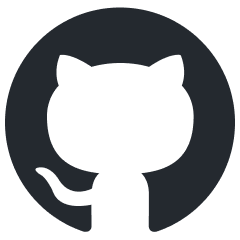
tracking-aircraft
None
Stars: 61
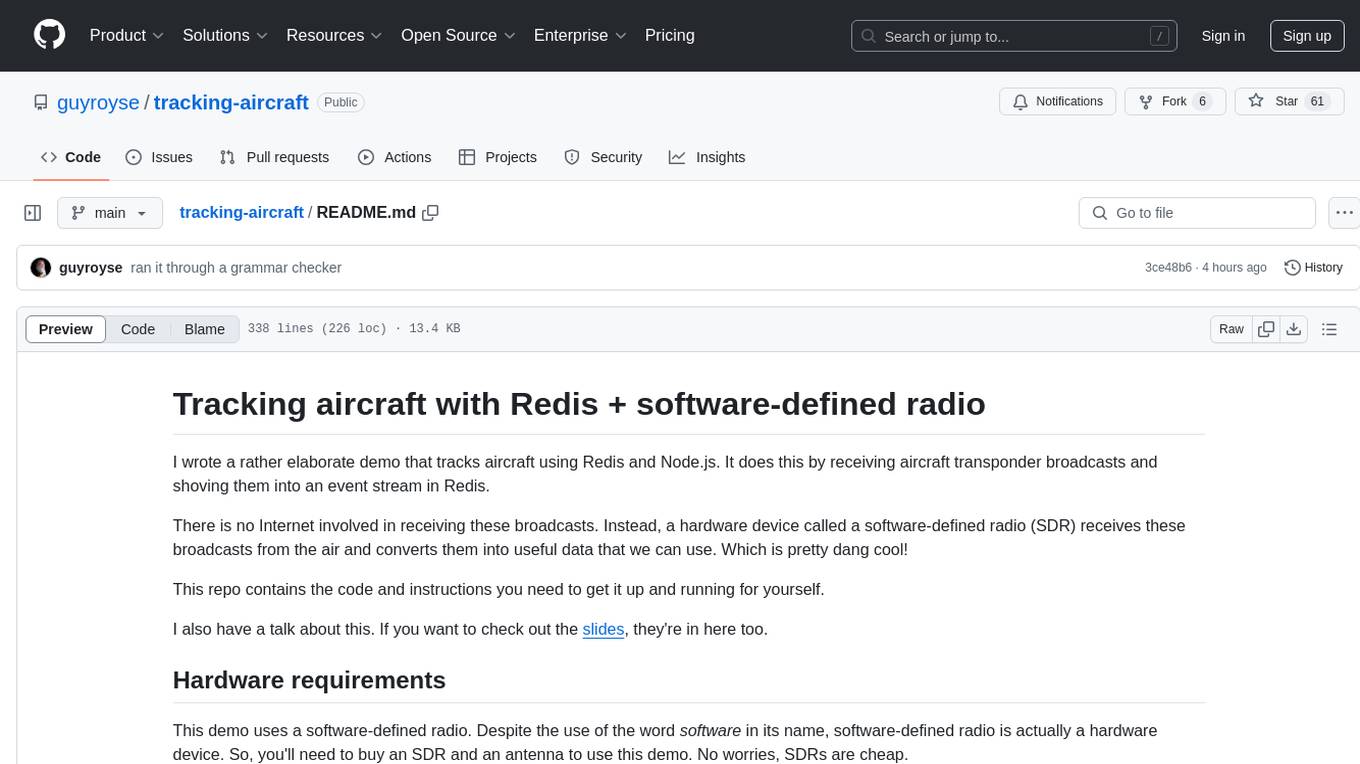
This repository provides a demo that tracks aircraft using Redis and Node.js by receiving aircraft transponder broadcasts through a software-defined radio (SDR) and storing them in Redis. The demo includes instructions for setting up the hardware and software components required for tracking aircraft. It consists of four main components: Radio Ingestor, Flight Server, Flight UI, and Redis. The Radio Ingestor captures transponder broadcasts and writes them to a Redis event stream, while the Flight Server consumes the event stream, enriches the data, and provides APIs to query aircraft status. The Flight UI presents flight data to users in map and detail views. Users can run the demo by setting up the hardware, installing SDR software, and running the components using Docker or Node.js.
README:
I wrote a rather elaborate demo that tracks aircraft using Redis and Node.js. It does this by receiving aircraft transponder broadcasts and shoving them into an event stream in Redis.
There is no Internet involved in receiving these broadcasts. Instead, a hardware device called a software-defined radio (SDR) receives these broadcasts from the air and converts them into useful data that we can use. Which is pretty dang cool!
This repo contains the code and instructions you need to get it up and running for yourself.
I also have a talk about this. If you want to check out the slides, they're in here too.
This demo uses a software-defined radio. Despite the use of the word software in its name, software-defined radio is actually a hardware device. So, you'll need to buy an SDR and an antenna to use this demo. No worries, SDRs are cheap.
Here's the load-out I like to use. It includes the SDR dongle and an antenna specifically designed for picking up aircraft transponder broadcasts.
- RTL SDR: https://www.amazon.com/RTL-SDR-Blog-RTL2832U-Software-Defined/dp/B0CD745394/
- ADS-B Antenna: https://www.amazon.com/1090MHz-Antenna-Fiberglass-SMA-Male-Extension/dp/B09BQ4H26L/
If you want something cheaper and more general-purpose, and this is probably a better choice, some bundles include the same SDR and some more flexible antennas. This is a good way to go if you want to do other things with your SDR. Which will happen.
You can also make your own antenna if you are ambitious. I'll leave that up to your own googling.
If you choose to make an antenna or to use the kit above, you'll want both legs of the antenna extended/cut to a length of ~69mm. Arrange them so that they are in a line, 180 degrees apart. This, in radio terms, is called a dipole.
Regardless of which antenna option you go with, you'll want to mount it vertically as this matches the way they are mounted on aircraft. Here's a picture of a homemade antenna (not mine) mounted vertically in an attic (not mine):
Thanks for the picture, random Internet stranger!
Installing SDR software can be a bit fiddly. You've been warned. That said, it has gotten easier over the years. You'll need two pieces of software to make this code work—besides, like, Node.js and stuff: the RTL SDR drivers, and dump1090.
These drivers allow your computer to talk to the SDR. And they provide lots of interesting command-line tools to boot. You'll need this to do, well, anything with your SDR.
sudo apt update
sudo apt install rtl-sdr
brew install rtl-sdr
You can download the RTL SDR drivers and tools from https://ftp.osmocom.org/binaries/windows/rtl-sdr/. They are built weekly so get the latest one for your platform—probably the 64-bit one. The download is simply a .ZIP file full of .EXE and .DLL files. Put these files in a folder somewhere on your system and add that folder to your PATH to get it working.
Details on the drivers themselves can be found at https://osmocom.org/projects/rtl-sdr/wiki/Rtl-sdr.
Regardless of your platform, testing is the same. Plug in your SDR and run the following command:
rtl_test
You should get back something like:
Found 1 device(s):
0: Realtek, RTL2838UHIDIR, SN: 00000001
Using device 0: Generic RTL2832U OEM
Detached kernel driver
Found Rafael Micro R820T tuner
Supported gain values (29): 0.0 0.9 1.4 2.7 3.7 7.7 8.7 12.5 14.4 15.7 16.6 19.7 20.7 22.9 25.4 28.0 29.7 32.8 33.8 36.4 37.2 38.6 40.2 42.1 43.4 43.9 44.5 48.0 49.6
[R82XX] PLL not locked!
Sampling at 2048000 S/s.
Info: This tool will continuously read from the device, and report if
samples get lost. If you observe no further output, everything is fine.
Reading samples in async mode...
Allocating 15 zero-copy buffers
lost at least 12 bytes
Hooray! Your drivers are working. Press Ctrl-C to stop and go to the next step.
Dump1090 is software that uses an RTL SDR to listen to aircraft transponder broadcasts, present them to the user, and make them available over a socket connection. The code in this repo uses that socket connection to read the broadcasts.
sudo apt install dump1090-mutability
You might be asked if you want to install this so that it always runs. I've only ever said no.
brew install dump1090-mutability
Windows has a lot of ports of dump1090. I like to use this one: https://github.com/MalcolmRobb/dump1090.
Just download the .ZIP file in the root of the repository—yes, it's really 10 years old—and unzip it into a folder of your choice. From there, you can just run it from that folder or, if you prefer, add it to your path.
You should now be able to run dump1090 using the following command:
dump1090 --net --interactive
If you installed to Linux, you'll need to run this instead:
dump1090-mutability --net --interactive
If you have your antenna attached, aircraft should start showing up. Here's some that I found today while writing this README:
Hex Mode Sqwk Flight Alt Spd Hdg Lat Long RSSI Msgs Ti/
-------------------------------------------------------------------------------
AD4C2A S -39.6 3 1
A097CE S 40000 -32.5 11 0
06A1DA S 47000 -33.4 7 0
A71ABB S 7143 43000 476 272 -33.1 18 1
A280FE S 40000 443 299 40.346 -83.589 -35.5 5 0
A1FF90 S 4000 230 294 39.920 -83.152 -28.2 13 0
A59398 S 1512 AAL464 34975 472 082 40.013 -82.266 -27.4 55 0
A05544 S 43000 474 107 40.163 -82.640 -29.5 21 2
A66312 S 1200 OSU51 1700 90 270 40.091 -83.082 -25.2 49 0
A537ED S 6646 RPA4723 31000 439 103 39.867 -82.767 -28.0 100 0
A05AAC S 6616 UCA4824 31000 431 102 39.984 -82.297 -23.8 39 0
A16B47 S 41000 491 045 40.486 -83.588 -31.7 39 1
A69939 S 1546 EJA524 5925 240 275 39.909 -82.957 -25.0 64 0
AC0B74 S SWA4635 36000 455 267 40.523 -83.089 -28.6 94 0
A0B990 S 6036 40000 423 322 39.488 -83.482 -26.7 56 0
C07C7A S 7276 37000 467 053 40.124 -82.966 -19.3 56 0
Now that we have the fiddly bits working, we can get the demo running. The demo itself is made up of four components: the Radio Ingestor, the Flight Server, the Flight UI, and Redis.
graph LR
subgraph "Atlanta"
ANT1{Antenna} --> SDR1((RTL SDR)) --> DMP1[dump1090] --> ING1("Radio Ingestor")
end
subgraph "Columbus"
ANT2{Antenna} --> SDR2((RTL SDR)) --> DMP2[dump1090] --> ING2("Radio Ingestor")
end
subgraph "Denver"
ANT3{Antenna} --> SDR3((RTL SDR)) --> DMP3[dump1090] --> ING3("Radio Ingestor")
end
ING1 --> RED[Redis]
ING2 --> RED
ING3 --> RED
RED <--> SRV("Flight Server")
subgraph "Consumer"
SRV <--> WEB("Flight UI")
end
The purpose of the Radio Ingestor is to take transponder broadcasts and write them to a Redis event stream. It is designed so that multiple instances can run at the same time feeding aircraft spots into Redis from multiple, geographically dispersed locations.
The Flight Server consumes the event stream, enriches it, and saves current flight statuses to Redis. It also publishes the enriched data as a WebSocket and provides simple HTTP APIs to query aircraft status and stats.
The Flight UI presents flight data to the end user providing both map and detail views. It is designed to work alongside the Flight Server and is useless without it.
In a dedicated window, launch dump1090 with one of the following commands:
dump1090 --net --interactive # for Mac or Windows
dump1090-mutability --net --interactive # for Linux
The --net
option tells dump1090 to publish transponder broadcasts on port 30003. The --interactive
option just makes it prettier.
Now, from the root of this repo run:
docker compose up --build
This will download Redis, build all the components, and start them up with defaults that will work. No fuss. No muss.
Once it's started, point your browser at http://localhost:8000 and watch the aircraft move about.
Redis is where we're storing our aircraft spots. If you're not going with the quickstart, you'll need Redis somewhere. You can either install it locally, use Docker, or use Redis Cloud.
-
To install Redis locally, follow the instructions at https://redis.io/docs/latest/operate/oss_and_stack/install/install-stack/.
-
To use Docker, run the following command:
docker run -d --name redis-stack-server -p 6379:6379 redis/redis-stack-server:latest
- To use Redis Cloud, sign up for a free account at https://cloud.redis.io/.
You might also want to snag Redis Insight so you can see what Redis is doing. You can find that on the App Store, the Microsoft Store, or directly from Redis.
You'll need an existing Redis instance to do this. This might be local, but will probably be a Redis Cloud instance.
Before you run the Radio Ingestor, it must be configured. Details are in the the sample.env
file in the radio-ingestor
folder. However, the tl;dr is:
cd radio-ingestor
cp sample.env .env
Then edit the Redis options in the .env
file to point to your Redis instance.
To run the Radio Ingestor you can just use Docker:
cd radio-ingestor
docker compose up --build
If you'd rather run it using Node.js directly, then make sure you have Node.js installed and run the following commands:
cd radio-ingestor
npm install
npm run build
npm start
If you'd like to run it in dev mode instead you can skip the build:
cd radio-ingestor
npm install
npm run dev
You should be able to see an event stream in Redis—using Redis Insight, of course—populating with aircraft transponder events.
You'll need an existing Redis instance to do this. This could be a local instance of Redis, but will probably be a shared instance like Redis Cloud. This instance should be fed by one or more instances of a Radio Ingestor. Technically, this'll work even if the instance isn't being fed, but it won't be very interesting. Nothing in. Nothing out.
Before you run the Flight Server, it must be configured. Details are in the sample.env
file in the flight-server
folder. However, the tl;dr is:
cd flight-server
cp sample.env .env
Then edit the Redis options in the .env
file to point to your Redis instance.
To run the Flight Server you can just use Docker:
cd flight-server
docker compose up --build
If you'd rather run it using Node.js directly, then make sure you have Node.js installed and run the following commands:
cd flight-server
npm install
npm run build
npm start
If you'd like to run it in dev mode instead you can skip the build:
cd flight-server
npm install
npm run dev
If these instructions seem familiar, that's because I copied them directly from the Radio Ingestor instructions. It's the same process.
