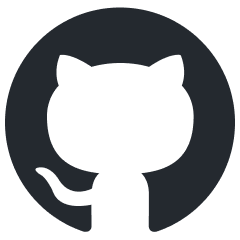
AirSLAM
AirSLAM is an upgrade version of AirVO
Stars: 489
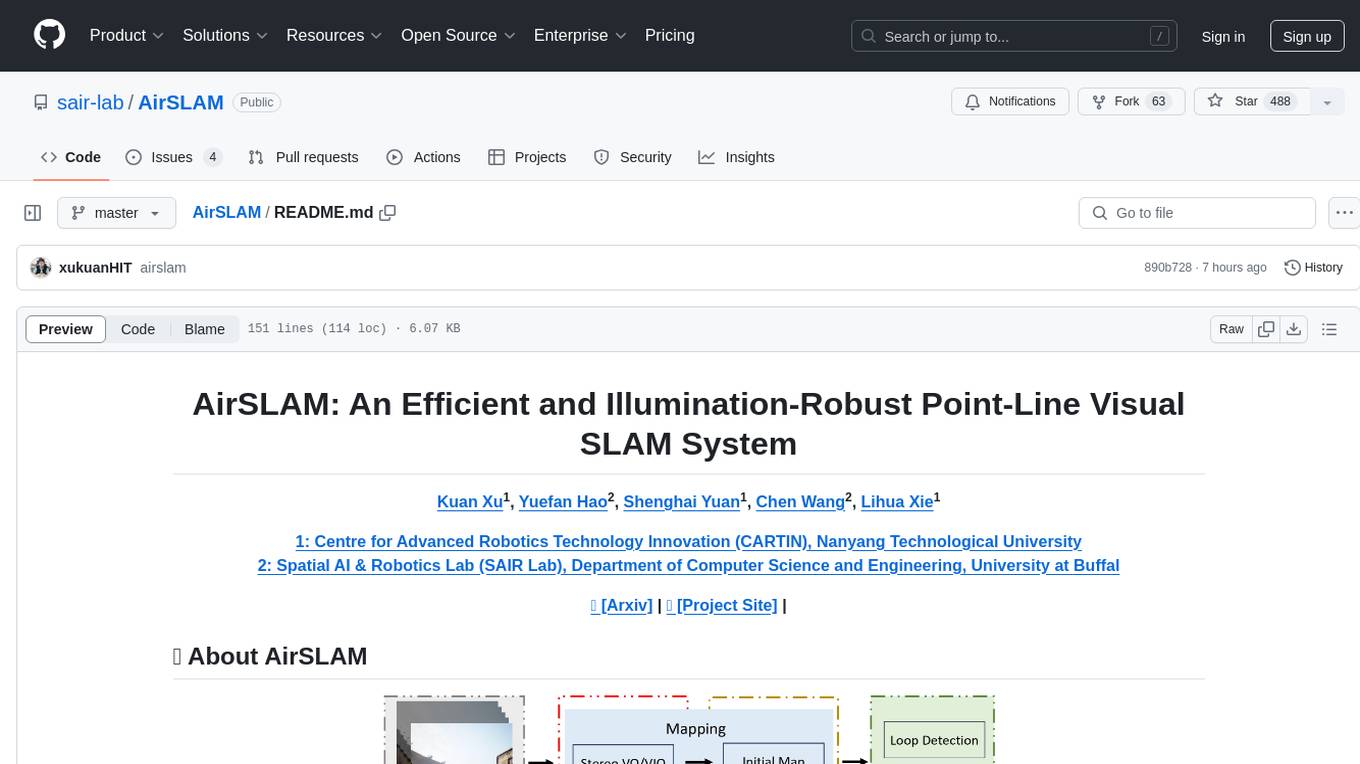
AirSLAM is an efficient visual SLAM system designed to tackle short-term and long-term illumination challenges. It combines deep learning techniques with traditional optimization methods, featuring a unified CNN for keypoint and structural line extraction. The system includes a relocalization pipeline for map reuse, accelerated using C++ and NVIDIA TensorRT. Outperforming other SLAM systems in challenging environments, it runs at 73Hz on PC and 40Hz on embedded platforms.
README:
Kuan Xu1, Yuefan Hao2, Shenghai Yuan1, Chen Wang2, Lihua Xie1
1: Centre for Advanced Robotics Technology Innovation (CARTIN), Nanyang Technological University
2: Spatial AI & Robotics Lab (SAIR Lab), Department of Computer Science and Engineering, University at Buffal
π [Arxiv] | πΎ [Project Site] |
AirSLAM is an efficient visual SLAM system designed to tackle both short-term and long-term illumination challenges. Our system adopts a hybrid approach that combines deep learning techniques for feature detection and matching with traditional backend optimization methods. Specifically, we proposea unified convolutional neural network (CNN) that simultaneously extracts keypoints and structural lines. These features are then associated, matched, triangulated, and optimized in a coupled manner. Additionally, we introduce a lightweight relocalization pipeline that reuses the built map, where keypoints, lines, anda structure graph are used to match the query frame with themap. To enhance the applicability of the proposed system to real-world robots, we deploy and accelerate the feature detection and matching networks using C++ and NVIDIA TensorRT. Extensive experiments conducted on various datasets demonstrate that our system outperforms other state-of-the-art visual SLAM systems in illumination-challenging environments. Efficiency evaluations show that our system can run at a rate of 73Hz on a PC and 40Hz on an embedded platform.
- [2024.08] We release the code and paper for AirSLAM.
- [2023.07] AriVO is accepted by IROS 2023.
- [2022.10] We release the code and paper for AirVO. The code for AirVO can now be found here.
- OpenCV 4.2
- Eigen 3
- Ceres 2.0.0
- G2O (tag:20230223_git)
- TensorRT 8.6
- CUDA 12
- python
- ROS noetic
- Boost
docker pull xukuanhit/air_slam:v4
docker run -it --env DISPLAY=$DISPLAY --volume /tmp/.X11-unix:/tmp/.X11-unix --privileged --runtime nvidia --gpus all --volume ${PWD}:/workspace --workdir /workspace --name air_slam xukuanhit/air_slam:v4 /bin/bash
The data for mapping should be organized in the following Automous Systems Lab (ASL) dataset format:
dataroot
βββ cam0
βΒ Β βββ data
βΒ Β βββ 00001.jpg
βΒ Β βββ 00002.jpg
βΒ Β βββ 00003.jpg
βΒ Β βββ ......
βββ cam1
β βββ data
β βββ 00001.jpg
β βββ 00002.jpg
β βββ 00003.jpg
β βββ ......
βββ imu0
βββ data.csv
After the map is built, the relocalization requires only moocular images. Therefore, you only need to place the query images in a folder.
cd ~/catkin_ws/src
git clone https://github.com/sair-lab/AirSLAM.git
cd ../
catkin_make
source ~/catkin_ws/devel/setup.bash
The launch files for VO/VIO, map optimization and relocalization are placed in VO folder, MR folder, and Reloc folder, respectively. Before running them, you need to modify the corresponding configurations according to you data path and the desired map saving path. The following is an example of mapping, optimization, and relocalization with the EuRoC dataset.
1: Change "dataroot" in VO launch file to your own data path. For the EuRoC dataset, "mav0" needs to be included in the path.
2: Change "saving_dir" in the same file to the path where you want to save the map and trajectory. It must be an existing folder.
3: Run the launch file:
roslaunch air_slam vo_euroc.launch
1: Change "map_root" in MR launch file to your own map path.
2: Run the launch file:
roslaunch air_slam mr_euroc.launch
1: Change "dataroot" in Reloc launch file to your own query data path.
2: Change "map_root" in the same file to your own map path.
3: Run the launch file:
roslaunch air_slam reloc_euroc.launch
Launch folder and config folder respectively provide the lauch files and configuration files for other datatsetsin the paper. If you want to run AirSLAM with your own dataset, you need to create your own camera file, configuration file, and launch file.
- [x] Initial release. π
- [ ] Support SuperGlue as feature matecher
- [ ] Optimize the TensorRT acceleration of PLNet
@article{xu2024airslam,
title = {{AirSLAM}: An Efficient and Illumination-Robust Point-Line Visual SLAM System},
author = {Xu, Kuan and Hao, Yuefan and Yuan, Shenghai and Wang, Chen and Xie, Lihua},
journal = {arXiv preprint arXiv:2408.03520},
year = {2024},
url = {https://arxiv.org/abs/2408.03520},
code = {https://github.com/sair-lab/AirSLAM},
}
@inproceedings{xu2023airvo,
title = {{AirVO}: An Illumination-Robust Point-Line Visual Odometry},
author = {Xu, Kuan and Hao, Yuefan and Yuan, Shenghai and Wang, Chen and Xie, Lihua},
booktitle = {IEEE/RSJ International Conference on Intelligent Robots and Systems (IROS)},
year = {2023},
url = {https://arxiv.org/abs/2212.07595},
code = {https://github.com/sair-lab/AirVO},
video = {https://youtu.be/YfOCLll_PfU},
addendum = {SAIR Lab Recommended}
}
For Tasks:
Click tags to check more tools for each tasksFor Jobs:
Alternative AI tools for AirSLAM
Similar Open Source Tools
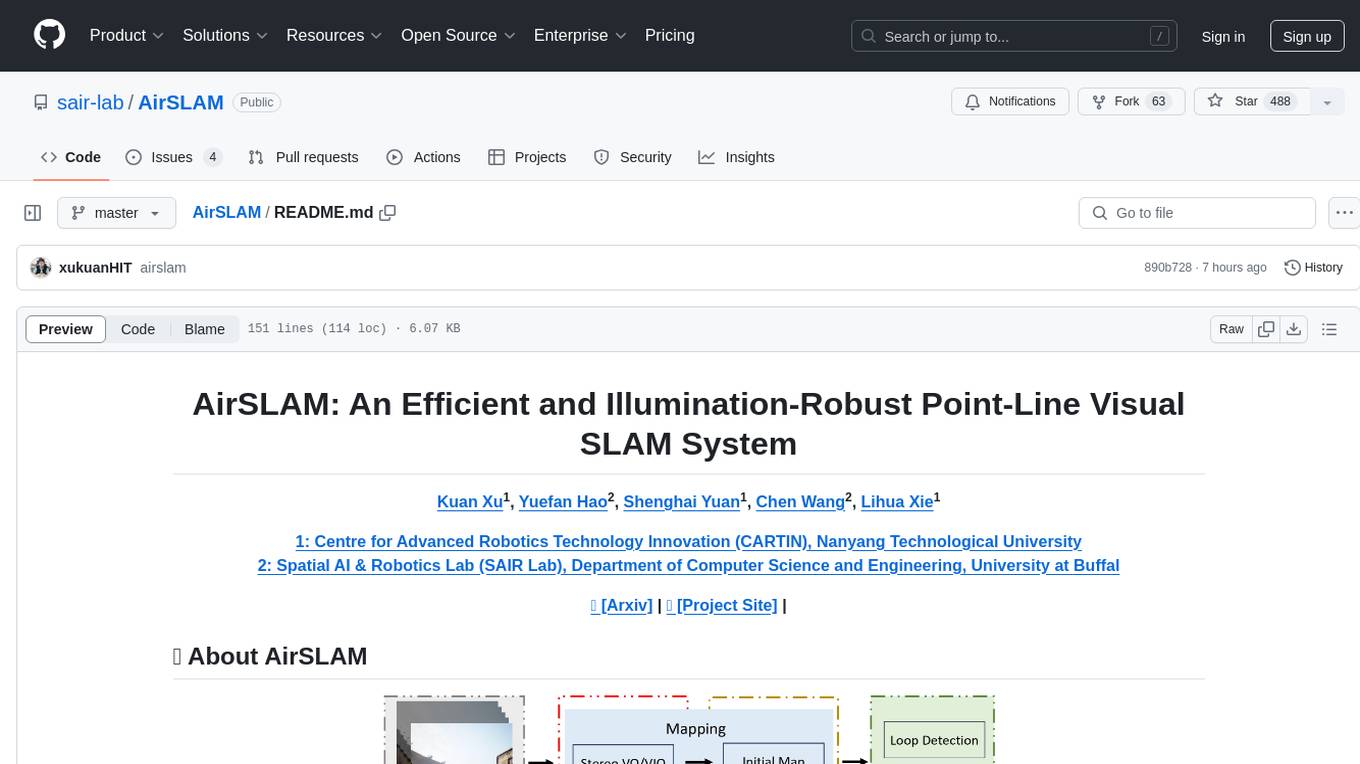
AirSLAM
AirSLAM is an efficient visual SLAM system designed to tackle short-term and long-term illumination challenges. It combines deep learning techniques with traditional optimization methods, featuring a unified CNN for keypoint and structural line extraction. The system includes a relocalization pipeline for map reuse, accelerated using C++ and NVIDIA TensorRT. Outperforming other SLAM systems in challenging environments, it runs at 73Hz on PC and 40Hz on embedded platforms.
