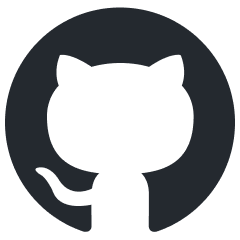
SeerAttention
SeerAttention: Learning Intrinsic Sparse Attention in Your LLMs
Stars: 73
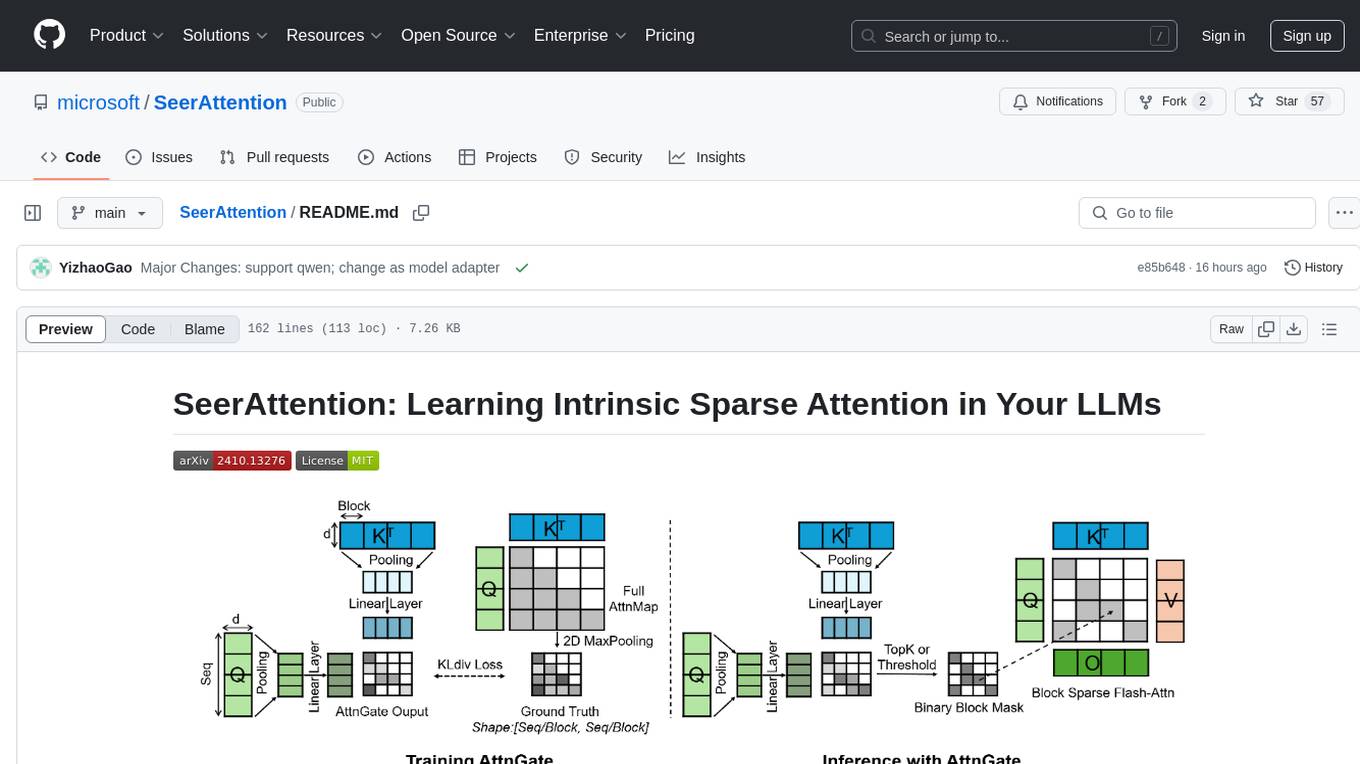
SeerAttention is a novel trainable sparse attention mechanism that learns intrinsic sparsity patterns directly from LLMs through self-distillation at post-training time. It achieves faster inference while maintaining accuracy for long-context prefilling. The tool offers features such as trainable sparse attention, block-level sparsity, self-distillation, efficient kernel, and easy integration with existing transformer architectures. Users can quickly start using SeerAttention for inference with AttnGate Adapter and training attention gates with self-distillation. The tool provides efficient evaluation methods and encourages contributions from the community.
README:
Official implementation of SeerAttention - a novel trainable sparse attention mechanism that learns intrinsic sparsity patterns directly from LLMs through self-distillation at post-training time. Achieves faster inference while maintaining accuracy for long-context prefilling.
- 2025/3/5: Release AttnGates of DeepSeek-R1-Distill-Qwen on HF. Release sparse flash-attn kernel with bwd for fine-tuning.
- 2025/2/23: Support Qwen! Change the distillation into model adapter so that only AttnGates are saved.
- 2025/2/18: Deepseek's Native Sparse Attention (NSA) and Kimi's Mixture of Block Attention (MoBA) all aquire similar trainable sparse attention concepts as us for pretrain models. Great works!
Trainable Sparse Attention - Outperform static/predefined attention sparsity
Block-level Sparsity - Hardware efficient sparsity at block level
Self-Distillation - Lightweight training of attention gates (original weights frozen)
Efficient Kernel - Block-sparse FlashAttention implementation
Easy Integration - Works with existing transformer architectures
The current codebase is improved by only saving the distilled AttnGates' weights. During inference, you can composed the AttnGates and original base model. Check the latest huggingface repos!
Base Model | HF Link | AttnGates Size |
---|---|---|
Llama-3.1-8B-Instruct | SeerAttention/SeerAttention-Llama-3.1-8B-AttnGates | 101 MB |
Llama-3.1-70B-Instruct | SeerAttention/SeerAttention-Llama-3.1-70B-AttnGates | 503 MB |
Qwen2.5-7B-Instruct | SeerAttention/SeerAttention-Qwen2.5-7B-AttnGates | 77 MB |
Qwen2.5-14B-Instruct | SeerAttention/SeerAttention-Qwen2.5-14B-AttnGates | 189 MB |
Qwen2.5-32B-Instruct | SeerAttention/SeerAttention-Qwen2.5-32B-AttnGates | 252 MB |
deepseek-ai/DeepSeek-R1-Distill-Qwen-14B | SeerAttention/SeerAttention-DeepSeek-R1-Distill-Qwen-14B-AttnGates | 189 MB |
deepseek-ai/DeepSeek-R1-Distill-Qwen-32B | SeerAttention/SeerAttention-DeepSeek-R1-Distill-Qwen-32B-AttnGates | 252 MB |
conda create -yn seer python=3.11
conda activate seer
pip install torch==2.4.0
pip install -r requirements.txt
pip install -e .
During inference, we automatically compose your original base model with our distilled AttnGates.
SeerAttention supports two sparse methods (Threshold / TopK) to convert a soft gating score to hard binary attention mask. Currently we simply use a single sparse configuration for all the attention heads. You are encourage to explore other configurations to tradeoff the speedup vs quality.
from transformers import AutoTokenizer, AutoConfig
from seer_attn import SeerAttnLlamaForCausalLM
model_name = "SeerAttention/SeerAttention-Llama-3.1-8B-AttnGates"
config = AutoConfig.from_pretrained(model_name)
tokenizer = AutoTokenizer.from_pretrained(
config.base_model,
padding_side="left",
)
## This will compose the AttnGates and base model
model = SeerAttnLlamaForCausalLM.from_pretrained(
model_name,
torch_dtype=torch.bfloat16,
seerattn_sparsity_method='threshold', # Using a threshold based sparse method
seerattn_threshold = 5e-4, # Higher = sparser, typical range 5e-4 ~ 5e-3
)
# Or using a TopK based sparse method
model = SeerAttnLlamaForCausalLM.from_pretrained(
model_name,
torch_dtype=torch.bfloat16,
seerattn_sparsity_method='nz_ratio',
seerattn_nz_ratio = 0.5, # Lower = sparser, typical range 0.1 ~ 0.9
)
model = model.cuda()
# Ready to inference
In the current self-distillation training setup, you can train the AttnGates for your own model. Here we give an example script for Llama-3.1-8B-Instruct. After the distillation process, the AttnGates' weights will be saved.
## scirpts to reproduce llama-3.1-8b
bash run_distillation.sh
We have a triton version and a CUDA version of 2D block-sparse flash-attn kernel for current SeerAttention inference. By default, the triton kernel is used as backend. The CUDA kernel is still being improved. See seer_attn/block_sparse_attention
for more details.
If first compute the intermediate attn-map (softmax(Q*K)) and then perform 2D maxpooled to generate the ground truth, it will cost huge GPU memory due to the quadratic size of the attn-map. Thus, we implement a kernel to directly generate the 2D maxpooled attn-map for efficient self-distillation training process.
### simple pseudo codo for self-distillation AttnGate training
from seer_attn.attn_pooling_kernel import attn_with_pooling
predict_mask = attn_gate(...)
attn_output, mask_ground_truth = attn_with_pooling(
query_states,
key_states,
value_states,
is_causal,
sm_scale,
block_size
)
###...
loss = self.loss_func(predict_mask, mask_ground_truth)
We implement two different kernels with backward for sparse-atttention-aware fine-tuning.
- Compress the sequence dimention for both Q, K and V. Similar to current SeerAttention Prefill.
from seer_attn import block_2d_sparse_attn_varlen_func
k = repeat_kv_varlen(k, self.num_key_value_groups)
v = repeat_kv_varlen(v, self.num_key_value_groups)
attn_output = block_2D_sparse_attn_varlen_func(
q, # [t, num_heads, head_dim]
k, # [t, num_heads, head_dim]
v, # [t, num_heads, head_dim]
cu_seqlens,
cu_seqlens,
max_seqlen,
1.0 / math.sqrt(self.head_dim),
block_mask, # [bsz, num_heads, ceil(t/block_size), ceil(t/block_size)]
block_size, # block_size of sparsity
)
- Compress only the sequence dimention of KV while enforcing all the heads within a GQA group share the same sparse mask. This is similar to the find-grained sparse branch of deepseek NSA.
from seer_attn import block_1d_gqa_sparse_attn_varlen_func
attn_output = block_1d_gqa_sparse_attn_varlen_func(
q, # [t, num_q_heads, head_dim]
k, # [t, num_kv_heads, head_dim]
v, # [t, num_kv_heads, head_dim]
cu_seqlens,
cu_seqlens,
max_seqlen,
1.0 / math.sqrt(self.head_dim),
block_mask, # [bsz, num_kv_heads, t, ceil(t/block_size)]
block_size, # block_size of sparsity
)
The code for fine-tuning with SeerAttention will be release soon.
For efficiency, we evaluate block_sparse_attn
compared with full attention by FlashAttention-2.
For model accuracy, we evaluate SeerAttention on PG19, Ruler and LongBench. Please refer to eval
folder for details.
If you find SeerAttention useful or want to use in your projects, please kindly cite our paper:
@article{gao2024seerattention,
title={SeerAttention: Learning Intrinsic Sparse Attention in Your LLMs},
author={Gao, Yizhao and Zeng, Zhichen and Du, Dayou and Cao, Shijie and So, Hayden Kwok-Hay and Cao, Ting and Yang, Fan and Yang, Mao},
journal={arXiv preprint arXiv:2410.13276},
year={2024}
}
This project welcomes contributions and suggestions. Most contributions require you to agree to a Contributor License Agreement (CLA) declaring that you have the right to, and actually do, grant us the rights to use your contribution. For details, visit https://cla.opensource.microsoft.com.
When you submit a pull request, a CLA bot will automatically determine whether you need to provide a CLA and decorate the PR appropriately (e.g., status check, comment). Simply follow the instructions provided by the bot. You will only need to do this once across all repos using our CLA.
This project has adopted the Microsoft Open Source Code of Conduct. For more information see the Code of Conduct FAQ or contact [email protected] with any additional questions or comments.
This project may contain trademarks or logos for projects, products, or services. Authorized use of Microsoft trademarks or logos is subject to and must follow Microsoft's Trademark & Brand Guidelines. Use of Microsoft trademarks or logos in modified versions of this project must not cause confusion or imply Microsoft sponsorship. Any use of third-party trademarks or logos are subject to those third-party's policies.
