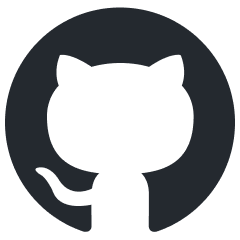
llmops-duke-aipi
Official curricula for the LLMOPs course at Duke University
Stars: 73
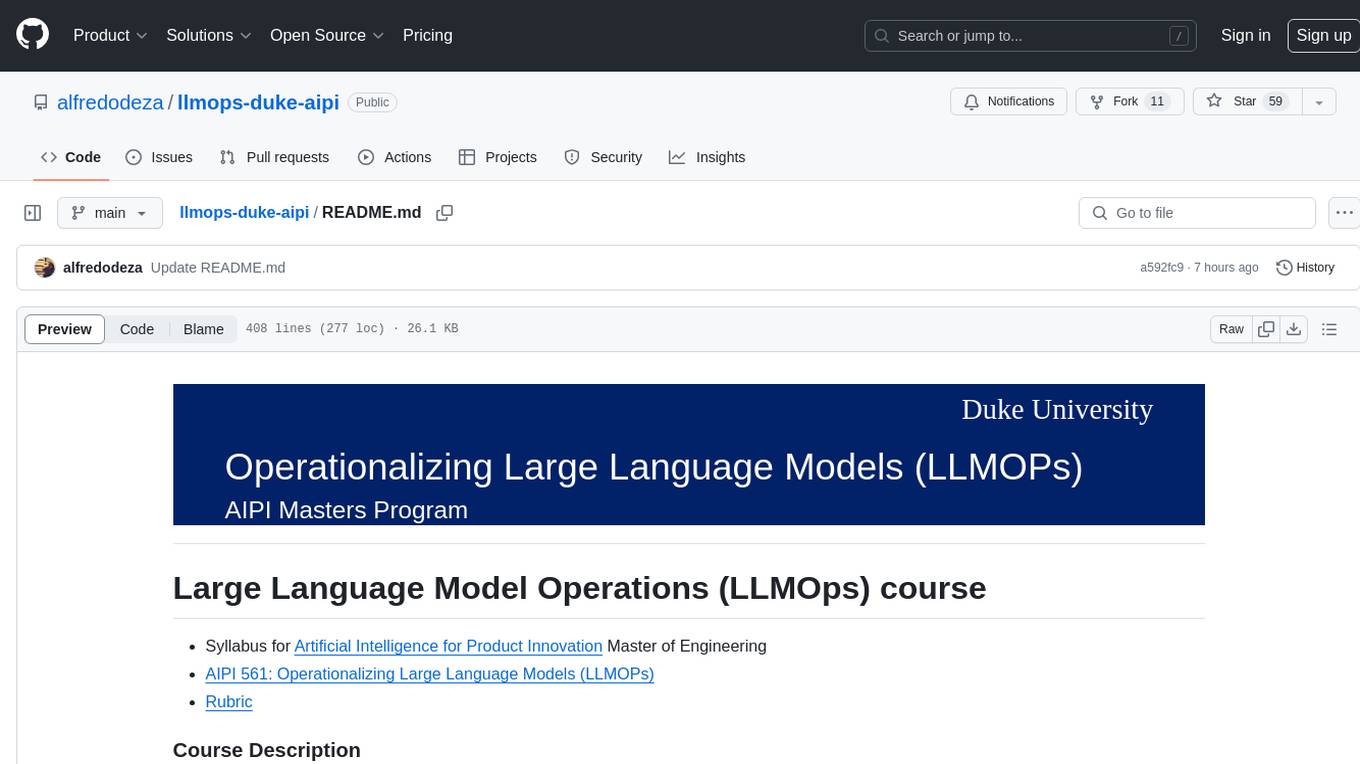
LLMOps Duke AIPI is a course focused on operationalizing Large Language Models, teaching methodologies for developing applications using software development best practices with large language models. The course covers various topics such as generative AI concepts, setting up development environments, interacting with large language models, using local large language models, applied solutions with LLMs, extensibility using plugins and functions, retrieval augmented generation, introduction to Python web frameworks for APIs, DevOps principles, deploying machine learning APIs, LLM platforms, and final presentations. Students will learn to build, share, and present portfolios using Github, YouTube, and Linkedin, as well as develop non-linear life-long learning skills. Prerequisites include basic Linux and programming skills, with coursework available in Python or Rust. Additional resources and references are provided for further learning and exploration.
README:
- Syllabus for Artificial Intelligence for Product Innovation Master of Engineering
- AIPI 561: Operationalizing Large Language Models (LLMOPs)
- Rubric
In this course, you will learn about the methodologies involved in operationalizing Large Language Models. By the end of the course, you will be able to develop applications that use software development best practices with large language models.
Faculty
Table of Contents
- Prerequisites
- Week 1: Introduction to Generative AI Concepts
- Week 2: Setup Development Environment
- Week 3: Interacting with Large Language Models
- Week 4: Using Local Large Language Models
- Week 5: Applied Solutions with LLMs
- Week 6: Extensibility Using Plugins and Functions
- Week 7: Using Retrieval Augmented Generation
- Week 8: Introduction to Python Web Frameworks for APIs
- Week 9: DevOps Principles
- Week 10: Deploying Machine Learning APIs
- Week 11: LLM Platforms
- Week 12: Final Presentations
By the end of the course, you will be able to:
- Build Large Language Model (LLM) or Small Language Model (SLM) solutions
- Build, share and present compelling portfolios using: Github, YouTube, and Linkedin.
- Setup a provisioned project environment
- Develop a non-linear life-long learning skill
[!NOTE] Diversity Statement: As educators and learners, we must share a commitment to diversity and equity, removing barriers to education so that everyone may participate fully in the community. In this course, we respect and embrace the unique experiences that brought each person here, including backgrounds, identities, learning styles, ways of expression, and academic interests. The broad spectrum of perspectives represented by our students enrich everyone’s experiences, and we strive to meet each perspective with openness and respect.
This course requires basic Linux and programming skills. You can complete all coursework with either Python or Rust. Use the following recommendations to get up to speed on these skills.
Linux If students lack basic Linux skills it is recommended to take this course: Duke+Coursera: Python, Bash and SQL Essentials for Data Engineering Specialization
[!IMPORTANT] You don't need both programming languages for this course, you can pick either or.
Python
These two modules from the Introduction to Python course covers the basics of Python:
- Python Essentials for MLOps-Week1-Introduction to Python
- Python Essentials for MLOps-Week2-Python Functions and Classes
Rust
This course on Rust Fundamentals covers the basics of the Rust Programming Language.
Although this course is based on the Large Language Model Operations (LLMOPs) specialization, you can use these additional resources as supporting material:
Courses:
- Duke+Coursera: Large Language Model Operations (LLMOps) Specialization
- Duke+Coursera: MLOps, Machine Learning Operations Specialization
- Rust Programming Specialization
- Duke+Coursera: Cloud Computing for Data Coursera Course
Books:
These are all the resources you need for this course.
[!IMPORTANT] You are not required to watch and read every single resource. You aren't graded on consumption of content or memorization of facts. Use the content as support for your project.
Rust:
Python:
- Python Essentials for MLOps-Week1-Introduction to Python
- Python Essentials for MLOps-Week2-Python Functions and Classes
- Python Essentials for MLOps-Week4-Introduction to Pandas and NumPy
- Introduction to Generative AI
- Public Speaking
- Developing Effective Technical Communication
- Exploring Cloud Onboarding
Weekly Demo video prompt: Discuss your plan and idea to build out your individual project and how you will do them on time. Use the Public Speaking guidelines to make sure you are delivering great demos.
Python:
- Python Development Environments_
- Pytest Master Class
- Python Essentials for MLOps-Week5-Applied Python for MLOps
Rust:
Weekly Demo video prompt: Describe your programming language choice and what are some of the advantages and potential pitfalls.
- Interacting with models
- Building robust Generative AI systems
- Introduction to MLOps Walkthrough
- MLOPs Foundations: Chapter 2 Walkthrough of Practical MLOps
- Chapter 1: Introduction to MLOps
- Chapter 2. MLOps Foundations
Weekly Demo video prompt: Explain what are some challenges for your application when working with Large Language Model output and what you will do to mitigate them.
- Beginning Llamafile for Local Large Language Models (LLMs)
- Getting Started with Open Source Ecosystem
- Foundations of Local Large Language Models
Weekly Demo video prompt: Describe your evaluation of the different Local Large Language and Small Language Models available with Llamafile and which one would work best for your project.
- Local LLMOps
- AI Pair Programming from CodeWhisperer to Prompt Engineering
- Using Local LLMs from Llamafile to Whisper.cpp
- Open Source Platforms for MLOps-Week2-Introduction to Hugging Face
- Open Source Platforms for MLOps-Week3-Deploying Hugging Face
- Open Source Platforms for MLOps-Week4-Applied Hugging Face
Weekly Demo video prompt: Explain the architectural overview of your application and what are some challenges with this type of design.
- Extending with Functions and Plugins
- Applications of LLMs
- MLOps Platforms: Amazon SageMaker and Azure ML-Week1
Weekly Demo video prompt: What are some benefits of adding plugins and functions to your application idea? What would be a challenge in a production environment?
Weekly Demo video prompt: How would adding RAG to your application affect the overall experience for an end user? Why or why wouldn't you consider using RAG in your application?
- Introduction to FastAPI Framework
- Introduction to Flask Framework
- Applied Python for MLOPs
- Cloud Virtualization, Containers and APIs
Weekly Demo video prompt: What are some benefits of using the framework you chose? Describe how you will make it work with your application.
- Responsible Generative AI
- Applying DevOps Principles
- MLOps Platforms: Amazon SageMaker and Azure ML-Week2
- MLOps Platforms: Amazon SageMaker and Azure ML-Week3
Weekly Demo video prompt: How would you apply DevOps, Responsible AI Principles, and automation for your application? What are some challenges you may face?
Weekly Demo video prompt: What are some difficulties of using automation? How will using automation benefit your application?
- Introduction to LLMOps with Azure
- mlflow-project-best-practices
- MLOps Platforms From Zero: Databricks, MLFlow/MLRun/SKLearn
- Azure Databricks, Pandas, and Opendatasets
- MLOps Platforms: Amazon SageMaker and Azure ML-Week5
- MLOps Platforms: Amazon SageMaker and Azure ML-Week4
Weekly Demo video prompt: How would adding a cloud platform enable your application? What are some potential drawbacks in using an LLM platform?
- Turn in Final Project
This class is based on a single and individual class project. You will start work on this project from Week 1 and will deliver the completed project with final presentation on Week 12.
See the rubric for more details.
[!IMPORTANT] Do not build a model yourself. Reuse a LLM or an SLM model. Use Mozilla Llamafile as a reference.
The primary two resources you will need to build an LLM solution using a local API are these:
- Beginning Llamafile for Local Large Language Models (LLMs)
- Getting Started with Open Source Ecosystem
- Python MLOps Cookbook
- databricks-zero-to-mlops
- Python Fire
- Refactoring a Python script into a library called by Python Click CLI
- Container Continuous Delivery
- Functions to Containerized Microservice Continuous Delivery to AWS App Runner with Fast API
- mlflow-project-best-practices
- databricks-zero-to-mlops
- Python MLOps Cookbook
- Edge Computer Vision
- Github Codespaces
- AWS Academy
- Azure for Students
- Google Qwiklabs
- Practical MLOps
- Pragmatic AI
- AWS Bootcamp
- Gift, N (2020) Python for DevOps Sebastopol, CA: O'Reilly.
- Gift, N (2021) Practical MLOps, Sebastopol, CA: O'Reilly
- Gift, N (2021) Cloud Computing for Data Analysis
- Gift, N (2020) Pragmatic AI: An Introduction to Cloud-Based Machine Learning
- AWS Training & Certification
- AWS Educate
- AWS Academy
- Google Qwiklabs - Hands-On Cloud Training
- Coursera
- Microsoft Learn
- edX
- Applied Computer Vision with Python Lectures: https://learning.oreilly.com/videos/applied-computer-vision/60652VIDEOPAIMLL/
- Learn Python in One Hour: https://learning.oreilly.com/videos/learn-python-in/60645VIDEOPAIML/
- Cloud Computing with Python: https://learning.oreilly.com/videos/cloud-computing-with/60650VIDEOPAIML/
- Python for Data Science with Colab and Pandas in One Hour: https://learning.oreilly.com/videos/python-for-data/62062021VIDEOPAIML/
- GCP Cloud Functions:
https://learning.oreilly.com/videos/learn-gcp-cloud/50101VIDEOPAIML/ - Azure AutoML
https://learning.oreilly.com/videos/learn-azure-ml/50104VIDEOPAIML/
- AWS Cloud Practitioner
- AWS ML
- AWS SA
-
Building AI Applications with GCP: https://learning.oreilly.com/videos/building-ai-applications/9780135973462/
-
Build GCP Cloud Functions:
https://learning.oreilly.com/videos/learn-gcp-cloud/50101VIDEOPAIML/
- Data Science, Pandas, and Colab
https://learning.oreilly.com/videos/python-for-data/62062021VIDEOPAIML/
- Python and DevOps
https://learning.oreilly.com/videos/python-devops-in/61272021VIDEOPAIML/ - Python Command-line Tools
https://learning.oreilly.com/videos/learn-python-command-line/50102VIDEOPAIML/
-
Docker containers:
https://learning.oreilly.com/videos/learn-docker-containers/50103VIDEOPAIML/ -
Learn the Vim Text Editor:
https://learning.oreilly.com/videos/learn-vim-in/50100VIDEOPAIML/
Grading and feedback turnaround will be one week from the due date. You will be notified if the turnaround will be longer than one week.
The discussion forums, written assignments, demo videos, and final project will be graded based on specific criteria or a rubric. The criteria or rubric for each type of assessment will be available in the course. To view the discussion forum rubric, click the gear icon in the upper right corner of the page and choose Show Rubric. The Written Assignment Rubric and Final Project Rubric will automatically appear on the page.
Late work will be accepted only in the event of an instructor-approved absence. Contact your instructor as soon as possible, at least 24 hours in advance.
The purpose of the discussion boards is to allow students to freely exchange ideas. It is imperative to remain respectful of all viewpoints and positions and, when necessary, agree to respectfully disagree. While active and frequent participation is encouraged, cluttering a discussion board with inappropriate, irrelevant, or insignificant material will not earn additional points and may result in receiving less than full credit. Frequency matters, but contributing content that adds value is paramount. Please remember to cite all sources—when relevant—in order to avoid plagiarism. Please post your viewpoints first and then discuss others’ viewpoints.
The quality of your posts and how others view and respond to them are the most valued. A single statement mostly implying “I agree” or “I do not agree” is not counted as a post. Explain, clarify, politely ask for details, provide details, persuade, and enrich communications for a great discussion experience. Please note, there is a requirement to respond to at least two fellow class members’ posts. Also, remember to cite all sources—when relevant—in order to avoid plagiarism.
Beyond interacting with your instructor and peers in discussions, you will be expected to communicate by Canvas message, email, and sync session. Your instructor may also make themselves available by phone or text. In all contexts, keep your communication professional and respect the instructor’s posted availability.
Just as you expect a response when you send a message to your instructor, please respond promptly when your instructor contacts you. Your instructor will expect a response within two business days. This will require that you log into the course site regularly and set up your notifications to inform you when the instructor posts an announcement, provides feedback on work or sends you a message.
This course will not meet at a particular time each week. All course goals, session learning objectives, and assessments are supported through classroom elements that can be accessed at any time. To measure class participation (or attendance), your participation in threaded discussion boards is required, graded, and paramount to your success in this course. Please note that any scheduled synchronous meetings are optional. While your attendance is highly encouraged, it is not required and you will not be graded on your attendance or participation.
This course will involve a number of different types of interactions. These interactions will take place primarily through Microsoft Teams. Please take the time to navigate through the course and become familiar with the course syllabus, structure, and content and review the list of resources below.
Students in an online program should be able to do the following:
- Communicate Teams discussion forums.
- Use web browsers and navigate the World Wide Web.
- Use the learning management system Teams.
- Use Teams
- Use applications to create documents and presentations (e.g., Microsoft Word, PowerPoint).
- Use applications to share files (e.g., Box, Google Drive).
In order to be successful in an online course, students should be able to locate, evaluate, apply, create, and communicate information using technology.
Students in this online course should be able to do the following:
- Create, name, compose, upload, and attach documents.
- Download, modify, upload, attach document templates.
- Create, name, design, and upload presentations.
- Access and download Course Reserve readings; read and review PDF documents.
- Access and use a digital textbook.
- Record and upload video taken with a webcam or smartphone.
- Use the library website for scholarly research tasks.
- Search the Internet strategically and assess the credibility of Internet sources.
- Participate in threaded discussions by contributing text responses, uploading images, sharing links.
- Coordinate remote work with peers, which may include contacting each other by e-mail, phone, video conference, or shared document.
- Edit and format pages in the course site using a WYSIWIG (What You See is What You Get) editor or basic HTML.
- Using a quizzing tool to answer multiple choice, true/false, matching, and short response questions within a given time period.
- Follow directions to engage with a remote proctor by text, webcam, and audio.
- Use a video player to review content, including pausing and restarting video.
