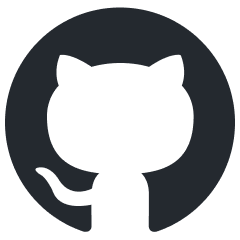
RAGEN
RAGEN leverages reinforcement learning to train LLM reasoning agents in interactive, stochastic environments.
Stars: 1307
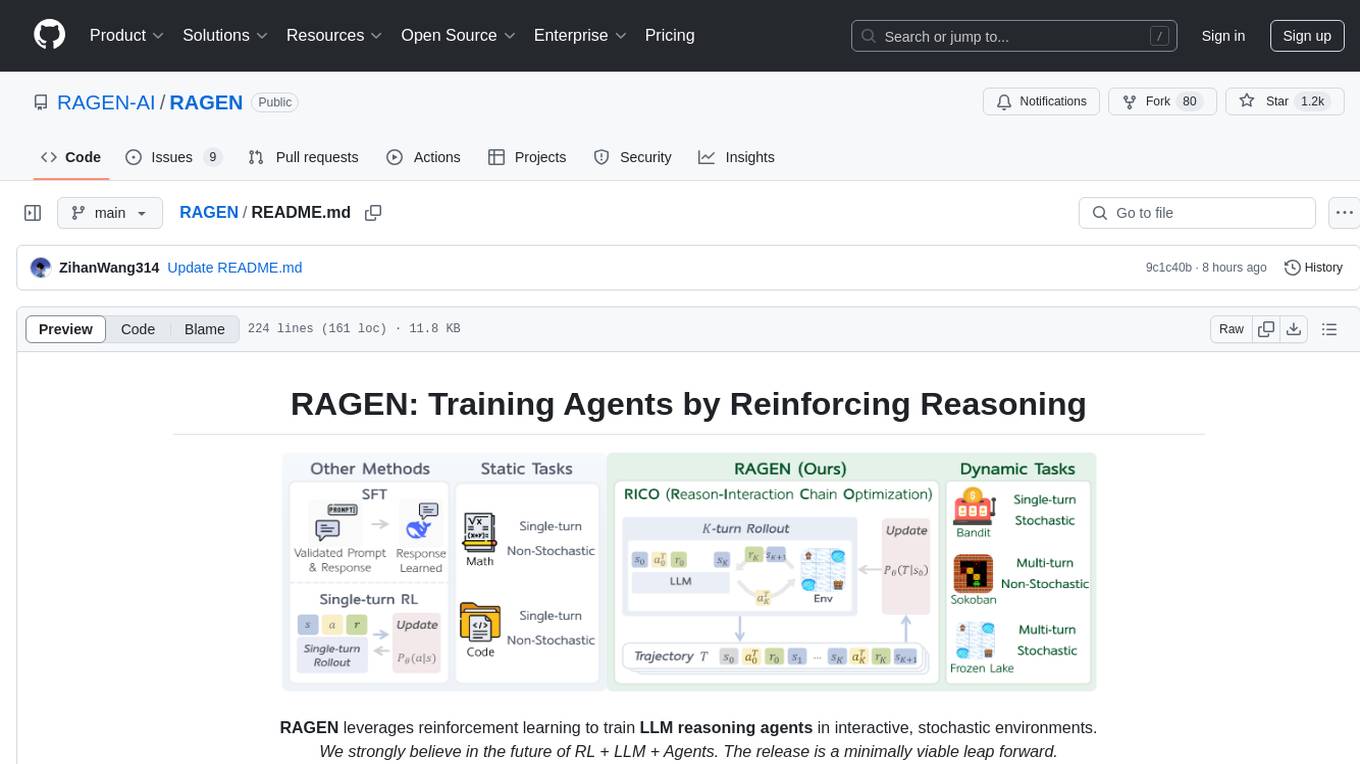
RAGEN is a reinforcement learning framework designed to train reasoning-capable large language model (LLM) agents in interactive, stochastic environments. It addresses challenges such as multi-turn interactions and stochastic environments through a Markov Decision Process (MDP) formulation, Reason-Interaction Chain Optimization (RICO) algorithm, and progressive reward normalization strategies. The framework consists of MDP formulation, RICO algorithm with rollout and update stages, and reward normalization strategies to stabilize training. RAGEN aims to optimize reasoning and action strategies for LLM agents operating in complex environments.
README:
RAGEN leverages reinforcement learning to train LLM reasoning agents in interactive, stochastic environments.
We strongly believe in the future of RL + LLM + Agents. The release is a minimally viable leap forward.
2025.3.13 Update:
We are recently refactoring RAGEN code to help you better develop your own idea on the codebase. Please checkout our developing branch. The first version decomposes RAGEN and veRL for better co-development, taking the latter as a submodule rather than a static directory.
2025.3.8 Update:
- In previous veRL implementation, there is a KL term issue, which has been fixed in recent versions.
- We find evidence from multiple sources that PPO could be more stable than GRPO training in Open-Reasoner-Zero, TinyZero, and Zhihu. We have changed the default advantage estimator to GAE (using PPO) and aim to find more stable while efficient RL optimization methods in later versions.
Reinforcement Learning (RL) with rule-based rewards has shown promise in enhancing reasoning capabilities of large language models (LLMs). However, existing approaches have primarily focused on static, single-turn tasks like math reasoning and coding. Extending these methods to agent scenarios introduces two fundamental challenges:
- Multi-turn Interactions: Agents must perform sequential decision-making and react to environment feedback
- Stochastic Environments: Uncertainty where identical actions can lead to different outcomes
RAGEN addresses these challenges through:
- A Markov Decision Process (MDP) formulation for agent tasks
- Reason-Interaction Chain Optimization (RICO) algorithm that optimizes entire trajectory distributions
- Progressive reward normalization strategies to handle diverse, complex environments
RAGEN introduces a reinforcement learning framework to train reasoning-capable LLM agents that can operate in interactive, stochastic environments.
The Reasoning-Interaction Chain Optimization (RICO) framework with two interleaved stages: rollout stage and update stage. LLM iteratively generates reasoning-guided actions to interact with the environment to obtain trajectory-level rewards, normalized for LLM update to jointly optimize reasoning and action strategies.
RAGEN introduces a reinforcement learning framework to train reasoning-capable LLM agents that can operate in interactive, stochastic environments. The framework consists of two key components:
We formulate agent-environment interactions as Markov Decision Processes (MDPs) where states and actions are token sequences, allowing LLMs to reason over environment dynamics. At time t, state $s_t$ transitions to the next state through action $a_t$ following a transition function. The policy generates actions given the trajectory history. The objective is to maximize expected cumulative rewards across multiple interaction turns.
RICO enables LLMs to jointly optimize reasoning and action strategies over entire trajectories. The algorithm alternates between two phases:
Given an initial state, the LLM generates multiple trajectories. At each step, the model receives the trajectory history and generates a reasoning-guided action: <think>...</think><ans> action </ans>
. The environment receives the action and returns feedback (reward and next state).
After generating trajectories, we train LLMs to optimize expected rewards. Instead of step-by-step optimization, RICO optimizes entire trajectories based on normalized trajectory-level rewards. This approach enables long-horizon reasoning while maintaining computational efficiency.
We implement three progressive normalization strategies to stabilize training:
- ARPO: Preserves raw rewards directly
- BRPO: Normalizes rewards across each training batch using batch statistics
- GRPO: Normalizes within prompt groups to balance learning across varying task difficulties
For detailed setup instructions, please check our documentation. Here's a quick start guide:
# Setup environment and download data (7MB)
bash scripts/setup_ragen.sh
python scripts/download_data.py
If this fails, you can follow the manual setup instructions in scripts/setup_ragen.md
.
Here's how to train models with RAGEN:
We provide 10k first-round-observation data for both Sokoban and FrozenLake tasks.
# Basic data creation
bash scripts/create_data.sh
# Or for research purposes, create more comprehensive data
bash scripts/create_data_full.sh
We provide default configuration in verl/trainer/config/ppo_trainer.yaml
. To train:
bash train.sh sokoban \
model.experiment_name=new_test
# Override config parameters as needed
bash train.sh sokoban \
model.experiment_name=new_test_debug \
training.train_batch_size=128 \
training.ppo_batch_size=64
For supervised finetuning with LoRA:
- Create supervised finetuning data:
bash sft/generate_data.sh <env_type>
- Finetune the model:
bash sft/finetune_lora.sh <env_type> <num_gpus> <save_path>
- Merge LoRA weights with the base model:
python sft/utils/merge_lora.py \
--base_model_name <base_model_name> \
--lora_model_path <lora_model_path> \
--output_path <output_path>
To visualize agent trajectories:
- Set visualization parameters in
train.sh
:
logging.log_images=True
logging.log_image_dir=log/trajectory
logging.log_image_step_size=4
logging.log_n_image_per_batch=32
- View the visualizations:
cd log/trajectory
python -m http.server 8000
# Access at http://localhost:8000/[EXP_NAME]/step_[STEP_NUM]/trajectory_data_[ID].html
- For proper font rendering:
sudo apt-get install fonts-noto-cjk
- Download visualization data from wandb:
from ragen.utils.wandb import download_wandb
download_wandb("RUN_ID") # e.g., 9o465jqj
We evaluate RAGEN across multiple model sizes and configurations. Below are results from our Sokoban experiments using Qwen-2.5-{0.5B, 3B}-{Instruct, None} and DeepSeek-R1-Distill-Qwen-1.5B.
NOTE: The loss shows the reward curve, where the KL term is considered.
Key observations:
- Instruct-finetuned models show early advantages but the gap narrows as training progresses
- Larger models (3B) generally outperform smaller models (0.5B), though the advantage is not dramatic
- The R1-distilled 1.5B model initially underperforms compared to 0.5B models
- Training has not yet converged in these experiments
Our analysis reveals two key aspects of LLM agent training with RL:
- Prompt diversity: Balancing observation variety and effective response comparison
- Online rollout frequency: Mediating between training stability and data recency
Visualization of agent reasoning on the Sokoban task:
The visualizations show how the agent reasons through sequential steps to solve the puzzle.
We provide several case studies showing the model's behavior:
More case studies will be added to showcase both successful reasoning patterns and failure modes.
We welcome all forms of feedback! Please raise an issue for bugs, questions, or suggestions. This helps our team address common problems efficiently and builds a more productive community.
- VAGEN: Training Visual Agents with multi-turn reinforcement learning
- Search-R1: Train your LLMs to reason and call a search engine with reinforcement learning
- Agent-R1: Training Powerful LLM Agents with End-to-End Reinforcement Learning
- OpenManus-RL: A live stream development of RL tunning for LLM agents
- MetaSpatial: Reinforcing 3D Spatial Reasoning in VLMs for the Metaverse
Zihan Wang*, Kangrui Wang*, Qineng Wang*, Pingyue Zhang*, Linjie Li*, Zhengyuan Yang, Kefan Yu, Minh Nhat Nguyen, Monica Lam, Yiping Lu, Kyunghyun Cho, Jiajun Wu, Li Fei-Fei, Lijuan Wang, Yejin Choi, Manling Li
*:Equal Contribution.
We thank DeepSeek for providing the DeepSeek-R1 model and ideas. We thank the veRL team for their infrastructure. We thank the TinyZero team for their discoveries that inspired our early exploration. We thank Licheng Liu, Eli Gottlieb, Jiajun Liu for helping explore stable RL algorithms. We thank Han Liu, Xinyu Xing, Li Erran Li, Akari Asai, Eiso Kant, Lu Lu, Runxin Xu, Huajian Xin, Zijun Liu, Weiyi Liu, Weimin Wu, Yibo Wen, Jiarui Liu, Lorenzo Xiao, Ishan Mukherjee, Anabella Isaro, Haosen Sun, How-Yeh Wan, Lester Xue, Weiyi Liu for insightful discussions.
@misc{RAGEN,
author = {Zihan Wang* and Kangrui Wang* and Qineng Wang* and Pingyue Zhang* and Linjie Li* and Zhengyuan Yang and Kefan Yu and Minh Nhat Nguyen and Monica Lam and Yiping Lu and Kyunghyun Cho and Jiajun Wu and Li Fei-Fei and Lijuan Wang and Yejin Choi and Manling Li},
title = {Training Agents by Reinforcing Reasoning},
year = {2025},
organization = {GitHub},
url = {https://github.com/ZihanWang314/ragen},
}
For Tasks:
Click tags to check more tools for each tasksFor Jobs:
Alternative AI tools for RAGEN
Similar Open Source Tools
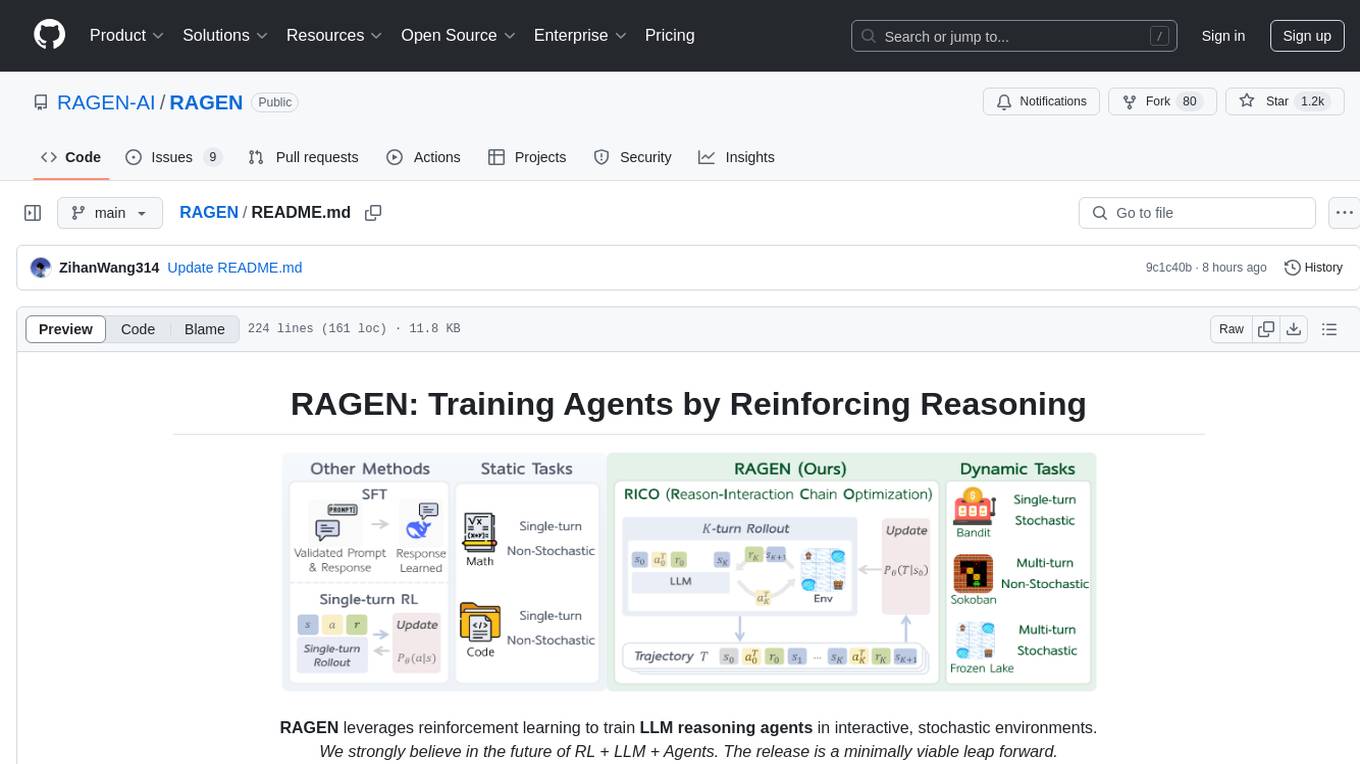
RAGEN
RAGEN is a reinforcement learning framework designed to train reasoning-capable large language model (LLM) agents in interactive, stochastic environments. It addresses challenges such as multi-turn interactions and stochastic environments through a Markov Decision Process (MDP) formulation, Reason-Interaction Chain Optimization (RICO) algorithm, and progressive reward normalization strategies. The framework consists of MDP formulation, RICO algorithm with rollout and update stages, and reward normalization strategies to stabilize training. RAGEN aims to optimize reasoning and action strategies for LLM agents operating in complex environments.
