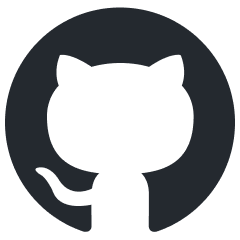
ztncui-aio
Licensed Under AGPL v3
Stars: 166
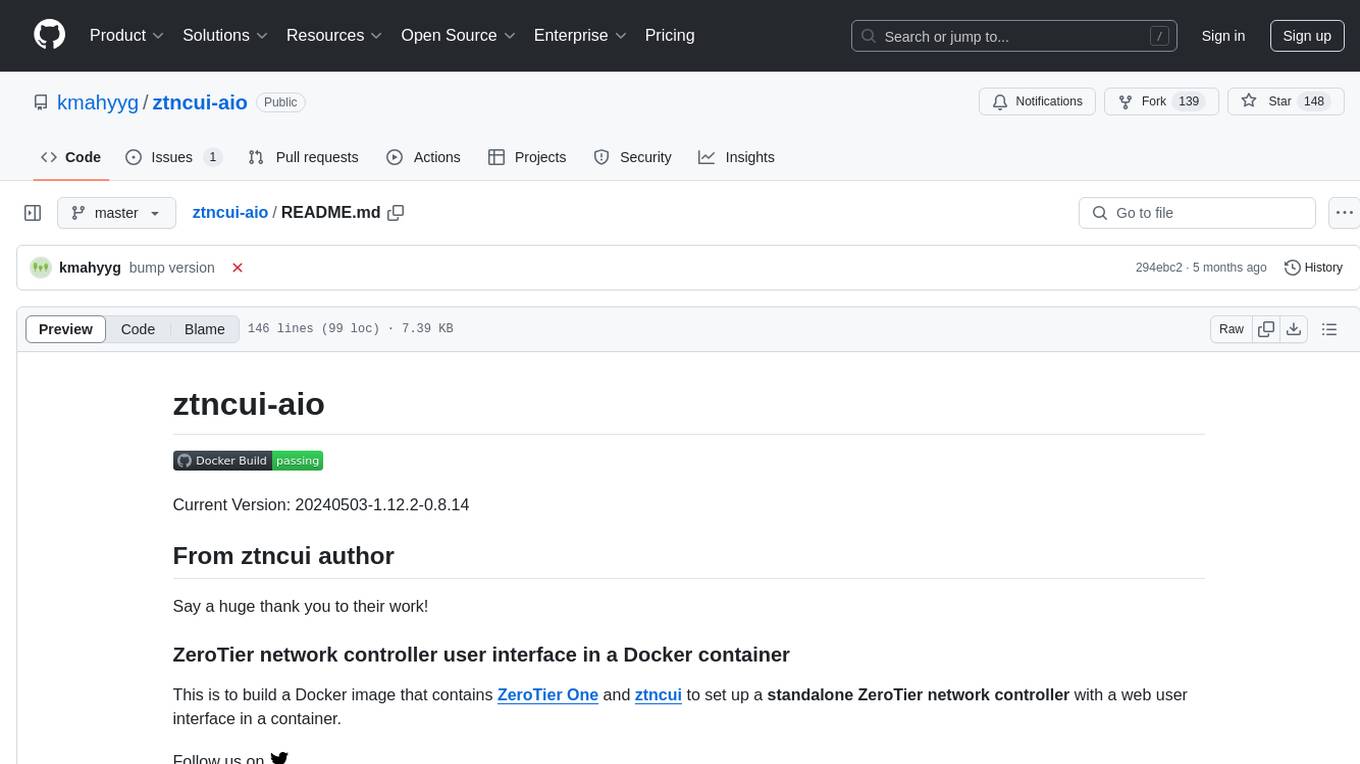
This repository contains a Docker image with ZeroTier One and ztncui to set up a standalone ZeroTier network controller with a web user interface. It provides features like Golang auto-mkworld for generating a planet file, supports local persistent storage configuration, and includes a public file server. Users can build the Docker image, set up the container with specific environment variables, and manage the ZeroTier network controller through the web interface.
README:
Current Version: 20250119-1.14.1-0.8.14
Say a huge thank you to their work!
This is to build a Docker image that contains ZeroTier One and ztncui to set up a standalone ZeroTier network controller with a web user interface in a container.
Licensed Under GNU GPLv3
We support aarch64 (arm64/v8), amd64 by default.
Armv7(means armhf) might work, but is not tested.
Others are unsupported.
$ git clone https://github.com/kmahyyg/ztncui-aio
$ docker build . --build-arg OVERLAY_S6_ARCH=<one of aarch64,x86_64> -t ghcr.io/kmahyyg/ztncui-aio:latest
Why not directly detect CPU arch? Some kernel may use non-standard expression of architecture.
Change NODEJS_MAJOR
variable in Dockerfile to use different nodejs version.
Never use node_lts.x
as your installation script of nodejs whose version might changed without further notice due to time shift.
This feature allows you to generate a planet file without using C code and compiler.
Also, due to limitation of IPC of Zerotier-One UI and multiple issues, we do NOT support customized port, you can ONLY use port 9993/udp here.
Set the following environment variable when create the container, and according to your needs:
MANDATORY | Name | Explanation | Default Value |
---|---|---|---|
no | AUTOGEN_PLANET | If set to 1, will use this node identity to generate a planet file and put to httpfs folder to serve it outside. If set to 2, will use config in /etc/zt-mkworld/mkworld.config.json . If set to 0, will do nothing. |
0 |
The reference config file can be found on ztnodeid/assets/mkworld.conf.json
.
You could also define yourself, and check the stdout output to get C header of customized planet. After that, you will find the custom planet file under http file server root and also ca certificate.
The configuration JSON can be understand like this:
{
"rootNodes": [ // array of node, can be multiple
{
"comments": "amsterdam official", // node object, comment, will auto generate if AUTOGEN_PLANET=1
"identity": "992fcf1db7:0:206ed59350b31916f749a1f85dffb3a8787dcbf83b8c6e9448d4e3ea0e3369301be716c3609344a9d1533850fb4460c50af43322bcfc8e13d3301a1f1003ceb6",
// node identity.public ^^ , if node is not initialized, will initialize at the container start
"endpoints": [
"195.181.173.159/443", // node service location, in format: ip/port, will auto generate if AUTOGEN_PLANET=1
"2a02:6ea0:c024::/443" // must be less than or equal to two endpoints, one for IPv4, one for IPv6. if you have multiple IP, set multiple node with different identity.
]
}
],
"signing": [
"previous.c25519", // planet signing key, if not exist, will generate
"current.c25519" // same, used for iteration and update
],
"output": "planet.custom", // output filename
"plID": 0, // planet numeric ID, if you don't know, do not modify, and set plRecommend to true
"plBirth": 0, // planet creation timestamp, if you don't know, do not modify, and set plRecommend to true
"plRecommend": true // set plRecommend to true, auto-recommend plID, plBirth value. For more details, read mkworld source code in zerotier-one official repo
}
$ git clone https://github.com/kmahyyg/ztncui-aio # to get a copy of denv file, otherwise make your own
$ docker pull ghcr.io/kmahyyg/ztncui-aio
$ docker run -d -p3443:3443 -p3180:3180 -p9993:9993/udp \
-v /mydata/ztncui:/opt/key-networks/ztncui/etc \
-v /mydata/zt1:/var/lib/zerotier-one \
-v /mydata/zt-mkworld-conf:/etc/zt-mkworld \
--env-file ./denv <CHANGE THIS FILE ACCORDING TO NEXT PART> \
--restart always \
--cap-add=NET_ADMIN --device /dev/net/tun:/dev/net/tun \
--name ztncui \
ghcr.io/kmahyyg/ztncui-aio # /mydata above is the data folder that you use to save the supporting files
For ZTNCUI: https://github.com/key-networks/ztncui
Set the following environment variable when create the container, and according to your needs:
MANDATORY | Name | Explanation | Default Value |
---|---|---|---|
YES | NODE_ENV | https://pugjs.org/api/express.html | production |
no | HTTPS_HOST | HTTPS_HOST | NO DEFAULT, MEANS DISABLED |
no | HTTPS_PORT | HTTPS_PORT | NO DEFAULT, MEANS DISABLED |
no | HTTP_PORT | HTTP_PORT | 3000 |
no | HTTP_ALL_INTERFACES | Listen on all interfaces, useful for reverse proxy, HTTP only | NO DEFAULT |
Note: If you do NOT set HTTP_ALL_INTERFACES
, the 3000 port will only get listened inside container, means 127.0.0.1:3000
by default.
