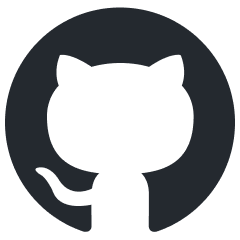
SuperAdapters
Finetune ALL LLMs with ALL Adapeters on ALL Platforms!
Stars: 293
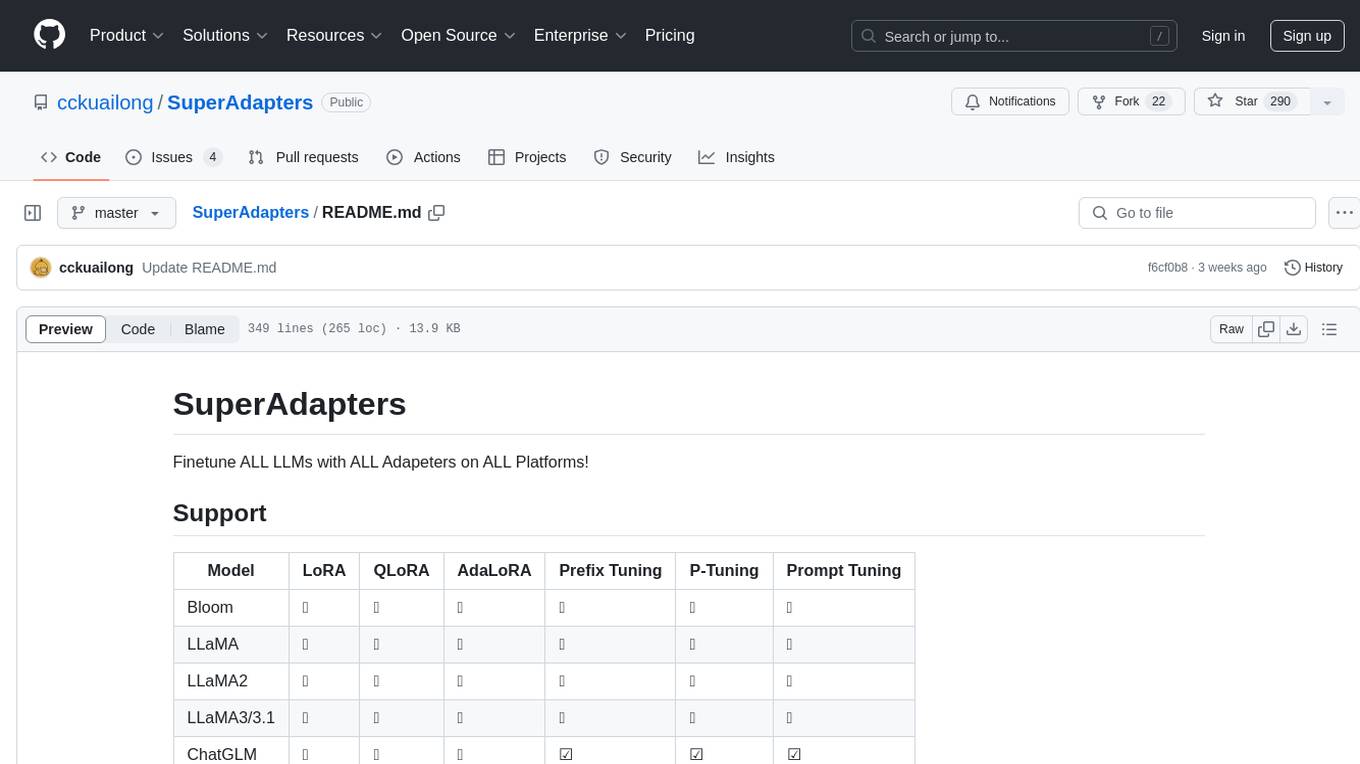
SuperAdapters is a tool designed to finetune Large Language Models (LLMs) with various adapters on different platforms. It supports models like Bloom, LLaMA, ChatGLM, Qwen, Baichuan, Mixtral, Phi, and more. Users can finetune LLMs on Windows, Linux, and Mac M1/2, handle train/test data with Terminal, File, or DataBase, and perform tasks like CausalLM and SequenceClassification. The tool provides detailed instructions on how to use different models with specific adapters for tasks like finetuning and inference. It also includes requirements for CentOS, Ubuntu, and MacOS, along with information on LLM downloads and data formats. Additionally, it offers parameters for finetuning and inference, as well as options for web and API-based inference.
README:
Finetune ALL LLMs with ALL Adapeters on ALL Platforms!
Model | LoRA | QLoRA | AdaLoRA | Prefix Tuning | P-Tuning | Prompt Tuning |
---|---|---|---|---|---|---|
Bloom | ✅ | ✅ | ✅ | ✅ | ✅ | ✅ |
LLaMA | ✅ | ✅ | ✅ | ✅ | ✅ | ✅ |
LLaMA2 | ✅ | ✅ | ✅ | ✅ | ✅ | ✅ |
LLaMA3/3.1 | ✅ | ✅ | ✅ | ✅ | ✅ | ✅ |
ChatGLM | ✅ | ✅ | ✅ | ☑️ | ☑️ | ☑️ |
ChatGLM2 | ✅ | ✅ | ✅ | ☑️ | ☑️ | ☑️ |
Qwen | ✅ | ✅ | ✅ | ✅ | ✅ | ✅ |
Baichuan | ✅ | ✅ | ✅ | ✅ | ✅ | ✅ |
Mixtral | ✅ | ✅ | ✅ | ✅ | ✅ | ✅ |
Phi | ✅ | ✅ | ✅ | ✅ | ✅ | ✅ |
Phi3 | ✅ | ✅ | ✅ | ✅ | ✅ | ✅ |
Gemma | ✅ | ✅ | ✅ | ✅ | ✅ | ✅ |
You can Finetune LLM on
- Windows
- Linux
- Mac M1/2
You can Handle train / test Data with
- Terminal
- File
- DataBase
You can Do various Task
- CausalLM (default)
- SequenceClassification
P.S. Unfortunately, SuperAdapters do not support qlora on Mac, please use lora/adalora instead.
CentOS:
yum install -y xz-devel
Ubuntu:
apt-get install -y liblzma-dev
MacOS:
brew install xz
P.S. Maybe you should recompile the python with xz
CPPFLAGS="-I$(brew --prefix xz)/include" pyenv install 3.10.0
If you want to use gpu on Mac, Please read How to use GPU on Mac
pip install --pre torch torchvision torchaudio --extra-index-url https://download.pytorch.org/whl/nightly/cpu
pip install -r requirements.txt
python finetune.py --model_type chatglm --data "data/train/" --model_path "LLMs/chatglm/chatglm-6b/" --adapter "lora" --output_dir "output/chatglm"
python inference.py --model_type chatglm --instruction "Who are you?" --model_path "LLMs/chatglm/chatglm-6b/" --adapter_weights "output/chatglm" --max_new_tokens 32
python finetune.py --model_type llama --data "data/train/" --model_path "LLMs/open-llama/open-llama-3b/" --adapter "lora" --output_dir "output/llama"
python inference.py --model_type llama --instruction "Who are you?" --model_path "LLMs/open-llama/open-llama-3b" --adapter_weights "output/llama" --max_new_tokens 32
python finetune.py --model_type qwen --data "data/train/" --model_path "LLMs/Qwen/Qwen-7b-chat" --adapter "lora" --output_dir "output/Qwen"
python inference.py --model_type qwen --instruction "Who are you?" --model_path "LLMs/Qwen/Qwen-7b-chat" --adapter_weights "output/Qwen" --max_new_tokens 32
Other LLMs are some usage of the above.
You need to specify task_type('classify') and labels
python finetune.py --model_type llama --data "data/train/alpaca_tiny_classify.json" --model_path "LLMs/open-llama/open-llama-3b" --adapter "lora" --output_dir "output/llama" --task_type classify --labels '["0", "1"]' --disable_wandb
python inference.py --model_type llama --data "data/train/alpaca_tiny_classify.json" --model_path "LLMs/open-llama/open-llama-3b" --adapter_weights "output/llama" --task_type classify --labels '["0", "1"]' --disable_wandb
- You need to install a MySQL, and put the db config into the system env.
Eg.
export LLM_DB_HOST='127.0.0.1'
export LLM_DB_PORT=3306
export LLM_DB_USERNAME='YOURUSERNAME'
export LLM_DB_PASSWORD='YOURPASSWORD'
export LLM_DB_NAME='YOURDBNAME'
- create the necessary tables
source xxxx.sql
- db_iteration: [train/test] The record's set name.
- db_type: [test] The record is whether "train" or "test".
- db_test_iteration: [test] The record's test set name.
- finetune (use chatglm for example)
python finetune.py --model_type chatglm --fromdb --db_iteration xxxxxx --model_path "LLMs/chatglm/chatglm-6b/" --adapter "lora" --output_dir "output/chatglm" --disable_wandb
- eval
python inference.py --model_type chatglm --fromdb --db_iteration xxxxxx --db_type 'test' --db_test_iteration yyyyyyy --model_path "LLMs/chatglm/chatglm-6b/" --adapter_weights "output/chatglm" --max_new_tokens 6
usage: finetune.py [-h] [--data DATA] [--model_type {llama,llama2,llama3,chatglm,chatglm2,bloom,qwen,baichuan,mixtral,phi,gemma}] [--task_type {seq2seq,classify}] [--labels LABELS] [--model_path MODEL_PATH]
[--output_dir OUTPUT_DIR] [--disable_wandb] [--adapter {lora,qlora,adalora,prompt,p_tuning,prefix}] [--lora_r LORA_R] [--lora_alpha LORA_ALPHA] [--lora_dropout LORA_DROPOUT]
[--lora_target_modules LORA_TARGET_MODULES [LORA_TARGET_MODULES ...]] [--adalora_init_r ADALORA_INIT_R] [--adalora_tinit ADALORA_TINIT] [--adalora_tfinal ADALORA_TFINAL]
[--adalora_delta_t ADALORA_DELTA_T] [--num_virtual_tokens NUM_VIRTUAL_TOKENS] [--mapping_hidden_dim MAPPING_HIDDEN_DIM] [--epochs EPOCHS] [--learning_rate LEARNING_RATE]
[--cutoff_len CUTOFF_LEN] [--val_set_size VAL_SET_SIZE] [--group_by_length] [--logging_steps LOGGING_STEPS] [--load_8bit] [--add_eos_token]
[--resume_from_checkpoint [RESUME_FROM_CHECKPOINT]] [--per_gpu_train_batch_size PER_GPU_TRAIN_BATCH_SIZE] [--gradient_accumulation_steps GRADIENT_ACCUMULATION_STEPS] [--fromdb]
[--db_iteration DB_ITERATION]
Finetune for all.
optional arguments:
-h, --help show this help message and exit
--data DATA the data used for instructing tuning
--model_type {llama,llama2,llama3,chatglm,chatglm2,bloom,qwen,baichuan,mixtral,phi,gemma}
--task_type {seq2seq,classify}
--labels LABELS Labels to classify, only used when task_type is classify
--model_path MODEL_PATH
--output_dir OUTPUT_DIR
The DIR to save the model
--disable_wandb Disable report to wandb
--adapter {lora,qlora,adalora,prompt,p_tuning,prefix}
--lora_r LORA_R
--lora_alpha LORA_ALPHA
--lora_dropout LORA_DROPOUT
--lora_target_modules LORA_TARGET_MODULES [LORA_TARGET_MODULES ...]
the module to be injected, e.g. q_proj/v_proj/k_proj/o_proj for llama, query_key_value for bloom&GLM
--adalora_init_r ADALORA_INIT_R
--adalora_tinit ADALORA_TINIT
number of warmup steps for AdaLoRA wherein no pruning is performed
--adalora_tfinal ADALORA_TFINAL
fix the resulting budget distribution and fine-tune the model for tfinal steps when using AdaLoRA
--adalora_delta_t ADALORA_DELTA_T
interval of steps for AdaLoRA to update rank
--num_virtual_tokens NUM_VIRTUAL_TOKENS
--mapping_hidden_dim MAPPING_HIDDEN_DIM
--epochs EPOCHS
--learning_rate LEARNING_RATE
--cutoff_len CUTOFF_LEN
--val_set_size VAL_SET_SIZE
--group_by_length
--logging_steps LOGGING_STEPS
--load_8bit
--add_eos_token
--resume_from_checkpoint [RESUME_FROM_CHECKPOINT]
resume from the specified or the latest checkpoint, e.g. `--resume_from_checkpoint [path]` or `--resume_from_checkpoint`
--per_gpu_train_batch_size PER_GPU_TRAIN_BATCH_SIZE
Batch size per GPU/CPU for training.
--gradient_accumulation_steps GRADIENT_ACCUMULATION_STEPS
--fromdb
--db_iteration DB_ITERATION
The record's set name.
--db_item_num DB_ITEM_NUM
The Limit Num of train/test items selected from DB.
usage: inference.py [-h] [--debug] [--web] [--api] [--instruction INSTRUCTION] [--input INPUT] [--max_input MAX_INPUT] [--test_data_path TEST_DATA_PATH]
[--model_type {llama,llama2,llama3,chatglm,chatglm2,bloom,qwen,baichuan,mixtral,phi,phi3,gemma}] [--task_type {seq2seq,classify}] [--labels LABELS] [--model_path MODEL_PATH]
[--adapter_weights ADAPTER_WEIGHTS] [--load_8bit] [--temperature TEMPERATURE] [--top_p TOP_P] [--top_k TOP_K] [--max_new_tokens MAX_NEW_TOKENS] [--vllm] [--fromdb] [--db_type DB_TYPE]
[--db_iteration DB_ITERATION] [--db_test_iteration DB_TEST_ITERATION] [--db_item_num DB_ITEM_NUM]
Inference for all.
optional arguments:
-h, --help show this help message and exit
--debug Debug Mode to output detail info
--web Web Demo to try the inference
--api API to try the inference
--instruction INSTRUCTION
--input INPUT
--max_input MAX_INPUT
Limit the input length to avoid OOM or other bugs
--test_data_path TEST_DATA_PATH
The DIR of test data
--model_type {llama,llama2,llama3,chatglm,chatglm2,bloom,qwen,baichuan,mixtral,phi,phi3,gemma}
--task_type {seq2seq,classify}
--labels LABELS Labels to classify, only used when task_type is classify
--model_path MODEL_PATH
--adapter_weights ADAPTER_WEIGHTS
The DIR of adapter weights
--load_8bit
--temperature TEMPERATURE
temperature higher, LLM is more creative
--top_p TOP_P
--top_k TOP_K
--max_new_tokens MAX_NEW_TOKENS
--vllm Use vllm to accelerate inference.
