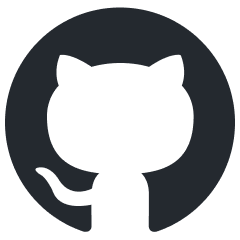
PraisonAI
PraisonAI application combines AutoGen and CrewAI or similar frameworks into a low-code solution for building and managing multi-agent LLM systems, focusing on simplicity, customisation, and efficient human-agent collaboration. Chat with your ENTIRE Codebase.
Stars: 2138
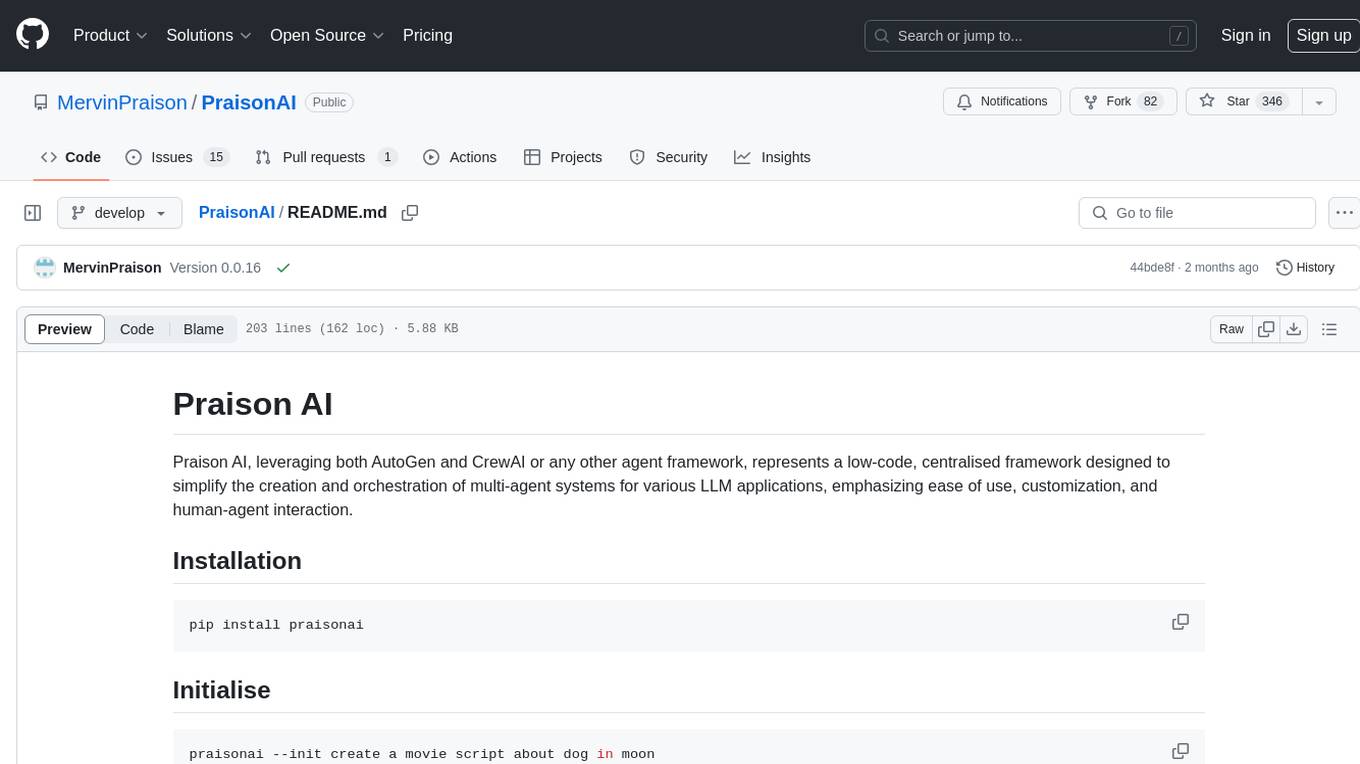
Praison AI is a low-code, centralised framework that simplifies the creation and orchestration of multi-agent systems for various LLM applications. It emphasizes ease of use, customization, and human-agent interaction. The tool leverages AutoGen and CrewAI frameworks to facilitate the development of AI-generated scripts and movie concepts. Users can easily create, run, test, and deploy agents for scriptwriting and movie concept development. Praison AI also provides options for full automatic mode and integration with OpenAI models for enhanced AI capabilities.
README:
Praison AI, leveraging both AutoGen and CrewAI or any other agent framework, represents a low-code, centralised framework designed to simplify the creation and orchestration of multi-agent systems for various LLM applications, emphasizing ease of use, customization, and human-agent interaction.
Interface | Description | URL |
---|---|---|
UI | Multi Agents such as CrewAI or AutoGen | https://docs.praison.ai/ui/ui |
Chat | Chat with 100+ LLMs, single AI Agent | https://docs.praison.ai/ui/chat |
Code | Chat with entire Codebase, single AI Agent | https://docs.praison.ai/ui/code |
Other Features | Description | Docs |
---|---|---|
Train | Fine-tune LLMs using your custom data | https://docs.praison.ai/train |
Cookbook | Open in Colab | |
---|---|---|
Basic | PraisonAI | |
Include Tools | PraisonAI Tools |
Installation | |
---|---|
PraisonAI | pip install praisonai |
PraisonAI Code | pip install "praisonai[code]" |
PraisonAI Chat | pip install "praisonai[chat]" |
PraisonAI Train | pip install "praisonai[train]" |
- Automated AI Agents Creation
- Use CrewAI or AutoGen Framework
- 100+ LLM Support
- Chat with ENTIRE Codebase
- Interactive UIs
- YAML-based Configuration
- Custom Tool Integration
- Internet Search Capability (using Crawl4AI and Tavily)
- Vision Language Model (VLM) Support
pip install praisonai
export OPENAI_API_KEY="Enter your API key"
praisonai --init create a movie script about dog in moon
praisonai
- Installation
- Initialise
- Run
- Full Automatic Mode
- User Interface
- Praison AI Chat
- Create Custom Tools
- Agents Playbook
- Include praisonai package in your project
- Commands to Install Dev Dependencies
- Other Models
- Contributing
- Star History
pip install praisonai
export OPENAI_API_KEY="Enter your API key"
Generate your OPENAI API KEY from here: https://platform.openai.com/api-keys
Note: You can use other providers such as Ollama, Mistral ... etc. Details are provided at the bottom.
praisonai --init create a movie script about dog in moon
This will automatically create agents.yaml file in the current directory.
praisonai --framework autogen --init create movie script about cat in mars
praisonai
or
python -m praisonai
praisonai --framework autogen
praisonai --auto create a movie script about Dog in Moon
Interface | Description | URL |
---|---|---|
UI | Multi Agents such as CrewAI or AutoGen | https://docs.praisonai.com/ui/ui |
Chat | Chat with 100+ LLMs, single AI Agent | https://docs.praisonai.com/ui/chat |
Code | Chat with entire Codebase, single AI Agent | https://docs.praisonai.com/ui/code |
pip install -U "praisonai[ui]"
export OPENAI_API_KEY="Enter your API key"
chainlit create-secret
export CHAINLIT_AUTH_SECRET=xxxxxxxx
praisonai ui
or
python -m praisonai ui
pip install "praisonai[chat]"
export OPENAI_API_KEY="Enter your API key"
praisonai chat
Praison AI Chat and Praison AI Code now includes internet search capabilities using Crawl4AI and Tavily, allowing you to retrieve up-to-date information during your conversations.
You can now upload images and ask questions based on them using Vision Language Models. This feature enables visual understanding and analysis within your chat sessions.
pip install "praisonai[code]"
export OPENAI_API_KEY="Enter your API key"
praisonai code
Praison AI Code also includes internet search functionality, enabling you to find relevant code snippets and programming information online.
framework: crewai
topic: Artificial Intelligence
roles:
screenwriter:
backstory: "Skilled in crafting scripts with engaging dialogue about {topic}."
goal: Create scripts from concepts.
role: Screenwriter
tasks:
scriptwriting_task:
description: "Develop scripts with compelling characters and dialogue about {topic}."
expected_output: "Complete script ready for production."
from praisonai import PraisonAI
# Example agent_yaml content
agent_yaml = """
framework: "crewai"
topic: "Space Exploration"
roles:
astronomer:
role: "Space Researcher"
goal: "Discover new insights about {topic}"
backstory: "You are a curious and dedicated astronomer with a passion for unraveling the mysteries of the cosmos."
tasks:
investigate_exoplanets:
description: "Research and compile information about exoplanets discovered in the last decade."
expected_output: "A summarized report on exoplanet discoveries, including their size, potential habitability, and distance from Earth."
"""
# Create a PraisonAI instance with the agent_yaml content
praisonai = PraisonAI(agent_yaml=agent_yaml)
# Run PraisonAI
result = praisonai.run()
# Print the result
print(result)
Note: Please create agents.yaml file before hand.
from praisonai import PraisonAI
def basic(): # Basic Mode
praisonai = PraisonAI(agent_file="agents.yaml")
praisonai.run()
if __name__ == "__main__":
basic()
-
Install all dependencies, including dev dependencies:
poetry install
-
Install only documentation dependencies:
poetry install --with docs
-
Install only test dependencies:
poetry install --with test
-
Install only dev dependencies:
poetry install --with dev
This configuration ensures that your development dependencies are correctly categorized and installed as needed.
- Fork on GitHub: Use the "Fork" button on the repository page.
- Clone your fork:
git clone https://github.com/yourusername/praisonAI.git
- Create a branch:
git checkout -b new-feature
- Make changes and commit:
git commit -am "Add some feature"
- Push to your fork:
git push origin new-feature
- Submit a pull request via GitHub's web interface.
- Await feedback from project maintainers.
Praison AI is an open-sourced software licensed under the MIT license.
For Tasks:
Click tags to check more tools for each tasksFor Jobs:
Alternative AI tools for PraisonAI
Similar Open Source Tools
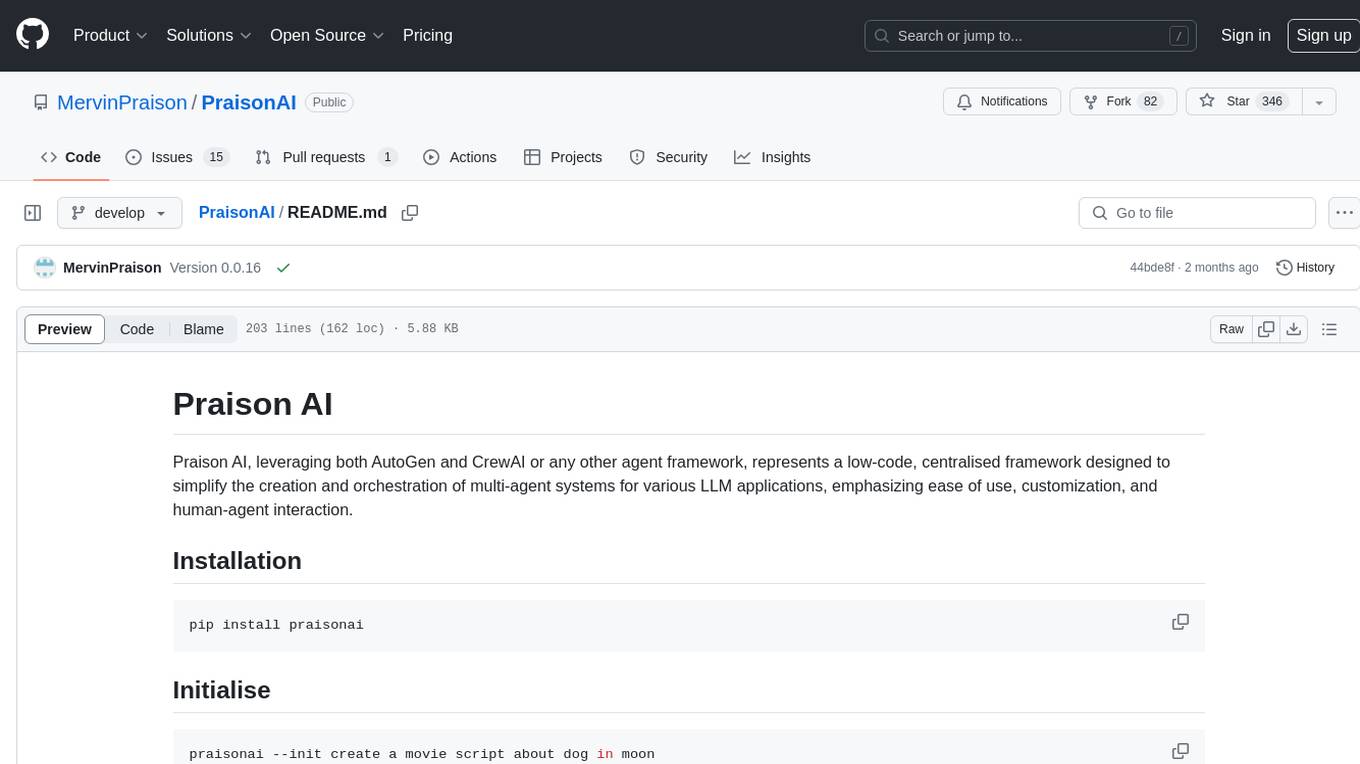
PraisonAI
Praison AI is a low-code, centralised framework that simplifies the creation and orchestration of multi-agent systems for various LLM applications. It emphasizes ease of use, customization, and human-agent interaction. The tool leverages AutoGen and CrewAI frameworks to facilitate the development of AI-generated scripts and movie concepts. Users can easily create, run, test, and deploy agents for scriptwriting and movie concept development. Praison AI also provides options for full automatic mode and integration with OpenAI models for enhanced AI capabilities.
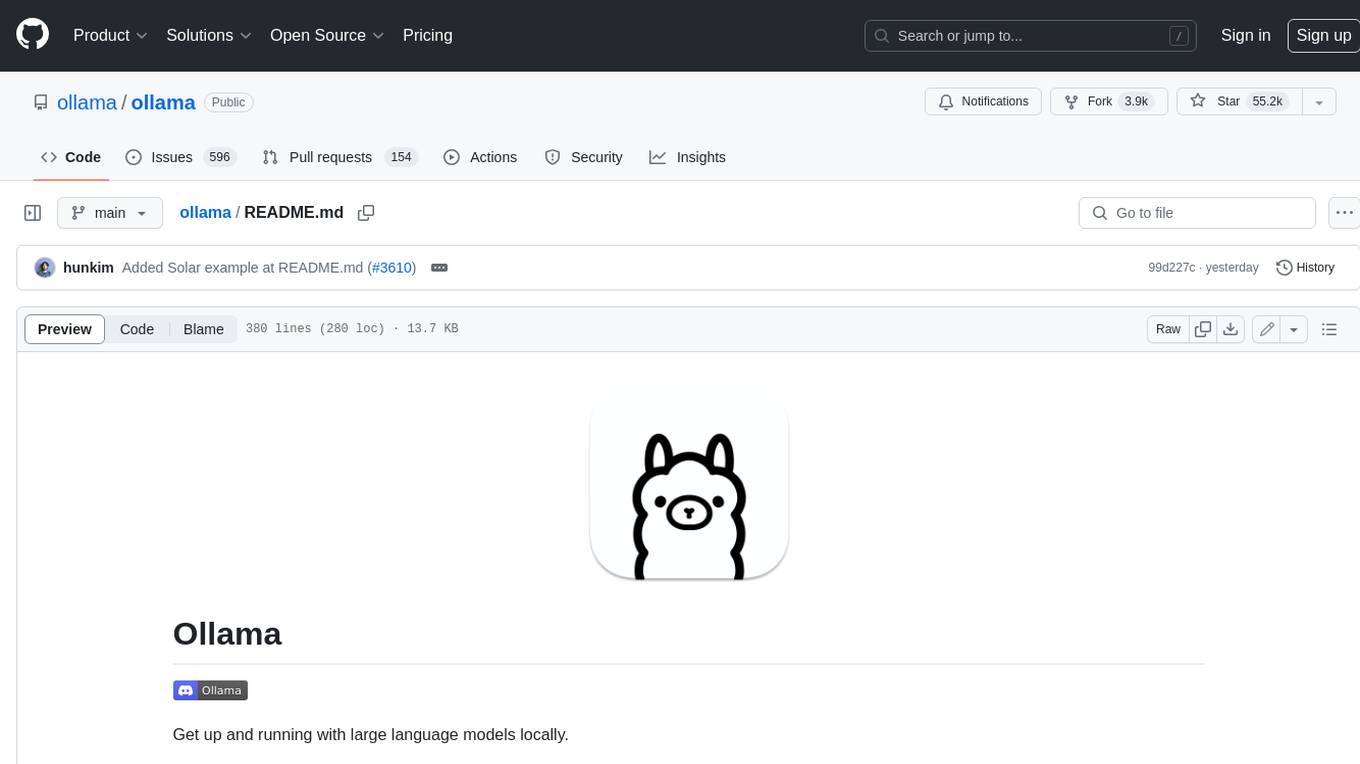
ollama
Ollama is a lightweight, extensible framework for building and running language models on the local machine. It provides a simple API for creating, running, and managing models, as well as a library of pre-built models that can be easily used in a variety of applications. Ollama is designed to be easy to use and accessible to developers of all levels. It is open source and available for free on GitHub.
