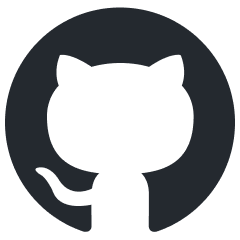
model2vec
The Fastest State-of-the-Art Static Embeddings in the World
Stars: 804
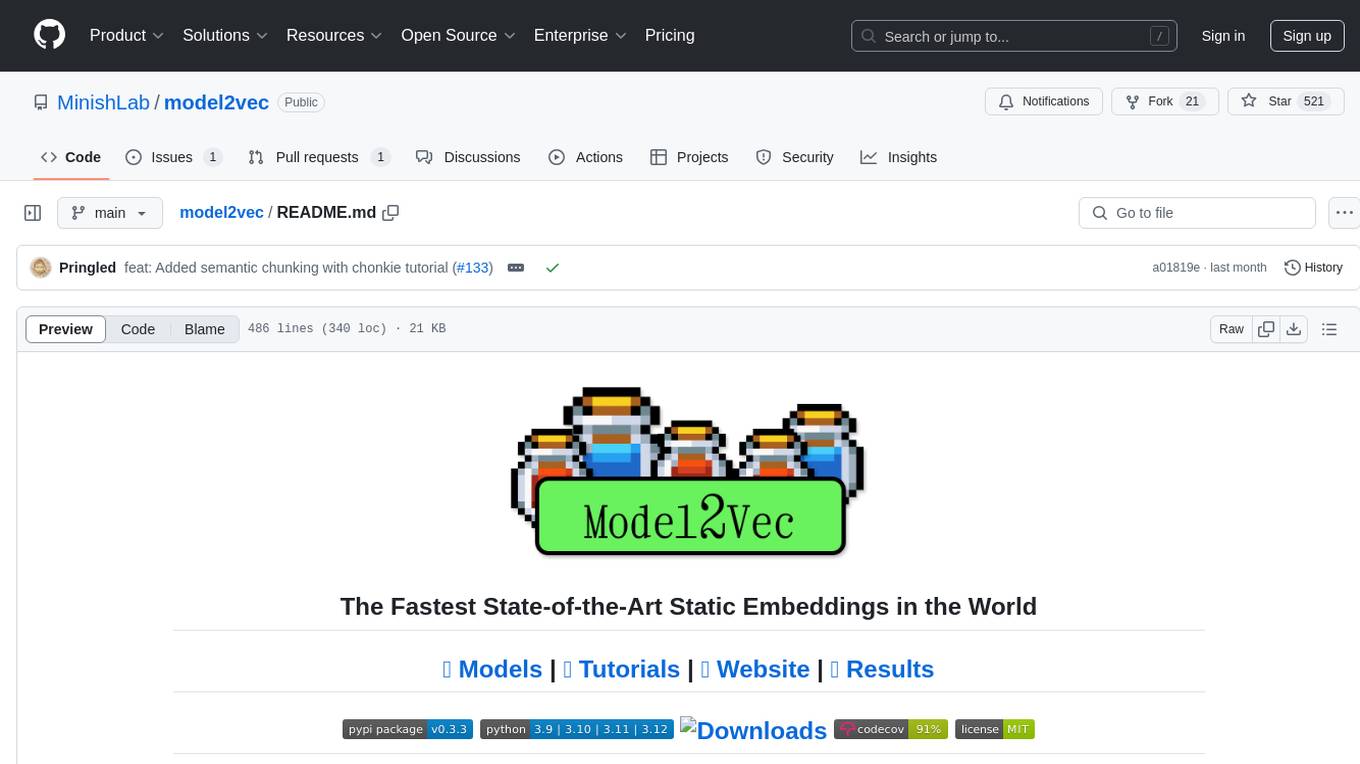
Model2Vec is a technique to turn any sentence transformer into a really small static model, reducing model size by 15x and making the models up to 500x faster, with a small drop in performance. It outperforms other static embedding models like GLoVe and BPEmb, is lightweight with only `numpy` as a major dependency, offers fast inference, dataset-free distillation, and is integrated into Sentence Transformers, txtai, and Chonkie. Model2Vec creates powerful models by passing a vocabulary through a sentence transformer model, reducing dimensionality using PCA, and weighting embeddings using zipf weighting. Users can distill their own models or use pre-trained models from the HuggingFace hub. Evaluation can be done using the provided evaluation package. Model2Vec is licensed under MIT.
README:
Model2Vec is a technique to turn any sentence transformer into a really small static model, reducing model size by 15x and making the models up to 500x faster, with a small drop in performance. Our best model is the most performant static embedding model in the world. See our results here, or dive in to see how it works.
- 30/10/2024: We released three new models: potion-base-8M, potion-base-4M, and potion-base-2M. These models are trained using Tokenlearn. Find out more in our blog post. NOTE: for users of any of our old English M2V models, we recommend switching to these new models as they perform better on all tasks.
Install the package with:
pip install model2vec
This will install the base inference package, which only depends on numpy
and a few other minor dependencies. If you want to distill your own models, you can install the distillation extras with:
pip install model2vec[distill]
The easiest way to get started with Model2Vec is to load one of our flagship models from the HuggingFace hub. These models are pre-trained and ready to use. The following code snippet shows how to load a model and make embeddings:
from model2vec import StaticModel
# Load a model from the HuggingFace hub (in this case the potion-base-8M model)
model = StaticModel.from_pretrained("minishlab/potion-base-8M")
# Make embeddings
embeddings = model.encode(["It's dangerous to go alone!", "It's a secret to everybody."])
# Make sequences of token embeddings
token_embeddings = model.encode_as_sequence(["It's dangerous to go alone!", "It's a secret to everybody."])
And that's it. You can use the model to classify texts, to cluster, or to build a RAG system.
Instead of using one of our models, you can also distill your own Model2Vec model from a Sentence Transformer model. The following code snippet shows how to distill a model:
from model2vec.distill import distill
# Distill a Sentence Transformer model, in this case the BAAI/bge-base-en-v1.5 model
m2v_model = distill(model_name="BAAI/bge-base-en-v1.5", pca_dims=256)
# Save the model
m2v_model.save_pretrained("m2v_model")
Distillation is really fast and only takes 30 seconds on CPU. Best of all, distillation requires no training data.
For advanced usage, such as using Model2Vec in the Sentence Transformers library, please refer to the Usage sections.
- State-of-the-Art Performance: Model2Vec models outperform any other static embeddings (such as GLoVe and BPEmb) by a large margin, as can be seen in our results.
- Small: Model2Vec reduces the size of a Sentence Transformer model by a factor of 15, from 120M params, down to 7.5M (30 MB on disk, making it the smallest model on MTEB!).
-
Lightweight Dependencies: the base package's only major dependency is
numpy
. - Lightning-fast Inference: up to 500 times faster on CPU than the original model. Go green or go home.
- Fast, Dataset-free Distillation: distill your own model in 30 seconds on a CPU, without a dataset. All you need is a model and (optionally) a custom vocabulary.
- Integrated in many popular libraries: Model2Vec can be used directly in popular libraries such as Sentence Transformers, LangChain, txtai, and Chonkie. See the Integrations section for more information.
-
Tightly integrated with HuggingFace hub: easily share and load models from the HuggingFace hub, using the familiar
from_pretrained
andpush_to_hub
. Our own models can be found here. Feel free to share your own.
Model2vec creates a small, fast, and powerful model that outperforms other static embedding models by a large margin on all tasks we could find, while being much faster to create than traditional static embedding models such as GloVe. Like BPEmb, it can create subword embeddings, but with much better performance. Distillation doesn't need any data, just a vocabulary and a model.
The base model2vec technique works by passing a vocabulary through a sentence transformer model, then reducing the dimensionality of the resulting embeddings using PCA, and finally weighting the embeddings using zipf weighting. During inference, we simply take the mean of all token embeddings occurring in a sentence.
Our potion models are pre-trained using tokenlearn, a technique to pre-train model2vec distillation models. These models are created with the following steps:
- Distillation: We distill a Model2Vec model from a Sentence Transformer model, using the method described above.
- Sentence Transformer inference: We use the Sentence Transformer model to create mean embeddings for a large number of texts from a corpus.
- Training: We train a model to minimize the cosine distance between the mean embeddings generated by the Sentence Transformer model and the mean embeddings generated by the Model2Vec model.
-
Post-training re-regularization: We re-regularize the trained embeddings by first performing PCA, and then weighting the embeddings using
smooth inverse frequency (SIF)
weighting using the following formula:w = 1e-3 / (1e-3 + proba)
. Here,proba
is the probability of the token in the corpus we used for training.
For a much more extensive deepdive, please refer to our Model2Vec blog post and our Tokenlearn blog post.
Inference with a pretrained model
Inference works as follows. The example shows one of our own models, but you can also just load a local one, or another one from the hub.
from model2vec import StaticModel
# Load a model from the Hub. You can optionally pass a token when loading a private model
model = StaticModel.from_pretrained(model_name="minishlab/potion-base-8M", token=None)
# Make embeddings
embeddings = model.encode(["It's dangerous to go alone!", "It's a secret to everybody."])
# Make sequences of token embeddings
token_embeddings = model.encode_as_sequence(["It's dangerous to go alone!", "It's a secret to everybody."])
Inference with the Sentence Transformers library
The following code snippet shows how to use a Model2Vec model in the Sentence Transformers library. This is useful if you want to use the model in a Sentence Transformers pipeline.
from sentence_transformers import SentenceTransformer
from sentence_transformers.models import StaticEmbedding
# Initialize a StaticEmbedding module
static_embedding = StaticEmbedding.from_model2vec("minishlab/potion-base-8M")
model = SentenceTransformer(modules=[static_embedding])
embeddings = model.encode(["It's dangerous to go alone!", "It's a secret to everybody."])
Distilling from a Sentence Transformer
The following code can be used to distill a model from a Sentence Transformer. As mentioned above, this leads to really small model that might be less performant.
from model2vec.distill import distill
# Distill a Sentence Transformer model
m2v_model = distill(model_name="BAAI/bge-base-en-v1.5", pca_dims=256)
# Save the model
m2v_model.save_pretrained("m2v_model")
Distilling from a loaded model
If you already have a model loaded, or need to load a model in some special way, we also offer an interface to distill models in memory.
from transformers import AutoModel, AutoTokenizer
from model2vec.distill import distill_from_model
# Assuming a loaded model and tokenizer
model_name = "baai/bge-base-en-v1.5"
model = AutoModel.from_pretrained(model_name)
tokenizer = AutoTokenizer.from_pretrained(model_name)
m2v_model = distill_from_model(model=model, tokenizer=tokenizer, pca_dims=256)
m2v_model.save_pretrained("m2v_model")
Distilling with the Sentence Transformers library
The following code snippet shows how to distill a model using the Sentence Transformers library. This is useful if you want to use the model in a Sentence Transformers pipeline.
from sentence_transformers import SentenceTransformer
from sentence_transformers.models import StaticEmbedding
static_embedding = StaticEmbedding.from_distillation("BAAI/bge-base-en-v1.5", device="cpu", pca_dims=256)
model = SentenceTransformer(modules=[static_embedding])
embeddings = model.encode(["It's dangerous to go alone!", "It's a secret to everybody."])
Distilling with a custom vocabulary
If you pass a vocabulary, you get a set of static word embeddings, together with a custom tokenizer for exactly that vocabulary. This is comparable to how you would use GLoVe or traditional word2vec, but doesn't actually require a corpus or data.
from model2vec.distill import distill
# Load a vocabulary as a list of strings
vocabulary = ["word1", "word2", "word3"]
# Distill a Sentence Transformer model with the custom vocabulary
m2v_model = distill(model_name="BAAI/bge-base-en-v1.5", vocabulary=vocabulary)
# Save the model
m2v_model.save_pretrained("m2v_model")
# Or push it to the hub
m2v_model.push_to_hub("my_organization/my_model", token="<it's a secret to everybody>")
By default, this will distill a model with a subword tokenizer, combining the models (subword) vocab with the new vocabulary. If you want to get a word-level tokenizer instead (with only the passed vocabulary), the use_subword
parameter can be set to False
, e.g.:
m2v_model = distill(model_name=model_name, vocabulary=vocabulary, use_subword=False)
Important note: we assume the passed vocabulary is sorted in rank frequency. i.e., we don't care about the actual word frequencies, but do assume that the most frequent word is first, and the least frequent word is last. If you're not sure whether this is case, set apply_zipf
to False
. This disables the weighting, but will also make performance a little bit worse.
Installation
Our models can be evaluated using our evaluation package. Install the evaluation package with:
pip install git+https://github.com/MinishLab/evaluation.git@main
Evaluation Code
The following code snippet shows how to evaluate a Model2Vec model:
from model2vec import StaticModel
from evaluation import CustomMTEB, get_tasks, parse_mteb_results, make_leaderboard, summarize_results
from mteb import ModelMeta
# Get all available tasks
tasks = get_tasks()
# Define the CustomMTEB object with the specified tasks
evaluation = CustomMTEB(tasks=tasks)
# Load the model
model_name = "m2v_model"
model = StaticModel.from_pretrained(model_name)
# Optionally, add model metadata in MTEB format
model.mteb_model_meta = ModelMeta(
name=model_name, revision="no_revision_available", release_date=None, languages=None
)
# Run the evaluation
results = evaluation.run(model, eval_splits=["test"], output_folder=f"results")
# Parse the results and summarize them
parsed_results = parse_mteb_results(mteb_results=results, model_name=model_name)
task_scores = summarize_results(parsed_results)
# Print the results in a leaderboard format
print(make_leaderboard(task_scores))
Sentence Transformers
Model2Vec can be used directly in Sentence Transformers using the StaticEmbedding
module.
The following code snippet shows how to load a Model2Vec model into a Sentence Transformer model:
from sentence_transformers import SentenceTransformer
from sentence_transformers.models import StaticEmbedding
# Initialize a StaticEmbedding module
static_embedding = StaticEmbedding.from_model2vec("minishlab/potion-base-8M")
model = SentenceTransformer(modules=[static_embedding])
embeddings = model.encode(["It's dangerous to go alone!", "It's a secret to everybody."])
The following code snippet shows how to distill a model directly into a Sentence Transformer model:
from sentence_transformers import SentenceTransformer
from sentence_transformers.models import StaticEmbedding
static_embedding = StaticEmbedding.from_distillation("BAAI/bge-base-en-v1.5", device="cpu", pca_dims=256)
model = SentenceTransformer(modules=[static_embedding])
embeddings = model.encode(["It's dangerous to go alone!", "It's a secret to everybody."])
For more documentation, please refer to the Sentence Transformers documentation.
