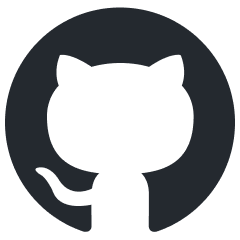
ollama-ebook-summary
LLM for Long Text Summary (Comprehensive Bulleted Notes)
Stars: 459
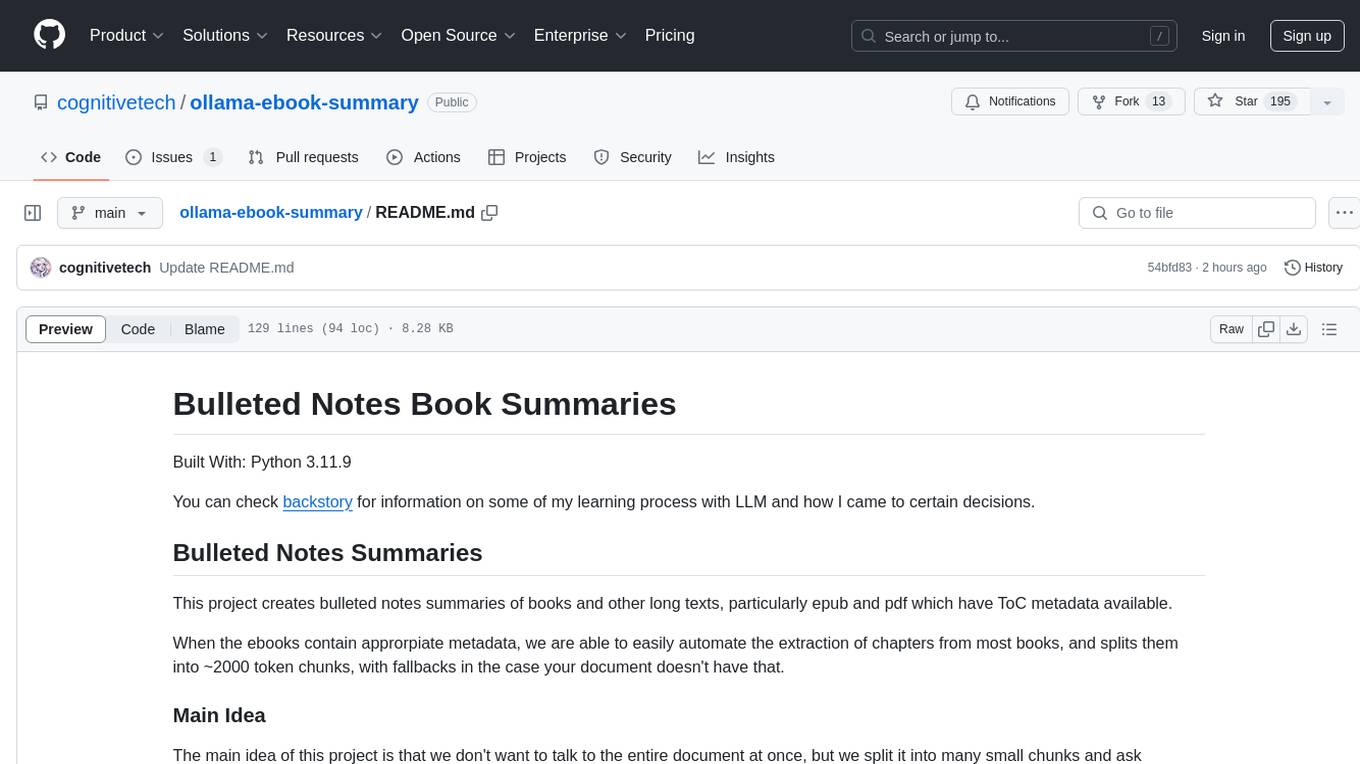
The 'ollama-ebook-summary' repository is a Python project that creates bulleted notes summaries of books and long texts, particularly in epub and pdf formats with ToC metadata. It automates the extraction of chapters, splits them into ~2000 token chunks, and allows for asking arbitrary questions to parts of the text for improved granularity of response. The tool aims to provide summaries for each page of a book rather than a one-page summary of the entire document, enhancing content curation and knowledge sharing capabilities.
README:
Built With: Python 3.11.9
This project creates bulleted notes summaries of books and other long texts, particularly epub and pdf which have ToC metadata available.
When the ebooks contain approrpiate metadata, we are able to easily automate the extraction of chapters from most books, and split them into ~2000 token chunks, with fallbacks in case we are unable to access a document outline.
Same Task, More Tokens: the Impact of Input Length on the Reasoning Performance of Large Language Models (2024-02-19; Mosh Levy, Alon Jacoby, Yoav Goldberg) suggests that reasoning capacity drops off pretty sharply from 250 to 1000 tokens, starting to flatten out between 2000-3000 tokens.
This corresponds my own experience while summarizing many long documents using local llm.
You can check the depreciated walkthroughs and rankings for more background on how I got here.
Similar to Retrieval Augmented Generation (RAG), we split the document into many parts, so they fit into the context. The difference is that RAG systems try to determine what is the best chunk to ask their question to. Instead, we ask the same questions to every part of the document.
Its very important towards unlocking the full capabilities of LLM without relying on a multitude of 3rd party apps.
Before starting, ensure you have Python 3.11.9 installed. If not, you can use conda or pyenv to manage Python versions:
Using conda:
- Install Anaconda from: https://www.anaconda.com/download/success
- Create a new environment:
conda create -n book_summary python=3.11.9
- Activate the environment:
conda activate book_summary
Using pyenv:
- Install pyenv: https://github.com/pyenv/pyenv#installation
- Install Python 3.11.9:
pyenv install 3.11.9
- Set local version:
pyenv local 3.11.9
pip install -r requirements.txt
ollama pull cognitivetech/obook_summary:q6_k
ollama pull cognitivetech/obook_title:q4_k_m
For your convenience Mistral 7b 0.3 is packaged with the necessary message history for title creation.
or
b) Append this message history to the Modelfile of your choice
ollama pull gemma2
Ensure the defaults are set accordingly!
This is an area subject to change which may differ from the documentation. Make sure you have the models on your system as noted in
summary
,general
, andtitle
in the current _config.yaml. I have to clean up this aspect of the code, but I'm still working on that.
defaults:
prompt: bnotes
summary: cognitivetech/obook_summary:q6_k # default model for summaries
general: gemma2 # default model for basic summary
title: cognitivetech/obook_title:q4_k_m # default model for title generation
prompts:
bnotes: # Default Prompt
prompt: Write comprehensive bulleted notes summarizing the provided text, with
headings and terms in bold.
research: # Also for use with summary model
prompt: Does this text make any arguments? If so list them here.
clean: # The following prompts should be used with a general purpose model.
prompt: Repeat back this text exactly, remove only garbage characters that do
not contribute to the flow of text. Output only the main text content, condensed
onto a single line. If you encounter any chapter boundaries or subheadings,
start a new line beginning with its title.
concise:
prompt: Repeat the provided passage, with Concision.
md:
prompt: 'Print these notes in proper markdown format, with headings marked as
bold with double asterisks and terms in bold also, and bullet points as `-`.
Print the notes exactly, word-for-word, do not elaborate, do not add headings
with #'
sum: # basic
prompt: Comprehensive bulleted notes with headings and terms in bold.
teacher:
prompt: 'Write a list of questions that can be answered by 3rd graders who are
reading the provided text. Topics we like to focus on include: Main idea, supporting
details, Point of view, Theme, Sequence, Elements of fiction (setting, characters,
BME)'
quotes:
prompt: 'write a few dozen quotes inspired by the provided text'
title_generation:
prompt: Write a title with fewer than 11 words to concisely describe this selection.
python3 book2text.py ebook-name.epub # or ebook-name.pdf (Epub is preferred)
This step produces two outputs:
-
out/ebook-name.csv
(split by chapter or section) -
out/ebook-name_processed.csv
(chunked)
or
2. Remove or escape all newlines within each chunk, so they may be placed line by line in a text file, with each line surrounded by double quotes.
*Note to be cautious of properly escaping or replacing double quotes from within each chunk.
$``python3 sum.py --help
Usage: python sum.py [OPTIONS] input_file
Options:
-c, --csv Process a CSV file. Expected columns: Title, Text
-t, --txt Process a text file. Each line should be a separate text chunk.
-m, --model Model name to use for generation (default from config)
-p, --prompt Alias of the prompt to use from config (default from config)
-v, --verbose Print markdown output additionally to terminal
--help Show this help message and exit.
For CSV input:
- Ensure your CSV has 'Title' and 'Text' columns.
For Text input:
- Each line should be a chunk of text surrounded by double quote.
The output CSV will include:
- Title: Final title chosen or generated
- Gen: Boolean indicating if the title was generated
- Text: Original input text
- model_name: Generated output
- Time: Processing time in seconds
- Len: Length of the output
If you have your defaults set, then all you need is to specify which type of input, manual text
, or automated csv
.
python3 sum.py -c ebook-name_processed.csv
In this example, I've used a prototype split_pdf.py to split the pdf not only by chapter but subsections (producing ebook-name_extracted.csv
), then manually process that output (using vscode) to place each chunk on a single line surrounded by double quotes.
Eventually that will be automated but provides challenges, which you will notice, that have prevented me from finishing that tool.
Split:
tools-prototype/split_pdf.py ebook-name.pdf # produces ebook-name_extracted.csv
Process:
python3 sum.py -t ebook-name_extracted.csv
This step generates two outputs:
-
ebook-name_extracted_processed_sum.md
(rendered markdown) -
ebook-name_extracted_processed_sum.csv
(csv with: input text, flattened md output, generation time, output length)
Download from one of two sources:
You can get any of them them right from ollama, template in all.
example: ollama pull obook_summary:q5_k_m
-
obook_summary - On Ollama.com
-
latest
• 7.7GB • Q_8 -
q3_k_m
• 3.5GB -
q4_k_m
• 4.4GB -
q5_k_m
• 5.1GB -
q6_k
• 5.9GB
-
-
obook_title - On Ollama.com
-
latest
• 7.7GB • Q_8 -
q3_k_m
• 3.5GB -
q4_k_m
• 4.4GB -
q5_k_m
• 5.1GB -
q6_k
• 5.9GB
-
There is also complete weights, lora and ggguf on huggingface
- Mistral Instruct Bulleted Notes - Collection on HuggingFace
Here you can see how to check whethere your eBook as the proper formatting, or not. With ePub it should fail gracefully.
* In some rare occasion, even with clickable toc the script will not find that.
You are responsible for verifying that the summary tool creates an accurate summary. There are a variety of issues which can interfere with a quality summary, and if you aren't paying attention may slip your notice.
1. References:
Personally, I don't trust references from my fine-tuned model without verifying them manually. Maybe this is solved in newer models, but during my testing phase I noticed some bad references with 7b models I was using. I never tested this out to see the quality of the app on references, my personal preference is to remove any long references sections before summarizing, and deal with those separate. I don't think this is a permenant blocker, just an area that I haven't fully dealt with or understood, yet.
2. Other:
There are a few other things to watch out for.
One of the reasons I keep the length of the input and output on CSV is that makes it easy to check when a summary is longer than the input, thats a red flag.
when the structure of the summary greatly deviates from the others, this can indicate issues with the summary. Some of these can be realated to special characters, or if the input is too long and the AI just doesn't grasp it all.
The inspiration for this app was my intention to manually summarize a dozen books so I could tie together psychological theory and practice which they discuss and make a cohesive argument based on that information.
I've already read the books a few times, but now I need easy access to the information within so that I can relate it to others in a cohesive fashion.
Originally, after working at it this project manually, for a week, I was only a few chapters into my first book, I could see this was going to take a loong time.
Over the next 6 months I began learning how to use LLM, discovering were the best for my task, with fine-tuning to deliver production quality consistency in the results.
Now with this tool, I'm able to review a lot more material more quickly. This is a content curation tool that empowers me to not only learn things but more readily share that knowledge, without having to spend ages that it takes to create quality content.
Moreover, it can be used to create custom datasets based on whatever source materials you throw at it.
For Tasks:
Click tags to check more tools for each tasksFor Jobs:
Alternative AI tools for ollama-ebook-summary
Similar Open Source Tools
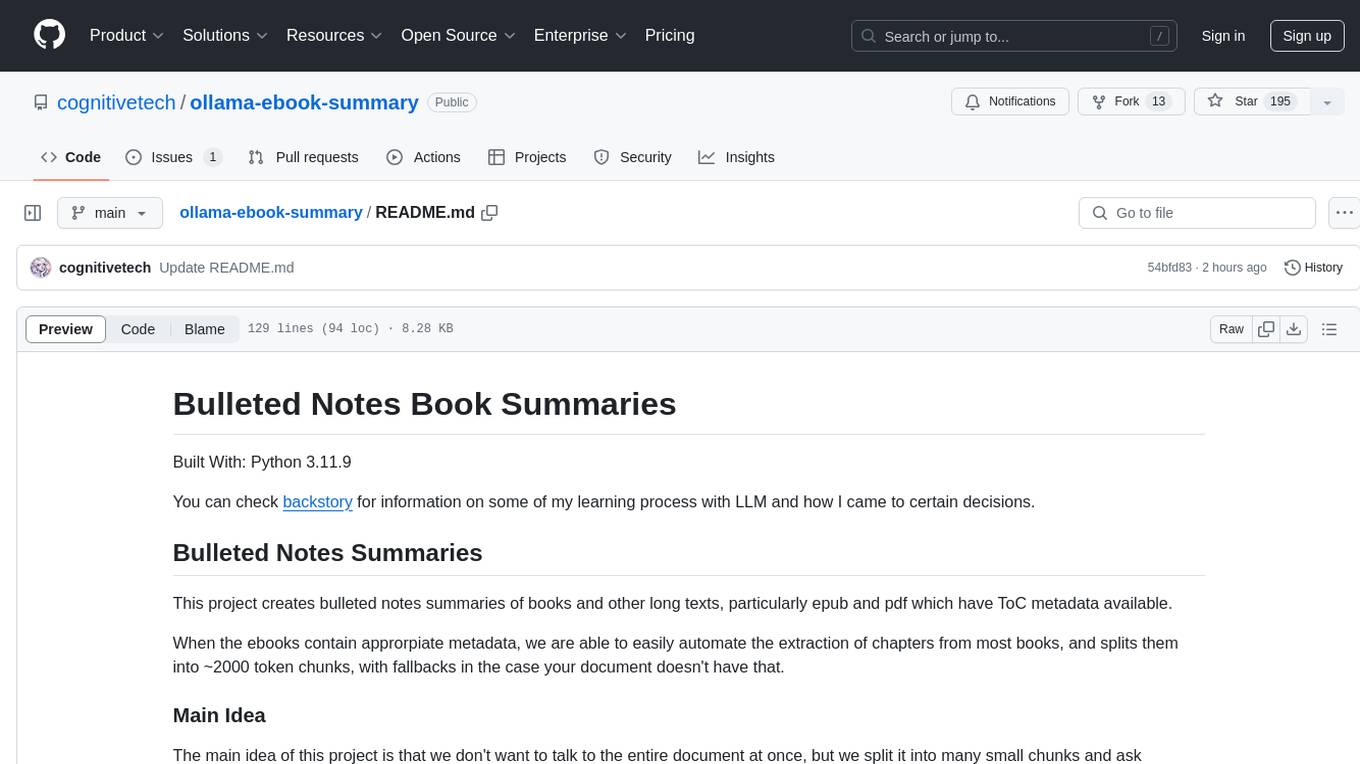
ollama-ebook-summary
The 'ollama-ebook-summary' repository is a Python project that creates bulleted notes summaries of books and long texts, particularly in epub and pdf formats with ToC metadata. It automates the extraction of chapters, splits them into ~2000 token chunks, and allows for asking arbitrary questions to parts of the text for improved granularity of response. The tool aims to provide summaries for each page of a book rather than a one-page summary of the entire document, enhancing content curation and knowledge sharing capabilities.
