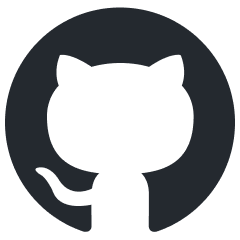
google-cloud-gcp-openai-api
🌴 Drop-in replacement REST API for Vertex AI (PaLM 2, Codey, Gemini) that is compatible with the OpenAI API specifications
Stars: 67
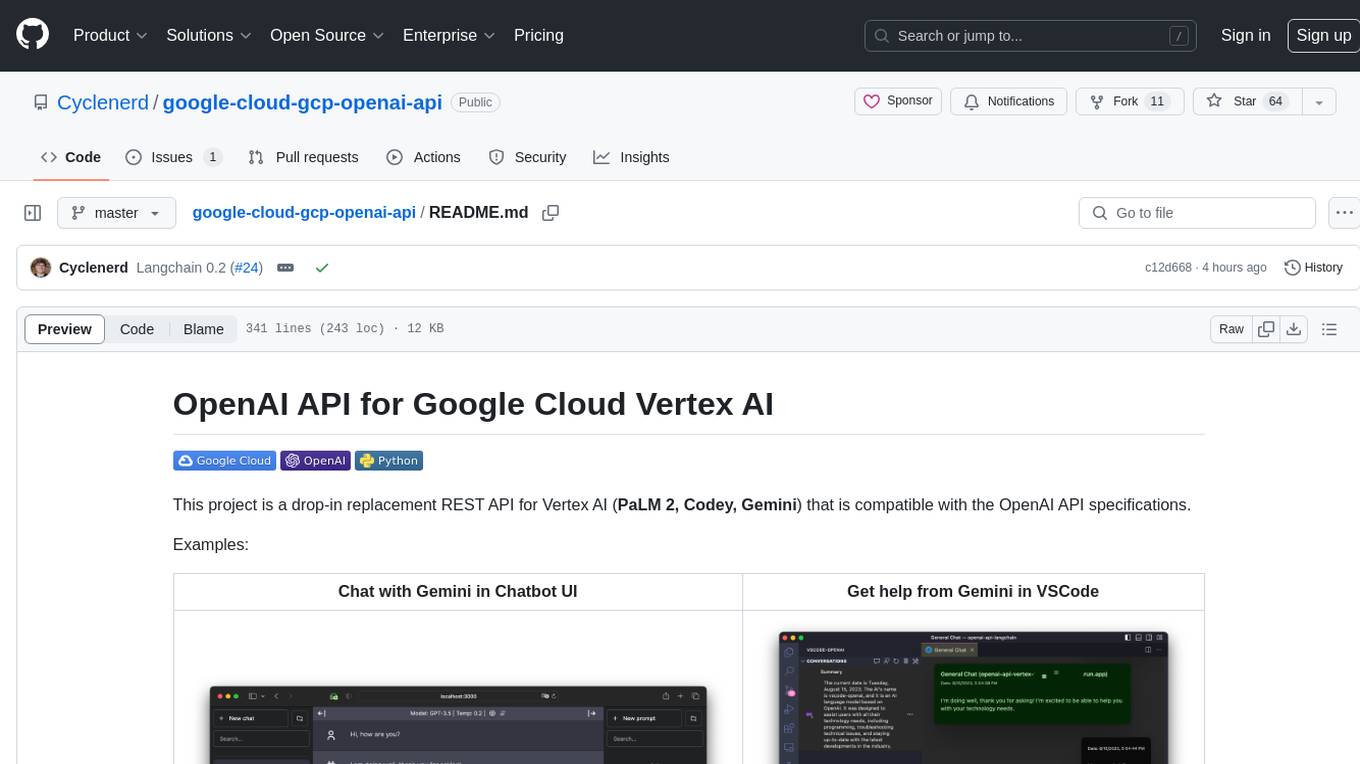
This project provides a drop-in replacement REST API for Google Cloud Vertex AI (PaLM 2, Codey, Gemini) that is compatible with the OpenAI API specifications. It aims to make Google Cloud Platform Vertex AI more accessible by translating OpenAI API calls to Vertex AI. The software is developed in Python and based on FastAPI and LangChain, designed to be simple and customizable for individual needs. It includes step-by-step guides for deployment, supports various OpenAI API services, and offers configuration through environment variables. Additionally, it provides examples for running locally and usage instructions consistent with the OpenAI API format.
README:
This project is a drop-in replacement REST API for Vertex AI (PaLM 2, Codey, Gemini) that is compatible with the OpenAI API specifications.
Examples:
Chat with Gemini in Chatbot UI | Get help from Gemini in VSCode |
---|---|
![]() |
![]() |
This project is inspired by the idea of LocalAI but with the focus on making Google Cloud Platform Vertex AI PaLM more accessible to anyone.
A Google Cloud Run service is installed that translates the OpenAI API calls to Vertex AI (PaLM 2, Codey, Gemini).
Supported OpenAI API services:
OpenAI | API | Supported |
---|---|---|
List models | /v1/models |
✅ |
Chat Completions | /v1/chat/completions |
✅ |
Completions (Legacy) | /v1/completions |
❌ |
Embeddings | /v1/embeddings |
❌ |
The software is developed in Python and based on FastAPI and LangChain.
Everything is designed to be very simple, so you can easily adjust the source code to your individual needs.
A Jupyter notebook Vertex_AI_Chat.ipynb
with step-by-step instructions is prepared.
It will help you to deploy the API backend and Chatbot UI frontend as Google Cloud Run service.
Requirements:
Your user (the one used for deployment) must have proper permissions in the project. For a fast and hassle-free deployemnt the "Owner" role is recommended.
In addition, the default compute service account ([PROJECT_NR][email protected]
)
must have the role "Role Vertex AI User" (roles/aiplatform.user
).
Authenticate:
gcloud auth login
Set default project:
gcloud config set project [PROJECT_ID]
Run the following script to create a container image and deploy that container as a public API (which allows unauthenticated calls) in Google Cloud Run:
bash deploy.sh
Note: You can change the generated fake OpenAI API key and Google Cloud region with environment variables:
export OPENAI_API_KEY="sk-XYZ" export GOOGLE_CLOUD_LOCATION="europe-west1" bash deploy.sh
The software was tested on GNU/Linux and macOS with Python 3.11 and 3.12.3 (3.12.4 currently not working).
If you want to use the software under Windows, you must set the environment variables with set
instead of export
.
You should also create a virtual environment with the version of Python you want to use, and activate it before proceeding.
You also need the Google Cloud CLI.
The Google Cloud CLI includes the gcloud
command-line tool.
Initiate a Python virtual environment and install requirements:
python3 -m venv .venv && \
source .venv/bin/activate && \
pip install -r requirements.txt
Authenticate:
gcloud auth application-default login
Set default project:
gcloud auth application-default set-quota-project [PROJECT_ID]
Run with default model:
export DEBUG="True"
export OPENAI_API_KEY="sk-XYZ"
uvicorn vertex:app --reload
Example for Windows:
set DEBUG=True
set OPENAI_API_KEY=sk-XYZ
uvicorn vertex:app --reload
Run with Gemini gemini-pro
model:
export DEBUG="True"
export OPENAI_API_KEY="sk-XYZ"
export MODEL_NAME="gemini-pro"
uvicorn vertex:app --reload
Run with Codey codechat-bison-32k
model:
export DEBUG="True"
export OPENAI_API_KEY="sk-XYZ"
export MODEL_NAME="codechat-bison-32k"
export MAX_OUTPUT_TOKENS="16000"
uvicorn vertex:app --reload
The application will now be running on your local computer. You can access it by opening a web browser and navigating to the following address:
http://localhost:8000/
HTTP request and response formats are consistent with the OpenAI API.
For example, to generate a chat completion, you can send a POST request to the /v1/chat/completions
endpoint with the instruction as the request body:
curl --location 'http://[ENDPOINT]/v1/chat/completions' \
--header 'Content-Type: application/json' \
--header 'Authorization: Bearer [API-KEY]' \
--data '{
"model": "gpt-3.5-turbo",
"messages": [
{
"role": "user",
"content": "Say this is a test!"
}
]
}'
Response:
{
"id": "cmpl-efccdeb3d2a6cfe144fdde11",
"created": 1691577522,
"object": "chat.completion",
"model": "gpt-3.5-turbo",
"usage": {
"prompt_tokens": 0,
"completion_tokens": 0,
"total_tokens": 0
},
"choices": [
{
"message": {
"role": "assistant",
"content": "Sure, this is a test."
},
"finish_reason": "stop",
"index": 0
}
]
}
Download export for Bruno API client: bruno-export.json
The configuration of the software can be done with environment variables.
The following variables with default values exist:
Variable | Default | Description |
---|---|---|
DEBUG | False | Show debug messages that help during development. |
GOOGLE_CLOUD_LOCATION | us-central1 | Google Cloud Platform region for API calls. |
GOOGLE_CLOUD_PROJECT_ID | [DEFAULT_AUTH_PROJECT] | Identifier for your project. If not specified, the project of authentication is used. |
HOST | 0.0.0.0 | Bind socket to this host. |
MAX_OUTPUT_TOKENS | 512 | Token limit determines the maximum amount of text output from one prompt. Can be overridden by the end user as required by the OpenAI API specification. |
MODEL_NAME | chat-bison | One of the foundation models that are available in Vertex AI. |
OPENAI_API_KEY | sk-[RANDOM_HEX] | Self-generated fake OpenAI API key used for authentication against the application. |
PORT | 8000 | Bind socket to this port. |
TEMPERATURE | 0.2 | Sampling temperature, it controls the degree of randomness in token selection. Can be overridden by the end user as required by the OpenAI API specification. |
TOP_K | 40 | How the model selects tokens for output, the next token is selected from. |
TOP_P | 0.8 | Tokens are selected from most probable to least until the sum of their. Can be overridden by the end user as required by the OpenAI API specification. |
If your application uses client libraries provided by OpenAI,
you only need to modify the OPENAI_API_BASE
environment variable to match your Google Cloud Run endpoint URL:
export OPENAI_API_BASE="https://openai-api-vertex-XYZ.a.run.app/v1"
python your_openai_app.py
When deploying the Chatbot UI application, the following environment variables must be set:
Variable | Value |
---|---|
OPENAI_API_KEY | API key generated during deployment |
OPENAI_API_HOST | Google Cloud Run URL |
Run the following script to create a container image from the GitHub source code and deploy that container as a public website (which allows unauthenticated calls) in Google Cloud Run:
export OPENAI_API_KEY="sk-XYZ"
export OPENAI_API_HOST="https://openai-api-vertex-XYZ.a.run.app"
bash chatbot-ui.sh
Set the following Chatbox settings:
Setting | Value |
---|---|
AI Provider | OpenAI API |
OpenAI API Key | API key generated during deployment |
API Host | Google Cloud Run URL |
The VSCode-OpenAI extension is a powerful and versatile tool designed to integrate OpenAI features seamlessly into your code editor.
To activate the setup, you have two options:
- either use the command "vscode-openai.configuration.show.quickpick" or
- access it through the vscode-openai Status Bar located at the bottom left corner of VSCode.
Select openai.com
and enter the Google Cloud Run URL with /v1
during setup.
When deploying the Discord Bot application, the following environment variables must be set:
Variable | Value |
---|---|
OPENAI_API_KEY | API key generated during deployment |
OPENAI_API_BASE | Google Cloud Run URL with /v1
|
When deploying the ChatGPT in Slack application, the following environment variables must be set:
Variable | Value |
---|---|
OPENAI_API_KEY | API key generated during deployment |
OPENAI_API_BASE | Google Cloud Run URL with /v1
|
When deploying the ChatGPT Telegram Bot application, the following environment variables must be set:
Variable | Value |
---|---|
OPENAI_API_KEY | API key generated during deployment |
OPENAI_API_BASE | Google Cloud Run URL with /v1
|
Have a patch that will benefit this project? Awesome! Follow these steps to have it accepted.
- Please read how to contribute.
- Fork this Git repository and make your changes.
- Create a Pull Request.
- Incorporate review feedback to your changes.
- Accepted!
All files in this repository are under the Apache License, Version 2.0 unless noted otherwise.
For Tasks:
Click tags to check more tools for each tasksFor Jobs:
Alternative AI tools for google-cloud-gcp-openai-api
Similar Open Source Tools
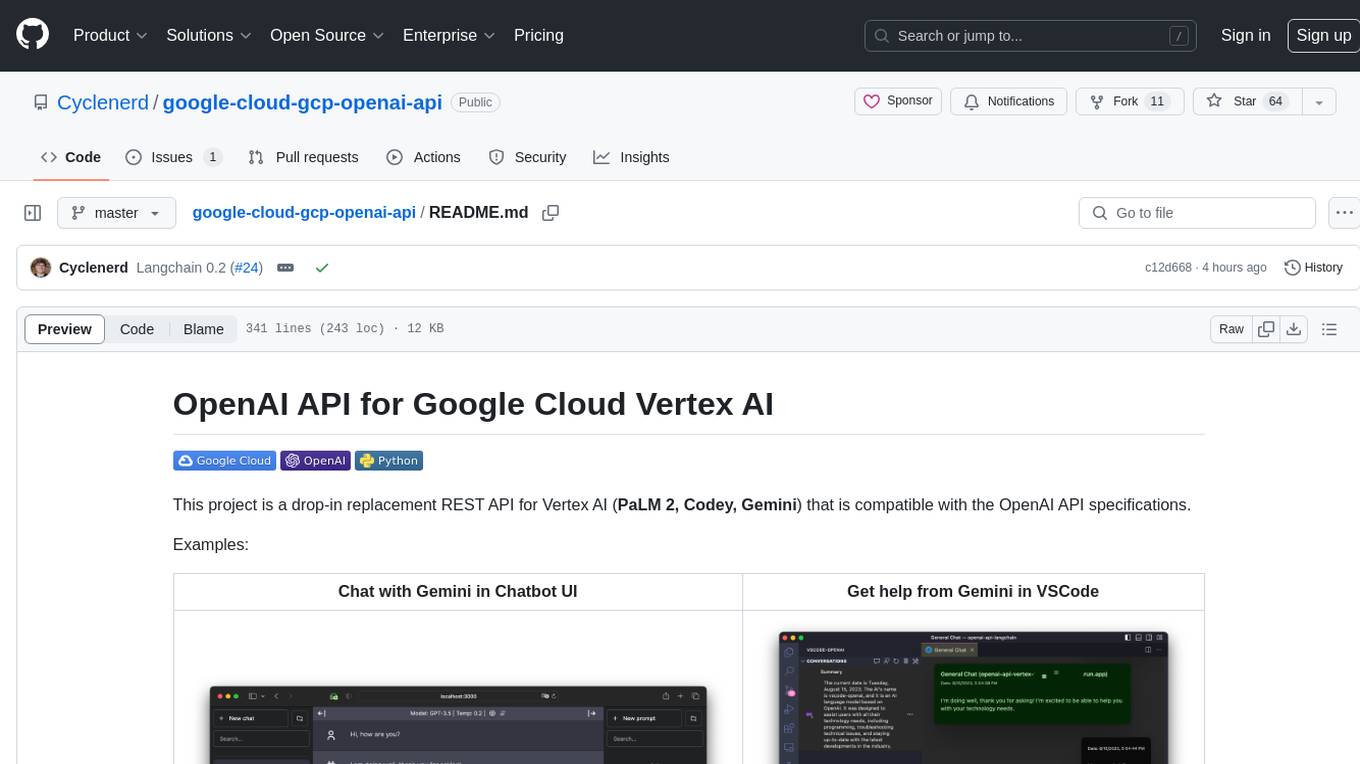
google-cloud-gcp-openai-api
This project provides a drop-in replacement REST API for Google Cloud Vertex AI (PaLM 2, Codey, Gemini) that is compatible with the OpenAI API specifications. It aims to make Google Cloud Platform Vertex AI more accessible by translating OpenAI API calls to Vertex AI. The software is developed in Python and based on FastAPI and LangChain, designed to be simple and customizable for individual needs. It includes step-by-step guides for deployment, supports various OpenAI API services, and offers configuration through environment variables. Additionally, it provides examples for running locally and usage instructions consistent with the OpenAI API format.
