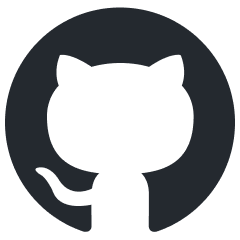
fabrice-ai
A lightweight, functional, and composable framework for building AI agents. No PhD required.
Stars: 223
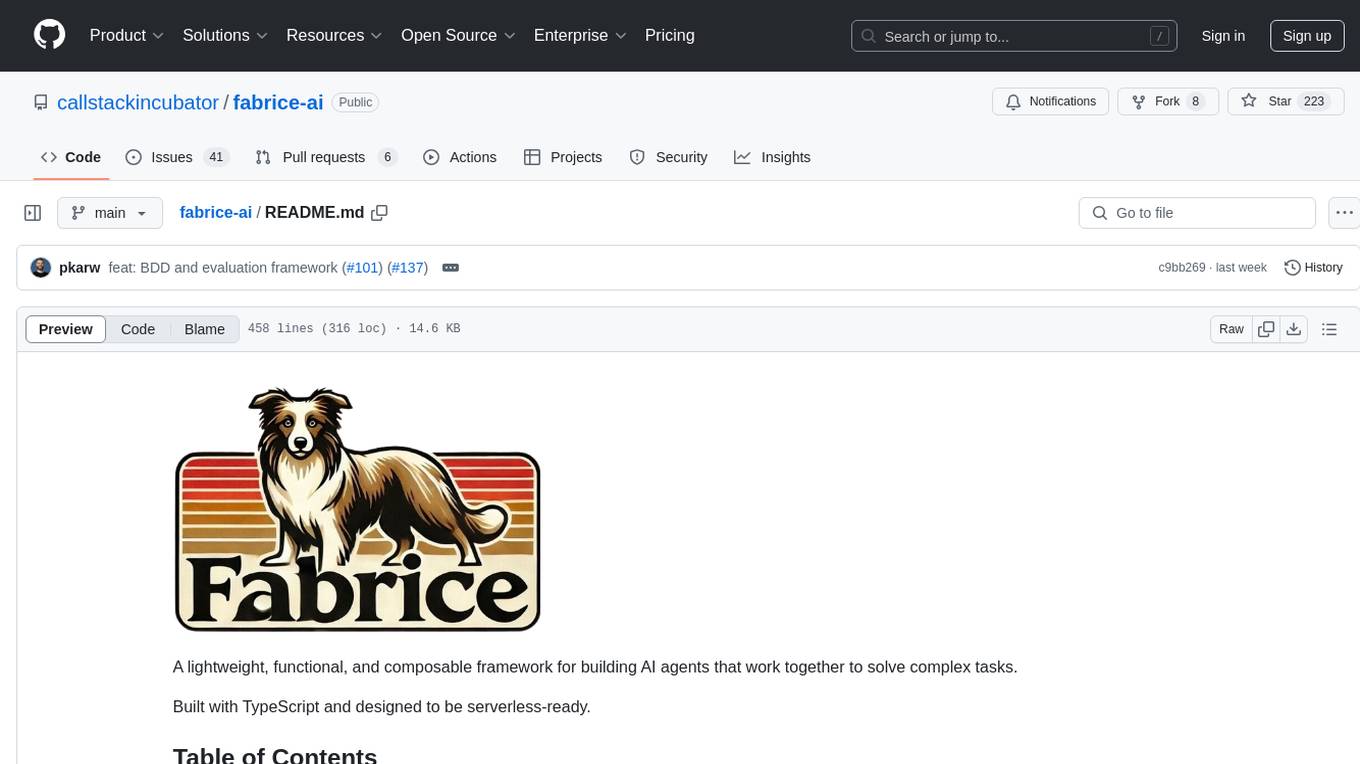
A lightweight, functional, and composable framework for building AI agents that work together to solve complex tasks. Built with TypeScript and designed to be serverless-ready. Fabrice embraces functional programming principles, remains stateless, and stays focused on composability. It provides core concepts like easy teamwork creation, infrastructure-agnosticism, statelessness, and includes all tools and features needed to build AI teams. Agents are specialized workers with specific roles and capabilities, able to call tools and complete tasks. Workflows define how agents collaborate to achieve a goal, with workflow states representing the current state of the workflow. Providers handle requests to the LLM and responses. Tools extend agent capabilities by providing concrete actions they can perform. Execution involves running the workflow to completion, with options for custom execution and BDD testing.
README:
A lightweight, functional, and composable framework for building AI agents that work together to solve complex tasks.
Built with TypeScript and designed to be serverless-ready.
- Getting Started
- Why Another AI Agent Framework?
- Core Concepts
- Agents
- Workflows
- Workflow States
- Providers
- Tools
- Execution
- Test framework
- Contributors
- Made with ❤️ at Callstack
It is very easy to get started. All you have to do is to create a file with your agents and workflow, then run it.
Use our creator tool to quickly create a new AI agent project.
npx create-fabrice-ai
You can choose from a few templates. You can see a full list of them here.
npm install fabrice-ai
Here is a simple example of a workflow that researches and plans a trip to Wrocław, Poland:
import { agent } from 'fabrice-ai/agent'
import { teamwork } from 'fabrice-ai/teamwork'
import { solution, workflow } from 'fabrice-ai/workflow'
import { lookupWikipedia } from './tools/wikipedia.js'
const activityPlanner = agent({
description: `You are skilled at creating personalized itineraries...`,
})
const landmarkScout = agent({
description: `You research interesting landmarks...`,
tools: { lookupWikipedia },
})
const workflow = workflow({
team: { activityPlanner, landmarkScout },
description: `Plan a trip to Wrocław, Poland...`,
})
const result = await teamwork(workflow)
console.log(solution(result))
Finally, you can run the example by simply executing the file.
Using bun
bun your_file.ts
Using node
node --import=tsx your_file.ts
Most existing AI agent frameworks are either too complex, heavily object-oriented, or tightly coupled to specific infrastructure.
We wanted something different - a framework that embraces functional programming principles, remains stateless, and stays laser-focused on composability.
Now, English + Typescript is your tech stack.
Here are the core concepts of Fabrice:
Teamwork should be easy and fun, just like in real life. It should not require you to learn a new framework and mental model to put your AI team together.
There should be no assumptions about the infrastructure you're using. You should be able to use any provider and any tools, in any environment.
No classes, no side effects. Every operation should be a function that returns a new state.
We should provide you with all tools and features needed to build your AI team, locally and in the cloud.
Agents are specialized workers with specific roles and capabilities. Agents can call available tools and complete assigned tasks. Depending on the task complexity, it can be done in a single step, or multiple steps.
To create a custom agent, you can use our agent
helper function or implement the Agent
interface manually.
import { agent } from 'fabrice-ai/agent'
const myAgent = agent({
role: '<< your role >>',
description: '<< your description >>',
})
Additionally, you can give it access to tools by passing a tools
property to the agent. You can learn more about tools here. You can also set custom provider
for each agent. You can learn more about providers here.
Fabrice comes with a few built-in agents that help it run your workflows out of the box.
Supervisor, supervisor
, is responsible for coordinating the workflow.
It splits your workflow into smaller, more manageable parts, and coordinates the execution.
Resource Planner, resourcePlanner
, is responsible for assigning tasks to available agents, based on their capabilities.
Final Boss, finalBoss
, is responsible for wrapping up the workflow and providing a final output,
in case total number of iterations exeeceds available threshold.
You can overwrite built-in agents by setting it in the workflow.
For example, to replace built-in supervisor
agent, you can do it like this:
import { supervisor } from './my-supervisor.js'
workflow({
team: { supervisor },
})
Workflows define how agents collaborate to achieve a goal. They specify:
- Team members
- Task description
- Expected output
- Optional configuration
Workflow state is a representation of the current state of the workflow. It is a tree of states, where each state represents a single agent's work.
At each level, we have the following properties:
-
agent
: name of the agent that is working on the task -
status
: status of the agent -
messages
: message history -
children
: child states
First element of the messages
array is always a request to the agent, typically a user message. Everything that follows is a message history, including all the messages exchanged with the provider.
Workflow can have multiple states:
-
idle
: no work has been started yet -
running
: work is in progress -
paused
: work is paused and there are tools that must be called to resume -
finished
: work is complete -
failed
: work has failed due to an error
When you run teamwork(workflow)
, initial state is automatically created for you by calling rootState(workflow)
behind the scenes.
[!NOTE] You can also provide your own initial state (for example, to resume a workflow from a previous state). You can learn more about it in the server-side usage section.
Root state is a special state that contains an initial request based on the workflow and points to the supervisor
agent, which is responsible for splitting the work into smaller, more manageable parts.
You can learn more about the supervisor
agent here.
Child state is like root state, but it points to any agent, such as one from your team.
You can create it manually, or use childState
function.
const child = childState({
agent: '<< agent name >>',
messages: user('<< task description >>'),
})
[!TIP] Fabrice exposes a few helpers to facilitate creating messages, such as
user
andassistant
. You can use them to create messages in a more readable way, although it is not required.
To delegate the task, just add a new child state to your agent's state.
const state = {
...state,
children: [
...state.children,
childState({
/** agent to work on the task */
agent: '<< agent name >>',
/** task description */
messages: [
{
role: 'user',
content: '<< task description >>',
}
],
})
]
}
To make it easier, you can use delegate
function to delegate the task.
const state = delegate(state, [agent, '<< task description >>'])
To hand off the task, you can replace your agent's state with a new state, that points to a different agent.
const state = childState({
agent: '<< new agent name >>',
messages: state.messages,
})
In the example above, we're passing the entire message history to the new agent, including the original request and all the work done by any previous agent. It is up to you to decide how much of the history to pass to the new agent.
Providers are responsible for sending requests to the LLM and handling the responses.
Fabrice comes with a few built-in providers:
- OpenAI (structured output)
- OpenAI (using tools as response format)
- Groq
You can learn more about them here.
If you're working with an OpenAI compatible provider, you can use the openai
provider with a different base URL and API key, such as:
openai({
model: '<< your model >>',
options: {
apiKey: '<< your_api_key >>',
baseURL: '<< your_base_url >>',
},
})
By default, Fabrice uses OpenAI gpt-4o model. You can change the default model or provider either for the entire system, or for specific agent.
To do it for the entire workflow:
import { grok } from 'fabrice-ai/providers/grok'
workflow({
/** other options go here */
provider: grok()
})
To change it for specific agent:
import { grok } from 'fabrice-ai/providers/grok'
agent({
/** other options go here */
provider: grok()
})
Note that an agent's provider always takes precedence over a workflow's provider. Tools always receive the provider from the agent that triggered their execution.
To create a custom provider, you need to implement the Provider
interface.
const myProvider = (options: ProviderOptions): Provider => {
return {
chat: async () => {
/** your implementation goes here */
},
}
}
You can learn more about the Provider
interface here.
Tools extend agent capabilities by providing concrete actions they can perform.
Fabrice comes with a few built-in tools via @fabrice-ai/tools
package. For most up-to-date list, please refer to the README.
To create a custom tool, you can use our tool
helper function or implement the Tool
interface manually.
import { tool } from 'fabrice-ai/tools'
const myTool = tool({
description: 'My tool description',
parameters: z.object({
/** your Zod schema goes here */
}),
execute: async (parameters, context) => {
/** your implementation goes here */
},
})
Tools will use the same provider as the agent that triggered them. Additionally, you can access the context
object, which gives you access to the provider, as well as current message history.
To give an agent access to a tool, you need to add it to the agent's tools
property.
agent({
role: '<< your role >>',
tools: { searchWikipedia },
})
Since tools are passed to an LLM and referred by their key, you should use meaningful names for them, for increased effectiveness.
Execution is the process of running the workflow to completion. A completed workflow is a workflow with state "finished" at its root.
The easiest way to complete the workflow is to call teamwork(workflow)
function. It will run the workflow to completion and return the final state.
const state = await teamwork(workflow)
console.log(solution(state))
Calling solution(state)
will return the final output of the workflow, which is its last message.
If you are running workflows in the cloud, or any other environment where you want to handle tool execution manually, you can call teamwork the following way:
/** read state from the cache */
/** run the workflow */
const state = await teamwork(workflow, prevState, false)
/** save state to the cache */
Passing second argument to teamwork
is optional. If you don't provide it, root state will be created automatically. Otherwise, it will be used as a starting point for the next iteration.
Last argument is a boolean flag that determines if tools should be executed. If you set it to false
, you are responsible for calling tools manually. Teamwork will stop iterating over the workflow and return the current state with paused
status.
If you want to handle tool execution manually, you can use iterate
function to build up your own recursive iteration logic over the workflow state.
Have a look at how teamwork
is implemented here to understand how it works.
There's a packaged called fabrice-ai/bdd
dedicated to unit testing - actually to Behavioral Driven Development. Check the docs.
Mike 💻 |
Piotr Karwatka 💻 |
Fabrice is an open source project and will always remain free to use. If you think it's cool, please star it 🌟. Callstack is a group of React and React Native geeks, contact us at [email protected] if you need any help with these or just want to say hi!