--fromdb
--db_type DB_TYPE The record is whether 'train' or 'test'.
--db_iteration DB_ITERATION
The record's set name.
--db_test_iteration DB_TEST_ITERATION
The record's test set name.
--db_item_num DB_ITEM_NUM
The Limit Num of train/test items selected from DB.
Use vllm:
- Combine the Base Model and Adapter weight
python tool.py combine --model_type llama3 --model_path "LLMs/llama3.1/" --adapter_weights "output/llama3.1/" --output_dir "output/llama3.1-combined/"
- Install the dependencies and start vllm server, Help Link.
- use option vllm
python inference.py --model_type llama3 --instruction "Who are you?" --model_path "/root/SuperAdapters/output/llama3.1-combined" --vllm --max_new_tokens 32
usage: tool.py combine [-h] [--model_type {llama,llama2,llama3,chatglm,chatglm2,bloom,qwen,baichuan,mixtral,phi,phi3,gemma}] [--model_path MODEL_PATH] [--adapter_weights ADAPTER_WEIGHTS]
[--output_dir OUTPUT_DIR] [--max_shard_size MAX_SHARD_SIZE]
optional arguments:
-h, --help show this help message and exit
--model_type {llama,llama2,llama3,chatglm,chatglm2,bloom,qwen,baichuan,mixtral,phi,phi3,gemma}
--model_path MODEL_PATH
--adapter_weights ADAPTER_WEIGHTS
The DIR of adapter weights
--output_dir OUTPUT_DIR
The DIR to save the model
--max_shard_size MAX_SHARD_SIZE
Max size of each of the combined model weight, like 1GB,5GB,etc.
python tool.py combine --model_type llama --model_path "LLMs/open-llama/open-llama-3b/" --adapter_weights "output/llama/" --output_dir "output/combine/"
Add the "--web" parameter
python inference.py --model_type phi --model_path "LLMs/phi/phi-2" --web
Add the "--api" parameter
python inference.py --model_type phi --model_path "LLMs/phi/phi-2" --api
python web/label.py
python web/label.py --type chat
For Tasks:
Click tags to check more tools for each tasksFor Jobs:
Alternative AI tools for SuperAdapters
Similar Open Source Tools
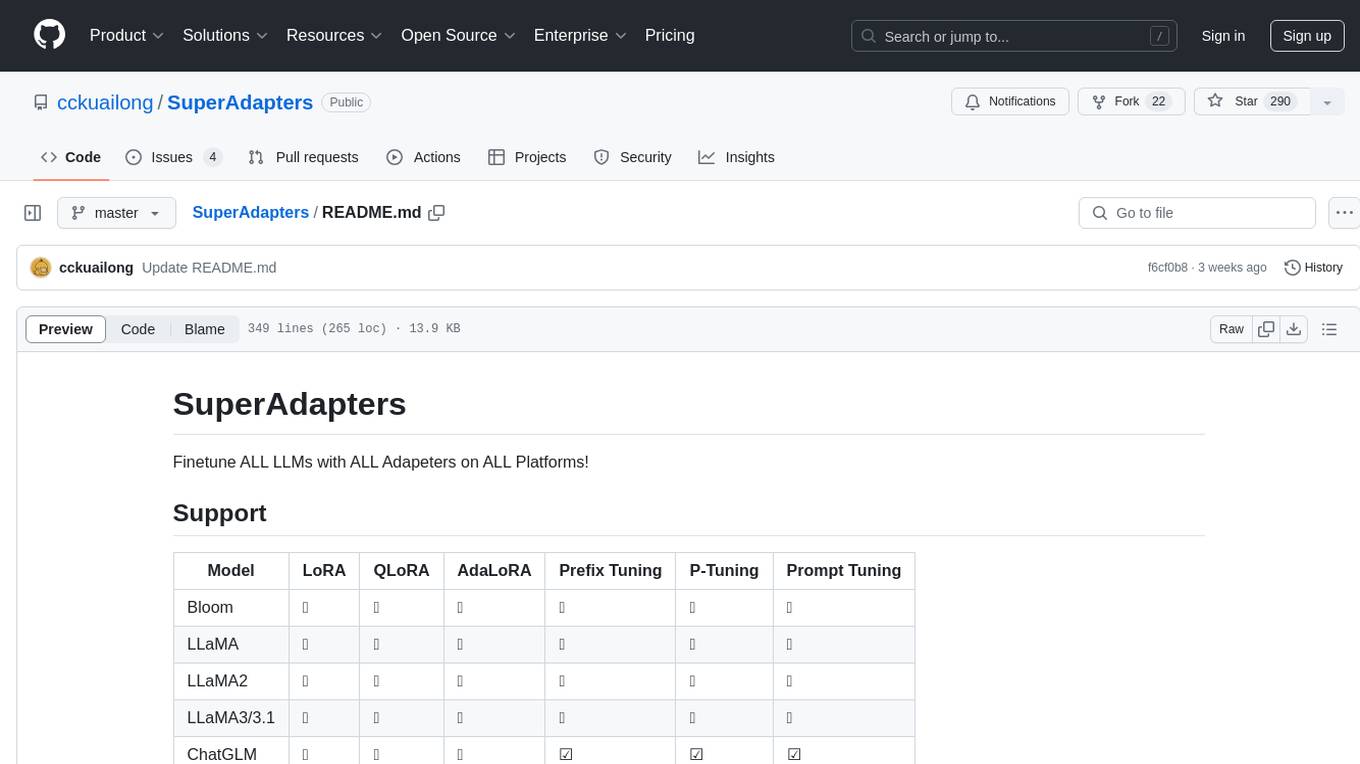
SuperAdapters
SuperAdapters is a tool designed to finetune Large Language Models (LLMs) with various adapters on different platforms. It supports models like Bloom, LLaMA, ChatGLM, Qwen, Baichuan, Mixtral, Phi, and more. Users can finetune LLMs on Windows, Linux, and Mac M1/2, handle train/test data with Terminal, File, or DataBase, and perform tasks like CausalLM and SequenceClassification. The tool provides detailed instructions on how to use different models with specific adapters for tasks like finetuning and inference. It also includes requirements for CentOS, Ubuntu, and MacOS, along with information on LLM downloads and data formats. Additionally, it offers parameters for finetuning and inference, as well as options for web and API-based inference.
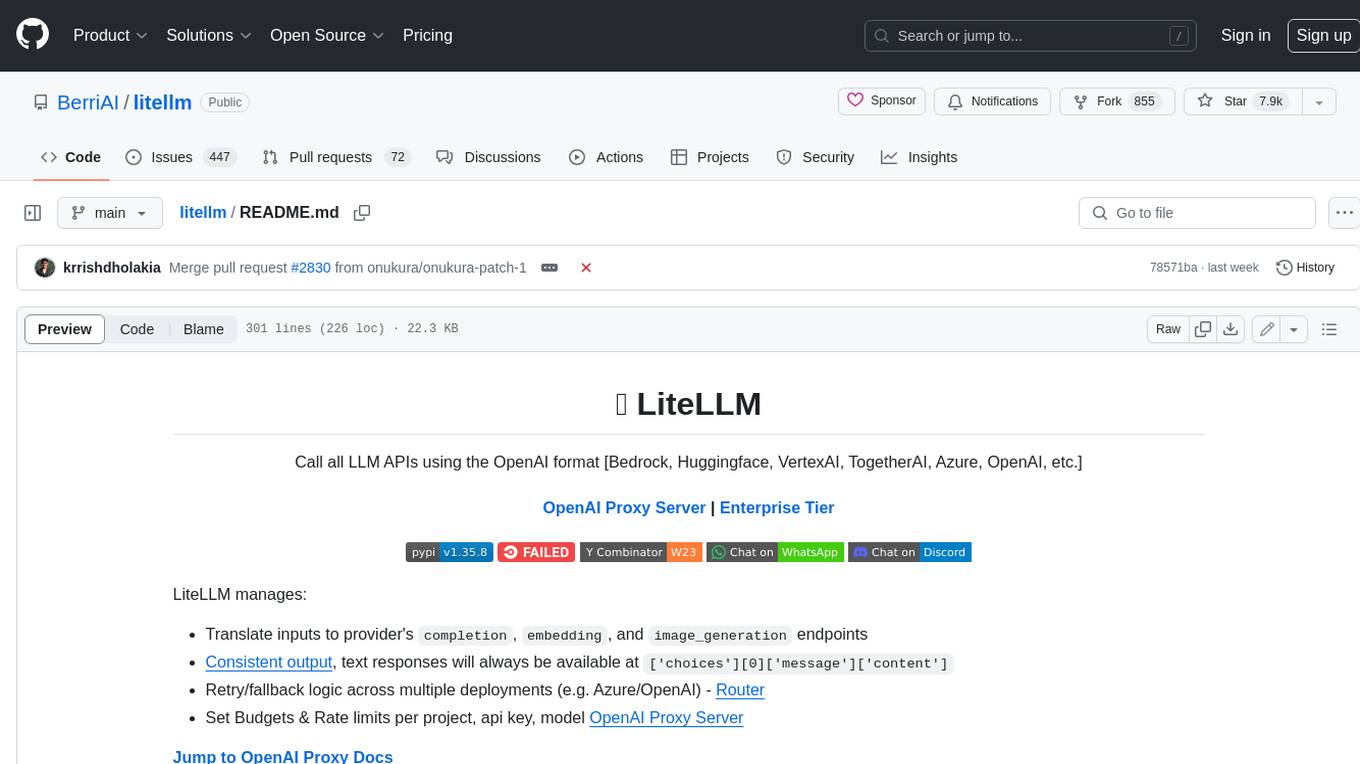
litellm
LiteLLM is a tool that allows you to call all LLM APIs using the OpenAI format. This includes Bedrock, Huggingface, VertexAI, TogetherAI, Azure, OpenAI, and more. LiteLLM manages translating inputs to provider's `completion`, `embedding`, and `image_generation` endpoints, providing consistent output, and retry/fallback logic across multiple deployments. It also supports setting budgets and rate limits per project, api key, and model.
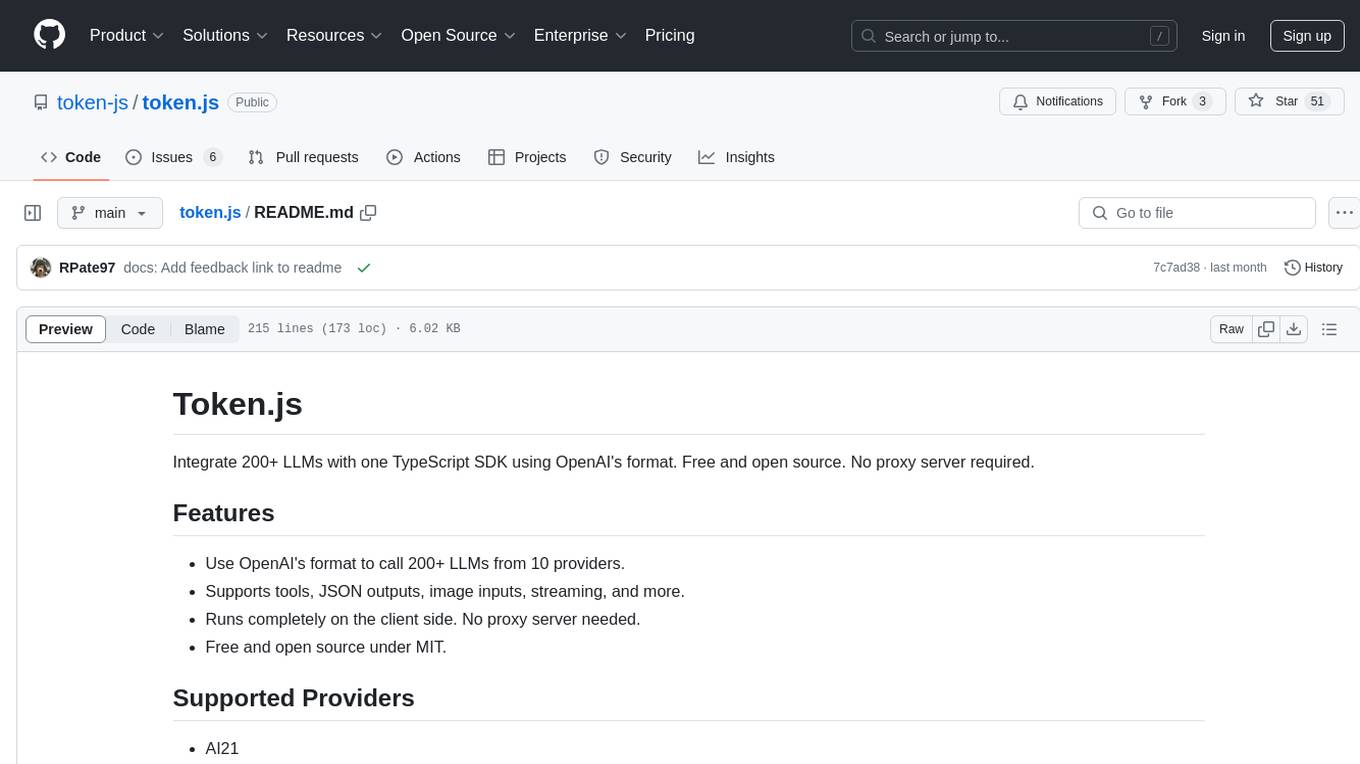
token.js
Token.js is a TypeScript SDK that integrates with over 200 LLMs from 10 providers using OpenAI's format. It allows users to call LLMs, supports tools, JSON outputs, image inputs, and streaming, all running on the client side without the need for a proxy server. The tool is free and open source under the MIT license.