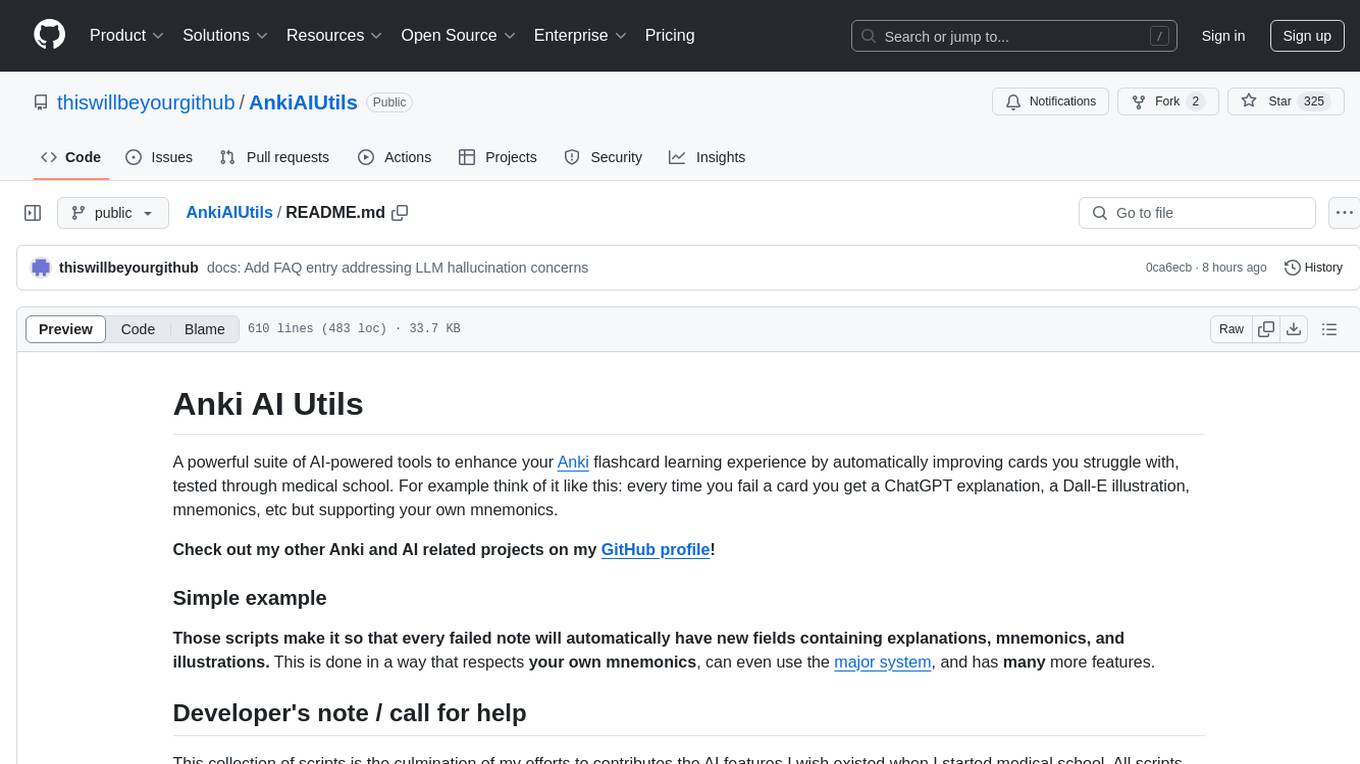
AnkiAIUtils
Anki AI Utils is a powerful suite of AI-powered tools designed to enhance your Anki flashcard learning experience by automatically improving cards you struggle with. The tools include features such as adaptive learning, personalized memory hooks, automation readiness, universal compatibility, provider agnosticism, and infinite extensibility. The toolkit consists of tools like Illustrator for creating custom mnemonic images, Reformulator for rephrasing flashcards, Mnemonics Creator for generating memorable mnemonics, Explainer for providing detailed explanations, and Mnemonics Helper for quick mnemonic generation. The project aims to motivate others to package the tools into addons for wider accessibility.
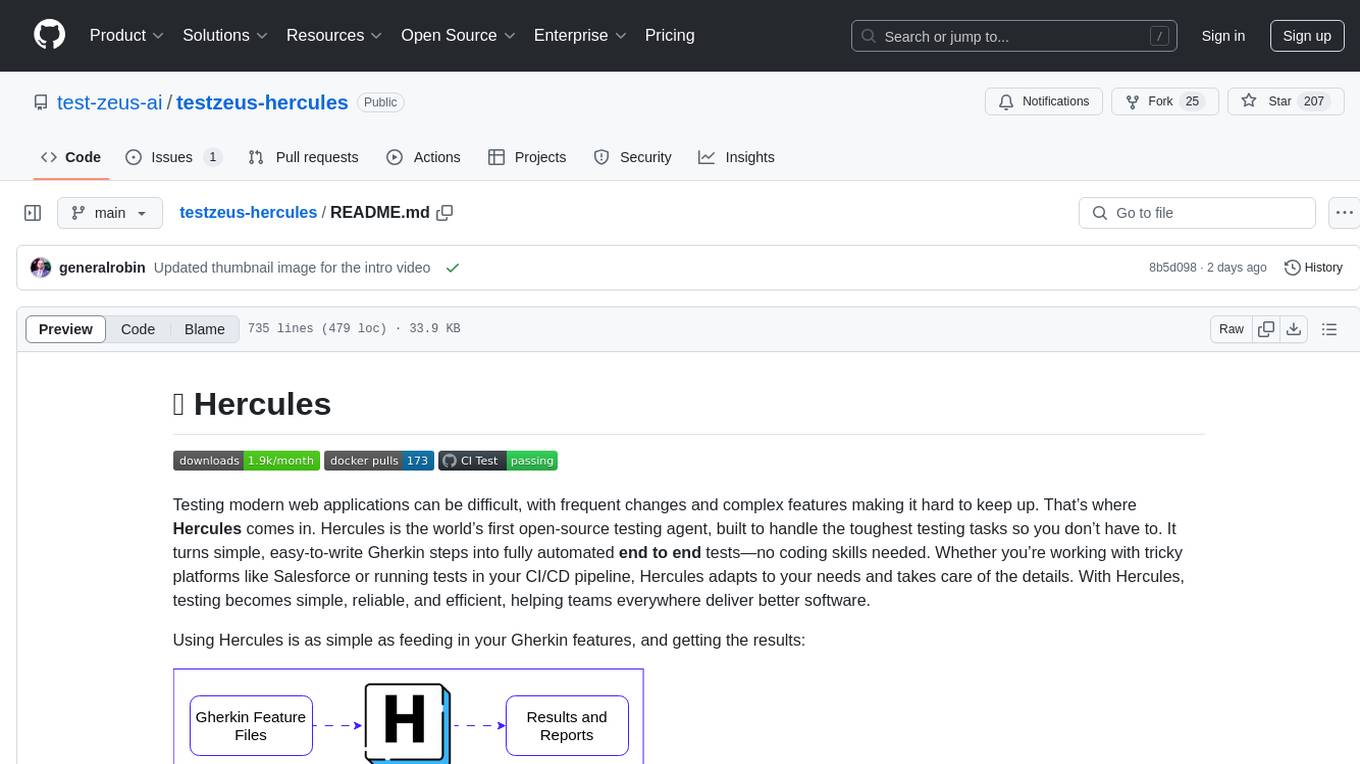
testzeus-hercules
Hercules is the world’s first open-source testing agent designed to handle the toughest testing tasks for modern web applications. It turns simple Gherkin steps into fully automated end-to-end tests, making testing simple, reliable, and efficient. Hercules adapts to various platforms like Salesforce and is suitable for CI/CD pipelines. It aims to democratize and disrupt test automation, making top-tier testing accessible to everyone. The tool is transparent, reliable, and community-driven, empowering teams to deliver better software. Hercules offers multiple ways to get started, including using PyPI package, Docker, or building and running from source code. It supports various AI models, provides detailed installation and usage instructions, and integrates with Nuclei for security testing and WCAG for accessibility testing. The tool is production-ready, open core, and open source, with plans for enhanced LLM support, advanced tooling, improved DOM distillation, community contributions, extensive documentation, and a bounty program.
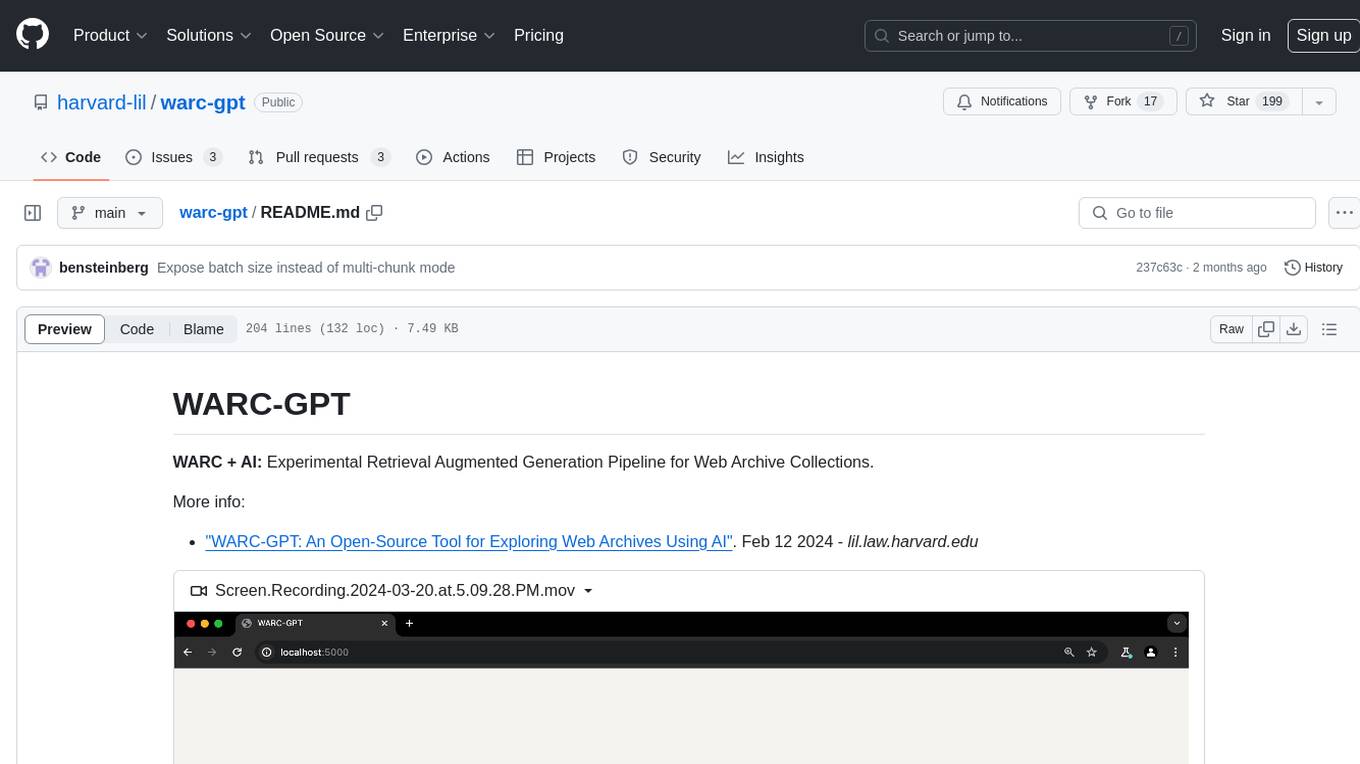
warc-gpt
WARC-GPT is an experimental retrieval augmented generation pipeline for web archive collections. It allows users to interact with WARC files, extract text, generate text embeddings, visualize embeddings, and interact with a web UI and API. The tool is highly customizable, supporting various LLMs, providers, and embedding models. Users can configure the application using environment variables, ingest WARC files, start the server, and interact with the web UI and API to search for content and generate text completions. WARC-GPT is designed for exploration and experimentation in exploring web archives using AI.
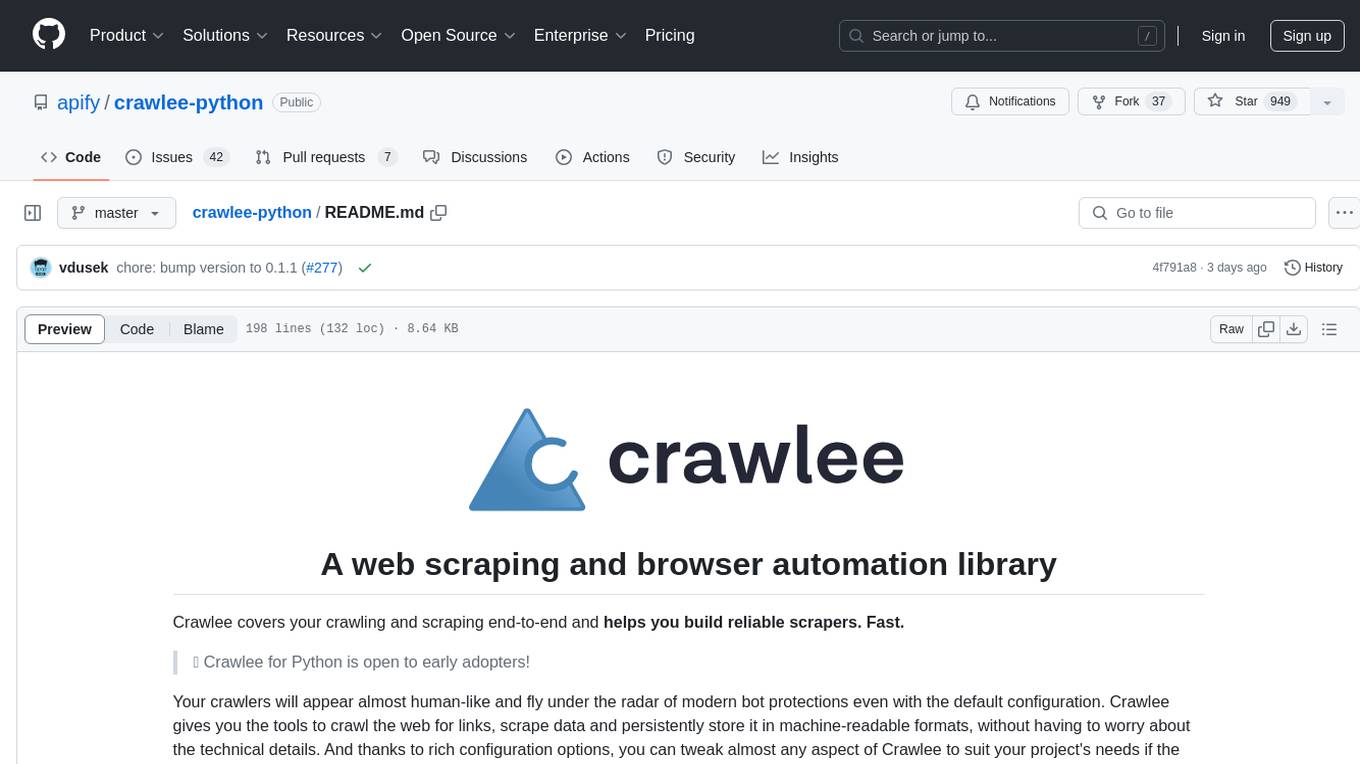
crawlee-python
Crawlee-python is a web scraping and browser automation library that covers crawling and scraping end-to-end, helping users build reliable scrapers fast. It allows users to crawl the web for links, scrape data, and store it in machine-readable formats without worrying about technical details. With rich configuration options, users can customize almost any aspect of Crawlee to suit their project's needs.
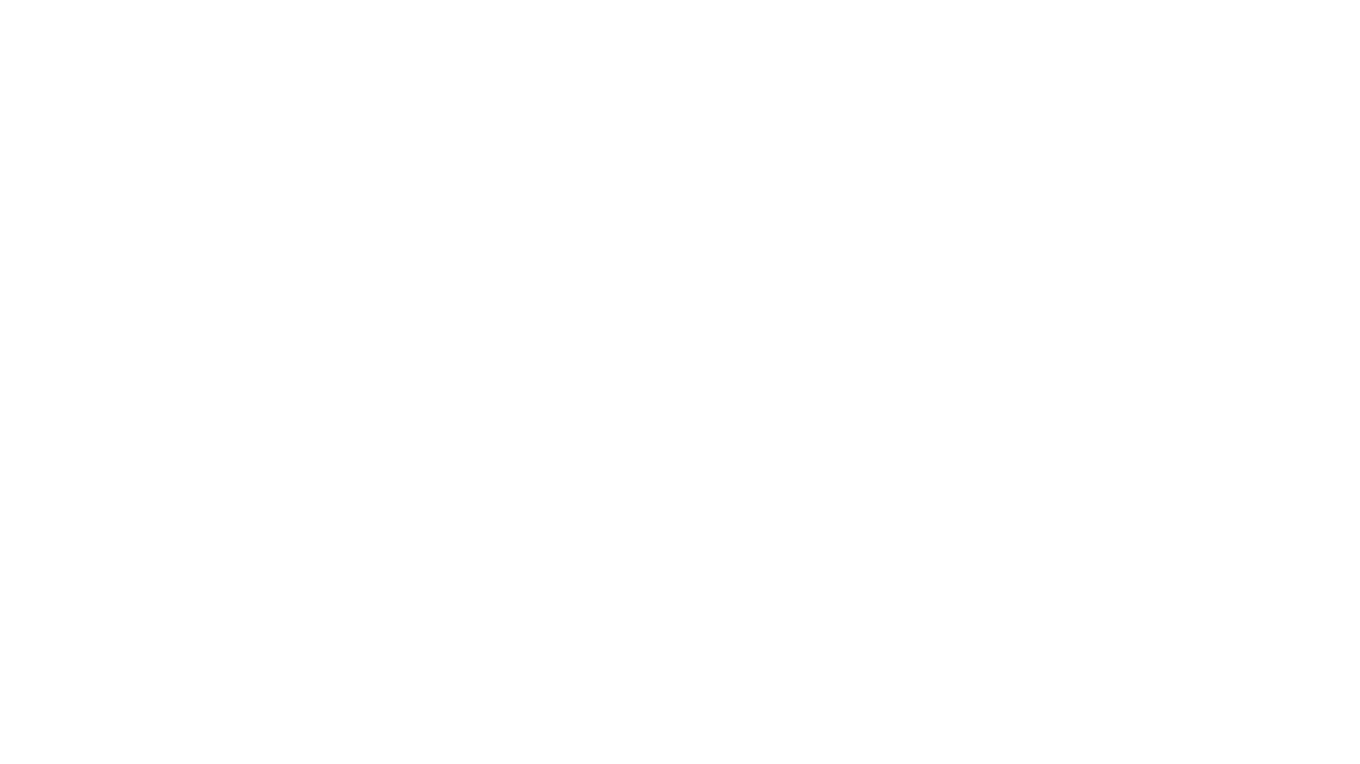
reader
Reader is a tool that converts any URL to an LLM-friendly input with a simple prefix `https://r.jina.ai/`. It improves the output for your agent and RAG systems at no cost. Reader supports image reading, captioning all images at the specified URL and adding `Image [idx]: [caption]` as an alt tag. This enables downstream LLMs to interact with the images in reasoning, summarizing, etc. Reader offers a streaming mode, useful when the standard mode provides an incomplete result. In streaming mode, Reader waits a bit longer until the page is fully rendered, providing more complete information. Reader also supports a JSON mode, which contains three fields: `url`, `title`, and `content`. Reader is backed by Jina AI and licensed under Apache-2.0.