For Tasks:
Click tags to check more tools for each tasksFor Jobs:
Alternative AI tools for SeerAttention
Similar Open Source Tools
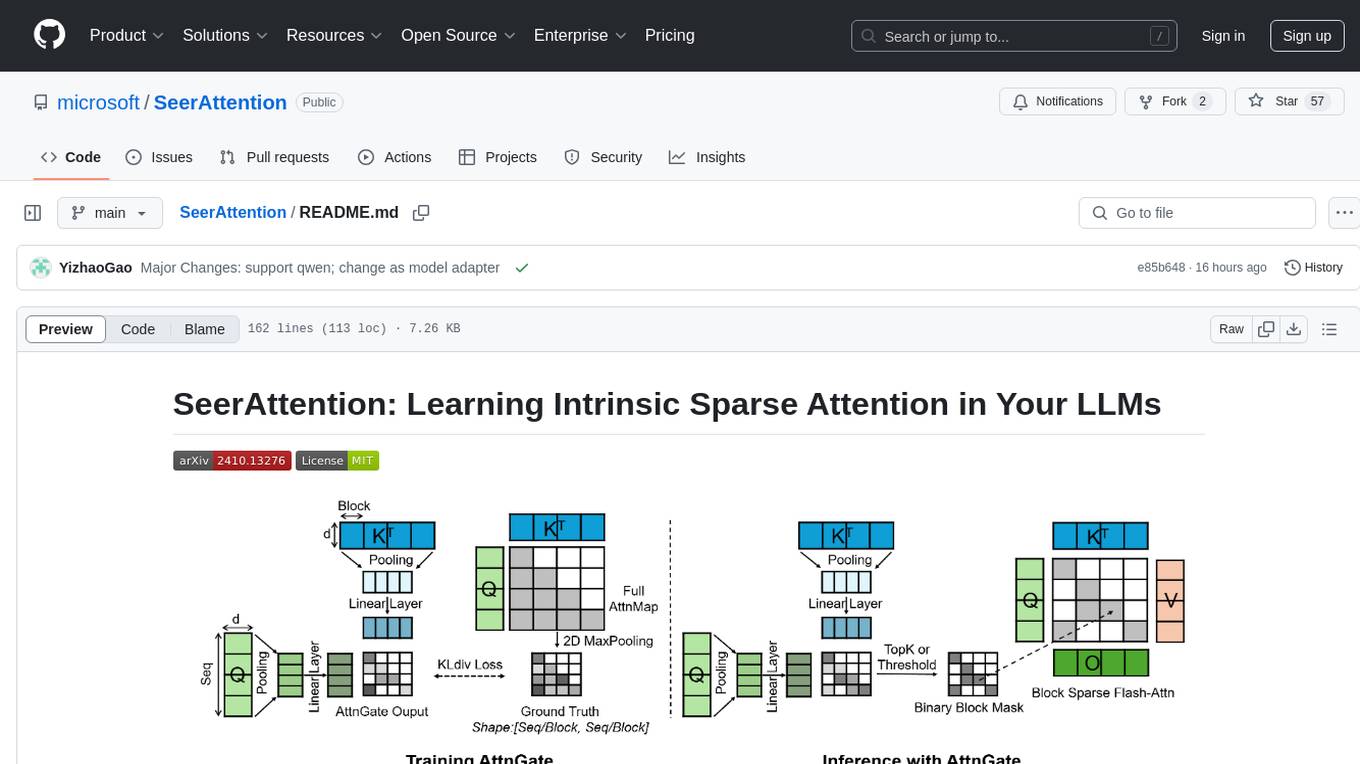
SeerAttention
SeerAttention is a novel trainable sparse attention mechanism that learns intrinsic sparsity patterns directly from LLMs through self-distillation at post-training time. It achieves faster inference while maintaining accuracy for long-context prefilling. The tool offers features such as trainable sparse attention, block-level sparsity, self-distillation, efficient kernel, and easy integration with existing transformer architectures. Users can quickly start using SeerAttention for inference with AttnGate Adapter and training attention gates with self-distillation. The tool provides efficient evaluation methods and encourages contributions from the community.
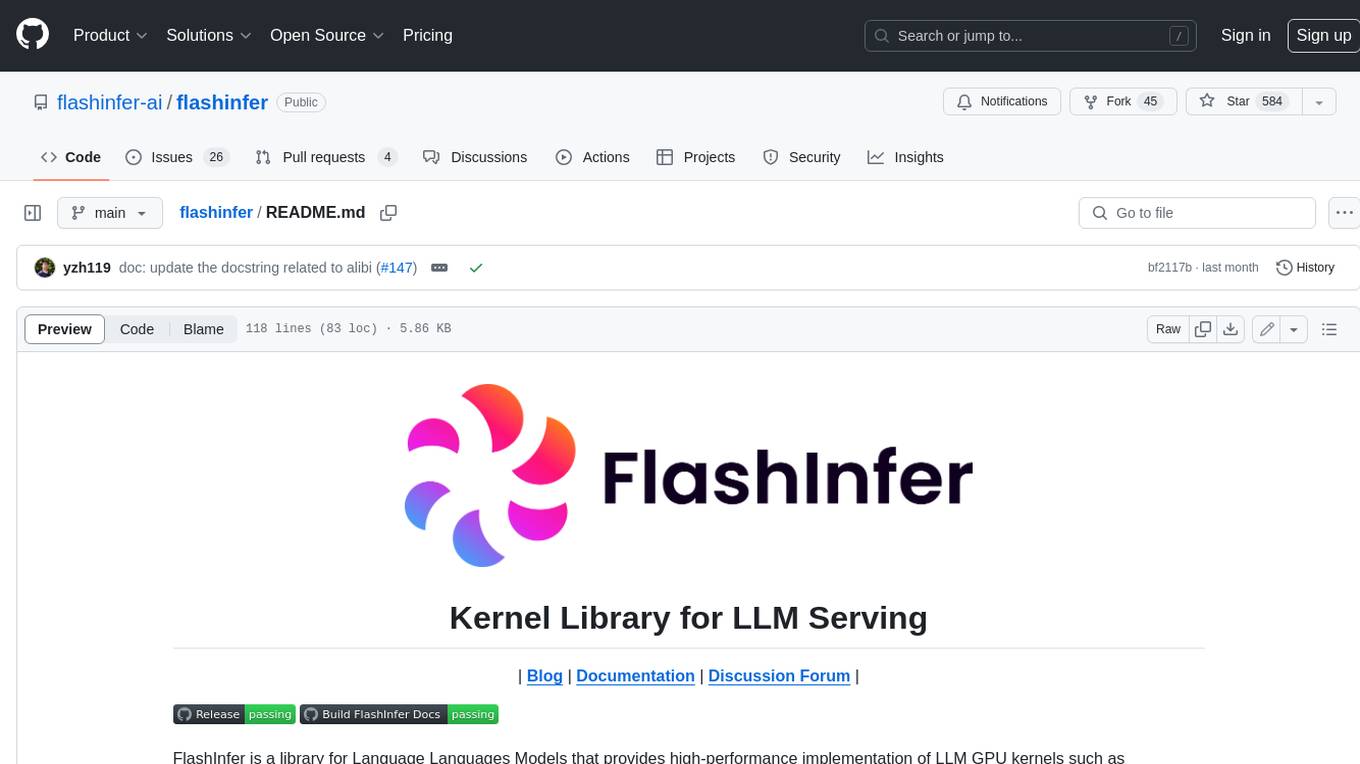
flashinfer
FlashInfer is a library for Language Languages Models that provides high-performance implementation of LLM GPU kernels such as FlashAttention, PageAttention and LoRA. FlashInfer focus on LLM serving and inference, and delivers state-the-art performance across diverse scenarios.
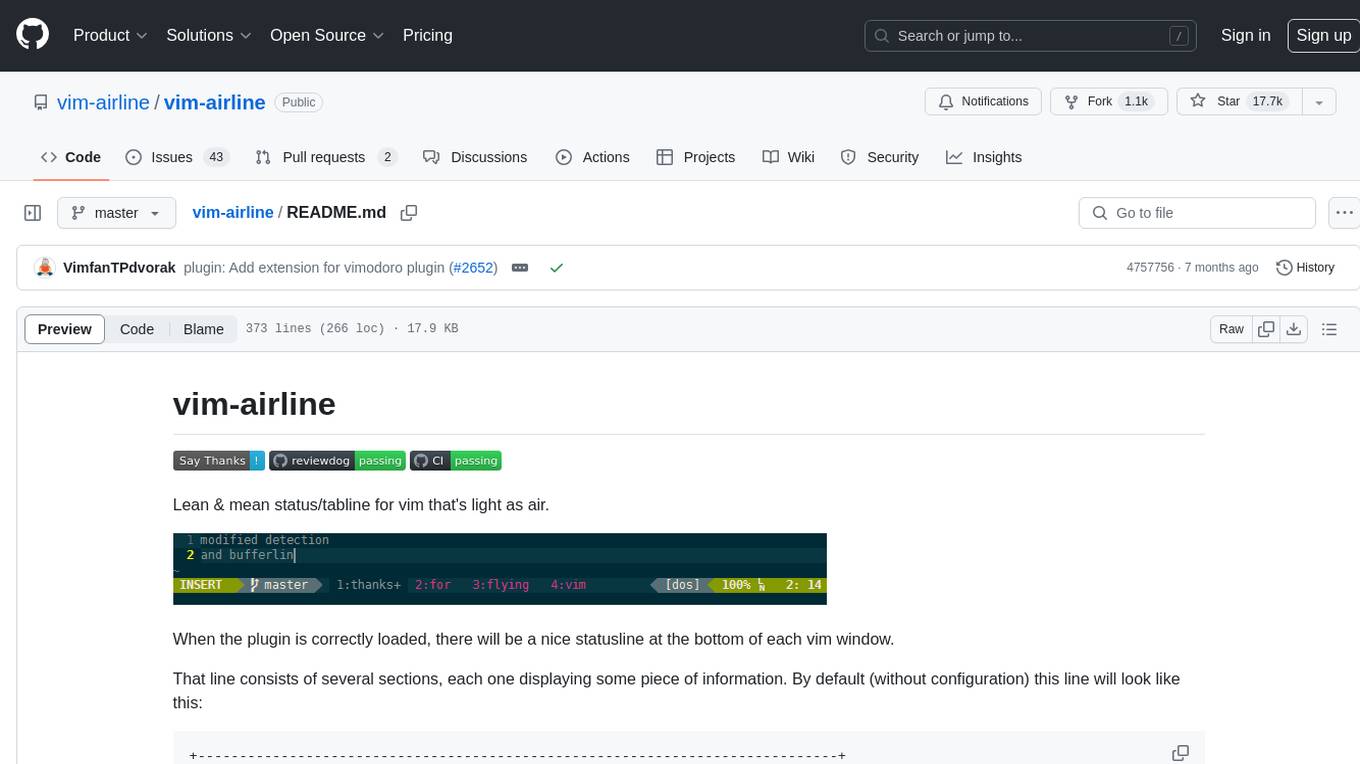
vim-airline
Vim-airline is a lean and mean status/tabline plugin for Vim that provides a nice statusline at the bottom of each Vim window. It consists of several sections displaying information such as mode, environment status, filename, filetype, file encoding, and current position in the file. The plugin is highly customizable and integrates with various plugins, providing a tiny core with extensibility in mind. It is optimized for speed, supports multiple themes, and integrates seamlessly with other plugins. Vim-airline is written in 100% Vimscript, eliminating the need for Python. The plugin aims to be stable and includes a unit testing suite for reliability.
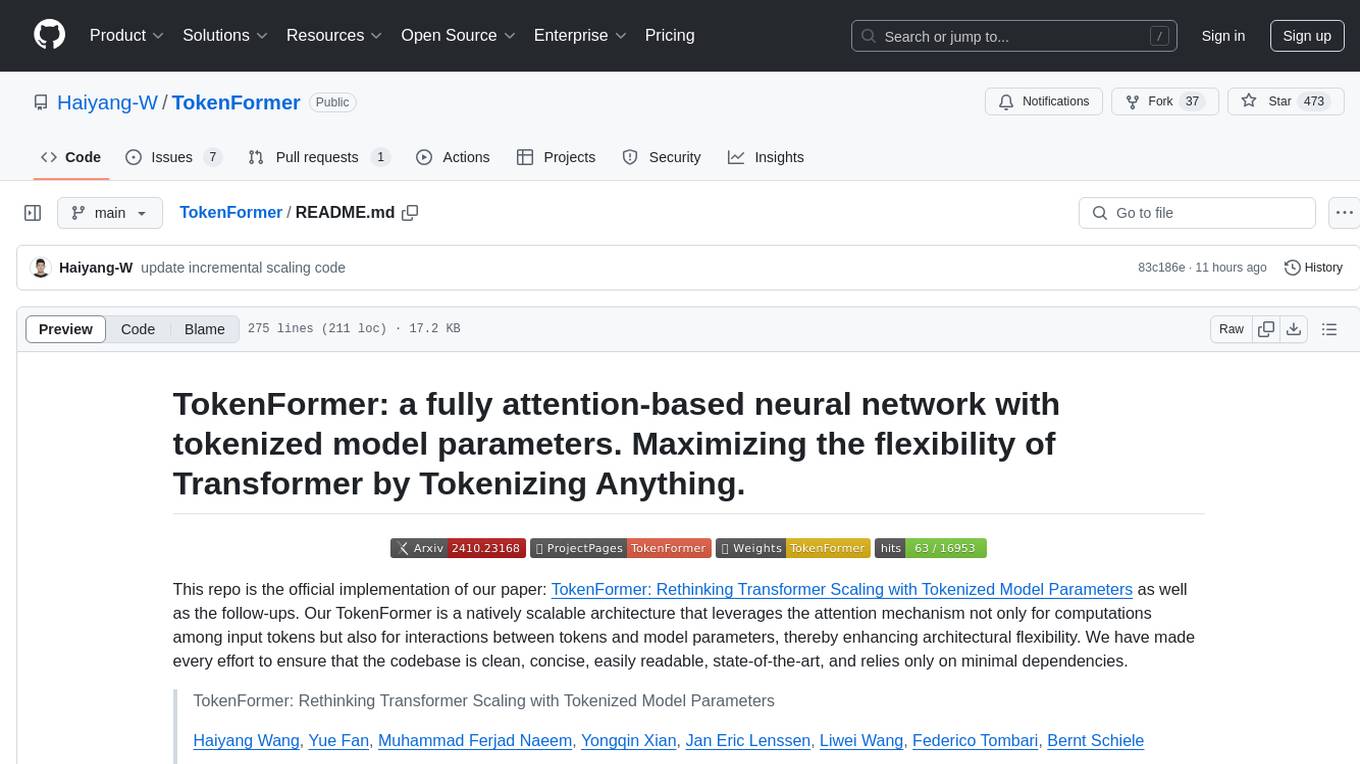
TokenFormer
TokenFormer is a fully attention-based neural network architecture that leverages tokenized model parameters to enhance architectural flexibility. It aims to maximize the flexibility of neural networks by unifying token-token and token-parameter interactions through the attention mechanism. The architecture allows for incremental model scaling and has shown promising results in language modeling and visual modeling tasks. The codebase is clean, concise, easily readable, state-of-the-art, and relies on minimal dependencies.