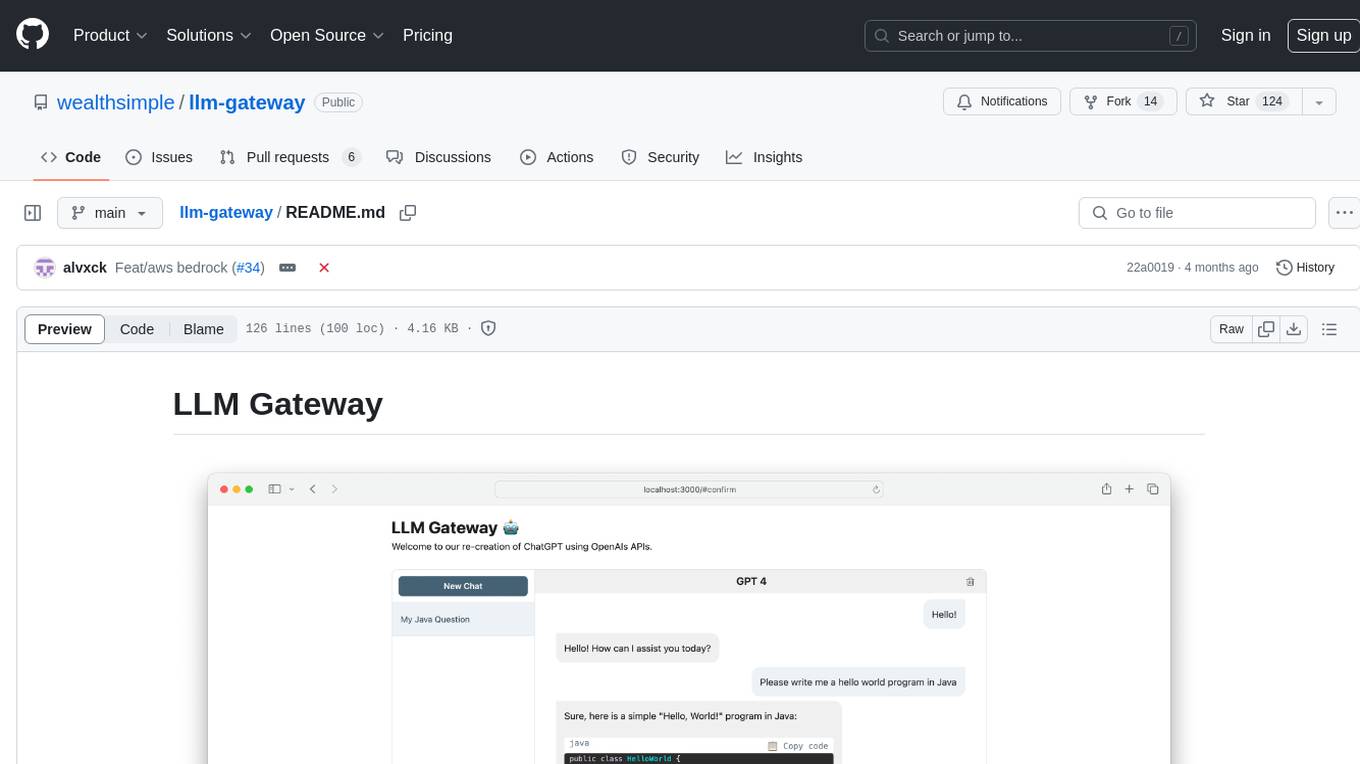
llm-gateway
llm-gateway is a gateway tool designed for interacting with third-party LLM providers such as OpenAI, Cohere, etc. It tracks data exchanged with these providers in a postgres database, applies PII scrubbing heuristics, and ensures safe communication with OpenAI's services. The tool supports various models from different providers and offers API and Python usage examples. Developers can set up the tool using Poetry, Pyenv, npm, and yarn for dependency management. The project also includes Docker setup for backend and frontend development.
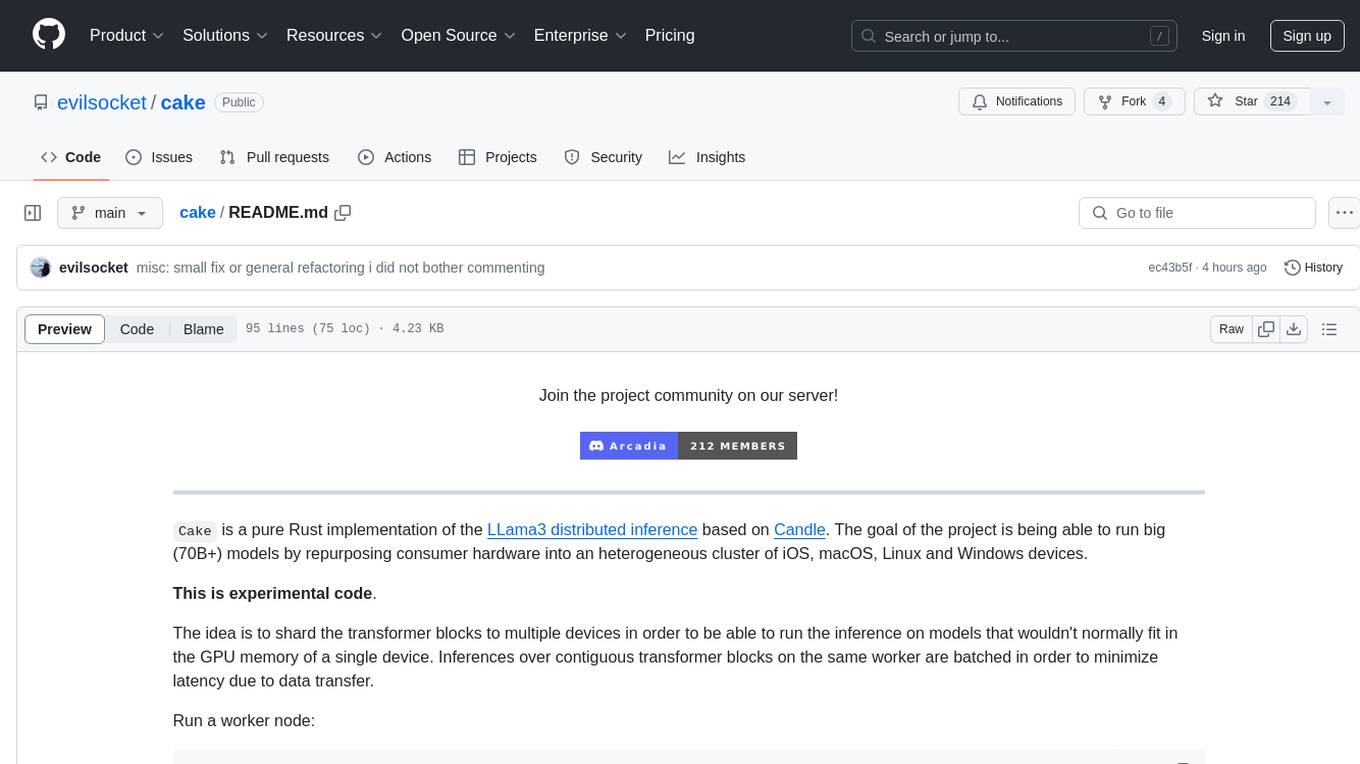
cake
cake is a pure Rust implementation of the llama3 LLM distributed inference based on Candle. The project aims to enable running large models on consumer hardware clusters of iOS, macOS, Linux, and Windows devices by sharding transformer blocks. It allows running inferences on models that wouldn't fit in a single device's GPU memory by batching contiguous transformer blocks on the same worker to minimize latency. The tool provides a way to optimize memory and disk space by splitting the model into smaller bundles for workers, ensuring they only have the necessary data. cake supports various OS, architectures, and accelerations, with different statuses for each configuration.
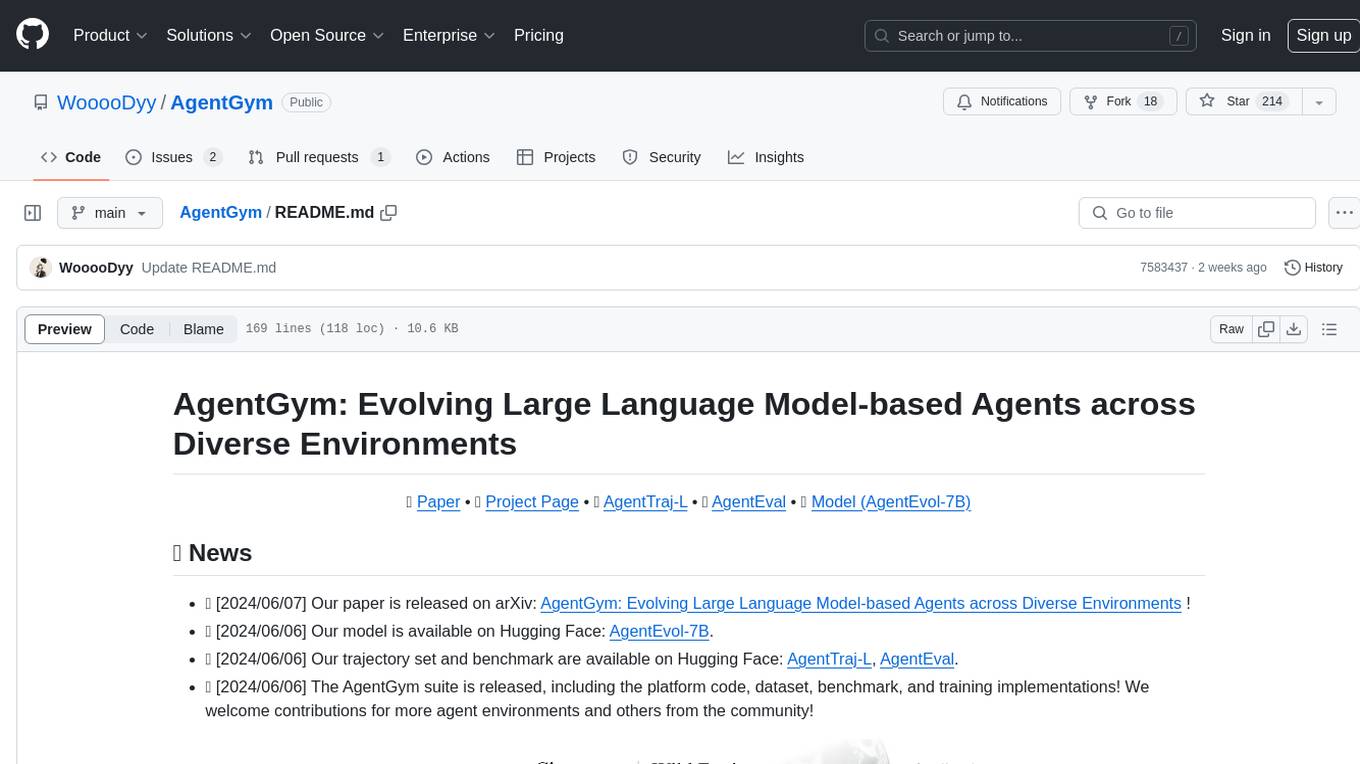
AgentGym
AgentGym is a framework designed to help the AI community evaluate and develop generally-capable Large Language Model-based agents. It features diverse interactive environments and tasks with real-time feedback and concurrency. The platform supports 14 environments across various domains like web navigating, text games, house-holding tasks, digital games, and more. AgentGym includes a trajectory set (AgentTraj) and a benchmark suite (AgentEval) to facilitate agent exploration and evaluation. The framework allows for agent self-evolution beyond existing data, showcasing comparable results to state-of-the-art models.
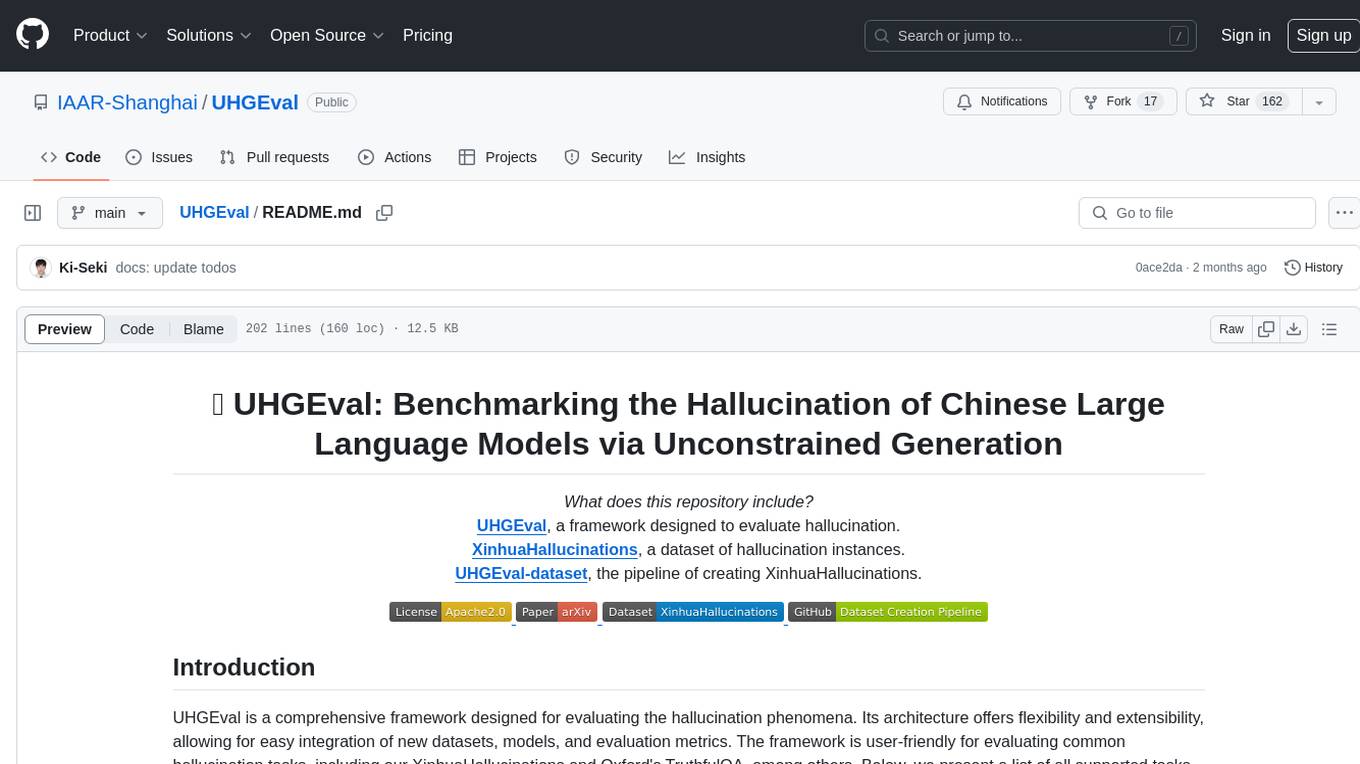
UHGEval
UHGEval is a comprehensive framework designed for evaluating the hallucination phenomena. It includes UHGEval, a framework for evaluating hallucination, XinhuaHallucinations dataset, and UHGEval-dataset pipeline for creating XinhuaHallucinations. The framework offers flexibility and extensibility for evaluating common hallucination tasks, supporting various models and datasets. Researchers can use the open-source pipeline to create customized datasets. Supported tasks include QA, dialogue, summarization, and multi-choice tasks.