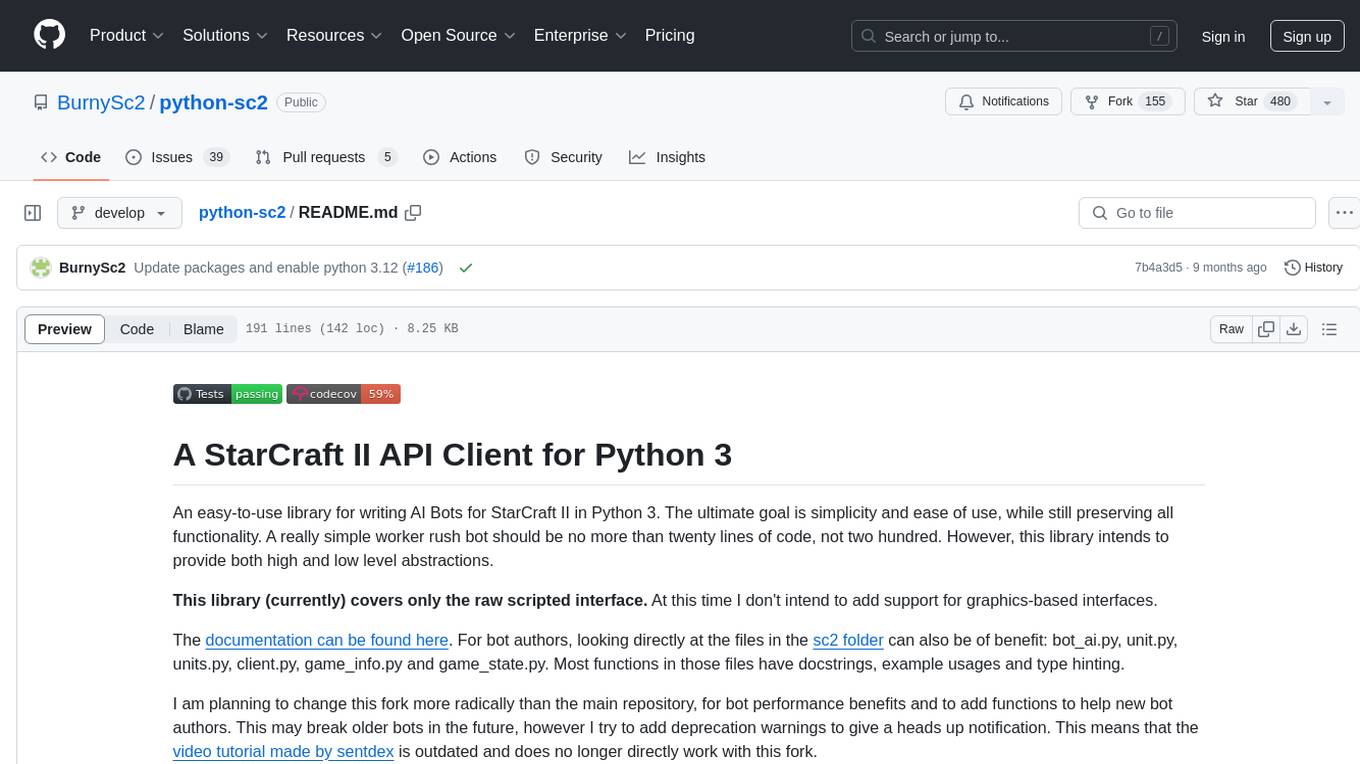
python-sc2
python-sc2 is an easy-to-use library for writing AI Bots for StarCraft II in Python 3. It aims for simplicity and ease of use while providing both high and low level abstractions. The library covers only the raw scripted interface and intends to help new bot authors with added functions. Users can install the library using pip and need a StarCraft II executable to run bots. The API configuration options allow users to customize bot behavior and performance. The community provides support through Discord servers, and users can contribute to the project by creating new issues or pull requests following style guidelines.
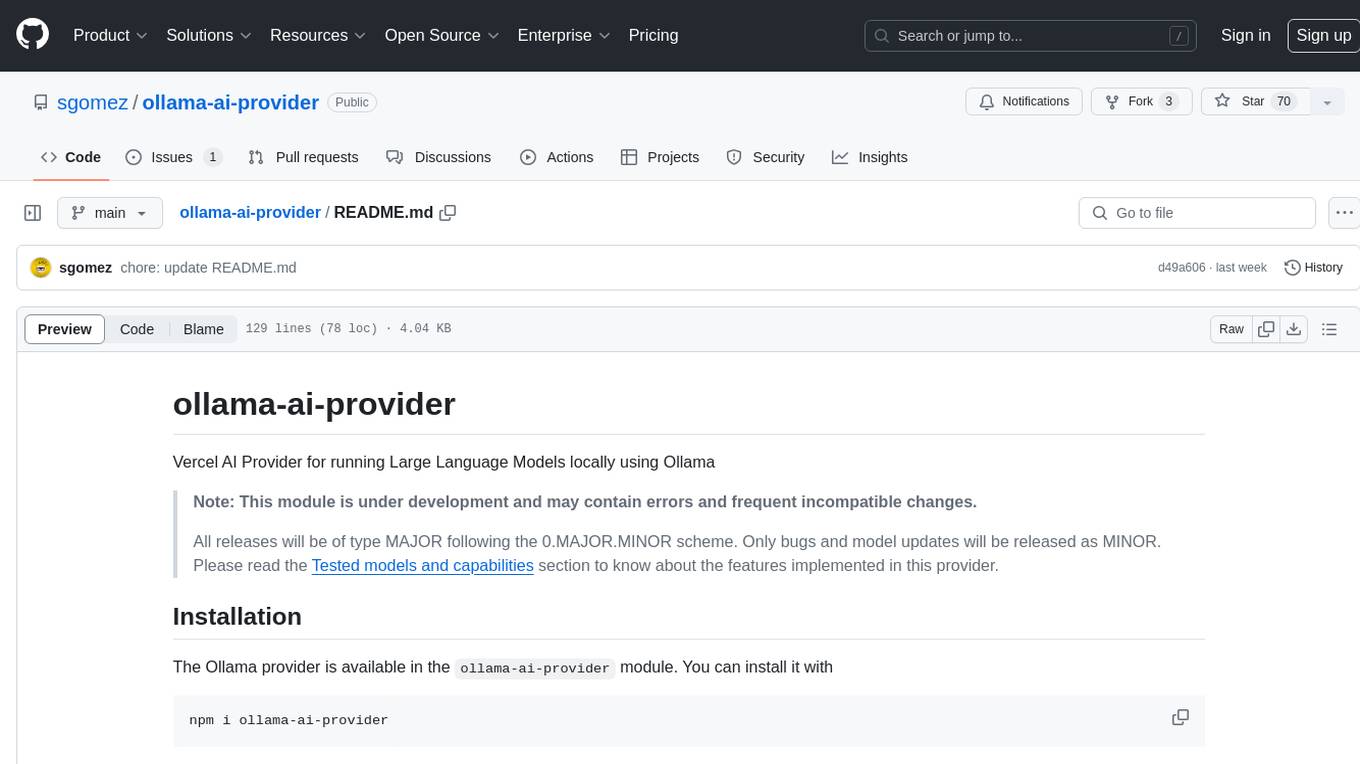
ollama-ai-provider
Vercel AI Provider for running Large Language Models locally using Ollama. This module is under development and may contain errors and frequent incompatible changes. It provides the capability of generating and streaming text and objects, with features like image input, object generation, tool usage simulation, tool streaming simulation, intercepting fetch requests, and provider management. The provider can be customized with optional settings like baseURL and headers.
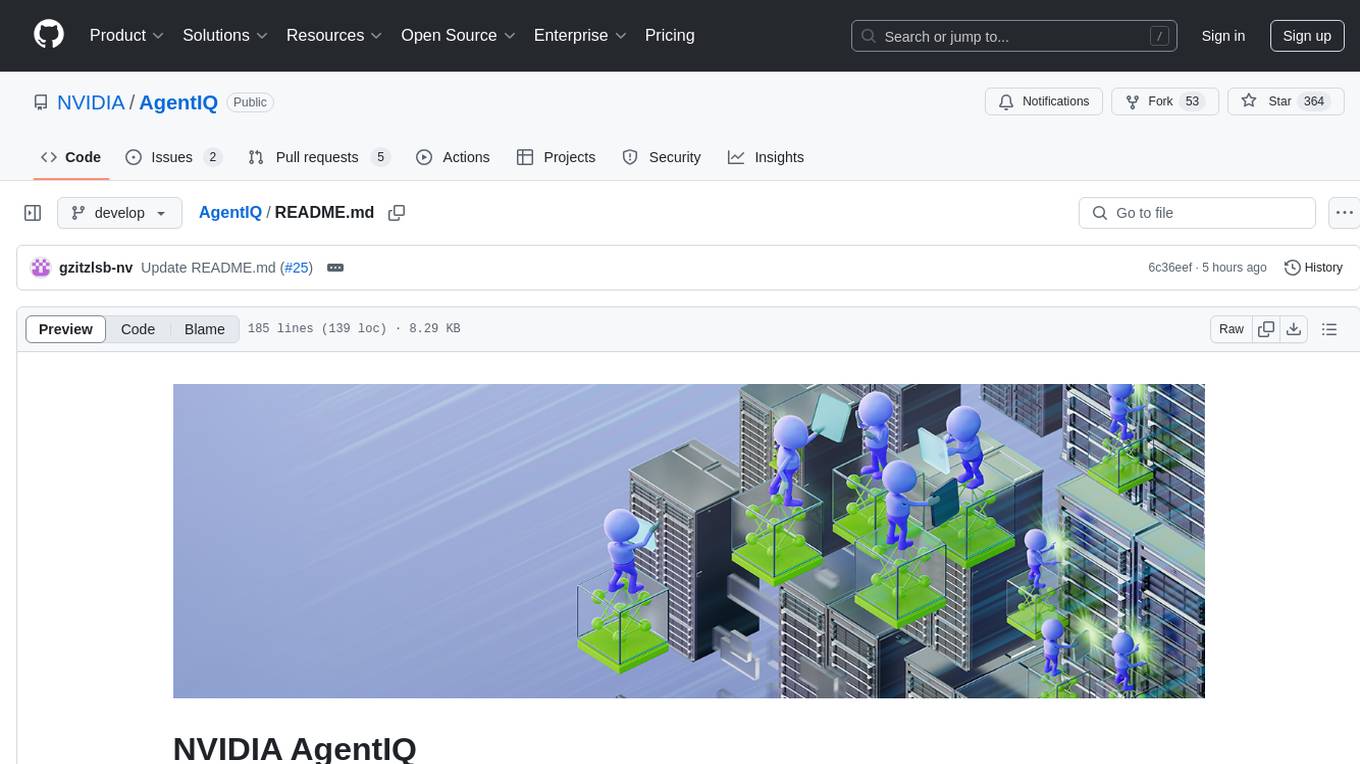
AgentIQ
AgentIQ is a flexible library designed to seamlessly integrate enterprise agents with various data sources and tools. It enables true composability by treating agents, tools, and workflows as simple function calls. With features like framework agnosticism, reusability, rapid development, profiling, observability, evaluation system, user interface, and MCP compatibility, AgentIQ empowers developers to move quickly, experiment freely, and ensure reliability across agent-driven projects.
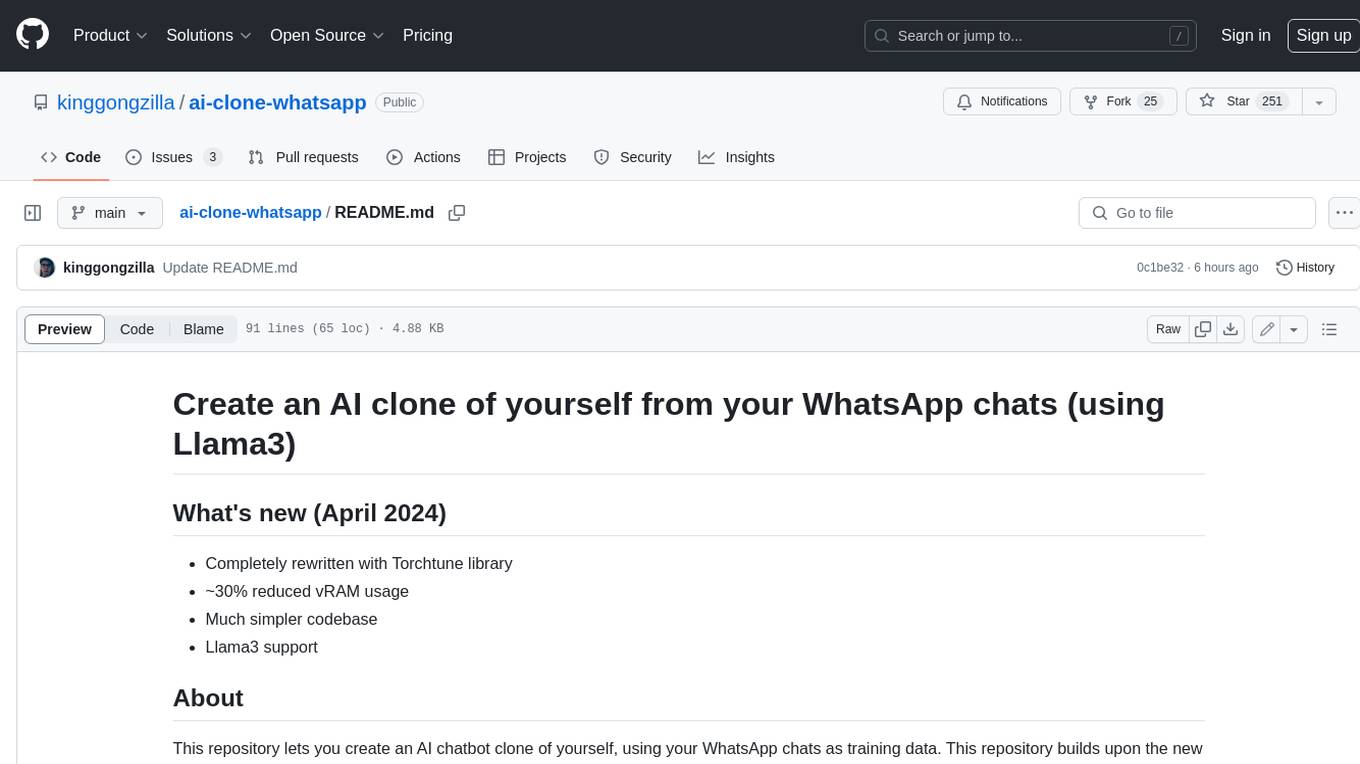
ai-clone-whatsapp
This repository provides a tool to create an AI chatbot clone of yourself using your WhatsApp chats as training data. It utilizes the Torchtune library for finetuning and inference. The code includes preprocessing of WhatsApp chats, finetuning models, and chatting with the AI clone via a command-line interface. Supported models are Llama3-8B-Instruct and Mistral-7B-Instruct-v0.2. Hardware requirements include approximately 16 GB vRAM for QLoRa Llama3 finetuning with a 4k context length. The repository addresses common issues like adjusting parameters for training and preprocessing non-English chats.