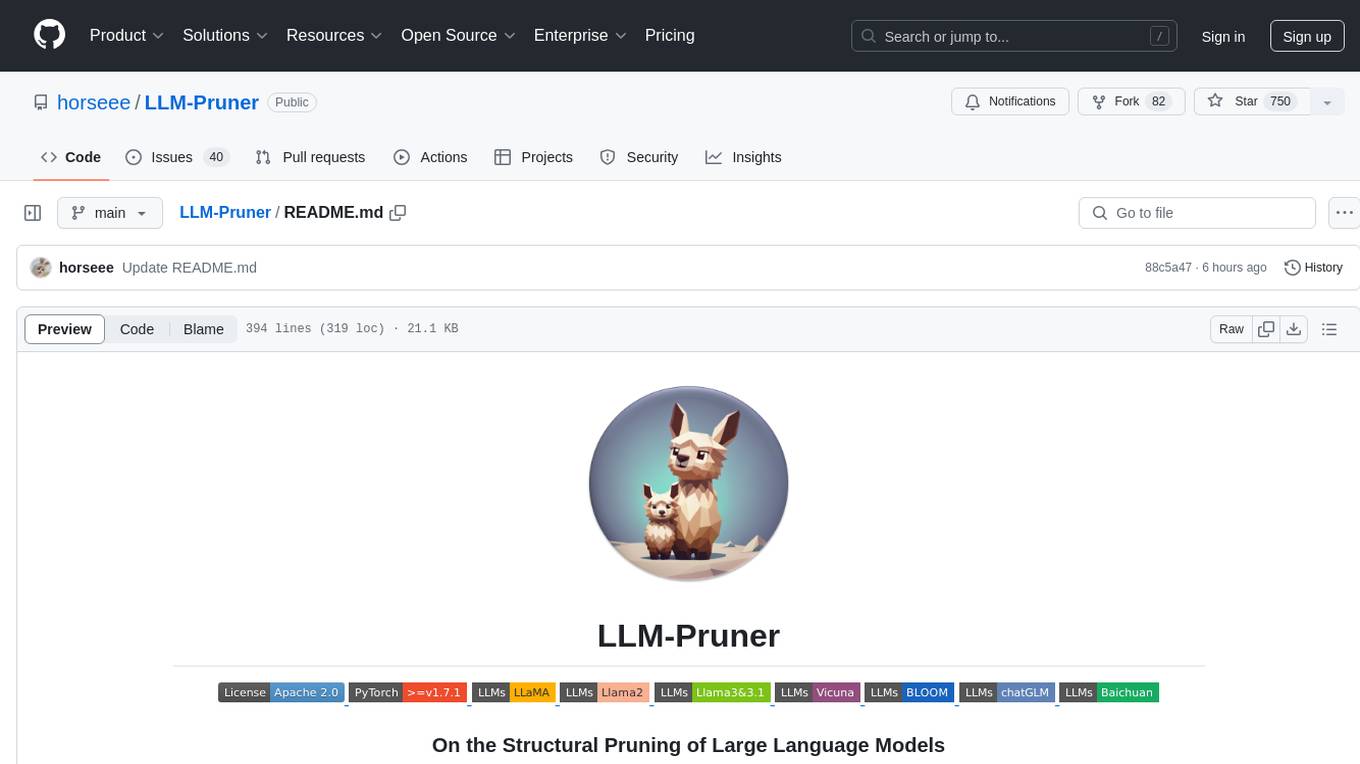
LLM-Pruner
LLM-Pruner is a tool for structural pruning of large language models, allowing task-agnostic compression while retaining multi-task solving ability. It supports automatic structural pruning of various LLMs with minimal human effort. The tool is efficient, requiring only 3 minutes for pruning and 3 hours for post-training. Supported LLMs include Llama-3.1, Llama-3, Llama-2, LLaMA, BLOOM, Vicuna, and Baichuan. Updates include support for new LLMs like GQA and BLOOM, as well as fine-tuning results achieving high accuracy. The tool provides step-by-step instructions for pruning, post-training, and evaluation, along with a Gradio interface for text generation. Limitations include issues with generating repetitive or nonsensical tokens in compressed models and manual operations for certain models.
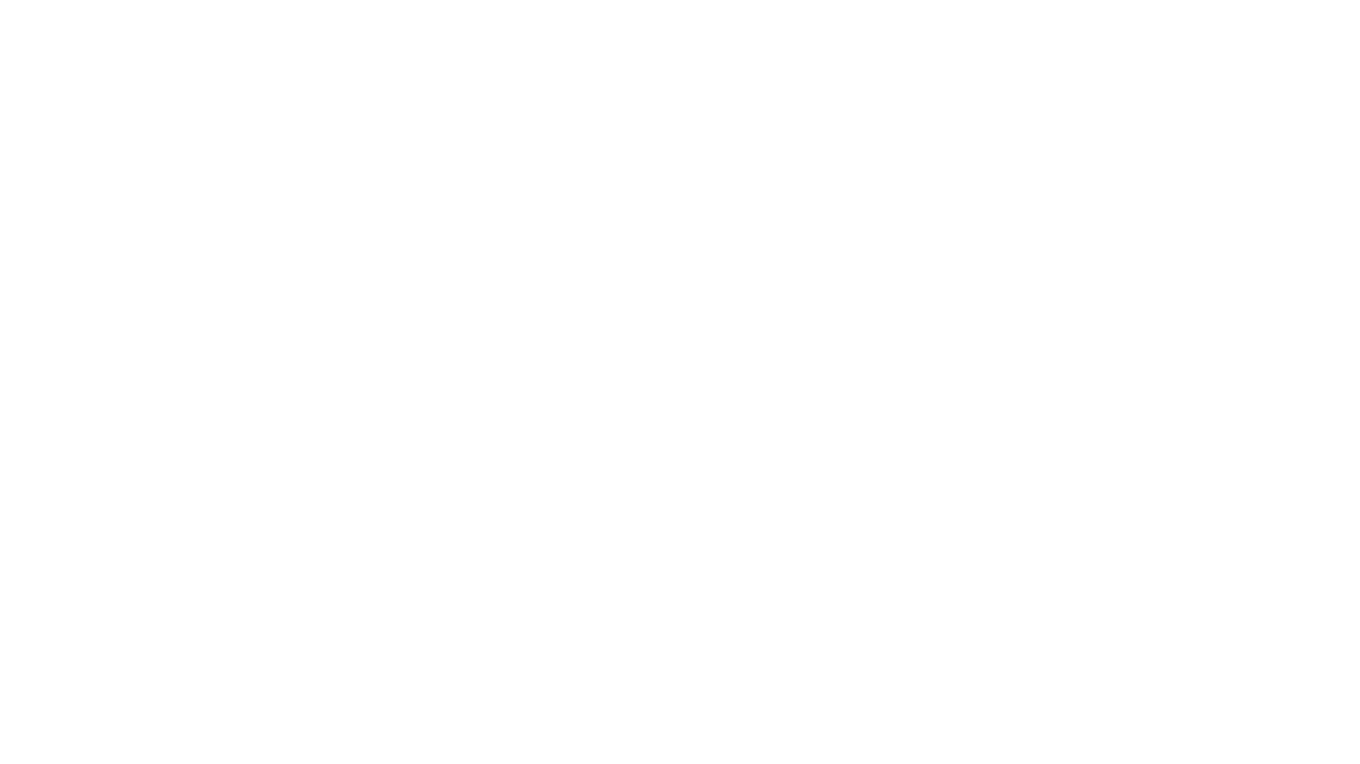
HolmesVAD
Holmes-VAD is a framework for unbiased and explainable Video Anomaly Detection using multimodal instructions. It addresses biased detection in challenging events by leveraging precise temporal supervision and rich multimodal instructions. The framework includes a largescale VAD instruction-tuning benchmark, VAD-Instruct50k, created with single-frame annotations and a robust video captioner. It offers accurate anomaly localization and comprehensive explanations through a customized solution for interpretable video anomaly detection.
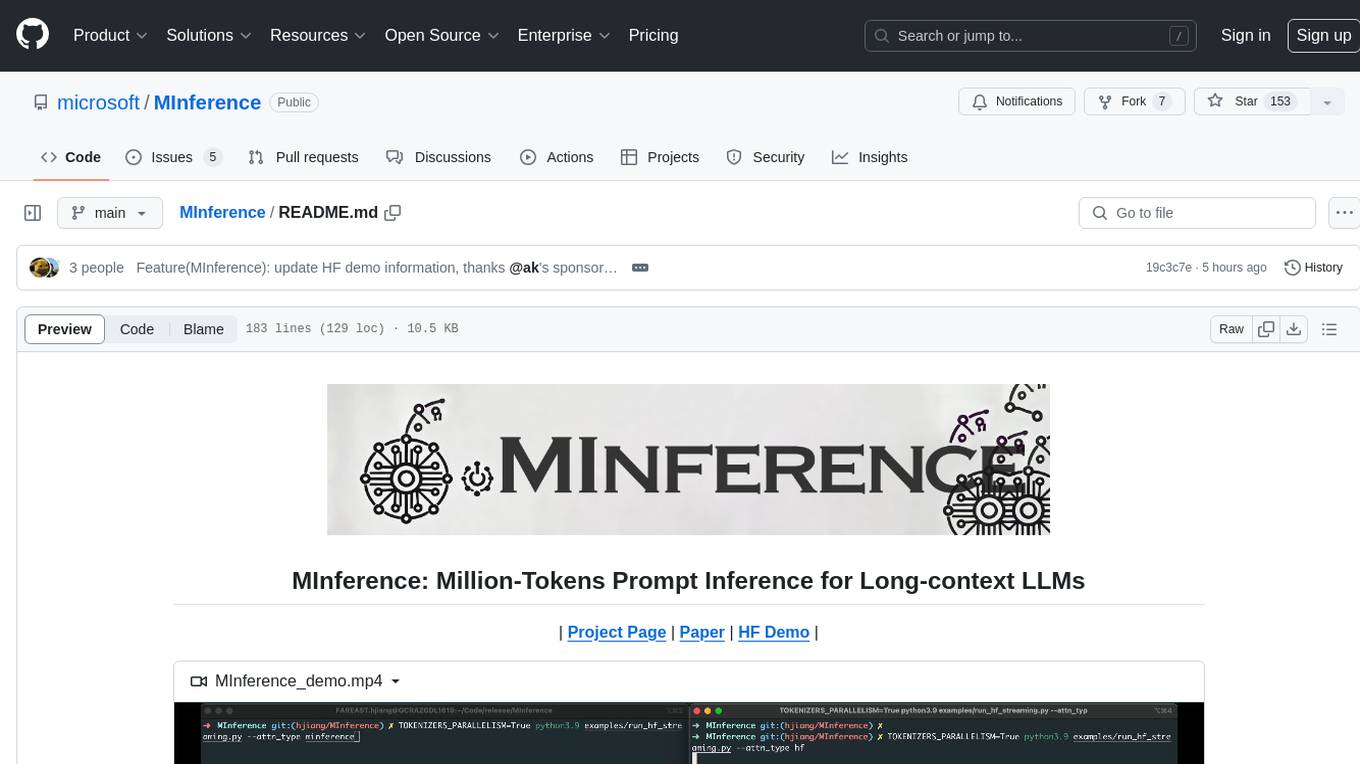
MInference
MInference is a tool designed to accelerate pre-filling for long-context Language Models (LLMs) by leveraging dynamic sparse attention. It achieves up to a 10x speedup for pre-filling on an A100 while maintaining accuracy. The tool supports various decoding LLMs, including LLaMA-style models and Phi models, and provides custom kernels for attention computation. MInference is useful for researchers and developers working with large-scale language models who aim to improve efficiency without compromising accuracy.
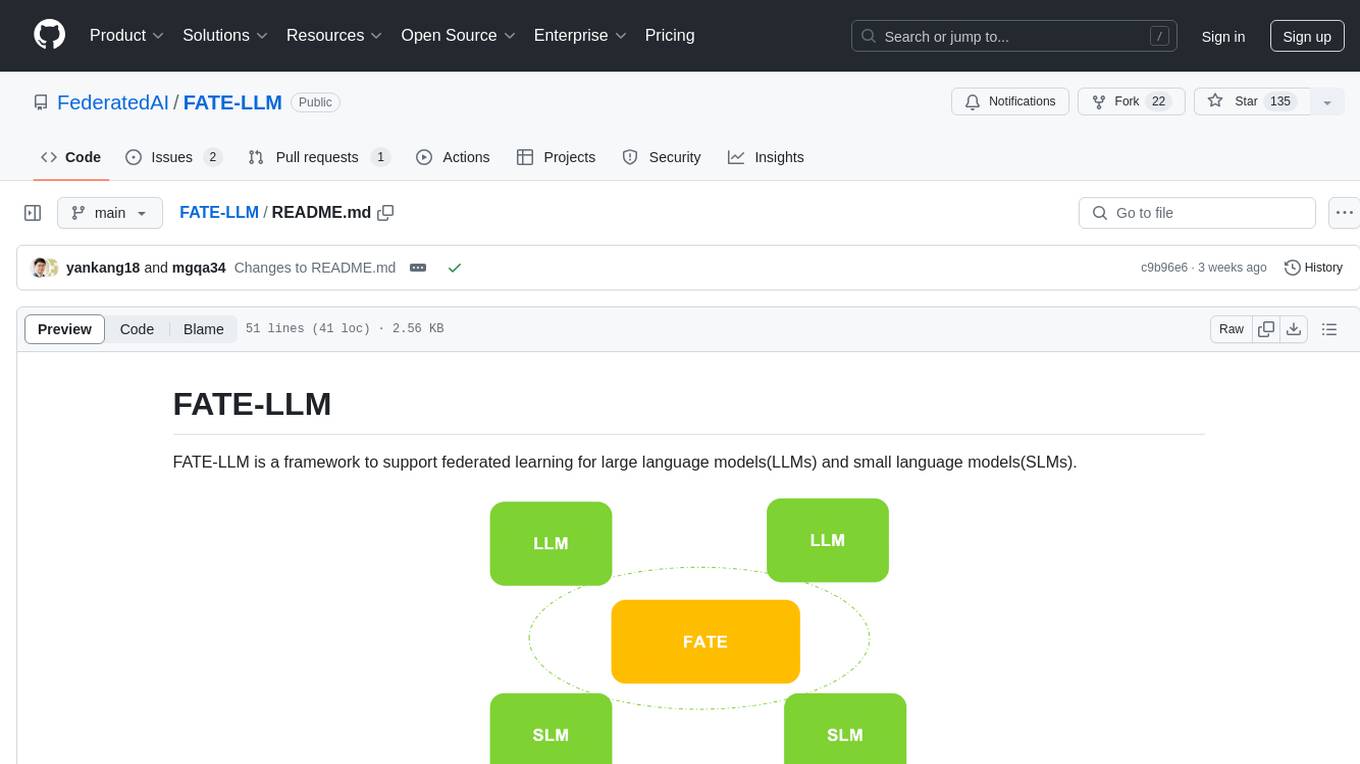
FATE-LLM
FATE-LLM is a framework supporting federated learning for large and small language models. It promotes training efficiency of federated LLMs using Parameter-Efficient methods, protects the IP of LLMs using FedIPR, and ensures data privacy during training and inference through privacy-preserving mechanisms.