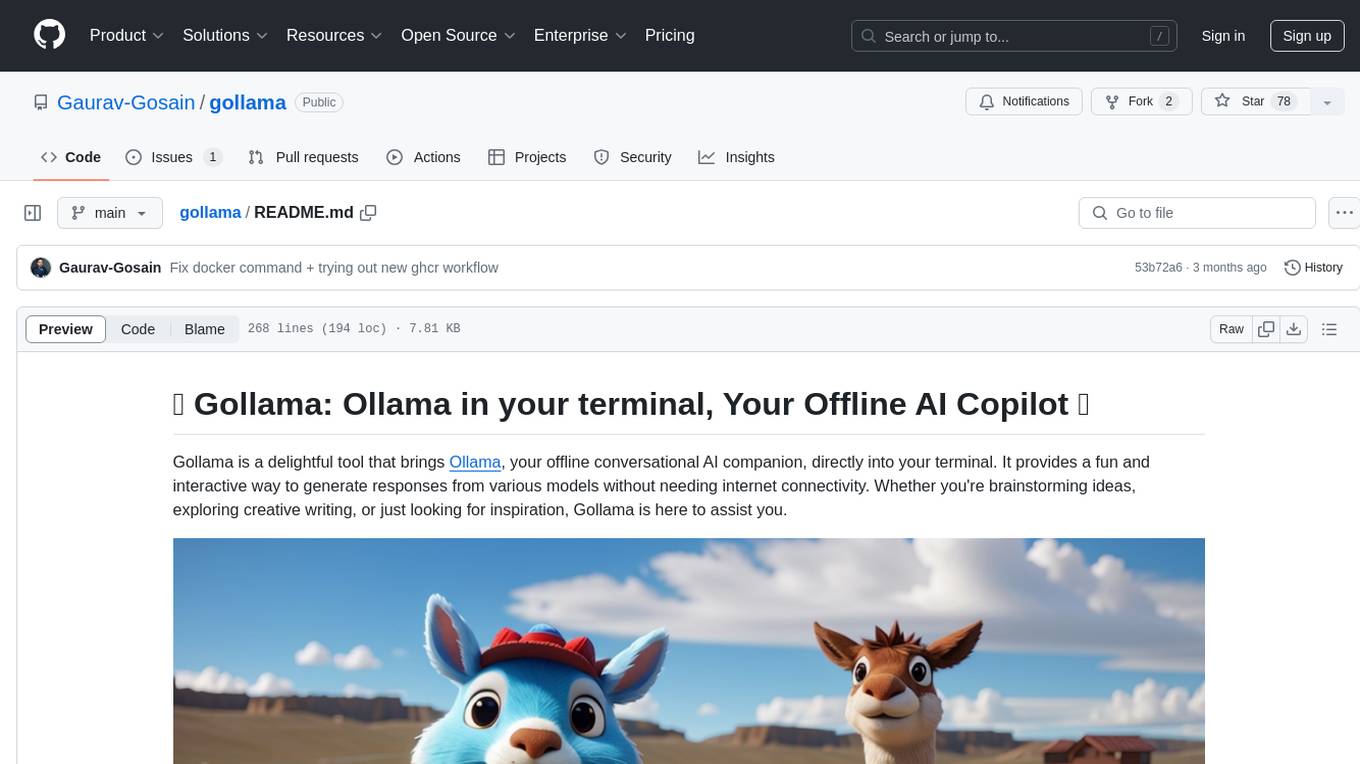
gollama
Gollama is a delightful tool that brings Ollama, your offline conversational AI companion, directly into your terminal. It provides a fun and interactive way to generate responses from various models without needing internet connectivity. Whether you're brainstorming ideas, exploring creative writing, or just looking for inspiration, Gollama is here to assist you. The tool offers an interactive interface, customizable prompts, multiple models selection, and visual feedback to enhance user experience. It can be installed via different methods like downloading the latest release, using Go, running with Docker, or building from source. Users can interact with Gollama through various options like specifying a custom base URL, prompt, model, and enabling raw output mode. The tool supports different modes like interactive, piped, CLI with image, and TUI with image. Gollama relies on third-party packages like bubbletea, glamour, huh, and lipgloss. The roadmap includes implementing piped mode, support for extracting codeblocks, copying responses/codeblocks to clipboard, GitHub Actions for automated releases, and downloading models directly from Ollama using the rest API. Contributions are welcome, and the project is licensed under the MIT License.
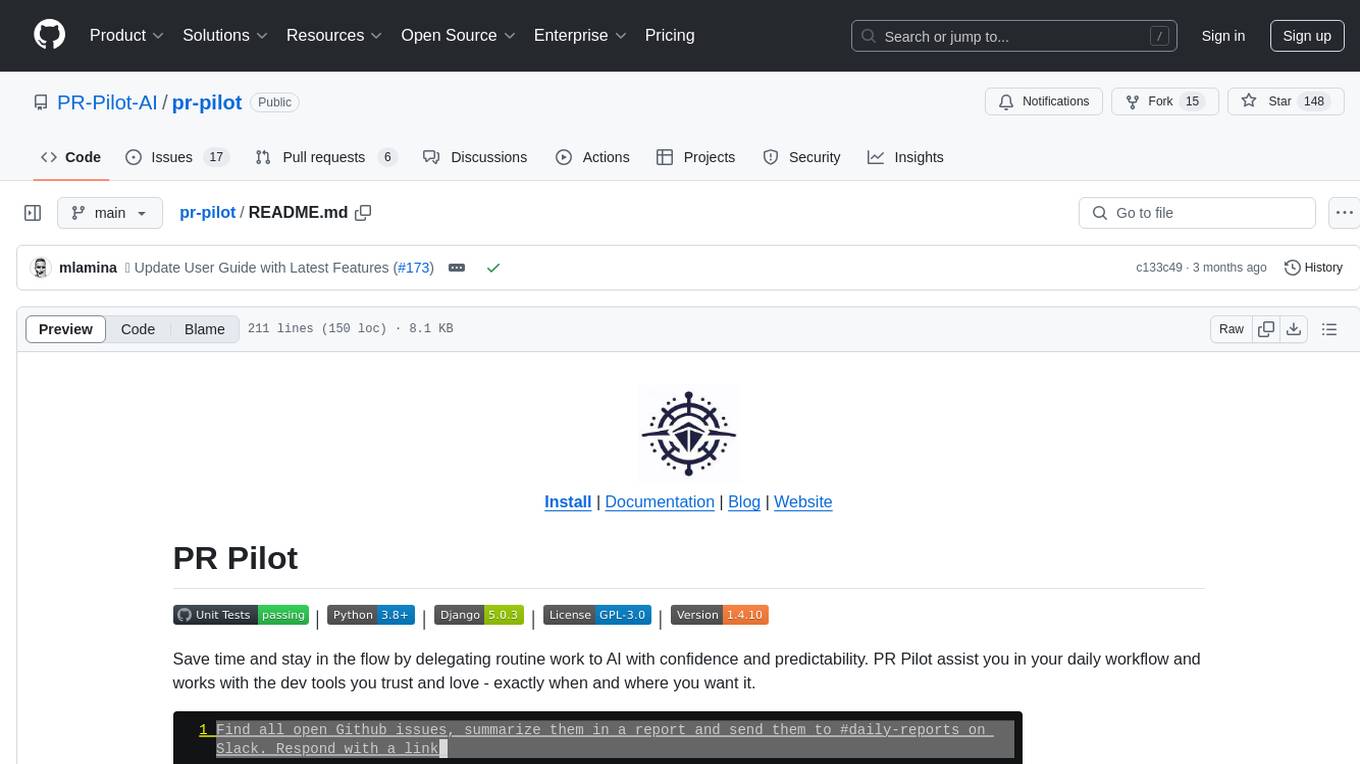
pr-pilot
PR Pilot is an AI-powered tool designed to assist users in their daily workflow by delegating routine work to AI with confidence and predictability. It integrates seamlessly with popular development tools and allows users to interact with it through a Command-Line Interface, Python SDK, REST API, and Smart Workflows. Users can automate tasks such as generating PR titles and descriptions, summarizing and posting issues, and formatting README files. The tool aims to save time and enhance productivity by providing AI-powered solutions for common development tasks.
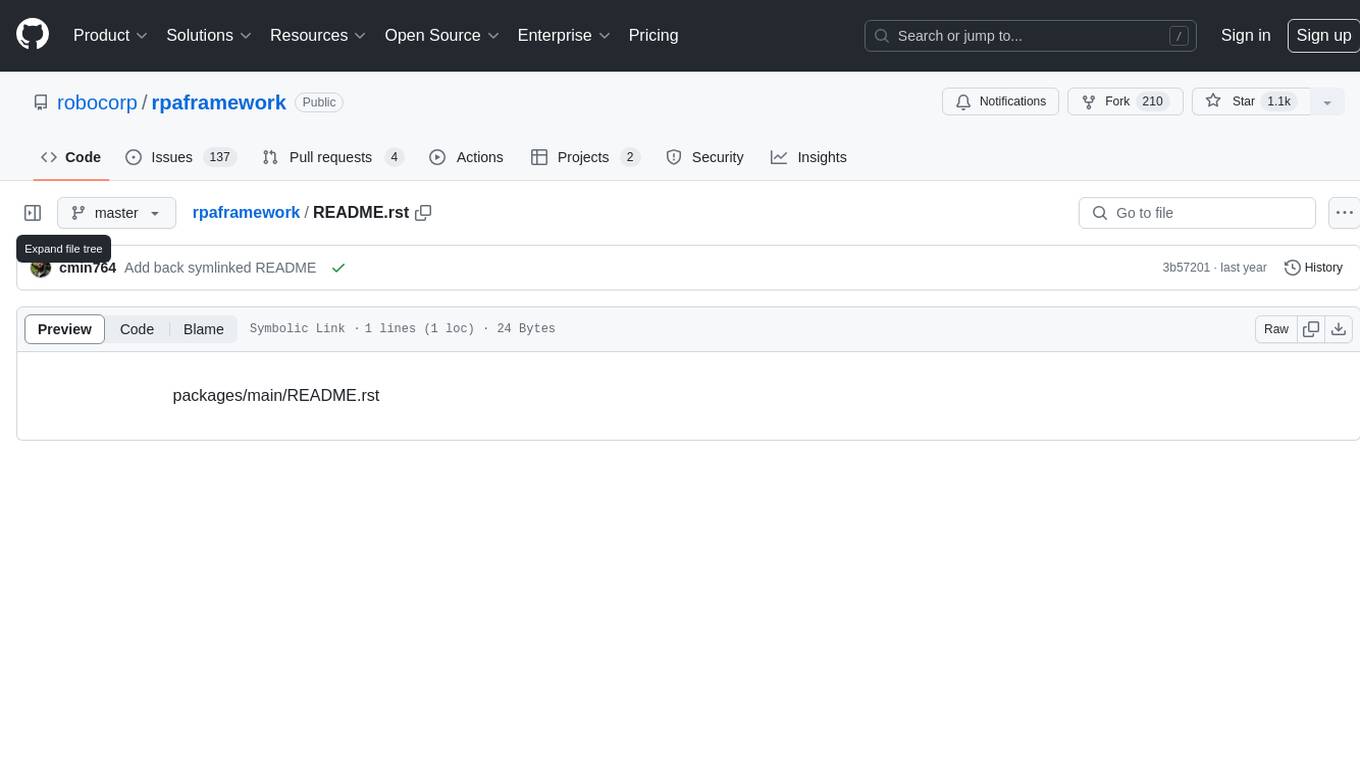
rpaframework
RPA Framework is an open-source collection of libraries and tools for Robotic Process Automation (RPA), designed to be used with Robot Framework and Python. It offers well-documented core libraries for Software Robot Developers, optimized for Robocorp Control Room and Developer Tools, and accepts external contributions. The project includes various libraries for tasks like archiving, browser automation, date/time manipulations, cloud services integration, encryption operations, database interactions, desktop automation, document processing, email operations, Excel manipulation, file system operations, FTP interactions, web API interactions, image manipulation, AI services, and more. The development of the repository is Python-based and requires Python version 3.8+, with tooling based on poetry and invoke for compiling, building, and running the package. The project is licensed under the Apache License 2.0.
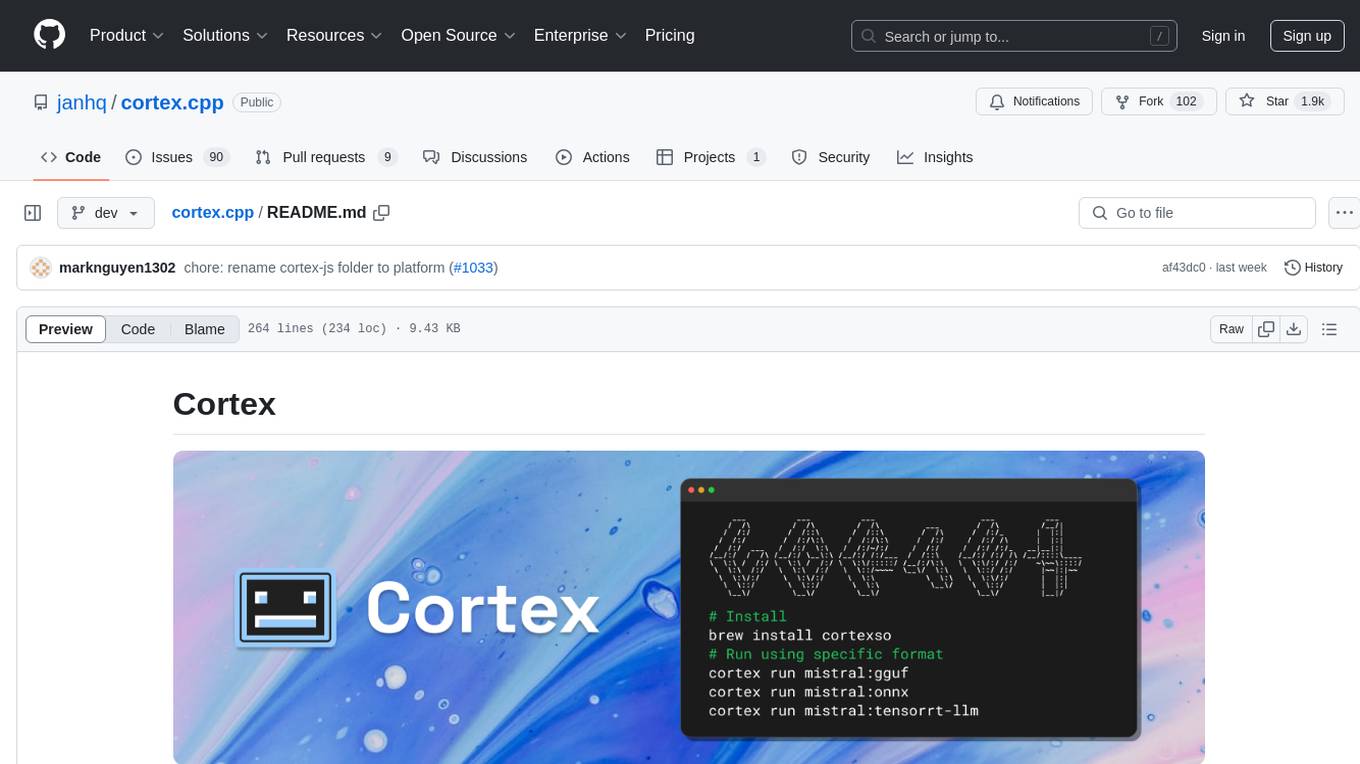
cortex.cpp
Cortex is a C++ AI engine with a Docker-like command-line interface and client libraries. It supports running AI models using ONNX, TensorRT-LLM, and llama.cpp engines. Cortex can function as a standalone server or be integrated as a library. The tool provides support for various engines and models, allowing users to easily deploy and interact with AI models. It offers a range of CLI commands for managing models, embeddings, and engines, as well as a REST API for interacting with models. Cortex is designed to simplify the deployment and usage of AI models in C++ applications.