You may want to complement this course with the following certifications:
For Tasks:
Click tags to check more tools for each tasksFor Jobs:
Alternative AI tools for llmops-duke-aipi
Similar Open Source Tools
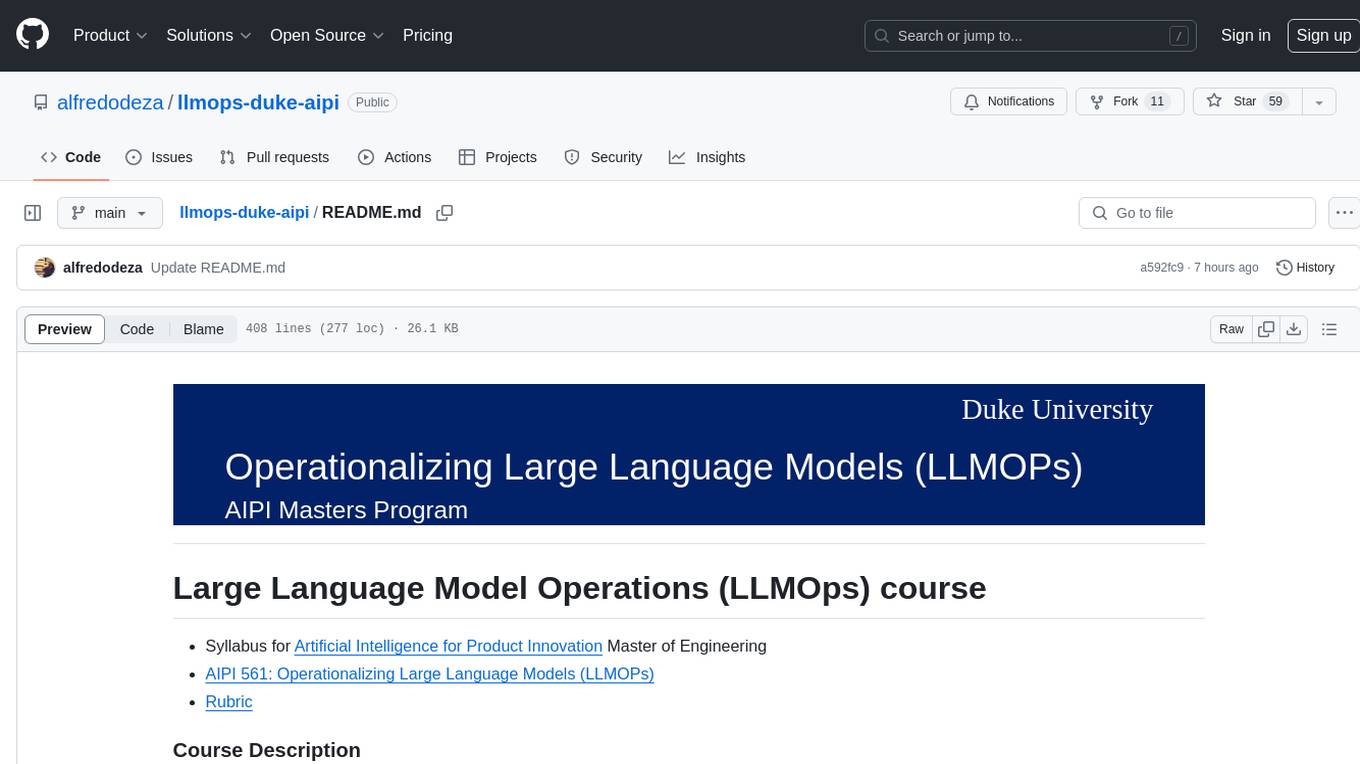
llmops-duke-aipi
LLMOps Duke AIPI is a course focused on operationalizing Large Language Models, teaching methodologies for developing applications using software development best practices with large language models. The course covers various topics such as generative AI concepts, setting up development environments, interacting with large language models, using local large language models, applied solutions with LLMs, extensibility using plugins and functions, retrieval augmented generation, introduction to Python web frameworks for APIs, DevOps principles, deploying machine learning APIs, LLM platforms, and final presentations. Students will learn to build, share, and present portfolios using Github, YouTube, and Linkedin, as well as develop non-linear life-long learning skills. Prerequisites include basic Linux and programming skills, with coursework available in Python or Rust. Additional resources and references are provided for further learning and exploration.
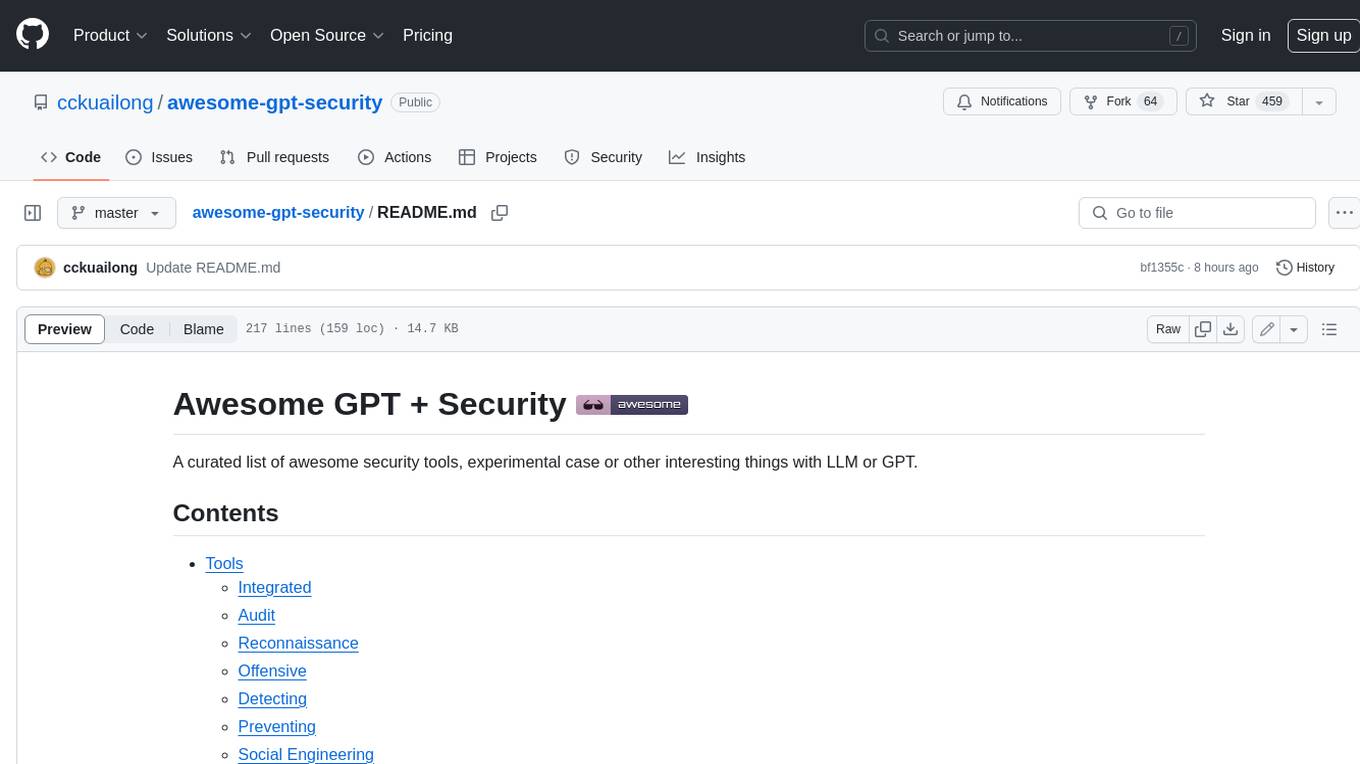
awesome-gpt-security
Awesome GPT + Security is a curated list of awesome security tools, experimental case or other interesting things with LLM or GPT. It includes tools for integrated security, auditing, reconnaissance, offensive security, detecting security issues, preventing security breaches, social engineering, reverse engineering, investigating security incidents, fixing security vulnerabilities, assessing security posture, and more. The list also includes experimental cases, academic research, blogs, and fun projects related to GPT security. Additionally, it provides resources on GPT security standards, bypassing security policies, bug bounty programs, cracking GPT APIs, and plugin security.
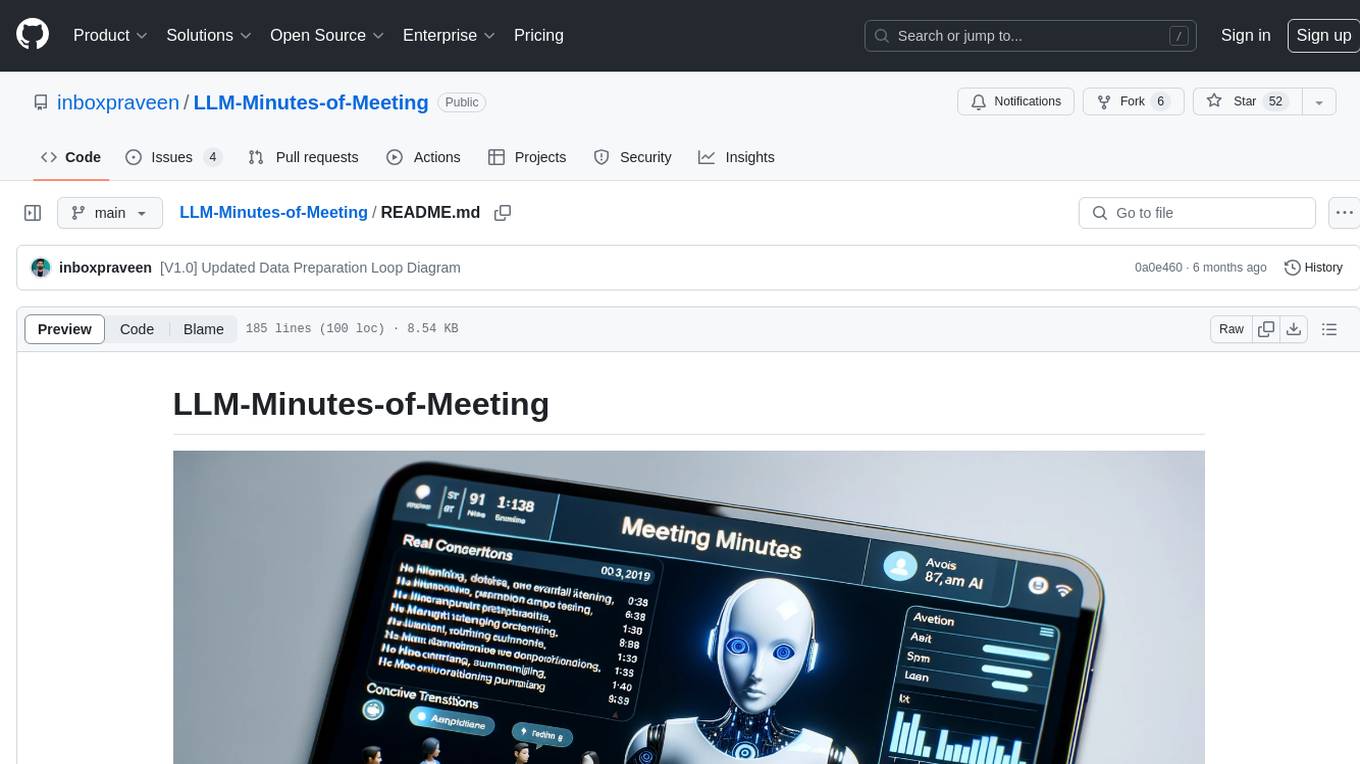
LLM-Minutes-of-Meeting
LLM-Minutes-of-Meeting is a project showcasing NLP & LLM's capability to summarize long meetings and automate the task of delegating Minutes of Meeting(MoM) emails. It converts audio/video files to text, generates editable MoM, and aims to develop a real-time python web-application for meeting automation. The tool features keyword highlighting, topic tagging, export in various formats, user-friendly interface, and uses Celery for asynchronous processing. It is designed for corporate meetings, educational institutions, legal and medical fields, accessibility, and event coverage.
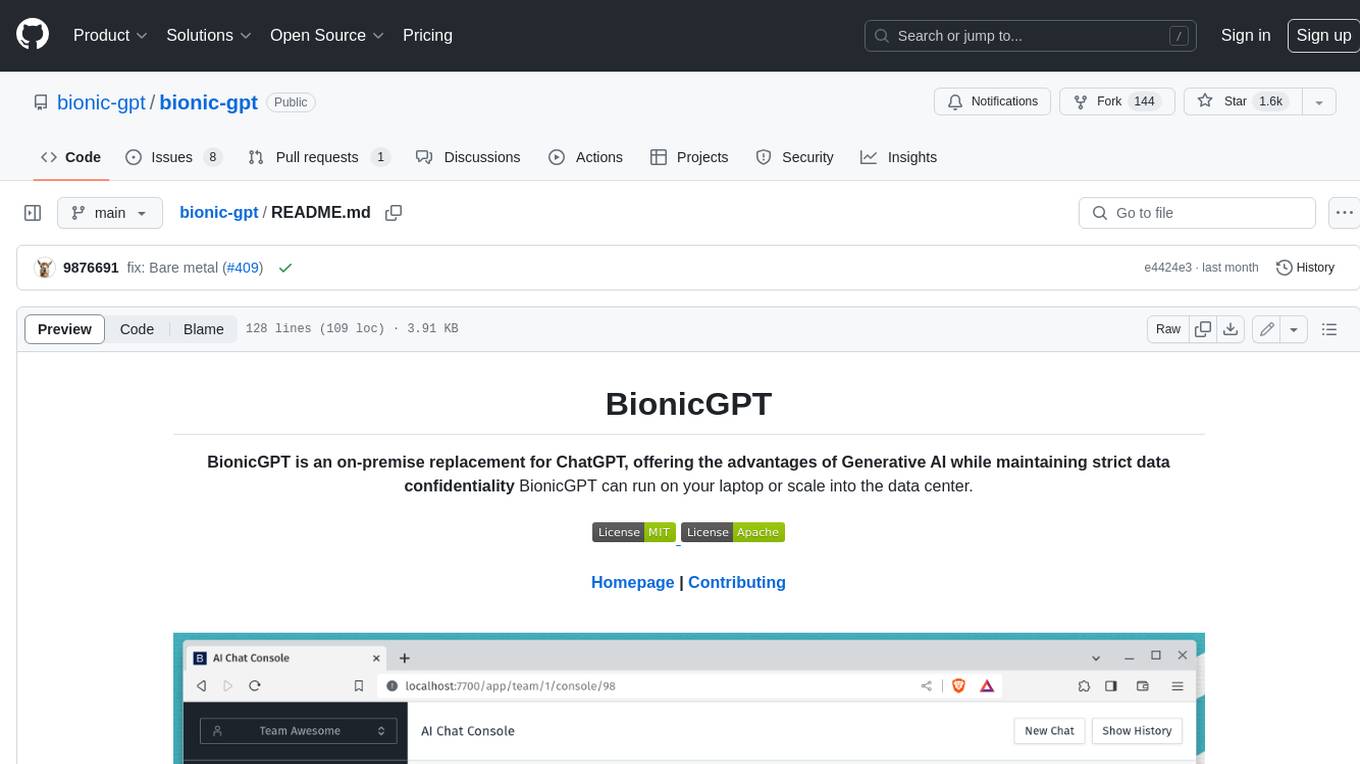
bionic-gpt
BionicGPT is an on-premise replacement for ChatGPT, offering the advantages of Generative AI while maintaining strict data confidentiality. BionicGPT can run on your laptop or scale into the data center.
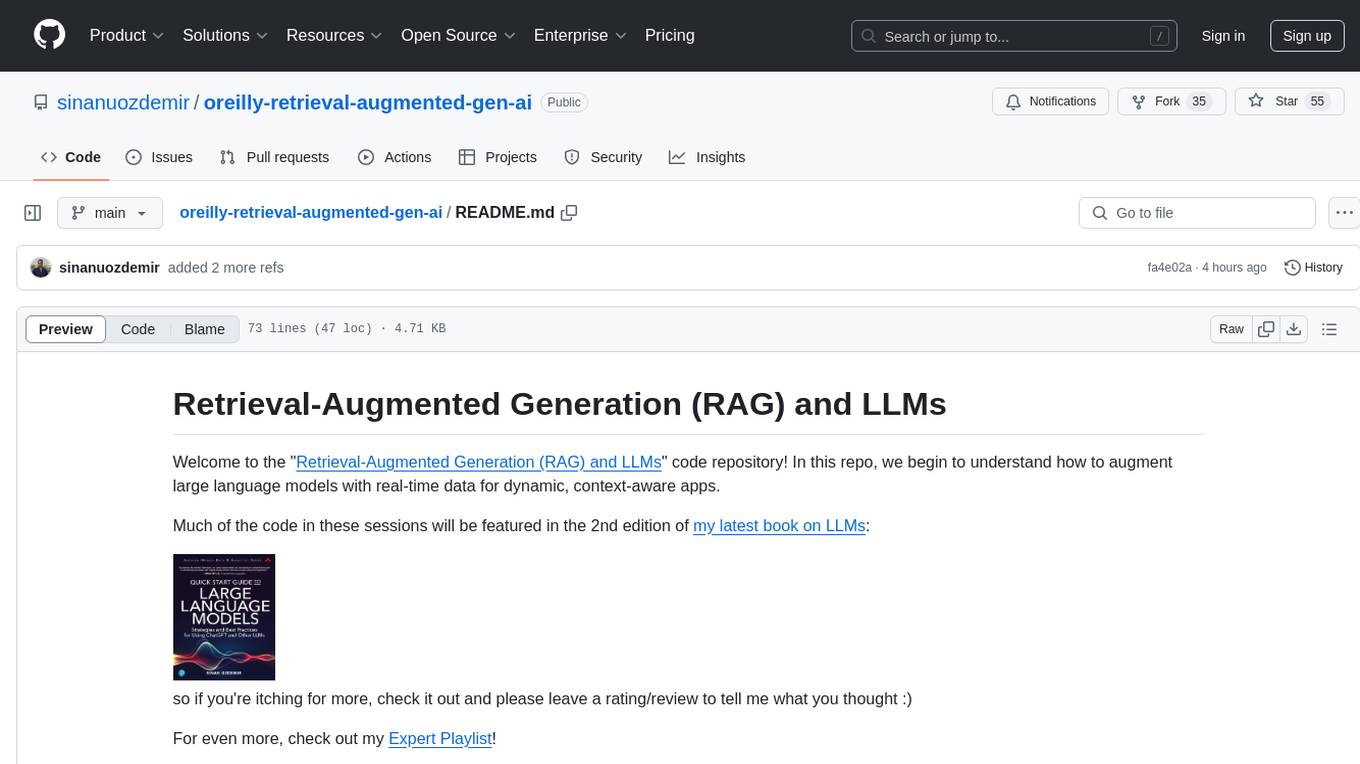
oreilly-retrieval-augmented-gen-ai
This repository focuses on Retrieval-Augmented Generation (RAG) and Large Language Models (LLMs). It provides code and resources to augment LLMs with real-time data for dynamic, context-aware applications. The content covers topics such as semantic search, fine-tuning embeddings, building RAG chatbots, evaluating LLMs, and using knowledge graphs in RAG. Prerequisites include Python skills, knowledge of machine learning and LLMs, and introductory experience with NLP and AI models.