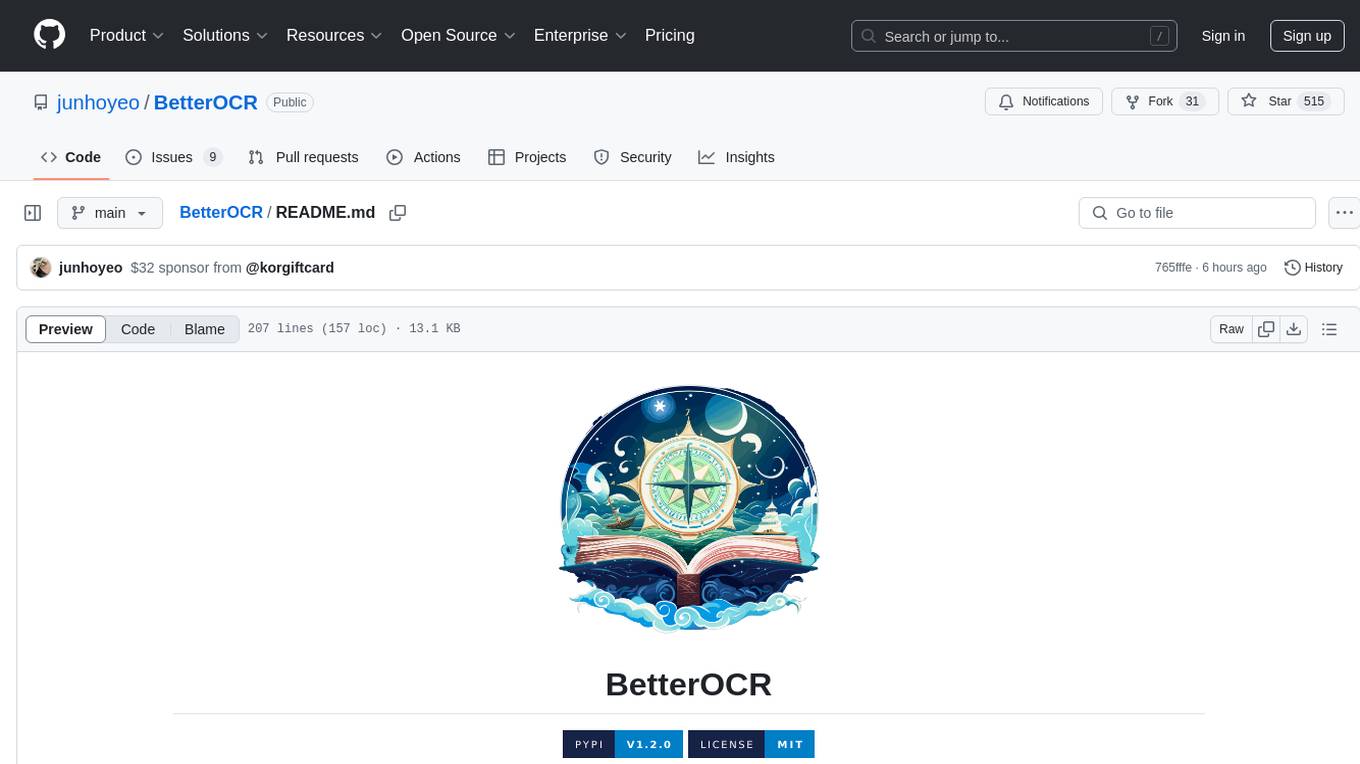
BetterOCR
BetterOCR is a tool that enhances text detection by combining multiple OCR engines with LLM (Language Model). It aims to improve OCR results, especially for languages with limited training data or noisy outputs. The tool combines results from EasyOCR, Tesseract, and Pororo engines, along with LLM support from OpenAI. Users can provide custom context for better accuracy, view performance examples by language, and upcoming features include box detection, improved interface, and async support. The package is under rapid development and contributions are welcomed.
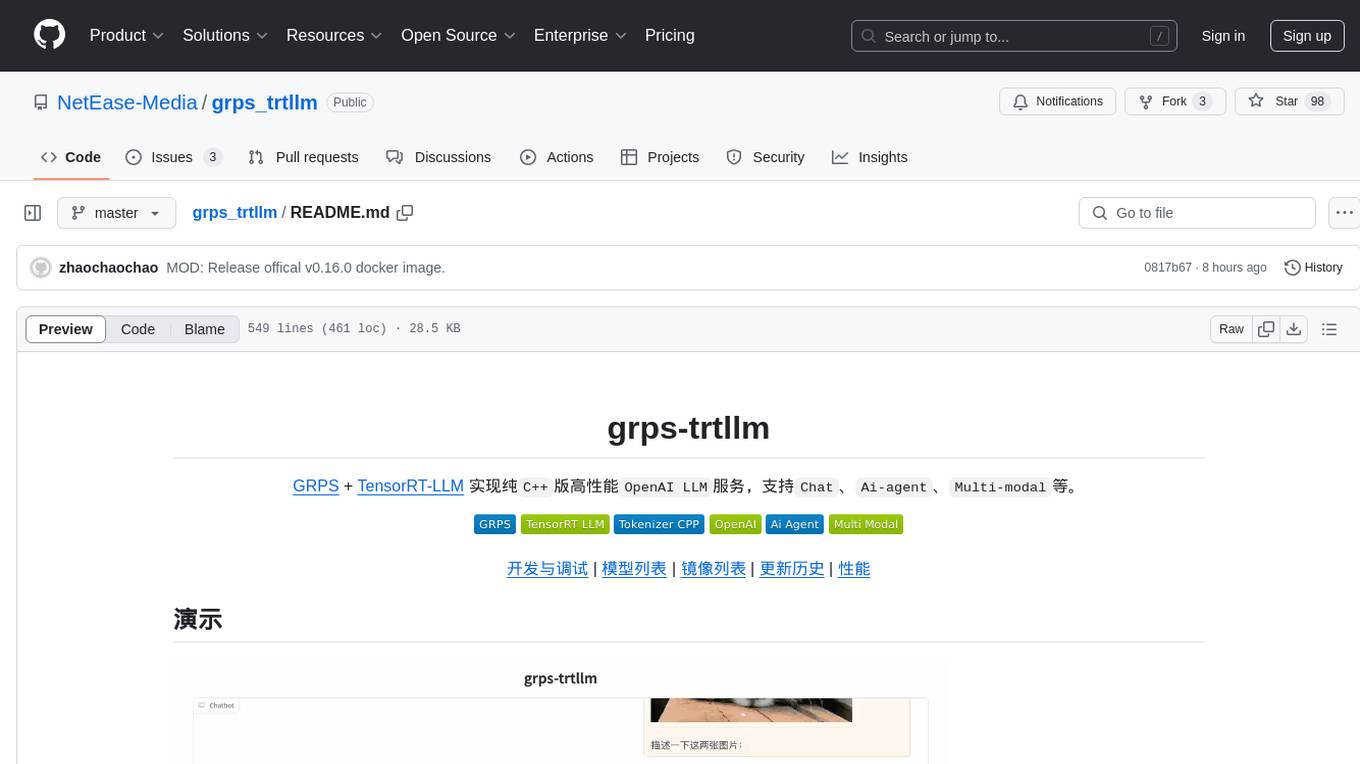
grps_trtllm
The grps-trtllm repository is a C++ implementation of a high-performance OpenAI LLM service, combining GRPS and TensorRT-LLM. It supports functionalities like Chat, Ai-agent, and Multi-modal. The repository offers advantages over triton-trtllm, including a complete LLM service implemented in pure C++, integrated tokenizer supporting huggingface and sentencepiece, custom HTTP functionality for OpenAI interface, support for different LLM prompt styles and result parsing styles, integration with tensorrt backend and opencv library for multi-modal LLM, and stable performance improvement compared to triton-trtllm.
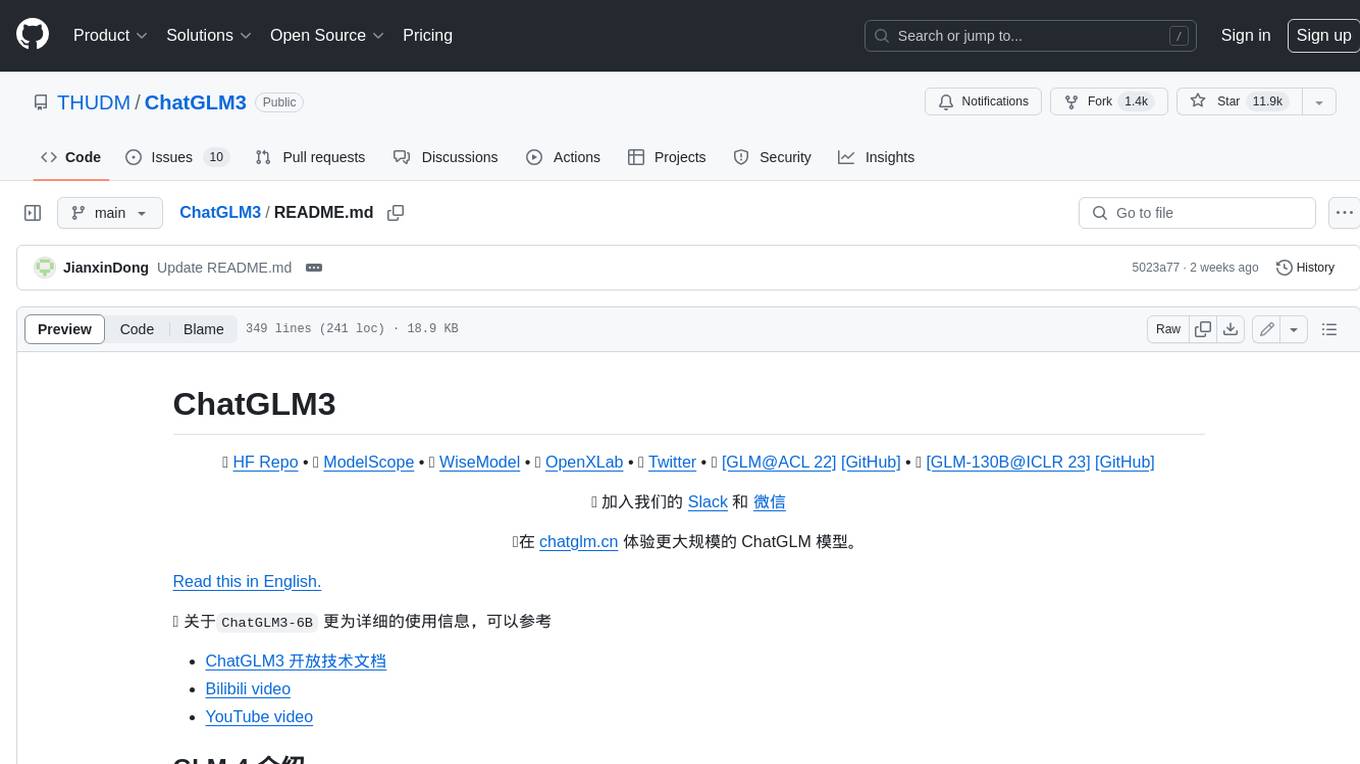
ChatGLM3
ChatGLM3 is a conversational pretrained model jointly released by Zhipu AI and THU's KEG Lab. ChatGLM3-6B is the open-sourced model in the ChatGLM3 series. It inherits the advantages of its predecessors, such as fluent conversation and low deployment threshold. In addition, ChatGLM3-6B introduces the following features: 1. A stronger foundation model: ChatGLM3-6B's foundation model ChatGLM3-6B-Base employs more diverse training data, more sufficient training steps, and more reasonable training strategies. Evaluation on datasets from different perspectives, such as semantics, mathematics, reasoning, code, and knowledge, shows that ChatGLM3-6B-Base has the strongest performance among foundation models below 10B parameters. 2. More complete functional support: ChatGLM3-6B adopts a newly designed prompt format, which supports not only normal multi-turn dialogue, but also complex scenarios such as tool invocation (Function Call), code execution (Code Interpreter), and Agent tasks. 3. A more comprehensive open-source sequence: In addition to the dialogue model ChatGLM3-6B, the foundation model ChatGLM3-6B-Base, the long-text dialogue model ChatGLM3-6B-32K, and ChatGLM3-6B-128K, which further enhances the long-text comprehension ability, are also open-sourced. All the above weights are completely open to academic research and are also allowed for free commercial use after filling out a questionnaire.
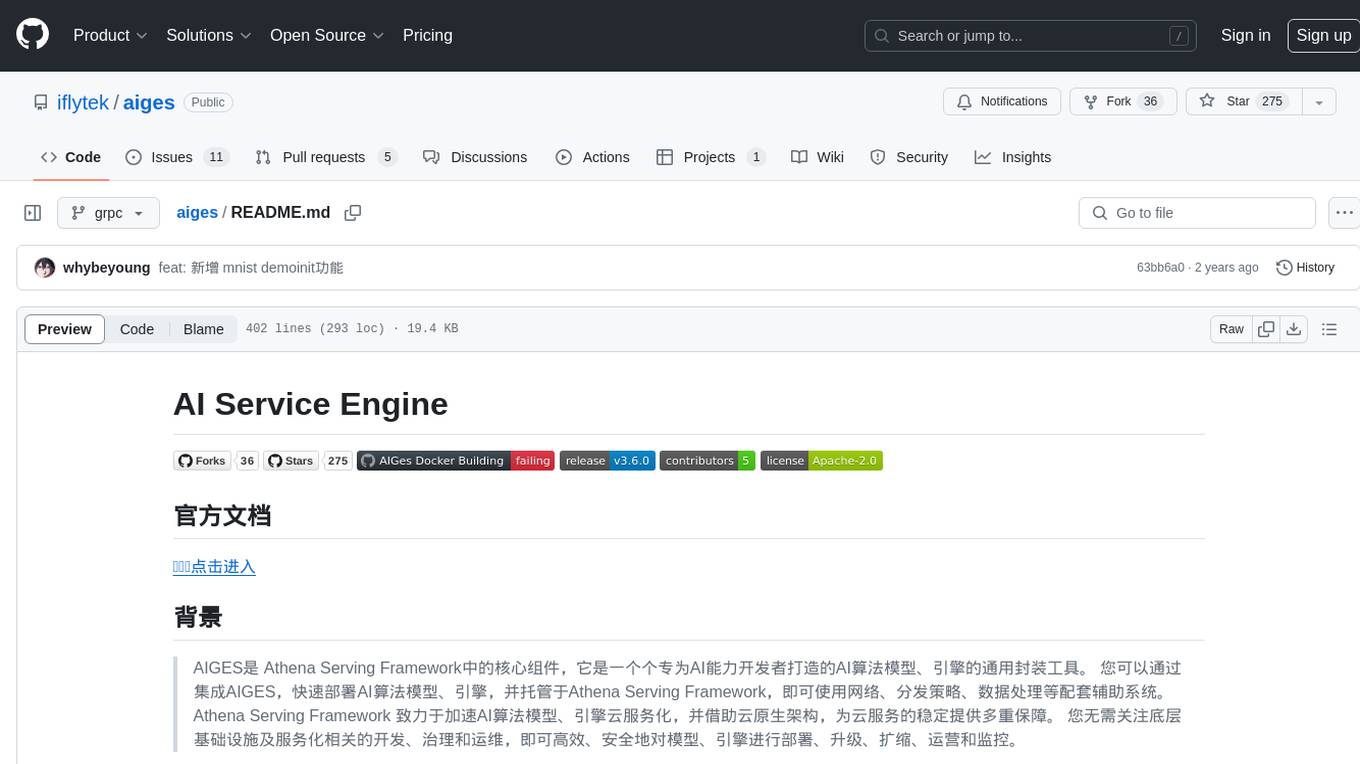
aiges
AIGES is a core component of the Athena Serving Framework, designed as a universal encapsulation tool for AI developers to deploy AI algorithm models and engines quickly. By integrating AIGES, you can deploy AI algorithm models and engines rapidly and host them on the Athena Serving Framework, utilizing supporting auxiliary systems for networking, distribution strategies, data processing, etc. The Athena Serving Framework aims to accelerate the cloud service of AI algorithm models and engines, providing multiple guarantees for cloud service stability through cloud-native architecture. You can efficiently and securely deploy, upgrade, scale, operate, and monitor models and engines without focusing on underlying infrastructure and service-related development, governance, and operations.
