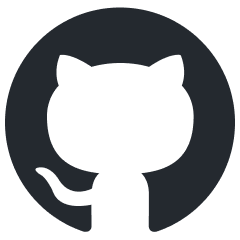
llm-gateway
Gateway for secure & reliable communications with OpenAI and other LLM providers
Stars: 182
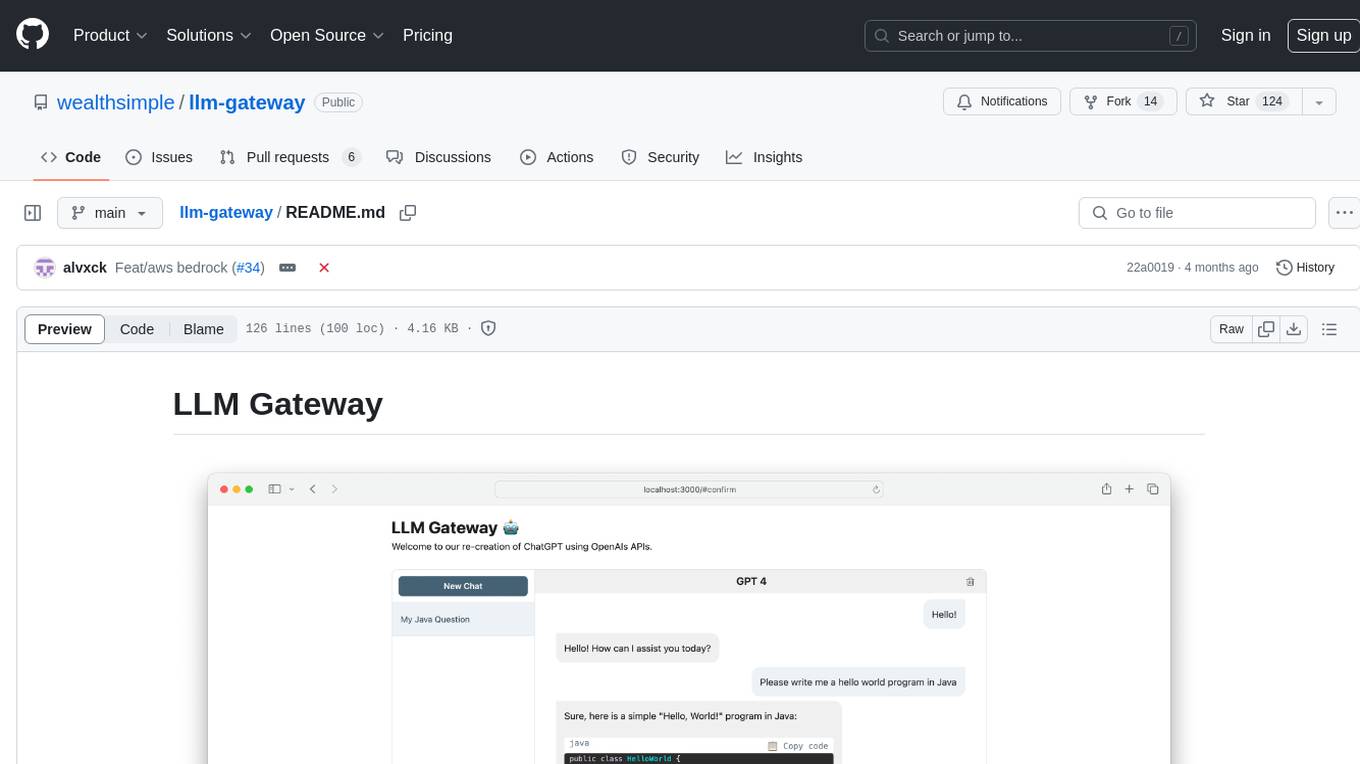
llm-gateway is a gateway tool designed for interacting with third-party LLM providers such as OpenAI, Cohere, etc. It tracks data exchanged with these providers in a postgres database, applies PII scrubbing heuristics, and ensures safe communication with OpenAI's services. The tool supports various models from different providers and offers API and Python usage examples. Developers can set up the tool using Poetry, Pyenv, npm, and yarn for dependency management. The project also includes Docker setup for backend and frontend development.
README:
llm-gateway
is a gateway for third party LLM providers such as OpenAI, Cohere, etc. It tracks data sent and received from these providers in a postgres database and runs PII scrubbing heuristics prior to sending.
Per OpenAI's non-API consumer products data usage policy, they "may use content such as prompts, responses, uploaded images, and generated images to improve our services" to improve products like ChatGPT and DALL-E.
Use llm-gateway
to interact with OpenAI in a safe manner. The gateway also recreates the ChatGPT frontend using OpenAI's /ChatCompletion
endpoint to keep all communication within the API.
Provider | Model |
---|---|
OpenAI | GPT 3.5 Turbo |
OpenAI | GPT 3.5 Turbo 16k |
OpenAI | GPT 4 |
AI21 Labs | Jurassic-2 Ultra |
AI21 Labs | Jurassic-2 Mid |
Amazon | Titan Text Lite |
Amazon | Titan Text Express |
Amazon | Titan Text Embeddings |
Anthropic | Claude 2.1 |
Anthropic | Claude 2.0 |
Anthropic | Claude 1.3 |
Anthropic | Claude Instant |
Cohere | Command |
Cohere | Command Light |
Cohere | Embed - English |
Cohere | Embed - Multilingual |
Meta | Llama-2-13b-chat |
Meta | Llama-2-70b-chat |
The provider's API key needs to be saved as an environment variable (see setup further down). If you are communicating with OpenAI, set OPENAI_API_KEY
.
For step-by-step setup instructions with Cohere, OpenAI, and AWS Bedrock, click here.
[OpenAI] Example cURL to /completion
endpoint:
curl -X 'POST' \
'http://<host>/api/openai/completion' \
-H 'accept: application/json' \
-H 'Content-Type: application/json' \
-d '{
"temperature": 0,
"prompt": "Tell me what is the meaning of life",
"max_tokens": 50,
"model": "text-davinci-003"
}'
[OpenAI] When using the /chat_completion
endpoint, formulate as conversation between user and assistant.
curl -X 'POST' \
'http://<host>/api/openai/chat_completion' \
-H 'accept: application/json' \
-H 'Content-Type: application/json' \
-d '{
"messages": [
{"role": "assistant", "content": "You are an intelligent assistant."},
{"role": "user", "content": "create a healthy recipe"}
],
"model": "gpt-3.5-turbo",
"temperature": 0
}'
from llm_gateway.providers.openai import OpenAIWrapper
wrapper = OpenAIWrapper()
wrapper.send_openai_request(
"Completion",
"create",
max_tokens=100,
prompt="What is the meaning of life?",
temperature=0,
model="text-davinci-003",
)
This project uses Poetry, Pyenv for dependency and environment management. Check out the official installation documentation for Poetry and Pyenv to get started. For front-end portion, this project use npm and yarn for dependency management. The most up-to-date node version required for this project is declared in .node-version.
If using Docker, steps 1-3 are optional. We recommend installing pre-commit hooks to speed up the development cycle.
- Install Poetry and Pyenv
- Install
pyenv install 3.11.3
- Install project requirements
brew install gitleaks
poetry install
poetry run pre-commit install
- Run
cp .envrc.example .envrc
and update with API secrets
To run in Docker:
# spin up docker-compose
make up
# open frontend in browser
make browse
# open FastAPI Swagger API
make browse-api
# delete docker-compose setup
make down
For Tasks:
Click tags to check more tools for each tasksFor Jobs:
Alternative AI tools for llm-gateway
Similar Open Source Tools
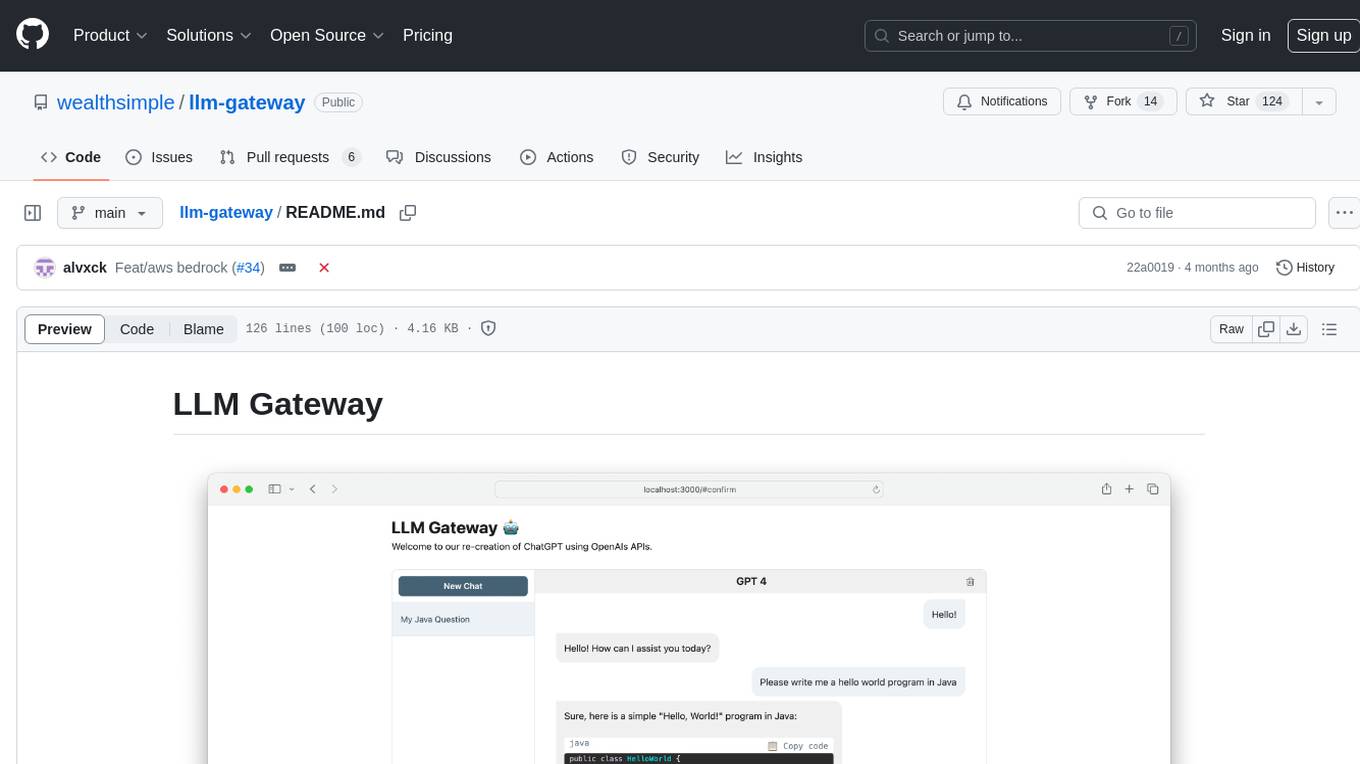
llm-gateway
llm-gateway is a gateway tool designed for interacting with third-party LLM providers such as OpenAI, Cohere, etc. It tracks data exchanged with these providers in a postgres database, applies PII scrubbing heuristics, and ensures safe communication with OpenAI's services. The tool supports various models from different providers and offers API and Python usage examples. Developers can set up the tool using Poetry, Pyenv, npm, and yarn for dependency management. The project also includes Docker setup for backend and frontend development.
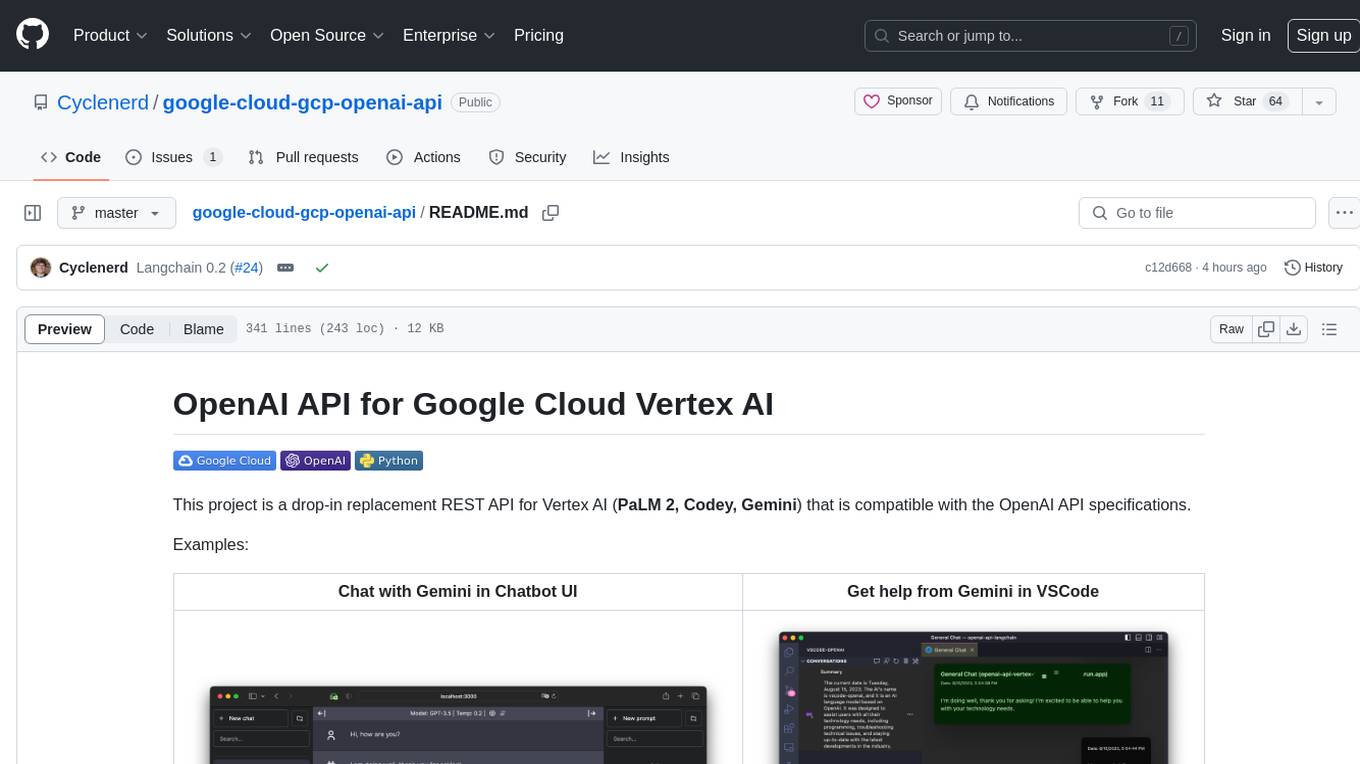
google-cloud-gcp-openai-api
This project provides a drop-in replacement REST API for Google Cloud Vertex AI (PaLM 2, Codey, Gemini) that is compatible with the OpenAI API specifications. It aims to make Google Cloud Platform Vertex AI more accessible by translating OpenAI API calls to Vertex AI. The software is developed in Python and based on FastAPI and LangChain, designed to be simple and customizable for individual needs. It includes step-by-step guides for deployment, supports various OpenAI API services, and offers configuration through environment variables. Additionally, it provides examples for running locally and usage instructions consistent with the OpenAI API format.