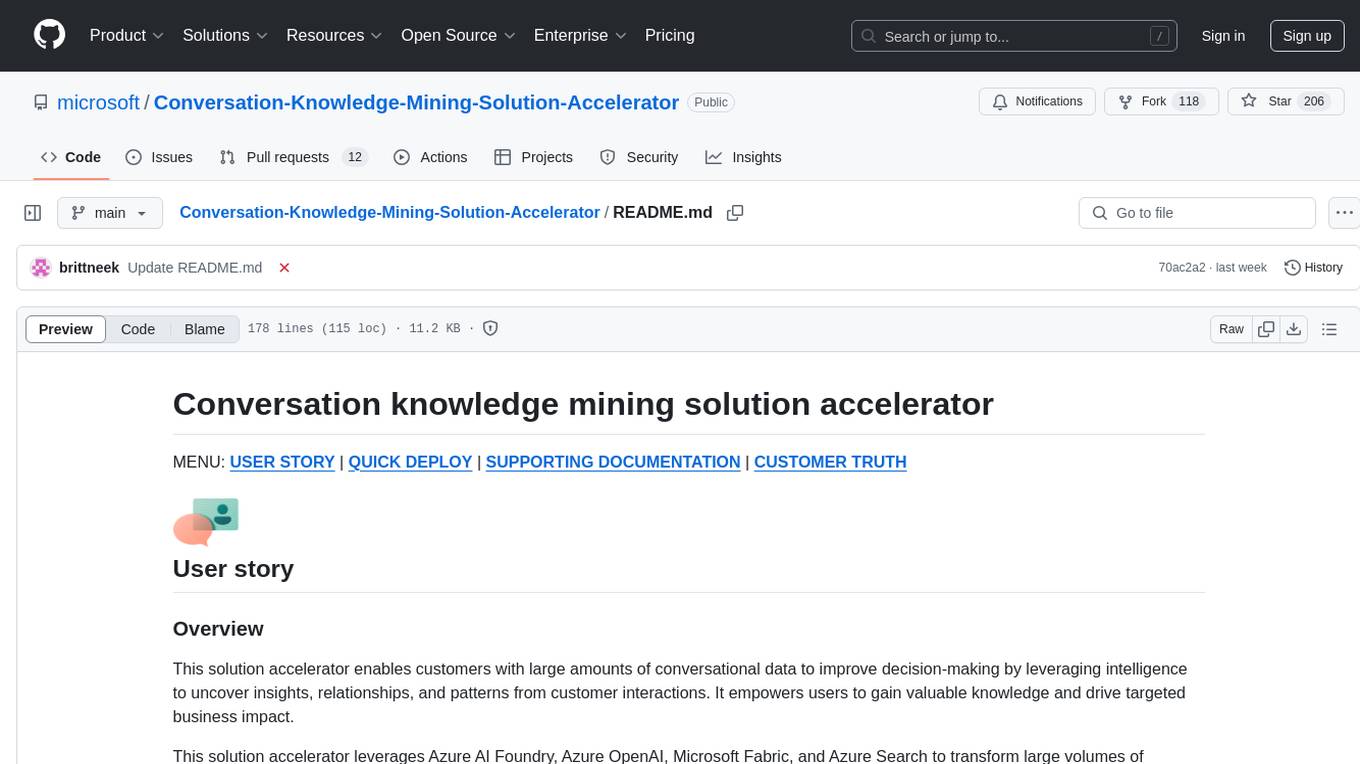
Conversation-Knowledge-Mining-Solution-Accelerator
The Conversation Knowledge Mining Solution Accelerator enables customers to leverage intelligence to uncover insights, relationships, and patterns from conversational data. It empowers users to gain valuable knowledge and drive targeted business impact by utilizing Azure AI Foundry, Azure OpenAI, Microsoft Fabric, and Azure Search for topic modeling, key phrase extraction, speech-to-text transcription, and interactive chat experiences.
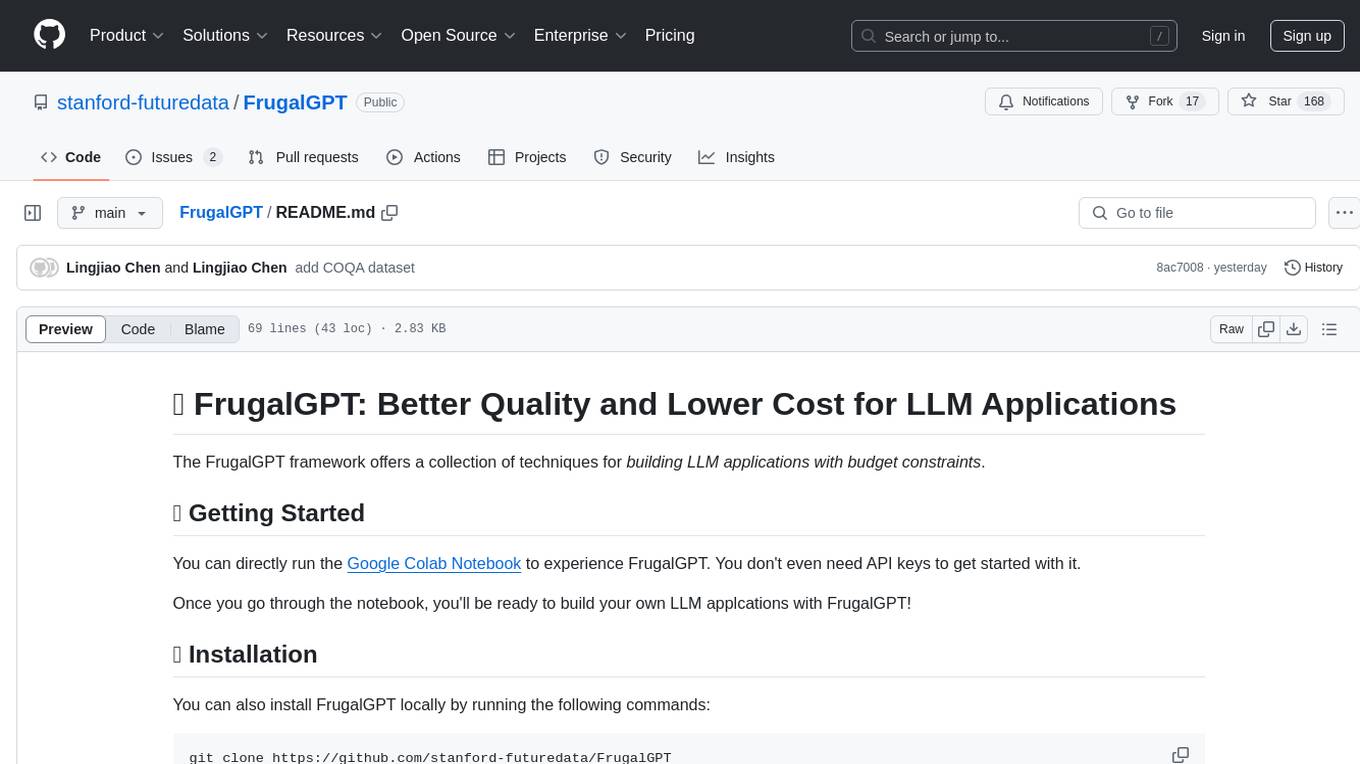
FrugalGPT
FrugalGPT is a framework that offers techniques for building Large Language Model (LLM) applications with budget constraints. It provides a cost-effective solution for utilizing LLMs while maintaining performance. The framework includes support for various models and offers resources for reducing costs and improving efficiency in LLM applications.
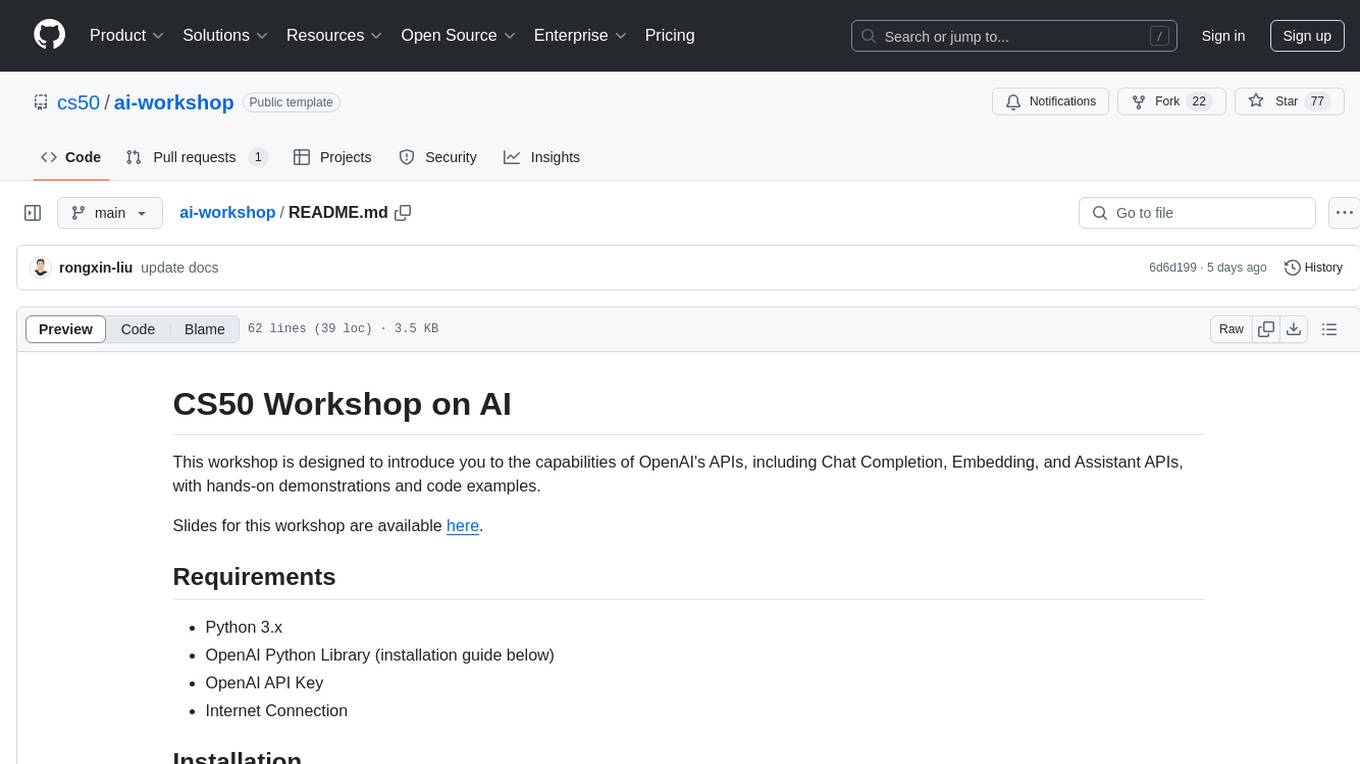
ai-workshop
The AI Workshop repository provides a comprehensive guide to utilizing OpenAI's APIs, including Chat Completion, Embedding, and Assistant APIs. It offers hands-on demonstrations and code examples to help users understand the capabilities of these APIs. The workshop covers topics such as creating interactive chatbots, performing semantic search using text embeddings, and building custom assistants with specific data and context. Users can enhance their understanding of AI applications in education, research, and other domains through practical examples and usage notes.
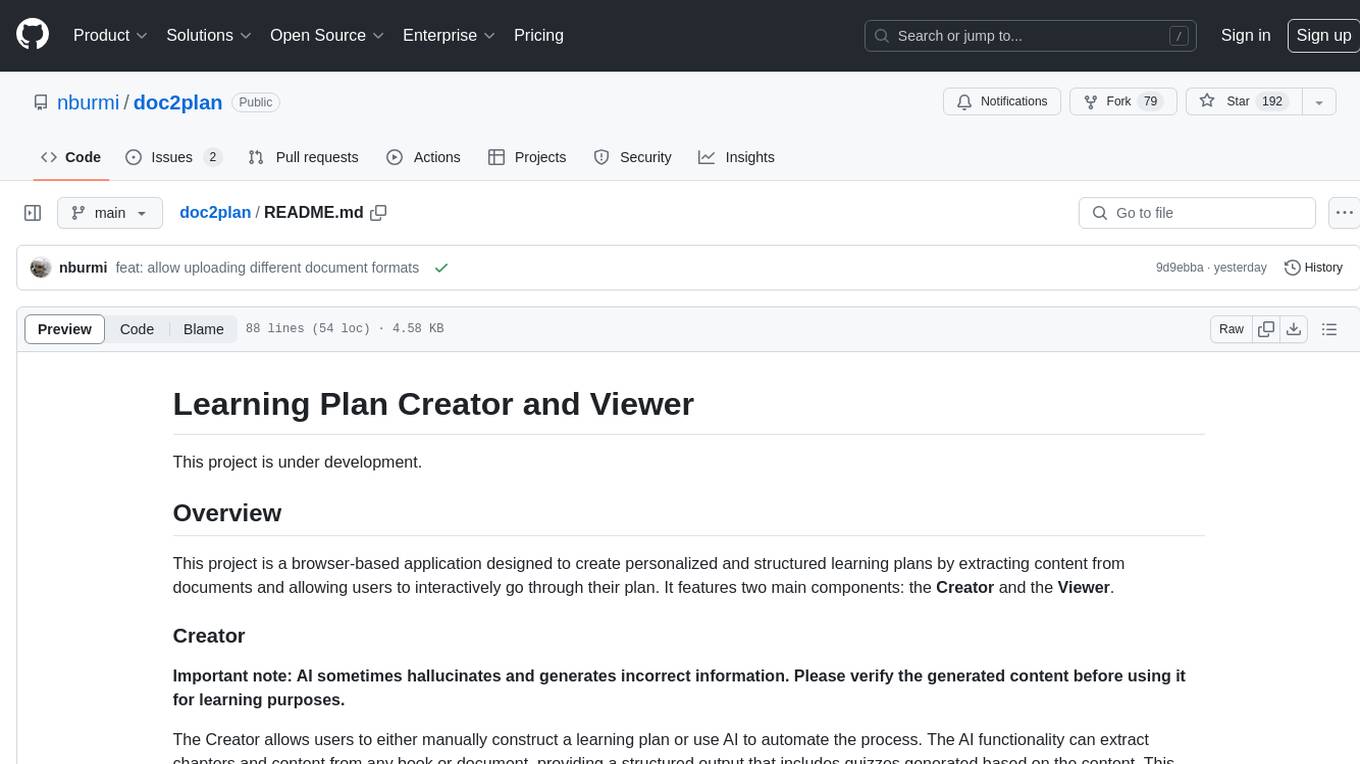
doc2plan
doc2plan is a browser-based application that helps users create personalized learning plans by extracting content from documents. It features a Creator for manual or AI-assisted plan construction and a Viewer for interactive plan navigation. Users can extract chapters, key topics, generate quizzes, and track progress. The application includes AI-driven content extraction, quiz generation, progress tracking, plan import/export, assistant management, customizable settings, viewer chat with text-to-speech and speech-to-text support, and integration with various Retrieval-Augmented Generation (RAG) models. It aims to simplify the creation of comprehensive learning modules tailored to individual needs.