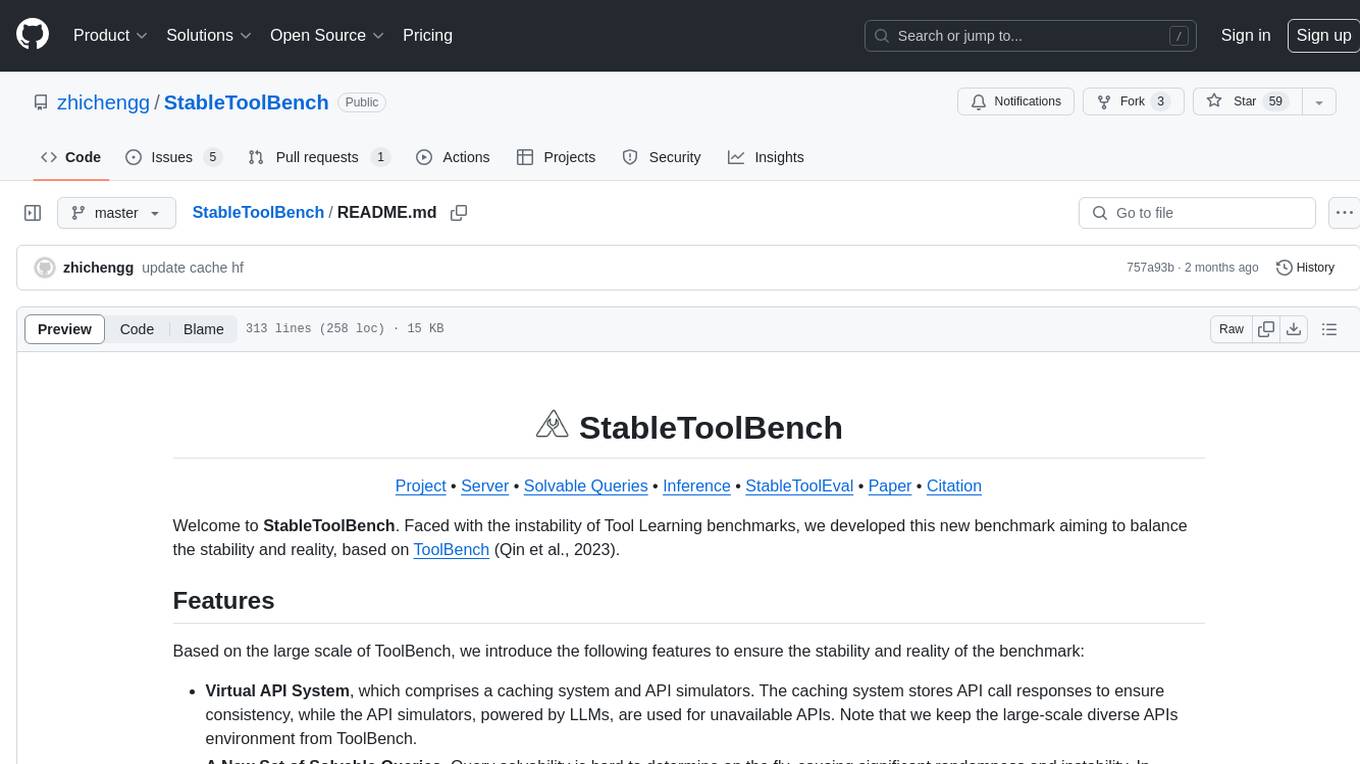
StableToolBench
StableToolBench is a new benchmark developed to address the instability of Tool Learning benchmarks. It aims to balance stability and reality by introducing features such as a Virtual API System with caching and API simulators, a new set of solvable queries determined by LLMs, and a Stable Evaluation System using GPT-4. The Virtual API Server can be set up either by building from source or using a prebuilt Docker image. Users can test the server using provided scripts and evaluate models with Solvable Pass Rate and Solvable Win Rate metrics. The tool also includes model experiments results comparing different models' performance.
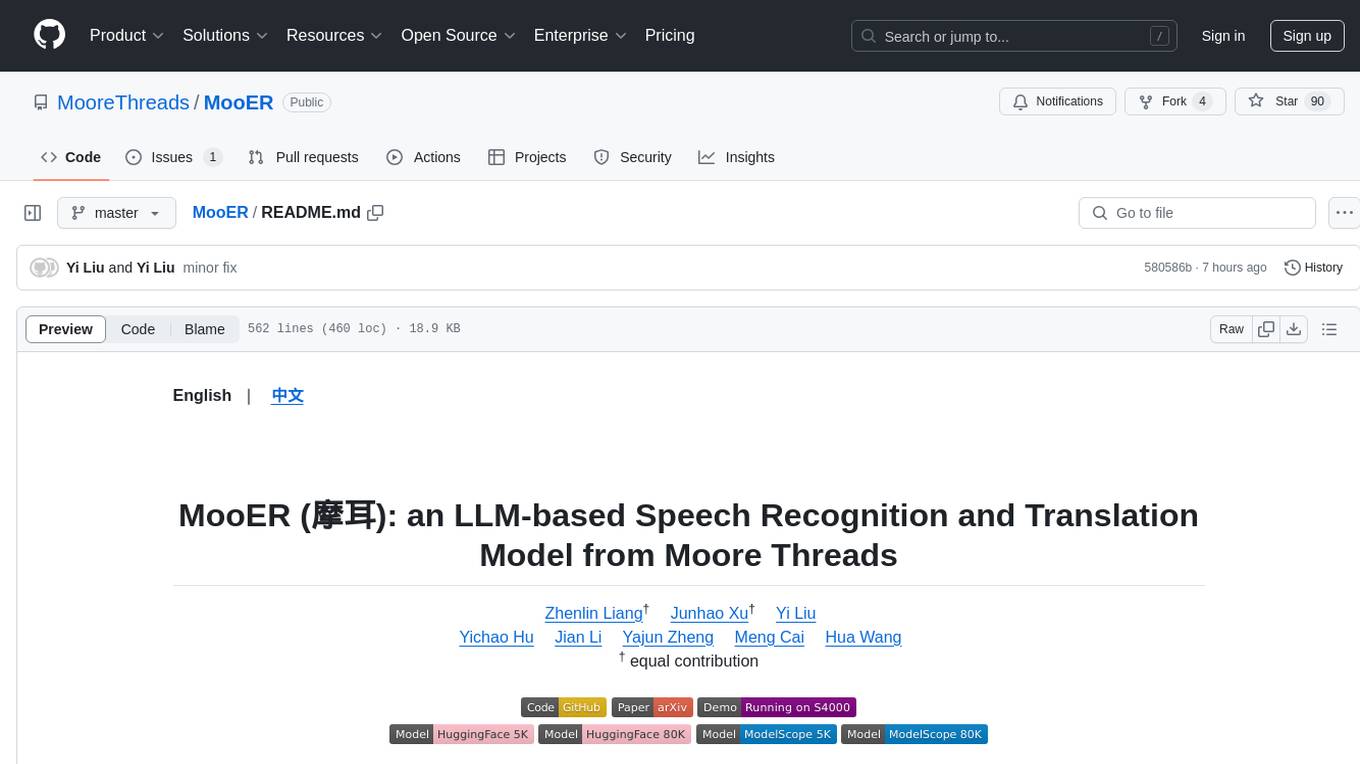
MooER
MooER (摩耳) is an LLM-based speech recognition and translation model developed by Moore Threads. It allows users to transcribe speech into text (ASR) and translate speech into other languages (AST) in an end-to-end manner. The model was trained using 5K hours of data and is now also available with an 80K hours version. MooER is the first LLM-based speech model trained and inferred using domestic GPUs. The repository includes pretrained models, inference code, and a Gradio demo for a better user experience.
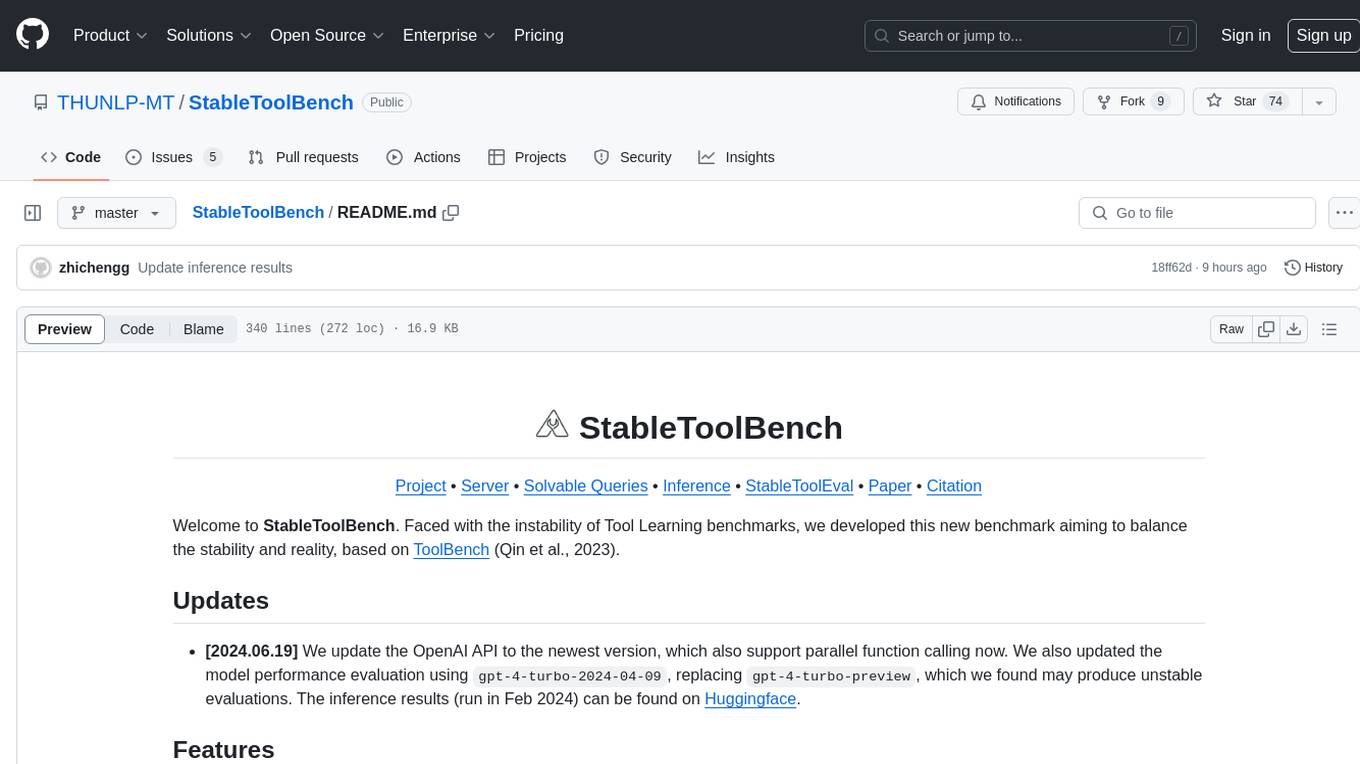
StableToolBench
StableToolBench is a new benchmark developed to address the instability of Tool Learning benchmarks. It aims to balance stability and reality by introducing features like Virtual API System, Solvable Queries, and Stable Evaluation System. The benchmark ensures consistency through a caching system and API simulators, filters queries based on solvability using LLMs, and evaluates model performance using GPT-4 with metrics like Solvable Pass Rate and Solvable Win Rate.
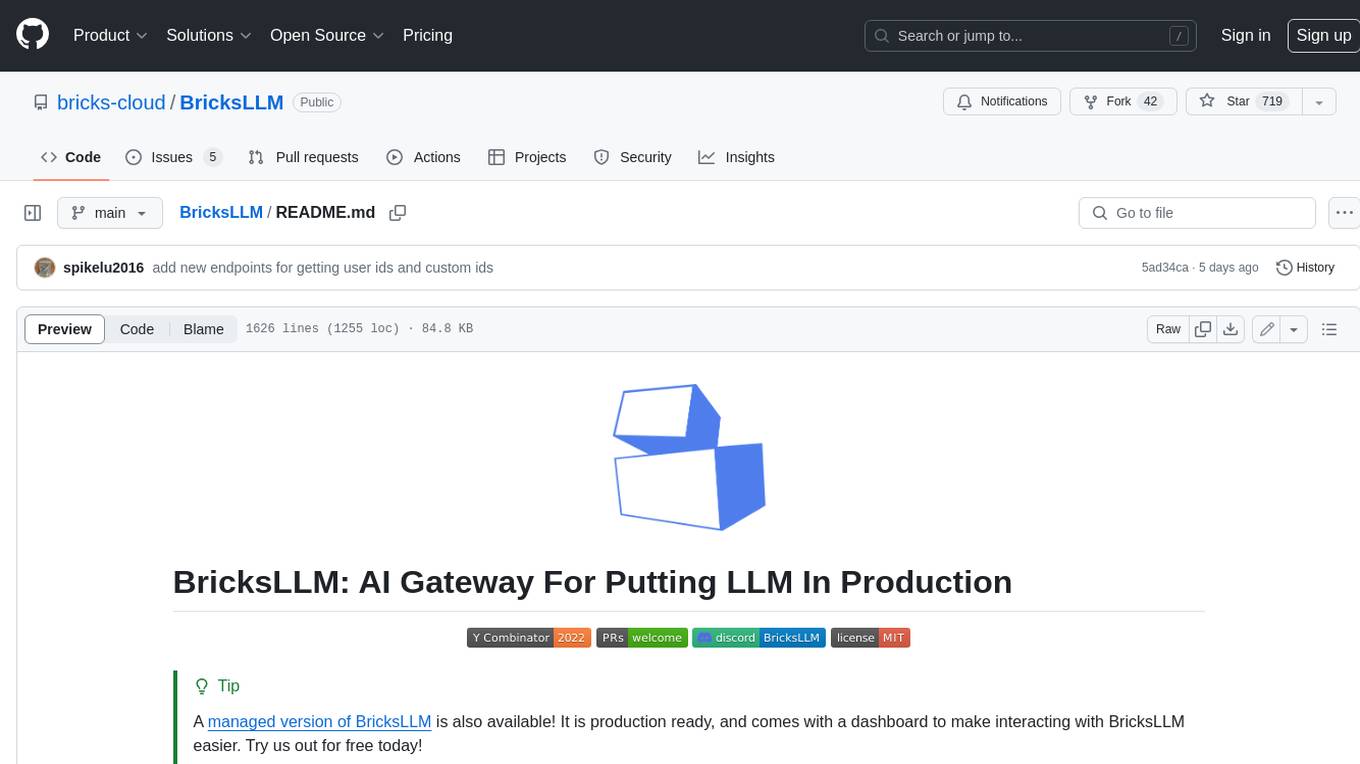
BricksLLM
BricksLLM is a cloud native AI gateway written in Go. Currently, it provides native support for OpenAI, Anthropic, Azure OpenAI and vLLM. BricksLLM aims to provide enterprise level infrastructure that can power any LLM production use cases. Here are some use cases for BricksLLM: * Set LLM usage limits for users on different pricing tiers * Track LLM usage on a per user and per organization basis * Block or redact requests containing PIIs * Improve LLM reliability with failovers, retries and caching * Distribute API keys with rate limits and cost limits for internal development/production use cases * Distribute API keys with rate limits and cost limits for students
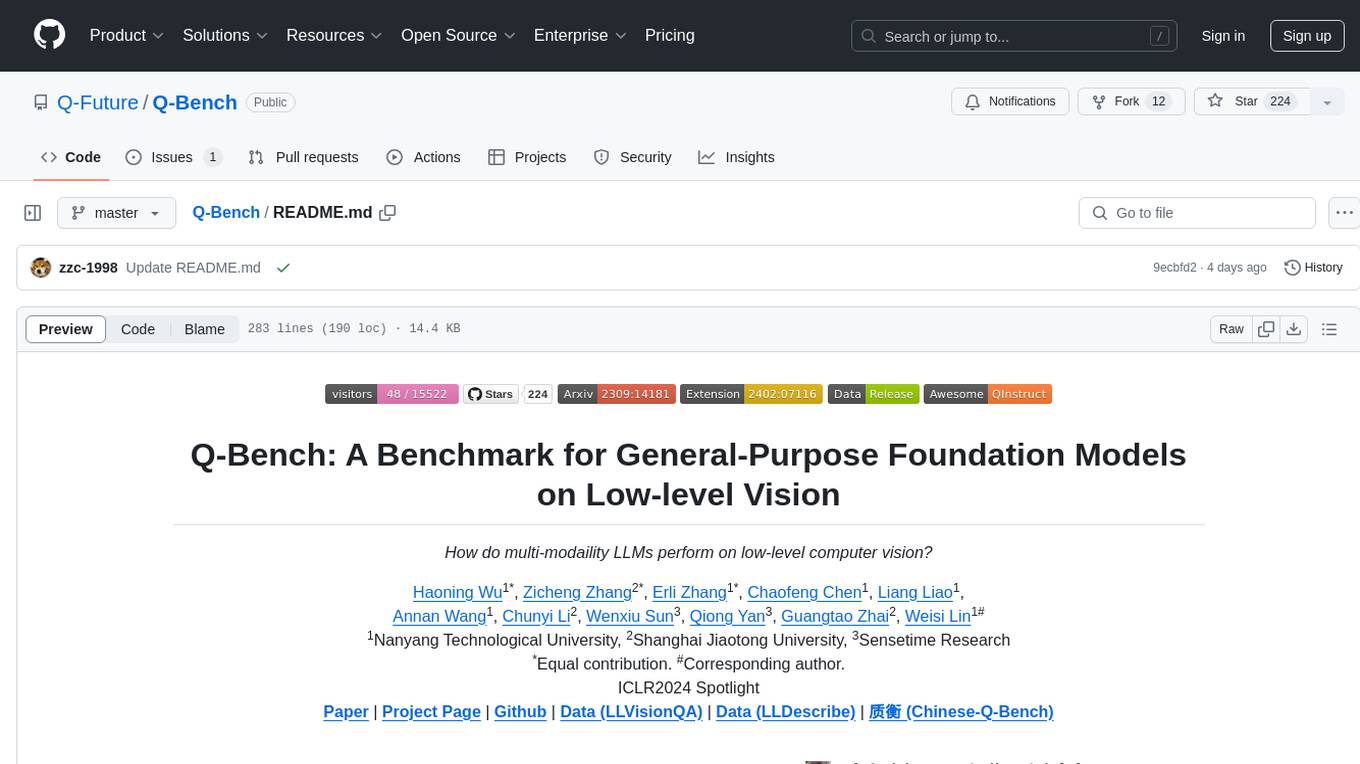
Q-Bench
Q-Bench is a benchmark for general-purpose foundation models on low-level vision, focusing on multi-modality LLMs performance. It includes three realms for low-level vision: perception, description, and assessment. The benchmark datasets LLVisionQA and LLDescribe are collected for perception and description tasks, with open submission-based evaluation. An abstract evaluation code is provided for assessment using public datasets. The tool can be used with the datasets API for single images and image pairs, allowing for automatic download and usage. Various tasks and evaluations are available for testing MLLMs on low-level vision tasks.