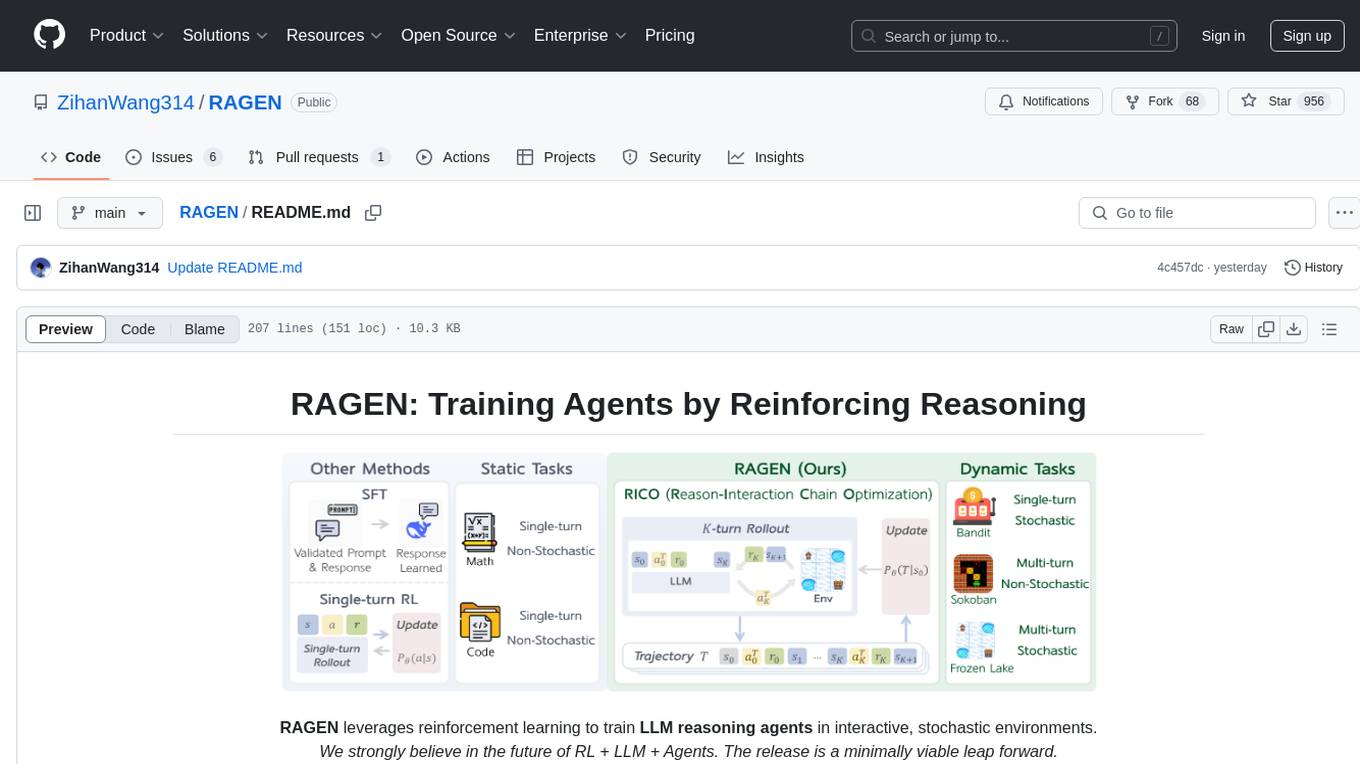
RAGEN
RAGEN is a reinforcement learning framework designed to train reasoning-capable large language model (LLM) agents in interactive, stochastic environments. It addresses challenges such as multi-turn interactions and stochastic environments through a Markov Decision Process (MDP) formulation, Reason-Interaction Chain Optimization (RICO) algorithm, and progressive reward normalization strategies. The framework enables LLMs to reason and interact with the environment, optimizing entire trajectories for long-horizon reasoning while maintaining computational efficiency.
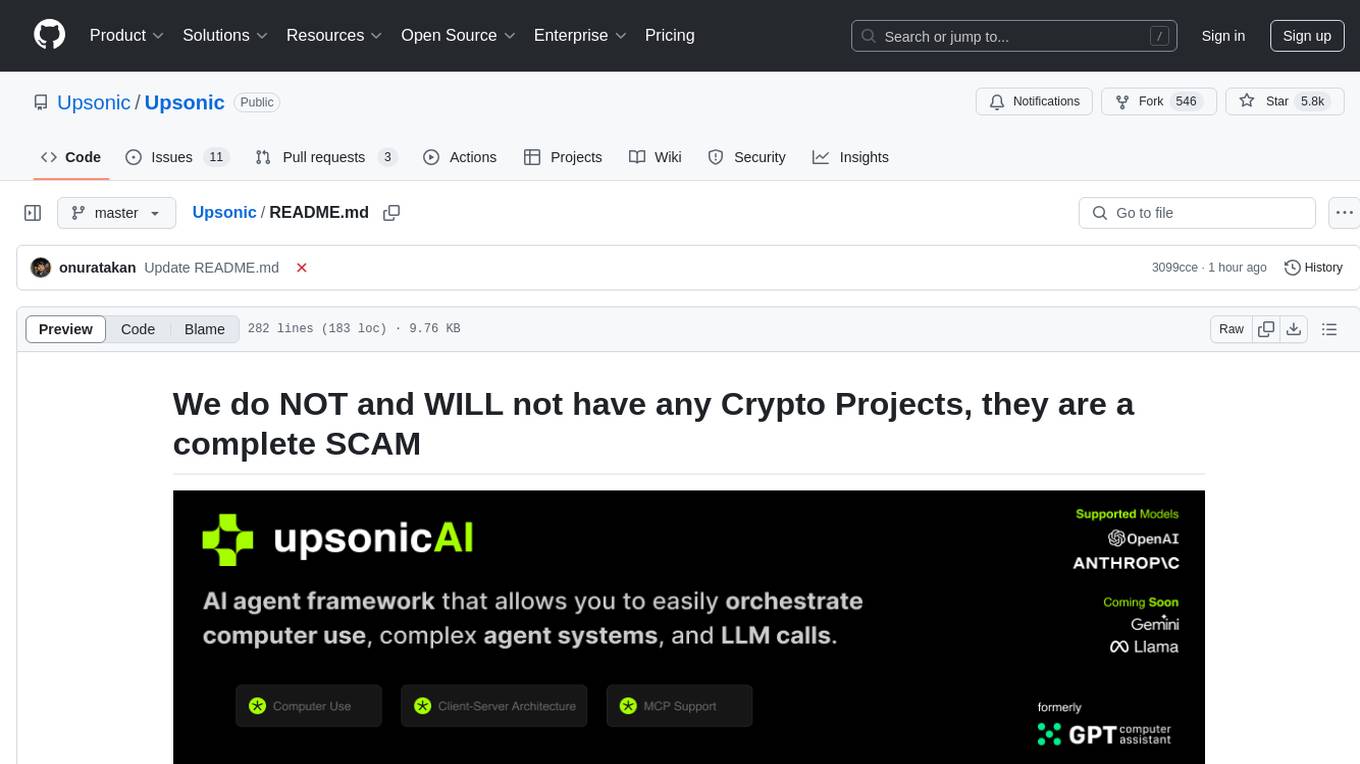
Upsonic
Upsonic offers a cutting-edge enterprise-ready framework for orchestrating LLM calls, agents, and computer use to complete tasks cost-effectively. It provides reliable systems, scalability, and a task-oriented structure for real-world cases. Key features include production-ready scalability, task-centric design, MCP server support, tool-calling server, computer use integration, and easy addition of custom tools. The framework supports client-server architecture and allows seamless deployment on AWS, GCP, or locally using Docker.
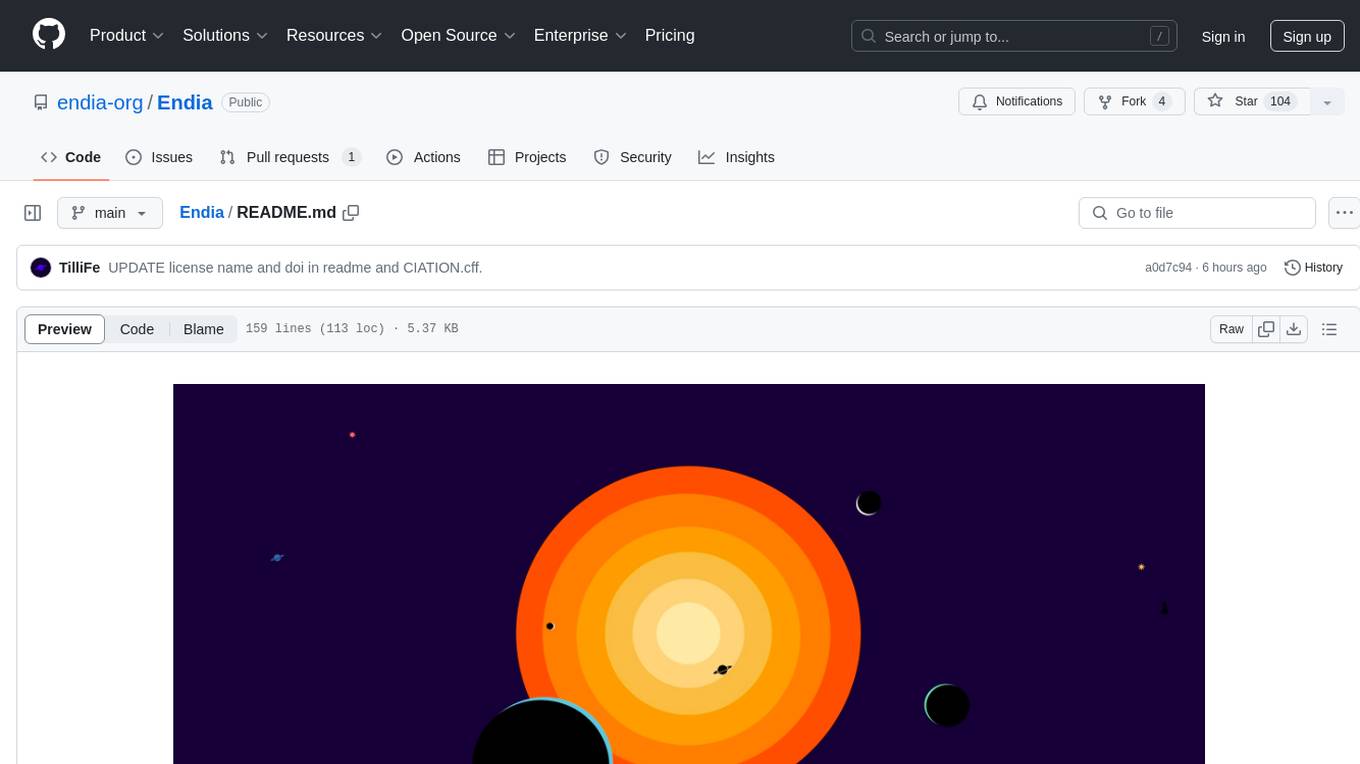
Endia
Endia is a dynamic Array library for Scientific Computing, offering automatic differentiation of arbitrary order, complex number support, dual API with PyTorch-like imperative or JAX-like functional interface, and JIT Compilation for speeding up training and inference. It can handle complex valued functions, perform both forward and reverse-mode automatic differentiation, and has a builtin JIT compiler. Endia aims to advance AI & Scientific Computing by pushing boundaries with clear algorithms, providing high-performance open-source code that remains readable and pythonic, and prioritizing clarity and educational value over exhaustive features.