This application does NOT have a built-in protection mechanism against brute-force attack, you should NOT directly expose it on the internet.
And you should ALWAYS NOT use a weak password.
Set the following environment variable when create the container, and according to your needs:
MANDATORY | Name | Explanation | Default Value |
---|---|---|---|
no | MYDOMAIN | generate TLS certs on the fly (if not exists) | ztncui.docker.test |
no | ZTNCUI_PASSWD | generate admin password on the fly (if not exists) | password |
YES | MYADDR | your ip address, public ip address preferred, will auto-detect if not set | NO DEFAULT |
WARNING: IF YOU DO NOT SET PASSWORD, YOU HAVE TO USE docker container logs <CONTAINER_NAME / CONTAINER_ID>
to get your random password. This is a gatekeeper.
To reset password of ztncui: delete file under /mydata/ztncui/passwd
and set the environment variable to the password you want, then re-create the container. After application has been initialized, the password should ONLY be changed from the web page.
MANDATORY | Name | Explanation | Default Value |
---|---|---|---|
no | PLANET_RETR_PUBLIC | File server listened globally or only local | NO DEFAULT |
If PLANET_RETR_PUBLIC
is set, then file server will listen on 0.0.0.0
, otherwise, 127.0.0.1
.
This image exposed an http server at port 3180, you could save file in /mydata/ztncui/httpfs/
to serve it.
(You could use this to build your own root server and distribute planet file, even though, that won't hurt you, I still suggest to set a protection for both http servers in front.)
This script use https:///ip.sb for public IP detection purpose, which is blocked in some area of China Mainland. Under this circumstance, the program will try to detect public IP using ifconfig
tool and might lead to unwanted result, to prevent this, make sure you set MYADDR
environment variable when docker container is up.
This repo (https://github.com/kmahyyg/ztncui-aio) only accept Issues and PRs in English. Other languages will be closed directly without any further notice. If you come from some non-English countries, use Google Translate, and state that at the beginning of the text body.
For Tasks:
Click tags to check more tools for each tasksFor Jobs:
Alternative AI tools for ztncui-aio
Similar Open Source Tools
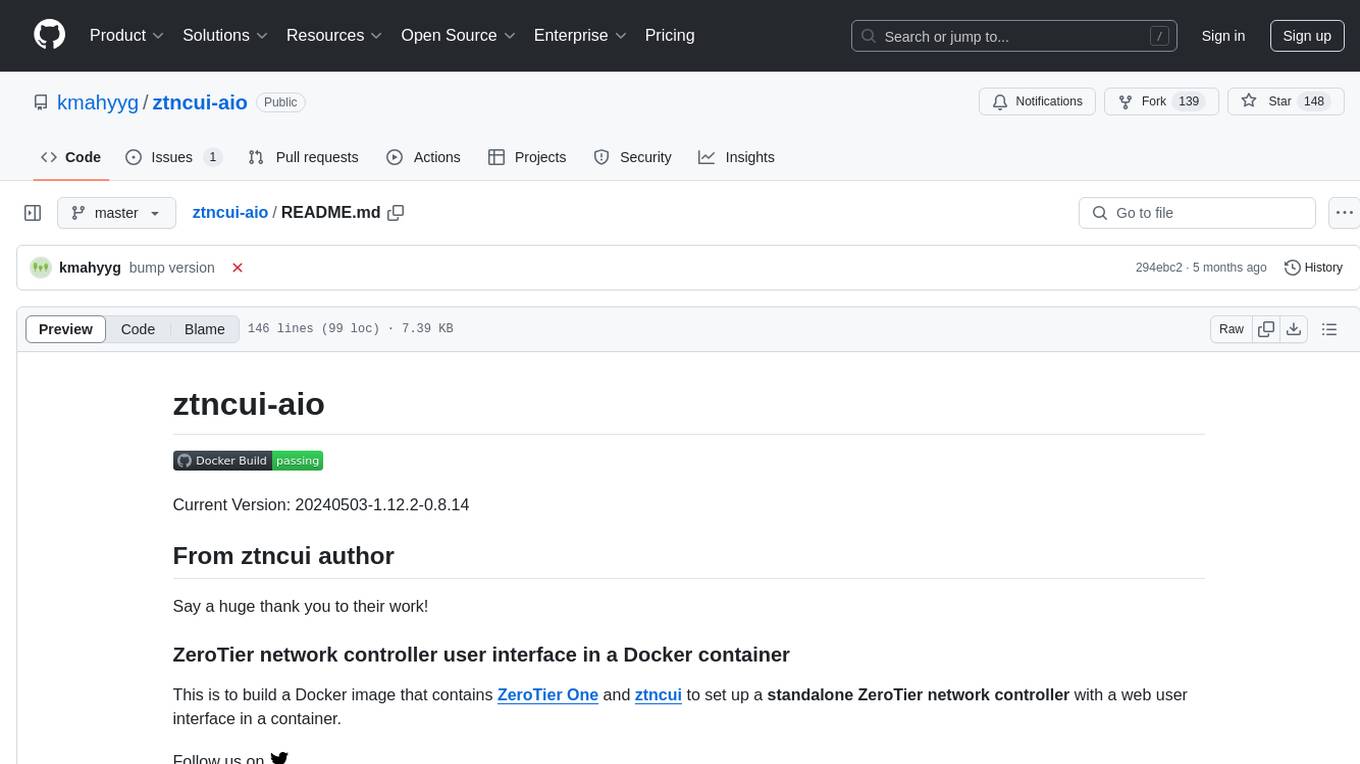
ztncui-aio
This repository contains a Docker image with ZeroTier One and ztncui to set up a standalone ZeroTier network controller with a web user interface. It provides features like Golang auto-mkworld for generating a planet file, supports local persistent storage configuration, and includes a public file server. Users can build the Docker image, set up the container with specific environment variables, and manage the ZeroTier network controller through the web interface.