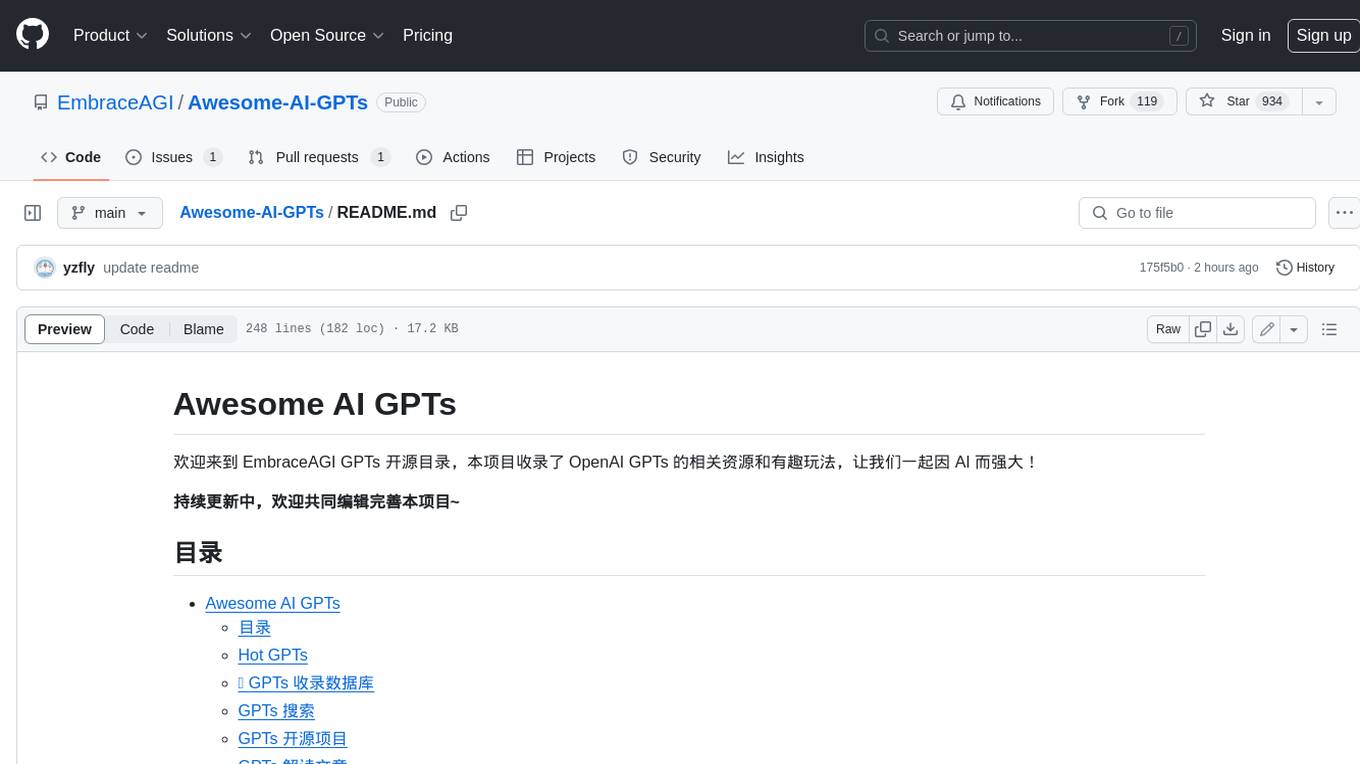
Awesome-AI-GPTs
Awesome AI GPTs is an open repository that collects resources and fun ways to use OpenAI GPTs. It includes databases, search tools, open-source projects, articles, attack and defense strategies, installation of custom plugins, knowledge bases, and community interactions related to GPTs. Users can find curated lists, leaked prompts, and various GPT applications in this repository. The project aims to empower users with AI capabilities and foster collaboration in the AI community.
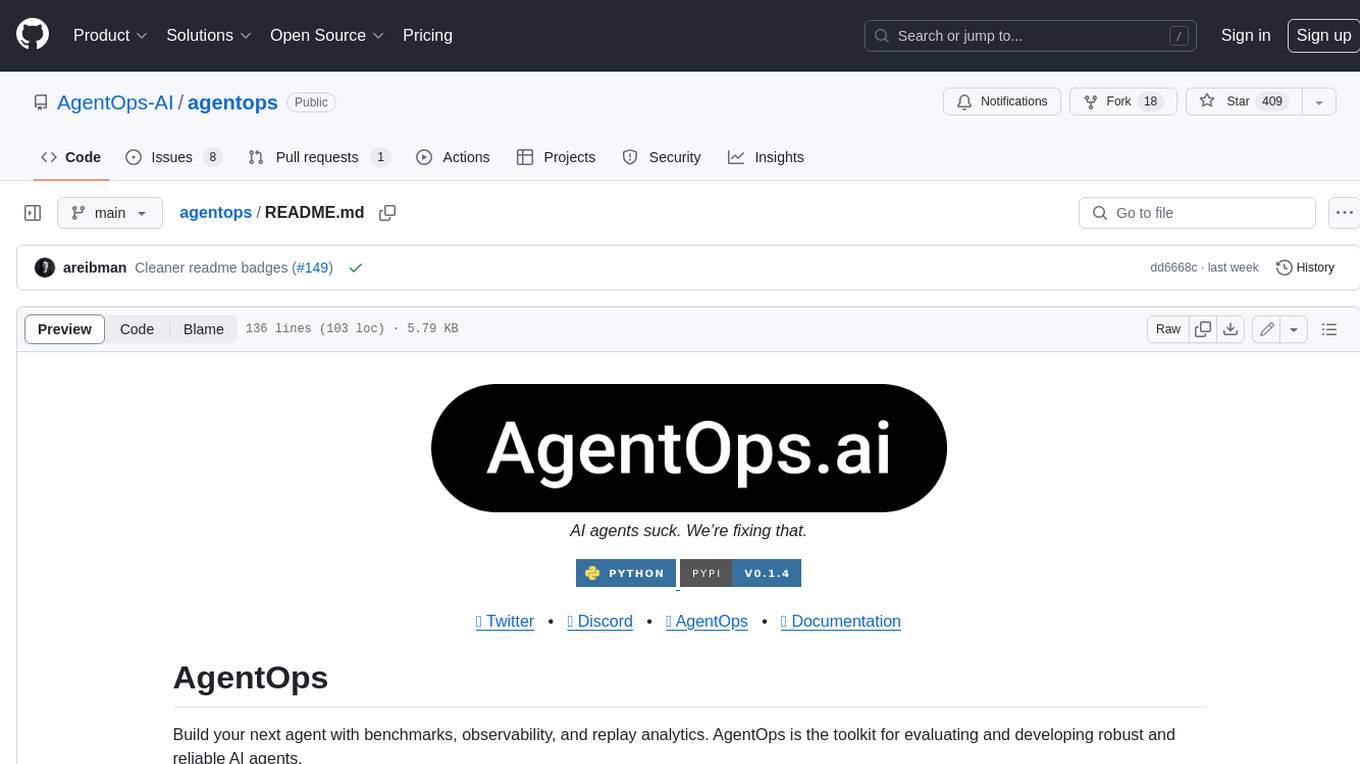
agentops
AgentOps is a toolkit for evaluating and developing robust and reliable AI agents. It provides benchmarks, observability, and replay analytics to help developers build better agents. AgentOps is open beta and can be signed up for here. Key features of AgentOps include: - Session replays in 3 lines of code: Initialize the AgentOps client and automatically get analytics on every LLM call. - Time travel debugging: (coming soon!) - Agent Arena: (coming soon!) - Callback handlers: AgentOps works seamlessly with applications built using Langchain and LlamaIndex.
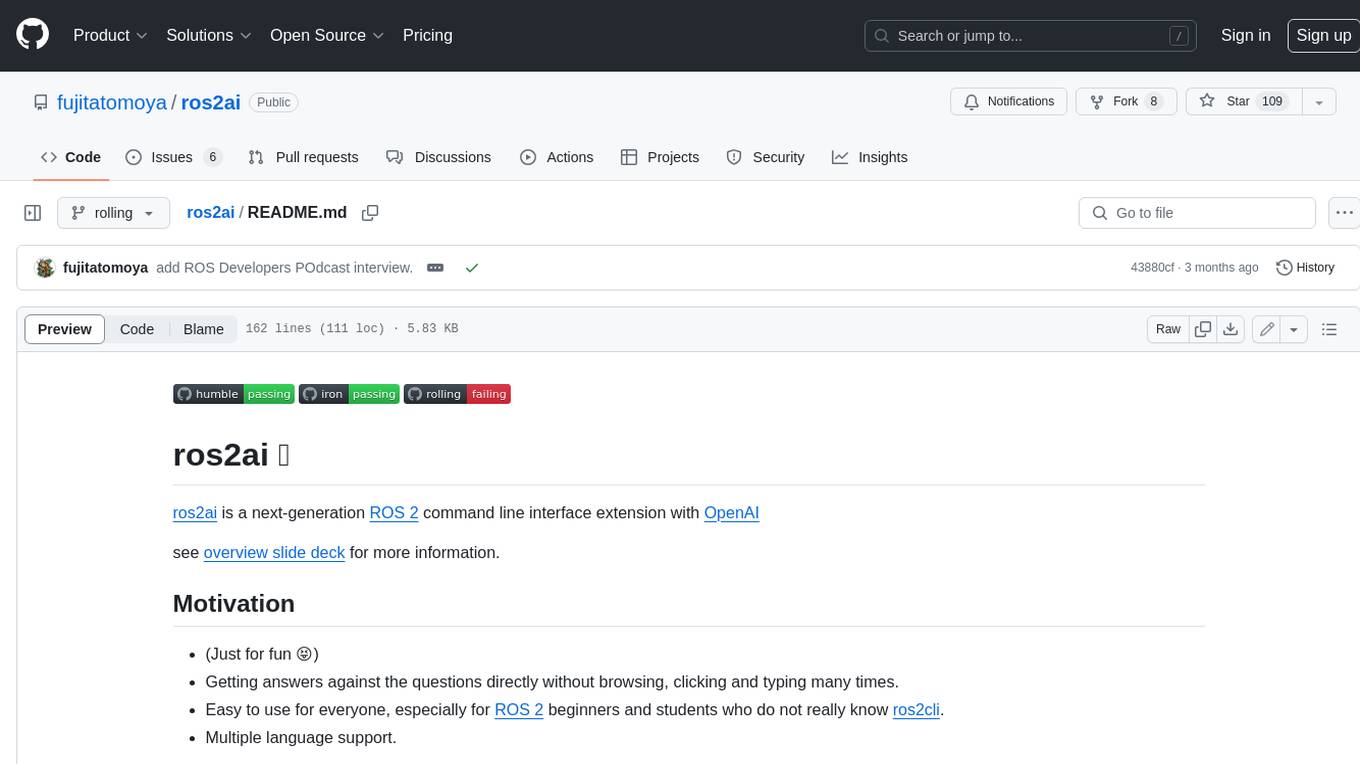
ros2ai
ros2ai is a next-generation ROS 2 command line interface extension with OpenAI. It allows users to ask questions about ROS 2, get answers, and execute commands using natural language. ros2ai is easy to use, especially for ROS 2 beginners and students who do not really know ros2cli. It supports multiple languages and is available as a Docker container or can be built from source.
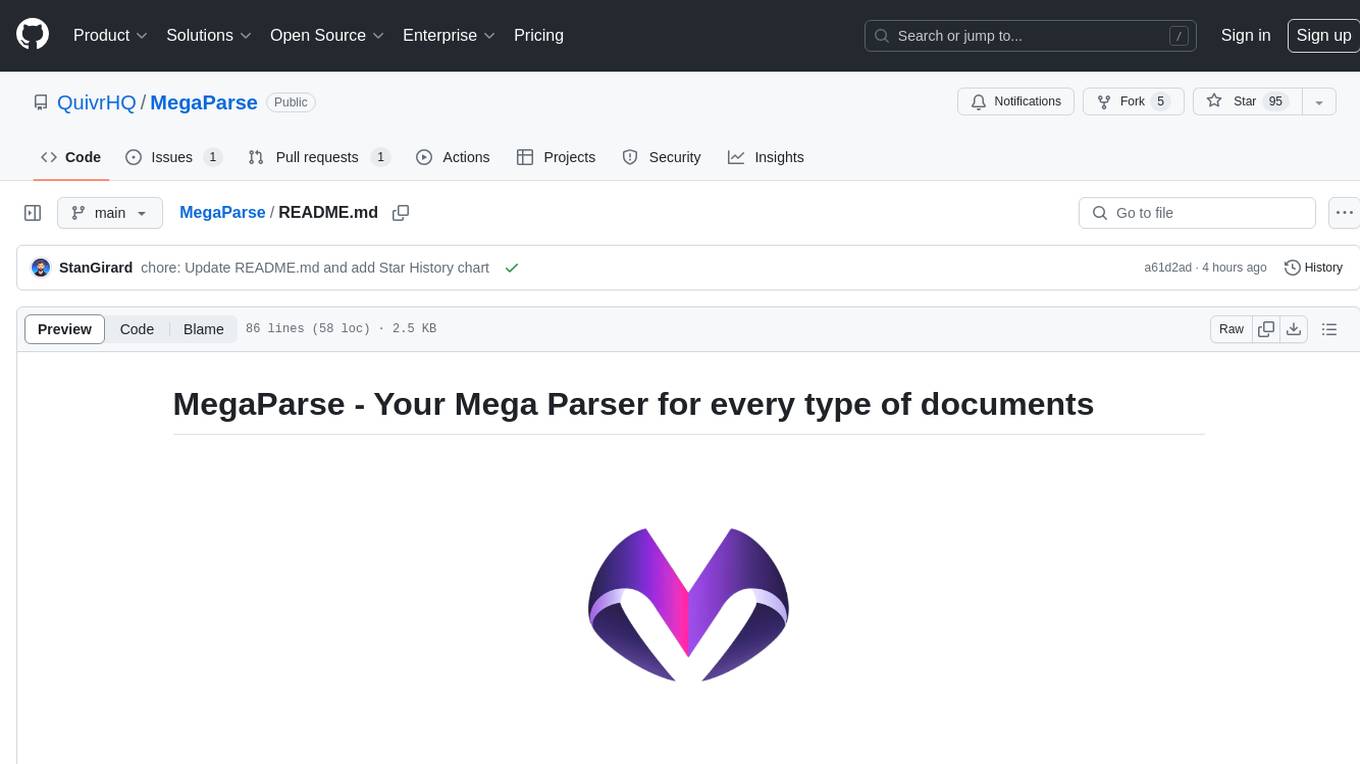
MegaParse
MegaParse is a powerful and versatile parser designed to handle various types of documents such as text, PDFs, Powerpoint presentations, and Word documents with no information loss. It is fast, efficient, and open source, supporting a wide range of file formats. MegaParse ensures compatibility with tables, table of contents, headers, footers, and images, making it a comprehensive solution for document parsing.