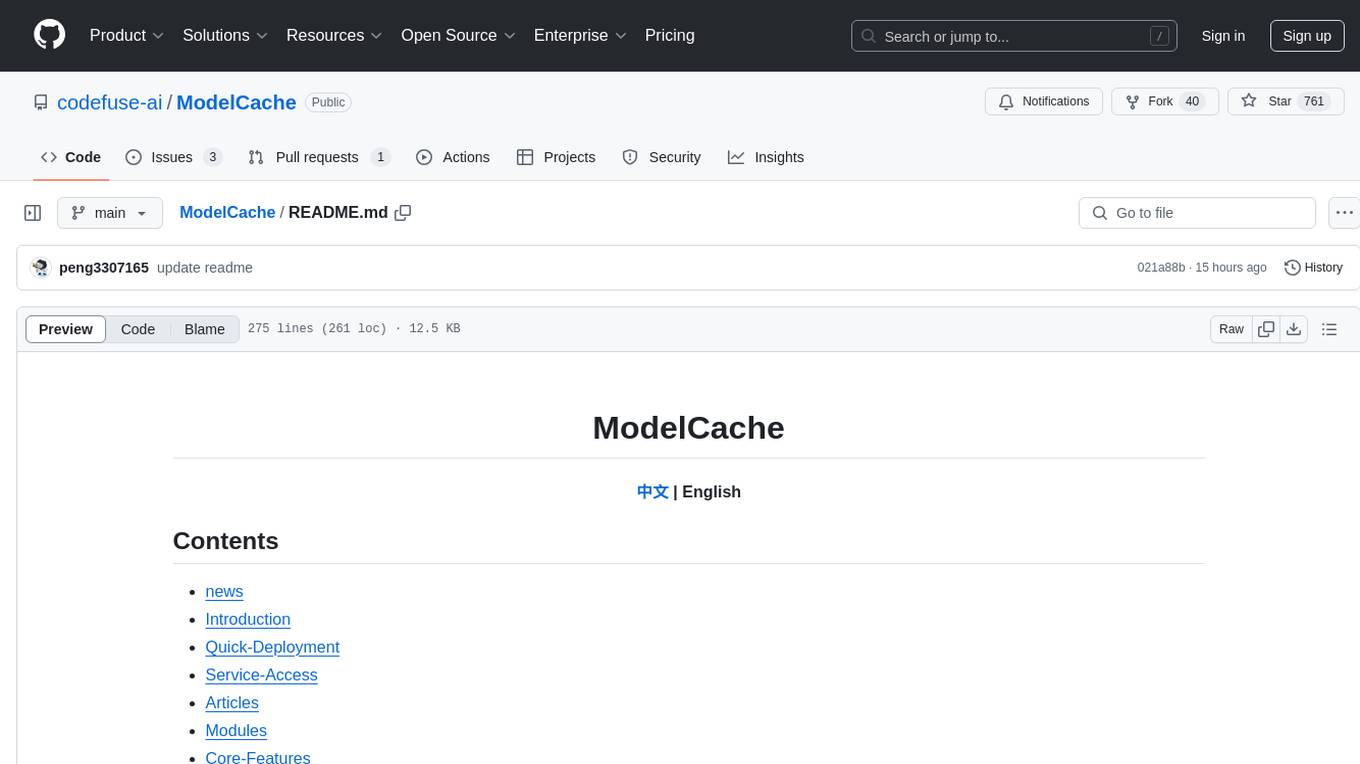
ModelCache
Codefuse-ModelCache is a semantic cache for large language models (LLMs) that aims to optimize services by introducing a caching mechanism. It helps reduce the cost of inference deployment, improve model performance and efficiency, and provide scalable services for large models. The project facilitates sharing and exchanging technologies related to large model semantic cache through open-source collaboration.
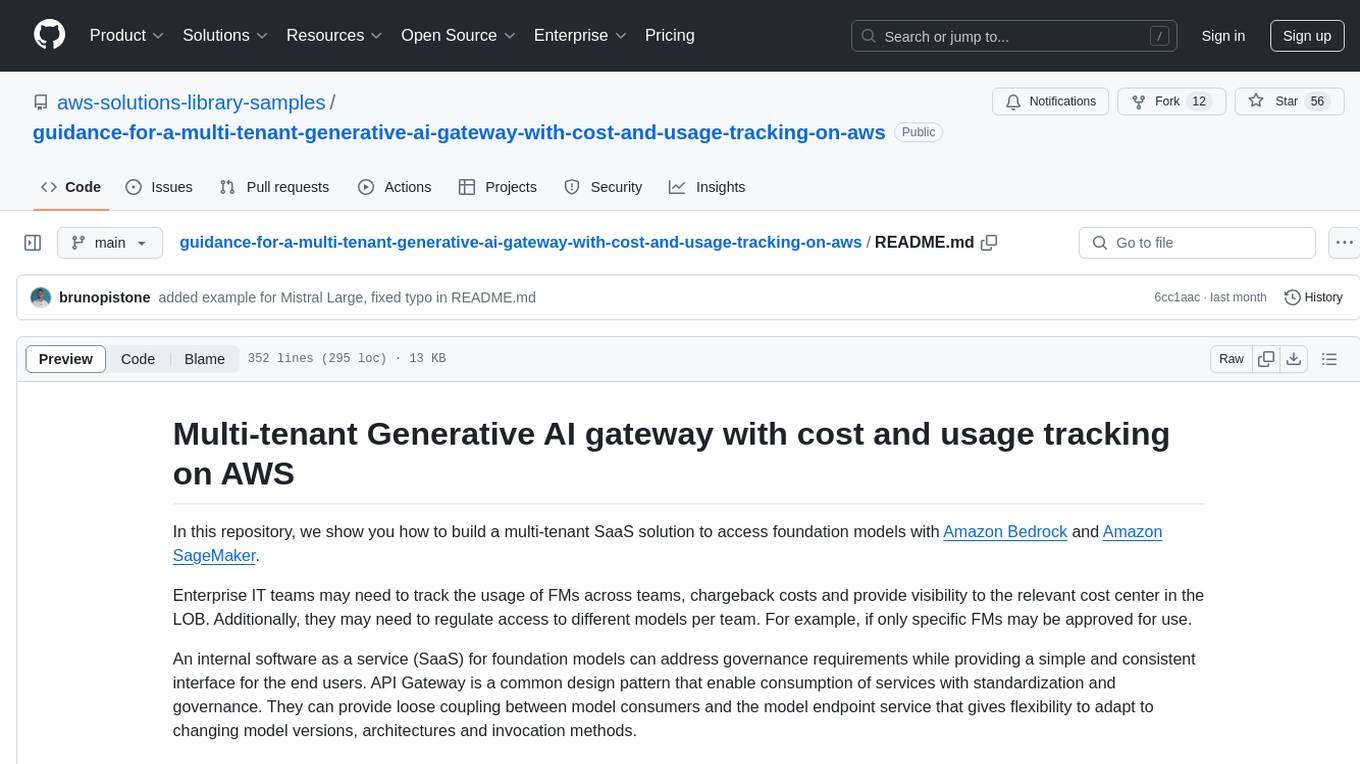
guidance-for-a-multi-tenant-generative-ai-gateway-with-cost-and-usage-tracking-on-aws
This repository provides guidance on building a multi-tenant SaaS solution for accessing foundation models using Amazon Bedrock and Amazon SageMaker. It helps enterprise IT teams track usage and costs of foundation models, regulate access, and provide visibility to cost centers. The solution includes an API Gateway design pattern for standardization and governance, enabling loose coupling between model consumers and endpoint services. The CDK Stack deploys resources for private networking, API Gateway, Lambda functions, DynamoDB table, EventBridge, S3 buckets, and Cloudwatch logs.
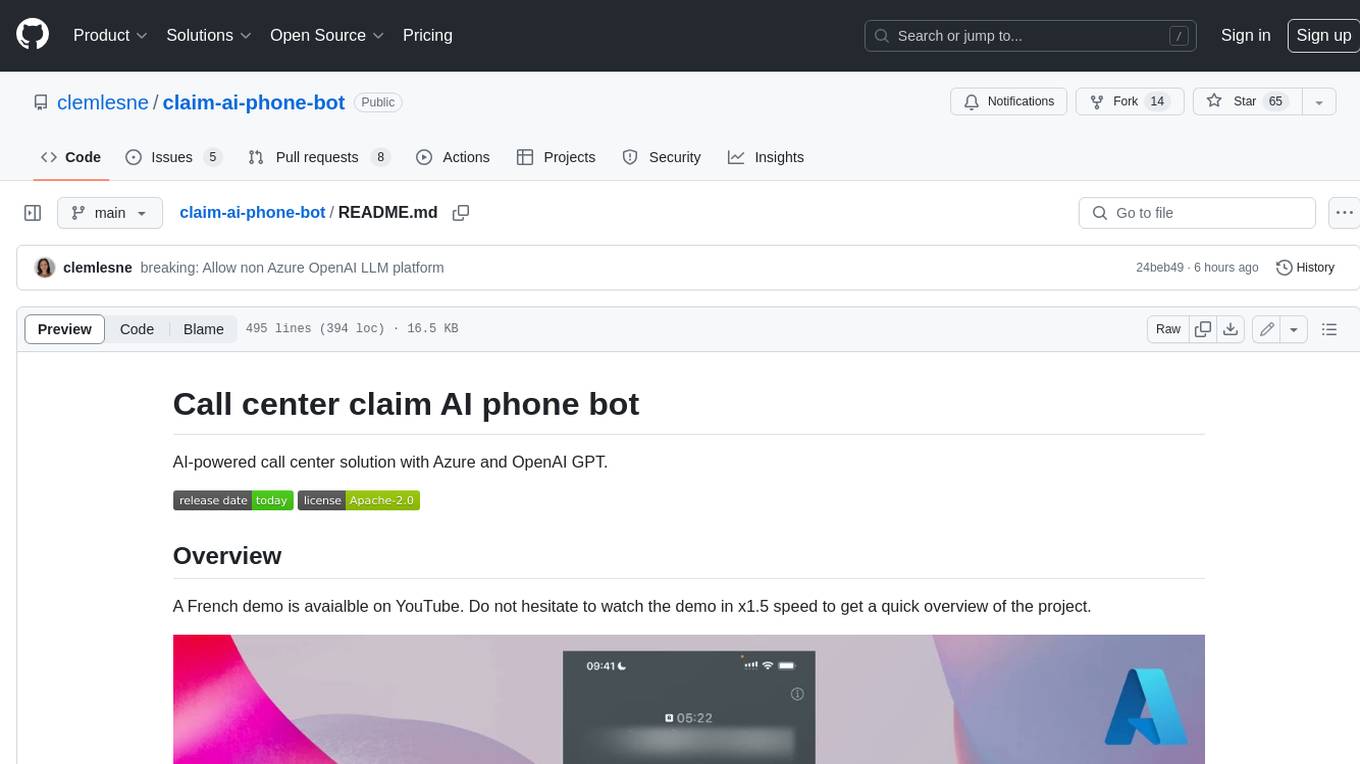
claim-ai-phone-bot
AI-powered call center solution with Azure and OpenAI GPT. The bot can answer calls, understand the customer's request, and provide relevant information or assistance. It can also create a todo list of tasks to complete the claim, and send a report after the call. The bot is customizable, and can be used in multiple languages.
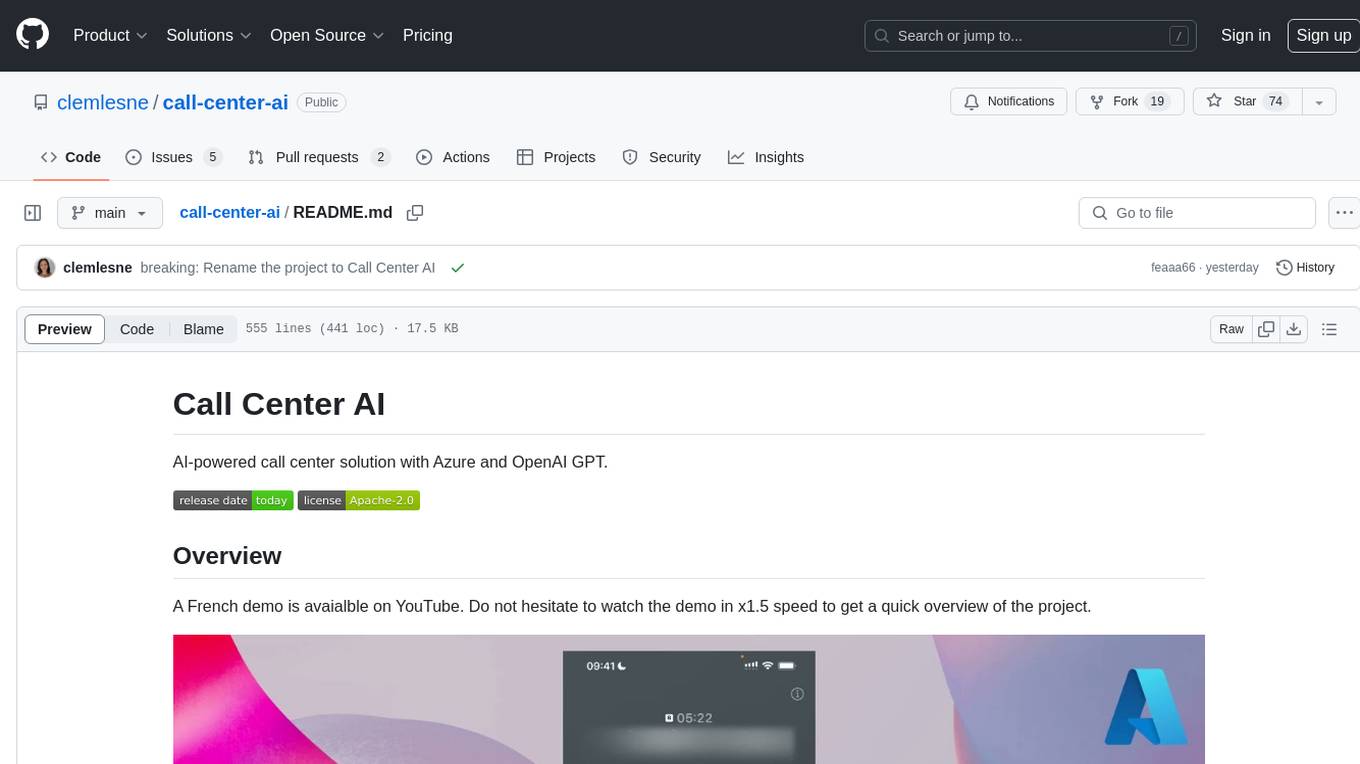
call-center-ai
Call Center AI is an AI-powered call center solution that leverages Azure and OpenAI GPT. It is a proof of concept demonstrating the integration of Azure Communication Services, Azure Cognitive Services, and Azure OpenAI to build an automated call center solution. The project showcases features like accessing claims on a public website, customer conversation history, language change during conversation, bot interaction via phone number, multiple voice tones, lexicon understanding, todo list creation, customizable prompts, content filtering, GPT-4 Turbo for customer requests, specific data schema for claims, documentation database access, SMS report sending, conversation resumption, and more. The system architecture includes components like RAG AI Search, SMS gateway, call gateway, moderation, Cosmos DB, event broker, GPT-4 Turbo, Redis cache, translation service, and more. The tool can be deployed remotely using GitHub Actions and locally with prerequisites like Azure environment setup, configuration file creation, and resource hosting. Advanced usage includes custom training data with AI Search, prompt customization, language customization, moderation level customization, claim data schema customization, OpenAI compatible model usage for the LLM, and Twilio integration for SMS.