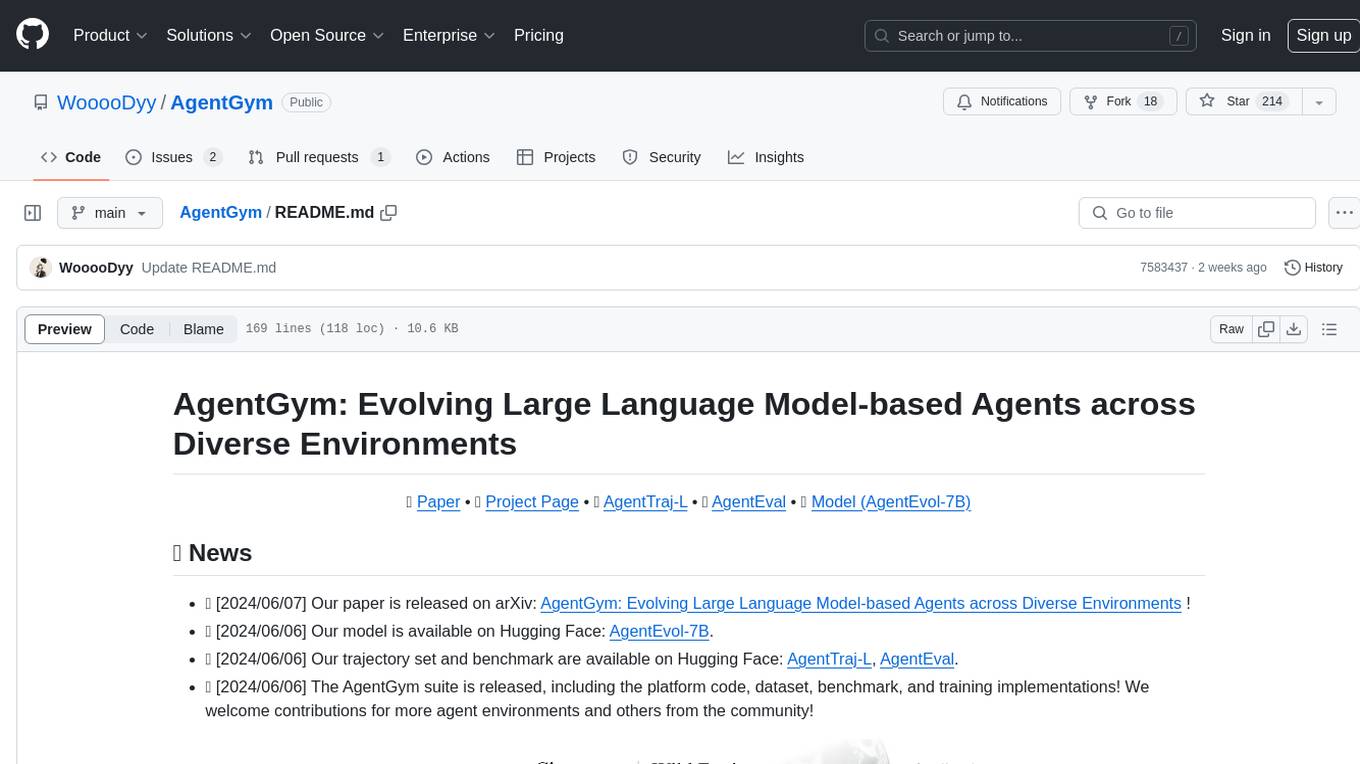
AgentGym
AgentGym is a framework designed to help the AI community evaluate and develop generally-capable Large Language Model-based agents. It features diverse interactive environments and tasks with real-time feedback and concurrency. The platform supports 14 environments across various domains like web navigating, text games, house-holding tasks, digital games, and more. AgentGym includes a trajectory set (AgentTraj) and a benchmark suite (AgentEval) to facilitate agent exploration and evaluation. The framework allows for agent self-evolution beyond existing data, showcasing comparable results to state-of-the-art models.
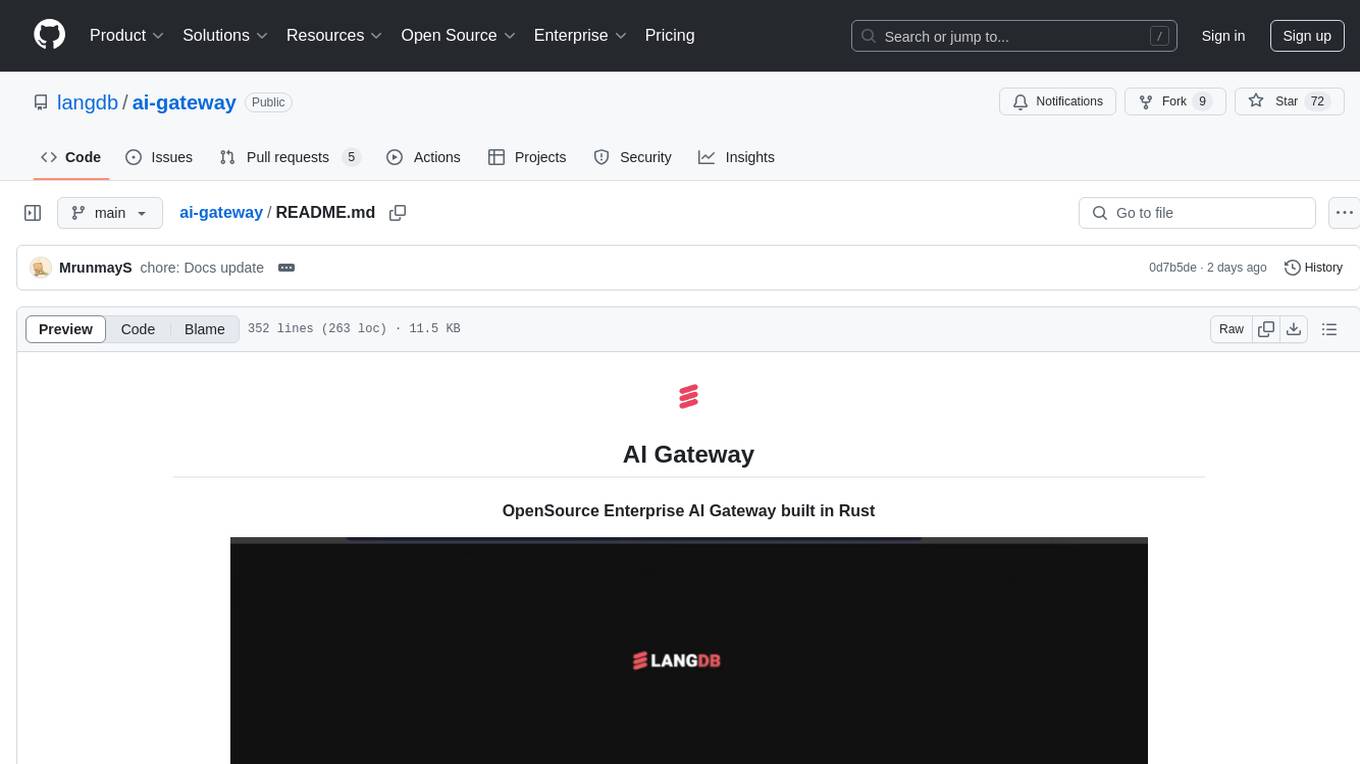
ai-gateway
LangDB AI Gateway is an open-source enterprise AI gateway built in Rust. It provides a unified interface to all LLMs using the OpenAI API format, focusing on high performance, enterprise readiness, and data control. The gateway offers features like comprehensive usage analytics, cost tracking, rate limiting, data ownership, and detailed logging. It supports various LLM providers and provides OpenAI-compatible endpoints for chat completions, model listing, embeddings generation, and image generation. Users can configure advanced settings, such as rate limiting, cost control, dynamic model routing, and observability with OpenTelemetry tracing. The gateway can be run with Docker Compose and integrated with MCP tools for server communication.
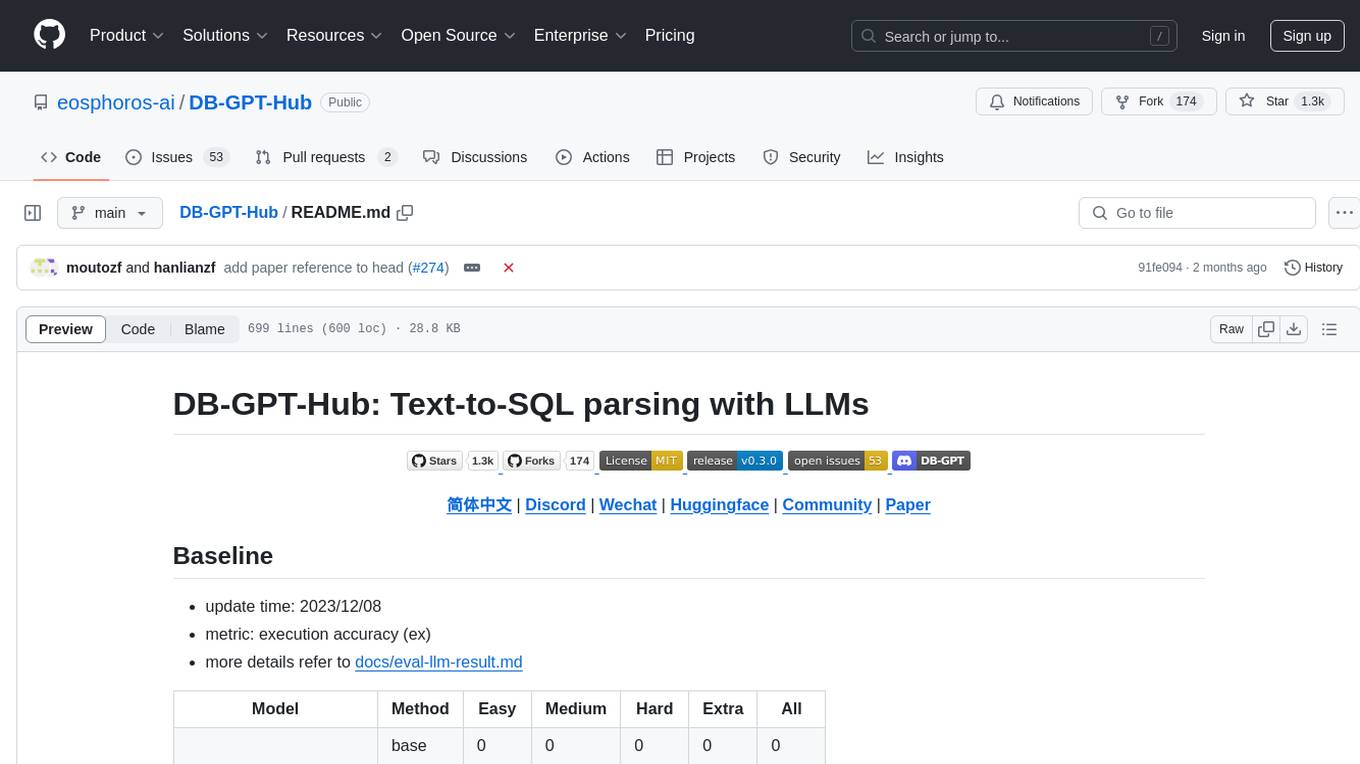
DB-GPT-Hub
DB-GPT-Hub is an experimental project leveraging Large Language Models (LLMs) for Text-to-SQL parsing. It includes stages like data collection, preprocessing, model selection, construction, and fine-tuning of model weights. The project aims to enhance Text-to-SQL capabilities, reduce model training costs, and enable developers to contribute to improving Text-to-SQL accuracy. The ultimate goal is to achieve automated question-answering based on databases, allowing users to execute complex database queries using natural language descriptions. The project has successfully integrated multiple large models and established a comprehensive workflow for data processing, SFT model training, prediction output, and evaluation.
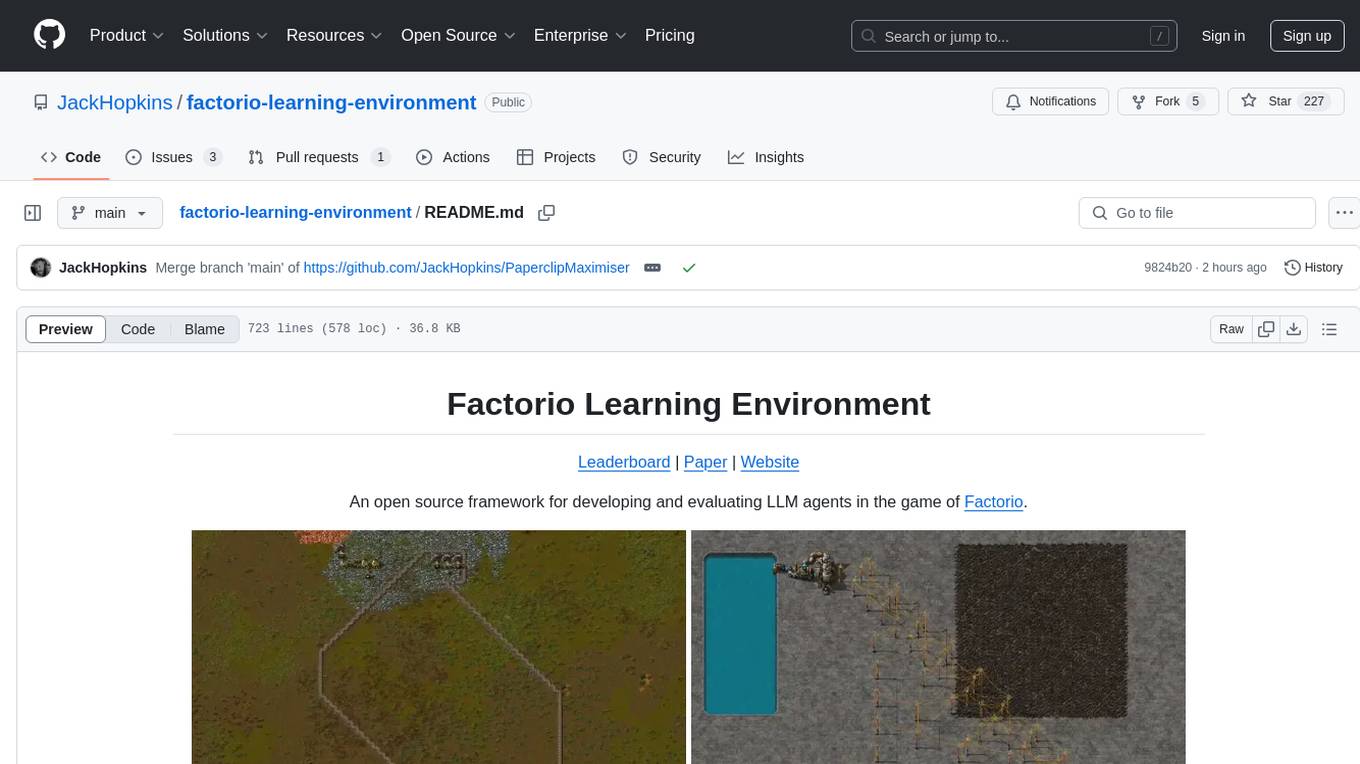
factorio-learning-environment
Factorio Learning Environment is an open source framework designed for developing and evaluating LLM agents in the game of Factorio. It provides two settings: Lab-play with structured tasks and Open-play for building large factories. Results show limitations in spatial reasoning and automation strategies. Agents interact with the environment through code synthesis, observation, action, and feedback. Tools are provided for game actions and state representation. Agents operate in episodes with observation, planning, and action execution. Tasks specify agent goals and are implemented in JSON files. The project structure includes directories for agents, environment, cluster, data, docs, eval, and more. A database is used for checkpointing agent steps. Benchmarks show performance metrics for different configurations.