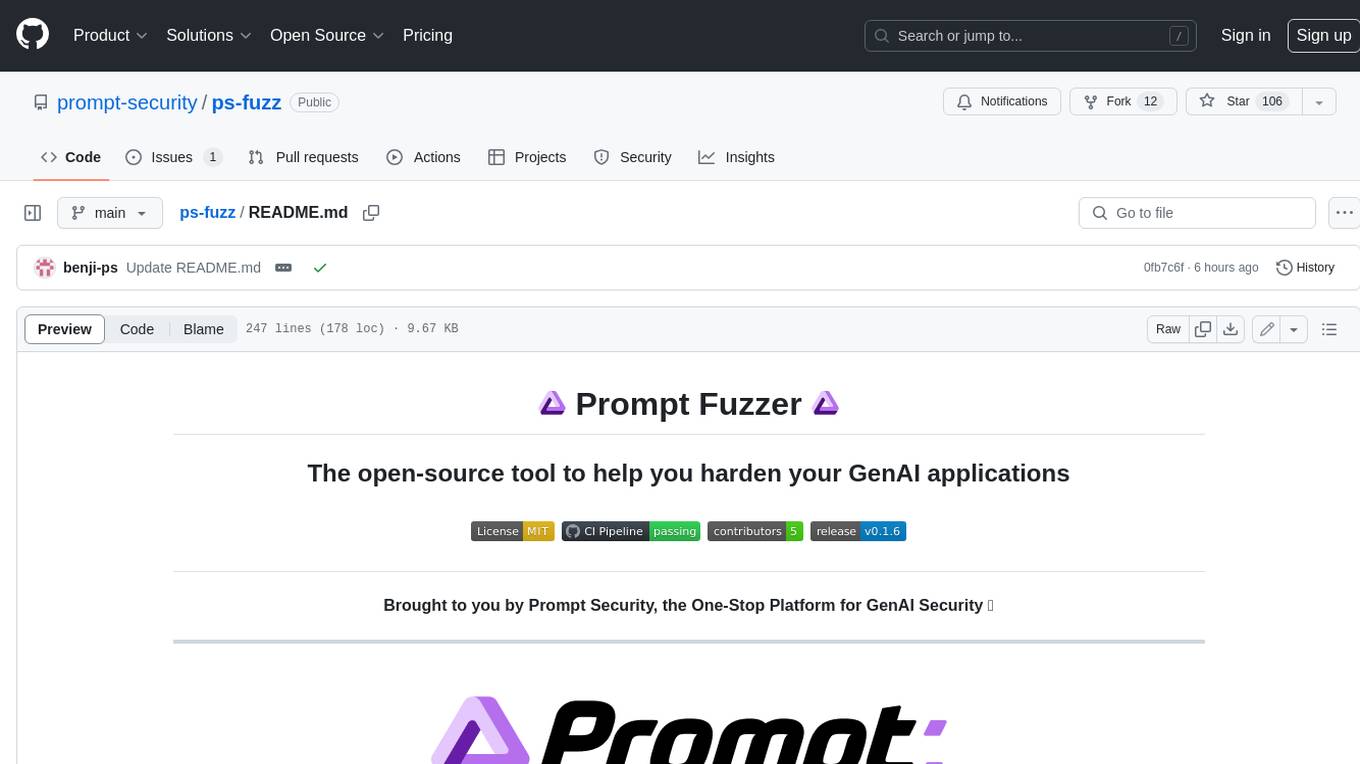
ps-fuzz
The Prompt Fuzzer is an open-source tool that helps you assess the security of your GenAI application's system prompt against various dynamic LLM-based attacks. It provides a security evaluation based on the outcome of these attack simulations, enabling you to strengthen your system prompt as needed. The Prompt Fuzzer dynamically tailors its tests to your application's unique configuration and domain. The Fuzzer also includes a Playground chat interface, giving you the chance to iteratively improve your system prompt, hardening it against a wide spectrum of generative AI attacks.
For similar tasks
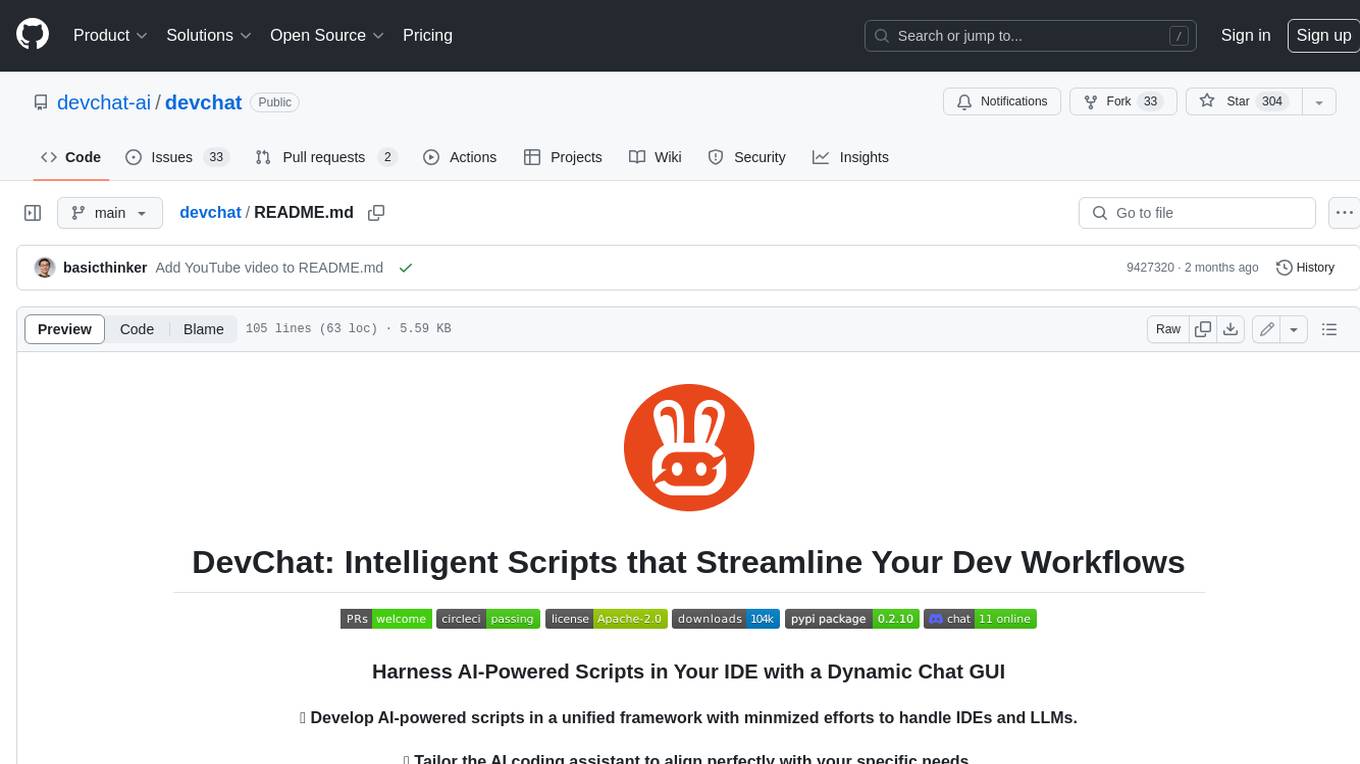
devchat
DevChat is an open-source workflow engine that enables developers to create intelligent, automated workflows for engaging with users through a chat panel within their IDEs. It combines script writing flexibility, latest AI models, and an intuitive chat GUI to enhance user experience and productivity. DevChat simplifies the integration of AI in software development, unlocking new possibilities for developers.
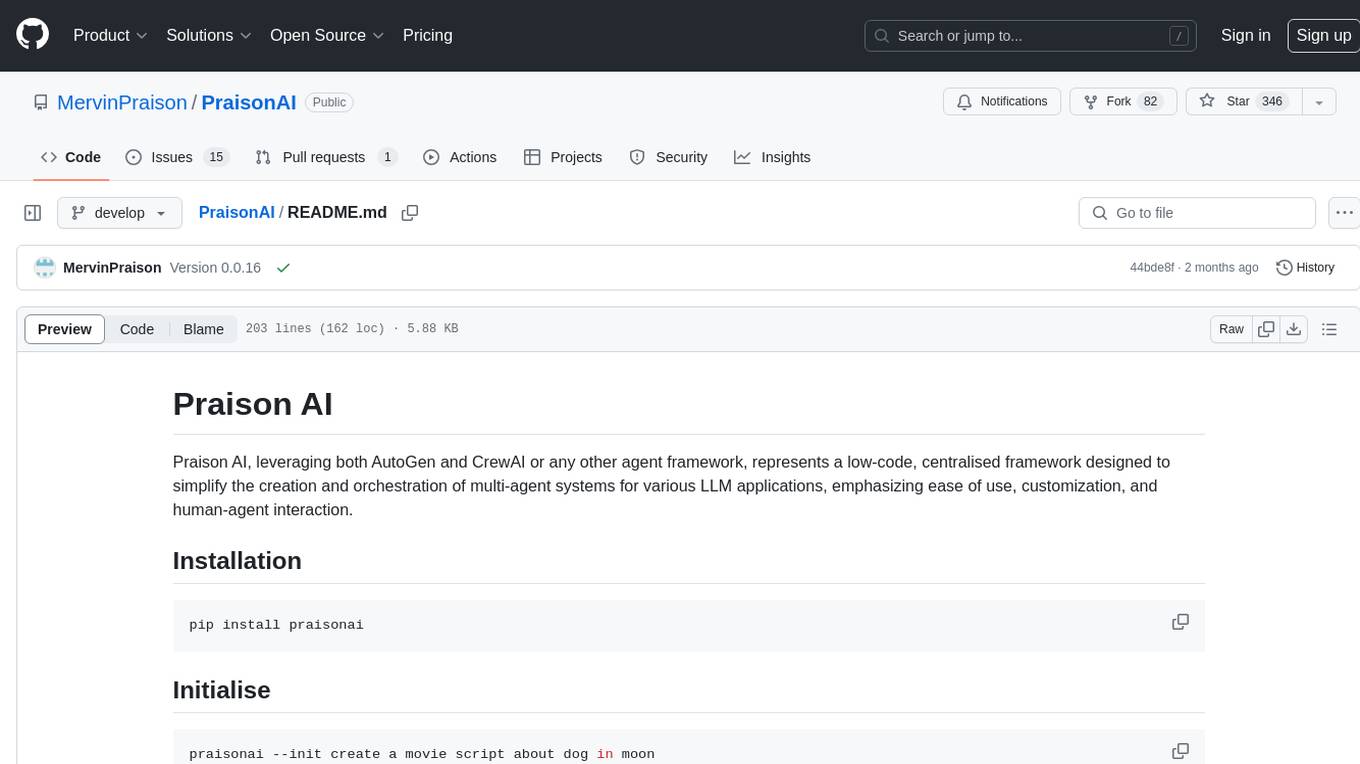
PraisonAI
Praison AI is a low-code, centralised framework that simplifies the creation and orchestration of multi-agent systems for various LLM applications. It emphasizes ease of use, customization, and human-agent interaction. The tool leverages AutoGen and CrewAI frameworks to facilitate the development of AI-generated scripts and movie concepts. Users can easily create, run, test, and deploy agents for scriptwriting and movie concept development. Praison AI also provides options for full automatic mode and integration with OpenAI models for enhanced AI capabilities.
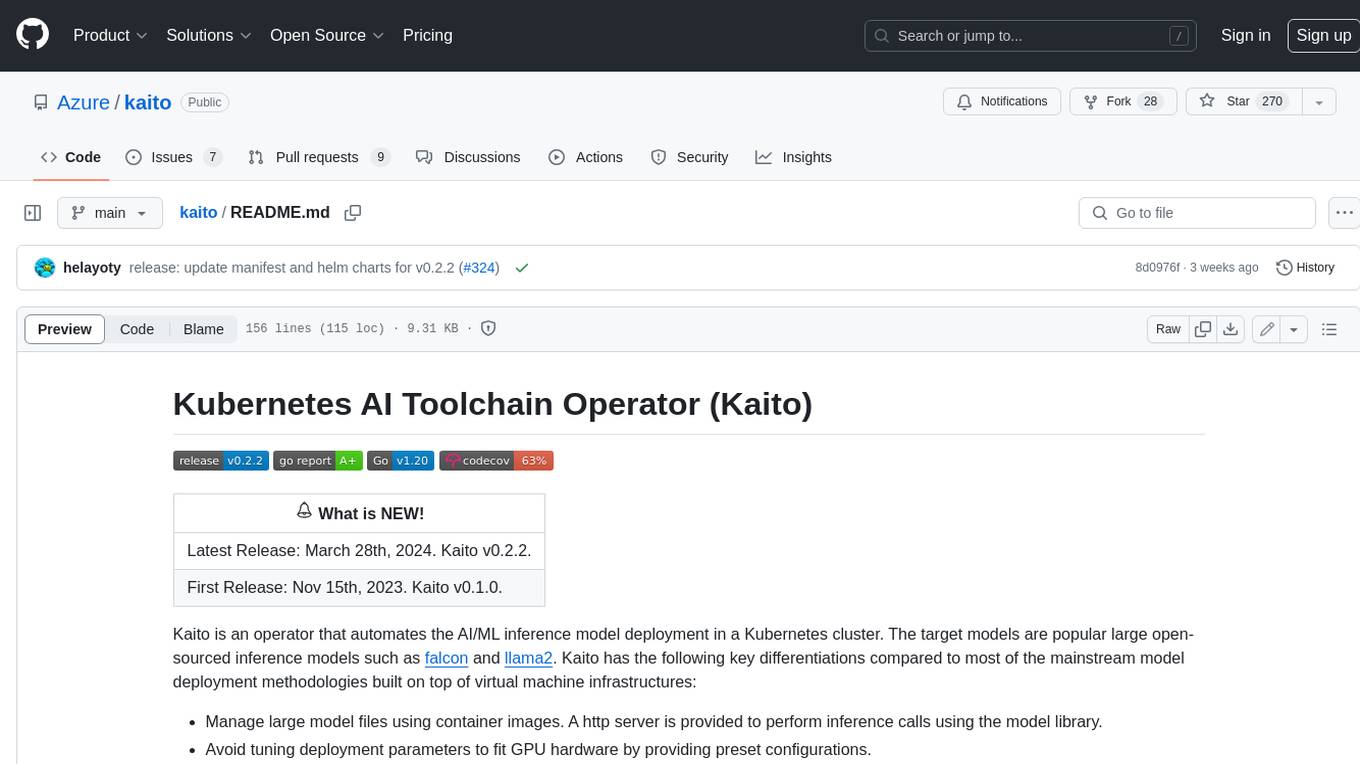
kaito
Kaito is an operator that automates the AI/ML inference model deployment in a Kubernetes cluster. It manages large model files using container images, avoids tuning deployment parameters to fit GPU hardware by providing preset configurations, auto-provisions GPU nodes based on model requirements, and hosts large model images in the public Microsoft Container Registry (MCR) if the license allows. Using Kaito, the workflow of onboarding large AI inference models in Kubernetes is largely simplified.
