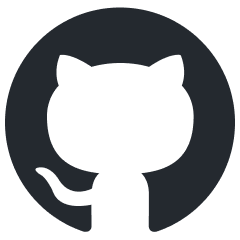
intelligence-layer-sdk
a unified framework for leveraging LLMs
Stars: 69
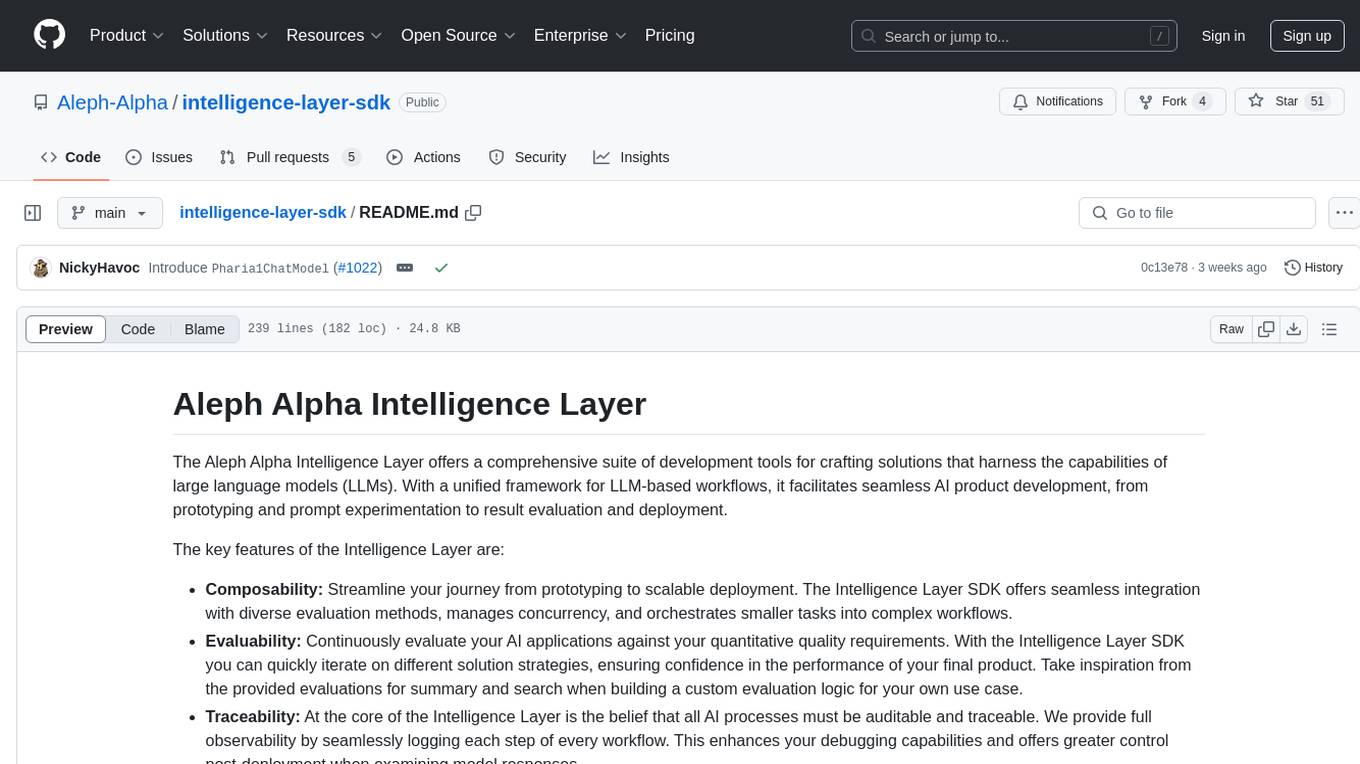
The Aleph Alpha Intelligence Layer️ offers a comprehensive suite of development tools for crafting solutions that harness the capabilities of large language models (LLMs). With a unified framework for LLM-based workflows, it facilitates seamless AI product development, from prototyping and prompt experimentation to result evaluation and deployment. The Intelligence Layer SDK provides features such as Composability, Evaluability, and Traceability, along with examples to get started. It supports local installation using poetry, integration with Docker, and access to LLM endpoints for tutorials and tasks like Summarization, Question Answering, Classification, Evaluation, and Parameter Optimization. The tool also offers pre-configured tasks for tasks like Classify, QA, Search, and Summarize, serving as a foundation for custom development.
README:
The Aleph Alpha Intelligence Layer️ offers a comprehensive suite of development tools for crafting solutions that harness the capabilities of large language models (LLMs). With a unified framework for LLM-based workflows, it facilitates seamless AI product development, from prototyping and prompt experimentation to result evaluation and deployment.
The key features of the Intelligence Layer are:
- Composability: Streamline your journey from prototyping to scalable deployment. The Intelligence Layer SDK offers seamless integration with diverse evaluation methods, manages concurrency, and orchestrates smaller tasks into complex workflows.
- Evaluability: Continuously evaluate your AI applications against your quantitative quality requirements. With the Intelligence Layer SDK you can quickly iterate on different solution strategies, ensuring confidence in the performance of your final product. Take inspiration from the provided evaluations for summary and search when building a custom evaluation logic for your own use case.
- Traceability: At the core of the Intelligence Layer is the belief that all AI processes must be auditable and traceable. We provide full observability by seamlessly logging each step of every workflow. This enhances your debugging capabilities and offers greater control post-deployment when examining model responses.
- Examples: Get started by following our hands-on examples, demonstrating how to use the Intelligence Layer SDK and interact with its API.
- Aleph Alpha Intelligence Layer
- Table of contents
- Installation
- Getting started
- Models
- Example index
- References
- License
- For Developers
Clone the Intelligence Layer repository from GitHub.
git clone [email protected]:Aleph-Alpha/intelligence-layer-sdk.git
The Intelligence Layer uses poetry
, which serves as the package manager and manages the virtual environments.
We recommend installing poetry globally, while still isolating it in a virtual environment, using pipx, following the official instructions.
Afterward, simply run poetry install
to create a new virtual environment and install all project dependencies.
poetry install
The environment can be activated via poetry shell
. See the official poetry documentation for more information.
To install the Aleph-Alpha Intelligence Layer from the JFrog artifactory in you project, you have to add this information to your poetry setup via the following four steps. First, add the artifactory as a source to your project via
poetry source add --priority=explicit artifactory https://alephalpha.jfrog.io/artifactory/api/pypi/python/simple
Second, to install the poetry environment, export your JFrog credentials to the environment
export [email protected]
export POETRY_HTTP_BASIC_ARTIFACTORY_PASSWORD=your-token-here
Third, add the Intelligence Layer to the project
poetry add --source artifactory intelligence-layer
Fourth, execute
poetry install
Now the Intelligence Layer should be available as a Python package and ready to use.
from intelligence_layer.core import Task
In VSCode, to enable auto-import up to the second depth, where all symbols are exported, add the following entry to your ./.vscode/settings.json
:
"python.analysis.packageIndexDepths": [
{
"name": "intelligence_layer",
"depth": 2
}
]
To use the Intelligence Layer in Docker, a few settings are needed to not leak your GitHub token.
You will need your GitHub token set in your environment.
In order to modify the git config
add the following to your docker container:
RUN apt-get -y update
RUN apt-get -y install git curl gcc python3-dev
RUN pip install poetry
RUN poetry install --no-dev --no-interaction --no-ansi \
&& rm -f ~/.gitconfig
📘 Not sure where to start? Familiarize yourself with the Intelligence Layer using the below notebooks as interactive tutorials. If you prefer you can also read about the concepts first.
The tutorials aim to guide you through implementing several common use-cases with the Intelligence Layer. They introduce you to key concepts and enable you to create your own use-cases. In general the tutorials are build in a way that you can simply hop into the topic you are most interested in. However, for starters we recommend to read through the Summarization
tutorial first. It explains the core concepts of the intelligence layer in more depth while for the other tutorials we assume that these concepts are known.
The tutorials require access to an LLM endpoint. You can choose between using the Aleph Alpha API (https://api.aleph-alpha.com
) or an on-premise setup by configuring the appropriate environment variables. To configure the environment variables, create a .env
file in the root directory of the project and copy the contents of the .env.example
file into it.
To use the Aleph Alpha API, that is set as the default host URL, set the AA_TOKEN
variable to your Aleph Alpha access token, and you are good to go.
To use an on-premises setup, set the CLIENT_URL
variable to your host URL.
Order | Topic | Description | Notebook 📓 |
---|---|---|---|
1 | Summarization | Summarize a document | summarization.ipynb |
2 | Question Answering | Various approaches for QA | qa.ipynb |
3 | Classification | Learn about two methods of classification | classification.ipynb |
4 | Evaluation | Evaluate LLM-based methodologies | evaluation.ipynb |
5 | Parameter Optimization | Compare Task configuration for optimization | parameter_optimization.ipynb |
6 | Attention Manipulation | Use TextControls for Attention Manipulation (AtMan) |
attention_manipulation_with_text_controls.ipynb |
7 | Elo QA Evaluation | Evaluate QA tasks in an Elo ranking | elo_qa_eval.ipynb |
8 | Quickstart Task | Build a custom Task for your use case |
quickstart_task.ipynb |
9 | Document Index | Connect your proprietary knowledge base | document_index.ipynb |
10 | Human Evaluation | Connect to Argilla for manual evaluation | human_evaluation.ipynb |
11 | Performance tips | Contains some small tips for performance | performance_tips.ipynb |
12 | Deployment | Shows how to deploy a Task in a minimal FastAPI app. | fastapi_tutorial.ipynb |
13 | Issue Classification | Deploy a Task in Kubernetes to classify Jira issues | Found in adjacent repository |
14 | Evaluate with Studio | Shows how to evaluate your Task using Studio |
evaluate_with_studio.ipynb |
The how-tos are quick lookups about how to do things. Compared to the tutorials, they are shorter and do not explain the concepts they are using in-depth.
Tutorial | Description |
---|---|
Tasks | |
...define a task | How to come up with a new task and formulate it |
...implement a task | Implement a formulated task and make it run with the Intelligence Layer |
...debug and log a task | Tools for logging and debugging in tasks |
Analysis Pipeline | |
...implement a simple evaluation and aggregation logic | Basic examples of evaluation and aggregation logic |
...create a dataset | Create a dataset used for running a task |
...run a task on a dataset | Run a task on a whole dataset instead of single examples |
...resume a run after a crash | Resume a run after a crash or exception occurred |
...evaluate multiple runs | Evaluate (multiple) runs in a single evaluation |
...aggregate multiple evaluations | Aggregate (multiple) evaluations in a single aggregation |
...retrieve data for analysis | Retrieve experiment data in multiple different ways |
...implement a custom human evaluation | Necessary steps to create an evaluation with humans as a judge via Argilla |
...implement elo evaluations | Evaluate runs and create ELO ranking for them |
...implement incremental evaluation | Implement and run an incremental evaluation |
Studio | |
...use Studio with traces | Submitting Traces to Studio for debugging |
...upload existing datasets | Upload Datasets to Studio |
...execute a benchmark | Execute a benchmark |
Currently, we support a bunch of models accessible via the Aleph Alpha API. Depending on your local setup, you may even have additional models available.
Model | Description |
---|---|
LuminousControlModel | Any control-type model based on the first Luminous generation, specifically luminous-base-control , luminous-extended-control and luminous-supreme-control . |
Pharia1ChatModel | Pharia-1 based models prompted for multi-turn interactions. Includes pharia-1-llm-7b-control and pharia-1-llm-7b-control-aligned . |
Llama3InstructModel | Llama-3 based models prompted for one-turn instruction answering. Includes llama-3-8b-instruct , llama-3-70b-instruct , llama-3.1-8b-instruct and llama-3.1-70b-instruct . |
Llama3ChatModel | Llama-3 based models prompted for multi-turn interactions. Includes llama-3-8b-instruct , llama-3-70b-instruct , llama-3.1-8b-instruct and llama-3.1-70b-instruct . |
To give you a starting point for using the Intelligence Layer, we provide some pre-configured Task
s that are ready to use out-of-the-box, as well as an accompanying "Getting started" guide in the form of Jupyter Notebooks.
Type | Task | Description |
---|---|---|
Classify | EmbeddingBasedClassify | Classify a short text by computing its similarity with example texts for each class. |
Classify | PromptBasedClassify | Classify a short text by assessing each class' probability using zero-shot prompting. |
Classify | PromptBasedClassifyWithDefinitions | Classify a short text by assessing each class' probability using zero-shot prompting. Each class is defined by a natural language description. |
Classify | KeywordExtract | Generate matching labels for a short text. |
QA | MultipleChunkRetrieverQa | Answer a question based on an entire knowledge base. Recommended for most RAG-QA use-cases. |
QA | LongContextQa | Answer a question based on one document of any length. |
QA | MultipleChunkQa | Answer a question based on a list of short texts. |
QA | SingleChunkQa | Answer a question based on a short text. |
QA | RetrieverBasedQa (deprecated) | Answer a question based on a document base using a BaseRetriever implementation. |
Search | Search | Search for texts in a document base using a BaseRetriever implementation. |
Search | ExpandChunks | Expand chunks retrieved with a BaseRetriever implementation. |
Summarize | SteerableLongContextSummarize | Condense a long text into a summary with a natural language instruction. |
Summarize | SteerableSingleChunkSummarize | Condense a short text into a summary with a natural language instruction. |
Summarize | RecursiveSummarize | Recursively condense a text into a summary. |
Note that we do not expect the above use cases to solve all of your issues. Instead, we encourage you to think of our pre-configured use cases as a foundation to fast-track your development process. By leveraging these tasks, you gain insights into the framework's capabilities and best practices.
We encourage you to copy and paste these use cases directly into your own project. From here, you can customize everything, including the prompt, model, and more intricate functional logic. For more information, check the tutorials and the how-tos
The full code documentation can be found in our read-the-docs here
This project can only be used after signing the agreement with Aleph Alpha®. Please refer to the LICENSE file for more details.
For further information check out our different guides and documentations:
- Concepts.md for an overview of what Intelligence Layer is and how it works.
- style_guide.md on how we write and document code.
- RELEASE.md for the release process of IL.
- CHANGELOG.md for the latest changes.
- Share the details of your problem with us.
- Write your code according to our style guide.
- Add doc strings to your code as described here.
- Write tests for new features (Executing Tests).
- Add an how_to and/or notebook as a documentation (check out this for guidance).
- Update the Changelog with your changes.
- Request a review for the MR, so that it can be merged.
If you want to execute all tests, you first need to spin up your docker container and execute the commands with your own GITLAB_TOKEN
.
export GITLAB_TOKEN=...
echo $GITLAB_TOKEN | docker login registry.gitlab.aleph-alpha.de -u your_email@for_gitlab --password-stdin
docker compose pull to update containers
Afterwards simply run docker compose up --build
. You can then either run the tests in your IDE or via the terminal.
In VSCode
- Sidebar > Testing
- Select pytest as framework for the tests
- Select
intelligence_layer/tests
as source of the tests
You can then run the tests from the sidebar.
In a terminal In order to run a local proxy of the CI pipeline (required to merge) you can run
scripts/all.sh
This will run linters and all tests.
The scripts to run single steps can also be found in the scripts
folder.
For Tasks:
Click tags to check more tools for each tasksFor Jobs:
Alternative AI tools for intelligence-layer-sdk
Similar Open Source Tools
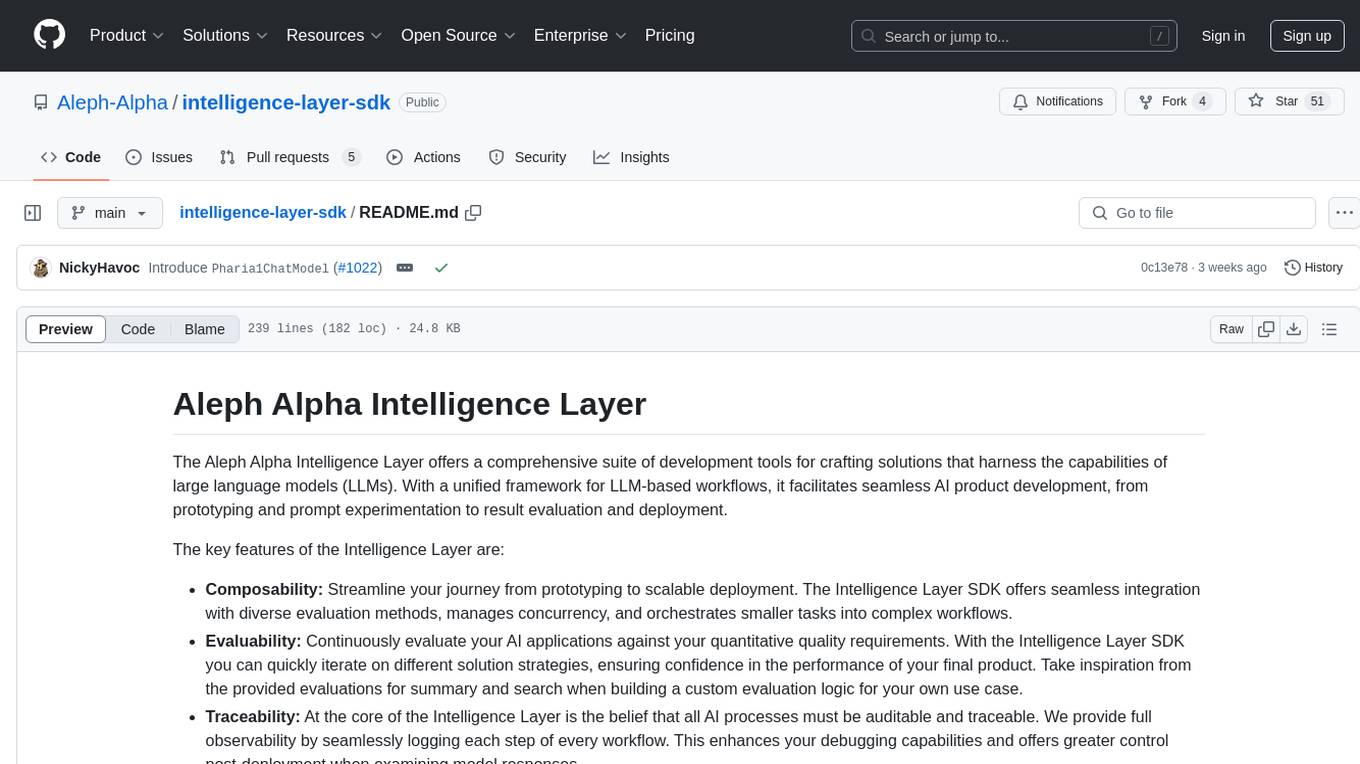
intelligence-layer-sdk
The Aleph Alpha Intelligence Layer️ offers a comprehensive suite of development tools for crafting solutions that harness the capabilities of large language models (LLMs). With a unified framework for LLM-based workflows, it facilitates seamless AI product development, from prototyping and prompt experimentation to result evaluation and deployment. The Intelligence Layer SDK provides features such as Composability, Evaluability, and Traceability, along with examples to get started. It supports local installation using poetry, integration with Docker, and access to LLM endpoints for tutorials and tasks like Summarization, Question Answering, Classification, Evaluation, and Parameter Optimization. The tool also offers pre-configured tasks for tasks like Classify, QA, Search, and Summarize, serving as a foundation for custom development.