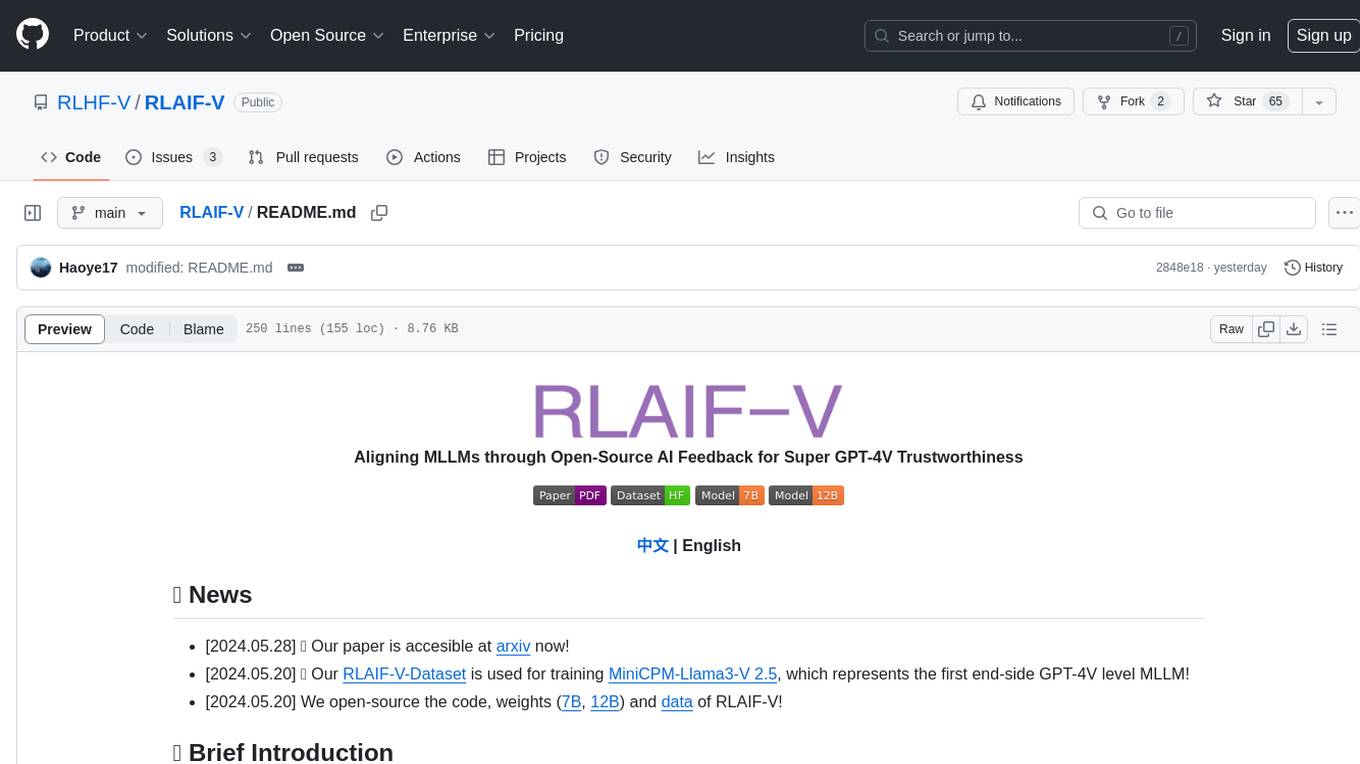
RLAIF-V
RLAIF-V is a novel framework that aligns MLLMs in a fully open-source paradigm for super GPT-4V trustworthiness. It maximally exploits open-source feedback from high-quality feedback data and online feedback learning algorithm. Notable features include achieving super GPT-4V trustworthiness in both generative and discriminative tasks, using high-quality generalizable feedback data to reduce hallucination of different MLLMs, and exhibiting better learning efficiency and higher performance through iterative alignment.
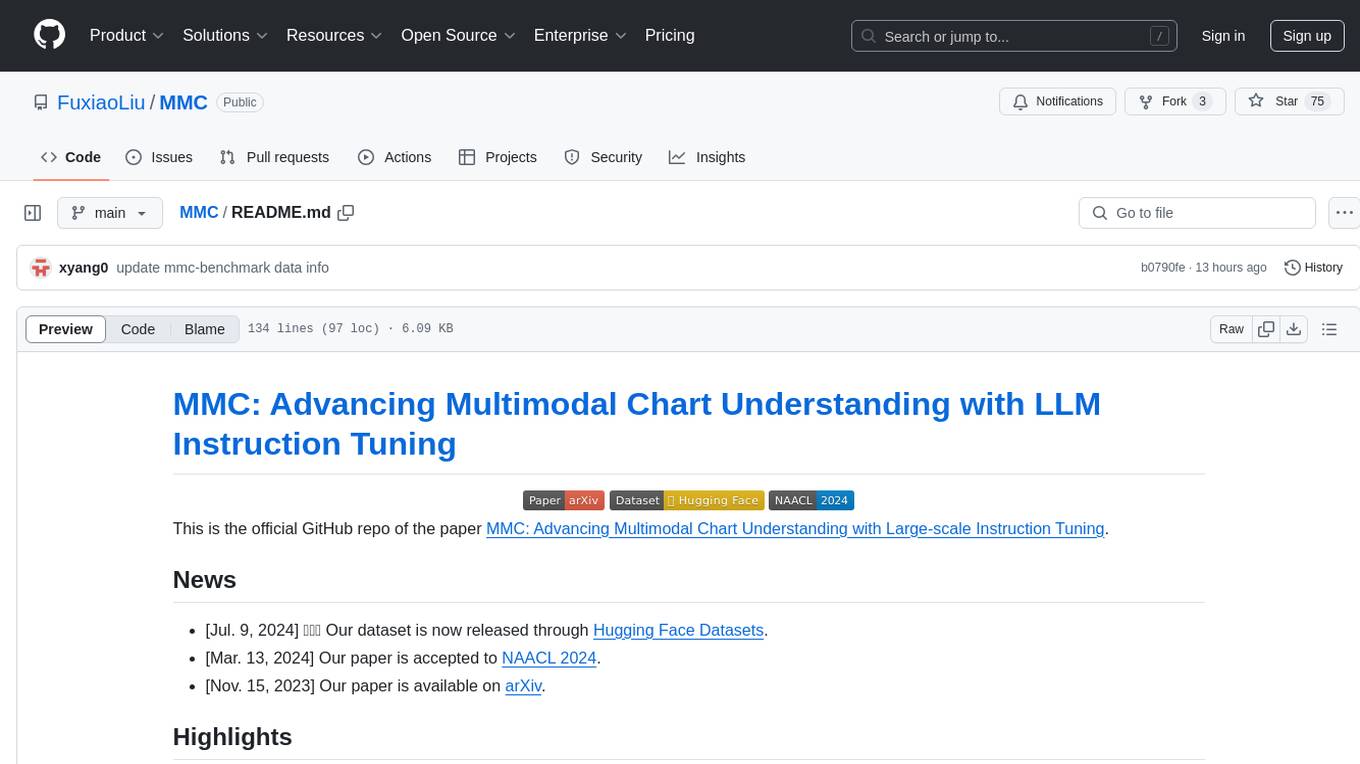
MMC
This repository, MMC, focuses on advancing multimodal chart understanding through large-scale instruction tuning. It introduces a dataset supporting various tasks and chart types, a benchmark for evaluating reasoning capabilities over charts, and an assistant achieving state-of-the-art performance on chart QA benchmarks. The repository provides data for chart-text alignment, benchmarking, and instruction tuning, along with existing datasets used in experiments. Additionally, it offers a Gradio demo for the MMCA model.
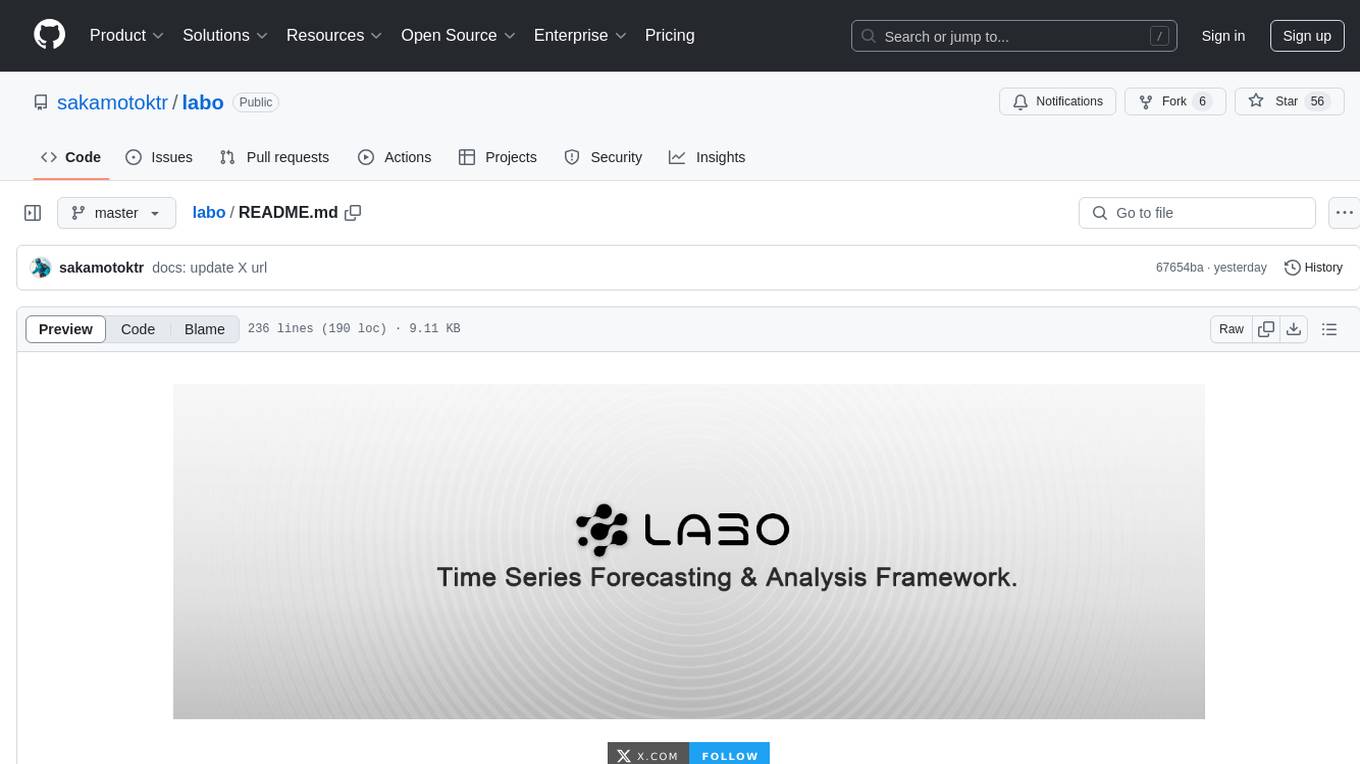
labo
LABO is a time series forecasting and analysis framework that integrates pre-trained and fine-tuned LLMs with multi-domain agent-based systems. It allows users to create and tune agents easily for various scenarios, such as stock market trend prediction and web public opinion analysis. LABO requires a specific runtime environment setup, including system requirements, Python environment, dependency installations, and configurations. Users can fine-tune their own models using LABO's Low-Rank Adaptation (LoRA) for computational efficiency and continuous model updates. Additionally, LABO provides a Python library for building model training pipelines and customizing agents for specific tasks.