Regardless, if you look in Redis Insight, you should see a lot more keys including JSON documents for each of the aircraft spotted; T-Digests gathering stats about altitude, velocity, and climb; a HyperLogLog counting unique aircraft; and a humble little string counting the number of messages received.
The Flight UI does not need to be configured. It's all ready to go. However, it is hard-coded to look for the Flight Server on localhost:8080
and to expose itself on port 8000
when run in developer or preview mode. Once you compile it, you can server up the files on any port you'd like.
At some point, I'd like to make the Flight Server host and port configurable. But this is what we have for now.
To run the Flight Server you can just use Docker. Docker will compile the site and then host it internally on an NGINX instance:
cd flight-ui
docker compose up --build
If you'd rather run it using Node.js and vite, then make sure you have Node.js installed and run the following commands:
cd flight-ui
npm install
npm run build
npm run preview
If you'd like to run it in dev mode instead you can skip the build:
cd flight-ui
npm install
npm run dev
In any of these cases, point your browser at http://localhost:8000 and you should see an aircraft map.
That's pretty much it. If you see a bug, a typo, or some small improvements, feel free to send a PR. If you see something big or would like to make major improvements, reach out and let's discuss it.
I hope you find this fun and instructive. Happy spotting!
For Tasks:
Click tags to check more tools for each tasksFor Jobs:
Alternative AI tools for tracking-aircraft
Similar Open Source Tools
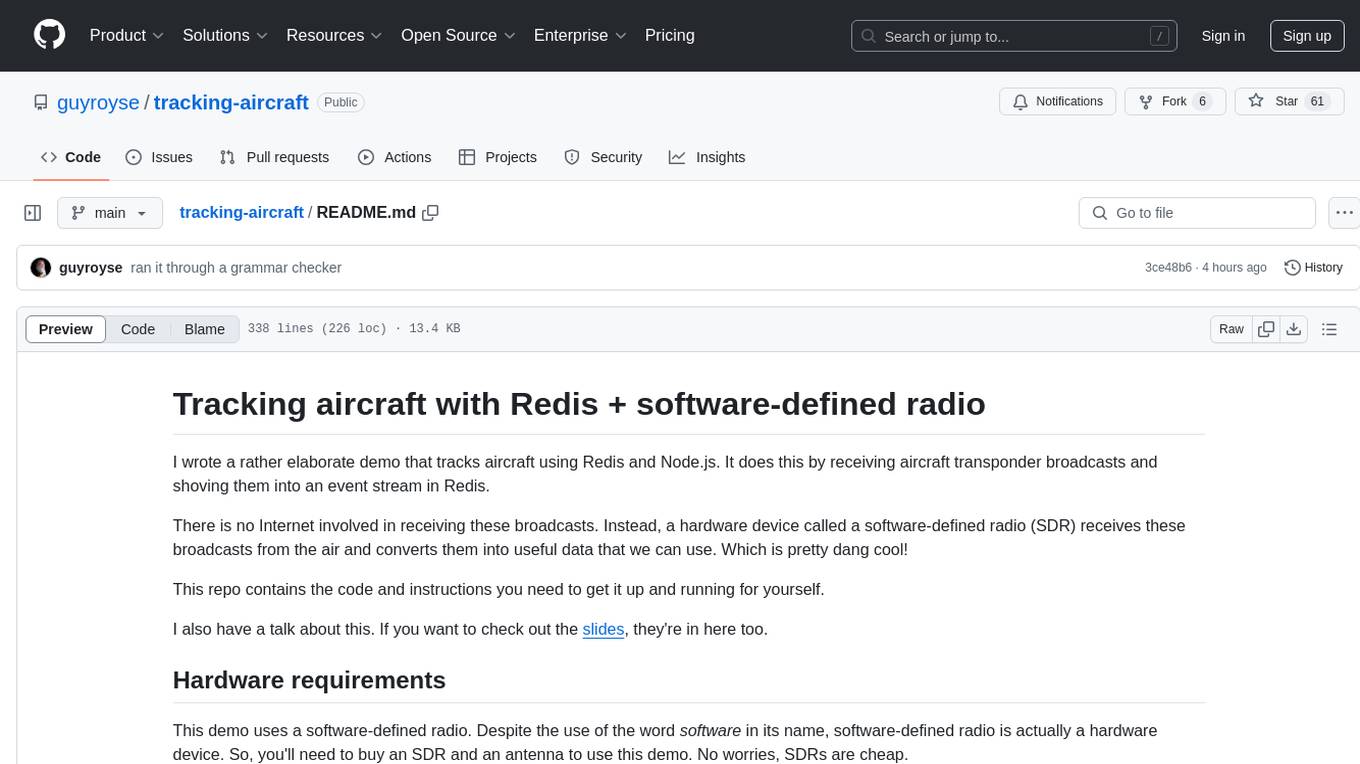
tracking-aircraft
This repository provides a demo that tracks aircraft using Redis and Node.js by receiving aircraft transponder broadcasts through a software-defined radio (SDR) and storing them in Redis. The demo includes instructions for setting up the hardware and software components required for tracking aircraft. It consists of four main components: Radio Ingestor, Flight Server, Flight UI, and Redis. The Radio Ingestor captures transponder broadcasts and writes them to a Redis event stream, while the Flight Server consumes the event stream, enriches the data, and provides APIs to query aircraft status. The Flight UI presents flight data to users in map and detail views. Users can run the demo by setting up the hardware, installing SDR software, and running the components using Docker or Node.js.
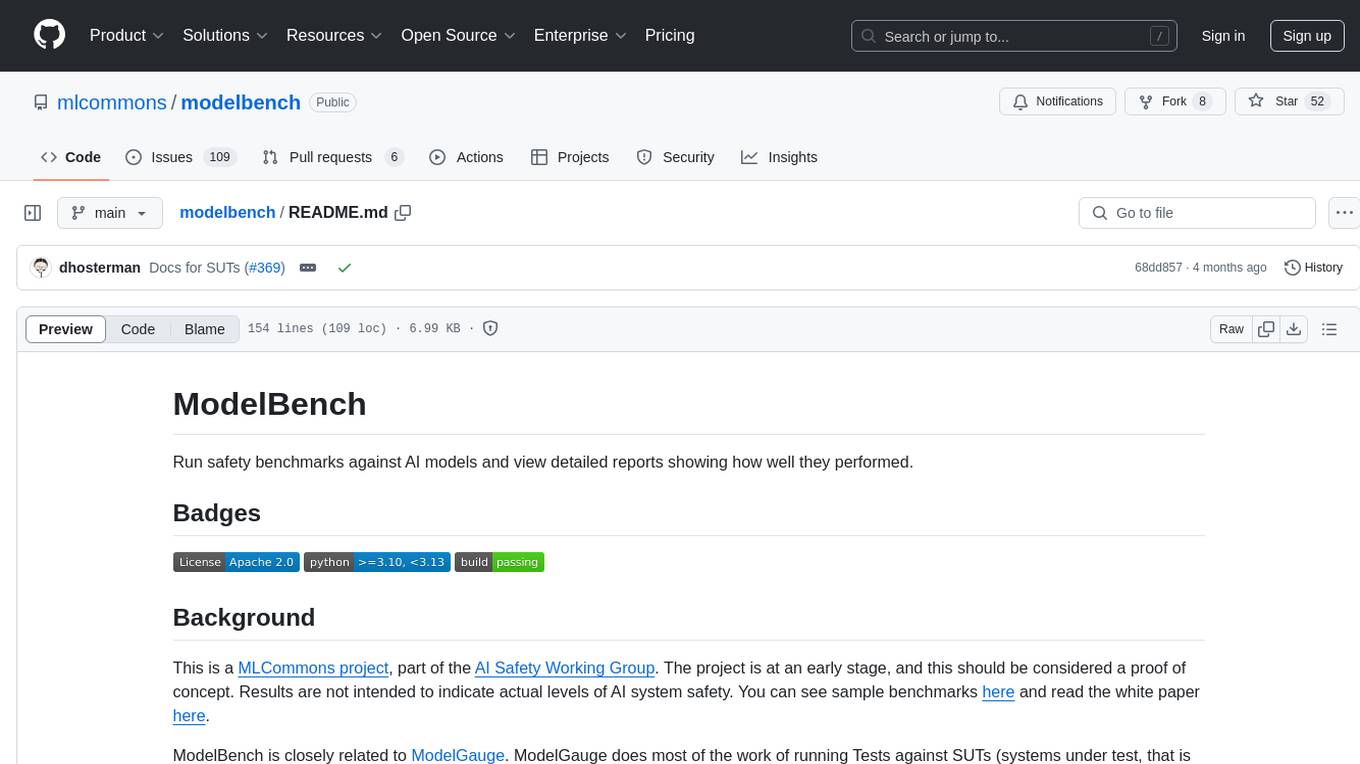
modelbench
ModelBench is a tool for running safety benchmarks against AI models and generating detailed reports. It is part of the MLCommons project and is designed as a proof of concept to aggregate measures, relate them to specific harms, create benchmarks, and produce reports. The tool requires LlamaGuard for evaluating responses and a TogetherAI account for running benchmarks. Users can install ModelBench from GitHub or PyPI, run tests using Poetry, and create benchmarks by providing necessary API keys. The tool generates static HTML pages displaying benchmark scores and allows users to dump raw scores and manage cache for faster runs. ModelBench is aimed at enabling users to test their own models and create tests and benchmarks.
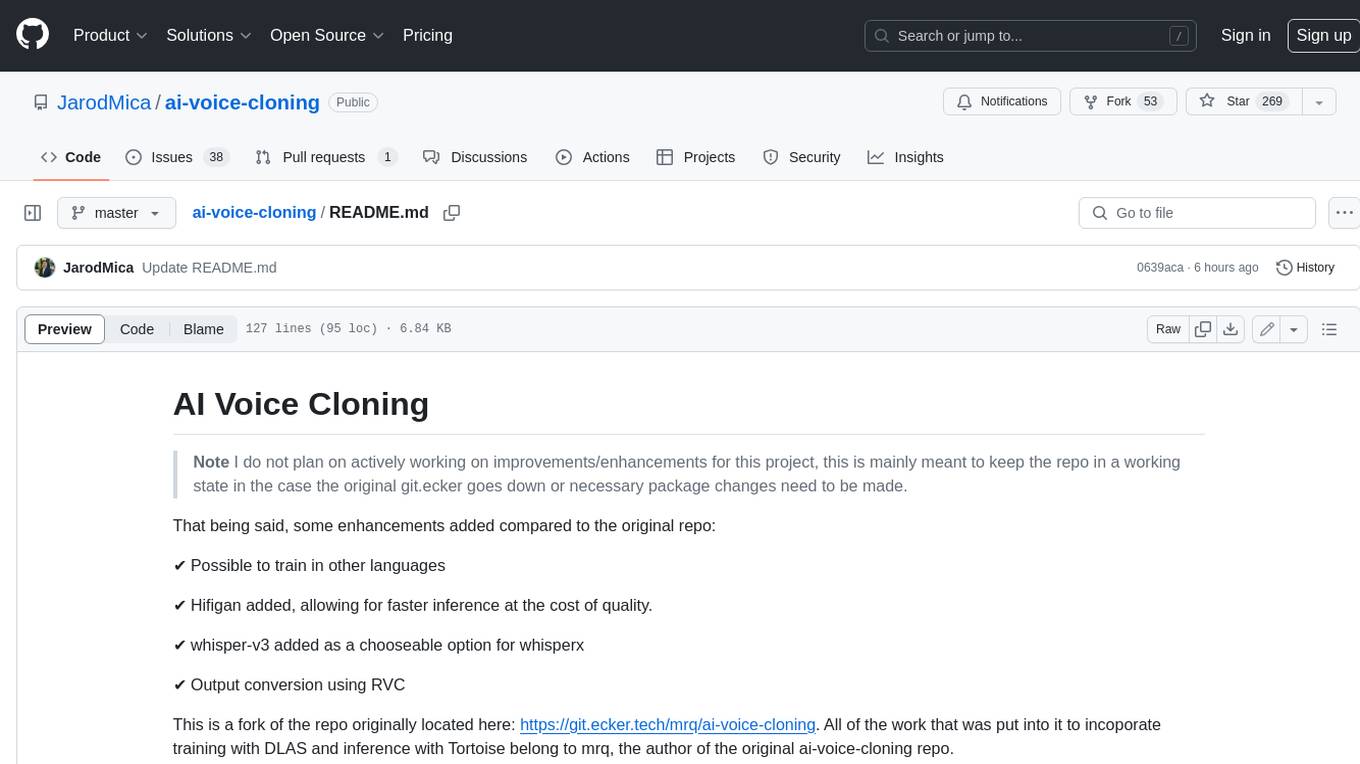
ai-voice-cloning
This repository provides a tool for AI voice cloning, allowing users to generate synthetic speech that closely resembles a target speaker's voice. The tool is designed to be user-friendly and accessible, with a graphical user interface that guides users through the process of training a voice model and generating synthetic speech. The tool also includes a variety of features that allow users to customize the generated speech, such as the pitch, volume, and speaking rate. Overall, this tool is a valuable resource for anyone interested in creating realistic and engaging synthetic speech.