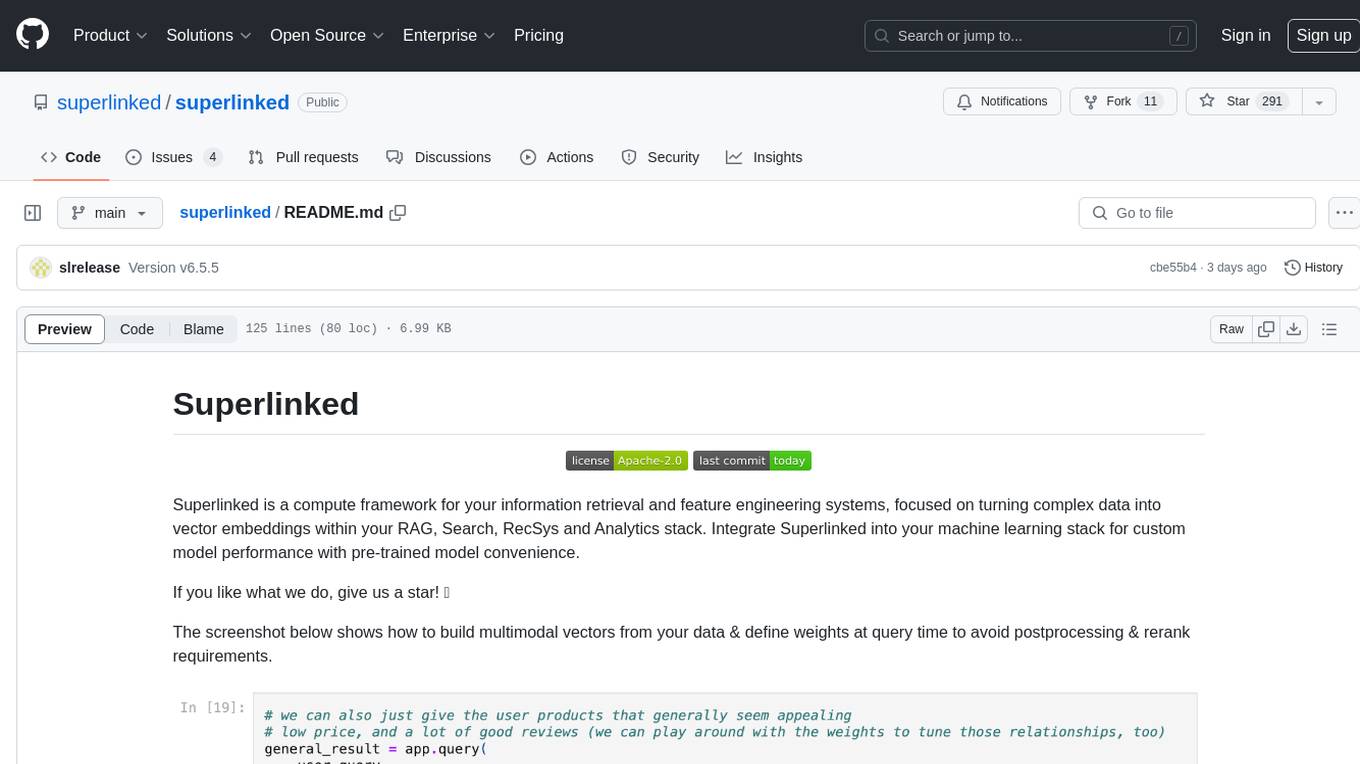
superlinked
Superlinked is a compute framework for information retrieval and feature engineering systems, focusing on converting complex data into vector embeddings for RAG, Search, RecSys, and Analytics stack integration. It enables custom model performance in machine learning with pre-trained model convenience. The tool allows users to build multimodal vectors, define weights at query time, and avoid postprocessing & rerank requirements. Users can explore the computational model through simple scripts and python notebooks, with a future release planned for production usage with built-in data infra and vector database integrations.
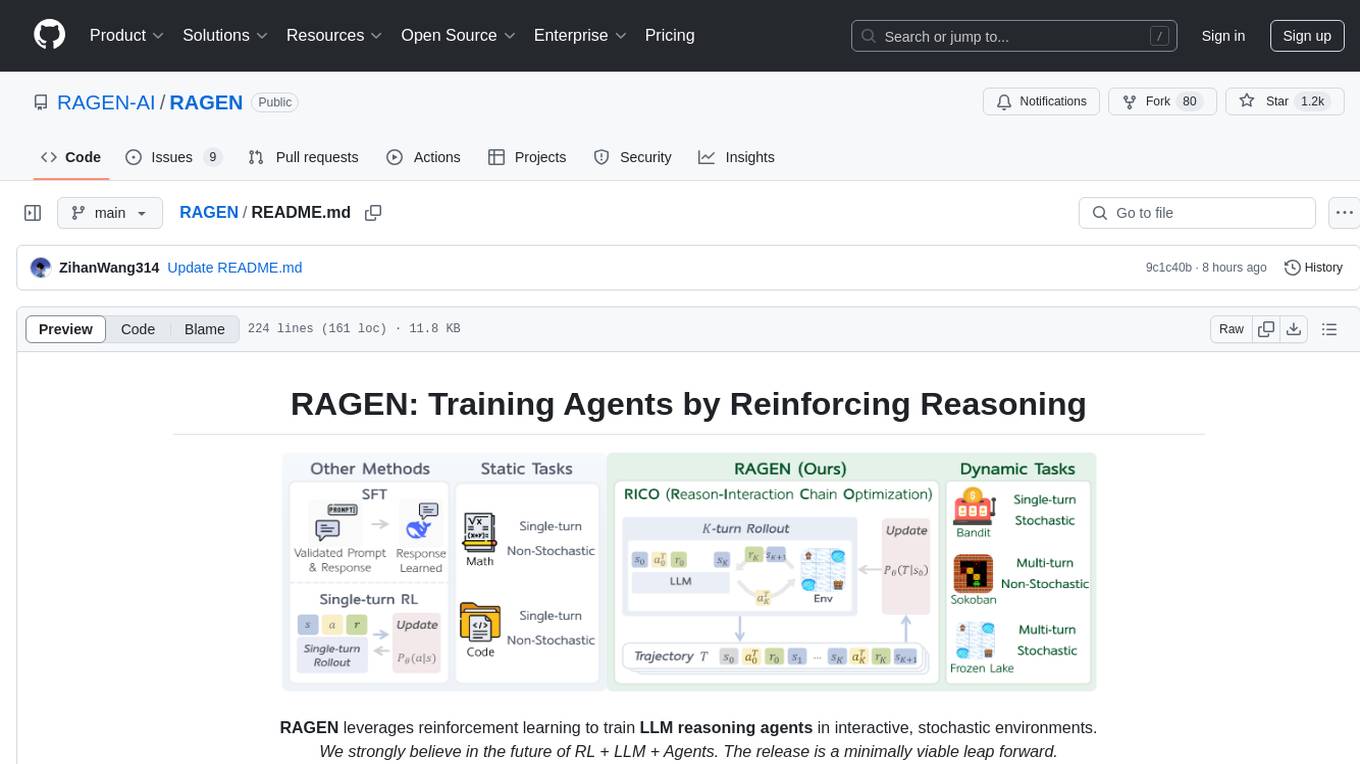
RAGEN
RAGEN is a reinforcement learning framework designed to train reasoning-capable large language model (LLM) agents in interactive, stochastic environments. It addresses challenges such as multi-turn interactions and stochastic environments through a Markov Decision Process (MDP) formulation, Reason-Interaction Chain Optimization (RICO) algorithm, and progressive reward normalization strategies. The framework consists of MDP formulation, RICO algorithm with rollout and update stages, and reward normalization strategies to stabilize training. RAGEN aims to optimize reasoning and action strategies for LLM agents operating in complex environments.
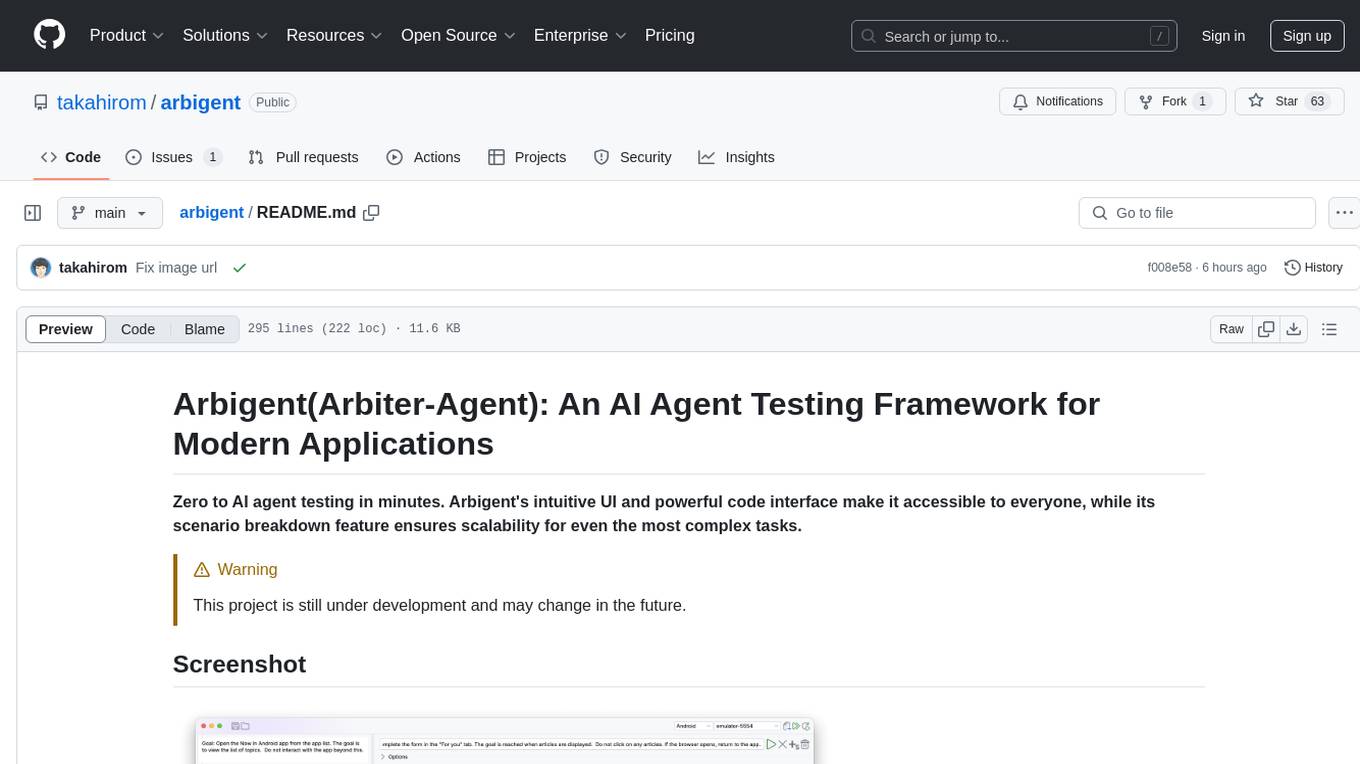
arbigent
Arbigent (Arbiter-Agent) is an AI agent testing framework designed to make AI agent testing practical for modern applications. It addresses challenges faced by traditional UI testing frameworks and AI agents by breaking down complex tasks into smaller, dependent scenarios. The framework is customizable for various AI providers, operating systems, and form factors, empowering users with extensive customization capabilities. Arbigent offers an intuitive UI for scenario creation and a powerful code interface for seamless test execution. It supports multiple form factors, optimizes UI for AI interaction, and is cost-effective by utilizing models like GPT-4o mini. With a flexible code interface and open-source nature, Arbigent aims to revolutionize AI agent testing in modern applications.
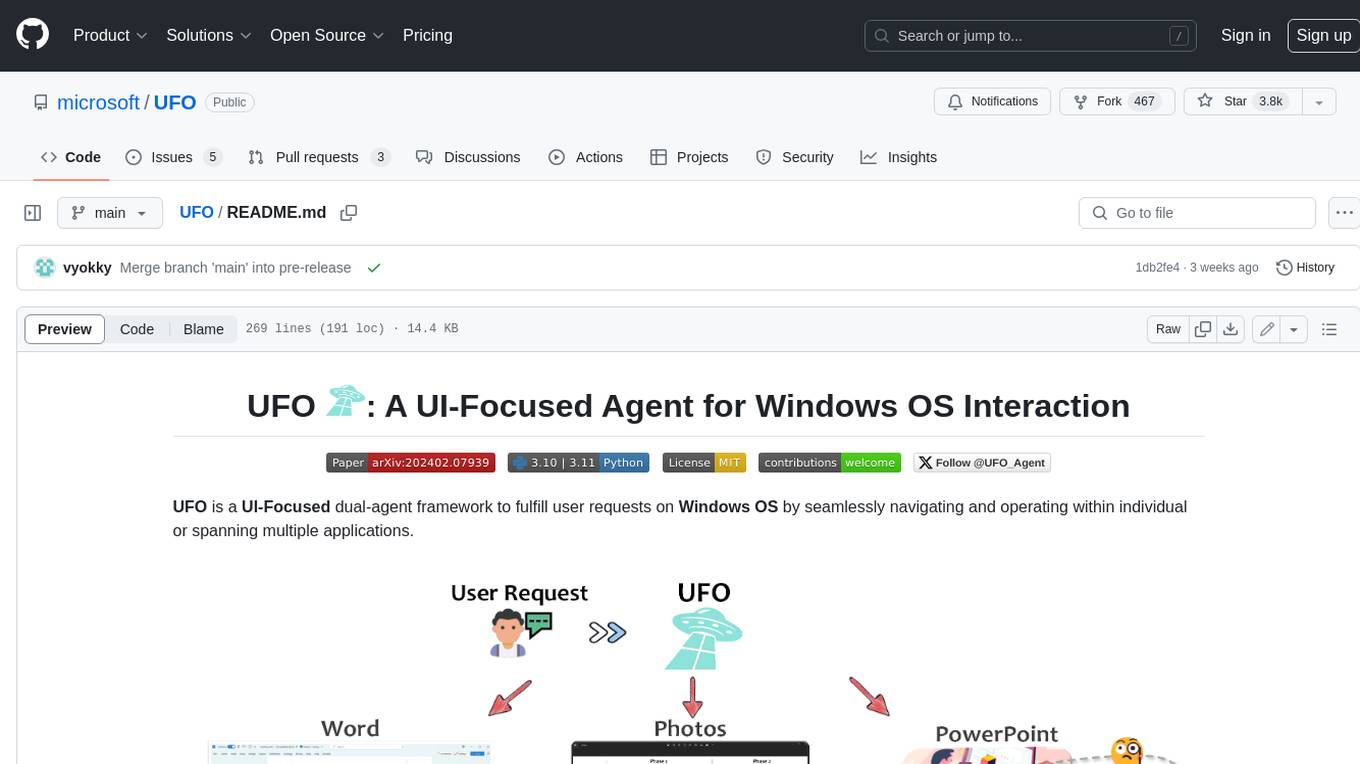
UFO
UFO is a UI-focused dual-agent framework to fulfill user requests on Windows OS by seamlessly navigating and operating within individual or spanning multiple applications.