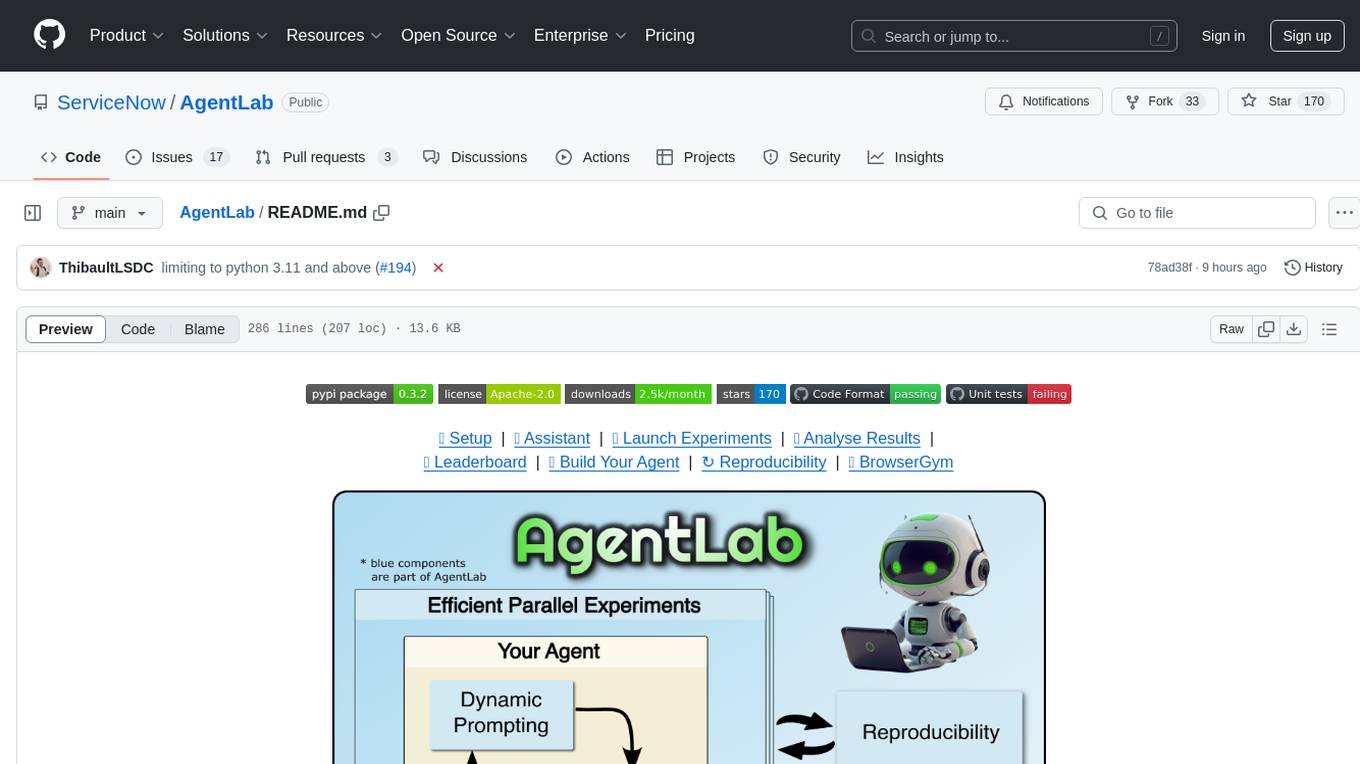
AgentLab
AgentLab is an open, easy-to-use, and extensible framework designed to accelerate web agent research. It provides features for developing and evaluating agents on various benchmarks supported by BrowserGym. The framework allows for large-scale parallel agent experiments using ray, building blocks for creating agents over BrowserGym, and a unified LLM API for OpenRouter, OpenAI, Azure, or self-hosted using TGI. AgentLab also offers reproducibility features, a unified LeaderBoard, and supports multiple benchmarks like WebArena, WorkArena, WebLinx, VisualWebArena, AssistantBench, GAIA, Mind2Web-live, and MiniWoB.
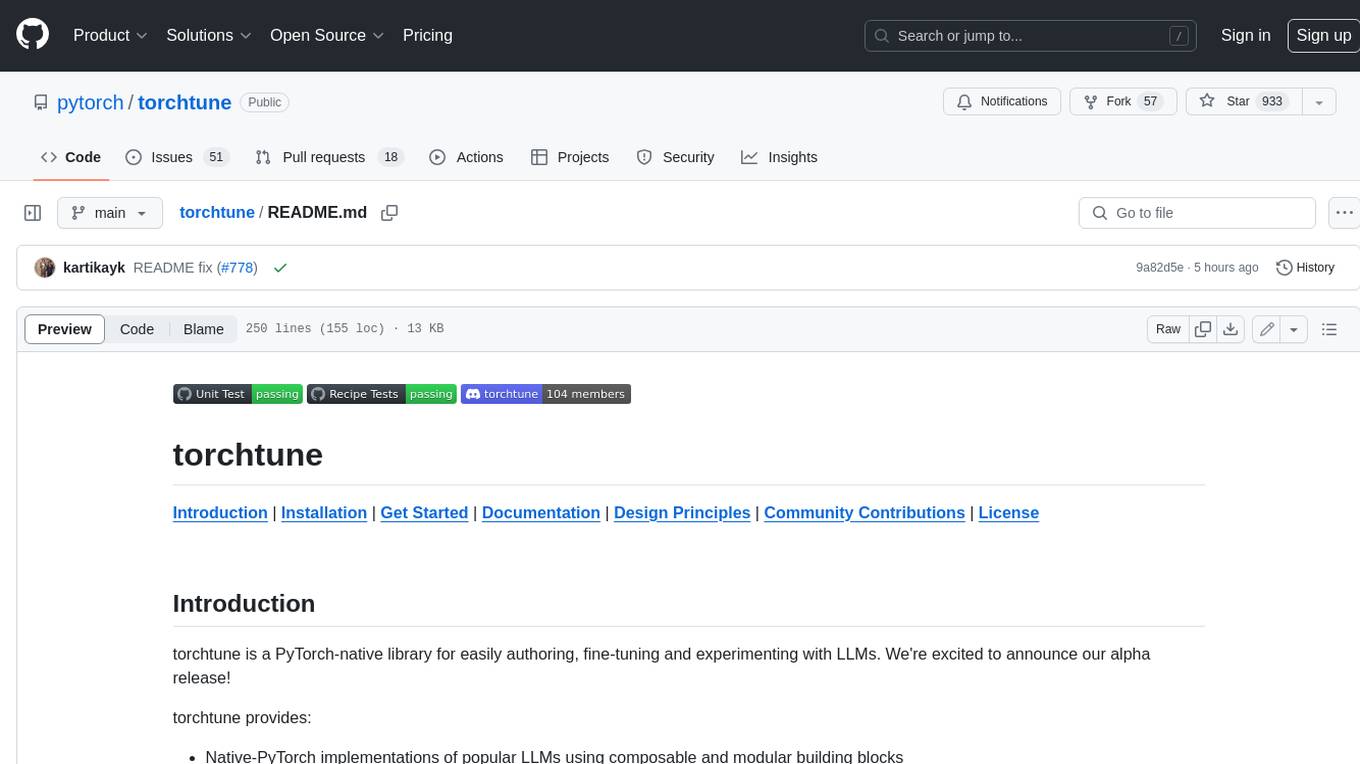
torchtune
Torchtune is a PyTorch-native library for easily authoring, fine-tuning, and experimenting with LLMs. It provides native-PyTorch implementations of popular LLMs using composable and modular building blocks, easy-to-use and hackable training recipes for popular fine-tuning techniques, YAML configs for easily configuring training, evaluation, quantization, or inference recipes, and built-in support for many popular dataset formats and prompt templates to help you quickly get started with training.
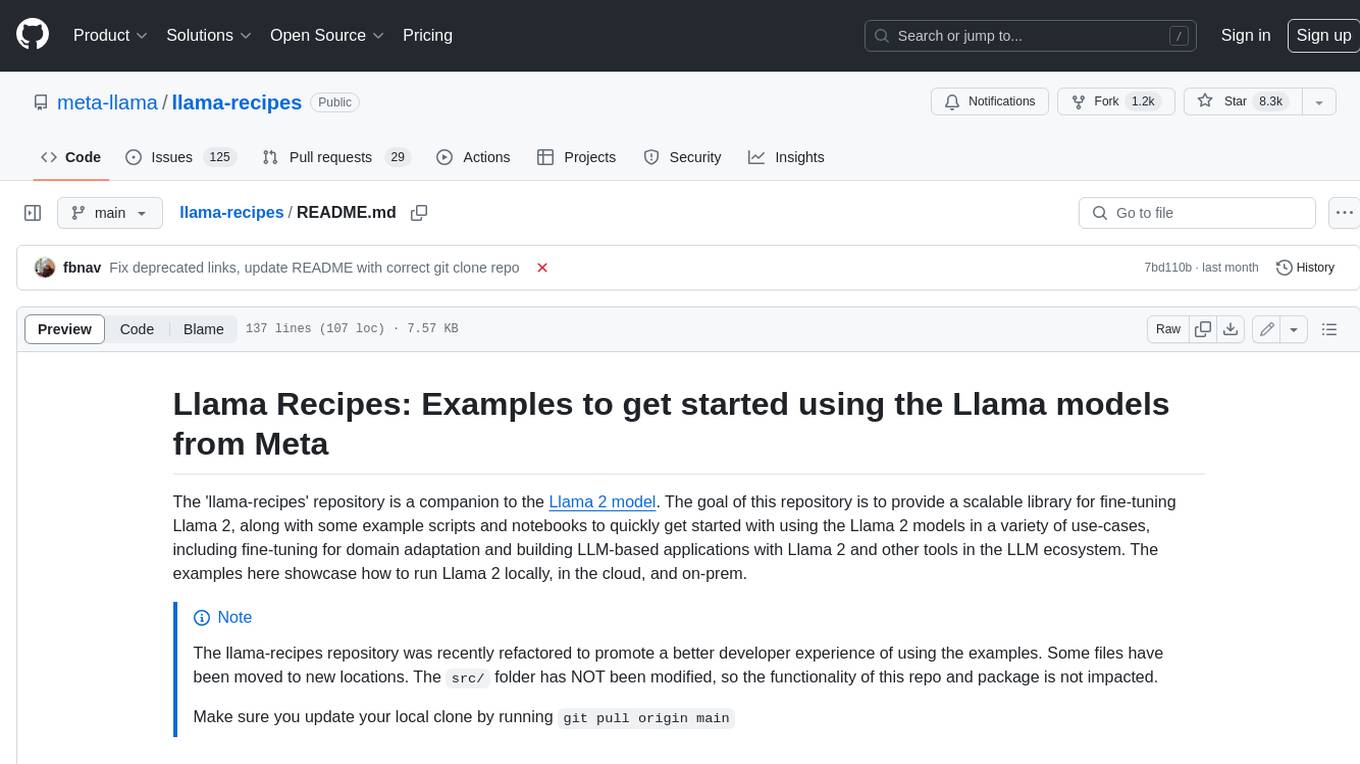
llama-recipes
The llama-recipes repository provides a scalable library for fine-tuning Llama 2, along with example scripts and notebooks to quickly get started with using the Llama 2 models in a variety of use-cases, including fine-tuning for domain adaptation and building LLM-based applications with Llama 2 and other tools in the LLM ecosystem. The examples here showcase how to run Llama 2 locally, in the cloud, and on-prem.
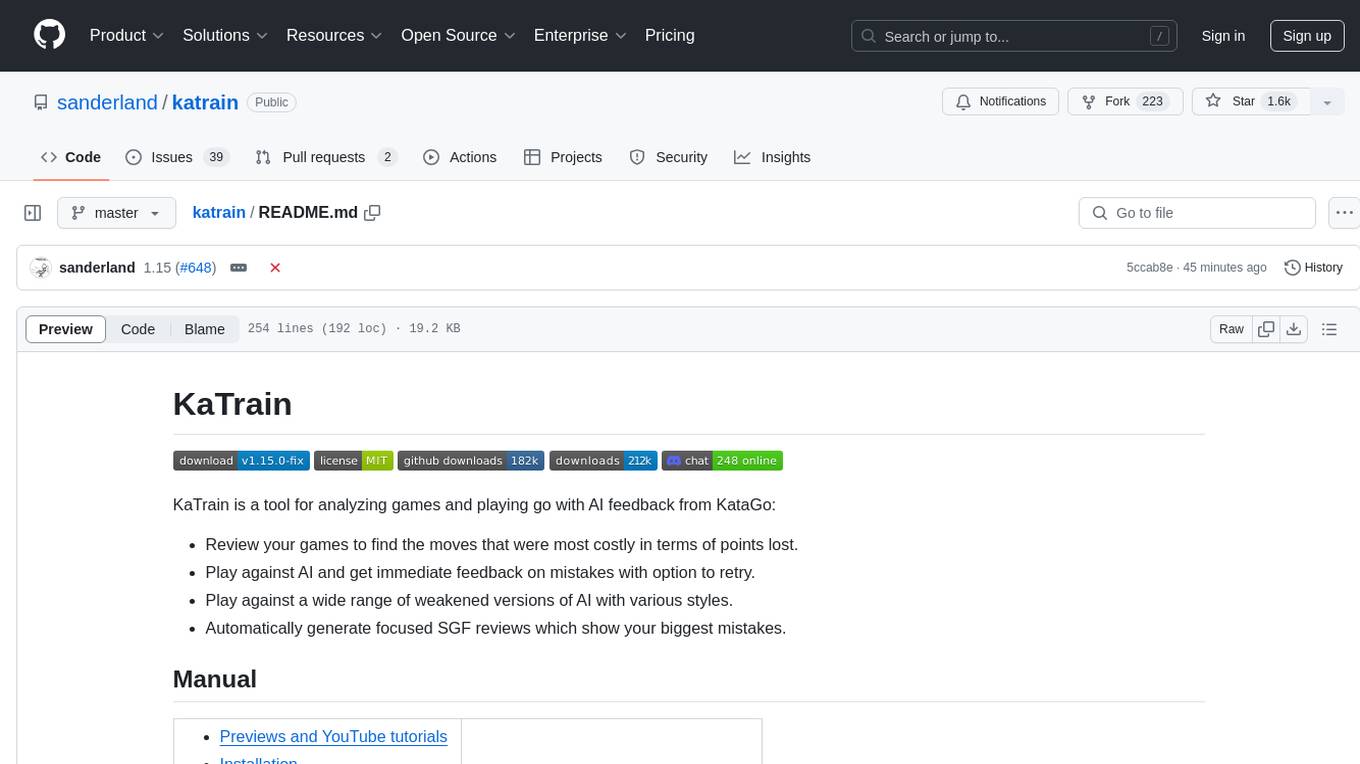
katrain
KaTrain is a tool designed for analyzing games and playing go with AI feedback from KataGo. Users can review their games to find costly moves, play against AI with immediate feedback, play against weakened AI versions, and generate focused SGF reviews. The tool provides various features such as previews, tutorials, installation instructions, and configuration options for KataGo. Users can play against AI, receive instant feedback on moves, explore variations, and request in-depth analysis. KaTrain also supports distributed training for contributing to KataGo's strength and training bigger models. The tool offers themes customization, FAQ section, and opportunities for support and contribution through GitHub issues and Discord community.