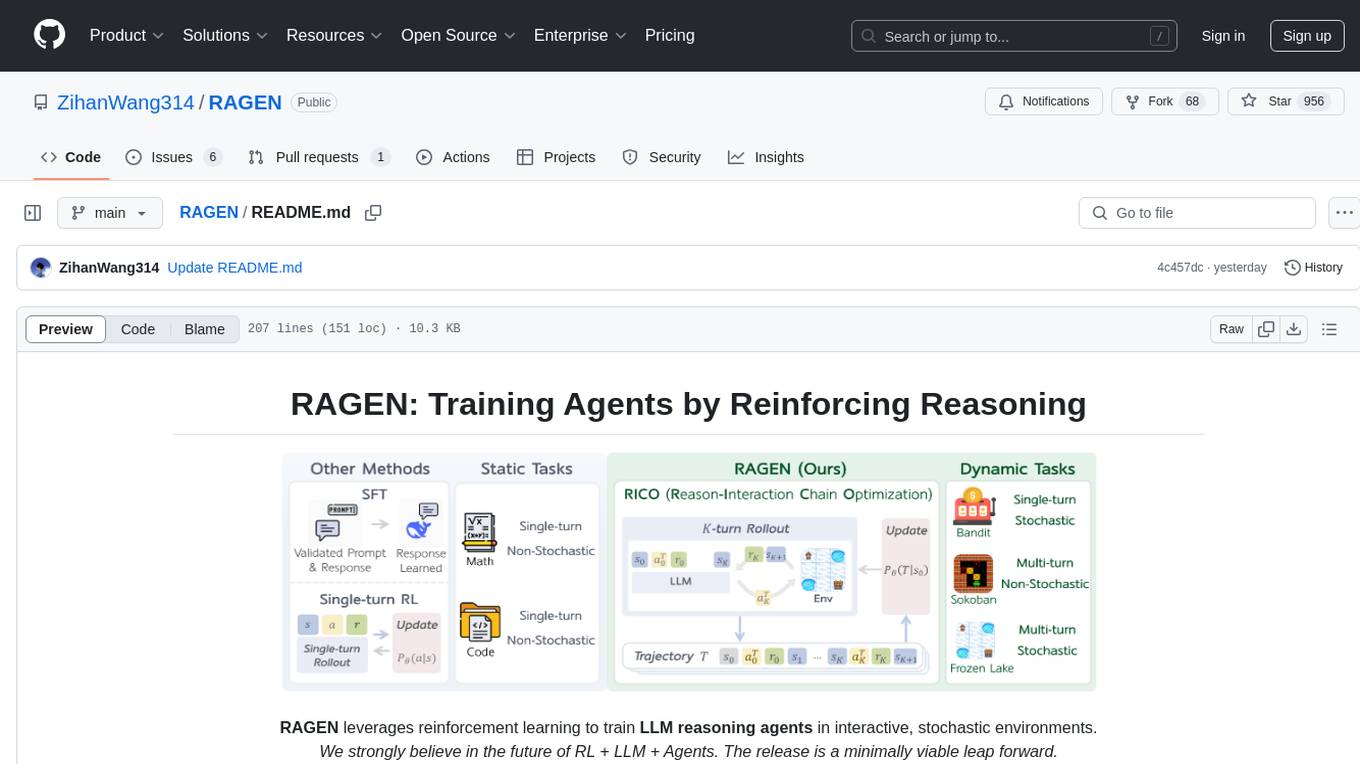
RAGEN
RAGEN is a reinforcement learning framework designed to train reasoning-capable large language model (LLM) agents in interactive, stochastic environments. It addresses challenges such as multi-turn interactions and stochastic environments through a Markov Decision Process (MDP) formulation, Reason-Interaction Chain Optimization (RICO) algorithm, and progressive reward normalization strategies. The framework enables LLMs to reason and interact with the environment, optimizing entire trajectories for long-horizon reasoning while maintaining computational efficiency.
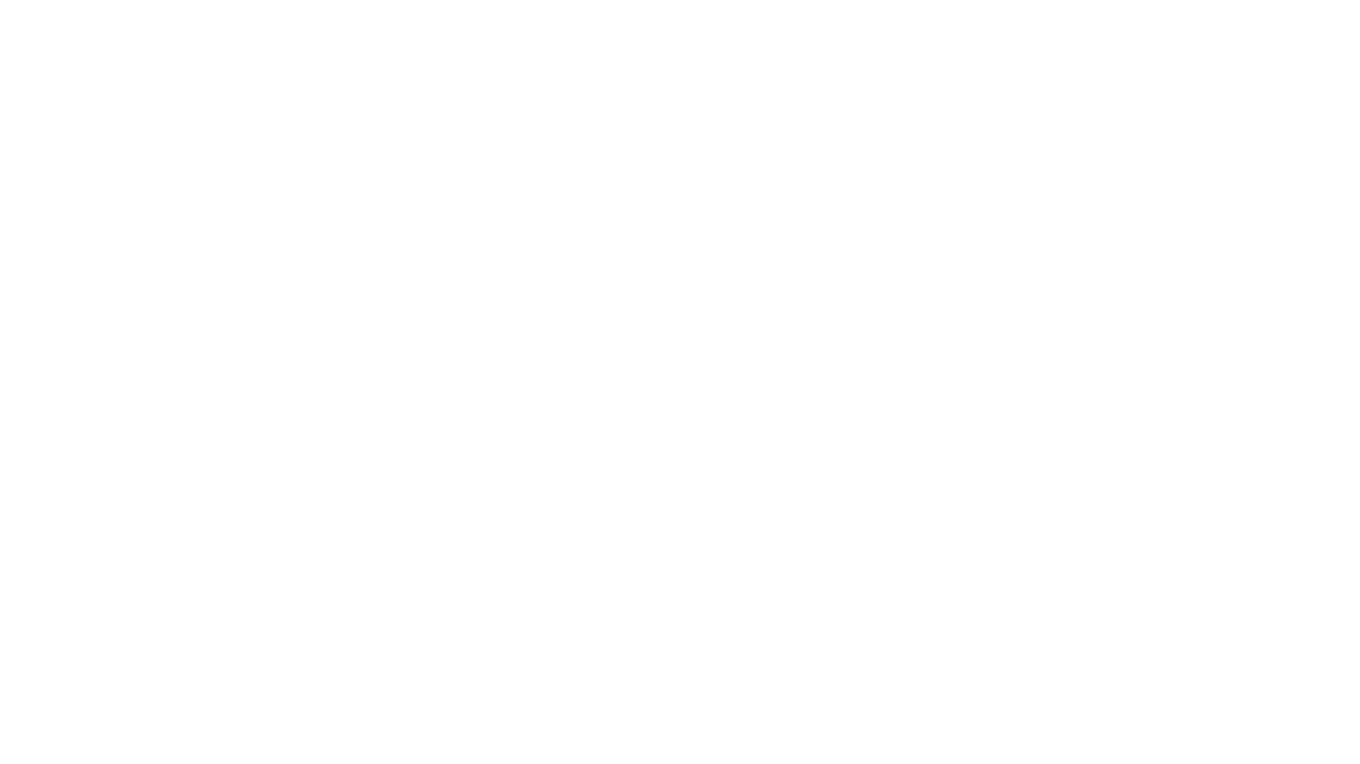
motia
Motia is an AI agent framework designed for software engineers to create, test, and deploy production-ready AI agents quickly. It provides a code-first approach, allowing developers to write agent logic in familiar languages and visualize execution in real-time. With Motia, developers can focus on business logic rather than infrastructure, offering zero infrastructure headaches, multi-language support, composable steps, built-in observability, instant APIs, and full control over AI logic. Ideal for building sophisticated agents and intelligent automations, Motia's event-driven architecture and modular steps enable the creation of GenAI-powered workflows, decision-making systems, and data processing pipelines.
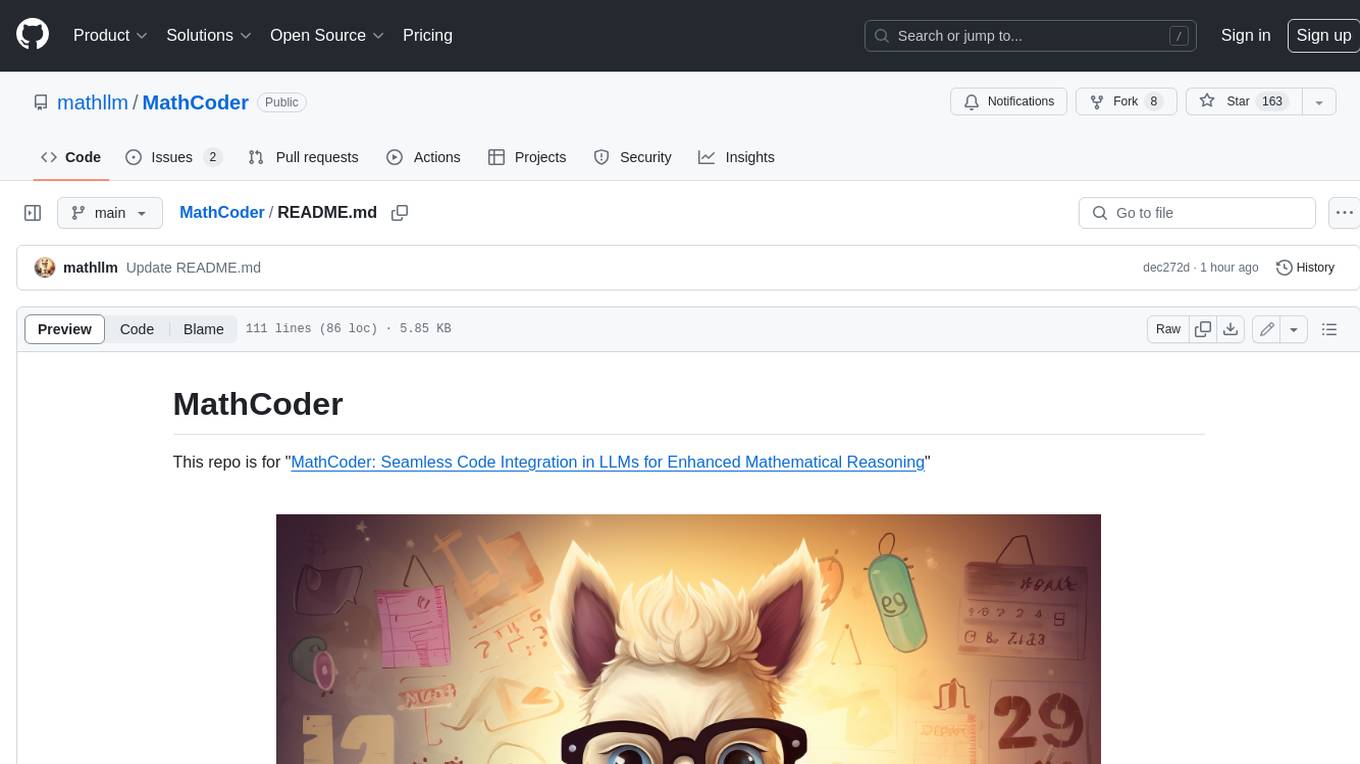
MathCoder
MathCoder is a repository focused on enhancing mathematical reasoning by fine-tuning open-source language models to use code for modeling and deriving math equations. It introduces MathCodeInstruct dataset with solutions interleaving natural language, code, and execution results. The repository provides MathCoder models capable of generating code-based solutions for challenging math problems, achieving state-of-the-art scores on MATH and GSM8K datasets. It offers tools for model deployment, inference, and evaluation, along with a citation for referencing the work.
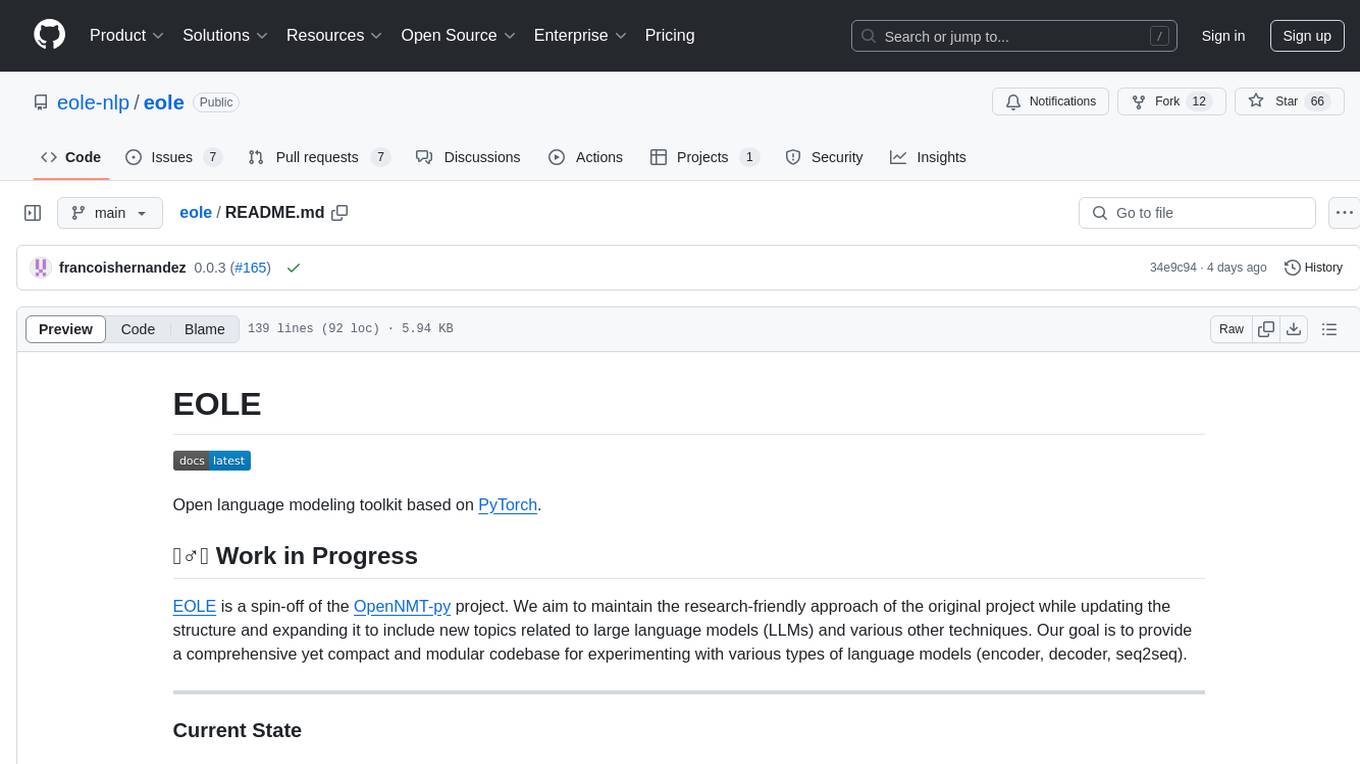
eole
EOLE is an open language modeling toolkit based on PyTorch. It aims to provide a research-friendly approach with a comprehensive yet compact and modular codebase for experimenting with various types of language models. The toolkit includes features such as versatile training and inference, dynamic data transforms, comprehensive large language model support, advanced quantization, efficient finetuning, flexible inference, and tensor parallelism. EOLE is a work in progress with ongoing enhancements in configuration management, command line entry points, reproducible recipes, core API simplification, and plans for further simplification, refactoring, inference server development, additional recipes, documentation enhancement, test coverage improvement, logging enhancements, and broader model support.