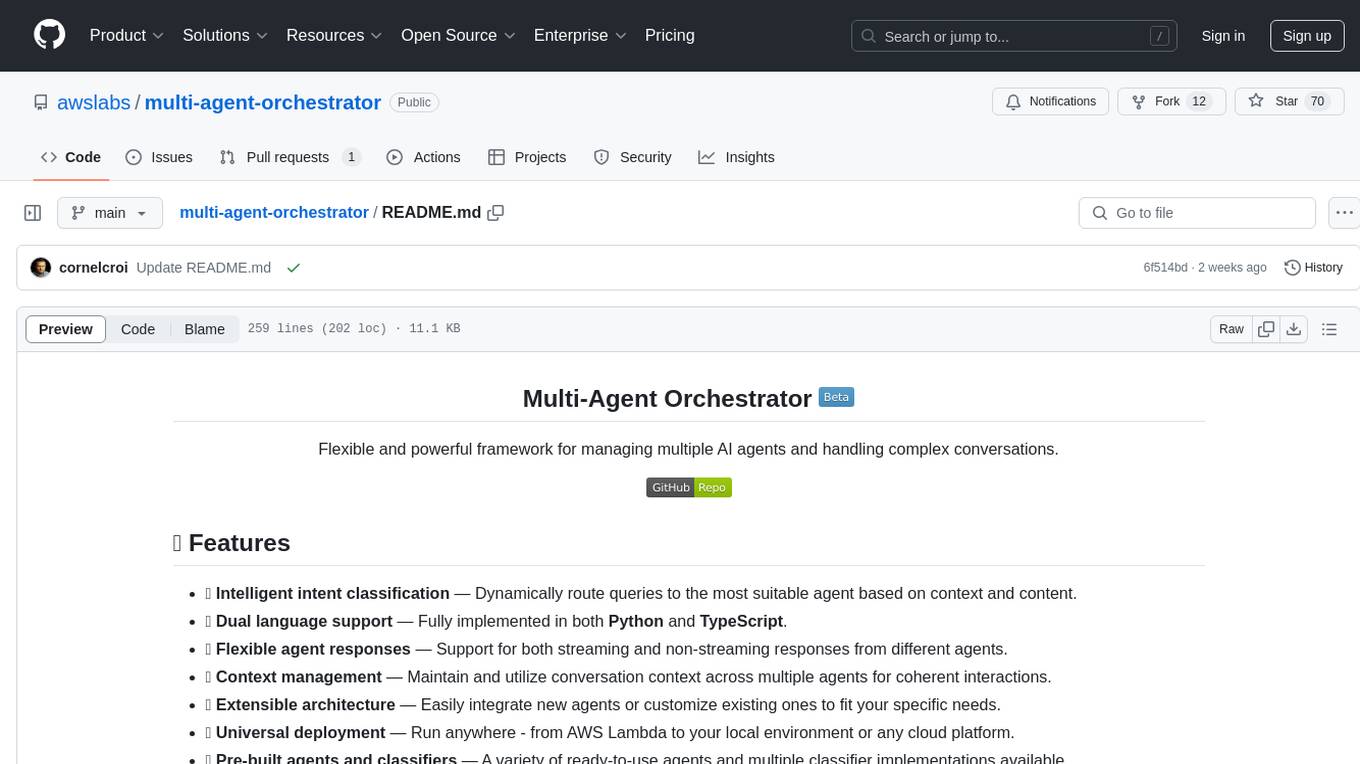
multi-agent-orchestrator
Multi-Agent Orchestrator is a flexible and powerful framework for managing multiple AI agents and handling complex conversations. It intelligently routes queries to the most suitable agent based on context and content, supports dual language implementation in Python and TypeScript, offers flexible agent responses, context management across agents, extensible architecture for customization, universal deployment options, and pre-built agents and classifiers. It is suitable for various applications, from simple chatbots to sophisticated AI systems, accommodating diverse requirements and scaling efficiently.
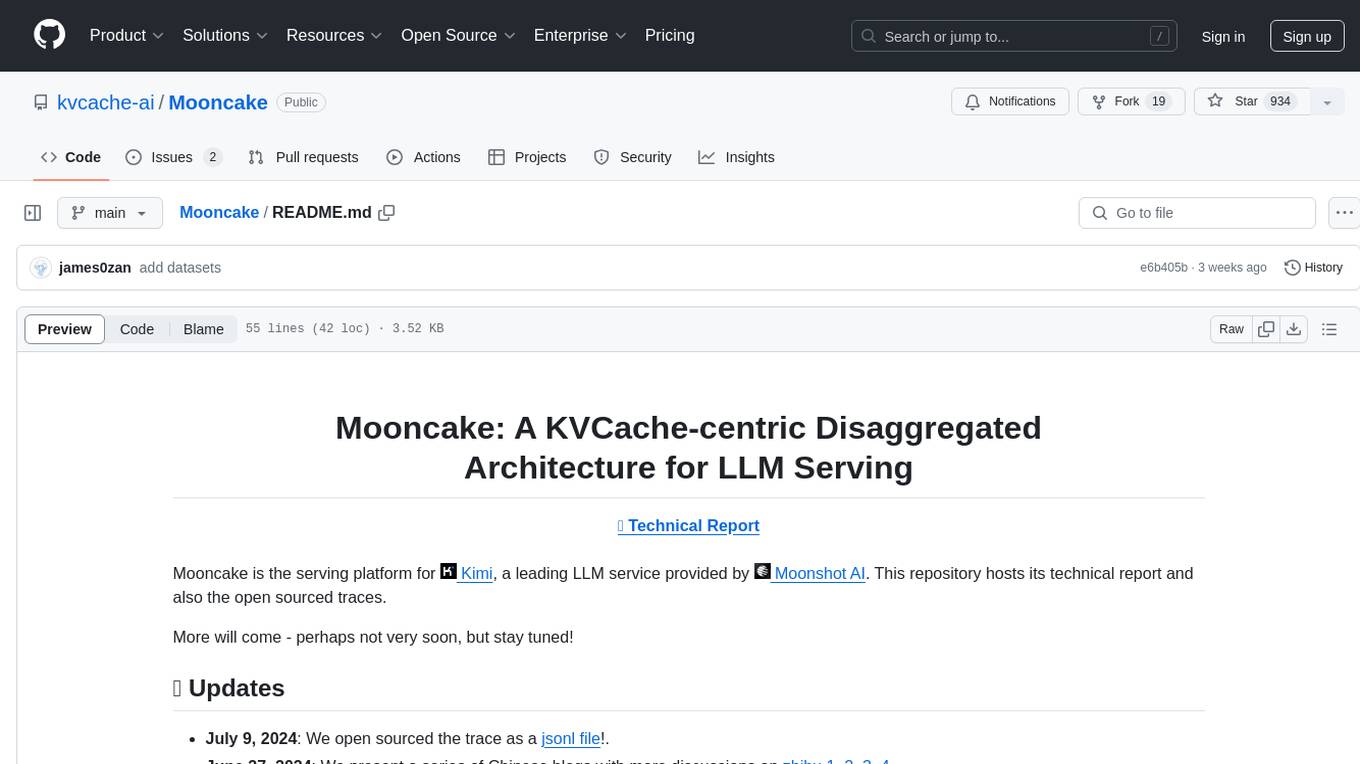
Mooncake
Mooncake is a serving platform for Kimi, a leading LLM service provided by Moonshot AI. It features a KVCache-centric disaggregated architecture that separates prefill and decoding clusters, leveraging underutilized CPU, DRAM, and SSD resources of the GPU cluster. Mooncake's scheduler balances throughput and latency-related SLOs, with a prediction-based early rejection policy for highly overloaded scenarios. It excels in long-context scenarios, achieving up to a 525% increase in throughput while handling 75% more requests under real workloads.
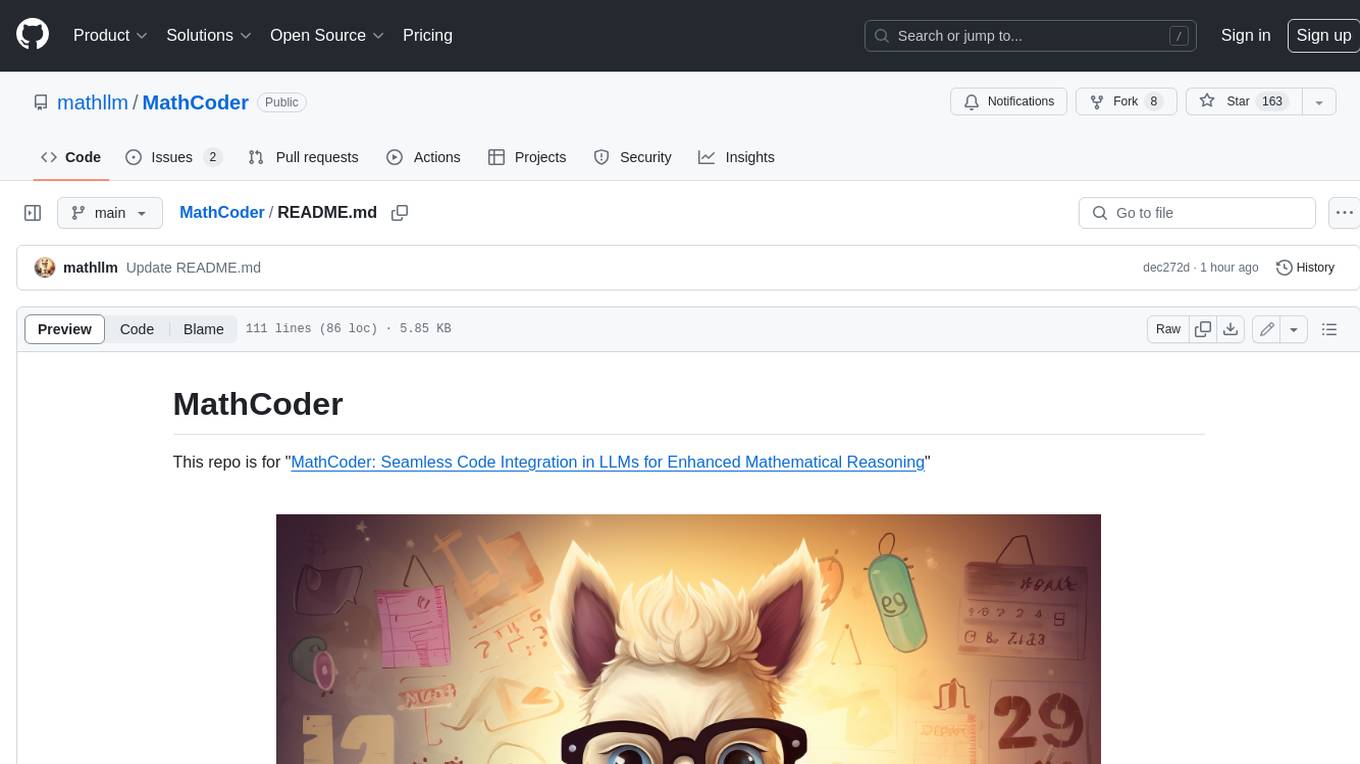
MathCoder
MathCoder is a repository focused on enhancing mathematical reasoning by fine-tuning open-source language models to use code for modeling and deriving math equations. It introduces MathCodeInstruct dataset with solutions interleaving natural language, code, and execution results. The repository provides MathCoder models capable of generating code-based solutions for challenging math problems, achieving state-of-the-art scores on MATH and GSM8K datasets. It offers tools for model deployment, inference, and evaluation, along with a citation for referencing the work.