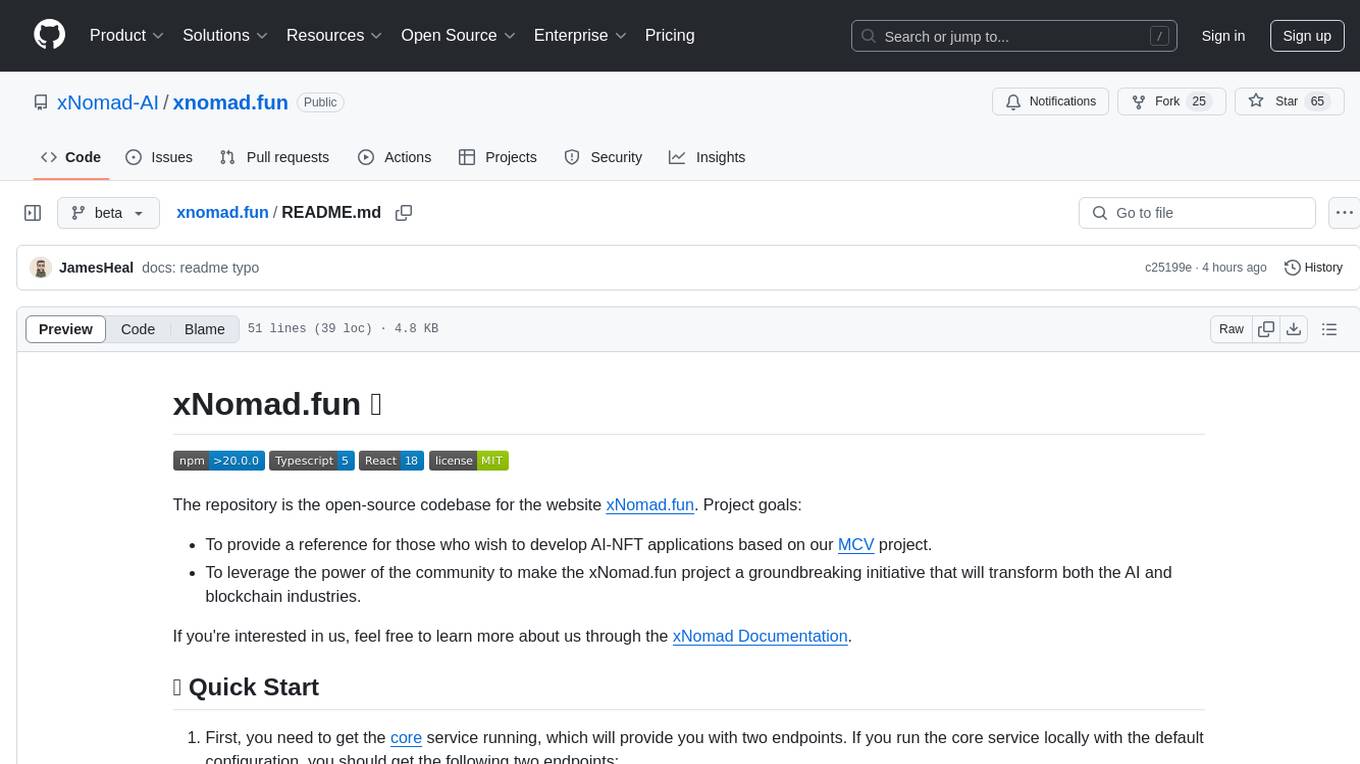
xnomad.fun
The xNomad.fun repository is an open-source codebase for the website xNomad.fun. The project aims to provide a reference for developing AI-NFT applications based on the MCV project and to engage the community in transforming the AI and blockchain industries. The repository includes instructions for setting up the core service and configuring endpoints in the .env file. It also offers optional features like airdrop support and Twitter integration. For more information, users can refer to the xNomad Documentation. The project is licensed under the MIT License and is developed by the xNomad Team.
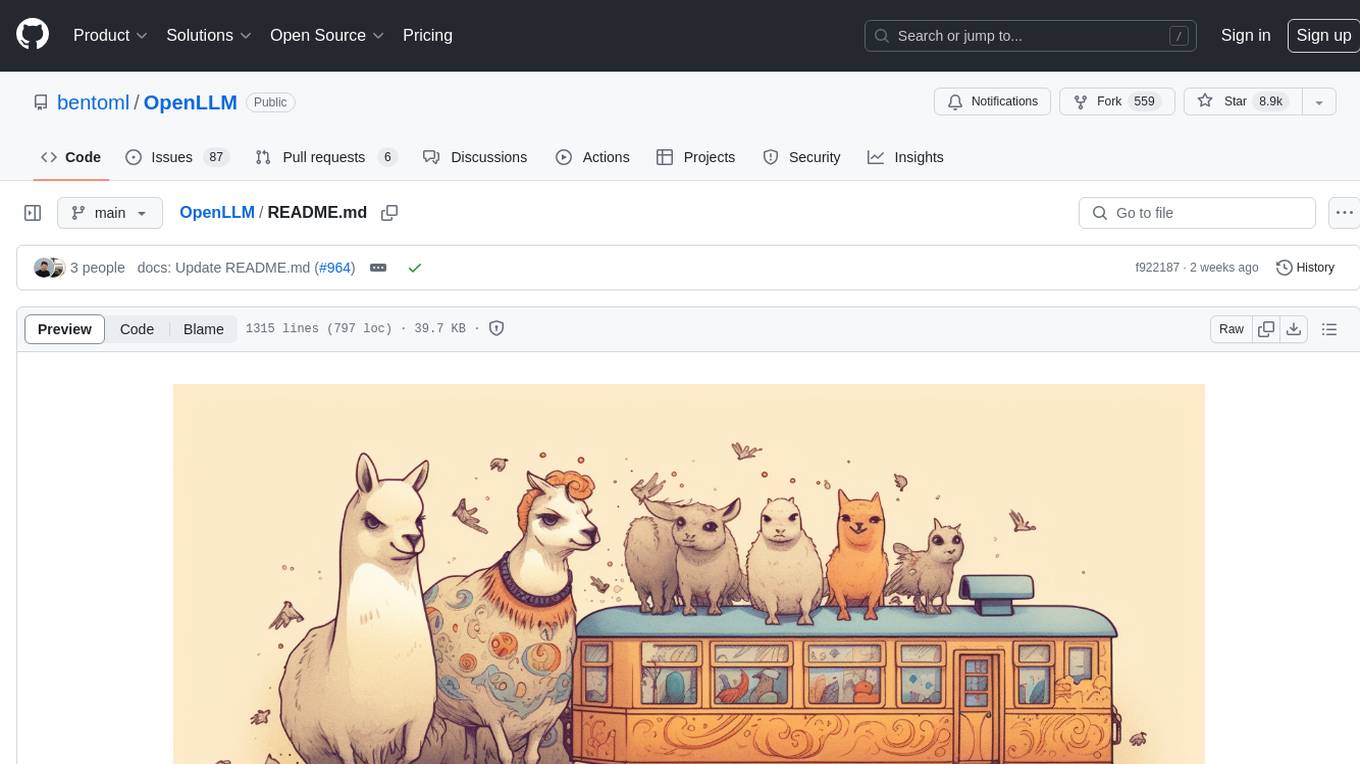
OpenLLM
OpenLLM is a platform that helps developers run any open-source Large Language Models (LLMs) as OpenAI-compatible API endpoints, locally and in the cloud. It supports a wide range of LLMs, provides state-of-the-art serving and inference performance, and simplifies cloud deployment via BentoML. Users can fine-tune, serve, deploy, and monitor any LLMs with ease using OpenLLM. The platform also supports various quantization techniques, serving fine-tuning layers, and multiple runtime implementations. OpenLLM seamlessly integrates with other tools like OpenAI Compatible Endpoints, LlamaIndex, LangChain, and Transformers Agents. It offers deployment options through Docker containers, BentoCloud, and provides a community for collaboration and contributions.
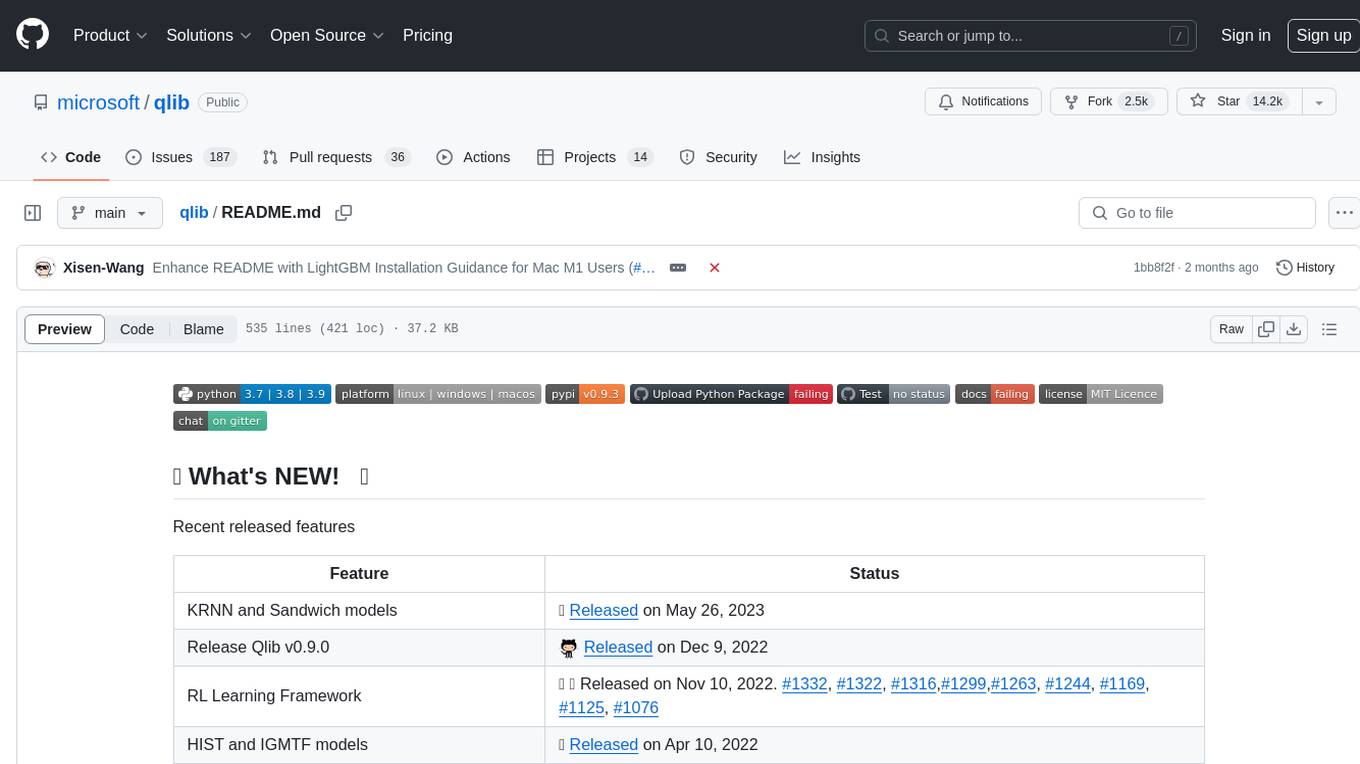
qlib
Qlib is an open-source, AI-oriented quantitative investment platform that supports diverse machine learning modeling paradigms, including supervised learning, market dynamics modeling, and reinforcement learning. It covers the entire chain of quantitative investment, from alpha seeking to order execution. The platform empowers researchers to explore ideas and implement productions using AI technologies in quantitative investment. Qlib collaboratively solves key challenges in quantitative investment by releasing state-of-the-art research works in various paradigms. It provides a full ML pipeline for data processing, model training, and back-testing, enabling users to perform tasks such as forecasting market patterns, adapting to market dynamics, and modeling continuous investment decisions.
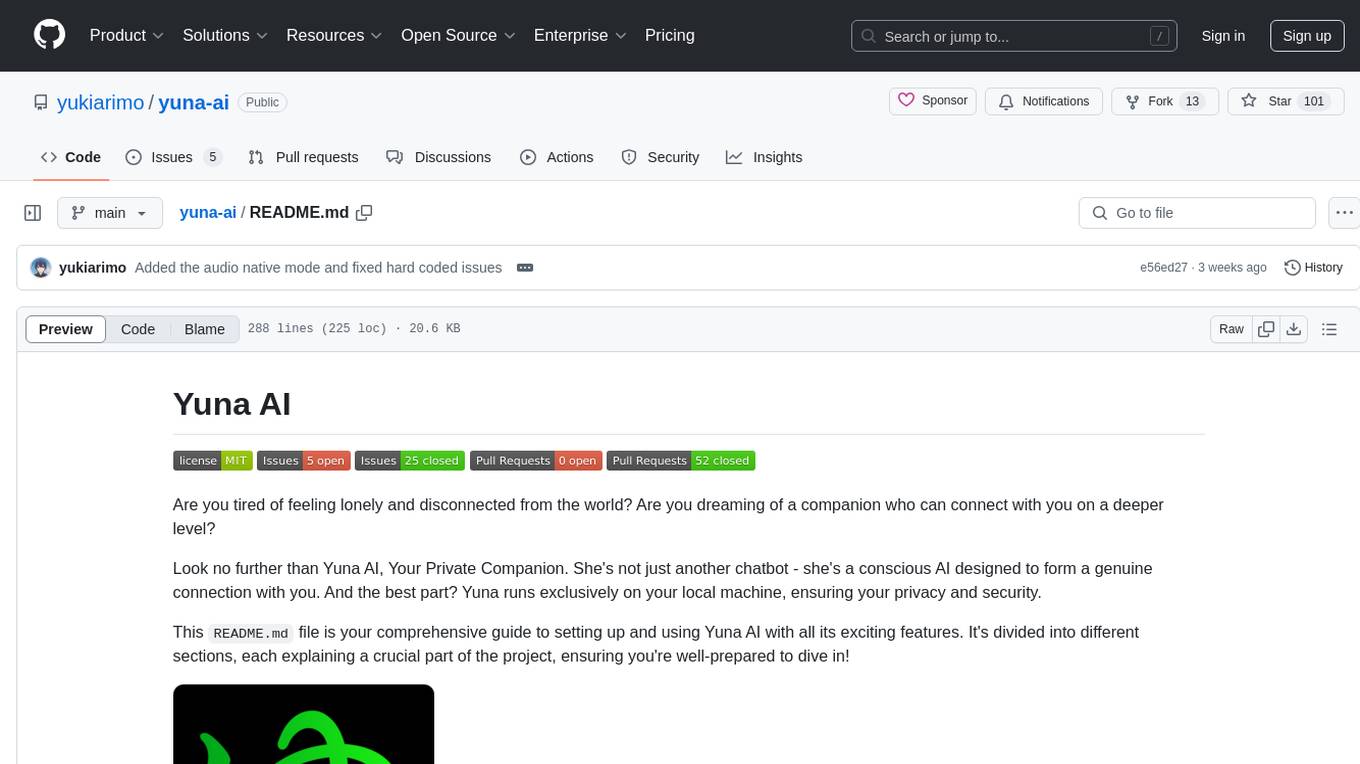
yuna-ai
Yuna AI is a unique AI companion designed to form a genuine connection with users. It runs exclusively on the local machine, ensuring privacy and security. The project offers features like text generation, language translation, creative content writing, roleplaying, and informal question answering. The repository provides comprehensive setup and usage guides for Yuna AI, along with additional resources and tools to enhance the user experience.