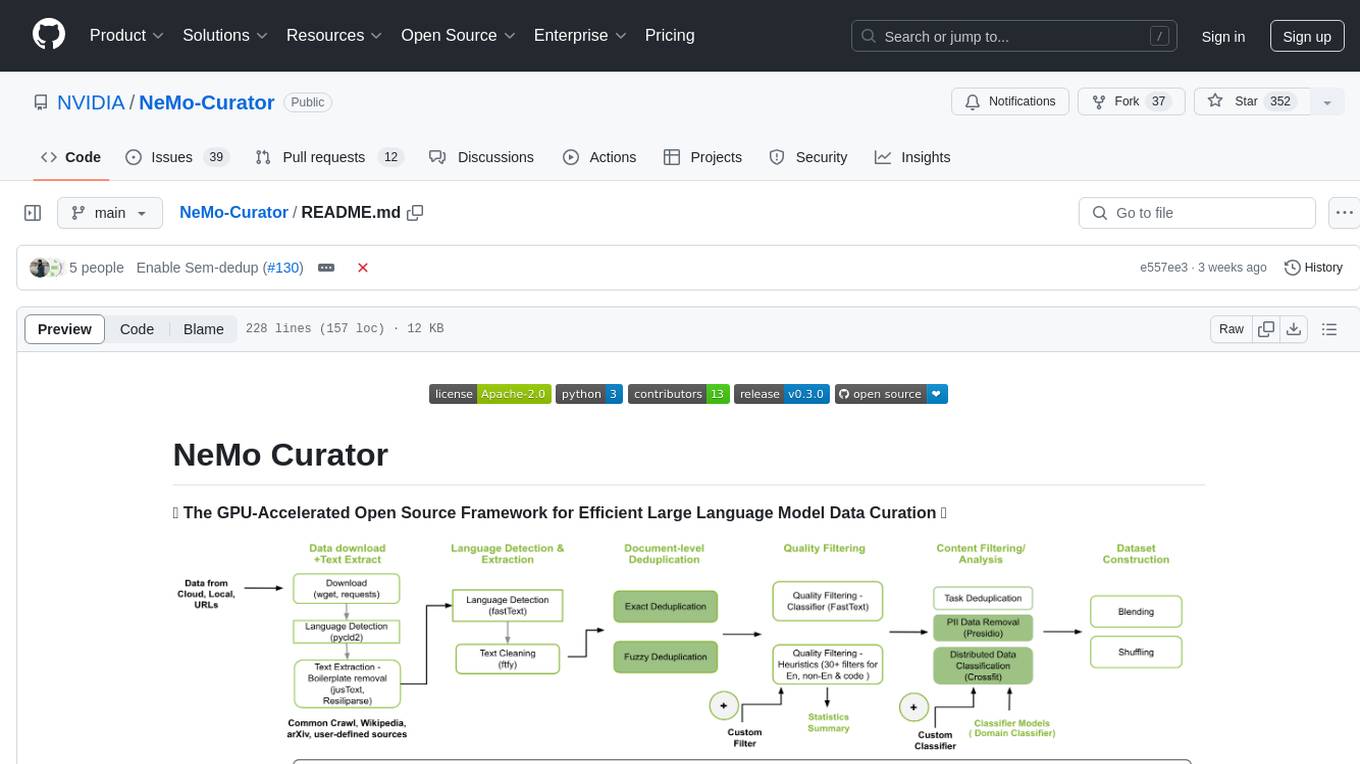
NeMo-Curator
NeMo Curator is a GPU-accelerated open-source framework designed for efficient large language model data curation. It provides scalable dataset preparation for tasks like foundation model pretraining, domain-adaptive pretraining, supervised fine-tuning, and parameter-efficient fine-tuning. The library leverages GPUs with Dask and RAPIDS to accelerate data curation, offering customizable and modular interfaces for pipeline expansion and model convergence. Key features include data download, text extraction, quality filtering, deduplication, downstream-task decontamination, distributed data classification, and PII redaction. NeMo Curator is suitable for curating high-quality datasets for large language model training.
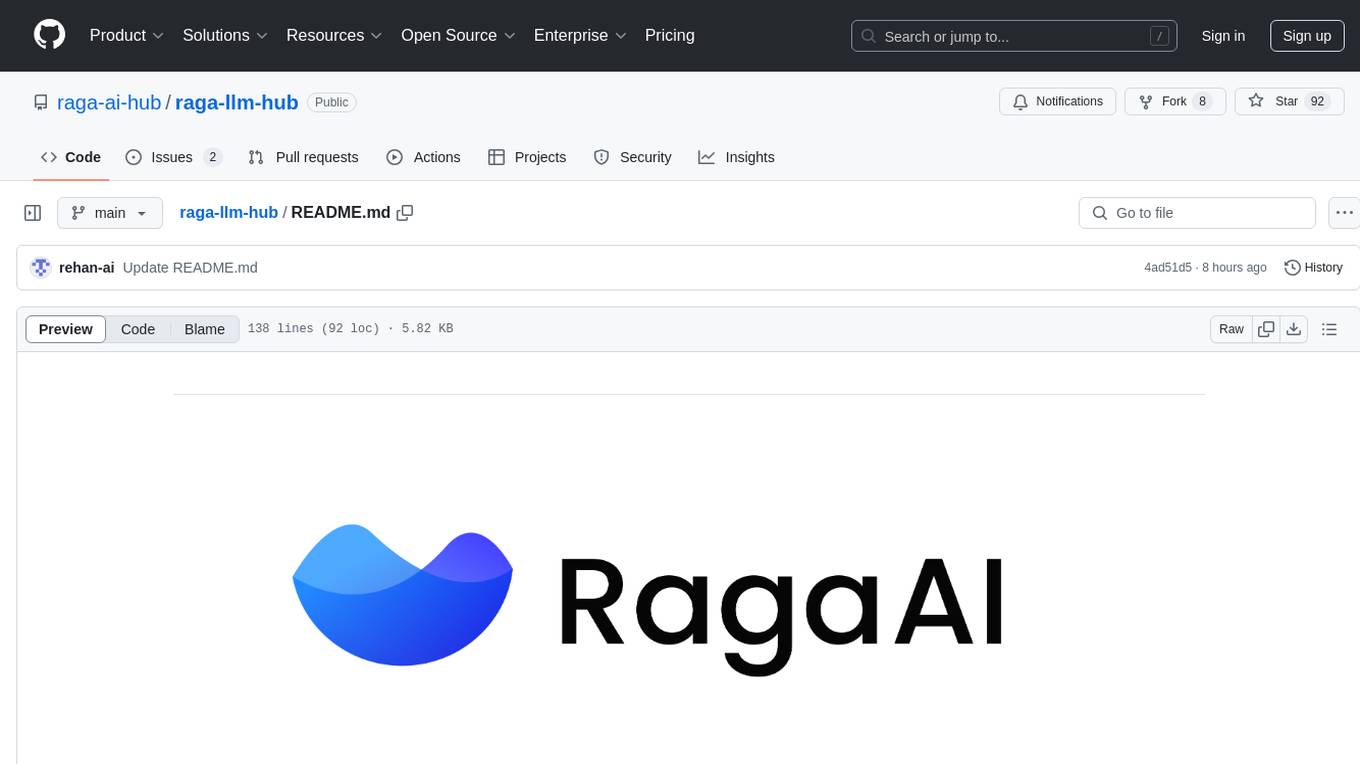
raga-llm-hub
Raga LLM Hub is a comprehensive evaluation toolkit for Language and Learning Models (LLMs) with over 100 meticulously designed metrics. It allows developers and organizations to evaluate and compare LLMs effectively, establishing guardrails for LLMs and Retrieval Augmented Generation (RAG) applications. The platform assesses aspects like Relevance & Understanding, Content Quality, Hallucination, Safety & Bias, Context Relevance, Guardrails, and Vulnerability scanning, along with Metric-Based Tests for quantitative analysis. It helps teams identify and fix issues throughout the LLM lifecycle, revolutionizing reliability and trustworthiness.
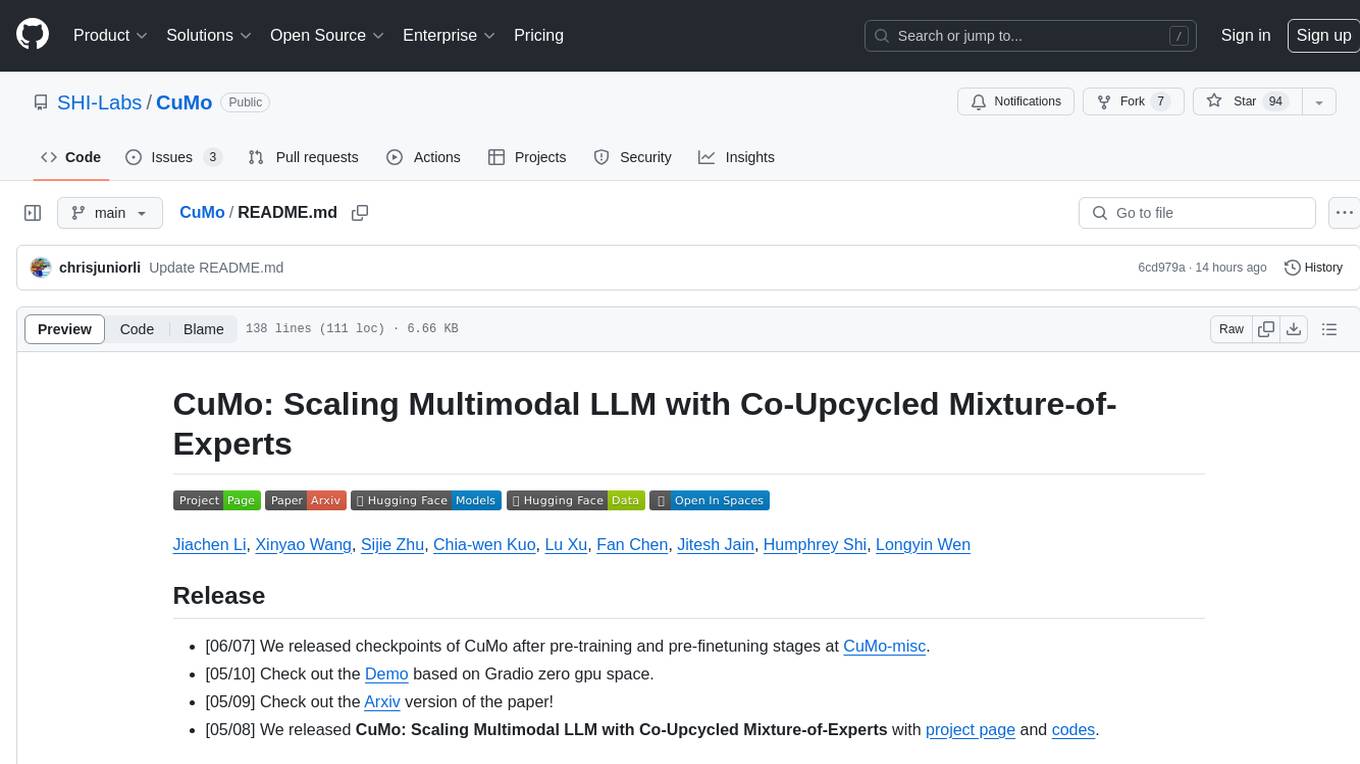
CuMo
CuMo is a project focused on scaling multimodal Large Language Models (LLMs) with Co-Upcycled Mixture-of-Experts. It introduces CuMo, which incorporates Co-upcycled Top-K sparsely-gated Mixture-of-experts blocks into the vision encoder and the MLP connector, enhancing the capabilities of multimodal LLMs. The project adopts a three-stage training approach with auxiliary losses to stabilize the training process and maintain a balanced loading of experts. CuMo achieves comparable performance to other state-of-the-art multimodal LLMs on various Visual Question Answering (VQA) and visual-instruction-following benchmarks.
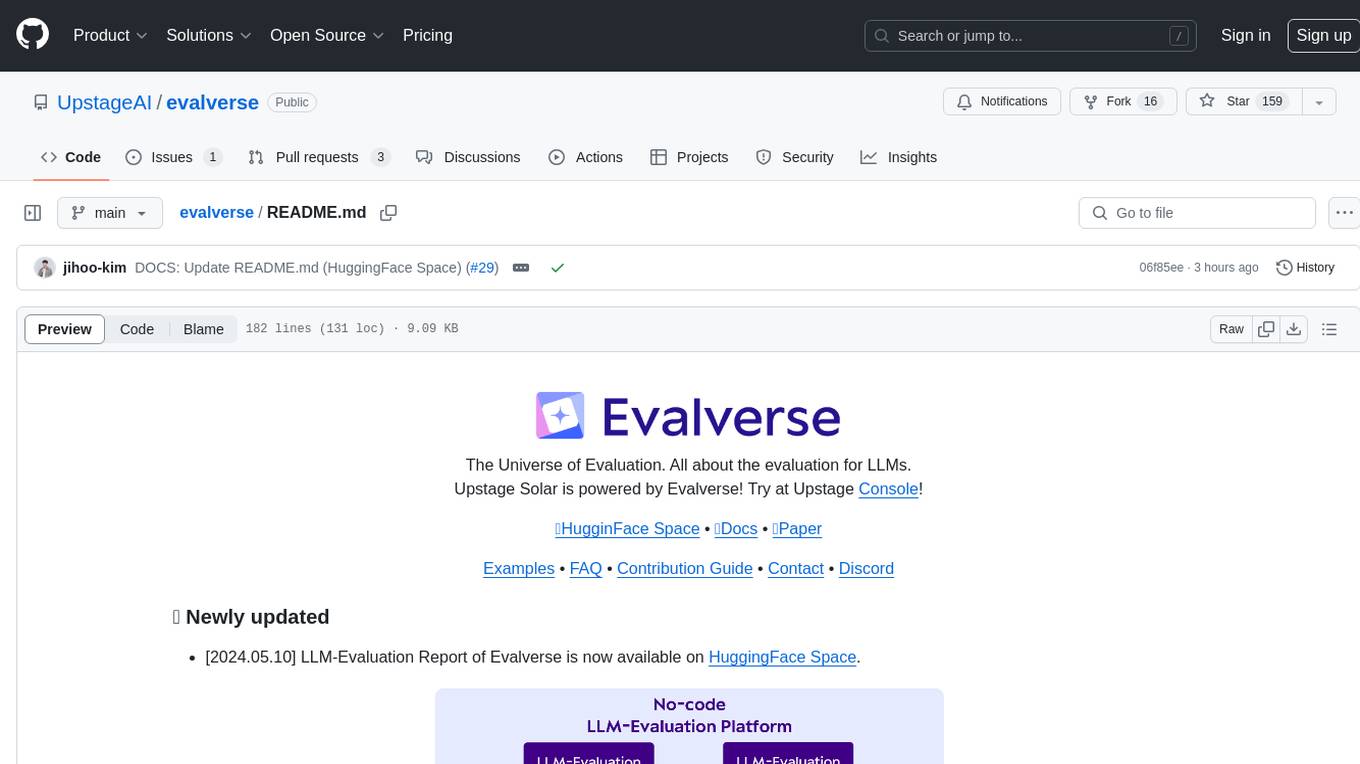
evalverse
Evalverse is an open-source project designed to support Large Language Model (LLM) evaluation needs. It provides a standardized and user-friendly solution for processing and managing LLM evaluations, catering to AI research engineers and scientists. Evalverse supports various evaluation methods, insightful reports, and no-code evaluation processes. Users can access unified evaluation with submodules, request evaluations without code via Slack bot, and obtain comprehensive reports with scores, rankings, and visuals. The tool allows for easy comparison of scores across different models and swift addition of new evaluation tools.