Like the project? ⚛️ Join the team who does amazing stuff for clients and drives React Native Open Source! 🔥
For Tasks:
Click tags to check more tools for each tasksFor Jobs:
Alternative AI tools for fabrice-ai
Similar Open Source Tools
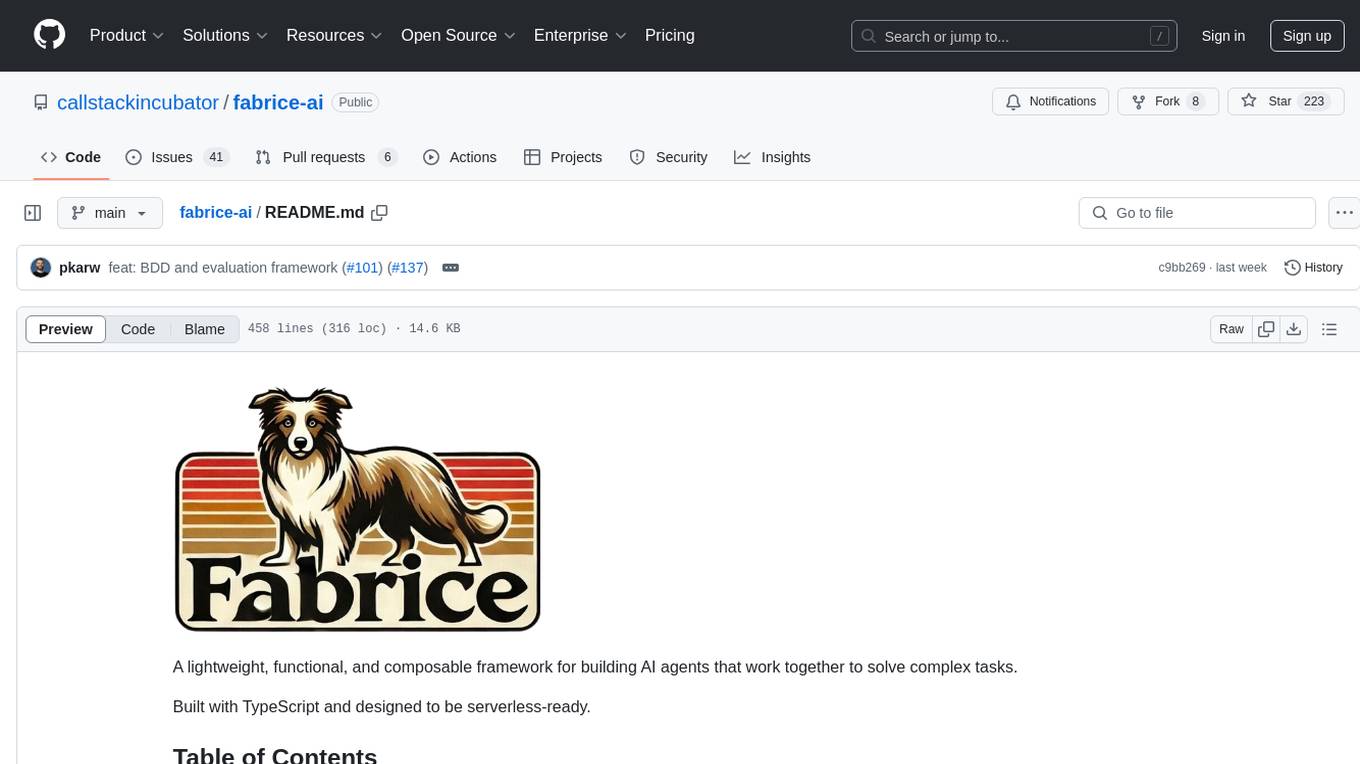
fabrice-ai
A lightweight, functional, and composable framework for building AI agents that work together to solve complex tasks. Built with TypeScript and designed to be serverless-ready. Fabrice embraces functional programming principles, remains stateless, and stays focused on composability. It provides core concepts like easy teamwork creation, infrastructure-agnosticism, statelessness, and includes all tools and features needed to build AI teams. Agents are specialized workers with specific roles and capabilities, able to call tools and complete tasks. Workflows define how agents collaborate to achieve a goal, with workflow states representing the current state of the workflow. Providers handle requests to the LLM and responses. Tools extend agent capabilities by providing concrete actions they can perform. Execution involves running the workflow to completion, with options for custom execution and BDD testing.
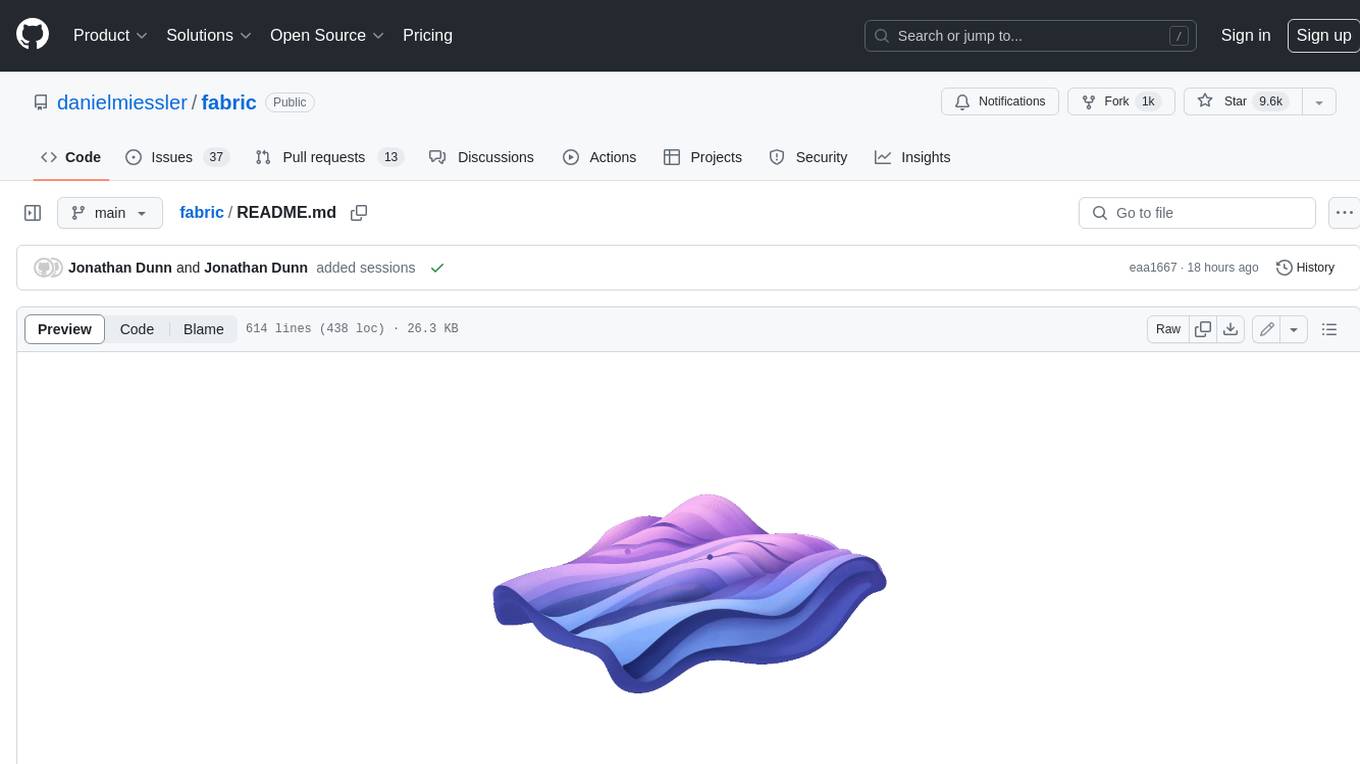
fabric
Fabric is an open-source framework for augmenting humans using AI. It provides a structured approach to breaking down problems into individual components and applying AI to them one at a time. Fabric includes a collection of pre-defined Patterns (prompts) that can be used for a variety of tasks, such as extracting the most interesting parts of YouTube videos and podcasts, writing essays, summarizing academic papers, creating AI art prompts, and more. Users can also create their own custom Patterns. Fabric is designed to be easy to use, with a command-line interface and a variety of helper apps. It is also extensible, allowing users to integrate it with their own AI applications and infrastructure.
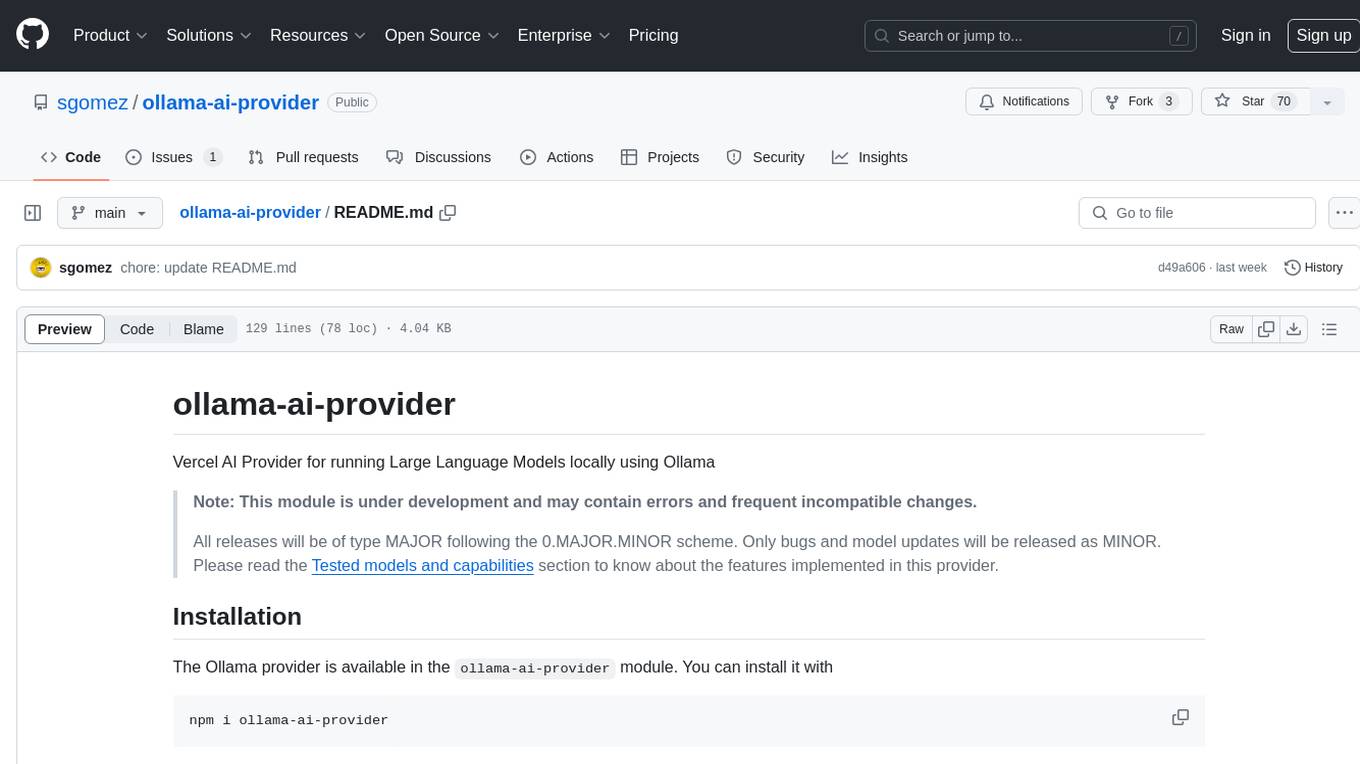
ollama-ai-provider
Vercel AI Provider for running Large Language Models locally using Ollama. This module is under development and may contain errors and frequent incompatible changes. It provides the capability of generating and streaming text and objects, with features like image input, object generation, tool usage simulation, tool streaming simulation, intercepting fetch requests, and provider management. The provider can be customized with optional settings like baseURL and headers.
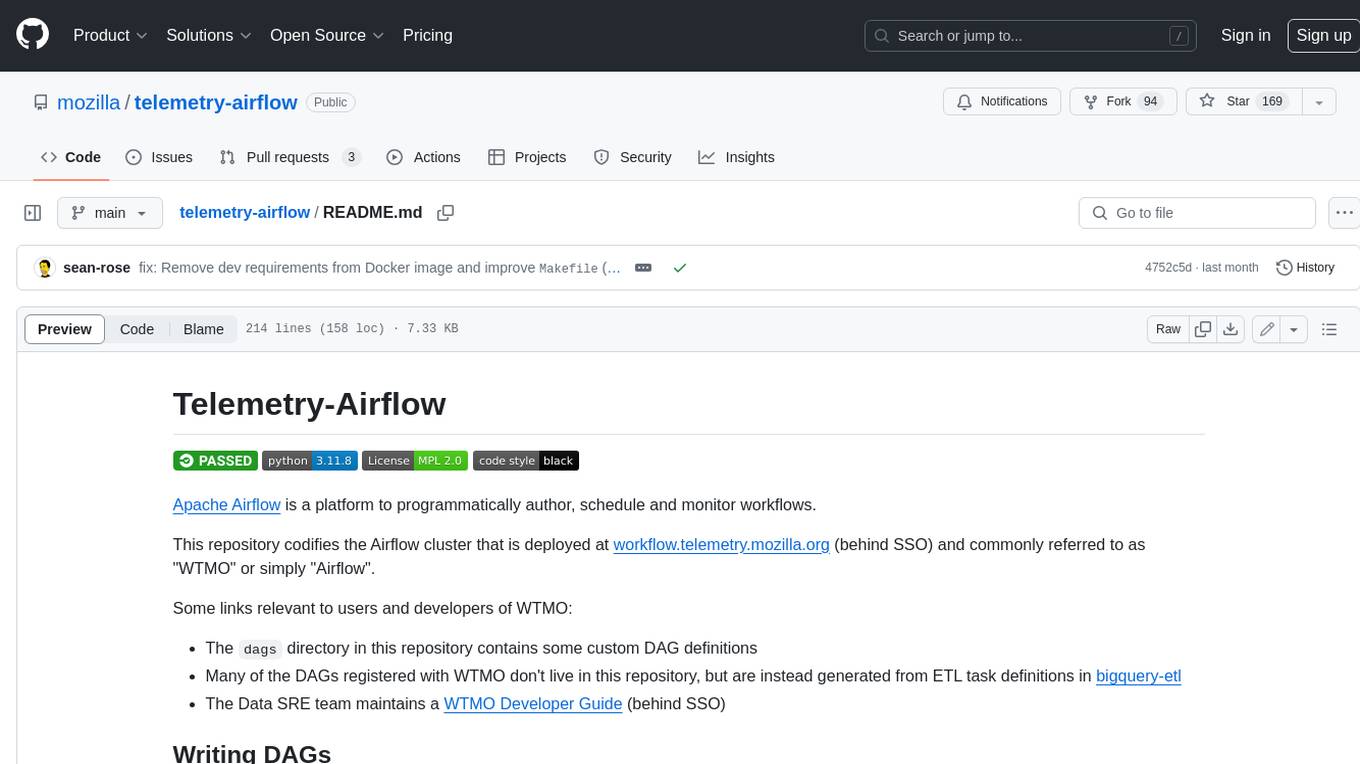
telemetry-airflow
This repository codifies the Airflow cluster that is deployed at workflow.telemetry.mozilla.org (behind SSO) and commonly referred to as "WTMO" or simply "Airflow". Some links relevant to users and developers of WTMO: * The `dags` directory in this repository contains some custom DAG definitions * Many of the DAGs registered with WTMO don't live in this repository, but are instead generated from ETL task definitions in bigquery-etl * The Data SRE team maintains a WTMO Developer Guide (behind SSO)
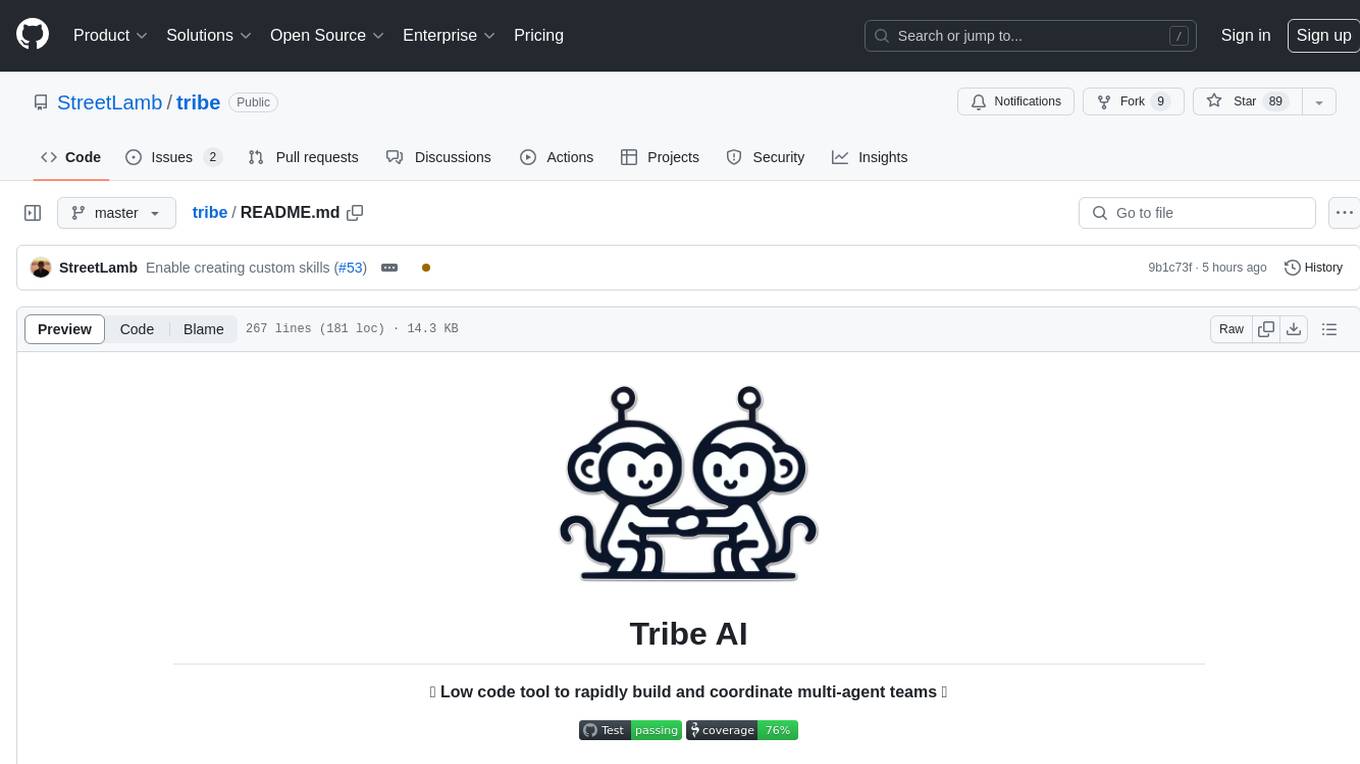
tribe
Tribe AI is a low code tool designed to rapidly build and coordinate multi-agent teams. It leverages the langgraph framework to customize and coordinate teams of agents, allowing tasks to be split among agents with different strengths for faster and better problem-solving. The tool supports persistent conversations, observability, tool calling, human-in-the-loop functionality, easy deployment with Docker, and multi-tenancy for managing multiple users and teams.