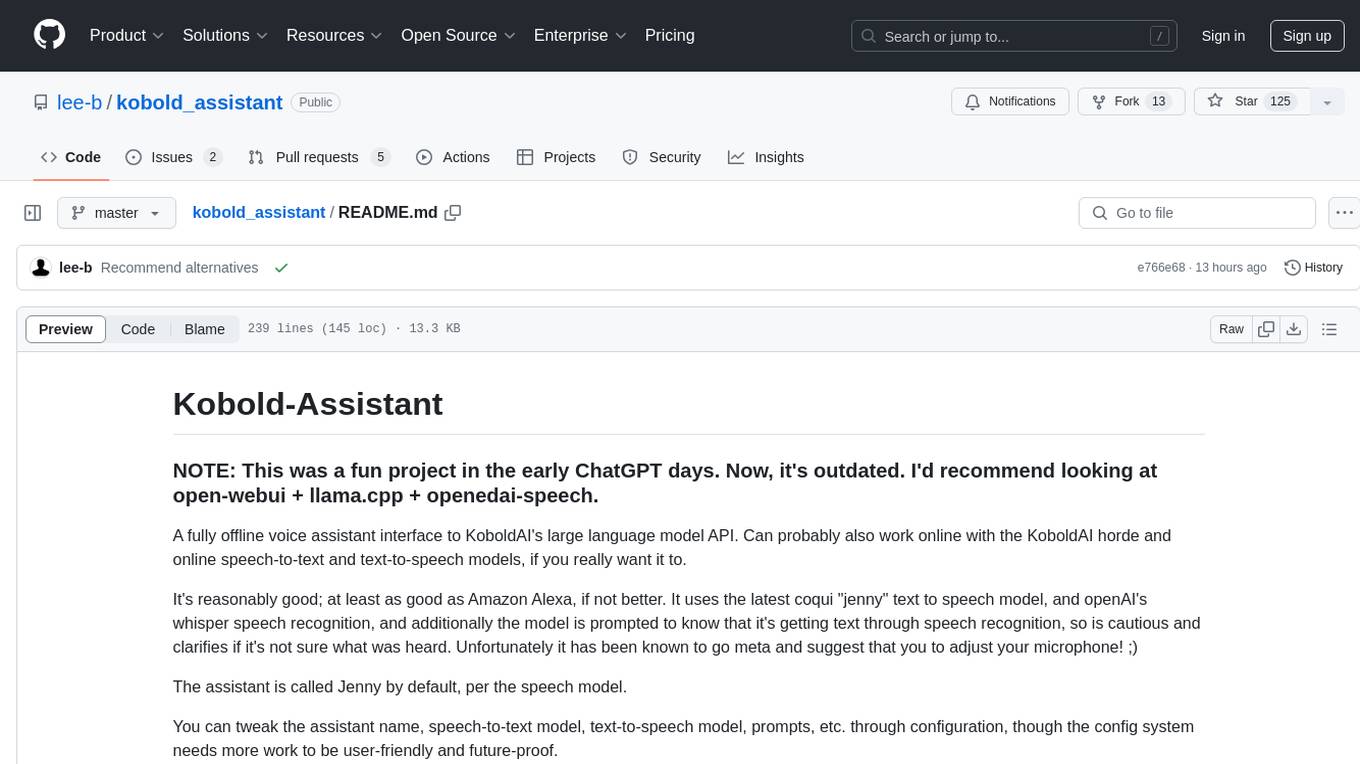
kobold_assistant
Kobold-Assistant is a fully offline voice assistant interface to KoboldAI's large language model API. It can work online with the KoboldAI horde and online speech-to-text and text-to-speech models. The assistant, called Jenny by default, uses the latest coqui 'jenny' text to speech model and openAI's whisper speech recognition. Users can customize the assistant name, speech-to-text model, text-to-speech model, and prompts through configuration. The tool requires system packages like GCC, portaudio development libraries, and ffmpeg, along with Python >=3.7, <3.11, and runs on Ubuntu/Debian systems. Users can interact with the assistant through commands like 'serve' and 'list-mics'.
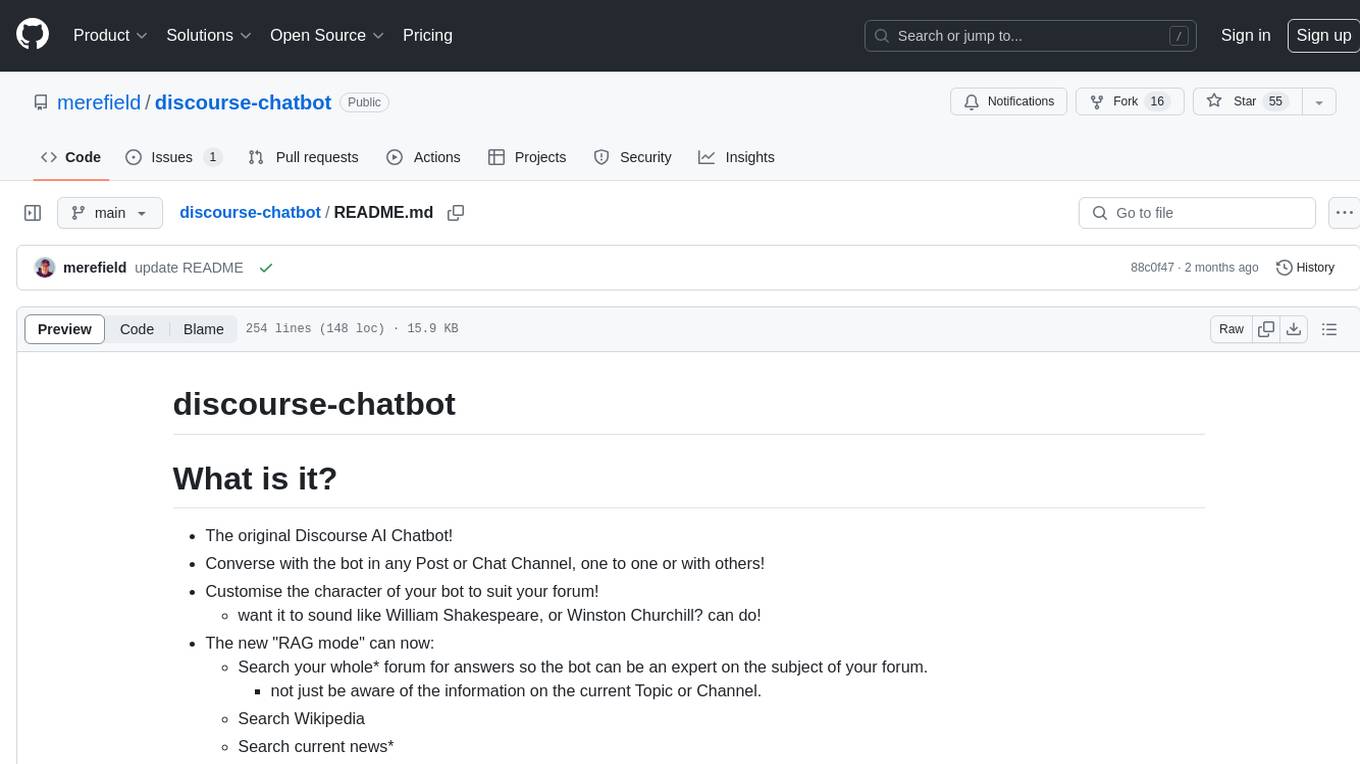
discourse-chatbot
The discourse-chatbot is an original AI chatbot for Discourse forums that allows users to converse with the bot in posts or chat channels. Users can customize the character of the bot, enable RAG mode for expert answers, search Wikipedia, news, and Google, provide market data, perform accurate math calculations, and experiment with vision support. The bot uses cutting-edge Open AI API and supports Azure and proxy server connections. It includes a quota system for access management and can be used in RAG mode or basic bot mode. The setup involves creating embeddings to make the bot aware of forum content and setting up bot access permissions based on trust levels. Users must obtain an API token from Open AI and configure group quotas to interact with the bot. The plugin is extensible to support other cloud bots and content search beyond the provided set.
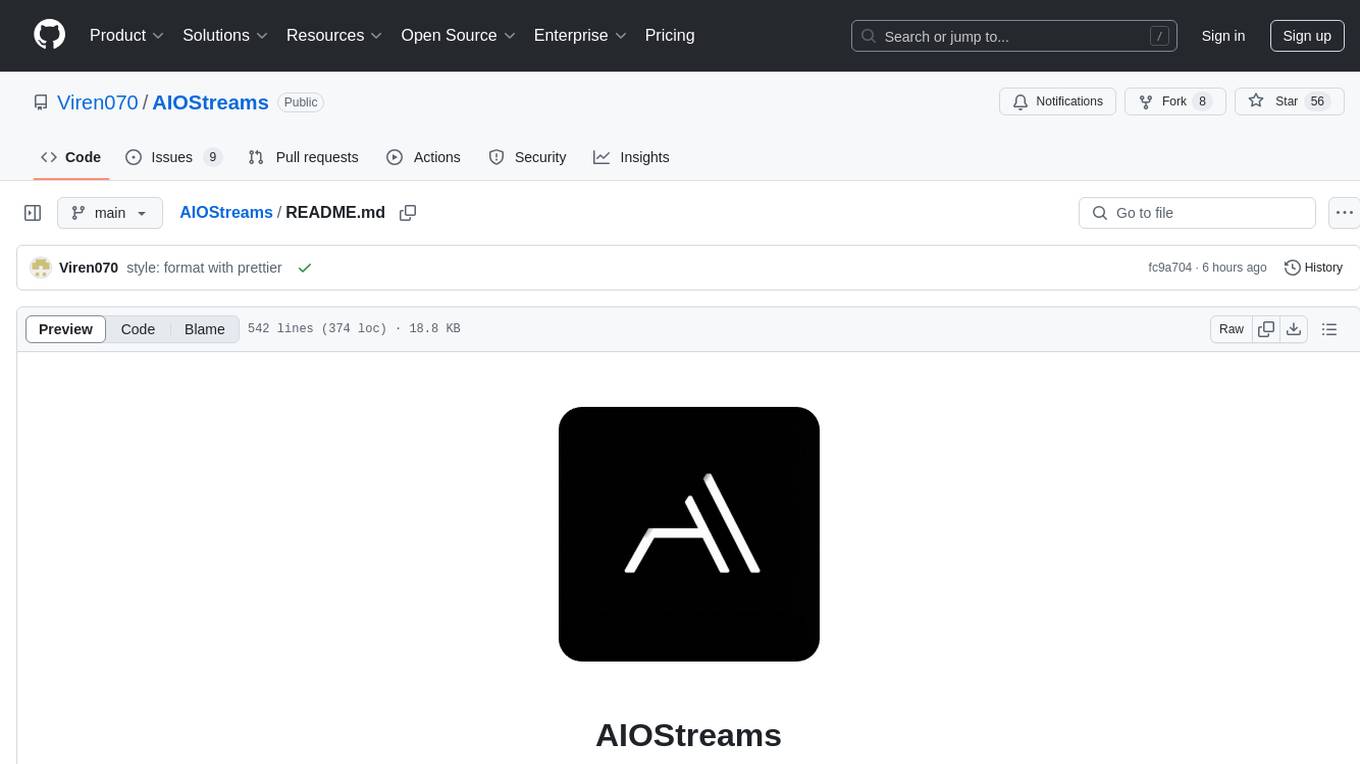
AIOStreams
AIOStreams is a versatile tool that combines streams from various addons into one platform, offering extensive customization options. Users can change result formats, filter results by various criteria, remove duplicates, prioritize services, sort results, specify size limits, and more. The tool scrapes results from selected addons, applies user configurations, and presents the results in a unified manner. It simplifies the process of finding and accessing desired content from multiple sources, enhancing user experience and efficiency.
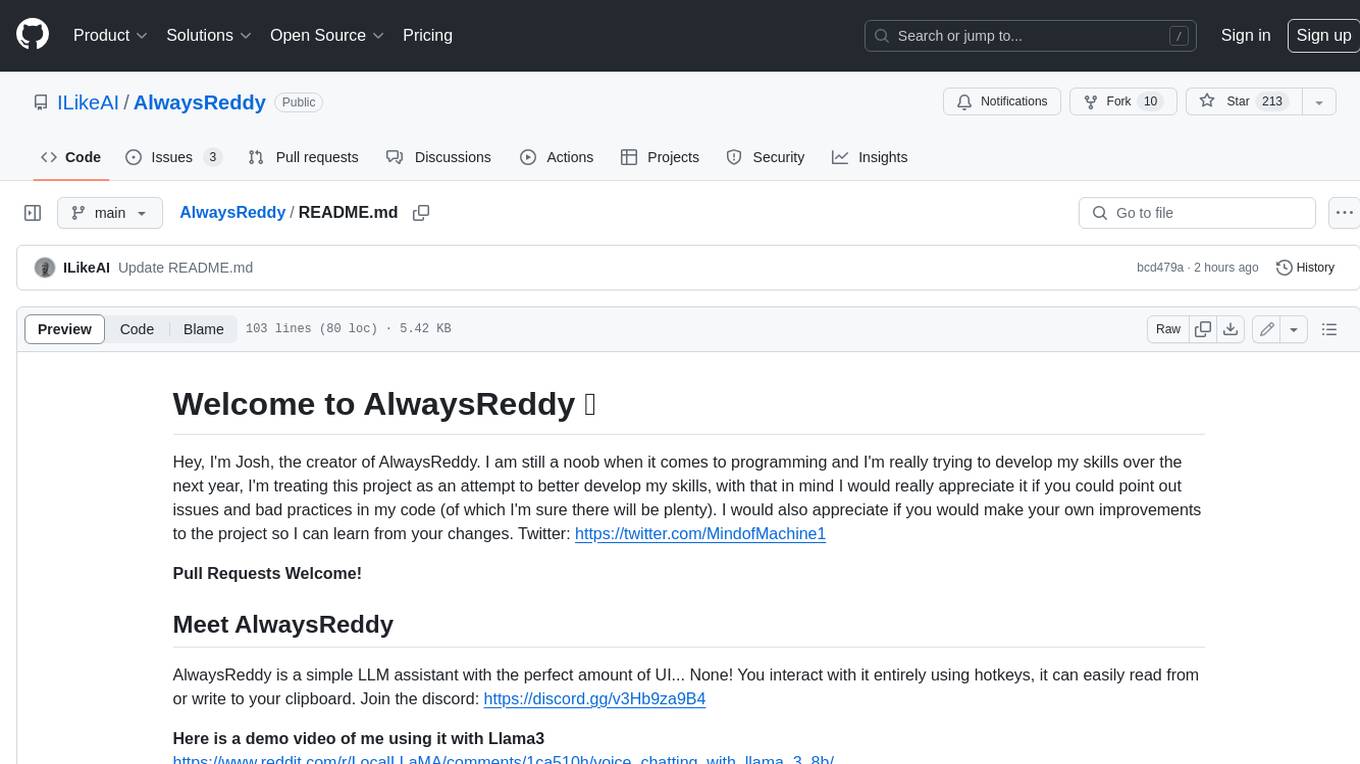
AlwaysReddy
AlwaysReddy is a simple LLM assistant with no UI that you interact with entirely using hotkeys. It can easily read from or write to your clipboard, and voice chat with you via TTS and STT. Here are some of the things you can use AlwaysReddy for: - Explain a new concept to AlwaysReddy and have it save the concept (in roughly your words) into a note. - Ask AlwaysReddy "What is X called?" when you know how to roughly describe something but can't remember what it is called. - Have AlwaysReddy proofread the text in your clipboard before you send it. - Ask AlwaysReddy "From the comments in my clipboard, what do the r/LocalLLaMA users think of X?" - Quickly list what you have done today and get AlwaysReddy to write a journal entry to your clipboard before you shutdown the computer for the day.