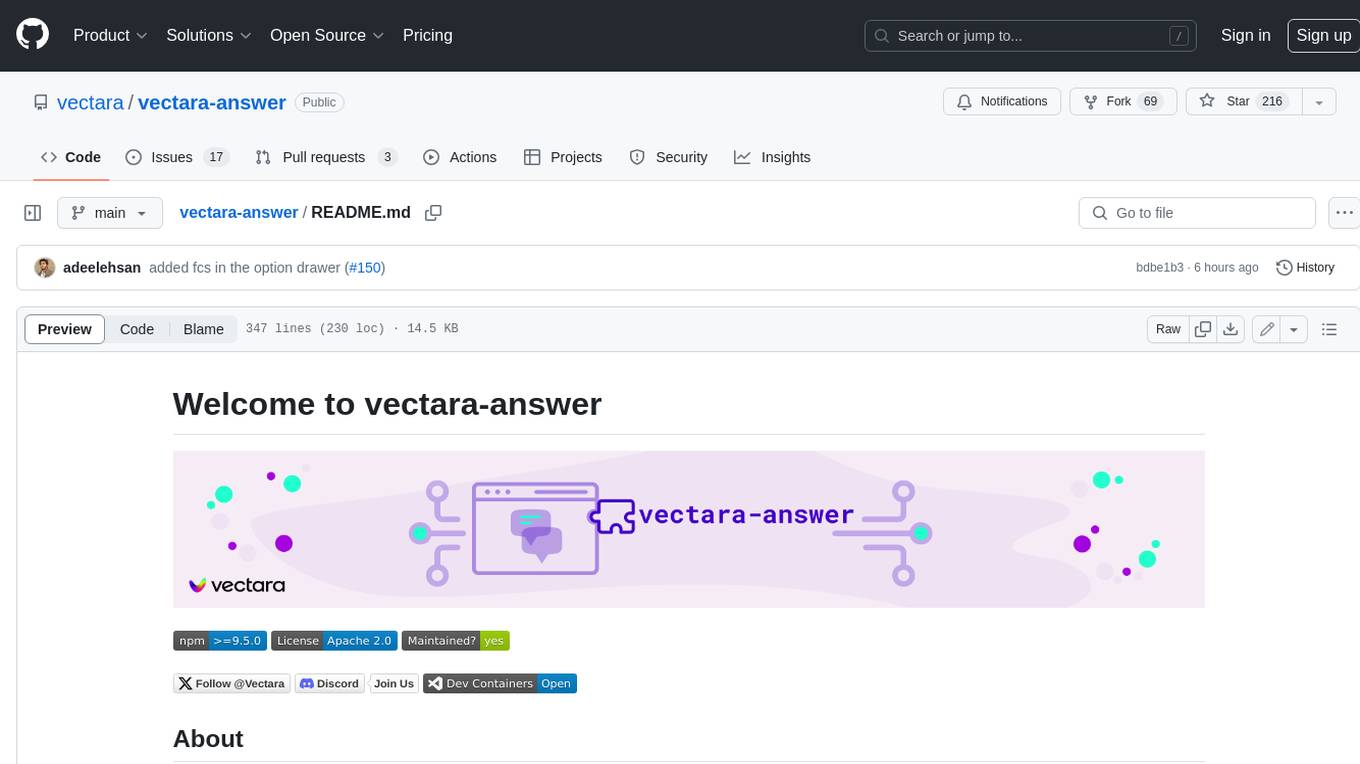
vectara-answer
Vectara Answer is a sample app for Vectara-powered Summarized Semantic Search (or question-answering) with advanced configuration options. For examples of what you can build with Vectara Answer, check out Ask News, LegalAid, or any of the other demo applications.
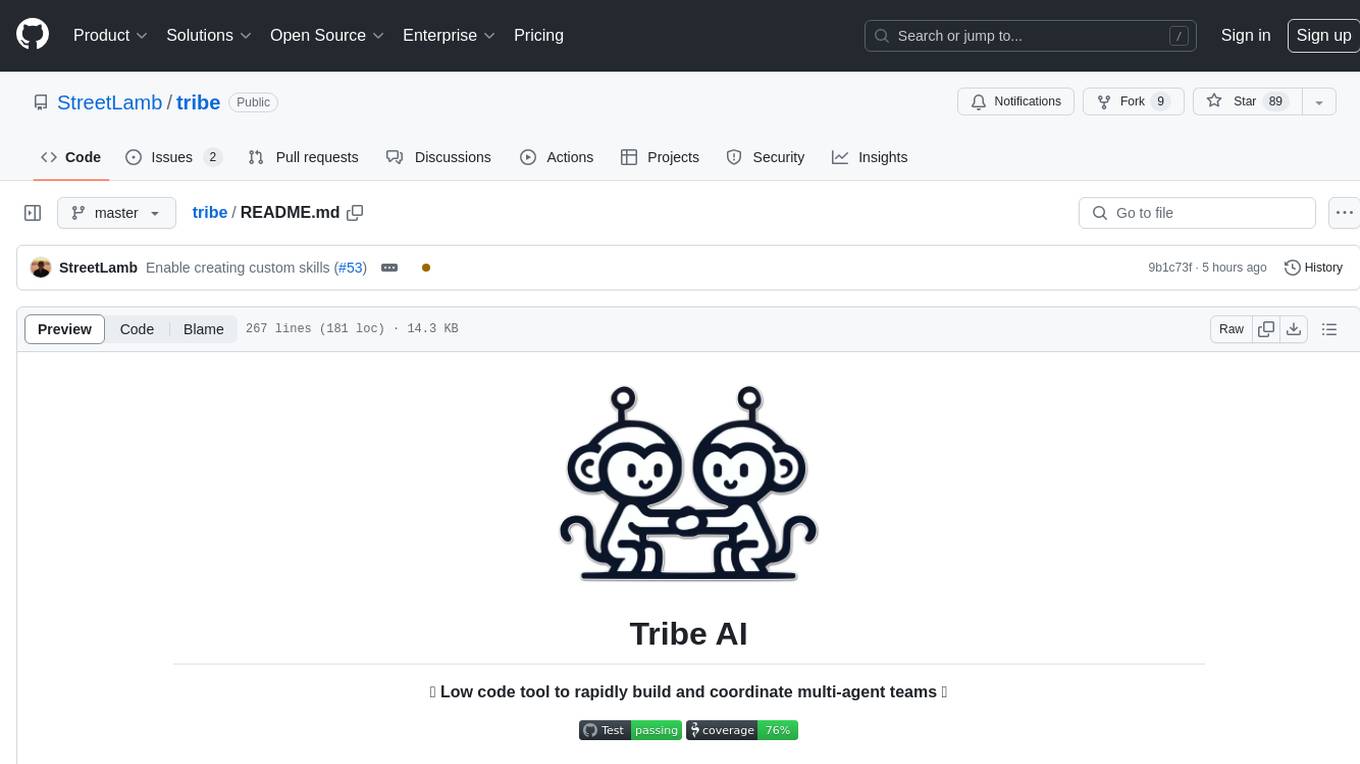
tribe
Tribe AI is a low code tool designed to rapidly build and coordinate multi-agent teams. It leverages the langgraph framework to customize and coordinate teams of agents, allowing tasks to be split among agents with different strengths for faster and better problem-solving. The tool supports persistent conversations, observability, tool calling, human-in-the-loop functionality, easy deployment with Docker, and multi-tenancy for managing multiple users and teams.
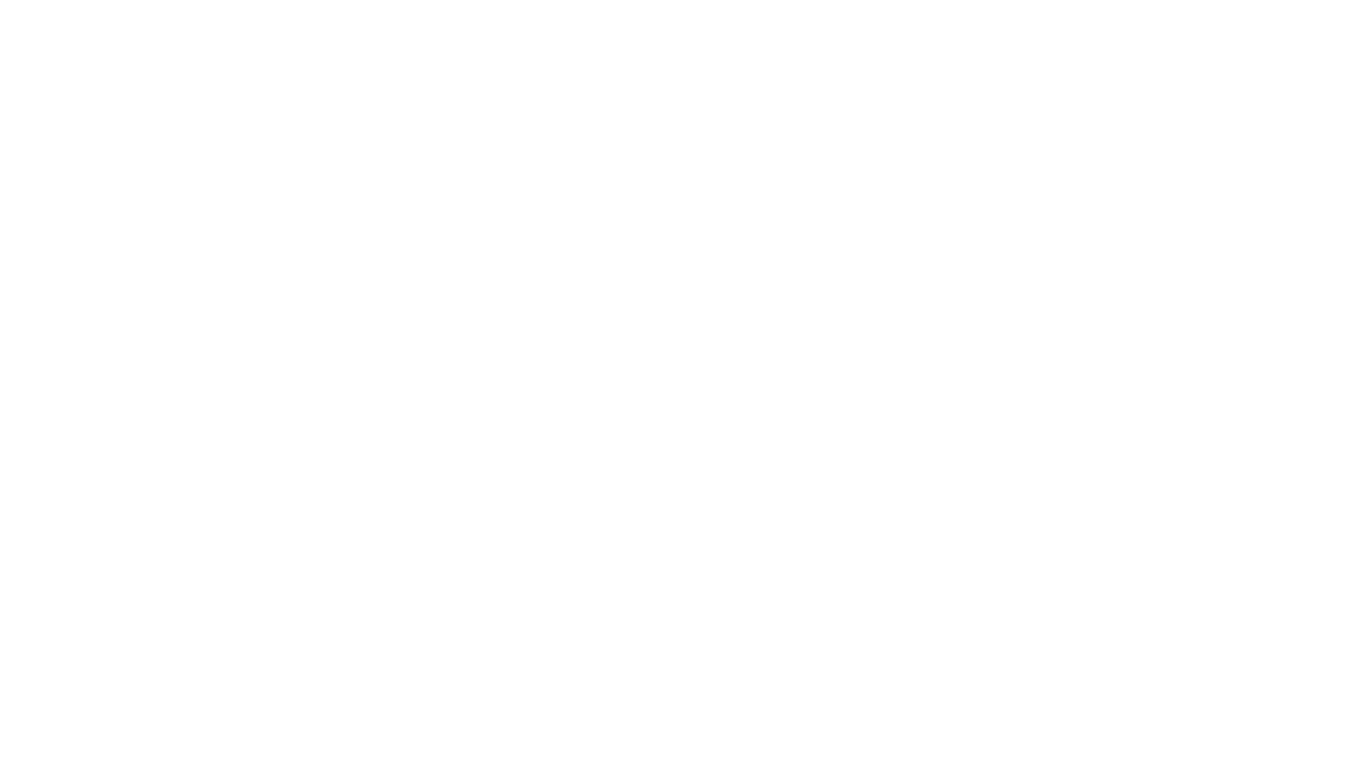
aider-composer
Aider Composer is a VSCode extension that integrates Aider into your development workflow. It allows users to easily add and remove files, toggle between read-only and editable modes, review code changes, use different chat modes, and reference files in the chat. The extension supports multiple models, code generation, code snippets, and settings customization. It has limitations such as lack of support for multiple workspaces, Git repository features, linting, testing, voice features, in-chat commands, and configuration options.
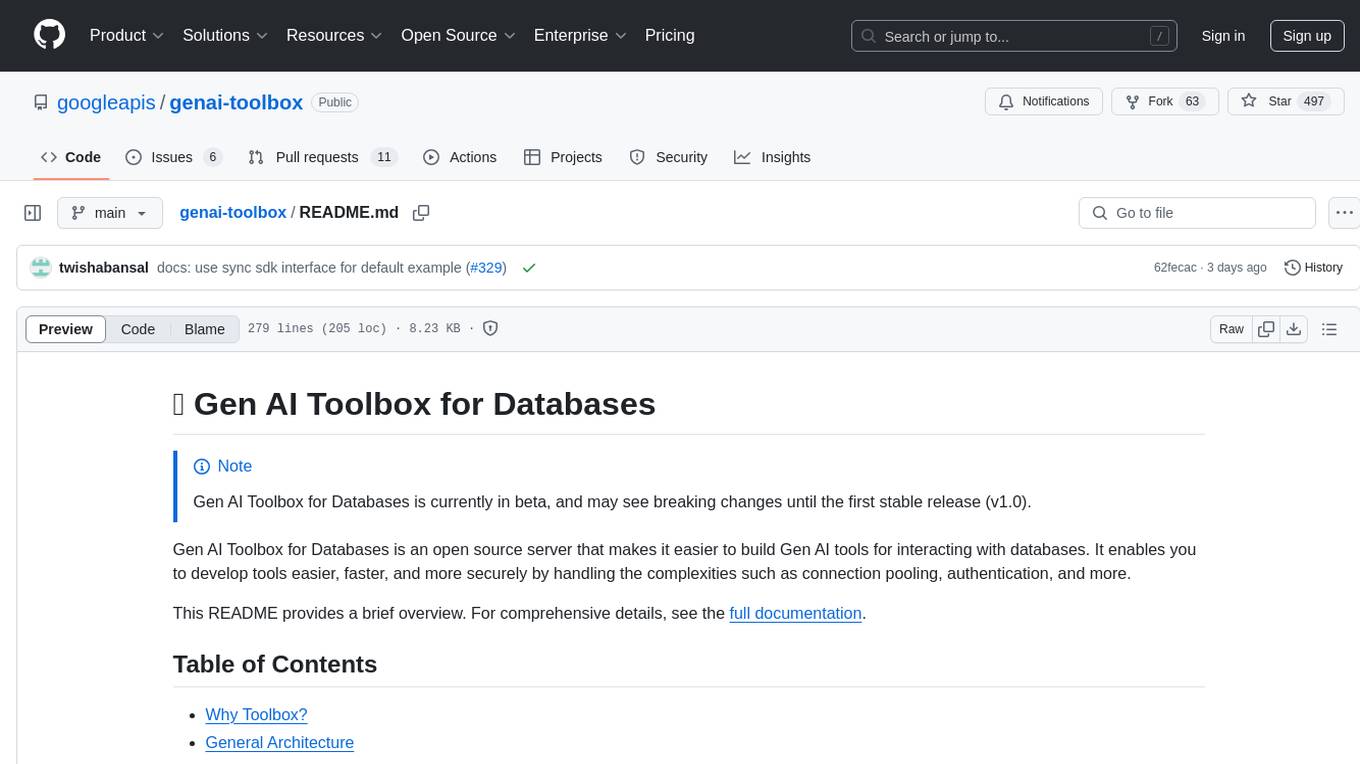
genai-toolbox
Gen AI Toolbox for Databases is an open source server that simplifies building Gen AI tools for interacting with databases. It handles complexities like connection pooling, authentication, and more, enabling easier, faster, and more secure tool development. The toolbox sits between the application's orchestration framework and the database, providing a control plane to modify, distribute, or invoke tools. It offers simplified development, better performance, enhanced security, and end-to-end observability. Users can install the toolbox as a binary, container image, or compile from source. Configuration is done through a 'tools.yaml' file, defining sources, tools, and toolsets. The project follows semantic versioning and welcomes contributions.