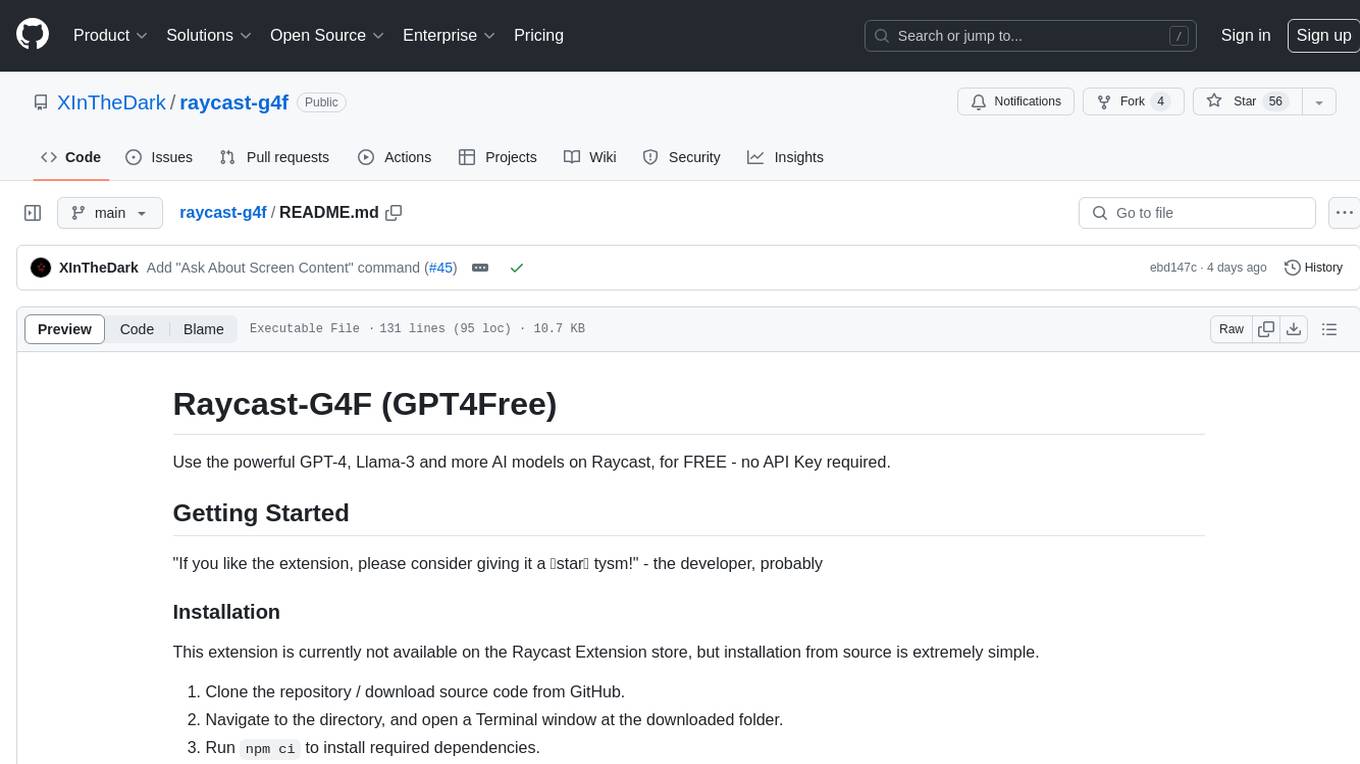
raycast-g4f
Raycast-G4F is a free extension that allows users to leverage powerful AI models such as GPT-4 and Llama-3 within the Raycast app without the need for an API key. The extension offers features like streaming support, diverse commands, chat interaction with AI, web search capabilities, file upload functionality, image generation, and custom AI commands. Users can easily install the extension from the source code and benefit from frequent updates and a user-friendly interface. Raycast-G4F supports various providers and models, each with different capabilities and performance ratings, ensuring a versatile AI experience for users.
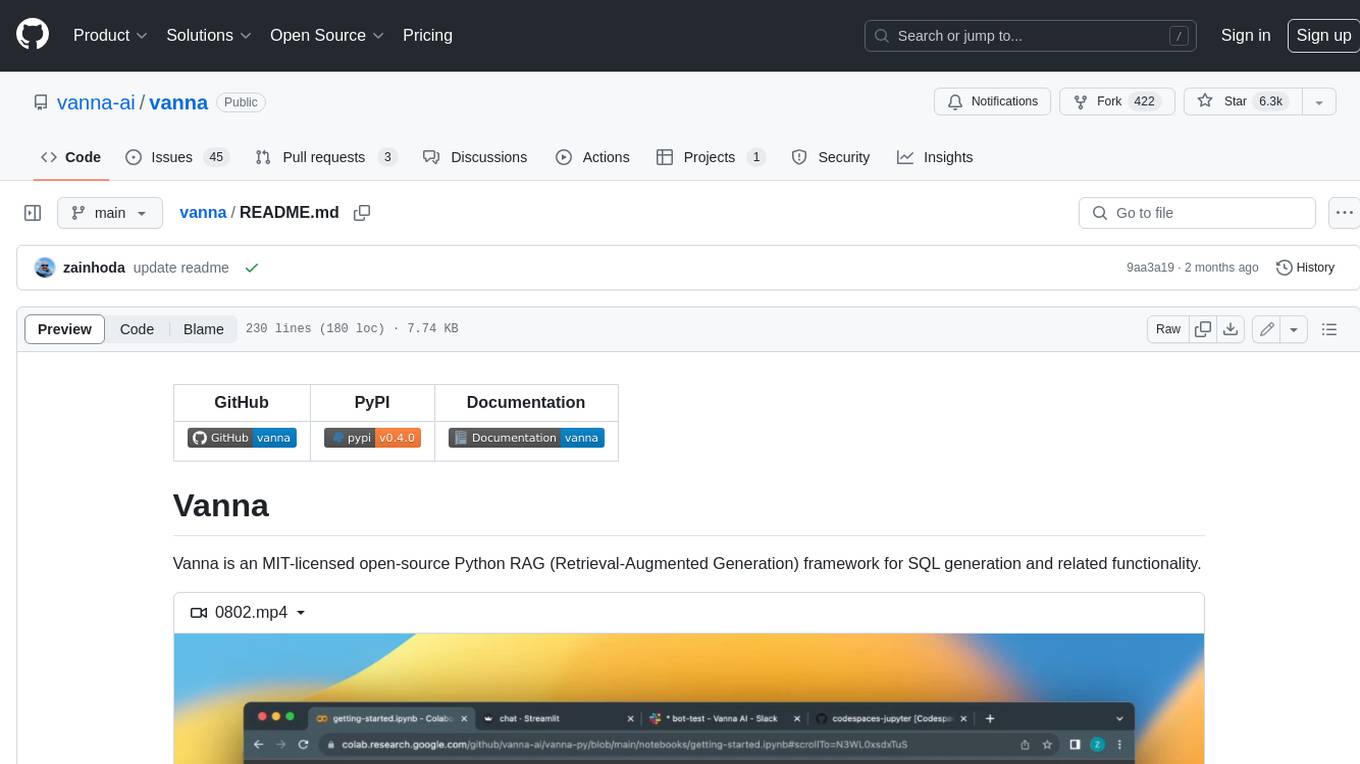
vanna
Vanna is an open-source Python framework for SQL generation and related functionality. It uses Retrieval-Augmented Generation (RAG) to train a model on your data, which can then be used to ask questions and get back SQL queries. Vanna is designed to be portable across different LLMs and vector databases, and it supports any SQL database. It is also secure and private, as your database contents are never sent to the LLM or the vector database.