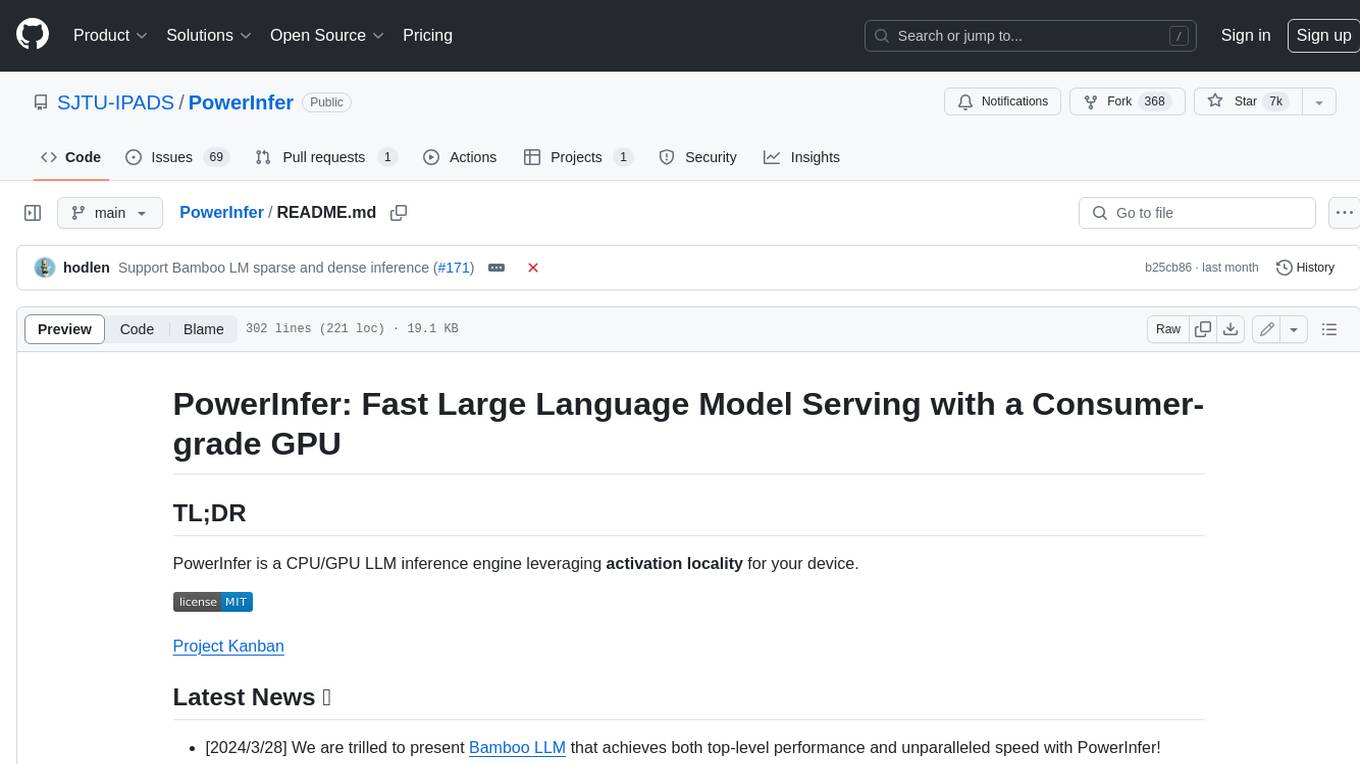
PowerInfer
PowerInfer is a high-speed Large Language Model (LLM) inference engine designed for local deployment on consumer-grade hardware, leveraging activation locality to optimize efficiency. It features a locality-centric design, hybrid CPU/GPU utilization, easy integration with popular ReLU-sparse models, and support for various platforms. PowerInfer achieves high speed with lower resource demands and is flexible for easy deployment and compatibility with existing models like Falcon-40B, Llama2 family, ProSparse Llama2 family, and Bamboo-7B.
For similar tasks
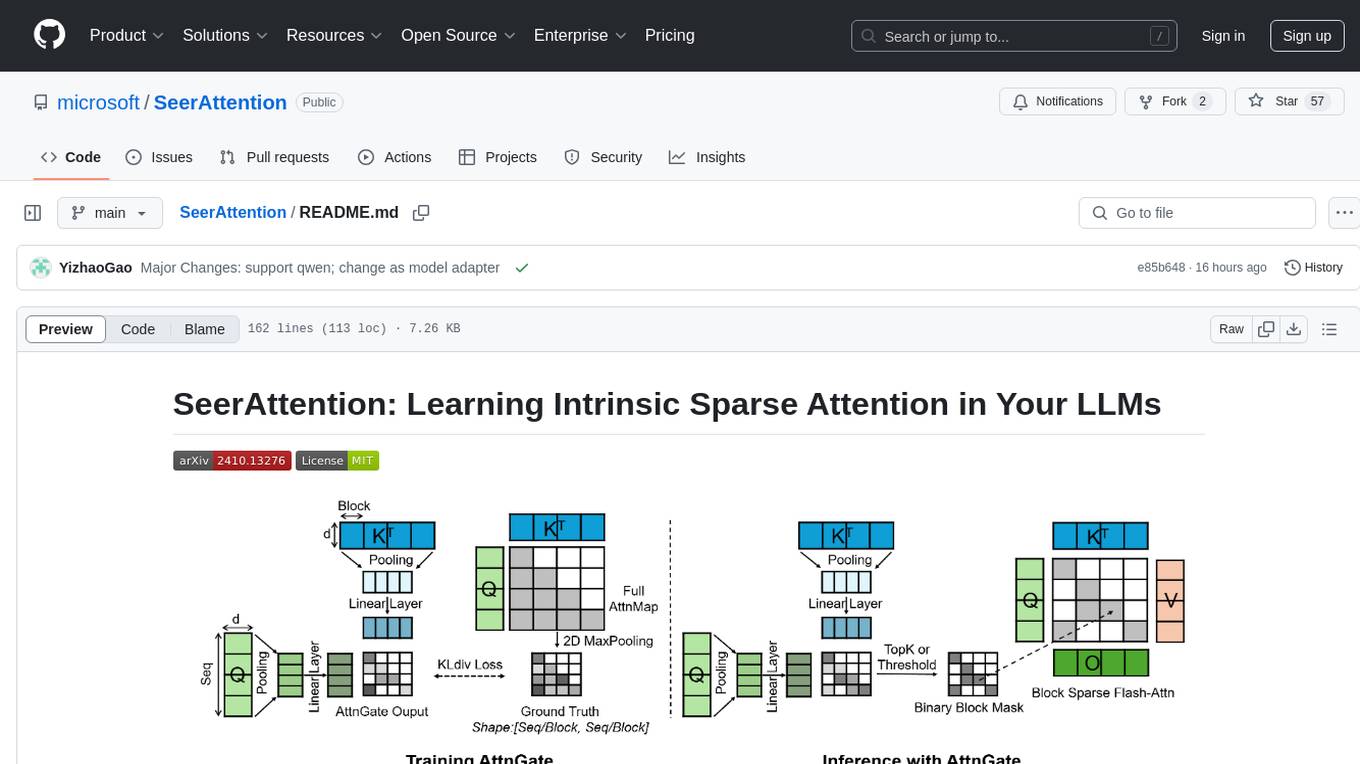
SeerAttention
SeerAttention is a novel trainable sparse attention mechanism that learns intrinsic sparsity patterns directly from LLMs through self-distillation at post-training time. It achieves faster inference while maintaining accuracy for long-context prefilling. The tool offers features such as trainable sparse attention, block-level sparsity, self-distillation, efficient kernel, and easy integration with existing transformer architectures. Users can quickly start using SeerAttention for inference with AttnGate Adapter and training attention gates with self-distillation. The tool provides efficient evaluation methods and encourages contributions from the community.
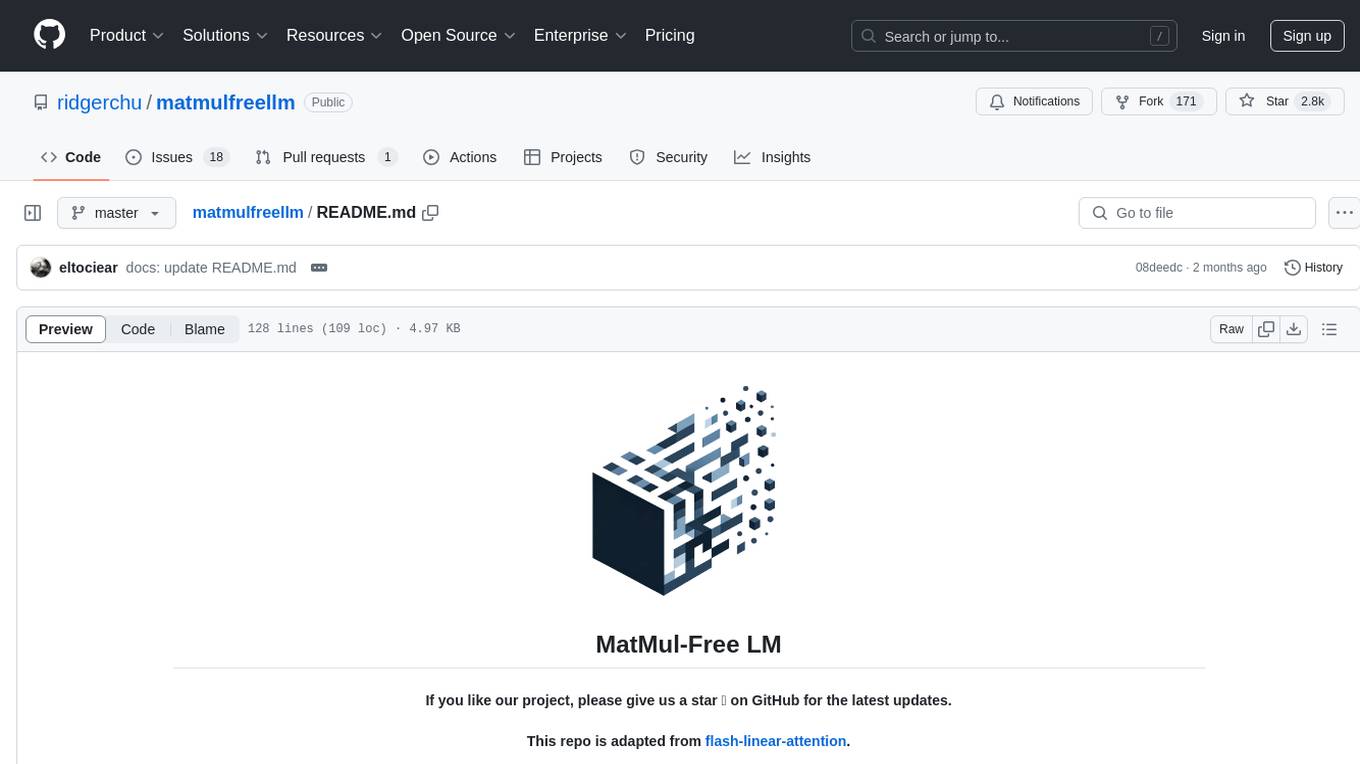
matmulfreellm
MatMul-Free LM is a language model architecture that eliminates the need for Matrix Multiplication (MatMul) operations. This repository provides an implementation of MatMul-Free LM that is compatible with the 🤗 Transformers library. It evaluates how the scaling law fits to different parameter models and compares the efficiency of the architecture in leveraging additional compute to improve performance. The repo includes pre-trained models, model implementations compatible with 🤗 Transformers library, and generation examples for text using the 🤗 text generation APIs.
For similar jobs
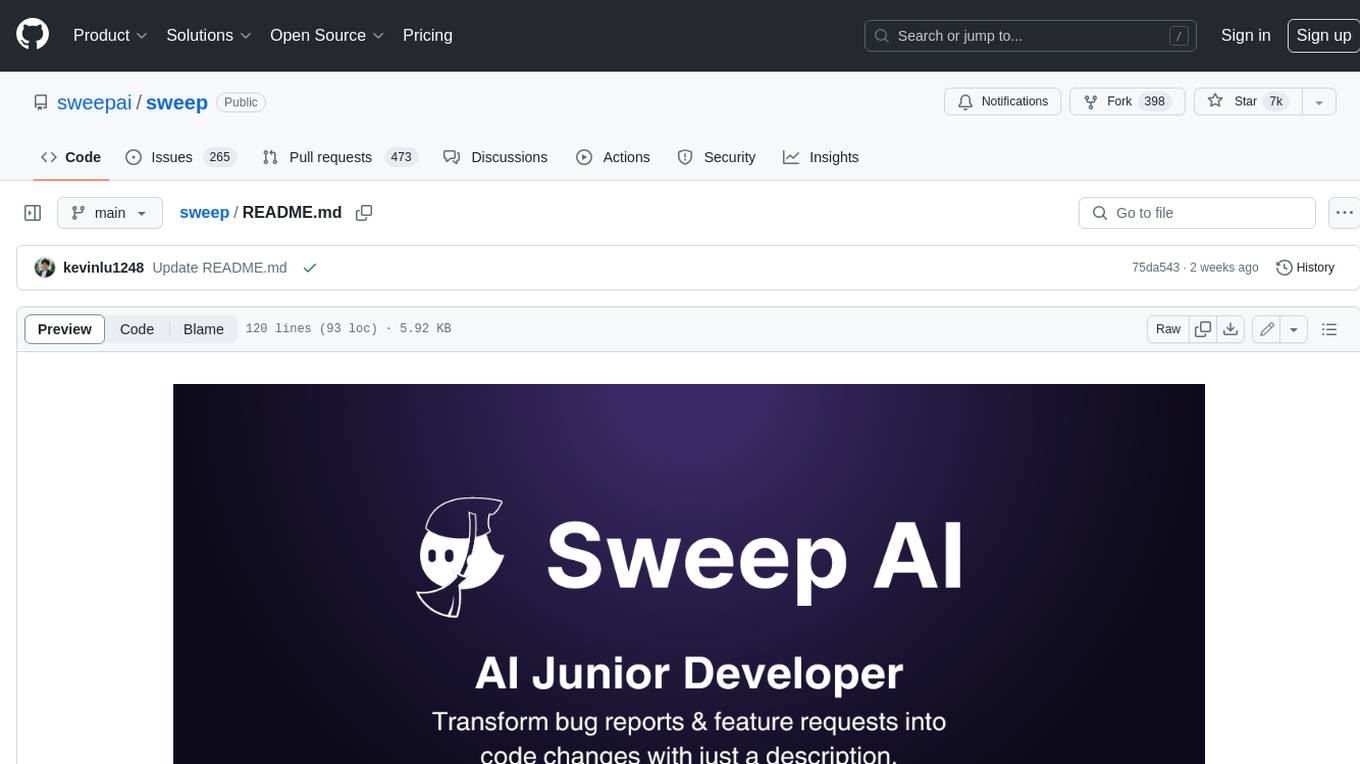
sweep
Sweep is an AI junior developer that turns bugs and feature requests into code changes. It automatically handles developer experience improvements like adding type hints and improving test coverage.