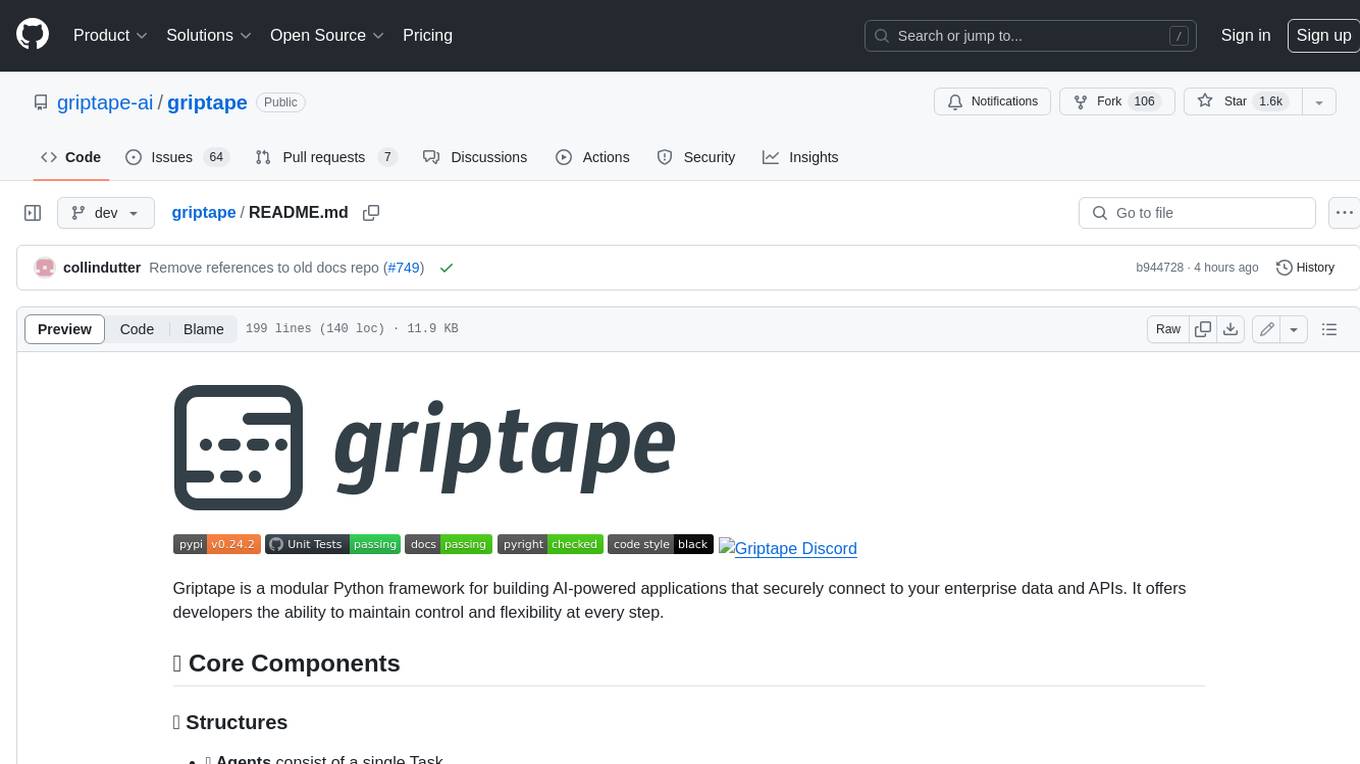
griptape
Griptape is a modular Python framework for building AI-powered applications that securely connect to your enterprise data and APIs. It offers developers the ability to maintain control and flexibility at every step. Griptape's core components include Structures (Agents, Pipelines, and Workflows), Tasks, Tools, Memory (Conversation Memory, Task Memory, and Meta Memory), Drivers (Prompt and Embedding Drivers, Vector Store Drivers, Image Generation Drivers, Image Query Drivers, SQL Drivers, Web Scraper Drivers, and Conversation Memory Drivers), Engines (Query Engines, Extraction Engines, Summary Engines, Image Generation Engines, and Image Query Engines), and additional components (Rulesets, Loaders, Artifacts, Chunkers, and Tokenizers). Griptape enables developers to create AI-powered applications with ease and efficiency.
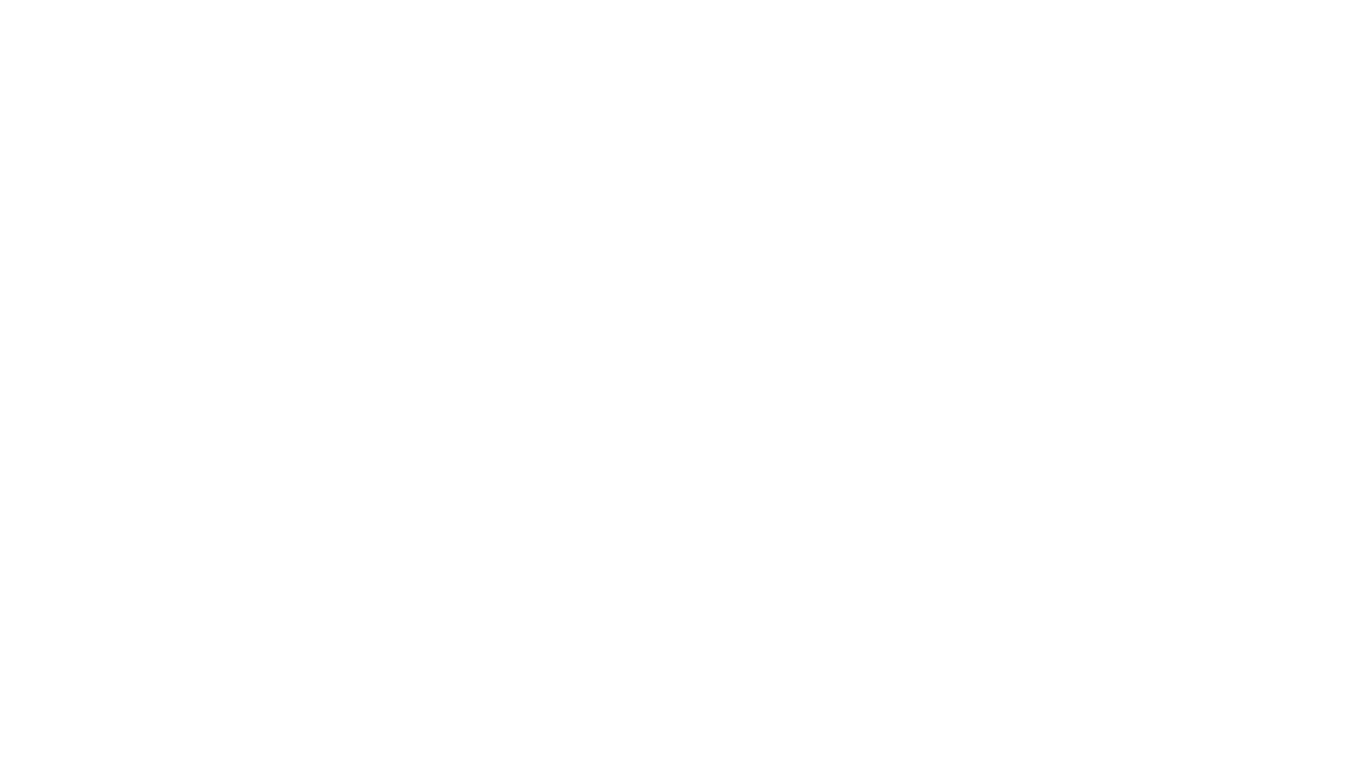
zep-python
Zep is an open-source platform for building and deploying large language model (LLM) applications. It provides a suite of tools and services that make it easy to integrate LLMs into your applications, including chat history memory, embedding, vector search, and data enrichment. Zep is designed to be scalable, reliable, and easy to use, making it a great choice for developers who want to build LLM-powered applications quickly and easily.
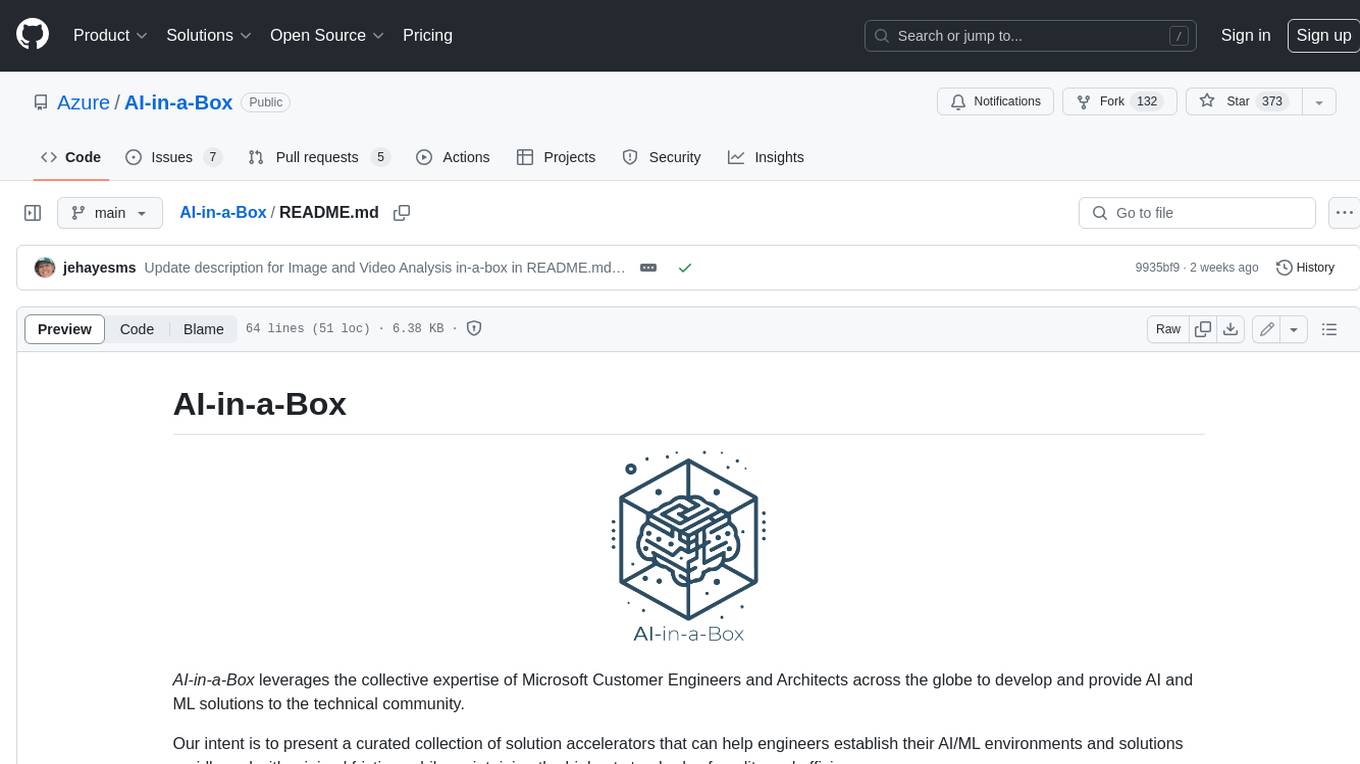
AI-in-a-Box
AI-in-a-Box is a curated collection of solution accelerators that can help engineers establish their AI/ML environments and solutions rapidly and with minimal friction, while maintaining the highest standards of quality and efficiency. It provides essential guidance on the responsible use of AI and LLM technologies, specific security guidance for Generative AI (GenAI) applications, and best practices for scaling OpenAI applications within Azure. The available accelerators include: Azure ML Operationalization in-a-box, Edge AI in-a-box, Doc Intelligence in-a-box, Image and Video Analysis in-a-box, Cognitive Services Landing Zone in-a-box, Semantic Kernel Bot in-a-box, NLP to SQL in-a-box, Assistants API in-a-box, and Assistants API Bot in-a-box.
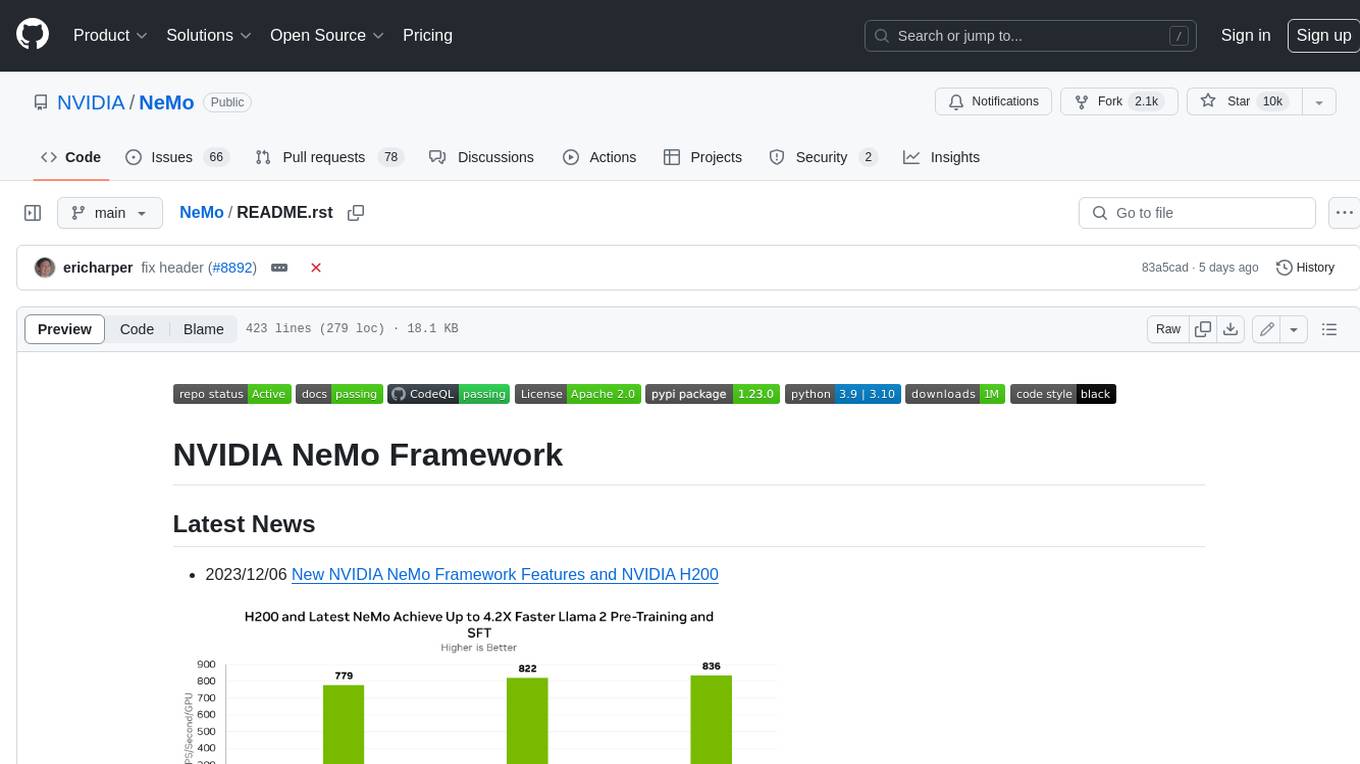
NeMo
NeMo Framework is a generative AI framework built for researchers and pytorch developers working on large language models (LLMs), multimodal models (MM), automatic speech recognition (ASR), and text-to-speech synthesis (TTS). The primary objective of NeMo is to provide a scalable framework for researchers and developers from industry and academia to more easily implement and design new generative AI models by being able to leverage existing code and pretrained models.
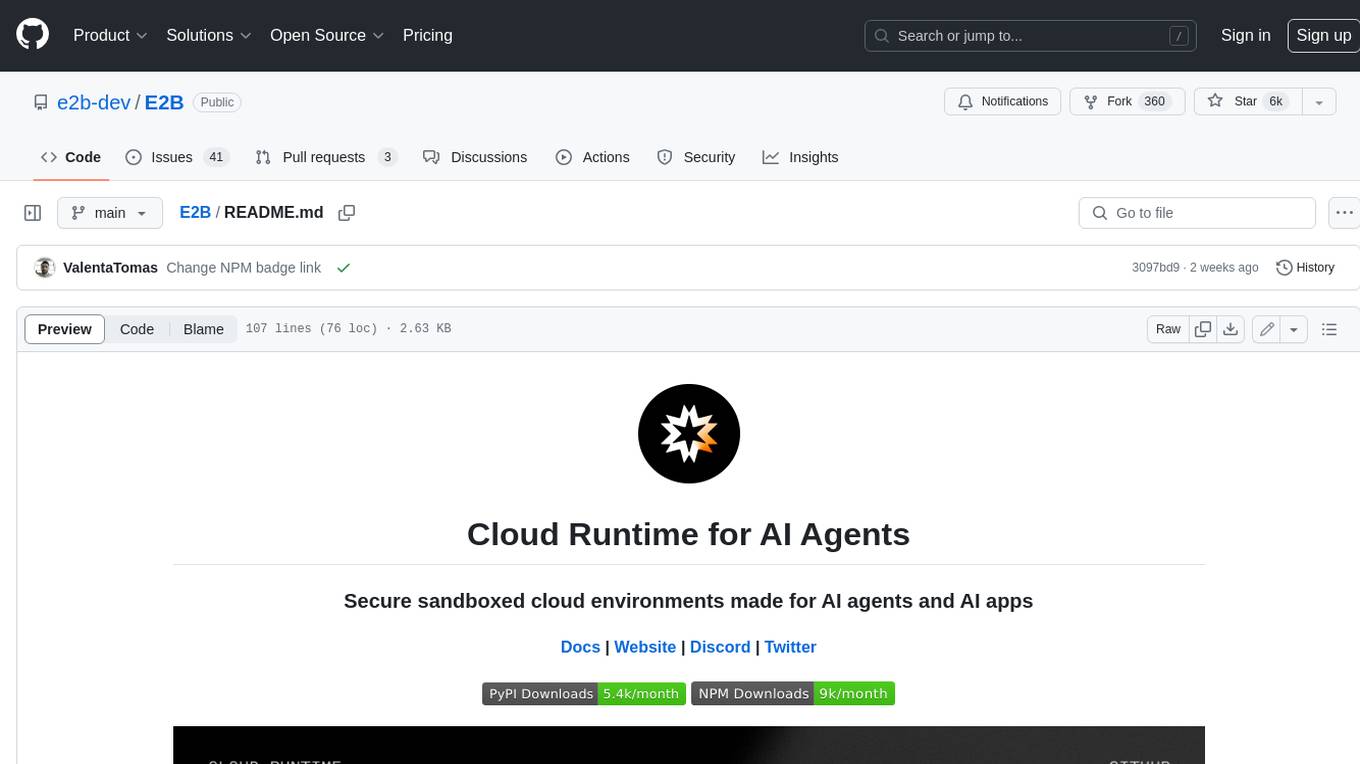
E2B
E2B Sandbox is a secure sandboxed cloud environment made for AI agents and AI apps. Sandboxes allow AI agents and apps to have long running cloud secure environments. In these environments, large language models can use the same tools as humans do. For example: * Cloud browsers * GitHub repositories and CLIs * Coding tools like linters, autocomplete, "go-to defintion" * Running LLM generated code * Audio & video editing The E2B sandbox can be connected to any LLM and any AI agent or app.
