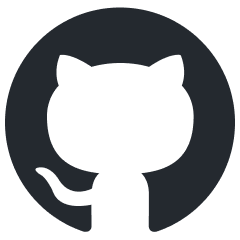
CoML
Interactive coding assistant for data scientists and machine learning developers, empowered by large language models.
Stars: 57
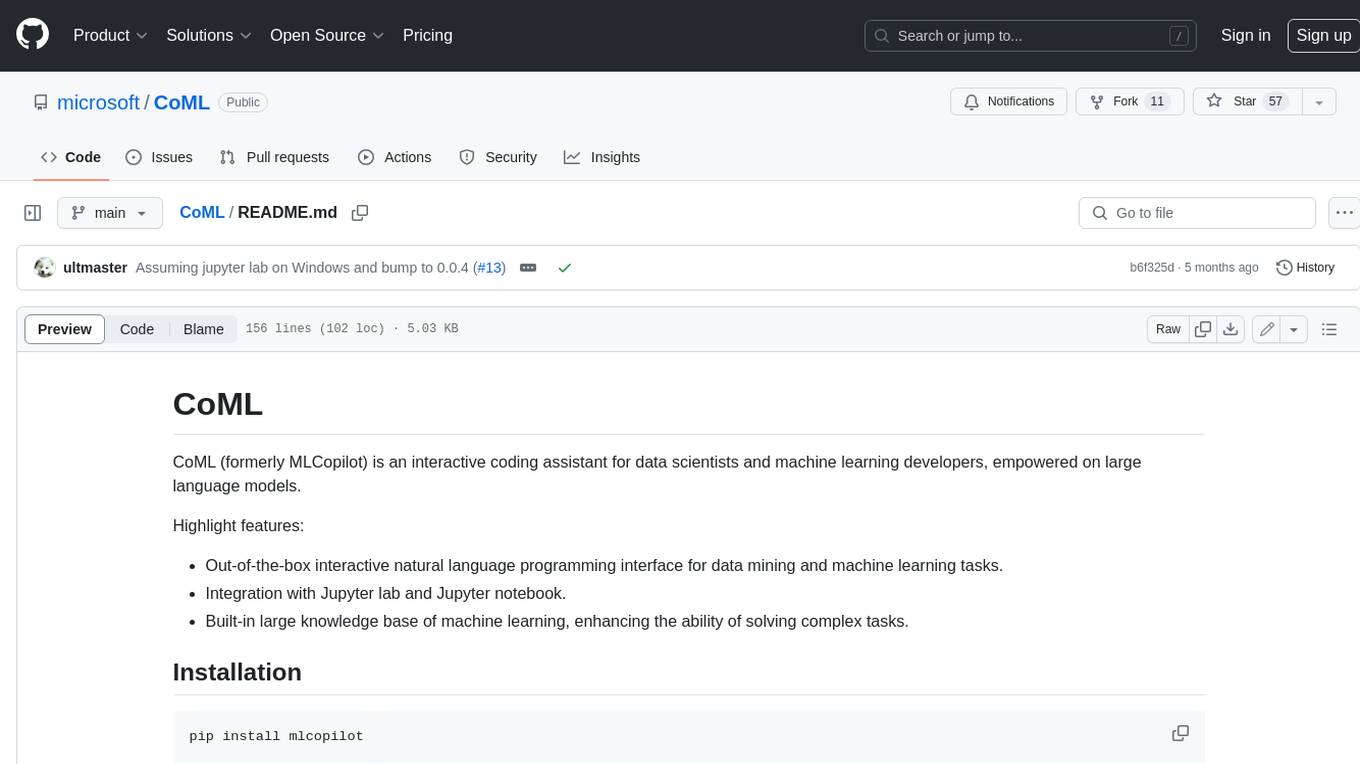
CoML (formerly MLCopilot) is an interactive coding assistant for data scientists and machine learning developers, empowered on large language models. It offers an out-of-the-box interactive natural language programming interface for data mining and machine learning tasks, integration with Jupyter lab and Jupyter notebook, and a built-in large knowledge base of machine learning to enhance the ability to solve complex tasks. The tool is designed to assist users in coding tasks related to data analysis and machine learning using natural language commands within Jupyter environments.
README:
CoML (formerly MLCopilot) is an interactive coding assistant for data scientists and machine learning developers, empowered on large language models.
Highlight features:
- Out-of-the-box interactive natural language programming interface for data mining and machine learning tasks.
- Integration with Jupyter lab and Jupyter notebook.
- Built-in large knowledge base of machine learning, enhancing the ability of solving complex tasks.
pip install mlcopilot
(We can't have the name coml
on PyPI, so we use mlcopilot
instead.)
We recommend trying CoML in a Jupyter Lab environment. Before using CoML, please make sure that:
- You have exported
OPENAI_API_KEY=sk-xxxx
in your environment. Alternatively, you can also use a.env
file. - Use
%load_ext coml
in your notebook to active CoML extension.
Then we have provided several commands to assist your journey with interactive coding in Jupyter Lab.
-
%coml <task>
to prompt CoML to write a cell for your task.
-
%comlfix
to fix the cell just above the current cell. You can also use%comlfix <reason>
to provide details for what's wrong.
-
%comlinspire
to inspire you with a cell describing what to do next.
Limitations:
- Currently, CoML only supports Jupyter Lab and classical Jupyter notebook (nbclassic, and only on Linux platforms). We are still working on supports of newer Jupyter notebook, Jupyter-vscode and Google Colab.
- CoML uses gpt-3.5-turbo-16k model in its implementation. There is no way to change the model for now. The cost of using this model is around $0.04 per request. Please be aware of this cost.
CoML config agent is the implementation of MLCopilot, which can suggest a ML configuration within a specific task, for a specific task. Currently, it is an independent component residing in coml.configagent
. In the future, we will integrate it into the CoML system.
(TODO: The demo needs an update.)
Please follow the steps to use CoML config agent:
- Clone this repo:
git clone REPO_URL; cd coml
- Put assets/coml.db in your home directory:
cp assets/coml.db ~/.coml/coml.db
- Copy
coml/.env.template
to~/.coml/.env
and put your API keys in the file.
Currently, it can only be invoked independently. You can use the following command line:
coml-configagent --space <space> --task <task>
If you feel uncertain about what to put into <space>
or <task>
, see the demo above, or try the interactive usage below:
coml-configagent --interactive
from coml.configagent.suggest import suggest
space = import_space("YOUR_SPACE_ID")
task_desc = "YOUR_TASK_DESCRIPTION_FOR_NEW_TASK"
suggest_configs, knowledge = suggest(space, task_desc)
Development documentation stays here for now. It shall be moved to a separate document later.
Important files and folders:
CoML
├── assets # data, examples, demos
├── coml # Python package
├── examples # example scripts
├── install.json # Jupyter lab extension installation file
├── package.json # Jupyter lab extension package file
├── pyproject.toml # Python package configuration
├── src # Jupyter lab extension source code
├── test # Python package tests
└── tsconfig.json # Jupyter lab extension TypeScript configuration
You can use the following command for development installation:
pip install -e .[dev]
If you are to develop Jupyter lab extension, you will also need to install NodeJS and npm, and run the following command:
# Link your development version of the extension with JupyterLab
jupyter labextension develop . --overwrite
# Rebuild extension Typescript source after making changes
jlpm run build
To uninstall, you can run the following commands:
# Server extension must be manually disabled in develop mode
jupyter server extension disable coml
# Uninstall the Python package
pip uninstall mlcopilot
In development mode, you will also need to remove the symlink created by jupyter labextension develop
command.
To find its location, you can run jupyter labextension list
to figure out where the labextensions
folder is located.
Then you can remove the symlink named coml
within that folder.
hatch build
If you find this work useful, please cite the paper as below:
@article{zhang2023mlcopilot,
title={MLCopilot: Unleashing the Power of Large Language Models in Solving Machine Learning Tasks},
author={Zhang, Lei and Zhang, Yuge and Ren, Kan and Li, Dongsheng and Yang, Yuqing},
journal={arXiv preprint arXiv:2304.14979},
year={2023}
}
The entire codebase is under MIT license.
For Tasks:
Click tags to check more tools for each tasksFor Jobs:
Alternative AI tools for CoML
Similar Open Source Tools
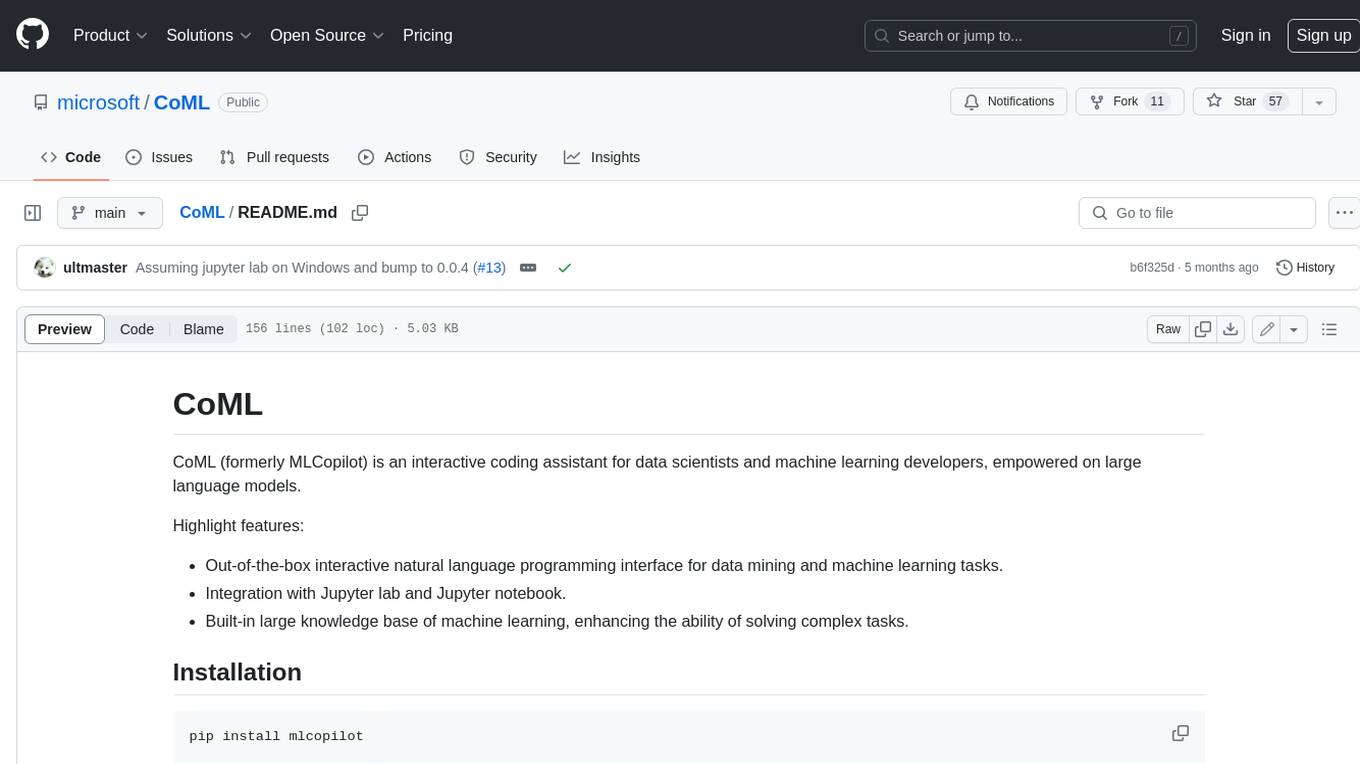
CoML
CoML (formerly MLCopilot) is an interactive coding assistant for data scientists and machine learning developers, empowered on large language models. It offers an out-of-the-box interactive natural language programming interface for data mining and machine learning tasks, integration with Jupyter lab and Jupyter notebook, and a built-in large knowledge base of machine learning to enhance the ability to solve complex tasks. The tool is designed to assist users in coding tasks related to data analysis and machine learning using natural language commands within Jupyter environments.