LangChain
Model2Vec can be used in LangChain using the langchain-community
package. For more information, see the LangChain Model2Vec docs. The following code snippet shows how to use Model2Vec in LangChain after installing the langchain-community
package with pip install langchain-community
:
from langchain_community.embeddings import Model2vecEmbeddings
from langchain_community.vectorstores import FAISS
from langchain.schema import Document
# Initialize a Model2Vec embedder
embedder = Model2vecEmbeddings("minishlab/potion-base-8M")
# Create some example texts
texts = [
"Enduring Stew",
"Hearty Elixir",
"Mighty Mushroom Risotto",
"Spicy Meat Skewer",
"Fruit Salad",
]
# Embed the texts
embeddings = embedder.embed_documents(texts)
# Or, create a vector store and query it
documents = [Document(page_content=text) for text in texts]
vector_store = FAISS.from_documents(documents, embedder)
query = "Risotto"
query_vector = embedder.embed_query(query)
retrieved_docs = vector_store.similarity_search_by_vector(query_vector, k=1)
Txtai
Model2Vec can be used in txtai for text embeddings, nearest-neighbors search, and any of the other functionalities that txtai offers. The following code snippet shows how to use Model2Vec in txtai after installing the txtai
package (including the vectors
dependency) with pip install txtai[vectors]
:
from txtai import Embeddings
# Load a model2vec model
embeddings = Embeddings(path="minishlab/potion-base-8M", method="model2vec", backend="numpy")
# Create some example texts
texts = ["Enduring Stew", "Hearty Elixir", "Mighty Mushroom Risotto", "Spicy Meat Skewer", "Chilly Fruit Salad"]
# Create embeddings for downstream tasks
vectors = embeddings.batchtransform(texts)
# Or create a nearest-neighbors index and search it
embeddings.index(texts)
result = embeddings.search("Risotto", 1)
Chonkie
Model2Vec is the default model for semantic chunking in Chonkie. To use Model2Vec for semantic chunking in Chonkie, simply install Chonkie with pip install chonkie[semantic]
and use one of the potion
models in the SemanticChunker
class. The following code snippet shows how to use Model2Vec in Chonkie:
from chonkie import SDPMChunker
# Create some example text to chunk
text = "It's dangerous to go alone! Take this."
# Initialize the SemanticChunker with a potion model
chunker = SDPMChunker(
embedding_model="minishlab/potion-base-8M",
similarity_threshold=0.3
)
# Chunk the text
chunks = chunker.chunk(text)
Transformers.js
To use a Model2Vec model in transformers.js, the following code snippet can be used as a starting point:
import { AutoModel, AutoTokenizer, Tensor } from '@huggingface/transformers';
const modelName = 'minishlab/potion-base-8M';
const modelConfig = {
config: { model_type: 'model2vec' },
dtype: 'fp32',
revision: 'refs/pr/1'
};
const tokenizerConfig = {
revision: 'refs/pr/2'
};
const model = await AutoModel.from_pretrained(modelName, modelConfig);
const tokenizer = await AutoTokenizer.from_pretrained(modelName, tokenizerConfig);
const texts = ['hello', 'hello world'];
const { input_ids } = await tokenizer(texts, { add_special_tokens: false, return_tensor: false });
const cumsum = arr => arr.reduce((acc, num, i) => [...acc, num + (acc[i - 1] || 0)], []);
const offsets = [0, ...cumsum(input_ids.slice(0, -1).map(x => x.length))];
const flattened_input_ids = input_ids.flat();
const modelInputs = {
input_ids: new Tensor('int64', flattened_input_ids, [flattened_input_ids.length]),
offsets: new Tensor('int64', offsets, [offsets.length])
};
const { embeddings } = await model(modelInputs);
console.log(embeddings.tolist()); // output matches python version
Note that this requires that the Model2Vec has a model.onnx
file and several required tokenizers file. To generate these for a model that does not have them yet, the following code snippet can be used:
python scripts/export_to_onnx.py --model_path <path-to-a-model2vec-model> --save_path "<path-to-save-the-onnx-model>"
We provide a number of models that can be used out of the box. These models are available on the HuggingFace hub and can be loaded using the from_pretrained
method. The models are listed below.
Model | Language | Vocab | Sentence Transformer | Tokenizer Type | Params | Tokenlearn |
---|---|---|---|---|---|---|
potion-base-8M | English | Output | bge-base-en-v1.5 | Subword | 7.5M | ✅ |
potion-base-4M | English | Output | bge-base-en-v1.5 | Subword | 3.7M | ✅ |
potion-base-2M | English | Output | bge-base-en-v1.5 | Subword | 1.8M | ✅ |
M2V_multilingual_output | Multilingual | Output | LaBSE | Subword | 471M | ❌ |
We have performed extensive experiments to evaluate the performance of Model2Vec models. The results are documented in the results folder. The results are presented in the following sections:
MIT
If you use Model2Vec in your research, please cite the following:
@software{minishlab2024model2vec,
authors = {Stephan Tulkens, Thomas van Dongen},
title = {Model2Vec: The Fastest State-of-the-Art Static Embeddings in the World},
year = {2024},
url = {https://github.com/MinishLab/model2vec},
}
For Tasks:
Click tags to check more tools for each tasksFor Jobs:
Alternative AI tools for model2vec
Similar Open Source Tools
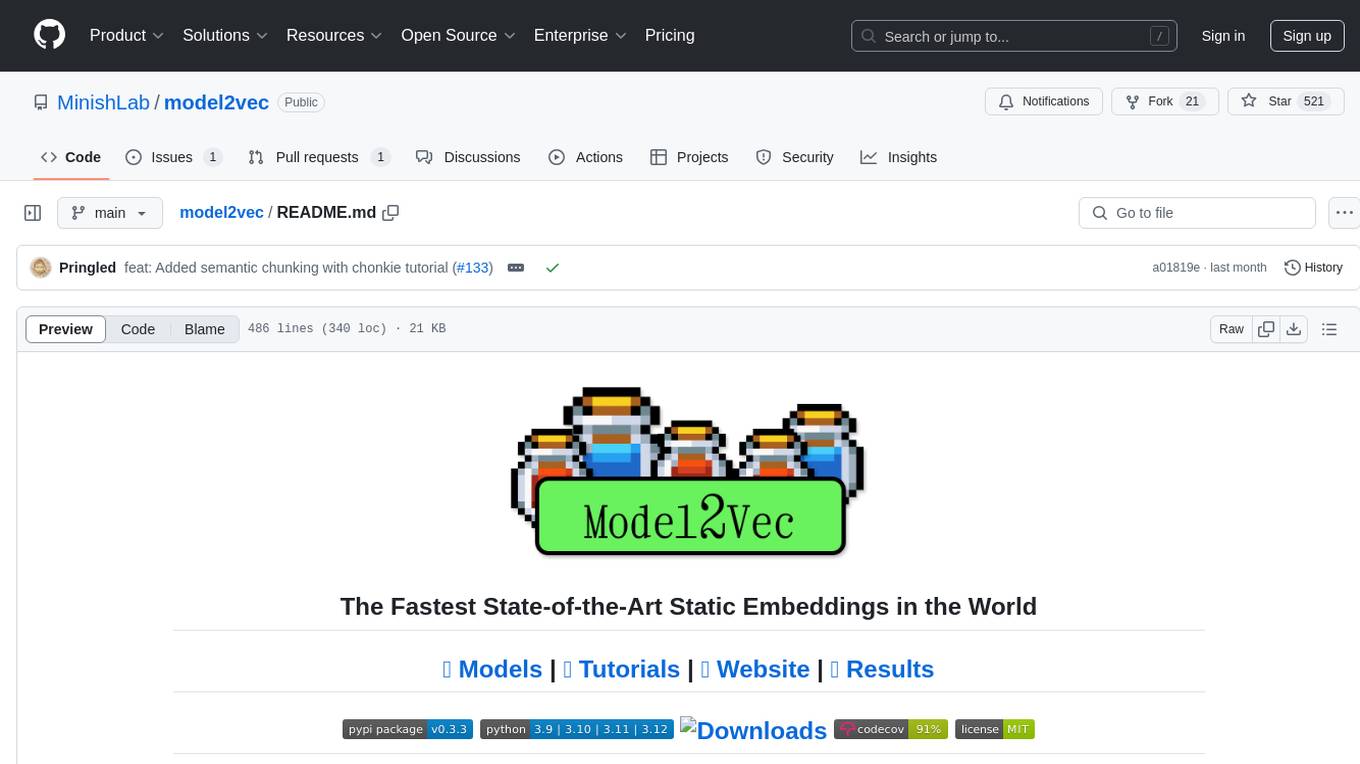
model2vec
Model2Vec is a technique to turn any sentence transformer into a really small static model, reducing model size by 15x and making the models up to 500x faster, with a small drop in performance. It outperforms other static embedding models like GLoVe and BPEmb, is lightweight with only `numpy` as a major dependency, offers fast inference, dataset-free distillation, and is integrated into Sentence Transformers, txtai, and Chonkie. Model2Vec creates powerful models by passing a vocabulary through a sentence transformer model, reducing dimensionality using PCA, and weighting embeddings using zipf weighting. Users can distill their own models or use pre-trained models from the HuggingFace hub. Evaluation can be done using the provided evaluation package. Model2Vec is licensed under MIT.
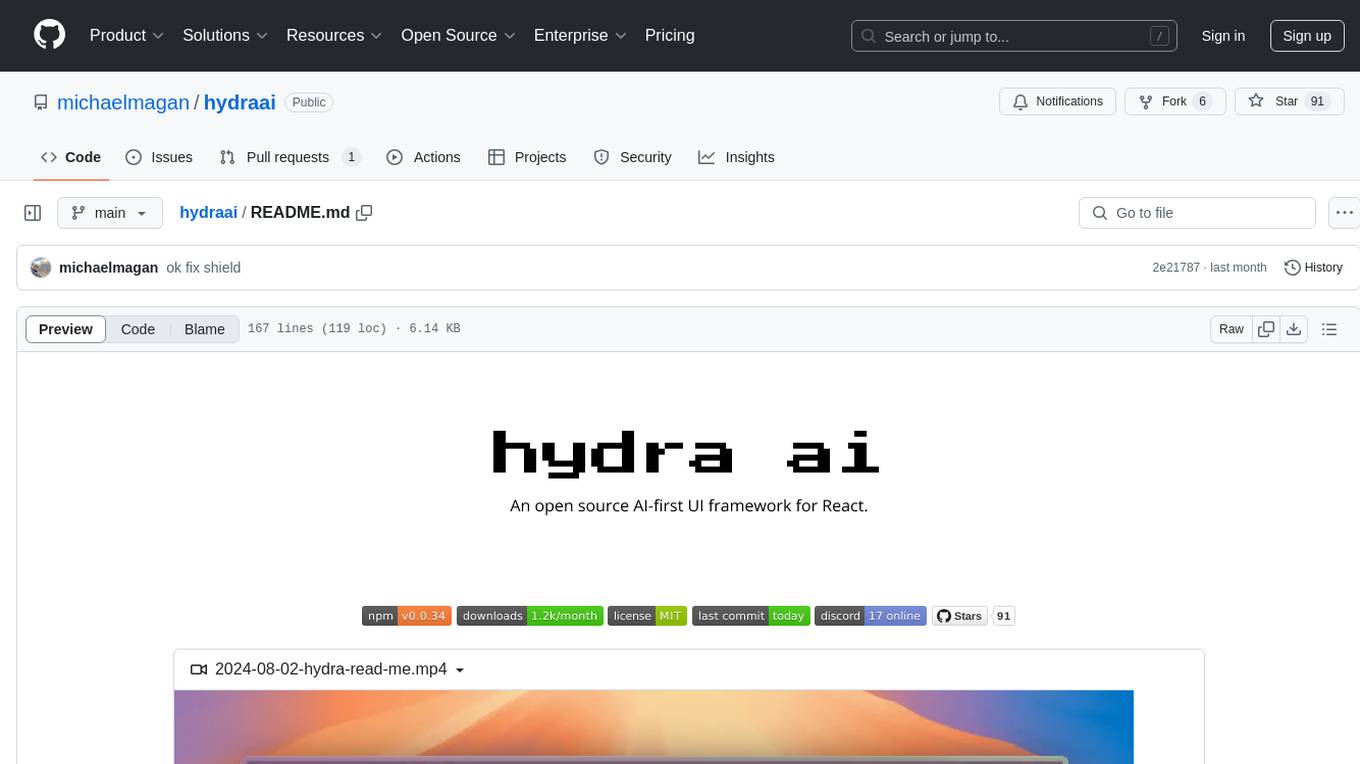
hydraai
Generate React components on-the-fly at runtime using AI. Register your components, and let Hydra choose when to show them in your App. Hydra development is still early, and patterns for different types of components and apps are still being developed. Join the discord to chat with the developers. Expects to be used in a NextJS project. Components that have function props do not work.
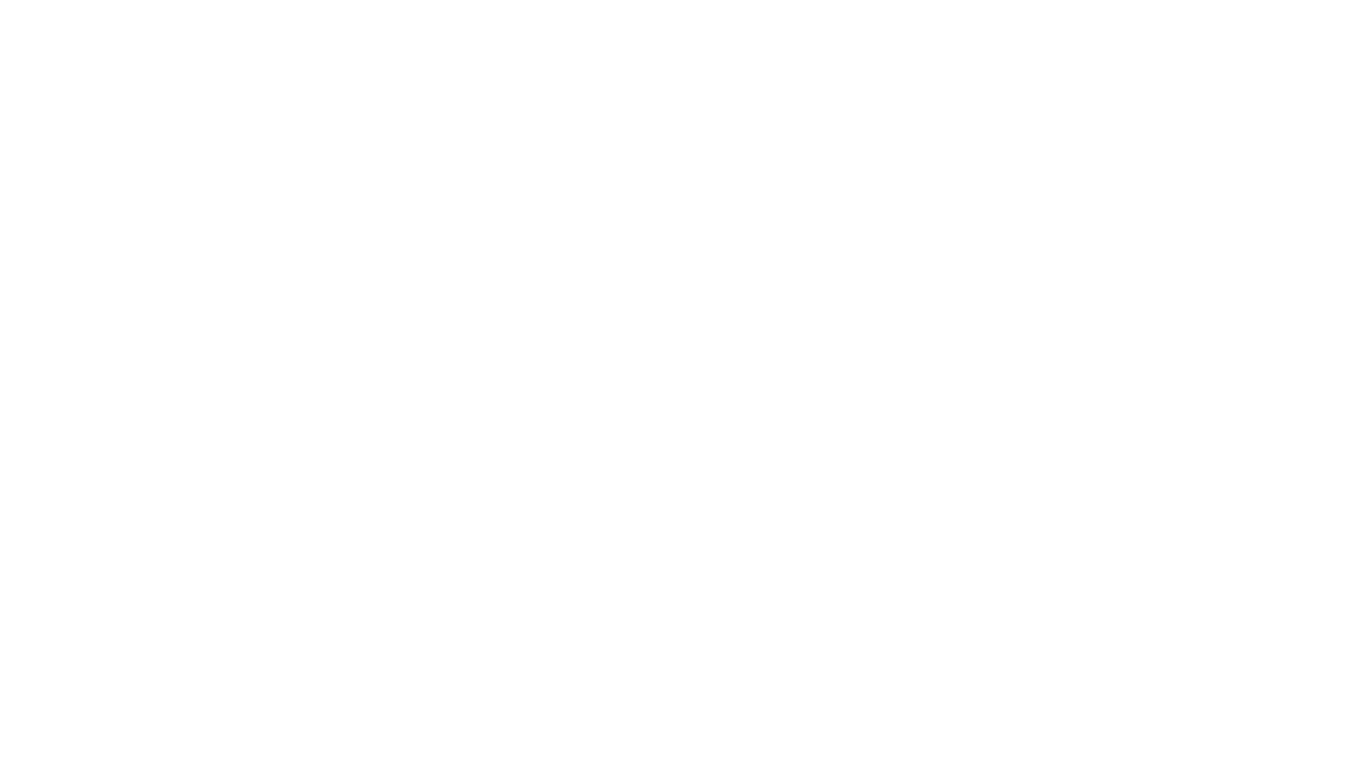
raglite
RAGLite is a Python toolkit for Retrieval-Augmented Generation (RAG) with PostgreSQL or SQLite. It offers configurable options for choosing LLM providers, database types, and rerankers. The toolkit is fast and permissive, utilizing lightweight dependencies and hardware acceleration. RAGLite provides features like PDF to Markdown conversion, multi-vector chunk embedding, optimal semantic chunking, hybrid search capabilities, adaptive retrieval, and improved output quality. It is extensible with a built-in Model Context Protocol server, customizable ChatGPT-like frontend, document conversion to Markdown, and evaluation tools. Users can configure RAGLite for various tasks like configuring, inserting documents, running RAG pipelines, computing query adapters, evaluating performance, running MCP servers, and serving frontends.