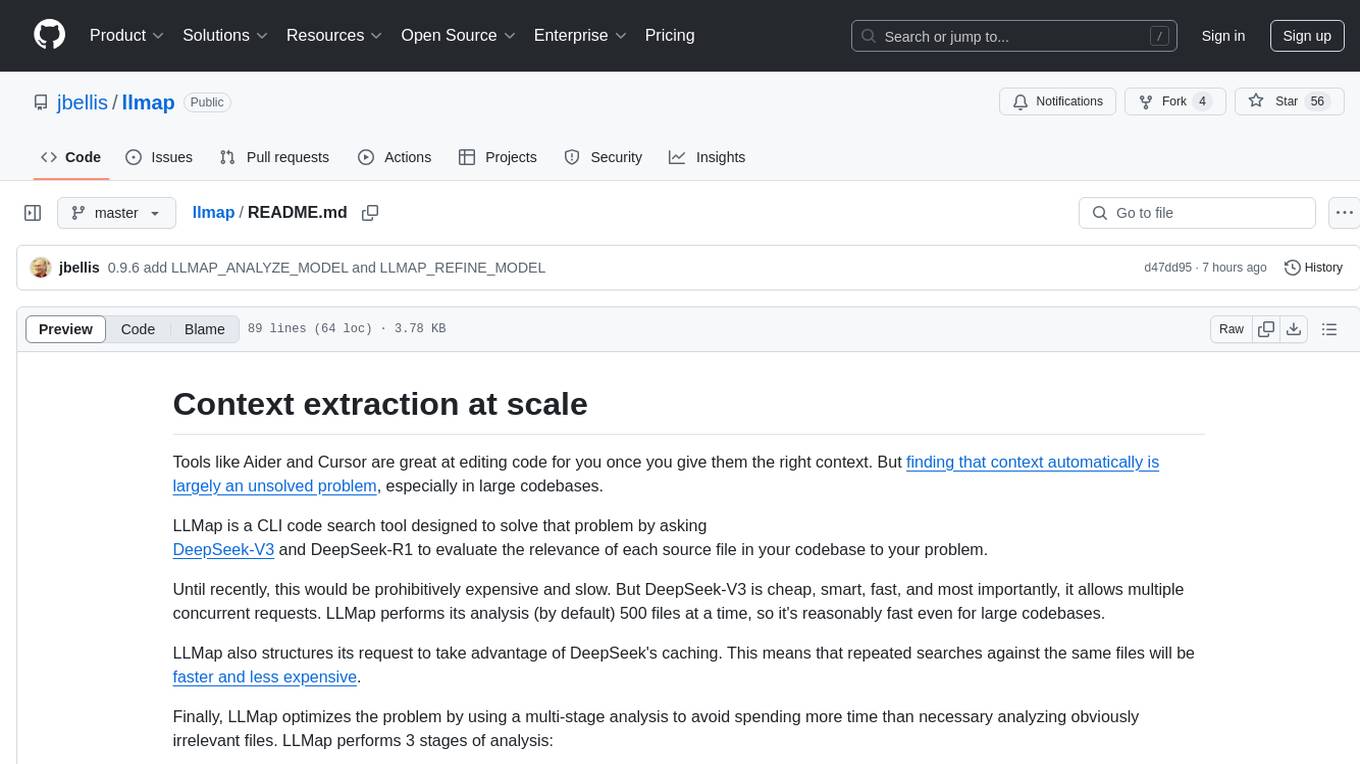
llmap
LLMap is a CLI code search tool designed to automatically find context in large codebases by evaluating the relevance of each source file using DeepSeek-V3 and DeepSeek-R1. It optimizes analysis by performing multi-stage analysis and caching results for faster searches. Currently supports Java and Python files, with potential for extension to other languages. Install with 'pip install llmap-ai' and use with a DeepSeek API key to search for specific context in code.
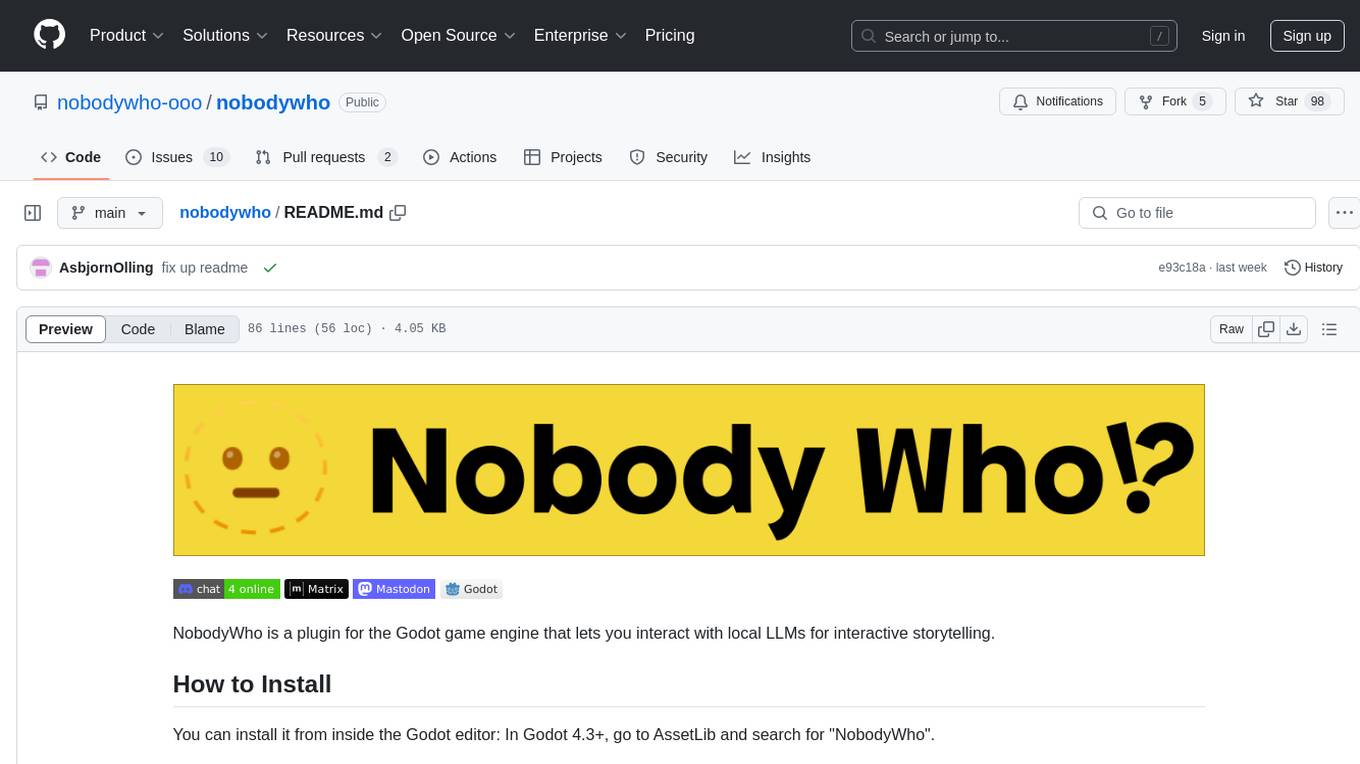
nobodywho
NobodyWho is a plugin for the Godot game engine that enables interaction with local LLMs for interactive storytelling. Users can install it from Godot editor or GitHub releases page, providing their own LLM in GGUF format. The plugin consists of `NobodyWhoModel` node for model file, `NobodyWhoChat` node for chat interaction, and `NobodyWhoEmbedding` node for generating embeddings. It offers a programming interface for sending text to LLM, receiving responses, and starting the LLM worker.
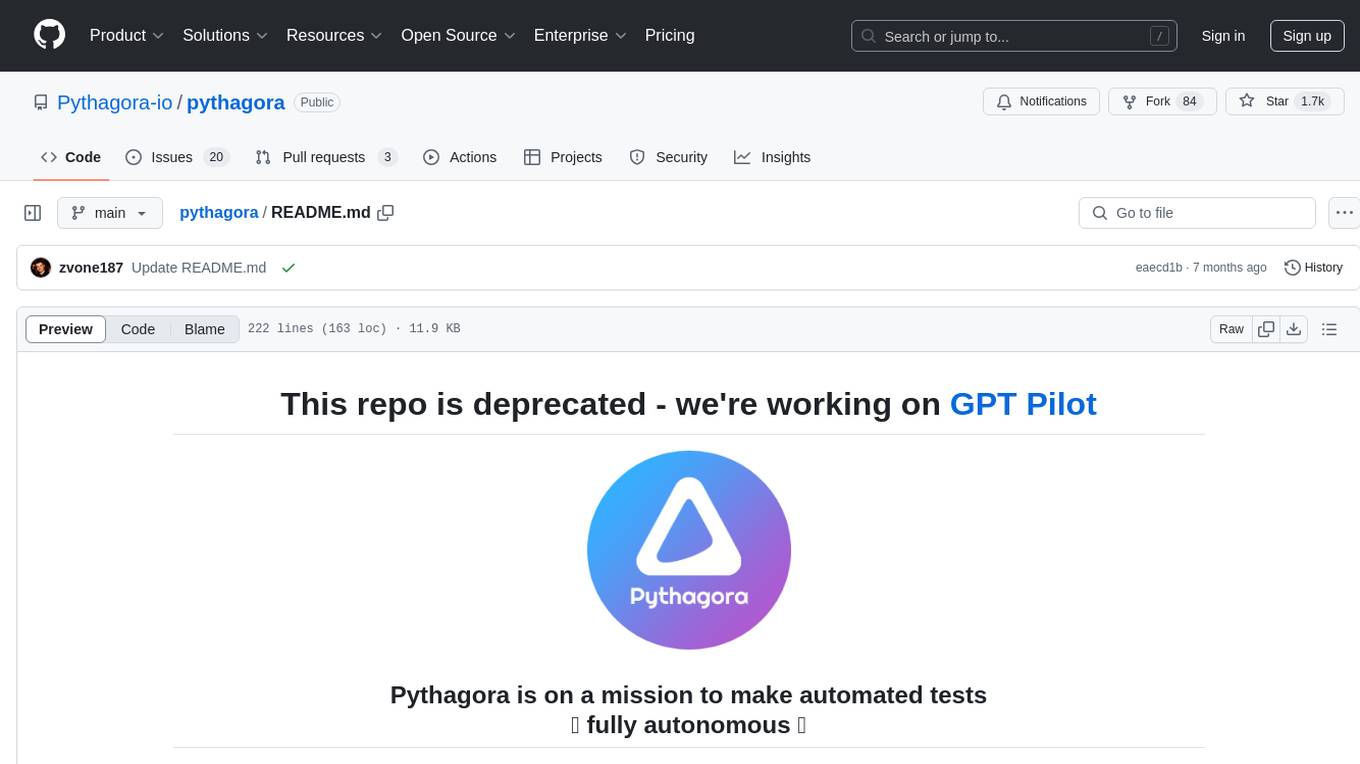
pythagora
Pythagora is an automated testing tool designed to generate unit tests using GPT-4. By running a single command, users can create tests for specific functions in their codebase. The tool leverages AST parsing to identify related functions and sends them to the Pythagora server for test generation. Pythagora primarily focuses on JavaScript code and supports Jest testing framework. Users can expand existing tests, increase code coverage, and find bugs efficiently. It is recommended to review the generated tests before committing them to the repository. Pythagora does not store user code on its servers but sends it to GPT and OpenAI for test generation.