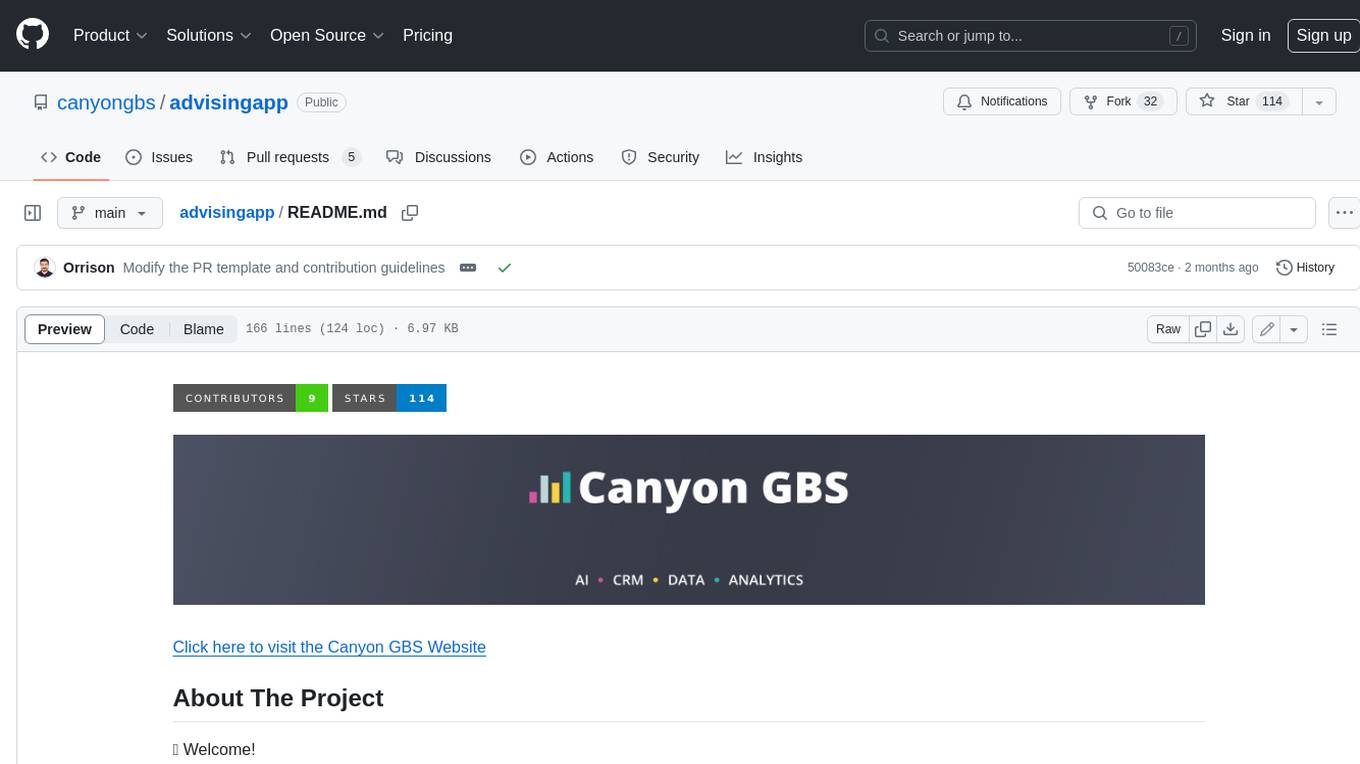
advisingapp
**Advising App™** is a software solution created by Canyon GBS™ that includes a robust personal assistant designed to support student service professionals in their day-to-day roles. The assistant can help with research tasks, draft communication, language translation, content creation, student profile analysis, project planning, ideation, and much more. The software also includes a student service CRM designed to support the management of prospective and enrolled students. Key features of the CRM include record management, email and SMS, service management, caseload management, task management, interaction tracking, files and documents, and much more.
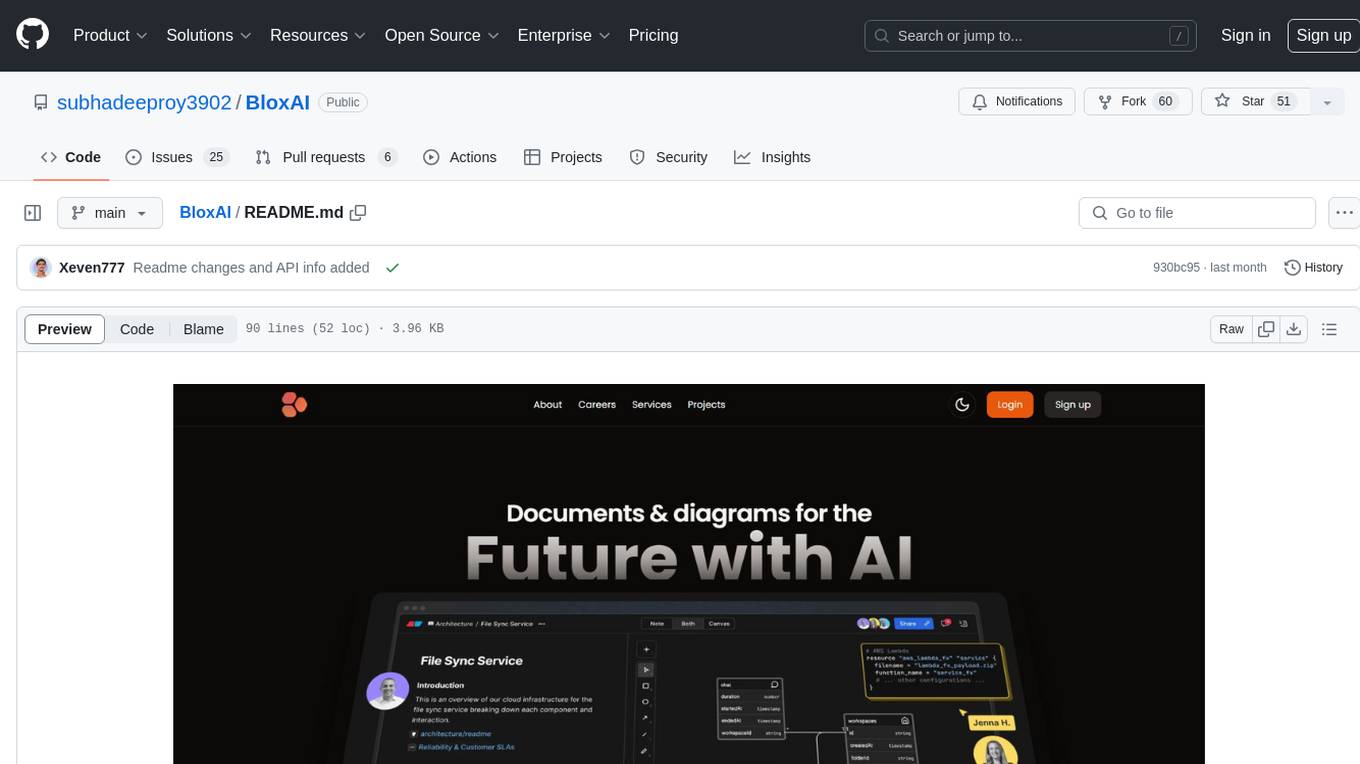
BloxAI
Blox AI is a platform that allows users to effortlessly create flowcharts and diagrams, collaborate with teams, and receive explanations from the Google Gemini model. It offers rich text editing, versatile visualizations, secure workspaces, and limited files allotment. Users can install it as an app and use it for wireframes, mind maps, and algorithms. The platform is built using Next.Js, Typescript, ShadCN UI, TailwindCSS, Convex, Kinde, EditorJS, and Excalidraw.
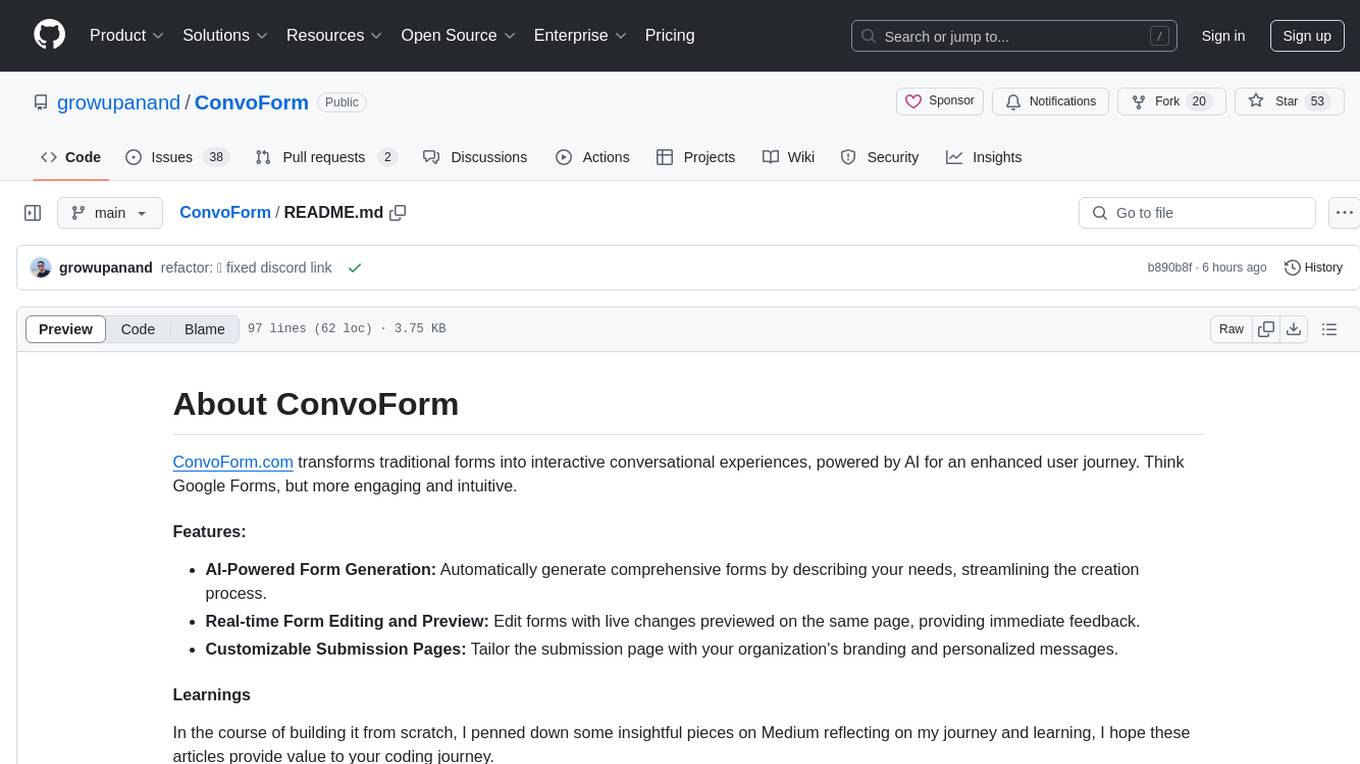
ConvoForm
ConvoForm.com transforms traditional forms into interactive conversational experiences, powered by AI for an enhanced user journey. It offers AI-Powered Form Generation, Real-time Form Editing and Preview, and Customizable Submission Pages. The tech stack includes Next.js for frontend, tRPC for backend, GPT-3.5-Turbo for AI integration, and Socket.io for real-time updates. Local setup requires Node.js, pnpm, Git, PostgreSQL database, Clerk for Authentication, OpenAI key, Redis Database, and Sentry for monitoring. The project is open for contributions and is licensed under the MIT License.
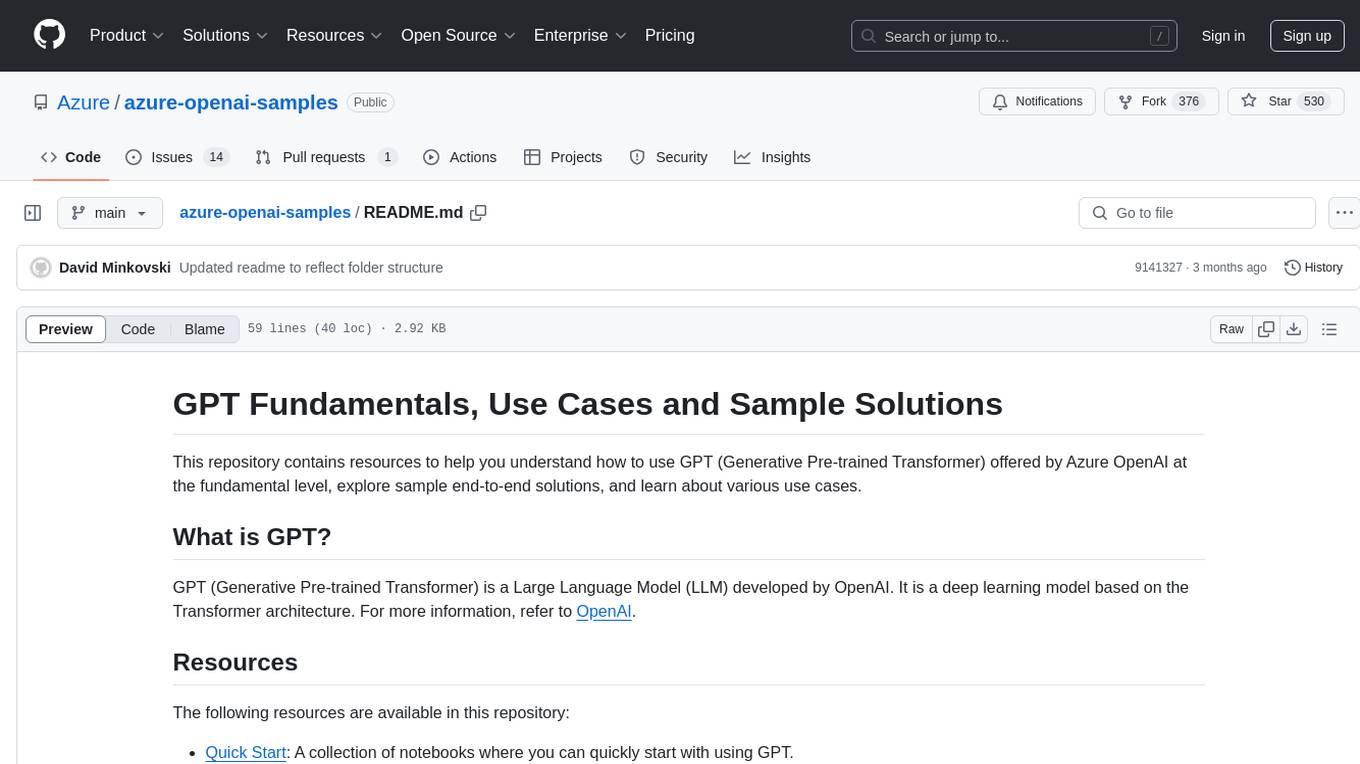
azure-openai-samples
This repository provides resources to understand and utilize GPT (Generative Pre-trained Transformer) by Azure OpenAI. It includes sample solutions, use cases, and quick start guides. Users can explore various applications of GPT, such as chatbots, customer service, and content generation. The repository also offers Langchain, Semantic Kernel, and Prompt Flow samples, along with Serverless SQL GPT for natural language processing in Azure Synapse Analytics. The samples are based on GPT 3.5, with plans to update for GPT-4. Users are encouraged to contribute to keep the repository updated with the latest technologies and solutions.
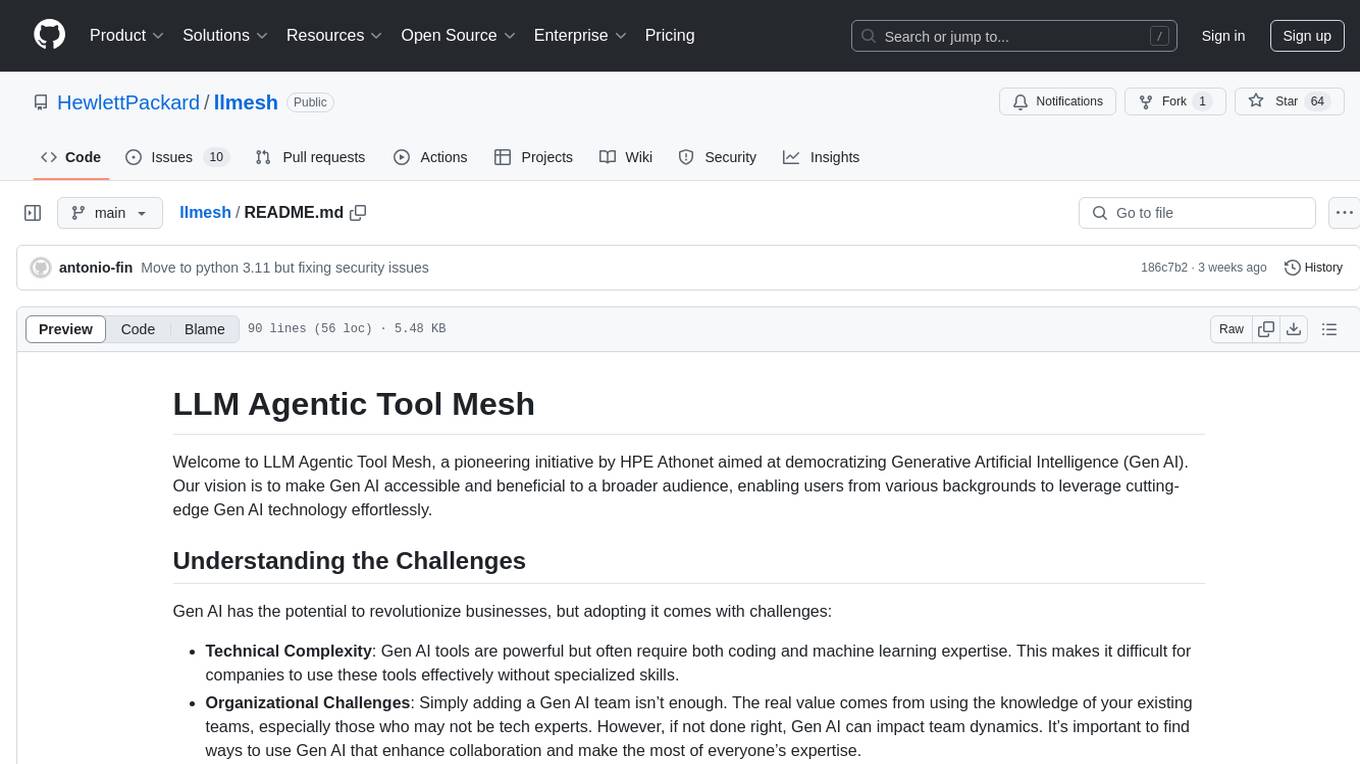
llmesh
LLM Agentic Tool Mesh is a platform by HPE Athonet that democratizes Generative Artificial Intelligence (Gen AI) by enabling users to create tools and web applications using Gen AI with Low or No Coding. The platform simplifies the integration process, focuses on key user needs, and abstracts complex libraries into easy-to-understand services. It empowers both technical and non-technical teams to develop tools related to their expertise and provides orchestration capabilities through an agentic Reasoning Engine based on Large Language Models (LLMs) to ensure seamless tool integration and enhance organizational functionality and efficiency.