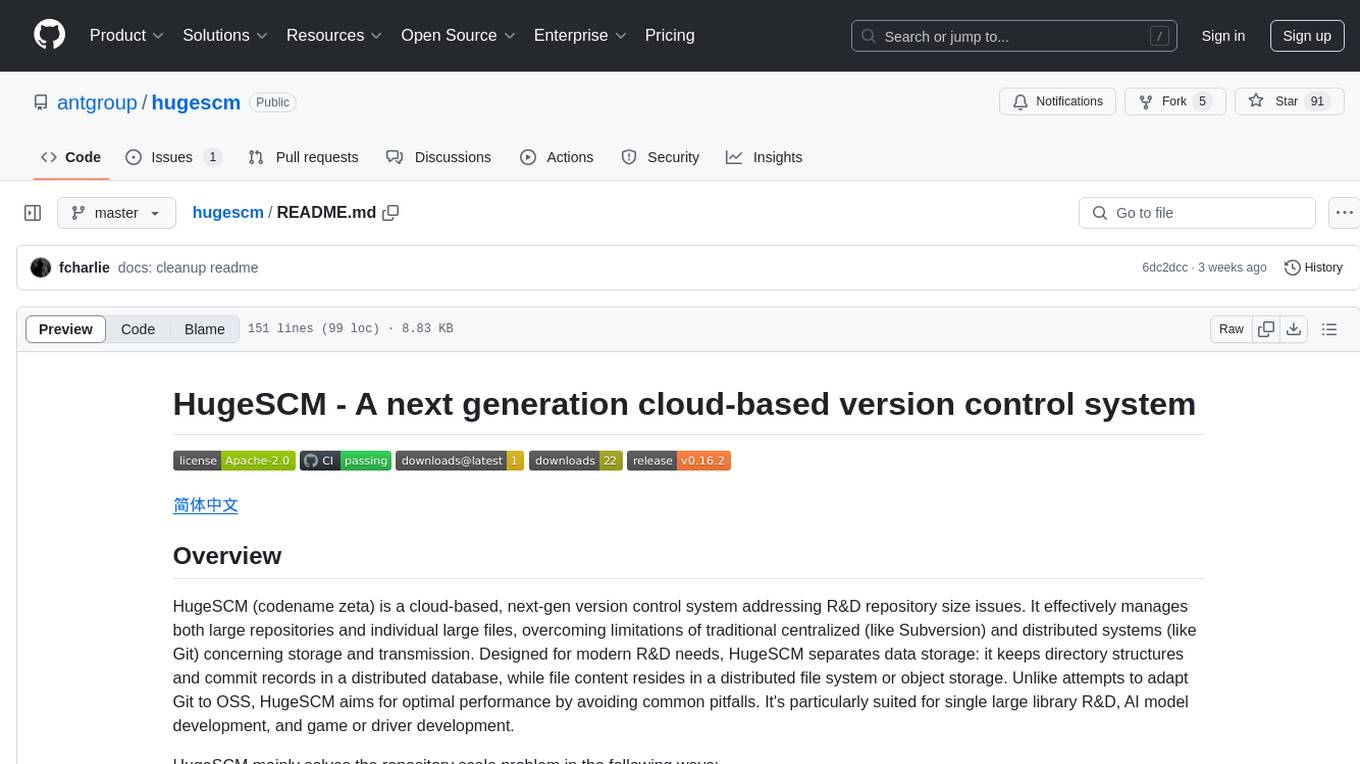
hugescm
HugeSCM is a cloud-based version control system designed to address R&D repository size issues. It effectively manages large repositories and individual large files by separating data storage and utilizing advanced algorithms and data structures. It aims for optimal performance in handling version control operations of large-scale repositories, making it suitable for single large library R&D, AI model development, and game or driver development.
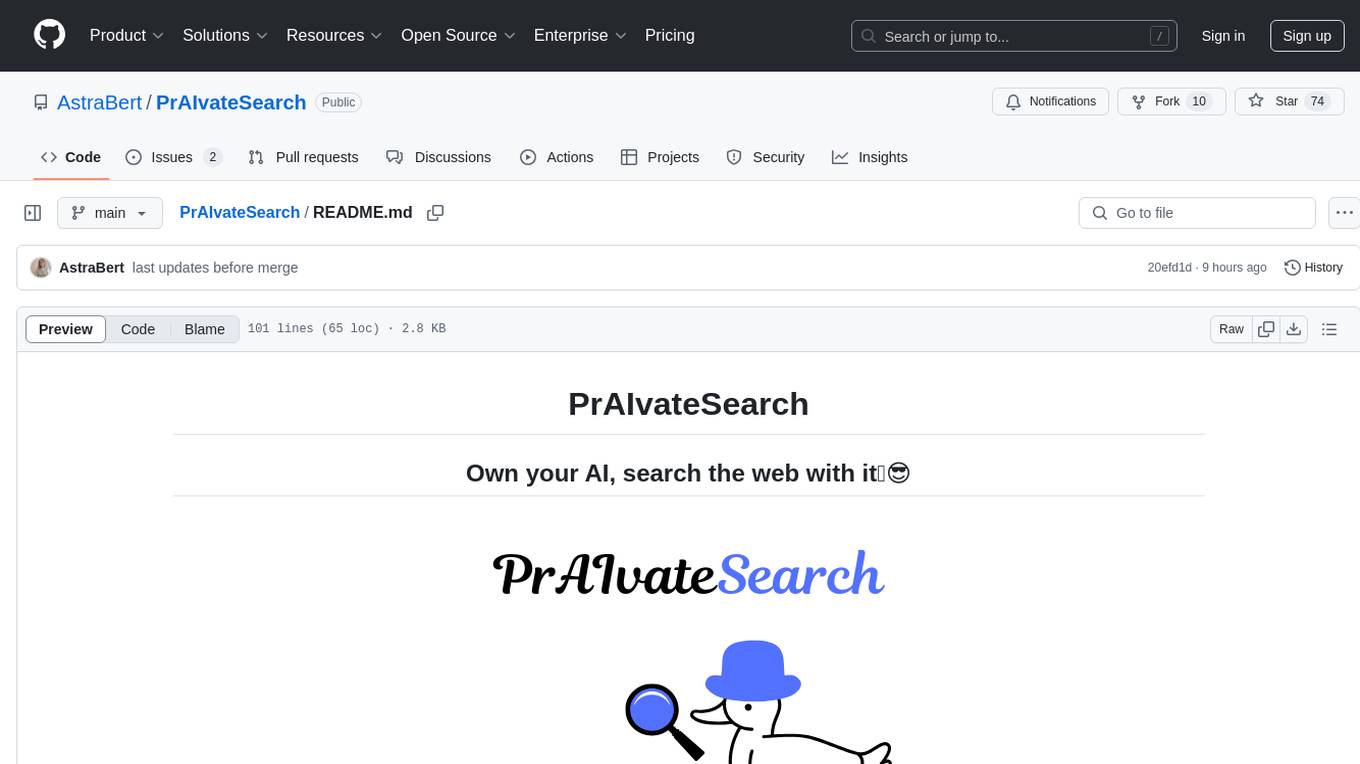
PrAIvateSearch
PrAIvateSearch is a NextJS web application that aims to implement similar features to SearchGPT in an open-source, local, and private way. It allows users to search the web using their own AI model. The application provides a user-friendly interface for interacting with the AI model and accessing search results. PrAIvateSearch is designed to be easy to install and use, with detailed instructions provided in the readme file. The project is in beta stage and welcomes contributions from the community to improve and enhance its functionality. Users are encouraged to support the project through funding to help it grow and continue to be maintained as an open-source tool under the MIT license.
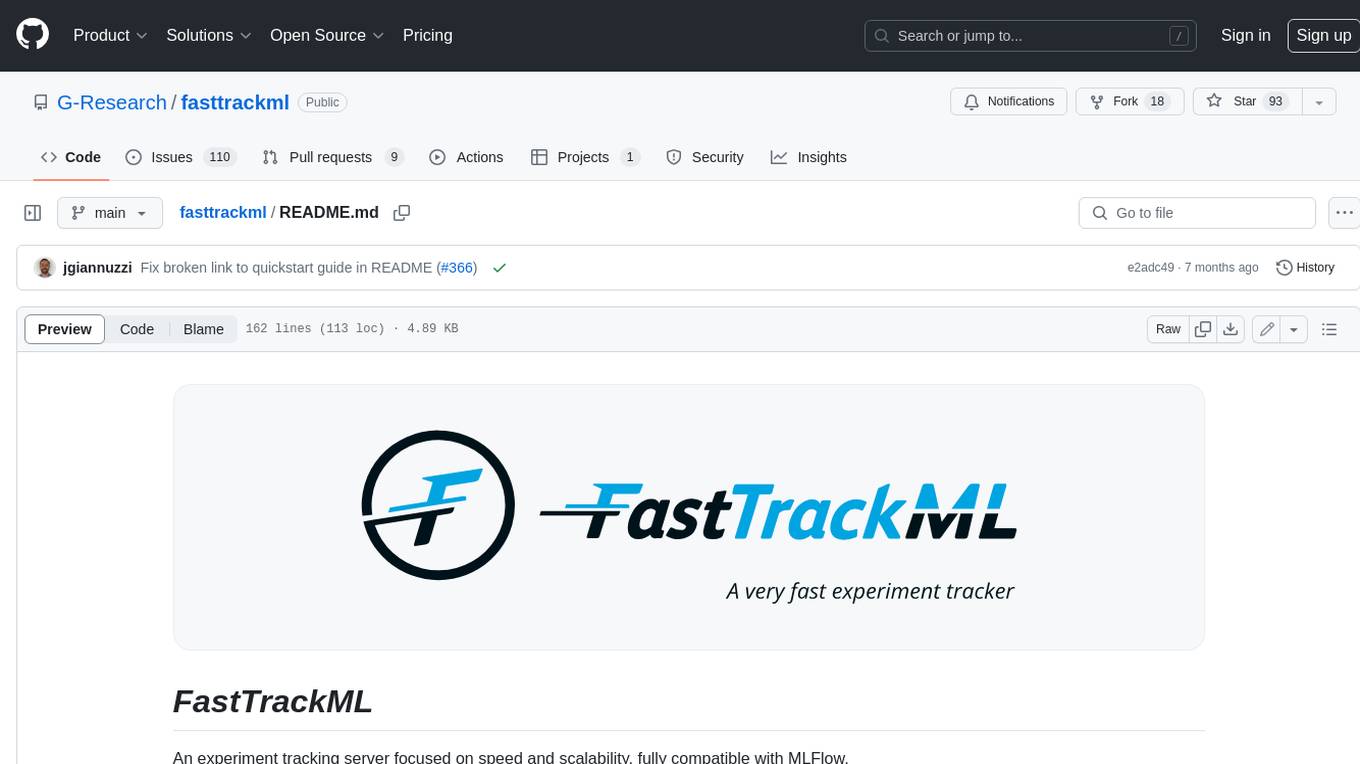
fasttrackml
FastTrackML is an experiment tracking server focused on speed and scalability, fully compatible with MLFlow. It provides a user-friendly interface to track and visualize your machine learning experiments, making it easy to compare different models and identify the best performing ones. FastTrackML is open source and can be easily installed and run with pip or Docker. It is also compatible with the MLFlow Python package, making it easy to integrate with your existing MLFlow workflows.
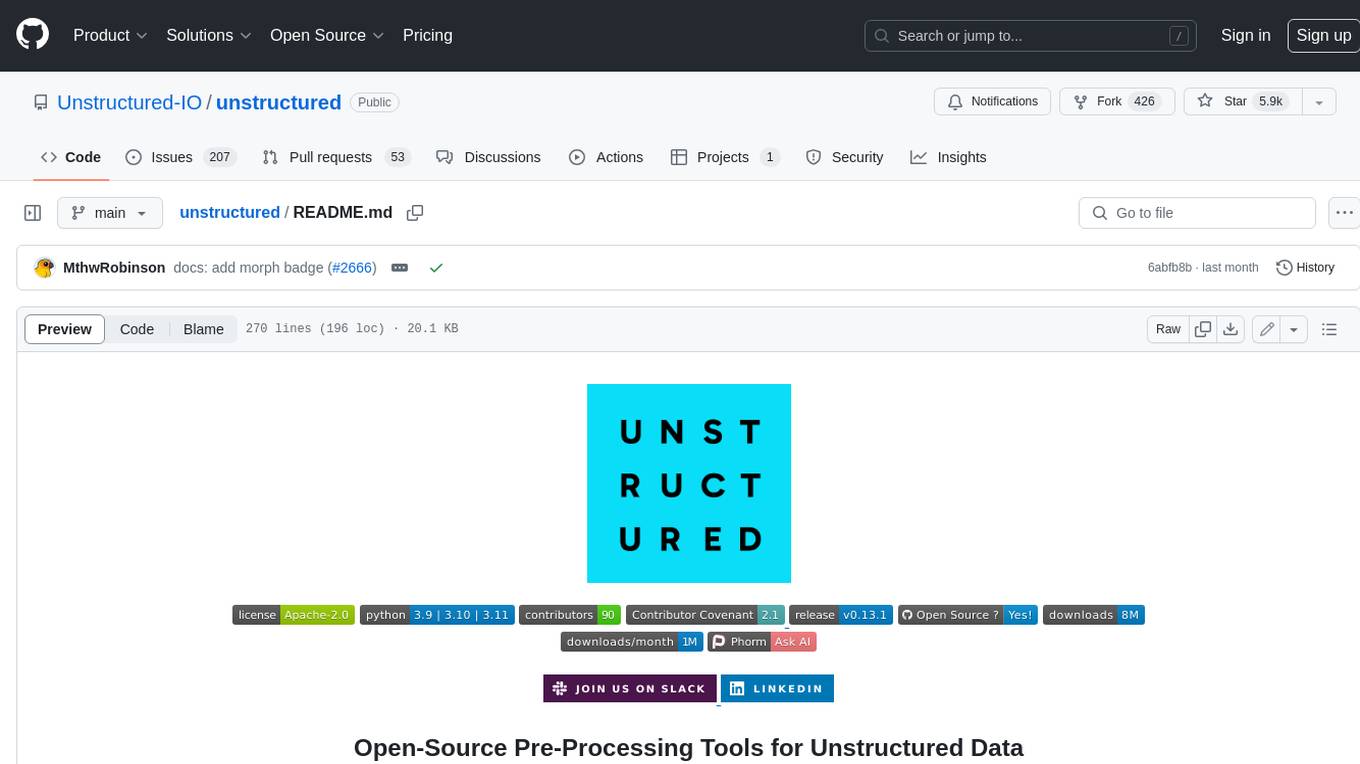
unstructured
The `unstructured` library provides open-source components for ingesting and pre-processing images and text documents, such as PDFs, HTML, Word docs, and many more. The use cases of `unstructured` revolve around streamlining and optimizing the data processing workflow for LLMs. `unstructured` modular functions and connectors form a cohesive system that simplifies data ingestion and pre-processing, making it adaptable to different platforms and efficient in transforming unstructured data into structured outputs.