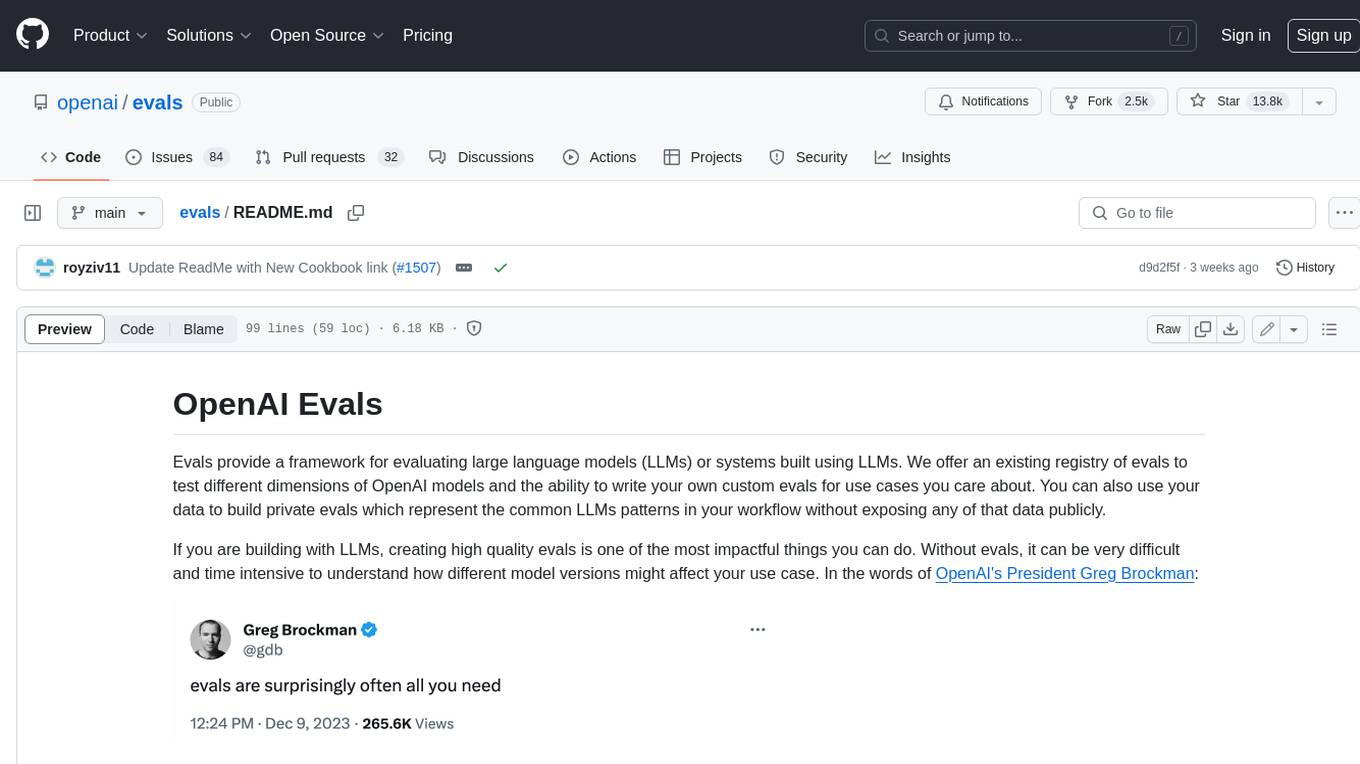
evals
Evals provide a framework for evaluating large language models (LLMs) or systems built using LLMs. We offer an existing registry of evals to test different dimensions of OpenAI models and the ability to write your own custom evals for use cases you care about. You can also use your data to build private evals which represent the common LLMs patterns in your workflow without exposing any of that data publicly.
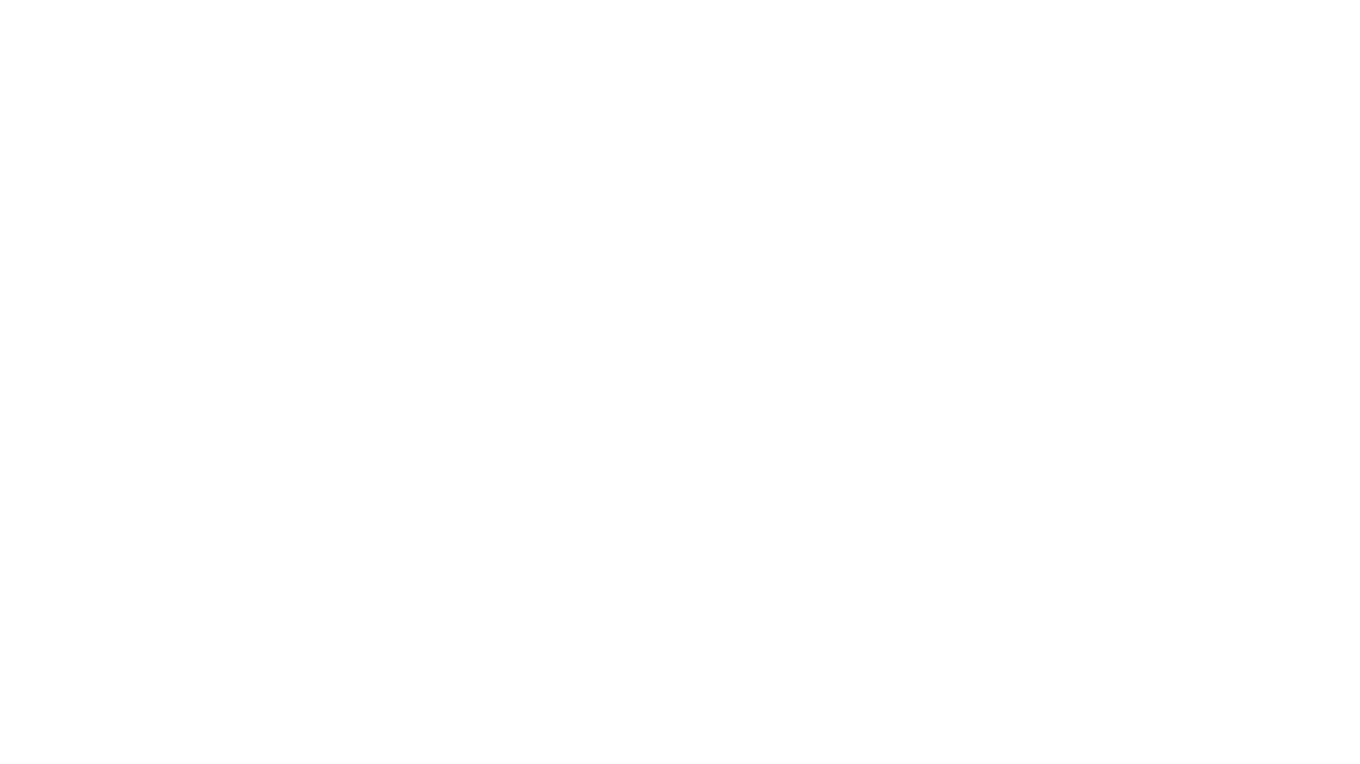
GlaDOS
This project aims to create a real-life version of GLaDOS, an aware, interactive, and embodied AI entity. It involves training a voice generator, developing a 'Personality Core,' implementing a memory system, providing vision capabilities, creating 3D-printable parts, and designing an animatronics system. The software architecture focuses on low-latency voice interactions, utilizing a circular buffer for data recording, text streaming for quick transcription, and a text-to-speech system. The project also emphasizes minimal dependencies for running on constrained hardware. The hardware system includes servo- and stepper-motors, 3D-printable parts for GLaDOS's body, animations for expression, and a vision system for tracking and interaction. Installation instructions cover setting up the TTS engine, required Python packages, compiling llama.cpp, installing an inference backend, and voice recognition setup. GLaDOS can be run using 'python glados.py' and tested using 'demo.ipynb'.
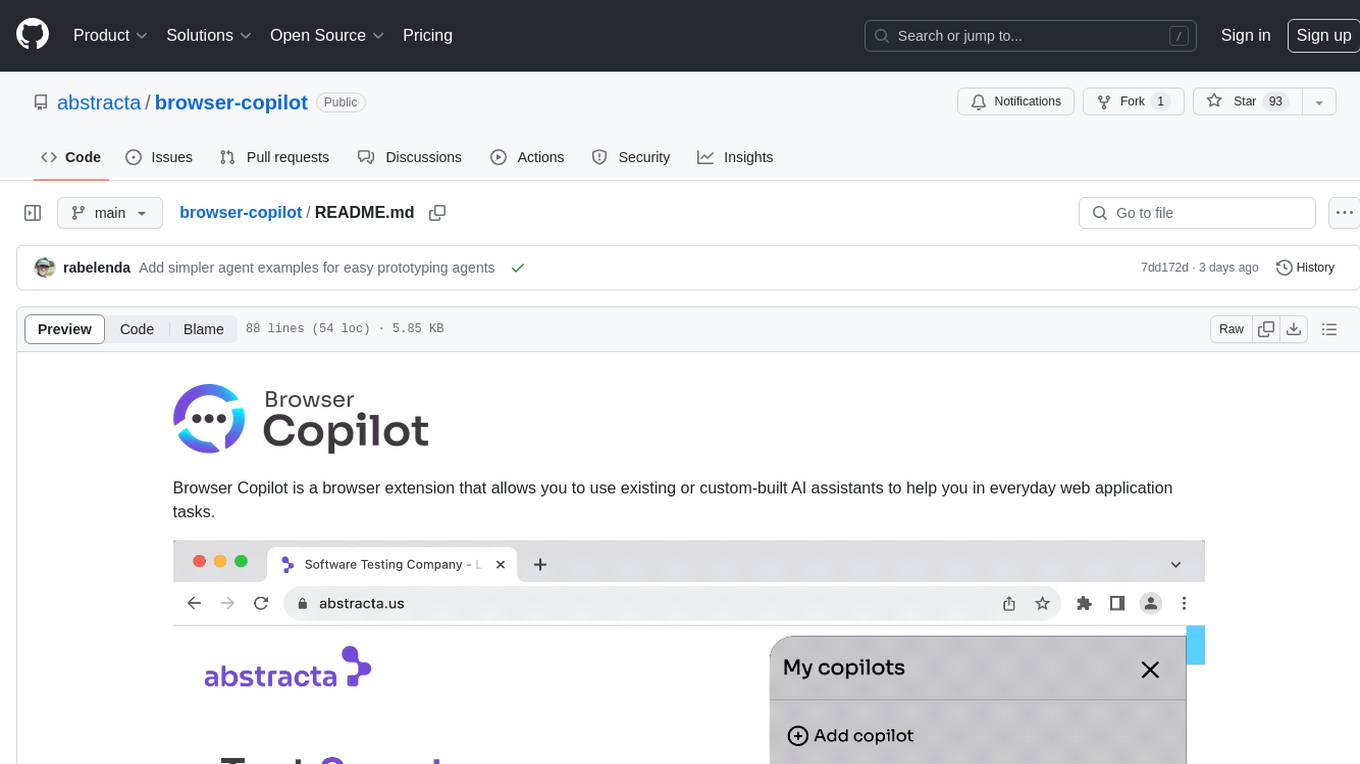
browser-copilot
Browser Copilot is a browser extension that enables users to utilize AI assistants for various web application tasks. It provides a versatile UI and framework to implement copilots that can automate tasks, extract information, interact with web applications, and utilize service APIs. Users can easily install copilots, start chats, save prompts, and toggle the copilot on or off. The project also includes a sample copilot implementation for testing purposes and encourages community contributions to expand the catalog of copilots.
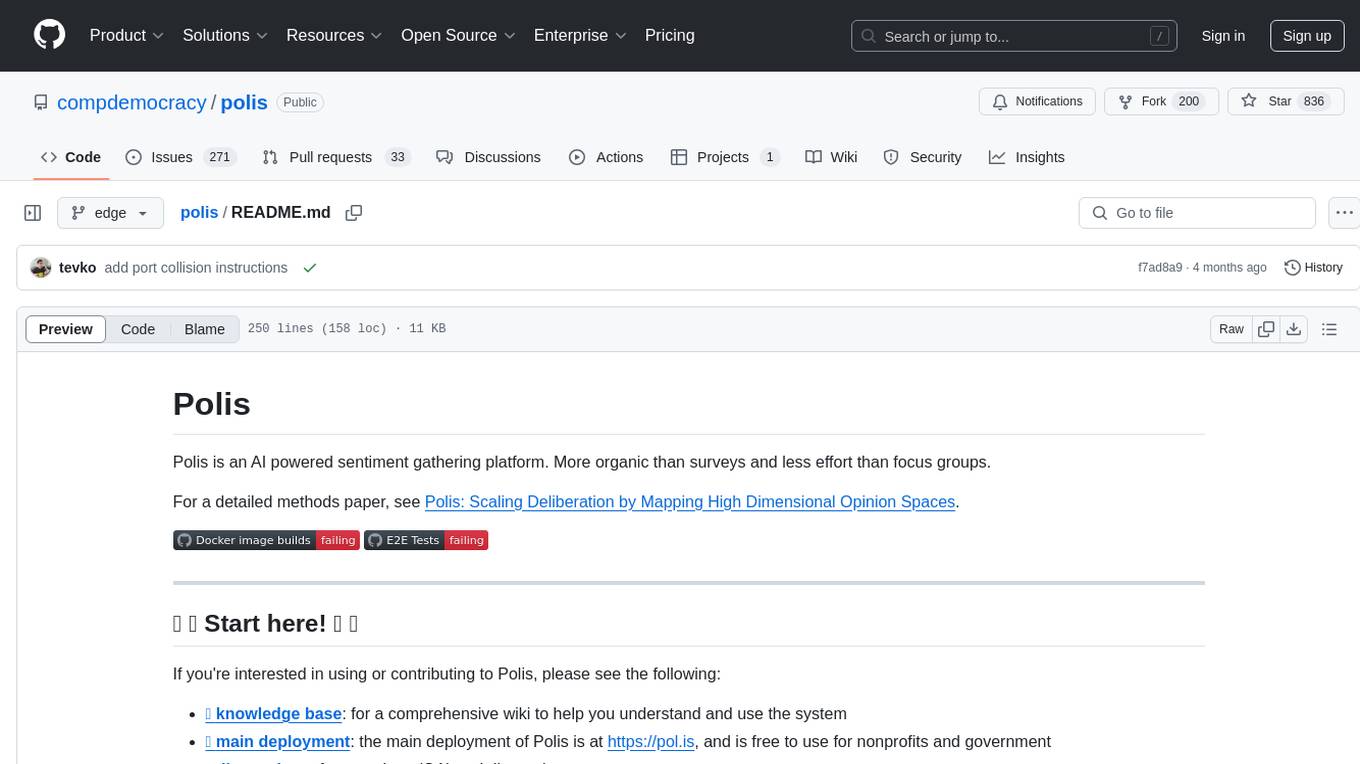
polis
Polis is an AI powered sentiment gathering platform that offers a more organic approach than surveys and requires less effort than focus groups. It provides a comprehensive wiki, main deployment at https://pol.is, discussions, issue tracking, and project board for users. Polis can be set up using Docker infrastructure and offers various commands for building and running containers. Users can test their instance, update the system, and deploy Polis for production. The tool also provides developer conveniences for code reloading, type checking, and database connections. Additionally, Polis supports end-to-end browser testing using Cypress and offers troubleshooting tips for common Docker and npm issues.