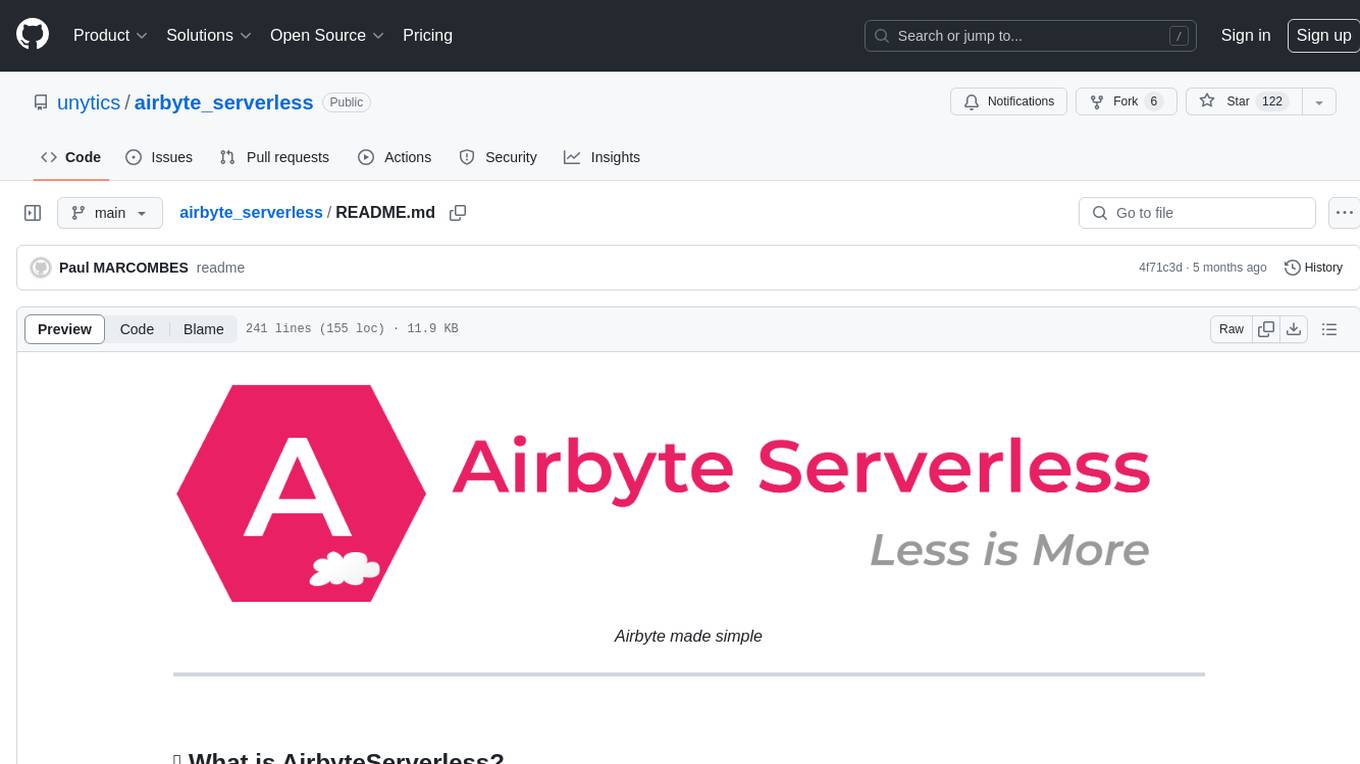
airbyte_serverless
AirbyteServerless is a lightweight tool designed to simplify the management of Airbyte connectors. It offers a serverless mode for running connectors, allowing users to easily move data from any source to their data warehouse. Unlike the full Airbyte-Open-Source-Platform, AirbyteServerless focuses solely on the Extract-Load process without a UI, database, or transform layer. It provides a CLI tool, 'abs', for managing connectors, creating connections, running jobs, selecting specific data streams, handling secrets securely, and scheduling remote runs. The tool is scalable, allowing independent deployment of multiple connectors. It aims to streamline the connector management process and provide a more agile alternative to the comprehensive Airbyte platform.
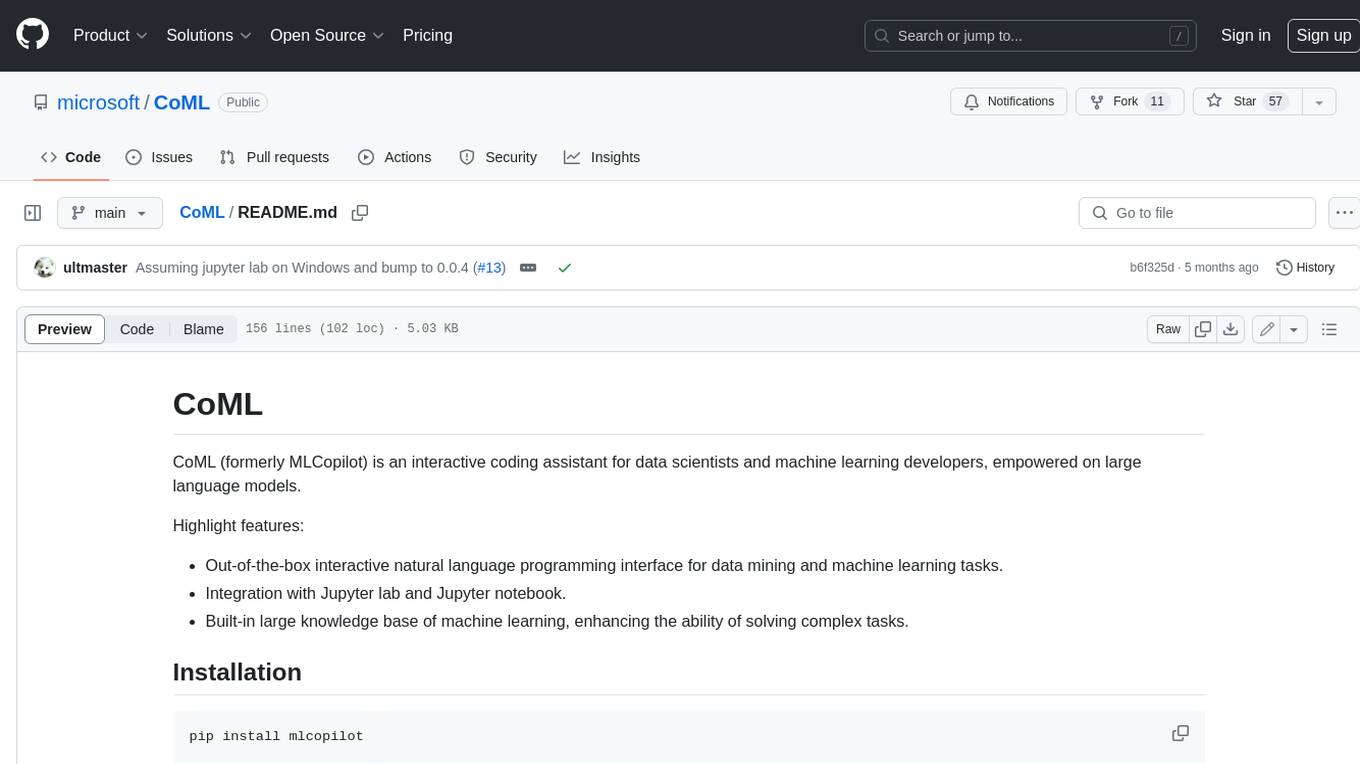
CoML
CoML (formerly MLCopilot) is an interactive coding assistant for data scientists and machine learning developers, empowered on large language models. It offers an out-of-the-box interactive natural language programming interface for data mining and machine learning tasks, integration with Jupyter lab and Jupyter notebook, and a built-in large knowledge base of machine learning to enhance the ability to solve complex tasks. The tool is designed to assist users in coding tasks related to data analysis and machine learning using natural language commands within Jupyter environments.
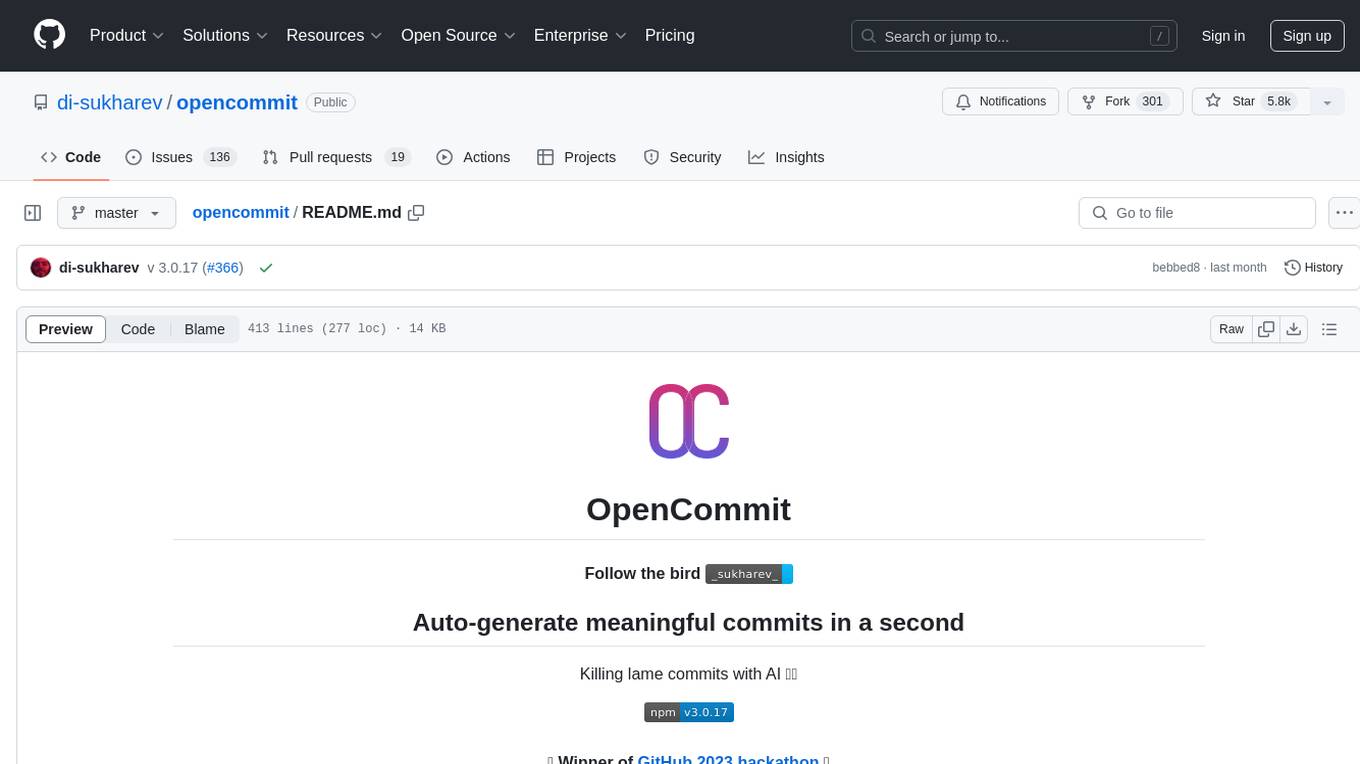
opencommit
OpenCommit is a tool that auto-generates meaningful commits using AI, allowing users to quickly create commit messages for their staged changes. It provides a CLI interface for easy usage and supports customization of commit descriptions, emojis, and AI models. Users can configure local and global settings, switch between different AI providers, and set up Git hooks for integration with IDE Source Control. Additionally, OpenCommit can be used as a GitHub Action to automatically improve commit messages on push events, ensuring all commits are meaningful and not generic. Payments for OpenAI API requests are handled by the user, with the tool storing API keys locally.