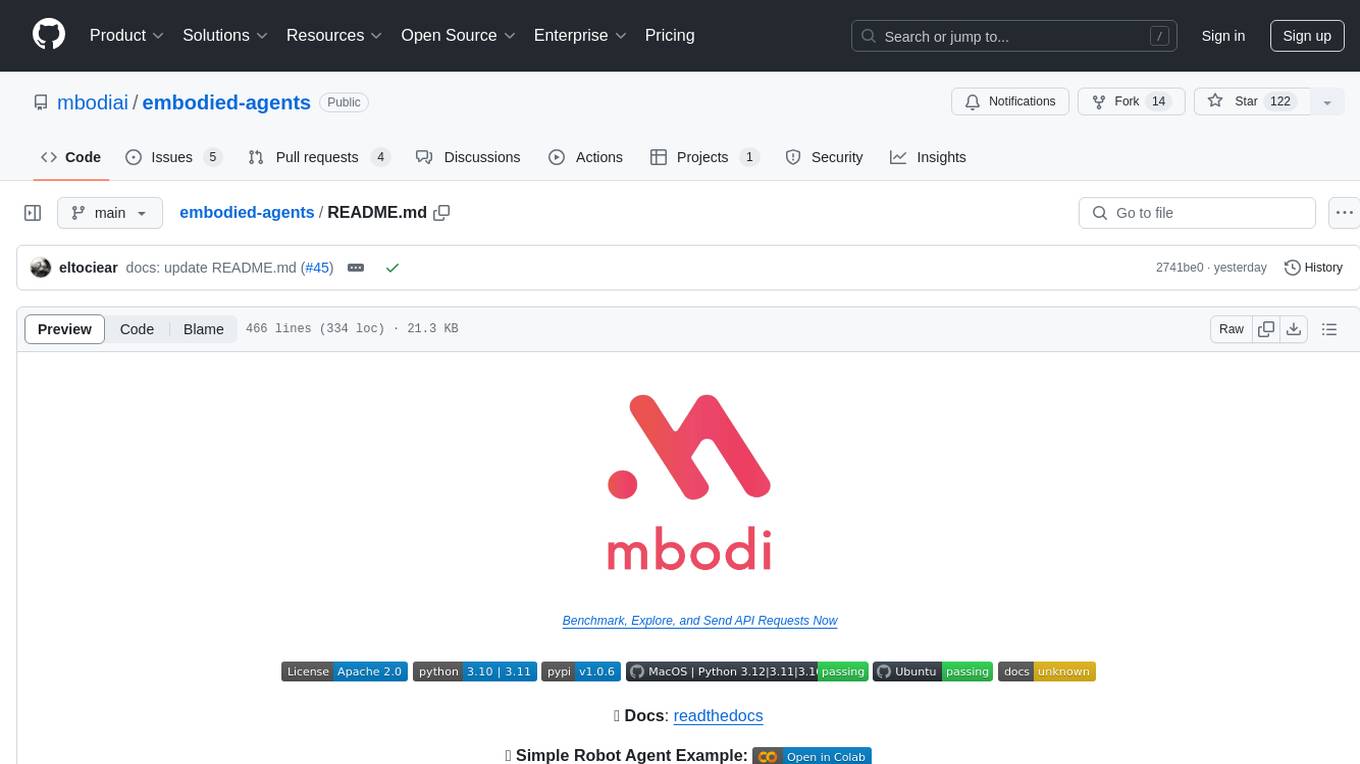
embodied-agents
Embodied Agents is a toolkit for integrating large multi-modal models into existing robot stacks with just a few lines of code. It provides consistency, reliability, scalability, and is configurable to any observation and action space. The toolkit is designed to reduce complexities involved in setting up inference endpoints, converting between different model formats, and collecting/storing datasets. It aims to facilitate data collection and sharing among roboticists by providing Python-first abstractions that are modular, extensible, and applicable to a wide range of tasks. The toolkit supports asynchronous and remote thread-safe agent execution for maximal responsiveness and scalability, and is compatible with various APIs like HuggingFace Spaces, Datasets, Gymnasium Spaces, Ollama, and OpenAI. It also offers automatic dataset recording and optional uploads to the HuggingFace hub.
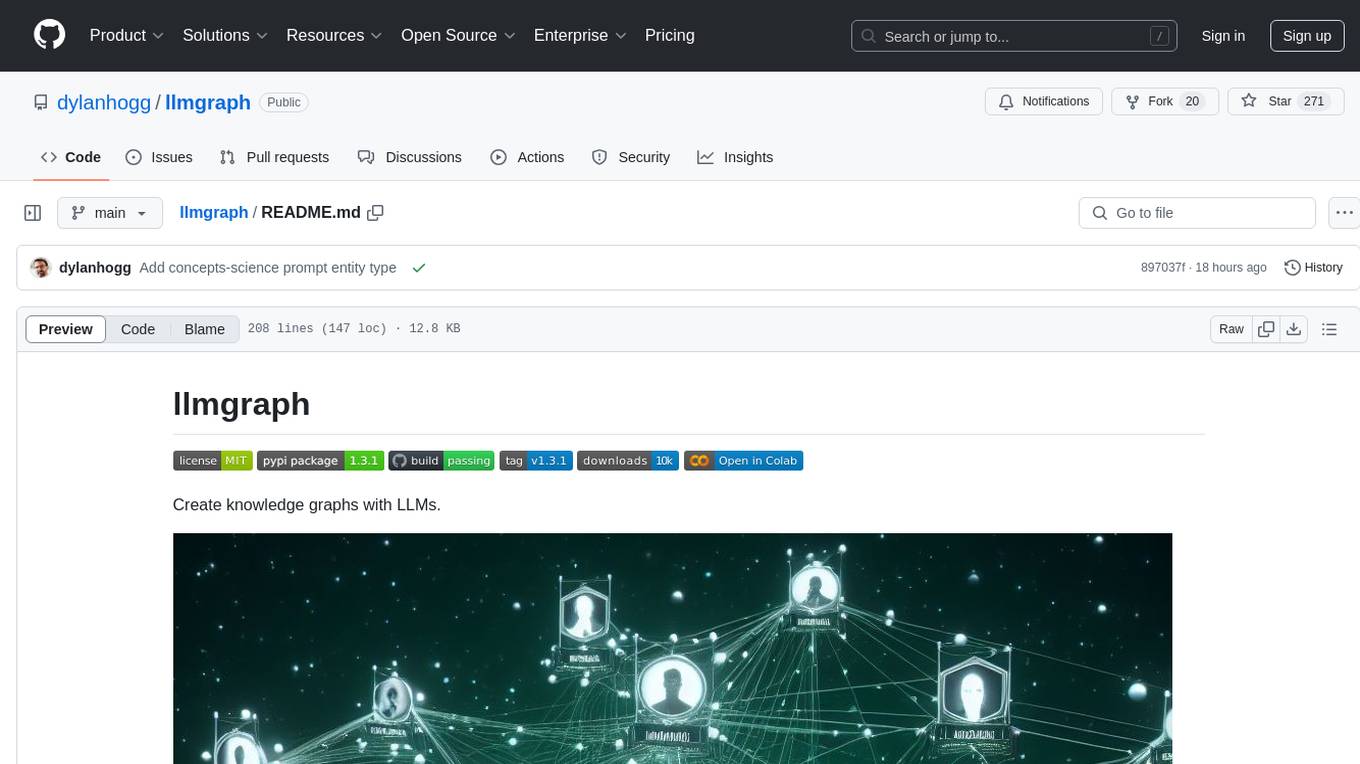
llmgraph
llmgraph is a tool that enables users to create knowledge graphs in GraphML, GEXF, and HTML formats by extracting world knowledge from large language models (LLMs) like ChatGPT. It supports various entity types and relationships, offers cache support for efficient graph growth, and provides insights into LLM costs. Users can customize the model used and interact with different LLM providers. The tool allows users to generate interactive graphs based on a specified entity type and Wikipedia link, making it a valuable resource for knowledge graph creation and exploration.
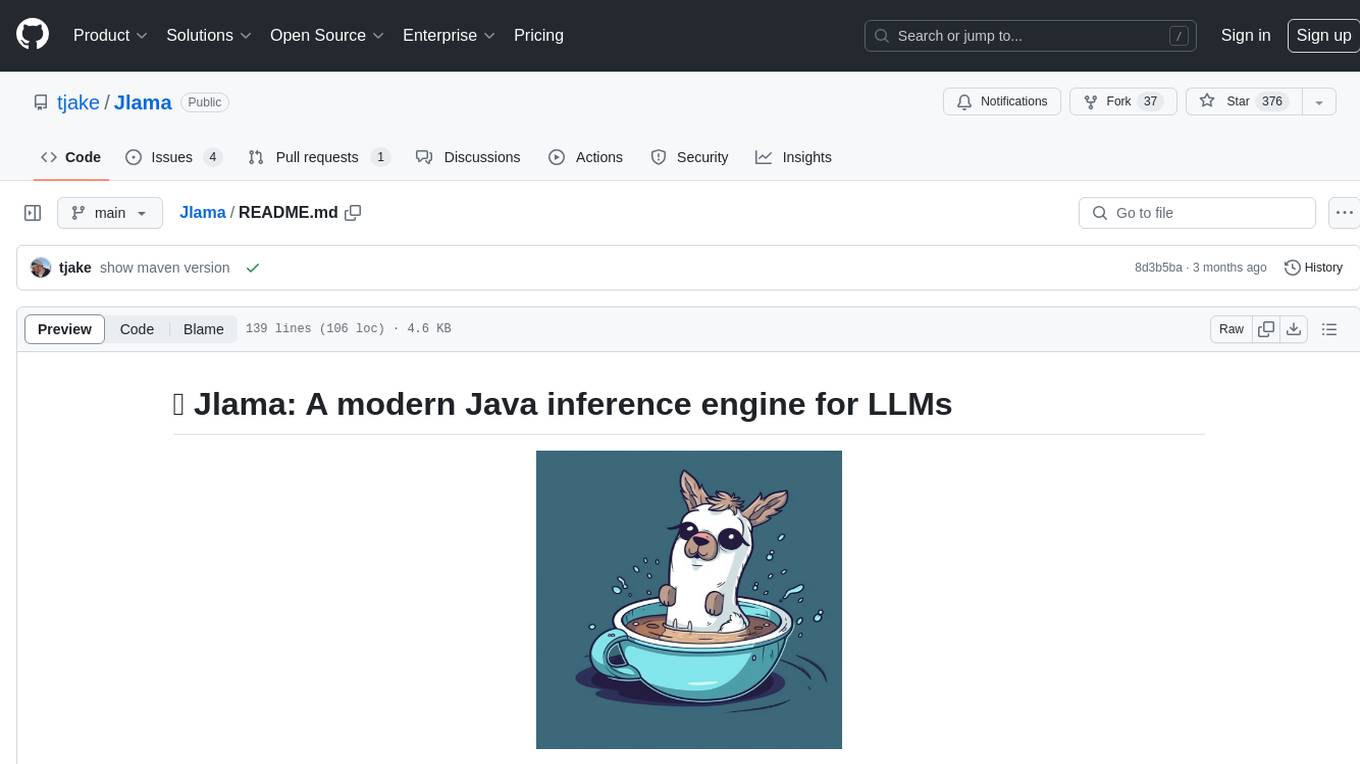
Jlama
Jlama is a modern Java inference engine designed for large language models. It supports various model types such as Gemma, Llama, Mistral, GPT-2, BERT, and more. The tool implements features like Flash Attention, Mixture of Experts, and supports different model quantization formats. Built with Java 21 and utilizing the new Vector API for faster inference, Jlama allows users to add LLM inference directly to their Java applications. The tool includes a CLI for running models, a simple UI for chatting with LLMs, and examples for different model types.