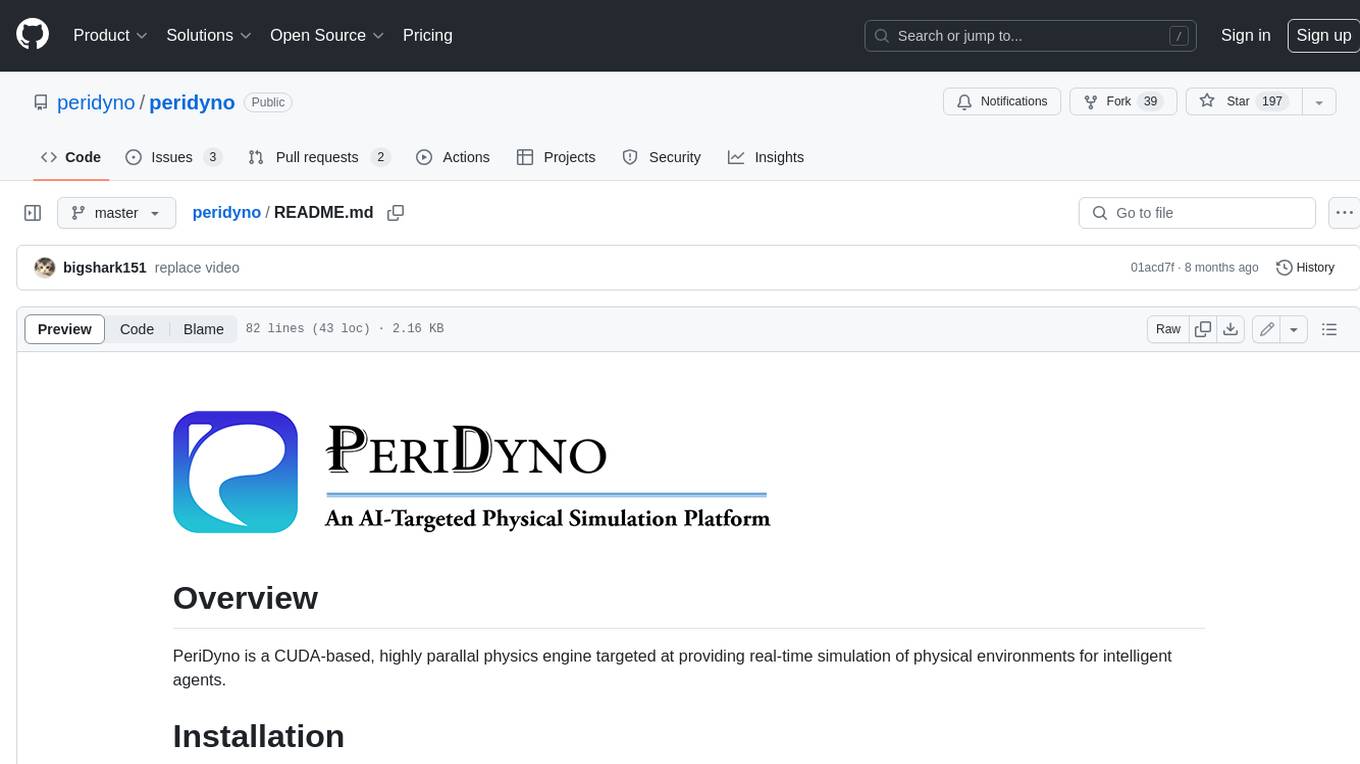
peridyno
PeriDyno is a CUDA-based, highly parallel physics engine targeted at providing real-time simulation of physical environments for intelligent agents. It is designed to be easy to use and integrate into existing projects, and it provides a wide range of features for simulating a variety of physical phenomena. PeriDyno is open source and available under the Apache 2.0 license.
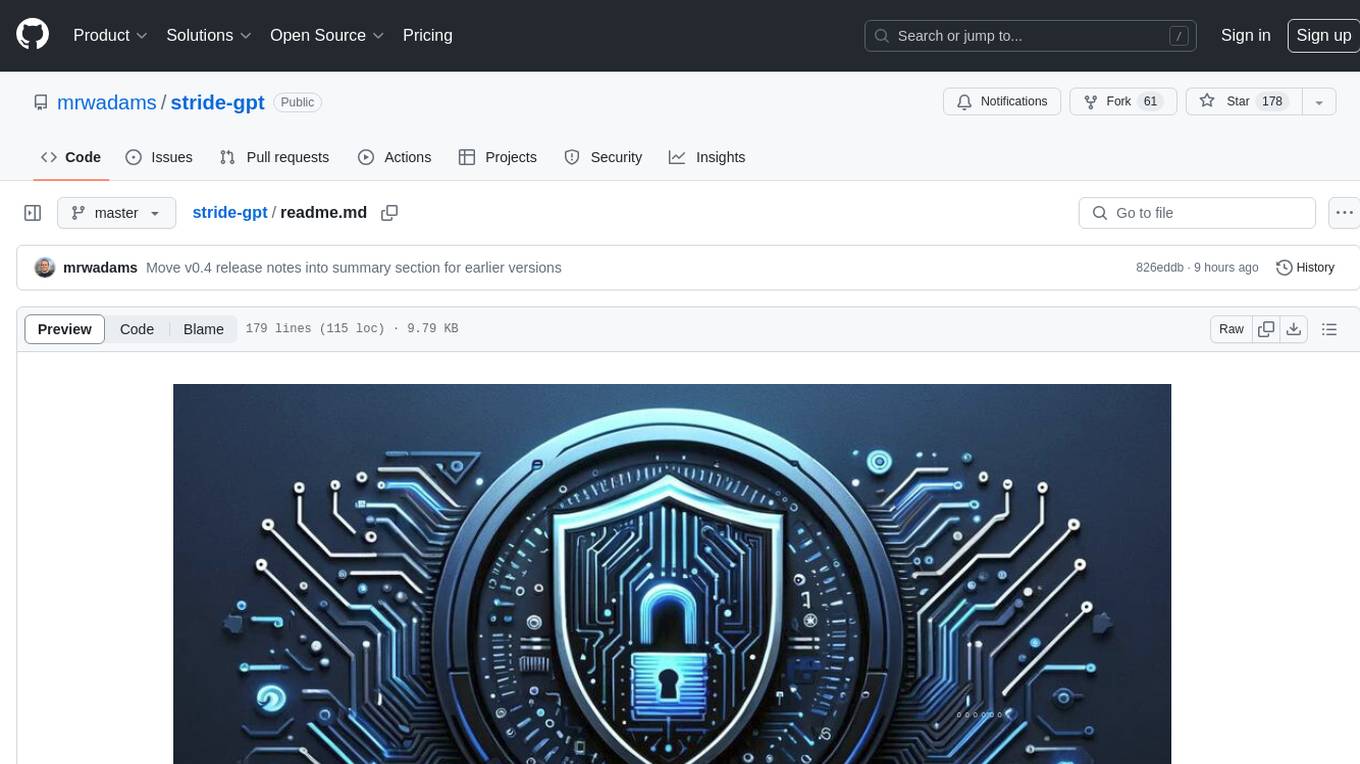
stride-gpt
STRIDE GPT is an AI-powered threat modelling tool that leverages Large Language Models (LLMs) to generate threat models and attack trees for a given application based on the STRIDE methodology. Users provide application details, such as the application type, authentication methods, and whether the application is internet-facing or processes sensitive data. The model then generates its output based on the provided information. It features a simple and user-friendly interface, supports multi-modal threat modelling, generates attack trees, suggests possible mitigations for identified threats, and does not store application details. STRIDE GPT can be accessed via OpenAI API, Azure OpenAI Service, Google AI API, or Mistral API. It is available as a Docker container image for easy deployment.
For similar tasks
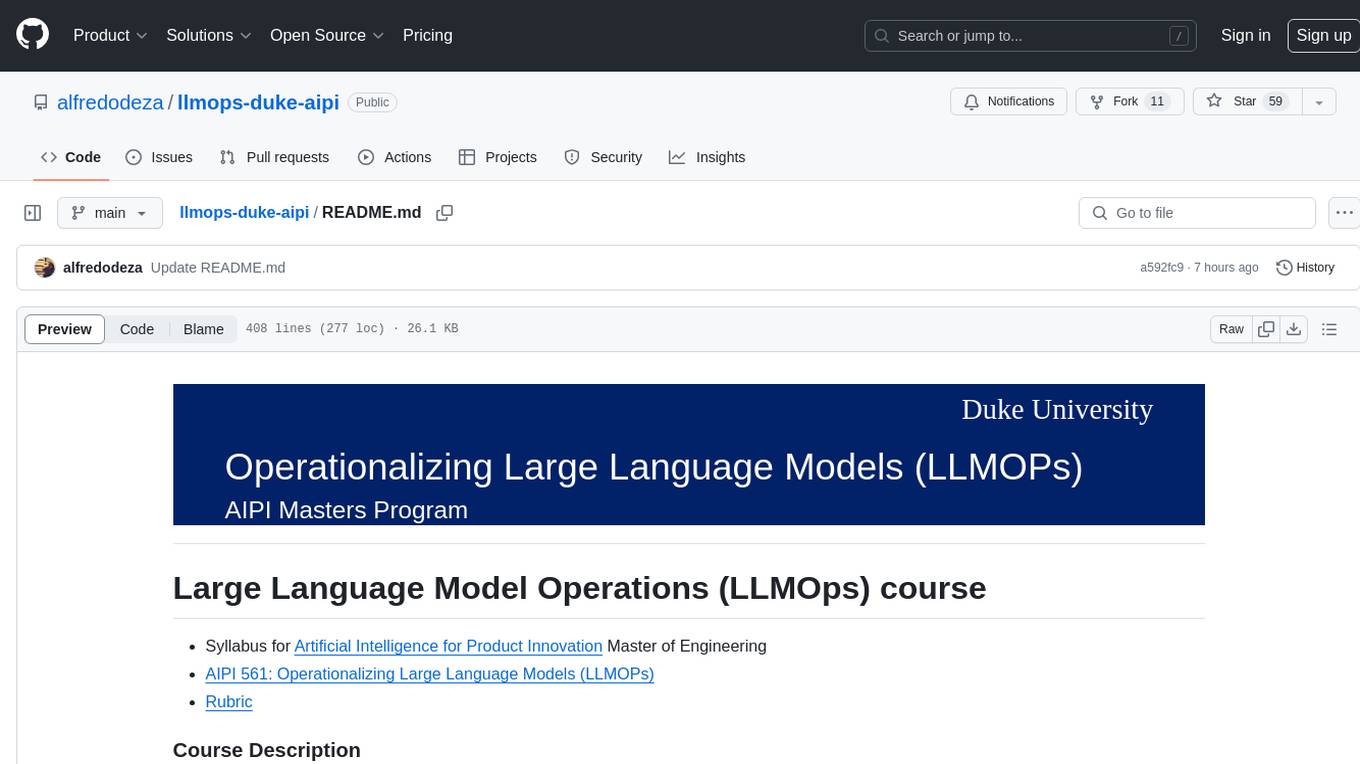
llmops-duke-aipi
LLMOps Duke AIPI is a course focused on operationalizing Large Language Models, teaching methodologies for developing applications using software development best practices with large language models. The course covers various topics such as generative AI concepts, setting up development environments, interacting with large language models, using local large language models, applied solutions with LLMs, extensibility using plugins and functions, retrieval augmented generation, introduction to Python web frameworks for APIs, DevOps principles, deploying machine learning APIs, LLM platforms, and final presentations. Students will learn to build, share, and present portfolios using Github, YouTube, and Linkedin, as well as develop non-linear life-long learning skills. Prerequisites include basic Linux and programming skills, with coursework available in Python or Rust. Additional resources and references are provided for further learning and exploration.
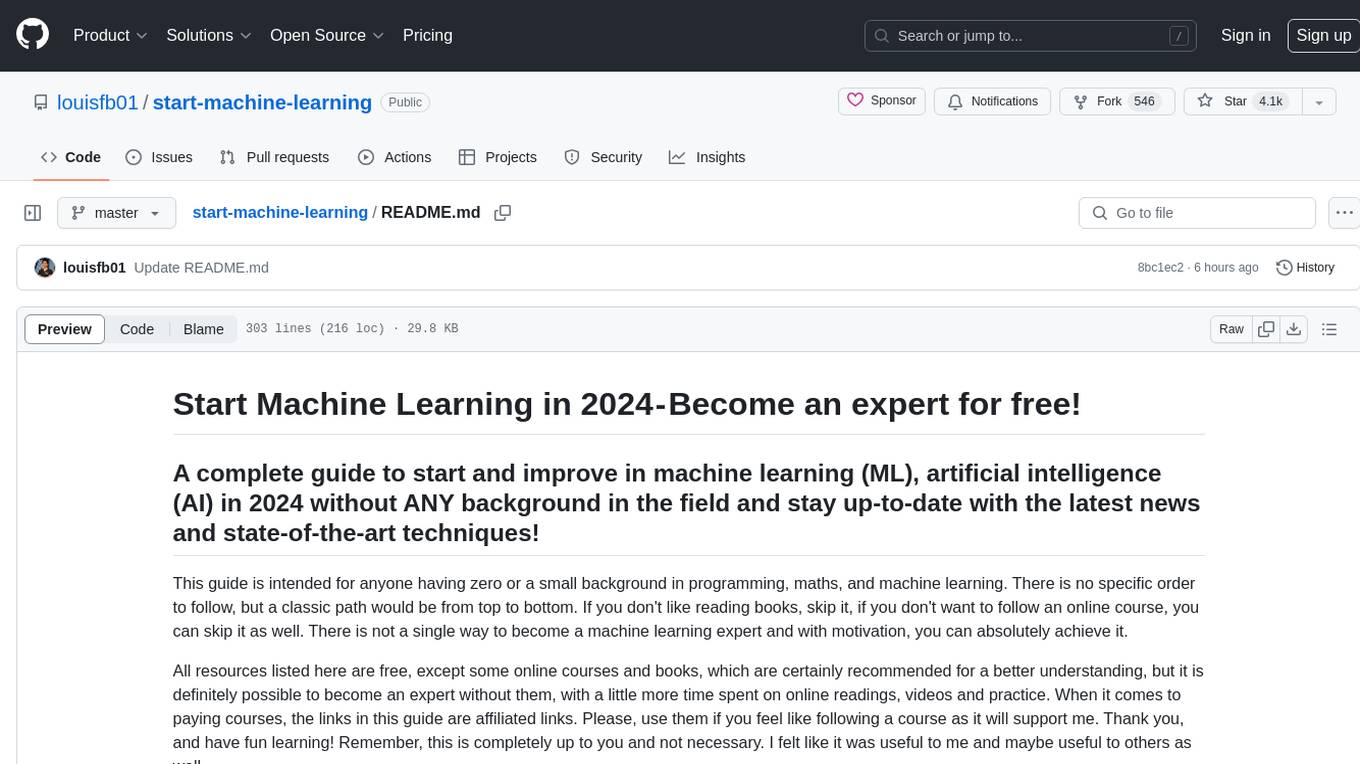
start-machine-learning
Start Machine Learning in 2024 is a comprehensive guide for beginners to advance in machine learning and artificial intelligence without any prior background. The guide covers various resources such as free online courses, articles, books, and practical tips to become an expert in the field. It emphasizes self-paced learning and provides recommendations for learning paths, including videos, podcasts, and online communities. The guide also includes information on building language models and applications, practicing through Kaggle competitions, and staying updated with the latest news and developments in AI. The goal is to empower individuals with the knowledge and resources to excel in machine learning and AI.