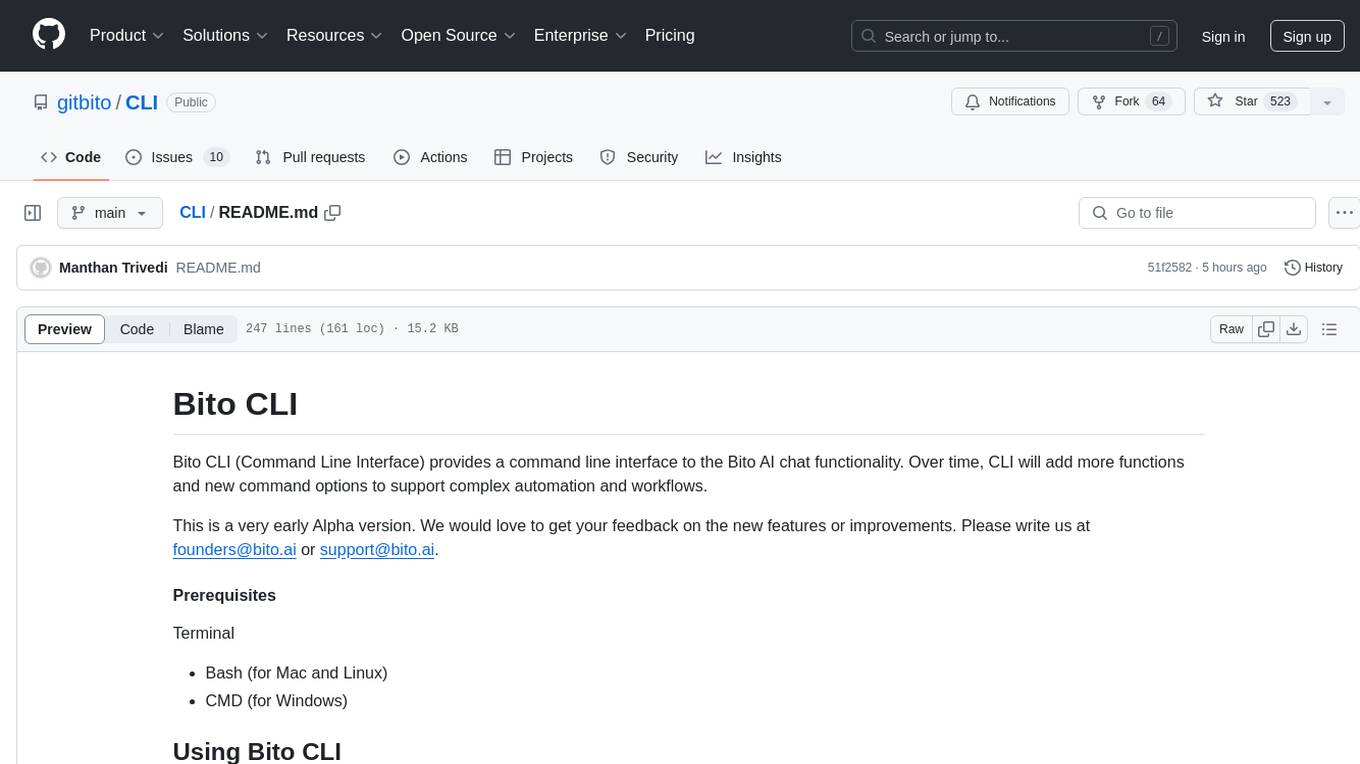
CLI
Bito CLI provides a command line interface to the Bito AI chat functionality, allowing users to interact with the AI through commands. It supports complex automation and workflows, with features like long prompts and slash commands. Users can install Bito CLI on Mac, Linux, and Windows systems using various methods. The tool also offers configuration options for AI model type, access key management, and output language customization. Bito CLI is designed to enhance user experience in querying AI models and automating tasks through the command line interface.
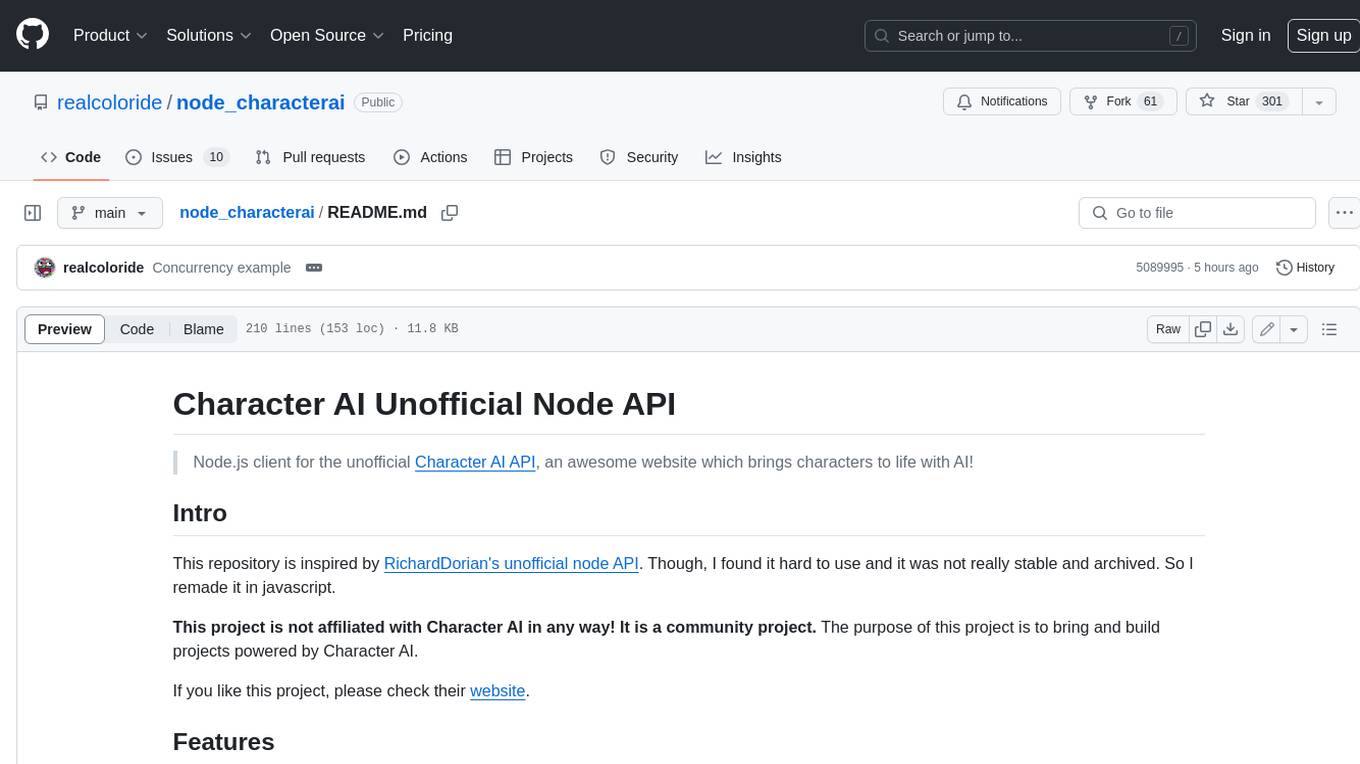
node_characterai
Node.js client for the unofficial Character AI API, an awesome website which brings characters to life with AI! This repository is inspired by RichardDorian's unofficial node API. Though, I found it hard to use and it was not really stable and archived. So I remade it in javascript. This project is not affiliated with Character AI in any way! It is a community project. The purpose of this project is to bring and build projects powered by Character AI. If you like this project, please check their website.
For similar tasks
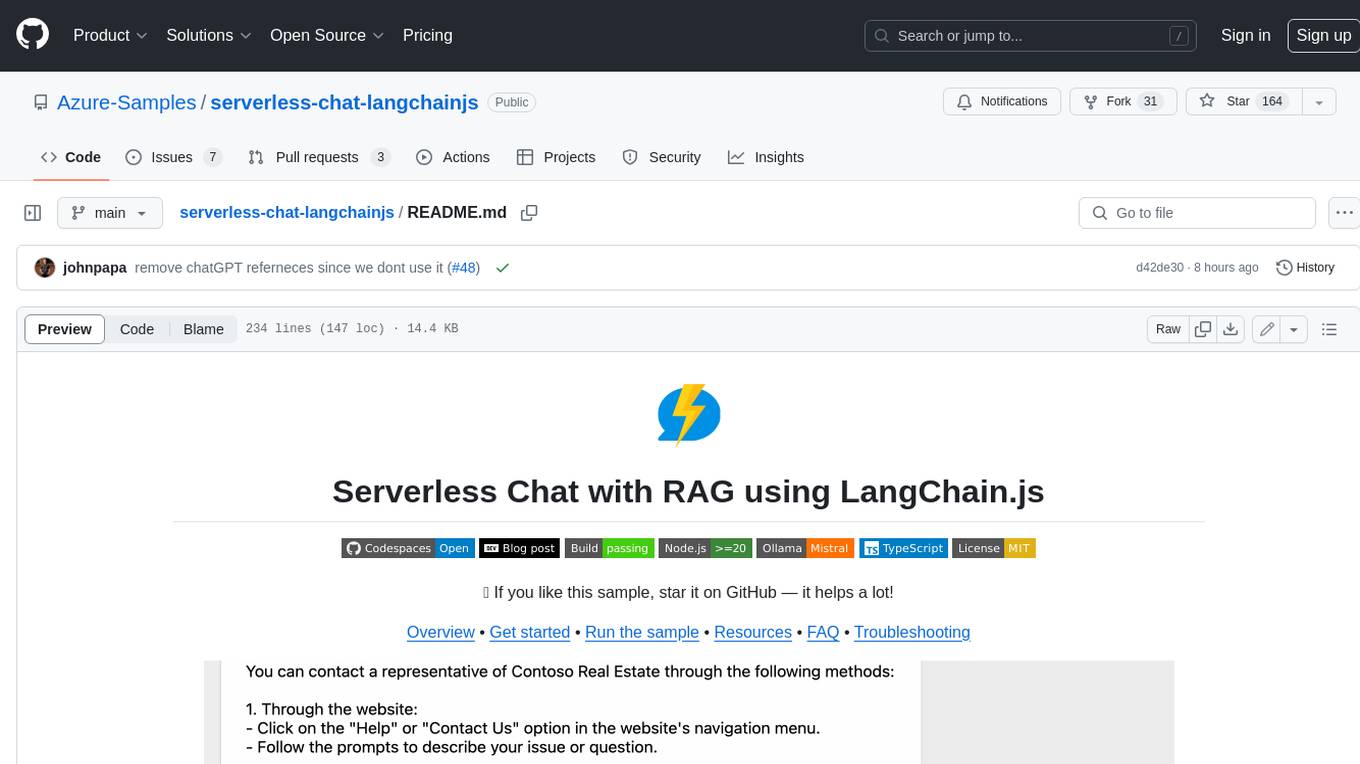
serverless-chat-langchainjs
This sample shows how to build a serverless chat experience with Retrieval-Augmented Generation using LangChain.js and Azure. The application is hosted on Azure Static Web Apps and Azure Functions, with Azure Cosmos DB for MongoDB vCore as the vector database. You can use it as a starting point for building more complex AI applications.
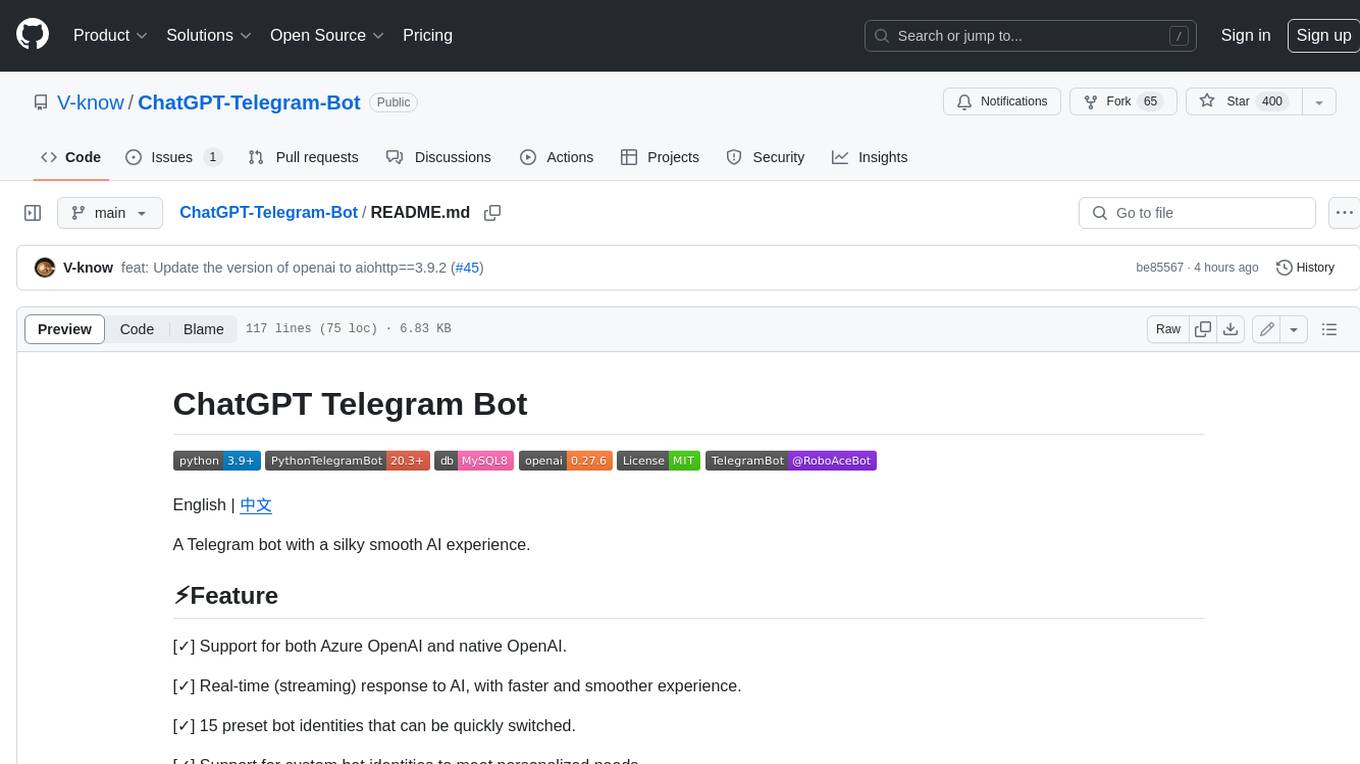
ChatGPT-Telegram-Bot
ChatGPT Telegram Bot is a Telegram bot that provides a smooth AI experience. It supports both Azure OpenAI and native OpenAI, and offers real-time (streaming) response to AI, with a faster and smoother experience. The bot also has 15 preset bot identities that can be quickly switched, and supports custom bot identities to meet personalized needs. Additionally, it supports clearing the contents of the chat with a single click, and restarting the conversation at any time. The bot also supports native Telegram bot button support, making it easy and intuitive to implement required functions. User level division is also supported, with different levels enjoying different single session token numbers, context numbers, and session frequencies. The bot supports English and Chinese on UI, and is containerized for easy deployment.
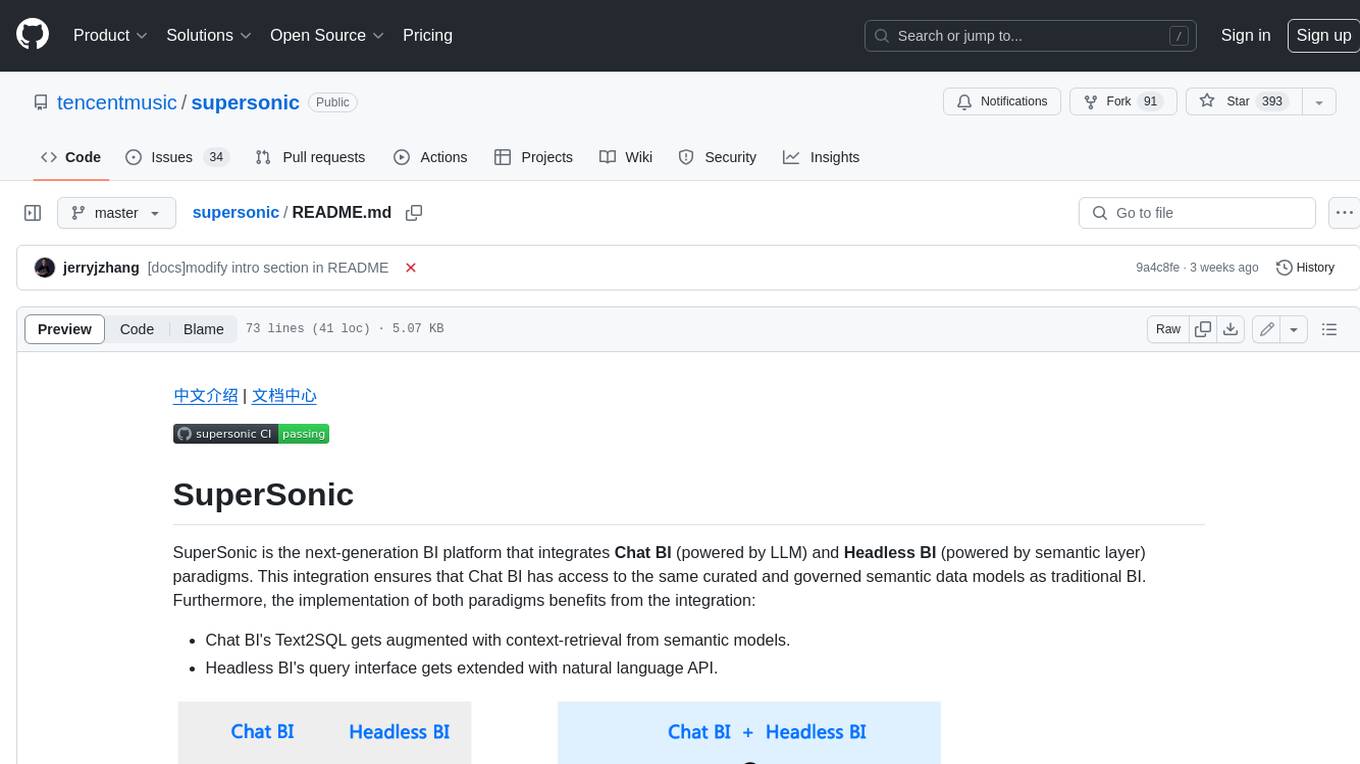
supersonic
SuperSonic is a next-generation BI platform that integrates Chat BI (powered by LLM) and Headless BI (powered by semantic layer) paradigms. This integration ensures that Chat BI has access to the same curated and governed semantic data models as traditional BI. Furthermore, the implementation of both paradigms benefits from the integration: * Chat BI's Text2SQL gets augmented with context-retrieval from semantic models. * Headless BI's query interface gets extended with natural language API. SuperSonic provides a Chat BI interface that empowers users to query data using natural language and visualize the results with suitable charts. To enable such experience, the only thing necessary is to build logical semantic models (definition of metric/dimension/tag, along with their meaning and relationships) through a Headless BI interface. Meanwhile, SuperSonic is designed to be extensible and composable, allowing custom implementations to be added and configured with Java SPI. The integration of Chat BI and Headless BI has the potential to enhance the Text2SQL generation in two dimensions: 1. Incorporate data semantics (such as business terms, column values, etc.) into the prompt, enabling LLM to better understand the semantics and reduce hallucination. 2. Offload the generation of advanced SQL syntax (such as join, formula, etc.) from LLM to the semantic layer to reduce complexity. With these ideas in mind, we develop SuperSonic as a practical reference implementation and use it to power our real-world products. Additionally, to facilitate further development we decide to open source SuperSonic as an extensible framework.