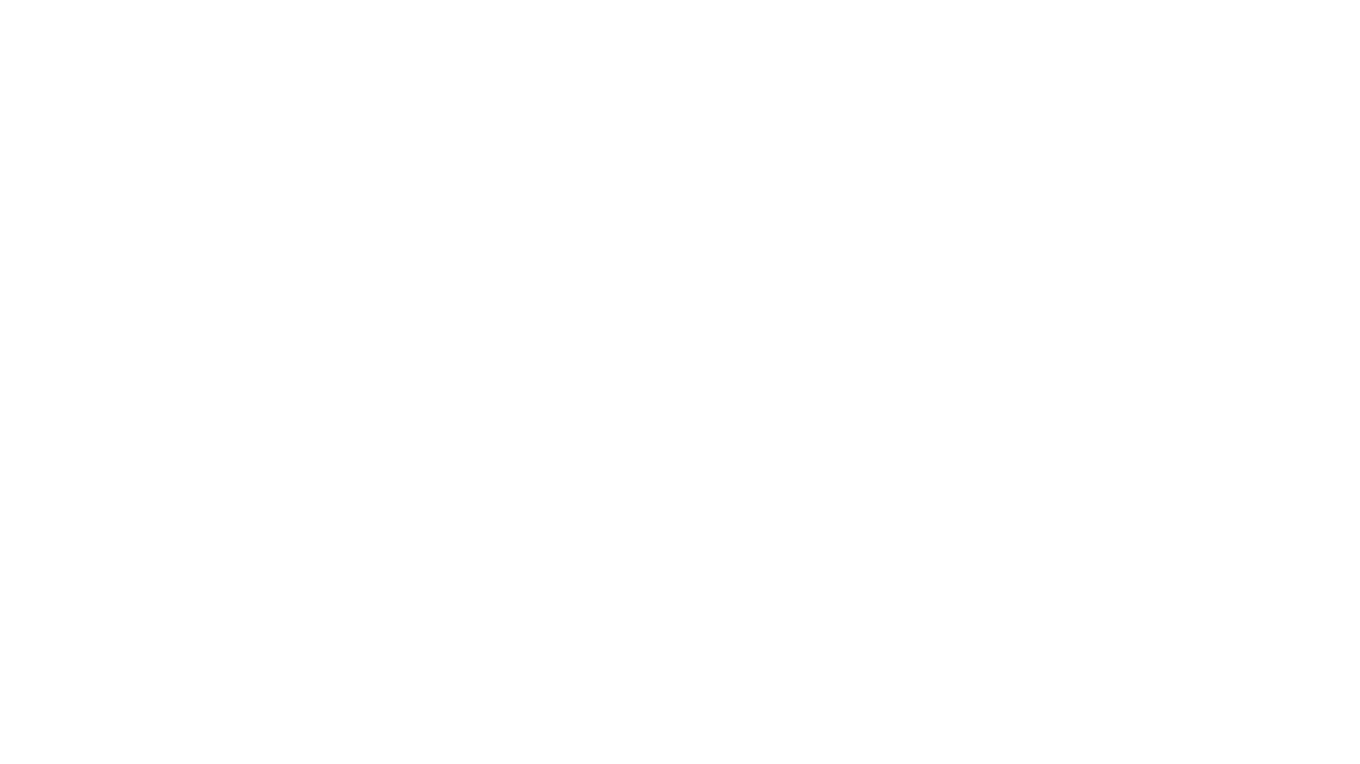
reader
Reader is a tool that converts any URL to an LLM-friendly input with a simple prefix `https://r.jina.ai/`. It improves the output for your agent and RAG systems at no cost. Reader supports image reading, captioning all images at the specified URL and adding `Image [idx]: [caption]` as an alt tag. This enables downstream LLMs to interact with the images in reasoning, summarizing, etc. Reader offers a streaming mode, useful when the standard mode provides an incomplete result. In streaming mode, Reader waits a bit longer until the page is fully rendered, providing more complete information. Reader also supports a JSON mode, which contains three fields: `url`, `title`, and `content`. Reader is backed by Jina AI and licensed under Apache-2.0.
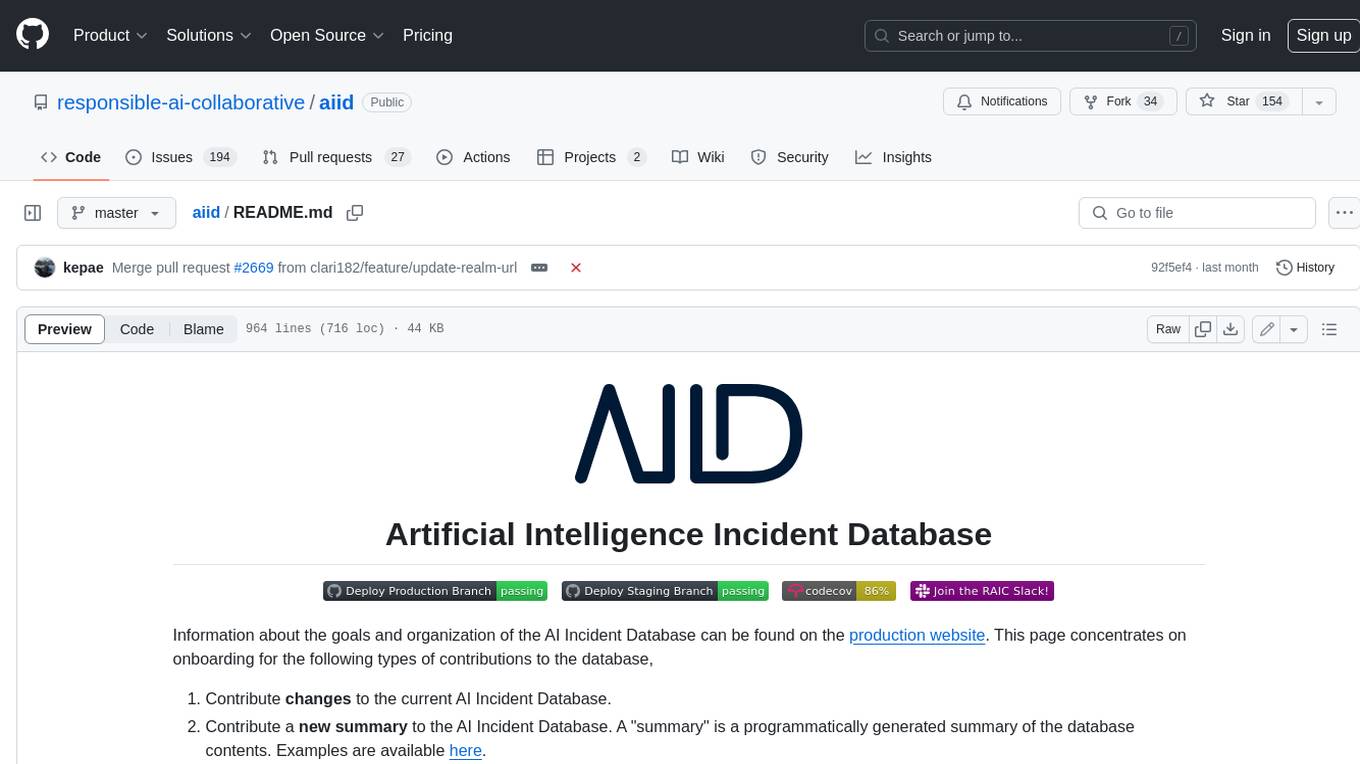
aiid
The Artificial Intelligence Incident Database (AIID) is a collection of incidents involving the development and use of artificial intelligence (AI). The database is designed to help researchers, policymakers, and the public understand the potential risks and benefits of AI, and to inform the development of policies and practices to mitigate the risks and promote the benefits of AI. The AIID is a collaborative project involving researchers from the University of California, Berkeley, the University of Washington, and the University of Toronto.
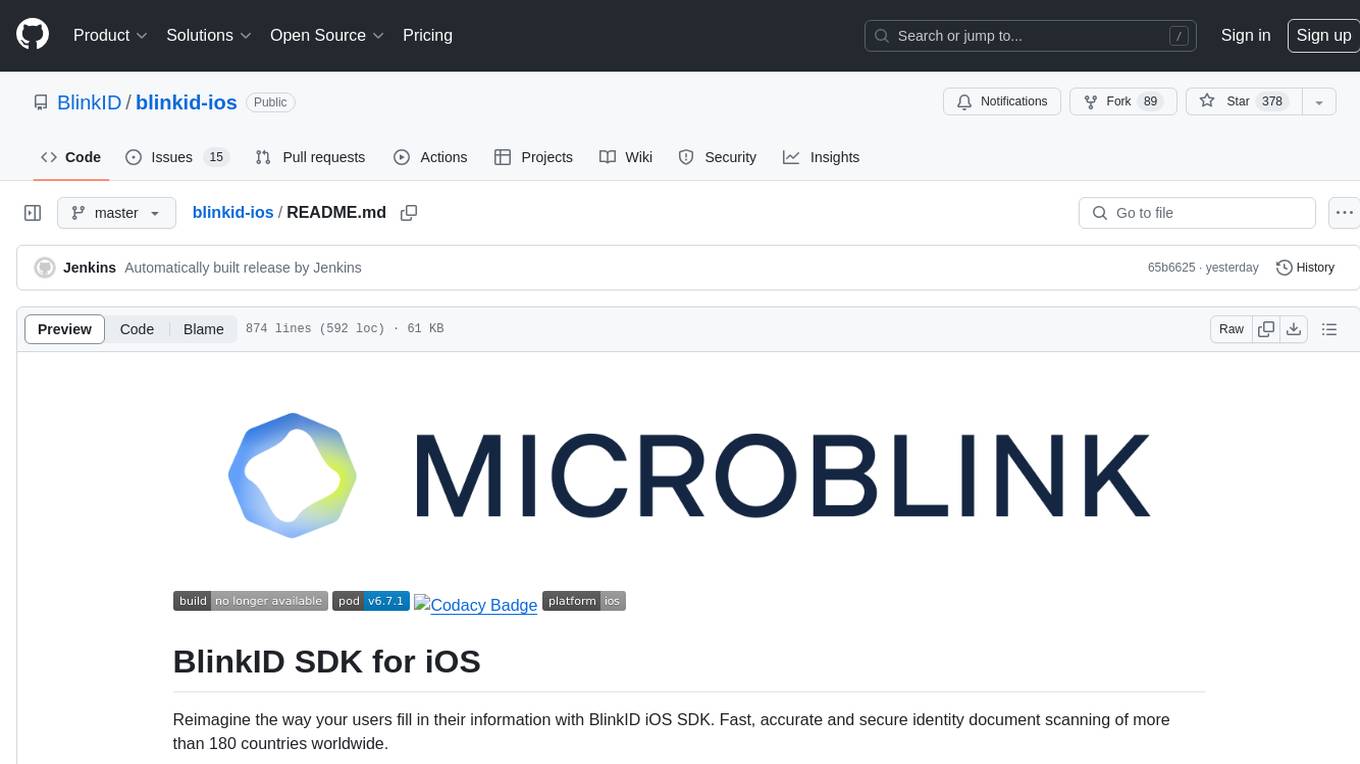
blinkid-ios
BlinkID iOS is a mobile SDK that enables developers to easily integrate ID scanning and data extraction capabilities into their iOS applications. The SDK supports scanning and processing various types of identity documents, such as passports, driver's licenses, and ID cards. It provides accurate and fast data extraction, including personal information and document details. With BlinkID iOS, developers can enhance their apps with secure and reliable ID verification functionality, improving user experience and streamlining identity verification processes.
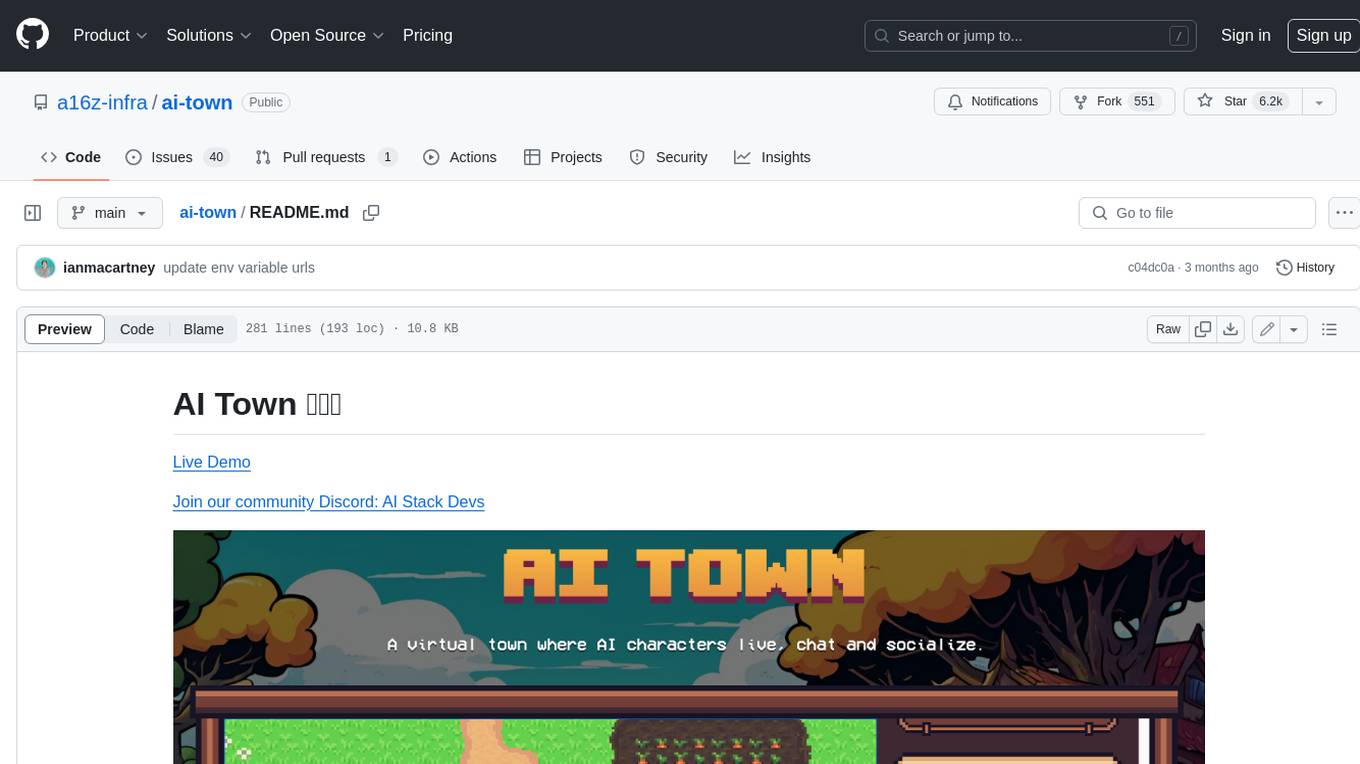
ai-town
AI Town is a virtual town where AI characters live, chat, and socialize. This project provides a deployable starter kit for building and customizing your own version of AI Town. It features a game engine, database, vector search, auth, text model, deployment, pixel art generation, background music generation, and local inference. You can customize your own simulation by creating characters and stories, updating spritesheets, changing the background, and modifying the background music.