For similar jobs
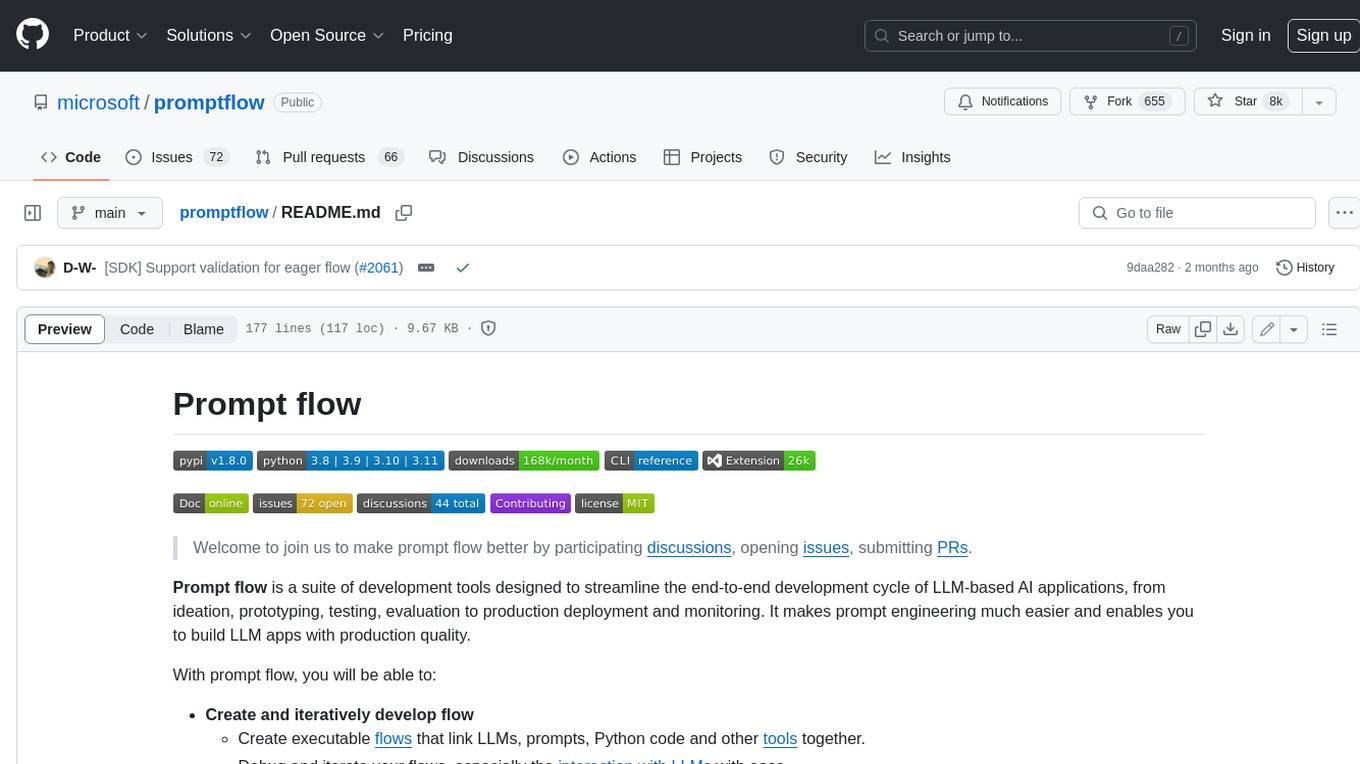
promptflow
**Prompt flow** is a suite of development tools designed to streamline the end-to-end development cycle of LLM-based AI applications, from ideation, prototyping, testing, evaluation to production deployment and monitoring. It makes prompt engineering much easier and enables you to build LLM apps with production quality.
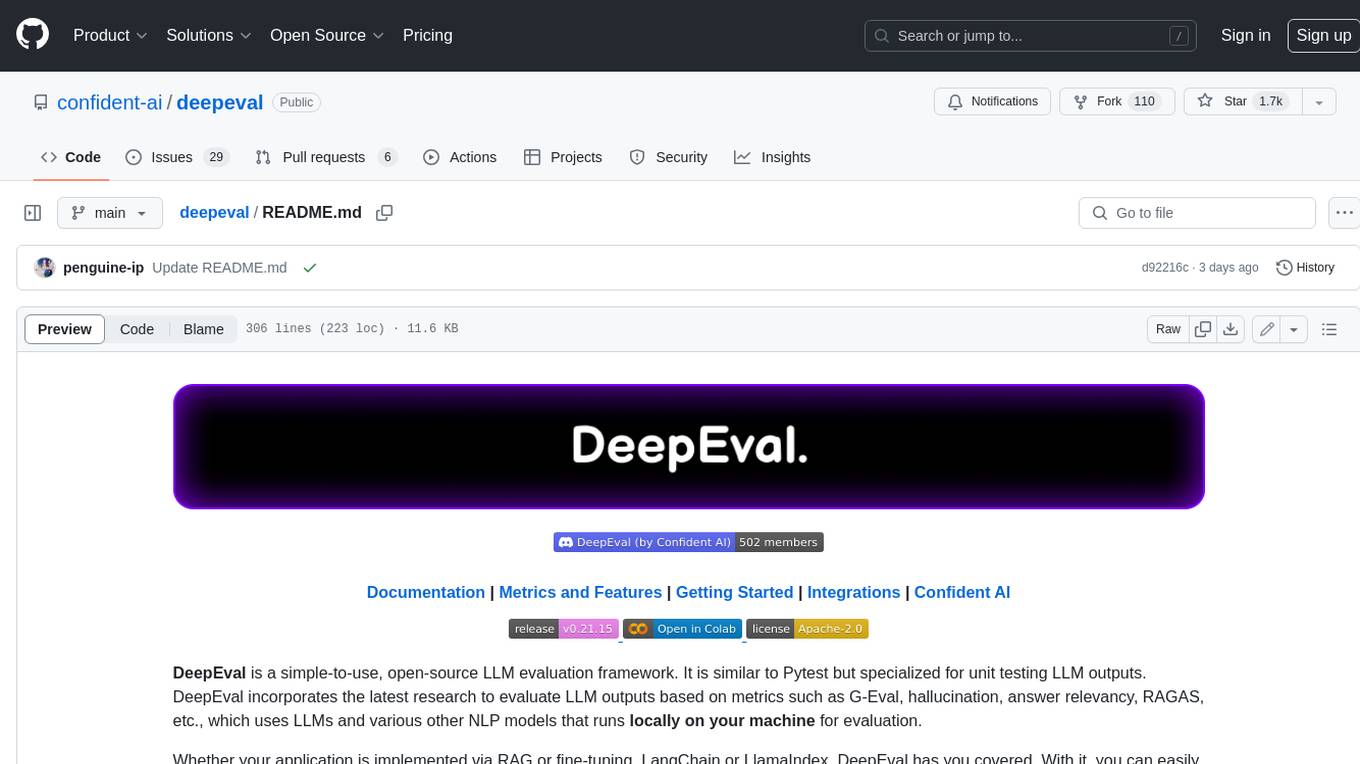
deepeval
DeepEval is a simple-to-use, open-source LLM evaluation framework specialized for unit testing LLM outputs. It incorporates various metrics such as G-Eval, hallucination, answer relevancy, RAGAS, etc., and runs locally on your machine for evaluation. It provides a wide range of ready-to-use evaluation metrics, allows for creating custom metrics, integrates with any CI/CD environment, and enables benchmarking LLMs on popular benchmarks. DeepEval is designed for evaluating RAG and fine-tuning applications, helping users optimize hyperparameters, prevent prompt drifting, and transition from OpenAI to hosting their own Llama2 with confidence.
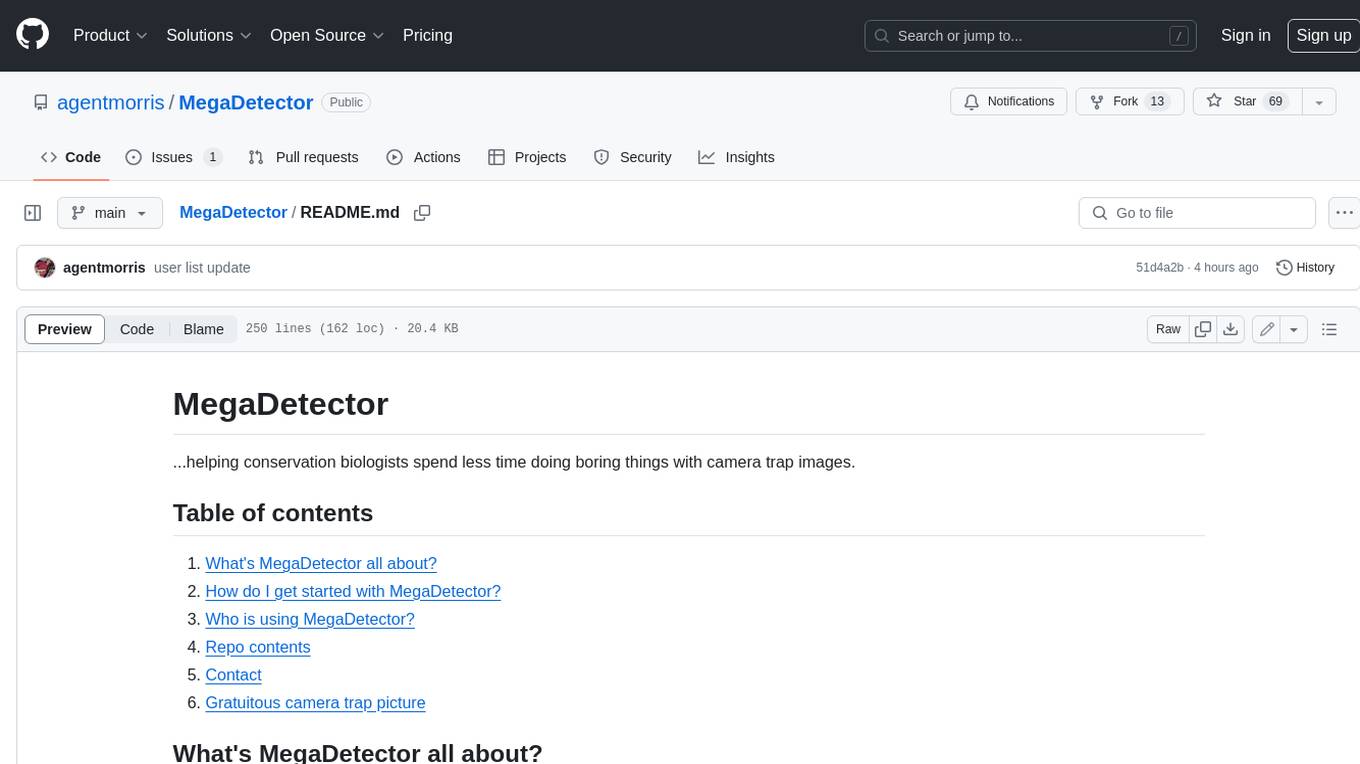
MegaDetector
MegaDetector is an AI model that identifies animals, people, and vehicles in camera trap images (which also makes it useful for eliminating blank images). This model is trained on several million images from a variety of ecosystems. MegaDetector is just one of many tools that aims to make conservation biologists more efficient with AI. If you want to learn about other ways to use AI to accelerate camera trap workflows, check out our of the field, affectionately titled "Everything I know about machine learning and camera traps".
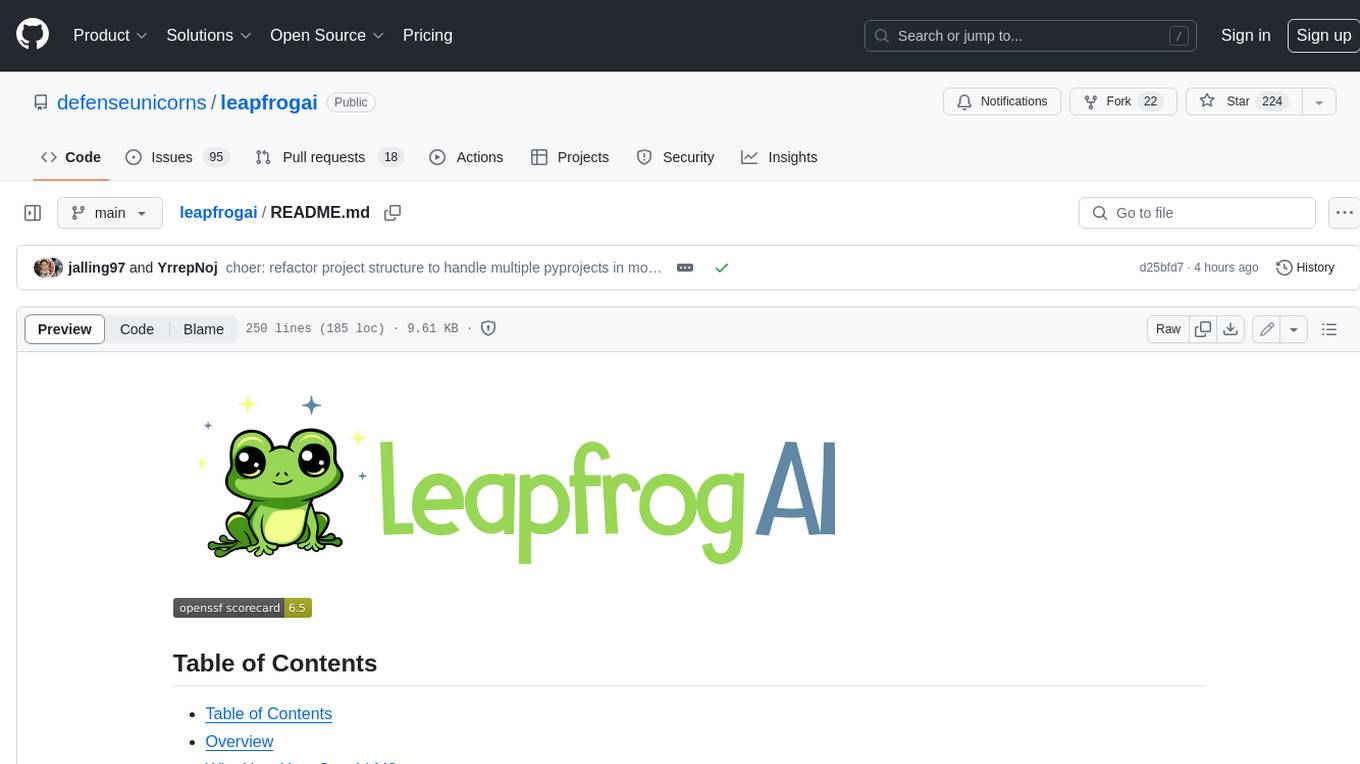
leapfrogai
LeapfrogAI is a self-hosted AI platform designed to be deployed in air-gapped resource-constrained environments. It brings sophisticated AI solutions to these environments by hosting all the necessary components of an AI stack, including vector databases, model backends, API, and UI. LeapfrogAI's API closely matches that of OpenAI, allowing tools built for OpenAI/ChatGPT to function seamlessly with a LeapfrogAI backend. It provides several backends for various use cases, including llama-cpp-python, whisper, text-embeddings, and vllm. LeapfrogAI leverages Chainguard's apko to harden base python images, ensuring the latest supported Python versions are used by the other components of the stack. The LeapfrogAI SDK provides a standard set of protobuffs and python utilities for implementing backends and gRPC. LeapfrogAI offers UI options for common use-cases like chat, summarization, and transcription. It can be deployed and run locally via UDS and Kubernetes, built out using Zarf packages. LeapfrogAI is supported by a community of users and contributors, including Defense Unicorns, Beast Code, Chainguard, Exovera, Hypergiant, Pulze, SOSi, United States Navy, United States Air Force, and United States Space Force.