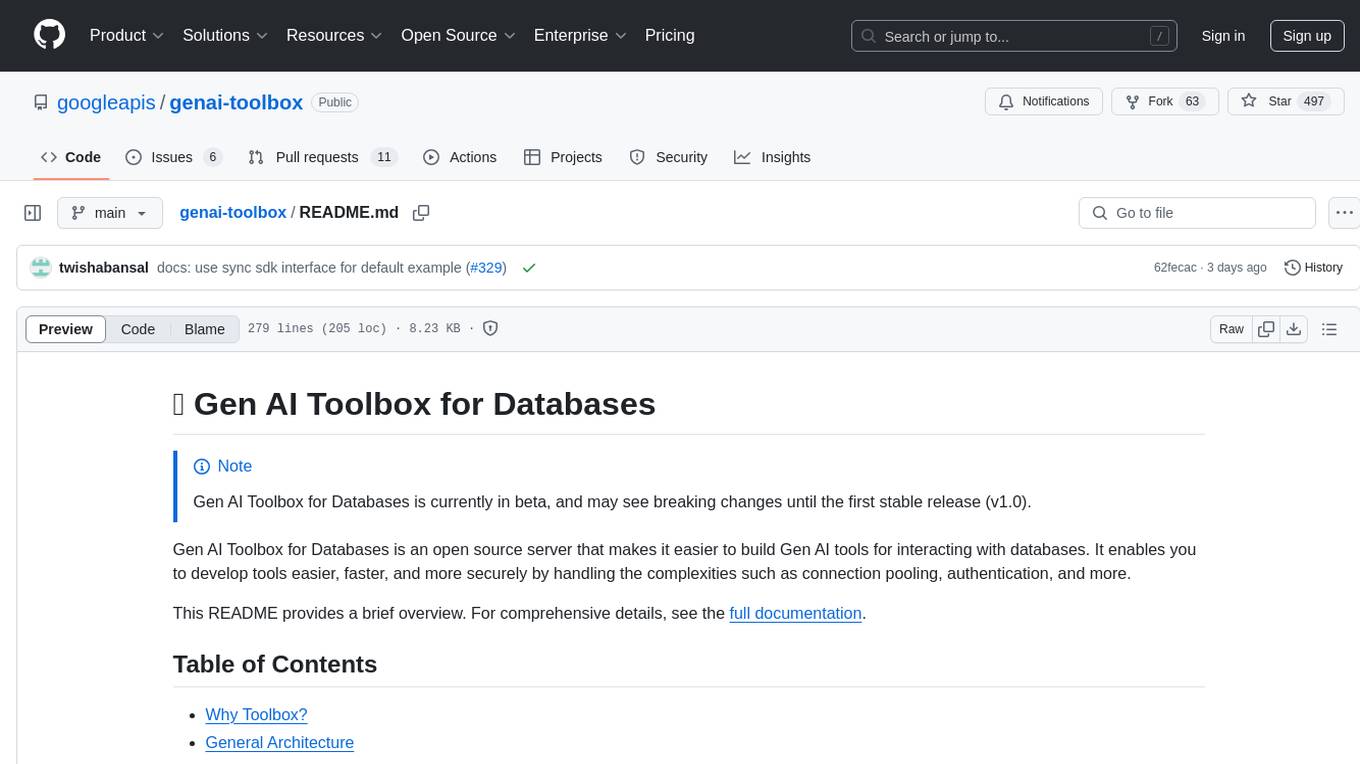
genai-toolbox
Gen AI Toolbox for Databases is an open source server that simplifies building Gen AI tools for interacting with databases. It handles complexities like connection pooling, authentication, and more, enabling easier, faster, and more secure tool development. The toolbox sits between the application's orchestration framework and the database, providing a control plane to modify, distribute, or invoke tools. It offers simplified development, better performance, enhanced security, and end-to-end observability. Users can install the toolbox as a binary, container image, or compile from source. Configuration is done through a 'tools.yaml' file, defining sources, tools, and toolsets. The project follows semantic versioning and welcomes contributions.
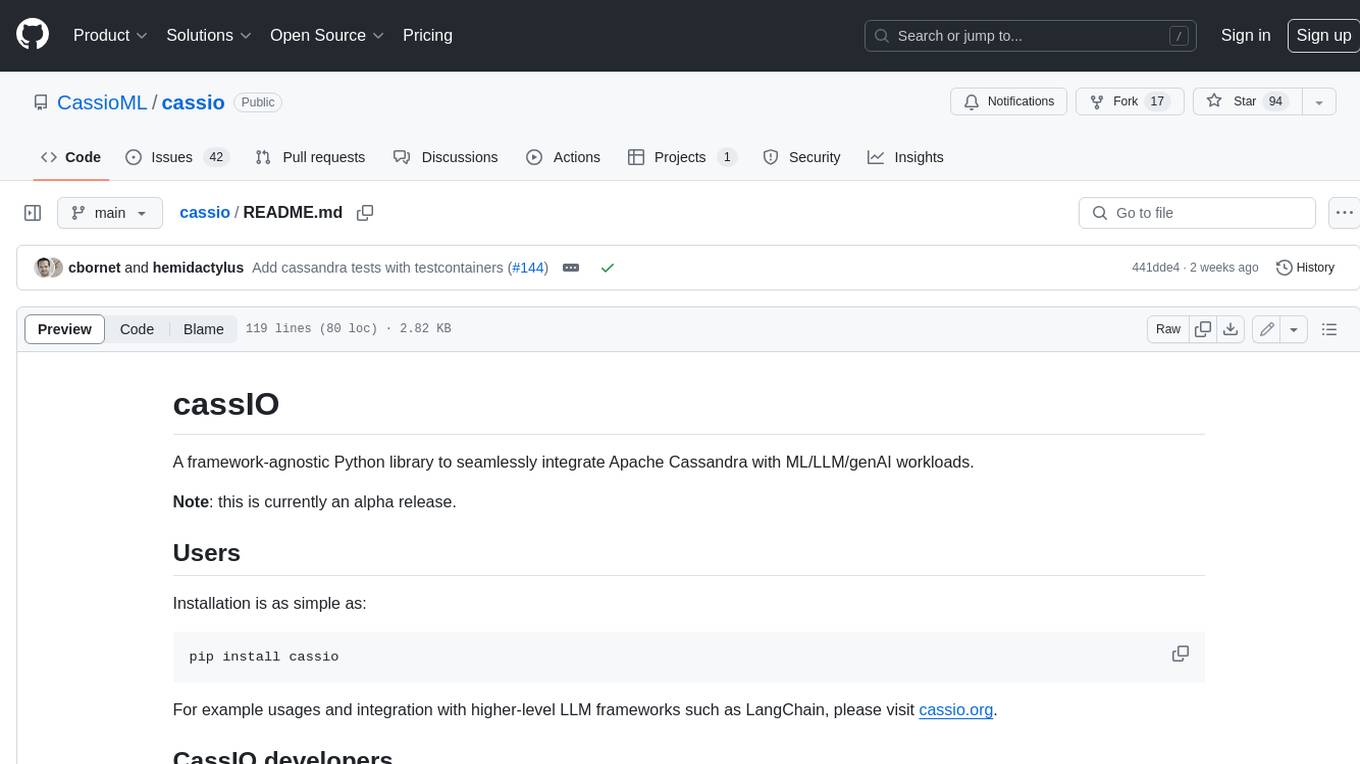
cassio
cassIO is a framework-agnostic Python library that seamlessly integrates Apache Cassandra with ML/LLM/genAI workloads. It provides an easy-to-use interface for developers to connect their Cassandra databases to machine learning models, allowing them to perform complex data analysis and AI-powered tasks directly on their Cassandra data. cassIO is designed to be flexible and extensible, making it suitable for a wide range of use cases, from data exploration and visualization to predictive modeling and natural language processing.