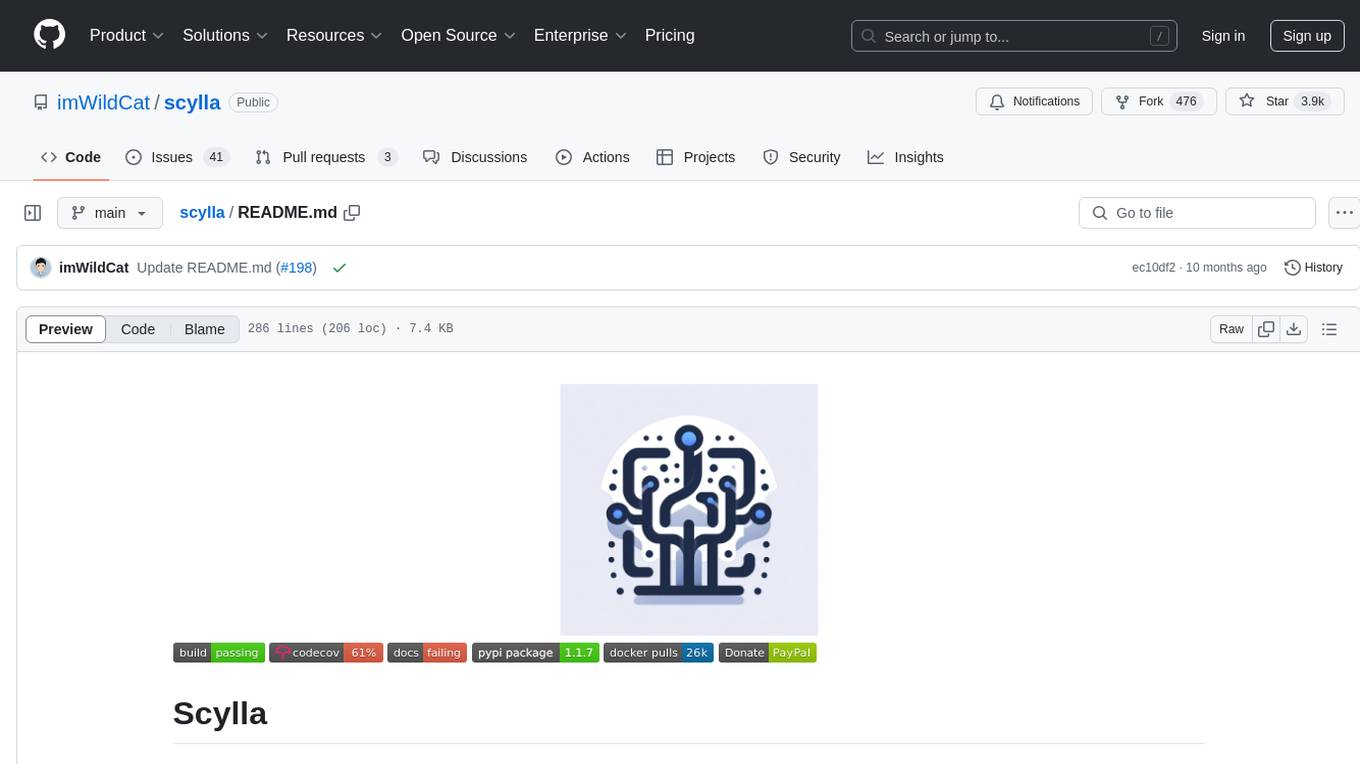
scylla
Scylla is an intelligent proxy pool tool designed for humanities, enabling users to extract content from the internet and build their own Large Language Models in the AI era. It features automatic proxy IP crawling and validation, an easy-to-use JSON API, a simple web-based user interface, HTTP forward proxy server, Scrapy and requests integration, and headless browser crawling. Users can start using Scylla with just one command, making it a versatile tool for various web scraping and content extraction tasks.
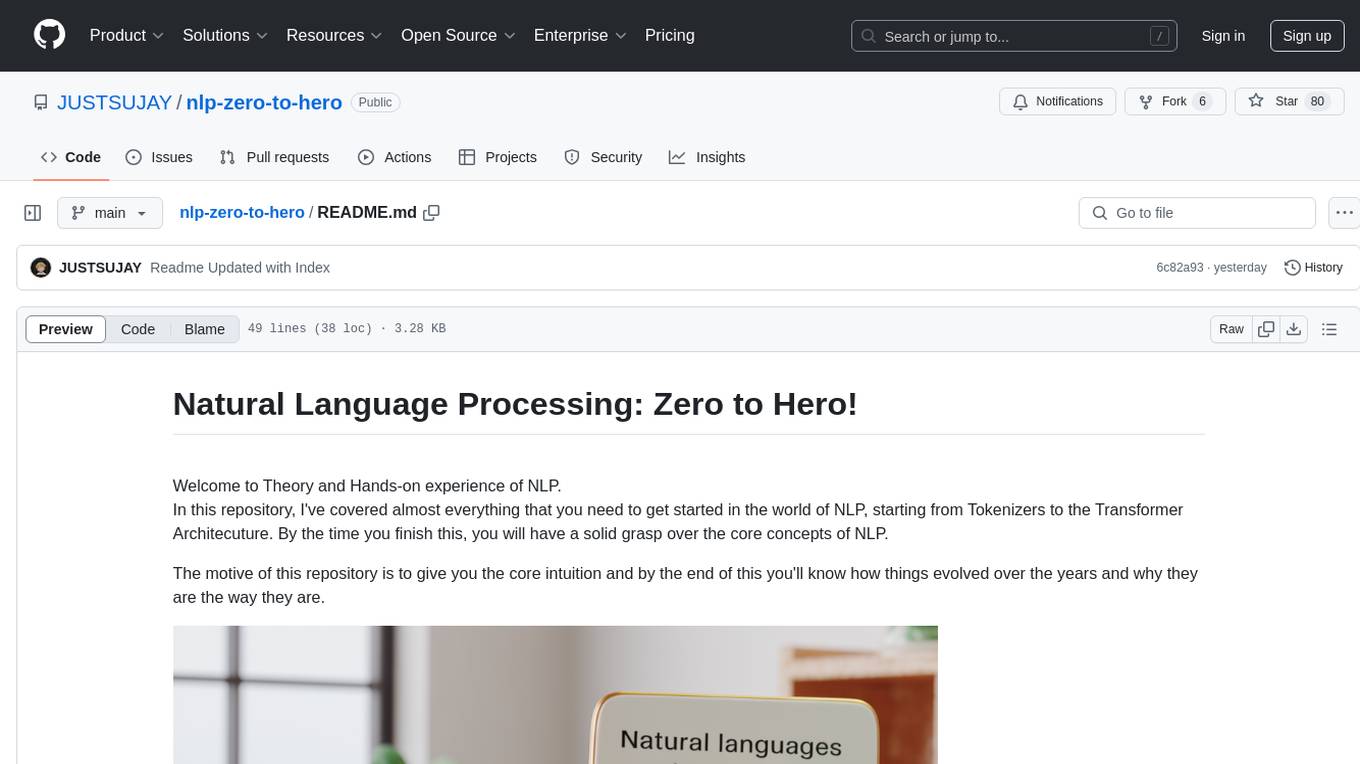
nlp-zero-to-hero
This repository provides a comprehensive guide to Natural Language Processing (NLP), covering topics from Tokenization to Transformer Architecture. It aims to equip users with a solid understanding of NLP concepts, evolution, and core intuition. The repository includes practical examples and hands-on experience to facilitate learning and exploration in the field of NLP.
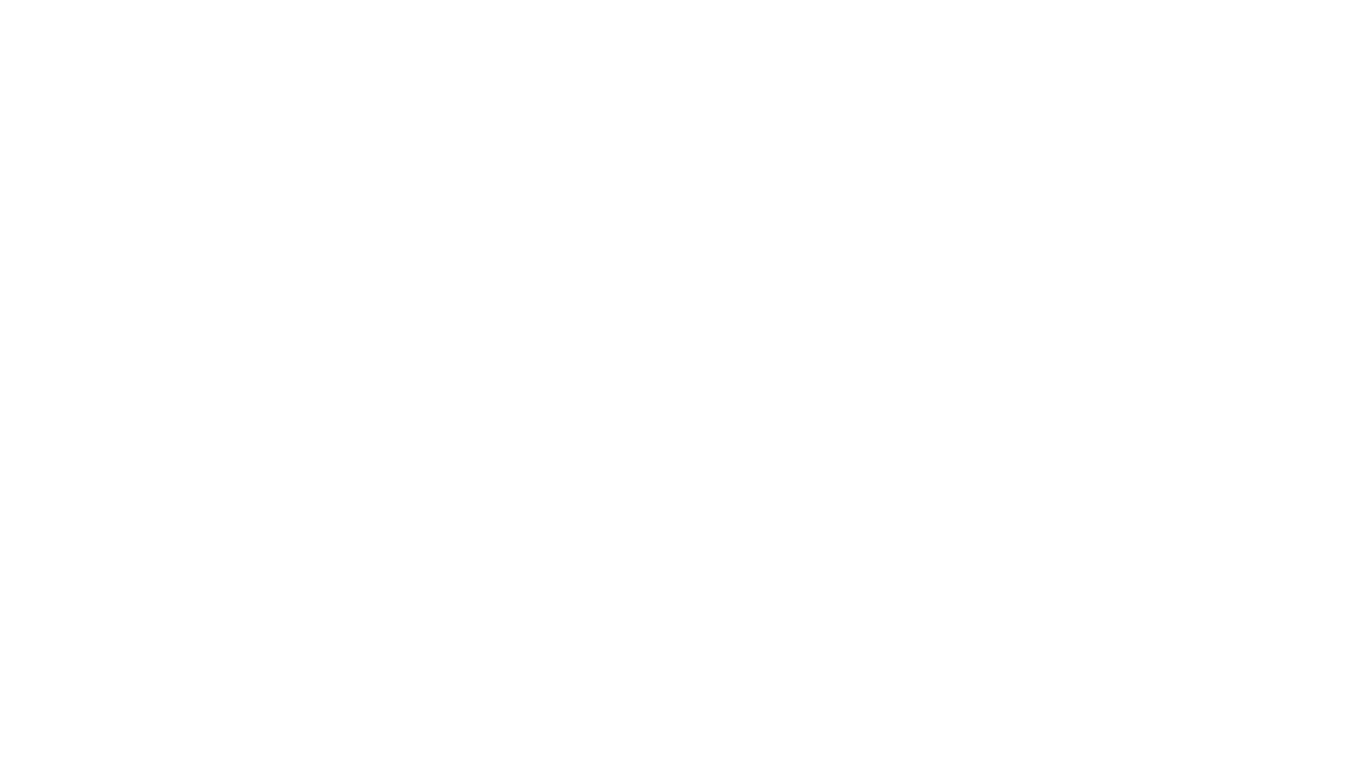
zep-python
Zep is an open-source platform for building and deploying large language model (LLM) applications. It provides a suite of tools and services that make it easy to integrate LLMs into your applications, including chat history memory, embedding, vector search, and data enrichment. Zep is designed to be scalable, reliable, and easy to use, making it a great choice for developers who want to build LLM-powered applications quickly and easily.
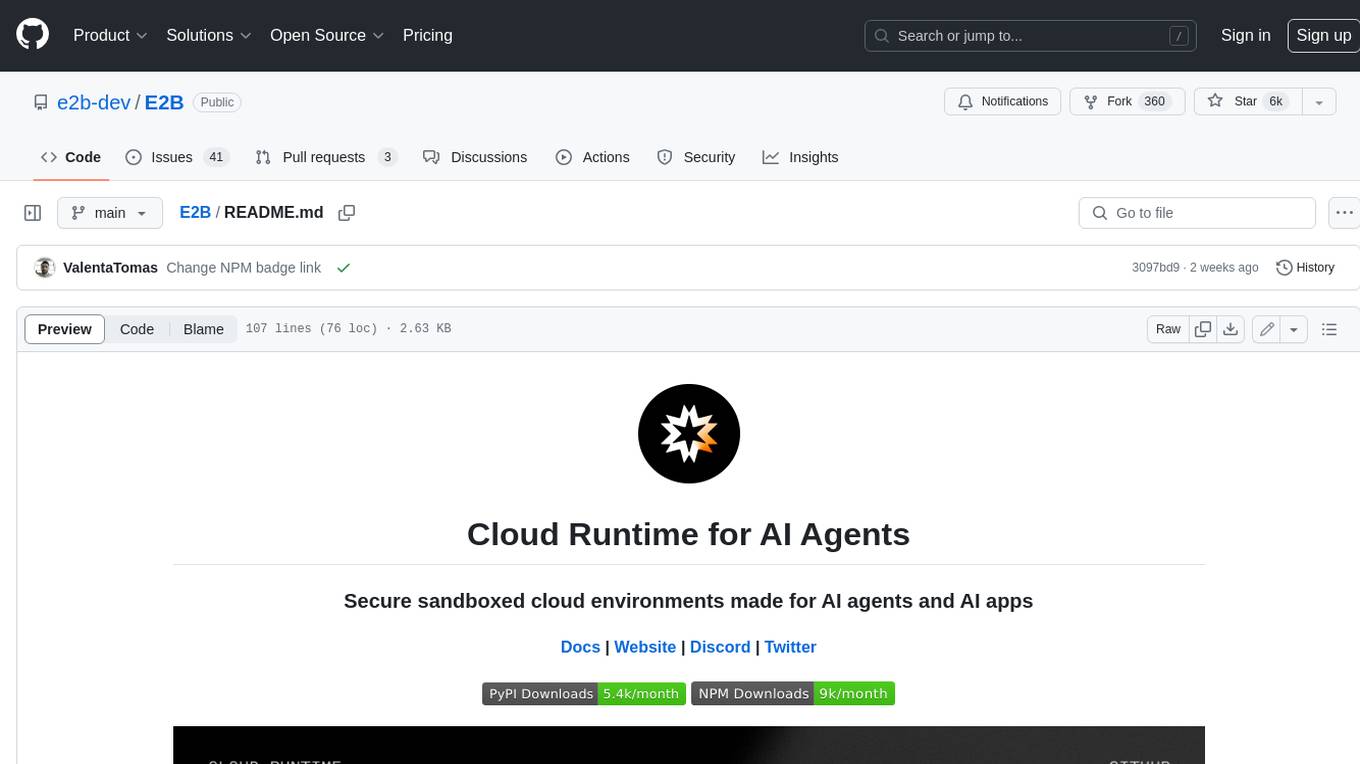
E2B
E2B Sandbox is a secure sandboxed cloud environment made for AI agents and AI apps. Sandboxes allow AI agents and apps to have long running cloud secure environments. In these environments, large language models can use the same tools as humans do. For example: * Cloud browsers * GitHub repositories and CLIs * Coding tools like linters, autocomplete, "go-to defintion" * Running LLM generated code * Audio & video editing The E2B sandbox can be connected to any LLM and any AI agent or app.
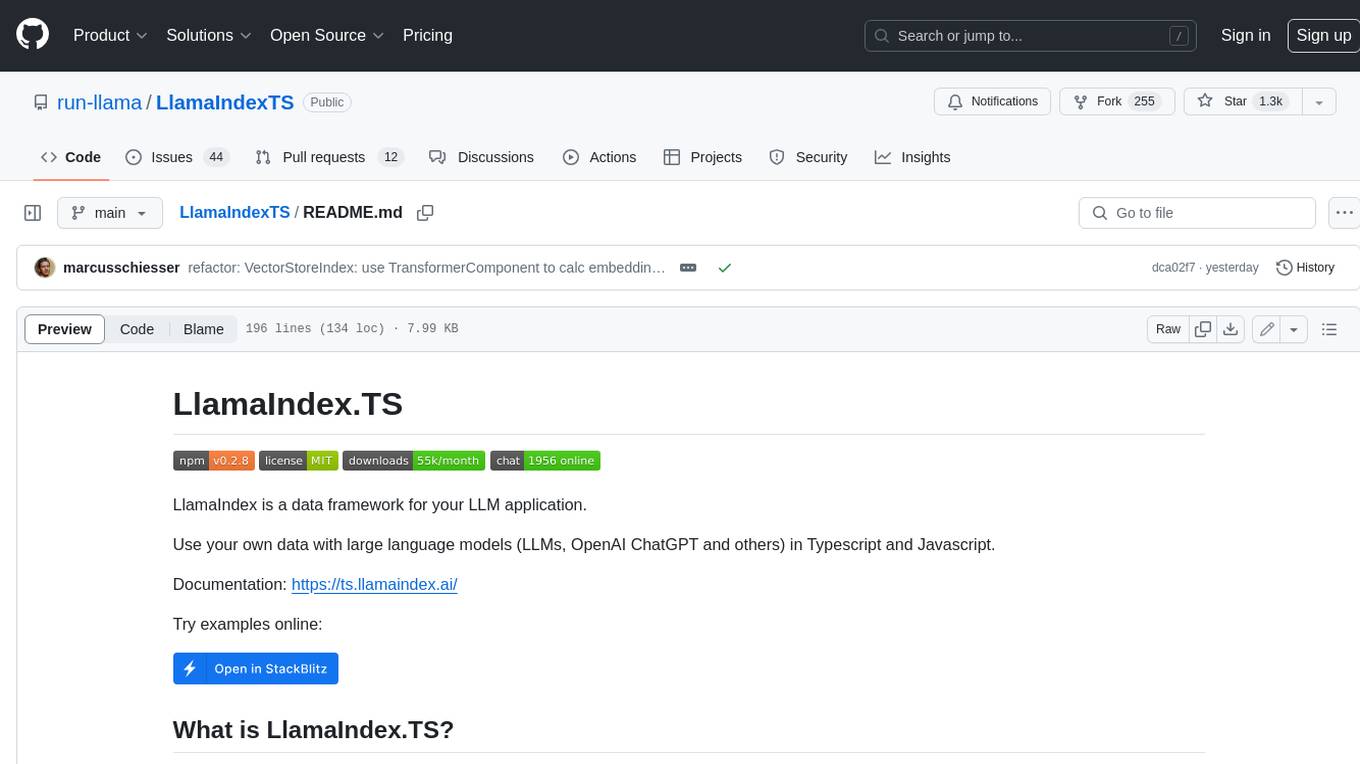
LlamaIndexTS
LlamaIndex.TS is a data framework for your LLM application. Use your own data with large language models (LLMs, OpenAI ChatGPT and others) in Typescript and Javascript.