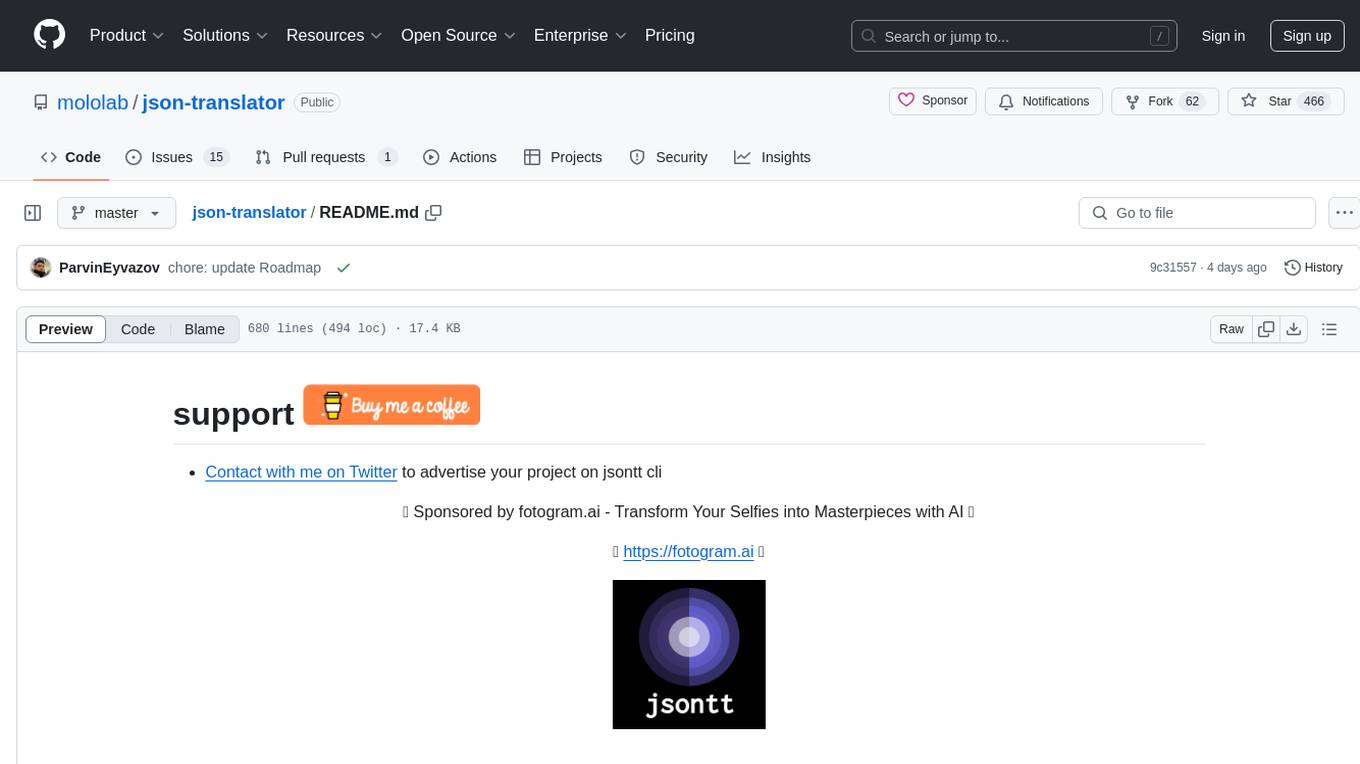
json-translator
The json-translator repository provides a free tool to translate JSON/YAML files or JSON objects into different languages using various translation modules. It supports CLI usage and package support, allowing users to translate words, sentences, JSON objects, and JSON files. The tool also offers multi-language translation, ignoring specific words, and safe translation practices. Users can contribute to the project by updating CLI, translation functions, JSON operations, and more. The roadmap includes features like Libre Translate option, Argos Translate option, Bing Translate option, and support for additional translation modules.
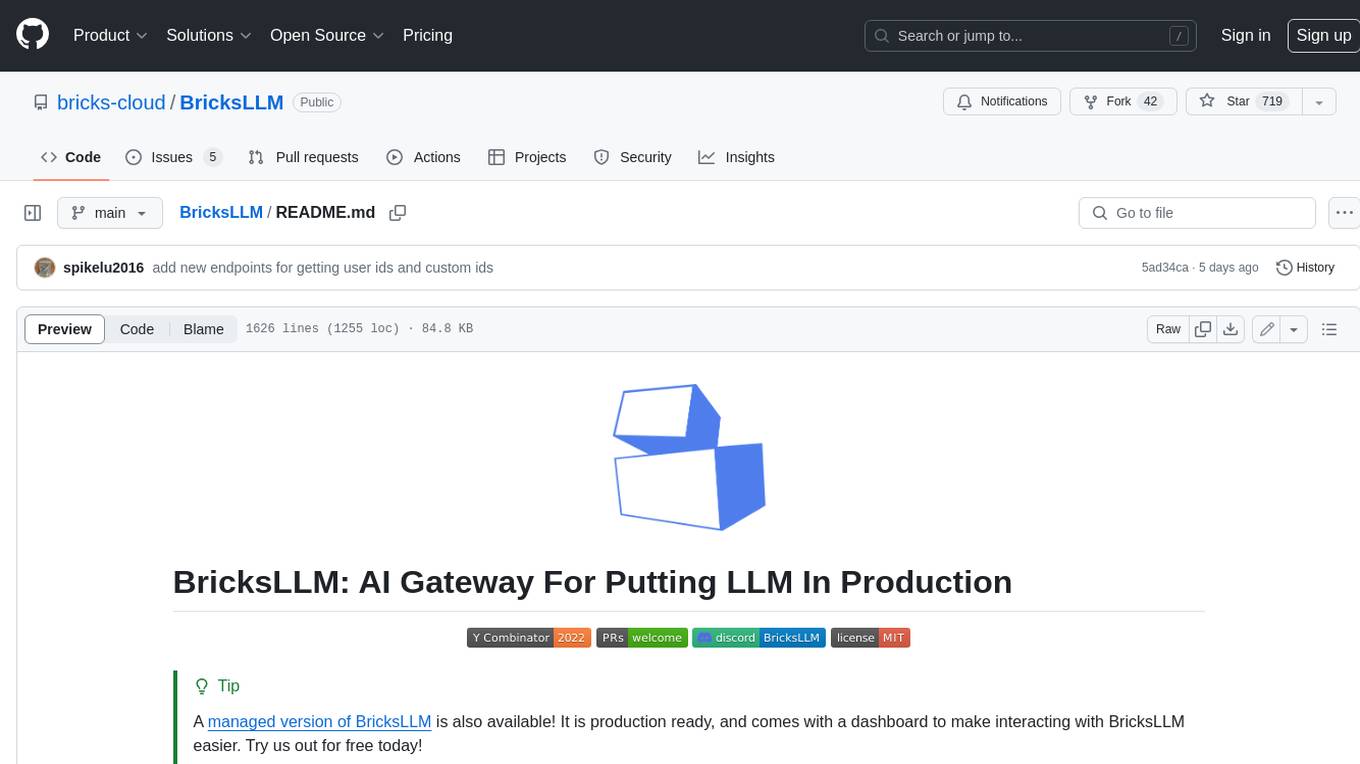
BricksLLM
BricksLLM is a cloud native AI gateway written in Go. Currently, it provides native support for OpenAI, Anthropic, Azure OpenAI and vLLM. BricksLLM aims to provide enterprise level infrastructure that can power any LLM production use cases. Here are some use cases for BricksLLM: * Set LLM usage limits for users on different pricing tiers * Track LLM usage on a per user and per organization basis * Block or redact requests containing PIIs * Improve LLM reliability with failovers, retries and caching * Distribute API keys with rate limits and cost limits for internal development/production use cases * Distribute API keys with rate limits and cost limits for students
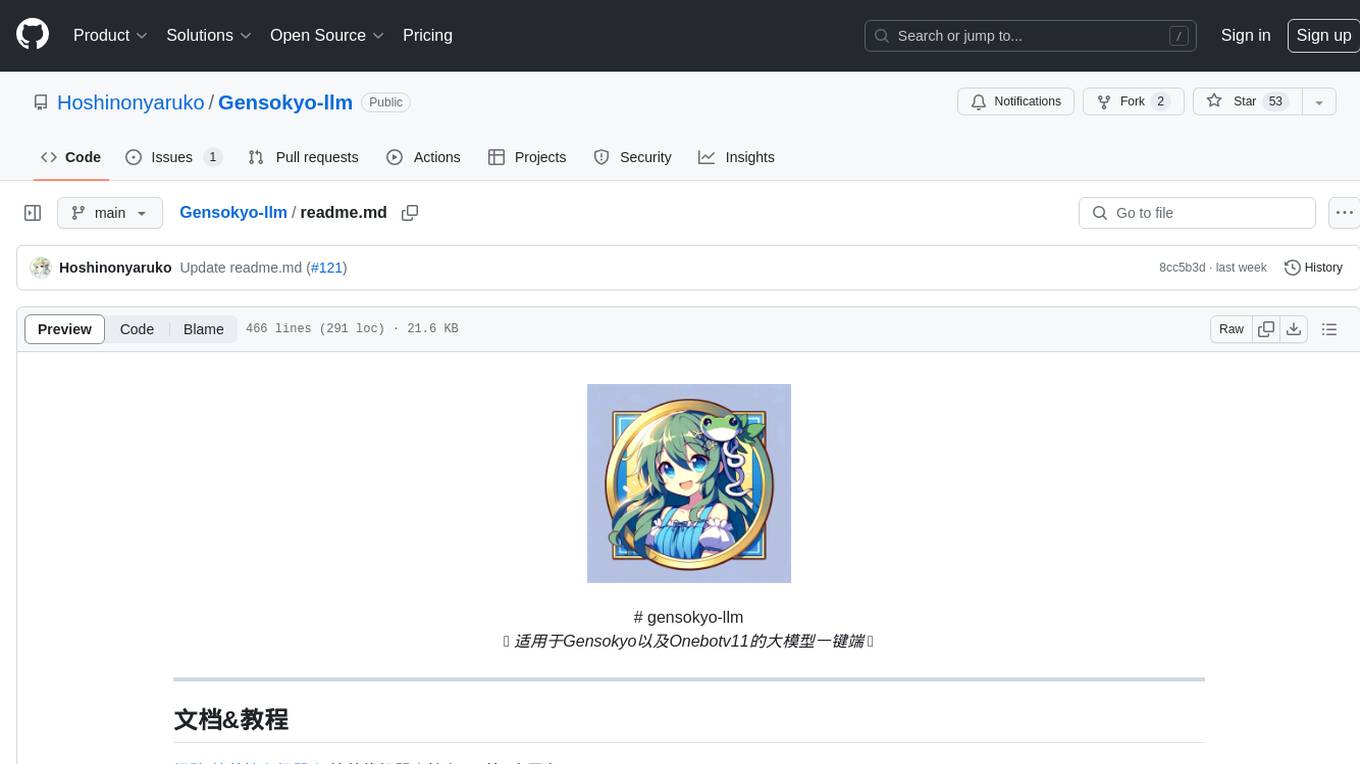
Gensokyo-llm
Gensokyo-llm is a tool designed for Gensokyo and Onebotv11, providing a one-click solution for large models. It supports various Onebotv11 standard frameworks, HTTP-API, and reverse WS. The tool is lightweight, with built-in SQLite for context maintenance and proxy support. It allows easy integration with the Gensokyo framework by configuring reverse HTTP and forward HTTP addresses. Users can set system settings, role cards, and context length. Additionally, it offers an openai original flavor API with automatic context. The tool can be used as an API or integrated with QQ channel robots. It supports converting GPT's SSE type and ensures memory safety in concurrent SSE environments. The tool also supports multiple users simultaneously transmitting SSE bidirectionally.
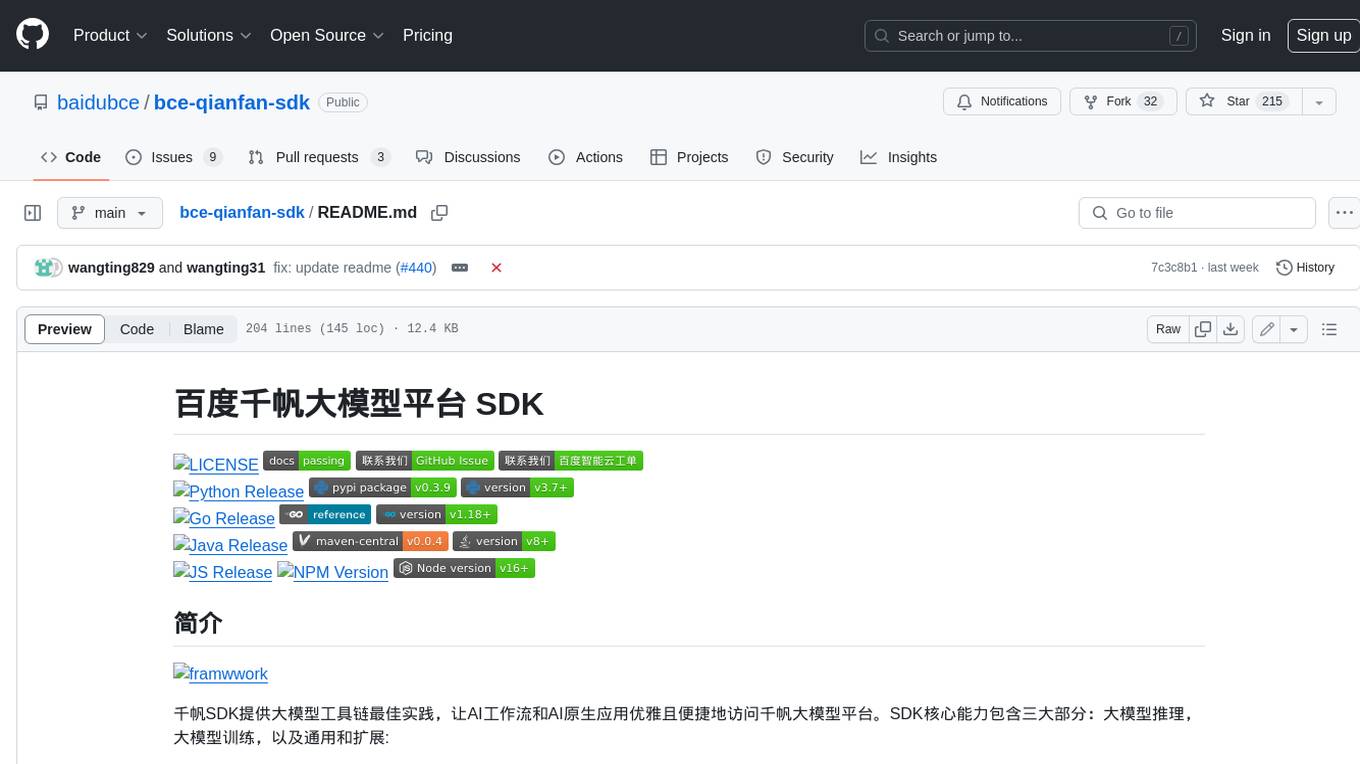
bce-qianfan-sdk
The Qianfan SDK provides best practices for large model toolchains, allowing AI workflows and AI-native applications to access the Qianfan large model platform elegantly and conveniently. The core capabilities of the SDK include three parts: large model reasoning, large model training, and general and extension: * `Large model reasoning`: Implements interface encapsulation for reasoning of Yuyan (ERNIE-Bot) series, open source large models, etc., supporting dialogue, completion, Embedding, etc. * `Large model training`: Based on platform capabilities, it supports end-to-end large model training process, including training data, fine-tuning/pre-training, and model services. * `General and extension`: General capabilities include common AI development tools such as Prompt/Debug/Client. The extension capability is based on the characteristics of Qianfan to adapt to common middleware frameworks.
