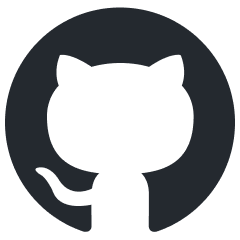
sailor-llm
Sailor: Open Language Models for South-East Asia
Stars: 71
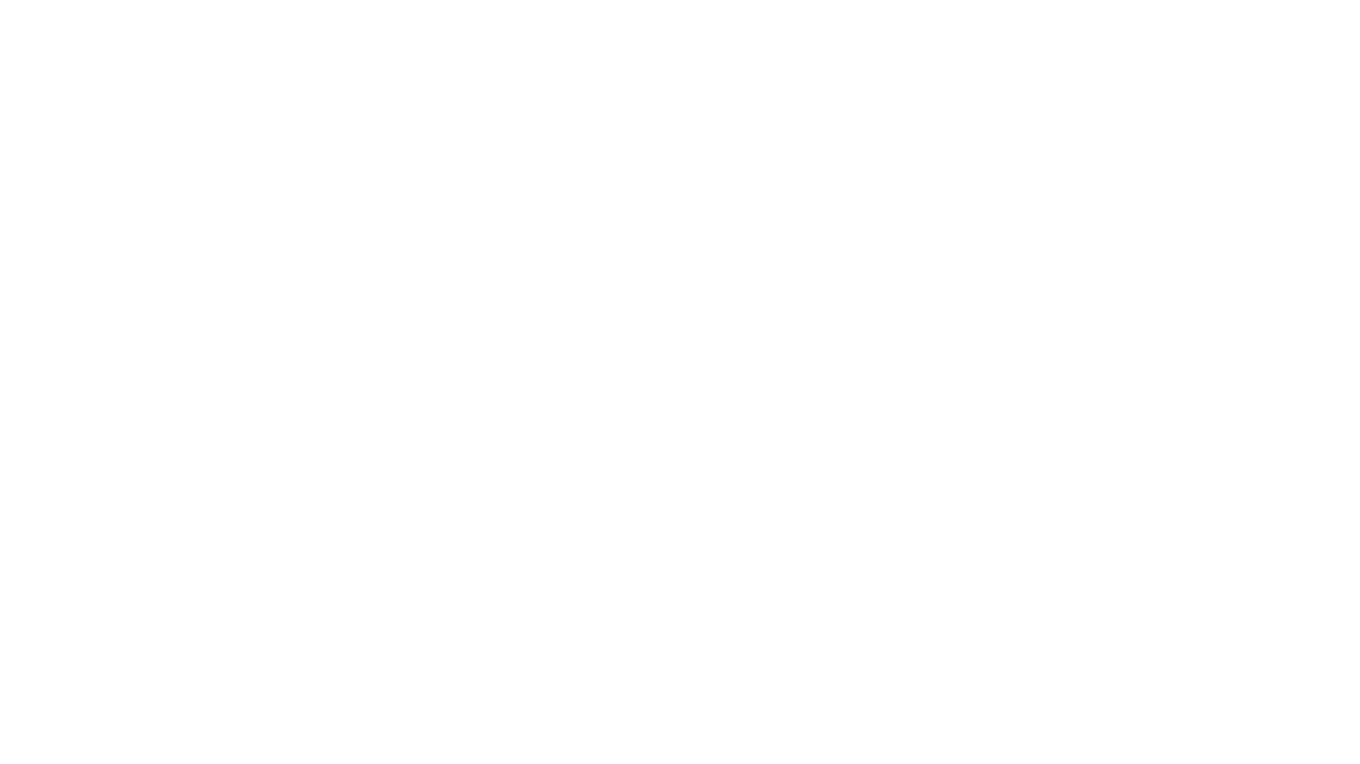
Sailor is a suite of open language models tailored for South-East Asia (SEA), focusing on languages such as Indonesian, Thai, Vietnamese, Malay, and Lao. Developed with careful data curation, Sailor models are designed to understand and generate text across diverse linguistic landscapes of the SEA region. Built from Qwen 1.5, Sailor encompasses models of varying sizes, spanning from 0.5B to 7B versions for different requirements. Benchmarking results demonstrate Sailor's proficiency in tasks such as question answering, commonsense reasoning, reading comprehension, and more in SEA languages.
README:
This repository contains the evaluation code for Sailor, a suite of open language models for South-East Asia.
Sailor is developed by the Sea AI Lab and Singapore University of Technology and Design.
Sailor is a suite of Open Language Models tailored for South-East Asia (SEA), focusing on languages such as 🇮🇩Indonesian, 🇹🇭Thai, 🇻🇳Vietnamese, 🇲🇾Malay, and 🇱🇦Lao. Developed with careful data curation, Sailor models are designed to understand and generate text across diverse linguistic landscapes of SEA region. Built from Qwen 1.5, Sailor encompasses models of varying sizes, spanning from 0.5B to 7B versions for different requirements. Benchmarking results demonstrate Sailor's proficiency in tasks such as question answering, commonsense reasoning, reading comprehension and etc. in SEA languages.
- Continually pretrained on 200 Billion to 400 Billion tokens over 7 languages, including Indonesian, Thai, Vietnamese, Malay, Lao, English and Chinese.
- Various model sizes (0.5B, 1.8B, 4B and 7B) to support different requirements.
- Strong performance on SEA benchmarks such as XQuAD, TydiQA, XCOPA, Belebele and M3Exam.
- No restrict on the research and the commercial use, but should comply with the Qwen 1.5 license.
To learn more details, please access the technical report.
You can find all the Sailor models in our Huggingface home page here:
- Sailor-0.5B
- Sailor-1.8B
- Sailor-4B
- Sailor-7B
- Sailor-0.5B-Chat
- Sailor-1.8B-Chat
- Sailor-4B-Chat
- Sailor-7B-Chat
Here are the results of the evaluation of the models on question answering tasks. The evaluation results are presented in the form of tables, where the first column is the model name, and the reset columns are the performance on Thai (th), Indonesian (id), and Vietnamese (vi) languages, respectively. The results of Sailor models are highlighted in bold. You can find the full evaluation results on the different tasks and our evaluation code to reproduce the results in the eval directory.
3-shot (EM / F1) | XQuAD (th) | TydiQA (id) | XQuAD (vi) |
---|---|---|---|
Qwen1.5-0.5B | 14.19 / 23.35 | 20.71 / 32.64 | 19.85 / 35.38 |
Sailor-0.5B | 15.84 / 27.58 | 30.44 / 54.74 | 21.13 / 40.57 |
Qwen1.5-1.8B | 27.24 / 43.56 | 29.73 / 53.76 | 29.17 / 48.15 |
Sailor-1.8B | 32.72 / 48.66 | 40.88 / 65.37 | 34.22 / 53.35 |
Qwen1.5-4B | 34.03 / 53.40 | 48.32 / 72.68 | 43.71 / 63.86 |
Sailor-4B | 46.82 / 63.34 | 53.98 / 73.48 | 47.65 / 67.09 |
Llama-2-7b | 30.64 / 43.80 | 56.64 / 72.14 | 46.96 / 66.16 |
Mistral-7B-v0.1 | 48.48 / 63.27 | 63.54 / 78.73 | 53.72 / 72.75 |
SeaLLM-7b-Hybrid | 49.70 / 67.62 | 50.62 / 75.21 | 49.62 / 70.74 |
SeaLLM-7b-v2 | 34.55 / 55.13 | 52.21 / 77.00 | 46.19 / 72.11 |
Qwen1.5-7B | 53.79 / 69.30 | 57.17 / 77.28 | 56.63 / 76.99 |
Sailor-7B | 57.88 / 71.06 | 60.53 / 75.42 | 53.81 / 74.62 |
We use OpenCompass to evaluate the models. To install the required packages, run the following command under this folder:
# setup opencompass environment
conda create --name opencompass python=3.10 pytorch torchvision pytorch-cuda -c nvidia -c pytorch -y
conda activate opencompass
git clone https://github.com/open-compass/opencompass opencompass
cd opencompass
pip install -e .
pip install pythainlp langid
mkdir data
To build the evaluation script, run the following command under this folder:
cp -r eval/configs/* opencompass/configs/
cp -r eval/data/* opencompass/data/
cp -r eval/datasets/* opencompass/opencompass/datasets/
cp eval/icl_sailor_evaluator.py opencompass/opencompass/openicl/icl_evaluator/
cp eval/sailor_text_postprocessors.py opencompass/opencompass/utils/
echo "from .icl_sailor_evaluator import AnsEvaluator, TextGenEvaluator # noqa" >> "opencompass/opencompass/openicl/icl_evaluator/__init__.py"
echo "from .sailor_text_postprocessors import * # noqa" >> "opencompass/opencompass/utils/__init__.py"
echo "from .xquad import * # noqa: F401, F403" >> "opencompass/opencompass/datasets/__init__.py"
echo "from .tydiqa_id import * # noqa: F401, F403" >> "opencompass/opencompass/datasets/__init__.py"
echo "from .xcopa_sea import * # noqa: F401, F403" >> "opencompass/opencompass/datasets/__init__.py"
echo "from .m3exam import * # noqa: F401, F403" >> "opencompass/opencompass/datasets/__init__.py"
echo "from .belebele import * # noqa: F401, F403" >> "opencompass/opencompass/datasets/__init__.py"
cp eval/eval_sailor.py opencompass/configs/
To run the evaluation, run the following command under this folder:
cd opencompass
python run.py configs/eval_sailor.py -w outputs/sailor --num-gpus 1 --max-num-workers 64
You can also modify the script to evaluate other models like Qwen1.5, Llama, Mistral, etc.
We provide a simple demo to chat with Sailor-7B-Chat . You can also develop it using the provided demo code.
If you use this repository or sailor models, please cite
@misc{dou2024sailor,
title={Sailor: Open Language Models for South-East Asia},
author={Longxu Dou and Qian Liu and Guangtao Zeng and Jia Guo and Jiahui Zhou and Wei Lu and Min Lin},
year={2024},
eprint={2404.03608},
archivePrefix={arXiv},
primaryClass={cs.CL}
}
If you have any questions, please raise an issue in our Github or contact us at [email protected] and [email protected].
For Tasks:
Click tags to check more tools for each tasksFor Jobs:
Alternative AI tools for sailor-llm
Similar Open Source Tools
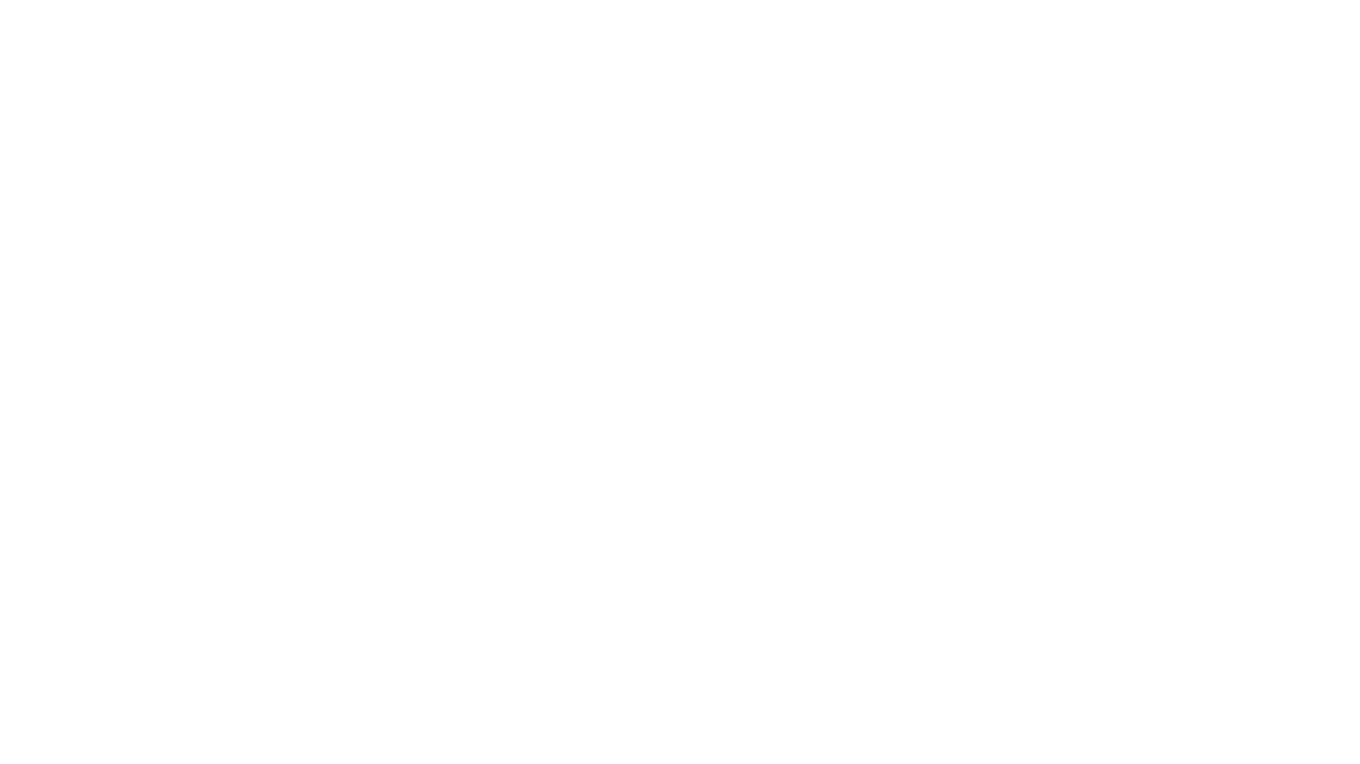
sailor-llm
Sailor is a suite of open language models tailored for South-East Asia (SEA), focusing on languages such as Indonesian, Thai, Vietnamese, Malay, and Lao. Developed with careful data curation, Sailor models are designed to understand and generate text across diverse linguistic landscapes of the SEA region. Built from Qwen 1.5, Sailor encompasses models of varying sizes, spanning from 0.5B to 7B versions for different requirements. Benchmarking results demonstrate Sailor's proficiency in tasks such as question answering, commonsense reasoning, reading comprehension, and more in SEA languages.
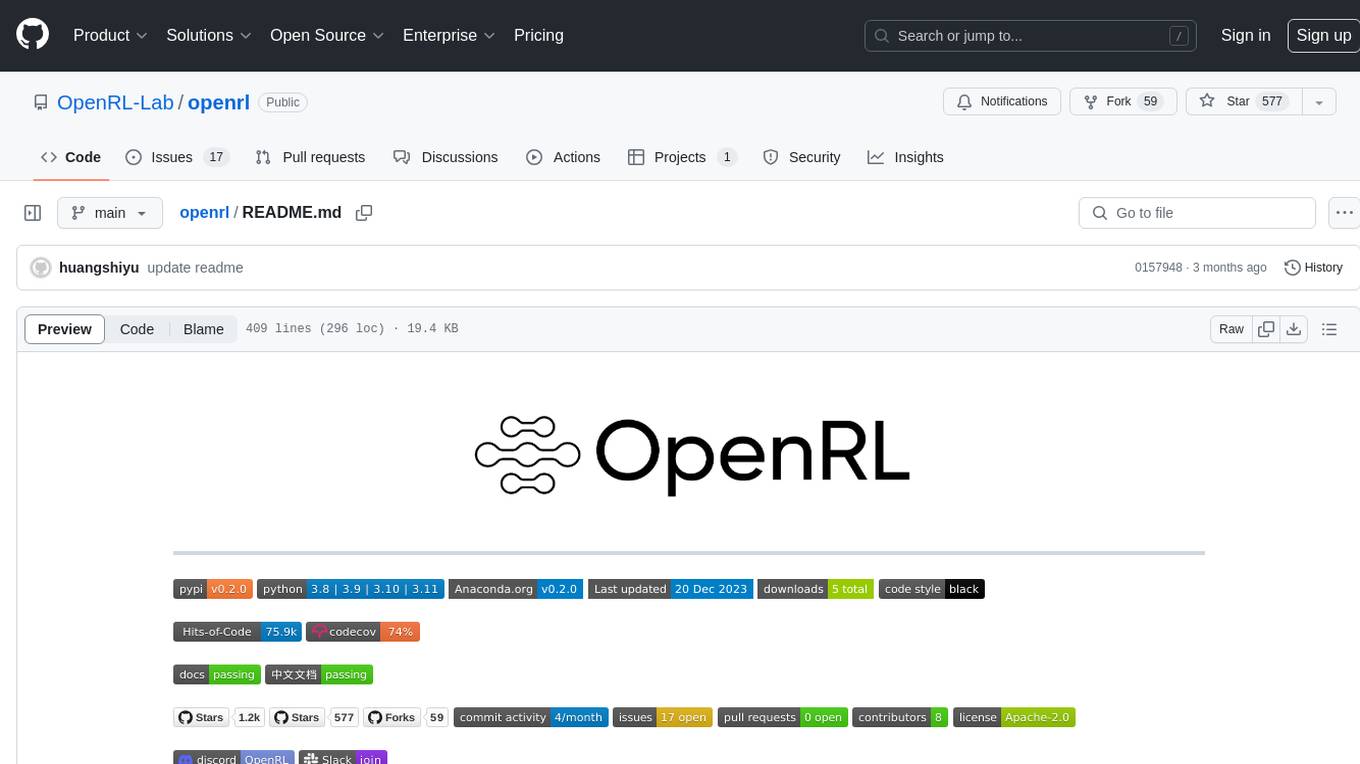
openrl
OpenRL is an open-source general reinforcement learning research framework that supports training for various tasks such as single-agent, multi-agent, offline RL, self-play, and natural language. Developed based on PyTorch, the goal of OpenRL is to provide a simple-to-use, flexible, efficient and sustainable platform for the reinforcement learning research community. It supports a universal interface for all tasks/environments, single-agent and multi-agent tasks, offline RL training with expert dataset, self-play training, reinforcement learning training for natural language tasks, DeepSpeed, Arena for evaluation, importing models and datasets from Hugging Face, user-defined environments, models, and datasets, gymnasium environments, callbacks, visualization tools, unit testing, and code coverage testing. It also supports various algorithms like PPO, DQN, SAC, and environments like Gymnasium, MuJoCo, Atari, and more.