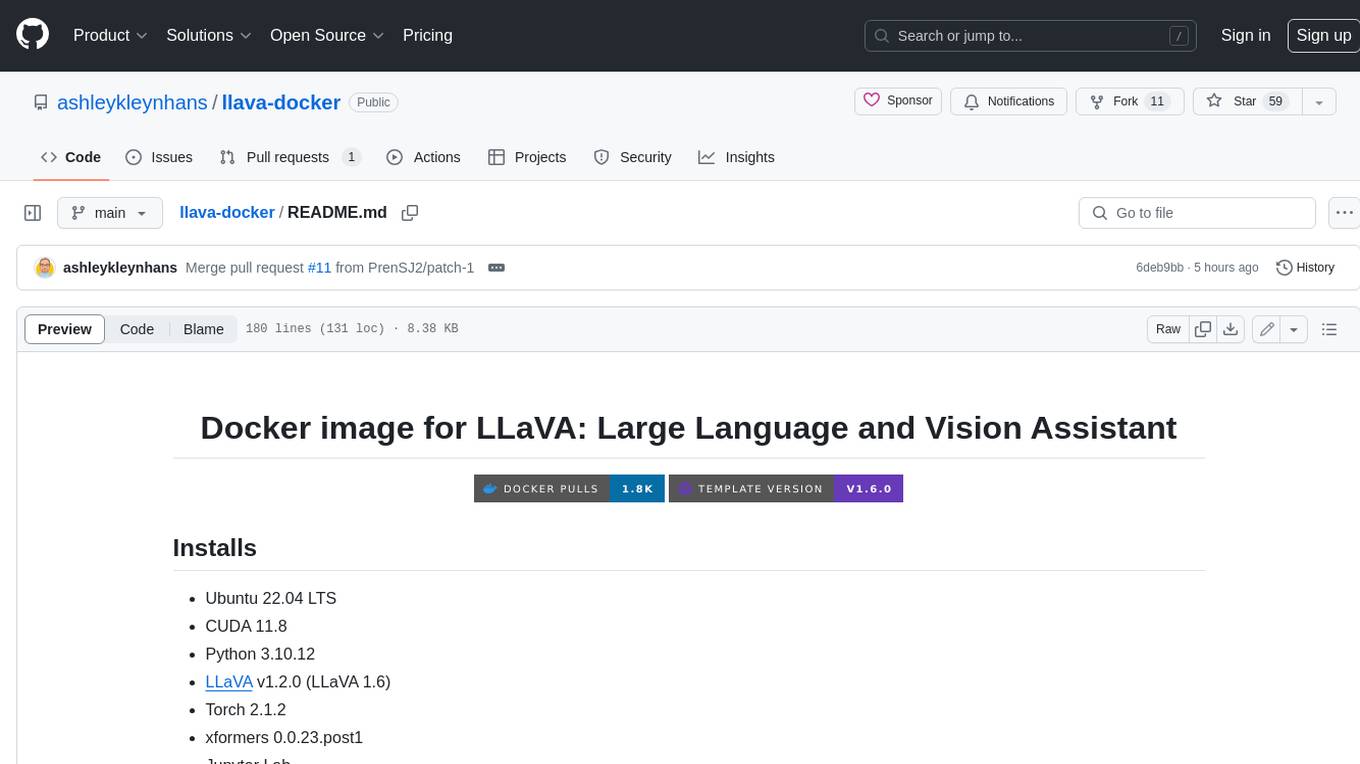
llava-docker
This Docker image for LLaVA (Large Language and Vision Assistant) provides a convenient way to run LLaVA locally or on RunPod. LLaVA is a powerful AI tool that combines natural language processing and computer vision capabilities. With this Docker image, you can easily access LLaVA's functionalities for various tasks, including image captioning, visual question answering, text summarization, and more. The image comes pre-installed with LLaVA v1.2.0, Torch 2.1.2, xformers 0.0.23.post1, and other necessary dependencies. You can customize the model used by setting the MODEL environment variable. The image also includes a Jupyter Lab environment for interactive development and exploration. Overall, this Docker image offers a comprehensive and user-friendly platform for leveraging LLaVA's capabilities.
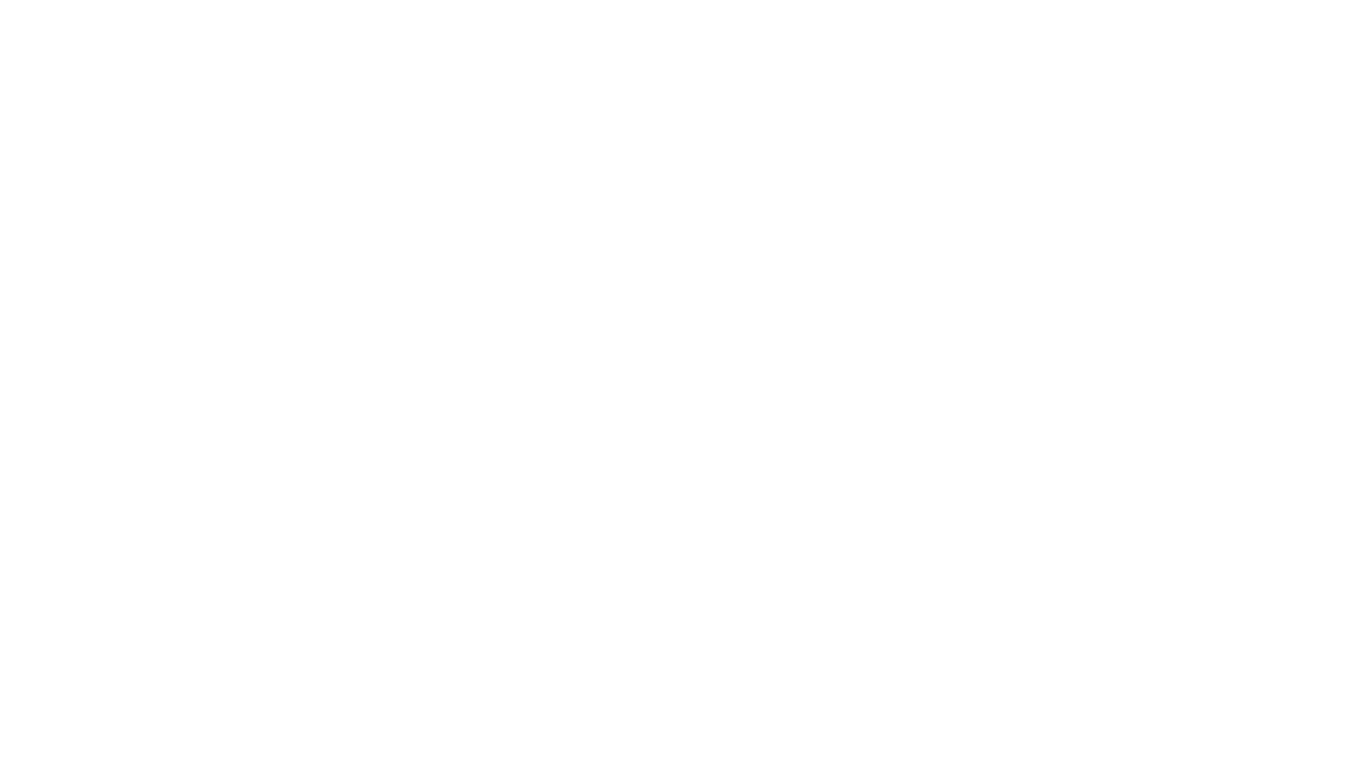
carrot
The 'carrot' repository on GitHub provides a list of free and user-friendly ChatGPT mirror sites for easy access. The repository includes sponsored sites offering various GPT models and services. Users can find and share sites, report errors, and access stable and recommended sites for ChatGPT usage. The repository also includes a detailed list of ChatGPT sites, their features, and accessibility options, making it a valuable resource for ChatGPT users seeking free and unlimited GPT services.
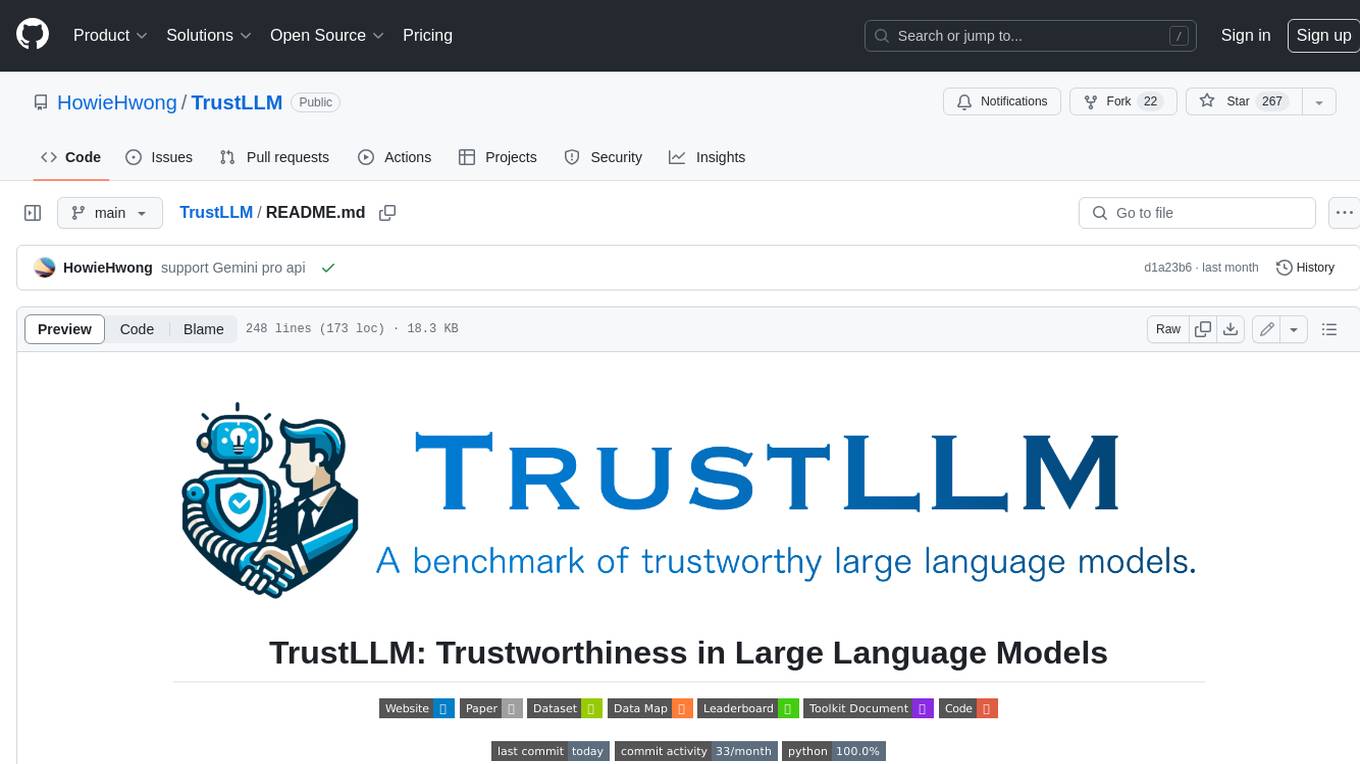
TrustLLM
TrustLLM is a comprehensive study of trustworthiness in LLMs, including principles for different dimensions of trustworthiness, established benchmark, evaluation, and analysis of trustworthiness for mainstream LLMs, and discussion of open challenges and future directions. Specifically, we first propose a set of principles for trustworthy LLMs that span eight different dimensions. Based on these principles, we further establish a benchmark across six dimensions including truthfulness, safety, fairness, robustness, privacy, and machine ethics. We then present a study evaluating 16 mainstream LLMs in TrustLLM, consisting of over 30 datasets. The document explains how to use the trustllm python package to help you assess the performance of your LLM in trustworthiness more quickly. For more details about TrustLLM, please refer to project website.
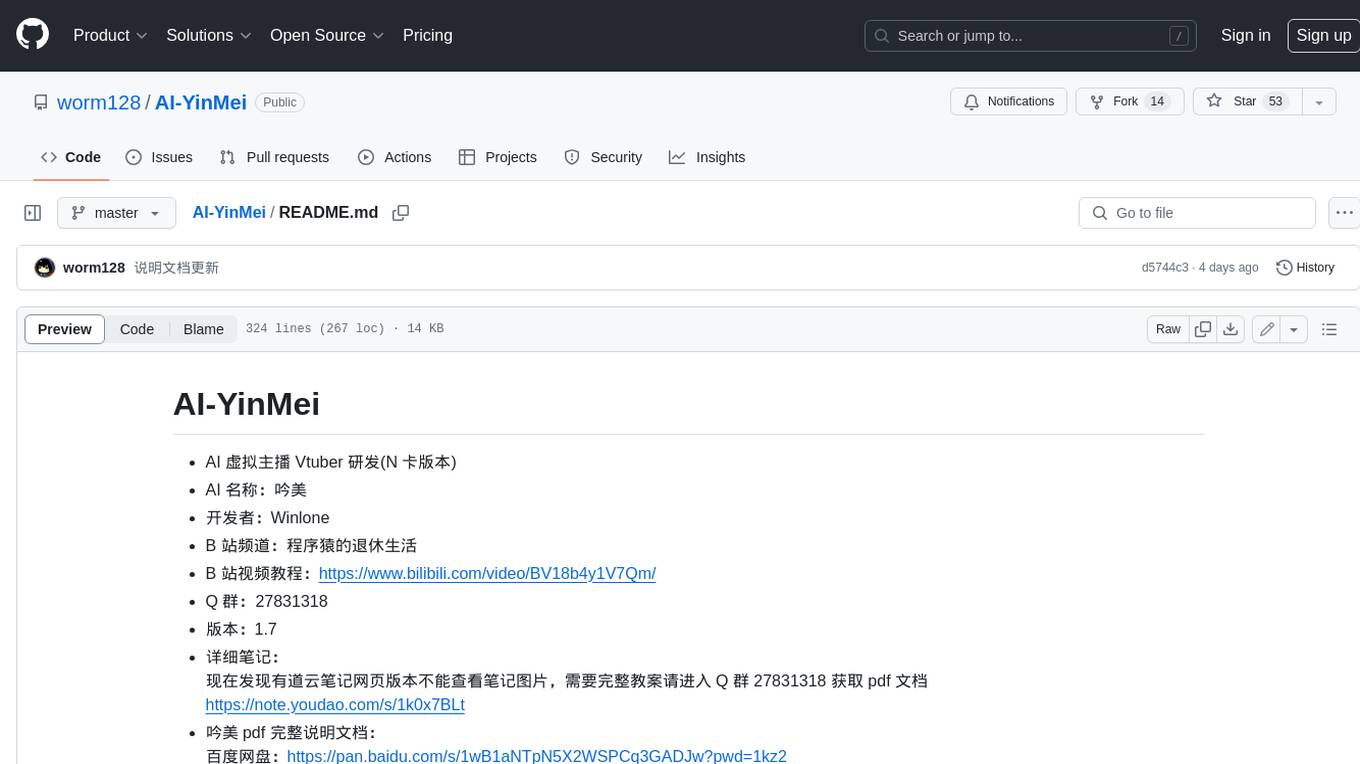
AI-YinMei
AI-YinMei is an AI virtual anchor Vtuber development tool (N card version). It supports fastgpt knowledge base chat dialogue, a complete set of solutions for LLM large language models: [fastgpt] + [one-api] + [Xinference], supports docking bilibili live broadcast barrage reply and entering live broadcast welcome speech, supports Microsoft edge-tts speech synthesis, supports Bert-VITS2 speech synthesis, supports GPT-SoVITS speech synthesis, supports expression control Vtuber Studio, supports painting stable-diffusion-webui output OBS live broadcast room, supports painting picture pornography public-NSFW-y-distinguish, supports search and image search service duckduckgo (requires magic Internet access), supports image search service Baidu image search (no magic Internet access), supports AI reply chat box [html plug-in], supports AI singing Auto-Convert-Music, supports playlist [html plug-in], supports dancing function, supports expression video playback, supports head touching action, supports gift smashing action, supports singing automatic start dancing function, chat and singing automatic cycle swing action, supports multi scene switching, background music switching, day and night automatic switching scene, supports open singing and painting, let AI automatically judge the content.