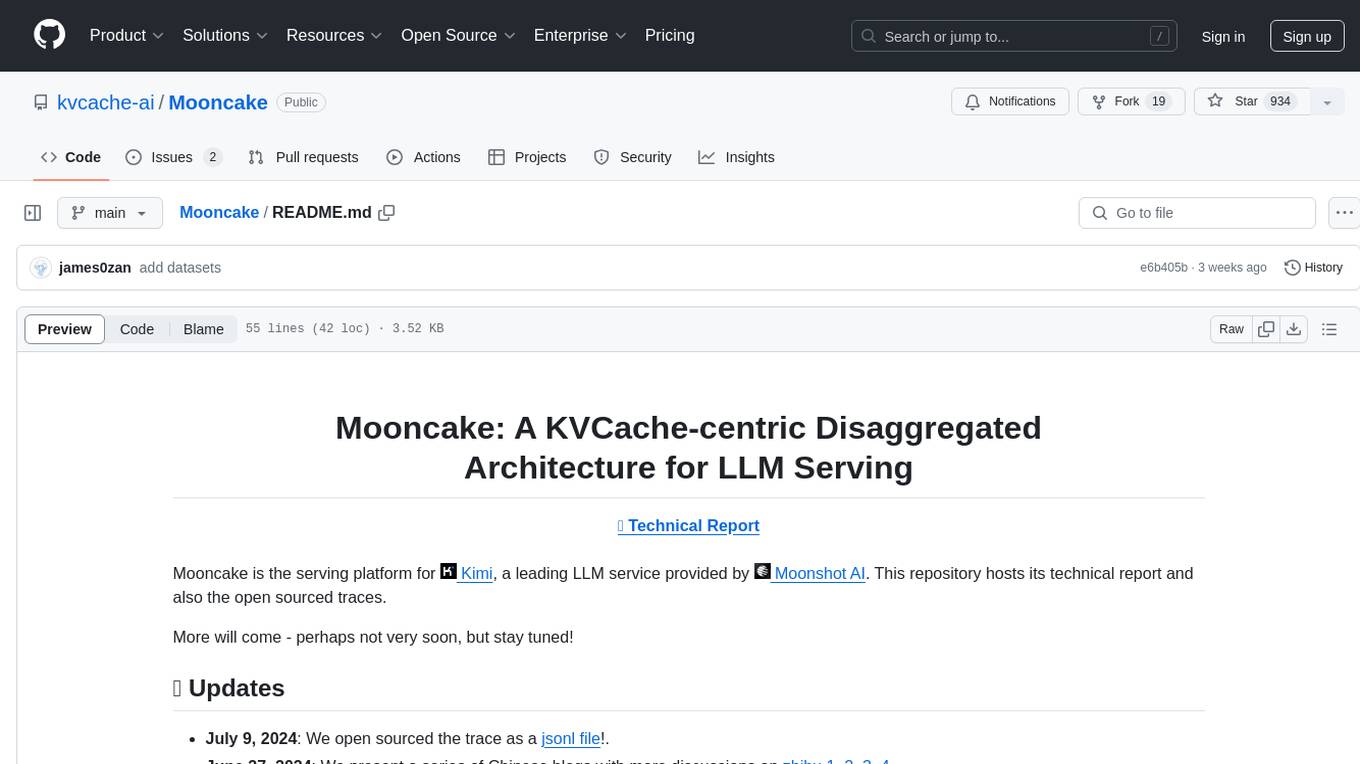
Mooncake
Mooncake is a serving platform for Kimi, a leading LLM service provided by Moonshot AI. It features a KVCache-centric disaggregated architecture that separates prefill and decoding clusters, leveraging underutilized CPU, DRAM, and SSD resources of the GPU cluster. Mooncake's scheduler balances throughput and latency-related SLOs, with a prediction-based early rejection policy for highly overloaded scenarios. It excels in long-context scenarios, achieving up to a 525% increase in throughput while handling 75% more requests under real workloads.
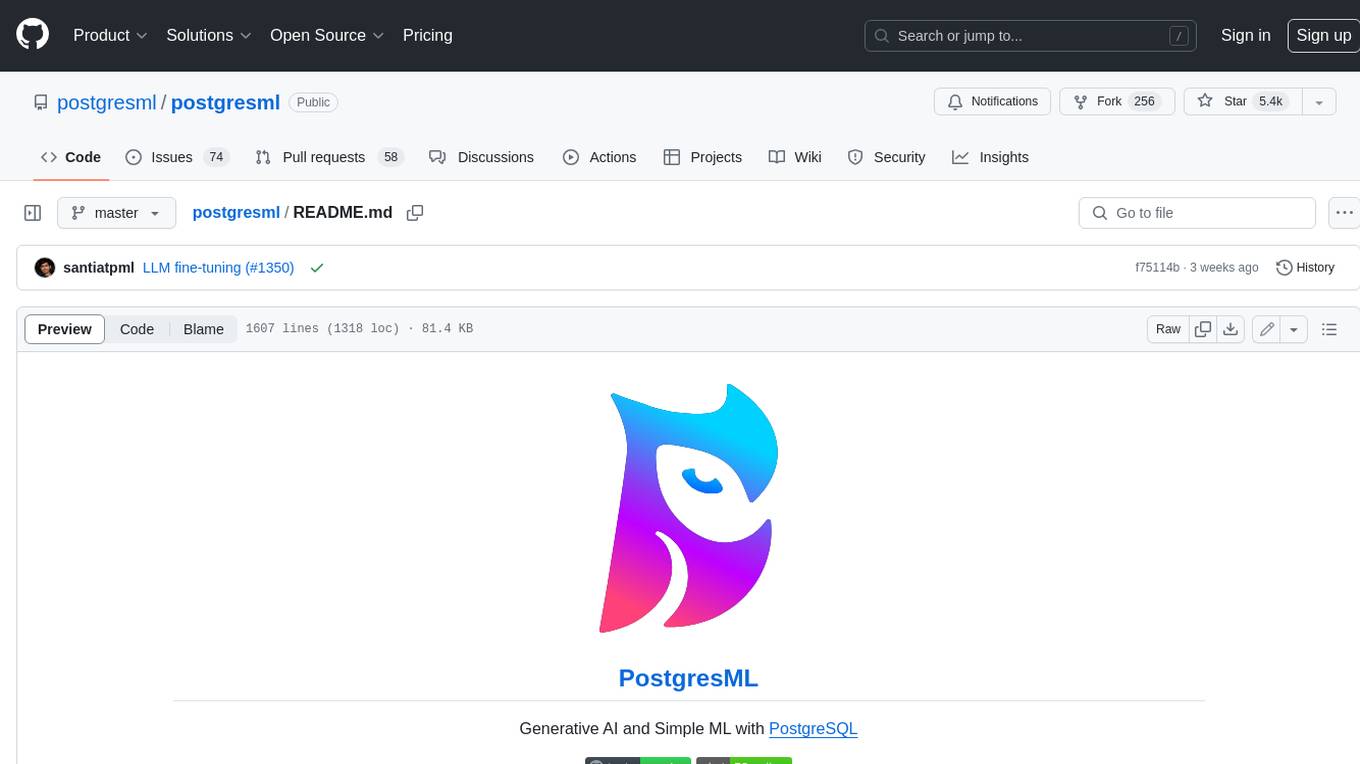
postgresml
PostgresML is a powerful Postgres extension that seamlessly combines data storage and machine learning inference within your database. It enables running machine learning and AI operations directly within PostgreSQL, leveraging GPU acceleration for faster computations, integrating state-of-the-art large language models, providing built-in functions for text processing, enabling efficient similarity search, offering diverse ML algorithms, ensuring high performance, scalability, and security, supporting a wide range of NLP tasks, and seamlessly integrating with existing PostgreSQL tools and client libraries.
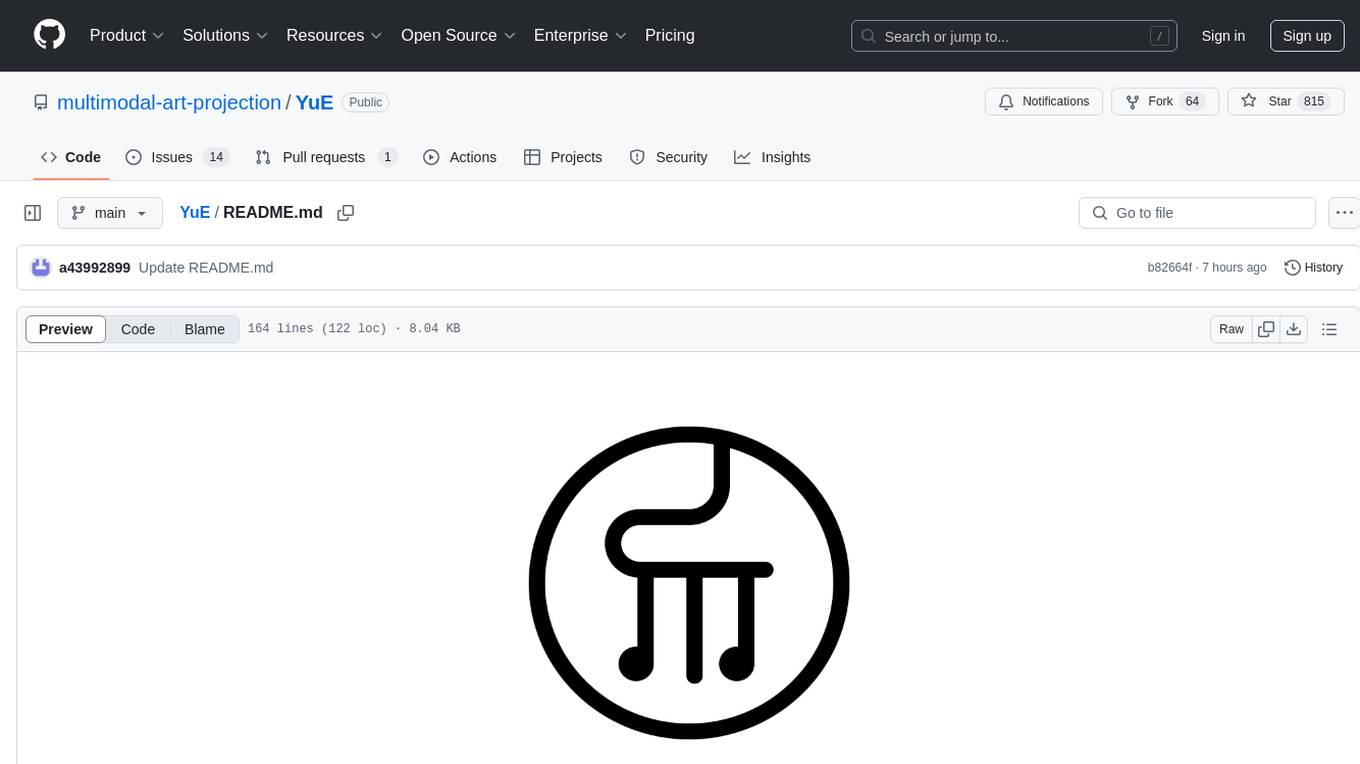
YuE
YuE (乐) is an open-source foundation model designed for music generation, specifically transforming lyrics into full songs. It can generate complete songs in various genres and vocal styles, ensuring a polished and cohesive result. The model requires significant GPU memory for generating long sequences and recommends specific configurations for optimal performance. Users can customize the number of sessions for memory usage. The tool provides a quickstart guide for generating music using Transformers and includes tips for execution time and tag selection. The project is licensed under Creative Commons Attribution Non Commercial 4.0.
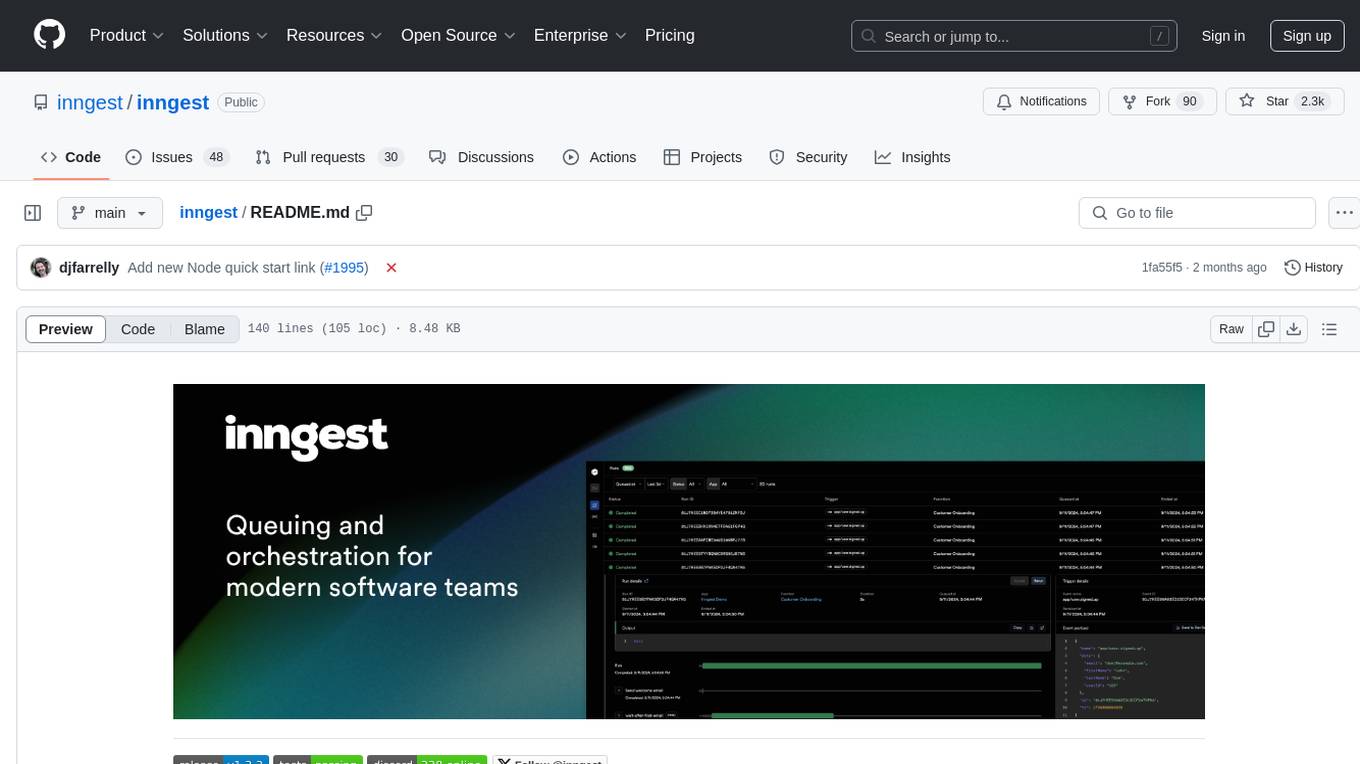
inngest
Inngest is a platform that offers durable functions to replace queues, state management, and scheduling for developers. It allows writing reliable step functions faster without dealing with infrastructure. Developers can create durable functions using various language SDKs, run a local development server, deploy functions to their infrastructure, sync functions with the Inngest Platform, and securely trigger functions via HTTPS. Inngest Functions support retrying, scheduling, and coordinating operations through triggers, flow control, and steps, enabling developers to build reliable workflows with robust support for various operations.
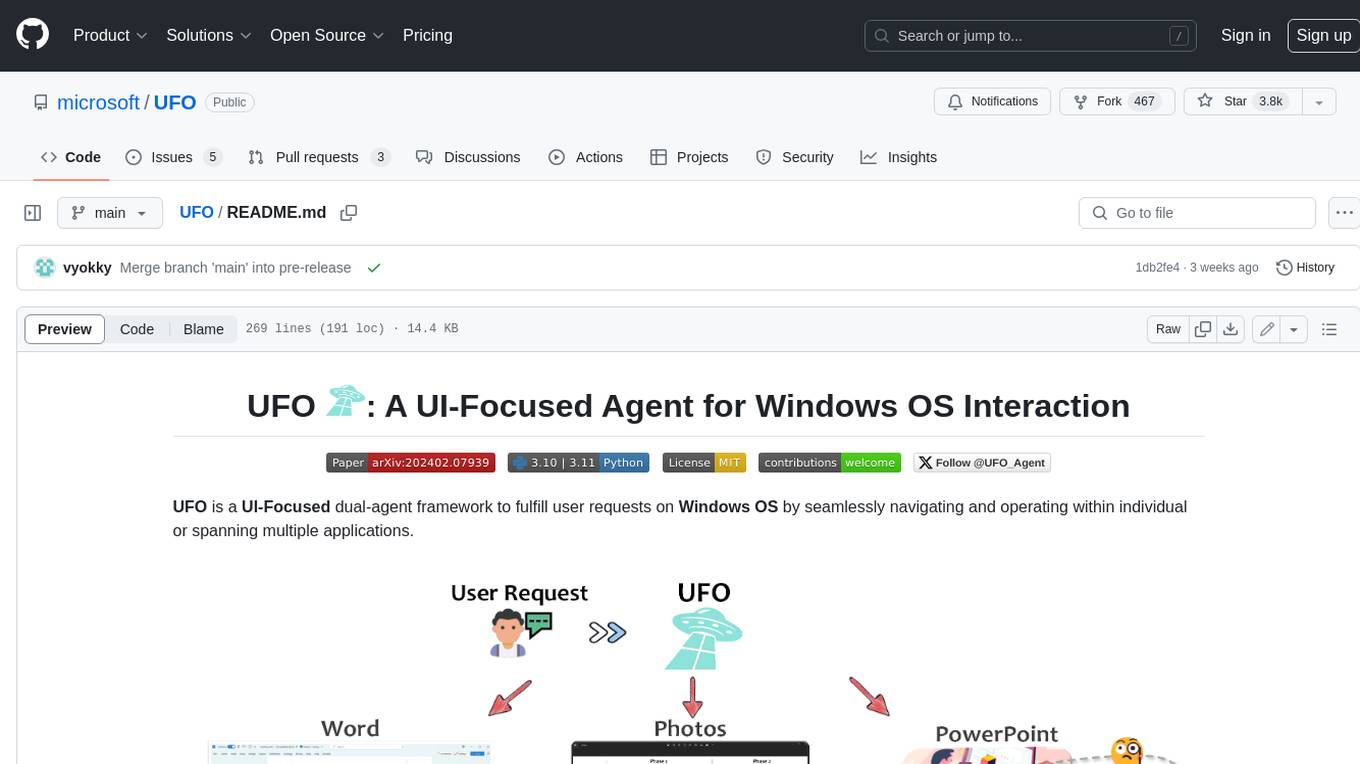
UFO
UFO is a UI-focused dual-agent framework to fulfill user requests on Windows OS by seamlessly navigating and operating within individual or spanning multiple applications.