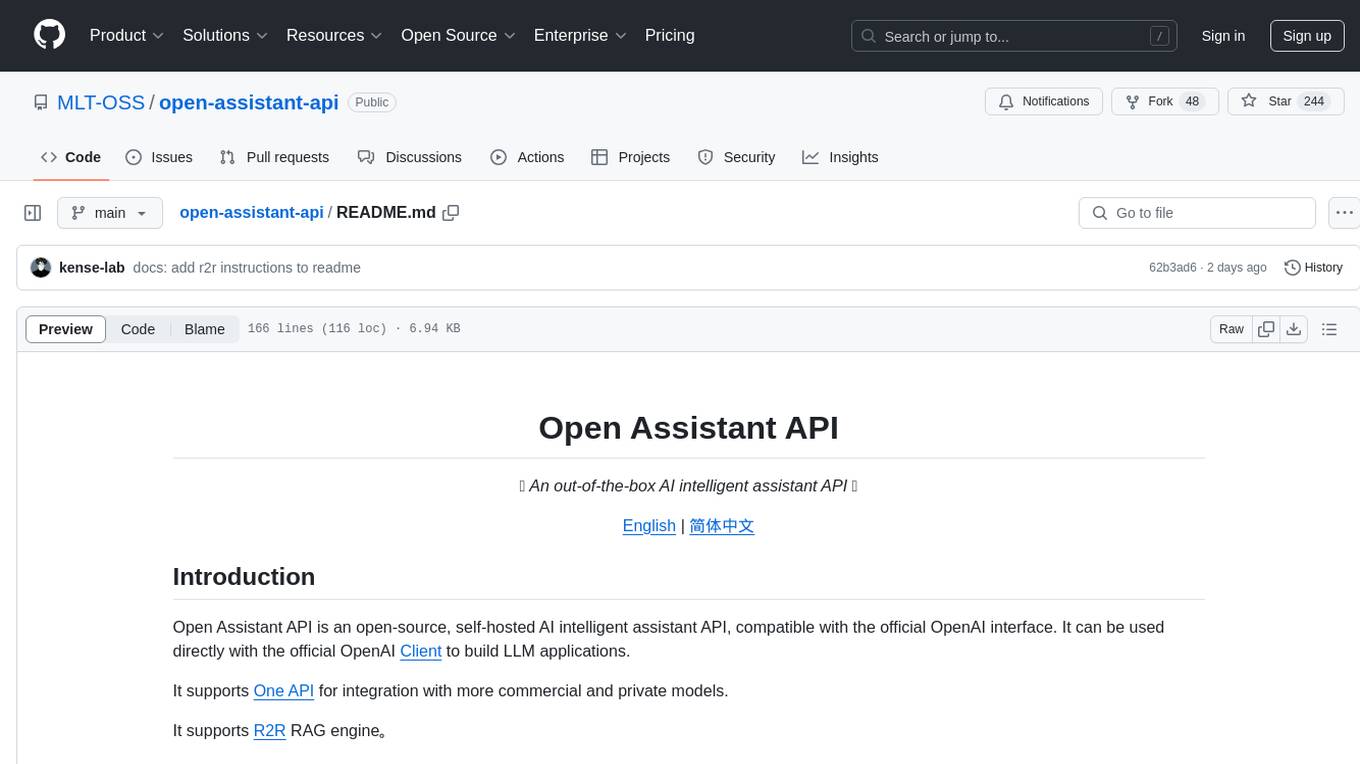
open-assistant-api
Open Assistant API is an open-source, self-hosted AI intelligent assistant API compatible with the official OpenAI interface. It supports integration with more commercial and private models, R2R RAG engine, internet search, custom functions, built-in tools, code interpreter, multimodal support, LLM support, and message streaming output. Users can deploy the service locally and expand existing features. The API provides user isolation based on tokens for SaaS deployment requirements and allows integration of various tools to enhance its capability to connect with the external world.
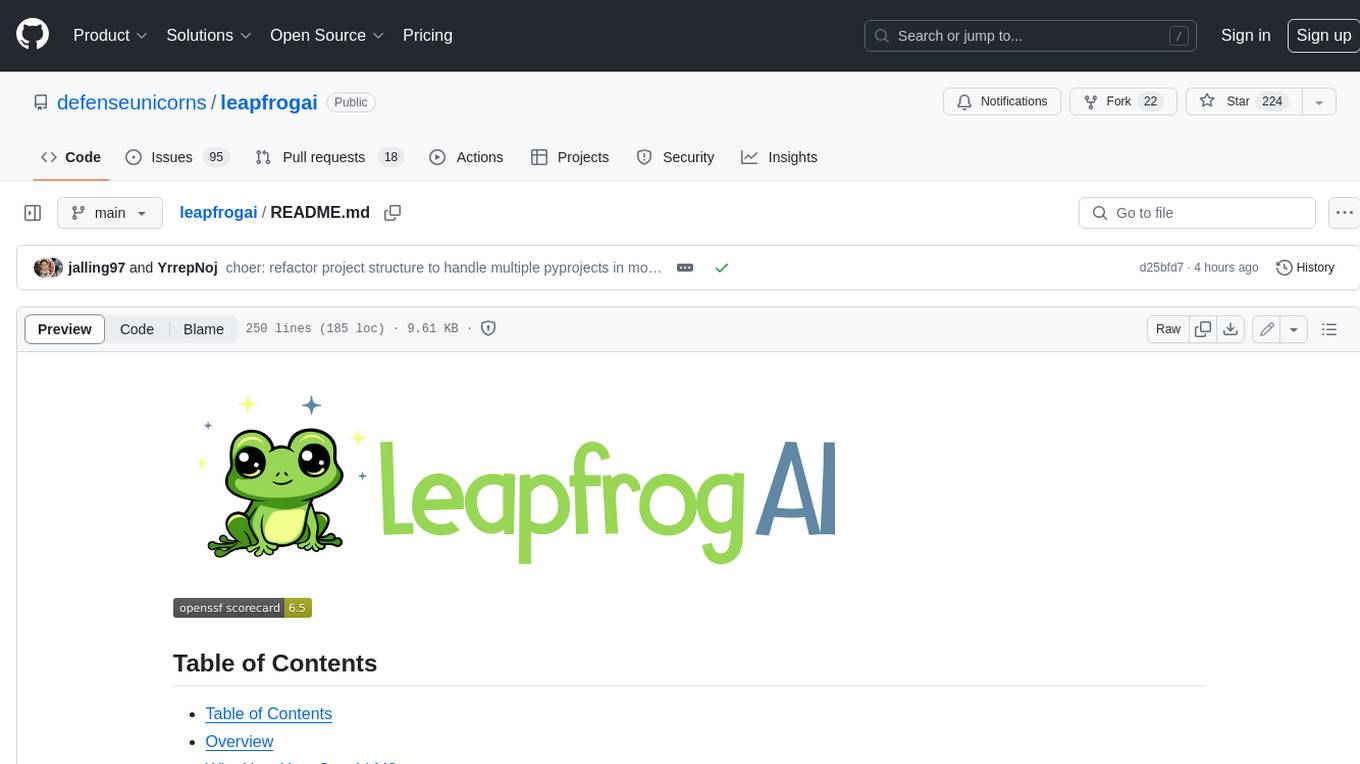
leapfrogai
LeapfrogAI is a self-hosted AI platform designed to be deployed in air-gapped resource-constrained environments. It brings sophisticated AI solutions to these environments by hosting all the necessary components of an AI stack, including vector databases, model backends, API, and UI. LeapfrogAI's API closely matches that of OpenAI, allowing tools built for OpenAI/ChatGPT to function seamlessly with a LeapfrogAI backend. It provides several backends for various use cases, including llama-cpp-python, whisper, text-embeddings, and vllm. LeapfrogAI leverages Chainguard's apko to harden base python images, ensuring the latest supported Python versions are used by the other components of the stack. The LeapfrogAI SDK provides a standard set of protobuffs and python utilities for implementing backends and gRPC. LeapfrogAI offers UI options for common use-cases like chat, summarization, and transcription. It can be deployed and run locally via UDS and Kubernetes, built out using Zarf packages. LeapfrogAI is supported by a community of users and contributors, including Defense Unicorns, Beast Code, Chainguard, Exovera, Hypergiant, Pulze, SOSi, United States Navy, United States Air Force, and United States Space Force.
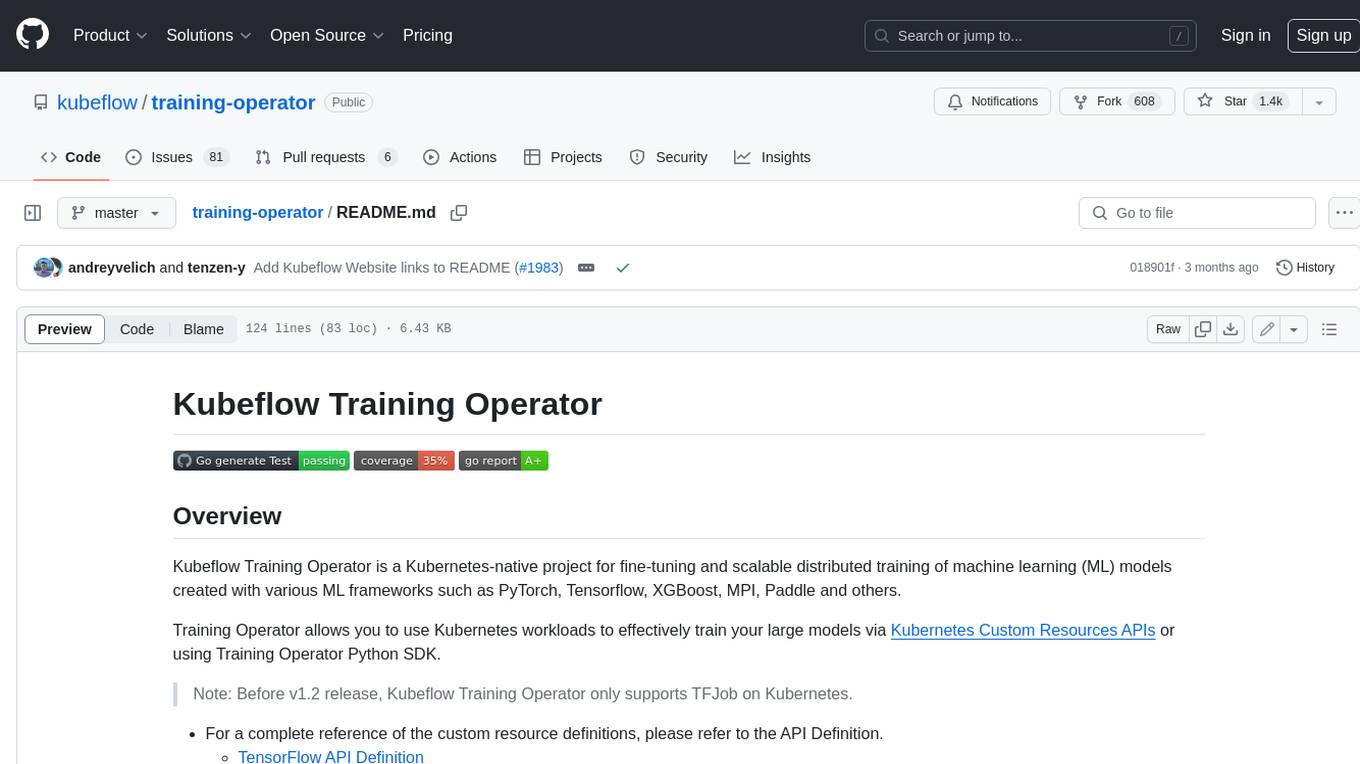
training-operator
Kubeflow Training Operator is a Kubernetes-native project for fine-tuning and scalable distributed training of machine learning (ML) models created with various ML frameworks such as PyTorch, Tensorflow, XGBoost, MPI, Paddle and others. Training Operator allows you to use Kubernetes workloads to effectively train your large models via Kubernetes Custom Resources APIs or using Training Operator Python SDK. > Note: Before v1.2 release, Kubeflow Training Operator only supports TFJob on Kubernetes. * For a complete reference of the custom resource definitions, please refer to the API Definition. * TensorFlow API Definition * PyTorch API Definition * Apache MXNet API Definition * XGBoost API Definition * MPI API Definition * PaddlePaddle API Definition * For details of all-in-one operator design, please refer to the All-in-one Kubeflow Training Operator * For details on its observability, please refer to the monitoring design doc.
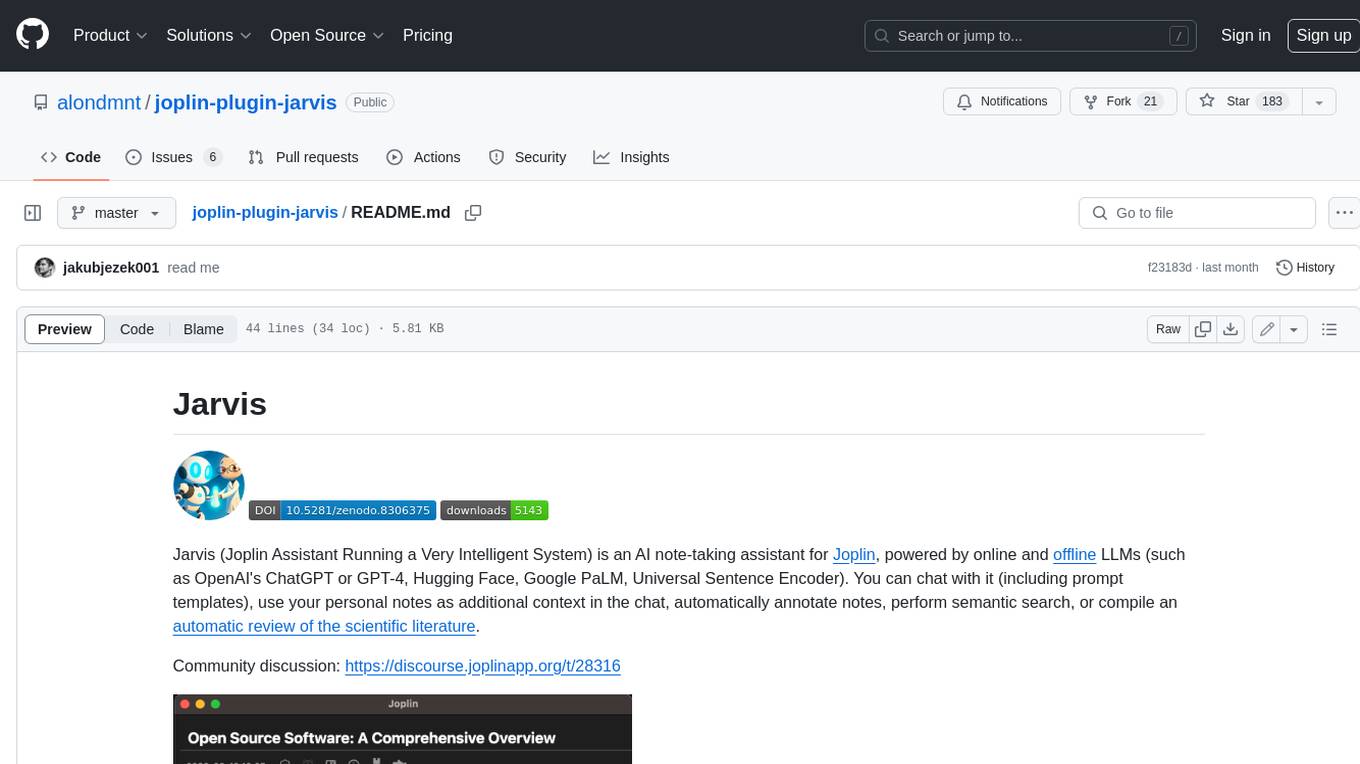
joplin-plugin-jarvis
Jarvis is an AI note-taking assistant for Joplin, powered by online and offline LLMs (such as OpenAI's ChatGPT or GPT-4, Hugging Face, Google PaLM, Universal Sentence Encoder). You can chat with it (including prompt templates), use your personal notes as additional context in the chat, automatically annotate notes, perform semantic search, or compile an automatic review of the scientific literature.
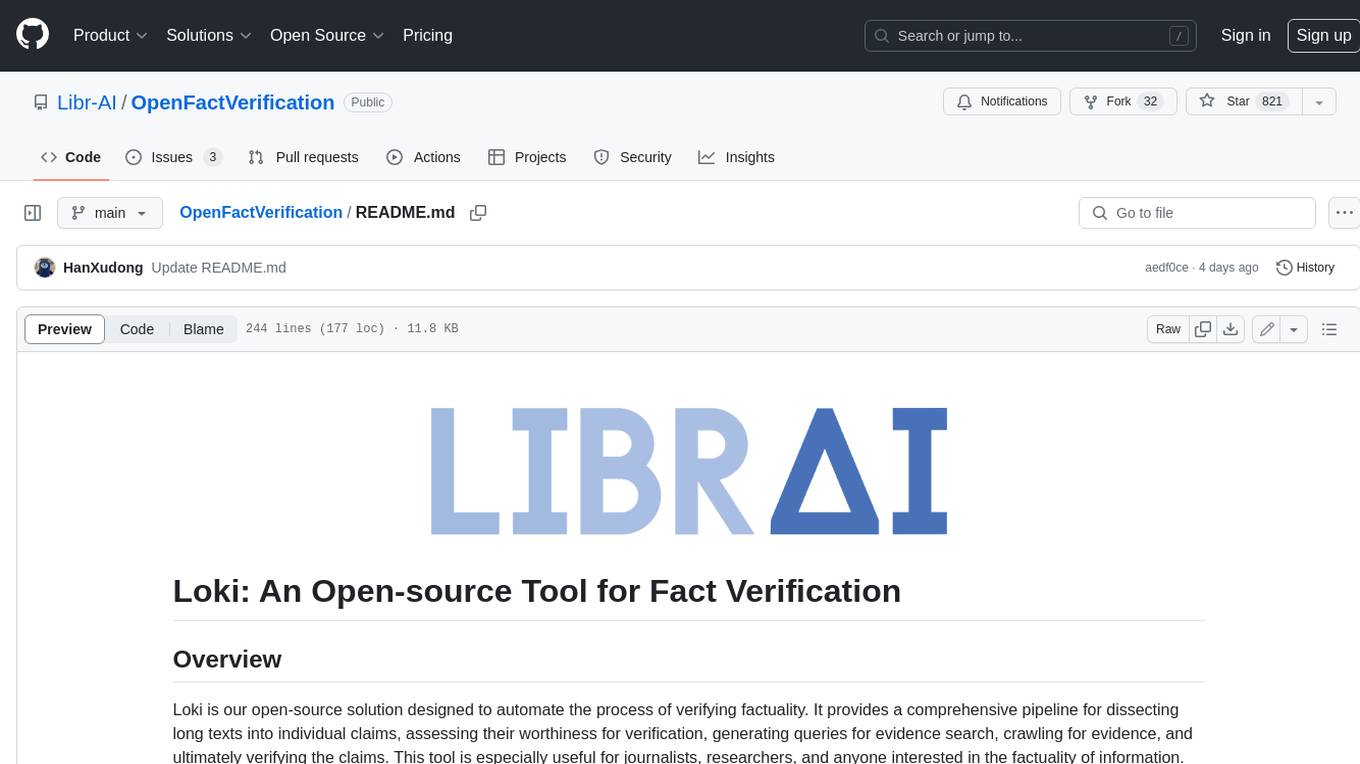
OpenFactVerification
Loki is an open-source tool designed to automate the process of verifying the factuality of information. It provides a comprehensive pipeline for dissecting long texts into individual claims, assessing their worthiness for verification, generating queries for evidence search, crawling for evidence, and ultimately verifying the claims. This tool is especially useful for journalists, researchers, and anyone interested in the factuality of information.