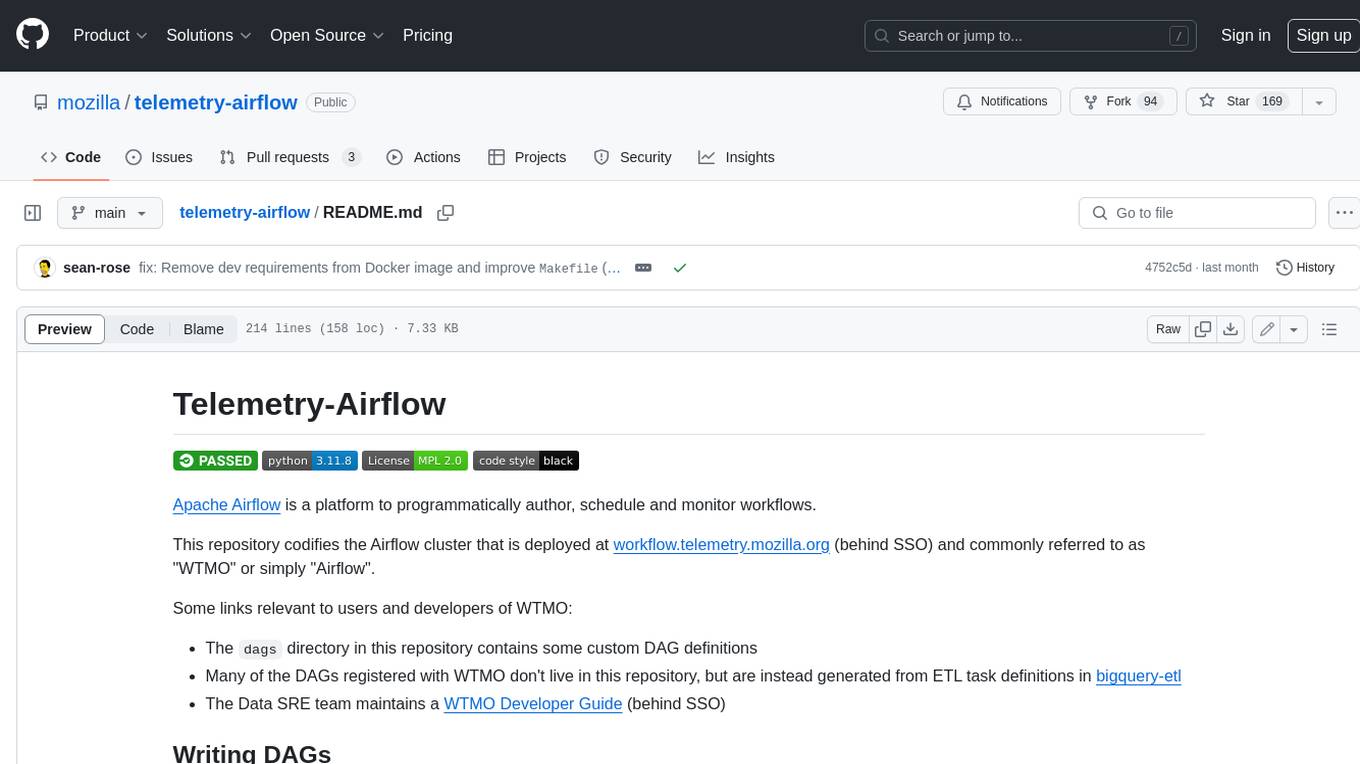
telemetry-airflow
This repository codifies the Airflow cluster that is deployed at workflow.telemetry.mozilla.org (behind SSO) and commonly referred to as "WTMO" or simply "Airflow". Some links relevant to users and developers of WTMO: * The `dags` directory in this repository contains some custom DAG definitions * Many of the DAGs registered with WTMO don't live in this repository, but are instead generated from ETL task definitions in bigquery-etl * The Data SRE team maintains a WTMO Developer Guide (behind SSO)
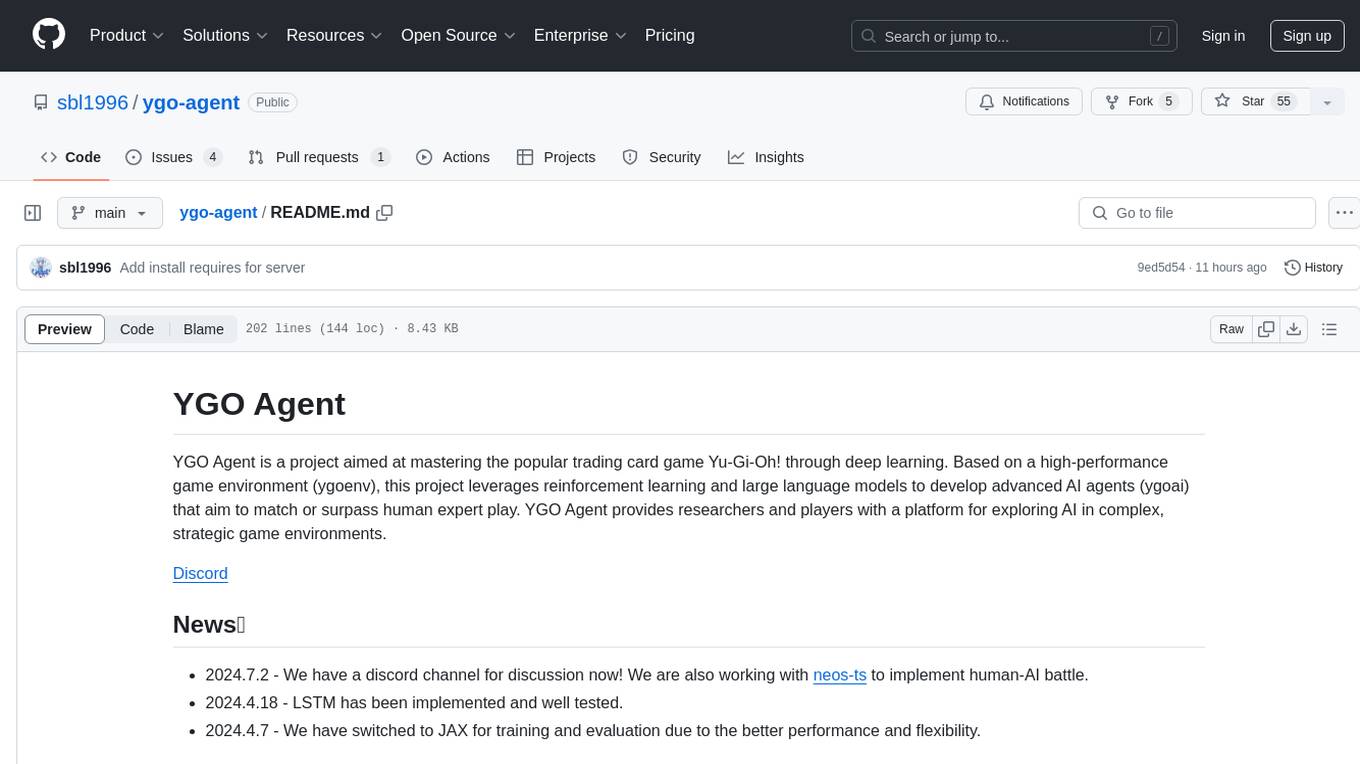
ygo-agent
YGO Agent is a project focused on using deep learning to master the Yu-Gi-Oh! trading card game. It utilizes reinforcement learning and large language models to develop advanced AI agents that aim to surpass human expert play. The project provides a platform for researchers and players to explore AI in complex, strategic game environments.
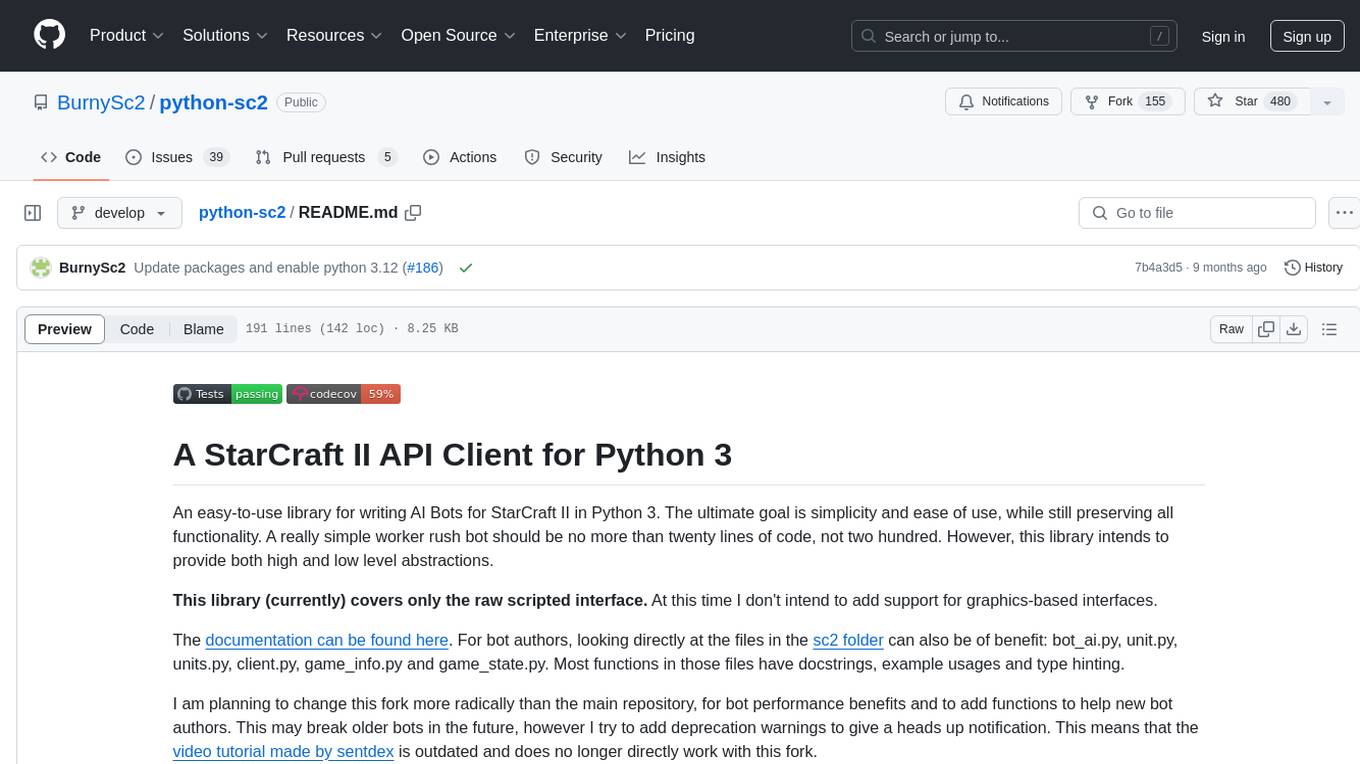
python-sc2
python-sc2 is an easy-to-use library for writing AI Bots for StarCraft II in Python 3. It aims for simplicity and ease of use while providing both high and low level abstractions. The library covers only the raw scripted interface and intends to help new bot authors with added functions. Users can install the library using pip and need a StarCraft II executable to run bots. The API configuration options allow users to customize bot behavior and performance. The community provides support through Discord servers, and users can contribute to the project by creating new issues or pull requests following style guidelines.
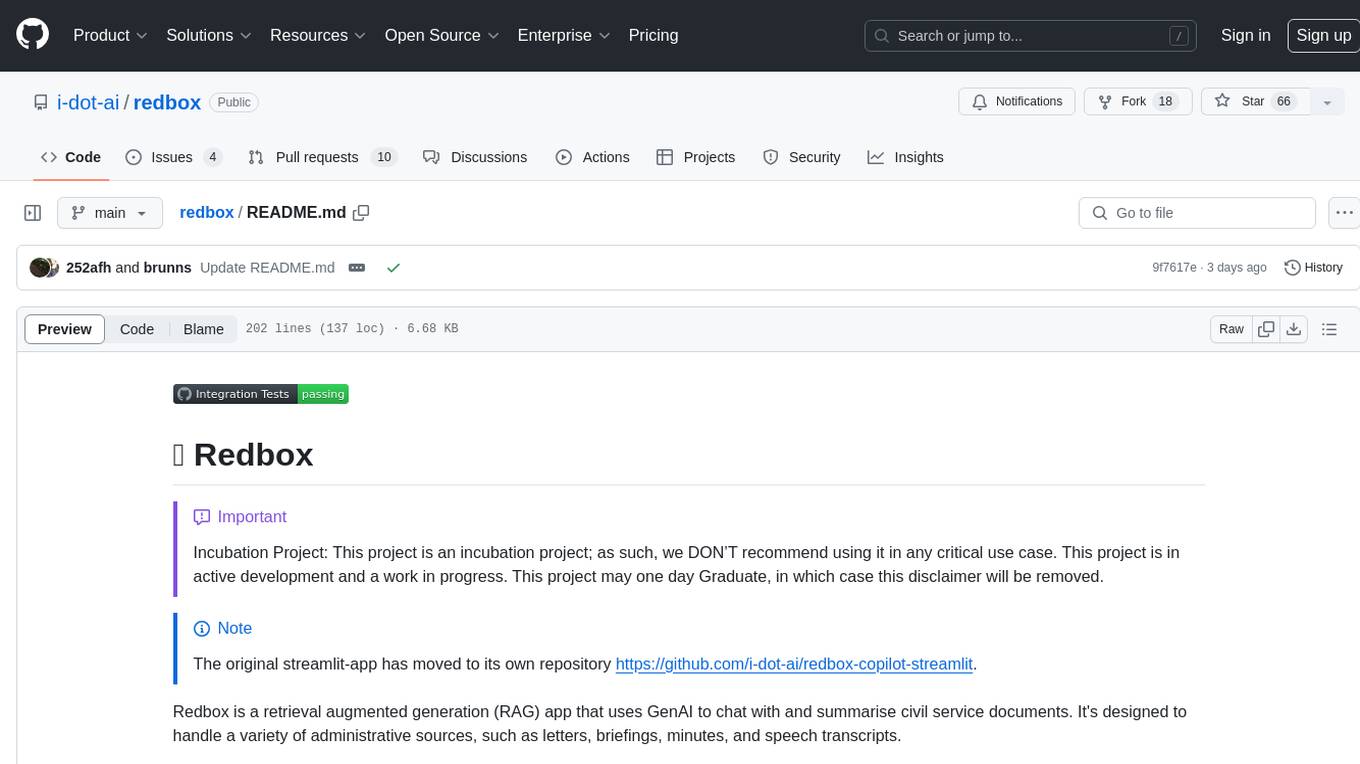
redbox
Redbox is a retrieval augmented generation (RAG) app that uses GenAI to chat with and summarise civil service documents. It increases organisational memory by indexing documents and can summarise reports read months ago, supplement them with current work, and produce a first draft that lets civil servants focus on what they do best. The project uses a microservice architecture with each microservice running in its own container defined by a Dockerfile. Dependencies are managed using Python Poetry. Contributions are welcome, and the project is licensed under the MIT License. Security measures are in place to ensure user data privacy and considerations are being made to make the core-api secure.