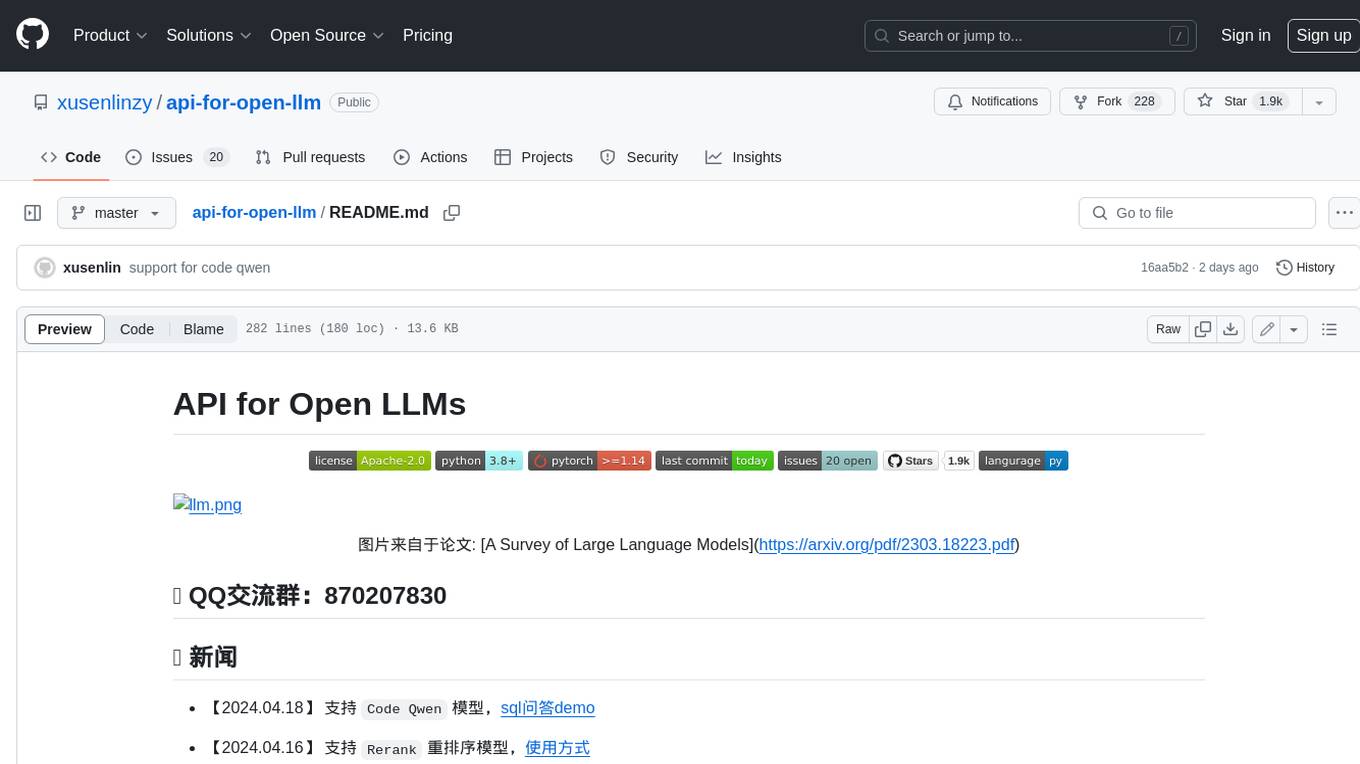
api-for-open-llm
This project provides a unified backend interface for open large language models (LLMs), offering a consistent experience with OpenAI's ChatGPT API. It supports various open-source LLMs, enabling developers to seamlessly integrate them into their applications. The interface features streaming responses, text embedding capabilities, and support for LangChain, a tool for developing LLM-based applications. By modifying environment variables, developers can easily use open-source models as alternatives to ChatGPT, providing a cost-effective and customizable solution for various use cases.
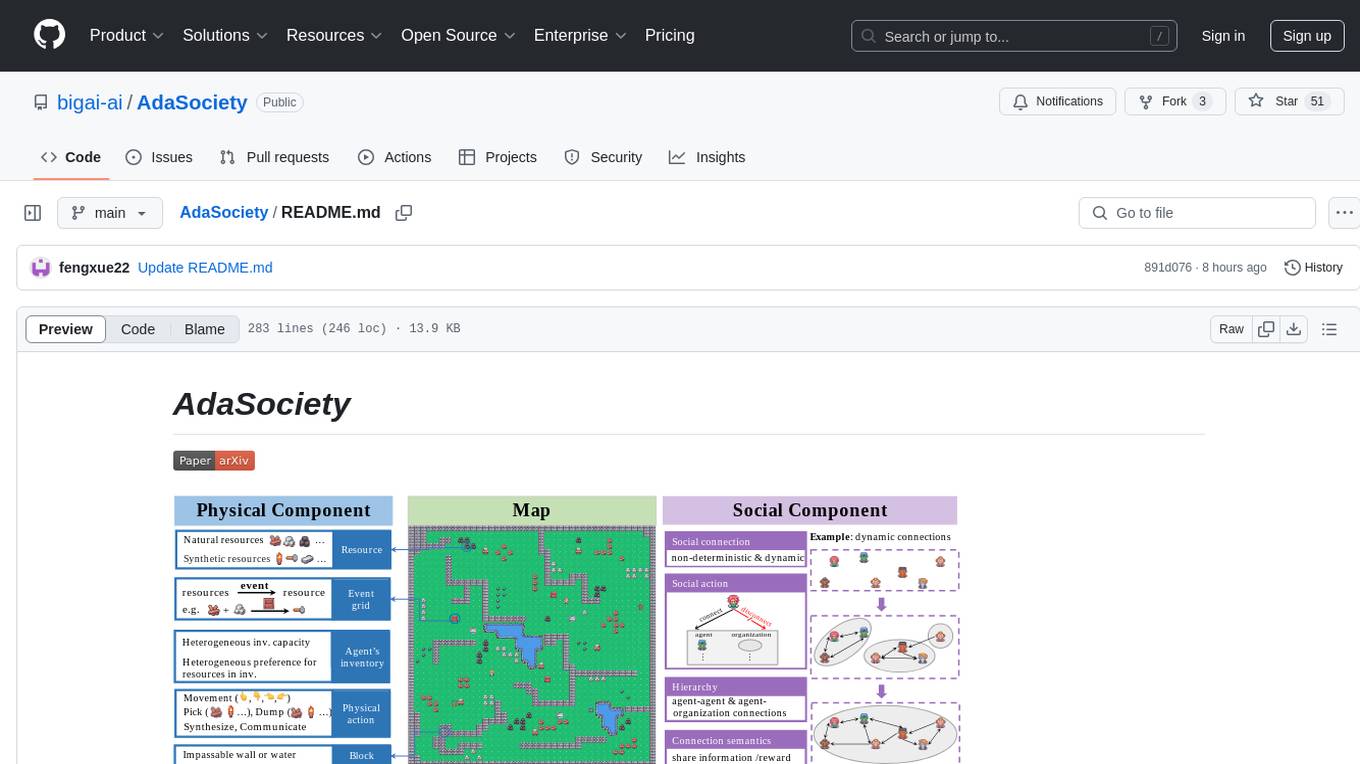
AdaSociety
AdaSociety is a multi-agent environment designed for simulating social structures and decision-making processes. It offers built-in resources, events, and player interactions. Users can customize the environment through JSON configuration or custom Python code. The environment supports training agents using RLlib and LLM frameworks. It provides a platform for studying multi-agent systems and social dynamics.
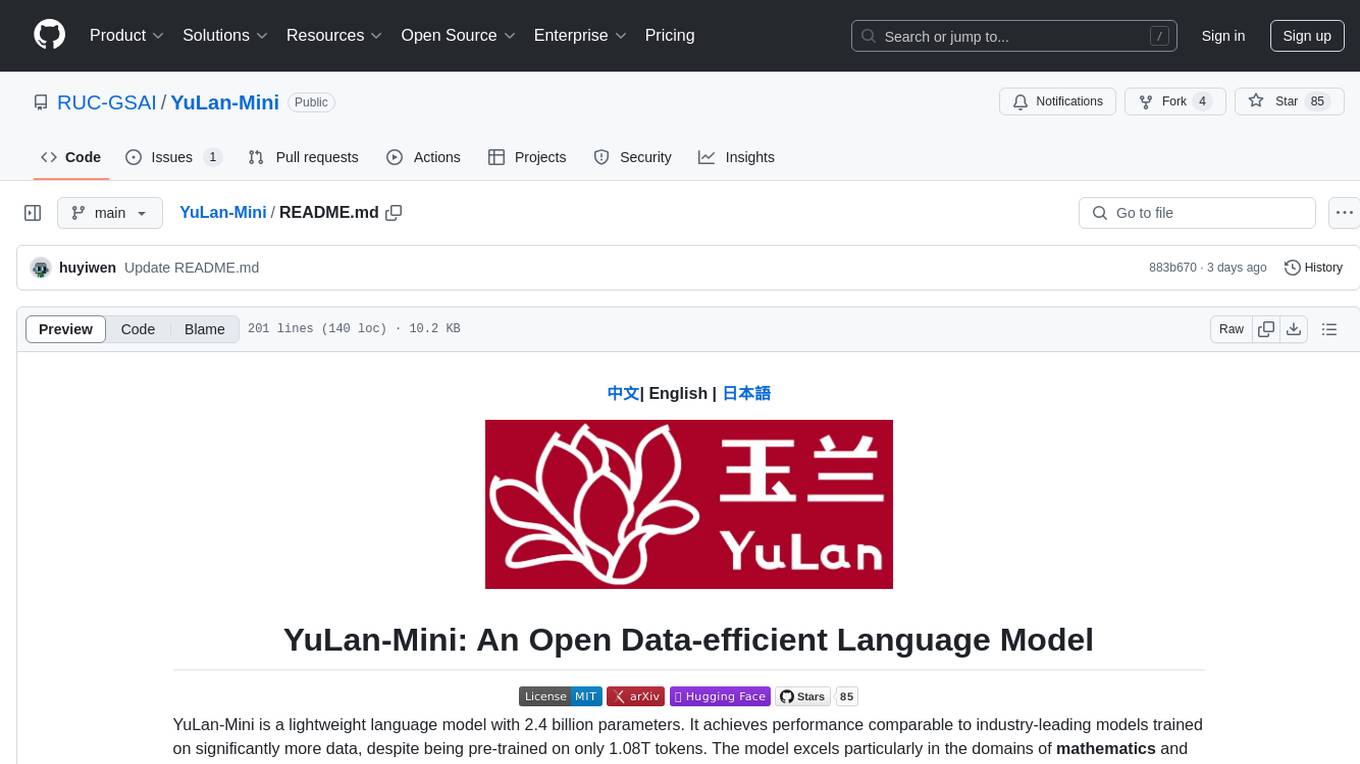
YuLan-Mini
YuLan-Mini is a lightweight language model with 2.4 billion parameters that achieves performance comparable to industry-leading models despite being pre-trained on only 1.08T tokens. It excels in mathematics and code domains. The repository provides pre-training resources, including data pipeline, optimization methods, and annealing approaches. Users can pre-train their own language models, perform learning rate annealing, fine-tune the model, research training dynamics, and synthesize data. The team behind YuLan-Mini is AI Box at Renmin University of China. The code is released under the MIT License with future updates on model weights usage policies. Users are advised on potential safety concerns and ethical use of the model.