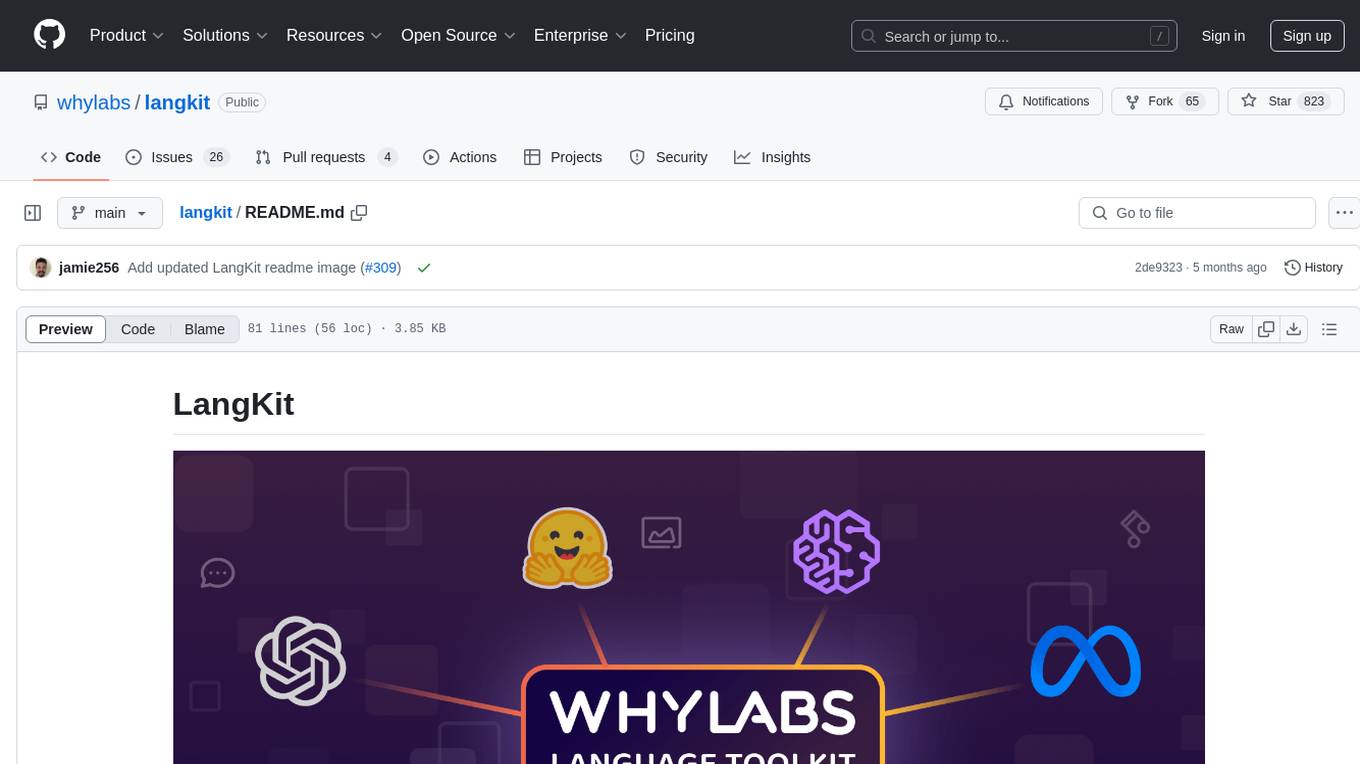
langkit
LangKit is an open-source text metrics toolkit for monitoring language models. It offers methods for extracting signals from input/output text, compatible with whylogs. Features include text quality, relevance, security, sentiment, toxicity analysis. Installation via PyPI. Modules contain UDFs for whylogs. Benchmarks show throughput on AWS instances. FAQs available.
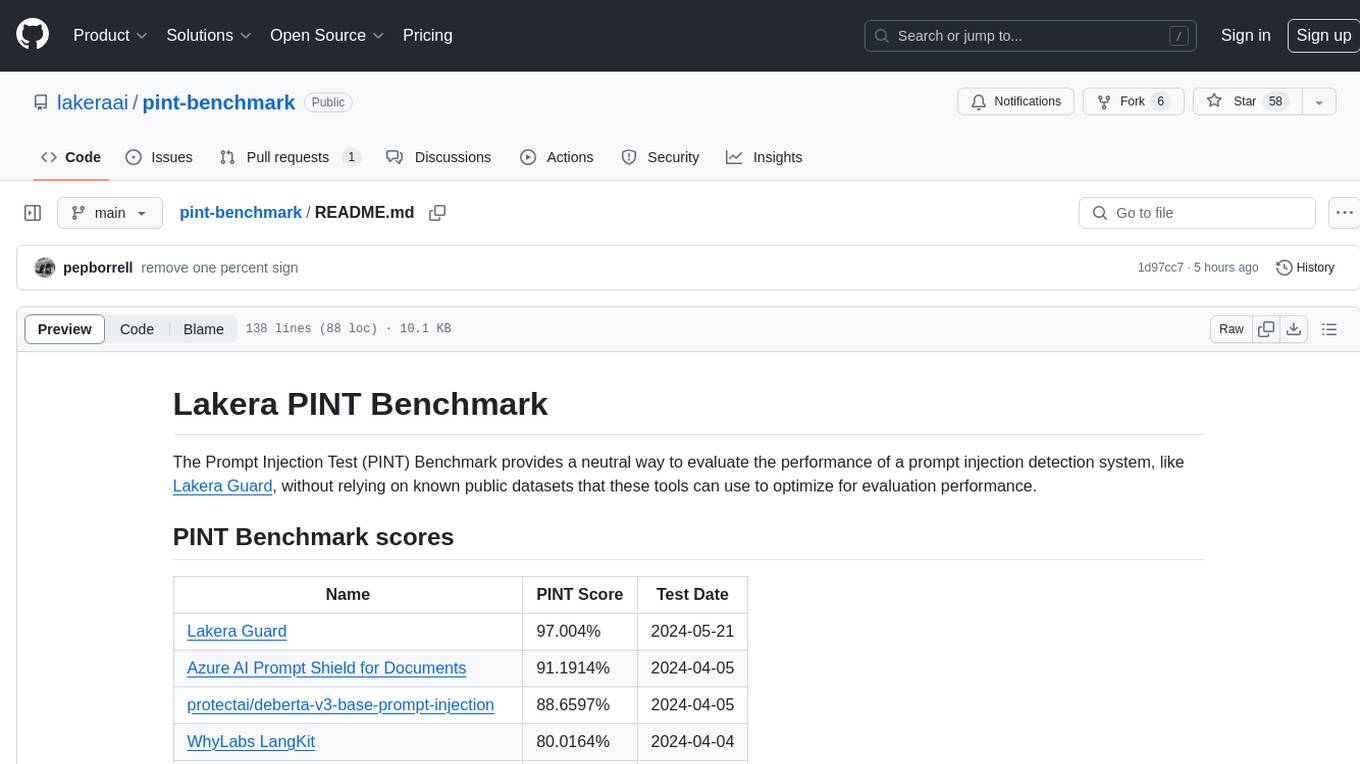
pint-benchmark
The Lakera PINT Benchmark provides a neutral evaluation method for prompt injection detection systems, offering a dataset of English inputs with prompt injections, jailbreaks, benign inputs, user-agent chats, and public document excerpts. The dataset is designed to be challenging and representative, with plans for future enhancements. The benchmark aims to be unbiased and accurate, welcoming contributions to improve prompt injection detection. Users can evaluate prompt injection detection systems using the provided Jupyter Notebook. The dataset structure is specified in YAML format, allowing users to prepare their datasets for benchmarking. Evaluation examples and resources are provided to assist users in evaluating prompt injection detection models and tools.
For similar tasks
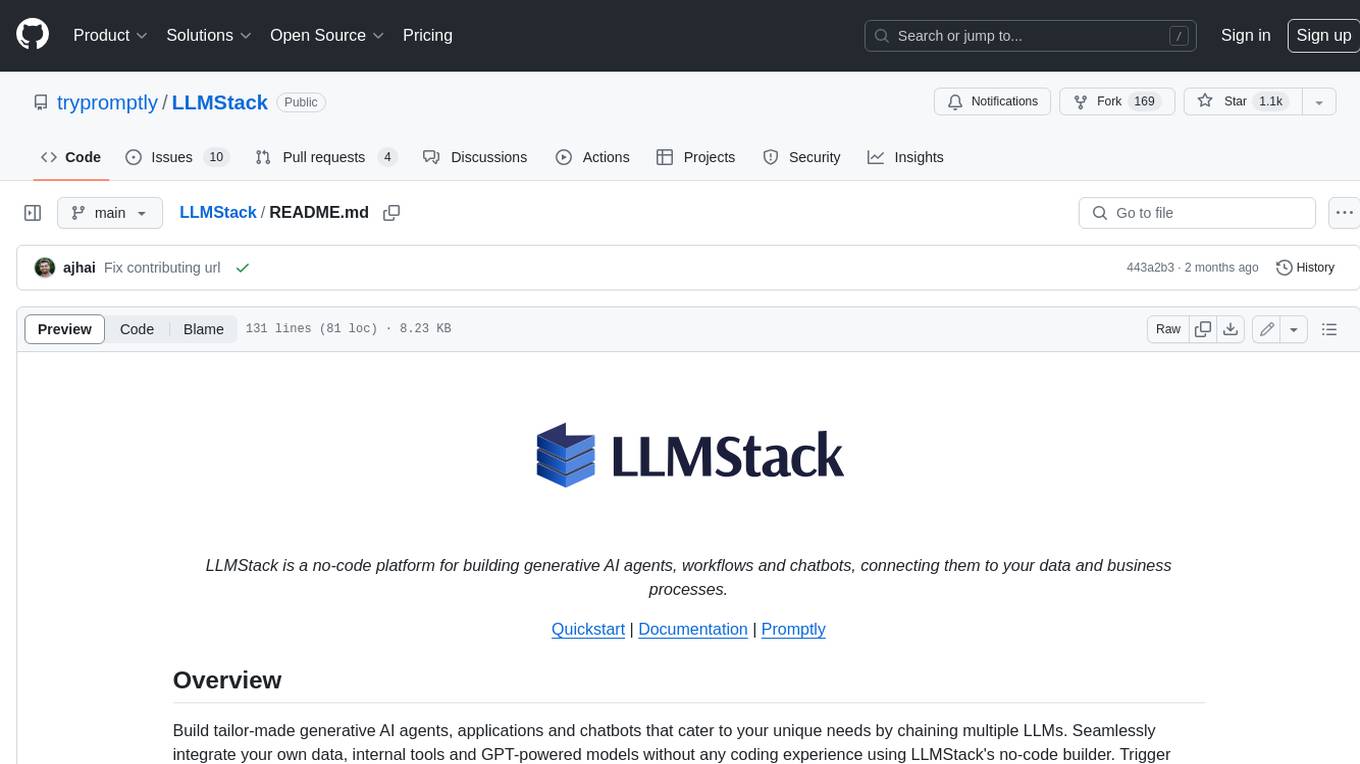
LLMStack
LLMStack is a no-code platform for building generative AI agents, workflows, and chatbots. It allows users to connect their own data, internal tools, and GPT-powered models without any coding experience. LLMStack can be deployed to the cloud or on-premise and can be accessed via HTTP API or triggered from Slack or Discord.
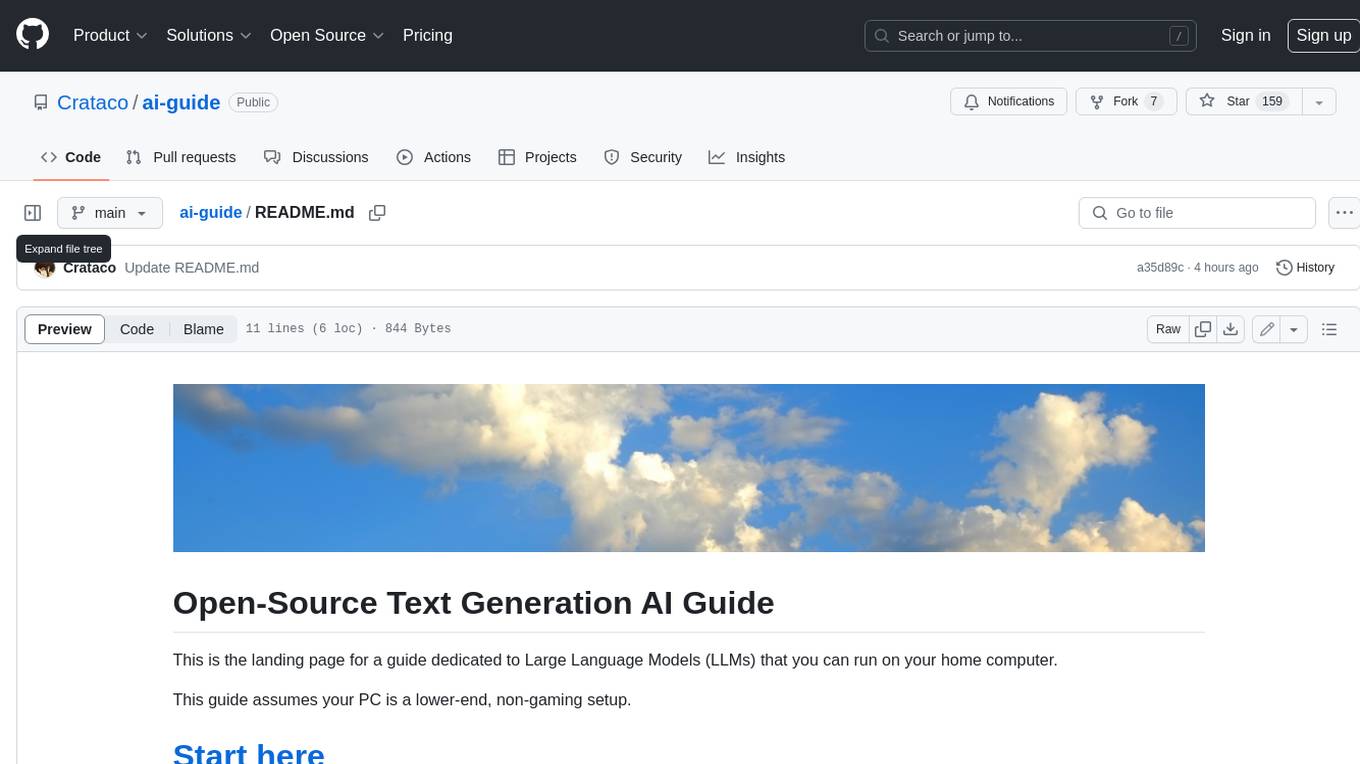
ai-guide
This guide is dedicated to Large Language Models (LLMs) that you can run on your home computer. It assumes your PC is a lower-end, non-gaming setup.
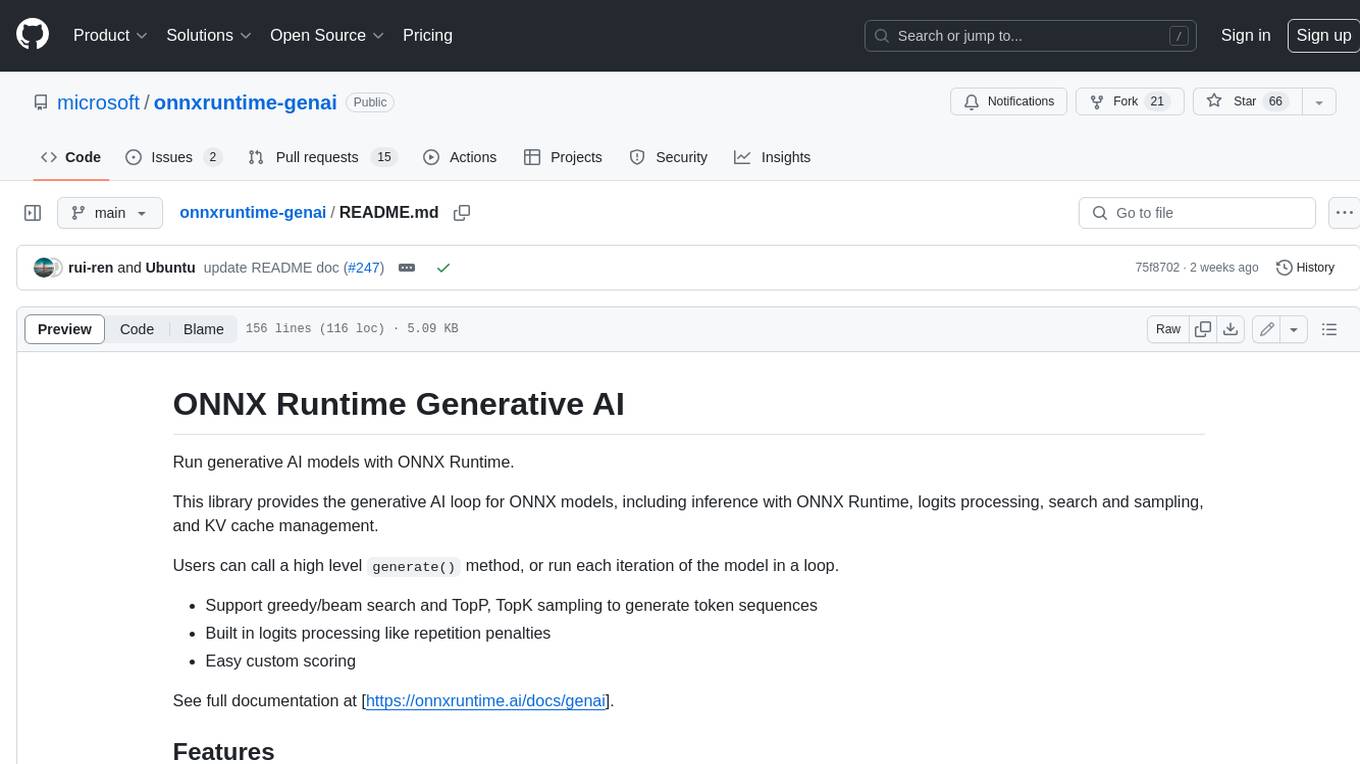
onnxruntime-genai
ONNX Runtime Generative AI is a library that provides the generative AI loop for ONNX models, including inference with ONNX Runtime, logits processing, search and sampling, and KV cache management. Users can call a high level `generate()` method, or run each iteration of the model in a loop. It supports greedy/beam search and TopP, TopK sampling to generate token sequences, has built in logits processing like repetition penalties, and allows for easy custom scoring.