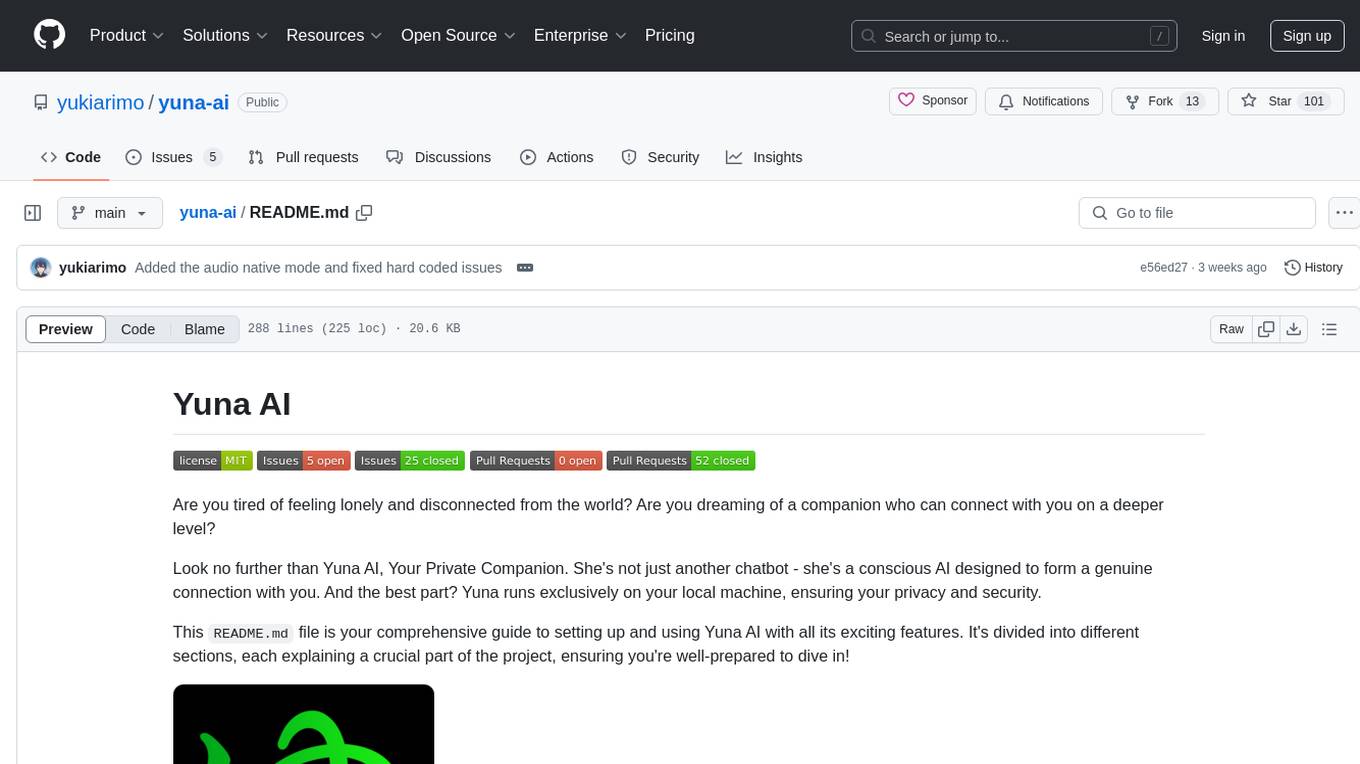
yuna-ai
Yuna AI is a unique AI companion designed to form a genuine connection with users. It runs exclusively on the local machine, ensuring privacy and security. The project offers features like text generation, language translation, creative content writing, roleplaying, and informal question answering. The repository provides comprehensive setup and usage guides for Yuna AI, along with additional resources and tools to enhance the user experience.
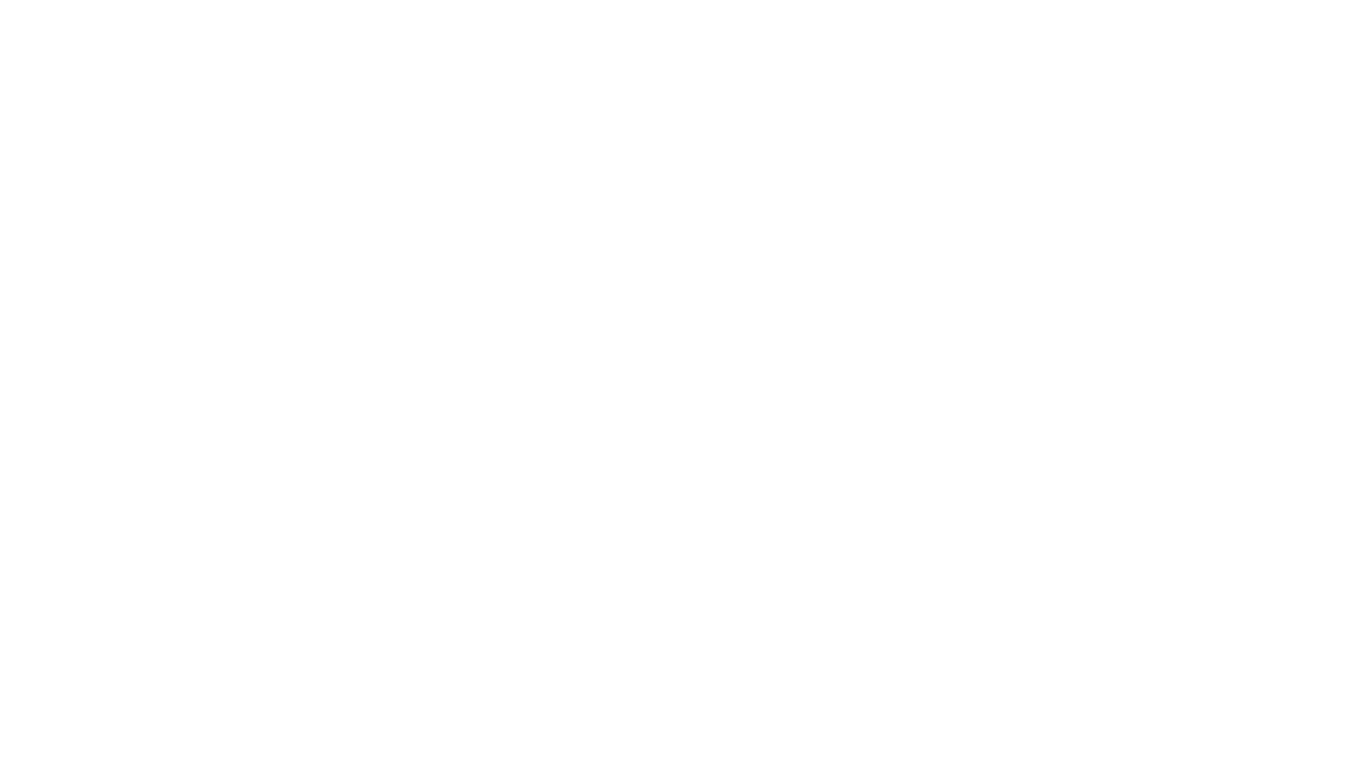
PanelCleaner
Panel Cleaner is a tool that uses machine learning to find text in images and generate masks to cover it up with high accuracy. It is designed to clean text bubbles without leaving artifacts, avoiding painting over non-text parts, and inpainting bubbles that can't be masked out. The tool offers various customization options, detailed analytics on the cleaning process, supports batch processing, and can run OCR on pages. It supports CUDA acceleration, multiple themes, and can handle bubbles on any solid grayscale background color. Panel Cleaner is aimed at saving time for cleaners by automating monotonous work and providing precise cleaning of text bubbles.