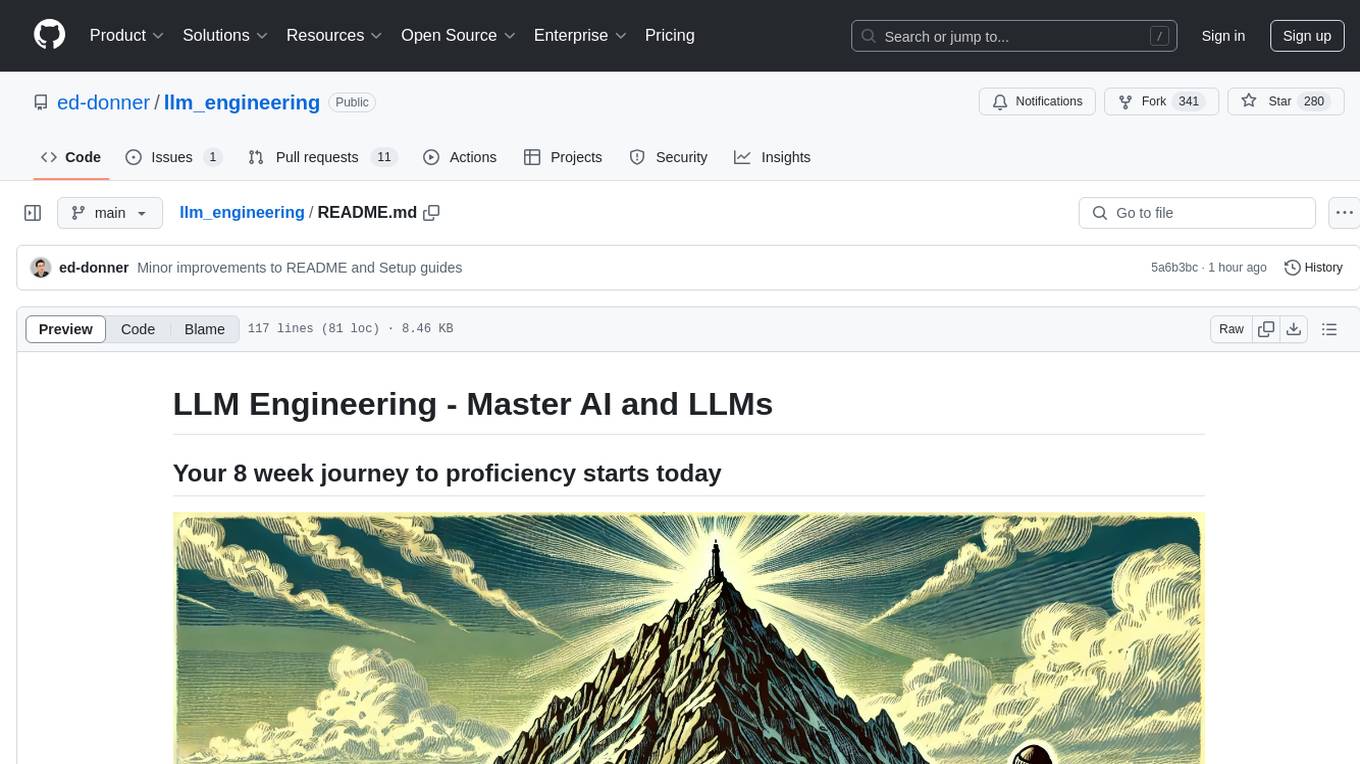
llm_engineering
LLM Engineering is an 8-week course designed to help learners master AI and LLMs through a series of projects that gradually increase in complexity. The course covers setting up the environment, working with APIs, using Google Colab for GPU processing, and building an autonomous Agentic AI solution. Learners are encouraged to actively participate, run code cells, tweak code, and share their progress with the community. The emphasis is on practical, educational projects that teach valuable business skills.
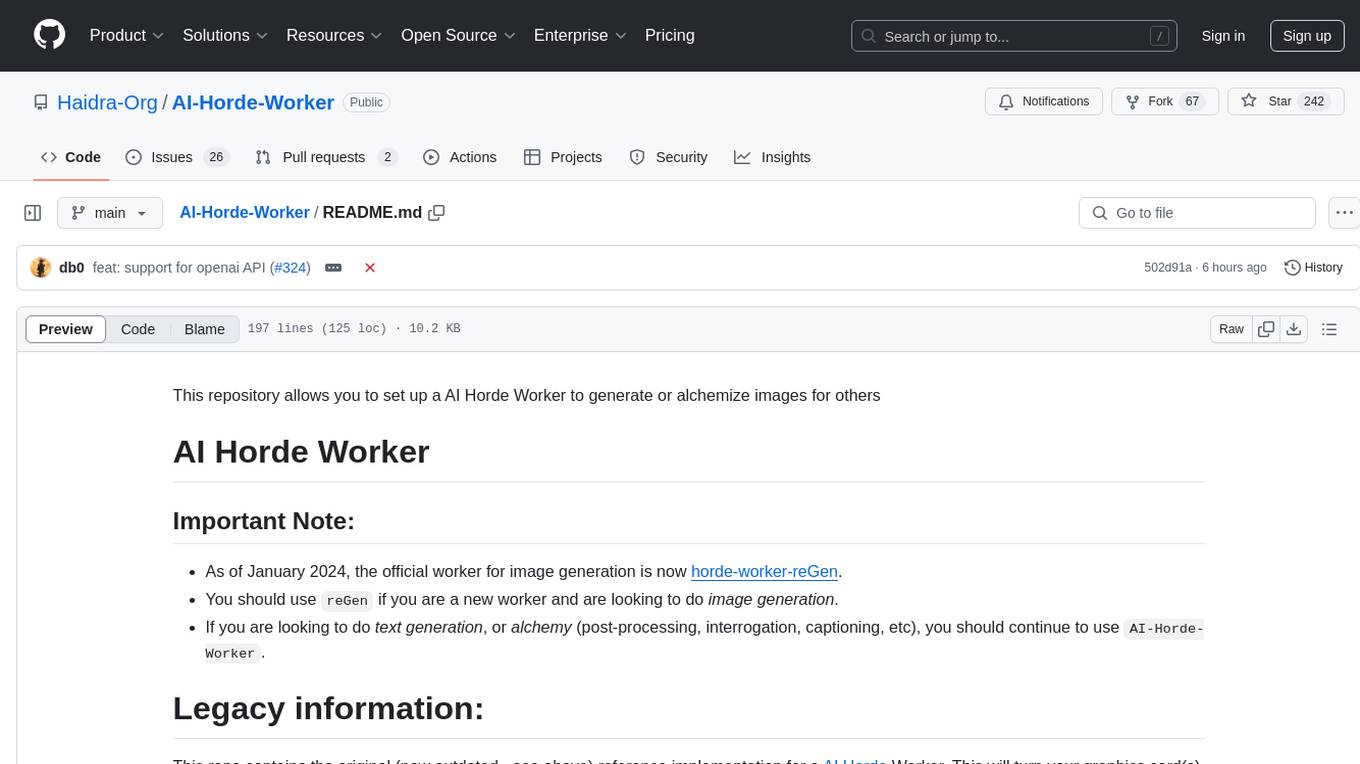
AI-Horde-Worker
AI-Horde-Worker is a repository containing the original reference implementation for a worker that turns your graphics card(s) into a worker for the AI Horde. It allows users to generate or alchemize images for others. The repository provides instructions for setting up the worker on Windows and Linux, updating the worker code, running with multiple GPUs, and stopping the worker. Users can configure the worker using a WebUI to connect to the horde with their username and API key. The repository also includes information on model usage and running the Docker container with specified environment variables.
For similar tasks
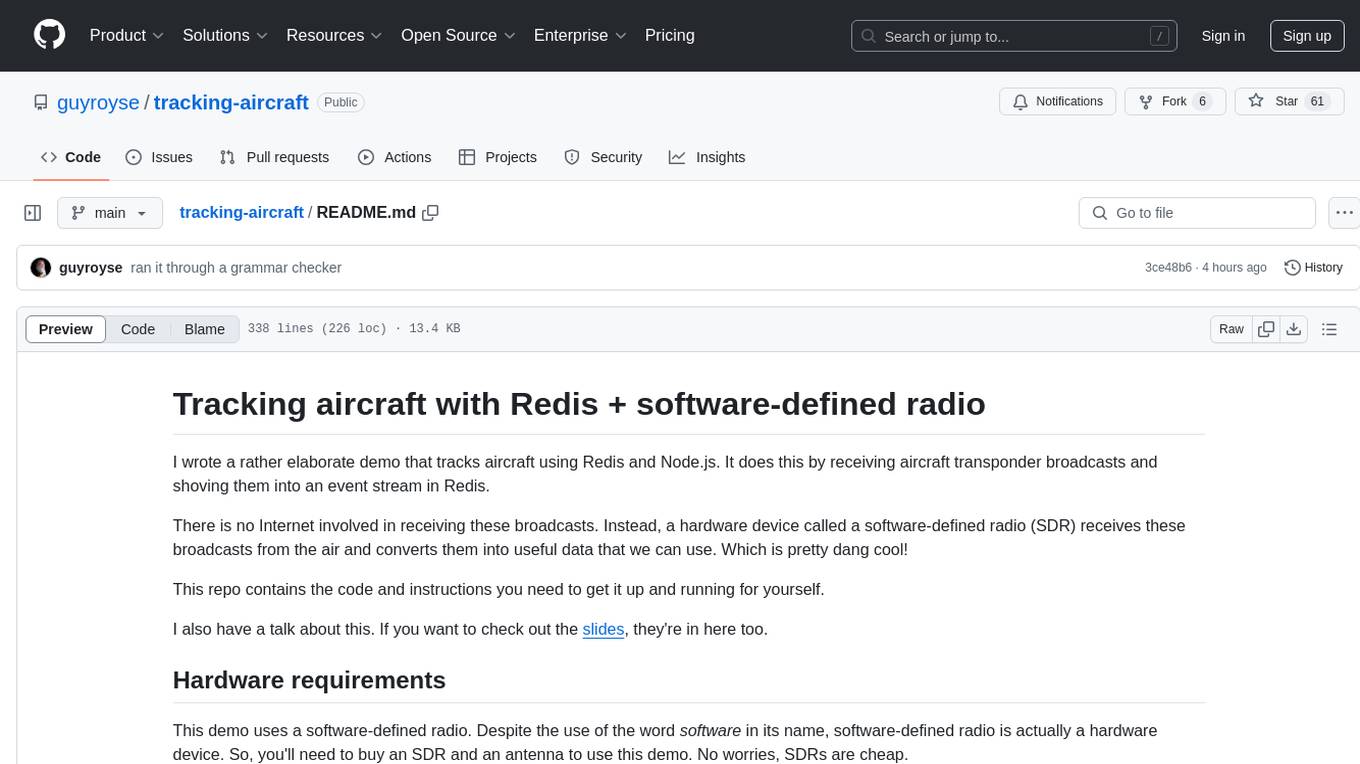
tracking-aircraft
This repository provides a demo that tracks aircraft using Redis and Node.js by receiving aircraft transponder broadcasts through a software-defined radio (SDR) and storing them in Redis. The demo includes instructions for setting up the hardware and software components required for tracking aircraft. It consists of four main components: Radio Ingestor, Flight Server, Flight UI, and Redis. The Radio Ingestor captures transponder broadcasts and writes them to a Redis event stream, while the Flight Server consumes the event stream, enriches the data, and provides APIs to query aircraft status. The Flight UI presents flight data to users in map and detail views. Users can run the demo by setting up the hardware, installing SDR software, and running the components using Docker or Node.js.
For similar jobs
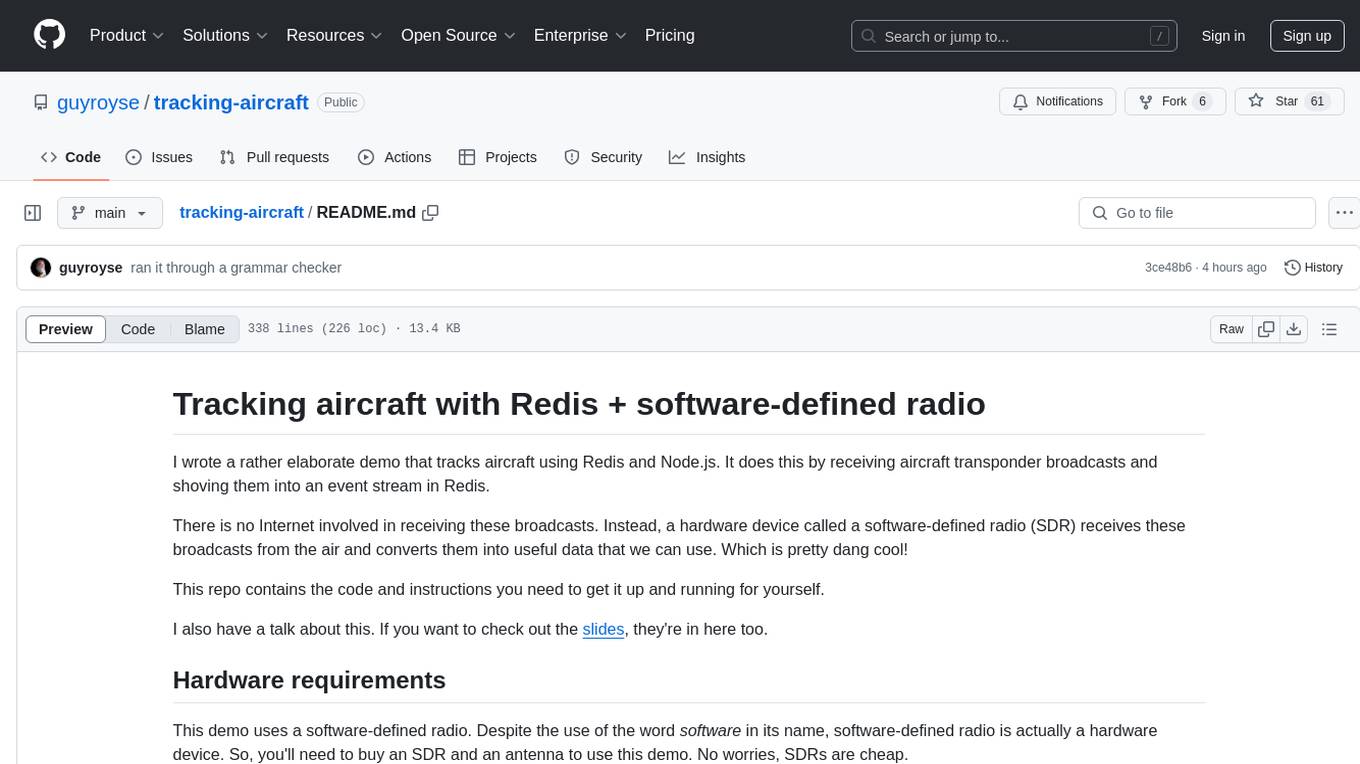
tracking-aircraft
This repository provides a demo that tracks aircraft using Redis and Node.js by receiving aircraft transponder broadcasts through a software-defined radio (SDR) and storing them in Redis. The demo includes instructions for setting up the hardware and software components required for tracking aircraft. It consists of four main components: Radio Ingestor, Flight Server, Flight UI, and Redis. The Radio Ingestor captures transponder broadcasts and writes them to a Redis event stream, while the Flight Server consumes the event stream, enriches the data, and provides APIs to query aircraft status. The Flight UI presents flight data to users in map and detail views. Users can run the demo by setting up the hardware, installing SDR software, and running the components using Docker or Node.js.