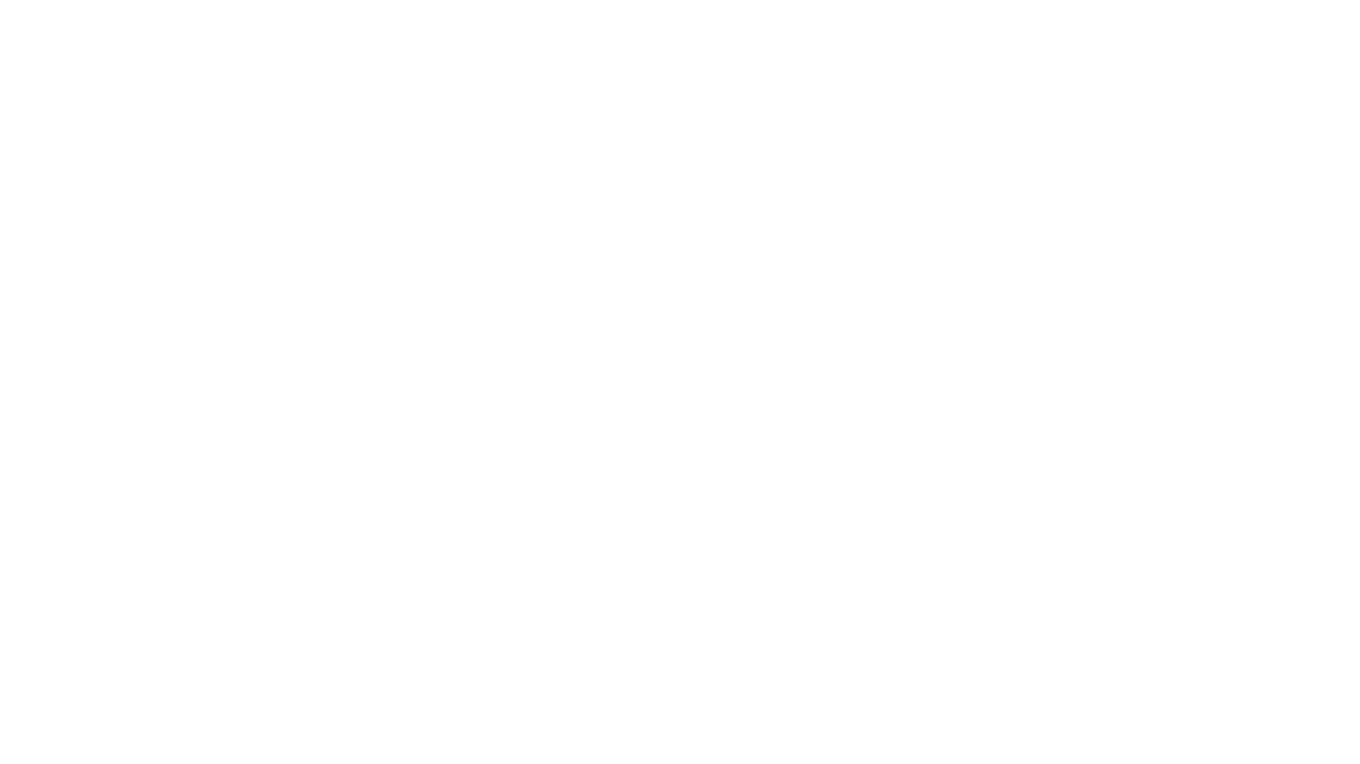
motia
Motia is an AI agent framework designed for software engineers to create, test, and deploy production-ready AI agents quickly. It provides a code-first approach, allowing developers to write agent logic in familiar languages and visualize execution in real-time. With Motia, developers can focus on business logic rather than infrastructure, offering zero infrastructure headaches, multi-language support, composable steps, built-in observability, instant APIs, and full control over AI logic. Ideal for building sophisticated agents and intelligent automations, Motia's event-driven architecture and modular steps enable the creation of GenAI-powered workflows, decision-making systems, and data processing pipelines.
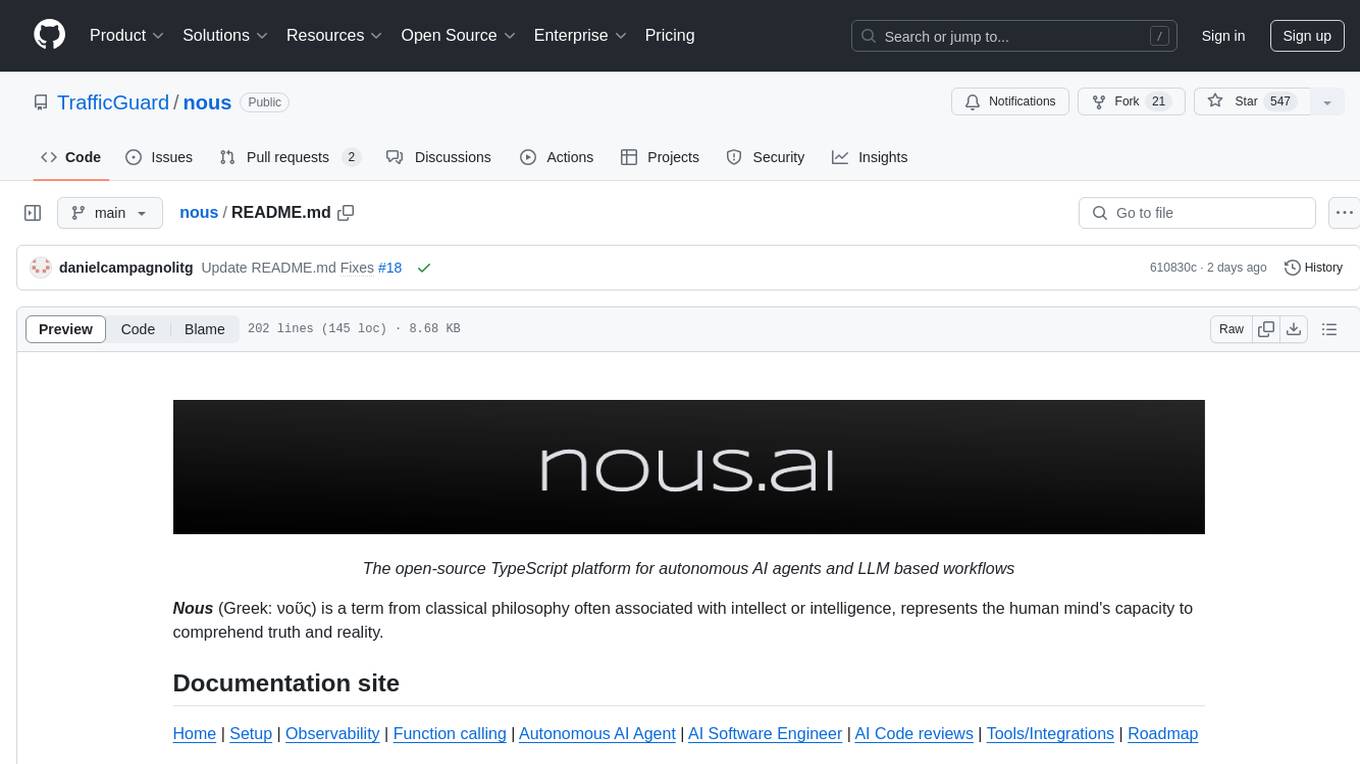
nous
Nous is an open-source TypeScript platform for autonomous AI agents and LLM based workflows. It aims to automate processes, support requests, review code, assist with refactorings, and more. The platform supports various integrations, multiple LLMs/services, CLI and web interface, human-in-the-loop interactions, flexible deployment options, observability with OpenTelemetry tracing, and specific agents for code editing, software engineering, and code review. It offers advanced features like reasoning/planning, memory and function call history, hierarchical task decomposition, and control-loop function calling options. Nous is designed to be a flexible platform for the TypeScript community to expand and support different use cases and integrations.
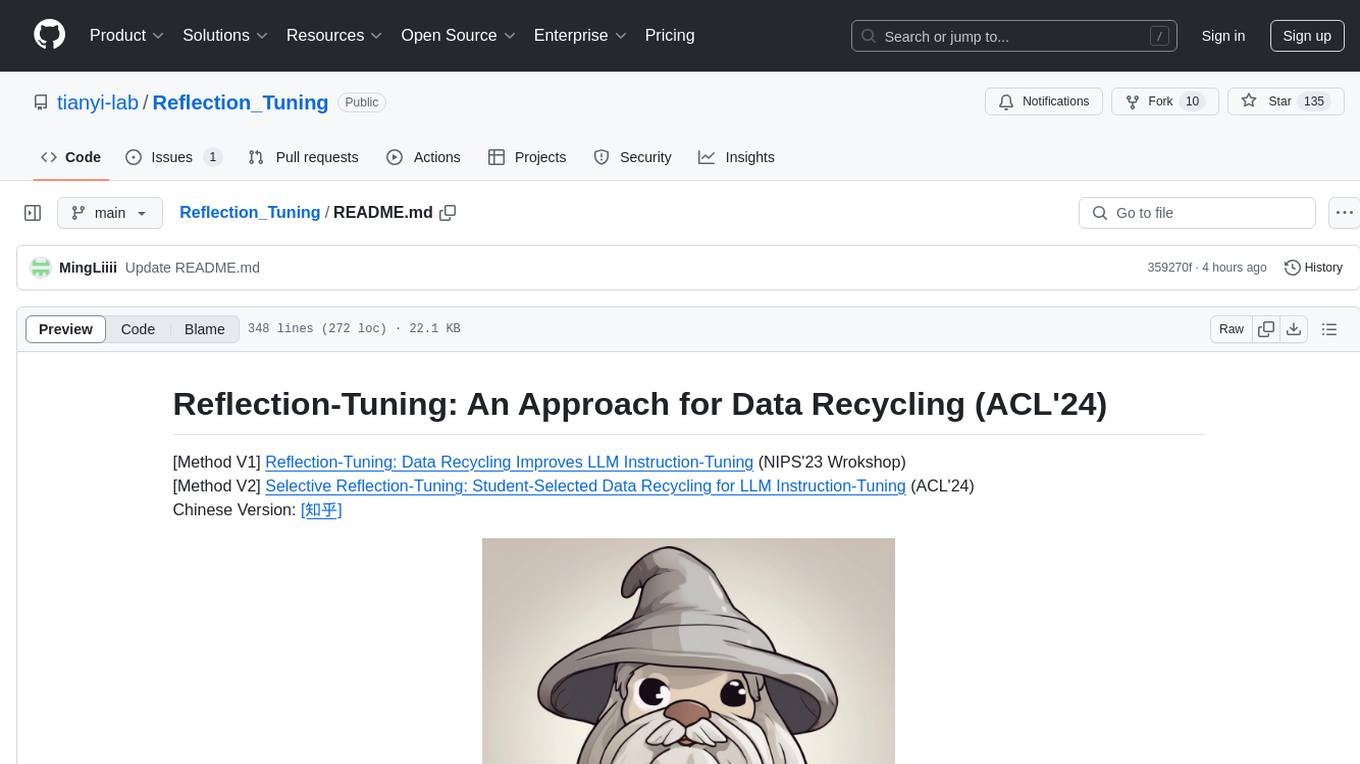
Reflection_Tuning
Reflection-Tuning is a project focused on improving the quality of instruction-tuning data through a reflection-based method. It introduces Selective Reflection-Tuning, where the student model can decide whether to accept the improvements made by the teacher model. The project aims to generate high-quality instruction-response pairs by defining specific criteria for the oracle model to follow and respond to. It also evaluates the efficacy and relevance of instruction-response pairs using the r-IFD metric. The project provides code for reflection and selection processes, along with data and model weights for both V1 and V2 methods.