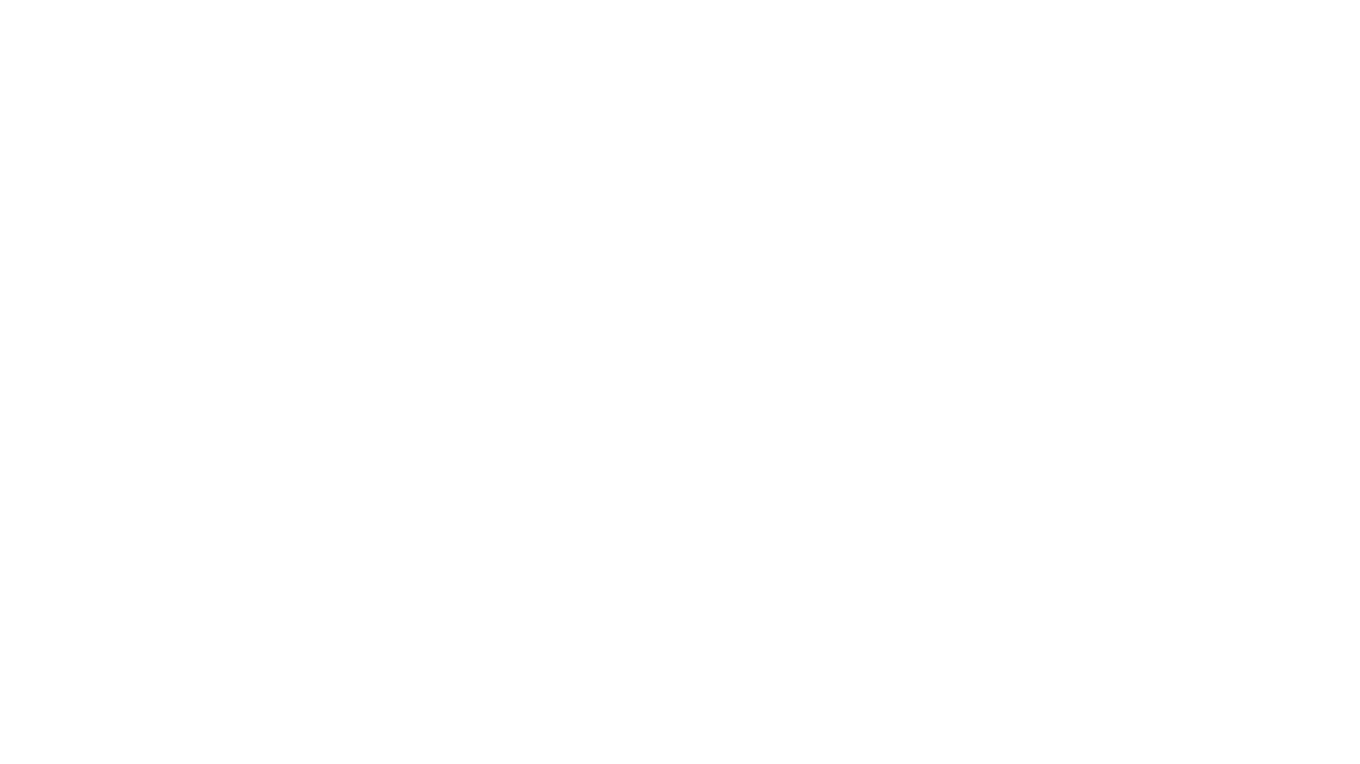
jupyter-ai
Jupyter AI connects generative AI with Jupyter notebooks. It provides a user-friendly and powerful way to explore generative AI models in notebooks and improve your productivity in JupyterLab and the Jupyter Notebook. Specifically, Jupyter AI offers: * An `%%ai` magic that turns the Jupyter notebook into a reproducible generative AI playground. This works anywhere the IPython kernel runs (JupyterLab, Jupyter Notebook, Google Colab, Kaggle, VSCode, etc.). * A native chat UI in JupyterLab that enables you to work with generative AI as a conversational assistant. * Support for a wide range of generative model providers, including AI21, Anthropic, AWS, Cohere, Gemini, Hugging Face, NVIDIA, and OpenAI. * Local model support through GPT4All, enabling use of generative AI models on consumer grade machines with ease and privacy.
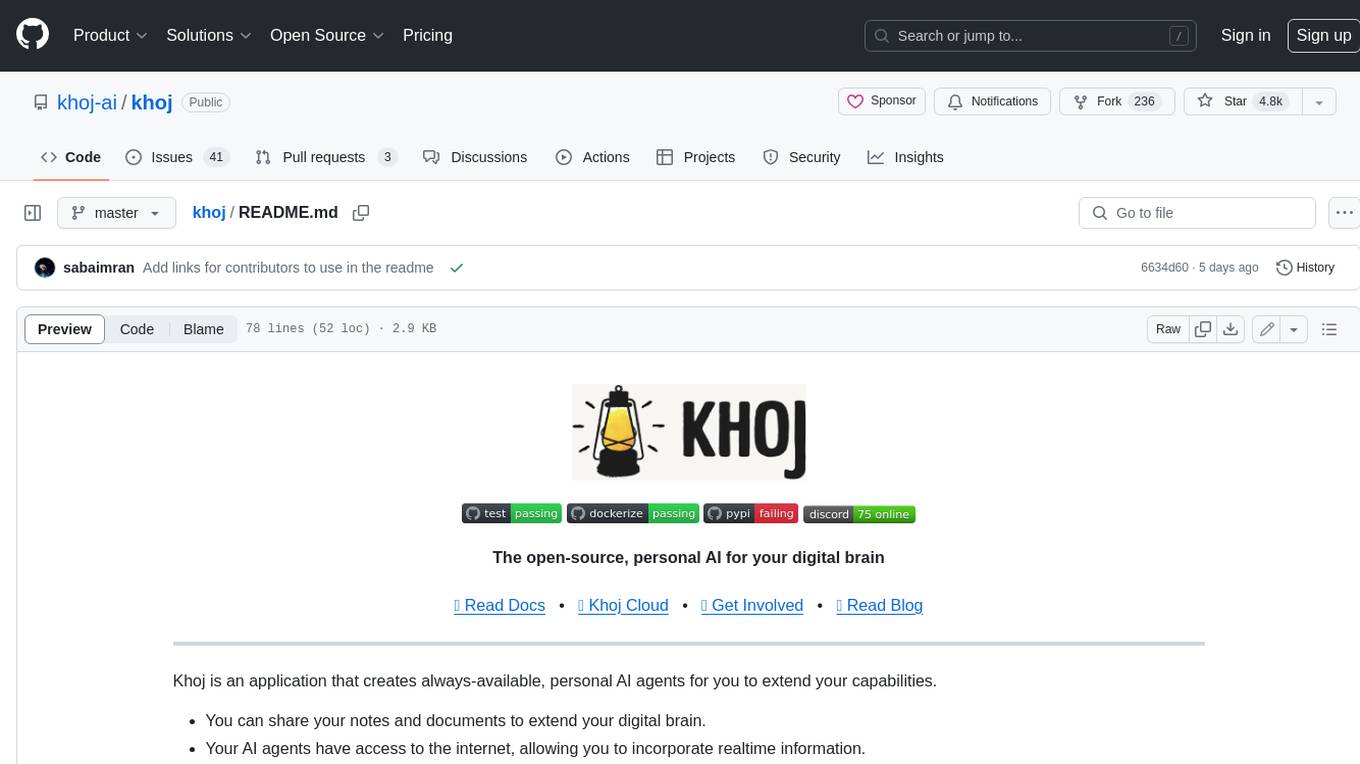
khoj
Khoj is an open-source, personal AI assistant that extends your capabilities by creating always-available AI agents. You can share your notes and documents to extend your digital brain, and your AI agents have access to the internet, allowing you to incorporate real-time information. Khoj is accessible on Desktop, Emacs, Obsidian, Web, and Whatsapp, and you can share PDF, markdown, org-mode, notion files, and GitHub repositories. You'll get fast, accurate semantic search on top of your docs, and your agents can create deeply personal images and understand your speech. Khoj is self-hostable and always will be.
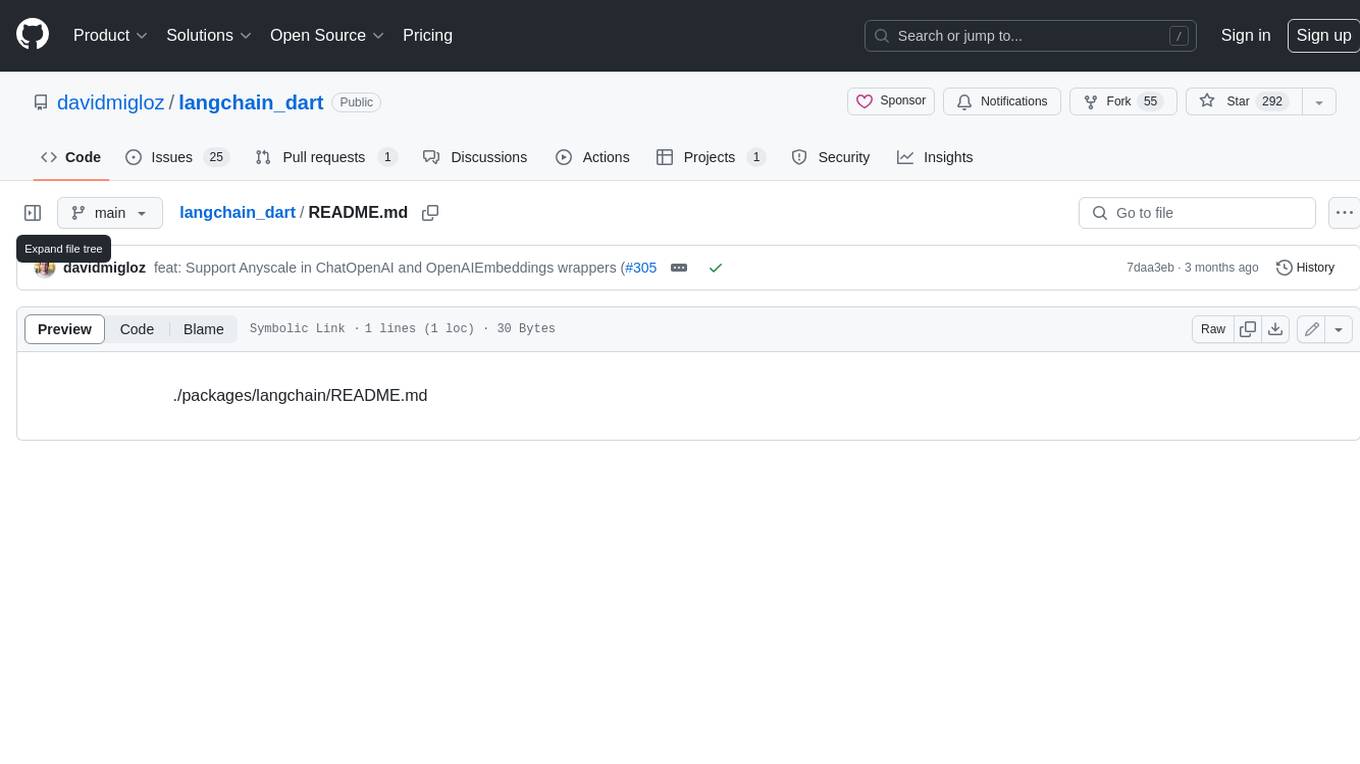
langchain_dart
LangChain.dart is a Dart port of the popular LangChain Python framework created by Harrison Chase. LangChain provides a set of ready-to-use components for working with language models and a standard interface for chaining them together to formulate more advanced use cases (e.g. chatbots, Q&A with RAG, agents, summarization, extraction, etc.). The components can be grouped into a few core modules: * **Model I/O:** LangChain offers a unified API for interacting with various LLM providers (e.g. OpenAI, Google, Mistral, Ollama, etc.), allowing developers to switch between them with ease. Additionally, it provides tools for managing model inputs (prompt templates and example selectors) and parsing the resulting model outputs (output parsers). * **Retrieval:** assists in loading user data (via document loaders), transforming it (with text splitters), extracting its meaning (using embedding models), storing (in vector stores) and retrieving it (through retrievers) so that it can be used to ground the model's responses (i.e. Retrieval-Augmented Generation or RAG). * **Agents:** "bots" that leverage LLMs to make informed decisions about which available tools (such as web search, calculators, database lookup, etc.) to use to accomplish the designated task. The different components can be composed together using the LangChain Expression Language (LCEL).
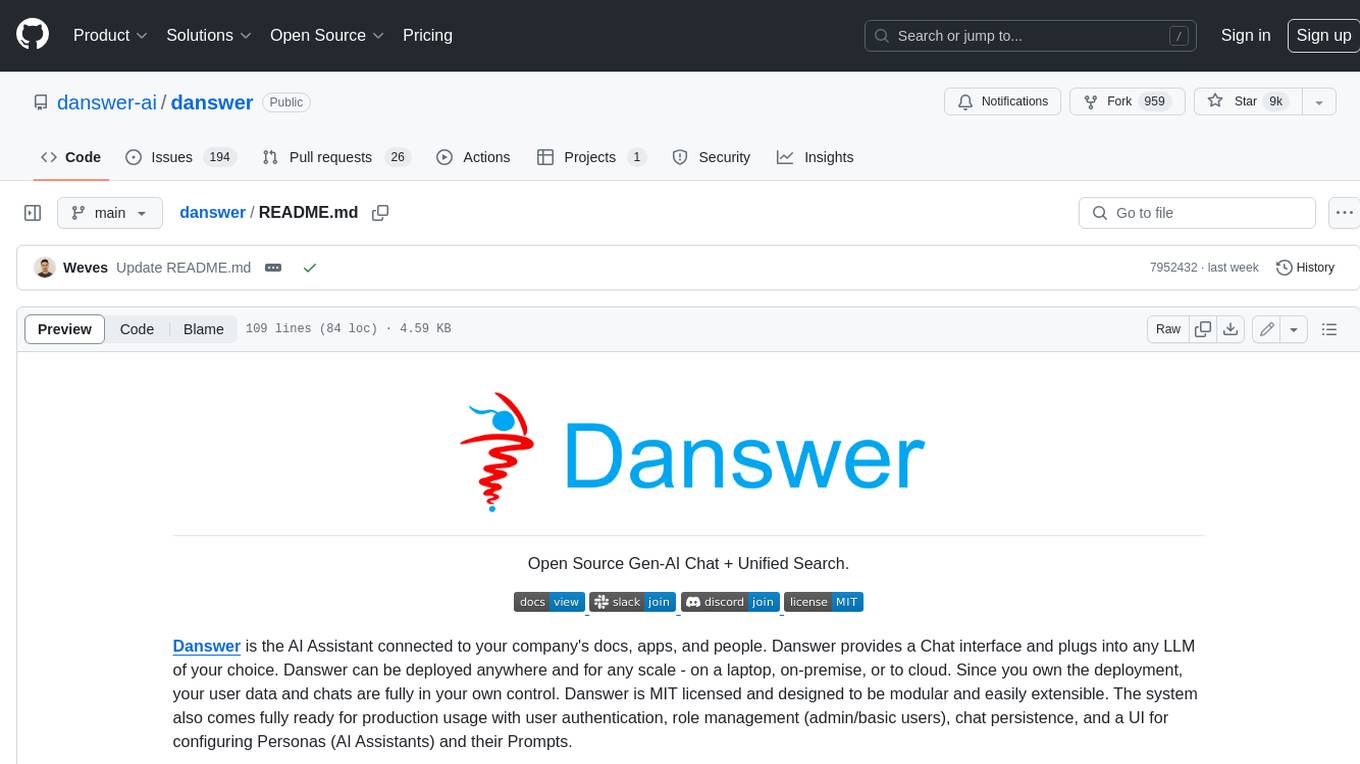
danswer
Danswer is an open-source Gen-AI Chat and Unified Search tool that connects to your company's docs, apps, and people. It provides a Chat interface and plugs into any LLM of your choice. Danswer can be deployed anywhere and for any scale - on a laptop, on-premise, or to cloud. Since you own the deployment, your user data and chats are fully in your own control. Danswer is MIT licensed and designed to be modular and easily extensible. The system also comes fully ready for production usage with user authentication, role management (admin/basic users), chat persistence, and a UI for configuring Personas (AI Assistants) and their Prompts. Danswer also serves as a Unified Search across all common workplace tools such as Slack, Google Drive, Confluence, etc. By combining LLMs and team specific knowledge, Danswer becomes a subject matter expert for the team. Imagine ChatGPT if it had access to your team's unique knowledge! It enables questions such as "A customer wants feature X, is this already supported?" or "Where's the pull request for feature Y?"