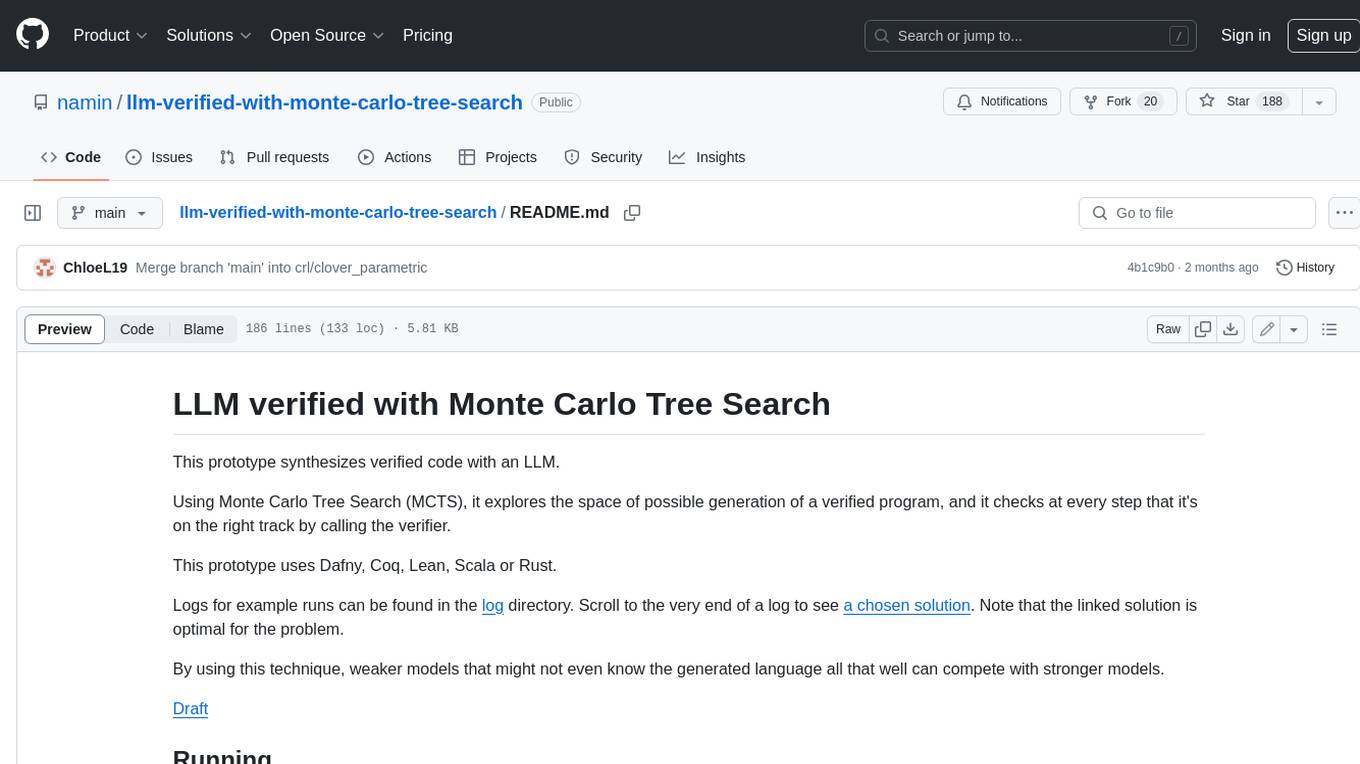
llm-verified-with-monte-carlo-tree-search
This prototype synthesizes verified code with an LLM using Monte Carlo Tree Search (MCTS). It explores the space of possible generation of a verified program and checks at every step that it's on the right track by calling the verifier. This prototype uses Dafny, Coq, Lean, Scala, or Rust. By using this technique, weaker models that might not even know the generated language all that well can compete with stronger models.
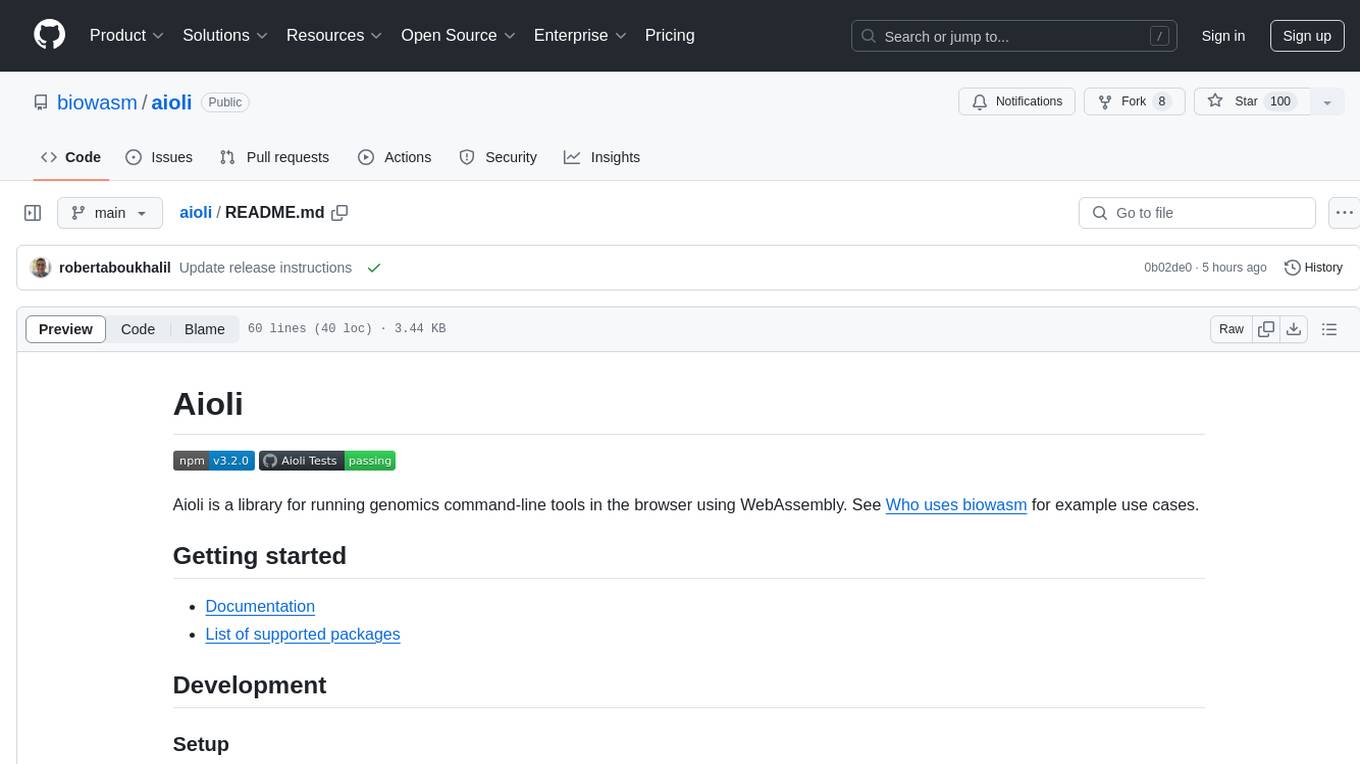
aioli
Aioli is a library for running genomics command-line tools in the browser using WebAssembly. It creates a single WebWorker to run all WebAssembly tools, shares a filesystem across modules, and efficiently mounts local files. The tool encapsulates each module for loading, does WebAssembly feature detection, and communicates with the WebWorker using the Comlink library. Users can deploy new releases and versions, and benefit from code reuse by porting existing C/C++/Rust/etc tools to WebAssembly for browser use.
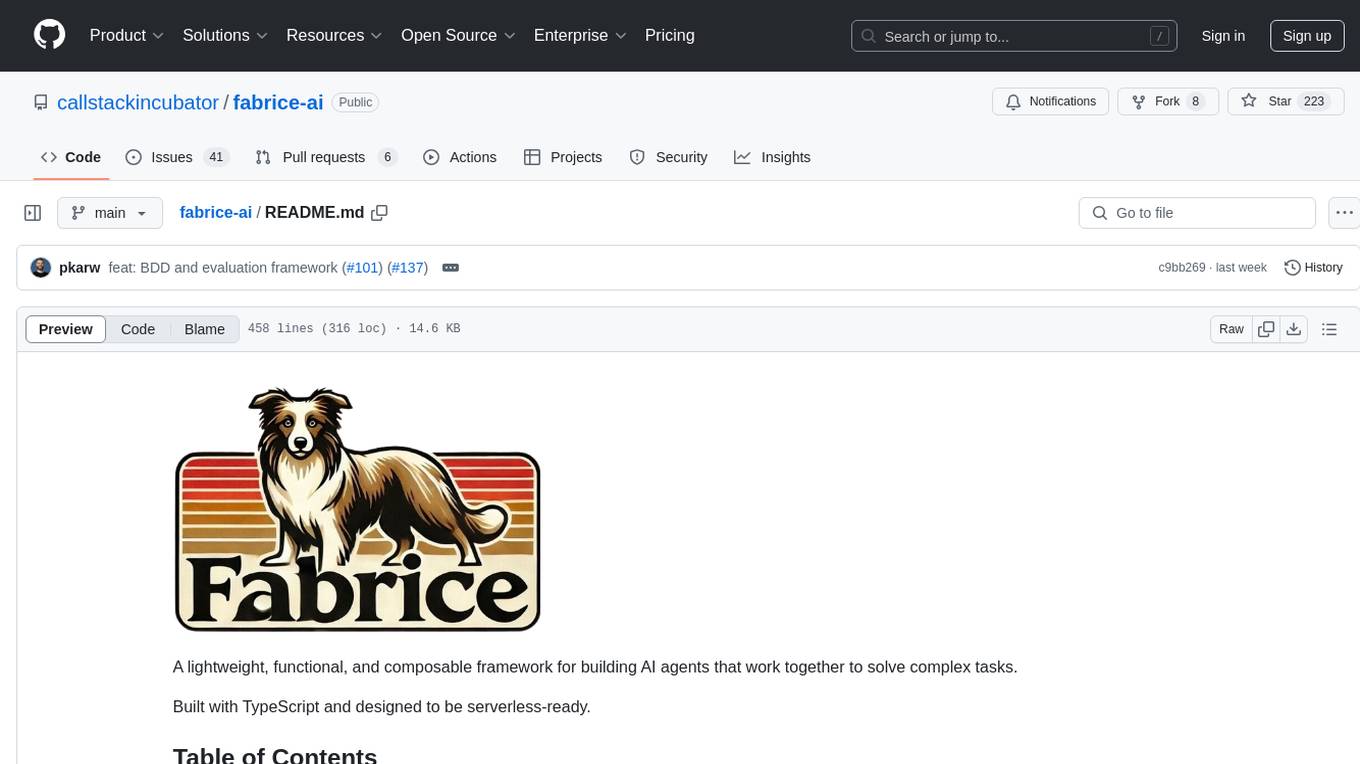
fabrice-ai
A lightweight, functional, and composable framework for building AI agents that work together to solve complex tasks. Built with TypeScript and designed to be serverless-ready. Fabrice embraces functional programming principles, remains stateless, and stays focused on composability. It provides core concepts like easy teamwork creation, infrastructure-agnosticism, statelessness, and includes all tools and features needed to build AI teams. Agents are specialized workers with specific roles and capabilities, able to call tools and complete tasks. Workflows define how agents collaborate to achieve a goal, with workflow states representing the current state of the workflow. Providers handle requests to the LLM and responses. Tools extend agent capabilities by providing concrete actions they can perform. Execution involves running the workflow to completion, with options for custom execution and BDD testing.
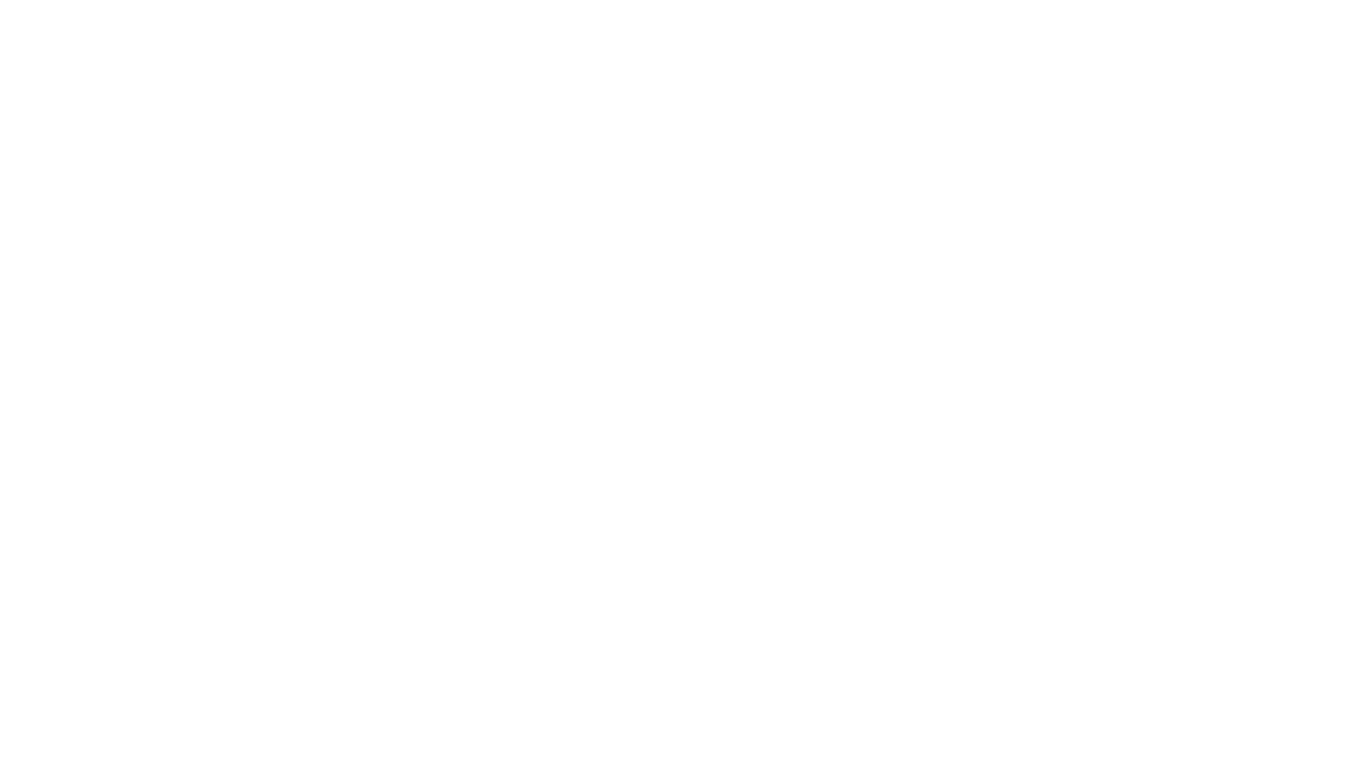
aider-composer
Aider Composer is a VSCode extension that integrates Aider into your development workflow. It allows users to easily add and remove files, toggle between read-only and editable modes, review code changes, use different chat modes, and reference files in the chat. The extension supports multiple models, code generation, code snippets, and settings customization. It has limitations such as lack of support for multiple workspaces, Git repository features, linting, testing, voice features, in-chat commands, and configuration options.