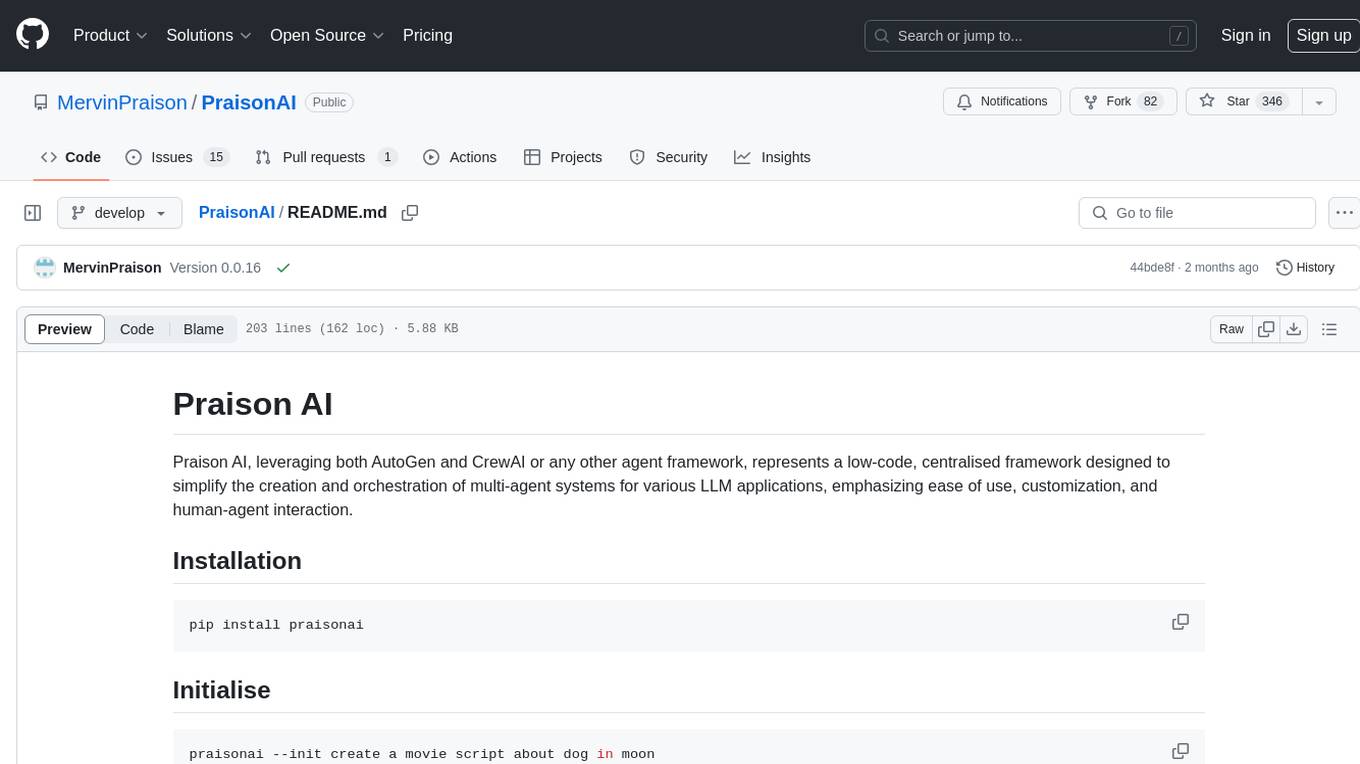
PraisonAI
Praison AI is a low-code, centralised framework that simplifies the creation and orchestration of multi-agent systems for various LLM applications. It emphasizes ease of use, customization, and human-agent interaction. The tool leverages AutoGen and CrewAI frameworks to facilitate the development of AI-generated scripts and movie concepts. Users can easily create, run, test, and deploy agents for scriptwriting and movie concept development. Praison AI also provides options for full automatic mode and integration with OpenAI models for enhanced AI capabilities.
For similar tasks
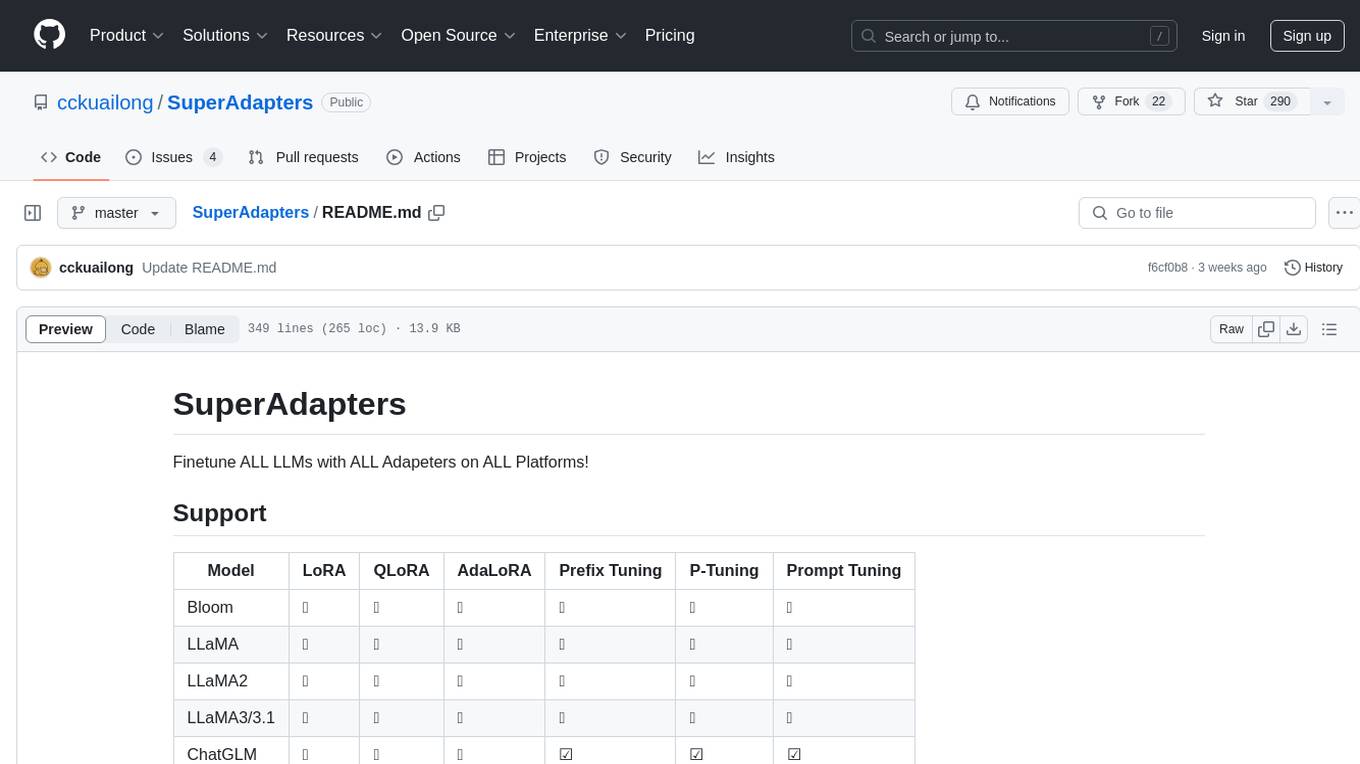
SuperAdapters
SuperAdapters is a tool designed to finetune Large Language Models (LLMs) with various adapters on different platforms. It supports models like Bloom, LLaMA, ChatGLM, Qwen, Baichuan, Mixtral, Phi, and more. Users can finetune LLMs on Windows, Linux, and Mac M1/2, handle train/test data with Terminal, File, or DataBase, and perform tasks like CausalLM and SequenceClassification. The tool provides detailed instructions on how to use different models with specific adapters for tasks like finetuning and inference. It also includes requirements for CentOS, Ubuntu, and MacOS, along with information on LLM downloads and data formats. Additionally, it offers parameters for finetuning and inference, as well as options for web and API-based inference.
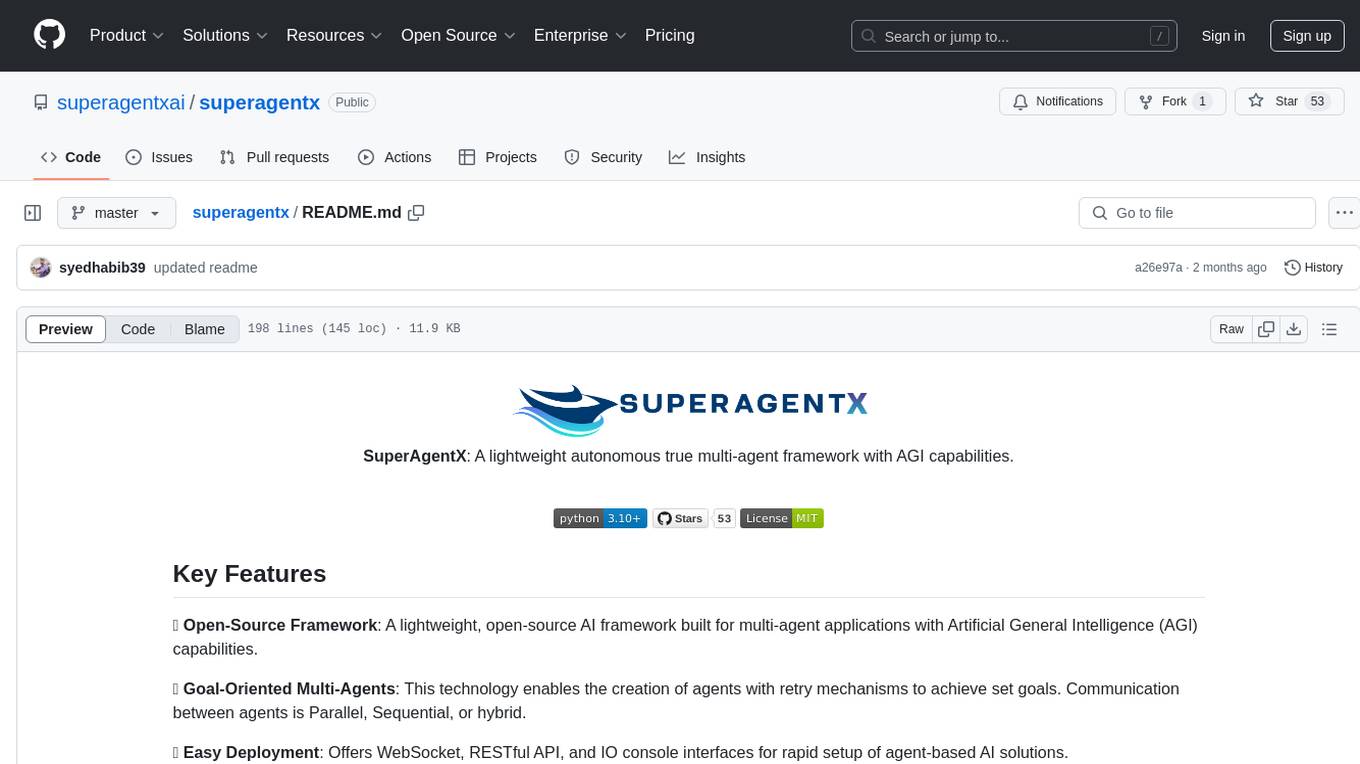
superagentx
SuperAgentX is a lightweight open-source AI framework designed for multi-agent applications with Artificial General Intelligence (AGI) capabilities. It offers goal-oriented multi-agents with retry mechanisms, easy deployment through WebSocket, RESTful API, and IO console interfaces, streamlined architecture with no major dependencies, contextual memory using SQL + Vector databases, flexible LLM configuration supporting various Gen AI models, and extendable handlers for integration with diverse APIs and data sources. It aims to accelerate the development of AGI by providing a powerful platform for building autonomous AI agents capable of executing complex tasks with minimal human intervention.
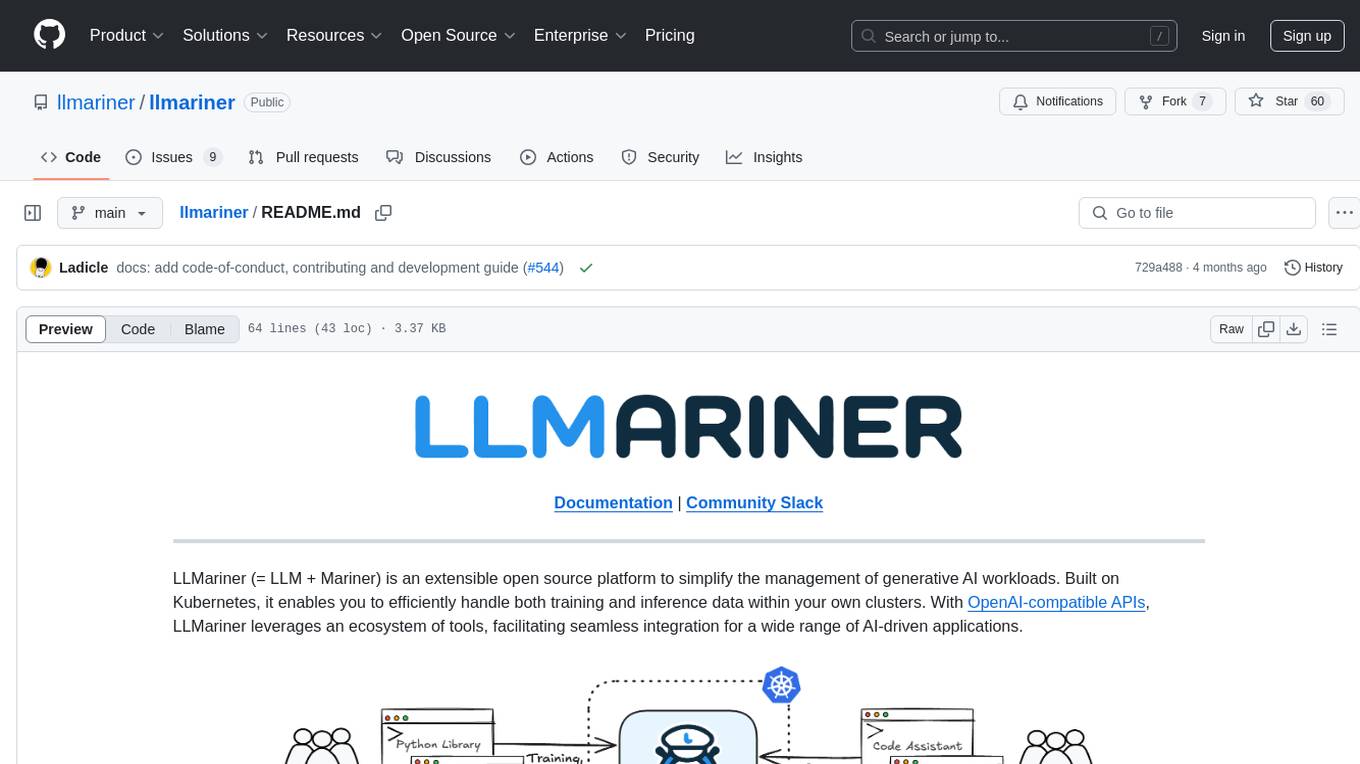
llmariner
LLMariner is an extensible open source platform built on Kubernetes to simplify the management of generative AI workloads. It enables efficient handling of training and inference data within clusters, with OpenAI-compatible APIs for seamless integration with a wide range of AI-driven applications.