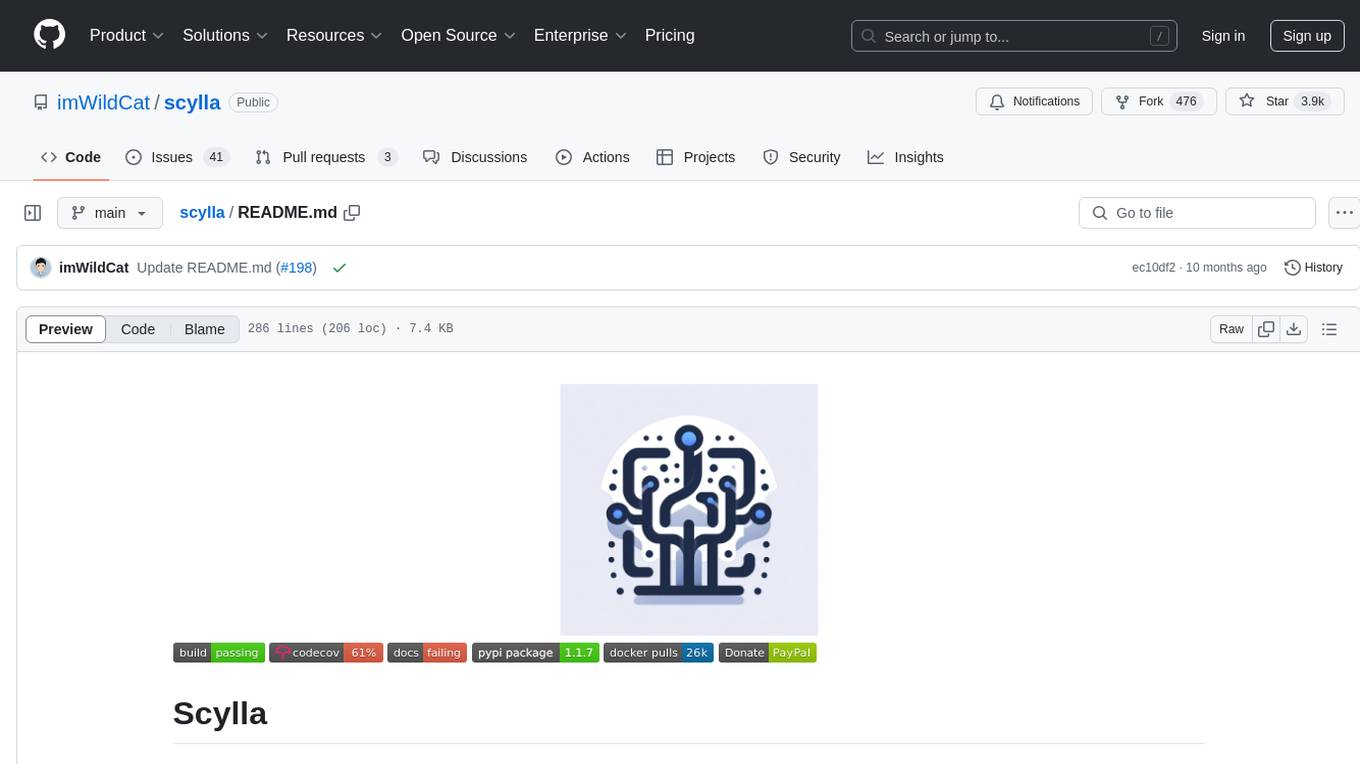
scylla
Scylla is an intelligent proxy pool tool designed for humanities, enabling users to extract content from the internet and build their own Large Language Models in the AI era. It features automatic proxy IP crawling and validation, an easy-to-use JSON API, a simple web-based user interface, HTTP forward proxy server, Scrapy and requests integration, and headless browser crawling. Users can start using Scylla with just one command, making it a versatile tool for various web scraping and content extraction tasks.
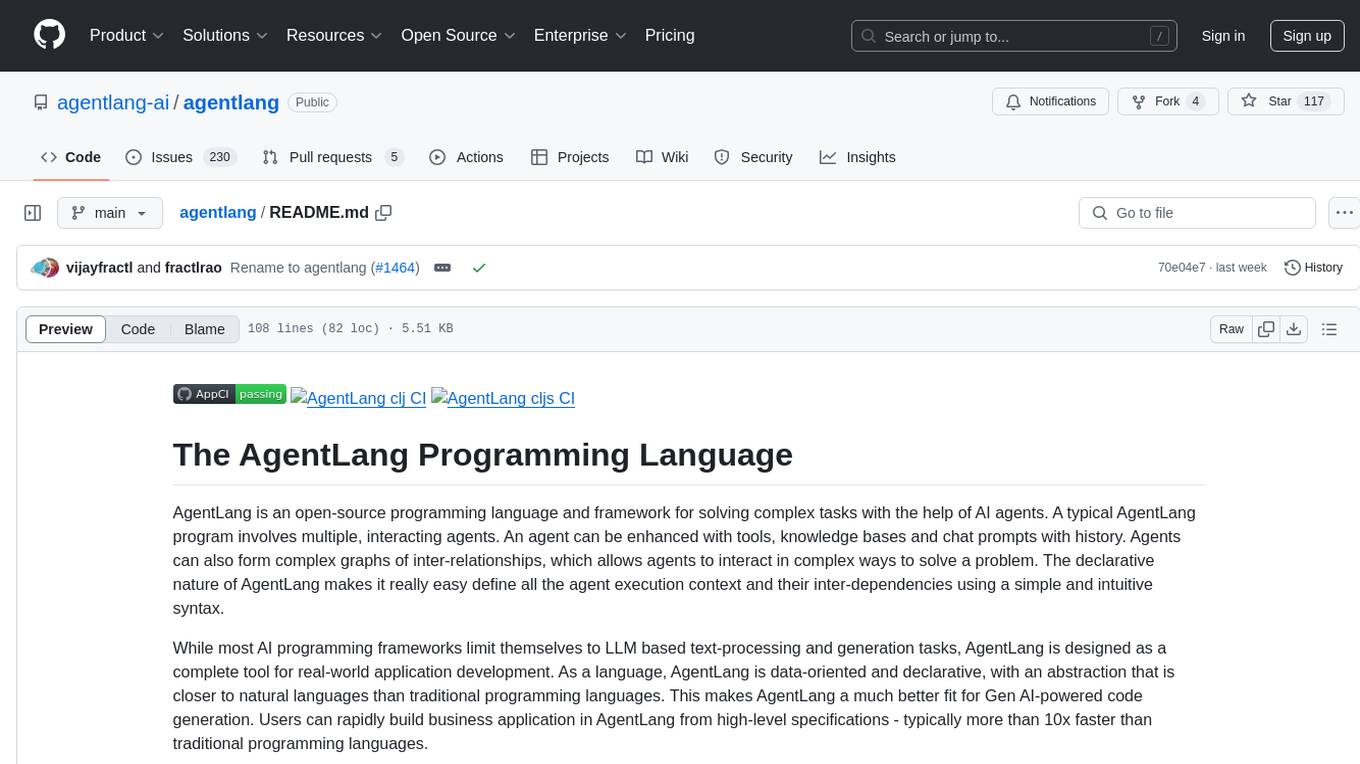
agentlang
AgentLang is an open-source programming language and framework designed for solving complex tasks with the help of AI agents. It allows users to build business applications rapidly from high-level specifications, making it more efficient than traditional programming languages. The language is data-oriented and declarative, with a syntax that is intuitive and closer to natural languages. AgentLang introduces innovative concepts such as first-class AI agents, graph-based hierarchical data model, zero-trust programming, declarative dataflow, resolvers, interceptors, and entity-graph-database mapping.
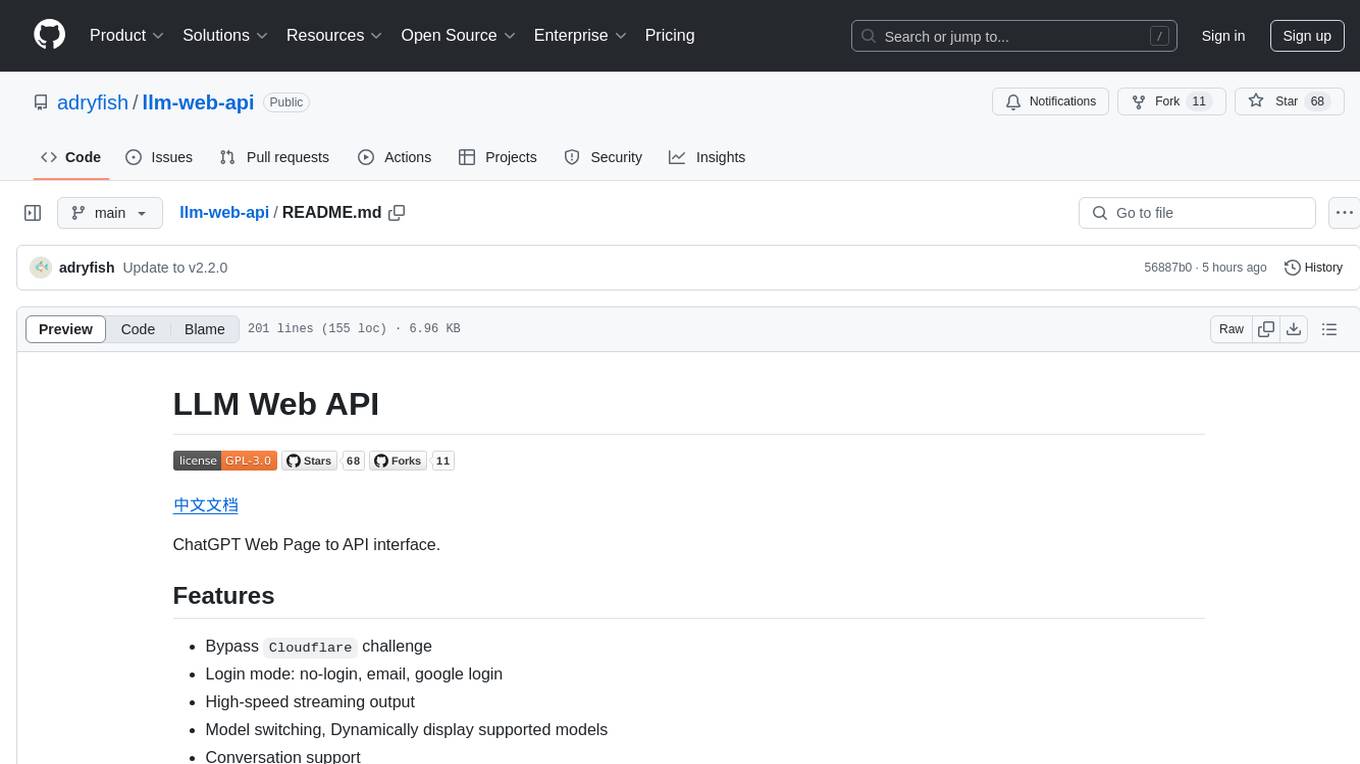
llm-web-api
LLM Web API is a tool that provides a web page to API interface for ChatGPT, allowing users to bypass Cloudflare challenges, switch models, and dynamically display supported models. It uses Playwright to control a fingerprint browser, simulating user operations to send requests to the OpenAI website and converting the responses into API interfaces. The API currently supports the OpenAI-compatible /v1/chat/completions API, accessible using OpenAI or other compatible clients.
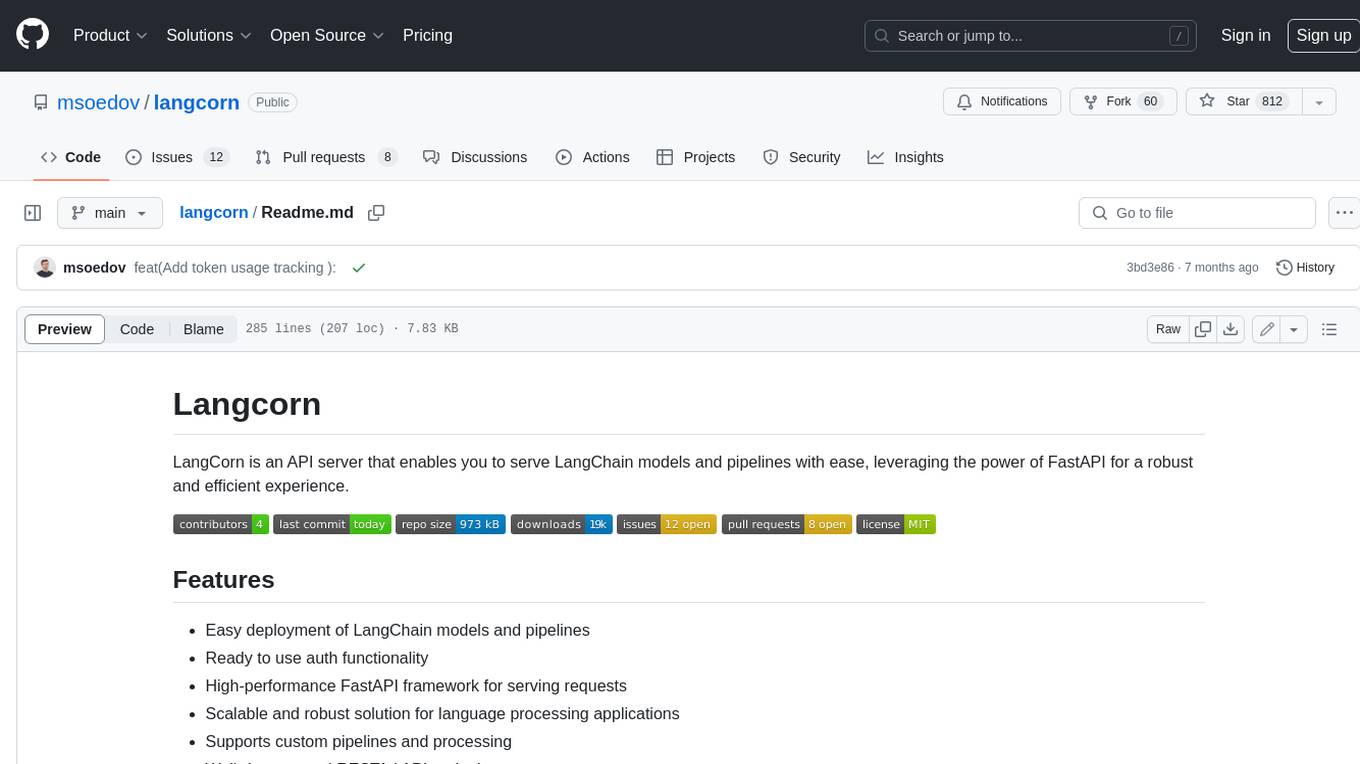
langcorn
LangCorn is an API server that enables you to serve LangChain models and pipelines with ease, leveraging the power of FastAPI for a robust and efficient experience. It offers features such as easy deployment of LangChain models and pipelines, ready-to-use authentication functionality, high-performance FastAPI framework for serving requests, scalability and robustness for language processing applications, support for custom pipelines and processing, well-documented RESTful API endpoints, and asynchronous processing for faster response times.