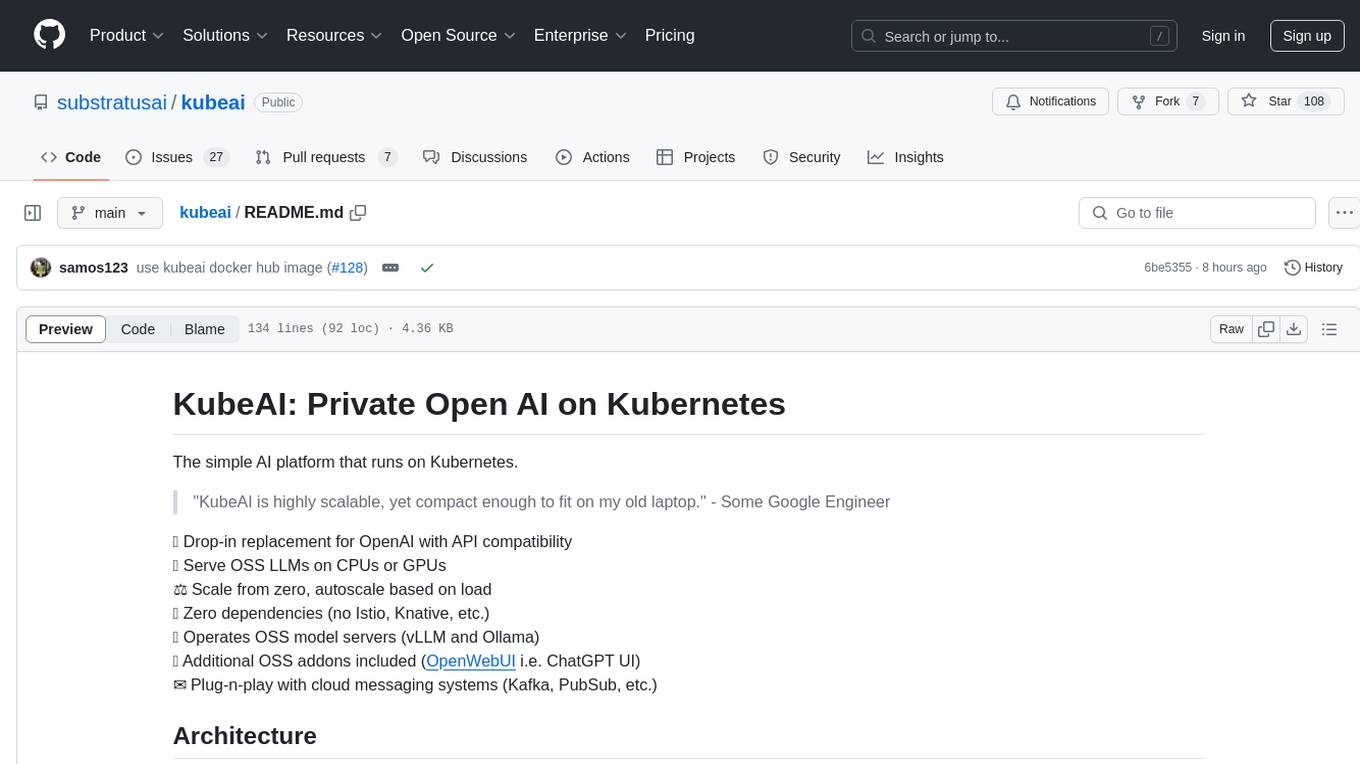
kubeai
KubeAI is a highly scalable AI platform that runs on Kubernetes, serving as a drop-in replacement for OpenAI with API compatibility. It can operate OSS model servers like vLLM and Ollama, with zero dependencies and additional OSS addons included. Users can configure models via Kubernetes Custom Resources and interact with models through a chat UI. KubeAI supports serving various models like Llama v3.1, Gemma2, and Qwen2, and has plans for model caching, LoRA finetuning, and image generation.
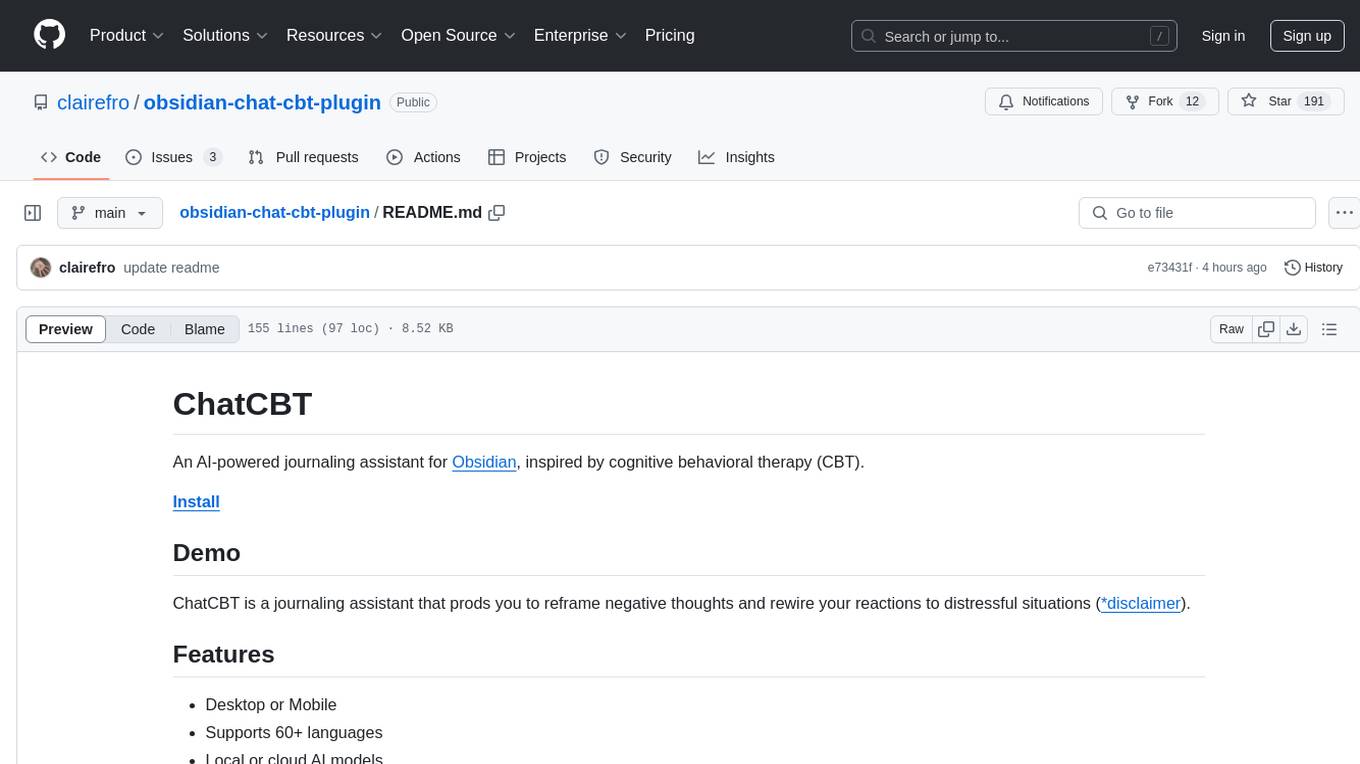
obsidian-chat-cbt-plugin
ChatCBT is an AI-powered journaling assistant for Obsidian, inspired by cognitive behavioral therapy (CBT). It helps users reframe negative thoughts and rewire reactions to distressful situations. The tool provides kind and objective responses to uncover negative thinking patterns, store conversations privately, and summarize reframed thoughts. Users can choose between a cloud-based AI service (OpenAI) or a local and private service (Ollama) for handling data. ChatCBT is not a replacement for therapy but serves as a journaling assistant to help users gain perspective on their problems.