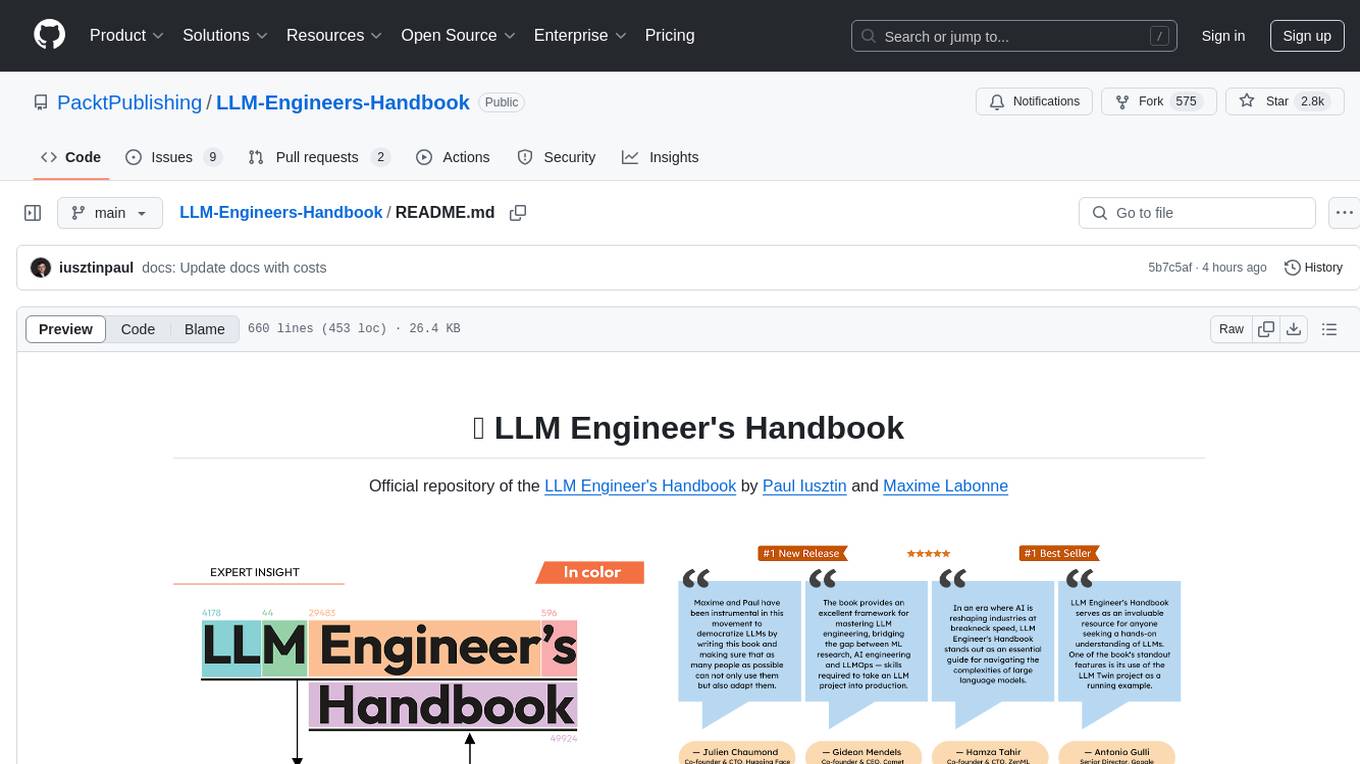
LLM-Engineers-Handbook
The LLM Engineer's Handbook is an official repository containing a comprehensive guide on creating an end-to-end LLM-based system using best practices. It covers data collection & generation, LLM training pipeline, a simple RAG system, production-ready AWS deployment, comprehensive monitoring, and testing and evaluation framework. The repository includes detailed instructions on setting up local and cloud dependencies, project structure, installation steps, infrastructure setup, pipelines for data processing, training, and inference, as well as QA, tests, and running the project end-to-end.
For similar tasks
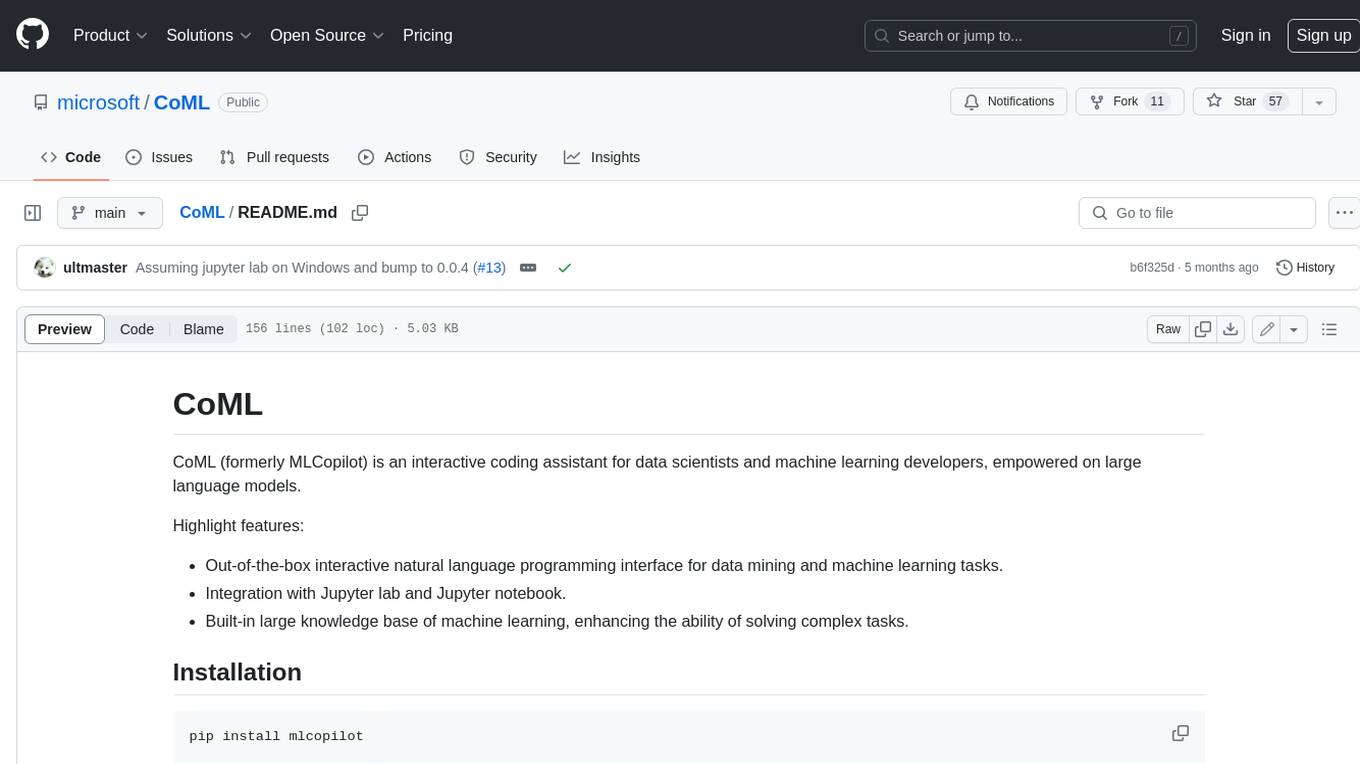
CoML
CoML (formerly MLCopilot) is an interactive coding assistant for data scientists and machine learning developers, empowered on large language models. It offers an out-of-the-box interactive natural language programming interface for data mining and machine learning tasks, integration with Jupyter lab and Jupyter notebook, and a built-in large knowledge base of machine learning to enhance the ability to solve complex tasks. The tool is designed to assist users in coding tasks related to data analysis and machine learning using natural language commands within Jupyter environments.
For similar jobs
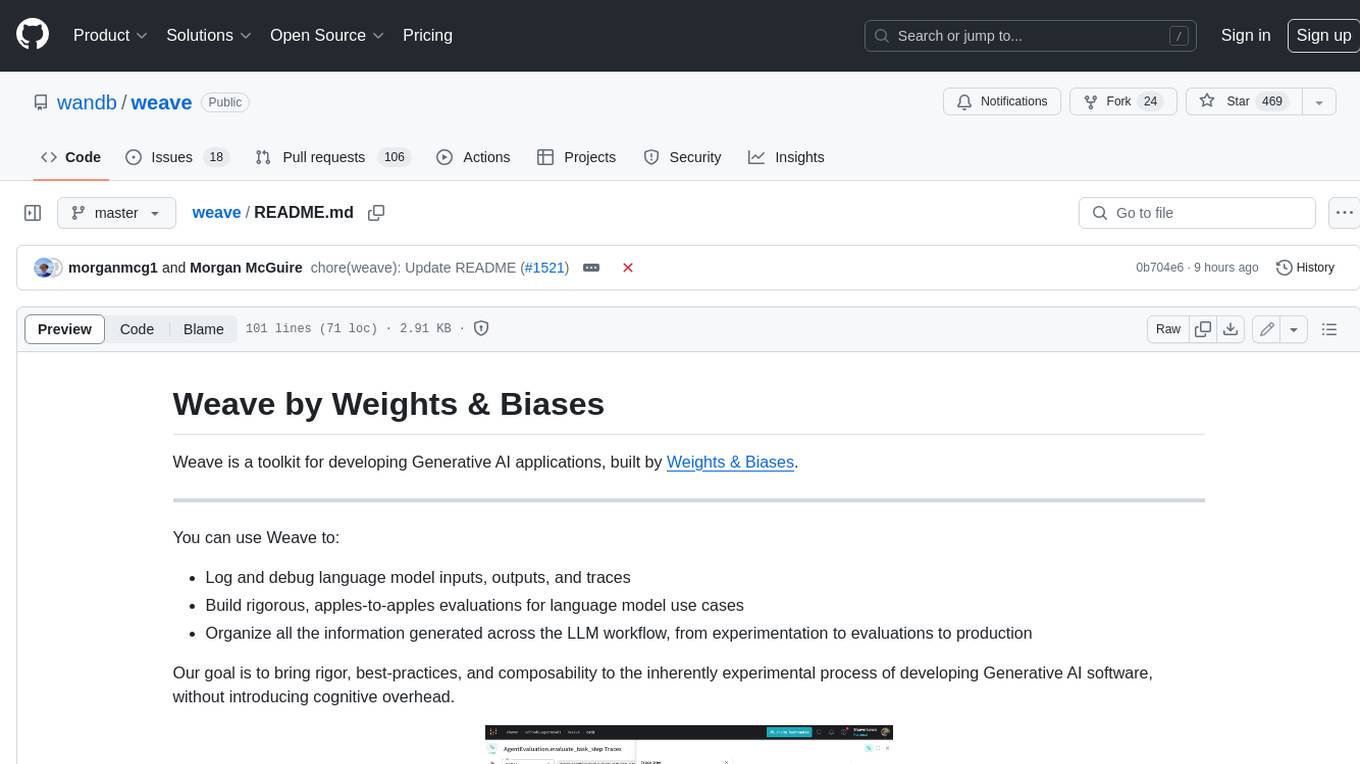
weave
Weave is a toolkit for developing Generative AI applications, built by Weights & Biases. With Weave, you can log and debug language model inputs, outputs, and traces; build rigorous, apples-to-apples evaluations for language model use cases; and organize all the information generated across the LLM workflow, from experimentation to evaluations to production. Weave aims to bring rigor, best-practices, and composability to the inherently experimental process of developing Generative AI software, without introducing cognitive overhead.