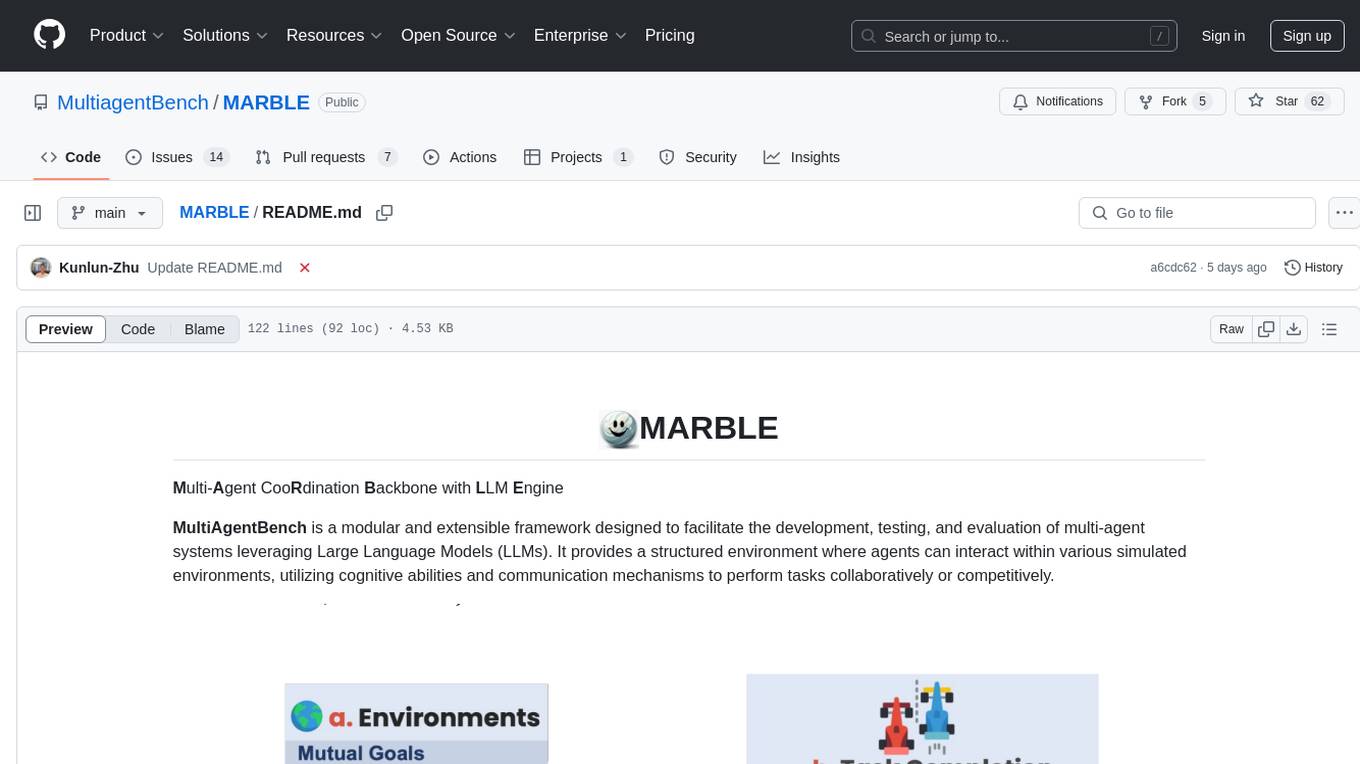
MARBLE
MARBLE (Multi-Agent Coordination Backbone with LLM Engine) is a modular framework for developing, testing, and evaluating multi-agent systems leveraging Large Language Models. It provides a structured environment for agents to interact in simulated environments, utilizing cognitive abilities and communication mechanisms for collaborative or competitive tasks. The framework features modular design, multi-agent support, LLM integration, shared memory, flexible environments, metrics and evaluation, industrial coding standards, and Docker support.
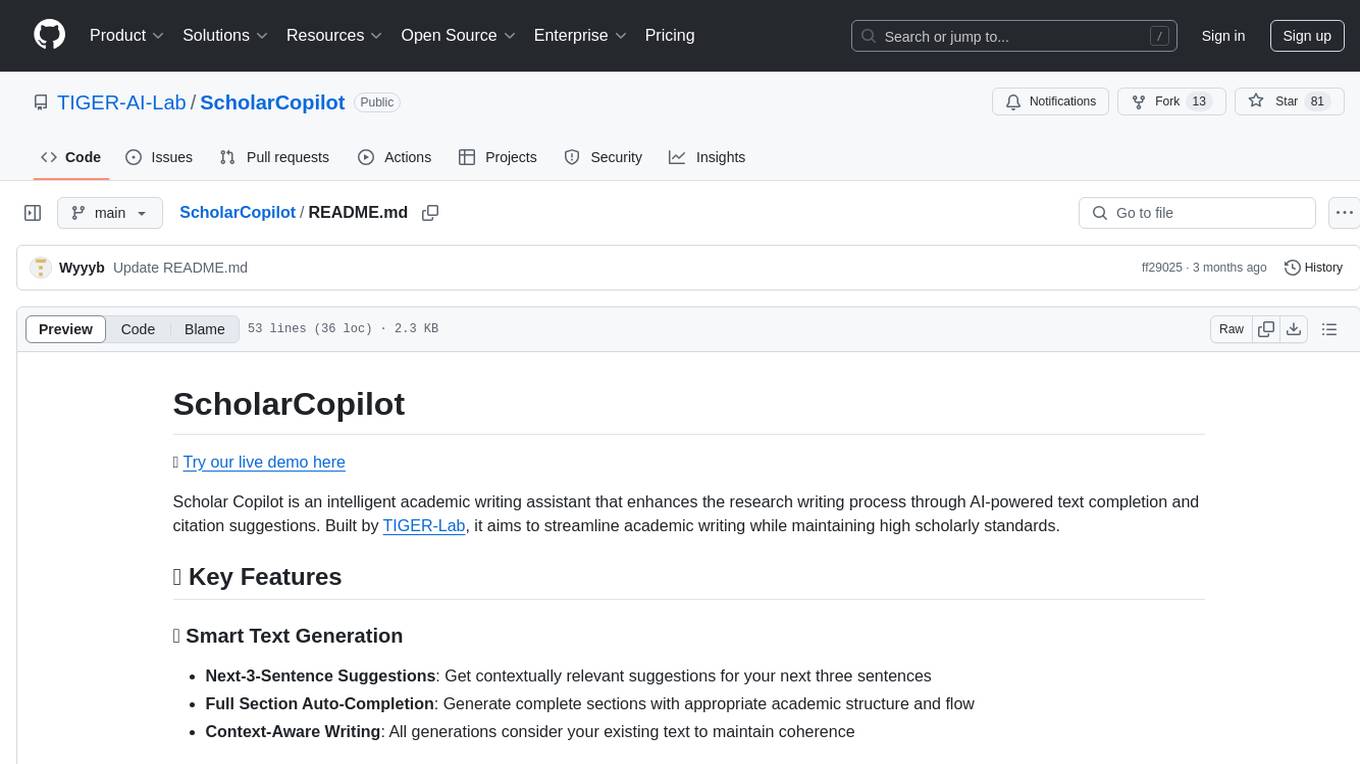
ScholarCopilot
Scholar Copilot is an intelligent academic writing assistant that enhances the research writing process through AI-powered text completion and citation suggestions. It aims to streamline academic writing while maintaining high scholarly standards. The tool provides features such as smart text generation with next-3-sentence suggestions, full section auto-completion, and context-aware writing. It also offers intelligent citation management with real-time citation suggestions, one-click citation insertion, and citation Bibtex generation. Scholar Copilot employs a unified model architecture that integrates retrieval and generation through a dynamic switching mechanism, ensuring coherent text generation with appropriate citation points.
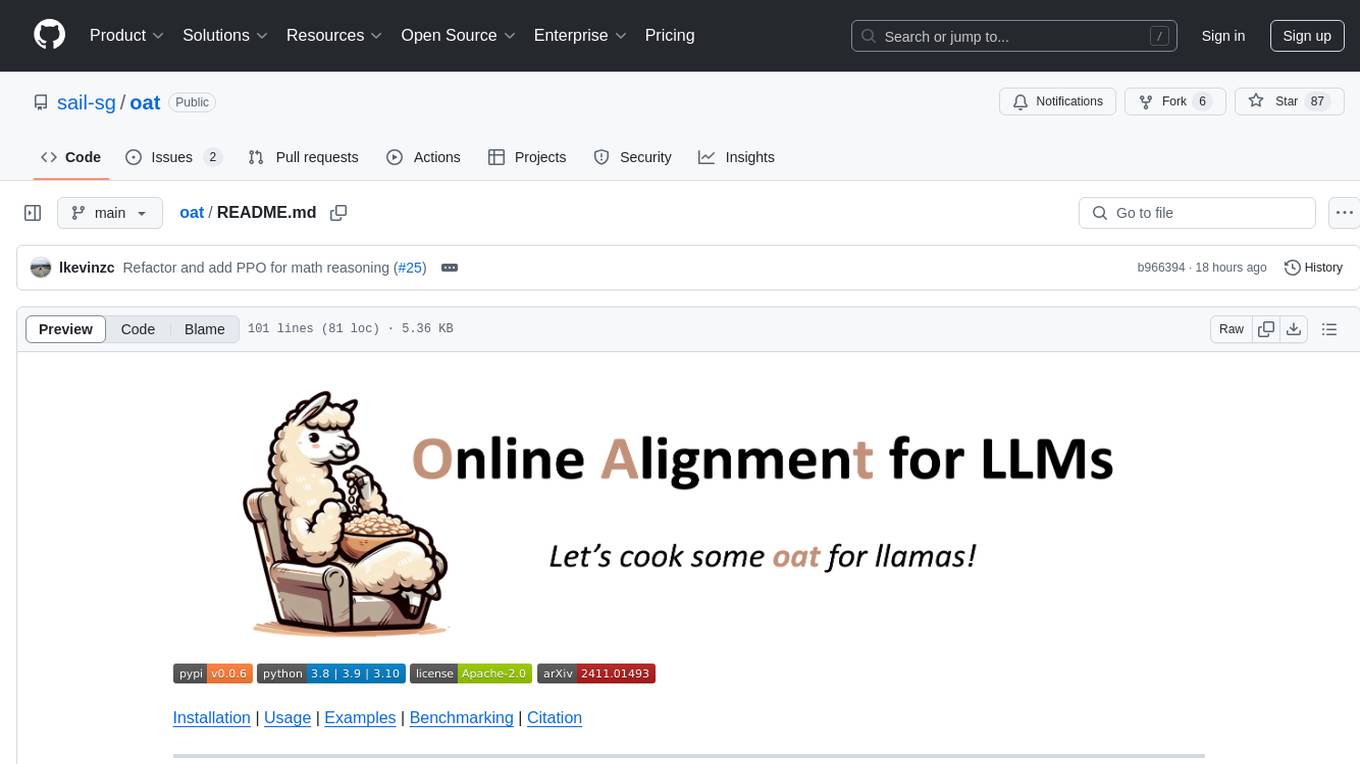
oat
Oat is a simple and efficient framework for running online LLM alignment algorithms. It implements a distributed Actor-Learner-Oracle architecture, with components optimized using state-of-the-art tools. Oat simplifies the experimental pipeline of LLM alignment by serving an Oracle online for preference data labeling and model evaluation. It provides a variety of oracles for simulating feedback and supports verifiable rewards. Oat's modular structure allows for easy inheritance and modification of classes, enabling rapid prototyping and experimentation with new algorithms. The framework implements cutting-edge online algorithms like PPO for math reasoning and various online exploration algorithms.
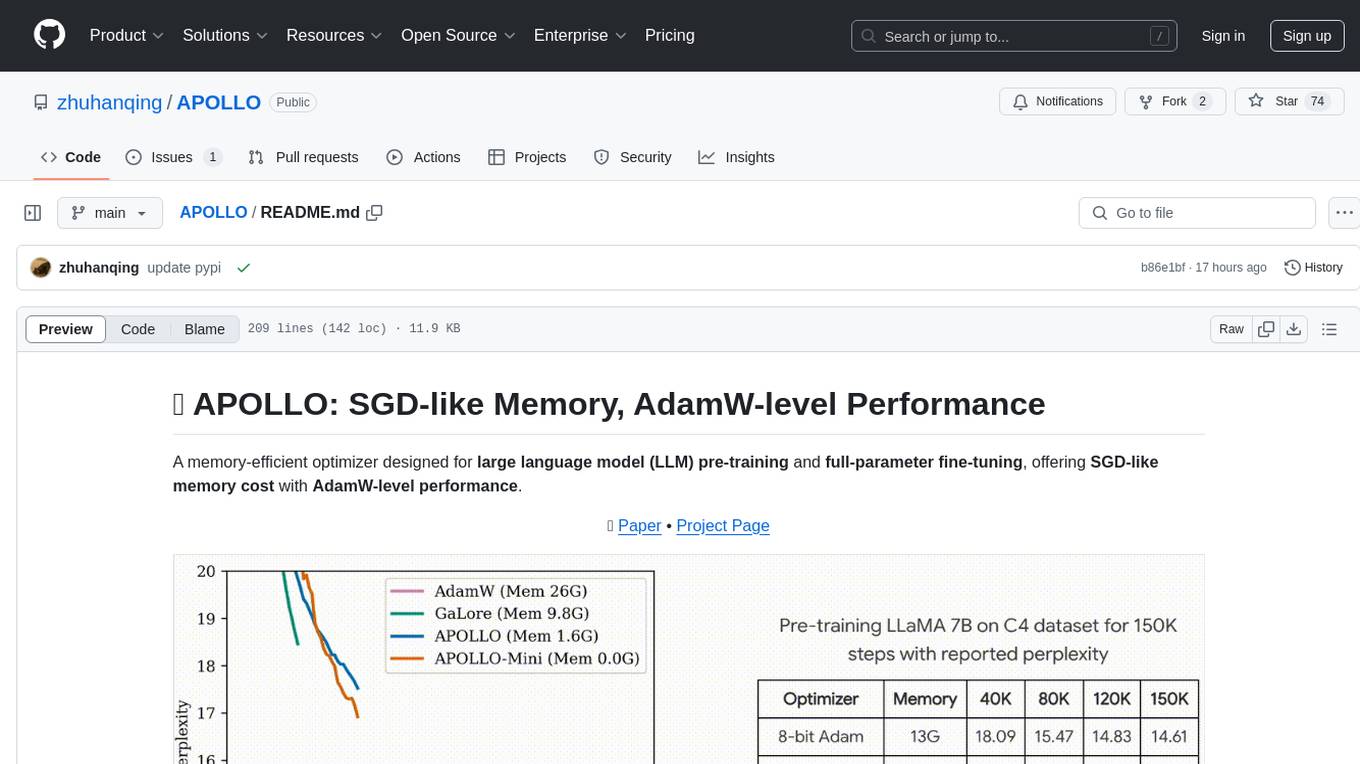
APOLLO
APOLLO is a memory-efficient optimizer designed for large language model (LLM) pre-training and full-parameter fine-tuning. It offers SGD-like memory cost with AdamW-level performance. The optimizer integrates low-rank approximation and optimizer state redundancy reduction to achieve significant memory savings while maintaining or surpassing the performance of Adam(W). Key contributions include structured learning rate updates for LLM training, approximated channel-wise gradient scaling in a low-rank auxiliary space, and minimal-rank tensor-wise gradient scaling. APOLLO aims to optimize memory efficiency during training large language models.