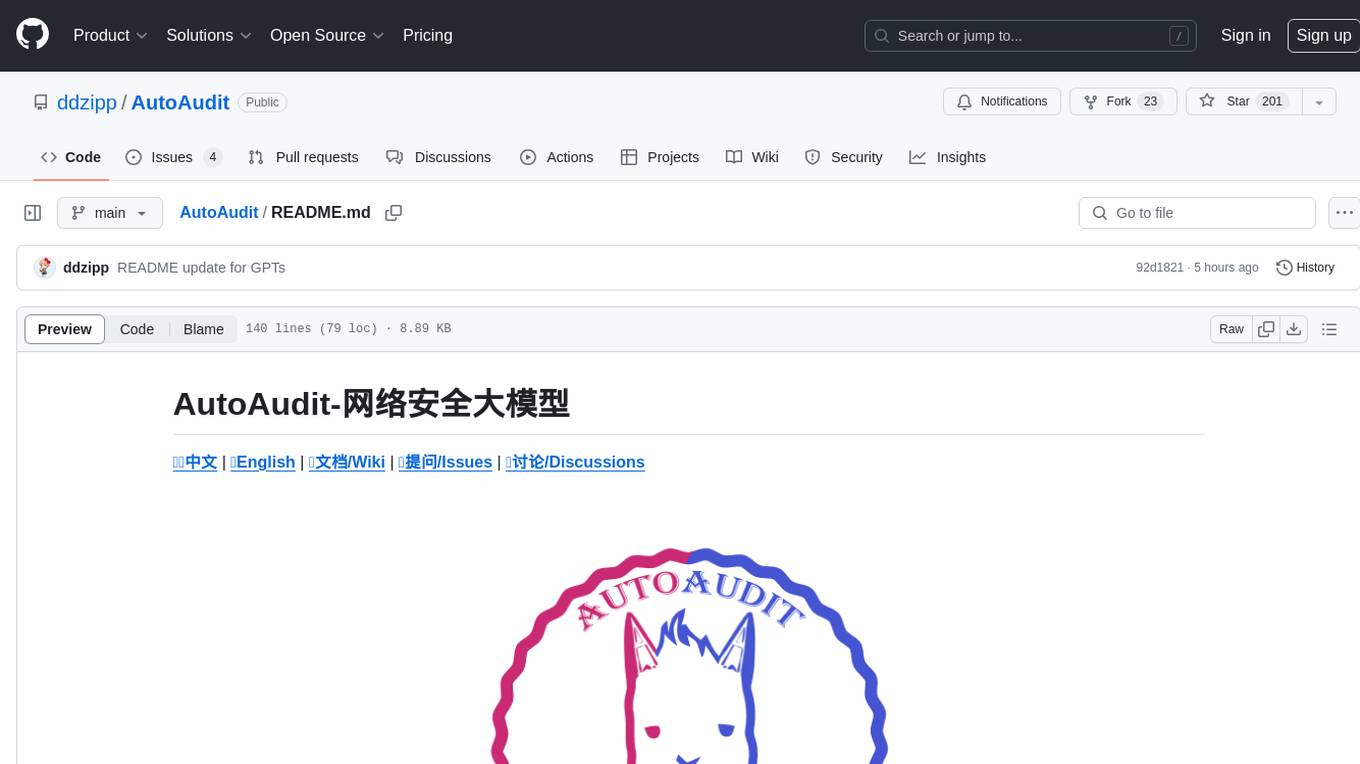
AutoAudit
AutoAudit is an open-source large language model specifically designed for the field of network security. It aims to provide powerful natural language processing capabilities for security auditing and network defense, including analyzing malicious code, detecting network attacks, and predicting security vulnerabilities. By coupling AutoAudit with ClamAV, a security scanning platform has been created for practical security audit applications. The tool is intended to assist security professionals with accurate and fast analysis and predictions to combat evolving network threats.
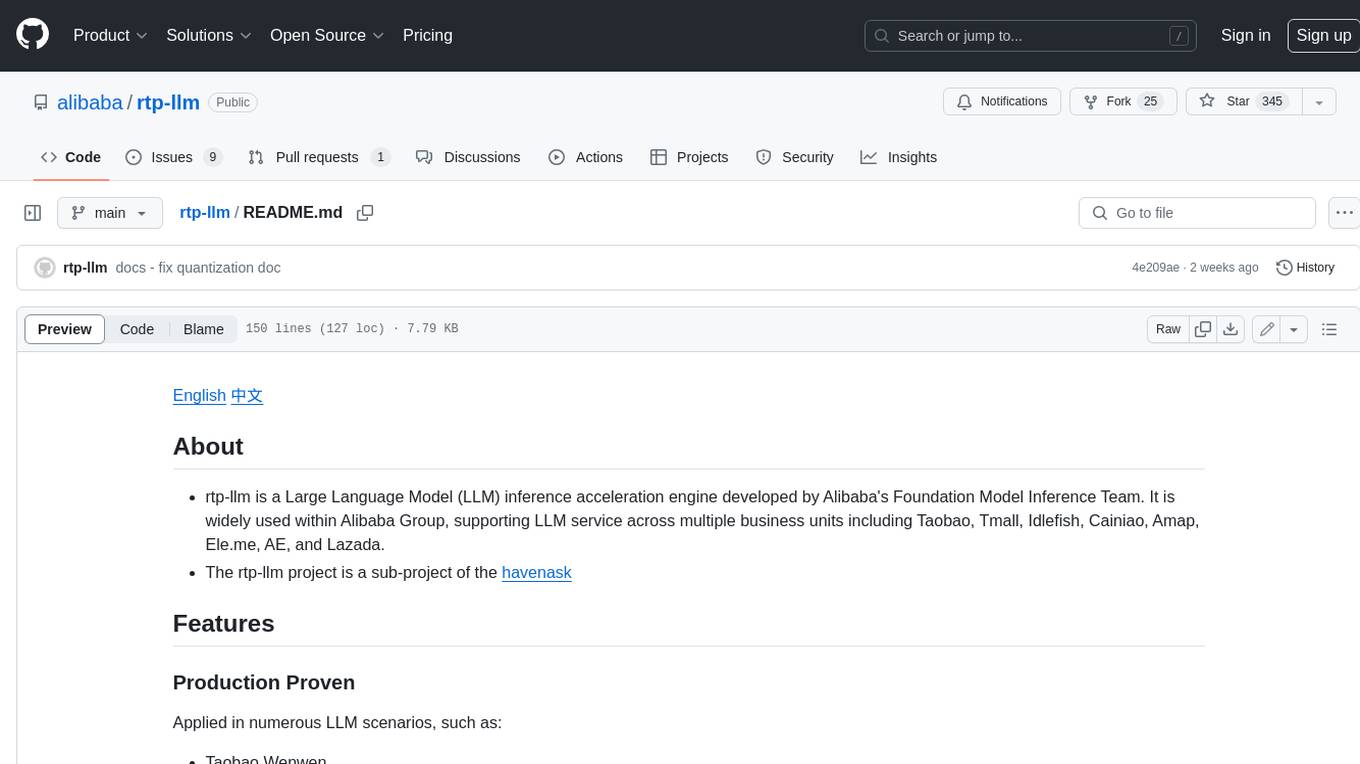
rtp-llm
**rtp-llm** is a Large Language Model (LLM) inference acceleration engine developed by Alibaba's Foundation Model Inference Team. It is widely used within Alibaba Group, supporting LLM service across multiple business units including Taobao, Tmall, Idlefish, Cainiao, Amap, Ele.me, AE, and Lazada. The rtp-llm project is a sub-project of the havenask.
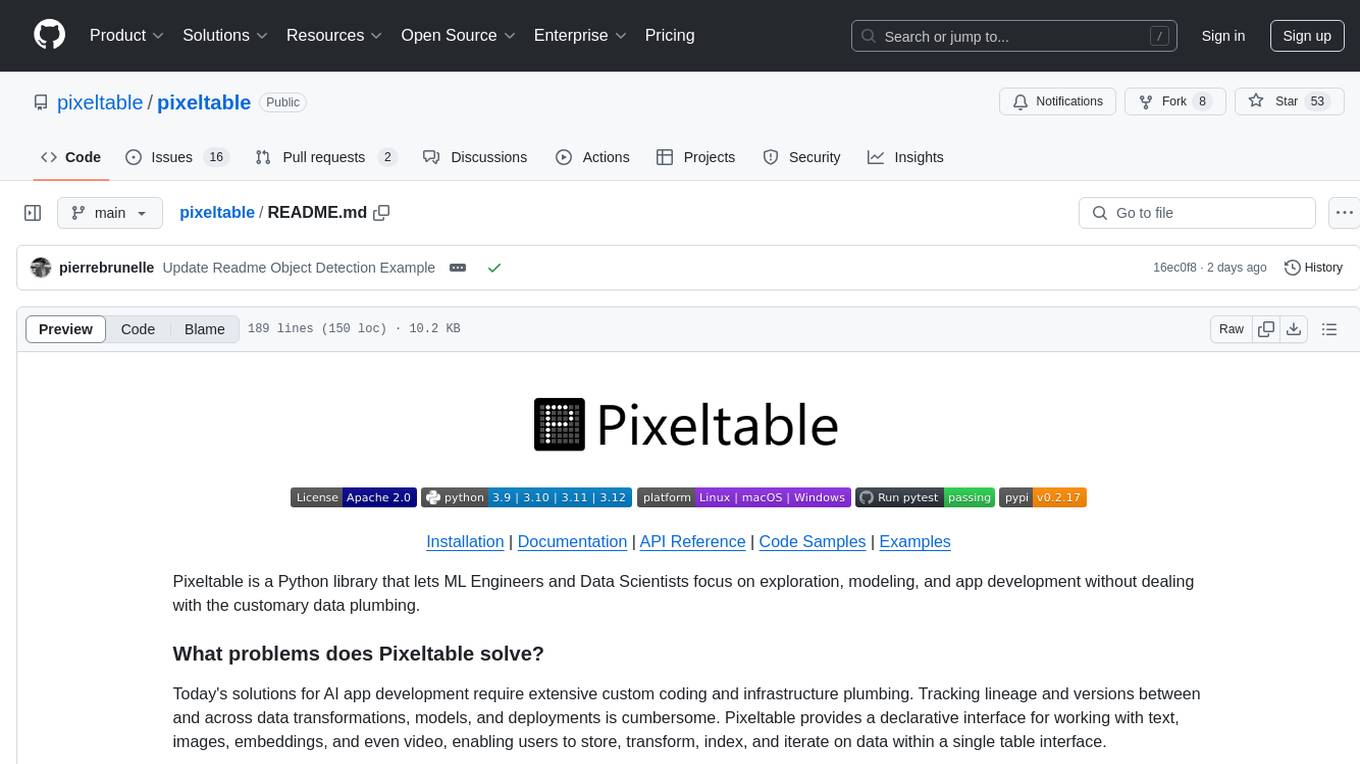
pixeltable
Pixeltable is a Python library designed for ML Engineers and Data Scientists to focus on exploration, modeling, and app development without the need to handle data plumbing. It provides a declarative interface for working with text, images, embeddings, and video, enabling users to store, transform, index, and iterate on data within a single table interface. Pixeltable is persistent, acting as a database unlike in-memory Python libraries such as Pandas. It offers features like data storage and versioning, combined data and model lineage, indexing, orchestration of multimodal workloads, incremental updates, and automatic production-ready code generation. The tool emphasizes transparency, reproducibility, cost-saving through incremental data changes, and seamless integration with existing Python code and libraries.
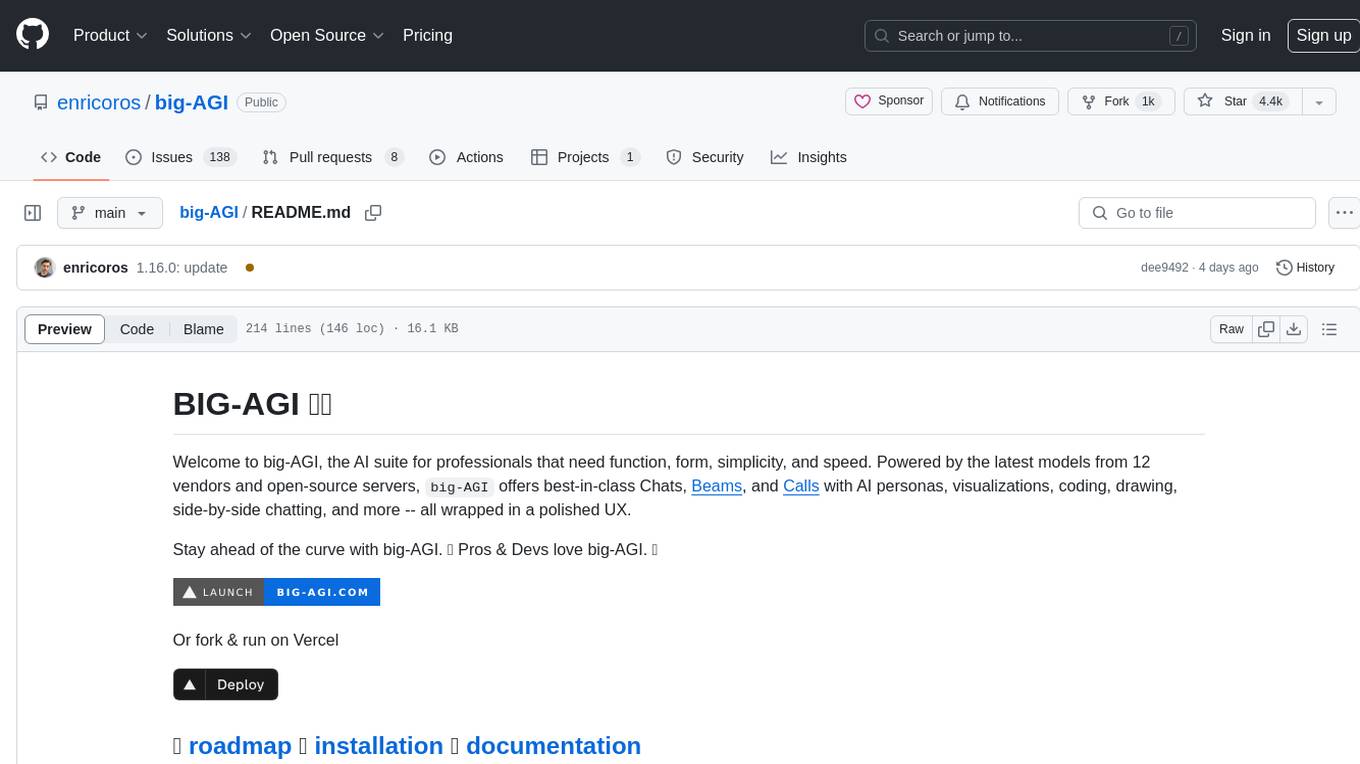
big-AGI
big-AGI is an AI suite designed for professionals seeking function, form, simplicity, and speed. It offers best-in-class Chats, Beams, and Calls with AI personas, visualizations, coding, drawing, side-by-side chatting, and more, all wrapped in a polished UX. The tool is powered by the latest models from 12 vendors and open-source servers, providing users with advanced AI capabilities and a seamless user experience. With continuous updates and enhancements, big-AGI aims to stay ahead of the curve in the AI landscape, catering to the needs of both developers and AI enthusiasts.