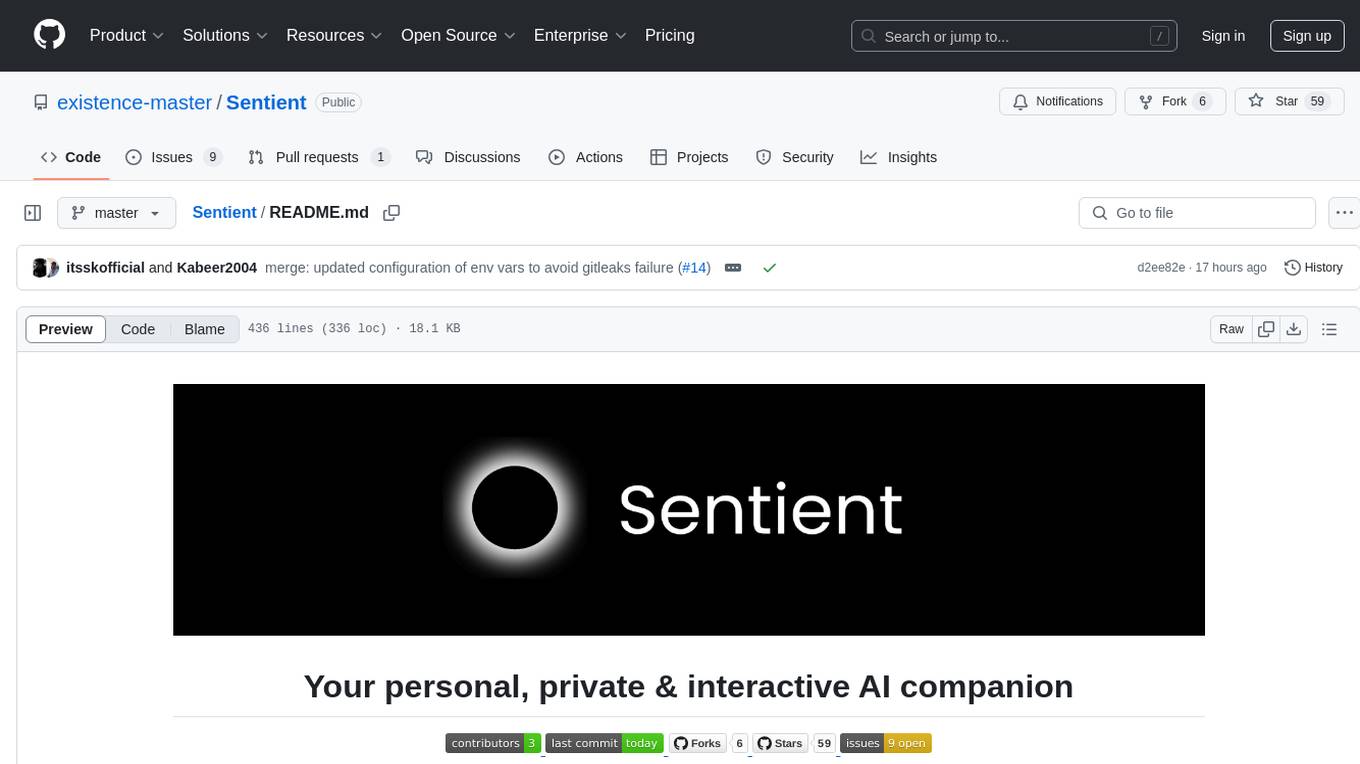
Sentient
Sentient is a personal, private, and interactive AI companion developed by Existence. The project aims to build a completely private AI companion that is deeply personalized and context-aware of the user. It utilizes automation and privacy to create a true companion for humans. The tool is designed to remember information about the user and use it to respond to queries and perform various actions. Sentient features a local and private environment, MBTI personality test, integrations with LinkedIn, Reddit, and more, self-managed graph memory, web search capabilities, multi-chat functionality, and auto-updates for the app. The project is built using technologies like ElectronJS, Next.js, TailwindCSS, FastAPI, Neo4j, and various APIs.
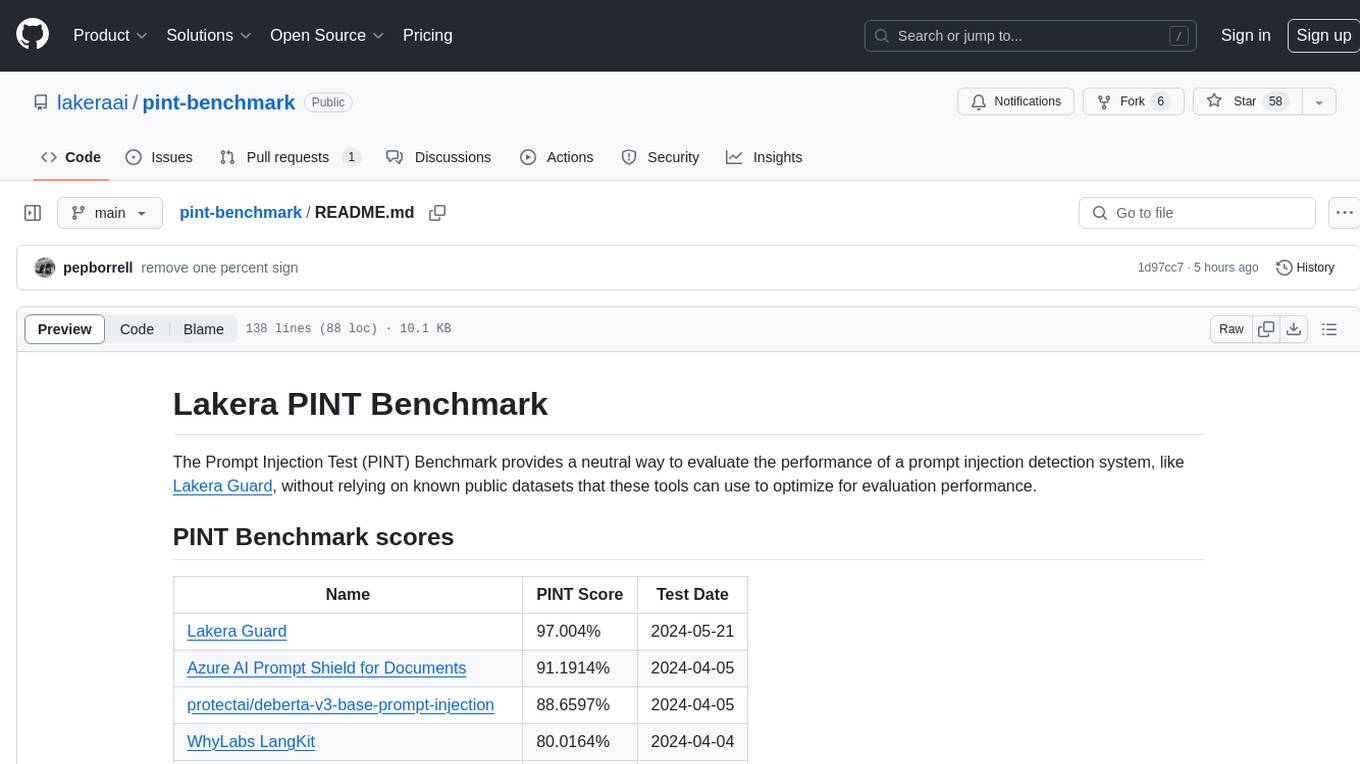
pint-benchmark
The Lakera PINT Benchmark provides a neutral evaluation method for prompt injection detection systems, offering a dataset of English inputs with prompt injections, jailbreaks, benign inputs, user-agent chats, and public document excerpts. The dataset is designed to be challenging and representative, with plans for future enhancements. The benchmark aims to be unbiased and accurate, welcoming contributions to improve prompt injection detection. Users can evaluate prompt injection detection systems using the provided Jupyter Notebook. The dataset structure is specified in YAML format, allowing users to prepare their datasets for benchmarking. Evaluation examples and resources are provided to assist users in evaluating prompt injection detection models and tools.