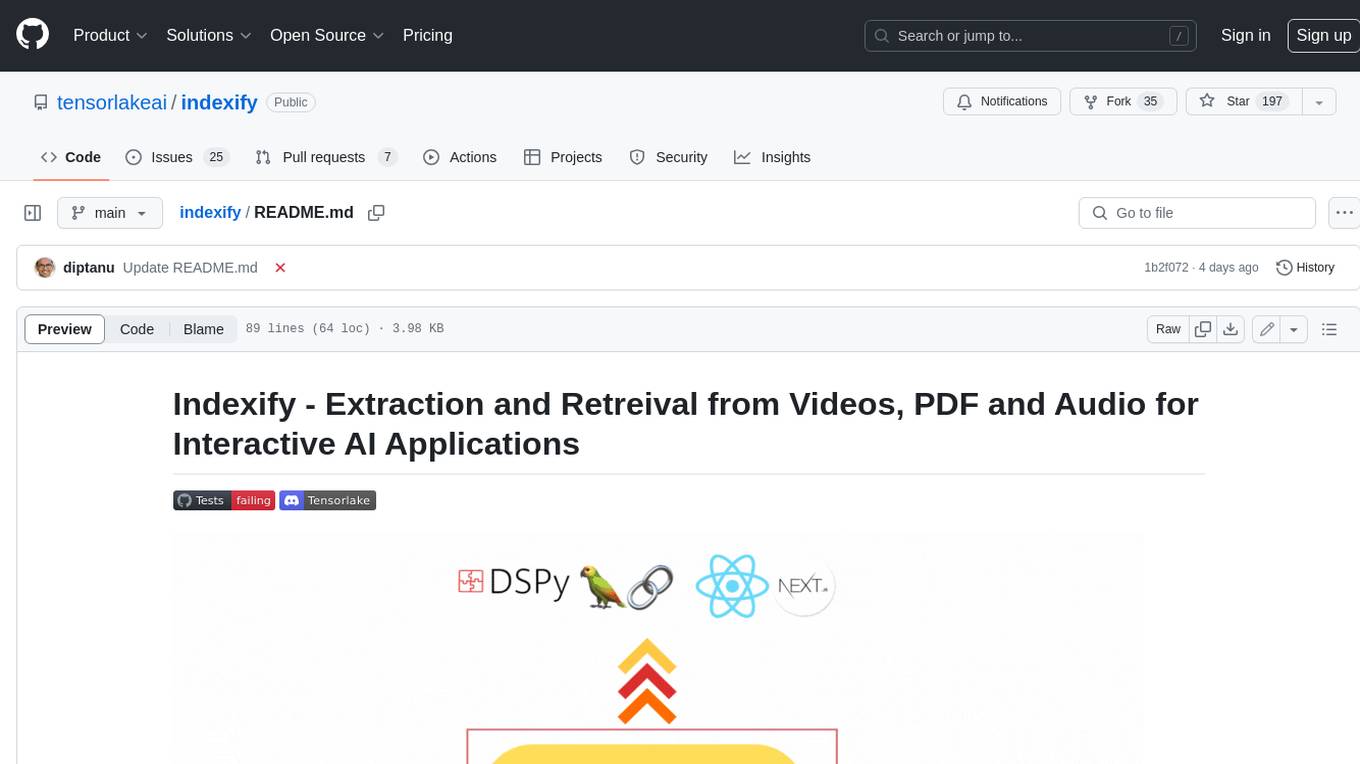
indexify
Indexify is an open-source engine for building fast data pipelines for unstructured data (video, audio, images, and documents) using reusable extractors for embedding, transformation, and feature extraction. LLM Applications can query transformed content friendly to LLMs by semantic search and SQL queries. Indexify keeps vector databases and structured databases (PostgreSQL) updated by automatically invoking the pipelines as new data is ingested into the system from external data sources. **Why use Indexify** * Makes Unstructured Data **Queryable** with **SQL** and **Semantic Search** * **Real-Time** Extraction Engine to keep indexes **automatically** updated as new data is ingested. * Create **Extraction Graph** to describe **data transformation** and extraction of **embedding** and **structured extraction**. * **Incremental Extraction** and **Selective Deletion** when content is deleted or updated. * **Extractor SDK** allows adding new extraction capabilities, and many readily available extractors for **PDF**, **Image**, and **Video** indexing and extraction. * Works with **any LLM Framework** including **Langchain**, **DSPy**, etc. * Runs on your laptop during **prototyping** and also scales to **1000s of machines** on the cloud. * Works with many **Blob Stores**, **Vector Stores**, and **Structured Databases** * We have even **Open Sourced Automation** to deploy to Kubernetes in production.
For similar tasks
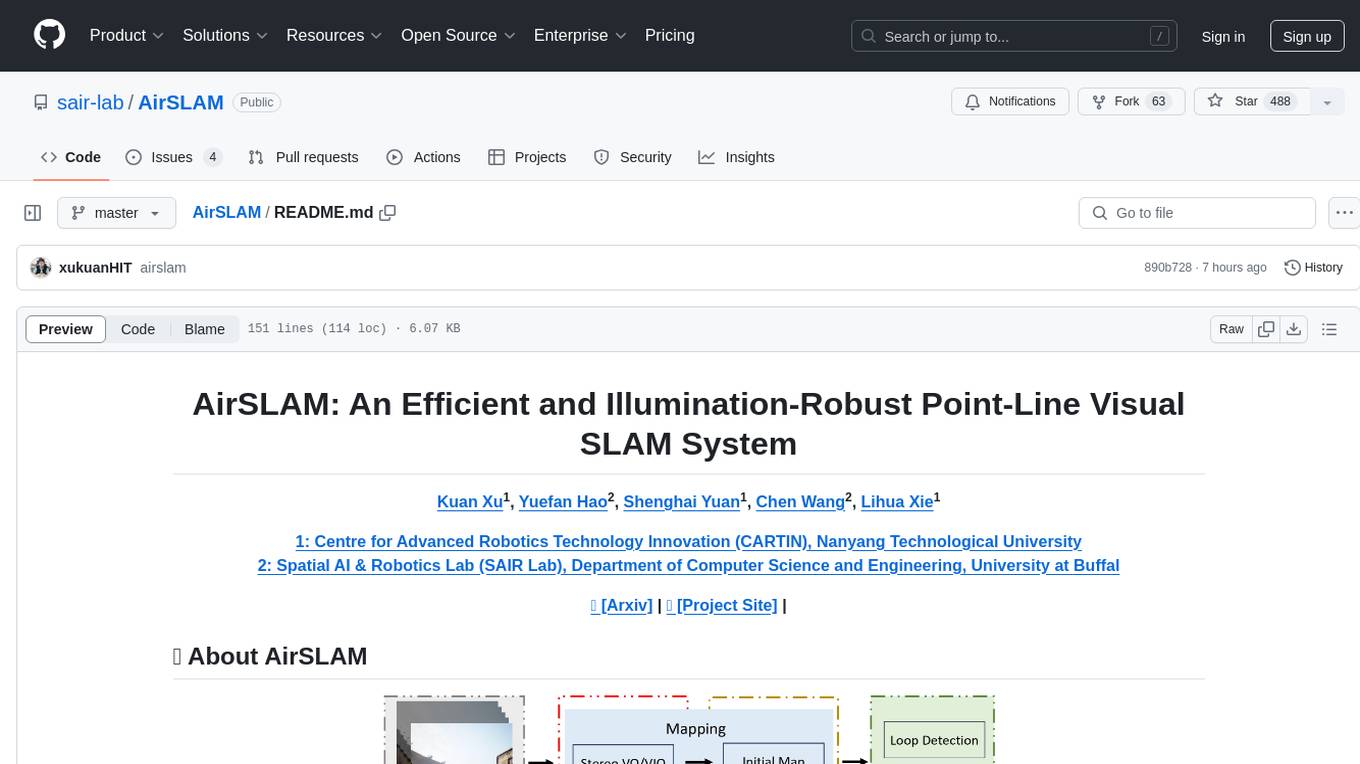
AirSLAM
AirSLAM is an efficient visual SLAM system designed to tackle short-term and long-term illumination challenges. It combines deep learning techniques with traditional optimization methods, featuring a unified CNN for keypoint and structural line extraction. The system includes a relocalization pipeline for map reuse, accelerated using C++ and NVIDIA TensorRT. Outperforming other SLAM systems in challenging environments, it runs at 73Hz on PC and 40Hz on embedded platforms.
For similar jobs
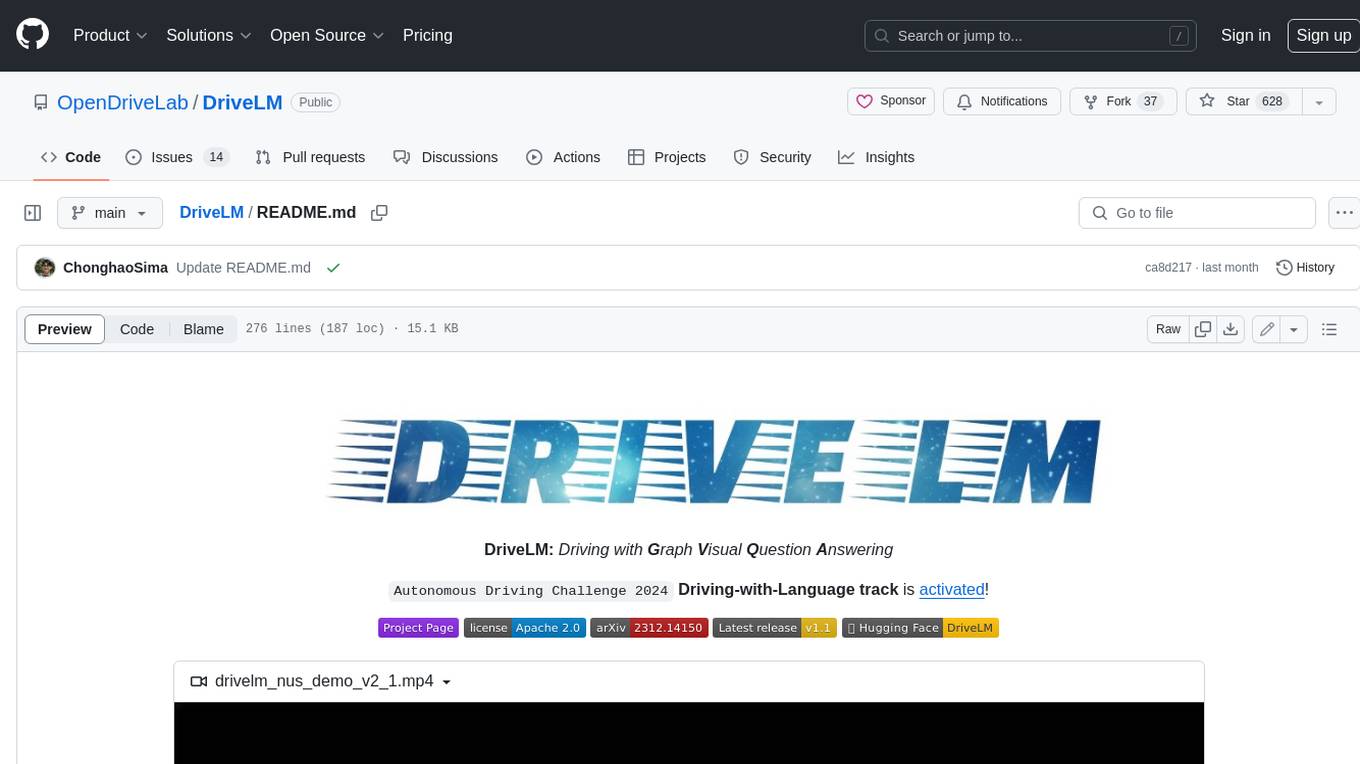
DriveLM
DriveLM is a multimodal AI model that enables autonomous driving by combining computer vision and natural language processing. It is designed to understand and respond to complex driving scenarios using visual and textual information. DriveLM can perform various tasks related to driving, such as object detection, lane keeping, and decision-making. It is trained on a massive dataset of images and text, which allows it to learn the relationships between visual cues and driving actions. DriveLM is a powerful tool that can help to improve the safety and efficiency of autonomous vehicles.