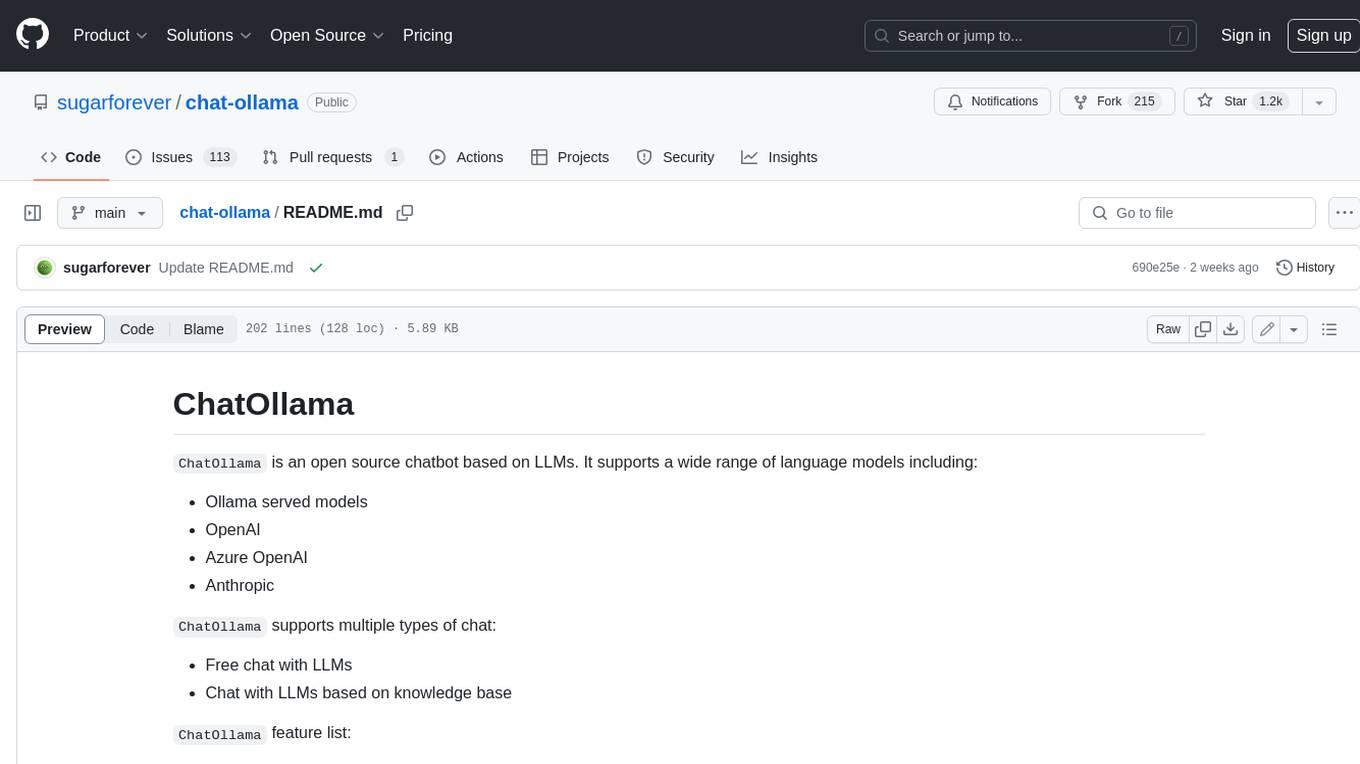
chat-ollama
ChatOllama is an open-source chatbot based on LLMs (Large Language Models). It supports a wide range of language models, including Ollama served models, OpenAI, Azure OpenAI, and Anthropic. ChatOllama supports multiple types of chat, including free chat with LLMs and chat with LLMs based on a knowledge base. Key features of ChatOllama include Ollama models management, knowledge bases management, chat, and commercial LLMs API keys management.
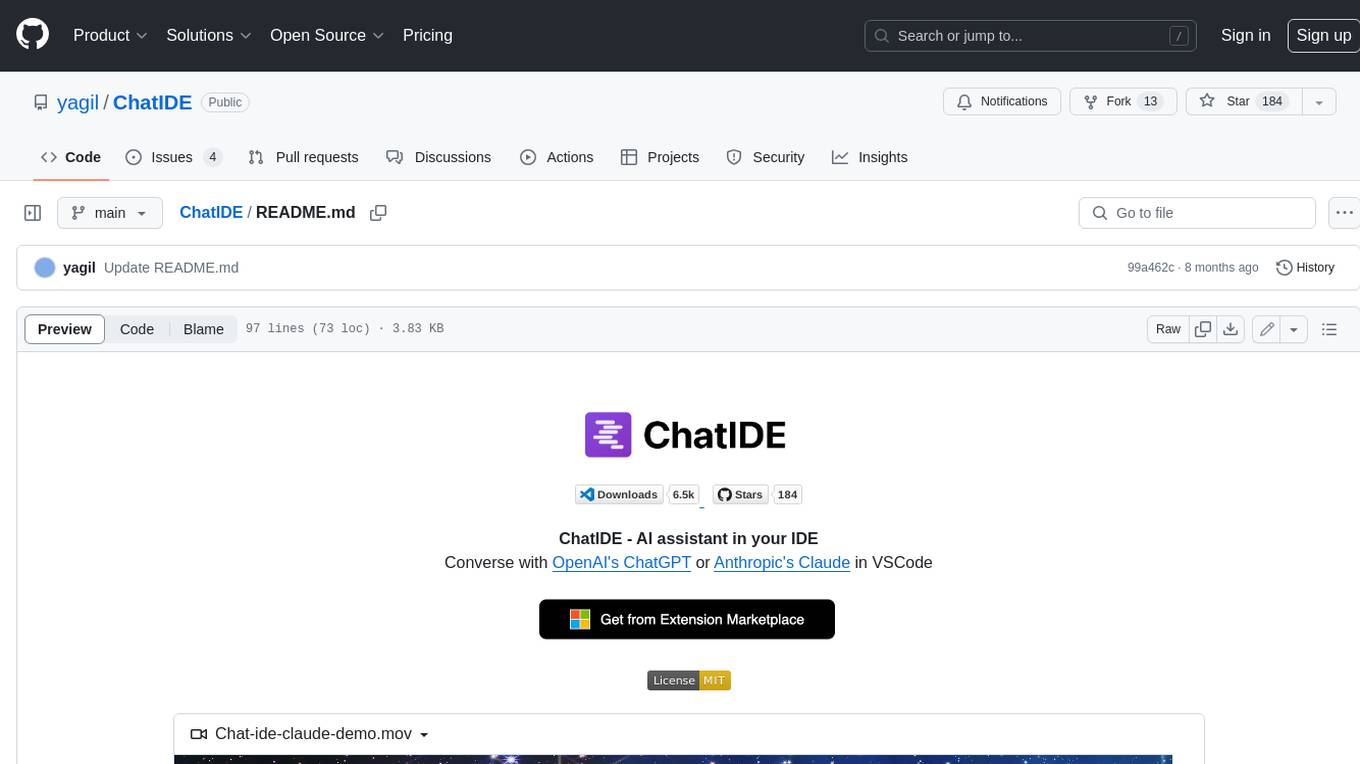
ChatIDE
ChatIDE is an AI assistant that integrates with your IDE, allowing you to converse with OpenAI's ChatGPT or Anthropic's Claude within your development environment. It provides a seamless way to access AI-powered assistance while coding, enabling you to get real-time help, generate code snippets, debug errors, and brainstorm ideas without leaving your IDE.
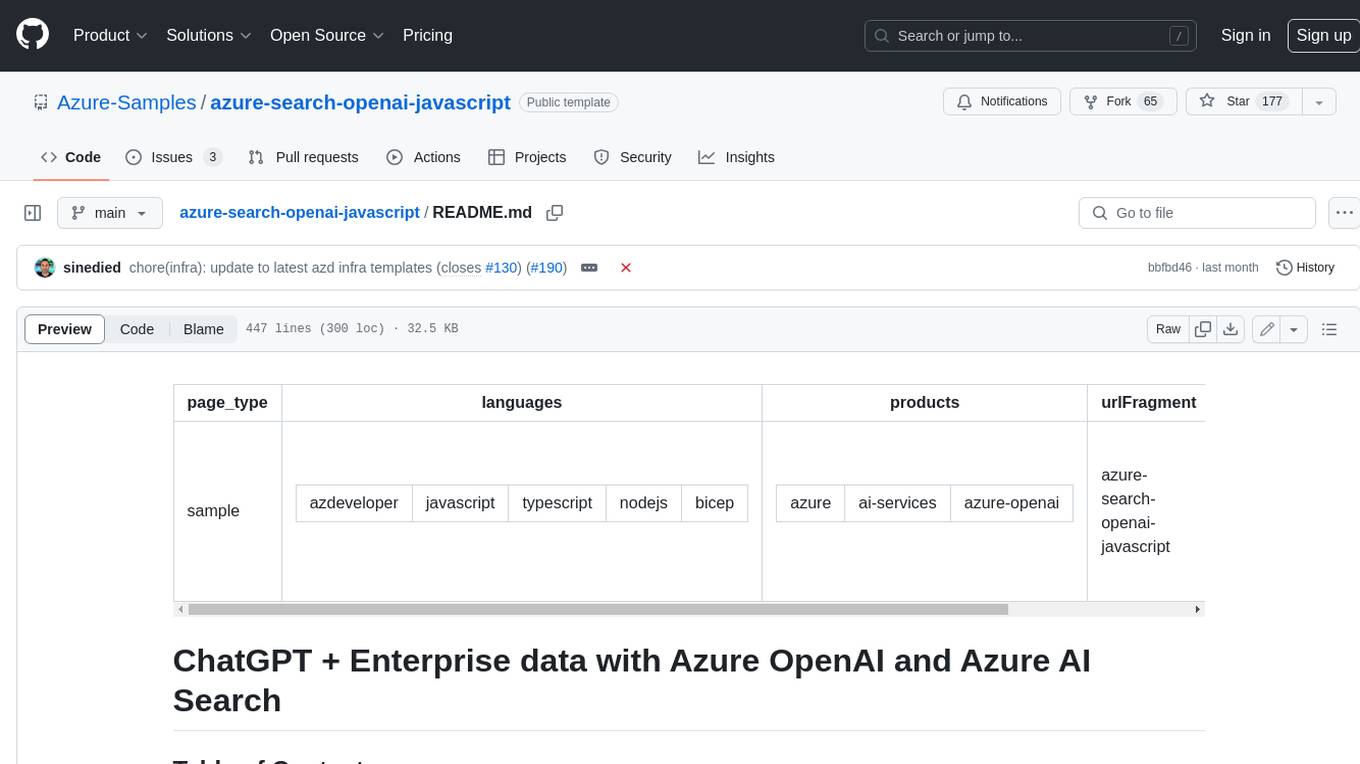
azure-search-openai-javascript
This sample demonstrates a few approaches for creating ChatGPT-like experiences over your own data using the Retrieval Augmented Generation pattern. It uses Azure OpenAI Service to access the ChatGPT model (gpt-35-turbo), and Azure AI Search for data indexing and retrieval.
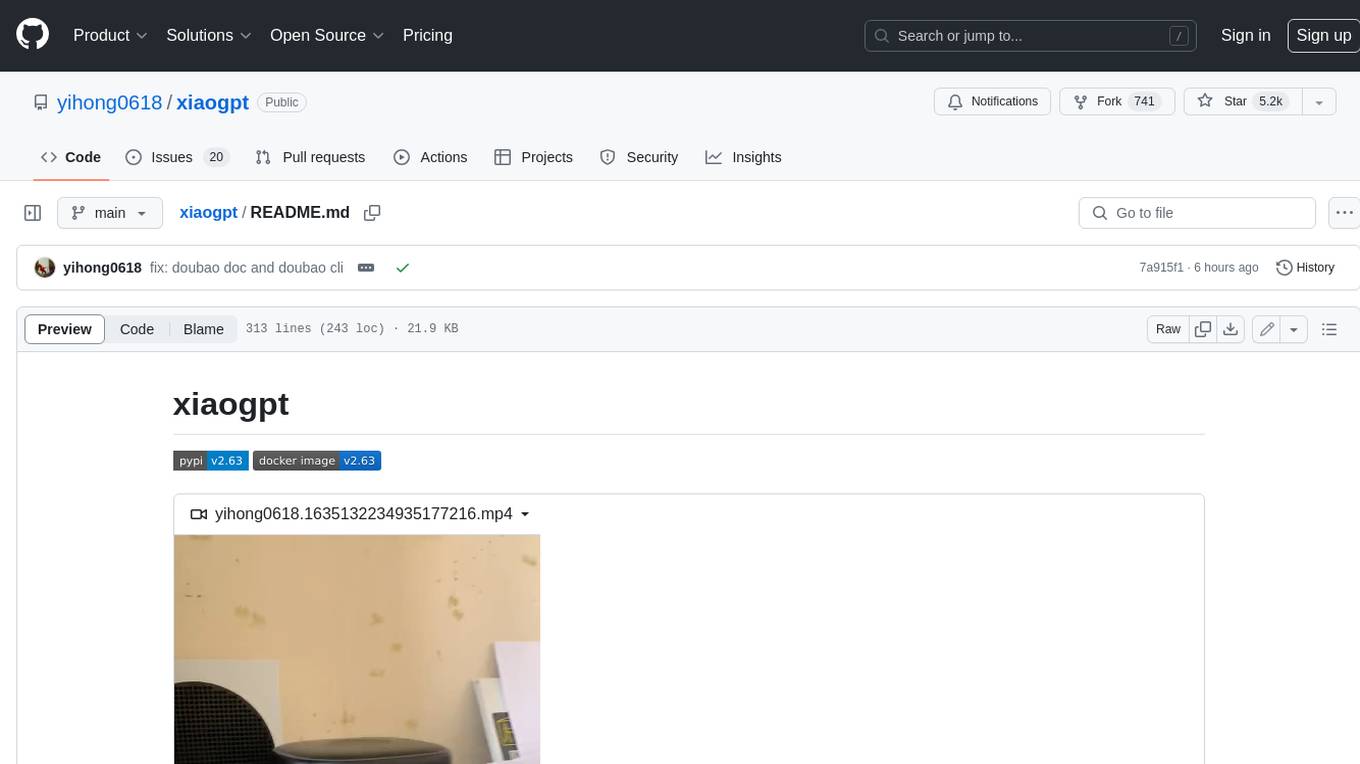
xiaogpt
xiaogpt is a tool that allows you to play ChatGPT and other LLMs with Xiaomi AI Speaker. It supports ChatGPT, New Bing, ChatGLM, Gemini, Doubao, and Tongyi Qianwen. You can use it to ask questions, get answers, and have conversations with AI assistants. xiaogpt is easy to use and can be set up in a few minutes. It is a great way to experience the power of AI and have fun with your Xiaomi AI Speaker.