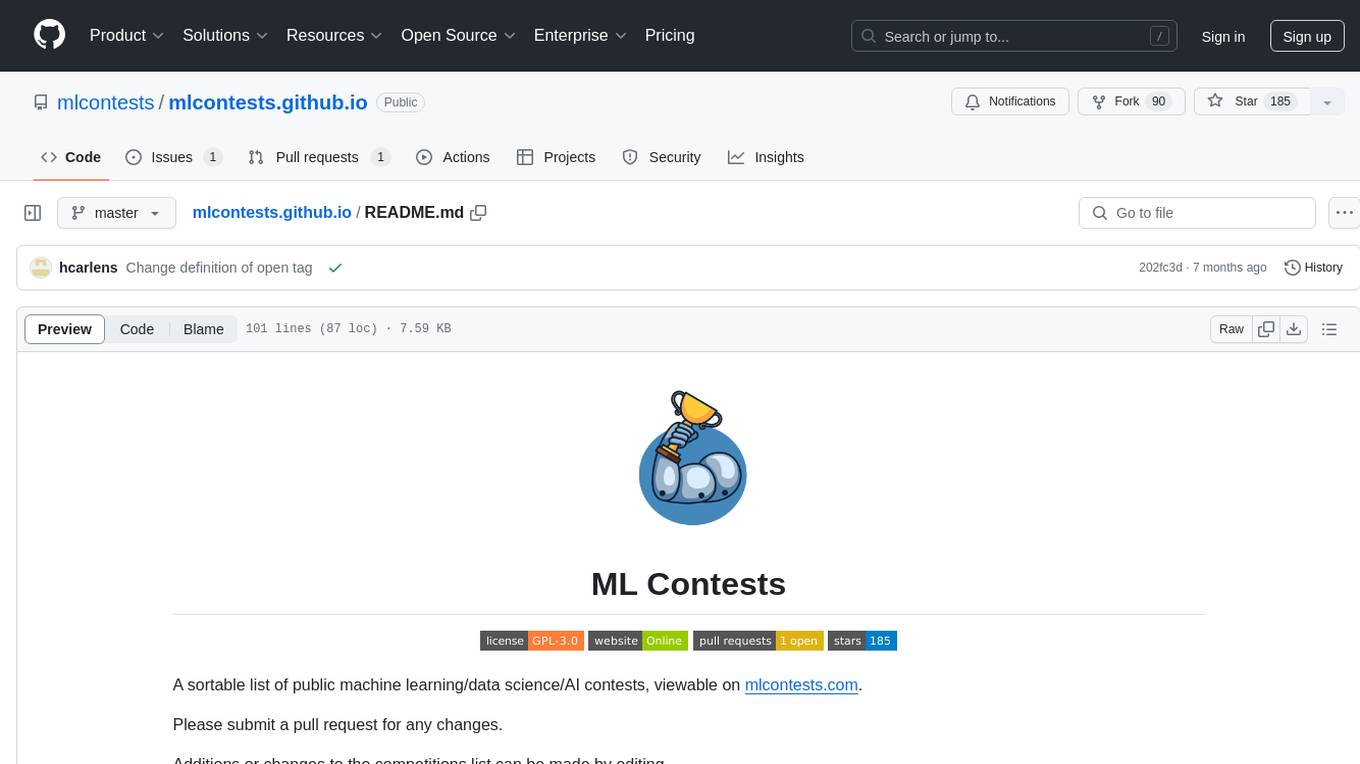
mlcontests.github.io
ML Contests is a platform that provides a sortable list of public machine learning/data science/AI contests, viewable on mlcontests.com. Users can submit pull requests for any changes or additions to the competitions list by editing the competitions.json file on the GitHub repository. The platform requires mandatory fields such as competition name, URL, type of ML, deadline for submissions, prize information, platform running the competition, and sponsorship details. Optional fields include conference affiliation, conference year, competition launch date, registration deadline, additional URLs, and tags relevant to the challenge type. The platform is transitioning towards assigning multiple tags to competitions for better categorization and searchability.
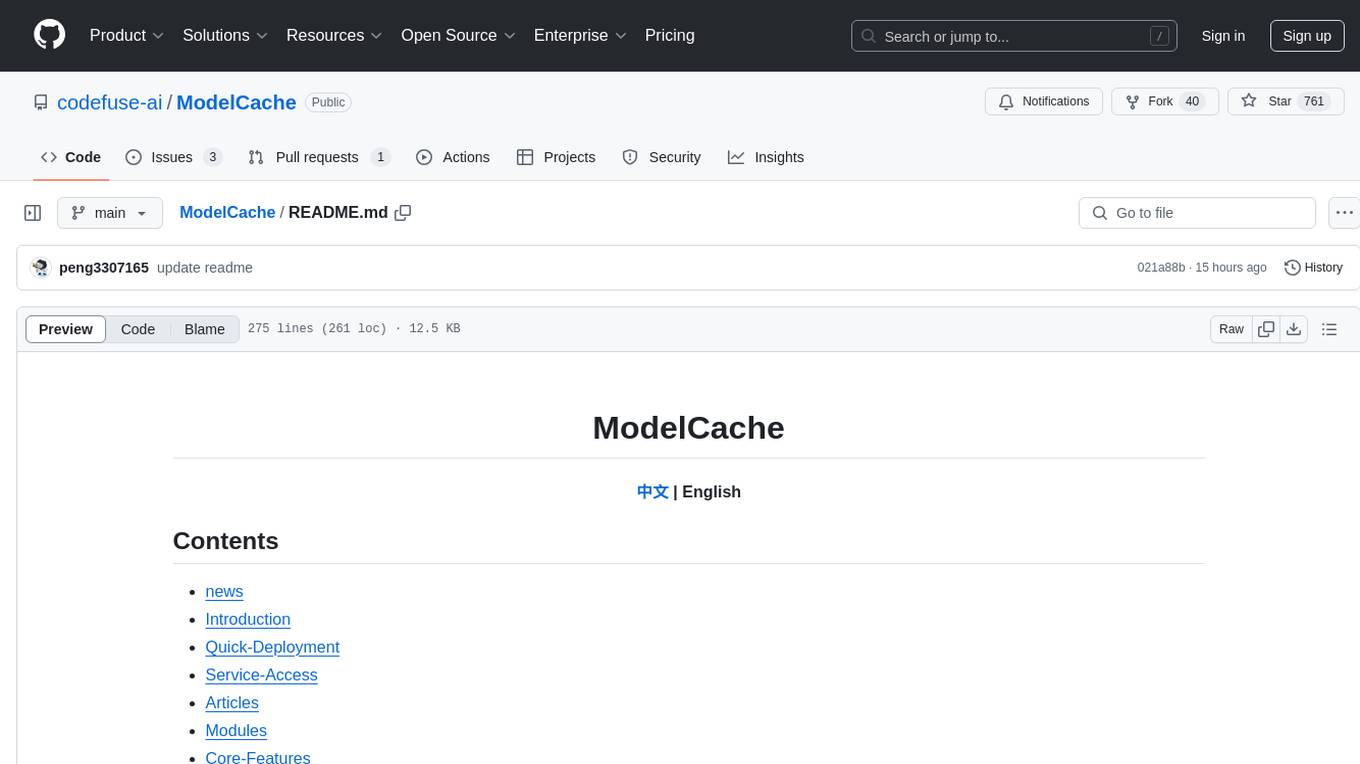
ModelCache
Codefuse-ModelCache is a semantic cache for large language models (LLMs) that aims to optimize services by introducing a caching mechanism. It helps reduce the cost of inference deployment, improve model performance and efficiency, and provide scalable services for large models. The project facilitates sharing and exchanging technologies related to large model semantic cache through open-source collaboration.
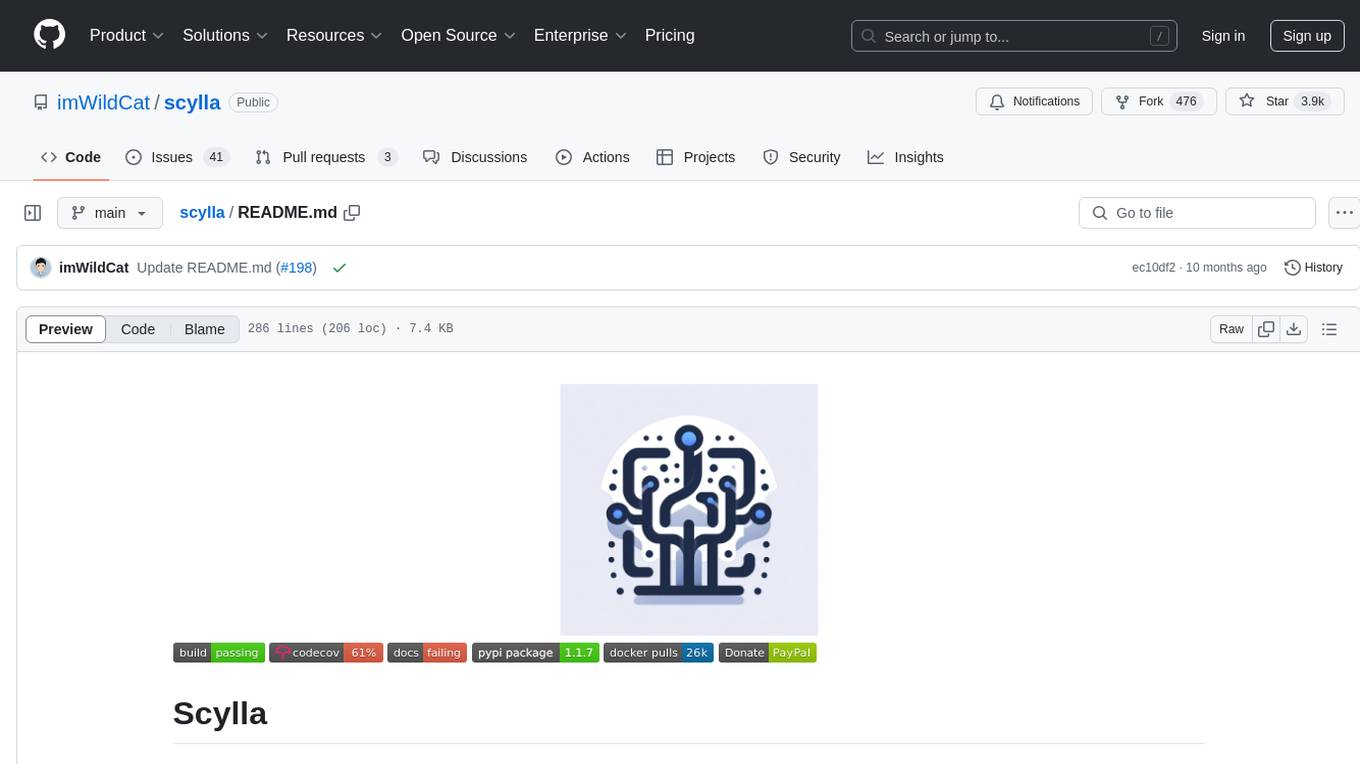
scylla
Scylla is an intelligent proxy pool tool designed for humanities, enabling users to extract content from the internet and build their own Large Language Models in the AI era. It features automatic proxy IP crawling and validation, an easy-to-use JSON API, a simple web-based user interface, HTTP forward proxy server, Scrapy and requests integration, and headless browser crawling. Users can start using Scylla with just one command, making it a versatile tool for various web scraping and content extraction tasks.
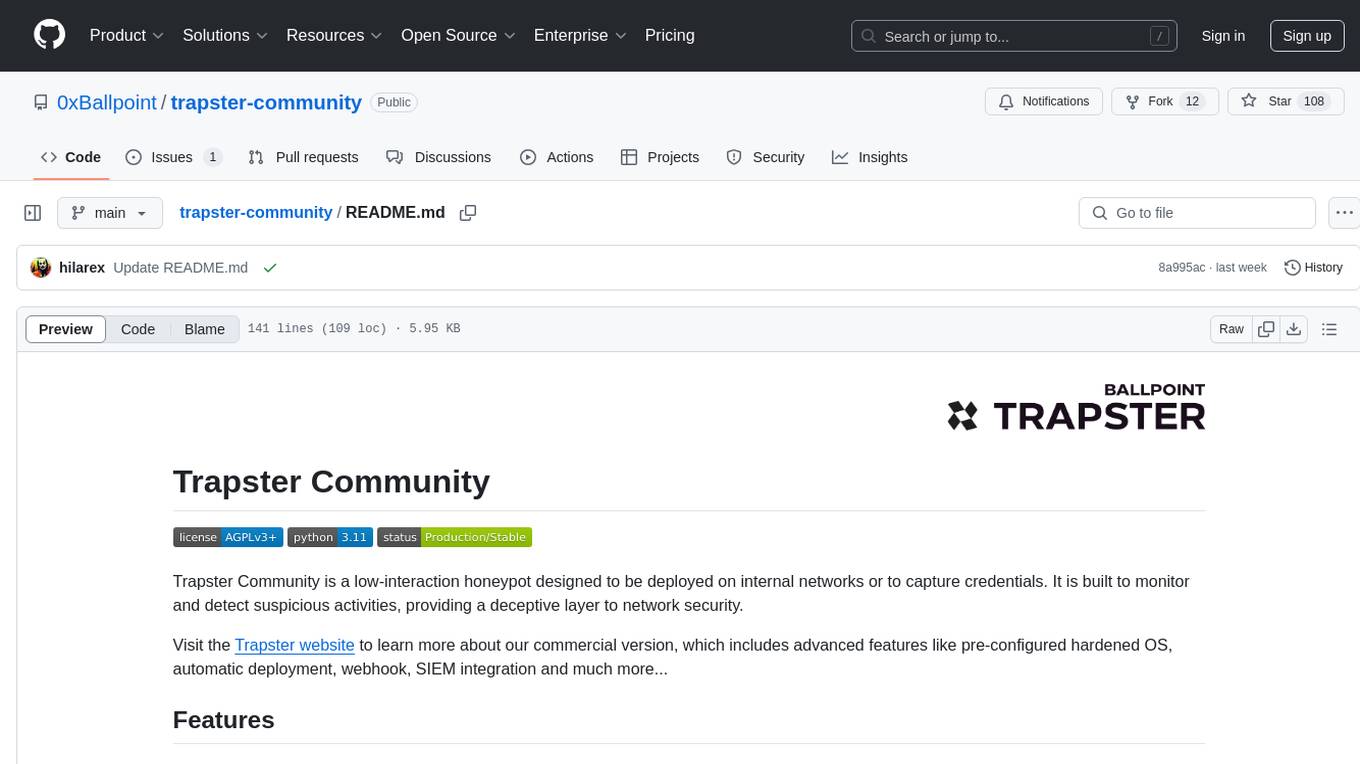
trapster-community
Trapster Community is a low-interaction honeypot designed for internal networks or credential capture. It monitors and detects suspicious activities, providing deceptive security layer. Features include mimicking network services, asynchronous framework, easy configuration, expandable services, and HTTP honeypot engine with AI capabilities. Supported protocols include DNS, HTTP/HTTPS, FTP, LDAP, MSSQL, POSTGRES, RDP, SNMP, SSH, TELNET, VNC, and RSYNC. The tool generates various types of logs and offers HTTP engine with AI capabilities to emulate websites using YAML configuration. Contributions are welcome under AGPLv3+ license.