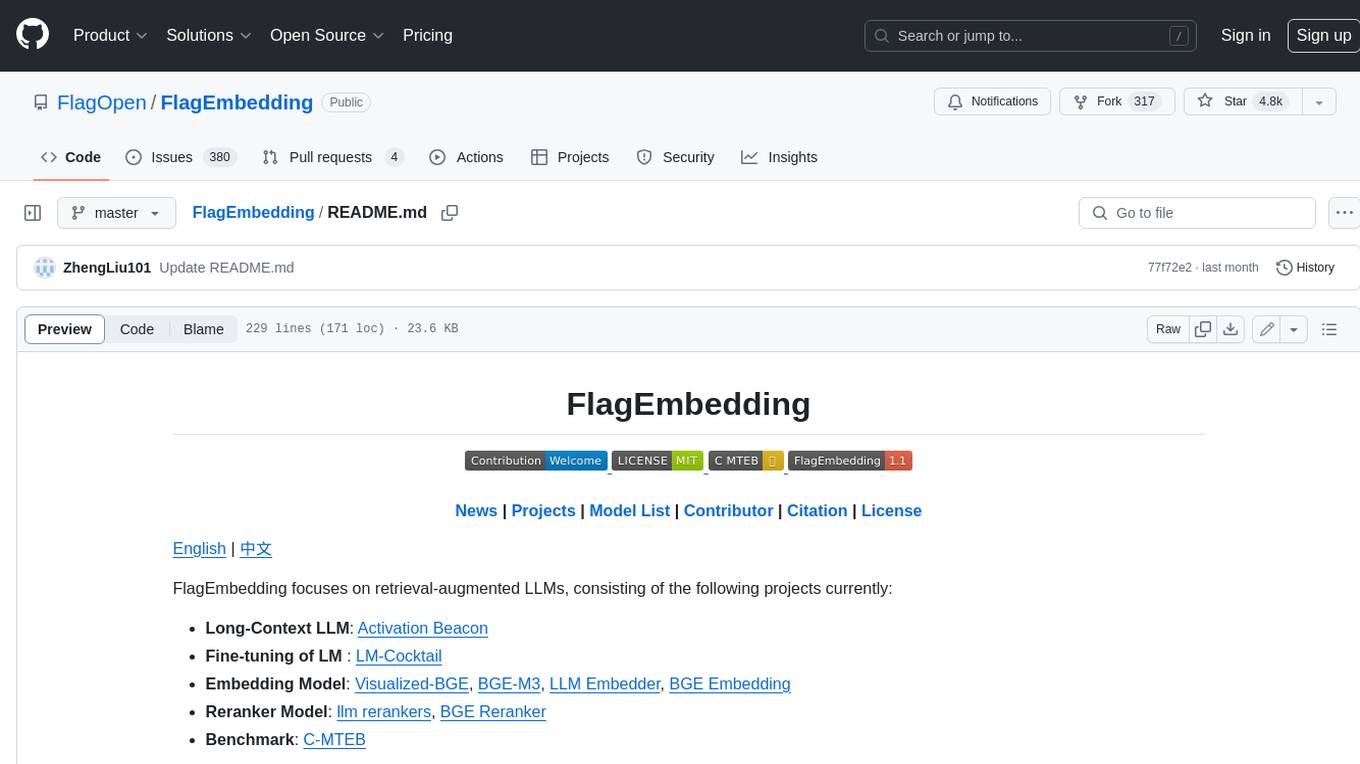
FlagEmbedding
FlagEmbedding focuses on retrieval-augmented LLMs, consisting of the following projects currently: * **Long-Context LLM** : Activation Beacon * **Fine-tuning of LM** : LM-Cocktail * **Embedding Model** : Visualized-BGE, BGE-M3, LLM Embedder, BGE Embedding * **Reranker Model** : llm rerankers, BGE Reranker * **Benchmark** : C-MTEB
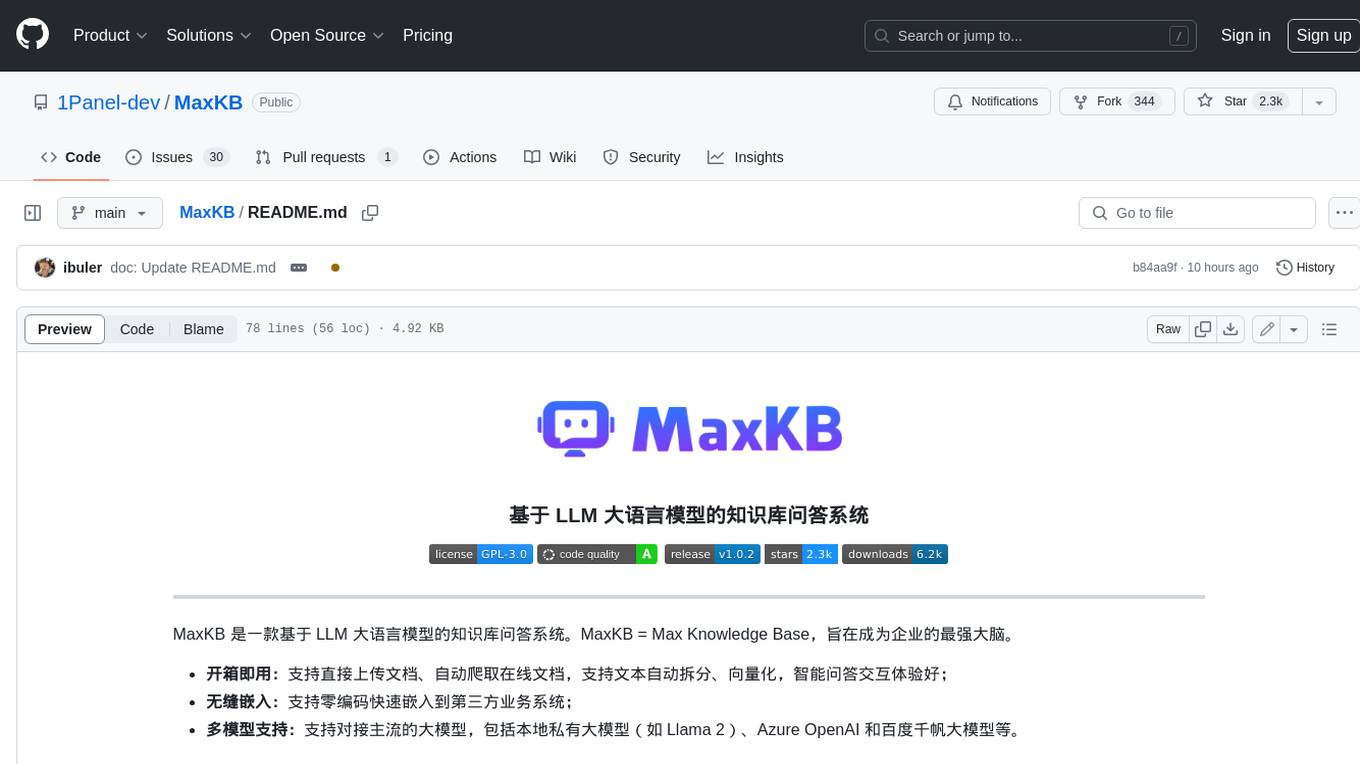
MaxKB
MaxKB is a knowledge base Q&A system based on the LLM large language model. MaxKB = Max Knowledge Base, which aims to become the most powerful brain of the enterprise.
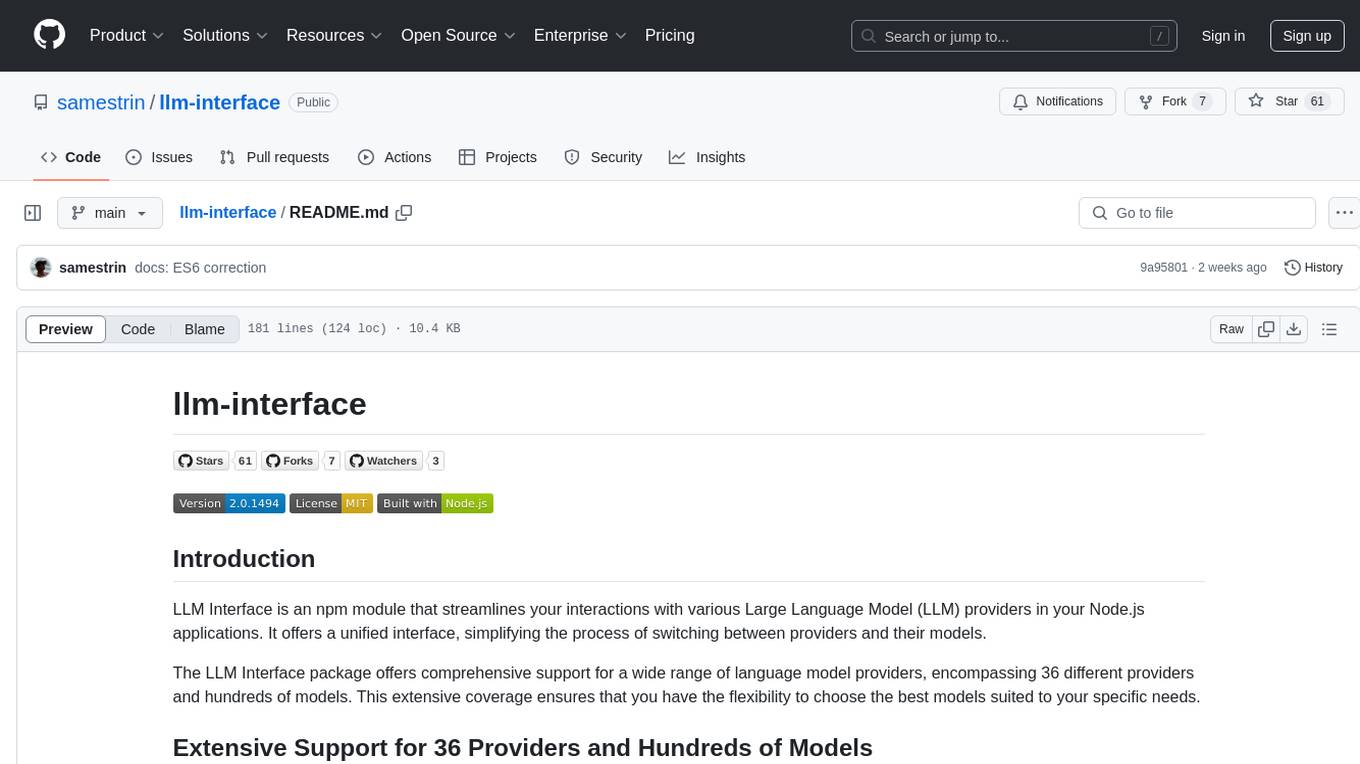
llm-interface
LLM Interface is an npm module that streamlines interactions with various Large Language Model (LLM) providers in Node.js applications. It offers a unified interface for switching between providers and models, supporting 36 providers and hundreds of models. Features include chat completion, streaming, error handling, extensibility, response caching, retries, JSON output, and repair. The package relies on npm packages like axios, @google/generative-ai, dotenv, jsonrepair, and loglevel. Installation is done via npm, and usage involves sending prompts to LLM providers. Tests can be run using npm test. Contributions are welcome under the MIT License.
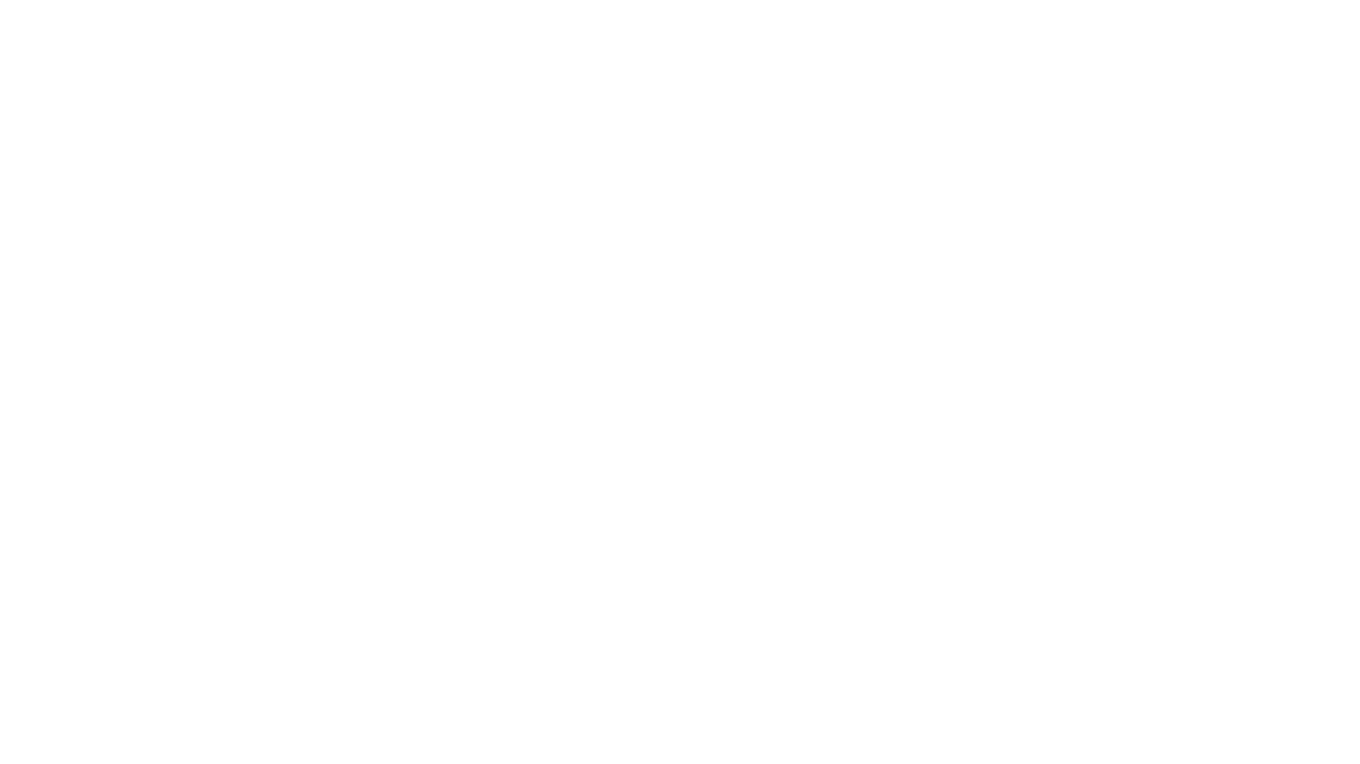
auto-news
Auto-News is an automatic news aggregator tool that utilizes Large Language Models (LLM) to pull information from various sources such as Tweets, RSS feeds, YouTube videos, web articles, Reddit, and journal notes. The tool aims to help users efficiently read and filter content based on personal interests, providing a unified reading experience and organizing information effectively. It features feed aggregation with summarization, transcript generation for videos and articles, noise reduction, task organization, and deep dive topic exploration. The tool supports multiple LLM backends, offers weekly top-k aggregations, and can be deployed on Linux/MacOS using docker-compose or Kubernetes.