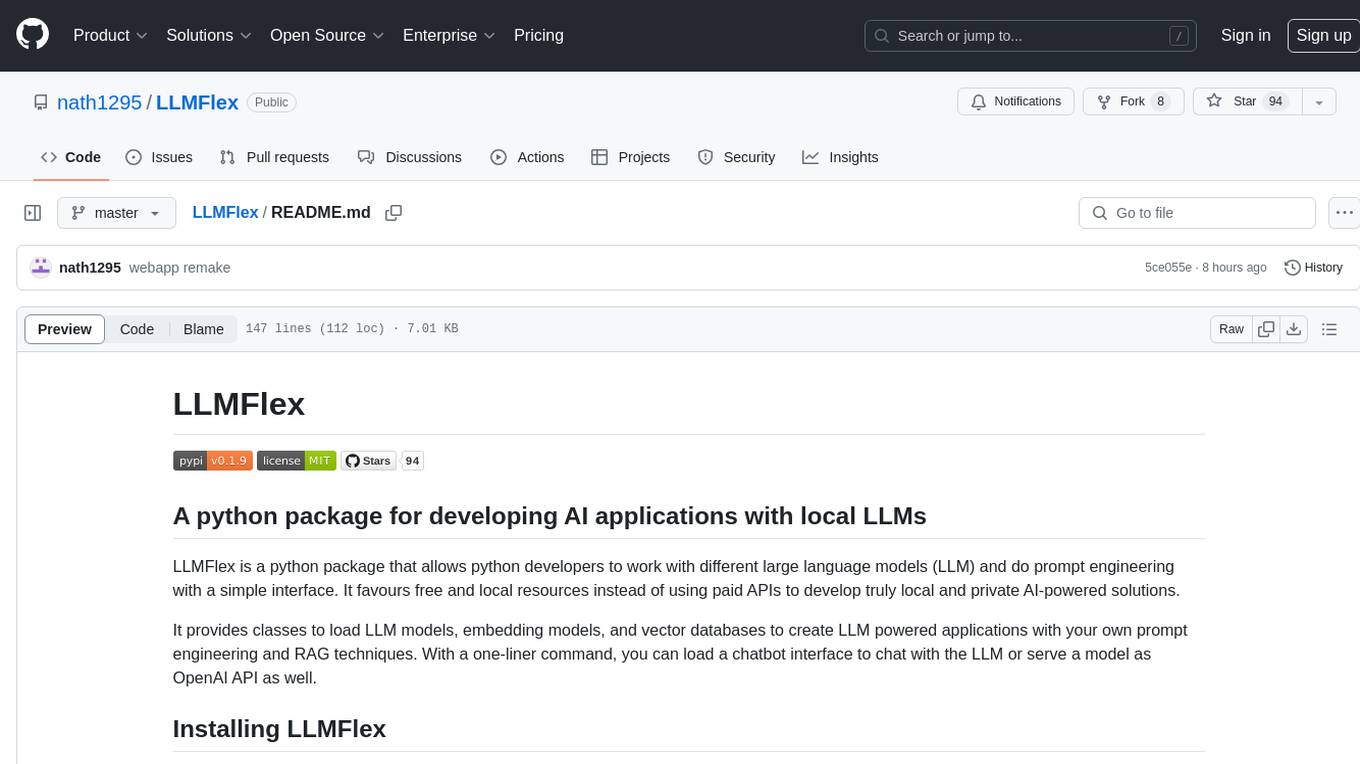
LLMFlex
LLMFlex is a python package designed for developing AI applications with local Large Language Models (LLMs). It provides classes to load LLM models, embedding models, and vector databases to create AI-powered solutions with prompt engineering and RAG techniques. The package supports multiple LLMs with different generation configurations, embedding toolkits, vector databases, chat memories, prompt templates, custom tools, and a chatbot frontend interface. Users can easily create LLMs, load embeddings toolkit, use tools, chat with models in a Streamlit web app, and serve an OpenAI API with a GGUF model. LLMFlex aims to offer a simple interface for developers to work with LLMs and build private AI solutions using local resources.
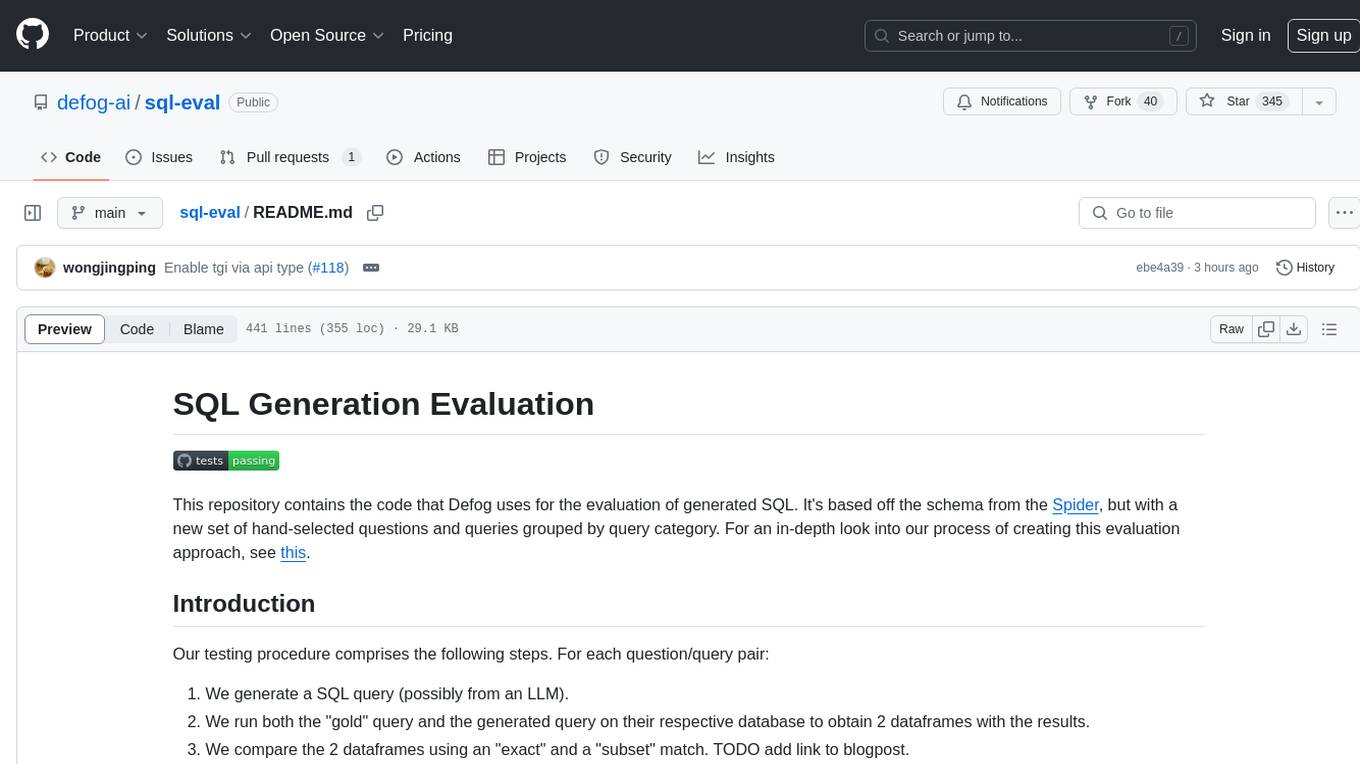
sql-eval
This repository contains the code that Defog uses for the evaluation of generated SQL. It's based off the schema from the Spider, but with a new set of hand-selected questions and queries grouped by query category. The testing procedure involves generating a SQL query, running both the 'gold' query and the generated query on their respective database to obtain dataframes with the results, comparing the dataframes using an 'exact' and a 'subset' match, logging these alongside other metrics of interest, and aggregating the results for reporting. The repository provides comprehensive instructions for installing dependencies, starting a Postgres instance, importing data into Postgres, importing data into Snowflake, using private data, implementing a query generator, and running the test with different runners.
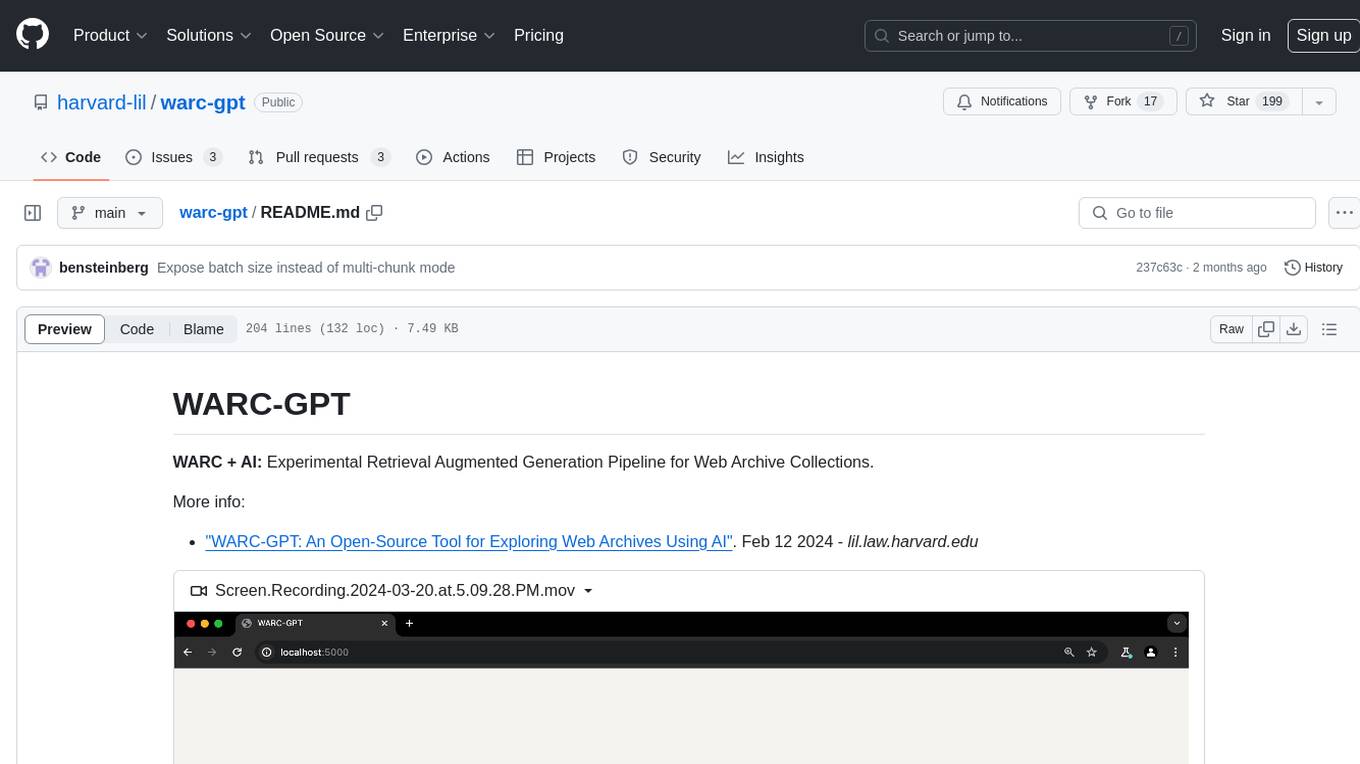
warc-gpt
WARC-GPT is an experimental retrieval augmented generation pipeline for web archive collections. It allows users to interact with WARC files, extract text, generate text embeddings, visualize embeddings, and interact with a web UI and API. The tool is highly customizable, supporting various LLMs, providers, and embedding models. Users can configure the application using environment variables, ingest WARC files, start the server, and interact with the web UI and API to search for content and generate text completions. WARC-GPT is designed for exploration and experimentation in exploring web archives using AI.