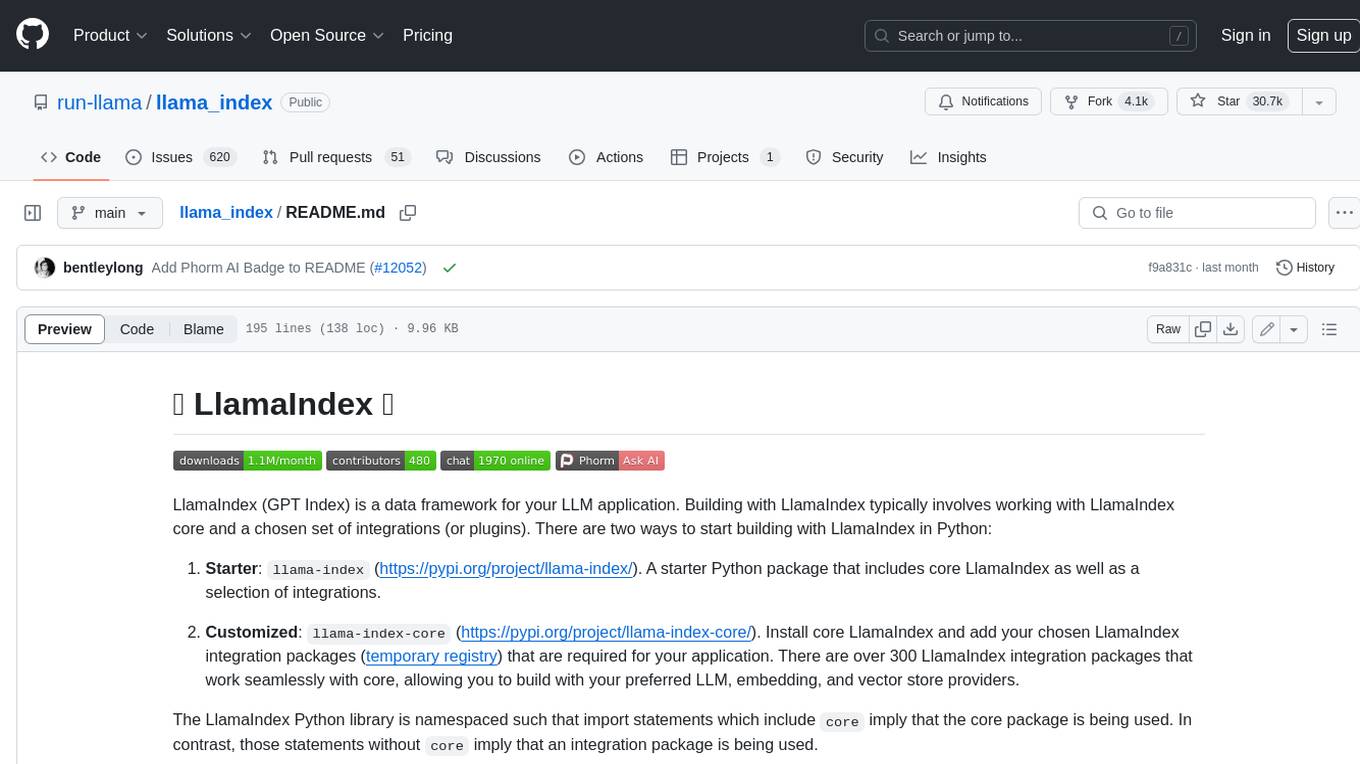
llama_index
LlamaIndex is a data framework for building LLM applications. It provides tools for ingesting, structuring, and querying data, as well as integrating with LLMs and other tools. LlamaIndex is designed to be easy to use for both beginner and advanced users, and it provides a comprehensive set of features for building LLM applications.
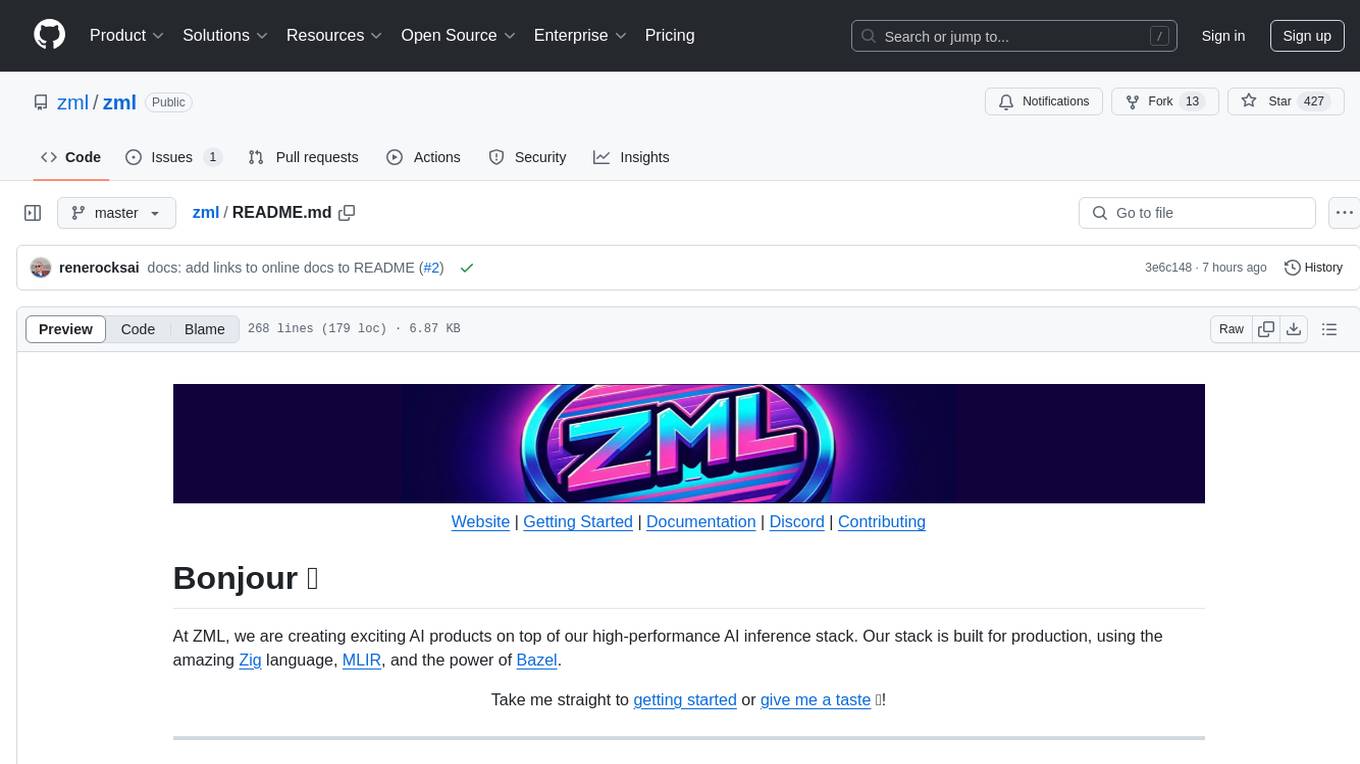
zml
ZML is a high-performance AI inference stack built for production, using Zig language, MLIR, and Bazel. It allows users to create exciting AI projects, run pre-packaged models like MNIST, TinyLlama, OpenLLama, and Meta Llama, and compile models for accelerator runtimes. Users can also run tests, explore examples, and contribute to the project. ZML is licensed under the Apache 2.0 license.
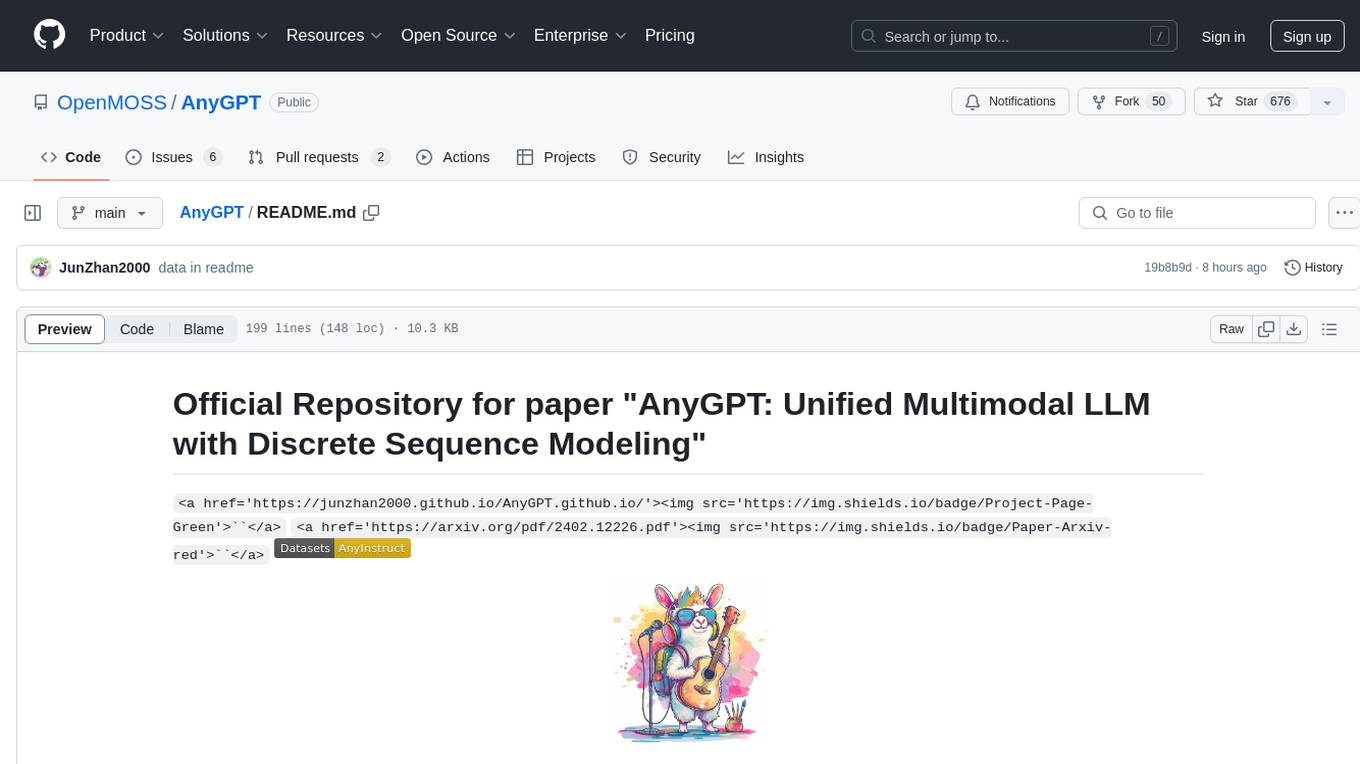
AnyGPT
AnyGPT is a unified multimodal language model that utilizes discrete representations for processing various modalities like speech, text, images, and music. It aligns the modalities for intermodal conversions and text processing. AnyInstruct dataset is constructed for generative models. The model proposes a generative training scheme using Next Token Prediction task for training on a Large Language Model (LLM). It aims to compress vast multimodal data on the internet into a single model for emerging capabilities. The tool supports tasks like text-to-image, image captioning, ASR, TTS, text-to-music, and music captioning.
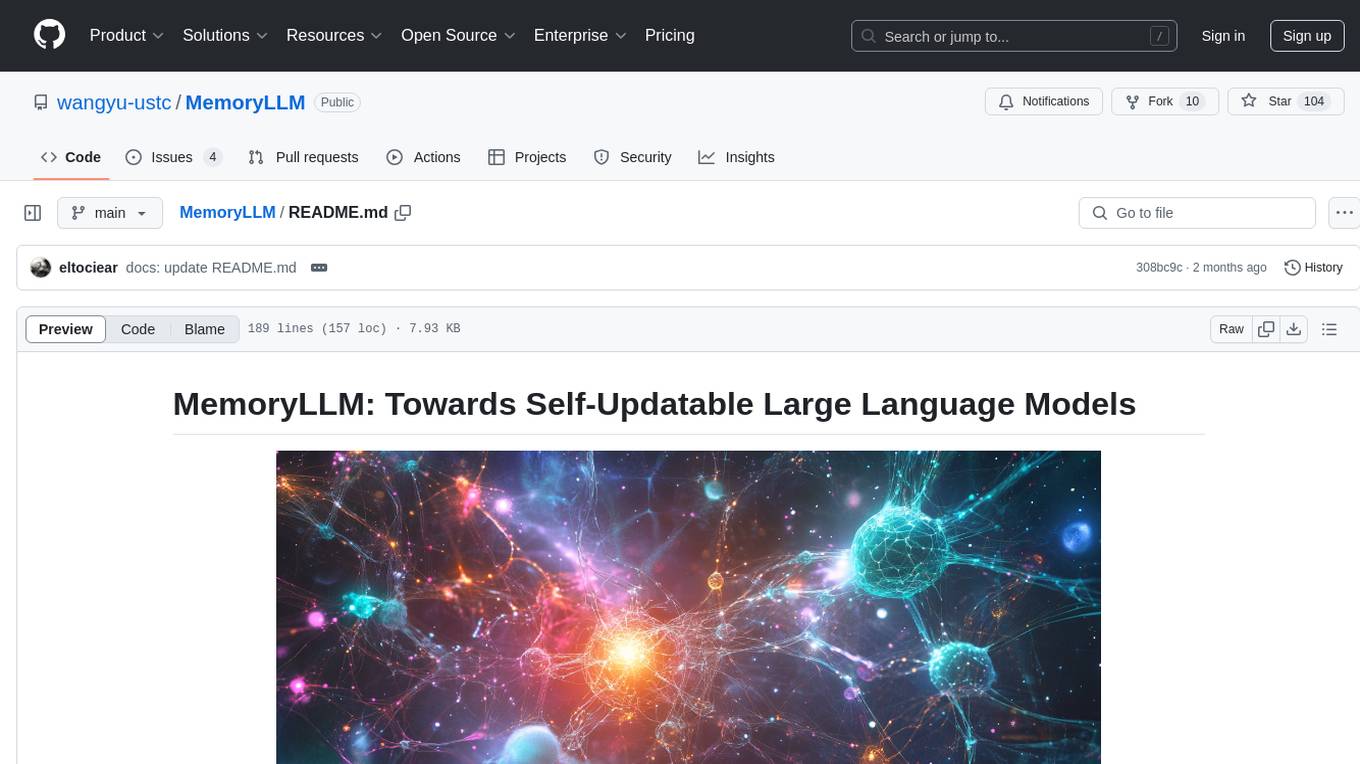
MemoryLLM
MemoryLLM is a large language model designed for self-updating capabilities. It offers pretrained models with different memory capacities and features, such as chat models. The repository provides training code, evaluation scripts, and datasets for custom experiments. MemoryLLM aims to enhance knowledge retention and performance on various natural language processing tasks.