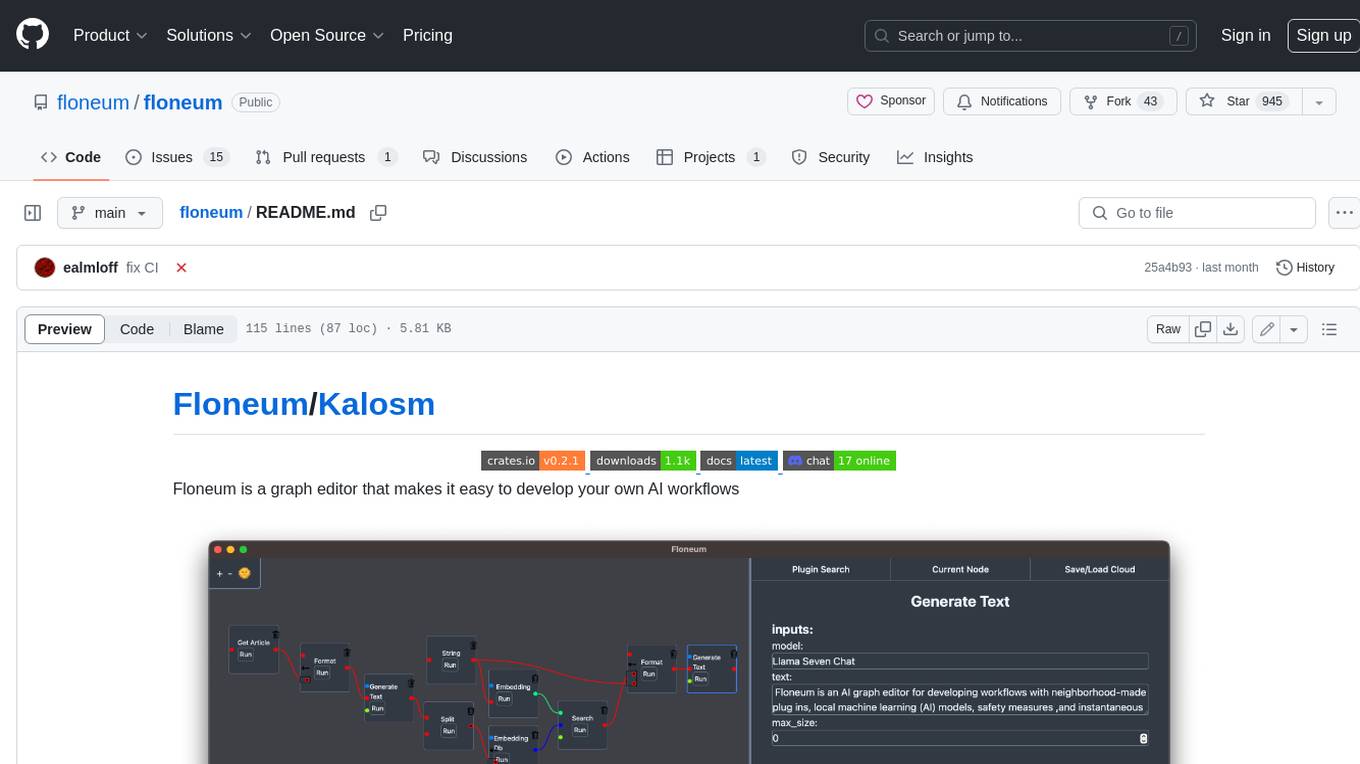
floneum
Floneum is a graph editor that makes it easy to develop your own AI workflows. It uses large language models (LLMs) to run AI models locally, without any external dependencies or even a GPU. This makes it easy to use LLMs with your own data, without worrying about privacy. Floneum also has a plugin system that allows you to improve the performance of LLMs and make them work better for your specific use case. Plugins can be used in any language that supports web assembly, and they can control the output of LLMs with a process similar to JSONformer or guidance.
For similar jobs
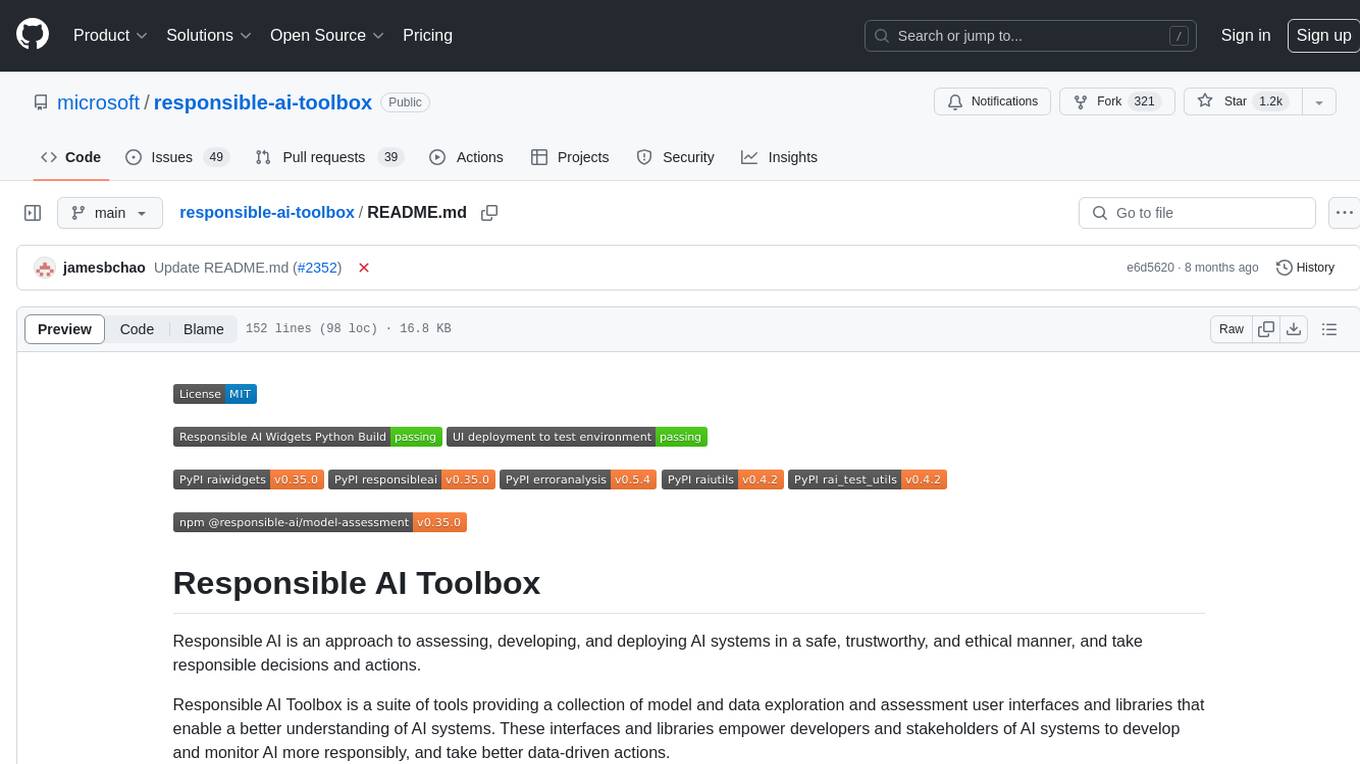
responsible-ai-toolbox
Responsible AI Toolbox is a suite of tools providing model and data exploration and assessment interfaces and libraries for understanding AI systems. It empowers developers and stakeholders to develop and monitor AI responsibly, enabling better data-driven actions. The toolbox includes visualization widgets for model assessment, error analysis, interpretability, fairness assessment, and mitigations library. It also offers a JupyterLab extension for managing machine learning experiments and a library for measuring gender bias in NLP datasets.
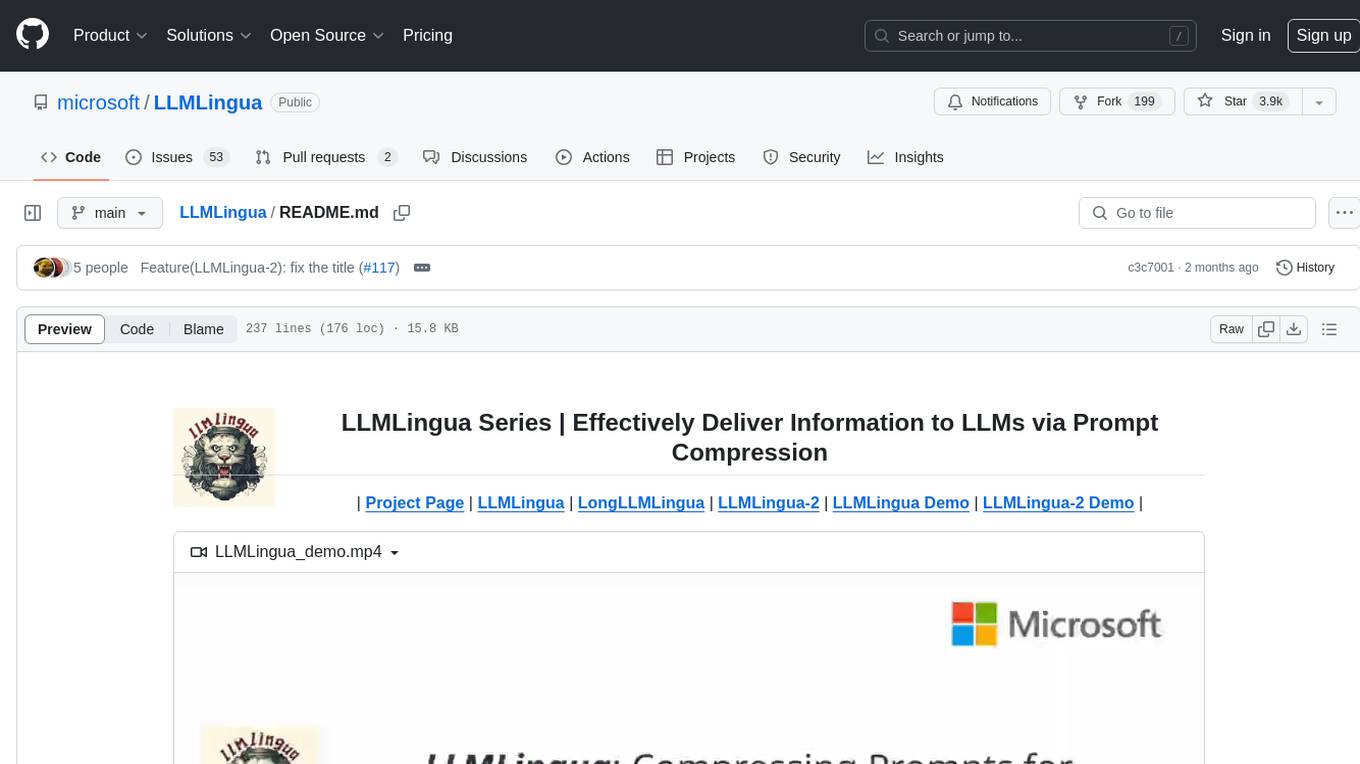
LLMLingua
LLMLingua is a tool that utilizes a compact, well-trained language model to identify and remove non-essential tokens in prompts. This approach enables efficient inference with large language models, achieving up to 20x compression with minimal performance loss. The tool includes LLMLingua, LongLLMLingua, and LLMLingua-2, each offering different levels of prompt compression and performance improvements for tasks involving large language models.
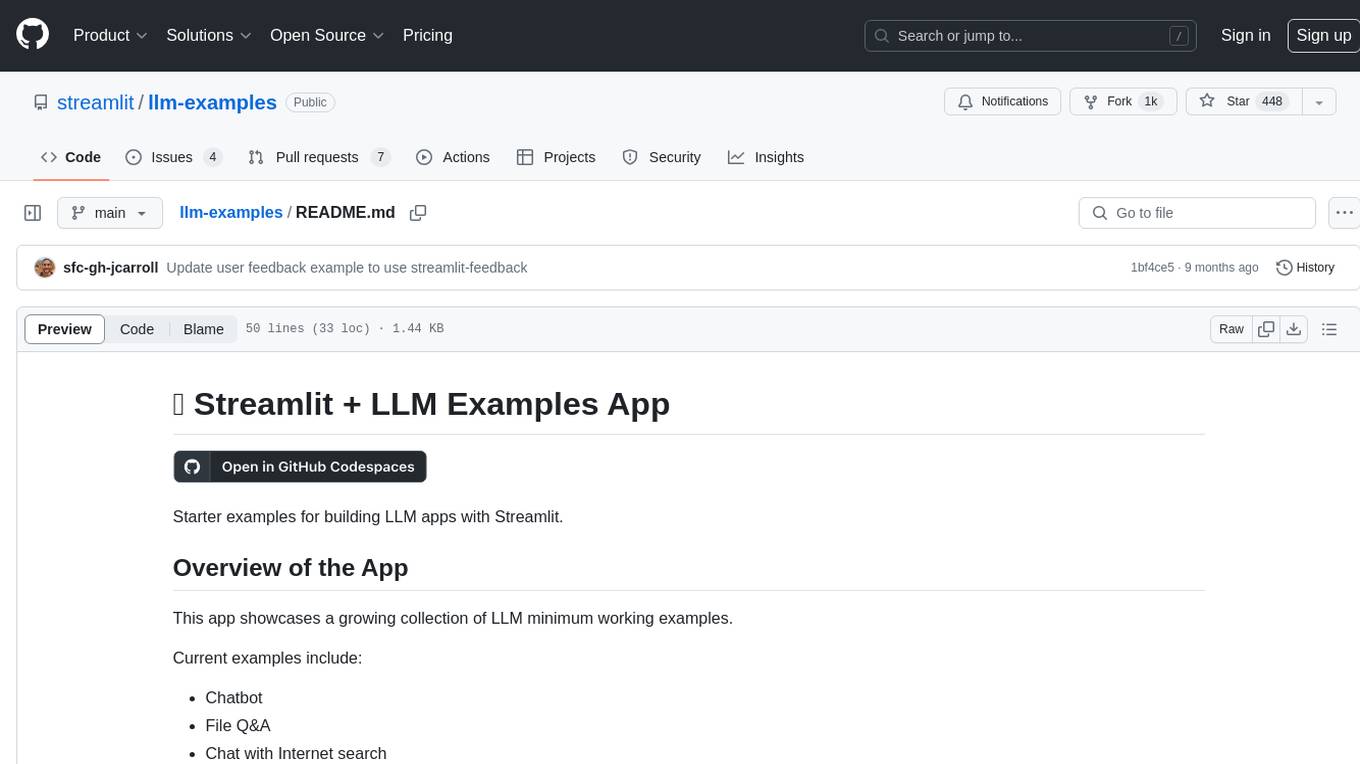
llm-examples
Starter examples for building LLM apps with Streamlit. This repository showcases a growing collection of LLM minimum working examples, including a Chatbot, File Q&A, Chat with Internet search, LangChain Quickstart, LangChain PromptTemplate, and Chat with user feedback. Users can easily get their own OpenAI API key and set it as an environment variable in Streamlit apps to run the examples locally.
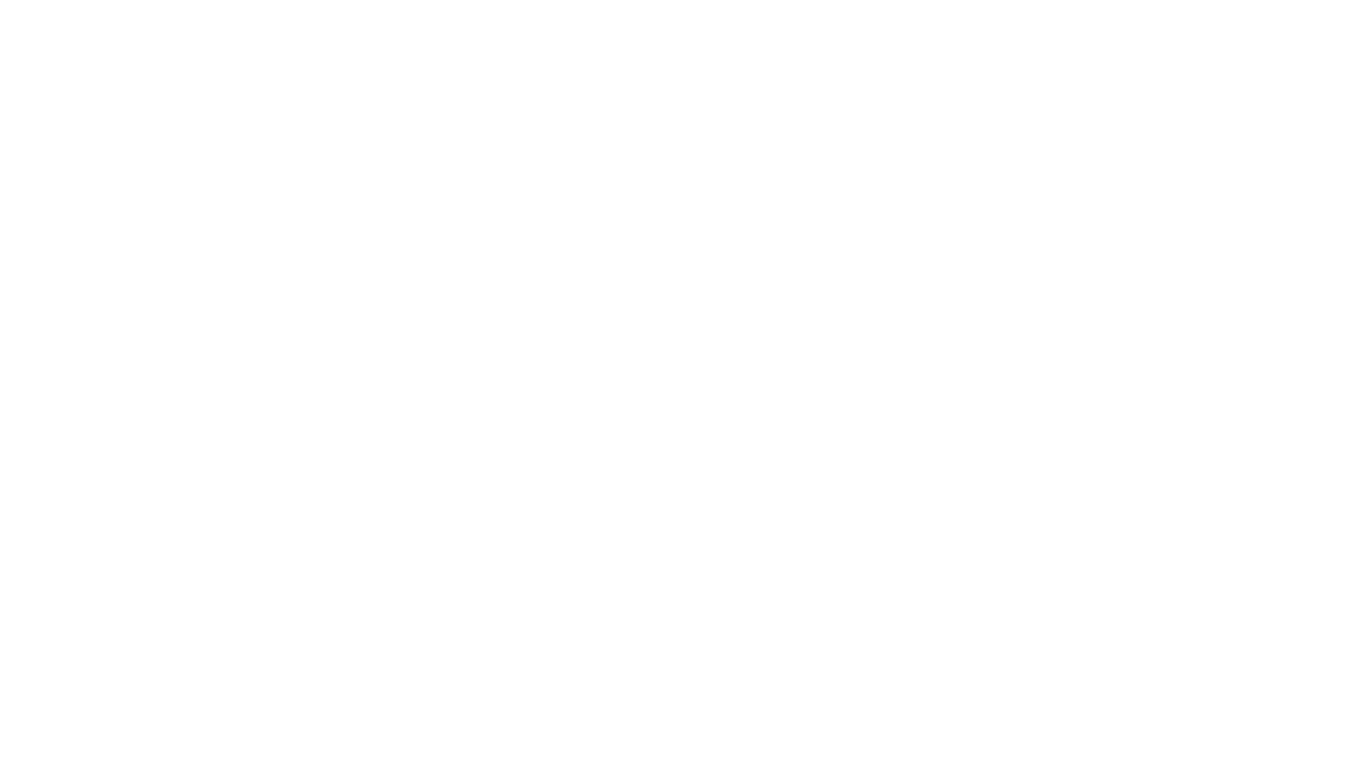
LMOps
LMOps is a research initiative focusing on fundamental research and technology for building AI products with foundation models, particularly enabling AI capabilities with Large Language Models (LLMs) and Generative AI models. The project explores various aspects such as prompt optimization, longer context handling, LLM alignment, acceleration of LLMs, LLM customization, and understanding in-context learning. It also includes tools like Promptist for automatic prompt optimization, Structured Prompting for efficient long-sequence prompts consumption, and X-Prompt for extensible prompts beyond natural language. Additionally, LLMA accelerators are developed to speed up LLM inference by referencing and copying text spans from documents. The project aims to advance technologies that facilitate prompting language models and enhance the performance of LLMs in various scenarios.