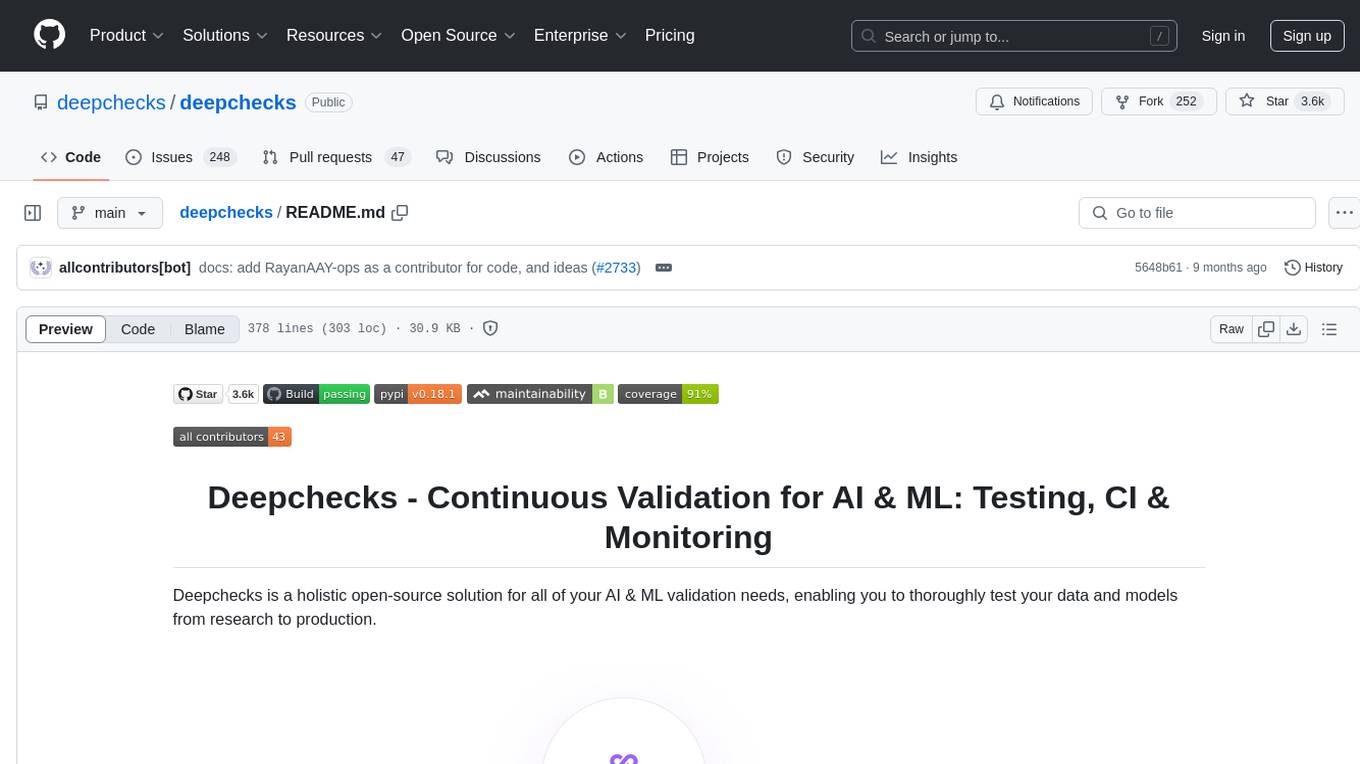
deepchecks
Deepchecks is a holistic open-source solution for AI & ML validation needs, enabling thorough testing of data and models from research to production. It includes components for testing, CI & testing management, and monitoring. Users can install and use Deepchecks for testing and monitoring their AI models, with customizable checks and suites for tabular, NLP, and computer vision data. The tool provides visual reports, pythonic/json output for processing, and a dynamic UI for collaboration and monitoring. Deepchecks is open source, with premium features available under a commercial license for monitoring components.
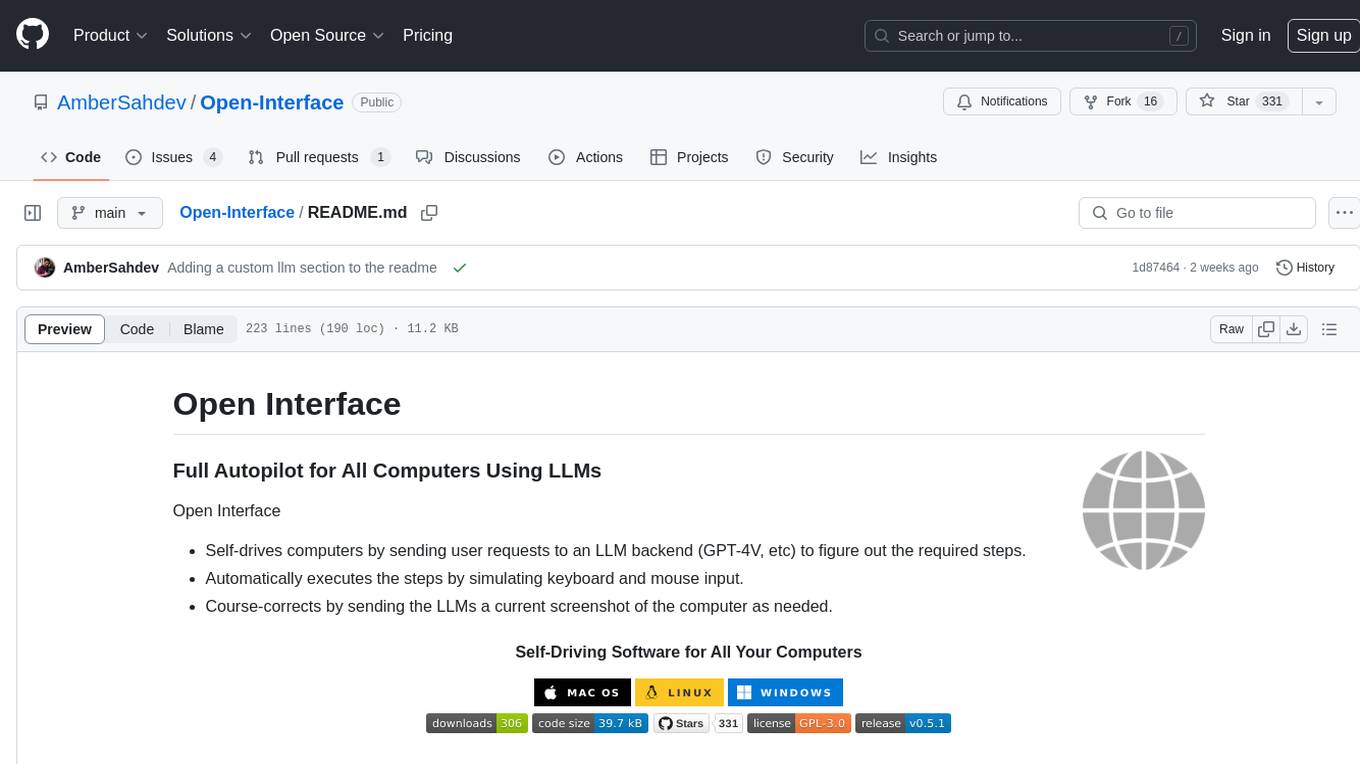
Open-Interface
Open Interface is a self-driving software that automates computer tasks by sending user requests to a language model backend (e.g., GPT-4V) and simulating keyboard and mouse inputs to execute the steps. It course-corrects by sending current screenshots to the language models. The tool supports MacOS, Linux, and Windows, and requires setting up the OpenAI API key for access to GPT-4V. It can automate tasks like creating meal plans, setting up custom language model backends, and more. Open Interface is currently not efficient in accurate spatial reasoning, tracking itself in tabular contexts, and navigating complex GUI-rich applications. Future improvements aim to enhance the tool's capabilities with better models trained on video walkthroughs. The tool is cost-effective, with user requests priced between $0.05 - $0.20, and offers features like interrupting the app and primary display visibility in multi-monitor setups.
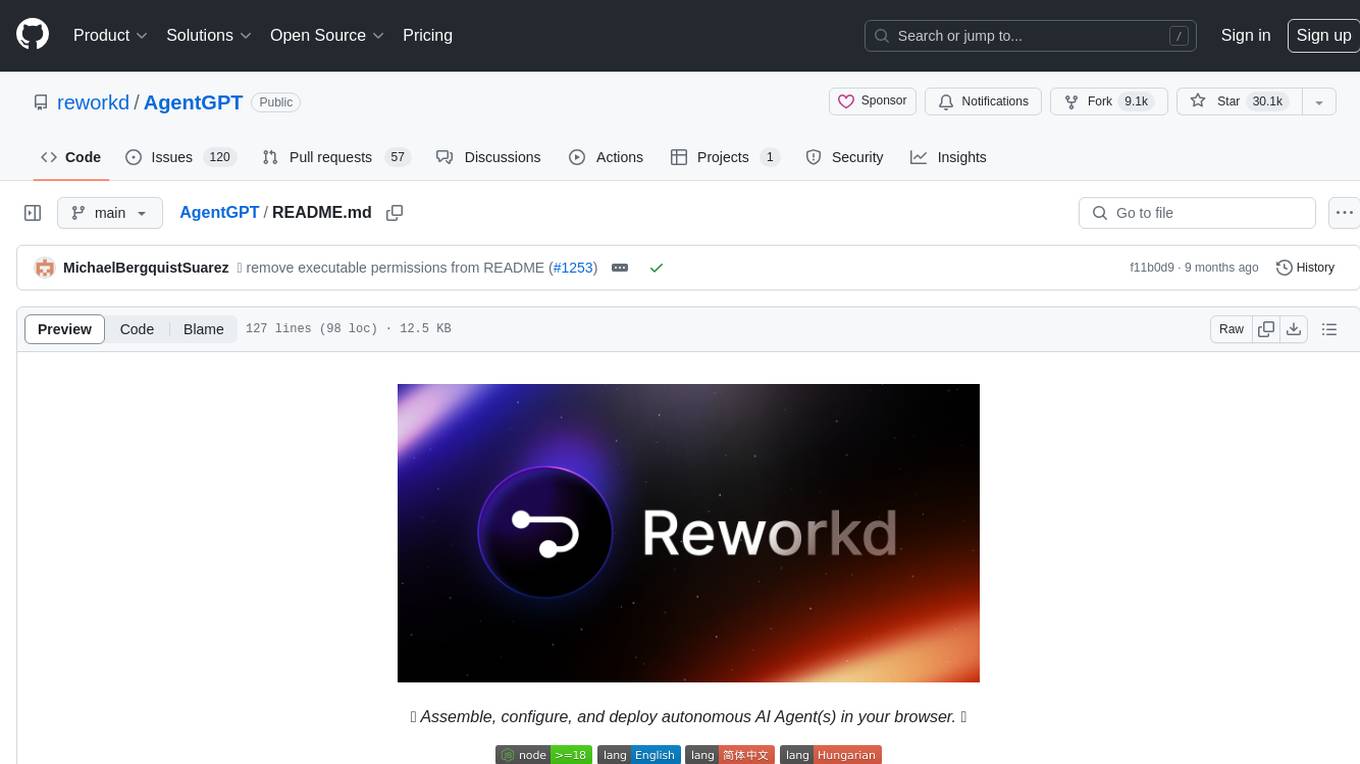
AgentGPT
AgentGPT is a platform that allows users to configure and deploy autonomous AI agents. Users can name their own custom AI and set it on any goal. The AI will think of tasks, execute them, and learn from the results to reach the goal. The platform provides a demo experience, automatic setup CLI, and a tech stack including Next.js, FastAPI, Prisma, TailwindCSS, Zod, and more. AgentGPT is designed to help users easily create and deploy AI agents for various tasks.
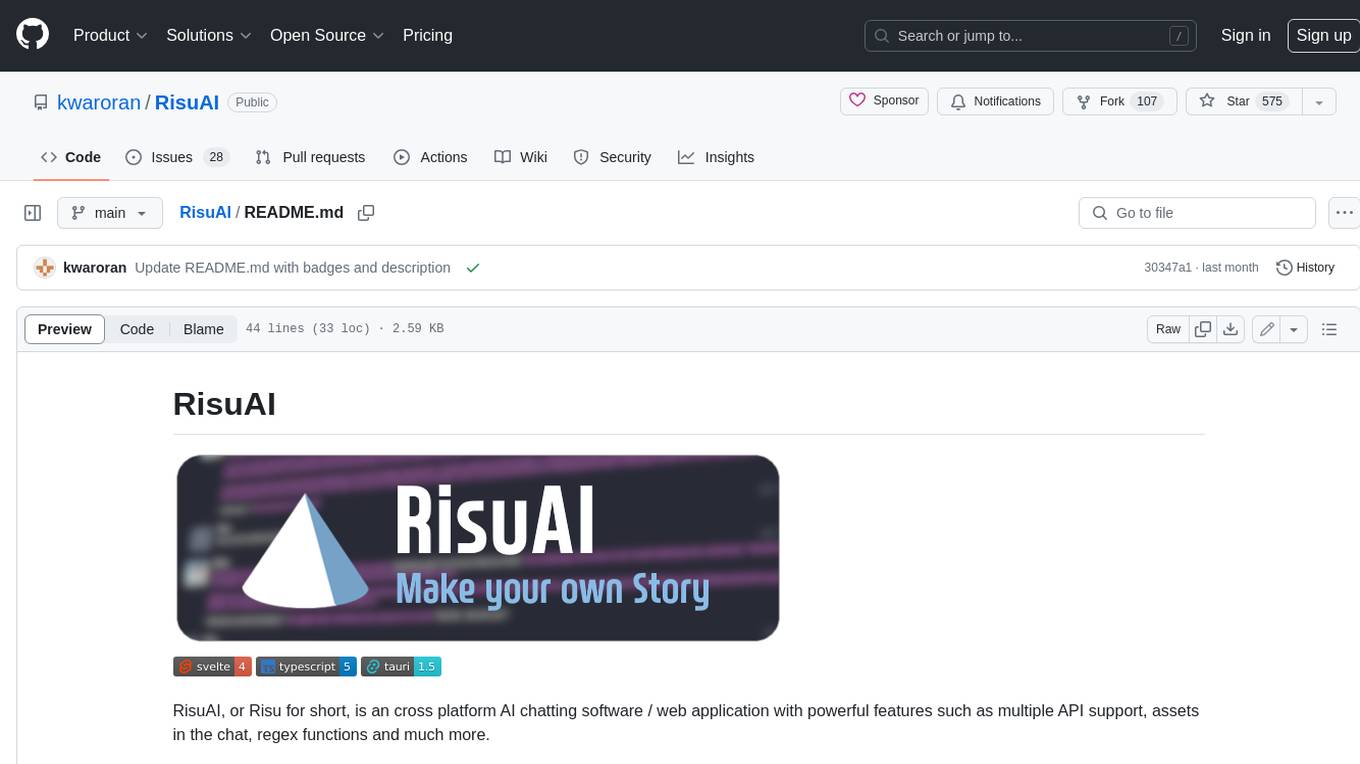
RisuAI
RisuAI, or Risu for short, is a cross-platform AI chatting software/web application with powerful features such as multiple API support, assets in the chat, regex functions, and much more.
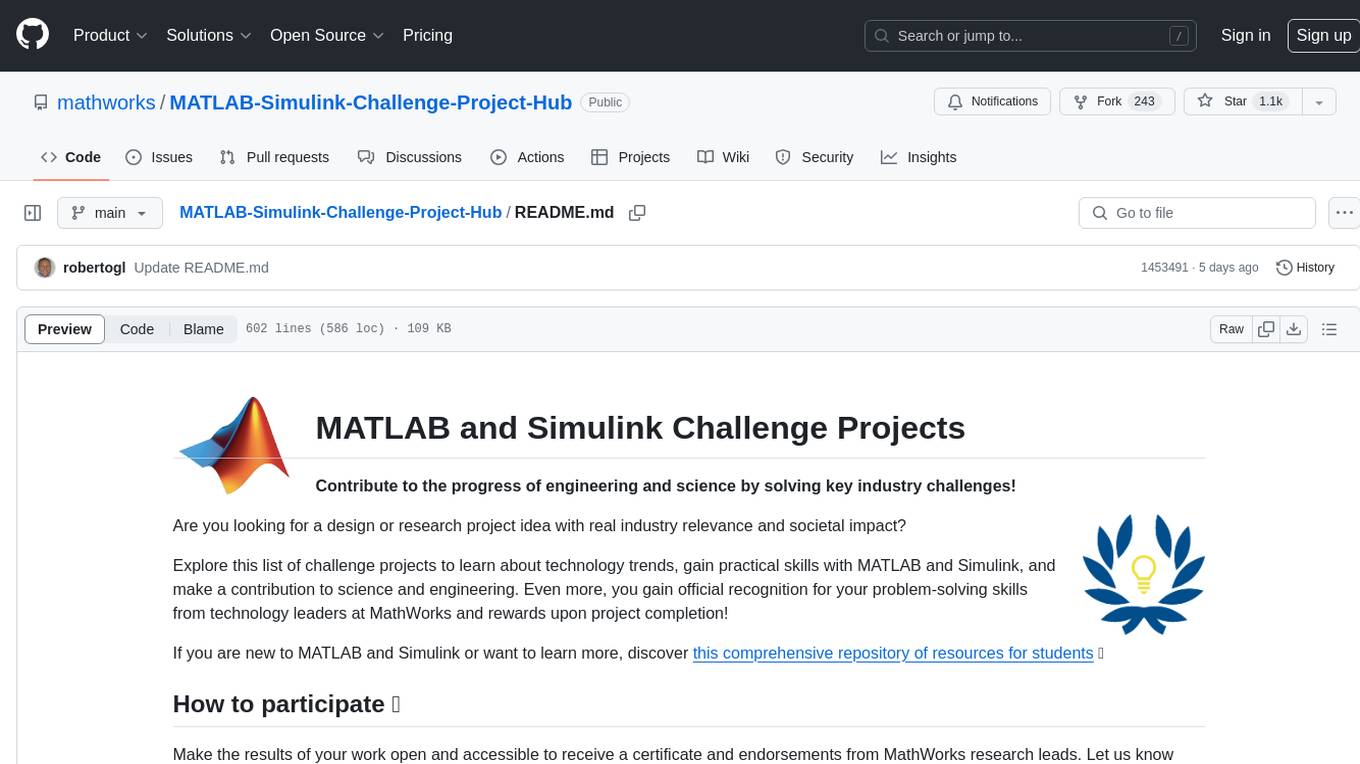
MATLAB-Simulink-Challenge-Project-Hub
MATLAB-Simulink-Challenge-Project-Hub is a repository aimed at contributing to the progress of engineering and science by providing challenge projects with real industry relevance and societal impact. The repository offers a wide range of projects covering various technology trends such as Artificial Intelligence, Autonomous Vehicles, Big Data, Computer Vision, and Sustainability. Participants can gain practical skills with MATLAB and Simulink while making a significant contribution to science and engineering. The projects are designed to enhance expertise in areas like Sustainability and Renewable Energy, Control, Modeling and Simulation, Machine Learning, and Robotics. By participating in these projects, individuals can receive official recognition for their problem-solving skills from technology leaders at MathWorks and earn rewards upon project completion.