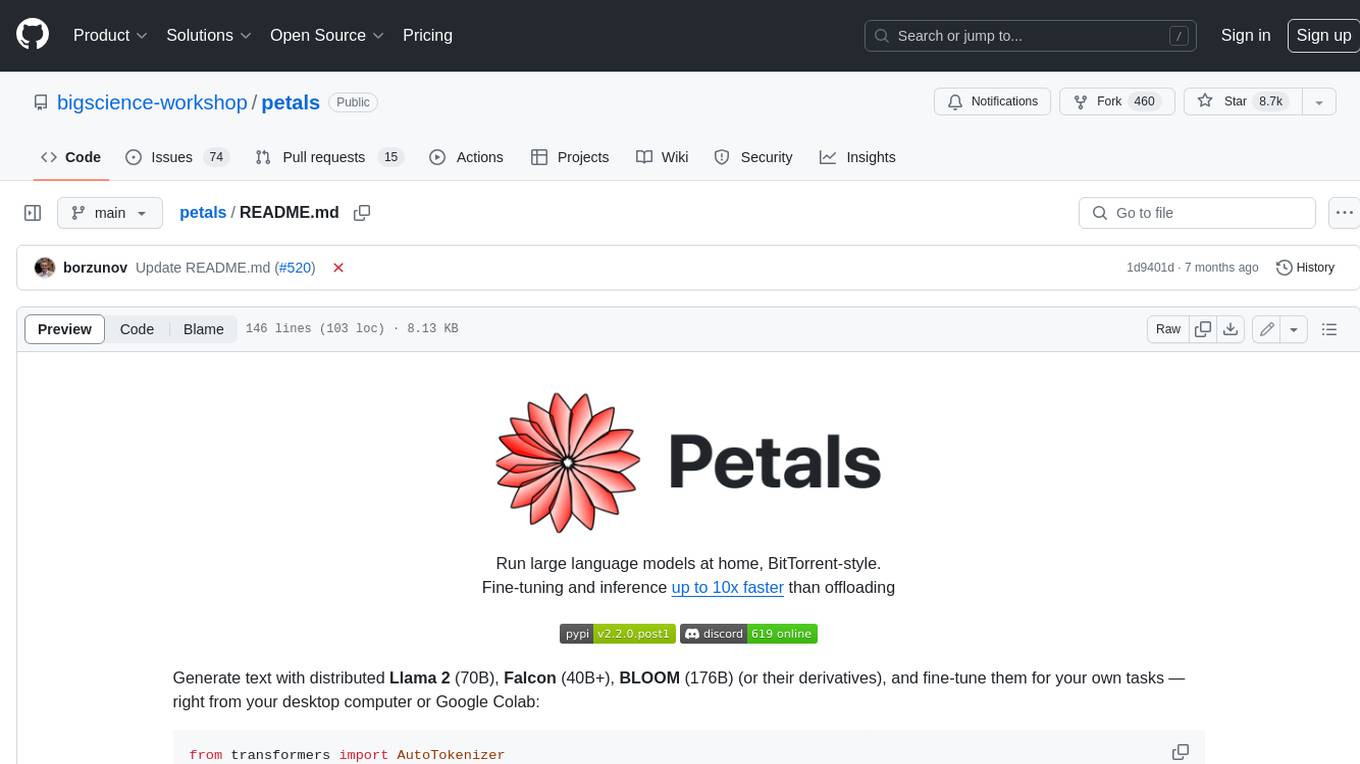
petals
Petals is a tool that allows users to run large language models at home in a BitTorrent-style manner. It enables fine-tuning and inference up to 10x faster than offloading. Users can generate text with distributed models like Llama 2, Falcon, and BLOOM, and fine-tune them for specific tasks directly from their desktop computer or Google Colab. Petals is a community-run system that relies on people sharing their GPUs to increase its capacity and offer a distributed network for hosting model layers.
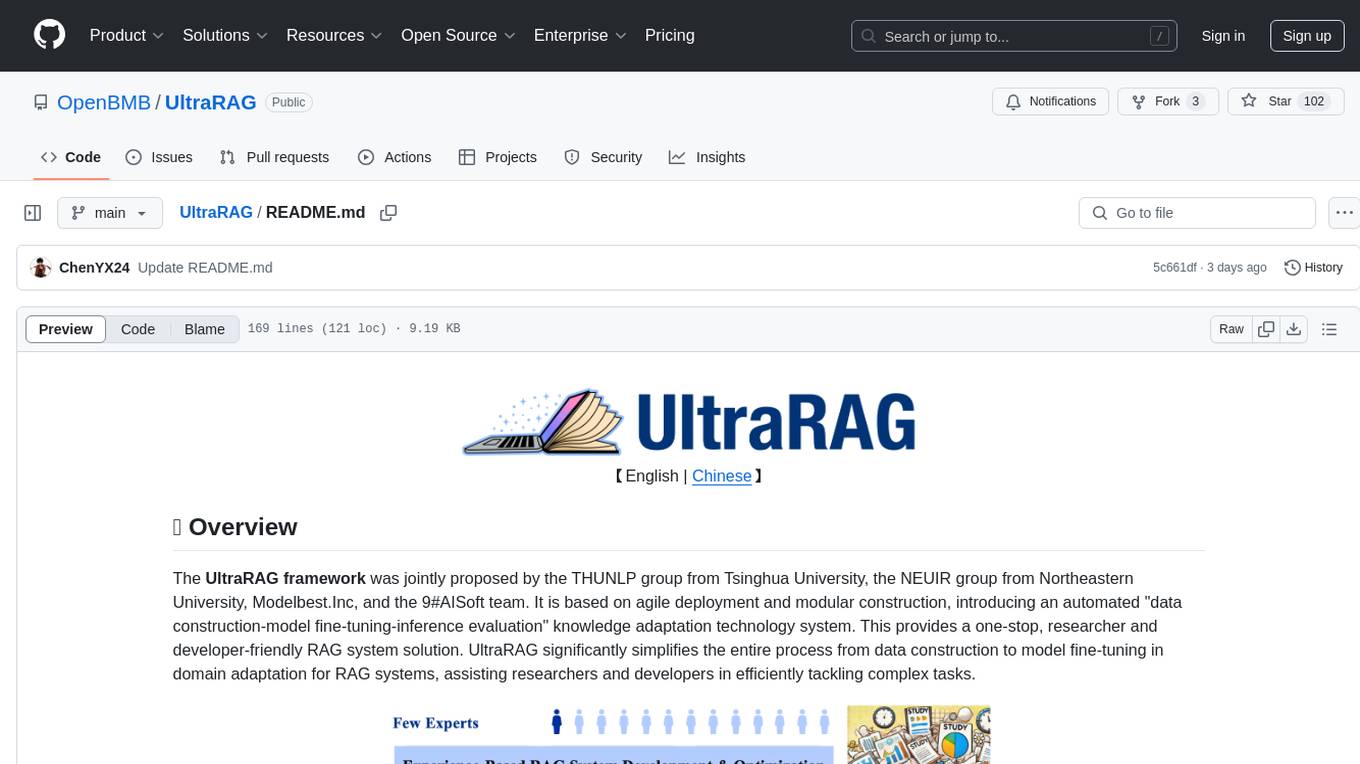
UltraRAG
The UltraRAG framework is a researcher and developer-friendly RAG system solution that simplifies the process from data construction to model fine-tuning in domain adaptation. It introduces an automated knowledge adaptation technology system, supporting no-code programming, one-click synthesis and fine-tuning, multidimensional evaluation, and research-friendly exploration work integration. The architecture consists of Frontend, Service, and Backend components, offering flexibility in customization and optimization. Performance evaluation in the legal field shows improved results compared to VanillaRAG, with specific metrics provided. The repository is licensed under Apache-2.0 and encourages citation for support.
For similar tasks
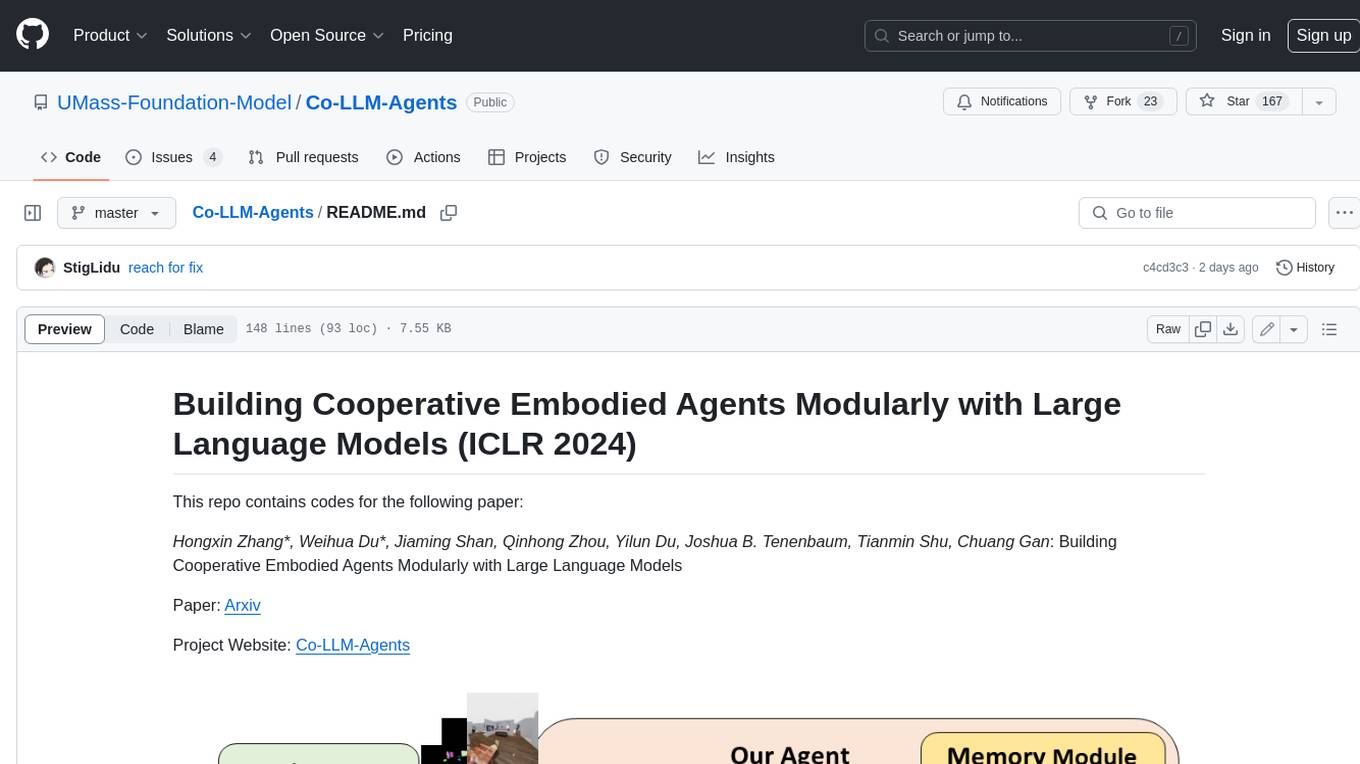
Co-LLM-Agents
This repository contains code for building cooperative embodied agents modularly with large language models. The agents are trained to perform tasks in two different environments: ThreeDWorld Multi-Agent Transport (TDW-MAT) and Communicative Watch-And-Help (C-WAH). TDW-MAT is a multi-agent environment where agents must transport objects to a goal position using containers. C-WAH is an extension of the Watch-And-Help challenge, which enables agents to send messages to each other. The code in this repository can be used to train agents to perform tasks in both of these environments.
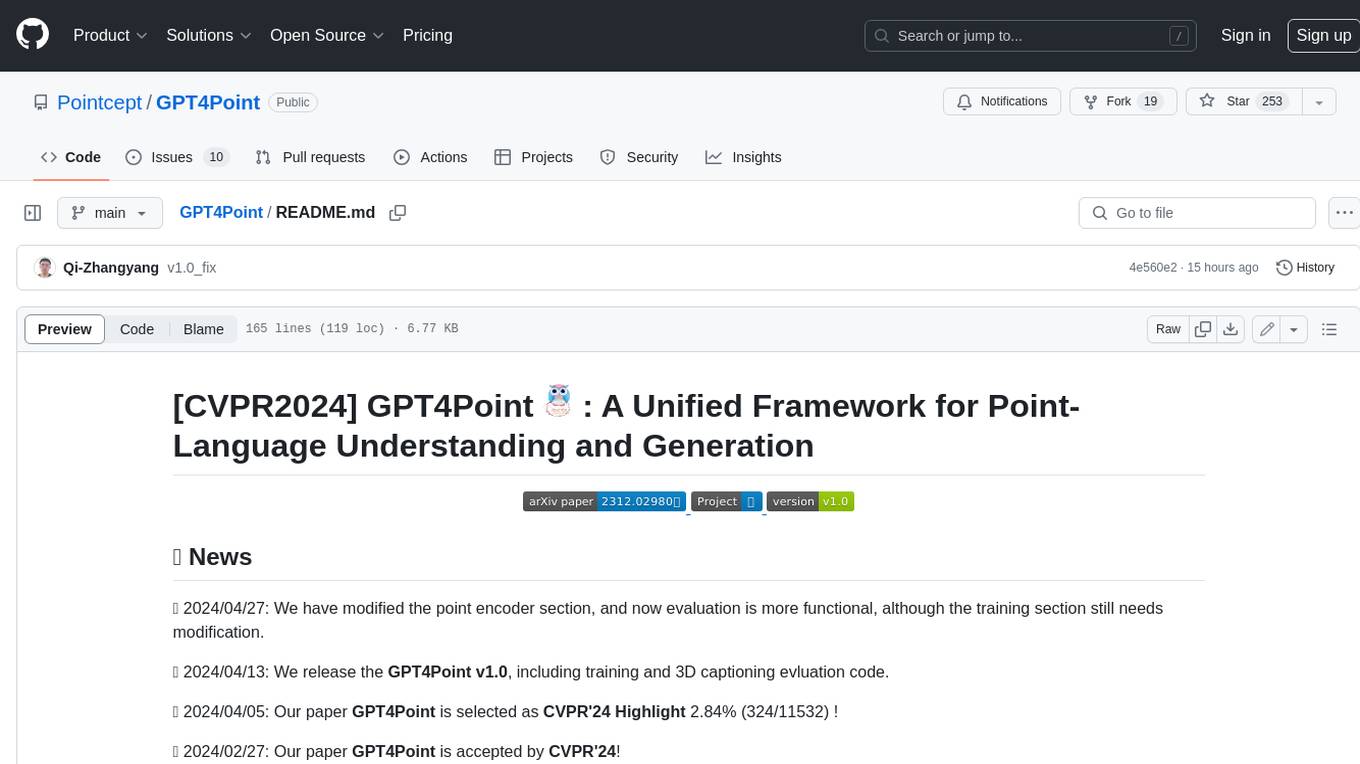
GPT4Point
GPT4Point is a unified framework for point-language understanding and generation. It aligns 3D point clouds with language, providing a comprehensive solution for tasks such as 3D captioning and controlled 3D generation. The project includes an automated point-language dataset annotation engine, a novel object-level point cloud benchmark, and a 3D multi-modality model. Users can train and evaluate models using the provided code and datasets, with a focus on improving models' understanding capabilities and facilitating the generation of 3D objects.
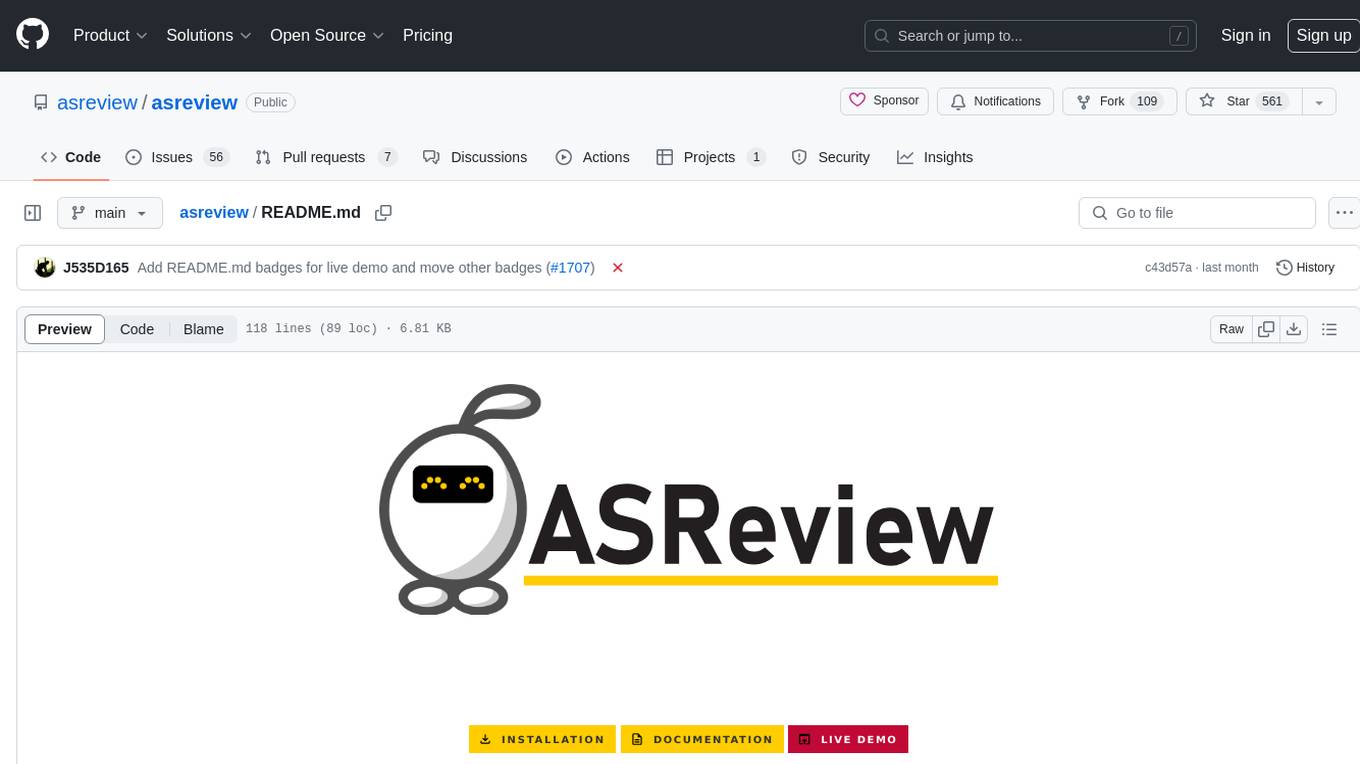
asreview
The ASReview project implements active learning for systematic reviews, utilizing AI-aided pipelines to assist in finding relevant texts for search tasks. It accelerates the screening of textual data with minimal human input, saving time and increasing output quality. The software offers three modes: Oracle for interactive screening, Exploration for teaching purposes, and Simulation for evaluating active learning models. ASReview LAB is designed to support decision-making in any discipline or industry by improving efficiency and transparency in screening large amounts of textual data.