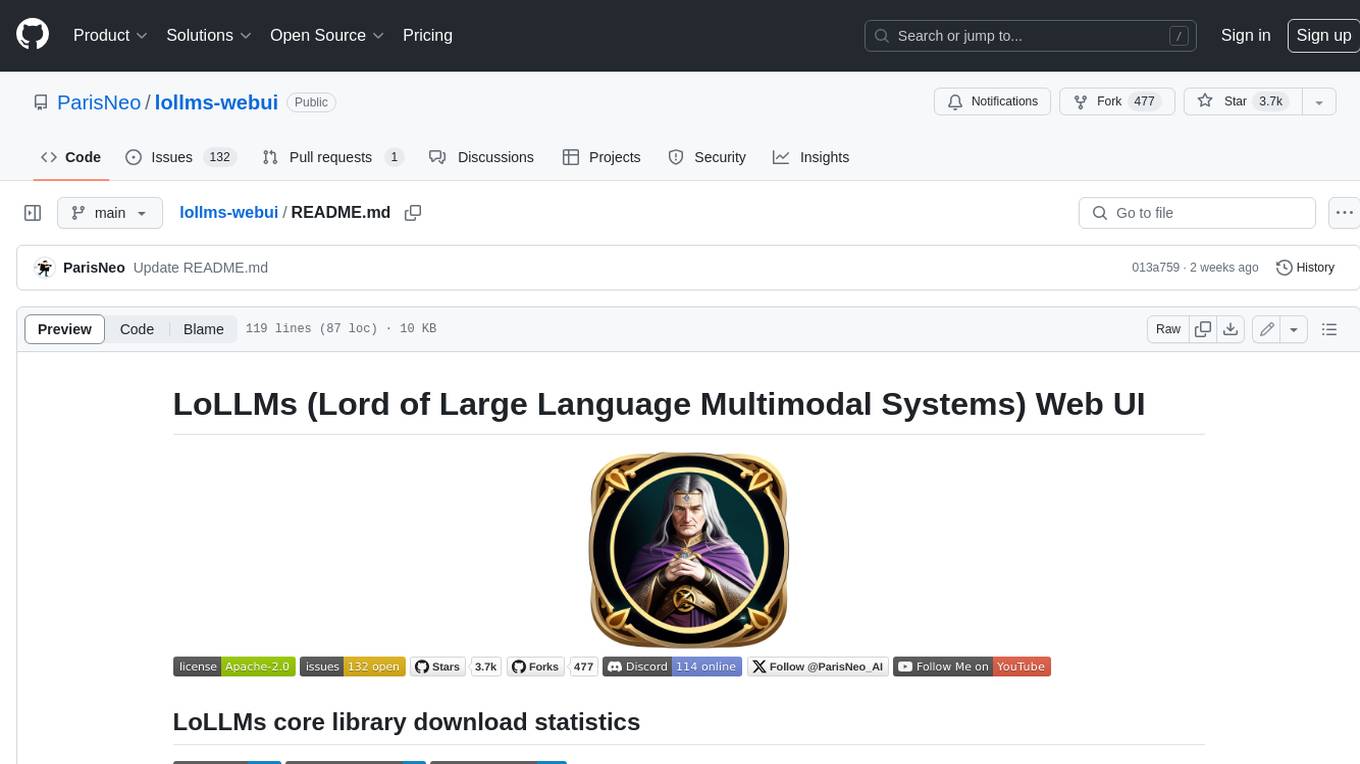
lollms-webui
LoLLMs WebUI (Lord of Large Language Multimodal Systems: One tool to rule them all) is a user-friendly interface to access and utilize various LLM (Large Language Models) and other AI models for a wide range of tasks. With over 500 AI expert conditionings across diverse domains and more than 2500 fine tuned models over multiple domains, LoLLMs WebUI provides an immediate resource for any problem, from car repair to coding assistance, legal matters, medical diagnosis, entertainment, and more. The easy-to-use UI with light and dark mode options, integration with GitHub repository, support for different personalities, and features like thumb up/down rating, copy, edit, and remove messages, local database storage, search, export, and delete multiple discussions, make LoLLMs WebUI a powerful and versatile tool.
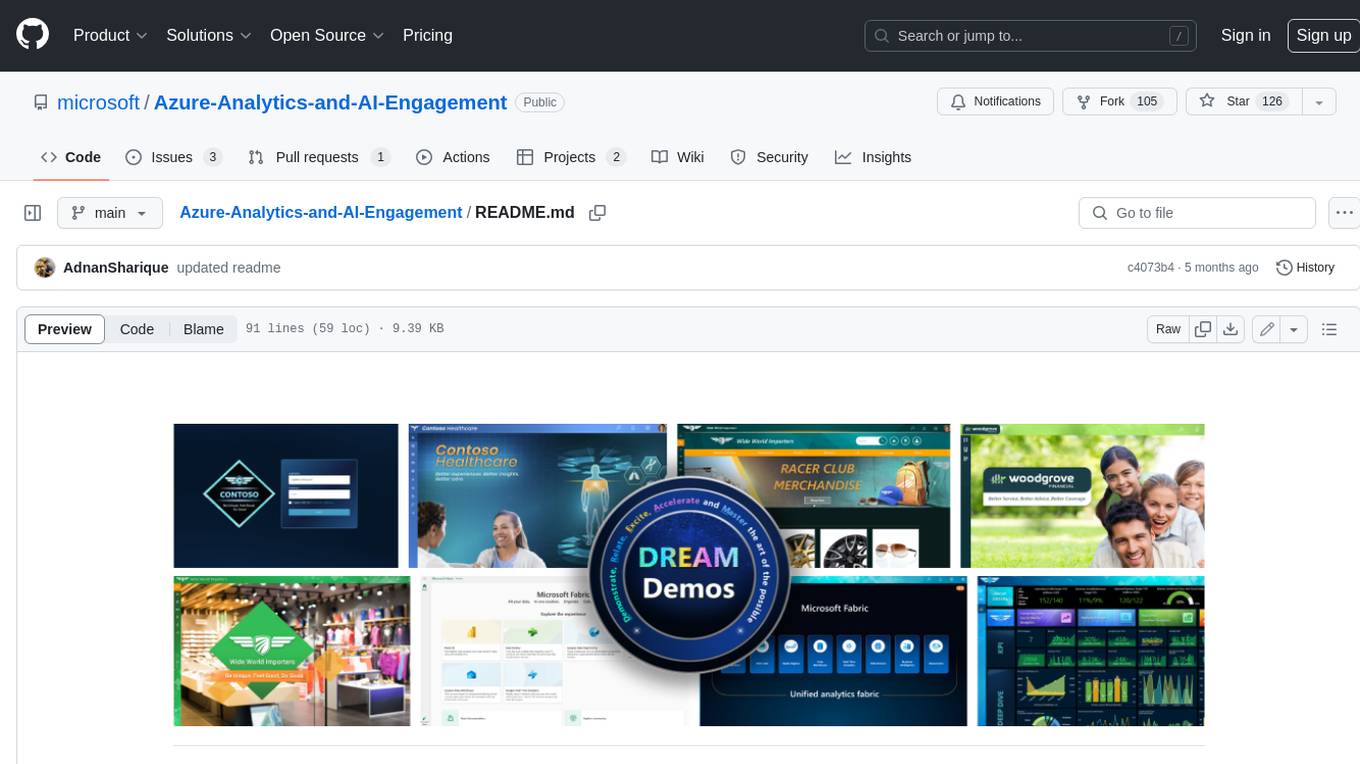
Azure-Analytics-and-AI-Engagement
The Azure-Analytics-and-AI-Engagement repository provides packaged Industry Scenario DREAM Demos with ARM templates (Containing a demo web application, Power BI reports, Synapse resources, AML Notebooks etc.) that can be deployed in a customer’s subscription using the CAPE tool within a matter of few hours. Partners can also deploy DREAM Demos in their own subscriptions using DPoC.
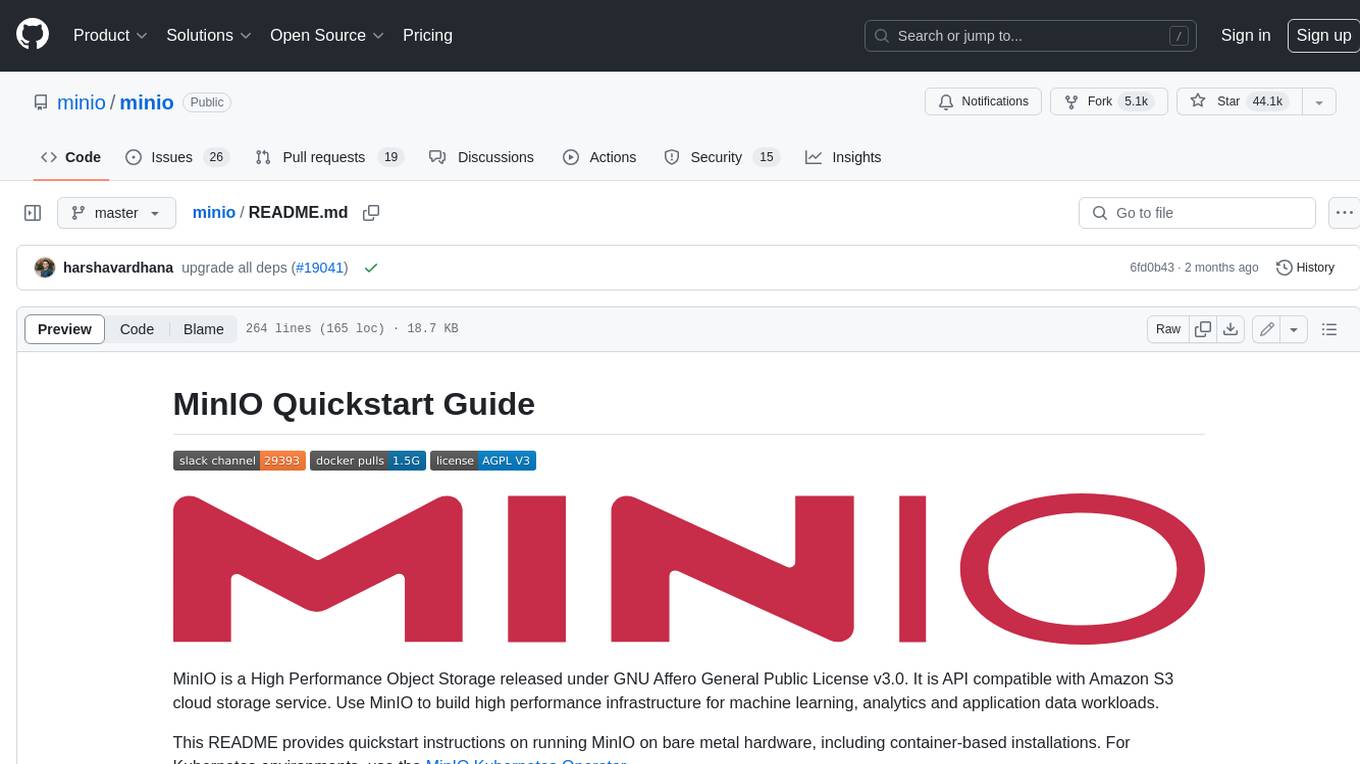
minio
MinIO is a High Performance Object Storage released under GNU Affero General Public License v3.0. It is API compatible with Amazon S3 cloud storage service. Use MinIO to build high performance infrastructure for machine learning, analytics and application data workloads.