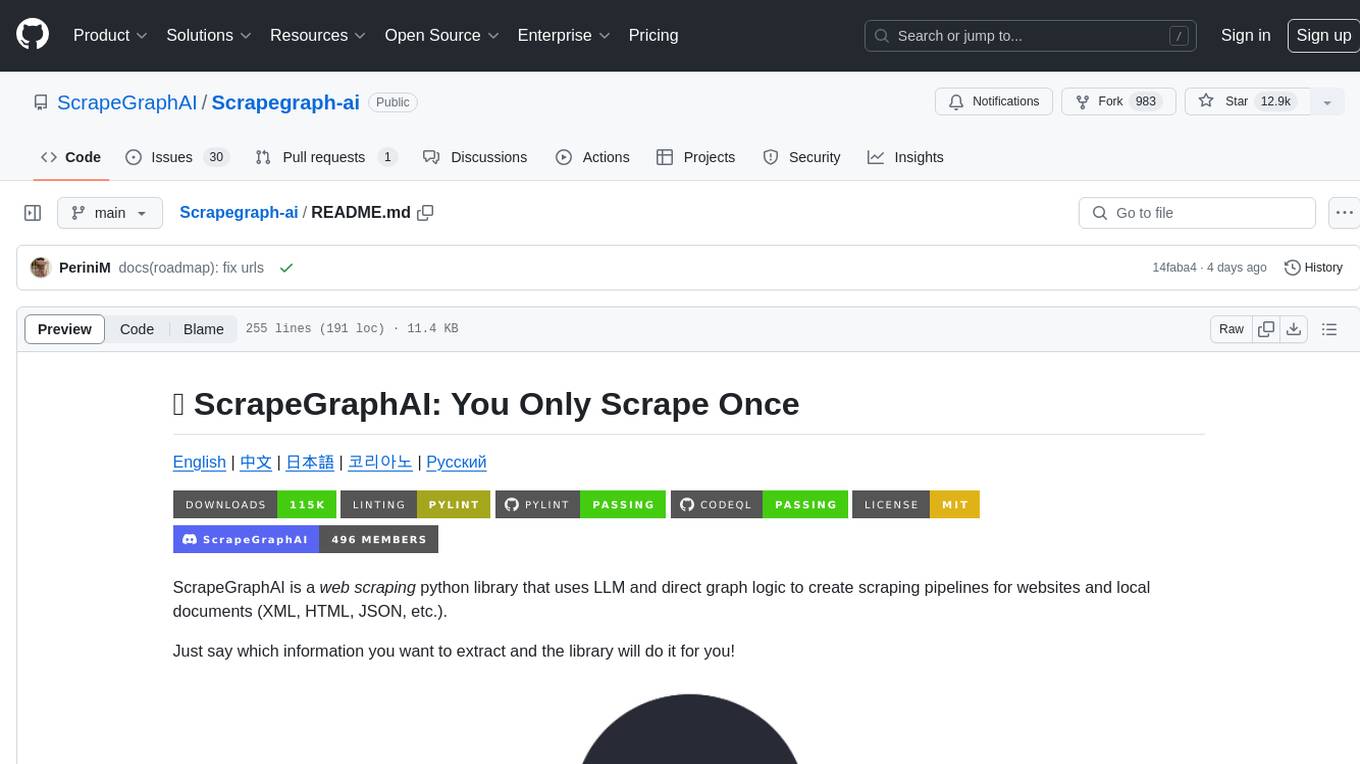
Scrapegraph-ai
ScrapeGraphAI is a web scraping Python library that utilizes LLM and direct graph logic to create scraping pipelines for websites and local documents. It offers various standard scraping pipelines like SmartScraperGraph, SearchGraph, SpeechGraph, and ScriptCreatorGraph. Users can extract information by specifying prompts and input sources. The library supports different LLM APIs such as OpenAI, Groq, Azure, and Gemini, as well as local models using Ollama. ScrapeGraphAI is designed for data exploration and research purposes, providing a versatile tool for extracting information from web pages and generating outputs like Python scripts, audio summaries, and search results.
For similar tasks
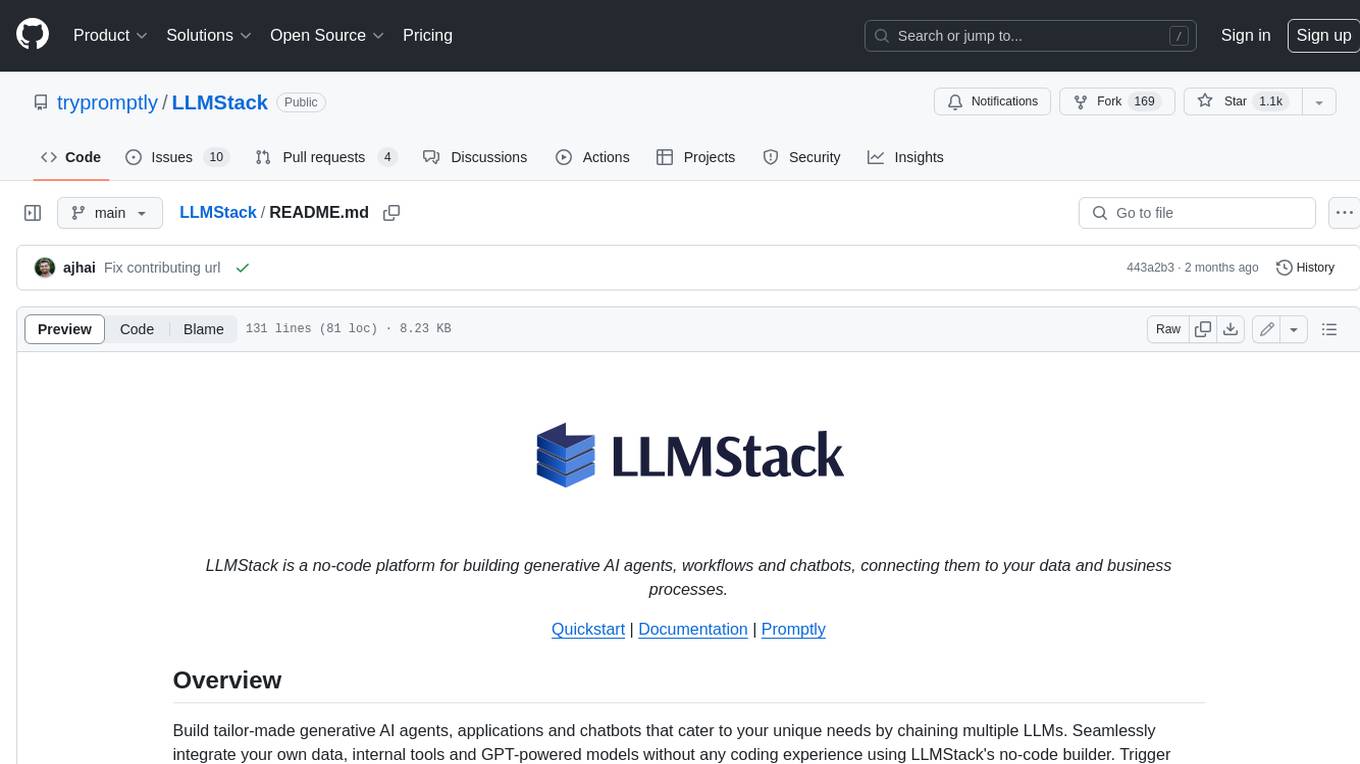
LLMStack
LLMStack is a no-code platform for building generative AI agents, workflows, and chatbots. It allows users to connect their own data, internal tools, and GPT-powered models without any coding experience. LLMStack can be deployed to the cloud or on-premise and can be accessed via HTTP API or triggered from Slack or Discord.
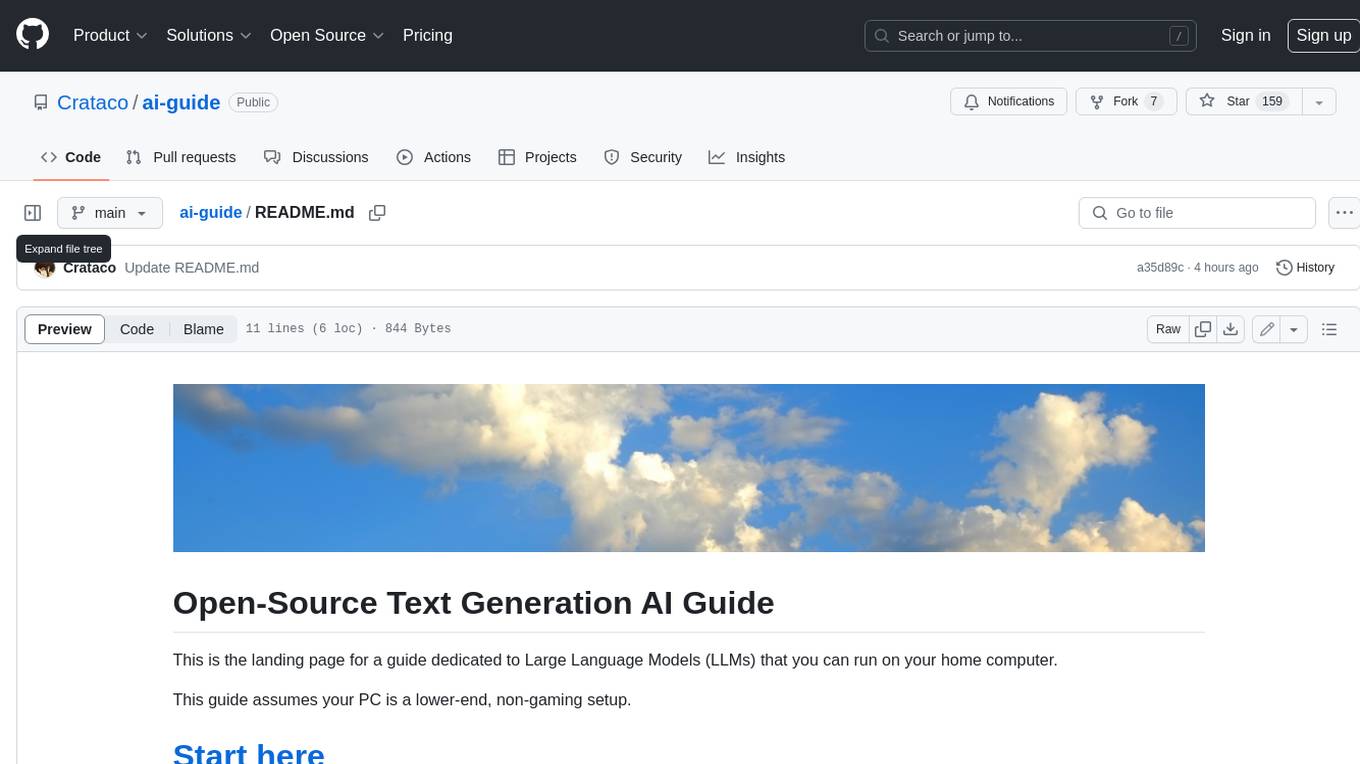
ai-guide
This guide is dedicated to Large Language Models (LLMs) that you can run on your home computer. It assumes your PC is a lower-end, non-gaming setup.
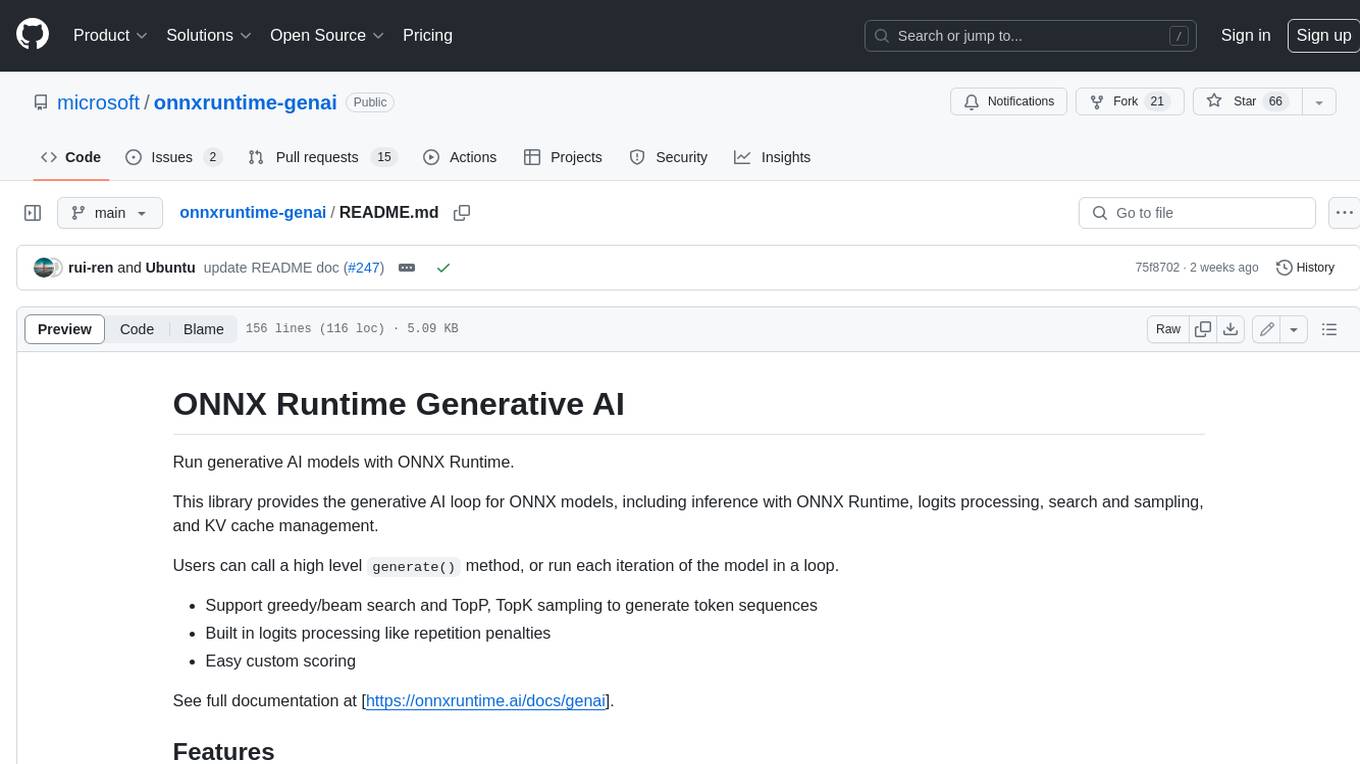
onnxruntime-genai
ONNX Runtime Generative AI is a library that provides the generative AI loop for ONNX models, including inference with ONNX Runtime, logits processing, search and sampling, and KV cache management. Users can call a high level `generate()` method, or run each iteration of the model in a loop. It supports greedy/beam search and TopP, TopK sampling to generate token sequences, has built in logits processing like repetition penalties, and allows for easy custom scoring.