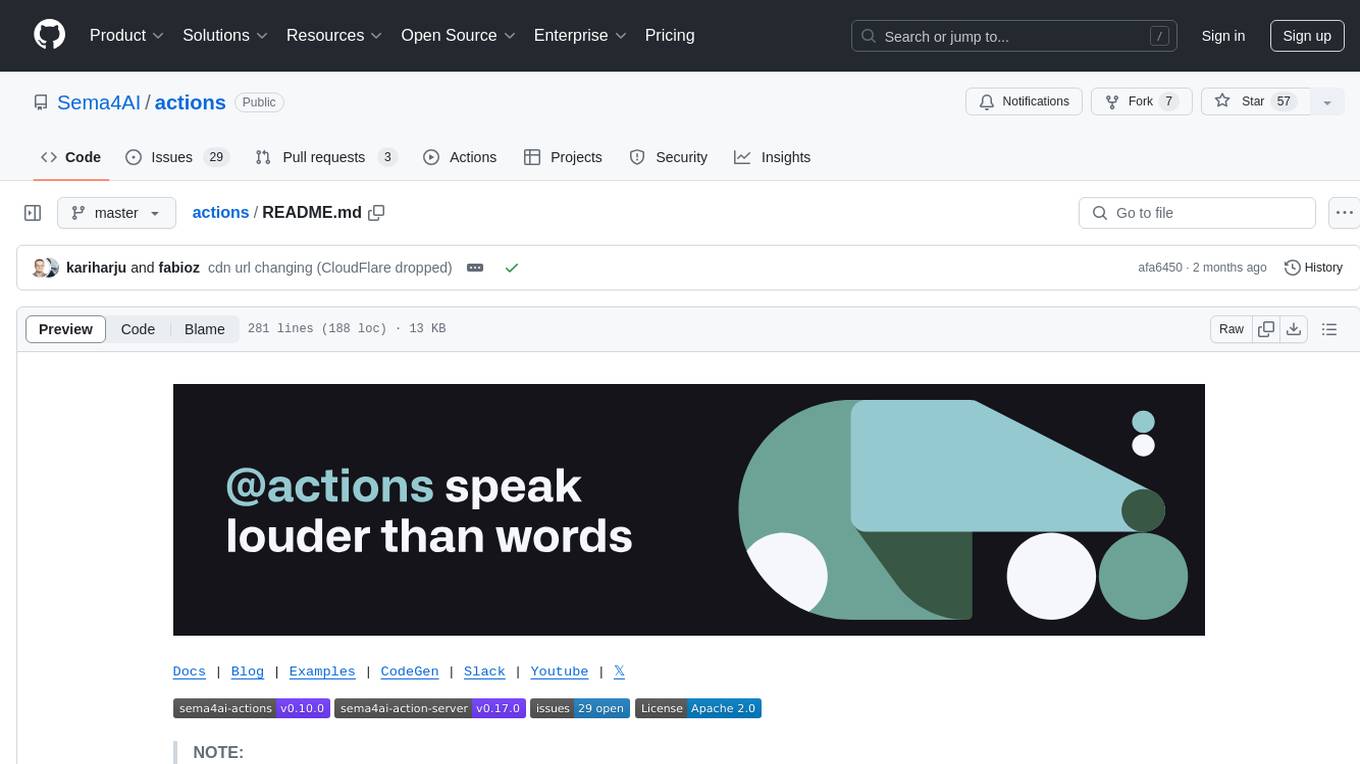
actions
Sema4.ai Action Server is a tool that allows users to build semantic actions in Python to connect AI agents with real-world applications. It enables users to create custom actions, skills, loaders, and plugins that securely connect any AI Assistant platform to data and applications. The tool automatically creates and exposes an API based on function declaration, type hints, and docstrings by adding '@action' to Python scripts. It provides an end-to-end stack supporting various connections between AI and user's apps and data, offering ease of use, security, and scalability.
For similar tasks
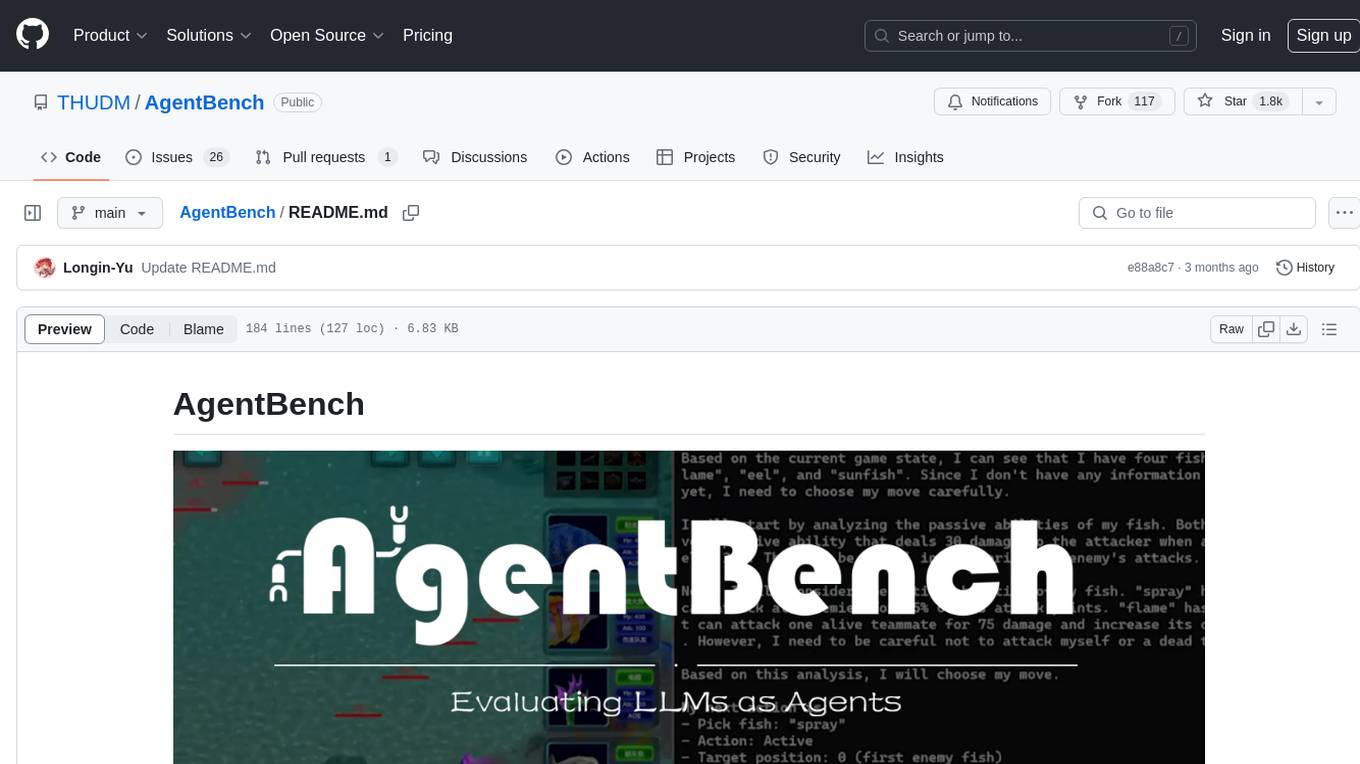
AgentBench
AgentBench is a benchmark designed to evaluate Large Language Models (LLMs) as autonomous agents in various environments. It includes 8 distinct environments such as Operating System, Database, Knowledge Graph, Digital Card Game, and Lateral Thinking Puzzles. The tool provides a comprehensive evaluation of LLMs' ability to operate as agents by offering Dev and Test sets for each environment. Users can quickly start using the tool by following the provided steps, configuring the agent, starting task servers, and assigning tasks. AgentBench aims to bridge the gap between LLMs' proficiency as agents and their practical usability.
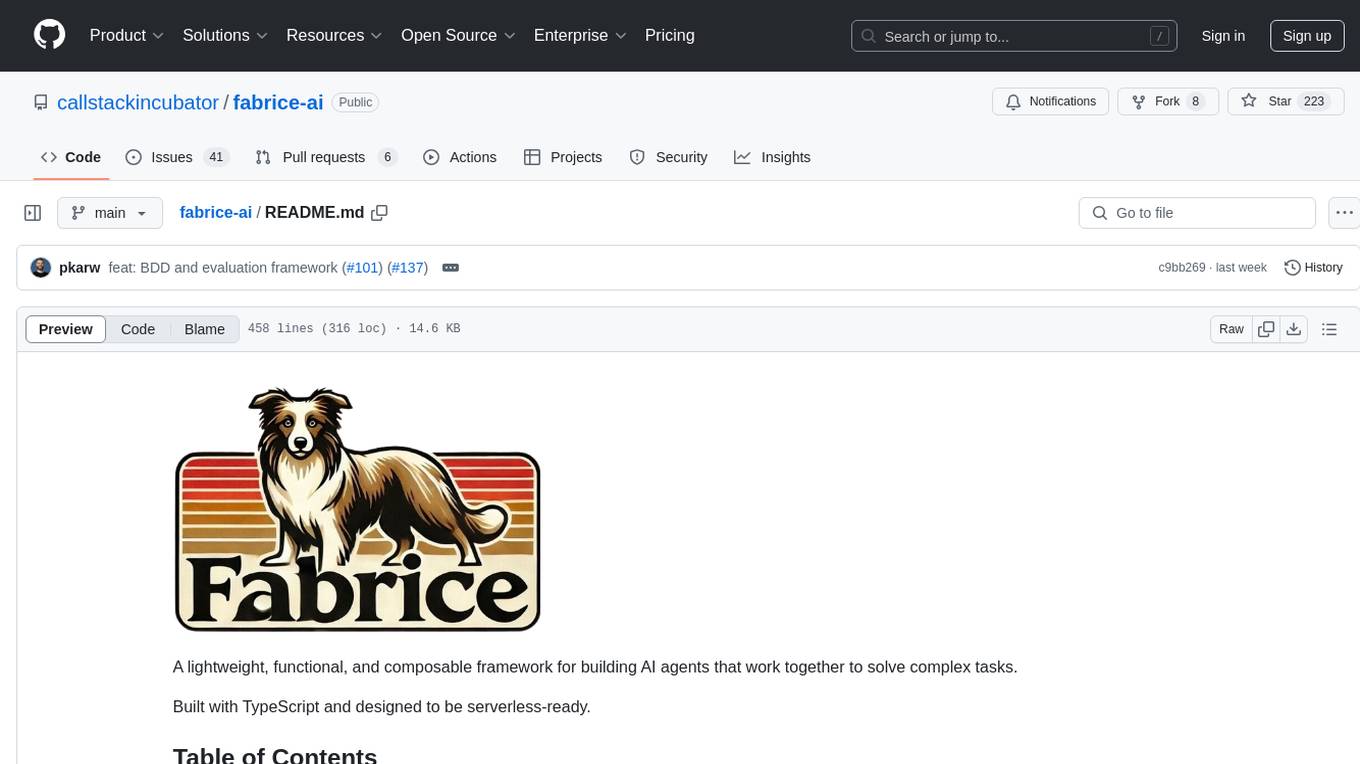
fabrice-ai
A lightweight, functional, and composable framework for building AI agents that work together to solve complex tasks. Built with TypeScript and designed to be serverless-ready. Fabrice embraces functional programming principles, remains stateless, and stays focused on composability. It provides core concepts like easy teamwork creation, infrastructure-agnosticism, statelessness, and includes all tools and features needed to build AI teams. Agents are specialized workers with specific roles and capabilities, able to call tools and complete tasks. Workflows define how agents collaborate to achieve a goal, with workflow states representing the current state of the workflow. Providers handle requests to the LLM and responses. Tools extend agent capabilities by providing concrete actions they can perform. Execution involves running the workflow to completion, with options for custom execution and BDD testing.
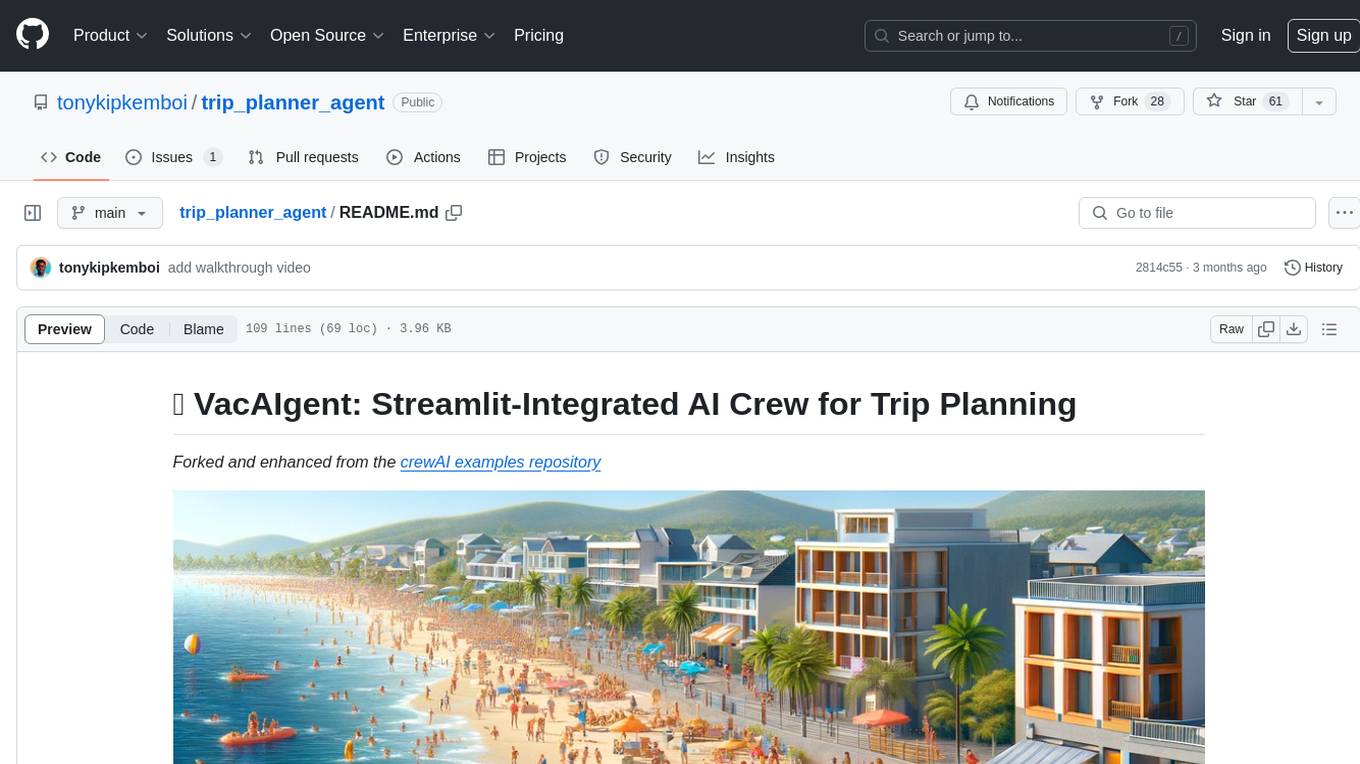
trip_planner_agent
VacAIgent is an AI tool that automates and enhances trip planning by leveraging the CrewAI framework. It integrates a user-friendly Streamlit interface for interactive travel planning. Users can input preferences and receive tailored travel plans with the help of autonomous AI agents. The tool allows for collaborative decision-making on cities and crafting complete itineraries based on specified preferences, all accessible via a streamlined Streamlit user interface. VacAIgent can be customized to use different AI models like GPT-3.5 or local models like Ollama for enhanced privacy and customization.