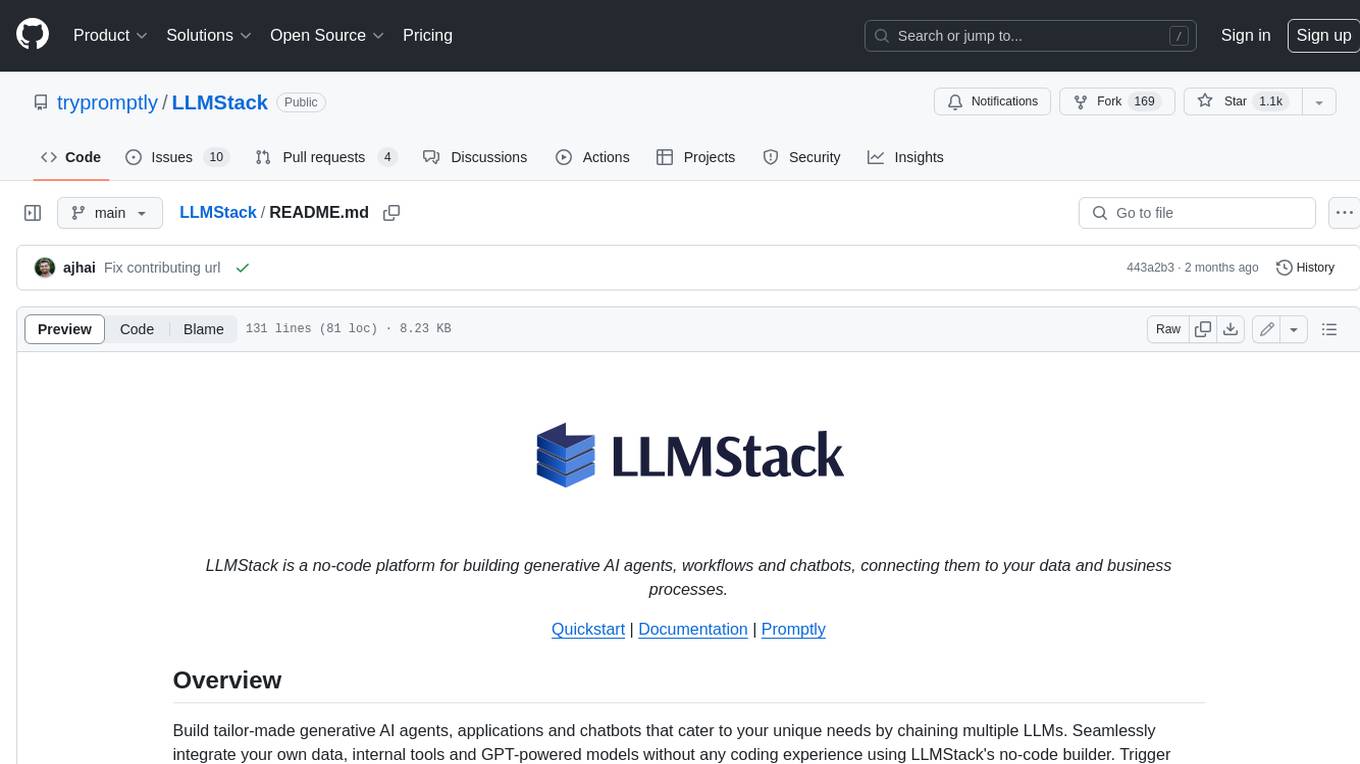
LLMStack
LLMStack is a no-code platform for building generative AI agents, workflows, and chatbots. It allows users to connect their own data, internal tools, and GPT-powered models without any coding experience. LLMStack can be deployed to the cloud or on-premise and can be accessed via HTTP API or triggered from Slack or Discord.
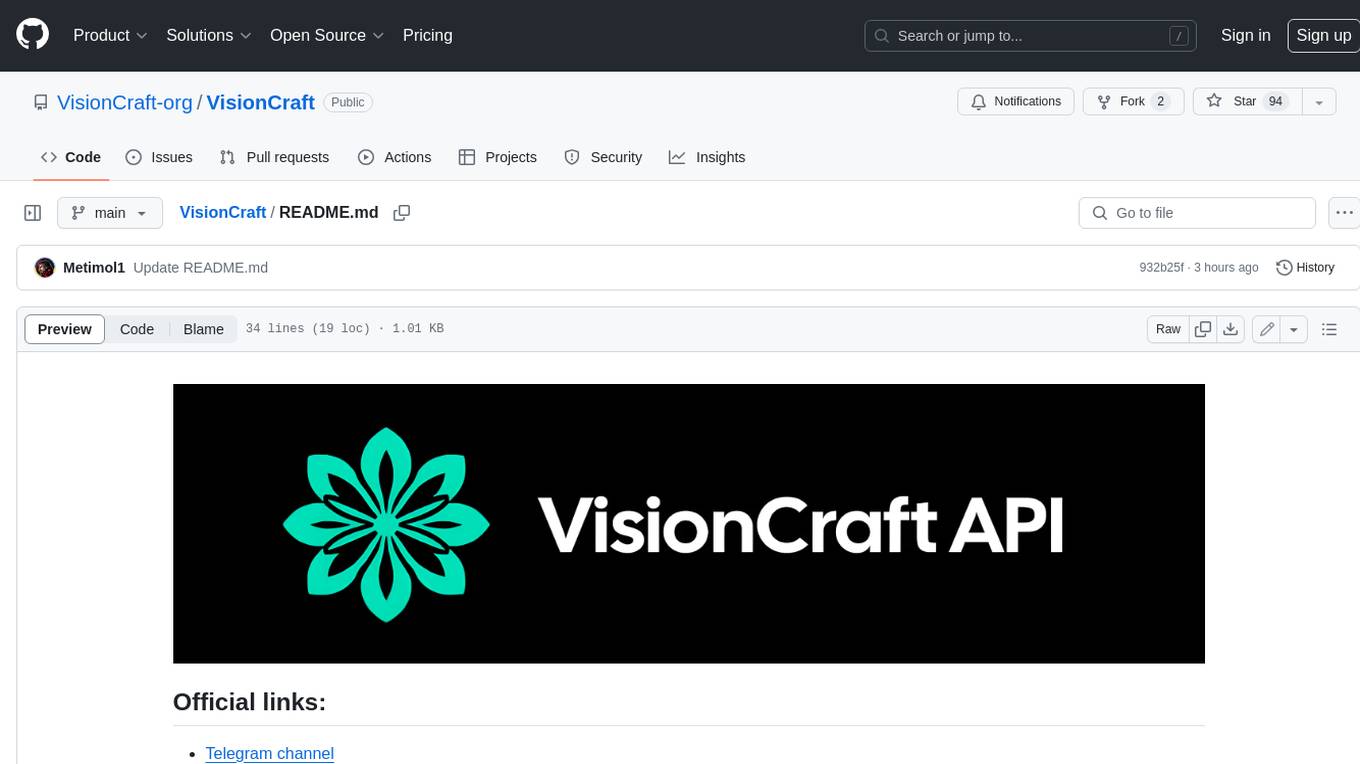
VisionCraft
The VisionCraft API is a free API for using over 100 different AI models. From images to sound.
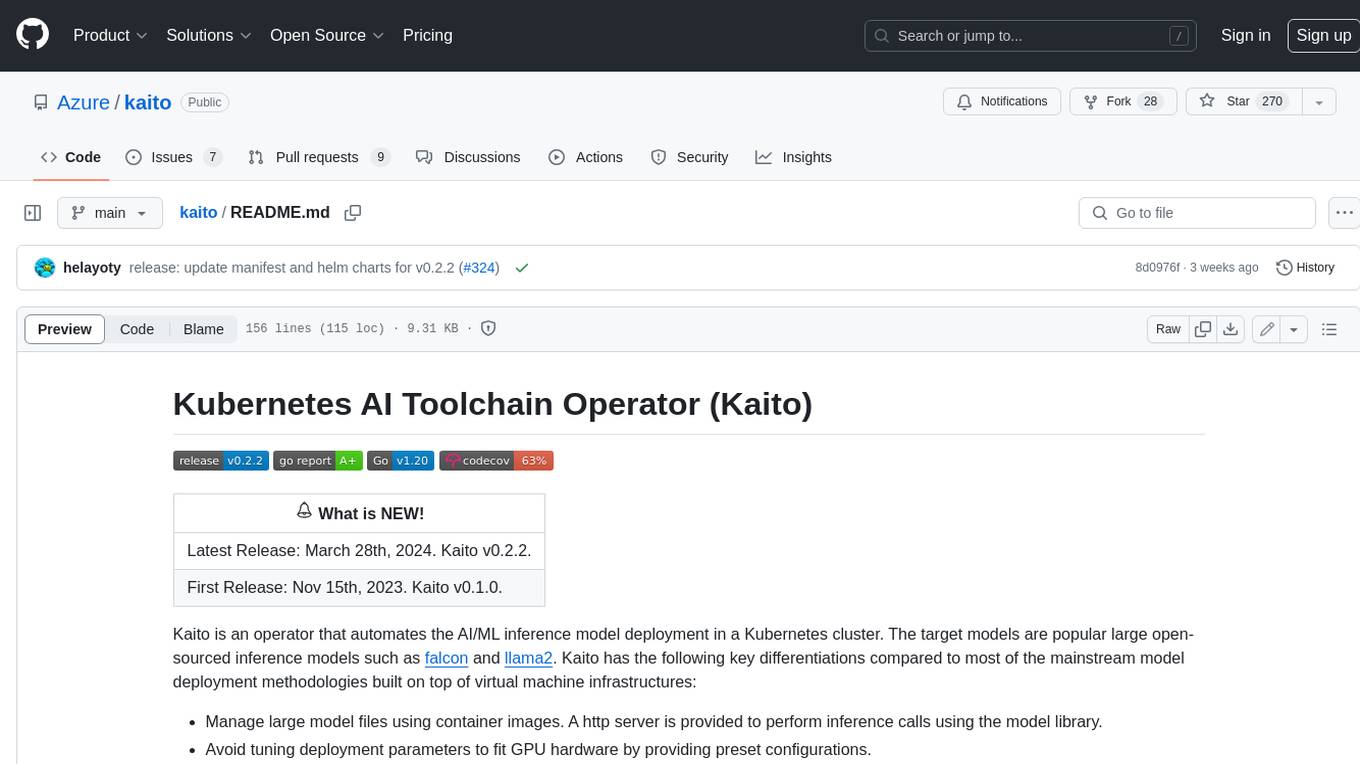
kaito
Kaito is an operator that automates the AI/ML inference model deployment in a Kubernetes cluster. It manages large model files using container images, avoids tuning deployment parameters to fit GPU hardware by providing preset configurations, auto-provisions GPU nodes based on model requirements, and hosts large model images in the public Microsoft Container Registry (MCR) if the license allows. Using Kaito, the workflow of onboarding large AI inference models in Kubernetes is largely simplified.
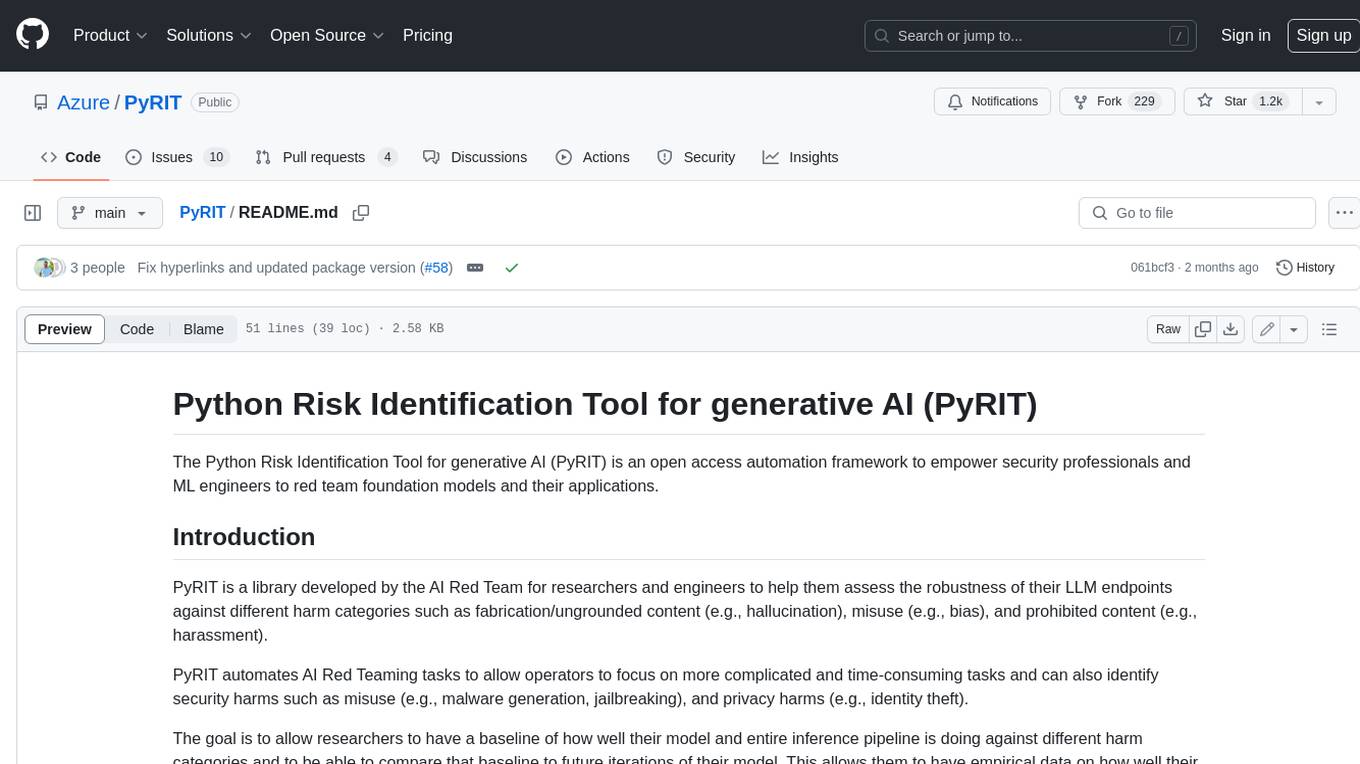
PyRIT
PyRIT is an open access automation framework designed to empower security professionals and ML engineers to red team foundation models and their applications. It automates AI Red Teaming tasks to allow operators to focus on more complicated and time-consuming tasks and can also identify security harms such as misuse (e.g., malware generation, jailbreaking), and privacy harms (e.g., identity theft). The goal is to allow researchers to have a baseline of how well their model and entire inference pipeline is doing against different harm categories and to be able to compare that baseline to future iterations of their model. This allows them to have empirical data on how well their model is doing today, and detect any degradation of performance based on future improvements.