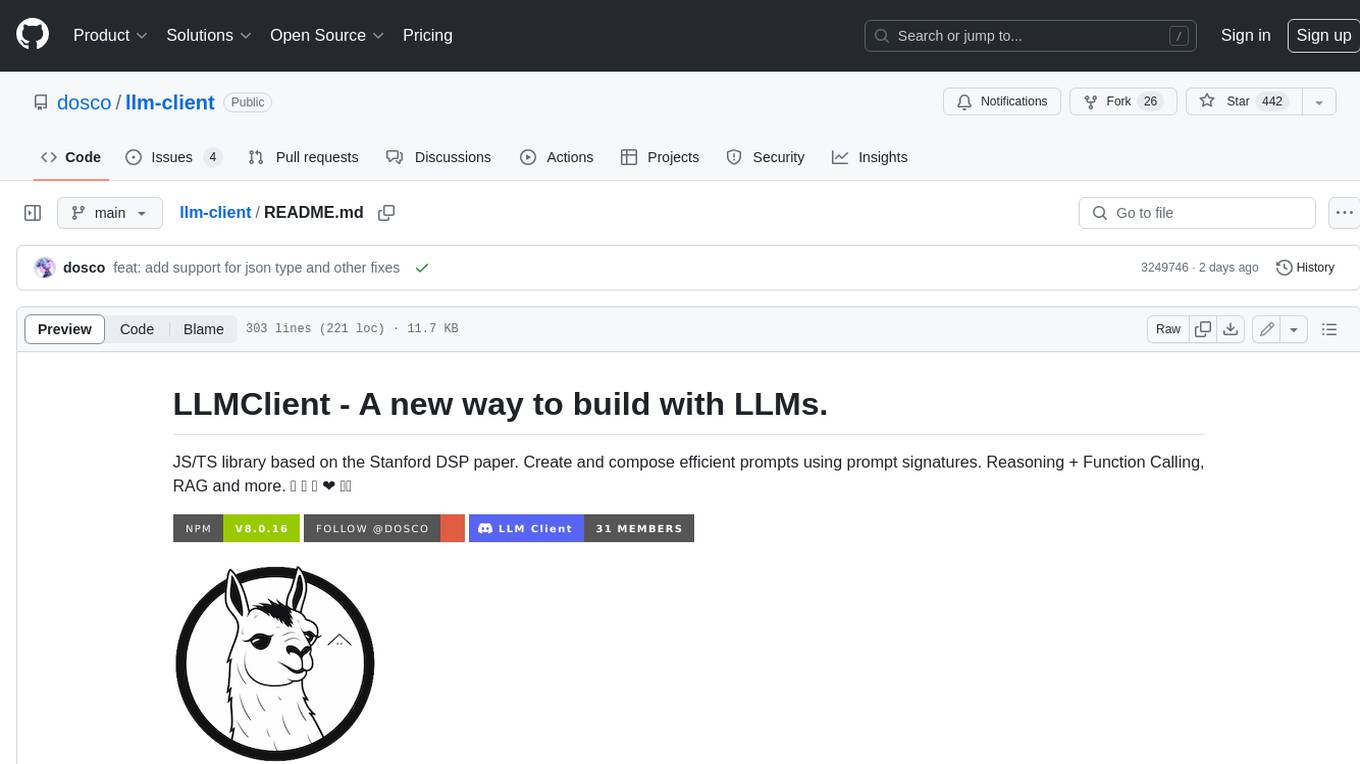
llm-client
LLMClient is a JavaScript/TypeScript library that simplifies working with large language models (LLMs) by providing an easy-to-use interface for building and composing efficient prompts using prompt signatures. These signatures enable the automatic generation of typed prompts, allowing developers to leverage advanced capabilities like reasoning, function calling, RAG, ReAcT, and Chain of Thought. The library supports various LLMs and vector databases, making it a versatile tool for a wide range of applications.
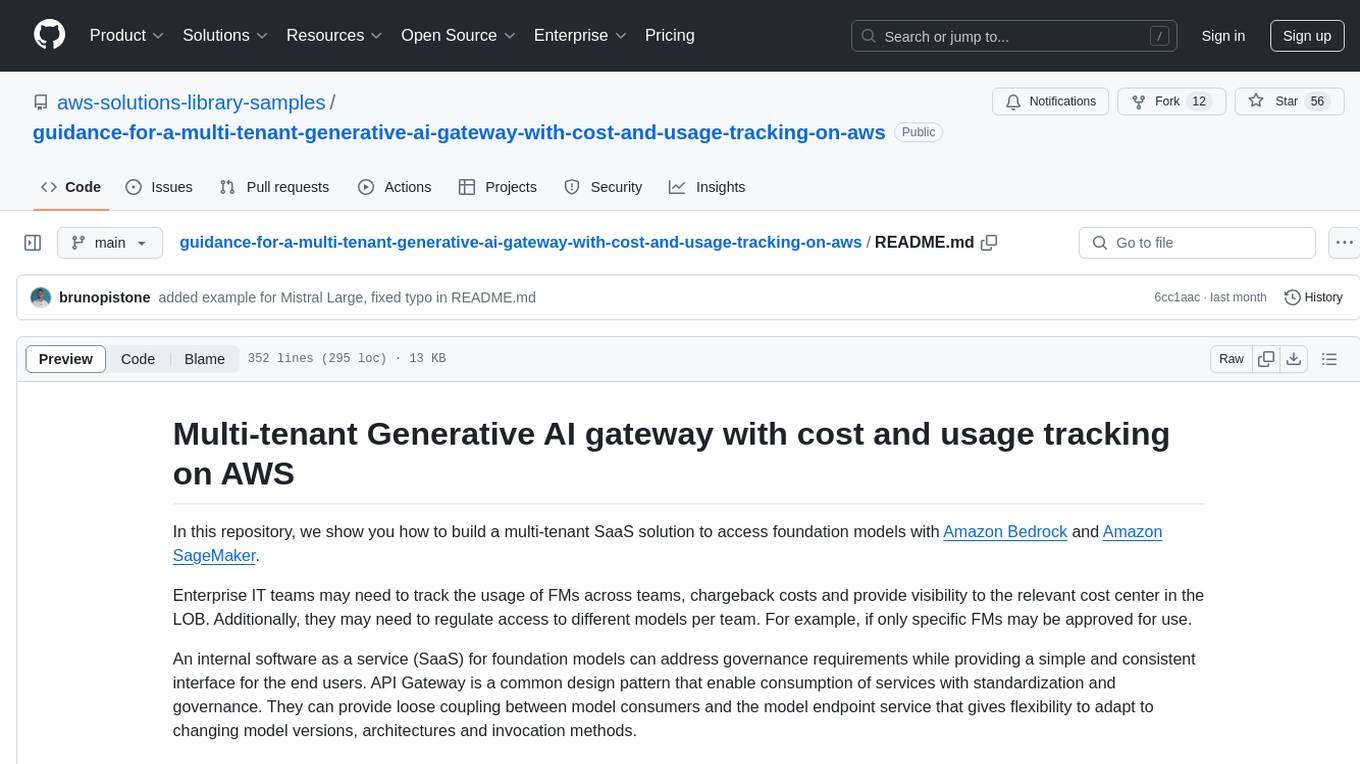
guidance-for-a-multi-tenant-generative-ai-gateway-with-cost-and-usage-tracking-on-aws
This repository provides guidance on building a multi-tenant SaaS solution for accessing foundation models using Amazon Bedrock and Amazon SageMaker. It helps enterprise IT teams track usage and costs of foundation models, regulate access, and provide visibility to cost centers. The solution includes an API Gateway design pattern for standardization and governance, enabling loose coupling between model consumers and endpoint services. The CDK Stack deploys resources for private networking, API Gateway, Lambda functions, DynamoDB table, EventBridge, S3 buckets, and Cloudwatch logs.
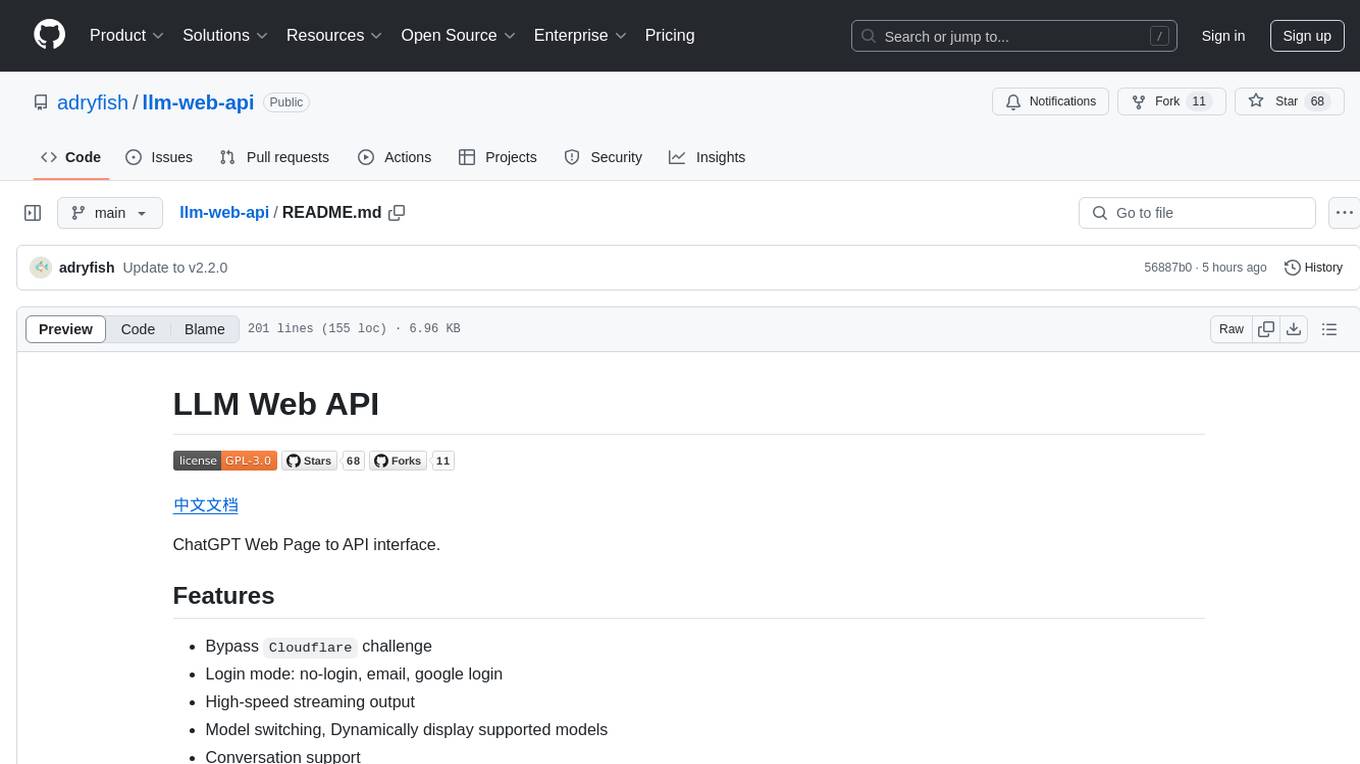
llm-web-api
LLM Web API is a tool that provides a web page to API interface for ChatGPT, allowing users to bypass Cloudflare challenges, switch models, and dynamically display supported models. It uses Playwright to control a fingerprint browser, simulating user operations to send requests to the OpenAI website and converting the responses into API interfaces. The API currently supports the OpenAI-compatible /v1/chat/completions API, accessible using OpenAI or other compatible clients.
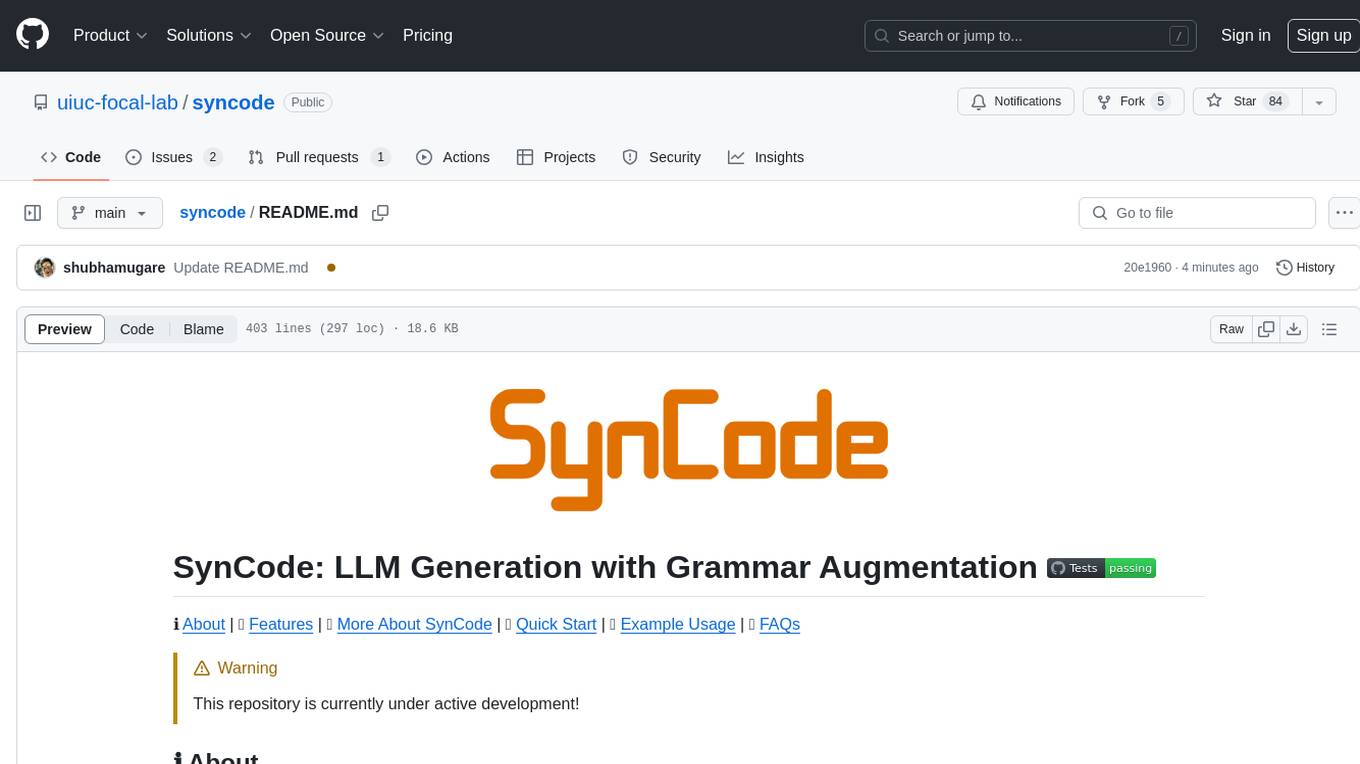
syncode
SynCode is a novel framework for the grammar-guided generation of Large Language Models (LLMs) that ensures syntactically valid output with respect to defined Context-Free Grammar (CFG) rules. It supports general-purpose programming languages like Python, Go, SQL, JSON, and more, allowing users to define custom grammars using EBNF syntax. The tool compares favorably to other constrained decoders and offers features like fast grammar-guided generation, compatibility with HuggingFace Language Models, and the ability to work with various decoding strategies.