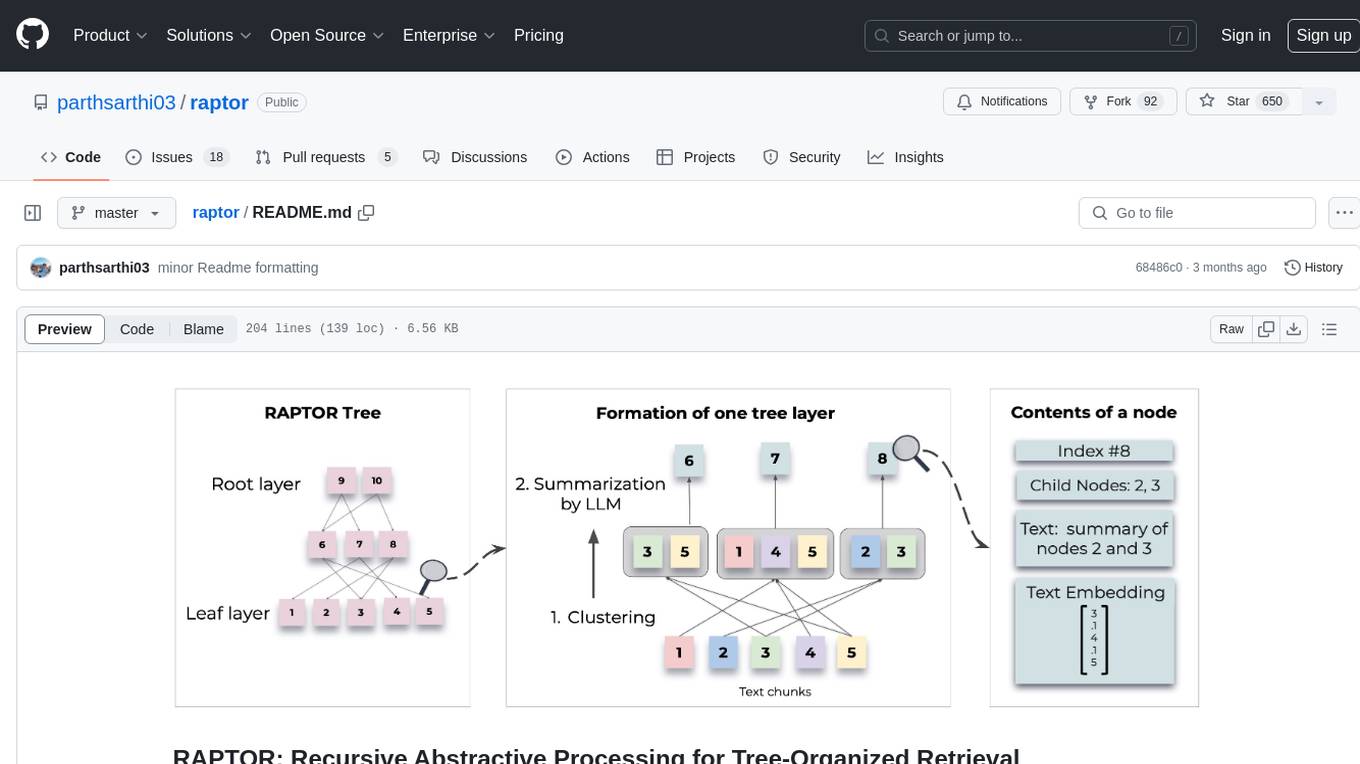
raptor
RAPTOR introduces a novel approach to retrieval-augmented language models by constructing a recursive tree structure from documents. This allows for more efficient and context-aware information retrieval across large texts, addressing common limitations in traditional language models. Users can add documents to the tree, answer questions based on indexed documents, save and load the tree, and extend RAPTOR with custom summarization, question-answering, and embedding models. The tool is designed to be flexible and customizable for various NLP tasks.
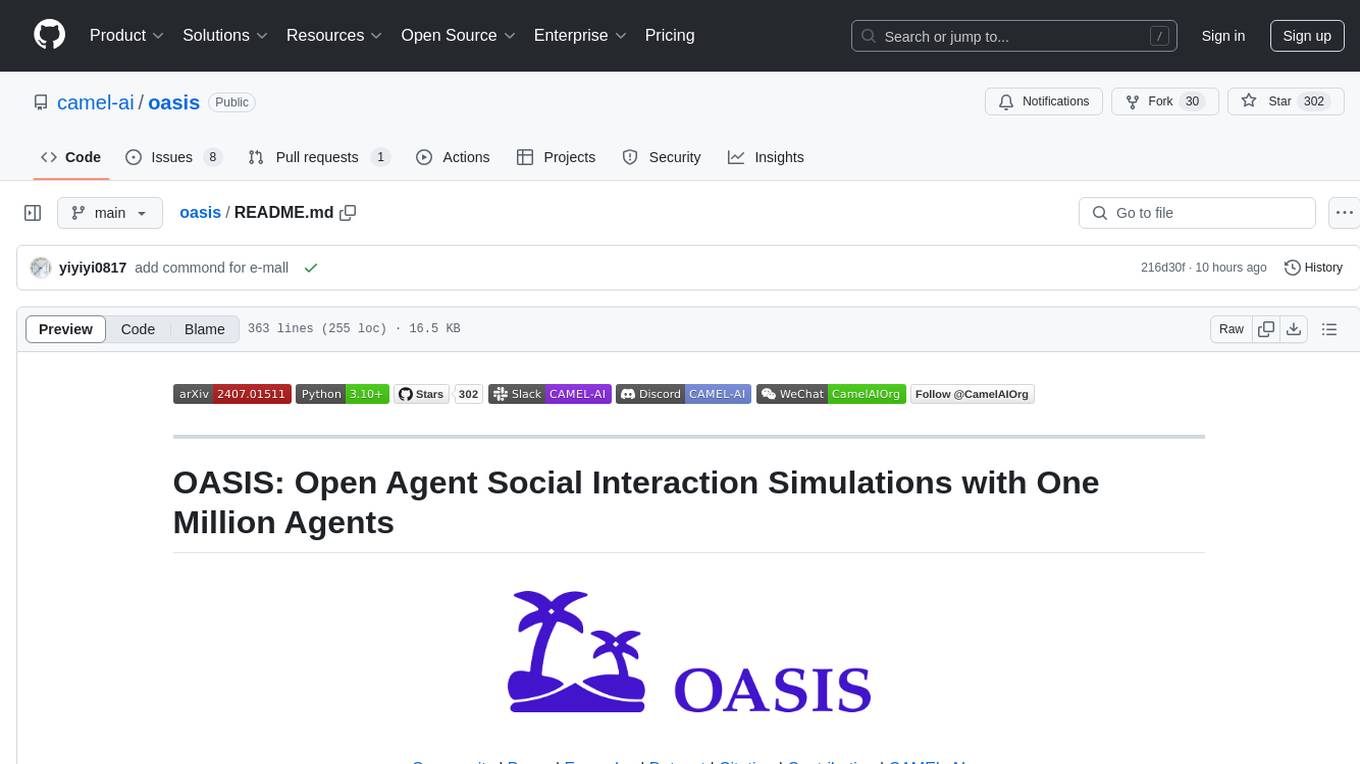
oasis
OASIS is a scalable, open-source social media simulator that integrates large language models with rule-based agents to realistically mimic the behavior of up to one million users on platforms like Twitter and Reddit. It facilitates the study of complex social phenomena such as information spread, group polarization, and herd behavior, offering a versatile tool for exploring diverse social dynamics and user interactions in digital environments. With features like scalability, dynamic environments, diverse action spaces, and integrated recommendation systems, OASIS provides a comprehensive platform for simulating social media interactions at a large scale.
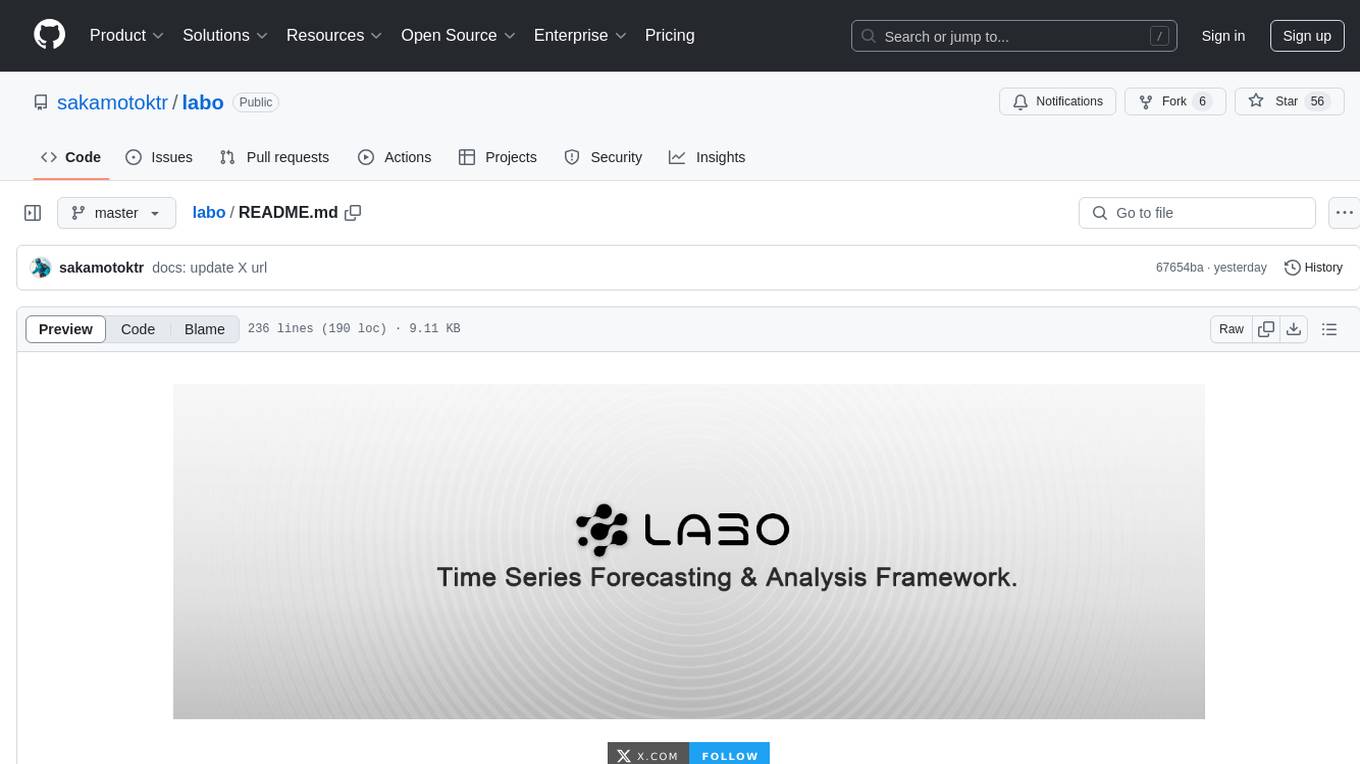
labo
LABO is a time series forecasting and analysis framework that integrates pre-trained and fine-tuned LLMs with multi-domain agent-based systems. It allows users to create and tune agents easily for various scenarios, such as stock market trend prediction and web public opinion analysis. LABO requires a specific runtime environment setup, including system requirements, Python environment, dependency installations, and configurations. Users can fine-tune their own models using LABO's Low-Rank Adaptation (LoRA) for computational efficiency and continuous model updates. Additionally, LABO provides a Python library for building model training pipelines and customizing agents for specific tasks.
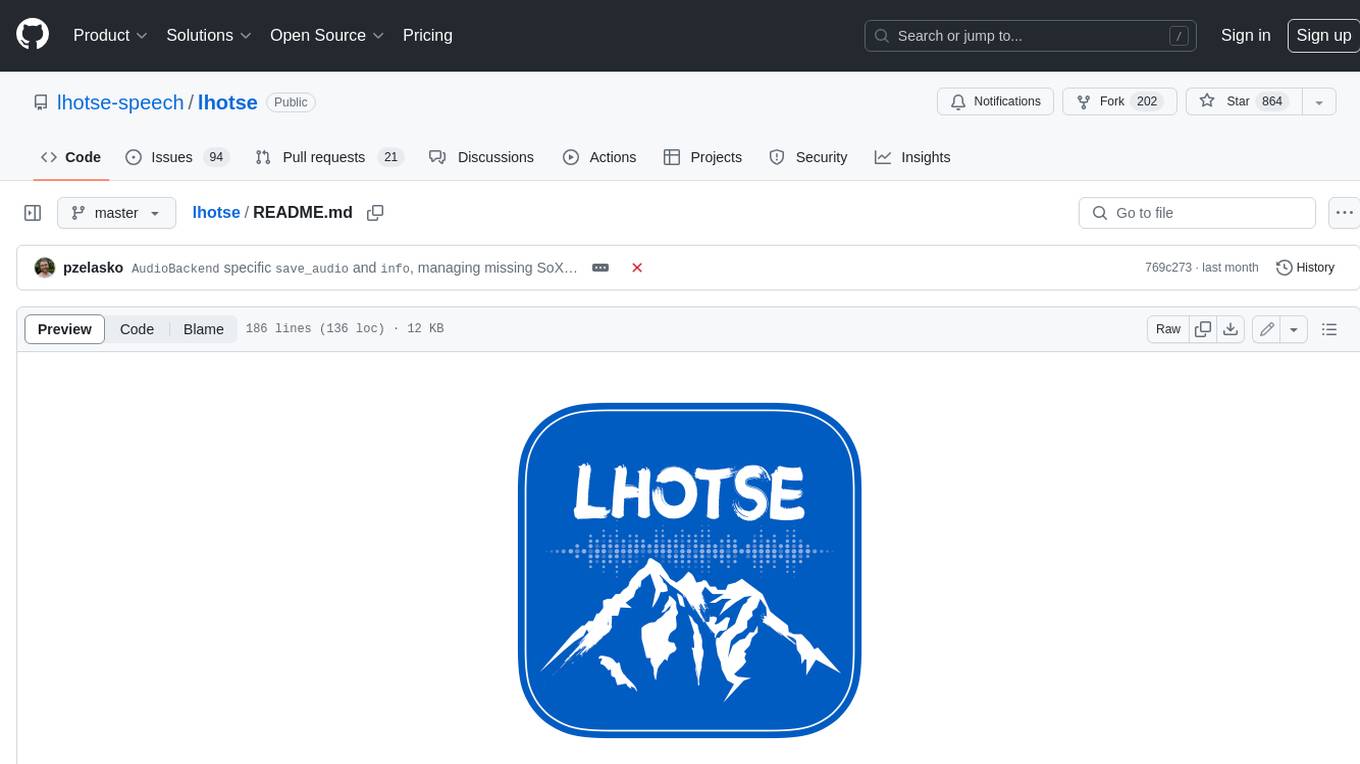
lhotse
Lhotse is a Python library designed to make speech and audio data preparation flexible and accessible. It aims to attract a wider community to speech processing tasks by providing a Python-centric design and an expressive command-line interface. Lhotse offers standard data preparation recipes, PyTorch Dataset classes for speech tasks, and efficient data preparation for model training with audio cuts. It supports data augmentation, feature extraction, and feature-space cut mixing. The tool extends Kaldi's data preparation recipes with seamless PyTorch integration, human-readable text manifests, and convenient Python classes.