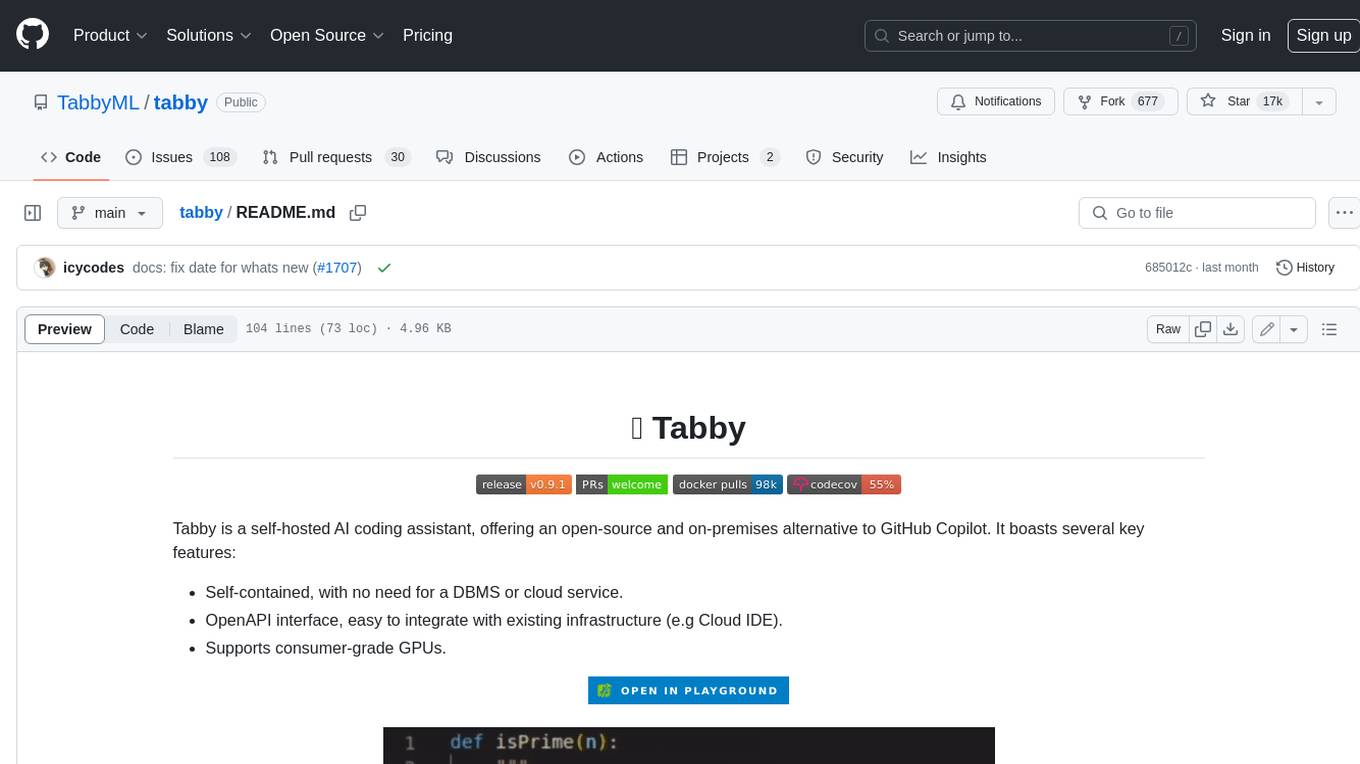
tabby
Tabby is a self-hosted AI coding assistant, offering an open-source and on-premises alternative to GitHub Copilot. It boasts several key features: * Self-contained, with no need for a DBMS or cloud service. * OpenAPI interface, easy to integrate with existing infrastructure (e.g Cloud IDE). * Supports consumer-grade GPUs.
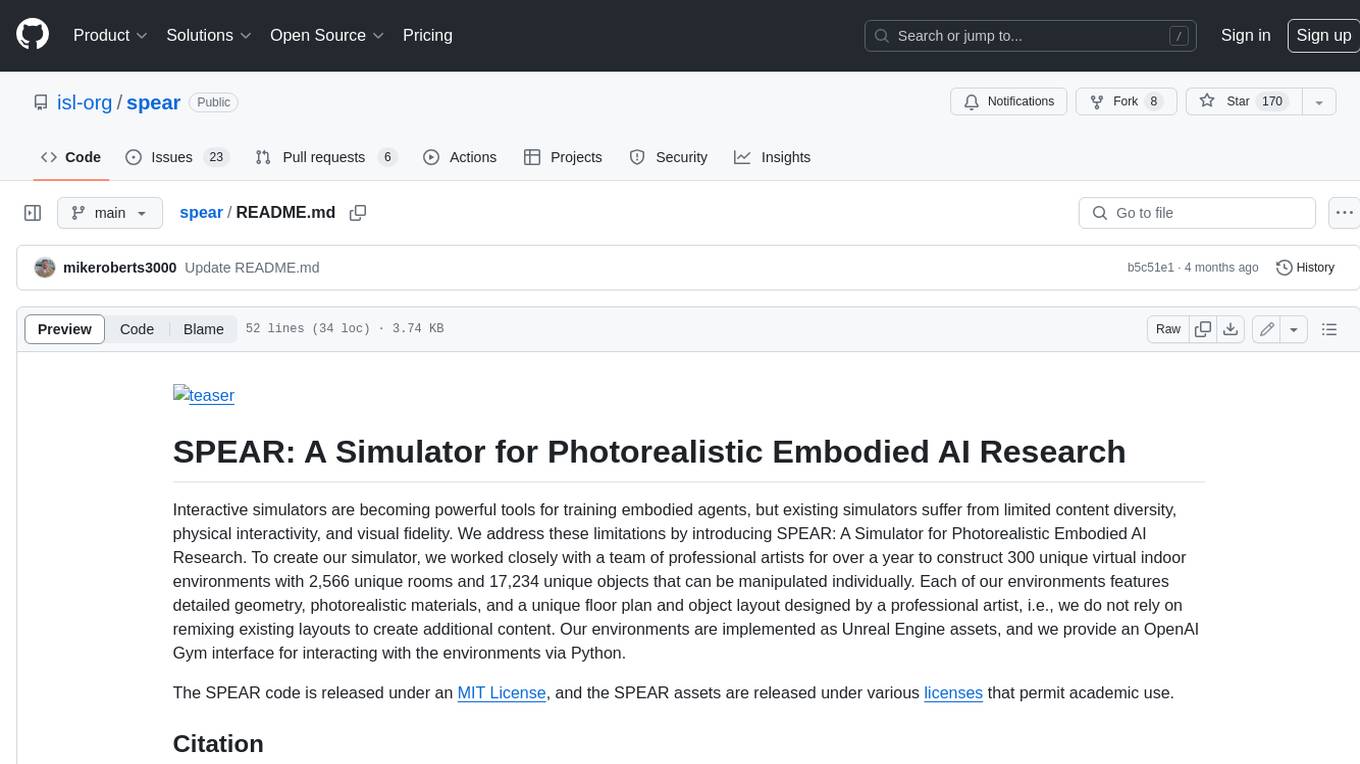
spear
SPEAR (Simulator for Photorealistic Embodied AI Research) is a powerful tool for training embodied agents. It features 300 unique virtual indoor environments with 2,566 unique rooms and 17,234 unique objects that can be manipulated individually. Each environment is designed by a professional artist and features detailed geometry, photorealistic materials, and a unique floor plan and object layout. SPEAR is implemented as Unreal Engine assets and provides an OpenAI Gym interface for interacting with the environments via Python.
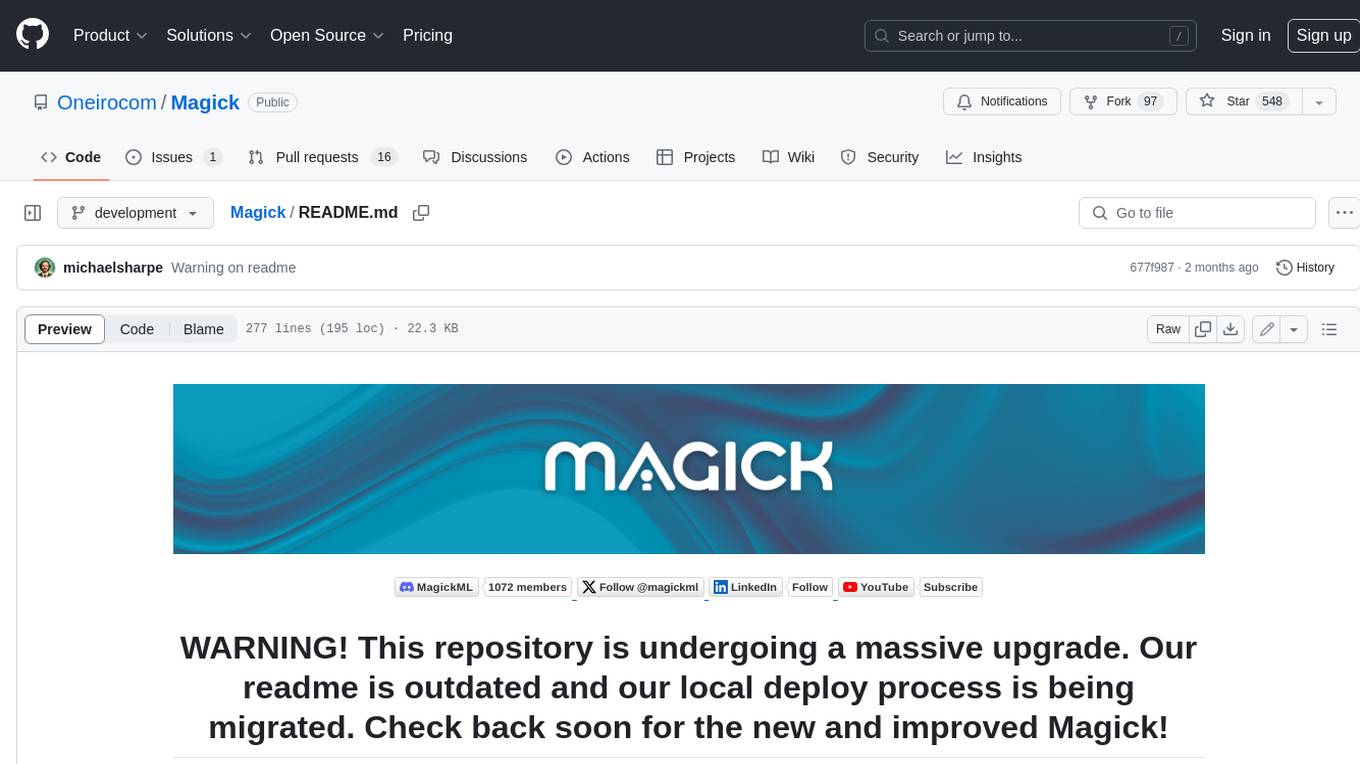
Magick
Magick is a groundbreaking visual AIDE (Artificial Intelligence Development Environment) for no-code data pipelines and multimodal agents. Magick can connect to other services and comes with nodes and templates well-suited for intelligent agents, chatbots, complex reasoning systems and realistic characters.