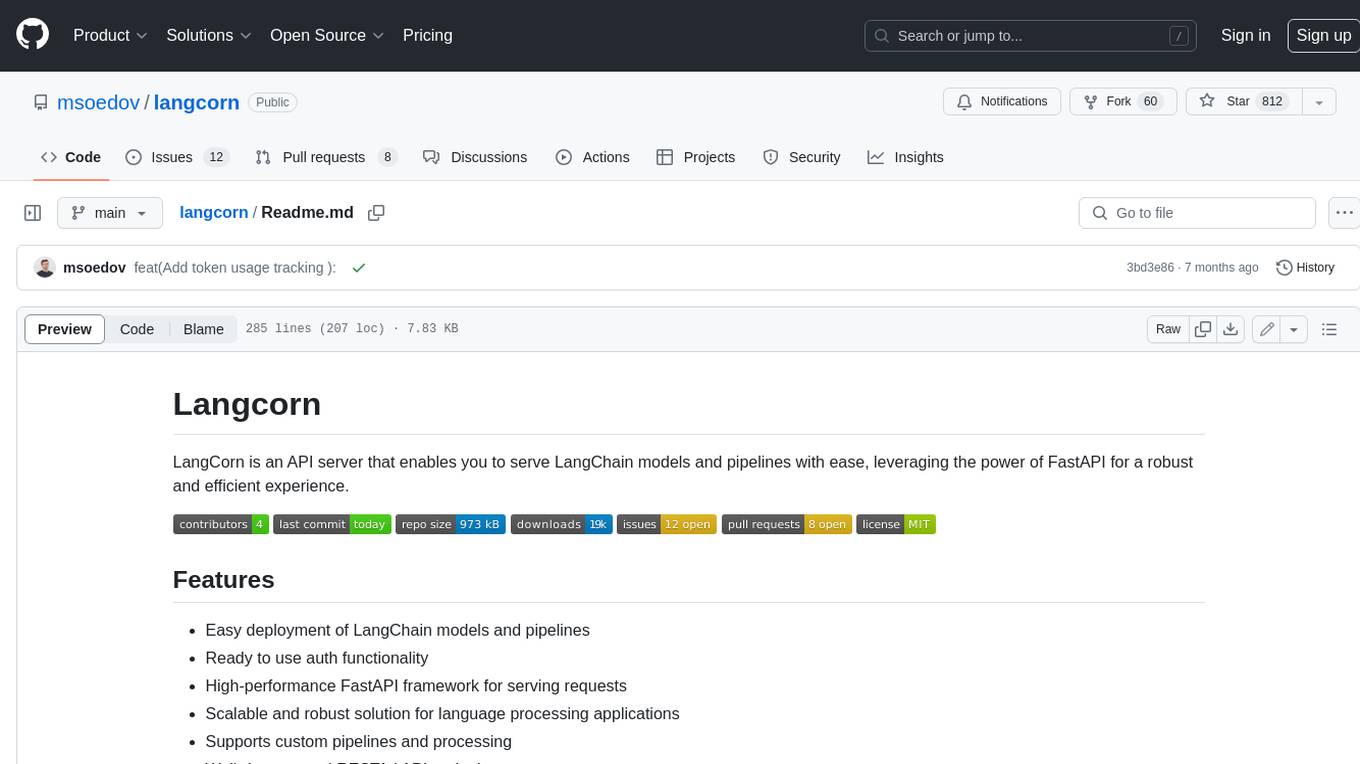
langcorn
LangCorn is an API server that enables you to serve LangChain models and pipelines with ease, leveraging the power of FastAPI for a robust and efficient experience. It offers features such as easy deployment of LangChain models and pipelines, ready-to-use authentication functionality, high-performance FastAPI framework for serving requests, scalability and robustness for language processing applications, support for custom pipelines and processing, well-documented RESTful API endpoints, and asynchronous processing for faster response times.
For similar tasks
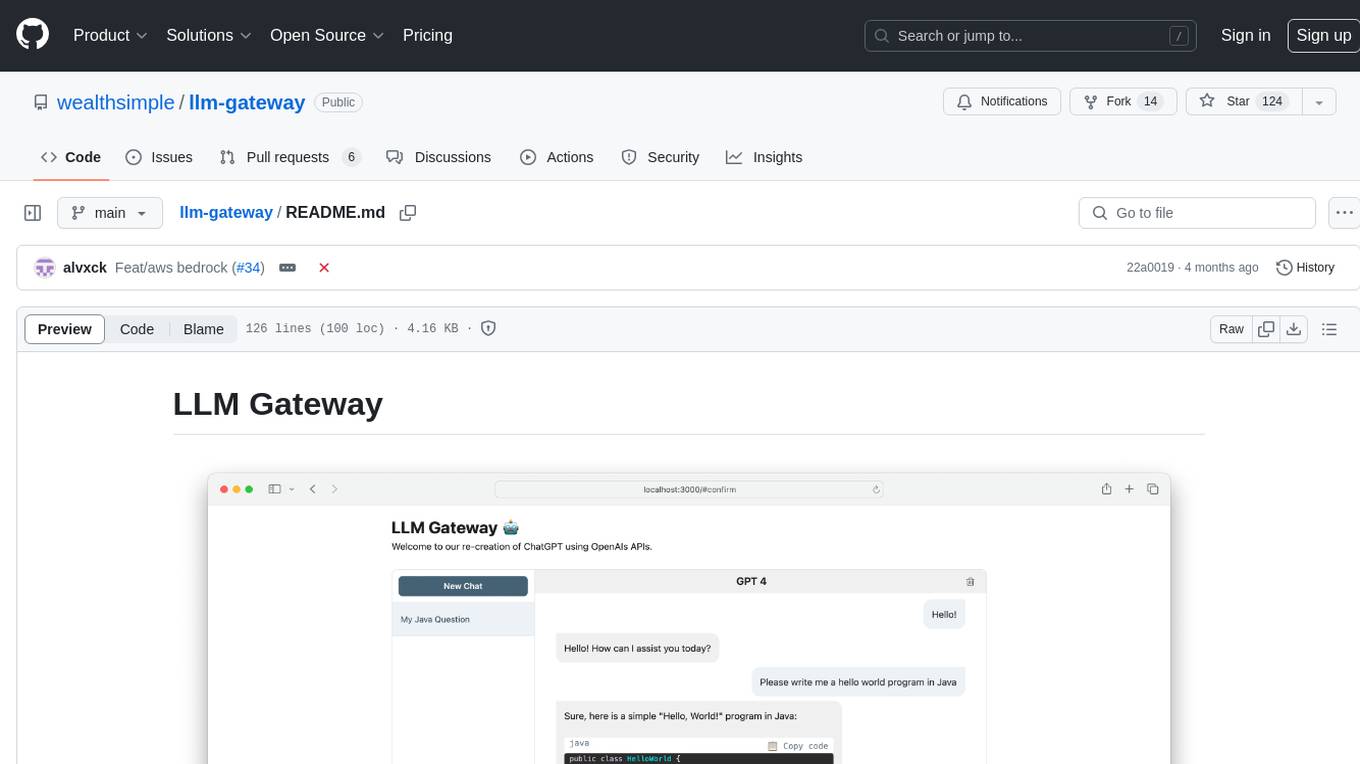
llm-gateway
llm-gateway is a gateway tool designed for interacting with third-party LLM providers such as OpenAI, Cohere, etc. It tracks data exchanged with these providers in a postgres database, applies PII scrubbing heuristics, and ensures safe communication with OpenAI's services. The tool supports various models from different providers and offers API and Python usage examples. Developers can set up the tool using Poetry, Pyenv, npm, and yarn for dependency management. The project also includes Docker setup for backend and frontend development.
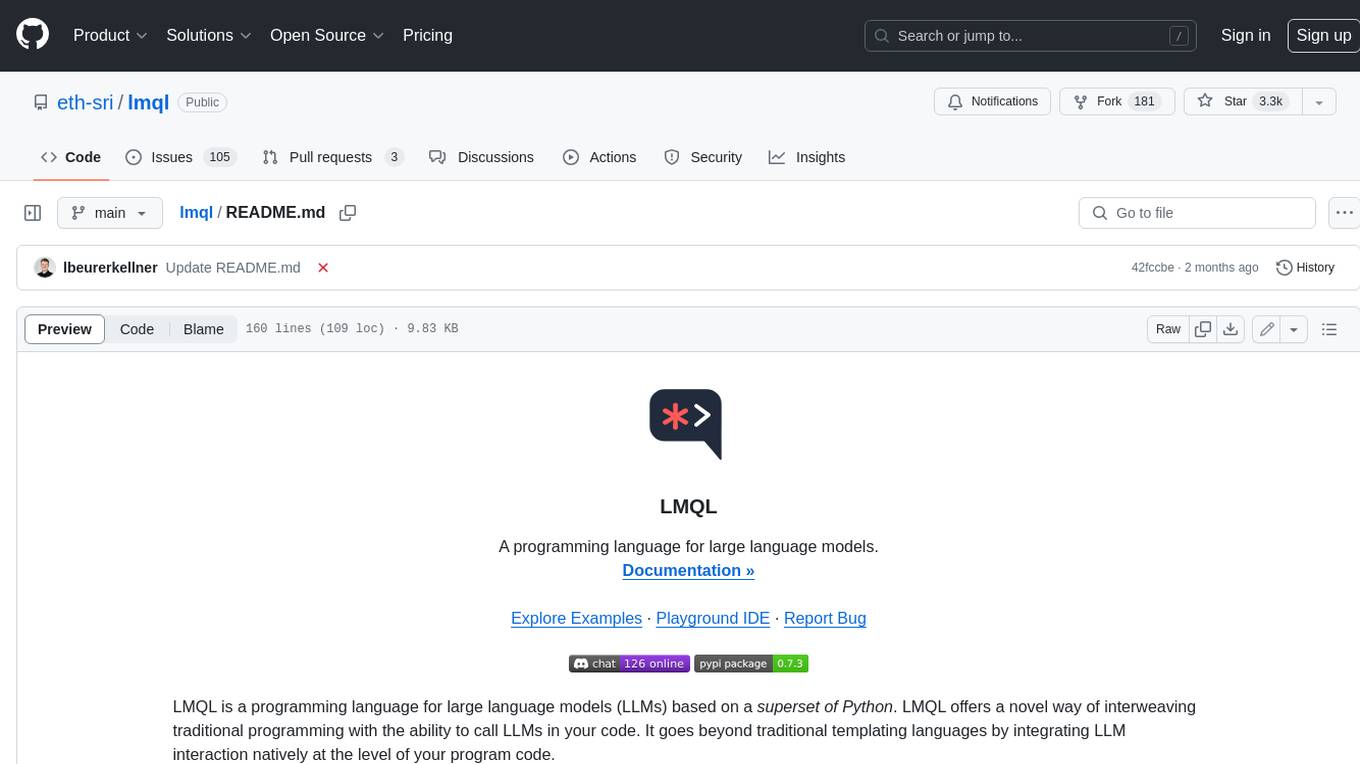
lmql
LMQL is a programming language designed for large language models (LLMs) that offers a unique way of integrating traditional programming with LLM interaction. It allows users to write programs that combine algorithmic logic with LLM calls, enabling model reasoning capabilities within the context of the program. LMQL provides features such as Python syntax integration, rich control-flow options, advanced decoding techniques, powerful constraints via logit masking, runtime optimization, sync and async API support, multi-model compatibility, and extensive applications like JSON decoding and interactive chat interfaces. The tool also offers library integration, flexible tooling, and output streaming options for easy model output handling.
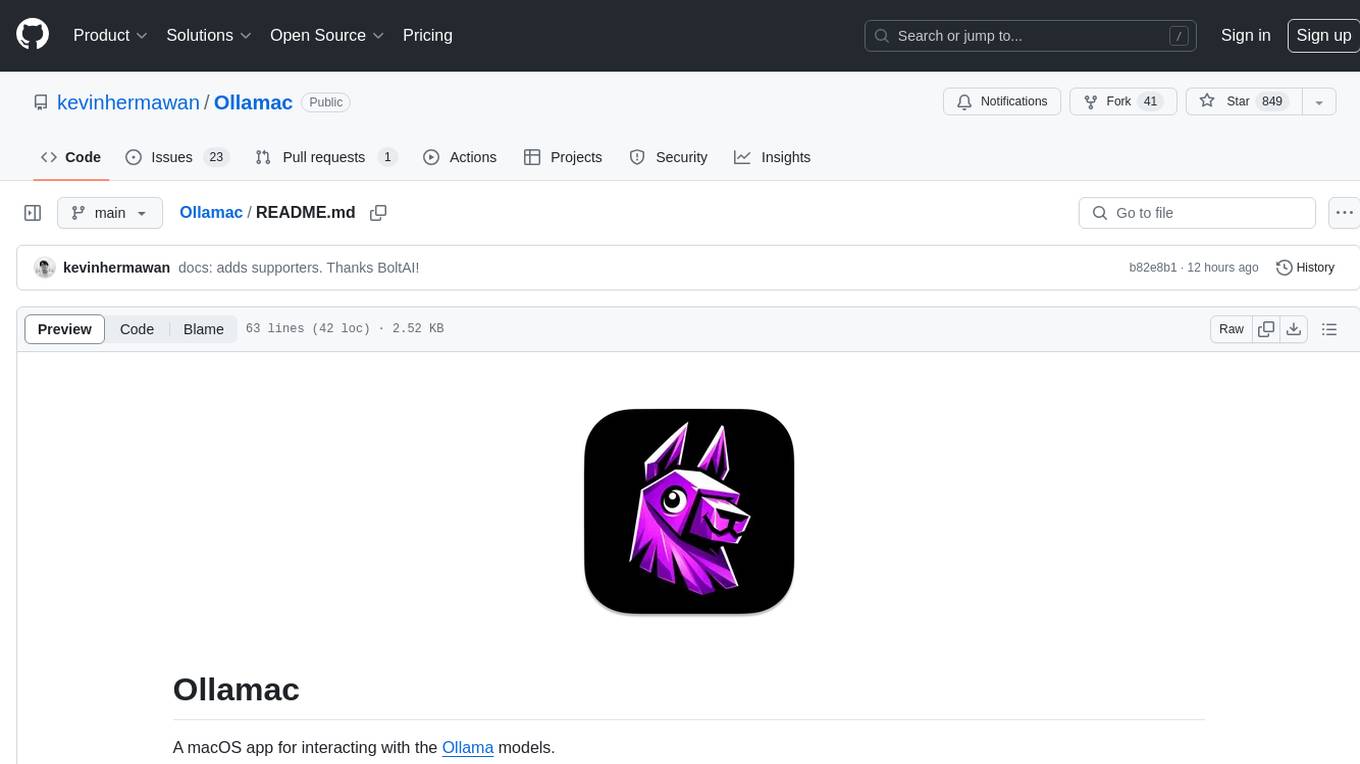
Ollamac
Ollamac is a macOS app designed for interacting with Ollama models. It is optimized for macOS, allowing users to easily use any model from the Ollama library. The app features a user-friendly interface, chat archive for saving interactions, and real-time communication using HTTP streaming technology. Ollamac is open-source, enabling users to contribute to its development and enhance its capabilities. It requires macOS 14 or later and the Ollama system to be installed on the user's Mac with at least one Ollama model downloaded.