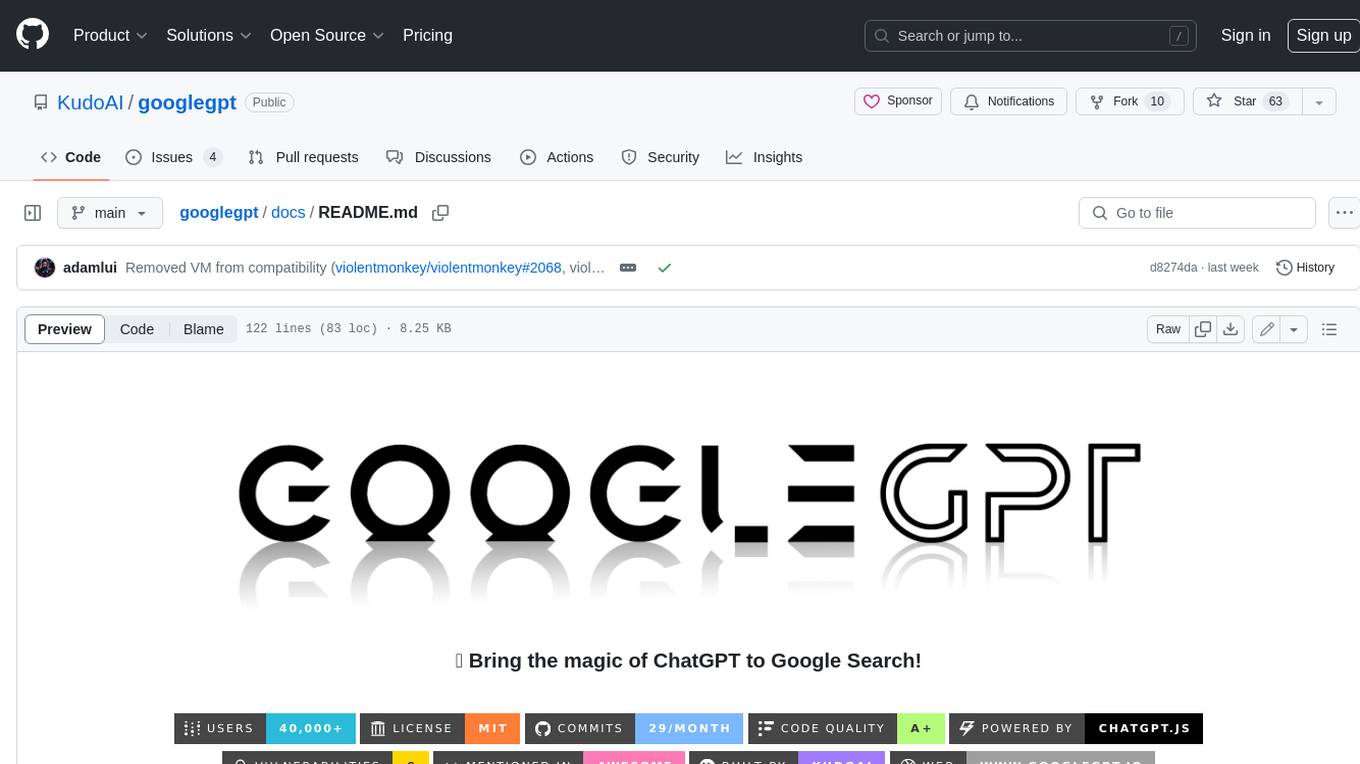
googlegpt
GoogleGPT is a browser extension that brings the power of ChatGPT to Google Search. With GoogleGPT, you can ask ChatGPT questions and get answers directly in your search results. You can also use GoogleGPT to generate text, translate languages, and more. GoogleGPT is compatible with all major browsers, including Chrome, Firefox, Edge, and Safari.
For similar jobs
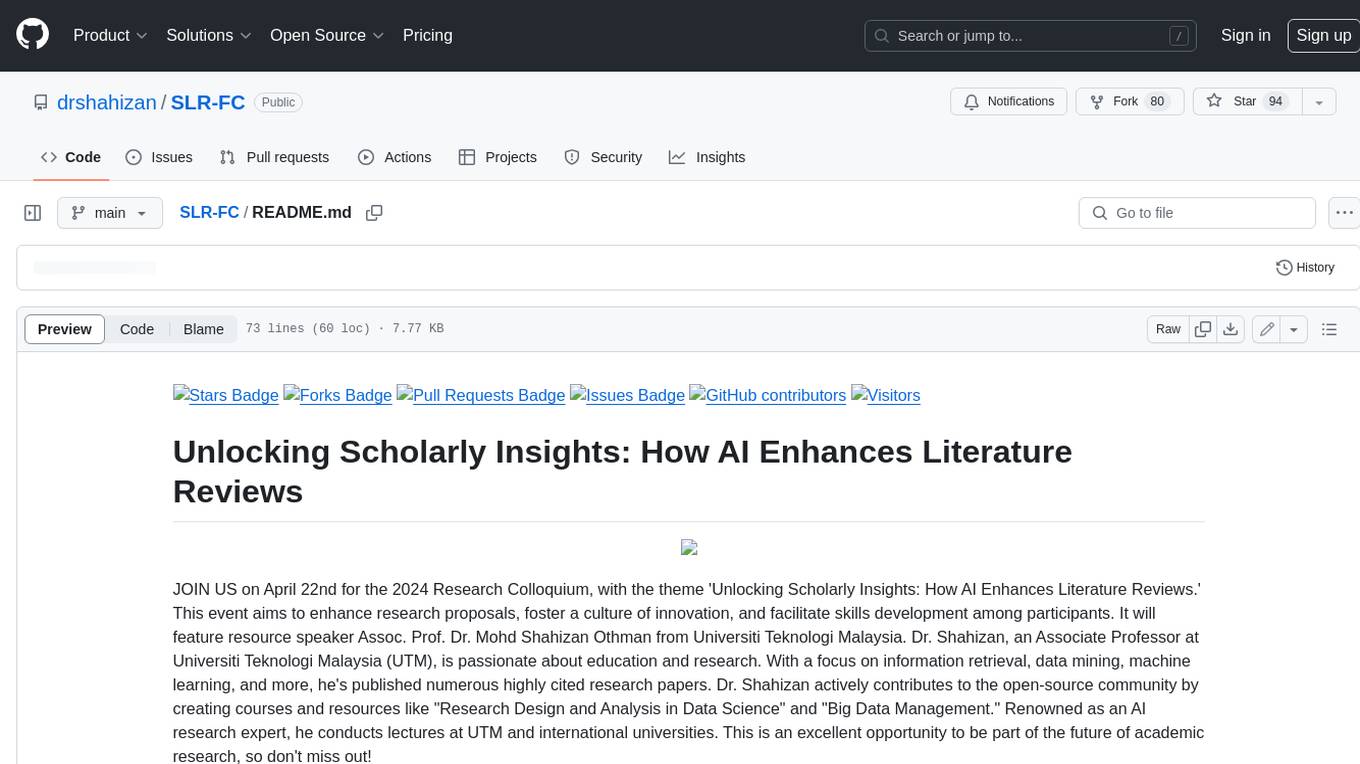
SLR-FC
This repository provides a comprehensive collection of AI tools and resources to enhance literature reviews. It includes a curated list of AI tools for various tasks, such as identifying research gaps, discovering relevant papers, visualizing paper content, and summarizing text. Additionally, the repository offers materials on generative AI, effective prompts, copywriting, image creation, and showcases of AI capabilities. By leveraging these tools and resources, researchers can streamline their literature review process, gain deeper insights from scholarly literature, and improve the quality of their research outputs.
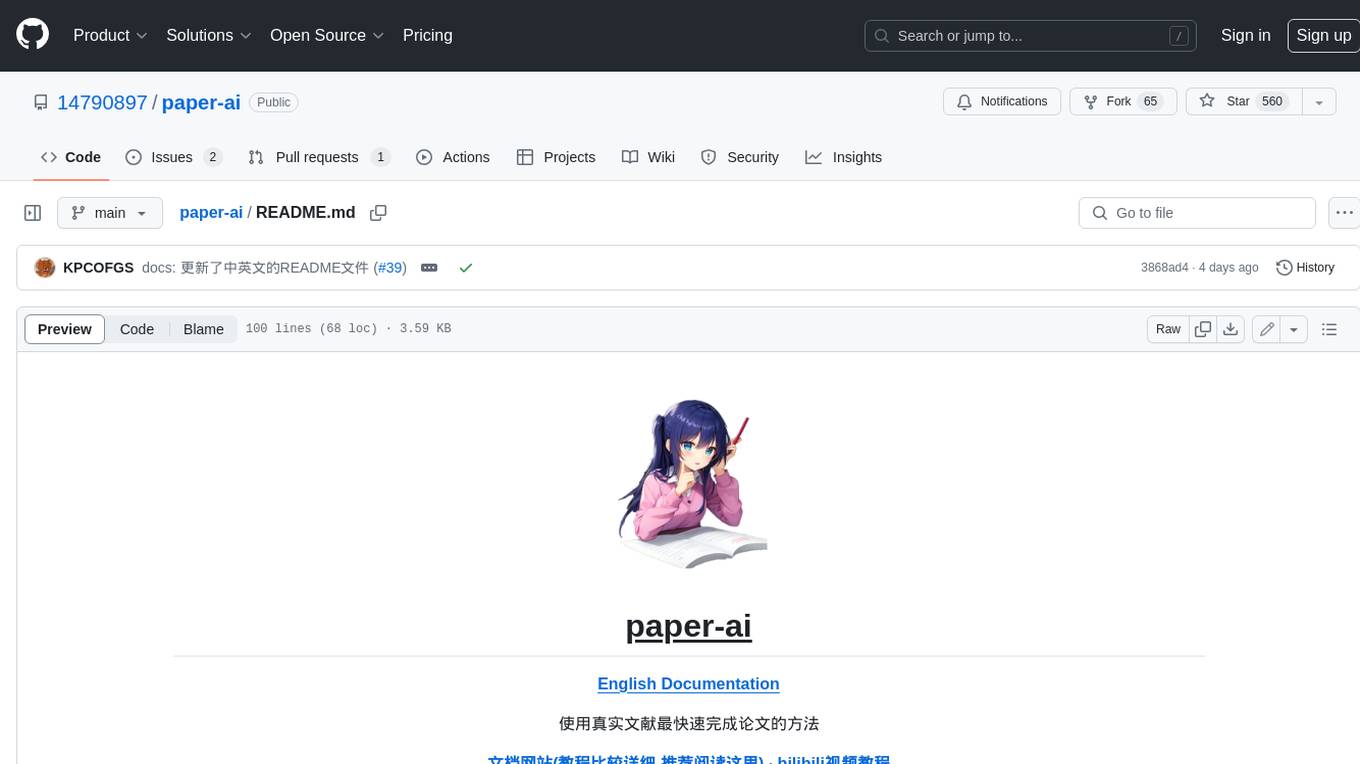
paper-ai
Paper-ai is a tool that helps you write papers using artificial intelligence. It provides features such as AI writing assistance, reference searching, and editing and formatting tools. With Paper-ai, you can quickly and easily create high-quality papers.
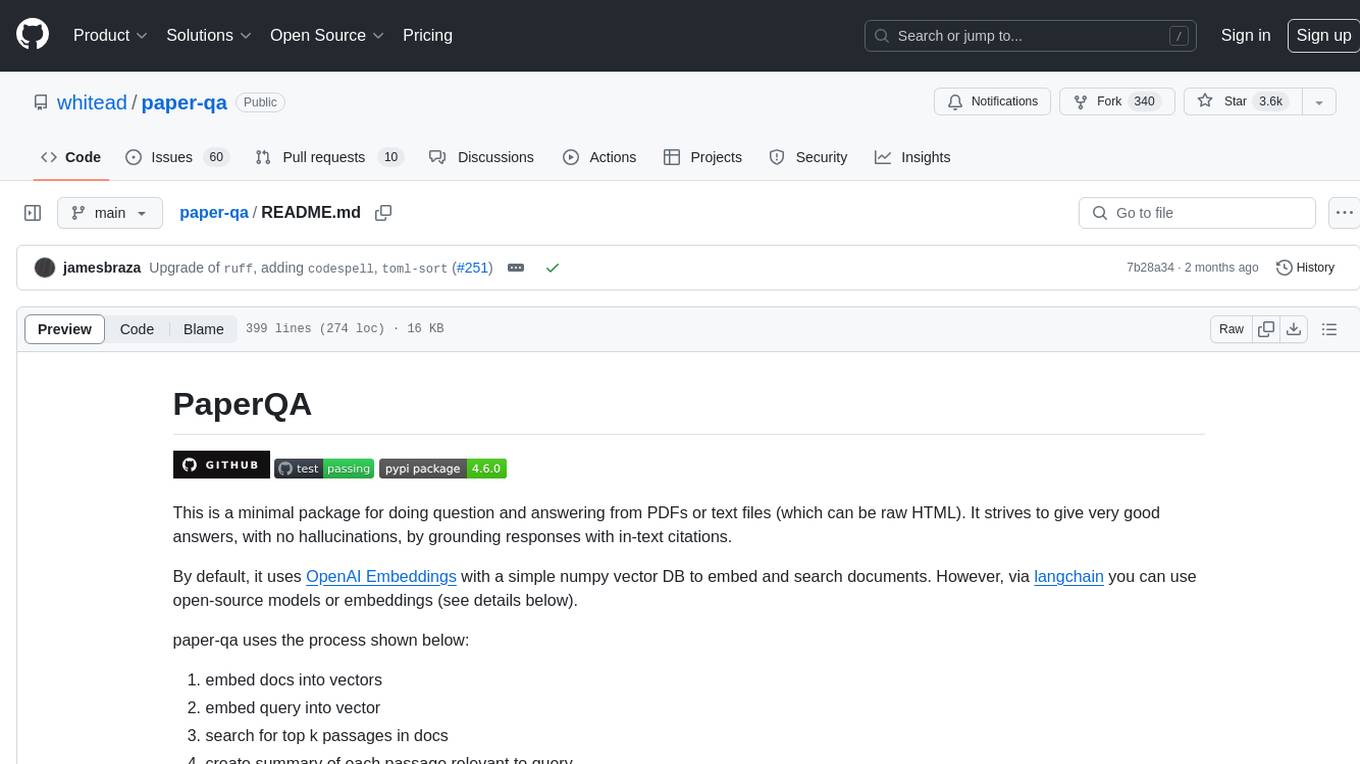
paper-qa
PaperQA is a minimal package for question and answering from PDFs or text files, providing very good answers with in-text citations. It uses OpenAI Embeddings to embed and search documents, and follows a process of embedding docs and queries, searching for top passages, creating summaries, scoring and selecting relevant summaries, putting summaries into prompt, and generating answers. Users can customize prompts and use various models for embeddings and LLMs. The tool can be used asynchronously and supports adding documents from paths, files, or URLs.
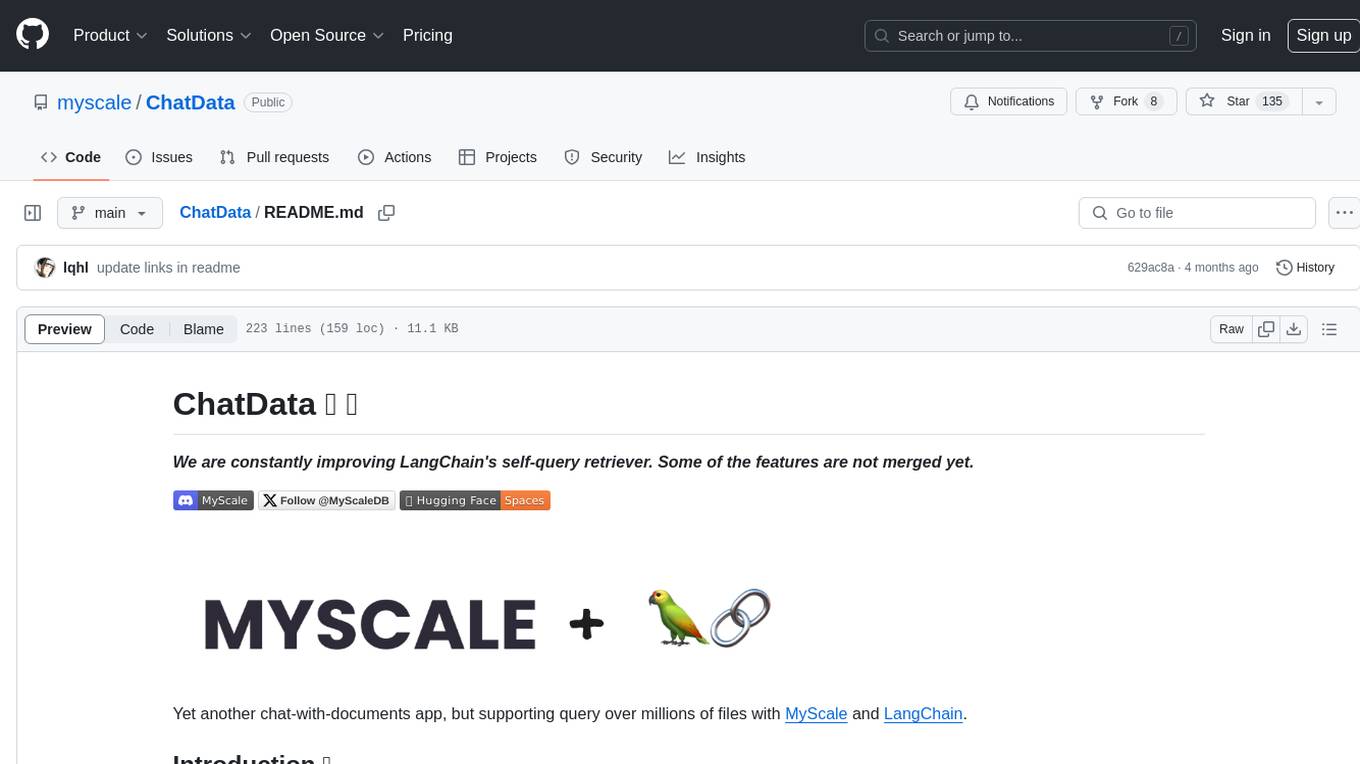
ChatData
ChatData is a robust chat-with-documents application designed to extract information and provide answers by querying the MyScale free knowledge base or uploaded documents. It leverages the Retrieval Augmented Generation (RAG) framework, millions of Wikipedia pages, and arXiv papers. Features include self-querying retriever, VectorSQL, session management, and building a personalized knowledge base. Users can effortlessly navigate vast data, explore academic papers, and research documents. ChatData empowers researchers, students, and knowledge enthusiasts to unlock the true potential of information retrieval.