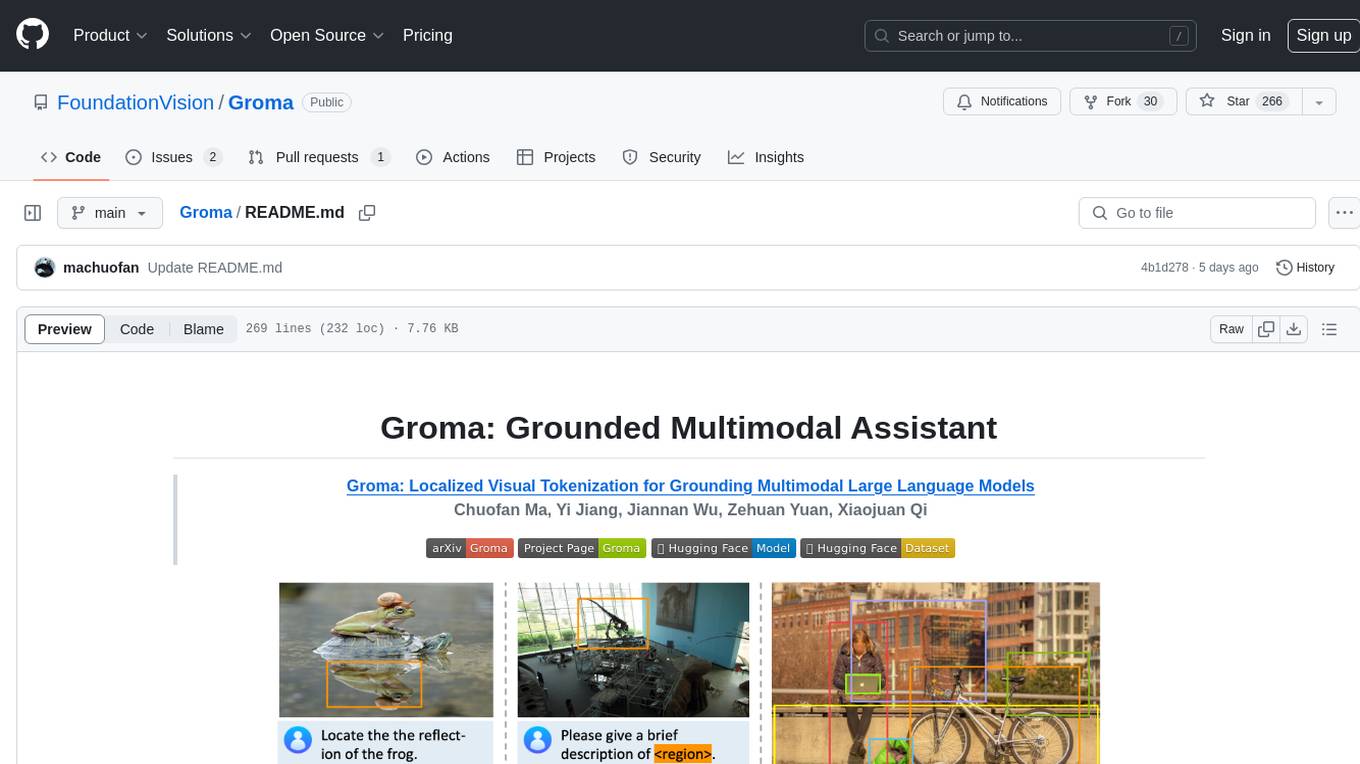
Groma
Groma is a grounded multimodal assistant that excels in region understanding and visual grounding. It can process user-defined region inputs and generate contextually grounded long-form responses. The tool presents a unique paradigm for multimodal large language models, focusing on visual tokenization for localization. Groma achieves state-of-the-art performance in referring expression comprehension benchmarks. The tool provides pretrained model weights and instructions for data preparation, training, inference, and evaluation. Users can customize training by starting from intermediate checkpoints. Groma is designed to handle tasks related to detection pretraining, alignment pretraining, instruction finetuning, instruction following, and more.
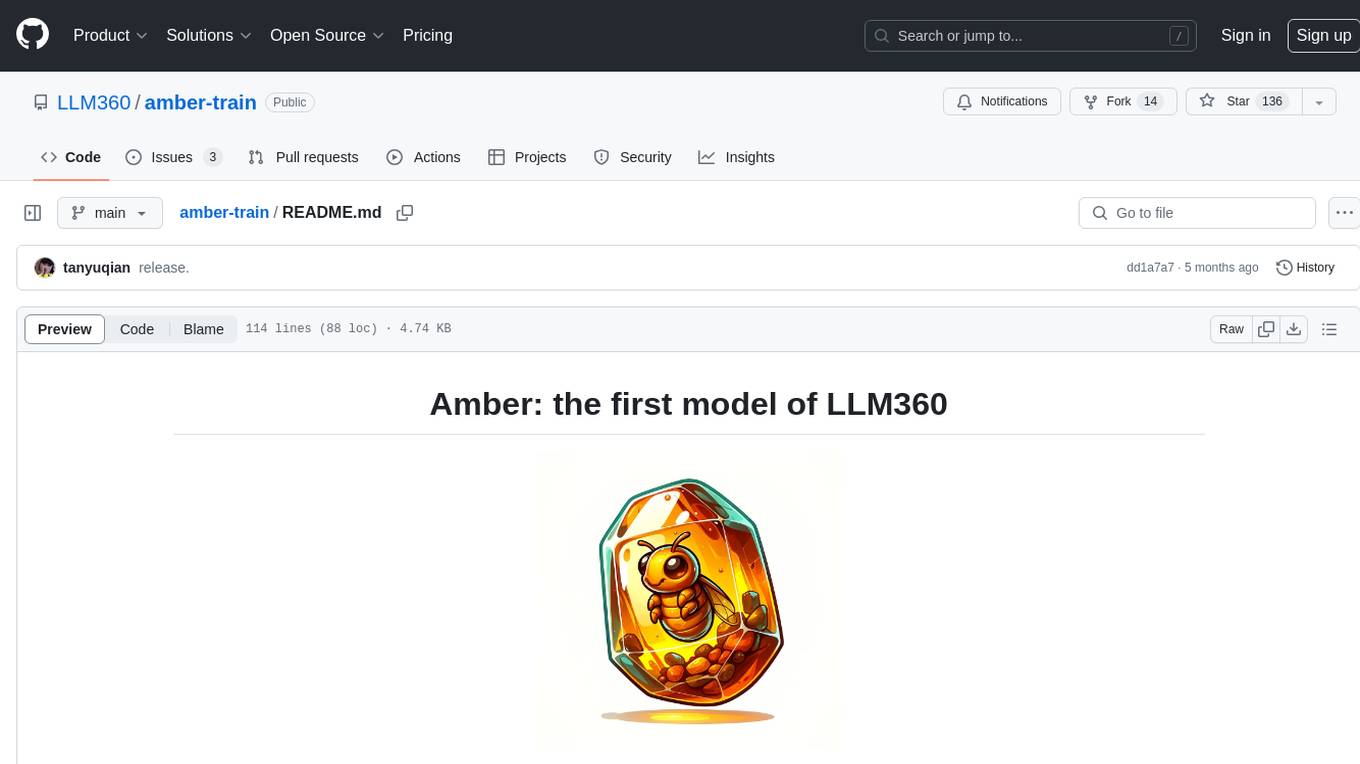
amber-train
Amber is the first model in the LLM360 family, an initiative for comprehensive and fully open-sourced LLMs. It is a 7B English language model with the LLaMA architecture. The model type is a language model with the same architecture as LLaMA-7B. It is licensed under Apache 2.0. The resources available include training code, data preparation, metrics, and fully processed Amber pretraining data. The model has been trained on various datasets like Arxiv, Book, C4, Refined-Web, StarCoder, StackExchange, and Wikipedia. The hyperparameters include a total of 6.7B parameters, hidden size of 4096, intermediate size of 11008, 32 attention heads, 32 hidden layers, RMSNorm ε of 1e^-6, max sequence length of 2048, and a vocabulary size of 32000.
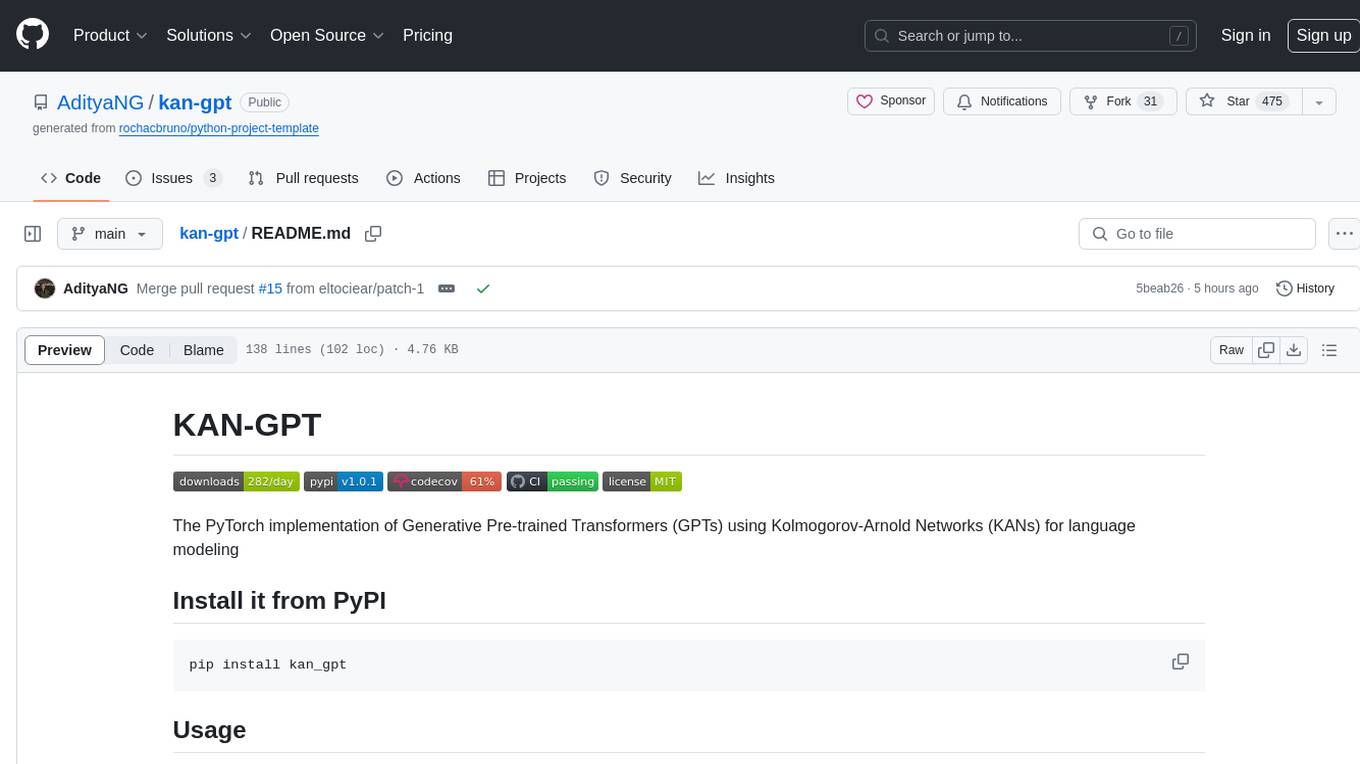
kan-gpt
The KAN-GPT repository is a PyTorch implementation of Generative Pre-trained Transformers (GPTs) using Kolmogorov-Arnold Networks (KANs) for language modeling. It provides a model for generating text based on prompts, with a focus on improving performance compared to traditional MLP-GPT models. The repository includes scripts for training the model, downloading datasets, and evaluating model performance. Development tasks include integrating with other libraries, testing, and documentation.
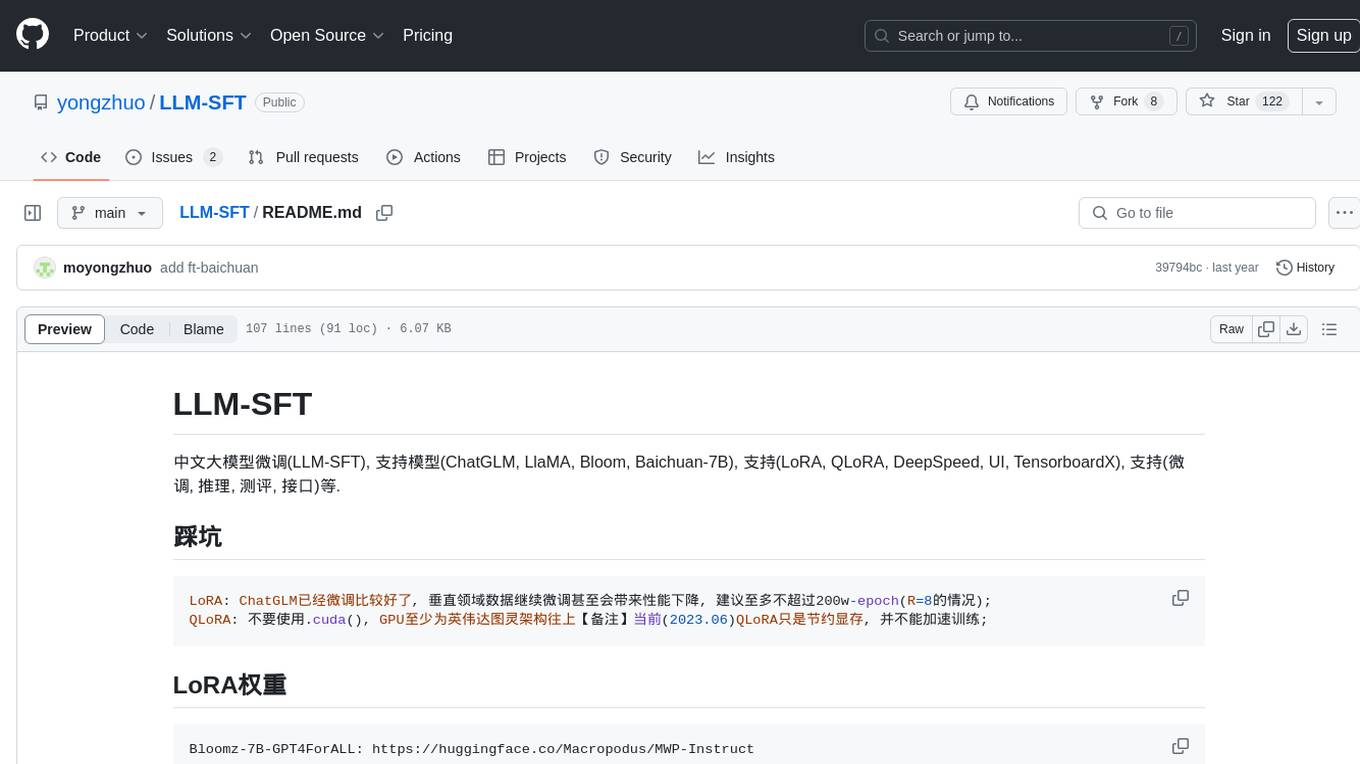
LLM-SFT
LLM-SFT is a Chinese large model fine-tuning tool that supports models such as ChatGLM, LlaMA, Bloom, Baichuan-7B, and frameworks like LoRA, QLoRA, DeepSpeed, UI, and TensorboardX. It facilitates tasks like fine-tuning, inference, evaluation, and API integration. The tool provides pre-trained weights for various models and datasets for Chinese language processing. It requires specific versions of libraries like transformers and torch for different functionalities.