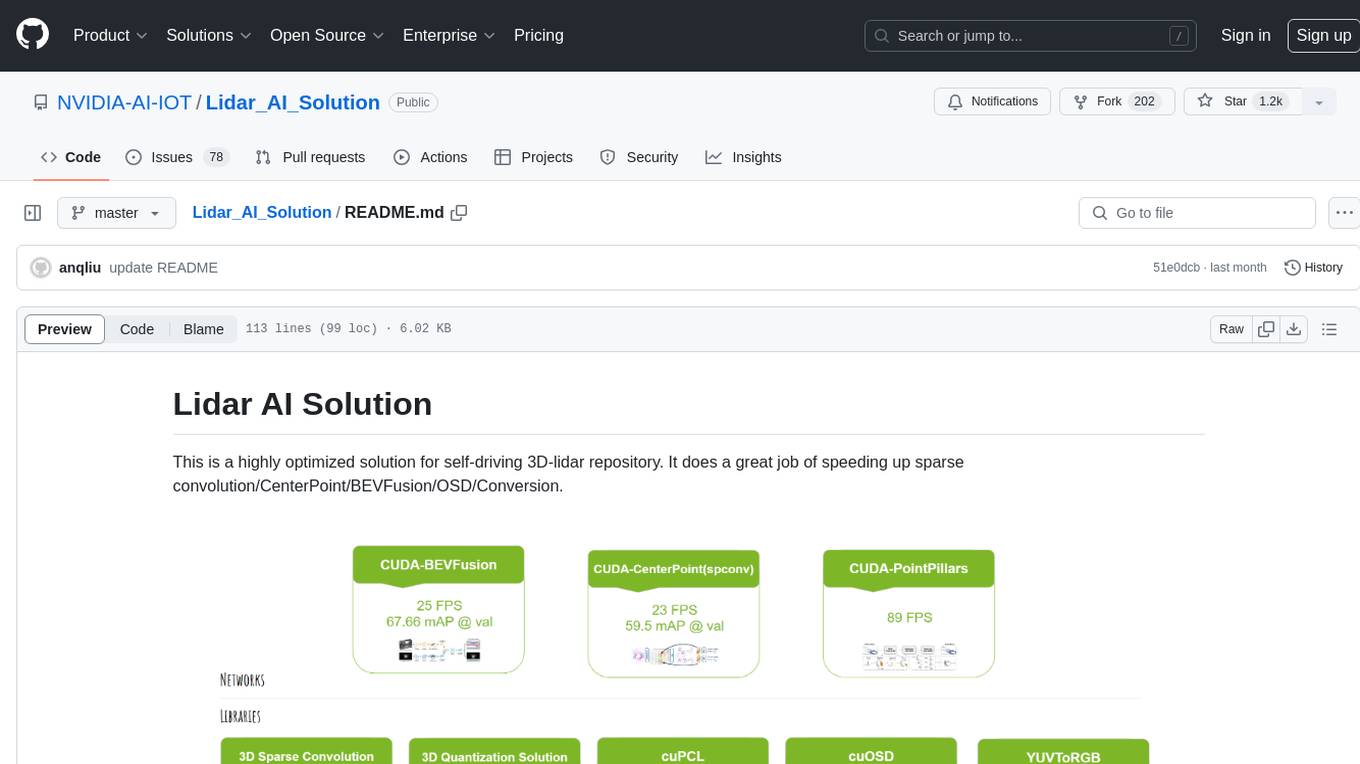
Lidar_AI_Solution
Lidar AI Solution is a highly optimized repository for self-driving 3D lidar, providing solutions for sparse convolution, BEVFusion, CenterPoint, OSD, and Conversion. It includes CUDA and TensorRT implementations for various tasks such as 3D sparse convolution, BEVFusion, CenterPoint, PointPillars, V2XFusion, cuOSD, cuPCL, and YUV to RGB conversion. The repository offers easy-to-use solutions, high accuracy, low memory usage, and quantization options for different tasks related to self-driving technology.
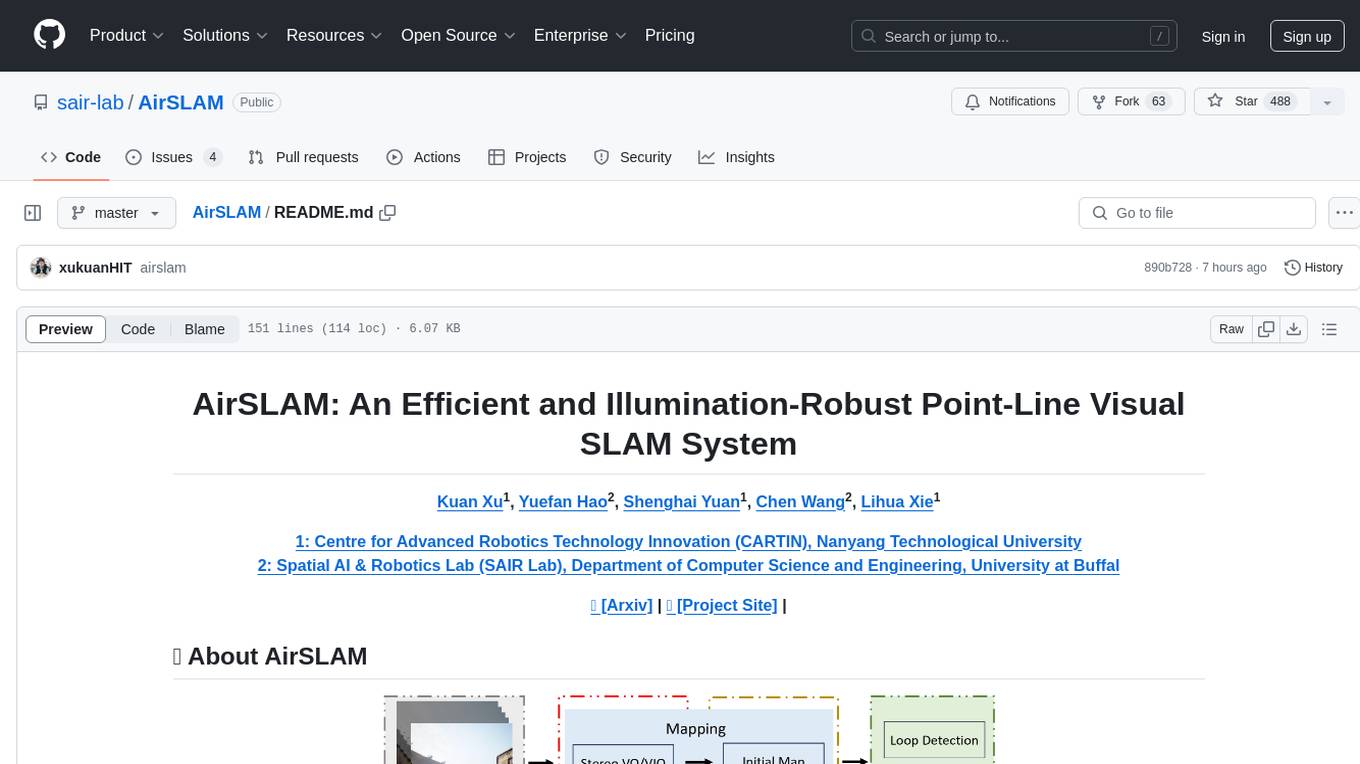
AirSLAM
AirSLAM is an efficient visual SLAM system designed to tackle short-term and long-term illumination challenges. It combines deep learning techniques with traditional optimization methods, featuring a unified CNN for keypoint and structural line extraction. The system includes a relocalization pipeline for map reuse, accelerated using C++ and NVIDIA TensorRT. Outperforming other SLAM systems in challenging environments, it runs at 73Hz on PC and 40Hz on embedded platforms.
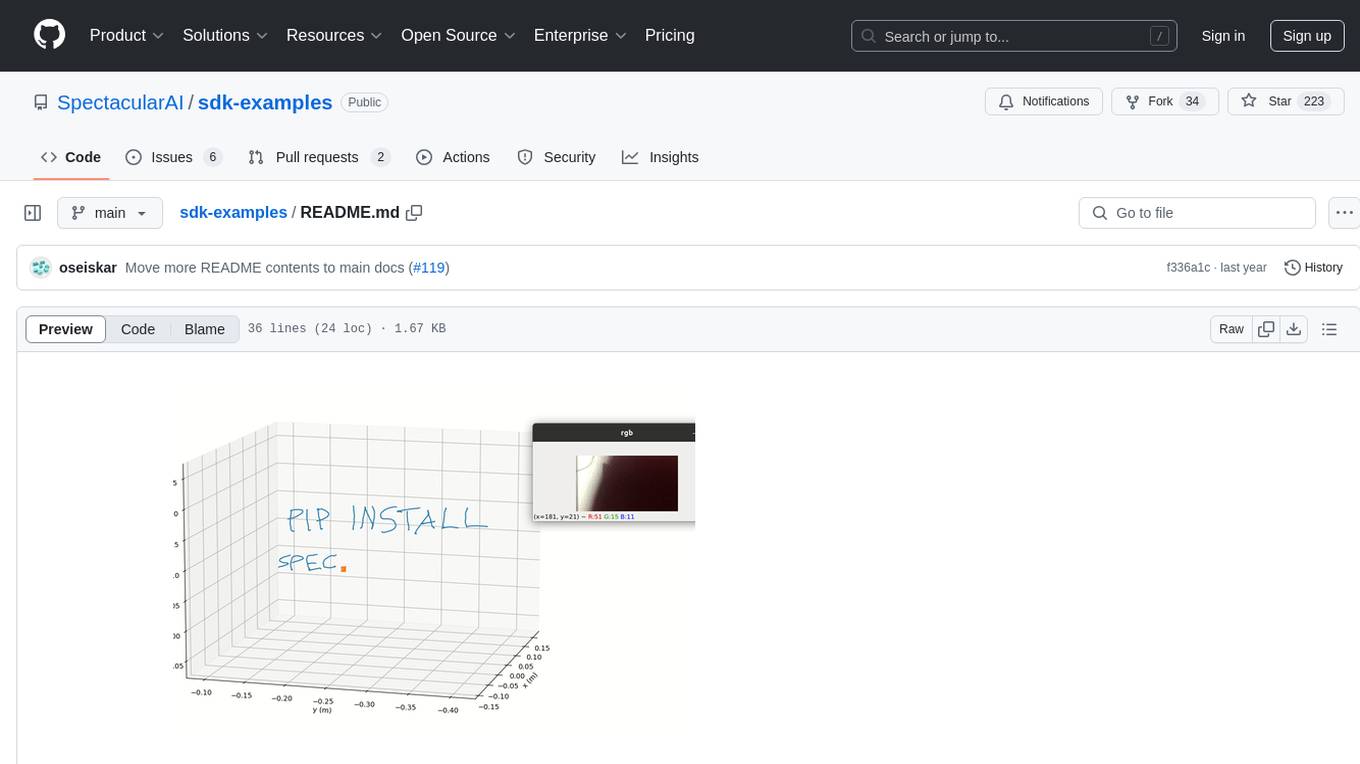
sdk-examples
Spectacular AI SDK fuses data from cameras and IMU sensors to output an accurate 6-degree-of-freedom pose of a device, enabling Visual-Inertial SLAM for tracking robots and vehicles, as well as Augmented, Mixed, and Virtual Reality. The SDK includes a Mapping API for real-time and offline 3D reconstruction use cases.
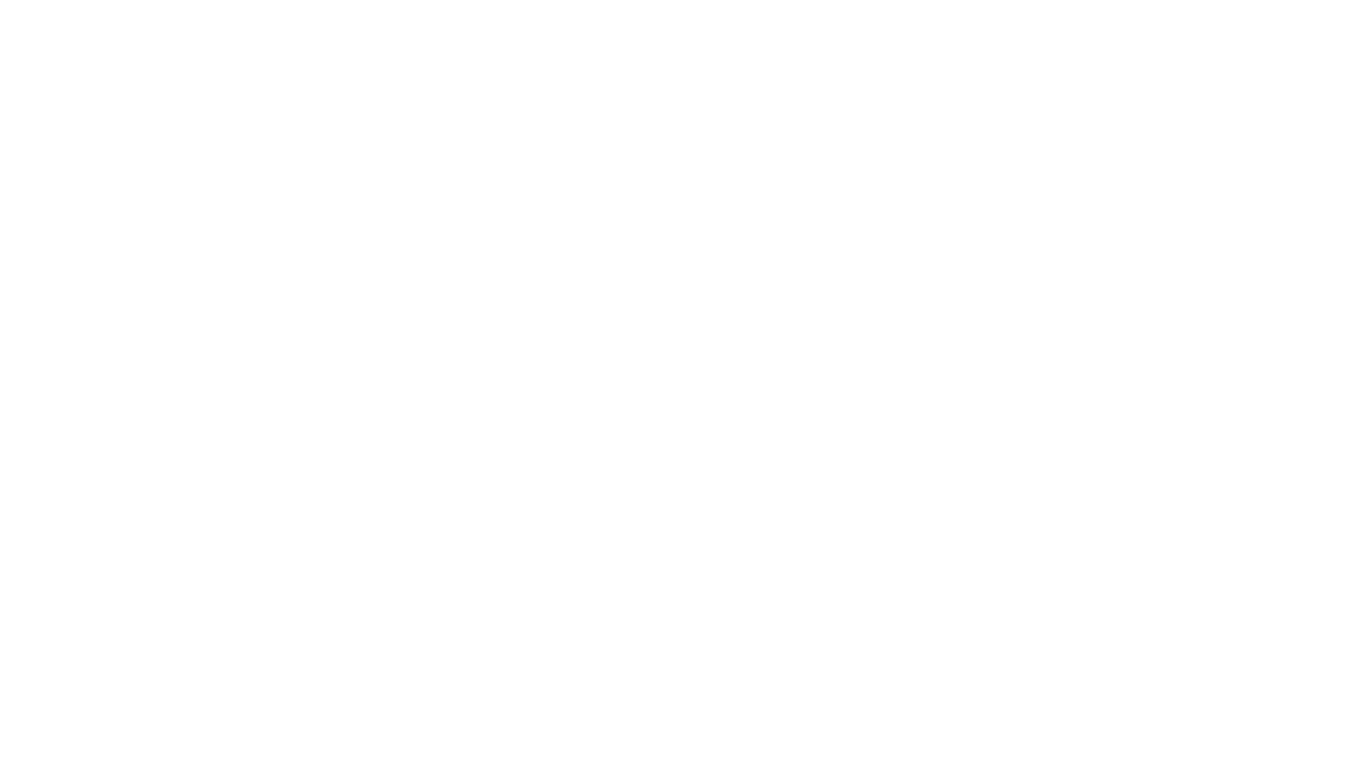
awesome-and-novel-works-in-slam
This repository contains a curated list of cutting-edge works in Simultaneous Localization and Mapping (SLAM). It includes research papers, projects, and tools related to various aspects of SLAM, such as 3D reconstruction, semantic mapping, novel algorithms, large-scale mapping, and more. The repository aims to showcase the latest advancements in SLAM technology and provide resources for researchers and practitioners in the field.