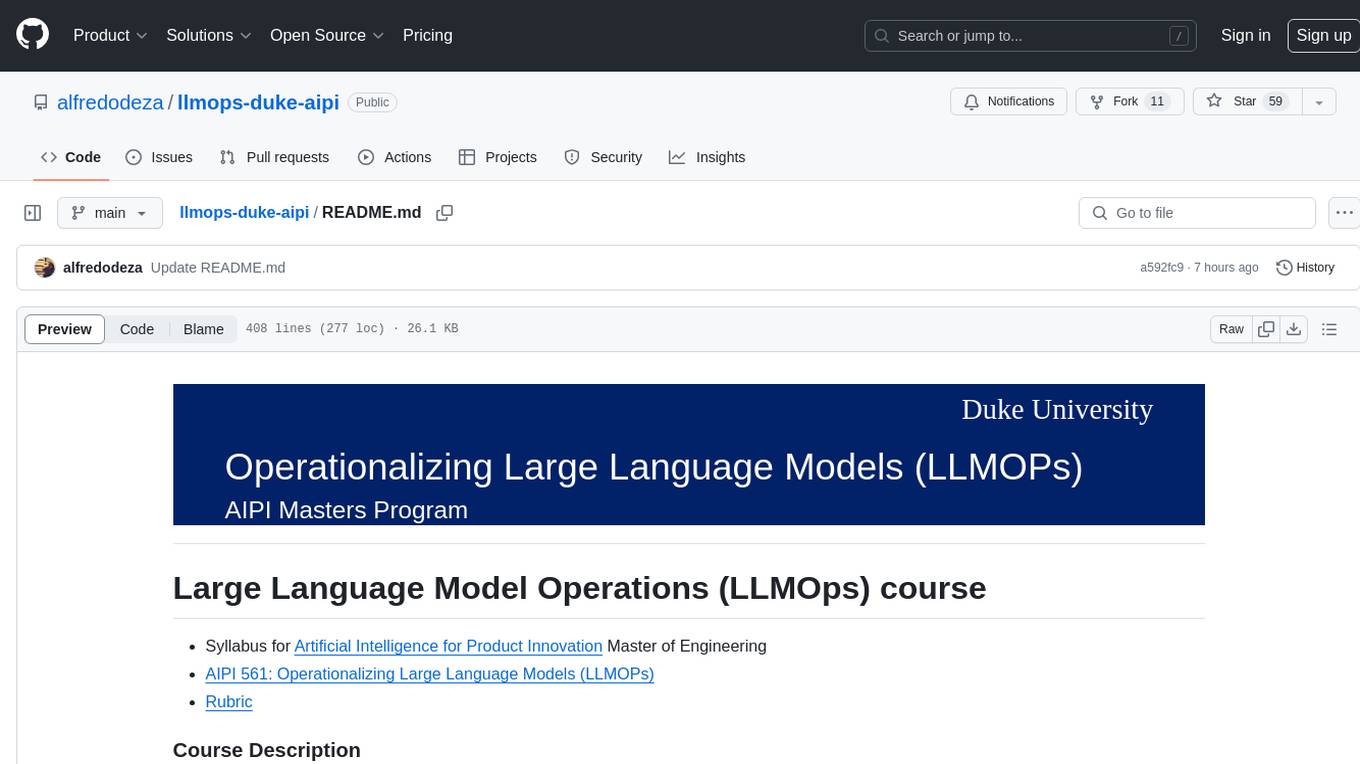
llmops-duke-aipi
LLMOps Duke AIPI is a course focused on operationalizing Large Language Models, teaching methodologies for developing applications using software development best practices with large language models. The course covers various topics such as generative AI concepts, setting up development environments, interacting with large language models, using local large language models, applied solutions with LLMs, extensibility using plugins and functions, retrieval augmented generation, introduction to Python web frameworks for APIs, DevOps principles, deploying machine learning APIs, LLM platforms, and final presentations. Students will learn to build, share, and present portfolios using Github, YouTube, and Linkedin, as well as develop non-linear life-long learning skills. Prerequisites include basic Linux and programming skills, with coursework available in Python or Rust. Additional resources and references are provided for further learning and exploration.
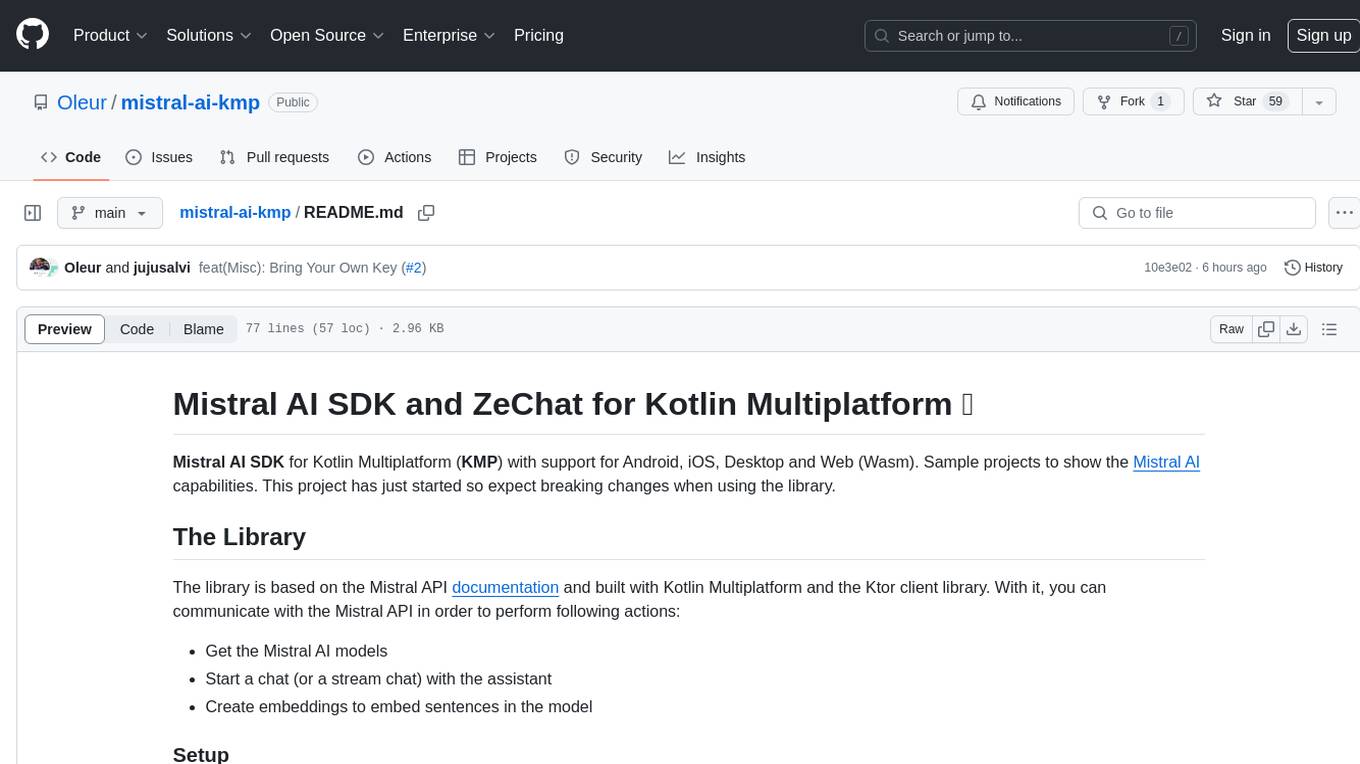
mistral-ai-kmp
Mistral AI SDK for Kotlin Multiplatform (KMP) allows communication with Mistral API to get AI models, start a chat with the assistant, and create embeddings. The library is based on Mistral API documentation and built with Kotlin Multiplatform and Ktor client library. Sample projects like ZeChat showcase the capabilities of Mistral AI SDK. Users can interact with different Mistral AI models through ZeChat apps on Android, Desktop, and Web platforms. The library is not yet published on Maven, but users can fork the project and use it as a module dependency in their apps.
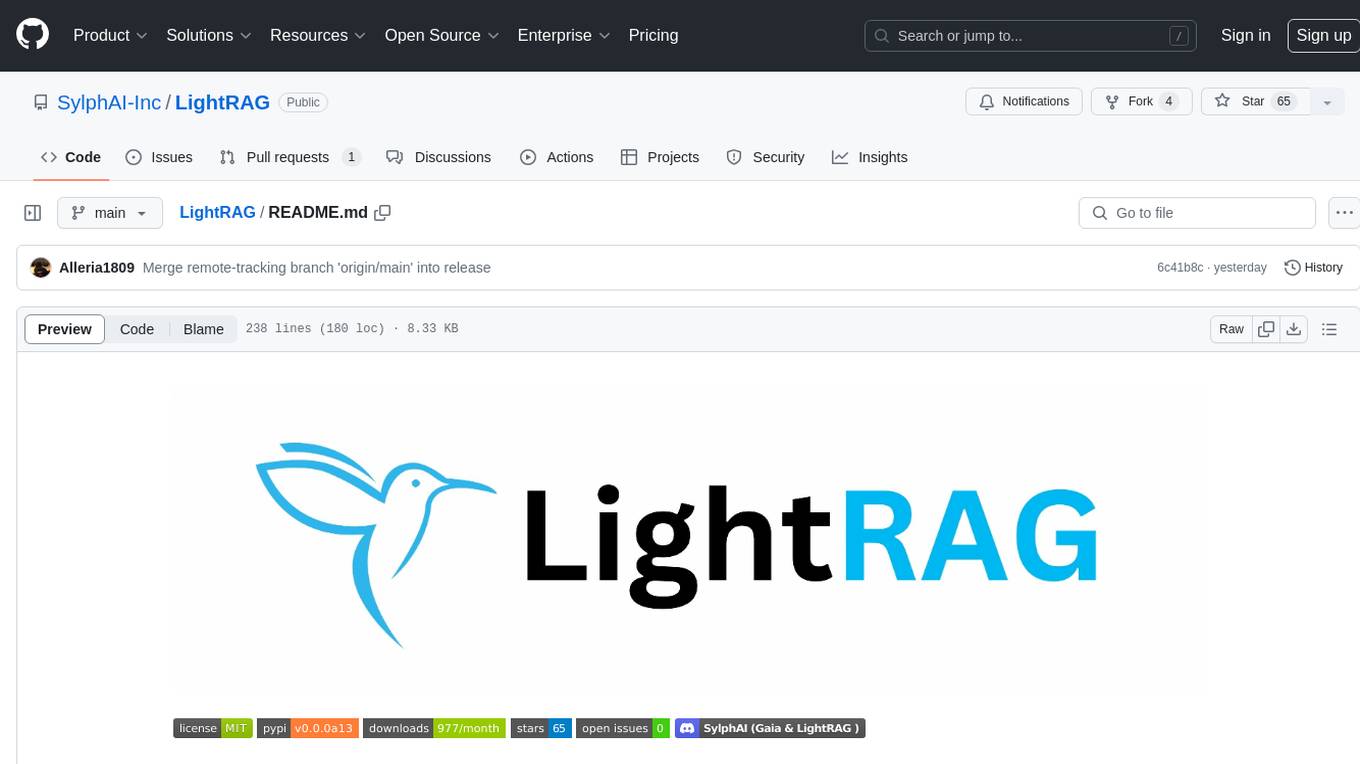
LightRAG
LightRAG is a PyTorch library designed for building and optimizing Retriever-Agent-Generator (RAG) pipelines. It follows principles of simplicity, quality, and optimization, offering developers maximum customizability with minimal abstraction. The library includes components for model interaction, output parsing, and structured data generation. LightRAG facilitates tasks like providing explanations and examples for concepts through a question-answering pipeline.
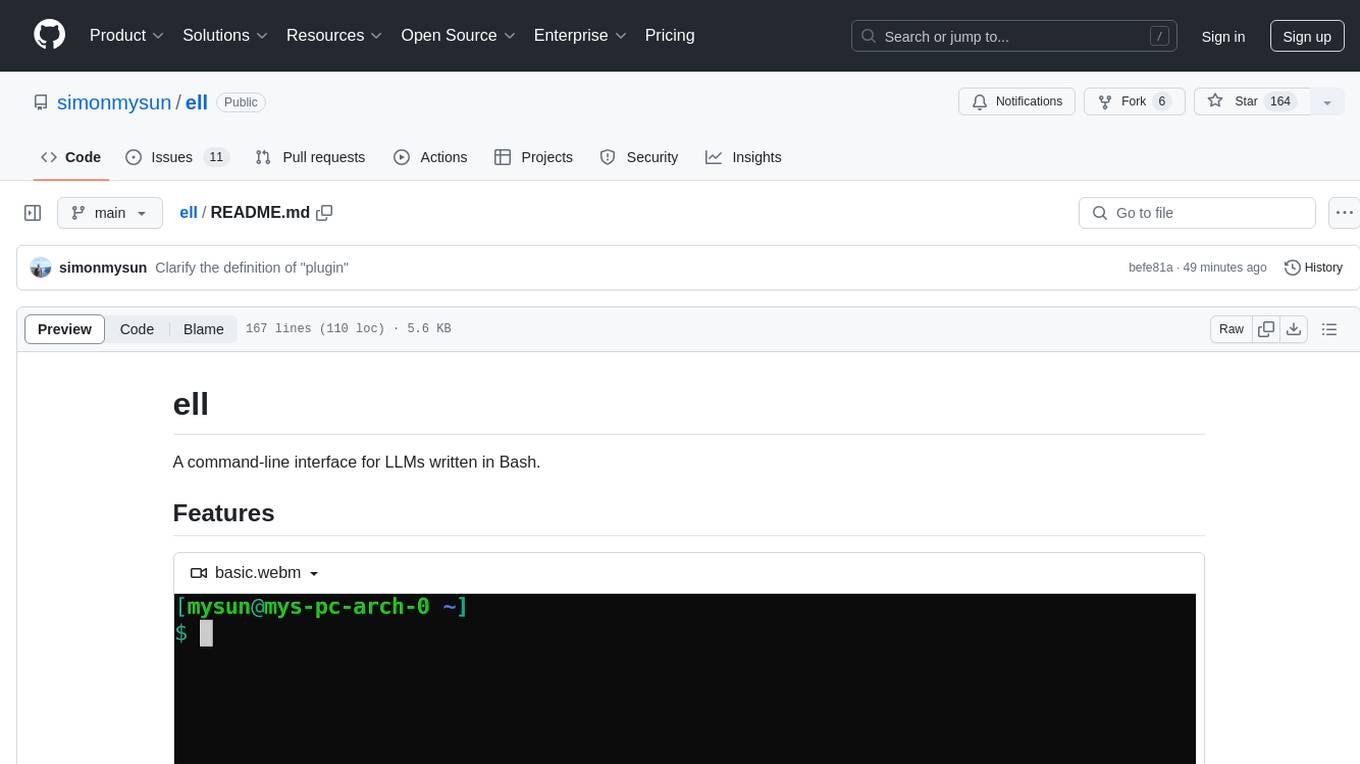
ell
ell is a command-line interface for Language Model Models (LLMs) written in Bash. It allows users to interact with LLMs from the terminal, supports piping, context bringing, and chatting with LLMs. Users can also call functions and use templates. The tool requires bash, jq for JSON parsing, curl for HTTPS requests, and perl for PCRE. Configuration involves setting variables for different LLM models and APIs. Usage examples include asking questions, specifying models, recording input/output, running in interactive mode, and using templates. The tool is lightweight, easy to install, and pipe-friendly, making it suitable for interacting with LLMs in a terminal environment.
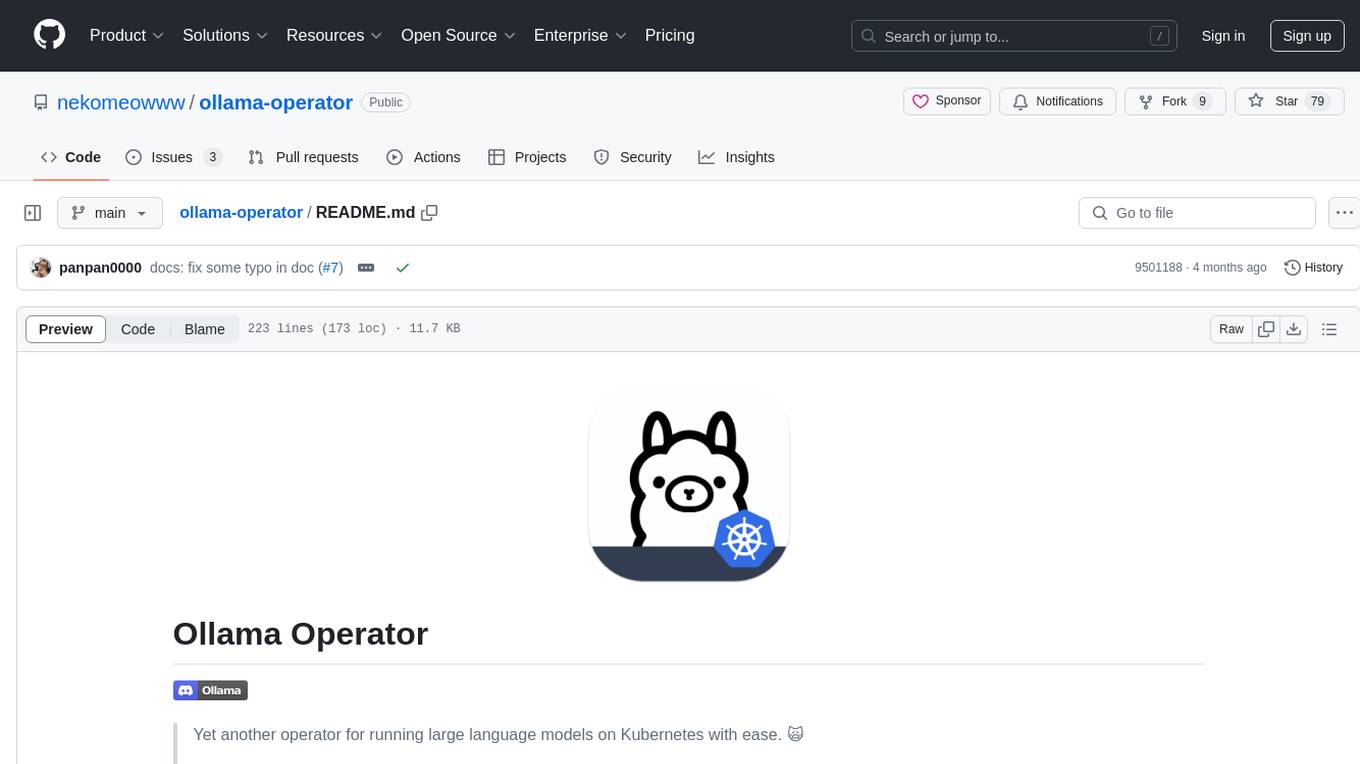
ollama-operator
Ollama Operator is a Kubernetes operator designed to facilitate running large language models on Kubernetes clusters. It simplifies the process of deploying and managing multiple models on the same cluster, providing an easy-to-use interface for users. With support for various Kubernetes environments and seamless integration with Ollama models, APIs, and CLI, Ollama Operator streamlines the deployment and management of language models. By leveraging the capabilities of lama.cpp, Ollama Operator eliminates the need to worry about Python environments and CUDA drivers, making it a reliable tool for running large language models on Kubernetes.