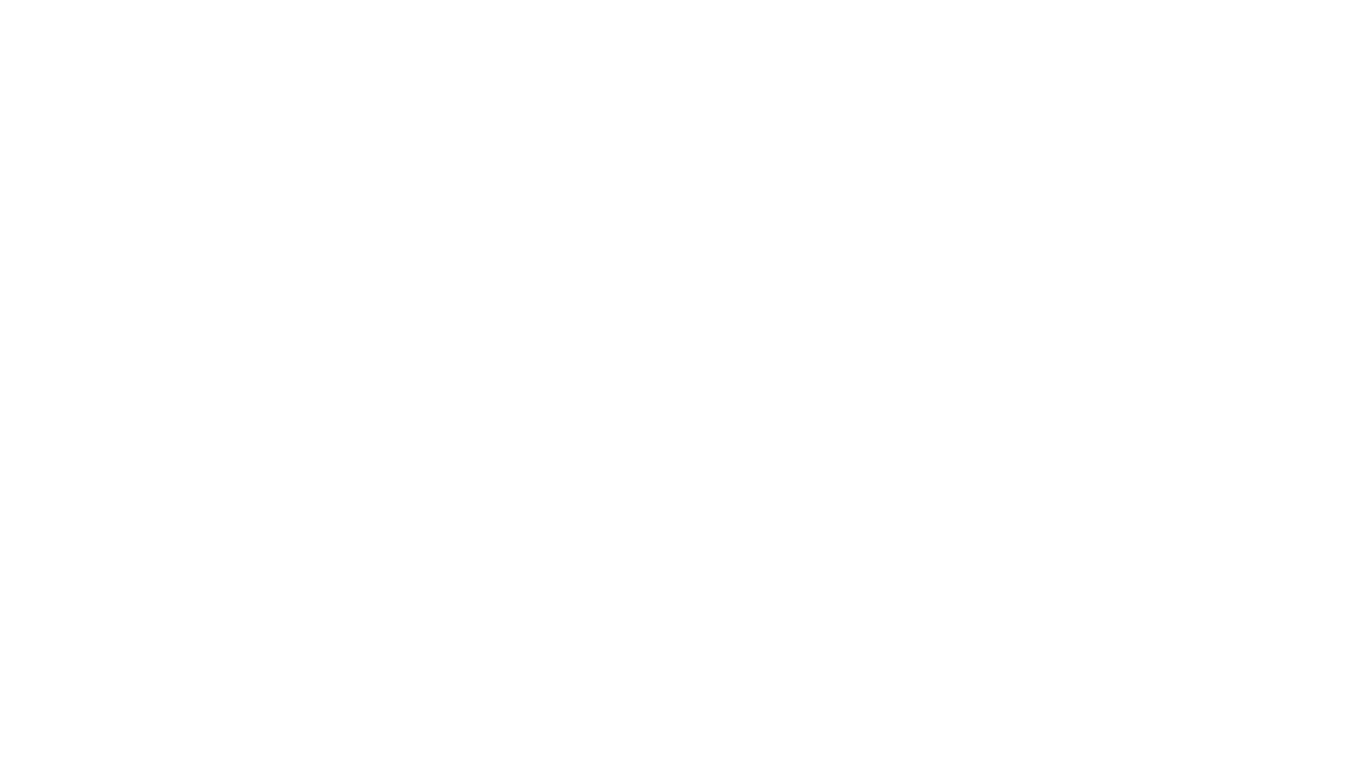
generative-ai
The 'Generative AI' repository provides a C# library for interacting with Google's Generative AI models, specifically the Gemini models. It allows users to access and integrate the Gemini API into .NET applications, supporting functionalities such as listing available models, generating content, creating tuned models, working with large files, starting chat sessions, and more. The repository also includes helper classes and enums for Gemini API aspects. Authentication methods include API key, OAuth, and various authentication modes for Google AI and Vertex AI. The package offers features for both Google AI Studio and Google Cloud Vertex AI, with detailed instructions on installation, usage, and troubleshooting.
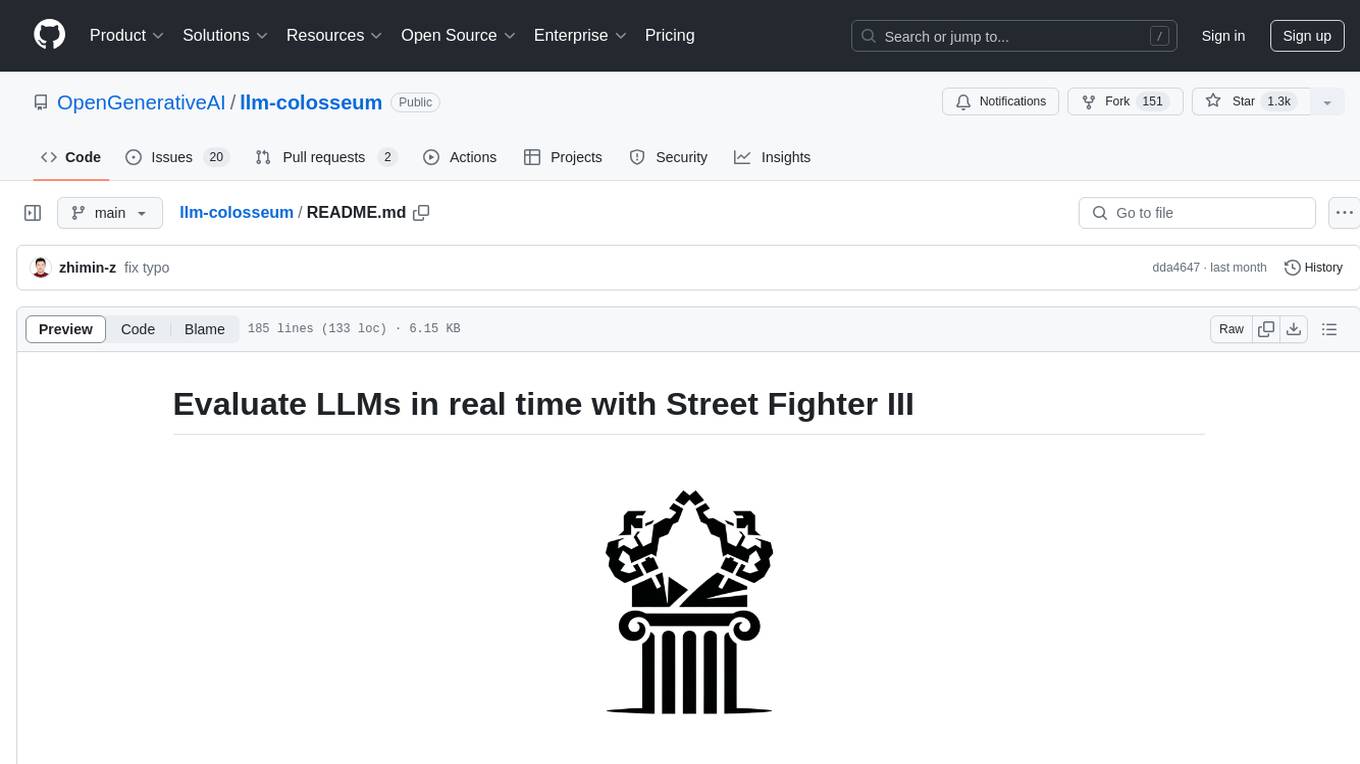
llm-colosseum
llm-colosseum is a tool designed to evaluate Language Model Models (LLMs) in real-time by making them fight each other in Street Fighter III. The tool assesses LLMs based on speed, strategic thinking, adaptability, out-of-the-box thinking, and resilience. It provides a benchmark for LLMs to understand their environment and take context-based actions. Users can analyze the performance of different LLMs through ELO rankings and win rate matrices. The tool allows users to run experiments, test different LLM models, and customize prompts for LLM interactions. It offers installation instructions, test mode options, logging configurations, and the ability to run the tool with local models. Users can also contribute their own LLM models for evaluation and ranking.
For similar tasks
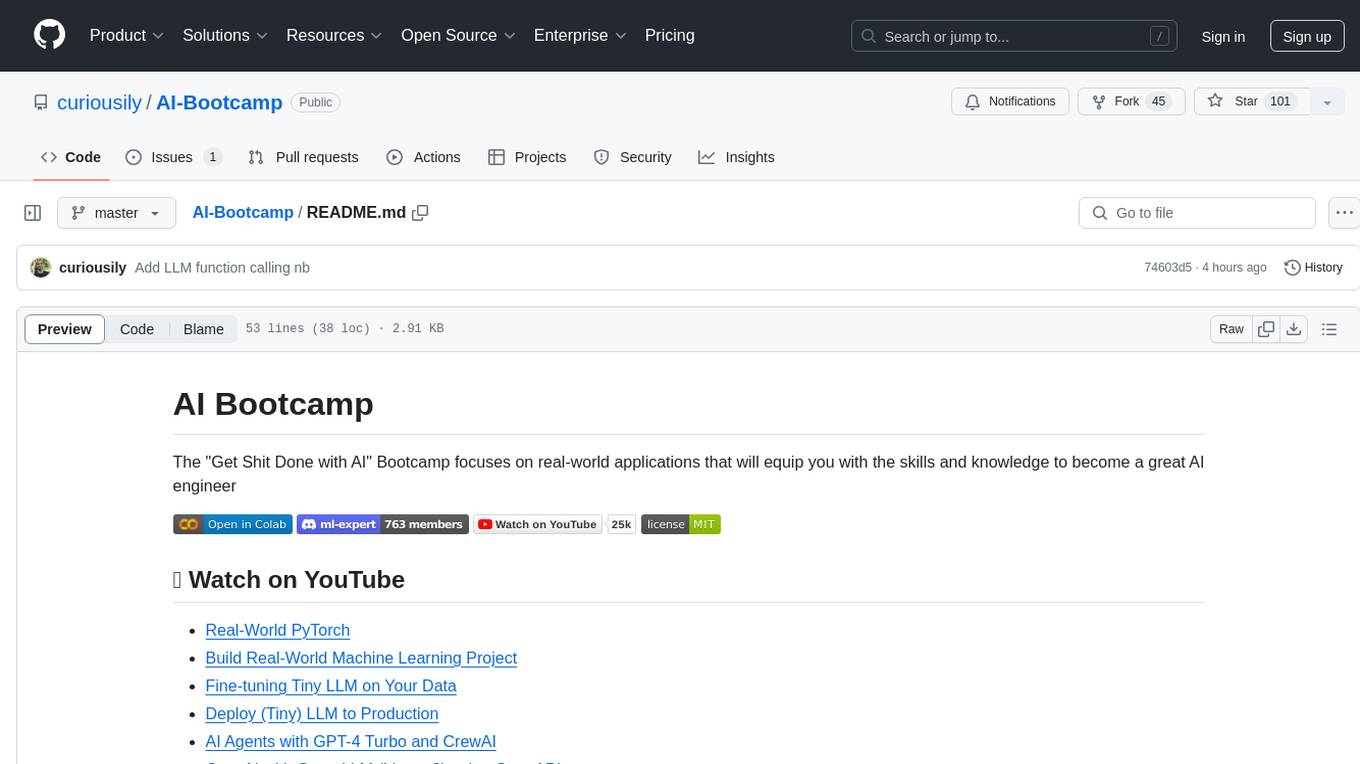
AI-Bootcamp
The AI Bootcamp is a comprehensive training program focusing on real-world applications to equip individuals with the skills and knowledge needed to excel as AI engineers. The bootcamp covers topics such as Real-World PyTorch, Machine Learning Projects, Fine-tuning Tiny LLM, Deployment of LLM to Production, AI Agents with GPT-4 Turbo, CrewAI, Llama 3, and more. Participants will learn foundational skills in Python for AI, ML Pipelines, Large Language Models (LLMs), AI Agents, and work on projects like RagBase for private document chat.
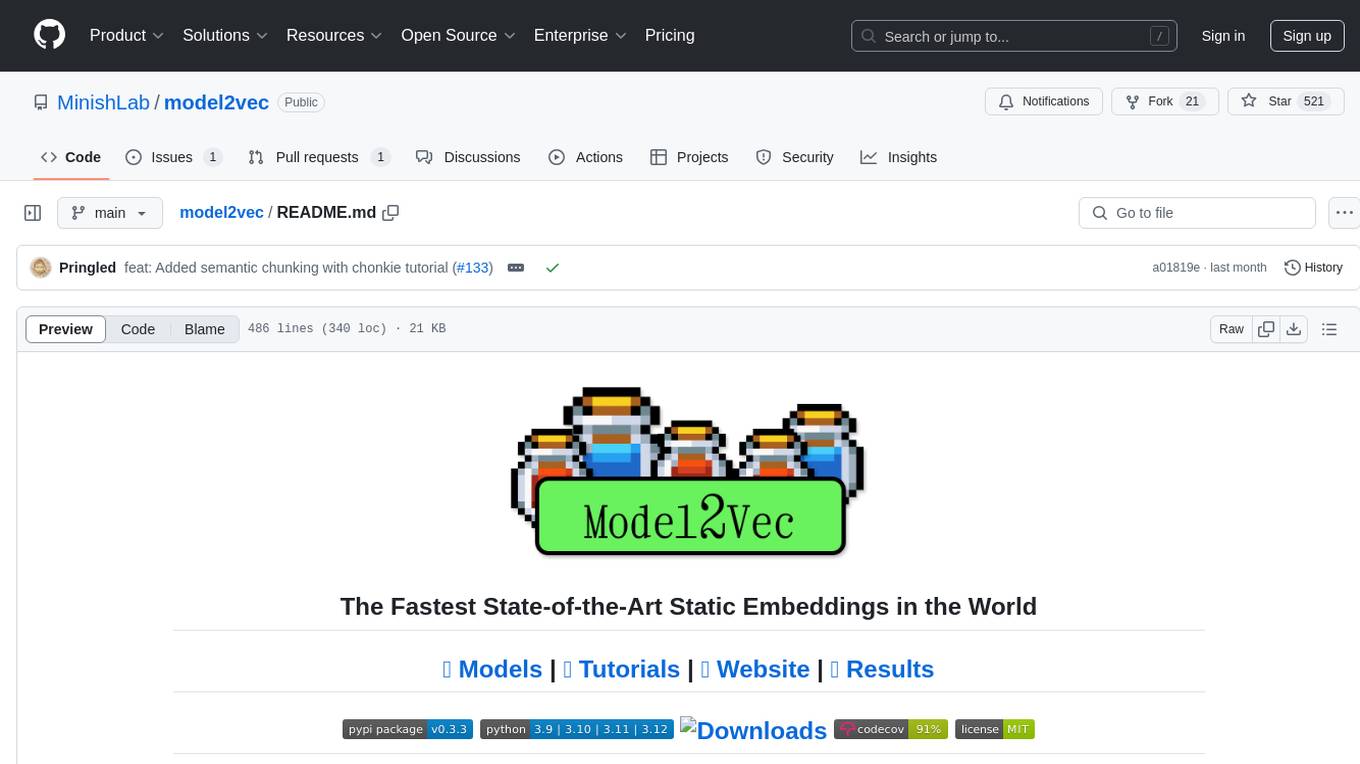
model2vec
Model2Vec is a technique to turn any sentence transformer into a really small static model, reducing model size by 15x and making the models up to 500x faster, with a small drop in performance. It outperforms other static embedding models like GLoVe and BPEmb, is lightweight with only `numpy` as a major dependency, offers fast inference, dataset-free distillation, and is integrated into Sentence Transformers, txtai, and Chonkie. Model2Vec creates powerful models by passing a vocabulary through a sentence transformer model, reducing dimensionality using PCA, and weighting embeddings using zipf weighting. Users can distill their own models or use pre-trained models from the HuggingFace hub. Evaluation can be done using the provided evaluation package. Model2Vec is licensed under MIT.