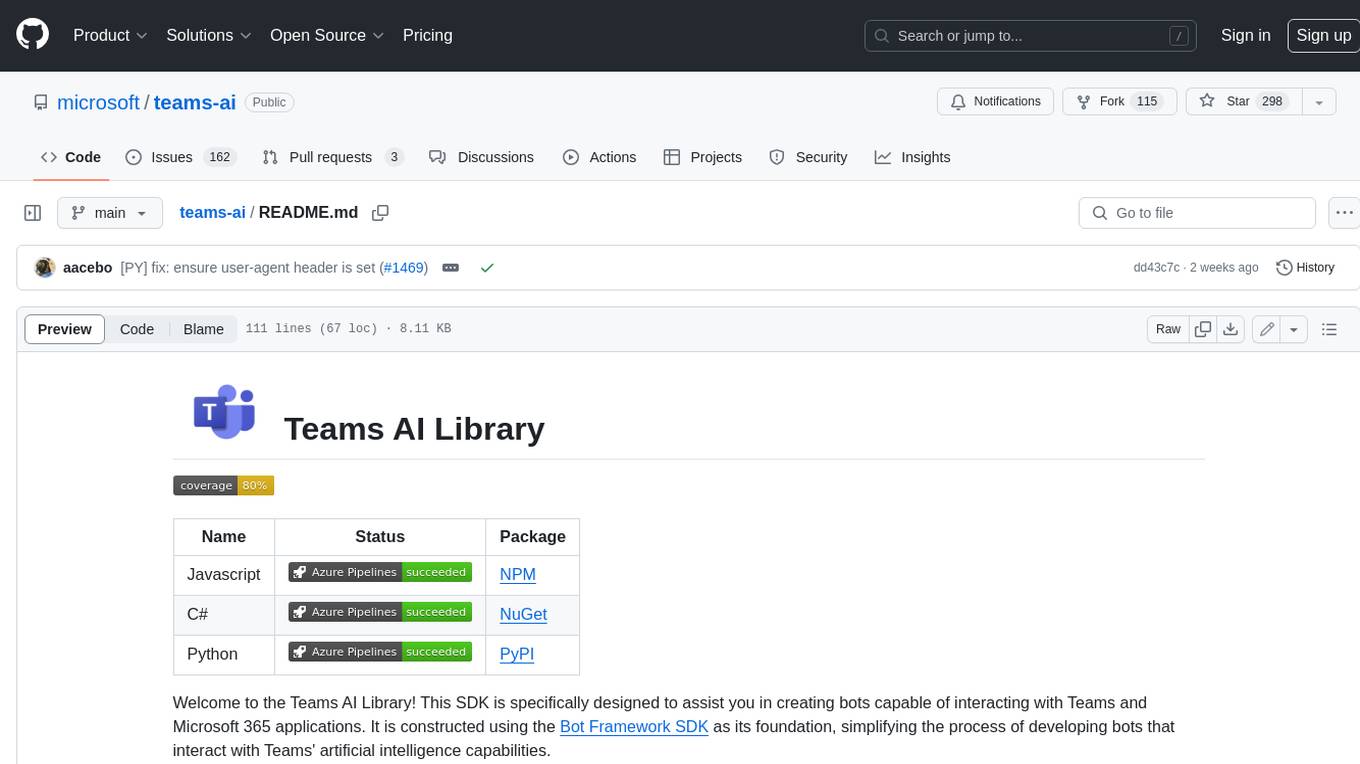
teams-ai
The Teams AI Library is a software development kit (SDK) that helps developers create bots that can interact with Teams and Microsoft 365 applications. It is built on top of the Bot Framework SDK and simplifies the process of developing bots that interact with Teams' artificial intelligence capabilities. The SDK is available for JavaScript/TypeScript, .NET, and Python.
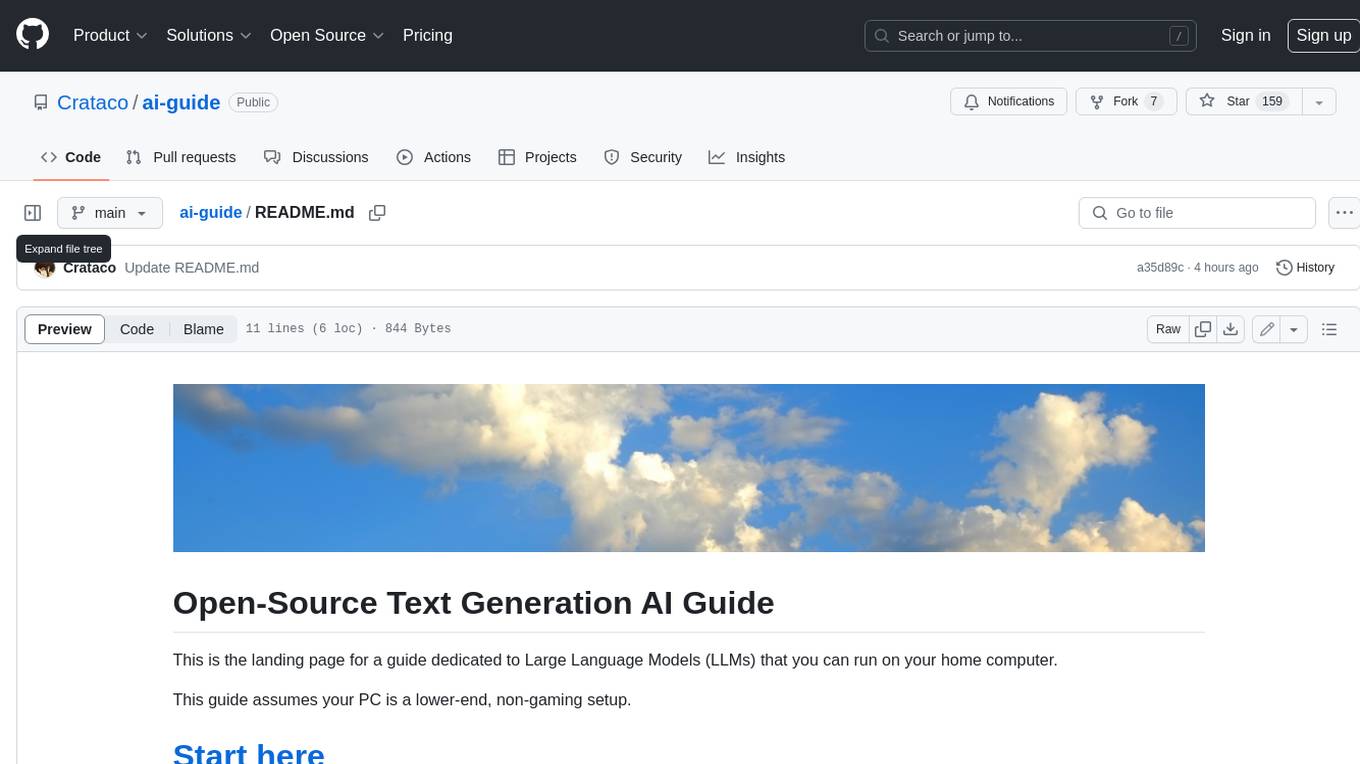
ai-guide
This guide is dedicated to Large Language Models (LLMs) that you can run on your home computer. It assumes your PC is a lower-end, non-gaming setup.
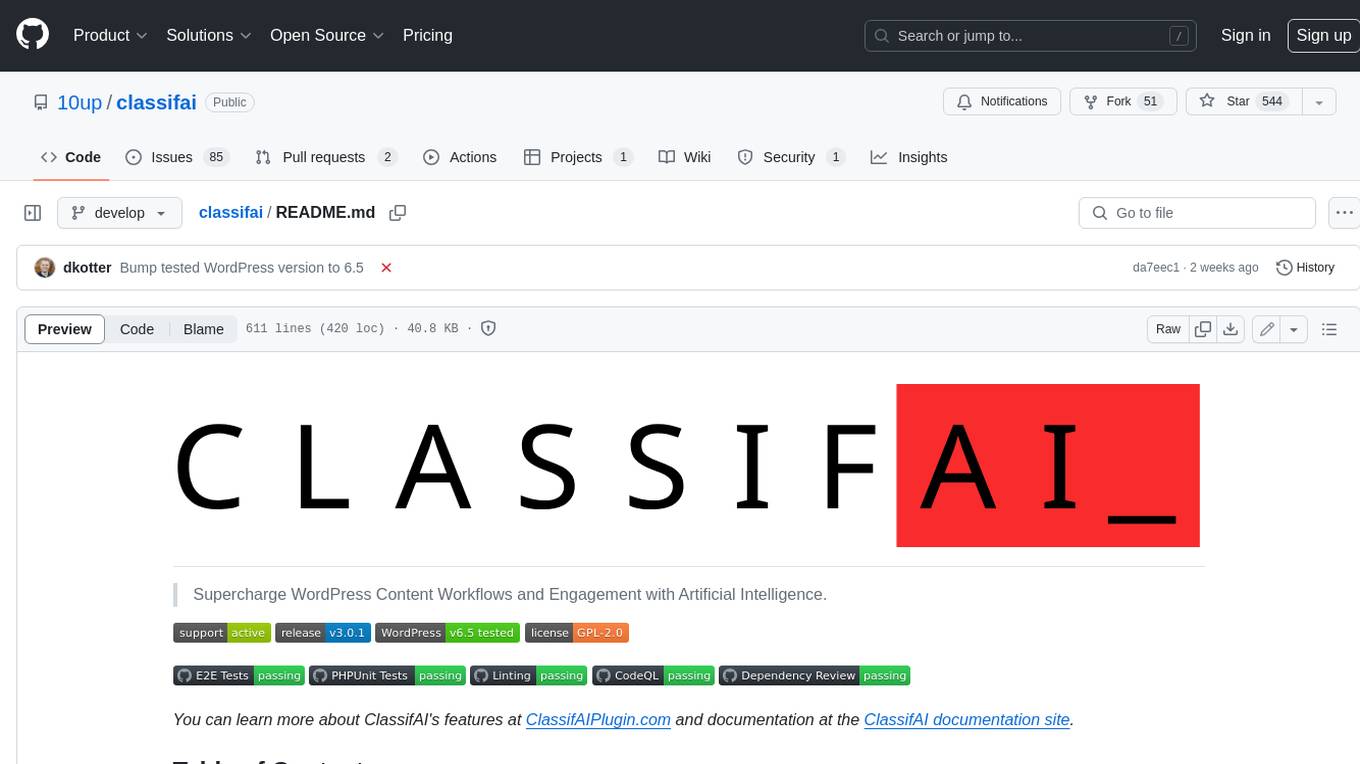
classifai
Supercharge WordPress Content Workflows and Engagement with Artificial Intelligence. Tap into leading cloud-based services like OpenAI, Microsoft Azure AI, Google Gemini and IBM Watson to augment your WordPress-powered websites. Publish content faster while improving SEO performance and increasing audience engagement. ClassifAI integrates Artificial Intelligence and Machine Learning technologies to lighten your workload and eliminate tedious tasks, giving you more time to create original content that matters.