For similar jobs
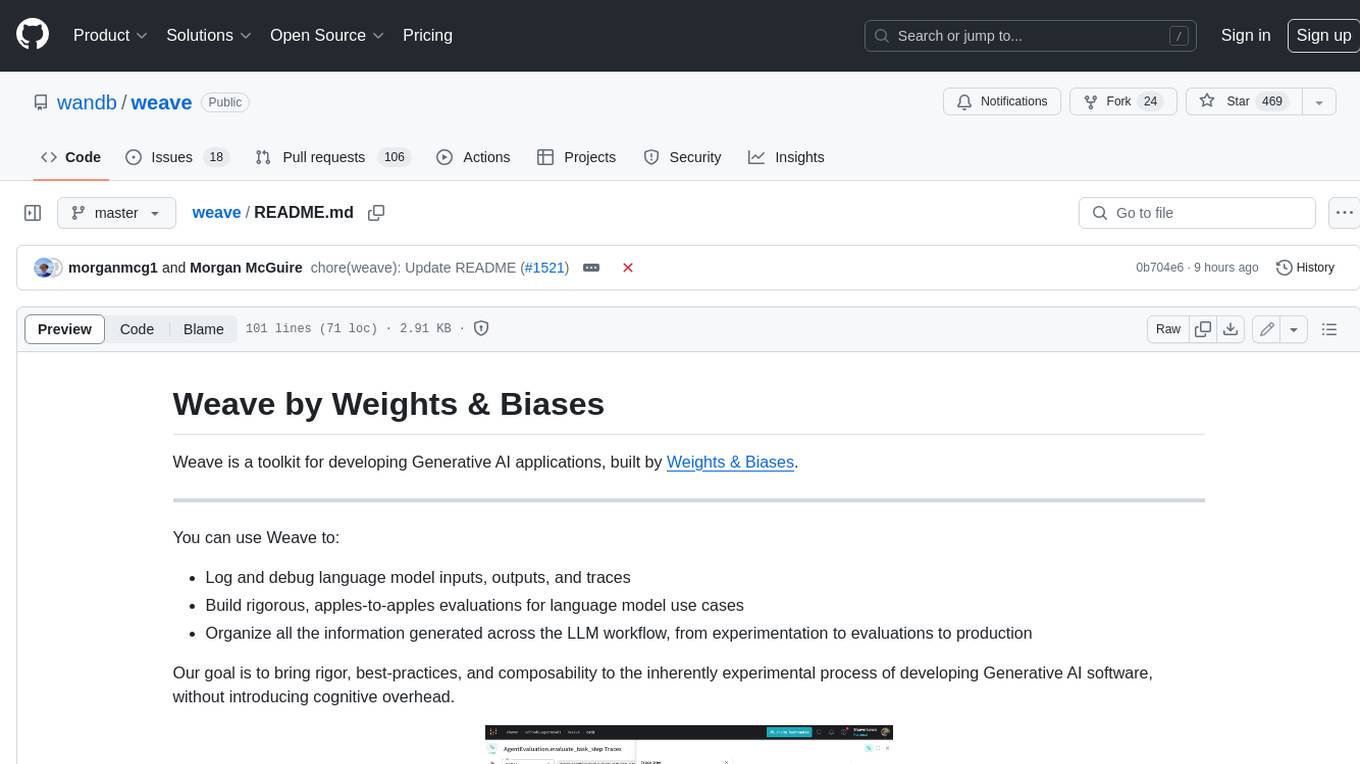
weave
Weave is a toolkit for developing Generative AI applications, built by Weights & Biases. With Weave, you can log and debug language model inputs, outputs, and traces; build rigorous, apples-to-apples evaluations for language model use cases; and organize all the information generated across the LLM workflow, from experimentation to evaluations to production. Weave aims to bring rigor, best-practices, and composability to the inherently experimental process of developing Generative AI software, without introducing cognitive overhead.
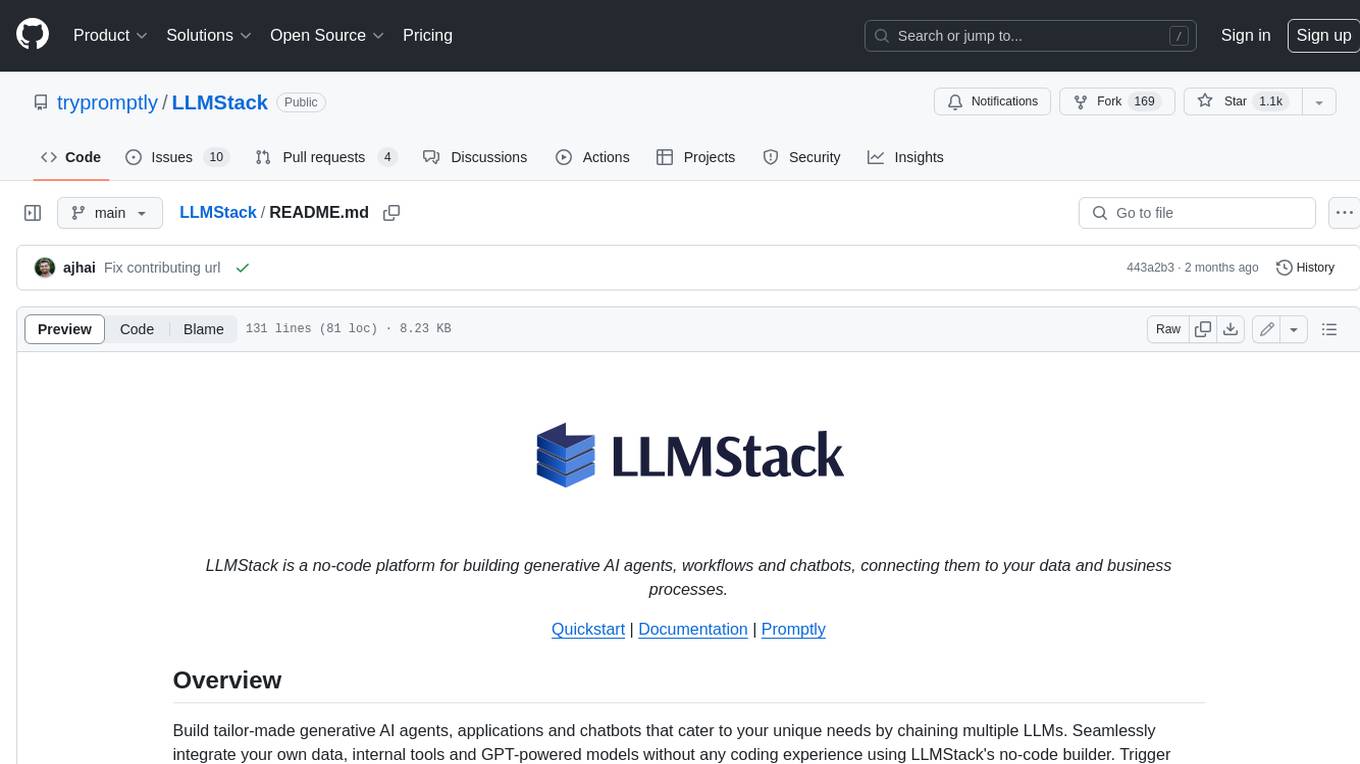
LLMStack
LLMStack is a no-code platform for building generative AI agents, workflows, and chatbots. It allows users to connect their own data, internal tools, and GPT-powered models without any coding experience. LLMStack can be deployed to the cloud or on-premise and can be accessed via HTTP API or triggered from Slack or Discord.
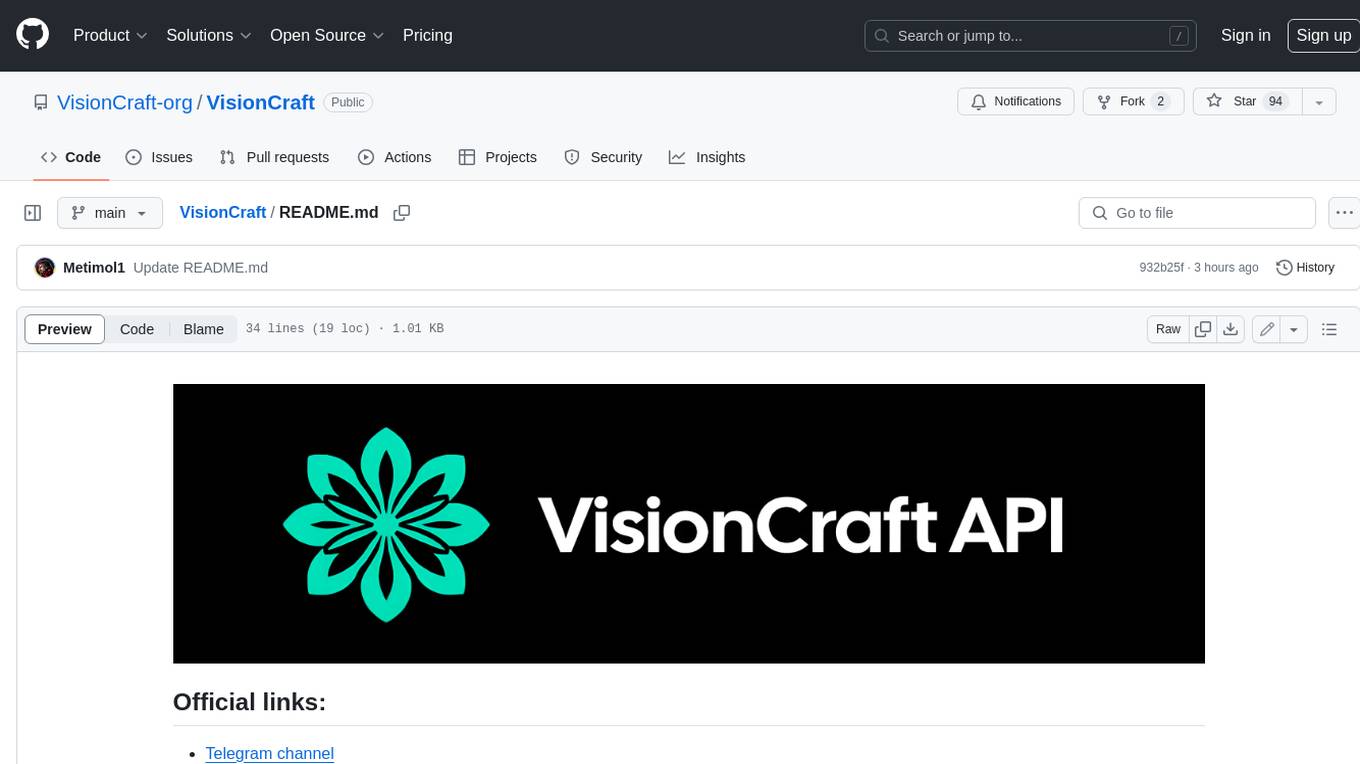
VisionCraft
The VisionCraft API is a free API for using over 100 different AI models. From images to sound.
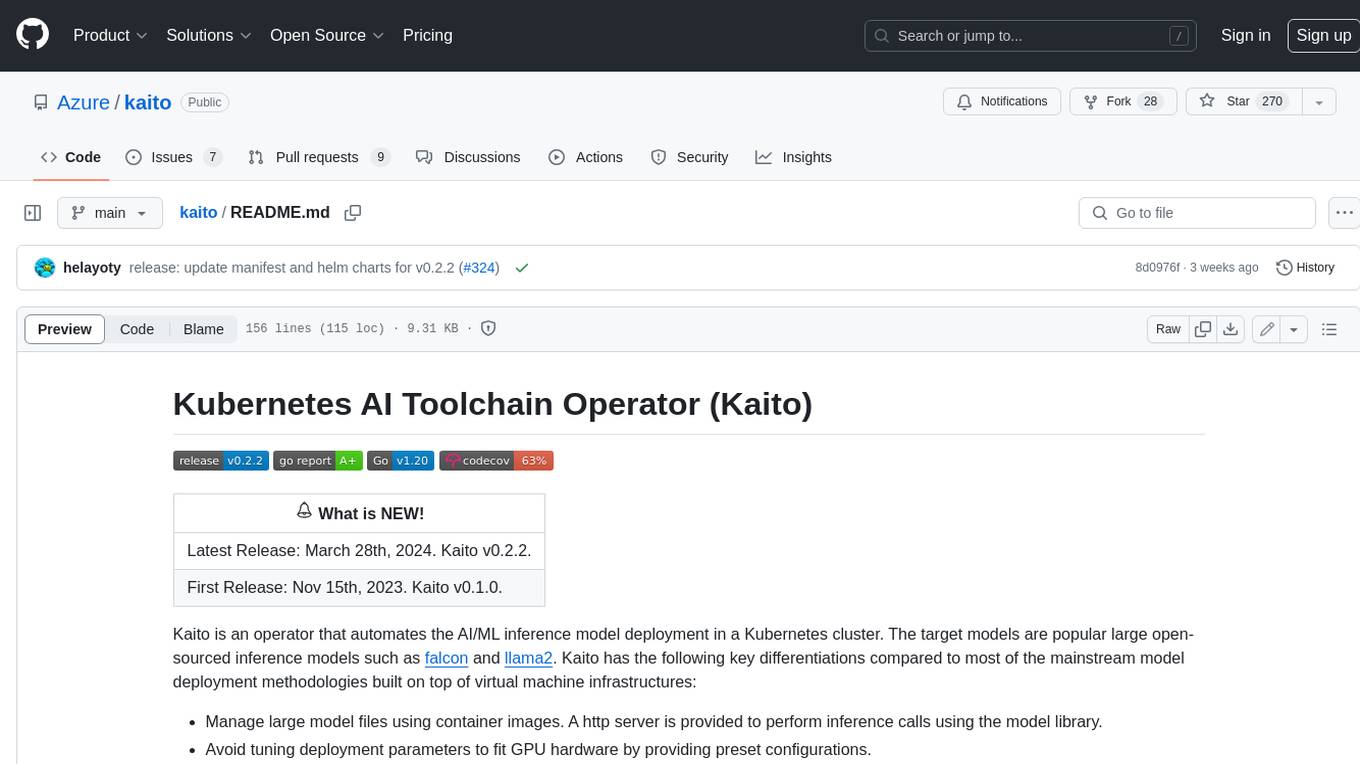
kaito
Kaito is an operator that automates the AI/ML inference model deployment in a Kubernetes cluster. It manages large model files using container images, avoids tuning deployment parameters to fit GPU hardware by providing preset configurations, auto-provisions GPU nodes based on model requirements, and hosts large model images in the public Microsoft Container Registry (MCR) if the license allows. Using Kaito, the workflow of onboarding large AI inference models in Kubernetes is largely simplified.