For similar jobs
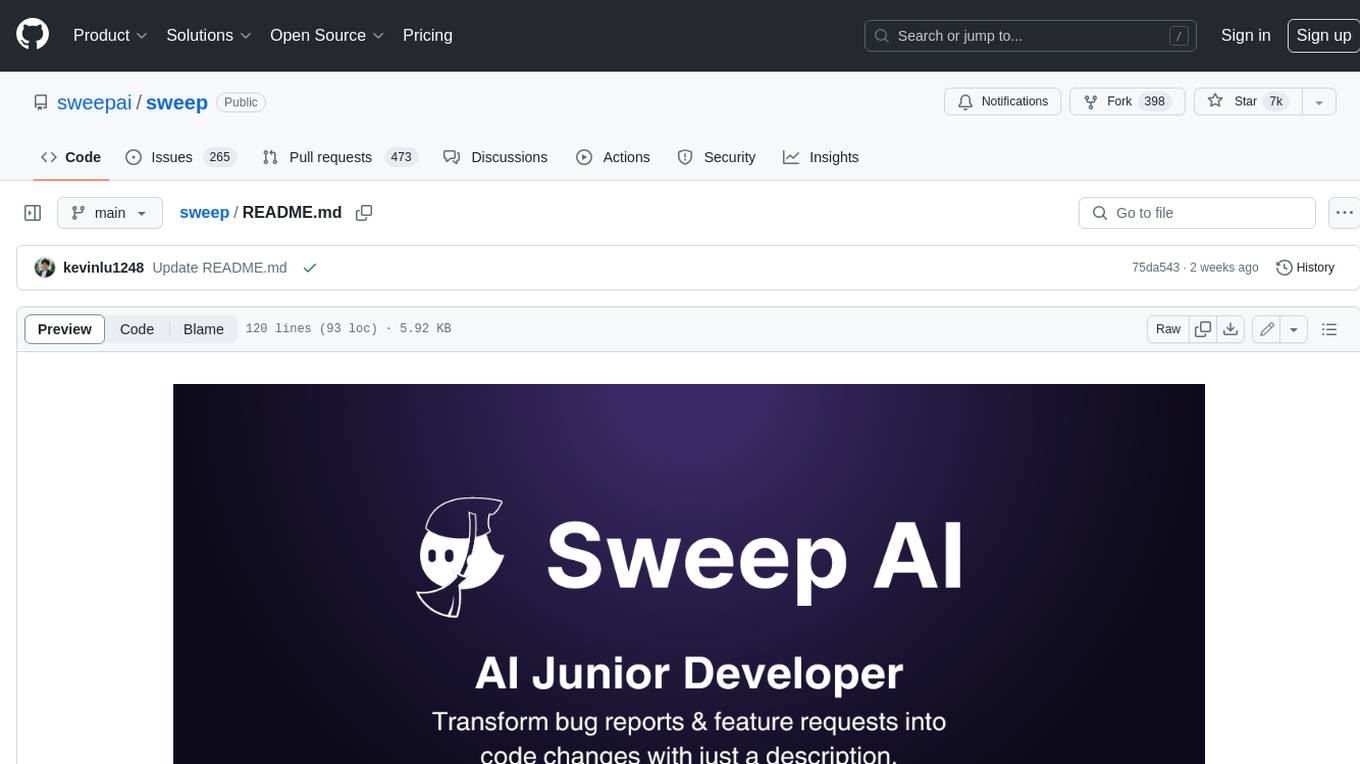
sweep
Sweep is an AI junior developer that turns bugs and feature requests into code changes. It automatically handles developer experience improvements like adding type hints and improving test coverage.
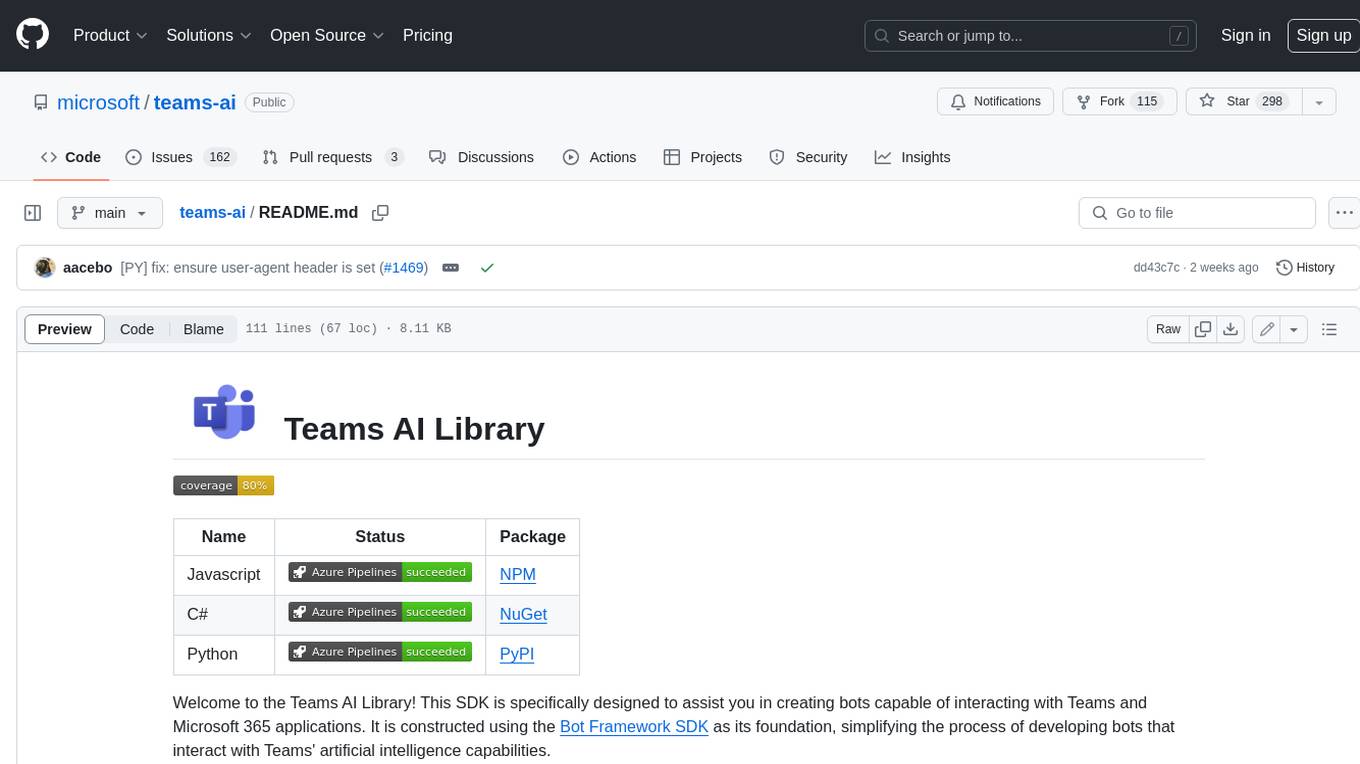
teams-ai
The Teams AI Library is a software development kit (SDK) that helps developers create bots that can interact with Teams and Microsoft 365 applications. It is built on top of the Bot Framework SDK and simplifies the process of developing bots that interact with Teams' artificial intelligence capabilities. The SDK is available for JavaScript/TypeScript, .NET, and Python.
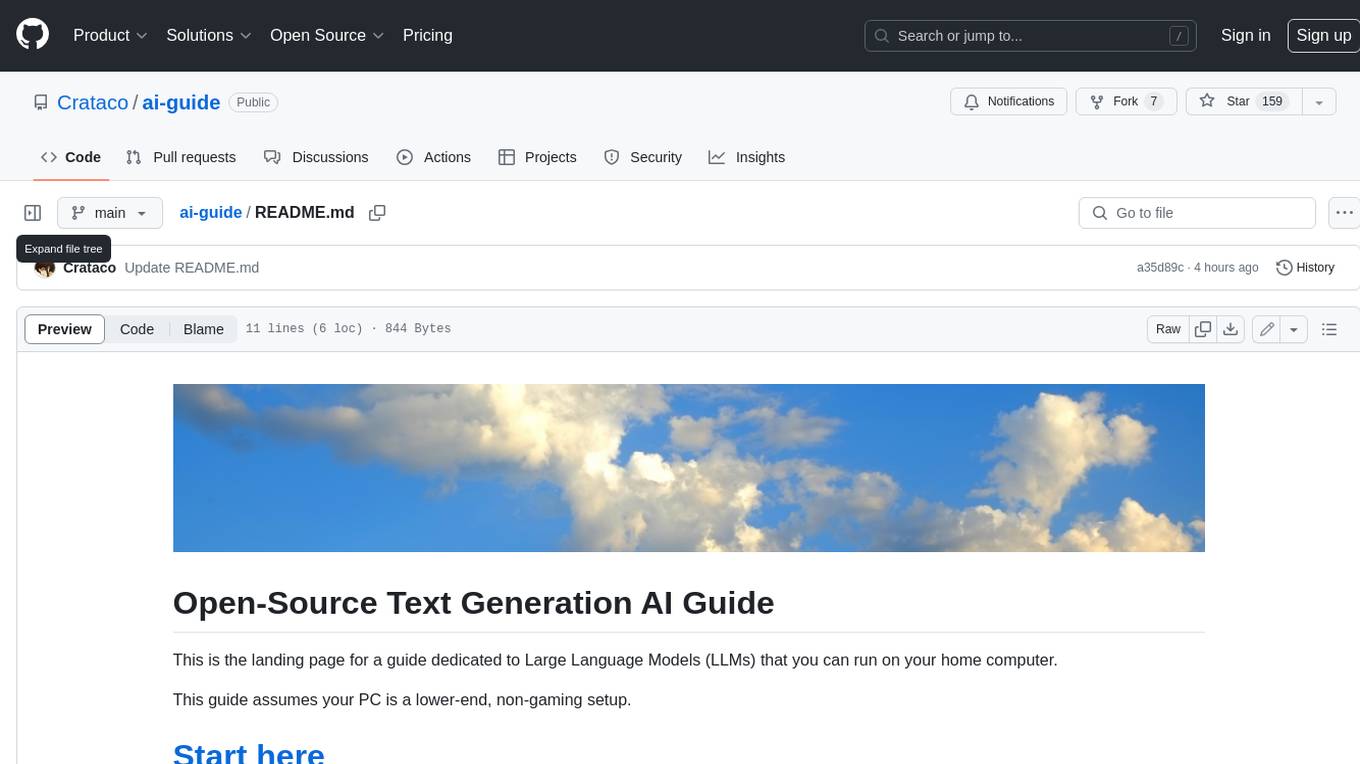
ai-guide
This guide is dedicated to Large Language Models (LLMs) that you can run on your home computer. It assumes your PC is a lower-end, non-gaming setup.
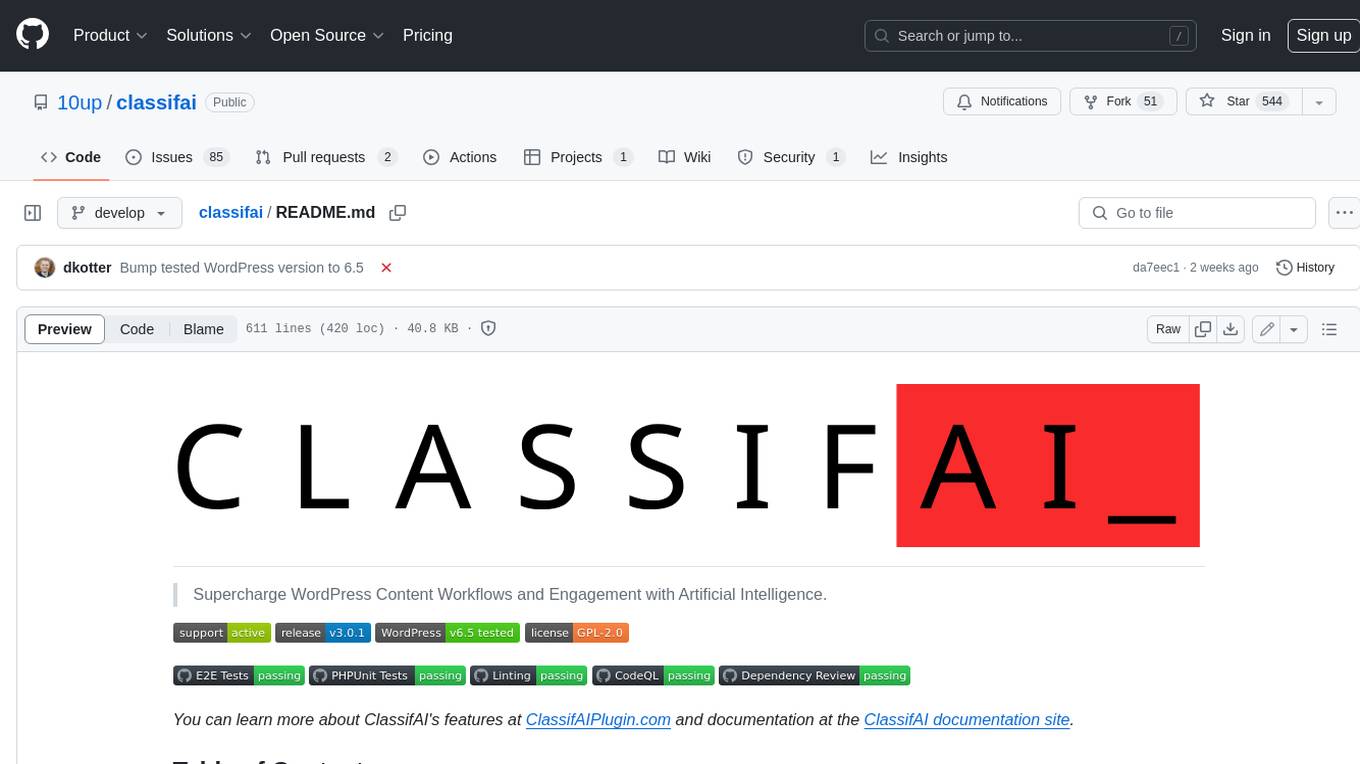
classifai
Supercharge WordPress Content Workflows and Engagement with Artificial Intelligence. Tap into leading cloud-based services like OpenAI, Microsoft Azure AI, Google Gemini and IBM Watson to augment your WordPress-powered websites. Publish content faster while improving SEO performance and increasing audience engagement. ClassifAI integrates Artificial Intelligence and Machine Learning technologies to lighten your workload and eliminate tedious tasks, giving you more time to create original content that matters.