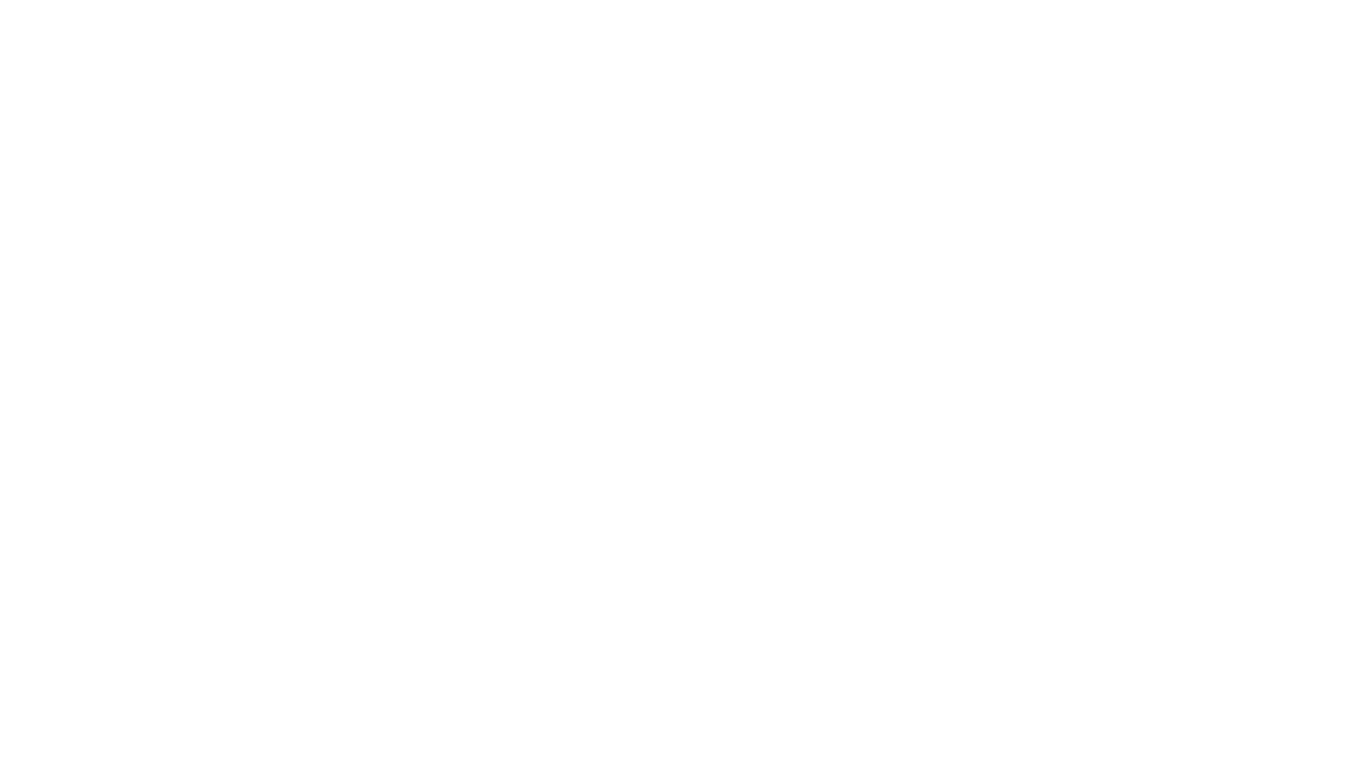
infinity
Infinity is an AI-native database designed for LLM applications, providing incredibly fast full-text and vector search capabilities. It supports a wide range of data types, including vectors, full-text, and structured data, and offers a fused search feature that combines multiple embeddings and full text. Infinity is easy to use, with an intuitive Python API and a single-binary architecture that simplifies deployment. It achieves high performance, with 0.1 milliseconds query latency on million-scale vector datasets and up to 15K QPS.
For similar jobs
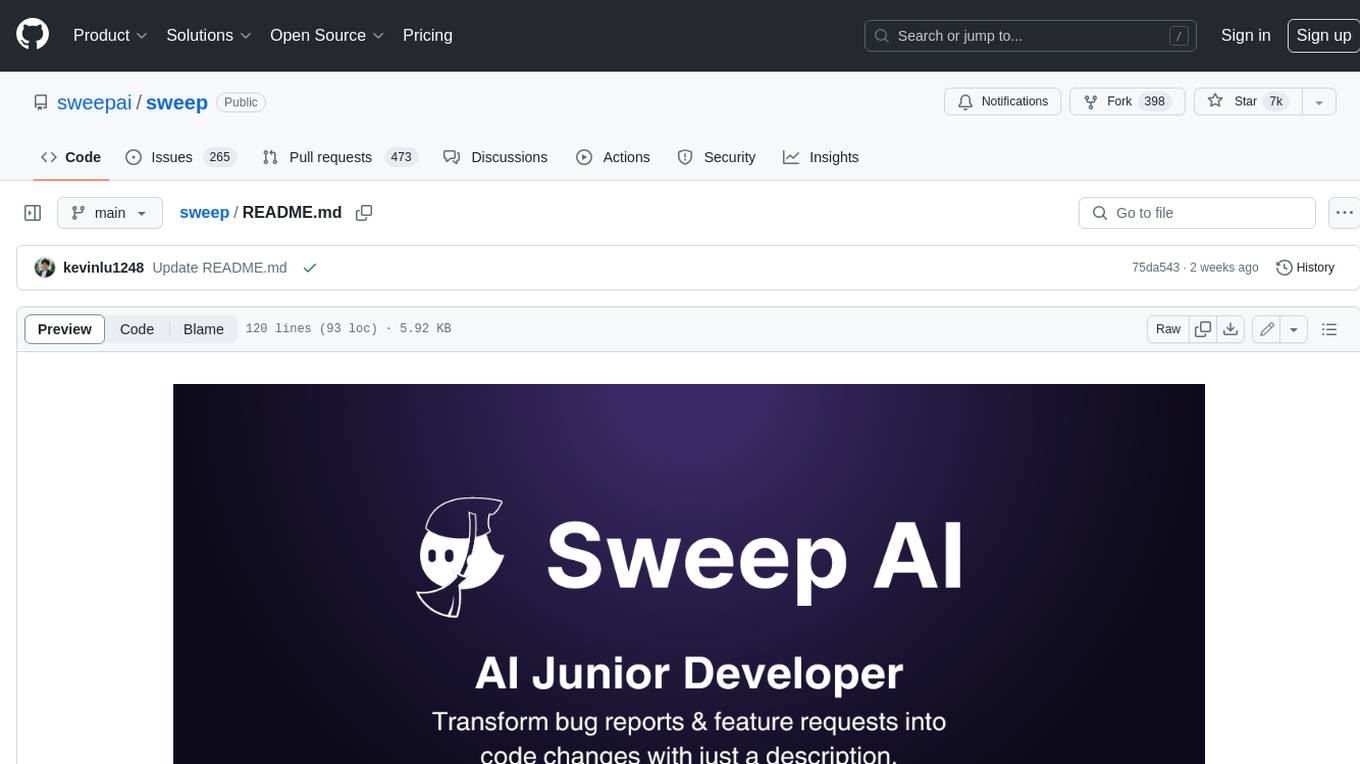
sweep
Sweep is an AI junior developer that turns bugs and feature requests into code changes. It automatically handles developer experience improvements like adding type hints and improving test coverage.
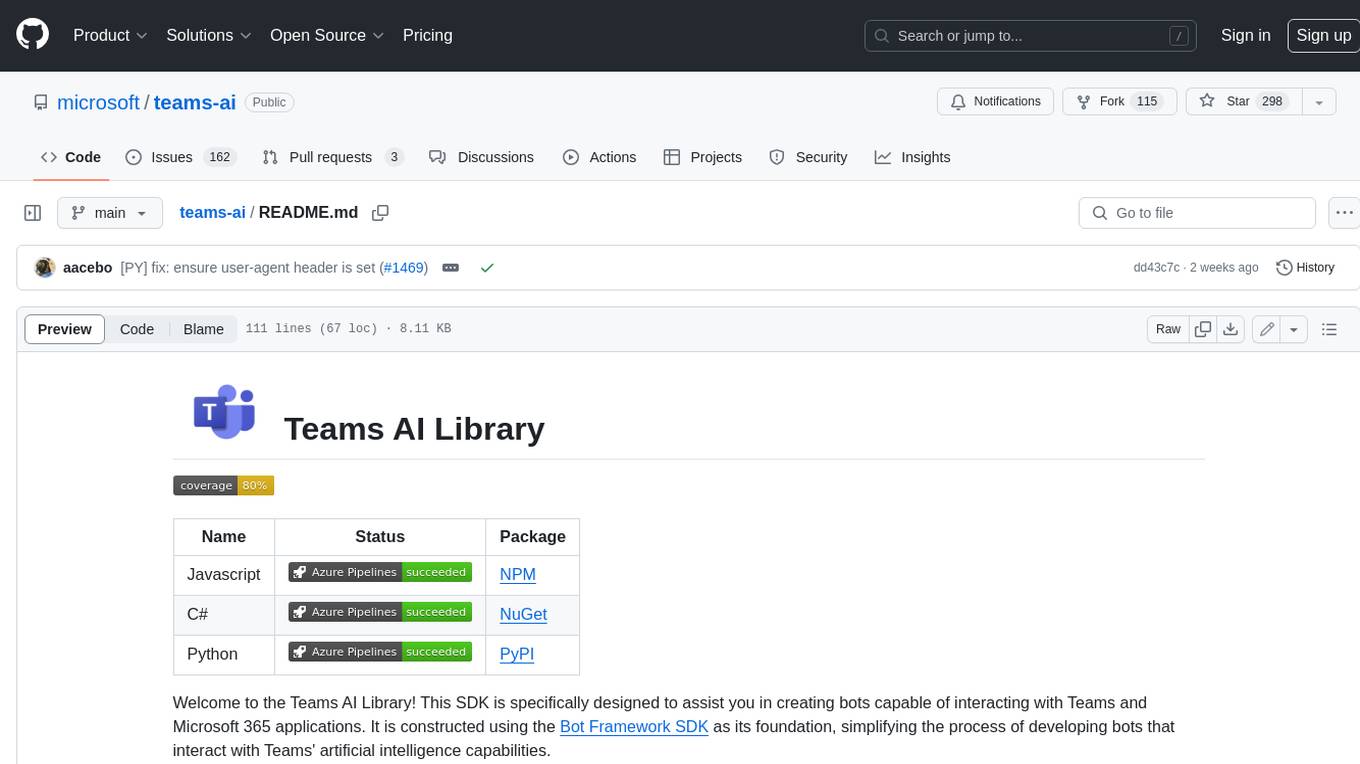
teams-ai
The Teams AI Library is a software development kit (SDK) that helps developers create bots that can interact with Teams and Microsoft 365 applications. It is built on top of the Bot Framework SDK and simplifies the process of developing bots that interact with Teams' artificial intelligence capabilities. The SDK is available for JavaScript/TypeScript, .NET, and Python.
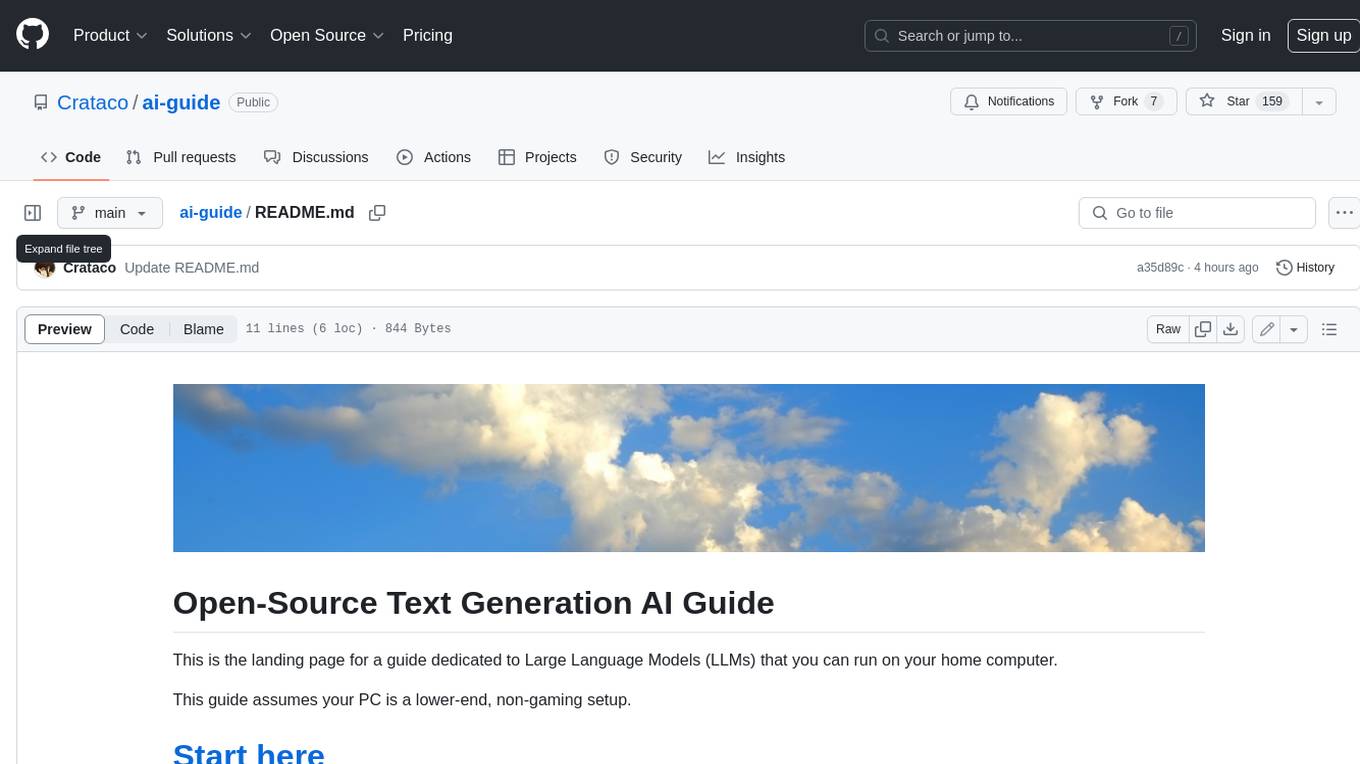
ai-guide
This guide is dedicated to Large Language Models (LLMs) that you can run on your home computer. It assumes your PC is a lower-end, non-gaming setup.