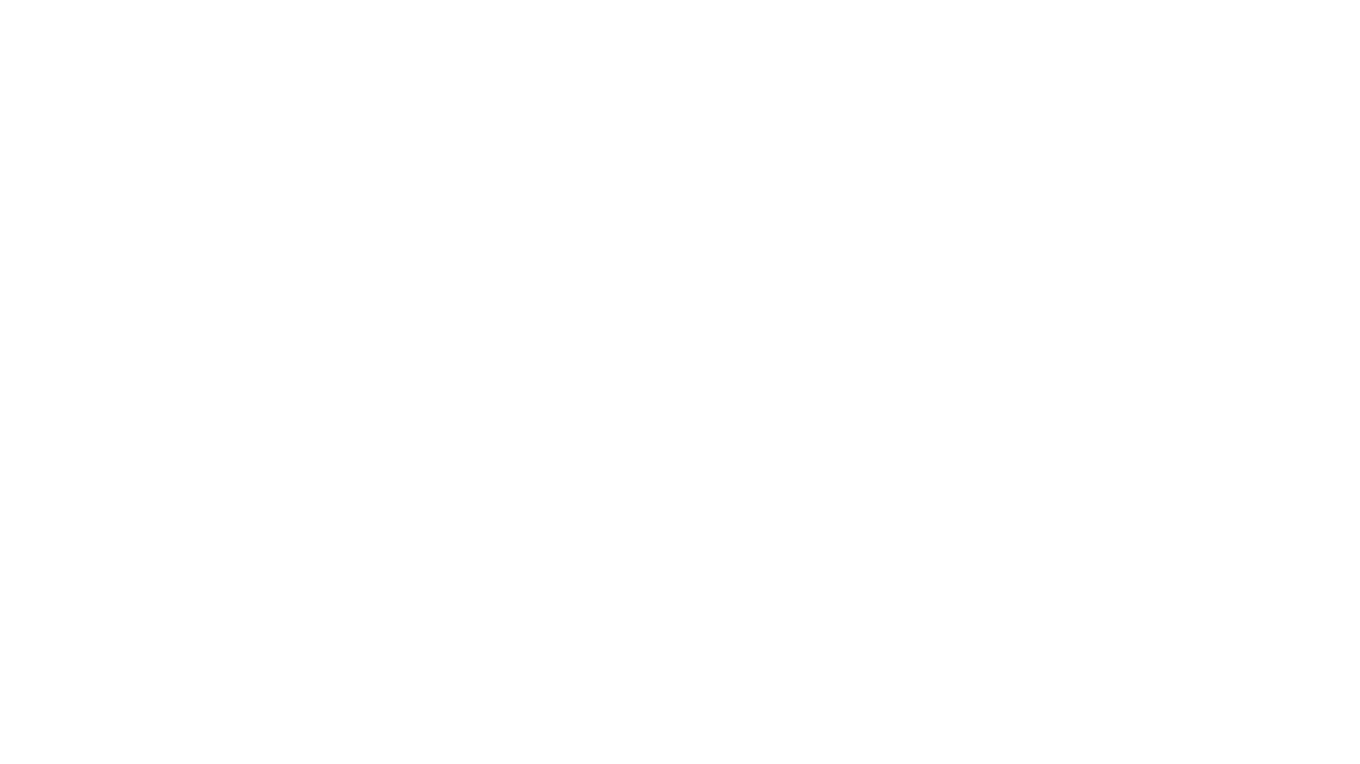
jupyter-ai
Jupyter AI connects generative AI with Jupyter notebooks. It provides a user-friendly and powerful way to explore generative AI models in notebooks and improve your productivity in JupyterLab and the Jupyter Notebook. Specifically, Jupyter AI offers: * An `%%ai` magic that turns the Jupyter notebook into a reproducible generative AI playground. This works anywhere the IPython kernel runs (JupyterLab, Jupyter Notebook, Google Colab, Kaggle, VSCode, etc.). * A native chat UI in JupyterLab that enables you to work with generative AI as a conversational assistant. * Support for a wide range of generative model providers, including AI21, Anthropic, AWS, Cohere, Gemini, Hugging Face, NVIDIA, and OpenAI. * Local model support through GPT4All, enabling use of generative AI models on consumer grade machines with ease and privacy.
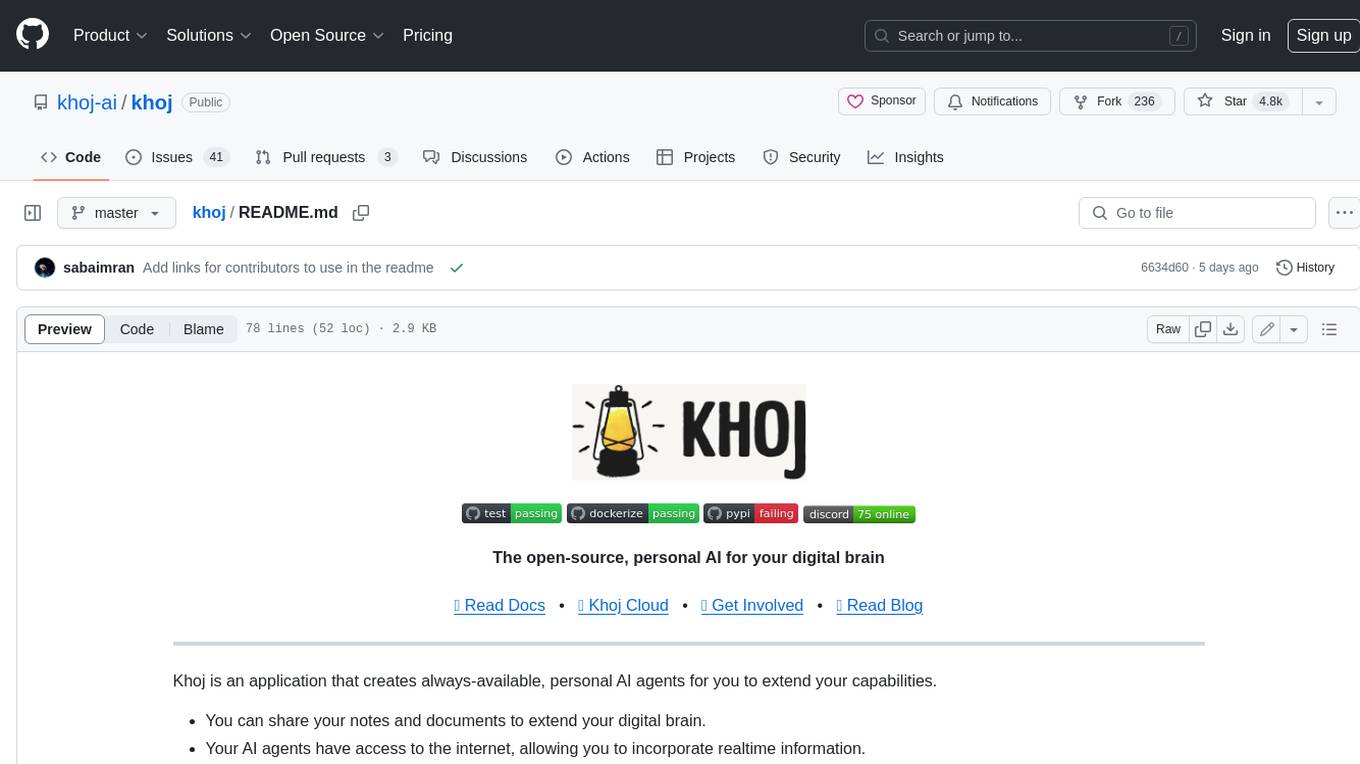
khoj
Khoj is an open-source, personal AI assistant that extends your capabilities by creating always-available AI agents. You can share your notes and documents to extend your digital brain, and your AI agents have access to the internet, allowing you to incorporate real-time information. Khoj is accessible on Desktop, Emacs, Obsidian, Web, and Whatsapp, and you can share PDF, markdown, org-mode, notion files, and GitHub repositories. You'll get fast, accurate semantic search on top of your docs, and your agents can create deeply personal images and understand your speech. Khoj is self-hostable and always will be.
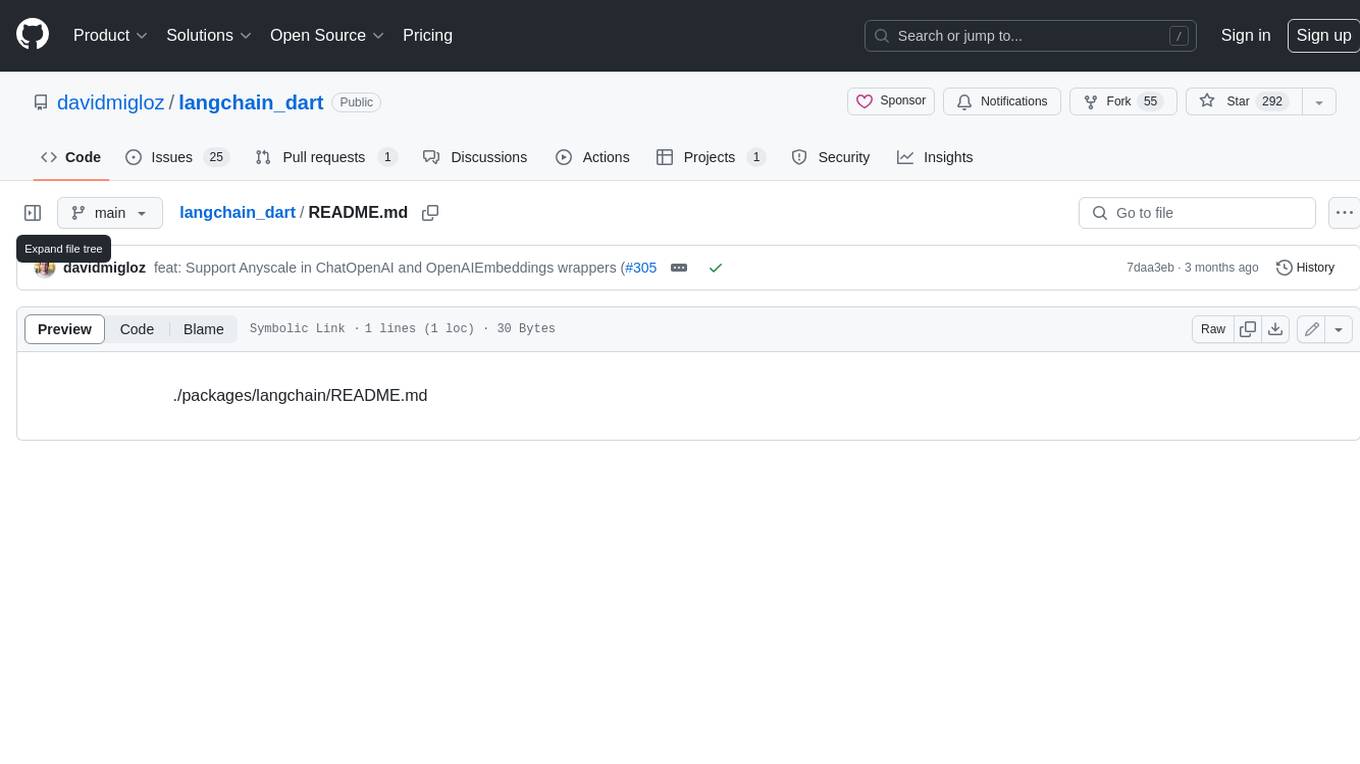
langchain_dart
LangChain.dart is a Dart port of the popular LangChain Python framework created by Harrison Chase. LangChain provides a set of ready-to-use components for working with language models and a standard interface for chaining them together to formulate more advanced use cases (e.g. chatbots, Q&A with RAG, agents, summarization, extraction, etc.). The components can be grouped into a few core modules: * **Model I/O:** LangChain offers a unified API for interacting with various LLM providers (e.g. OpenAI, Google, Mistral, Ollama, etc.), allowing developers to switch between them with ease. Additionally, it provides tools for managing model inputs (prompt templates and example selectors) and parsing the resulting model outputs (output parsers). * **Retrieval:** assists in loading user data (via document loaders), transforming it (with text splitters), extracting its meaning (using embedding models), storing (in vector stores) and retrieving it (through retrievers) so that it can be used to ground the model's responses (i.e. Retrieval-Augmented Generation or RAG). * **Agents:** "bots" that leverage LLMs to make informed decisions about which available tools (such as web search, calculators, database lookup, etc.) to use to accomplish the designated task. The different components can be composed together using the LangChain Expression Language (LCEL).
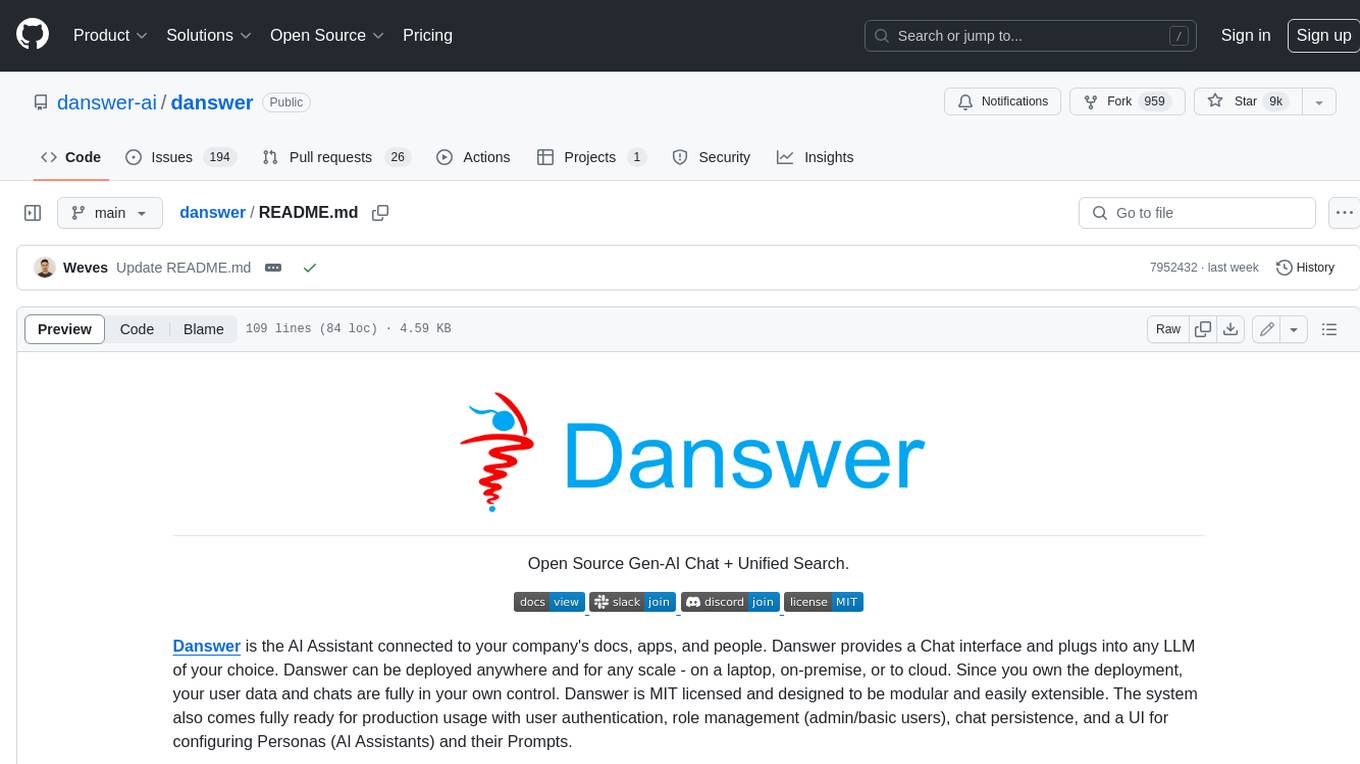
danswer
Danswer is an open-source Gen-AI Chat and Unified Search tool that connects to your company's docs, apps, and people. It provides a Chat interface and plugs into any LLM of your choice. Danswer can be deployed anywhere and for any scale - on a laptop, on-premise, or to cloud. Since you own the deployment, your user data and chats are fully in your own control. Danswer is MIT licensed and designed to be modular and easily extensible. The system also comes fully ready for production usage with user authentication, role management (admin/basic users), chat persistence, and a UI for configuring Personas (AI Assistants) and their Prompts. Danswer also serves as a Unified Search across all common workplace tools such as Slack, Google Drive, Confluence, etc. By combining LLMs and team specific knowledge, Danswer becomes a subject matter expert for the team. Imagine ChatGPT if it had access to your team's unique knowledge! It enables questions such as "A customer wants feature X, is this already supported?" or "Where's the pull request for feature Y?"