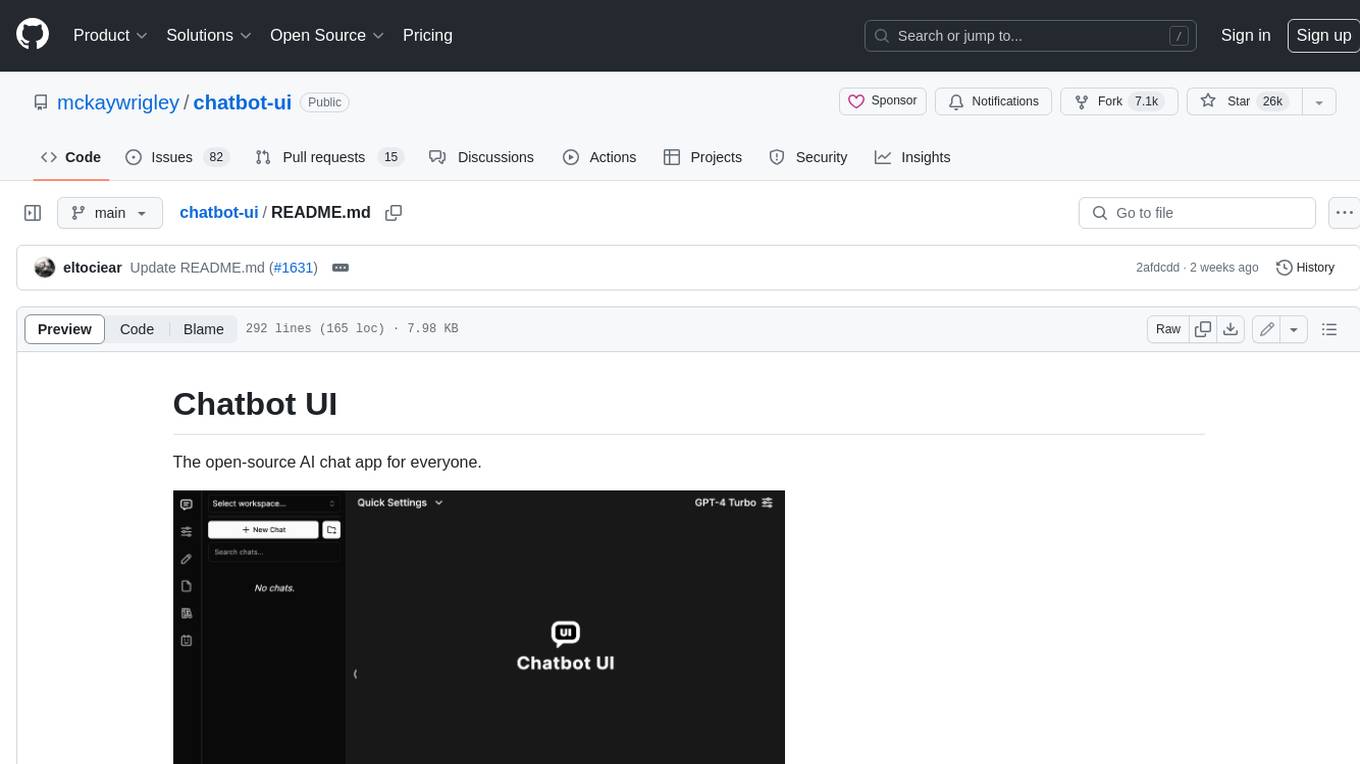
chatbot-ui
Chatbot UI is an open-source AI chat app that allows users to create and deploy their own AI chatbots. It is easy to use and can be customized to fit any need. Chatbot UI is perfect for businesses, developers, and anyone who wants to create a chatbot.
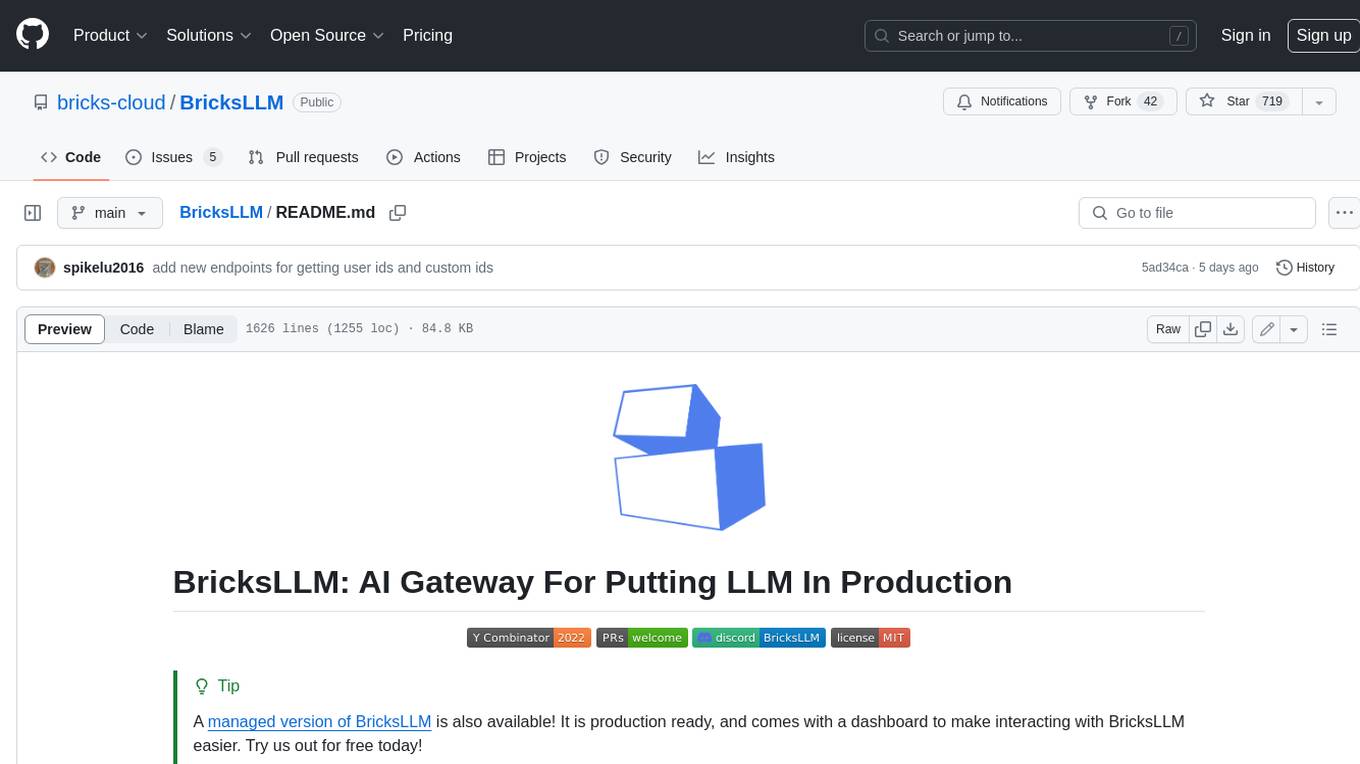
BricksLLM
BricksLLM is a cloud native AI gateway written in Go. Currently, it provides native support for OpenAI, Anthropic, Azure OpenAI and vLLM. BricksLLM aims to provide enterprise level infrastructure that can power any LLM production use cases. Here are some use cases for BricksLLM: * Set LLM usage limits for users on different pricing tiers * Track LLM usage on a per user and per organization basis * Block or redact requests containing PIIs * Improve LLM reliability with failovers, retries and caching * Distribute API keys with rate limits and cost limits for internal development/production use cases * Distribute API keys with rate limits and cost limits for students
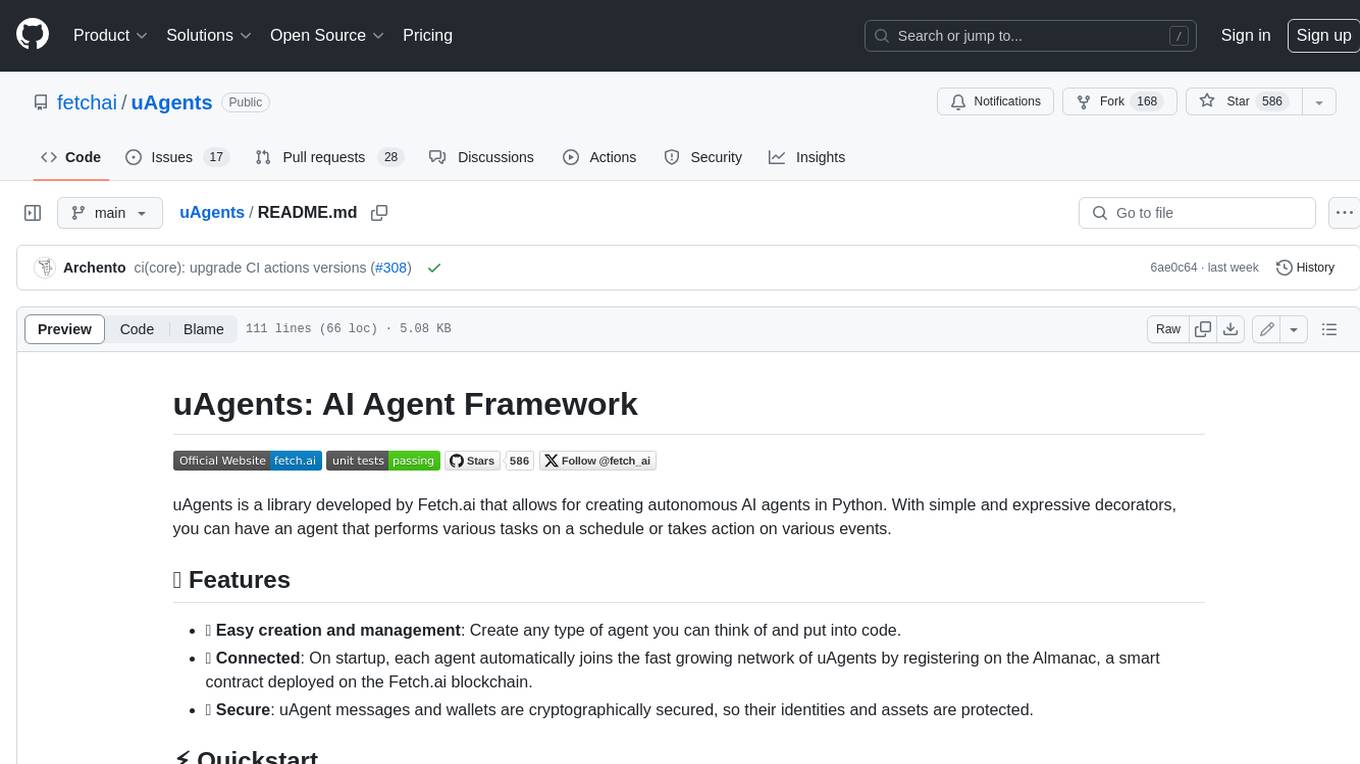
uAgents
uAgents is a Python library developed by Fetch.ai that allows for the creation of autonomous AI agents. These agents can perform various tasks on a schedule or take action on various events. uAgents are easy to create and manage, and they are connected to a fast-growing network of other uAgents. They are also secure, with cryptographically secured messages and wallets.
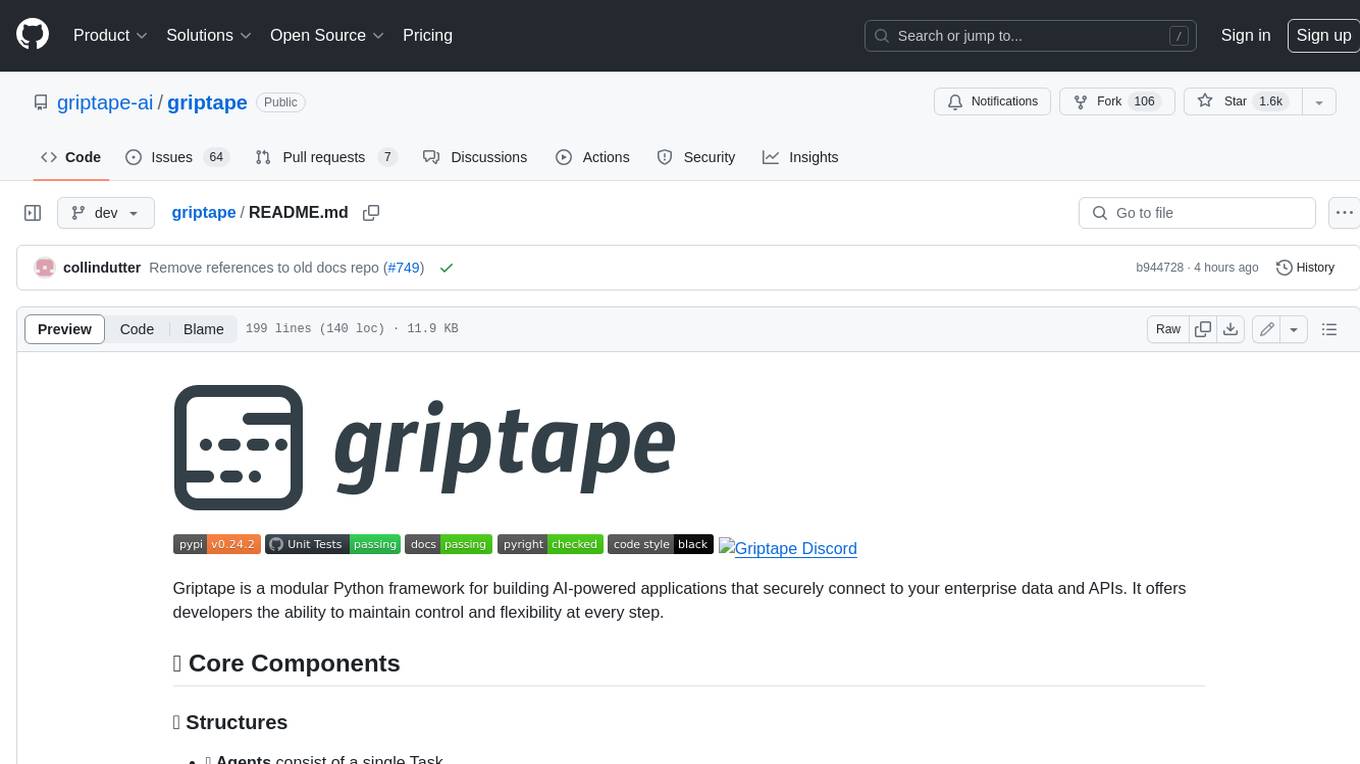
griptape
Griptape is a modular Python framework for building AI-powered applications that securely connect to your enterprise data and APIs. It offers developers the ability to maintain control and flexibility at every step. Griptape's core components include Structures (Agents, Pipelines, and Workflows), Tasks, Tools, Memory (Conversation Memory, Task Memory, and Meta Memory), Drivers (Prompt and Embedding Drivers, Vector Store Drivers, Image Generation Drivers, Image Query Drivers, SQL Drivers, Web Scraper Drivers, and Conversation Memory Drivers), Engines (Query Engines, Extraction Engines, Summary Engines, Image Generation Engines, and Image Query Engines), and additional components (Rulesets, Loaders, Artifacts, Chunkers, and Tokenizers). Griptape enables developers to create AI-powered applications with ease and efficiency.