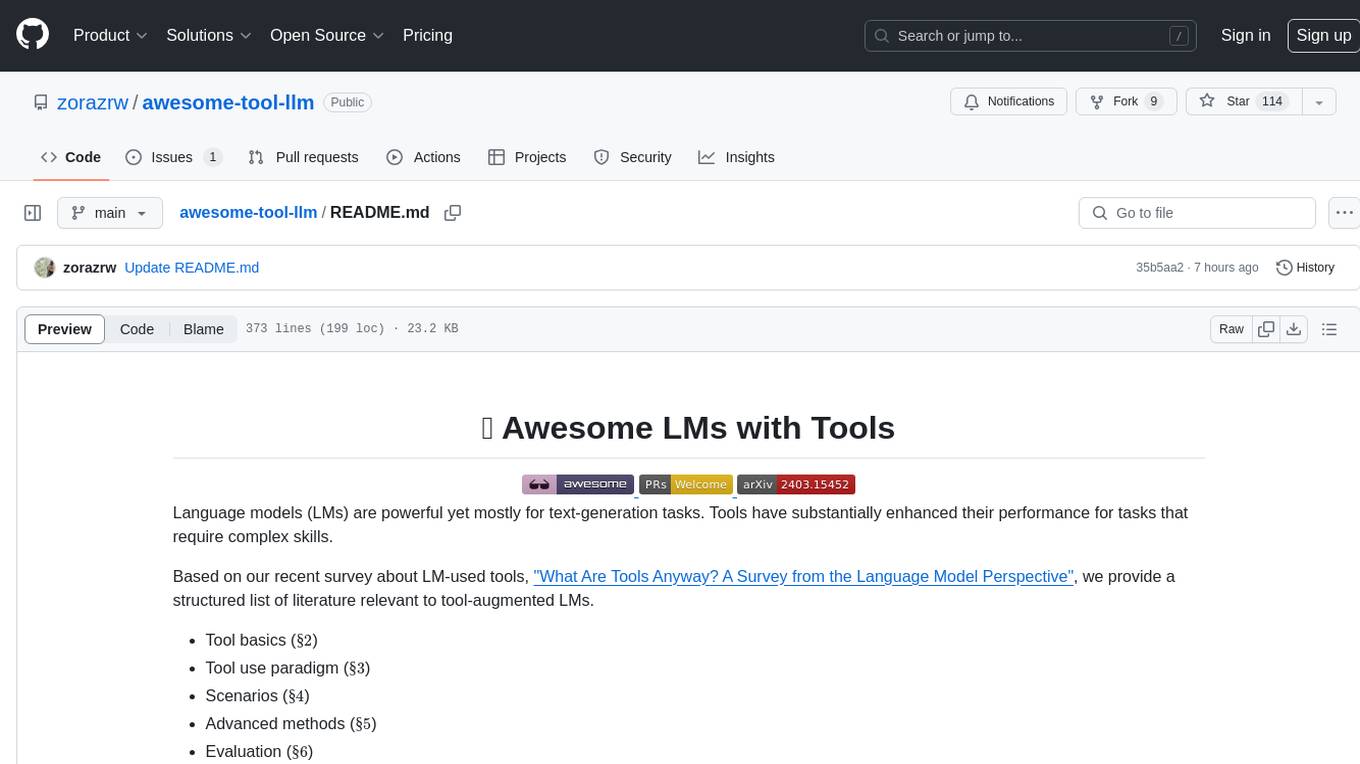
awesome-tool-llm
This repository focuses on exploring tools that enhance the performance of language models for various tasks. It provides a structured list of literature relevant to tool-augmented language models, covering topics such as tool basics, tool use paradigm, scenarios, advanced methods, and evaluation. The repository includes papers, preprints, and books that discuss the use of tools in conjunction with language models for tasks like reasoning, question answering, mathematical calculations, accessing knowledge, interacting with the world, and handling non-textual modalities.
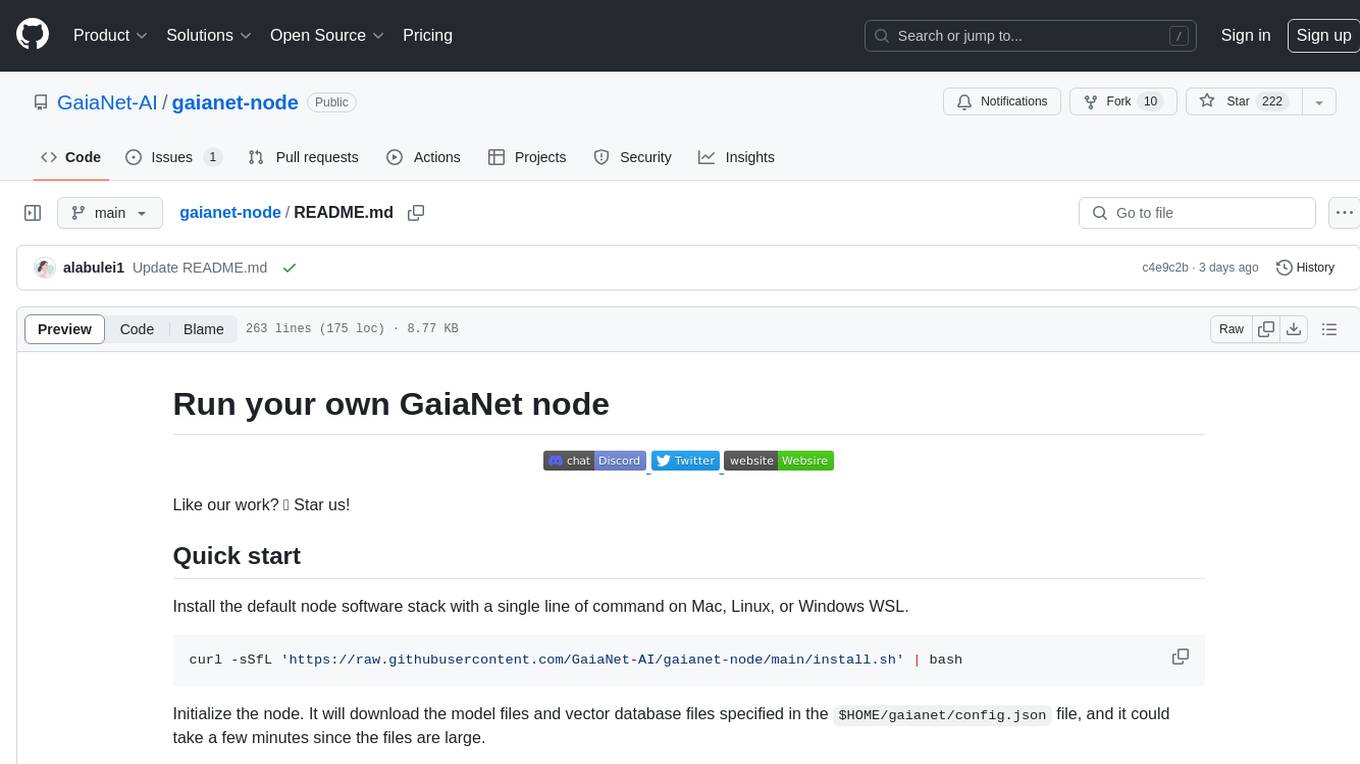
gaianet-node
GaiaNet-node is a tool that allows users to run their own GaiaNet node, enabling them to interact with an AI agent. The tool provides functionalities to install the default node software stack, initialize the node with model files and vector database files, start the node, stop the node, and update configurations. Users can use pre-set configurations or pass a custom URL for initialization. The tool is designed to facilitate communication with the AI agent and access node information via a browser. GaiaNet-node requires sudo privilege for installation but can also be installed without sudo privileges with specific commands.
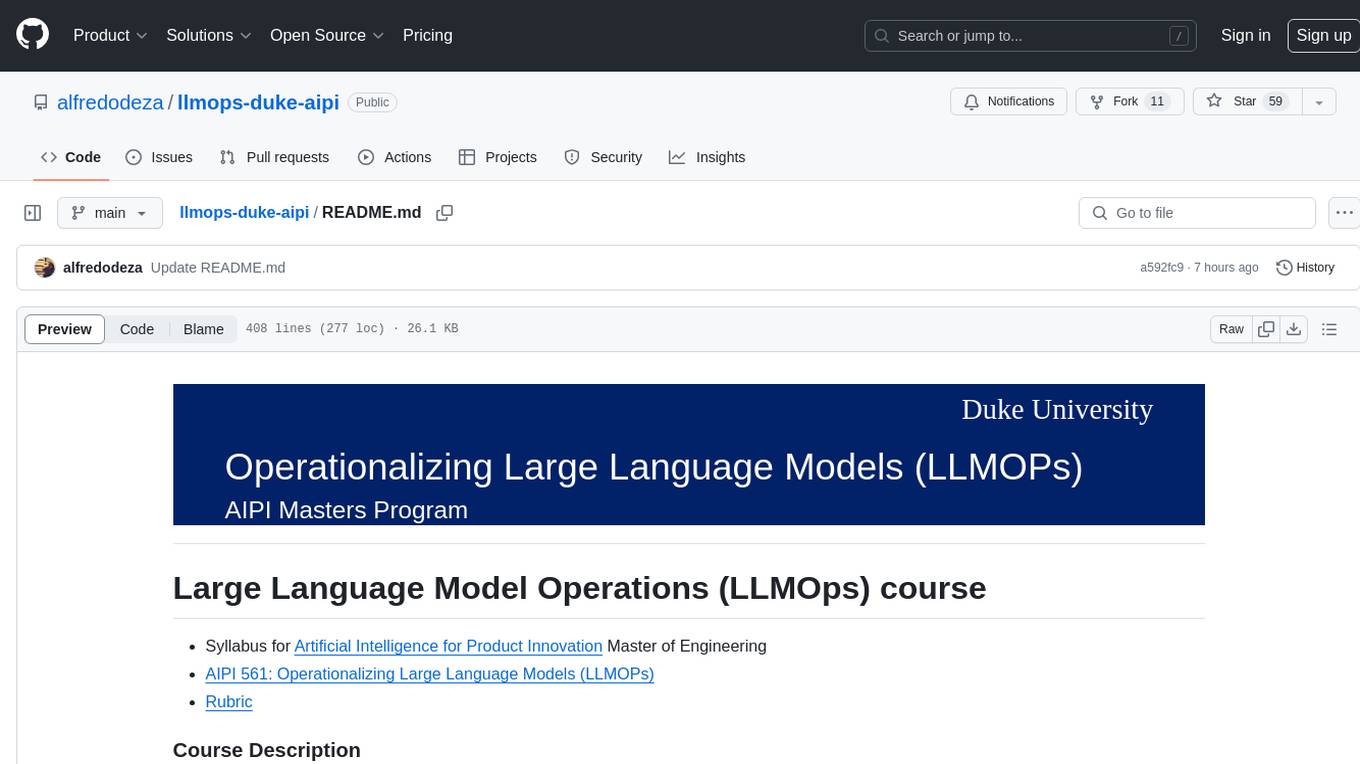
llmops-duke-aipi
LLMOps Duke AIPI is a course focused on operationalizing Large Language Models, teaching methodologies for developing applications using software development best practices with large language models. The course covers various topics such as generative AI concepts, setting up development environments, interacting with large language models, using local large language models, applied solutions with LLMs, extensibility using plugins and functions, retrieval augmented generation, introduction to Python web frameworks for APIs, DevOps principles, deploying machine learning APIs, LLM platforms, and final presentations. Students will learn to build, share, and present portfolios using Github, YouTube, and Linkedin, as well as develop non-linear life-long learning skills. Prerequisites include basic Linux and programming skills, with coursework available in Python or Rust. Additional resources and references are provided for further learning and exploration.
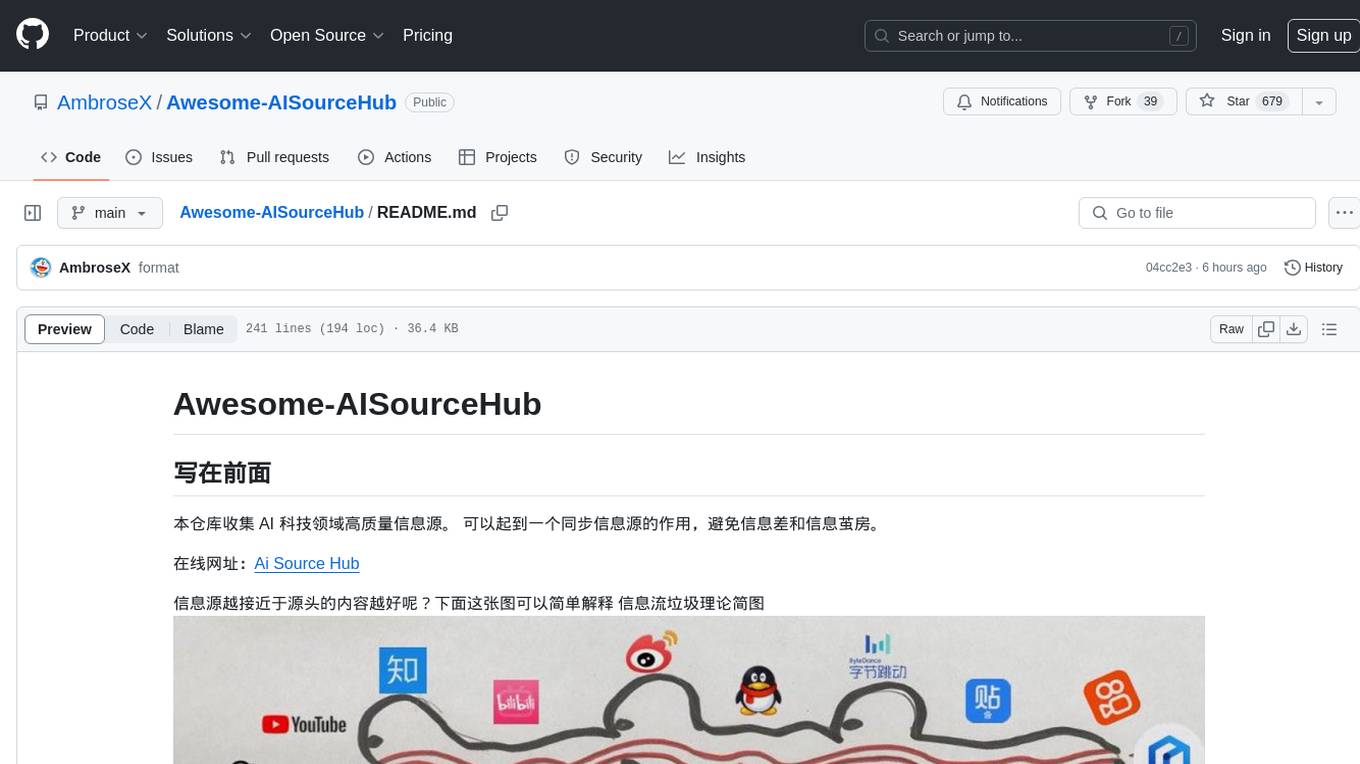
Awesome-AISourceHub
Awesome-AISourceHub is a repository that collects high-quality information sources in the field of AI technology. It serves as a synchronized source of information to avoid information gaps and information silos. The repository aims to provide valuable resources for individuals such as AI book authors, enterprise decision-makers, and tool developers who frequently use Twitter to share insights and updates related to AI advancements. The platform emphasizes the importance of accessing information closer to the source for better quality content. Users can contribute their own high-quality information sources to the repository by following specific steps outlined in the contribution guidelines. The repository covers various platforms such as Twitter, public accounts, knowledge planets, podcasts, blogs, websites, YouTube channels, and more, offering a comprehensive collection of AI-related resources for individuals interested in staying updated with the latest trends and developments in the AI field.