For similar jobs
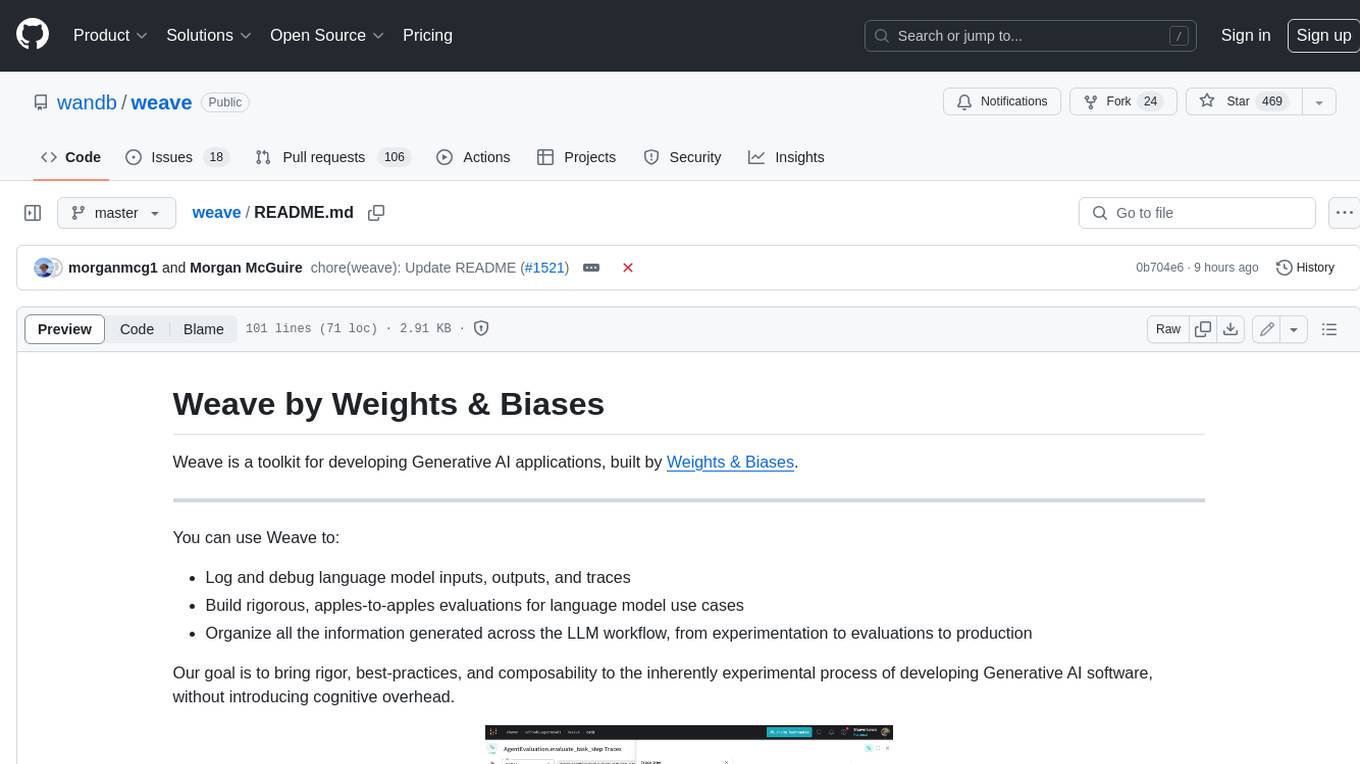
weave
Weave is a toolkit for developing Generative AI applications, built by Weights & Biases. With Weave, you can log and debug language model inputs, outputs, and traces; build rigorous, apples-to-apples evaluations for language model use cases; and organize all the information generated across the LLM workflow, from experimentation to evaluations to production. Weave aims to bring rigor, best-practices, and composability to the inherently experimental process of developing Generative AI software, without introducing cognitive overhead.
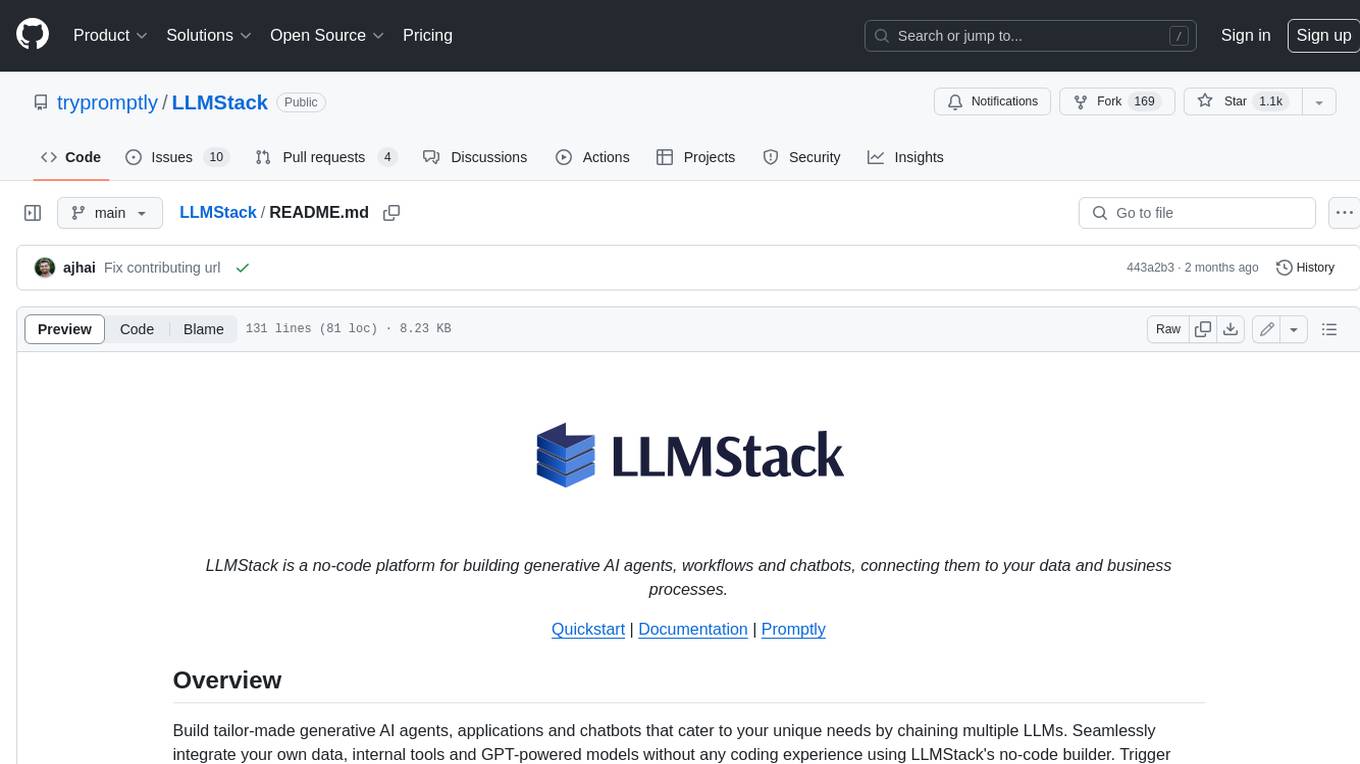
LLMStack
LLMStack is a no-code platform for building generative AI agents, workflows, and chatbots. It allows users to connect their own data, internal tools, and GPT-powered models without any coding experience. LLMStack can be deployed to the cloud or on-premise and can be accessed via HTTP API or triggered from Slack or Discord.
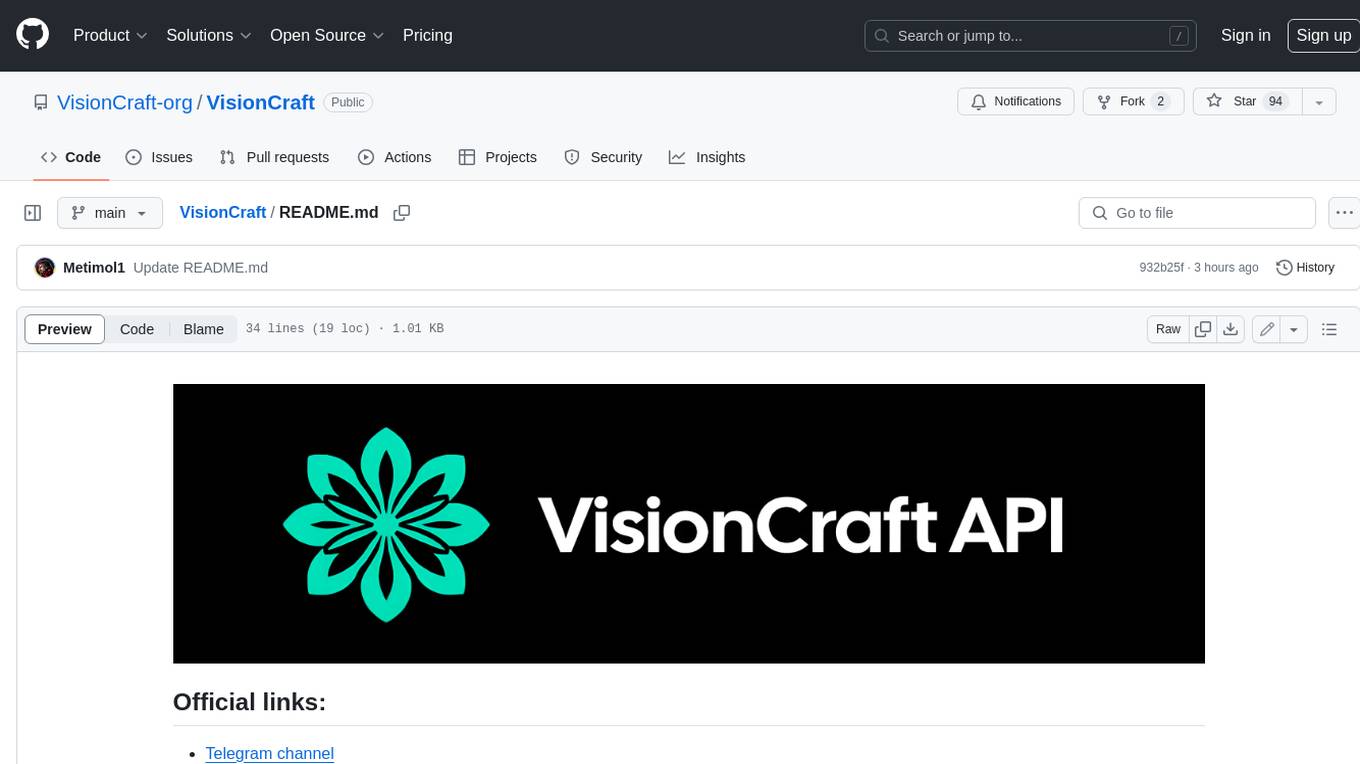
VisionCraft
The VisionCraft API is a free API for using over 100 different AI models. From images to sound.
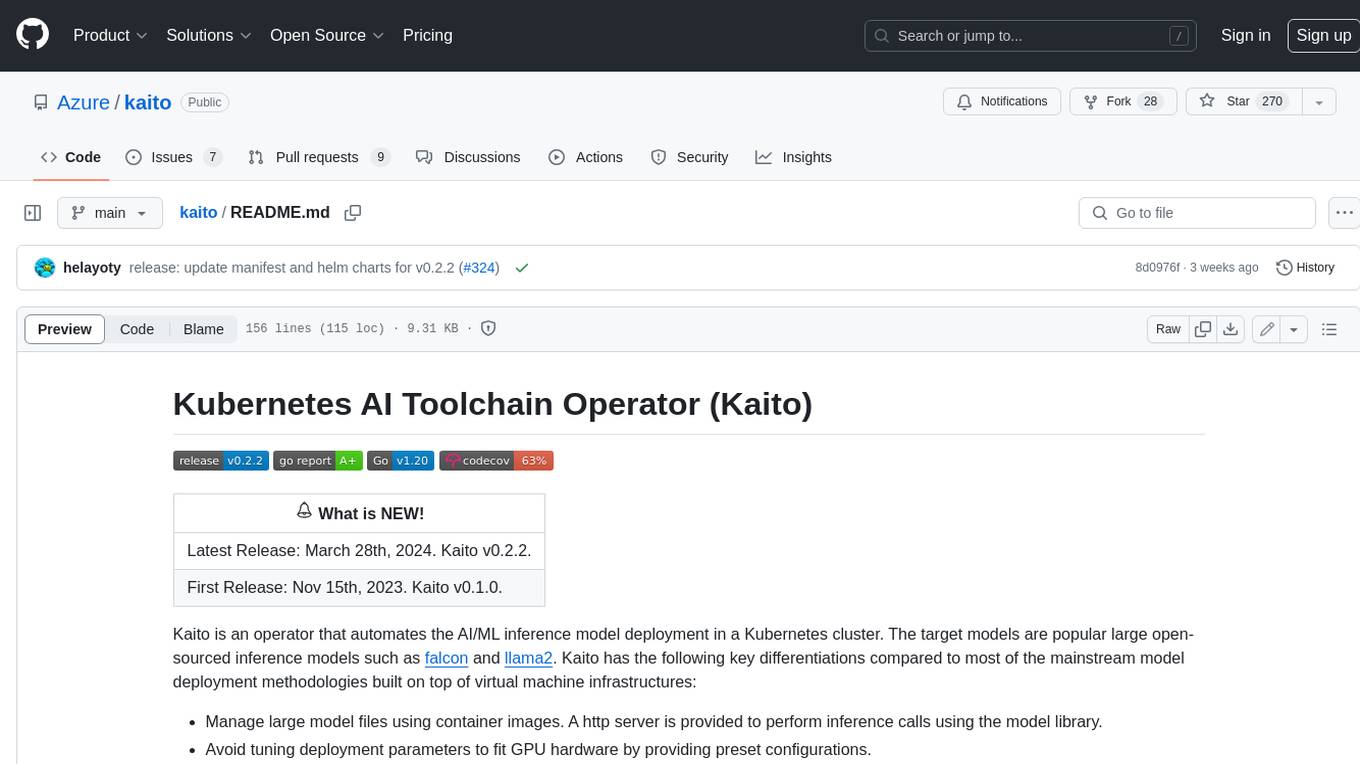
kaito
Kaito is an operator that automates the AI/ML inference model deployment in a Kubernetes cluster. It manages large model files using container images, avoids tuning deployment parameters to fit GPU hardware by providing preset configurations, auto-provisions GPU nodes based on model requirements, and hosts large model images in the public Microsoft Container Registry (MCR) if the license allows. Using Kaito, the workflow of onboarding large AI inference models in Kubernetes is largely simplified.