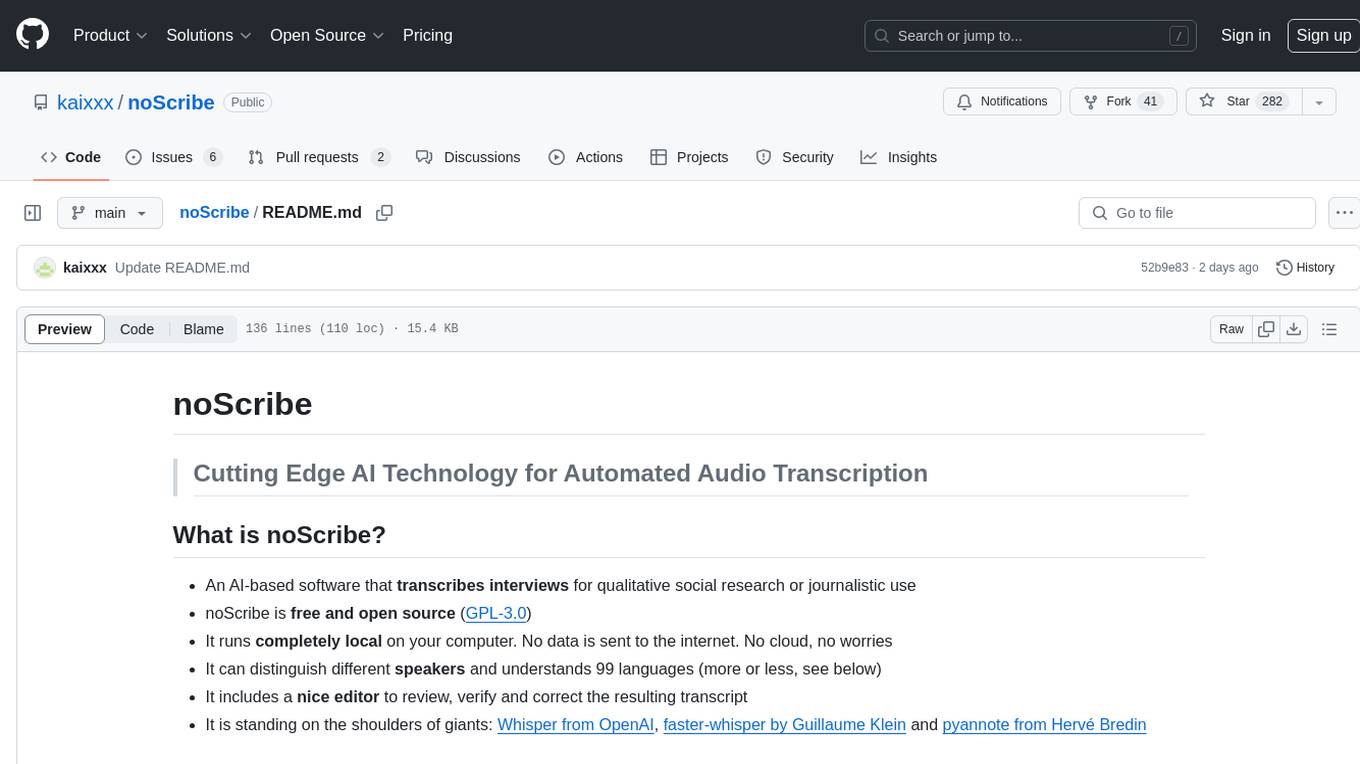
noScribe
noScribe is an AI-based software designed for automated audio transcription, specifically tailored for transcribing interviews for qualitative social research or journalistic purposes. It is a free and open-source tool that runs locally on the user's computer, ensuring data privacy. The software can differentiate between speakers and supports transcription in 99 languages. It includes a user-friendly editor for reviewing and correcting transcripts. Developed by Kai Dröge, a PhD in sociology with a background in computer science, noScribe aims to streamline the transcription process and enhance the efficiency of qualitative analysis.
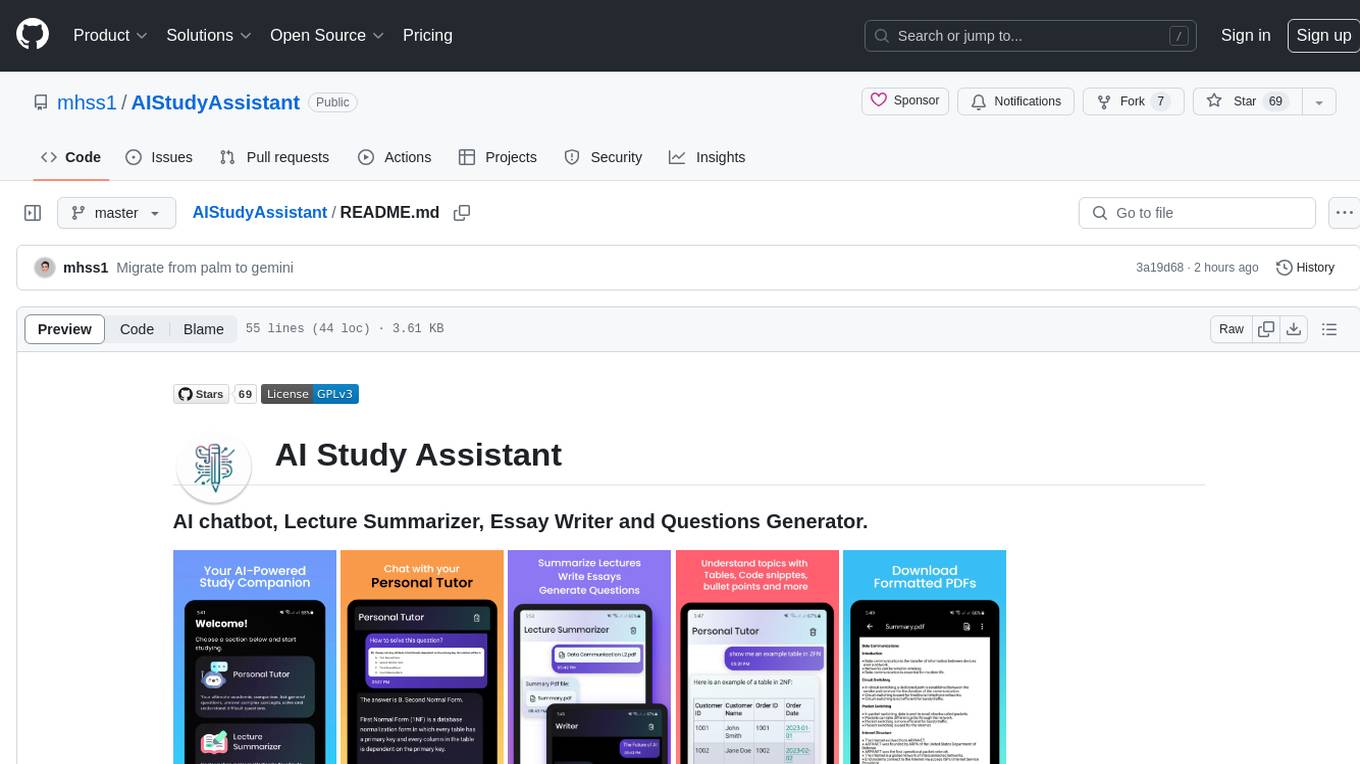
AIStudyAssistant
AI Study Assistant is an app designed to enhance learning experience and boost academic performance. It serves as a personal tutor, lecture summarizer, writer, and question generator powered by Google PaLM 2. Features include interacting with an AI chatbot, summarizing lectures, generating essays, and creating practice questions. The app is built using 100% Kotlin, Jetpack Compose, Clean Architecture, and MVVM design pattern, with technologies like Ktor, Room DB, Hilt, and Kotlin coroutines. AI Study Assistant aims to provide comprehensive AI-powered assistance for students in various academic tasks.
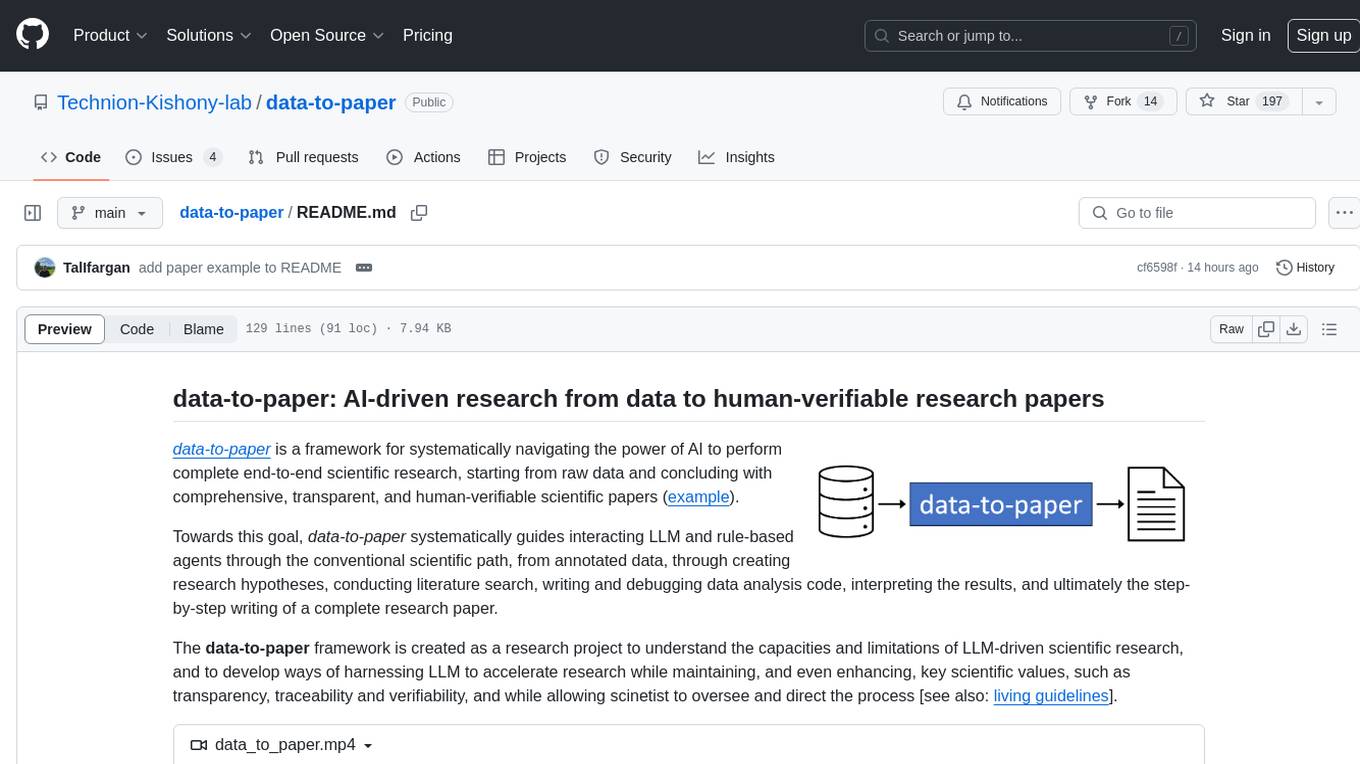
data-to-paper
Data-to-paper is an AI-driven framework designed to guide users through the process of conducting end-to-end scientific research, starting from raw data to the creation of comprehensive and human-verifiable research papers. The framework leverages a combination of LLM and rule-based agents to assist in tasks such as hypothesis generation, literature search, data analysis, result interpretation, and paper writing. It aims to accelerate research while maintaining key scientific values like transparency, traceability, and verifiability. The framework is field-agnostic, supports both open-goal and fixed-goal research, creates data-chained manuscripts, involves human-in-the-loop interaction, and allows for transparent replay of the research process.
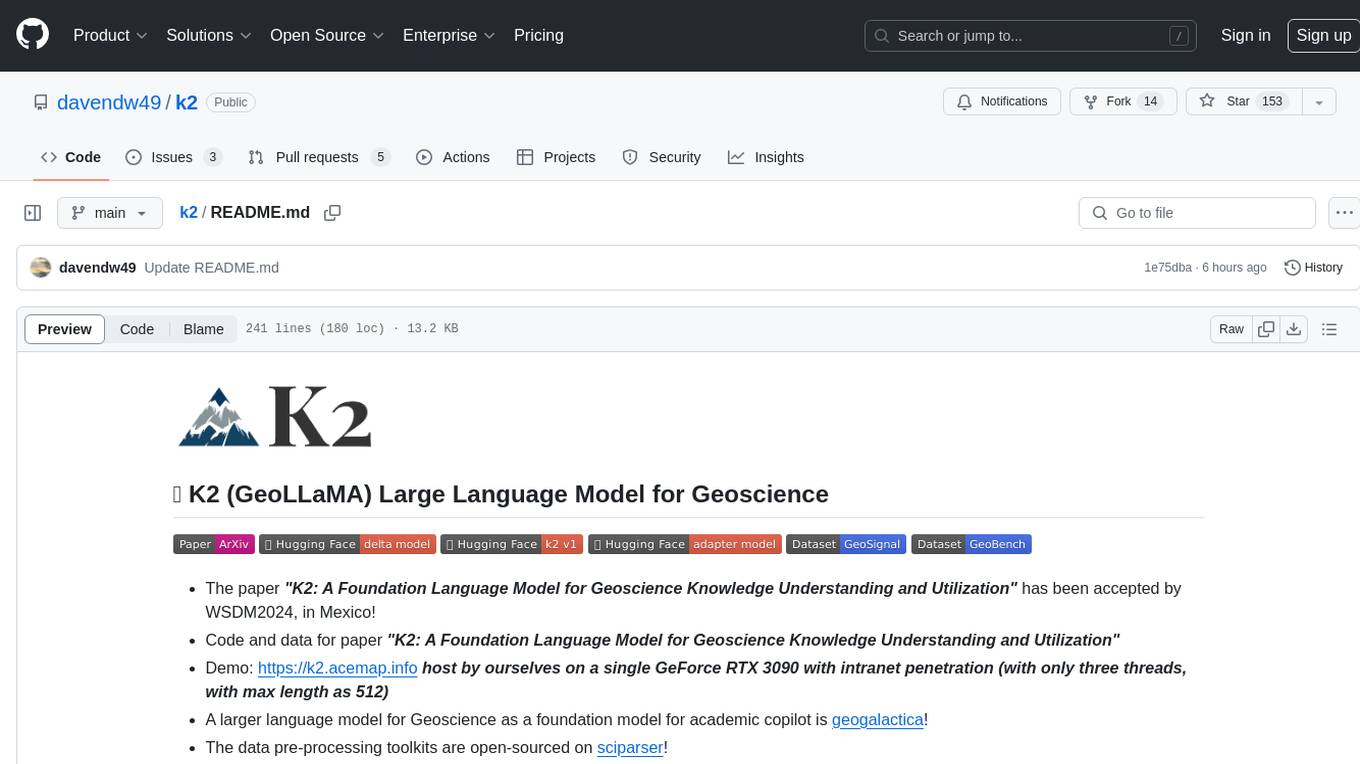
k2
K2 (GeoLLaMA) is a large language model for geoscience, trained on geoscience literature and fine-tuned with knowledge-intensive instruction data. It outperforms baseline models on objective and subjective tasks. The repository provides K2 weights, core data of GeoSignal, GeoBench benchmark, and code for further pretraining and instruction tuning. The model is available on Hugging Face for use. The project aims to create larger and more powerful geoscience language models in the future.