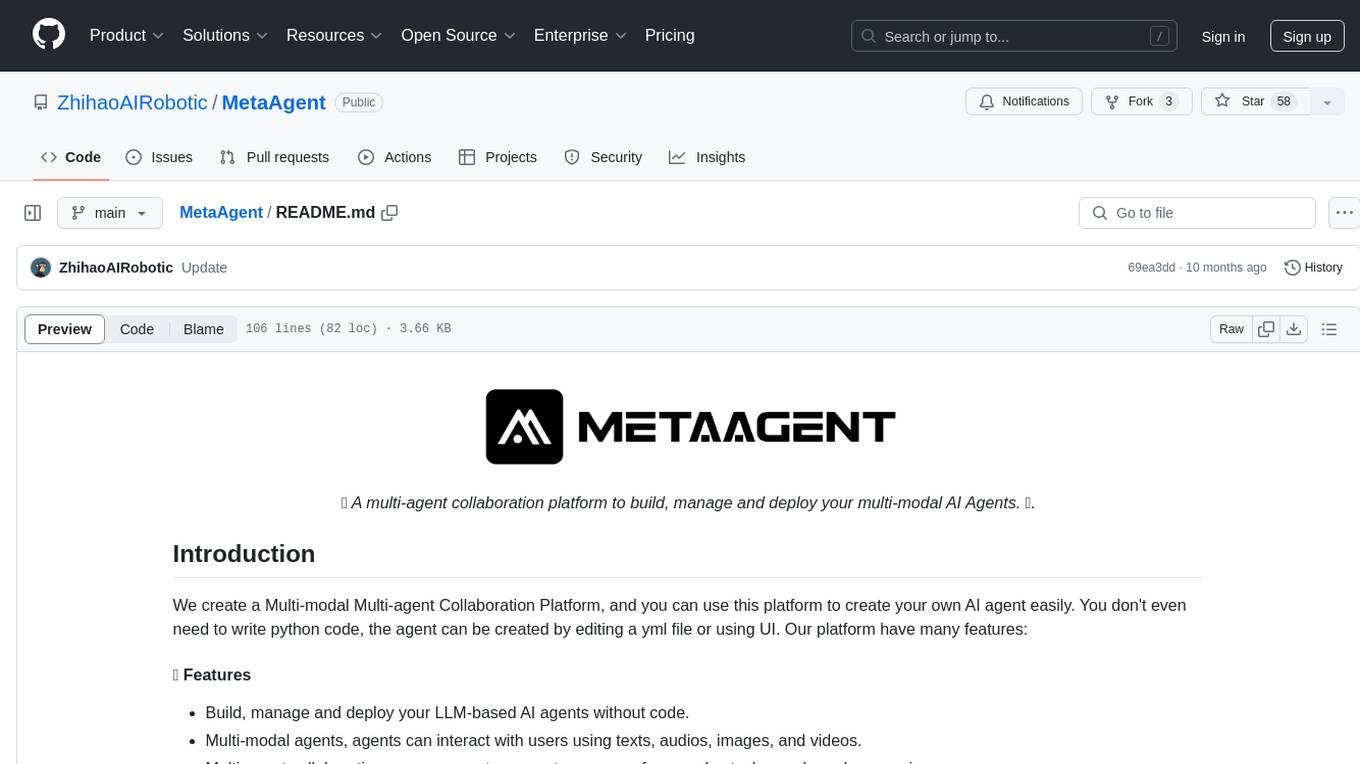
MetaAgent
MetaAgent is a multi-agent collaboration platform designed to build, manage, and deploy multi-modal AI agents without the need for coding. Users can easily create AI agents by editing a yml file or using the provided UI. The platform supports features such as building LLM-based AI agents, multi-modal interactions with users using texts, audios, images, and videos, creating a company of agents for complex tasks like drawing comics, vector database and knowledge embeddings, and upcoming features like UI for creating and using AI agents, fine-tuning, and RLHF. The tool simplifies the process of creating and deploying AI agents for various tasks.
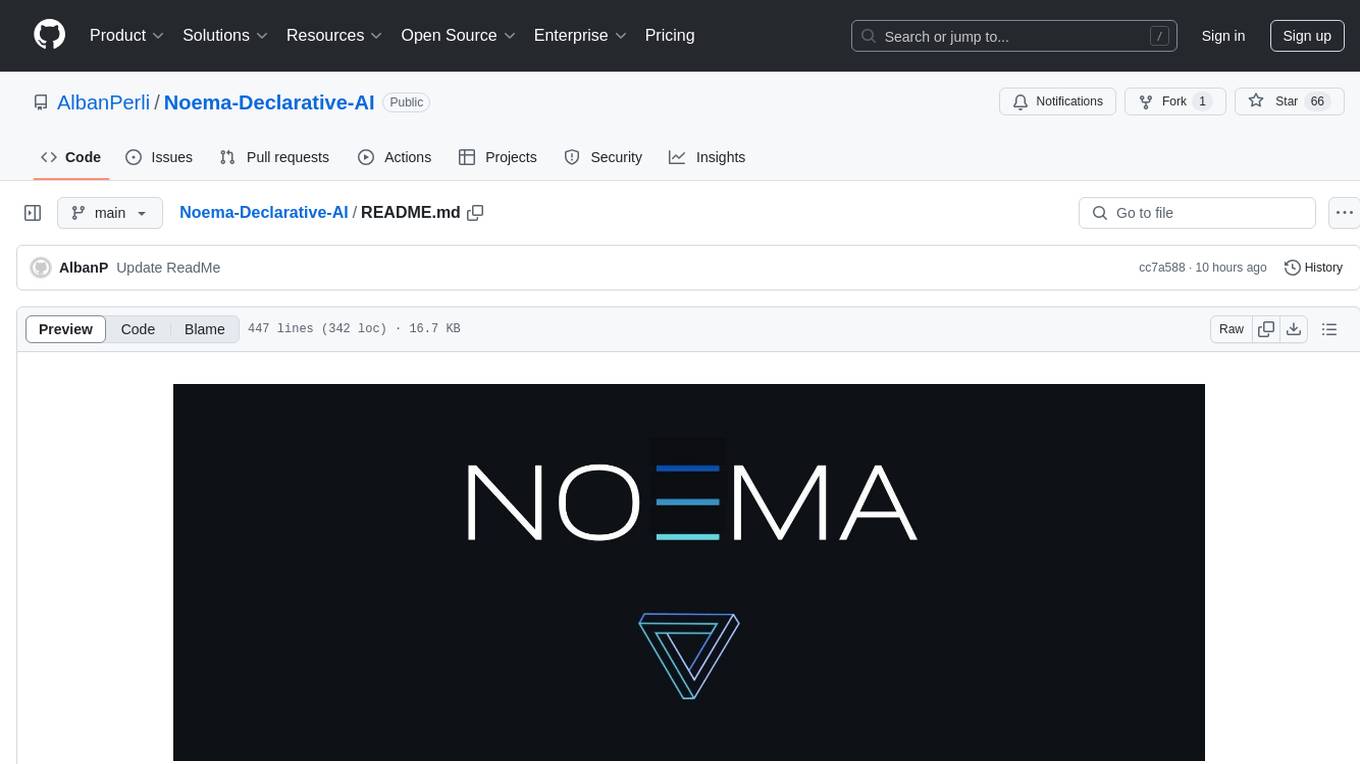
Noema-Declarative-AI
Noema is a framework that enables developers to control a language model and choose the path it will follow. It integrates Python with llm's generations, allowing users to use LLM as a thought interpreter rather than a source of truth. Noema is built on llama.cpp and guidance's shoulders. It applies the declarative programming paradigm to a language model, providing a way to represent functions, descriptions, and transformations. Users can create subjects, think about tasks, and generate content through generators, selectors, and code generators. Noema supports ReAct prompting, visualization, and semantic Python functionalities, offering a versatile tool for automating tasks and guiding language models.
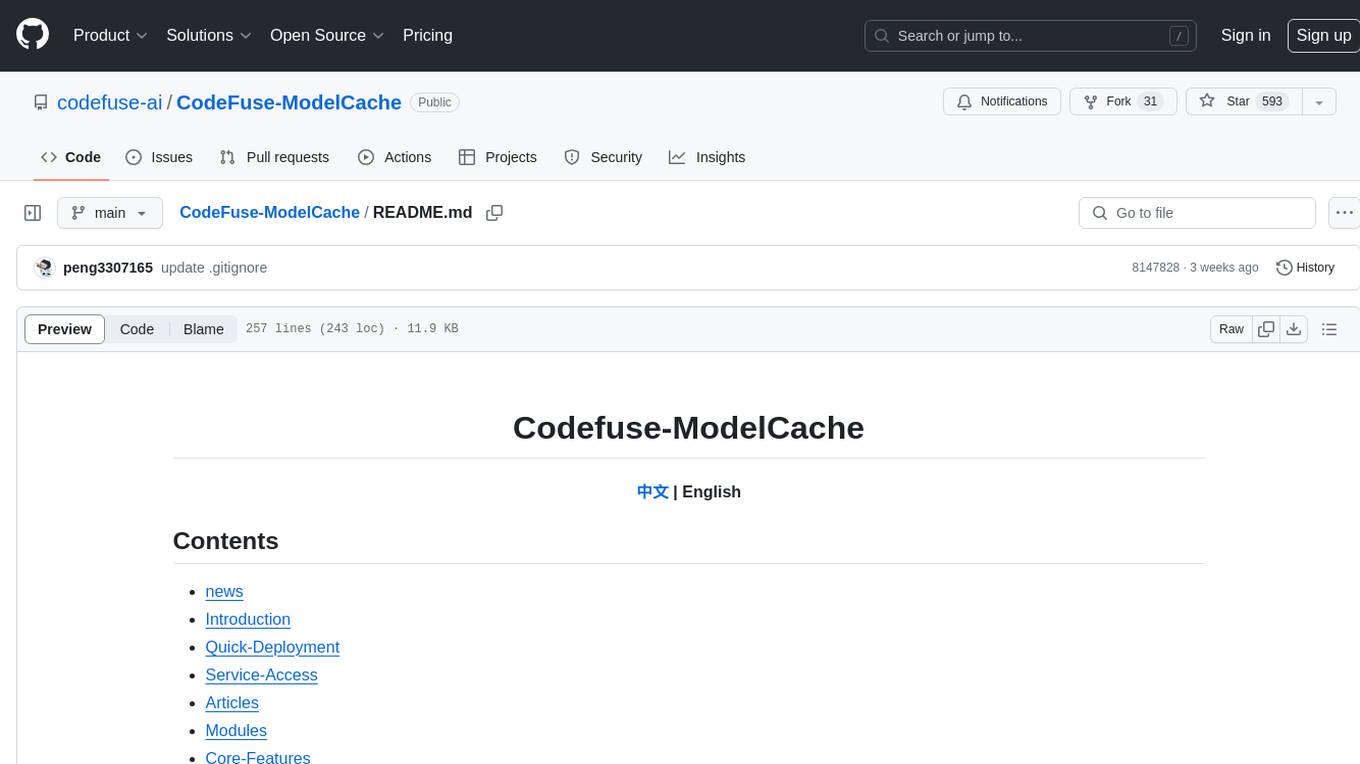
CodeFuse-ModelCache
Codefuse-ModelCache is a semantic cache for large language models (LLMs) that aims to optimize services by introducing a caching mechanism. It helps reduce the cost of inference deployment, improve model performance and efficiency, and provide scalable services for large models. The project caches pre-generated model results to reduce response time for similar requests and enhance user experience. It integrates various embedding frameworks and local storage options, offering functionalities like cache-writing, cache-querying, and cache-clearing through RESTful API. The tool supports multi-tenancy, system commands, and multi-turn dialogue, with features for data isolation, database management, and model loading schemes. Future developments include data isolation based on hyperparameters, enhanced system prompt partitioning storage, and more versatile embedding models and similarity evaluation algorithms.
For similar tasks
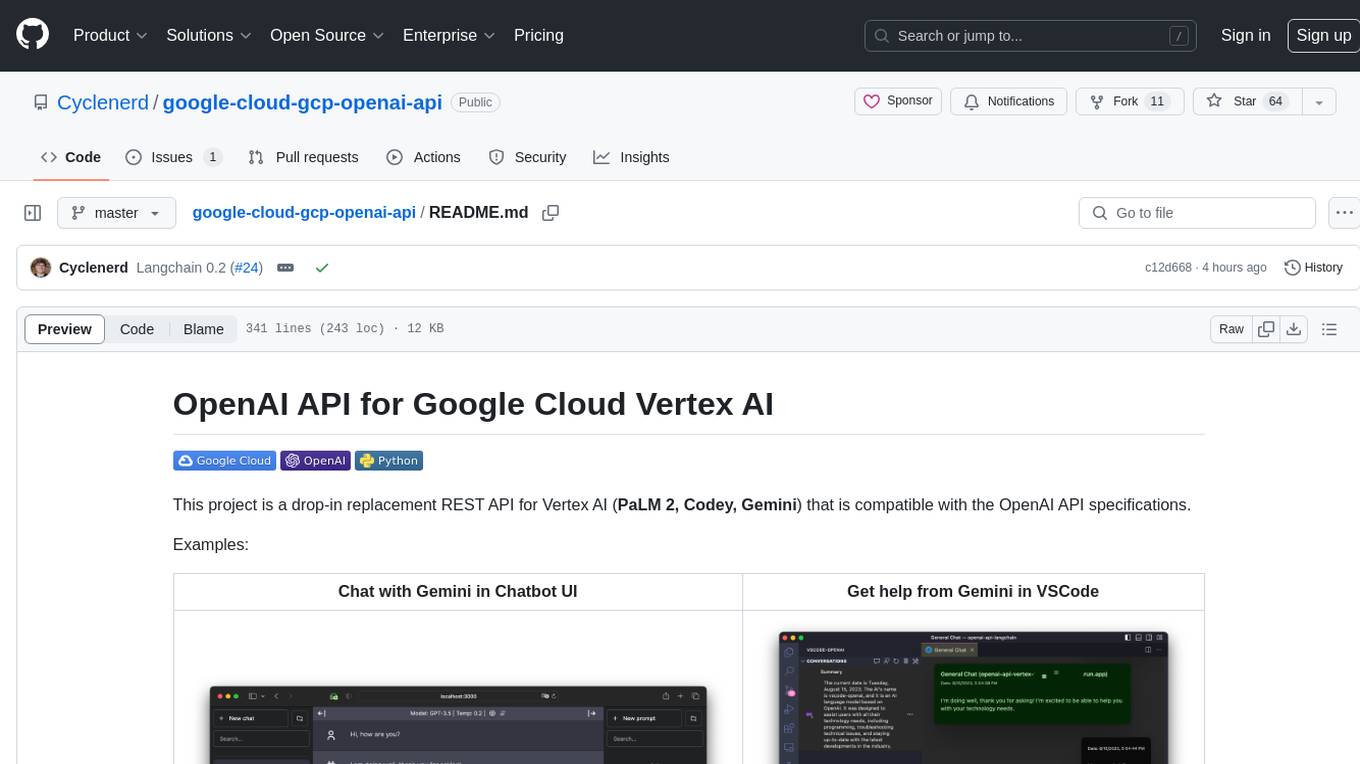
google-cloud-gcp-openai-api
This project provides a drop-in replacement REST API for Google Cloud Vertex AI (PaLM 2, Codey, Gemini) that is compatible with the OpenAI API specifications. It aims to make Google Cloud Platform Vertex AI more accessible by translating OpenAI API calls to Vertex AI. The software is developed in Python and based on FastAPI and LangChain, designed to be simple and customizable for individual needs. It includes step-by-step guides for deployment, supports various OpenAI API services, and offers configuration through environment variables. Additionally, it provides examples for running locally and usage instructions consistent with the OpenAI API format.