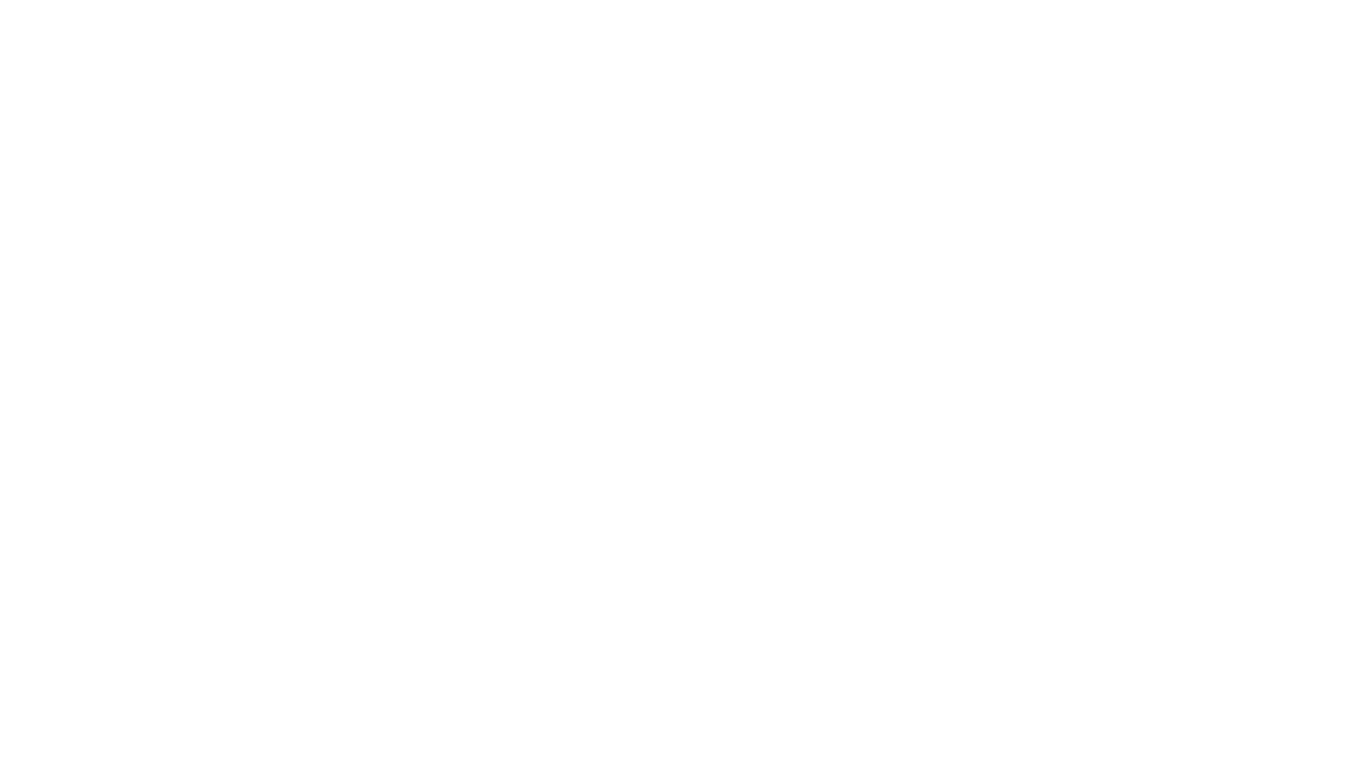
infinity
Infinity is an AI-native database designed for LLM applications, providing incredibly fast full-text and vector search capabilities. It supports a wide range of data types, including vectors, full-text, and structured data, and offers a fused search feature that combines multiple embeddings and full text. Infinity is easy to use, with an intuitive Python API and a single-binary architecture that simplifies deployment. It achieves high performance, with 0.1 milliseconds query latency on million-scale vector datasets and up to 15K QPS.
For similar jobs
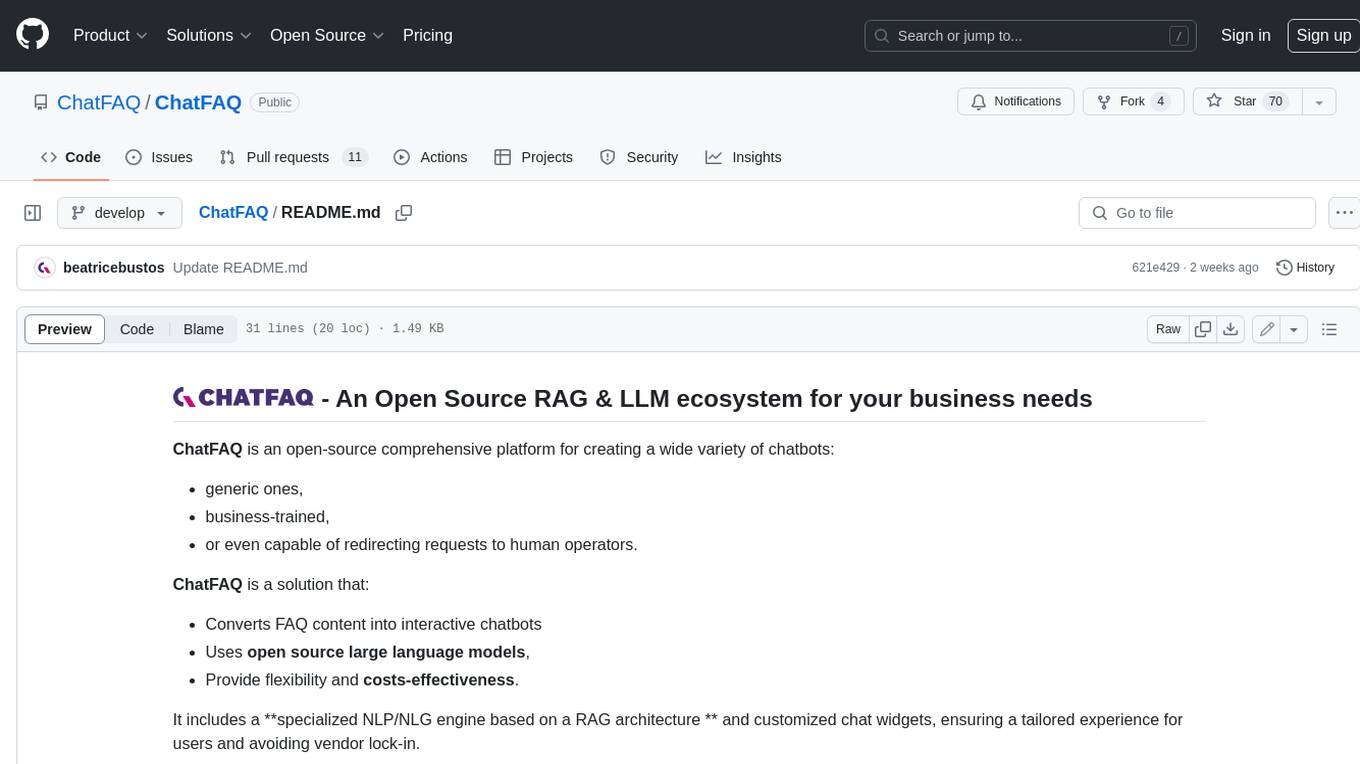
ChatFAQ
ChatFAQ is an open-source comprehensive platform for creating a wide variety of chatbots: generic ones, business-trained, or even capable of redirecting requests to human operators. It includes a specialized NLP/NLG engine based on a RAG architecture and customized chat widgets, ensuring a tailored experience for users and avoiding vendor lock-in.
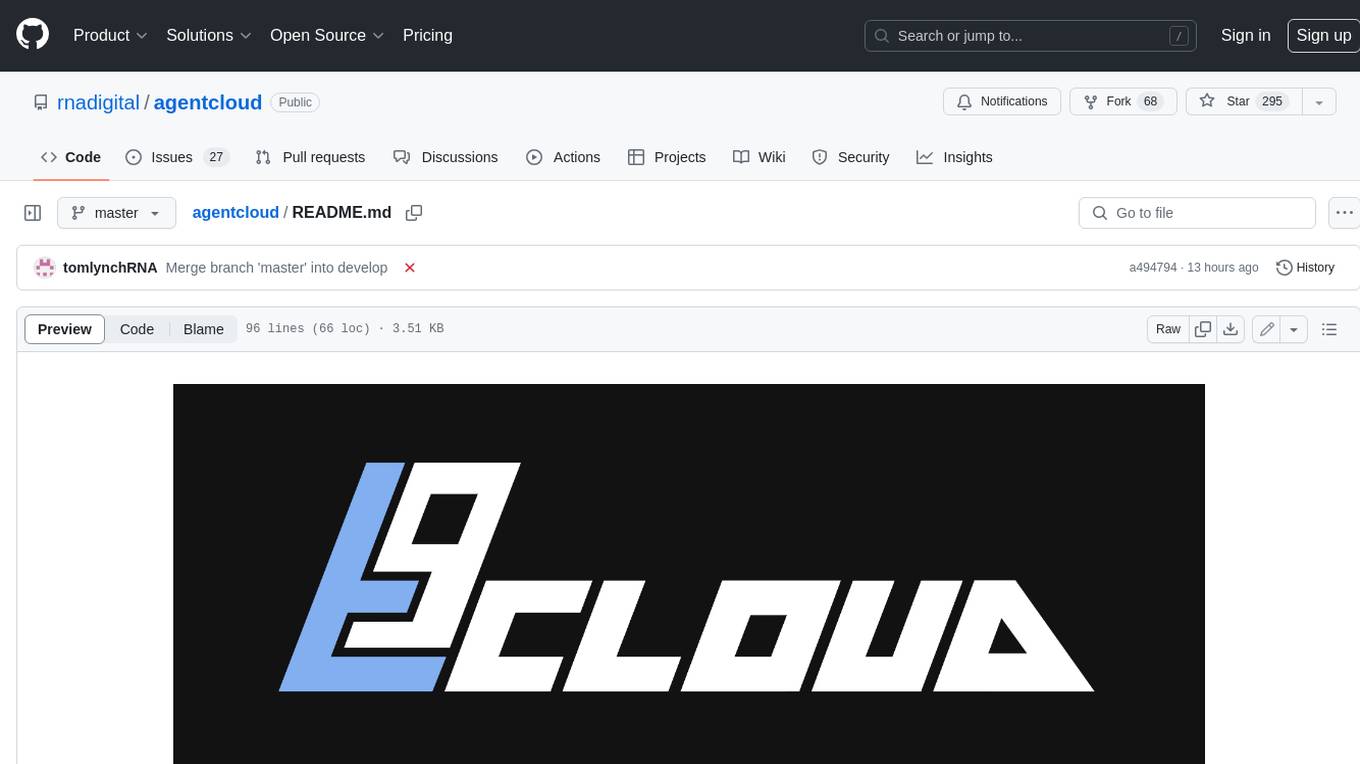
agentcloud
AgentCloud is an open-source platform that enables companies to build and deploy private LLM chat apps, empowering teams to securely interact with their data. It comprises three main components: Agent Backend, Webapp, and Vector Proxy. To run this project locally, clone the repository, install Docker, and start the services. The project is licensed under the GNU Affero General Public License, version 3 only. Contributions and feedback are welcome from the community.
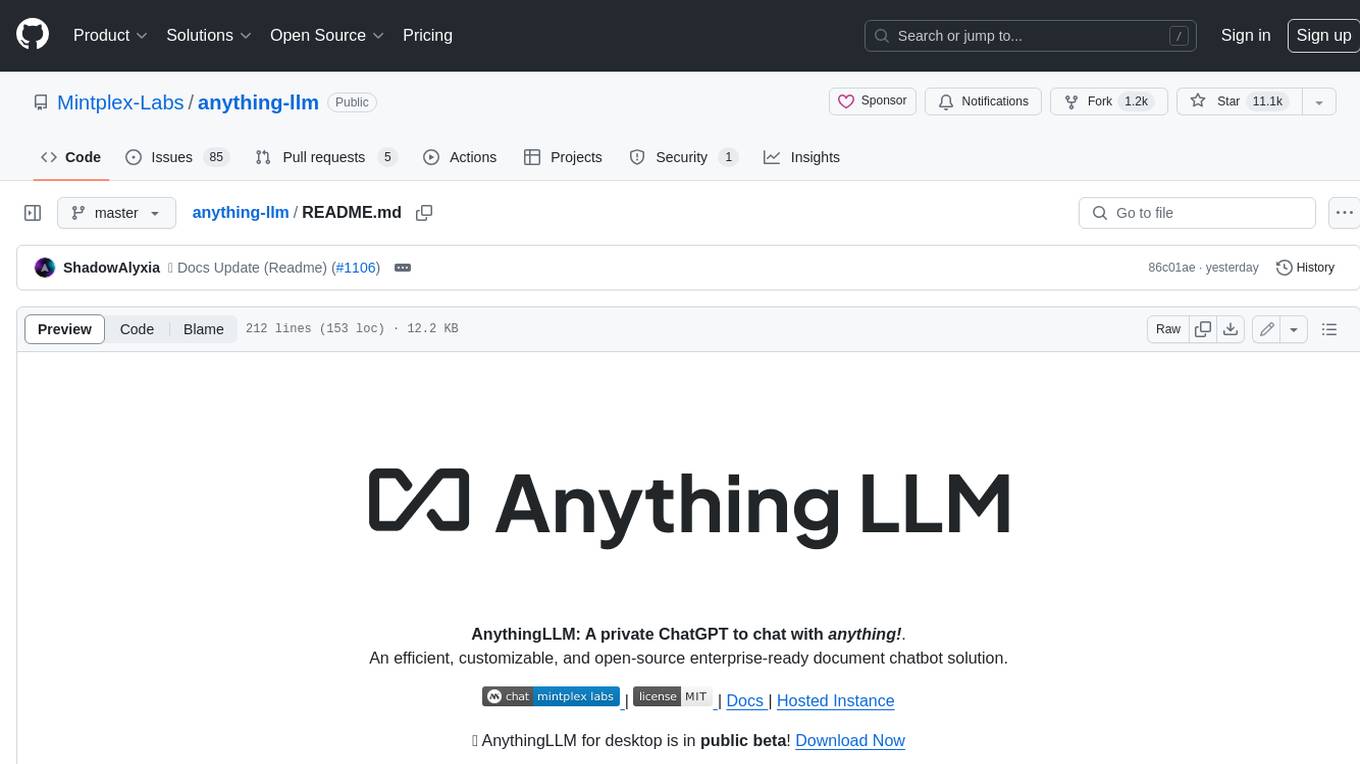
anything-llm
AnythingLLM is a full-stack application that enables you to turn any document, resource, or piece of content into context that any LLM can use as references during chatting. This application allows you to pick and choose which LLM or Vector Database you want to use as well as supporting multi-user management and permissions.