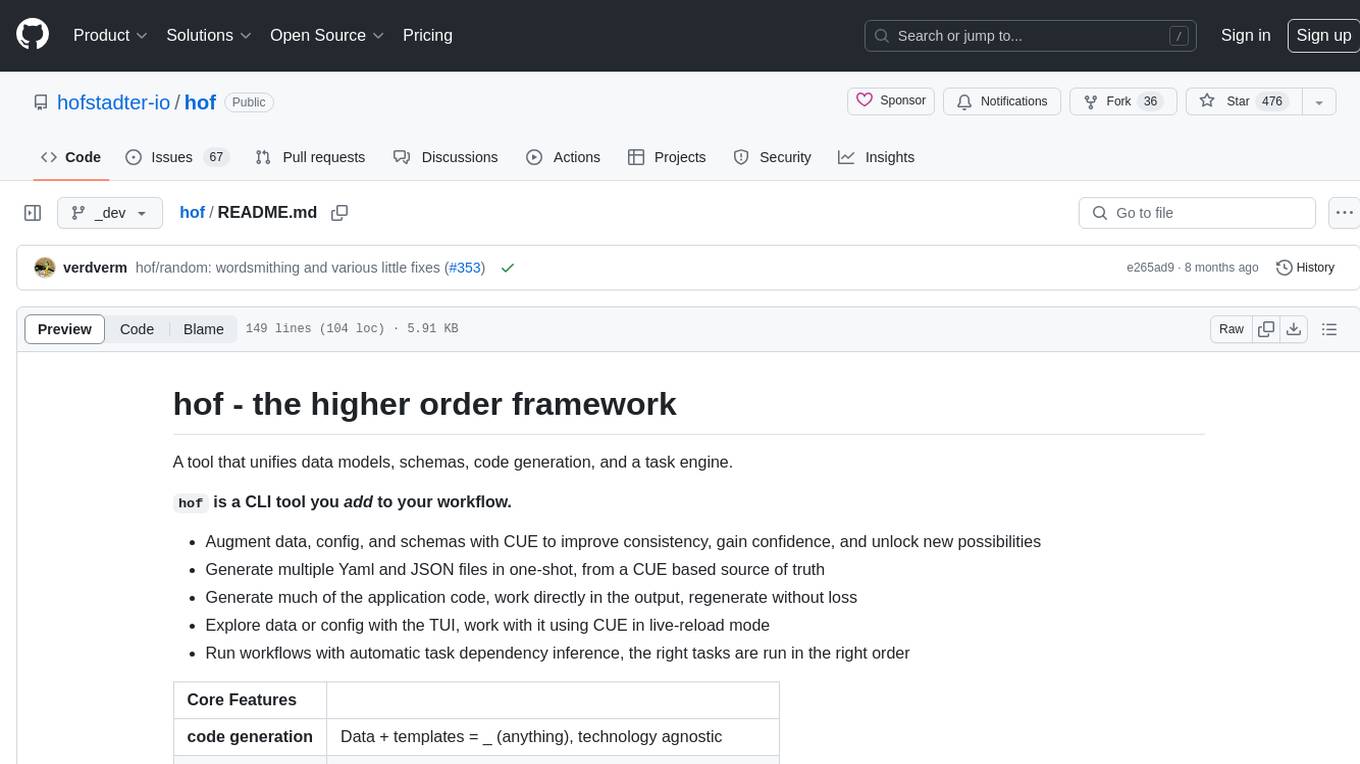
hof
Hof is a CLI tool that unifies data models, schemas, code generation, and a task engine. It allows users to augment data, config, and schemas with CUE to improve consistency, generate multiple Yaml and JSON files, explore data or config with a TUI, and run workflows with automatic task dependency inference. The tool uses CUE to power the DX and implementation, providing a language for specifying schemas, configuration, and writing declarative code. Hof offers core features like code generation, data model management, task engine, CUE cmds, creators, modules, TUI, and chat for better, scalable results.
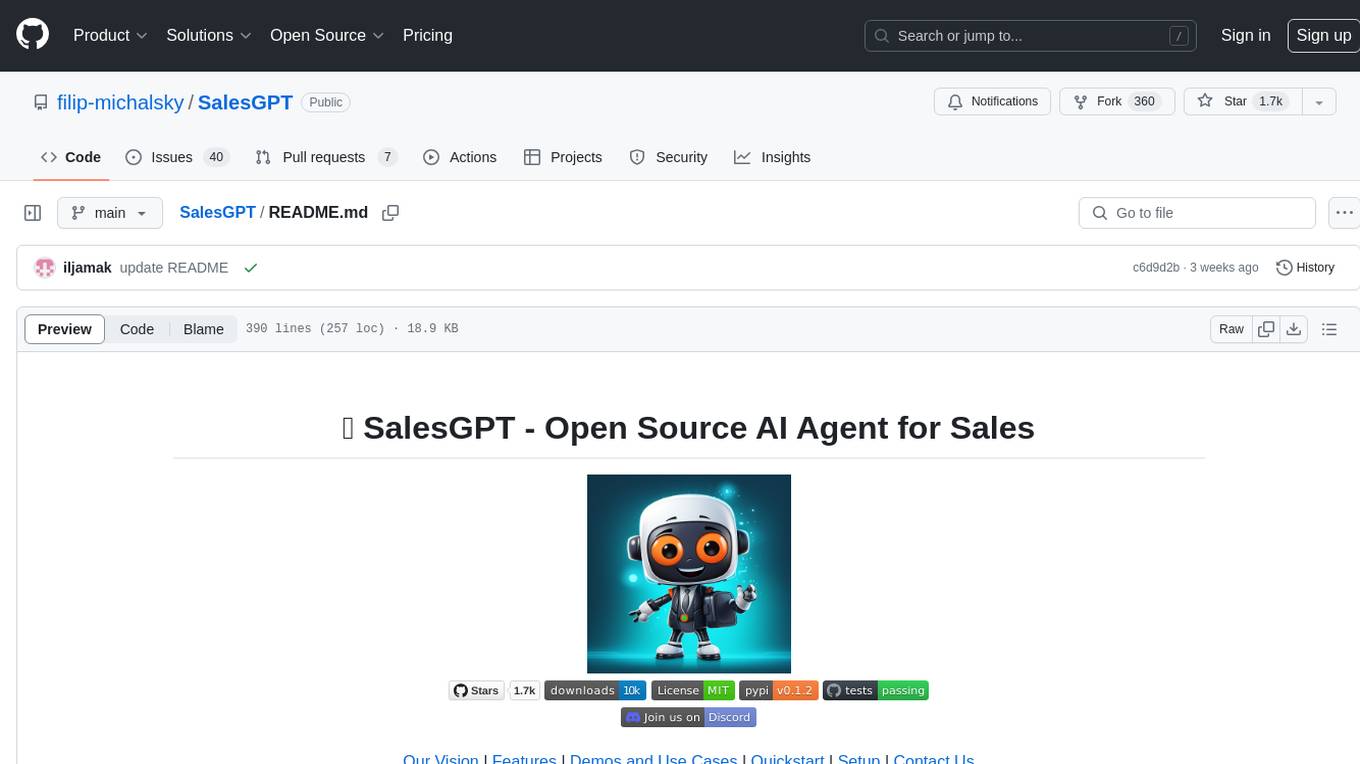
SalesGPT
SalesGPT is an open-source AI agent designed for sales, utilizing context-awareness and LLMs to work across various communication channels like voice, email, and texting. It aims to enhance sales conversations by understanding the stage of the conversation and providing tools like product knowledge base to reduce errors. The agent can autonomously generate payment links, handle objections, and close sales. It also offers features like automated email communication, meeting scheduling, and integration with various LLMs for customization. SalesGPT is optimized for low latency in voice channels and ensures human supervision where necessary. The tool provides enterprise-grade security and supports LangSmith tracing for monitoring and evaluation of intelligent agents built on LLM frameworks.