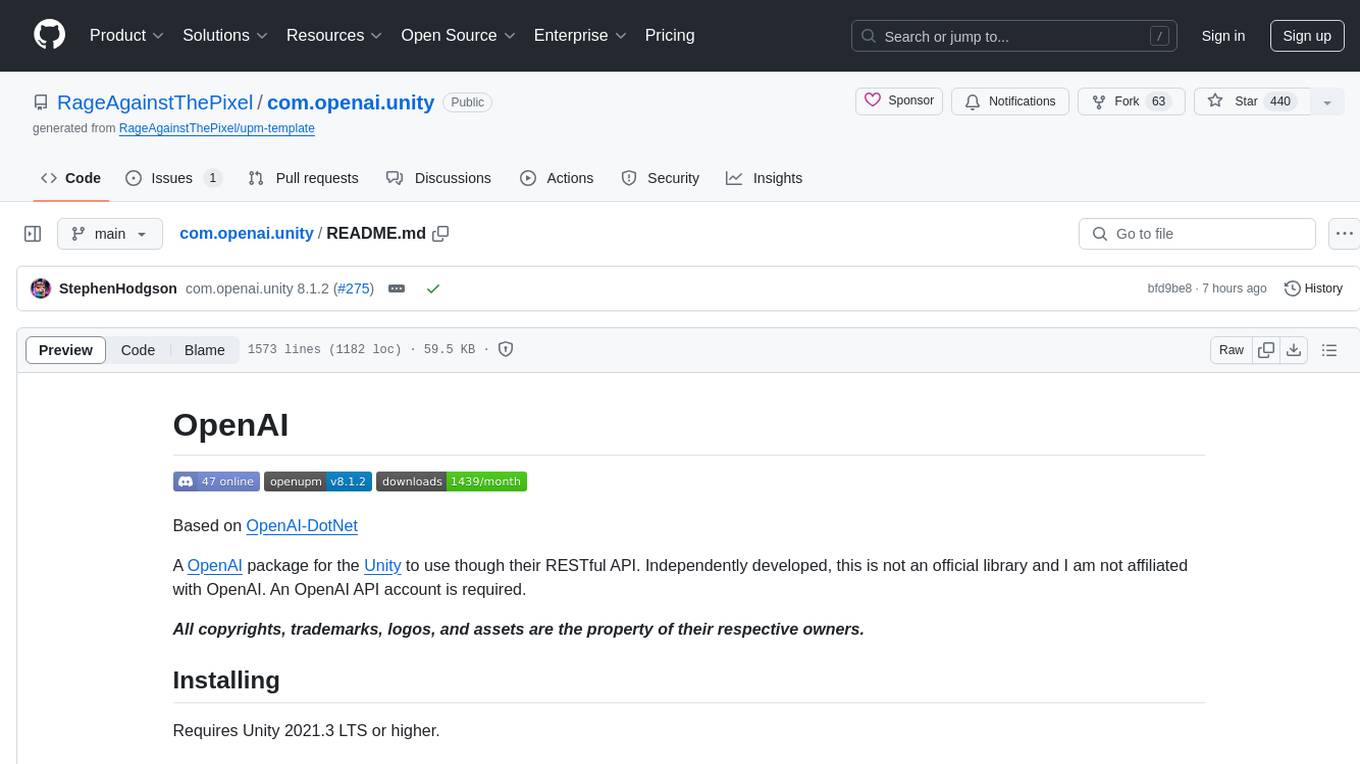
com.openai.unity
com.openai.unity is an OpenAI package for Unity that allows users to interact with OpenAI's API through RESTful requests. It is independently developed and not an official library affiliated with OpenAI. Users can fine-tune models, create assistants, chat completions, and more. The package requires Unity 2021.3 LTS or higher and can be installed via Unity Package Manager or Git URL. Various features like authentication, Azure OpenAI integration, model management, thread creation, chat completions, audio processing, image generation, file management, fine-tuning, batch processing, embeddings, and content moderation are available.
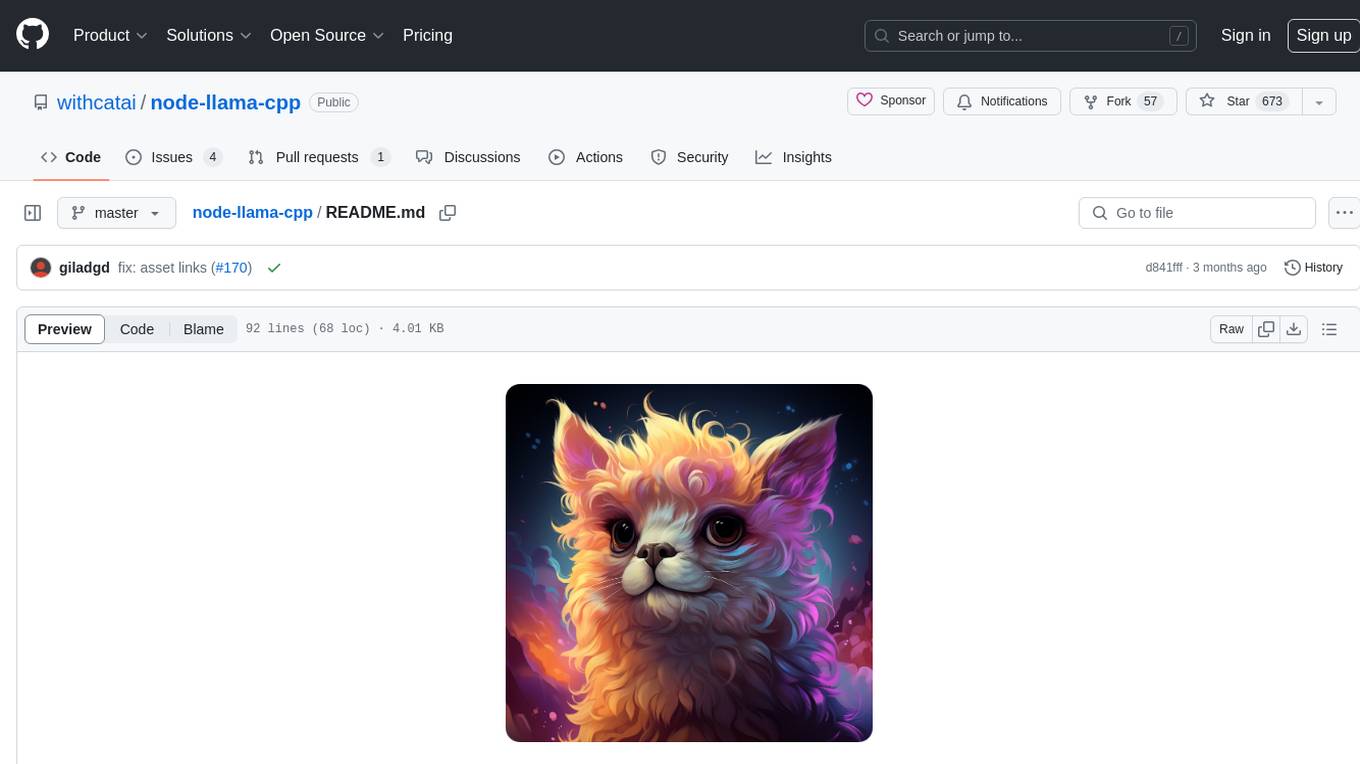
node-llama-cpp
node-llama-cpp is a tool that allows users to run AI models locally on their machines. It provides pre-built bindings with the option to build from source using cmake. Users can interact with text generation models, chat with models using a chat wrapper, and force models to generate output in a parseable format like JSON. The tool supports Metal and CUDA, offers CLI functionality for chatting with models without coding, and ensures up-to-date compatibility with the latest version of llama.cpp. Installation includes pre-built binaries for macOS, Linux, and Windows, with the option to build from source if binaries are not available for the platform.
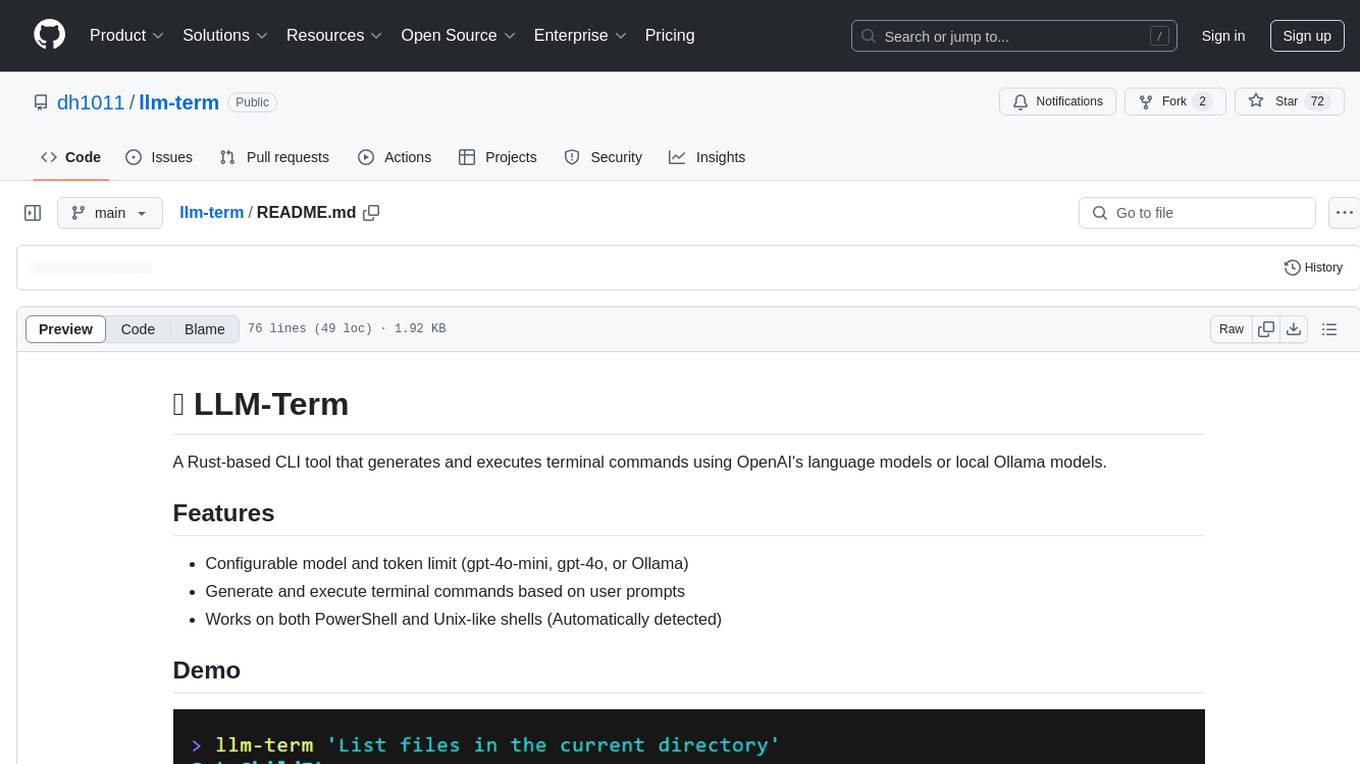
llm-term
LLM-Term is a Rust-based CLI tool that generates and executes terminal commands using OpenAI's language models or local Ollama models. It offers configurable model and token limits, works on both PowerShell and Unix-like shells, and provides a seamless user experience for generating commands based on prompts. Users can easily set up the tool, customize configurations, and leverage different models for command generation.
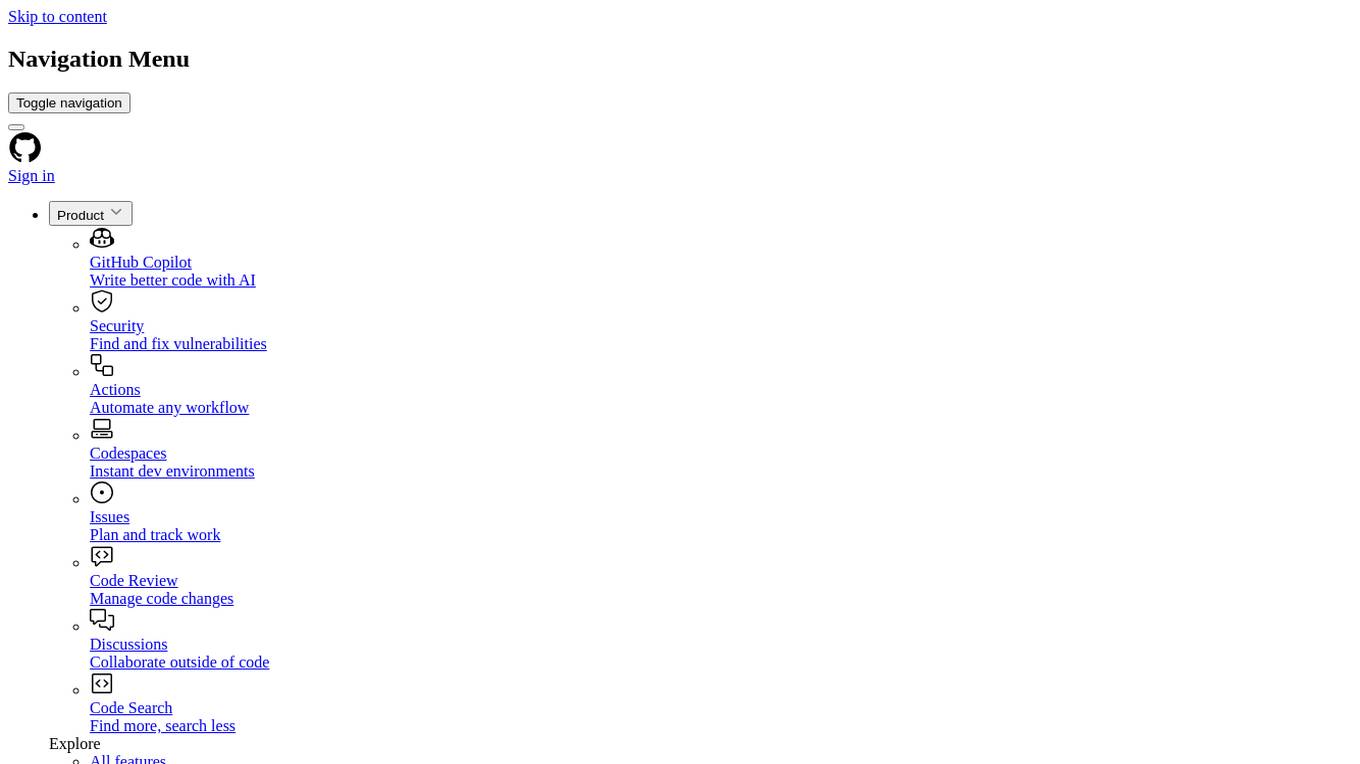
ai-analyst
AI Analyst by E2B is an AI-powered code and data analysis tool built with Next.js and the E2B SDK. It allows users to analyze data with Meta's Llama 3.1, upload CSV files, and create interactive charts. The tool is powered by E2B Sandbox, Vercel's AI SDK, Next.js, and echarts library for interactive charts. Supported LLM providers include TogetherAI and Fireworks, with various chart types available for visualization.