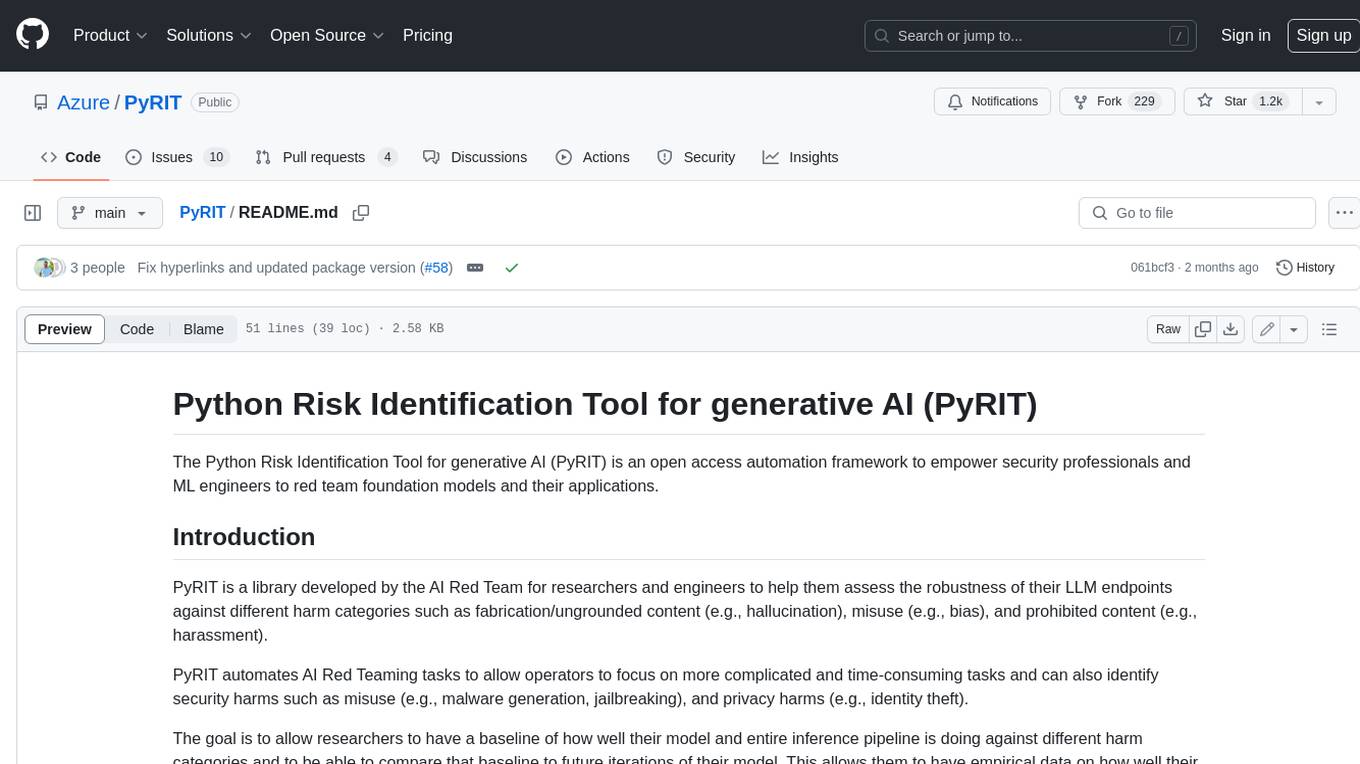
PyRIT
PyRIT is an open access automation framework designed to empower security professionals and ML engineers to red team foundation models and their applications. It automates AI Red Teaming tasks to allow operators to focus on more complicated and time-consuming tasks and can also identify security harms such as misuse (e.g., malware generation, jailbreaking), and privacy harms (e.g., identity theft). The goal is to allow researchers to have a baseline of how well their model and entire inference pipeline is doing against different harm categories and to be able to compare that baseline to future iterations of their model. This allows them to have empirical data on how well their model is doing today, and detect any degradation of performance based on future improvements.
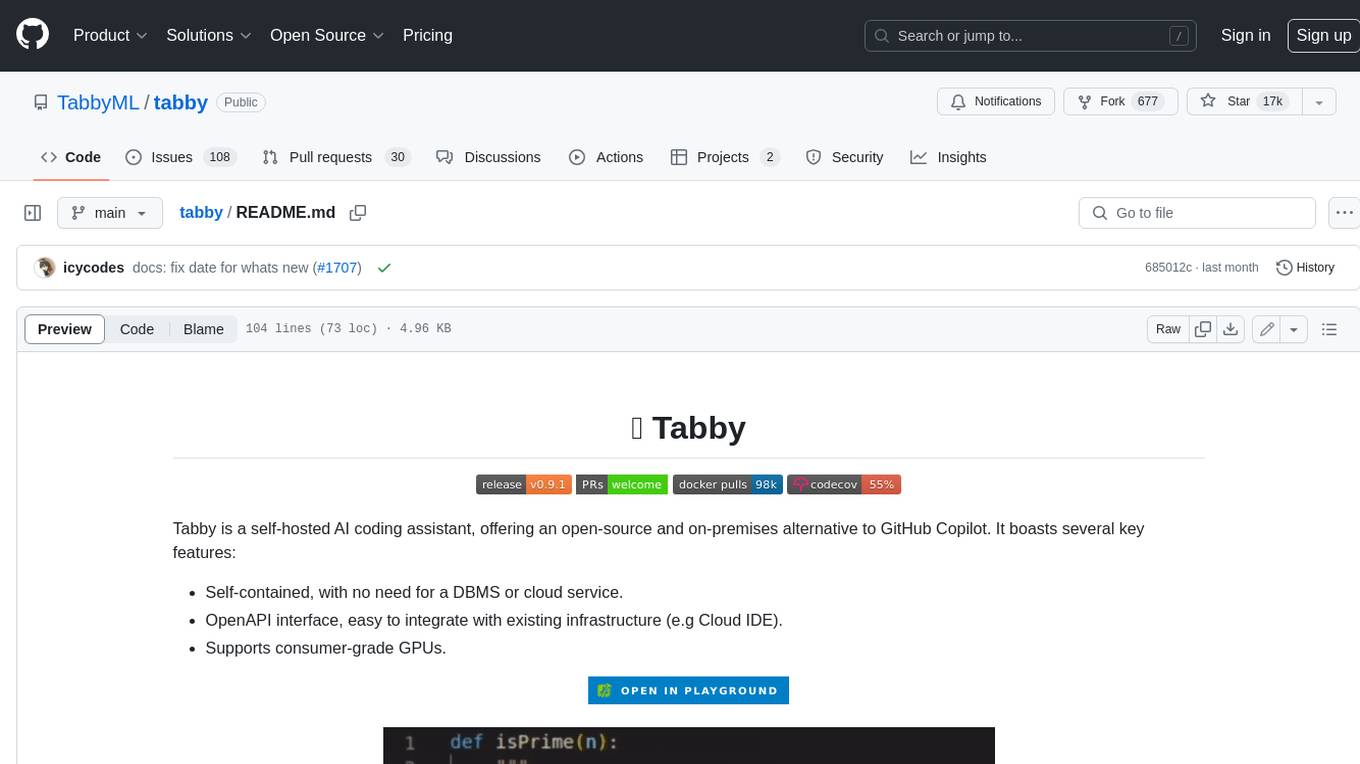
tabby
Tabby is a self-hosted AI coding assistant, offering an open-source and on-premises alternative to GitHub Copilot. It boasts several key features: * Self-contained, with no need for a DBMS or cloud service. * OpenAPI interface, easy to integrate with existing infrastructure (e.g Cloud IDE). * Supports consumer-grade GPUs.
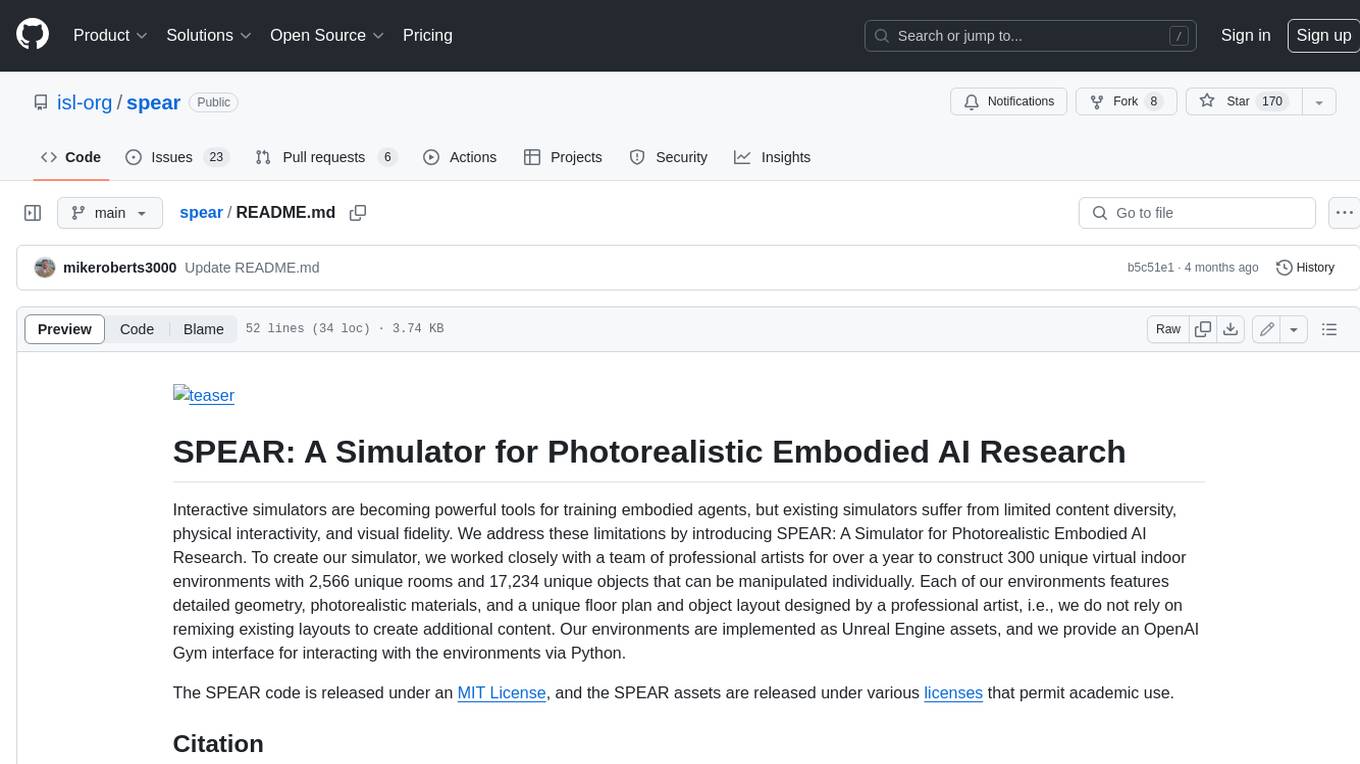
spear
SPEAR (Simulator for Photorealistic Embodied AI Research) is a powerful tool for training embodied agents. It features 300 unique virtual indoor environments with 2,566 unique rooms and 17,234 unique objects that can be manipulated individually. Each environment is designed by a professional artist and features detailed geometry, photorealistic materials, and a unique floor plan and object layout. SPEAR is implemented as Unreal Engine assets and provides an OpenAI Gym interface for interacting with the environments via Python.
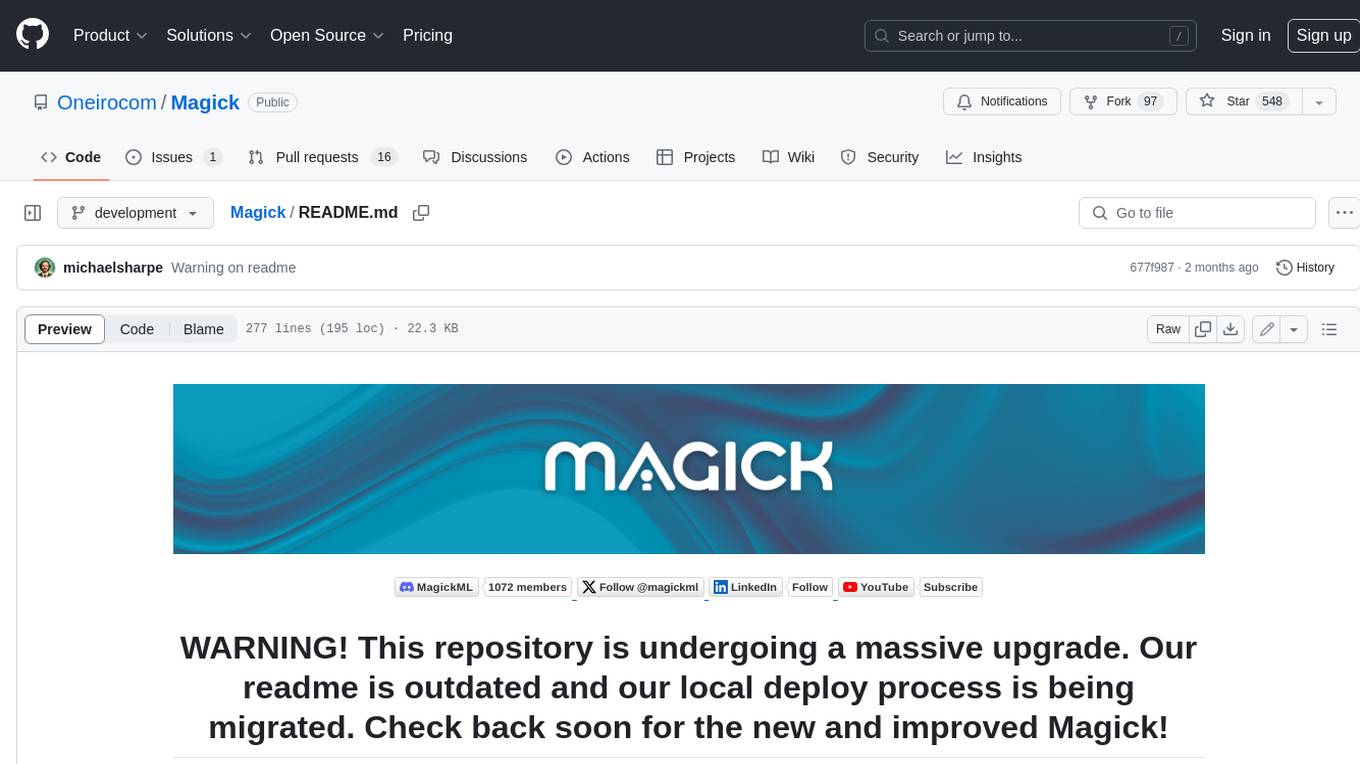
Magick
Magick is a groundbreaking visual AIDE (Artificial Intelligence Development Environment) for no-code data pipelines and multimodal agents. Magick can connect to other services and comes with nodes and templates well-suited for intelligent agents, chatbots, complex reasoning systems and realistic characters.