For similar jobs
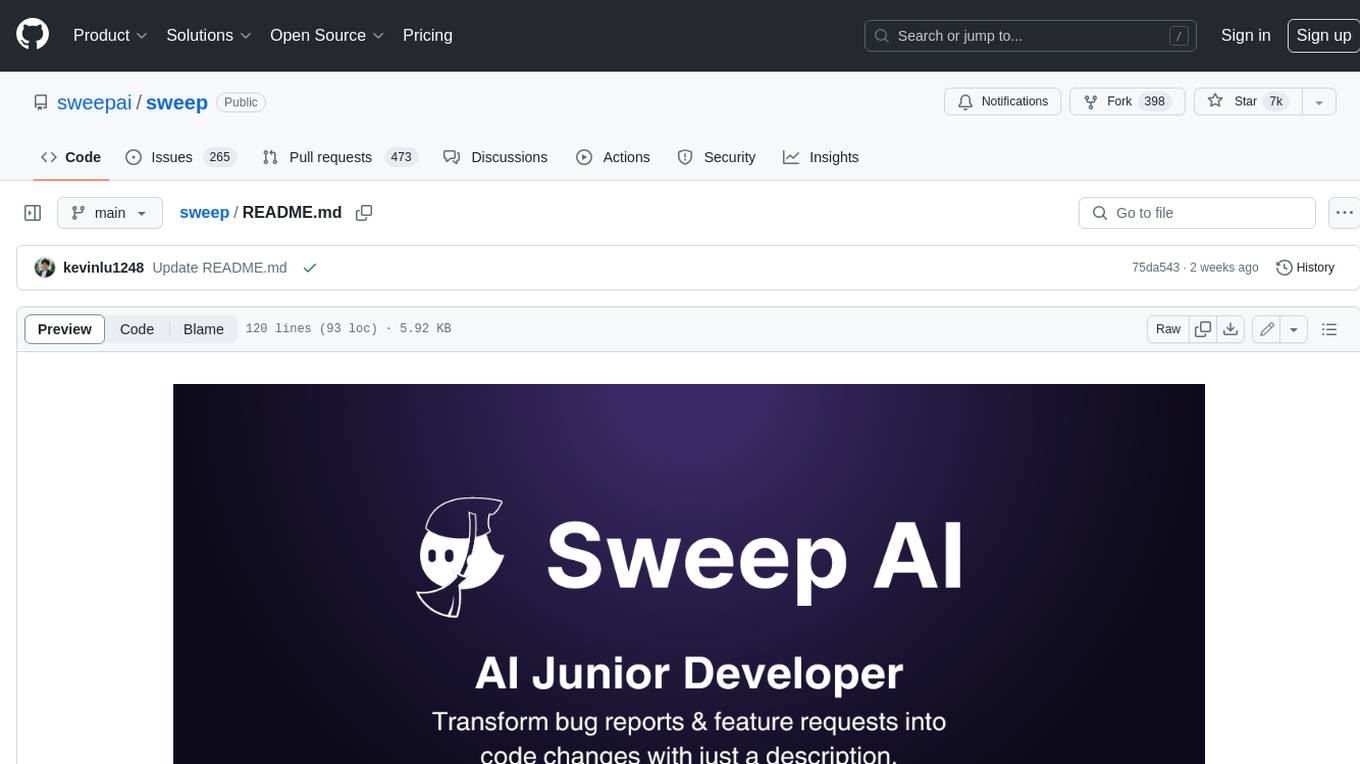
sweep
Sweep is an AI junior developer that turns bugs and feature requests into code changes. It automatically handles developer experience improvements like adding type hints and improving test coverage.
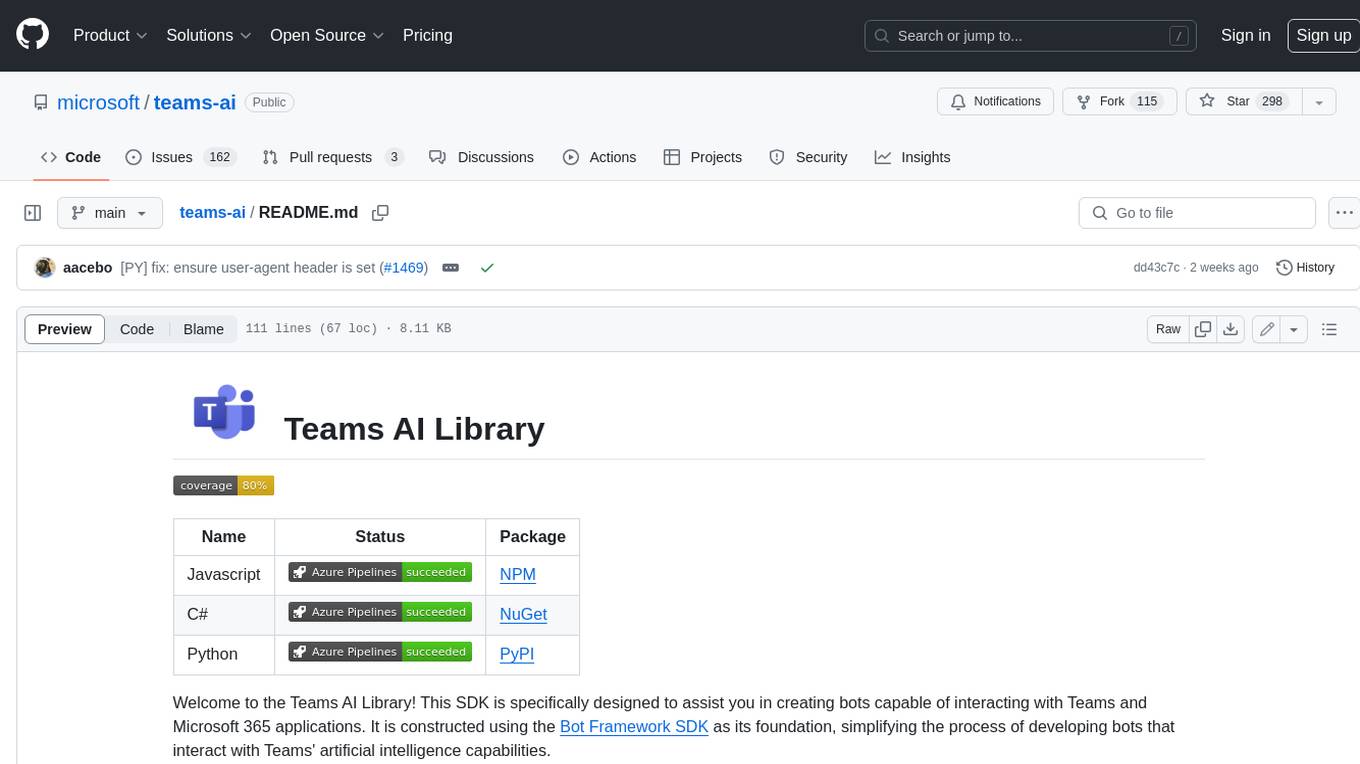
teams-ai
The Teams AI Library is a software development kit (SDK) that helps developers create bots that can interact with Teams and Microsoft 365 applications. It is built on top of the Bot Framework SDK and simplifies the process of developing bots that interact with Teams' artificial intelligence capabilities. The SDK is available for JavaScript/TypeScript, .NET, and Python.
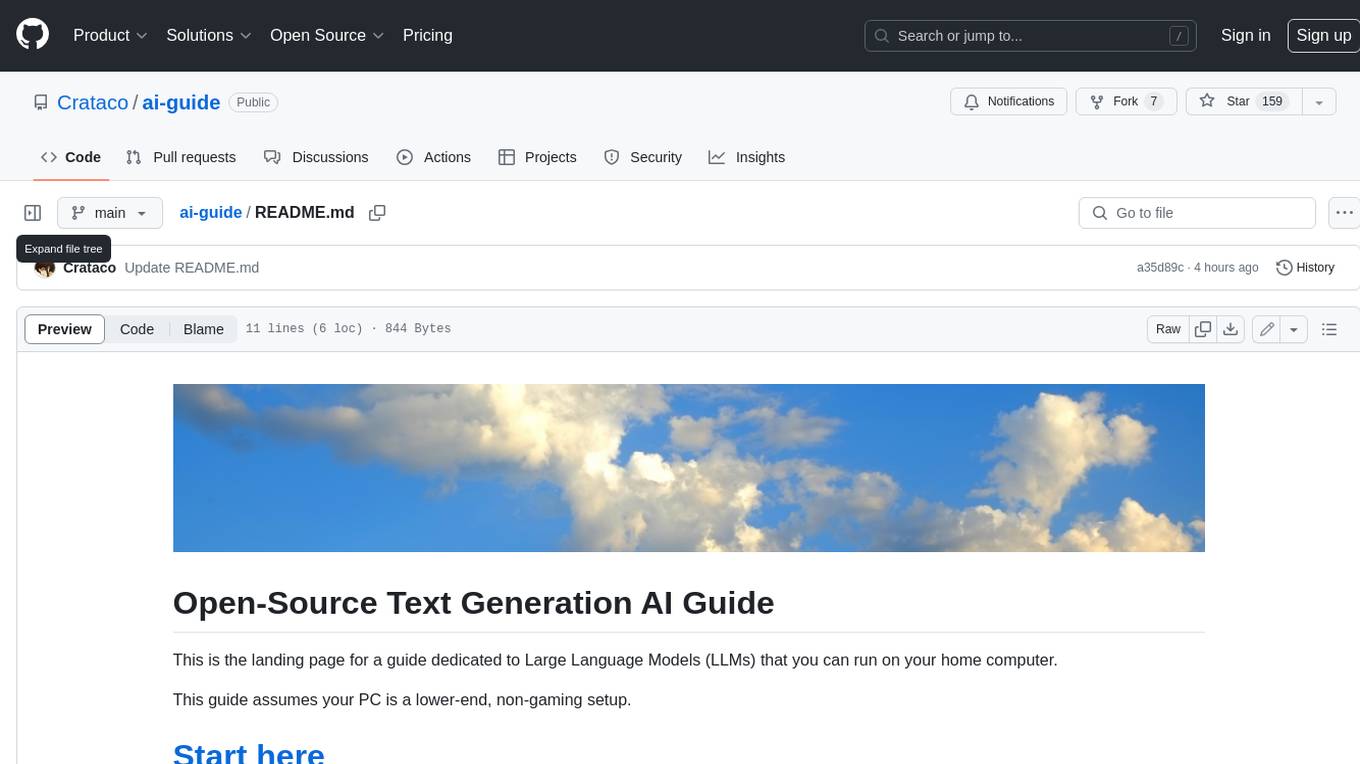
ai-guide
This guide is dedicated to Large Language Models (LLMs) that you can run on your home computer. It assumes your PC is a lower-end, non-gaming setup.
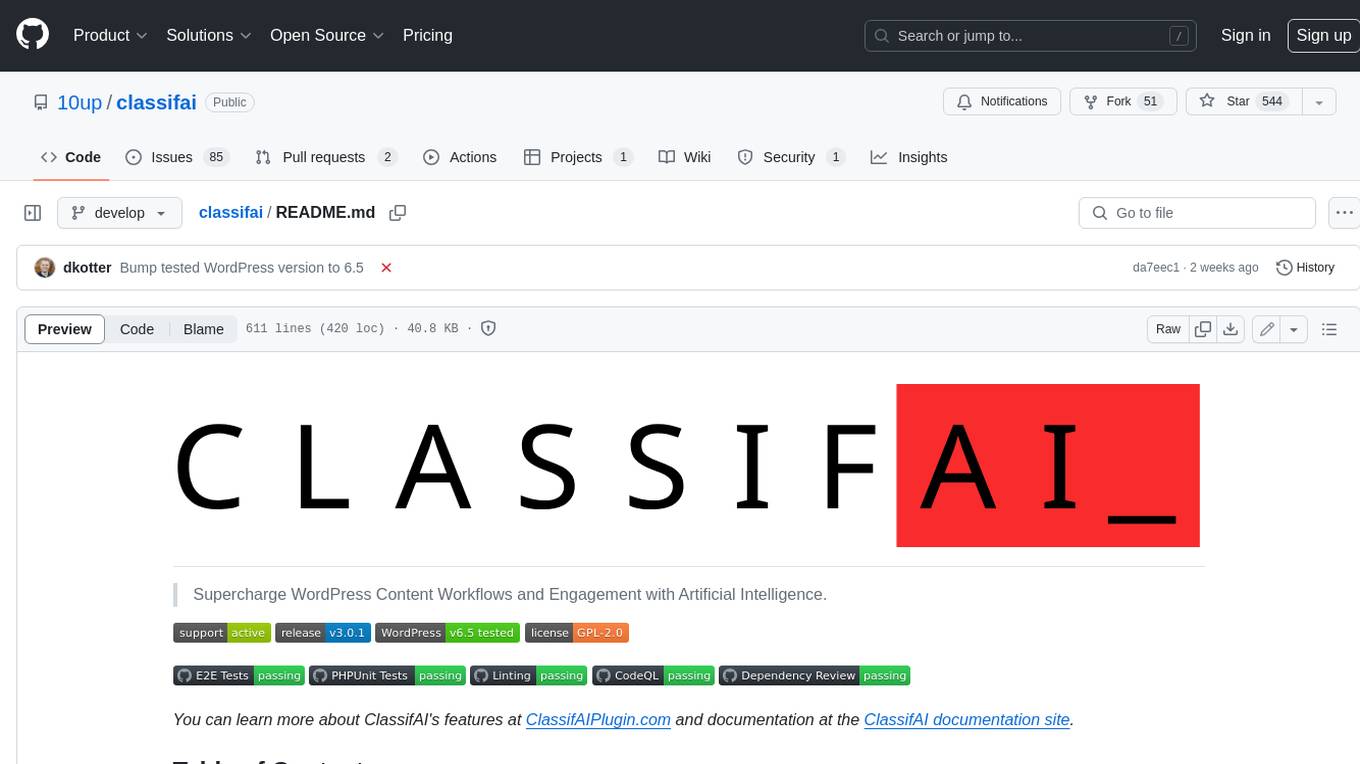
classifai
Supercharge WordPress Content Workflows and Engagement with Artificial Intelligence. Tap into leading cloud-based services like OpenAI, Microsoft Azure AI, Google Gemini and IBM Watson to augment your WordPress-powered websites. Publish content faster while improving SEO performance and increasing audience engagement. ClassifAI integrates Artificial Intelligence and Machine Learning technologies to lighten your workload and eliminate tedious tasks, giving you more time to create original content that matters.