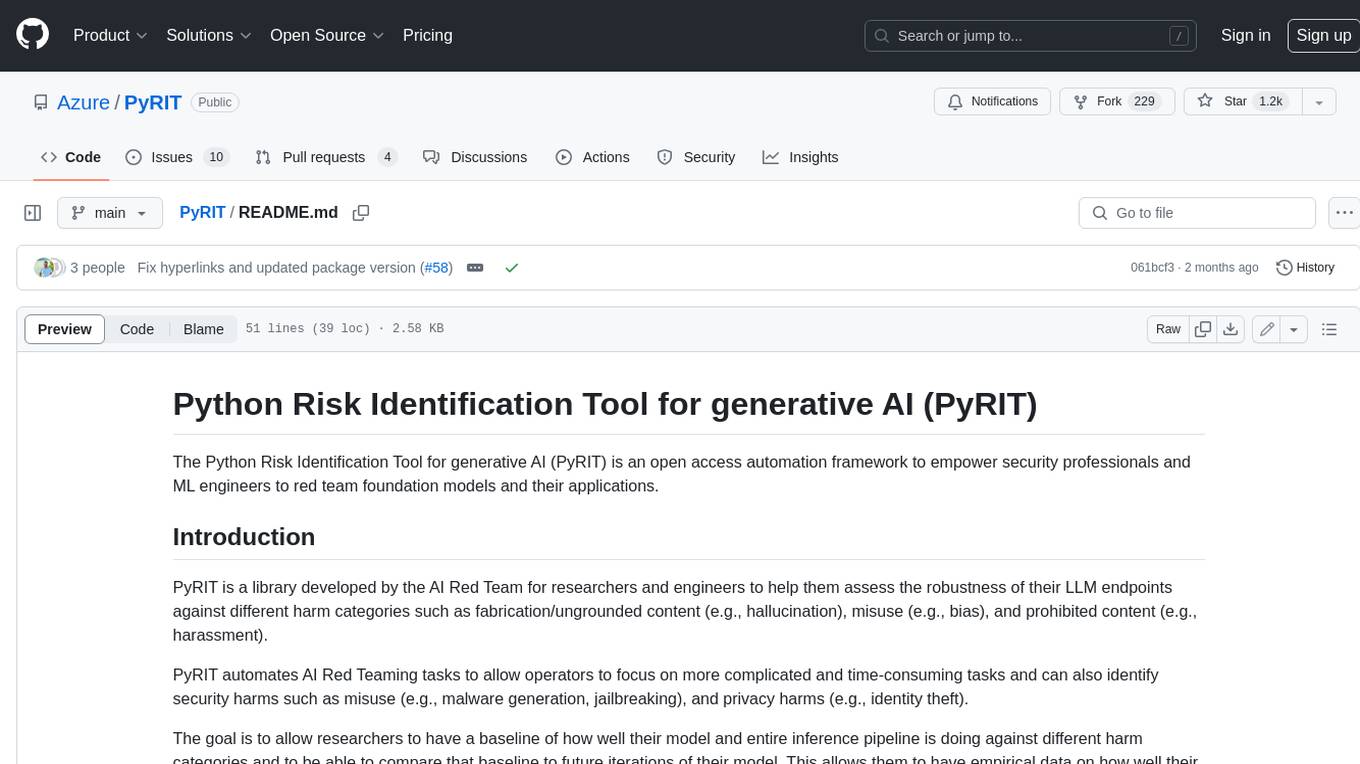
PyRIT
PyRIT is an open access automation framework designed to empower security professionals and ML engineers to red team foundation models and their applications. It automates AI Red Teaming tasks to allow operators to focus on more complicated and time-consuming tasks and can also identify security harms such as misuse (e.g., malware generation, jailbreaking), and privacy harms (e.g., identity theft). The goal is to allow researchers to have a baseline of how well their model and entire inference pipeline is doing against different harm categories and to be able to compare that baseline to future iterations of their model. This allows them to have empirical data on how well their model is doing today, and detect any degradation of performance based on future improvements.
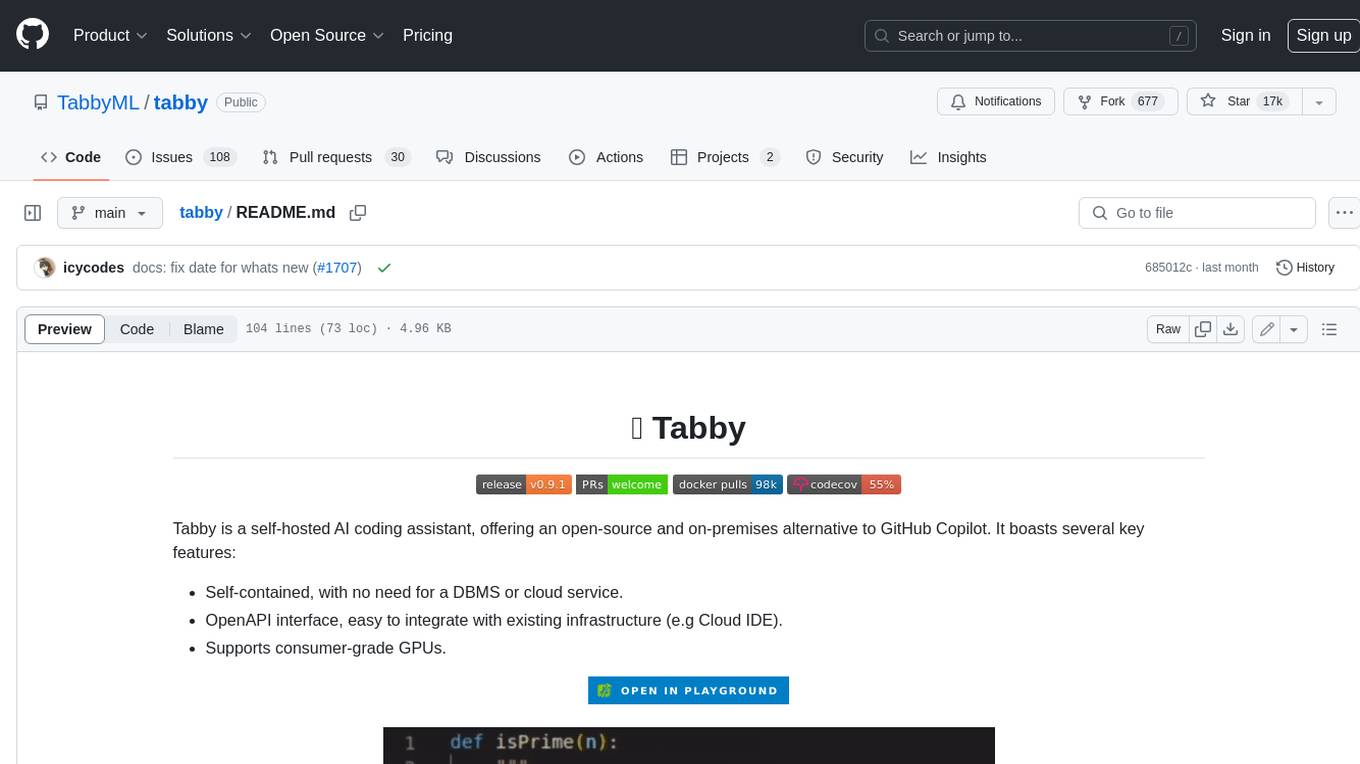
tabby
Tabby is a self-hosted AI coding assistant, offering an open-source and on-premises alternative to GitHub Copilot. It boasts several key features: * Self-contained, with no need for a DBMS or cloud service. * OpenAPI interface, easy to integrate with existing infrastructure (e.g Cloud IDE). * Supports consumer-grade GPUs.
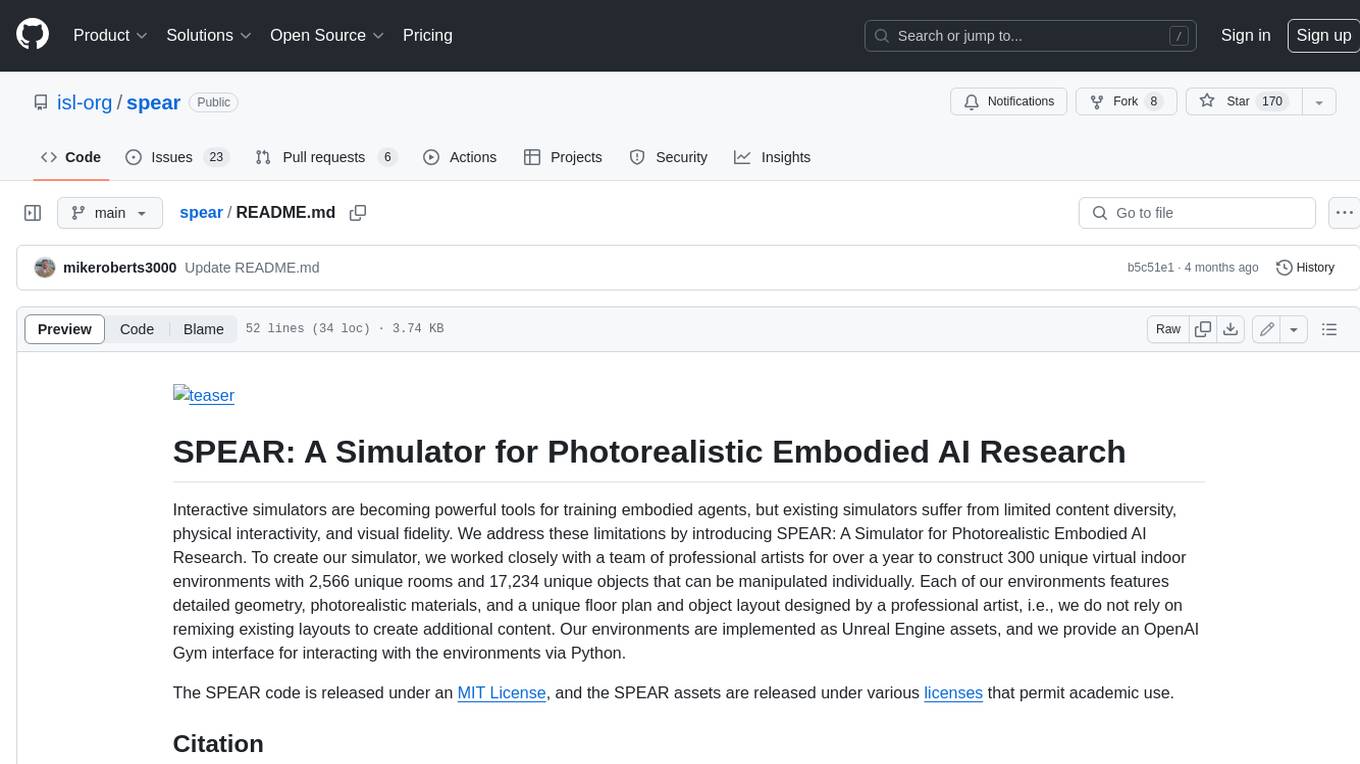
spear
SPEAR (Simulator for Photorealistic Embodied AI Research) is a powerful tool for training embodied agents. It features 300 unique virtual indoor environments with 2,566 unique rooms and 17,234 unique objects that can be manipulated individually. Each environment is designed by a professional artist and features detailed geometry, photorealistic materials, and a unique floor plan and object layout. SPEAR is implemented as Unreal Engine assets and provides an OpenAI Gym interface for interacting with the environments via Python.
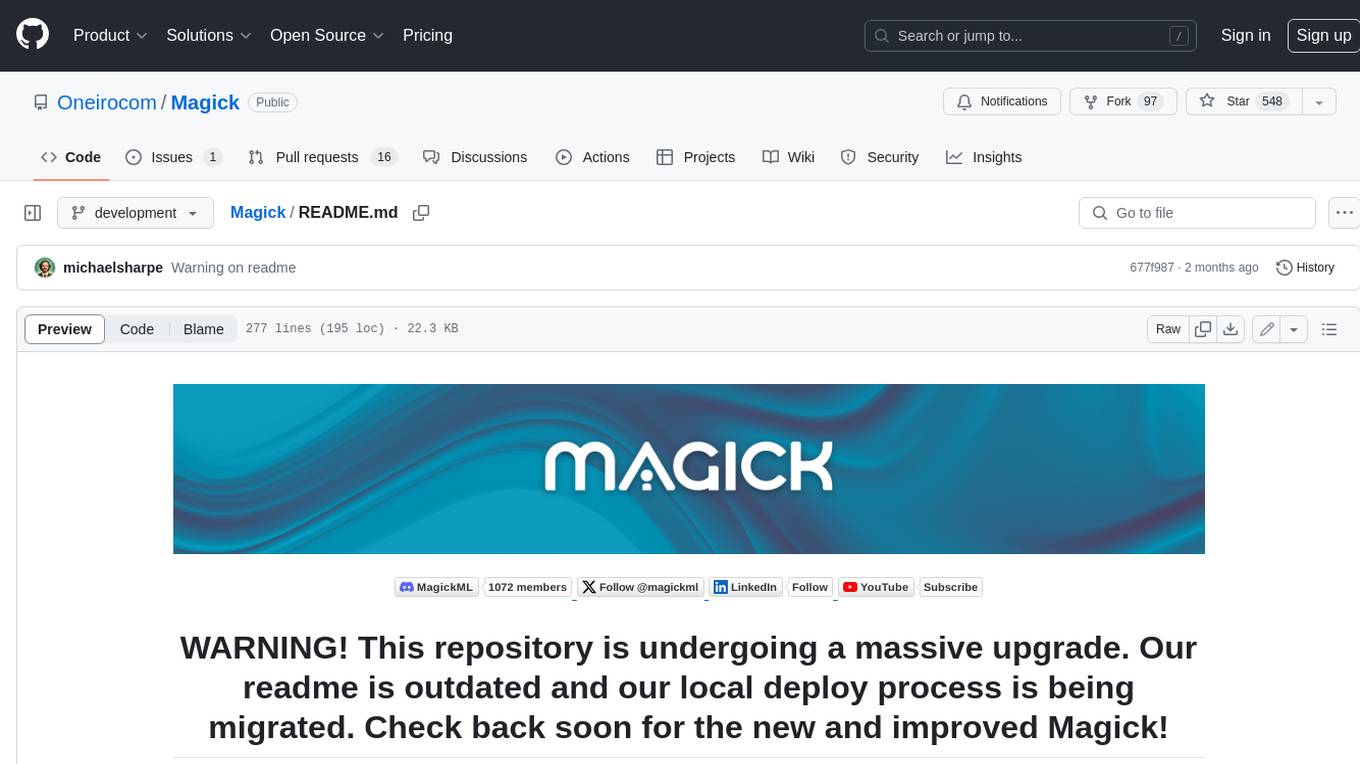
Magick
Magick is a groundbreaking visual AIDE (Artificial Intelligence Development Environment) for no-code data pipelines and multimodal agents. Magick can connect to other services and comes with nodes and templates well-suited for intelligent agents, chatbots, complex reasoning systems and realistic characters.