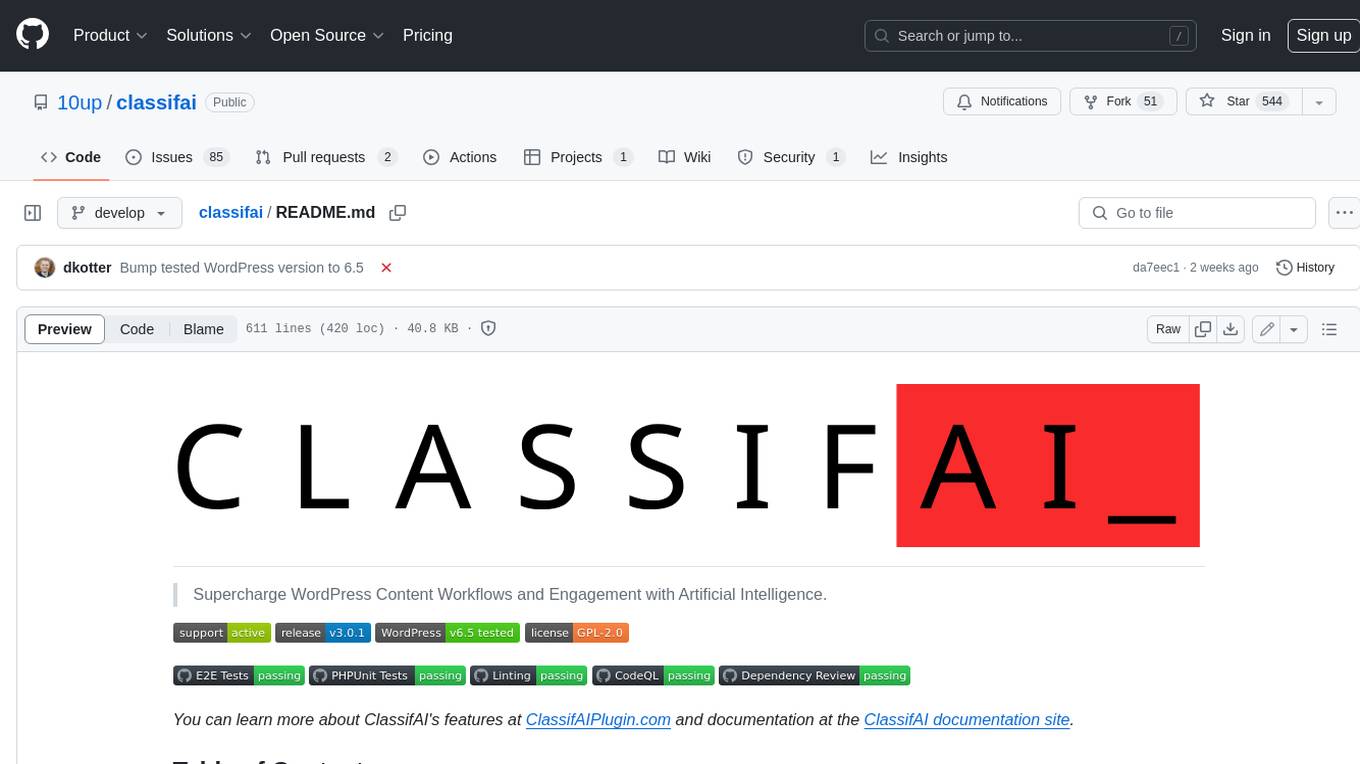
classifai
Supercharge WordPress Content Workflows and Engagement with Artificial Intelligence. Tap into leading cloud-based services like OpenAI, Microsoft Azure AI, Google Gemini and IBM Watson to augment your WordPress-powered websites. Publish content faster while improving SEO performance and increasing audience engagement. ClassifAI integrates Artificial Intelligence and Machine Learning technologies to lighten your workload and eliminate tedious tasks, giving you more time to create original content that matters.
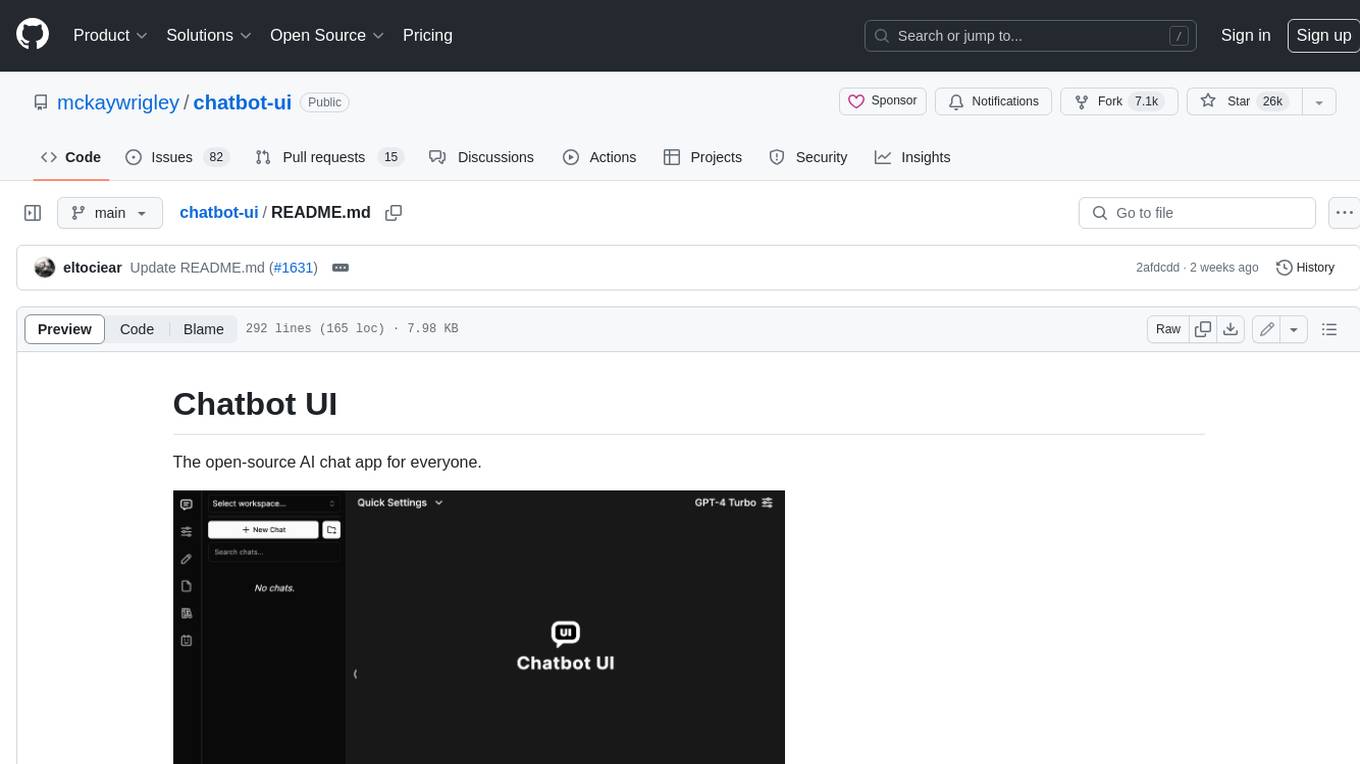
chatbot-ui
Chatbot UI is an open-source AI chat app that allows users to create and deploy their own AI chatbots. It is easy to use and can be customized to fit any need. Chatbot UI is perfect for businesses, developers, and anyone who wants to create a chatbot.
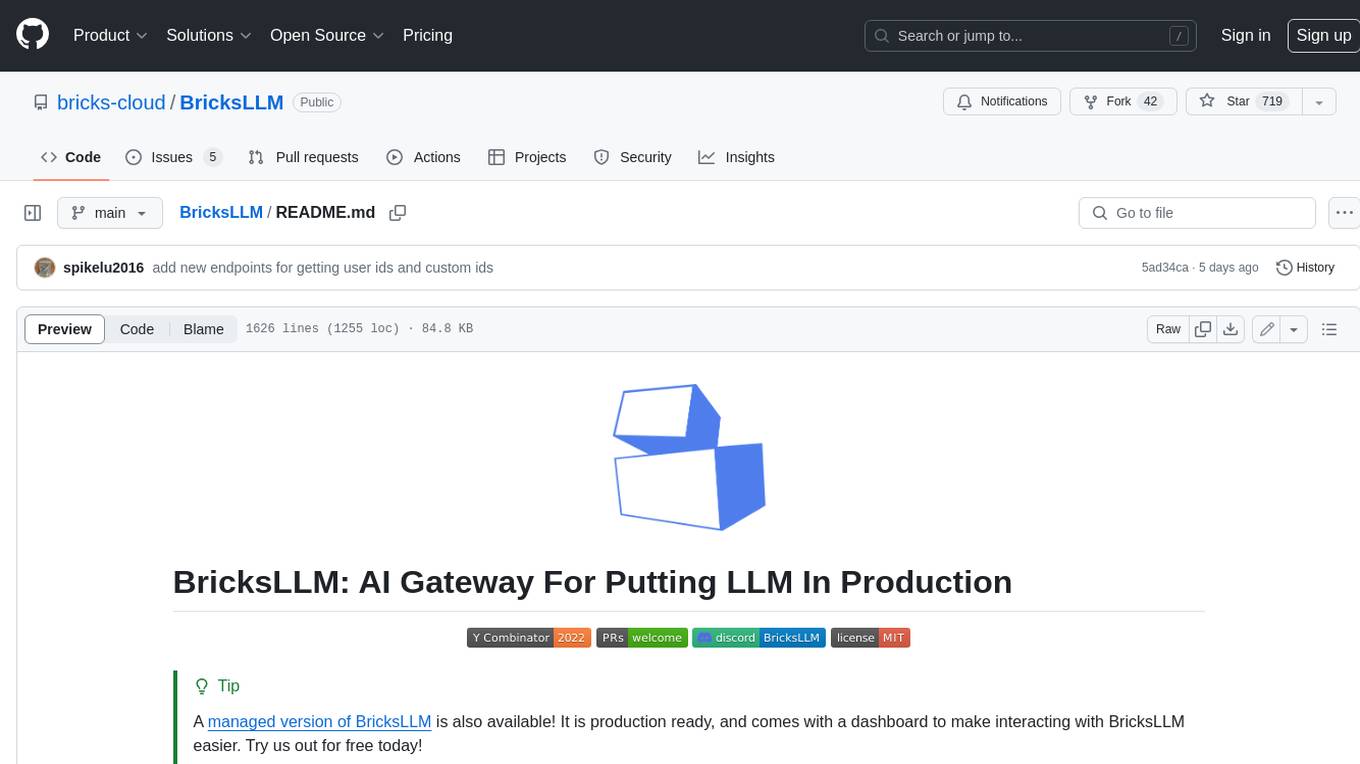
BricksLLM
BricksLLM is a cloud native AI gateway written in Go. Currently, it provides native support for OpenAI, Anthropic, Azure OpenAI and vLLM. BricksLLM aims to provide enterprise level infrastructure that can power any LLM production use cases. Here are some use cases for BricksLLM: * Set LLM usage limits for users on different pricing tiers * Track LLM usage on a per user and per organization basis * Block or redact requests containing PIIs * Improve LLM reliability with failovers, retries and caching * Distribute API keys with rate limits and cost limits for internal development/production use cases * Distribute API keys with rate limits and cost limits for students
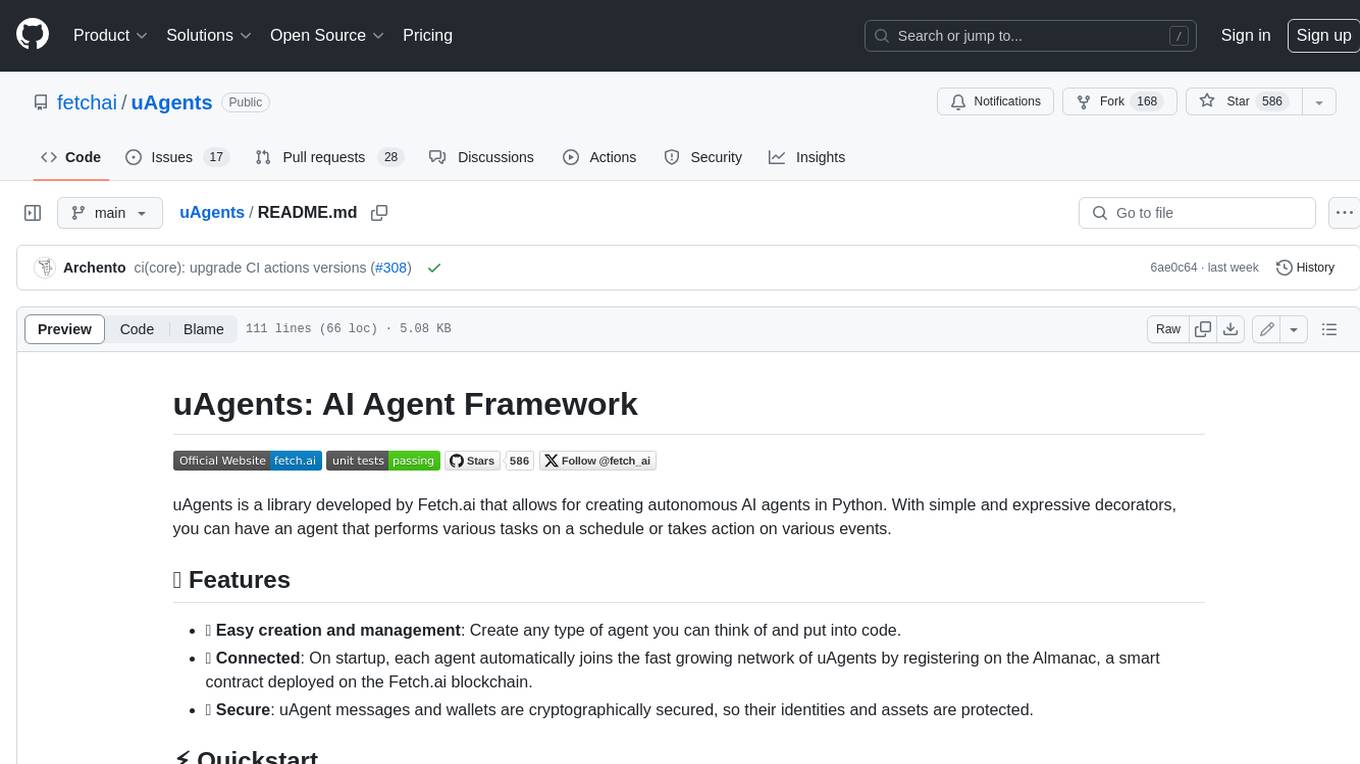
uAgents
uAgents is a Python library developed by Fetch.ai that allows for the creation of autonomous AI agents. These agents can perform various tasks on a schedule or take action on various events. uAgents are easy to create and manage, and they are connected to a fast-growing network of other uAgents. They are also secure, with cryptographically secured messages and wallets.
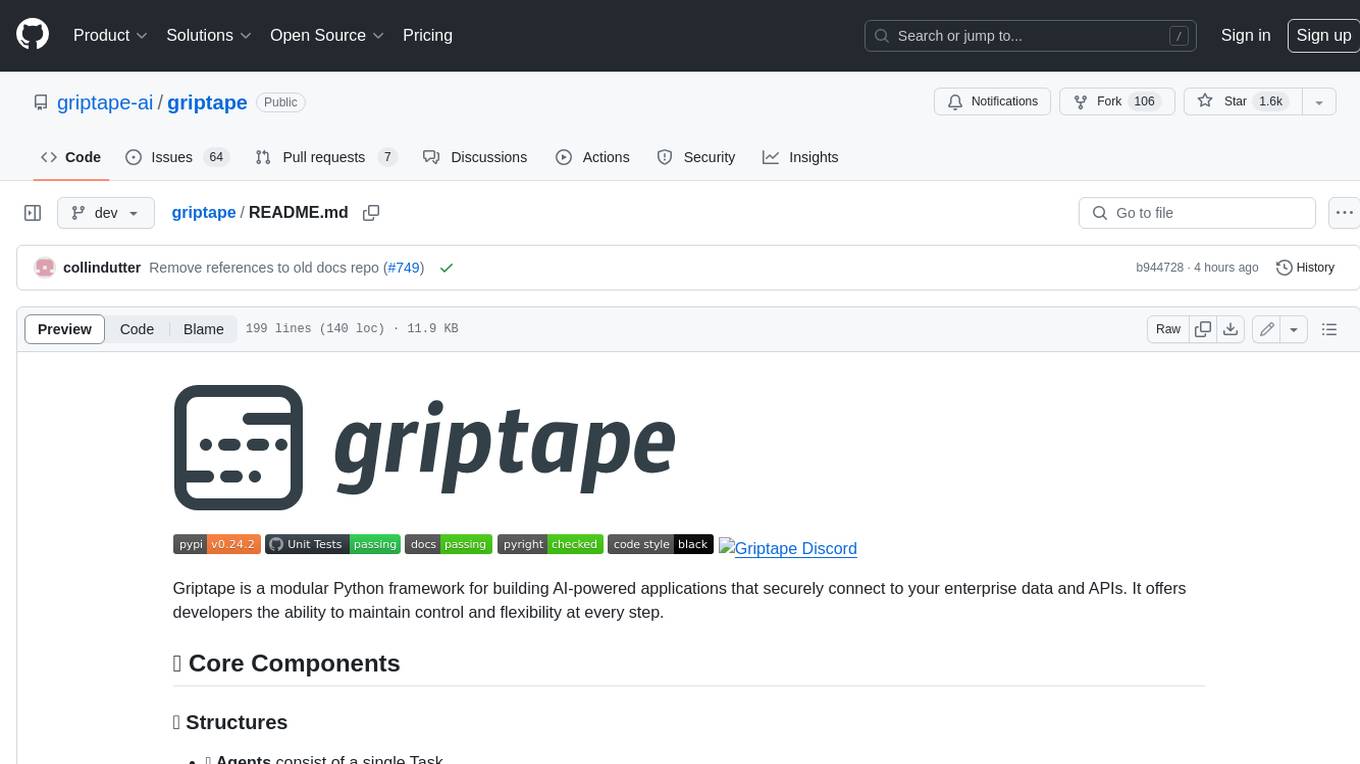
griptape
Griptape is a modular Python framework for building AI-powered applications that securely connect to your enterprise data and APIs. It offers developers the ability to maintain control and flexibility at every step. Griptape's core components include Structures (Agents, Pipelines, and Workflows), Tasks, Tools, Memory (Conversation Memory, Task Memory, and Meta Memory), Drivers (Prompt and Embedding Drivers, Vector Store Drivers, Image Generation Drivers, Image Query Drivers, SQL Drivers, Web Scraper Drivers, and Conversation Memory Drivers), Engines (Query Engines, Extraction Engines, Summary Engines, Image Generation Engines, and Image Query Engines), and additional components (Rulesets, Loaders, Artifacts, Chunkers, and Tokenizers). Griptape enables developers to create AI-powered applications with ease and efficiency.