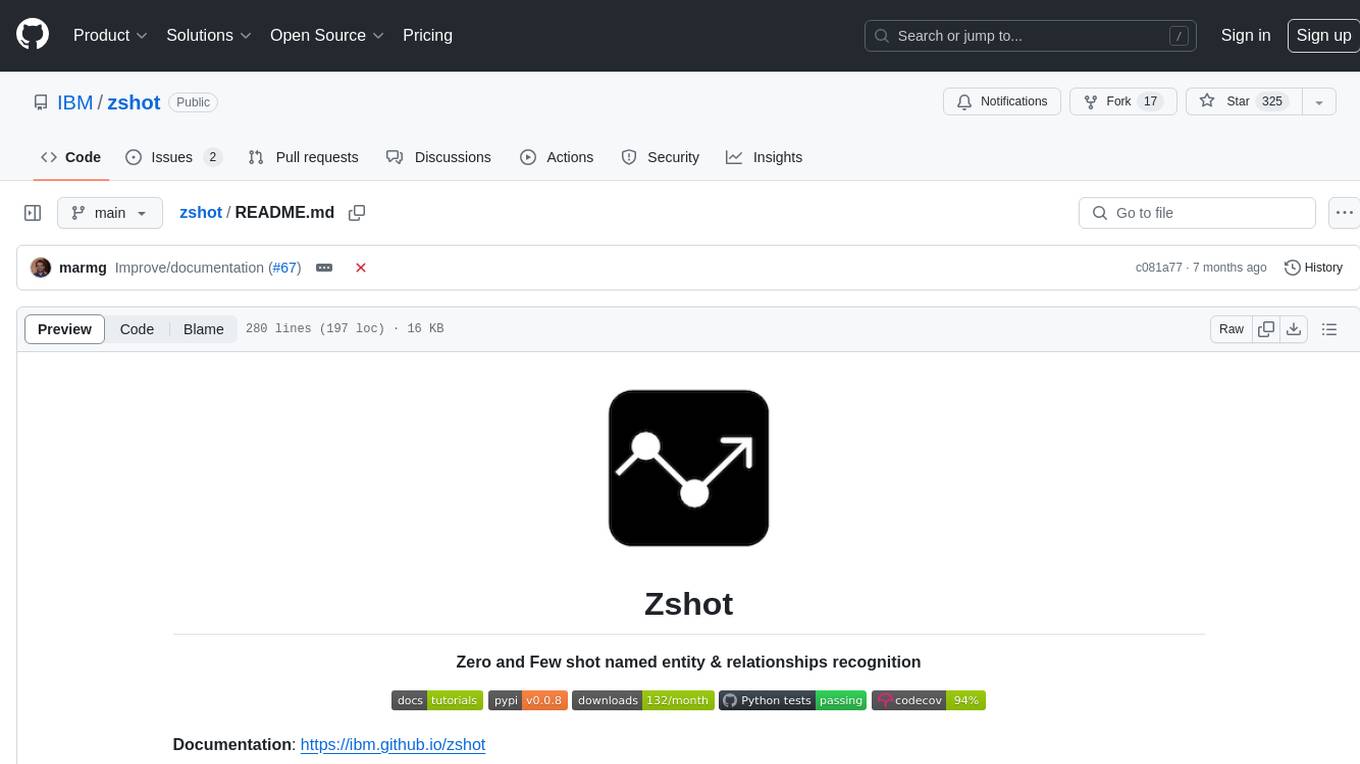
zshot
Zshot is a highly customizable framework for performing Zero and Few shot named entity and relationships recognition. It can be used for mentions extraction, wikification, zero and few shot named entity recognition, zero and few shot named relationship recognition, and visualization of zero-shot NER and RE extraction. The framework consists of two main components: the mentions extractor and the linker. There are multiple mentions extractors and linkers available, each serving a specific purpose. Zshot also includes a relations extractor and a knowledge extractor for extracting relations among entities and performing entity classification. The tool requires Python 3.6+ and dependencies like spacy, torch, transformers, evaluate, and datasets for evaluation over datasets like OntoNotes. Optional dependencies include flair and blink for additional functionalities. Zshot provides examples, tutorials, and evaluation methods to assess the performance of the components.
For similar jobs
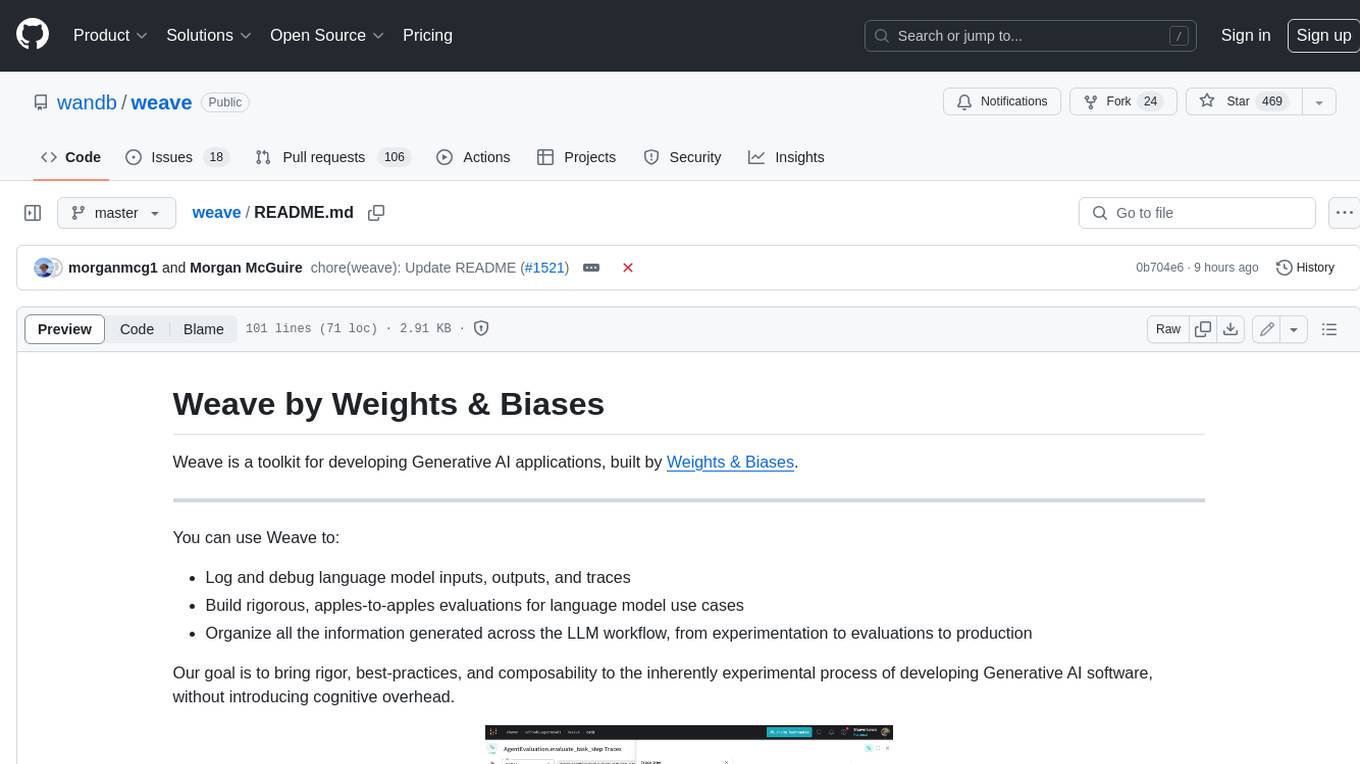
weave
Weave is a toolkit for developing Generative AI applications, built by Weights & Biases. With Weave, you can log and debug language model inputs, outputs, and traces; build rigorous, apples-to-apples evaluations for language model use cases; and organize all the information generated across the LLM workflow, from experimentation to evaluations to production. Weave aims to bring rigor, best-practices, and composability to the inherently experimental process of developing Generative AI software, without introducing cognitive overhead.
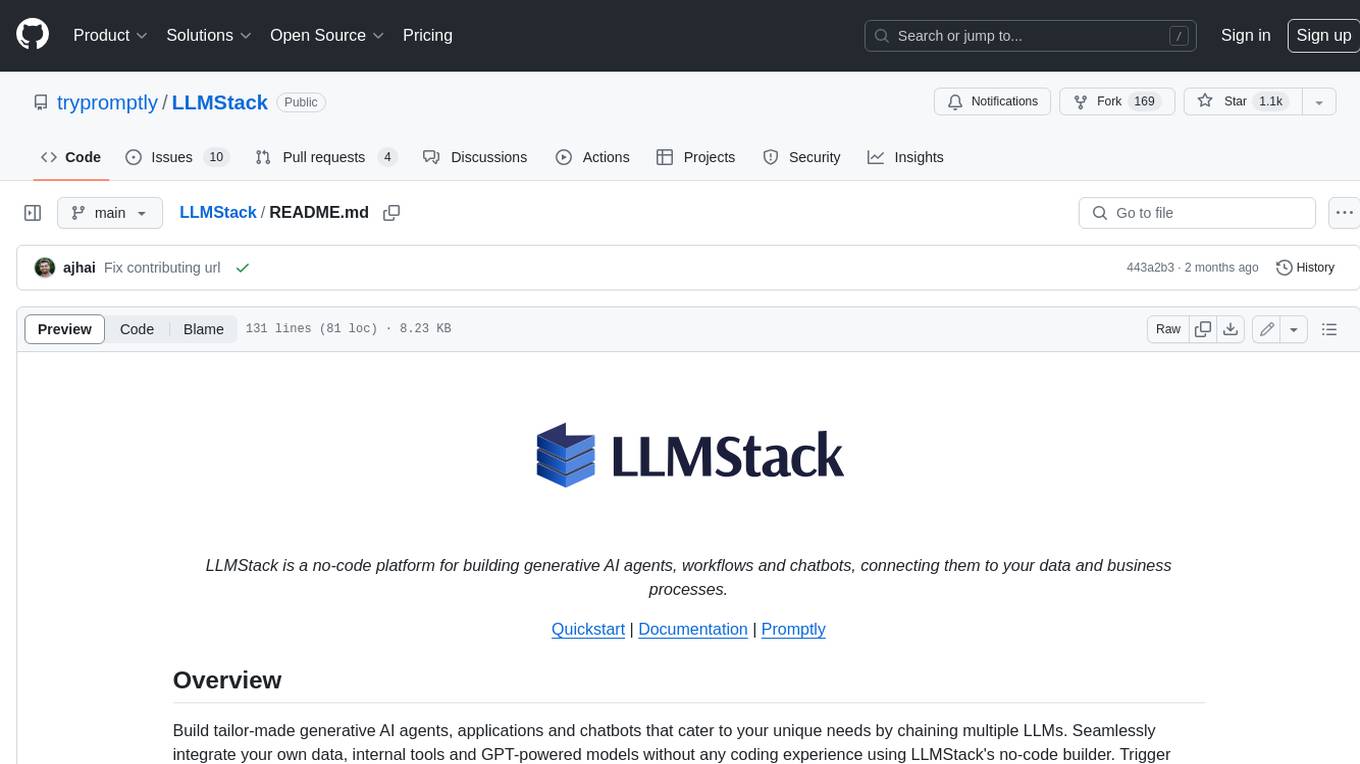
LLMStack
LLMStack is a no-code platform for building generative AI agents, workflows, and chatbots. It allows users to connect their own data, internal tools, and GPT-powered models without any coding experience. LLMStack can be deployed to the cloud or on-premise and can be accessed via HTTP API or triggered from Slack or Discord.
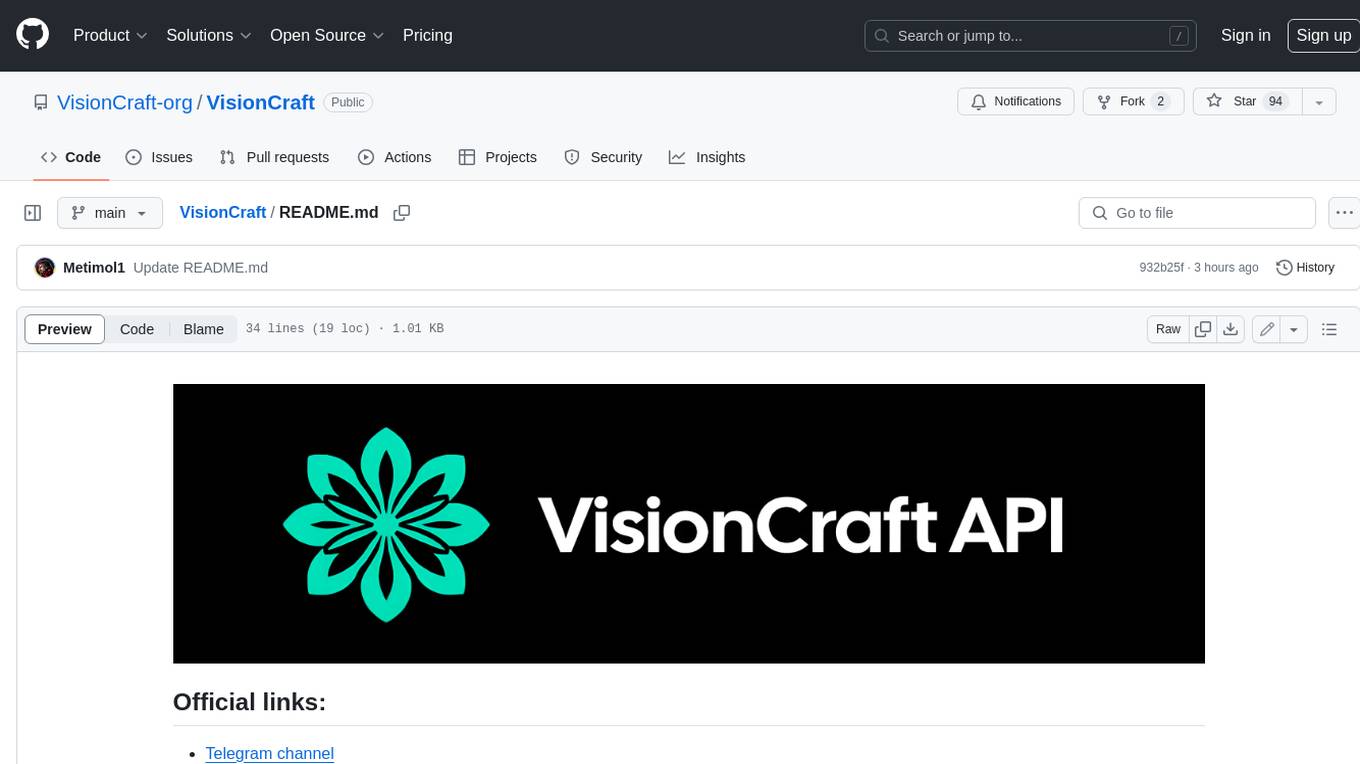
VisionCraft
The VisionCraft API is a free API for using over 100 different AI models. From images to sound.
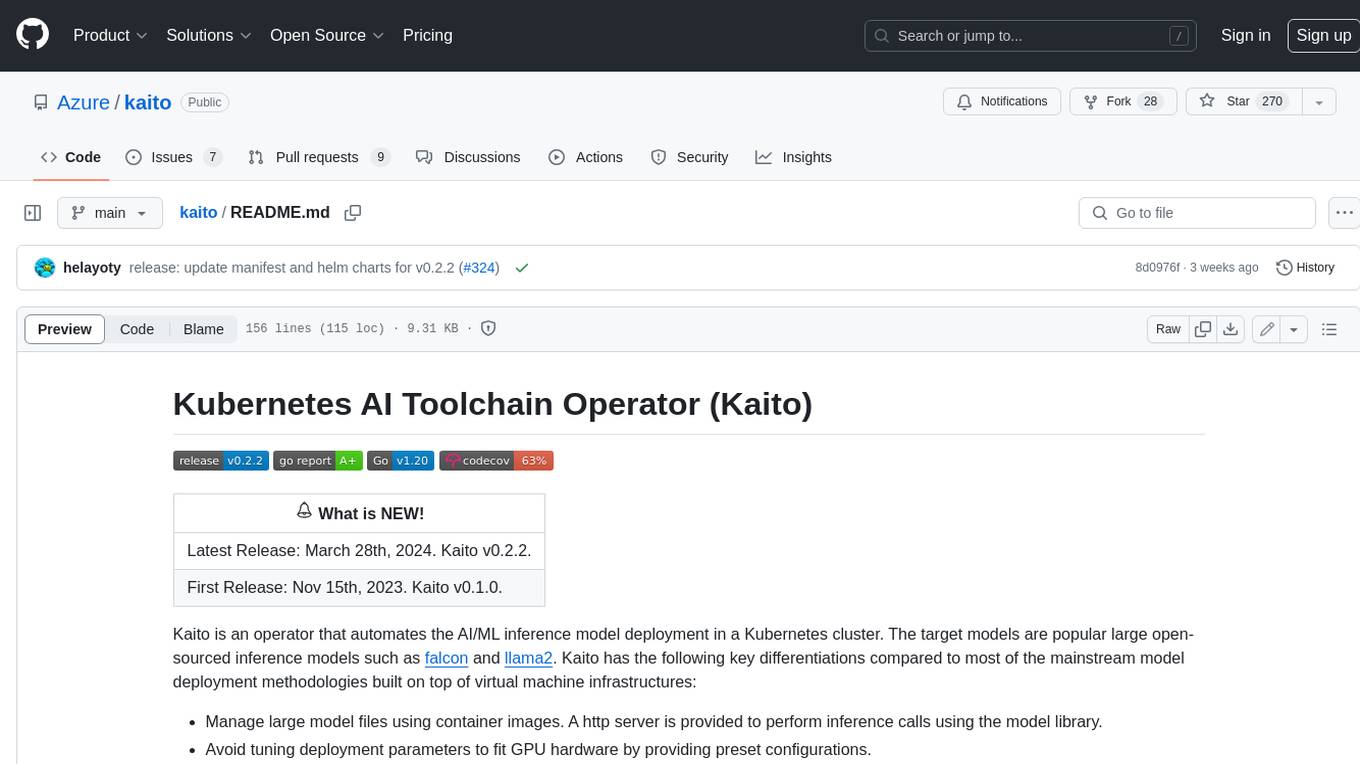
kaito
Kaito is an operator that automates the AI/ML inference model deployment in a Kubernetes cluster. It manages large model files using container images, avoids tuning deployment parameters to fit GPU hardware by providing preset configurations, auto-provisions GPU nodes based on model requirements, and hosts large model images in the public Microsoft Container Registry (MCR) if the license allows. Using Kaito, the workflow of onboarding large AI inference models in Kubernetes is largely simplified.