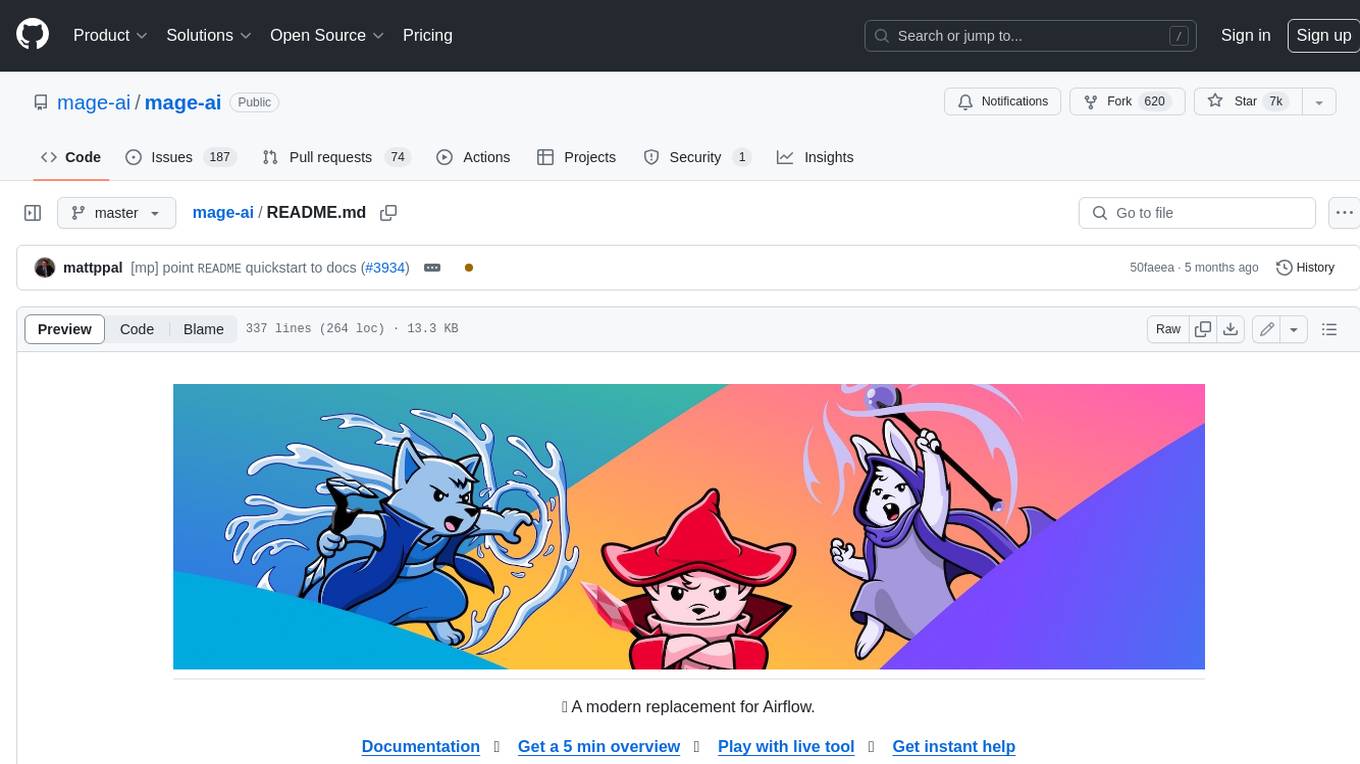
mage-ai
Mage is an open-source data pipeline tool for transforming and integrating data. It offers an easy developer experience, engineering best practices built-in, and data as a first-class citizen. Mage makes it easy to build, preview, and launch data pipelines, and provides observability and scaling capabilities. It supports data integrations, streaming pipelines, and dbt integration.
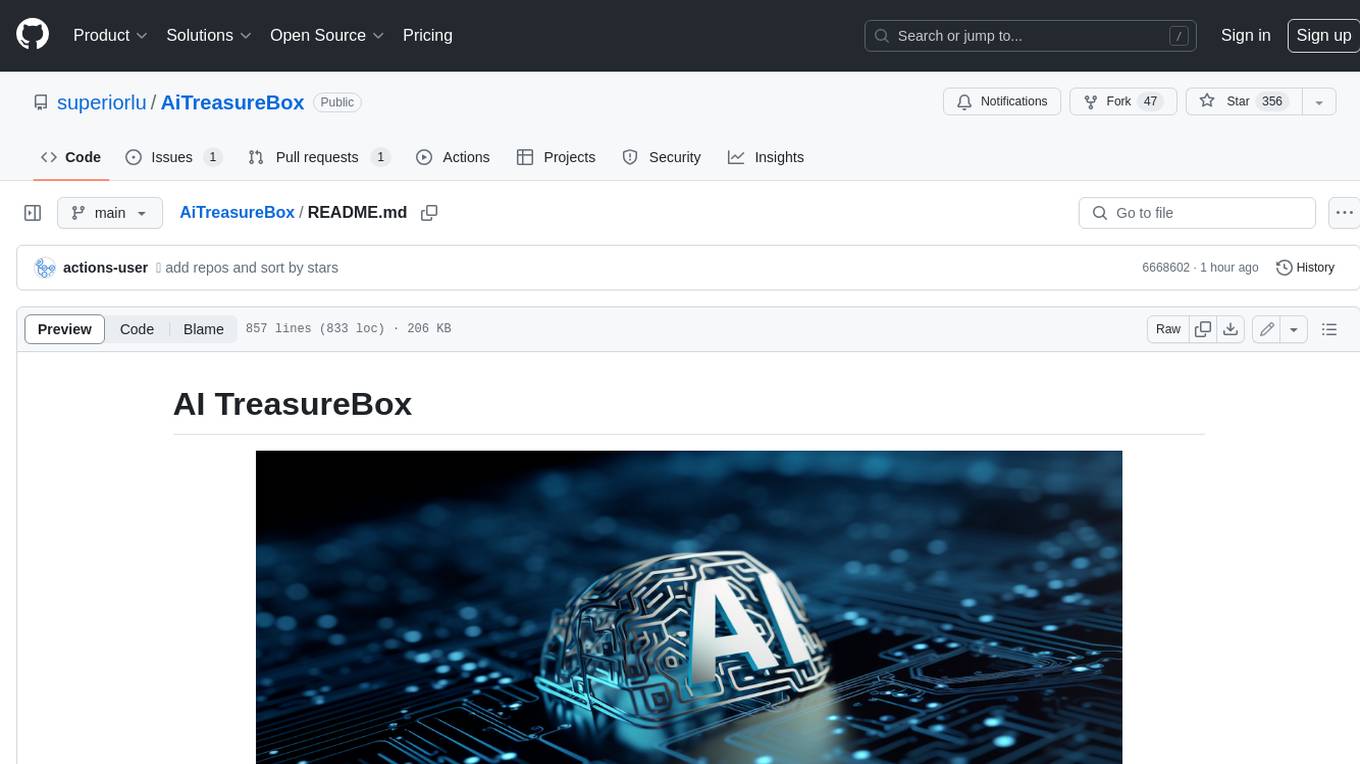
AiTreasureBox
AiTreasureBox is a versatile AI tool that provides a collection of pre-trained models and algorithms for various machine learning tasks. It simplifies the process of implementing AI solutions by offering ready-to-use components that can be easily integrated into projects. With AiTreasureBox, users can quickly prototype and deploy AI applications without the need for extensive knowledge in machine learning or deep learning. The tool covers a wide range of tasks such as image classification, text generation, sentiment analysis, object detection, and more. It is designed to be user-friendly and accessible to both beginners and experienced developers, making AI development more efficient and accessible to a wider audience.
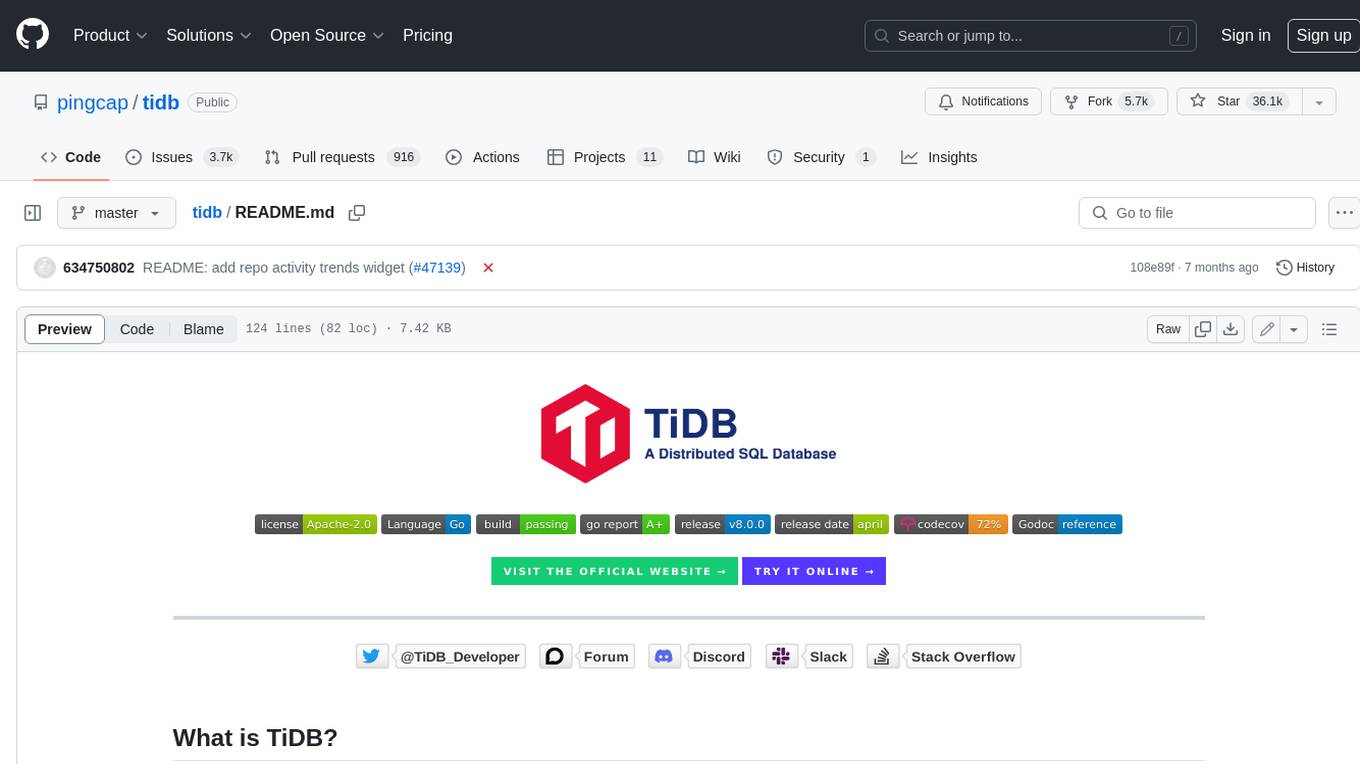
tidb
TiDB is an open-source distributed SQL database that supports Hybrid Transactional and Analytical Processing (HTAP) workloads. It is MySQL compatible and features horizontal scalability, strong consistency, and high availability.
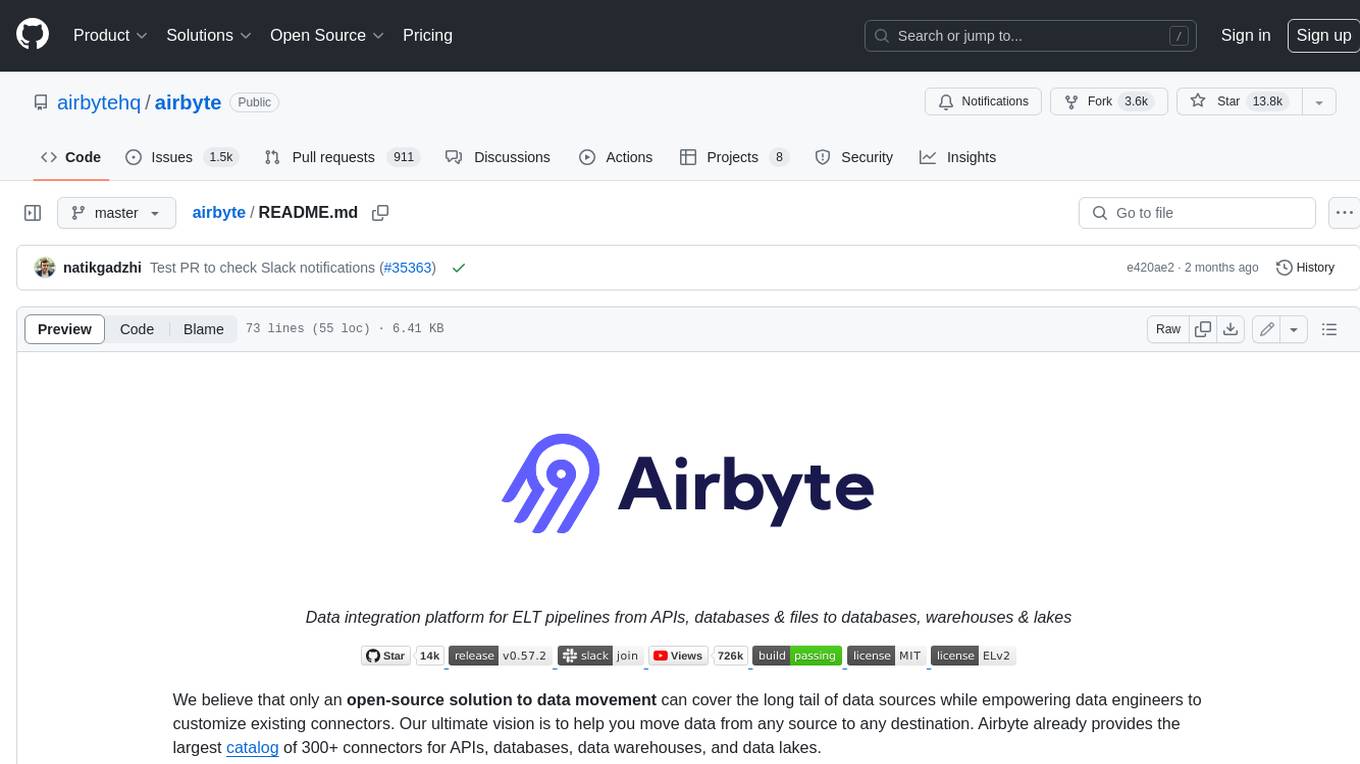
airbyte
Airbyte is an open-source data integration platform that makes it easy to move data from any source to any destination. With Airbyte, you can build and manage data pipelines without writing any code. Airbyte provides a library of pre-built connectors that make it easy to connect to popular data sources and destinations. You can also create your own connectors using Airbyte's no-code Connector Builder or low-code CDK. Airbyte is used by data engineers and analysts at companies of all sizes to build and manage their data pipelines.