For similar jobs
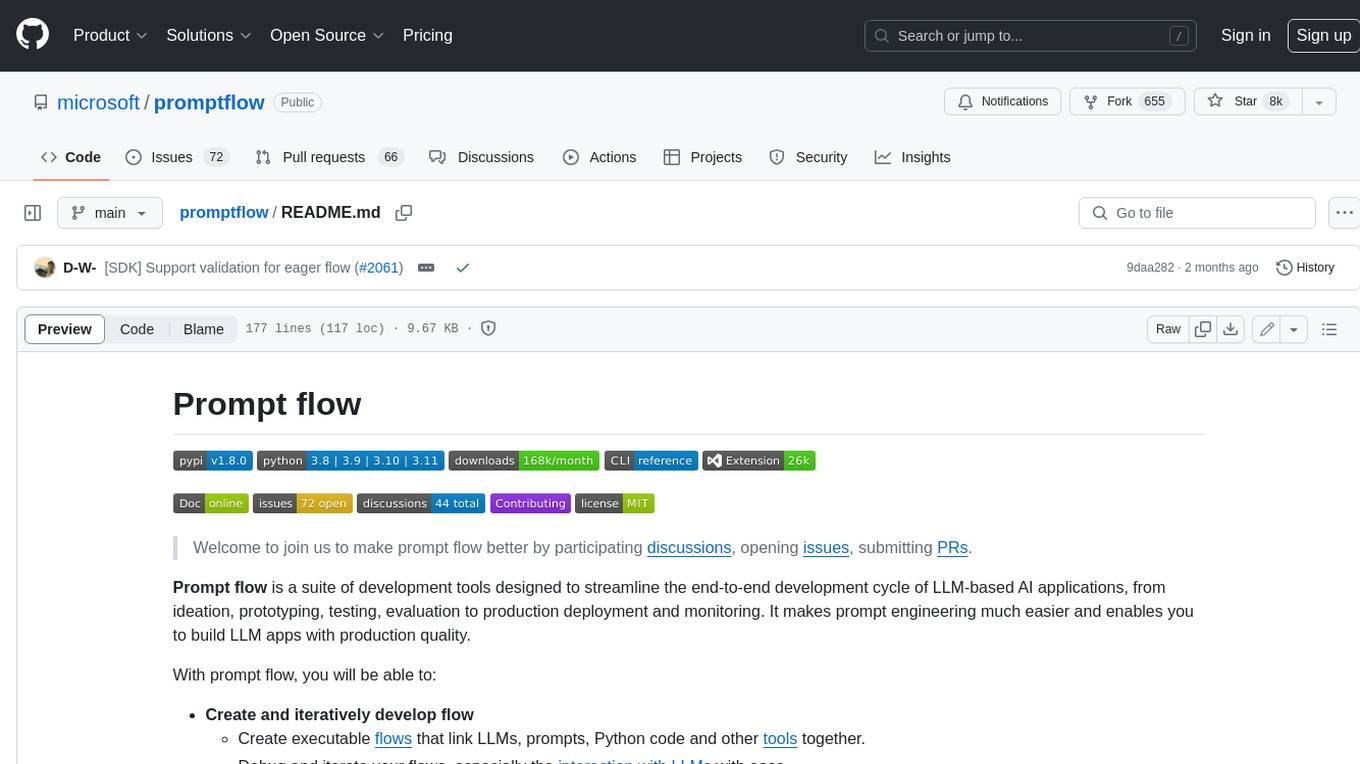
promptflow
**Prompt flow** is a suite of development tools designed to streamline the end-to-end development cycle of LLM-based AI applications, from ideation, prototyping, testing, evaluation to production deployment and monitoring. It makes prompt engineering much easier and enables you to build LLM apps with production quality.
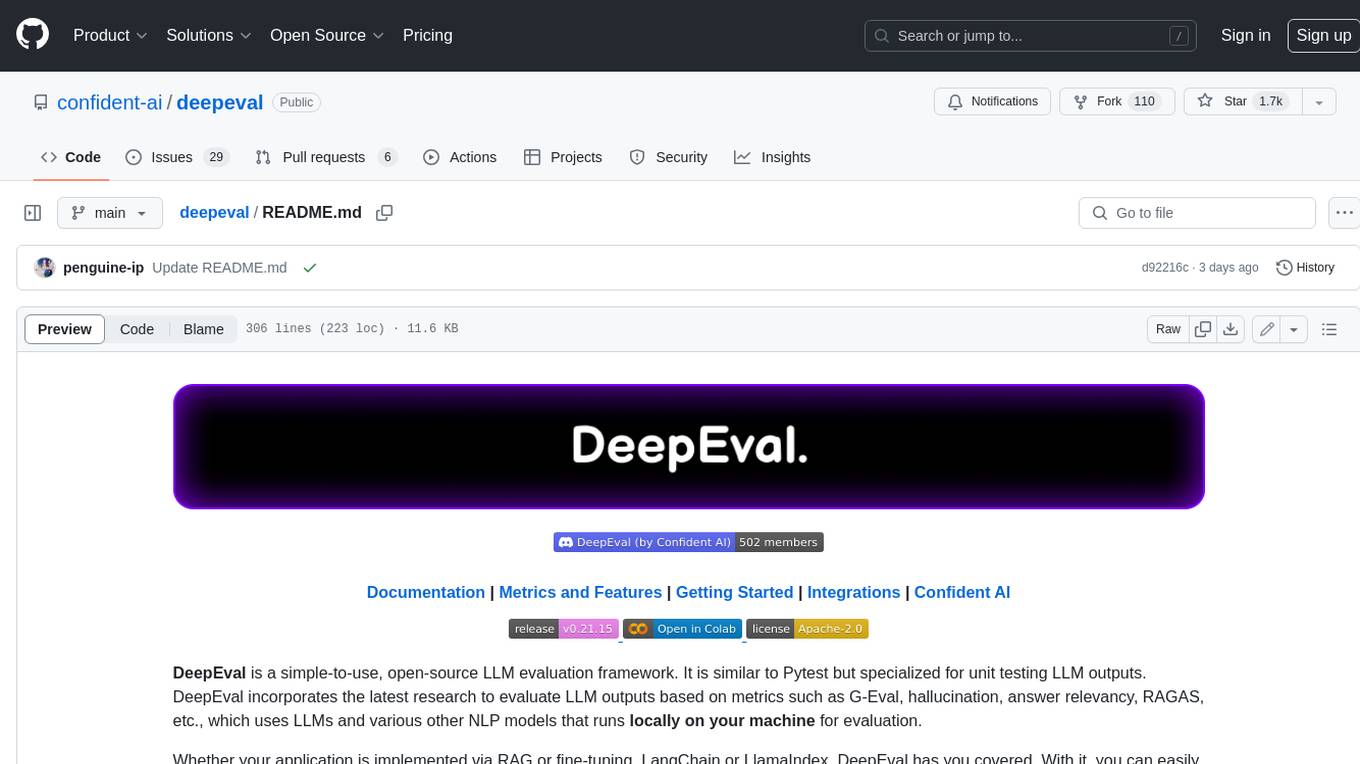
deepeval
DeepEval is a simple-to-use, open-source LLM evaluation framework specialized for unit testing LLM outputs. It incorporates various metrics such as G-Eval, hallucination, answer relevancy, RAGAS, etc., and runs locally on your machine for evaluation. It provides a wide range of ready-to-use evaluation metrics, allows for creating custom metrics, integrates with any CI/CD environment, and enables benchmarking LLMs on popular benchmarks. DeepEval is designed for evaluating RAG and fine-tuning applications, helping users optimize hyperparameters, prevent prompt drifting, and transition from OpenAI to hosting their own Llama2 with confidence.
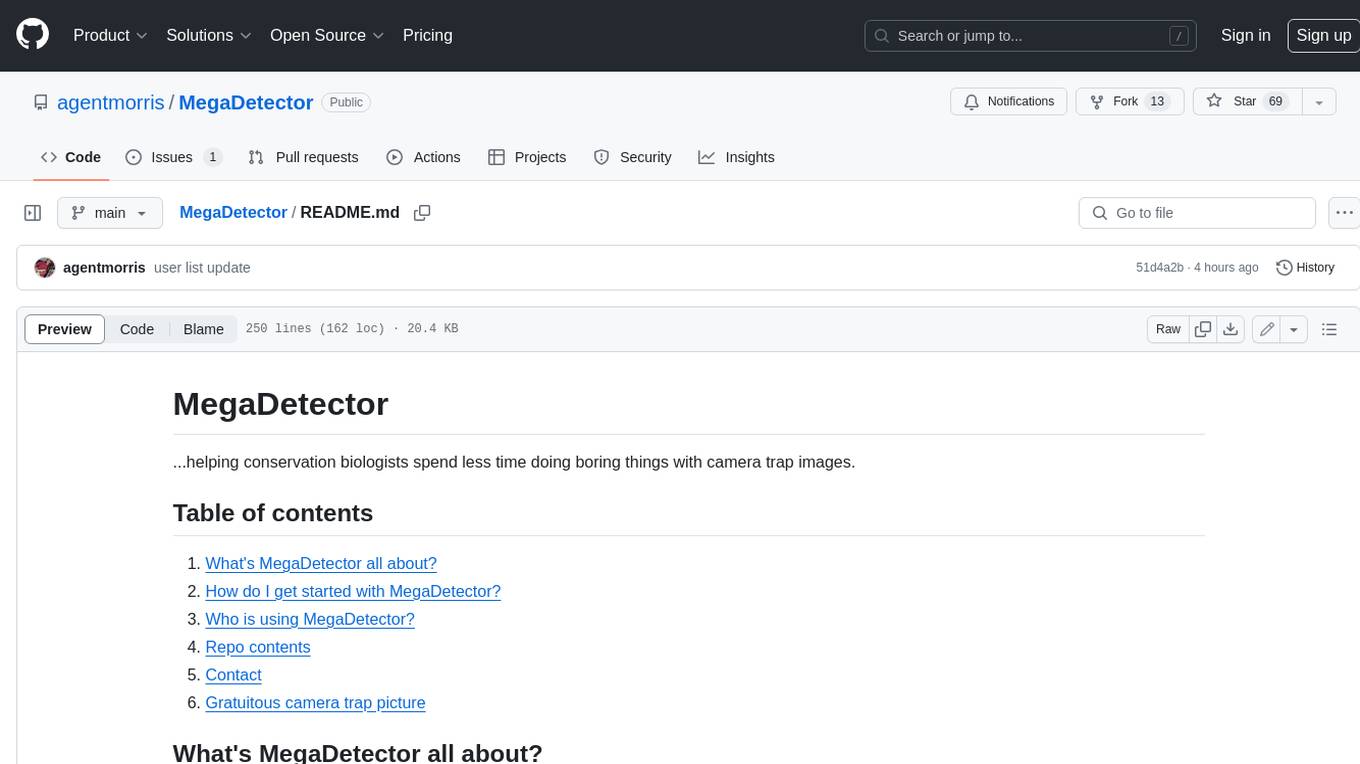
MegaDetector
MegaDetector is an AI model that identifies animals, people, and vehicles in camera trap images (which also makes it useful for eliminating blank images). This model is trained on several million images from a variety of ecosystems. MegaDetector is just one of many tools that aims to make conservation biologists more efficient with AI. If you want to learn about other ways to use AI to accelerate camera trap workflows, check out our of the field, affectionately titled "Everything I know about machine learning and camera traps".
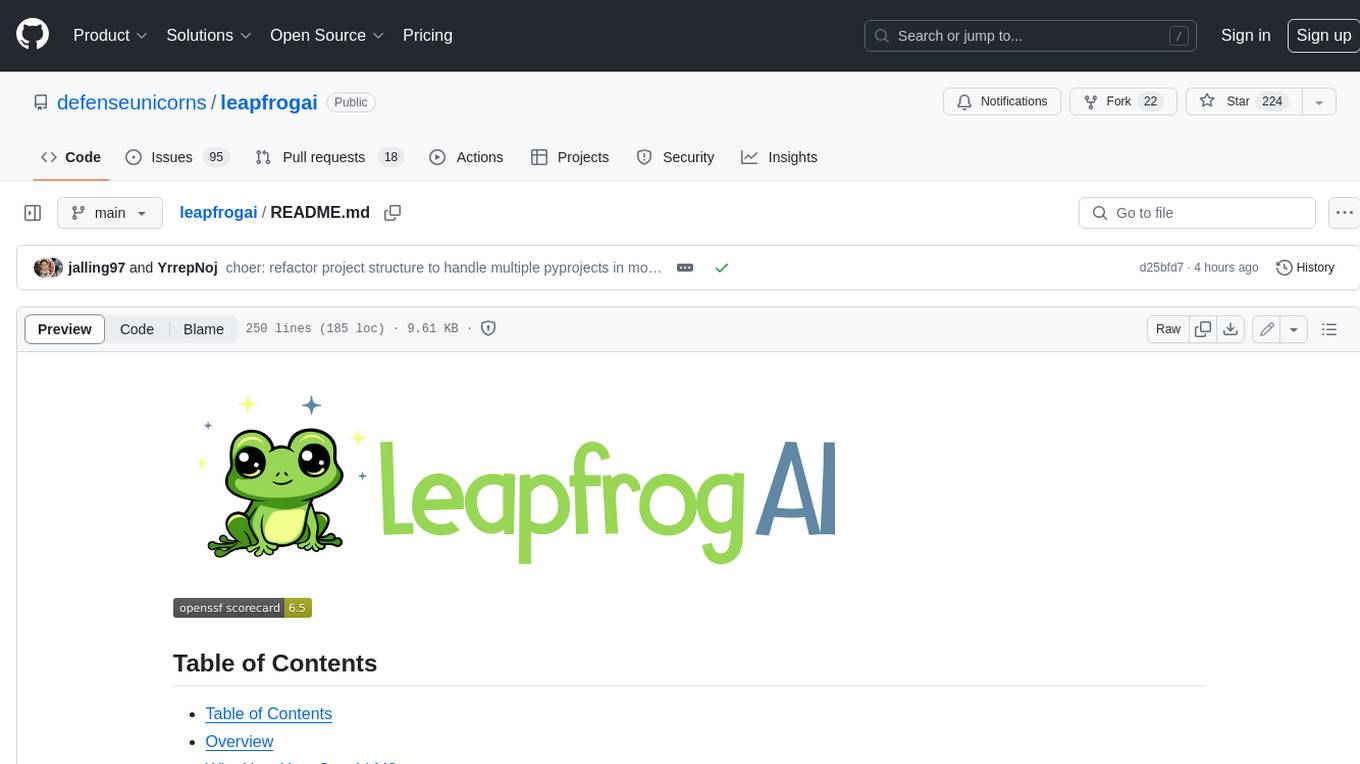
leapfrogai
LeapfrogAI is a self-hosted AI platform designed to be deployed in air-gapped resource-constrained environments. It brings sophisticated AI solutions to these environments by hosting all the necessary components of an AI stack, including vector databases, model backends, API, and UI. LeapfrogAI's API closely matches that of OpenAI, allowing tools built for OpenAI/ChatGPT to function seamlessly with a LeapfrogAI backend. It provides several backends for various use cases, including llama-cpp-python, whisper, text-embeddings, and vllm. LeapfrogAI leverages Chainguard's apko to harden base python images, ensuring the latest supported Python versions are used by the other components of the stack. The LeapfrogAI SDK provides a standard set of protobuffs and python utilities for implementing backends and gRPC. LeapfrogAI offers UI options for common use-cases like chat, summarization, and transcription. It can be deployed and run locally via UDS and Kubernetes, built out using Zarf packages. LeapfrogAI is supported by a community of users and contributors, including Defense Unicorns, Beast Code, Chainguard, Exovera, Hypergiant, Pulze, SOSi, United States Navy, United States Air Force, and United States Space Force.