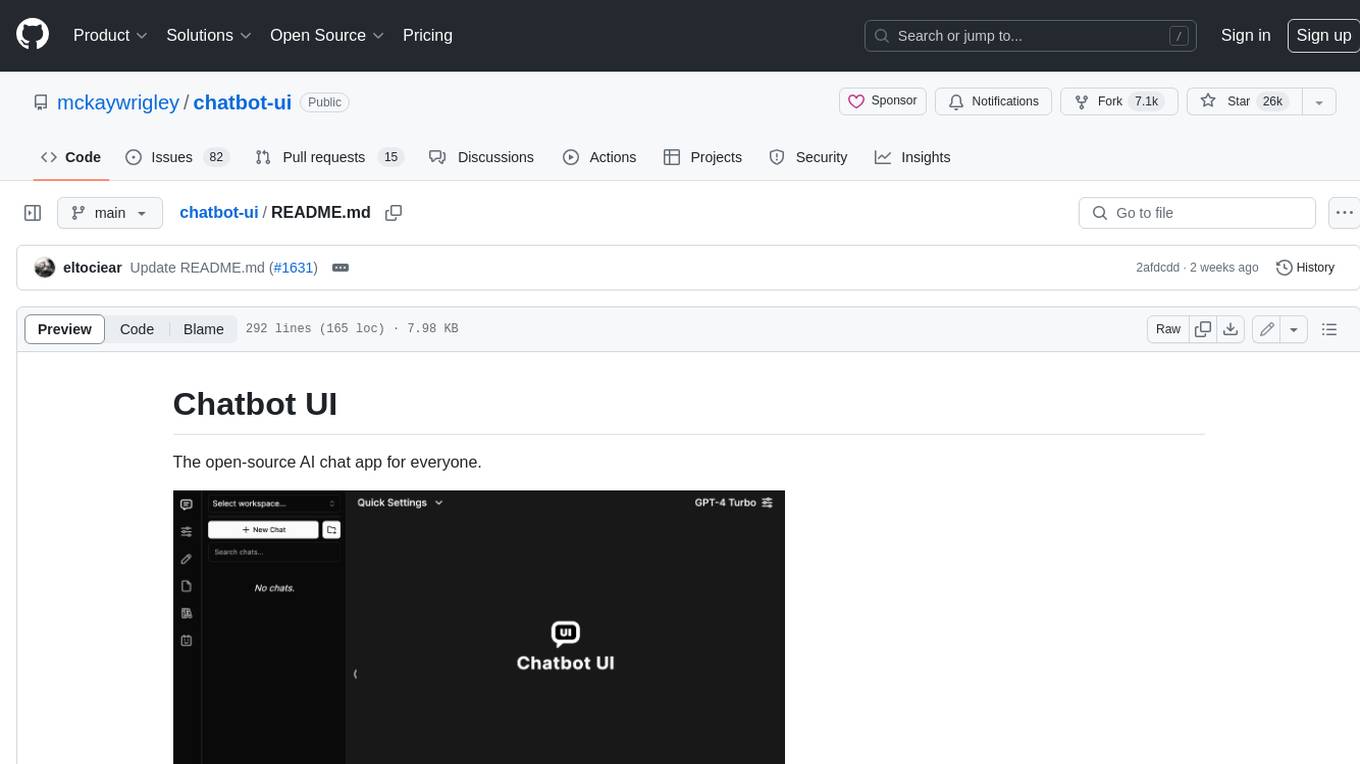
chatbot-ui
Chatbot UI is an open-source AI chat app that allows users to create and deploy their own AI chatbots. It is easy to use and can be customized to fit any need. Chatbot UI is perfect for businesses, developers, and anyone who wants to create a chatbot.
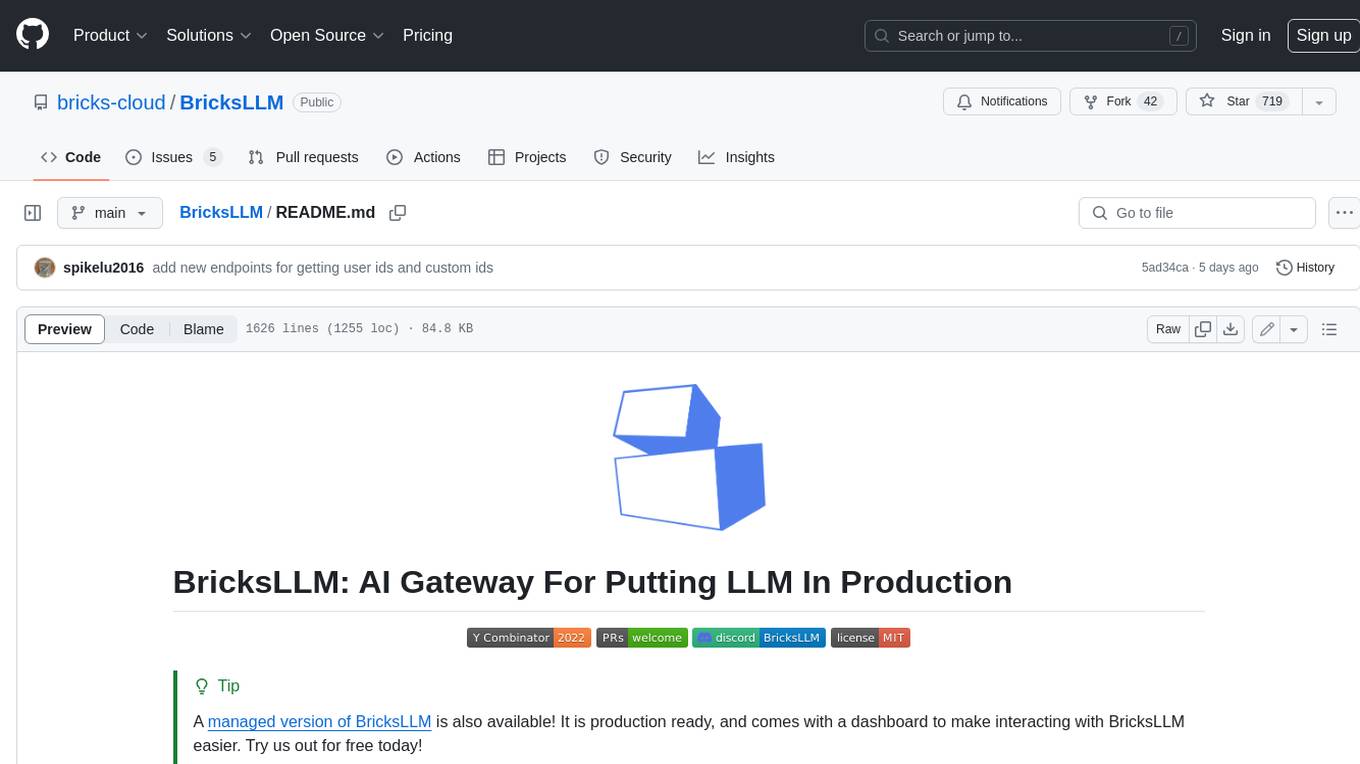
BricksLLM
BricksLLM is a cloud native AI gateway written in Go. Currently, it provides native support for OpenAI, Anthropic, Azure OpenAI and vLLM. BricksLLM aims to provide enterprise level infrastructure that can power any LLM production use cases. Here are some use cases for BricksLLM: * Set LLM usage limits for users on different pricing tiers * Track LLM usage on a per user and per organization basis * Block or redact requests containing PIIs * Improve LLM reliability with failovers, retries and caching * Distribute API keys with rate limits and cost limits for internal development/production use cases * Distribute API keys with rate limits and cost limits for students
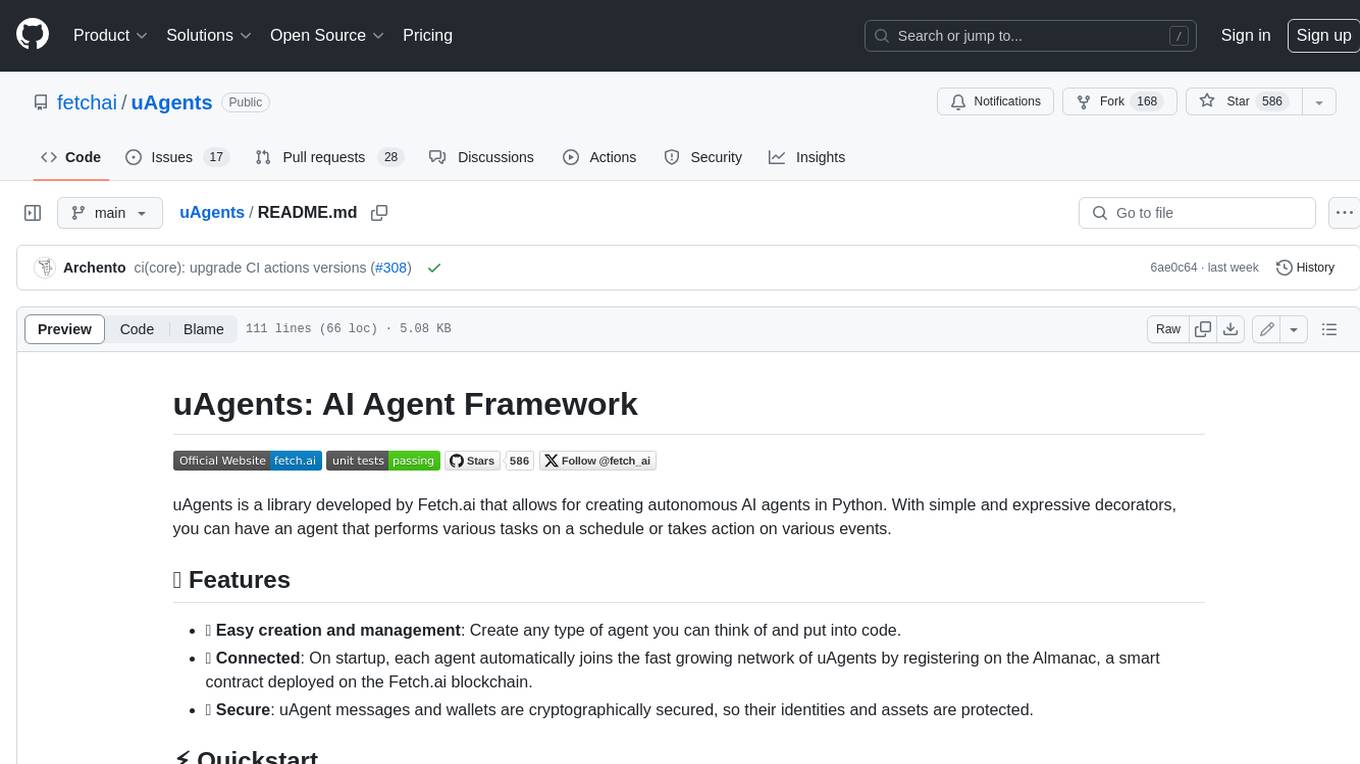
uAgents
uAgents is a Python library developed by Fetch.ai that allows for the creation of autonomous AI agents. These agents can perform various tasks on a schedule or take action on various events. uAgents are easy to create and manage, and they are connected to a fast-growing network of other uAgents. They are also secure, with cryptographically secured messages and wallets.
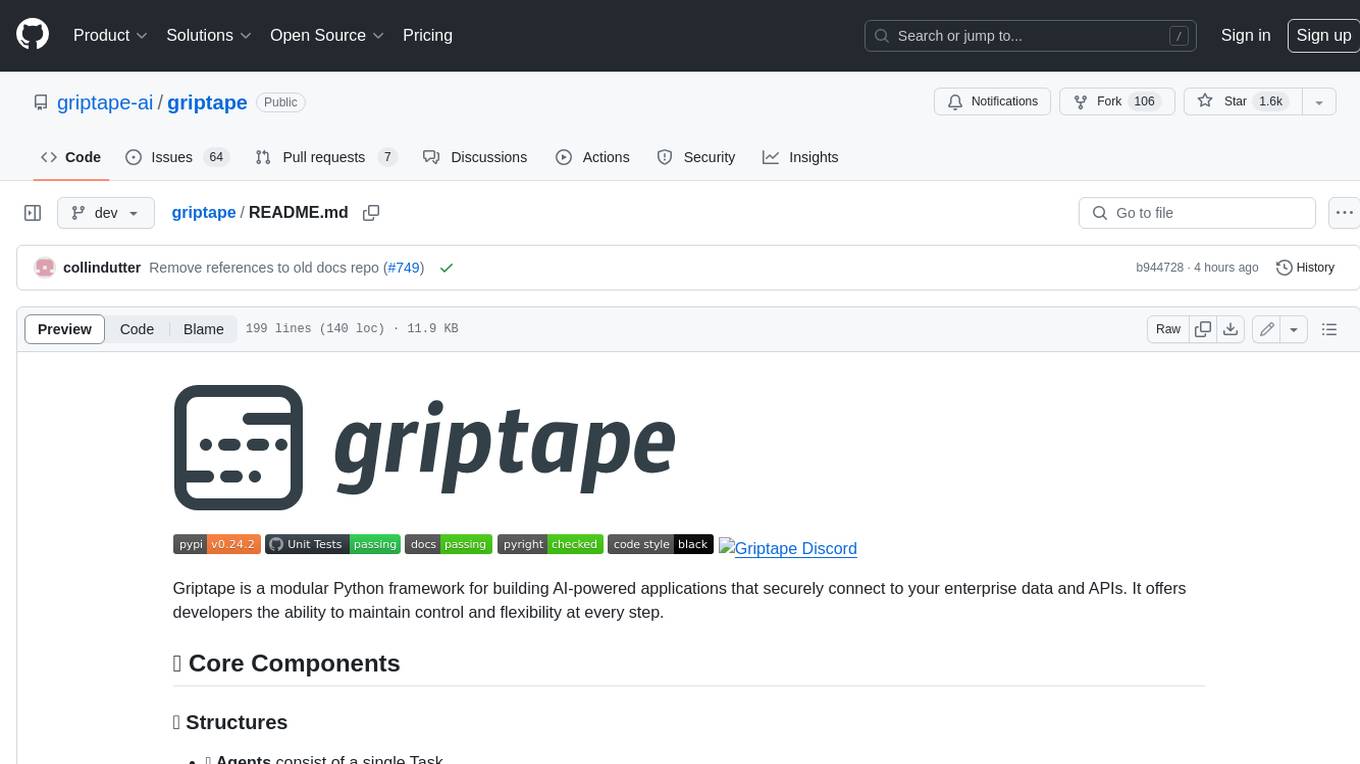
griptape
Griptape is a modular Python framework for building AI-powered applications that securely connect to your enterprise data and APIs. It offers developers the ability to maintain control and flexibility at every step. Griptape's core components include Structures (Agents, Pipelines, and Workflows), Tasks, Tools, Memory (Conversation Memory, Task Memory, and Meta Memory), Drivers (Prompt and Embedding Drivers, Vector Store Drivers, Image Generation Drivers, Image Query Drivers, SQL Drivers, Web Scraper Drivers, and Conversation Memory Drivers), Engines (Query Engines, Extraction Engines, Summary Engines, Image Generation Engines, and Image Query Engines), and additional components (Rulesets, Loaders, Artifacts, Chunkers, and Tokenizers). Griptape enables developers to create AI-powered applications with ease and efficiency.