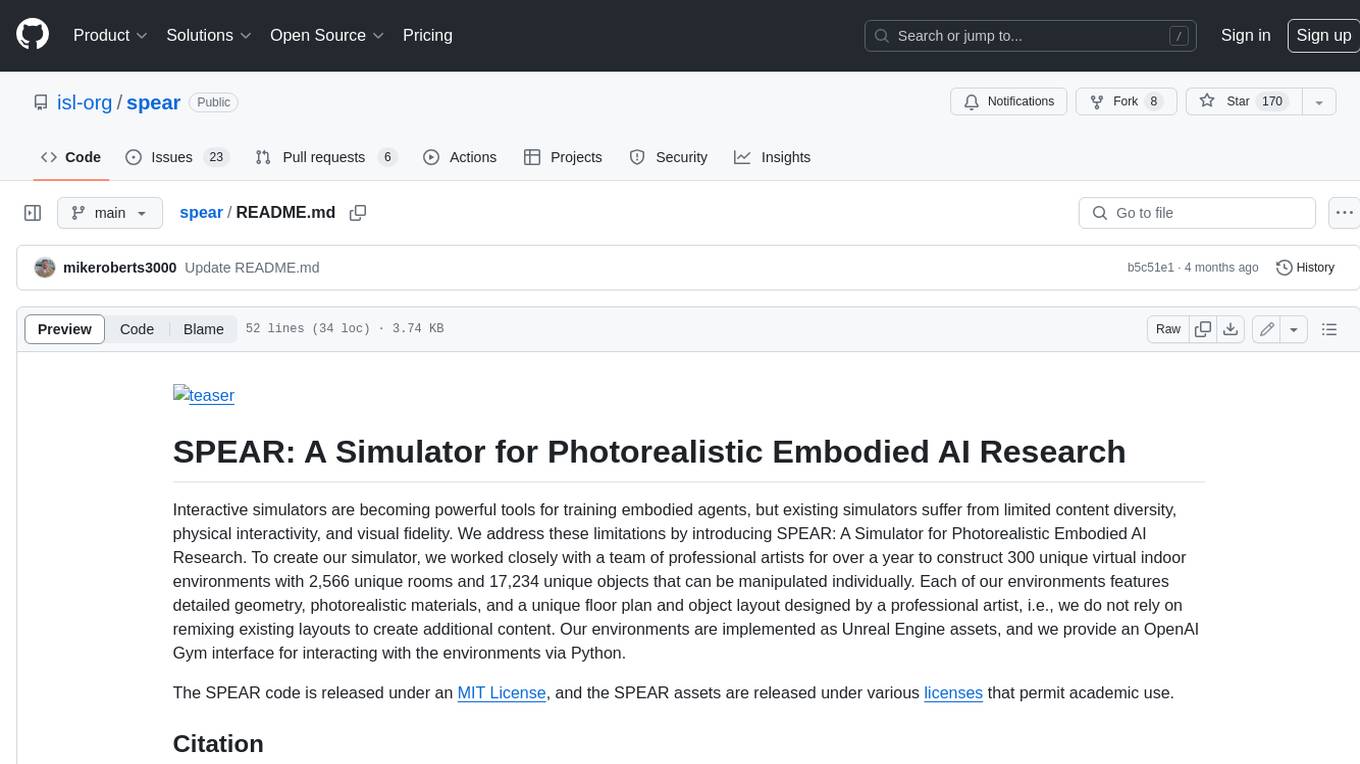
spear
SPEAR (Simulator for Photorealistic Embodied AI Research) is a powerful tool for training embodied agents. It features 300 unique virtual indoor environments with 2,566 unique rooms and 17,234 unique objects that can be manipulated individually. Each environment is designed by a professional artist and features detailed geometry, photorealistic materials, and a unique floor plan and object layout. SPEAR is implemented as Unreal Engine assets and provides an OpenAI Gym interface for interacting with the environments via Python.
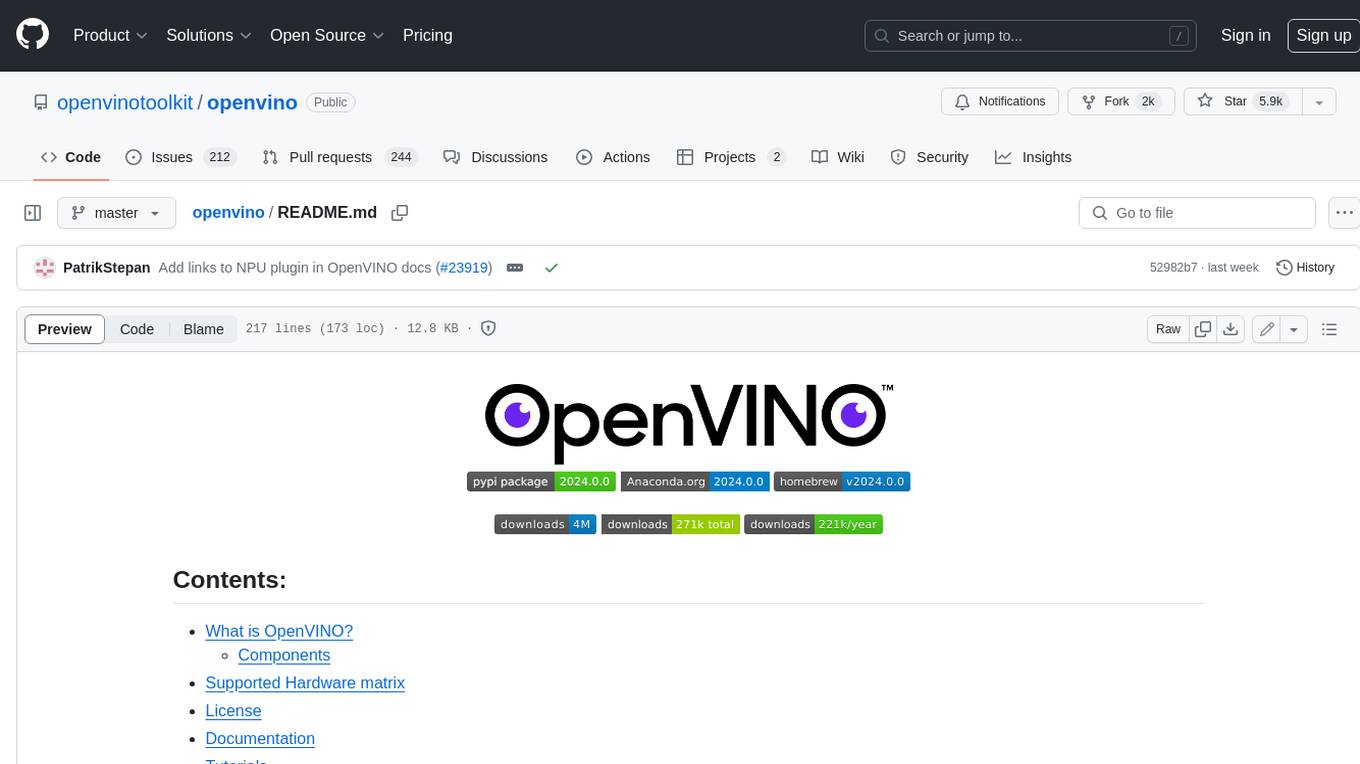
openvino
OpenVINOβ’ is an open-source toolkit for optimizing and deploying AI inference. It provides a common API to deliver inference solutions on various platforms, including CPU, GPU, NPU, and heterogeneous devices. OpenVINOβ’ supports pre-trained models from Open Model Zoo and popular frameworks like TensorFlow, PyTorch, and ONNX. Key components of OpenVINOβ’ include the OpenVINOβ’ Runtime, plugins for different hardware devices, frontends for reading models from native framework formats, and the OpenVINO Model Converter (OVC) for adjusting models for optimal execution on target devices.
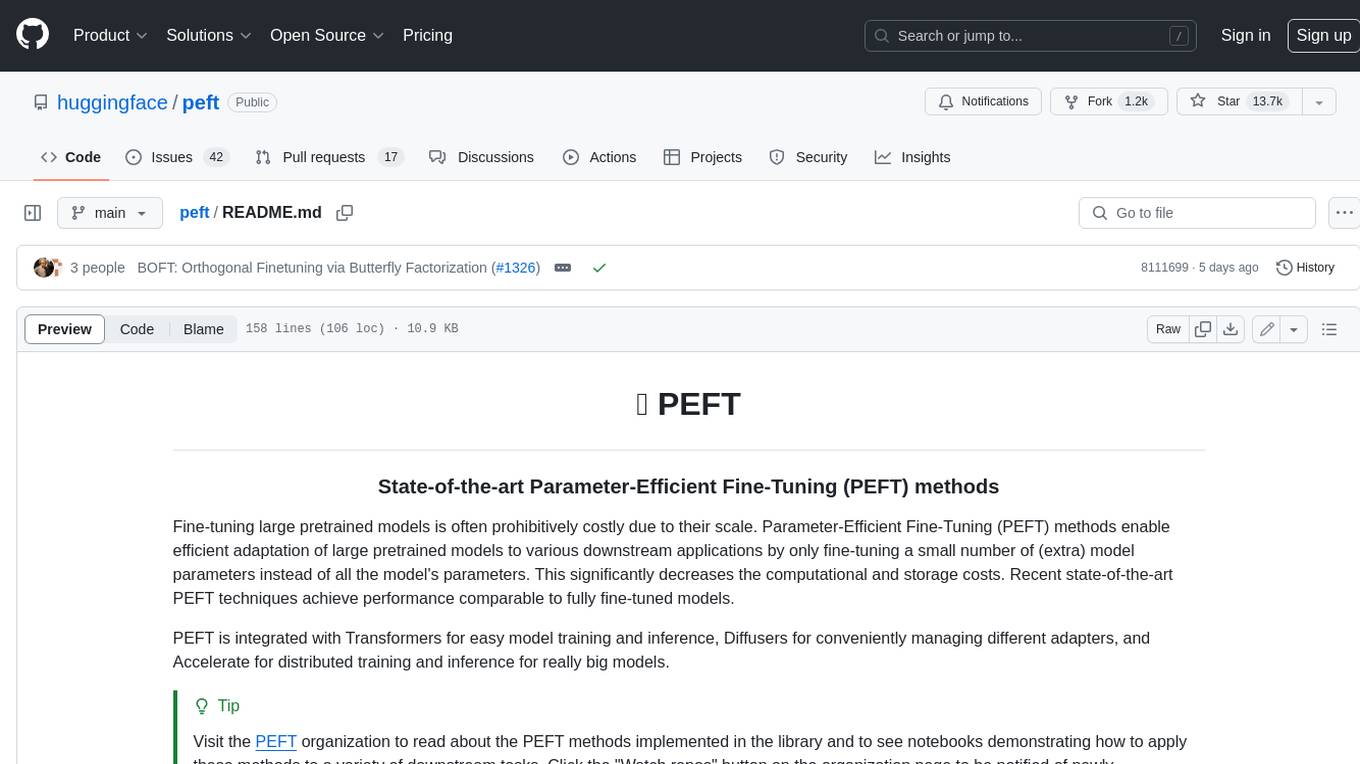
peft
PEFT (Parameter-Efficient Fine-Tuning) is a collection of state-of-the-art methods that enable efficient adaptation of large pretrained models to various downstream applications. By only fine-tuning a small number of extra model parameters instead of all the model's parameters, PEFT significantly decreases the computational and storage costs while achieving performance comparable to fully fine-tuned models.