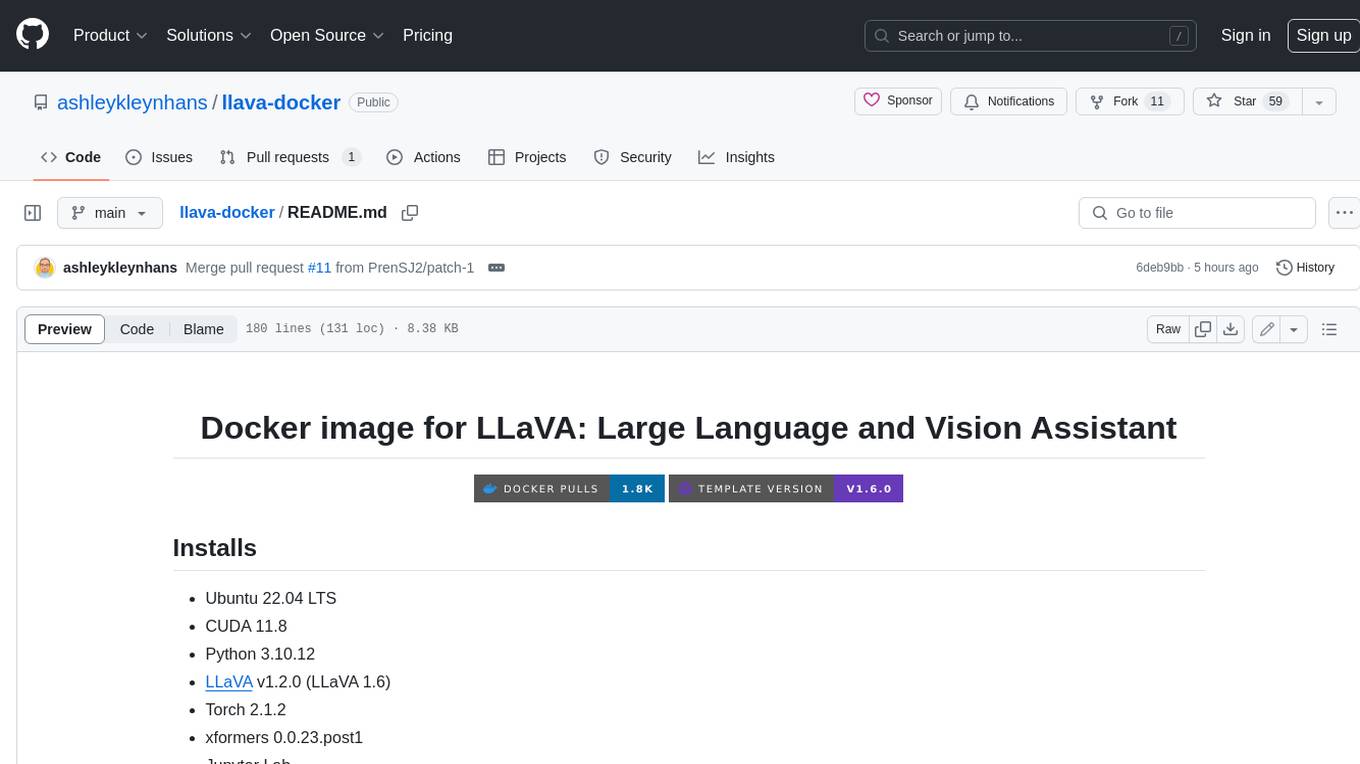
llava-docker
This Docker image for LLaVA (Large Language and Vision Assistant) provides a convenient way to run LLaVA locally or on RunPod. LLaVA is a powerful AI tool that combines natural language processing and computer vision capabilities. With this Docker image, you can easily access LLaVA's functionalities for various tasks, including image captioning, visual question answering, text summarization, and more. The image comes pre-installed with LLaVA v1.2.0, Torch 2.1.2, xformers 0.0.23.post1, and other necessary dependencies. You can customize the model used by setting the MODEL environment variable. The image also includes a Jupyter Lab environment for interactive development and exploration. Overall, this Docker image offers a comprehensive and user-friendly platform for leveraging LLaVA's capabilities.
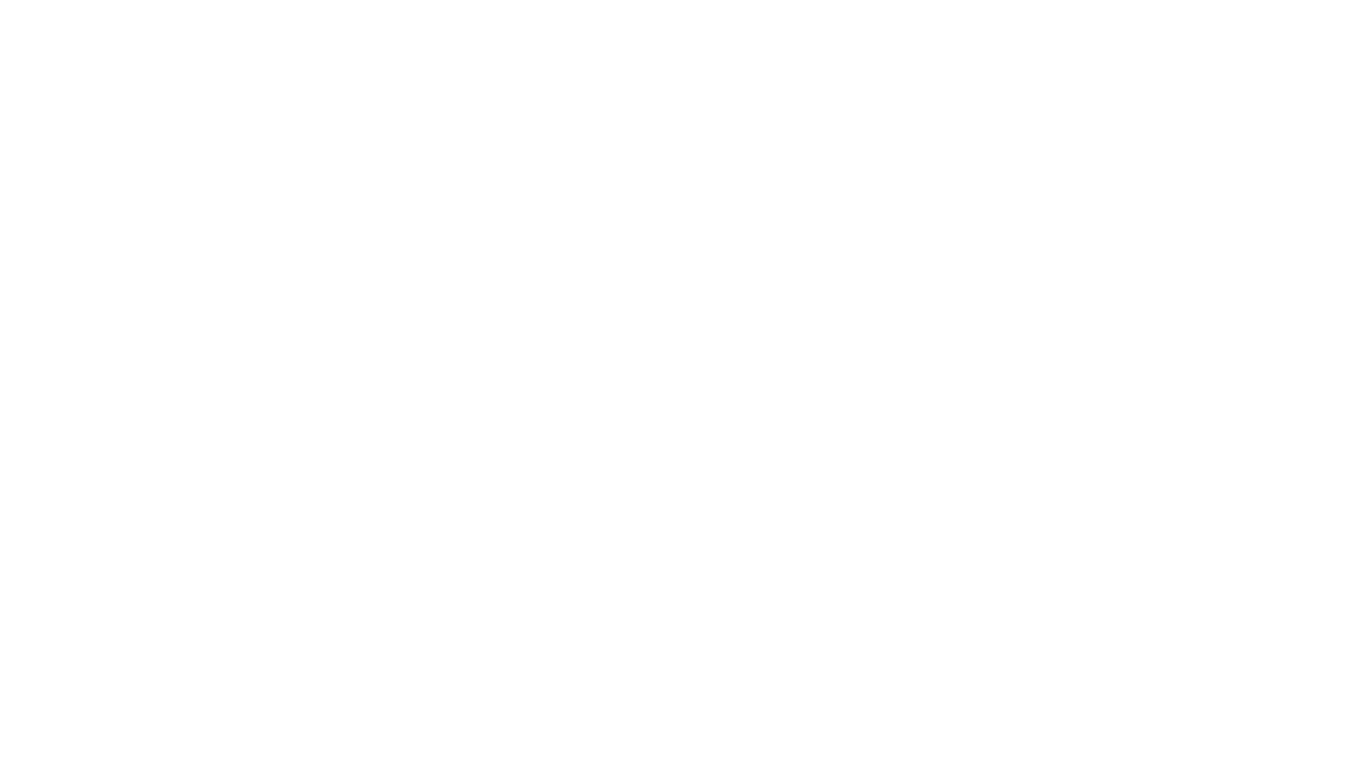
carrot
The 'carrot' repository on GitHub provides a list of free and user-friendly ChatGPT mirror sites for easy access. The repository includes sponsored sites offering various GPT models and services. Users can find and share sites, report errors, and access stable and recommended sites for ChatGPT usage. The repository also includes a detailed list of ChatGPT sites, their features, and accessibility options, making it a valuable resource for ChatGPT users seeking free and unlimited GPT services.
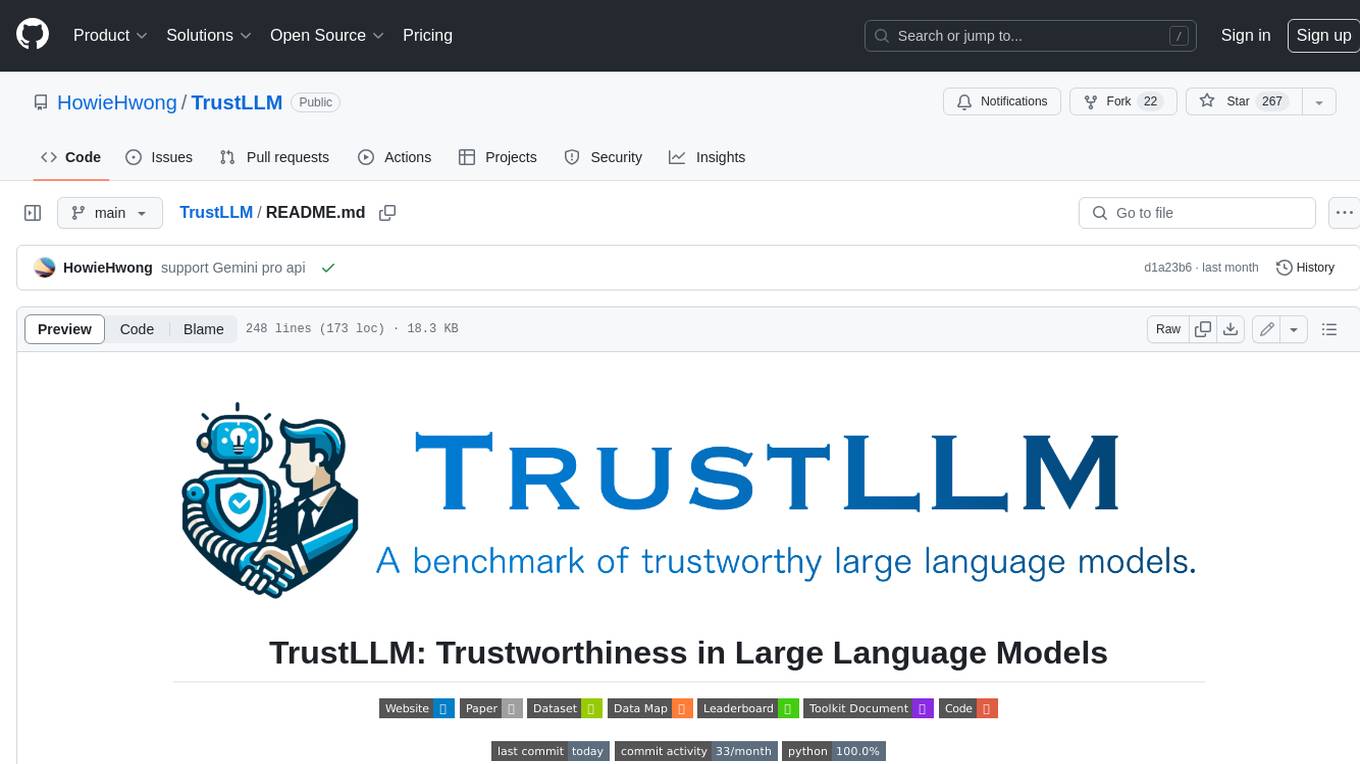
TrustLLM
TrustLLM is a comprehensive study of trustworthiness in LLMs, including principles for different dimensions of trustworthiness, established benchmark, evaluation, and analysis of trustworthiness for mainstream LLMs, and discussion of open challenges and future directions. Specifically, we first propose a set of principles for trustworthy LLMs that span eight different dimensions. Based on these principles, we further establish a benchmark across six dimensions including truthfulness, safety, fairness, robustness, privacy, and machine ethics. We then present a study evaluating 16 mainstream LLMs in TrustLLM, consisting of over 30 datasets. The document explains how to use the trustllm python package to help you assess the performance of your LLM in trustworthiness more quickly. For more details about TrustLLM, please refer to project website.
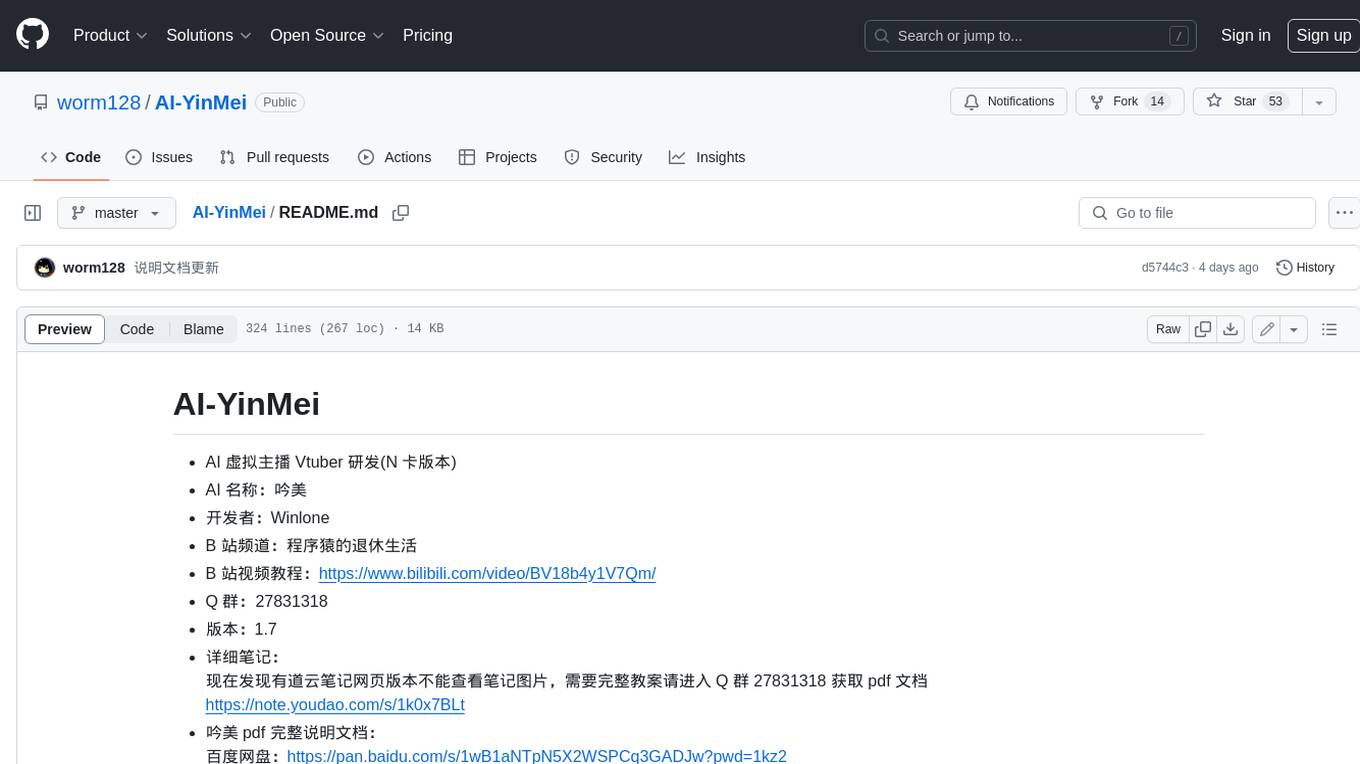
AI-YinMei
AI-YinMei is an AI virtual anchor Vtuber development tool (N card version). It supports fastgpt knowledge base chat dialogue, a complete set of solutions for LLM large language models: [fastgpt] + [one-api] + [Xinference], supports docking bilibili live broadcast barrage reply and entering live broadcast welcome speech, supports Microsoft edge-tts speech synthesis, supports Bert-VITS2 speech synthesis, supports GPT-SoVITS speech synthesis, supports expression control Vtuber Studio, supports painting stable-diffusion-webui output OBS live broadcast room, supports painting picture pornography public-NSFW-y-distinguish, supports search and image search service duckduckgo (requires magic Internet access), supports image search service Baidu image search (no magic Internet access), supports AI reply chat box [html plug-in], supports AI singing Auto-Convert-Music, supports playlist [html plug-in], supports dancing function, supports expression video playback, supports head touching action, supports gift smashing action, supports singing automatic start dancing function, chat and singing automatic cycle swing action, supports multi scene switching, background music switching, day and night automatic switching scene, supports open singing and painting, let AI automatically judge the content.