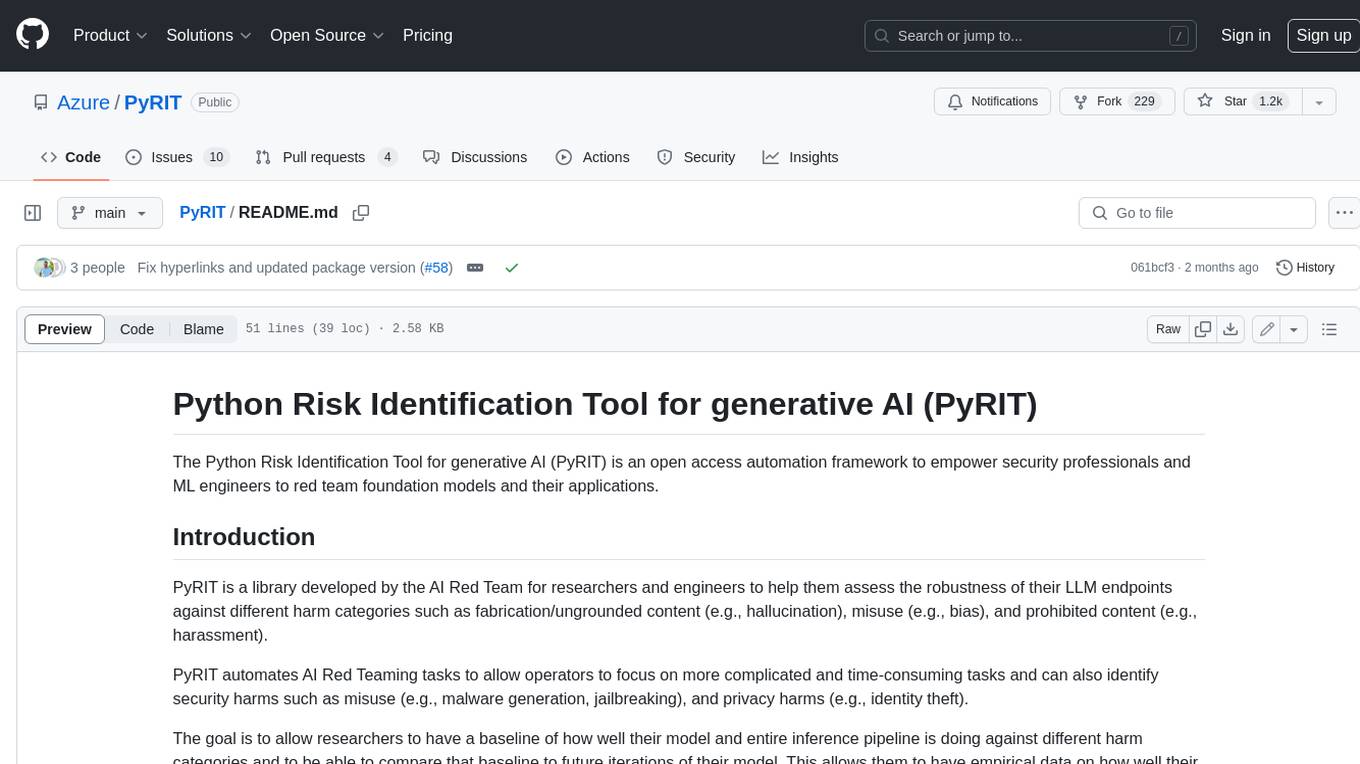
PyRIT
PyRIT is an open access automation framework designed to empower security professionals and ML engineers to red team foundation models and their applications. It automates AI Red Teaming tasks to allow operators to focus on more complicated and time-consuming tasks and can also identify security harms such as misuse (e.g., malware generation, jailbreaking), and privacy harms (e.g., identity theft). The goal is to allow researchers to have a baseline of how well their model and entire inference pipeline is doing against different harm categories and to be able to compare that baseline to future iterations of their model. This allows them to have empirical data on how well their model is doing today, and detect any degradation of performance based on future improvements.
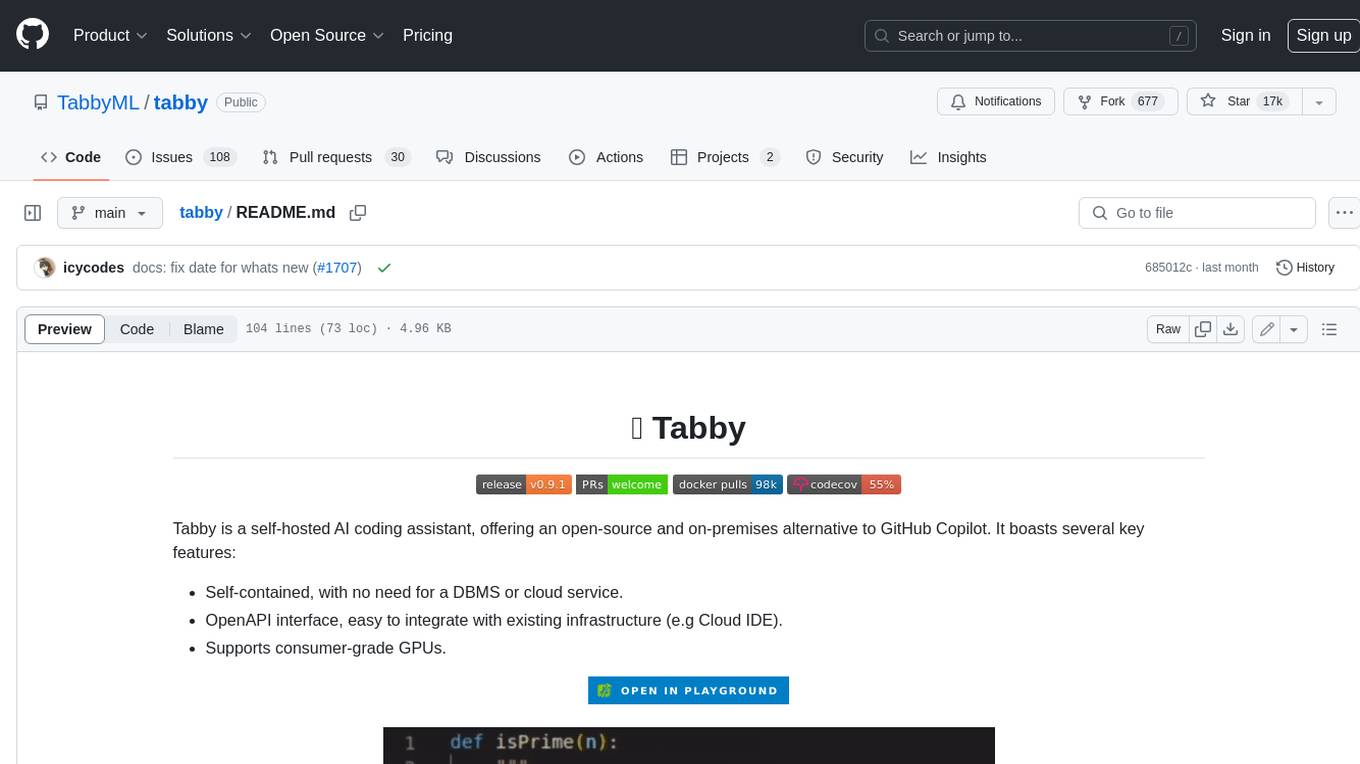
tabby
Tabby is a self-hosted AI coding assistant, offering an open-source and on-premises alternative to GitHub Copilot. It boasts several key features: * Self-contained, with no need for a DBMS or cloud service. * OpenAPI interface, easy to integrate with existing infrastructure (e.g Cloud IDE). * Supports consumer-grade GPUs.
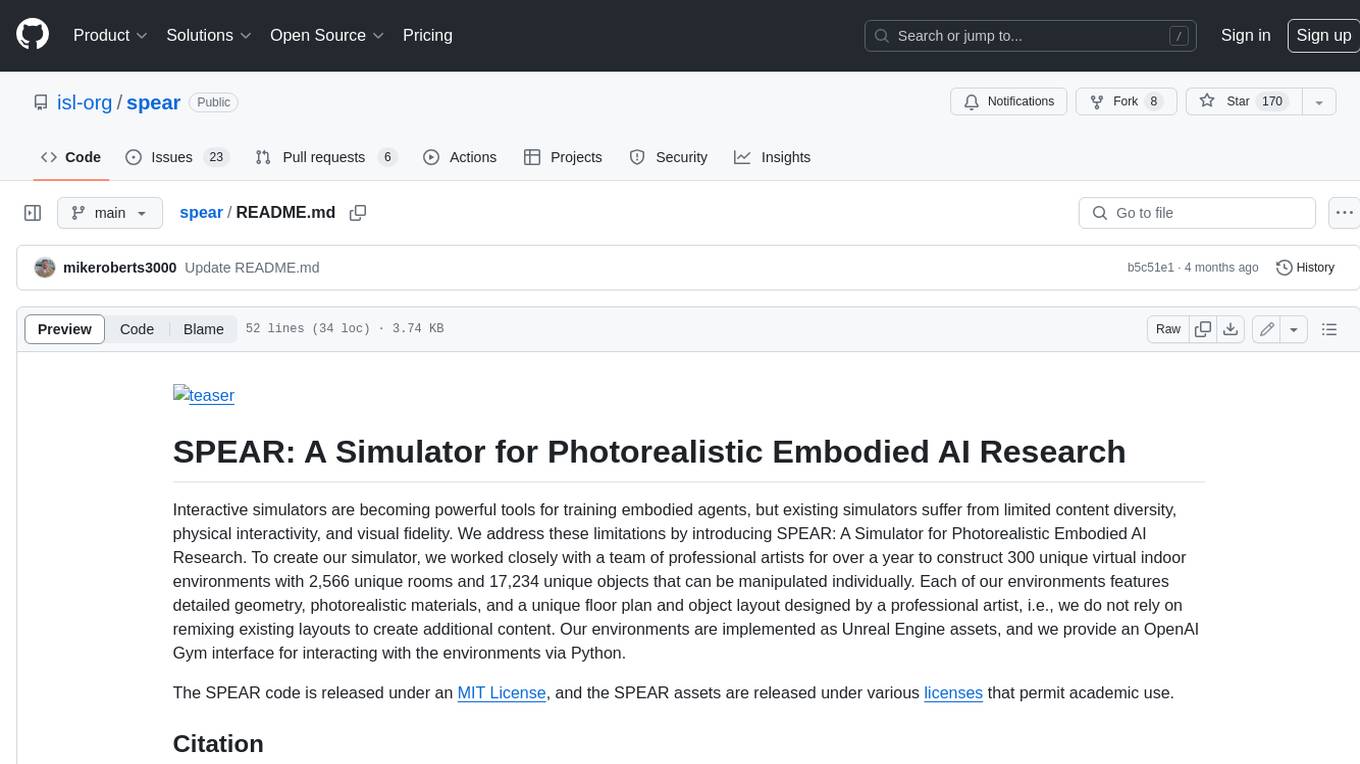
spear
SPEAR (Simulator for Photorealistic Embodied AI Research) is a powerful tool for training embodied agents. It features 300 unique virtual indoor environments with 2,566 unique rooms and 17,234 unique objects that can be manipulated individually. Each environment is designed by a professional artist and features detailed geometry, photorealistic materials, and a unique floor plan and object layout. SPEAR is implemented as Unreal Engine assets and provides an OpenAI Gym interface for interacting with the environments via Python.
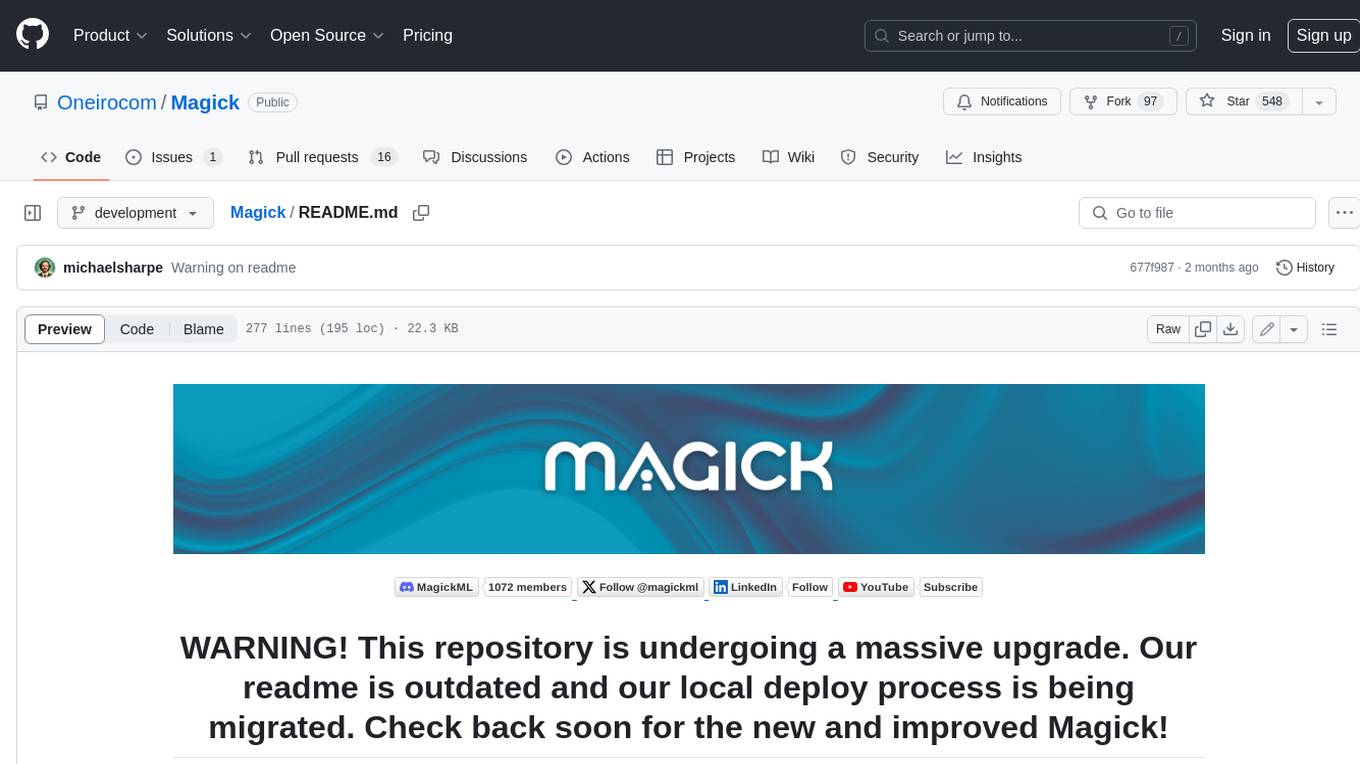
Magick
Magick is a groundbreaking visual AIDE (Artificial Intelligence Development Environment) for no-code data pipelines and multimodal agents. Magick can connect to other services and comes with nodes and templates well-suited for intelligent agents, chatbots, complex reasoning systems and realistic characters.