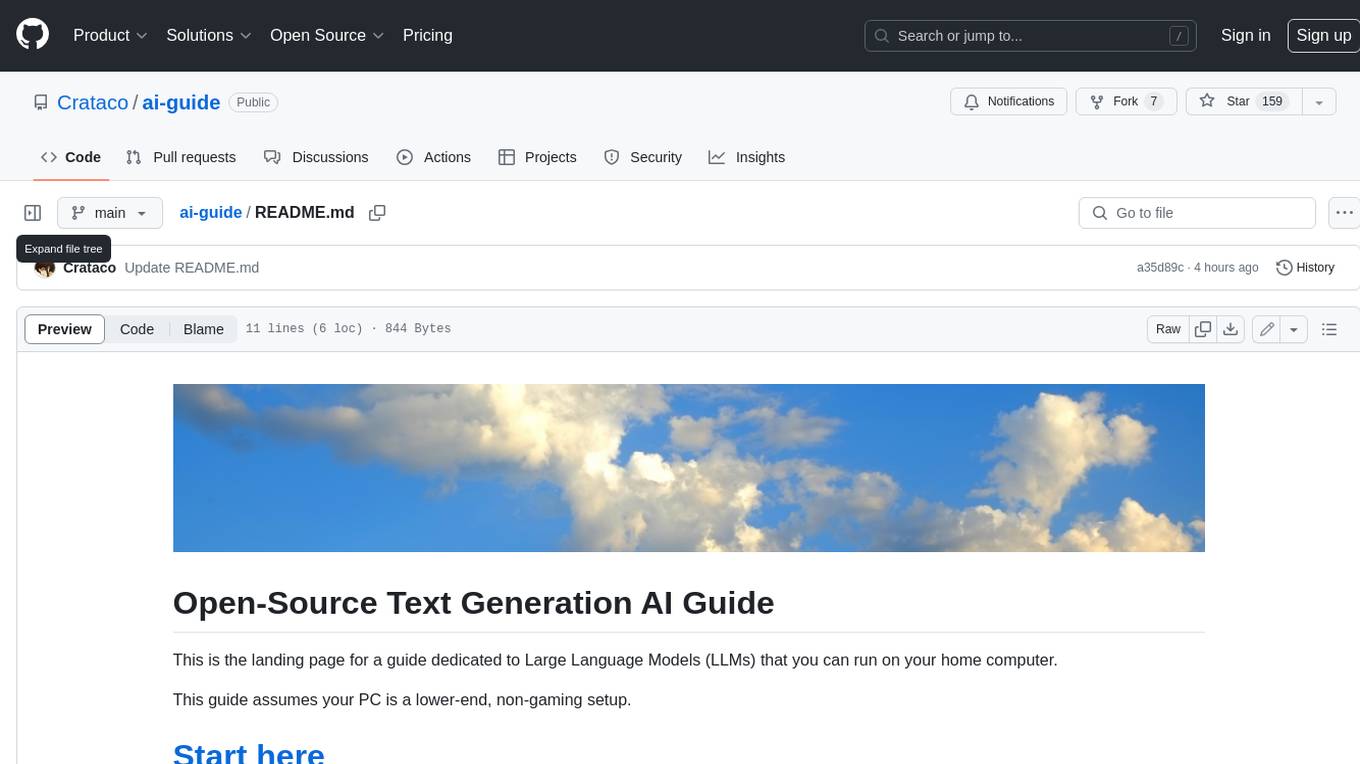
ai-guide
This guide is dedicated to Large Language Models (LLMs) that you can run on your home computer. It assumes your PC is a lower-end, non-gaming setup.
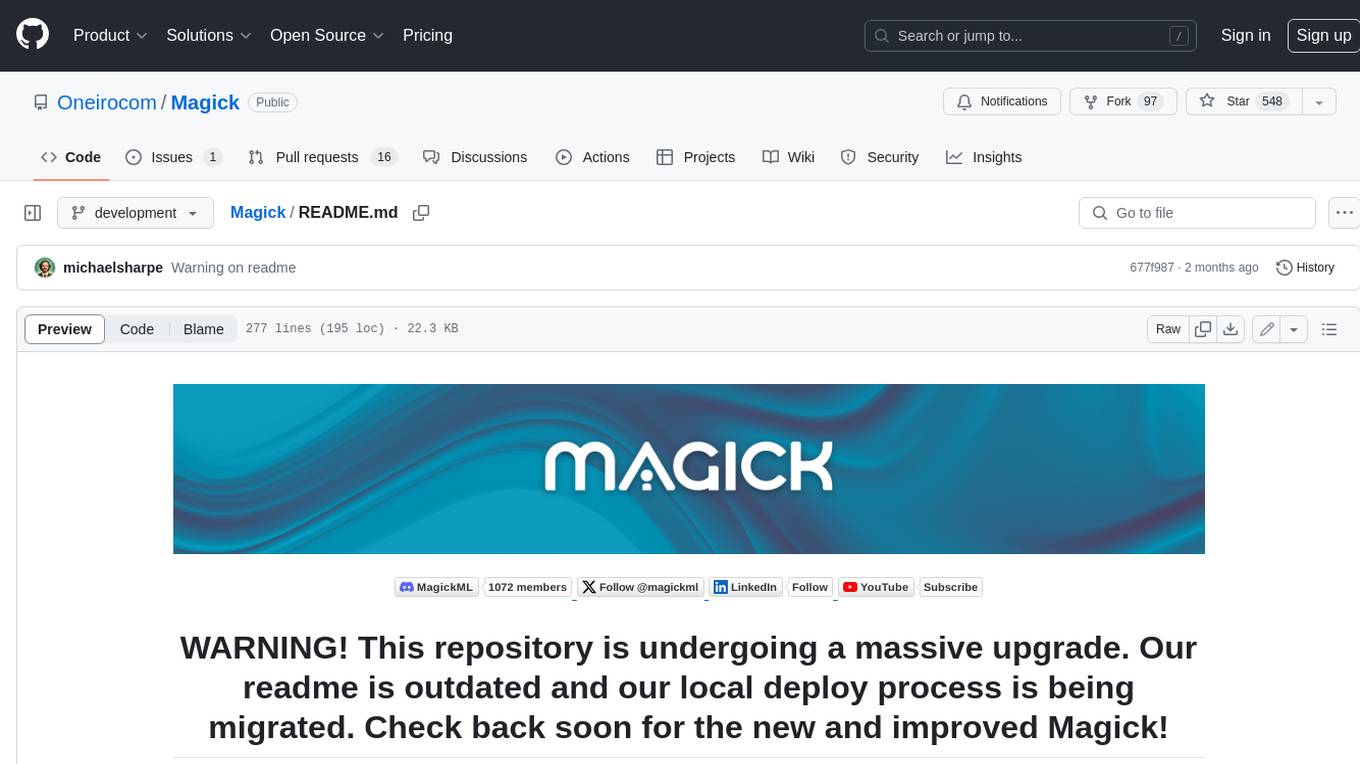
Magick
Magick is a groundbreaking visual AIDE (Artificial Intelligence Development Environment) for no-code data pipelines and multimodal agents. Magick can connect to other services and comes with nodes and templates well-suited for intelligent agents, chatbots, complex reasoning systems and realistic characters.
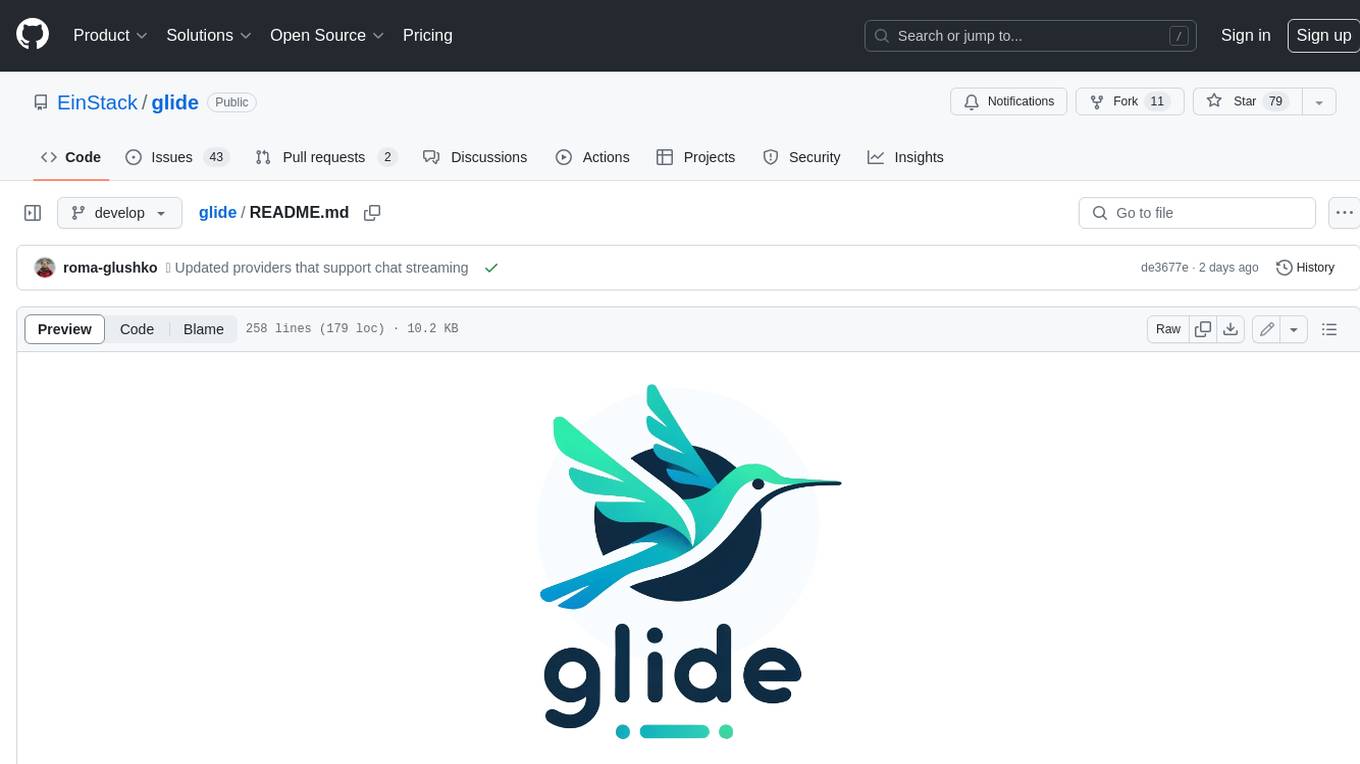
glide
Glide is a cloud-native LLM gateway that provides a unified REST API for accessing various large language models (LLMs) from different providers. It handles LLMOps tasks such as model failover, caching, key management, and more, making it easy to integrate LLMs into applications. Glide supports popular LLM providers like OpenAI, Anthropic, Azure OpenAI, AWS Bedrock (Titan), Cohere, Google Gemini, OctoML, and Ollama. It offers high availability, performance, and observability, and provides SDKs for Python and NodeJS to simplify integration.
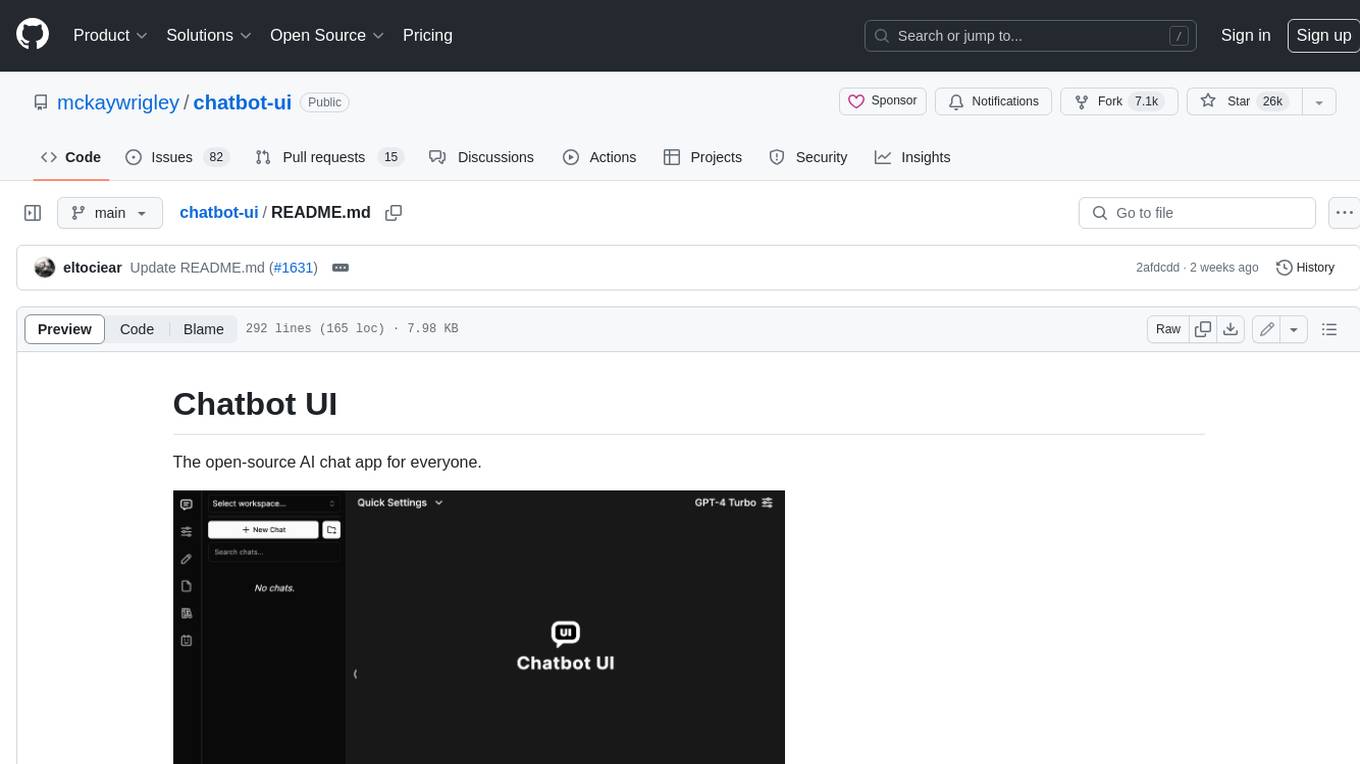
chatbot-ui
Chatbot UI is an open-source AI chat app that allows users to create and deploy their own AI chatbots. It is easy to use and can be customized to fit any need. Chatbot UI is perfect for businesses, developers, and anyone who wants to create a chatbot.
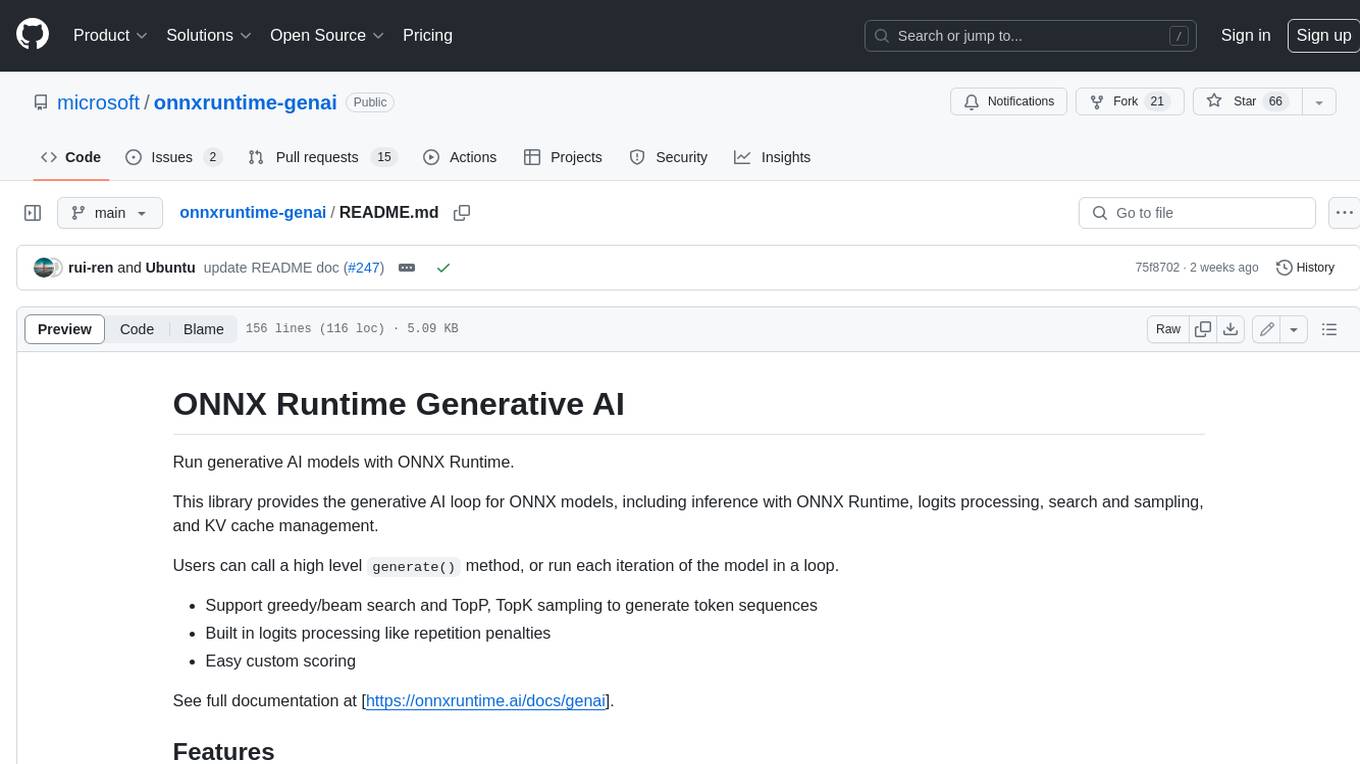
onnxruntime-genai
ONNX Runtime Generative AI is a library that provides the generative AI loop for ONNX models, including inference with ONNX Runtime, logits processing, search and sampling, and KV cache management. Users can call a high level `generate()` method, or run each iteration of the model in a loop. It supports greedy/beam search and TopP, TopK sampling to generate token sequences, has built in logits processing like repetition penalties, and allows for easy custom scoring.