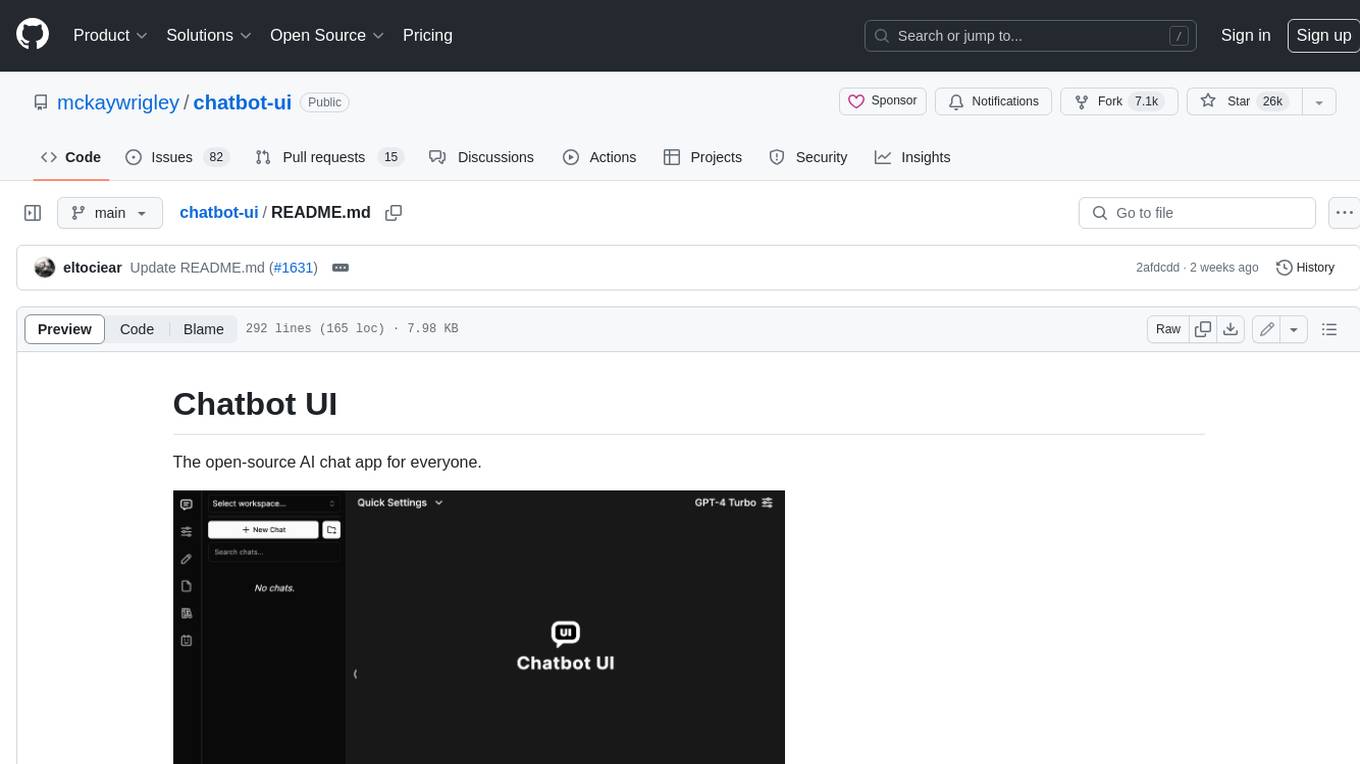
chatbot-ui
Chatbot UI is an open-source AI chat app that allows users to create and deploy their own AI chatbots. It is easy to use and can be customized to fit any need. Chatbot UI is perfect for businesses, developers, and anyone who wants to create a chatbot.
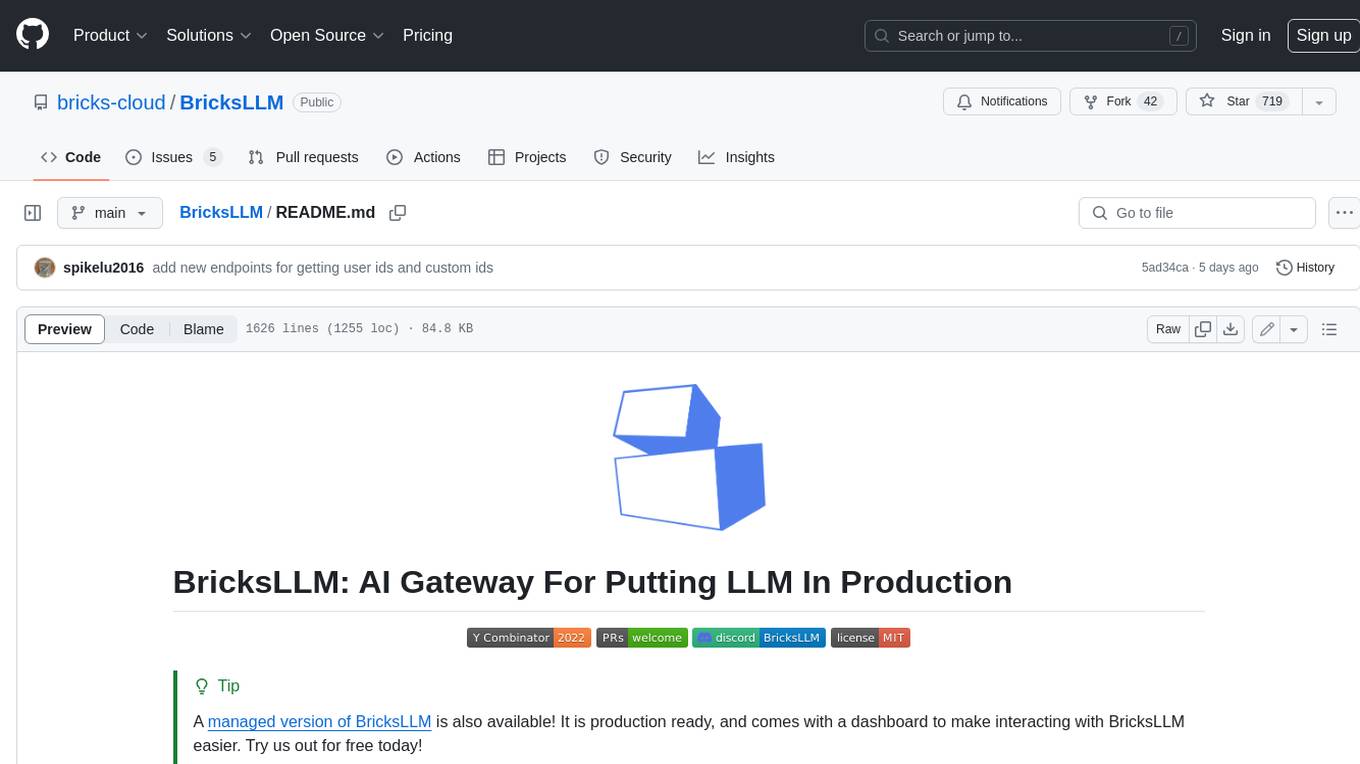
BricksLLM
BricksLLM is a cloud native AI gateway written in Go. Currently, it provides native support for OpenAI, Anthropic, Azure OpenAI and vLLM. BricksLLM aims to provide enterprise level infrastructure that can power any LLM production use cases. Here are some use cases for BricksLLM: * Set LLM usage limits for users on different pricing tiers * Track LLM usage on a per user and per organization basis * Block or redact requests containing PIIs * Improve LLM reliability with failovers, retries and caching * Distribute API keys with rate limits and cost limits for internal development/production use cases * Distribute API keys with rate limits and cost limits for students
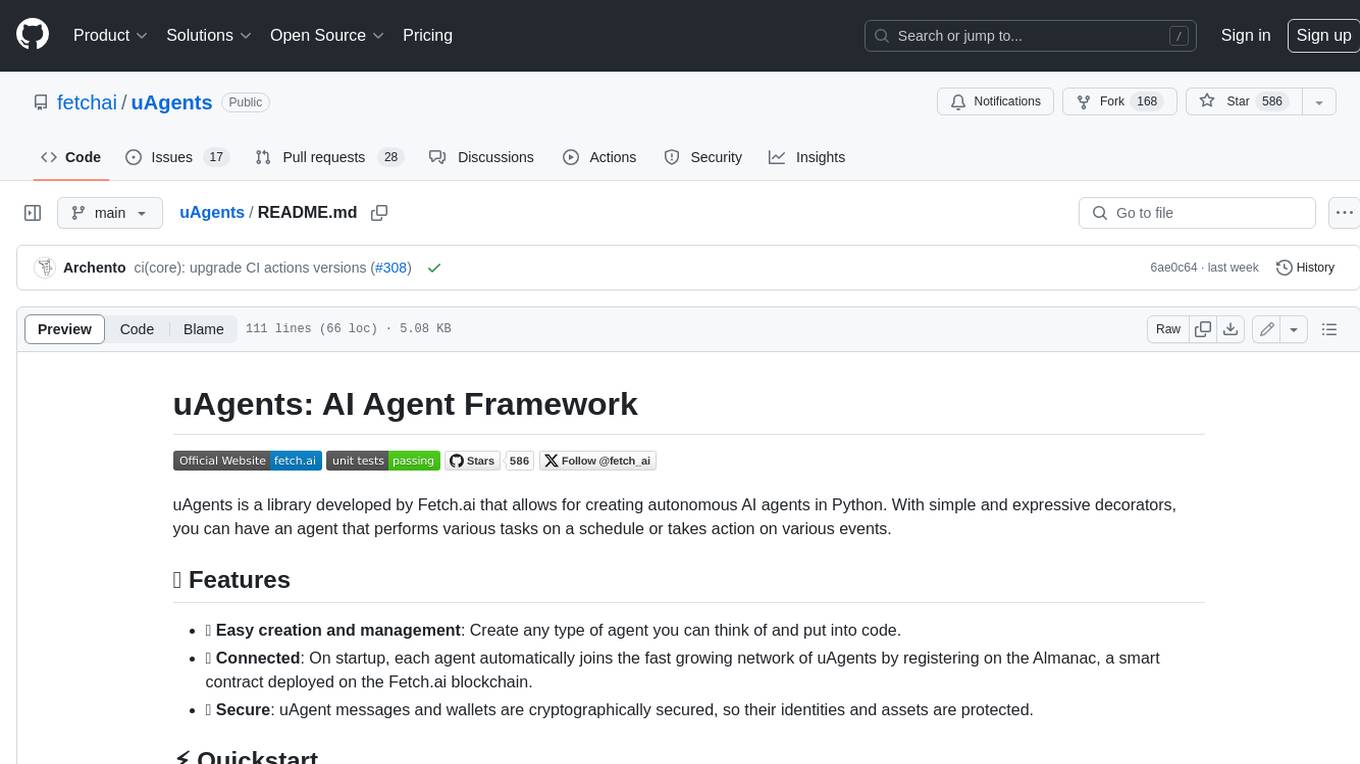
uAgents
uAgents is a Python library developed by Fetch.ai that allows for the creation of autonomous AI agents. These agents can perform various tasks on a schedule or take action on various events. uAgents are easy to create and manage, and they are connected to a fast-growing network of other uAgents. They are also secure, with cryptographically secured messages and wallets.
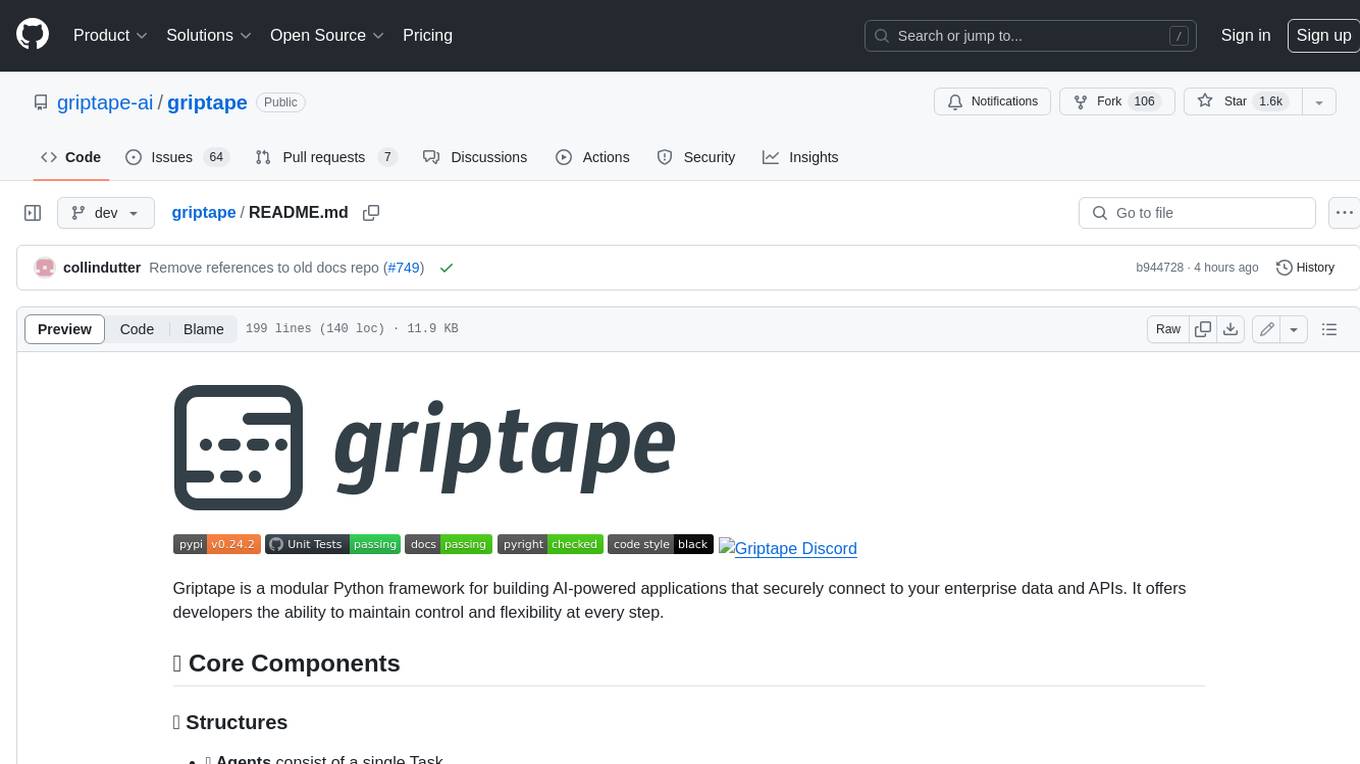
griptape
Griptape is a modular Python framework for building AI-powered applications that securely connect to your enterprise data and APIs. It offers developers the ability to maintain control and flexibility at every step. Griptape's core components include Structures (Agents, Pipelines, and Workflows), Tasks, Tools, Memory (Conversation Memory, Task Memory, and Meta Memory), Drivers (Prompt and Embedding Drivers, Vector Store Drivers, Image Generation Drivers, Image Query Drivers, SQL Drivers, Web Scraper Drivers, and Conversation Memory Drivers), Engines (Query Engines, Extraction Engines, Summary Engines, Image Generation Engines, and Image Query Engines), and additional components (Rulesets, Loaders, Artifacts, Chunkers, and Tokenizers). Griptape enables developers to create AI-powered applications with ease and efficiency.