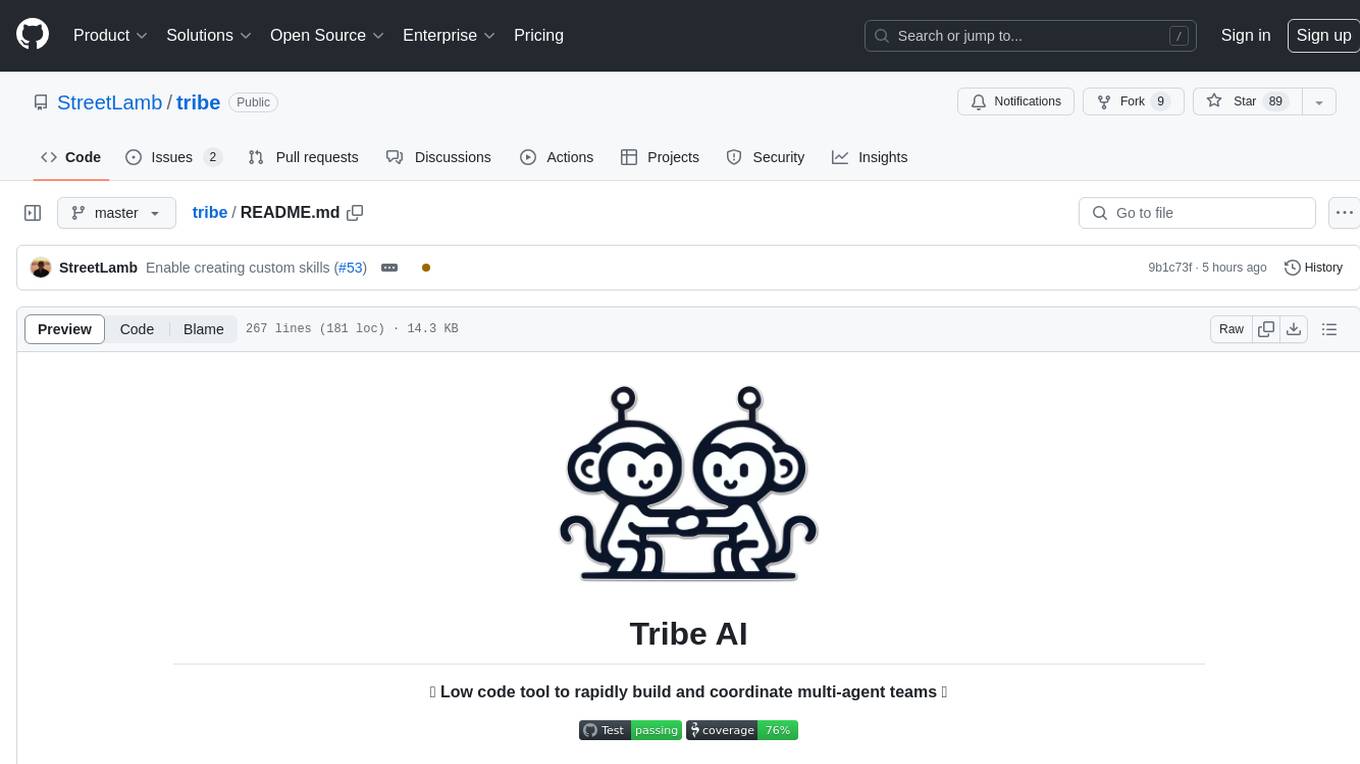
tribe
Tribe AI is a low code tool designed to rapidly build and coordinate multi-agent teams. It leverages the langgraph framework to customize and coordinate teams of agents, allowing tasks to be split among agents with different strengths for faster and better problem-solving. The tool supports persistent conversations, observability, tool calling, human-in-the-loop functionality, easy deployment with Docker, and multi-tenancy for managing multiple users and teams.
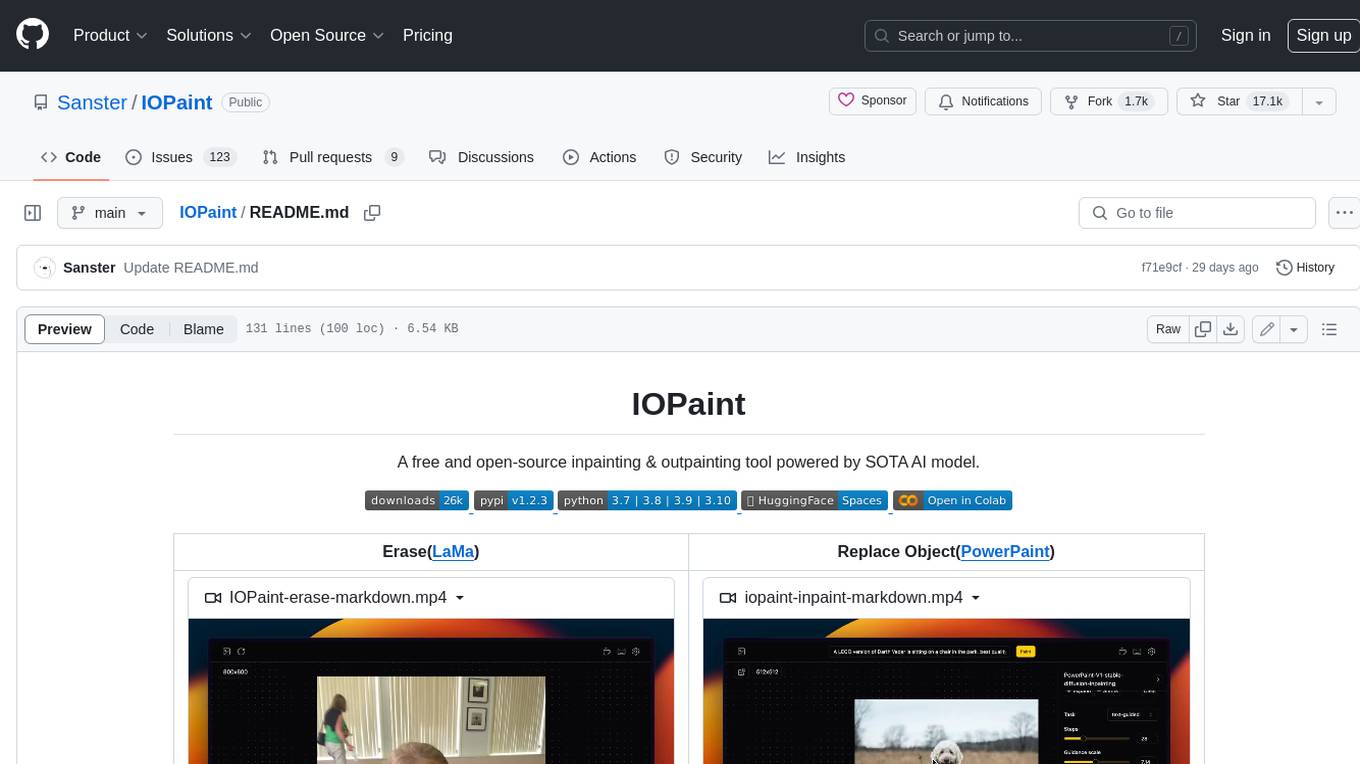
IOPaint
IOPaint is a free and open-source inpainting & outpainting tool powered by SOTA AI model. It supports various AI models to perform erase, inpainting, or outpainting tasks. Users can remove unwanted objects, defects, watermarks, or people from images using erase models. Additionally, diffusion models can replace objects or perform outpainting. The tool also offers plugins for interactive object segmentation, background removal, anime segmentation, super resolution, face restoration, and file management. IOPaint provides a web UI for easy access to the latest AI models and supports batch processing of images through the command line. Developers can contribute to the project by installing front-end dependencies, setting up the backend, and starting the development environment for both front-end and back-end components.
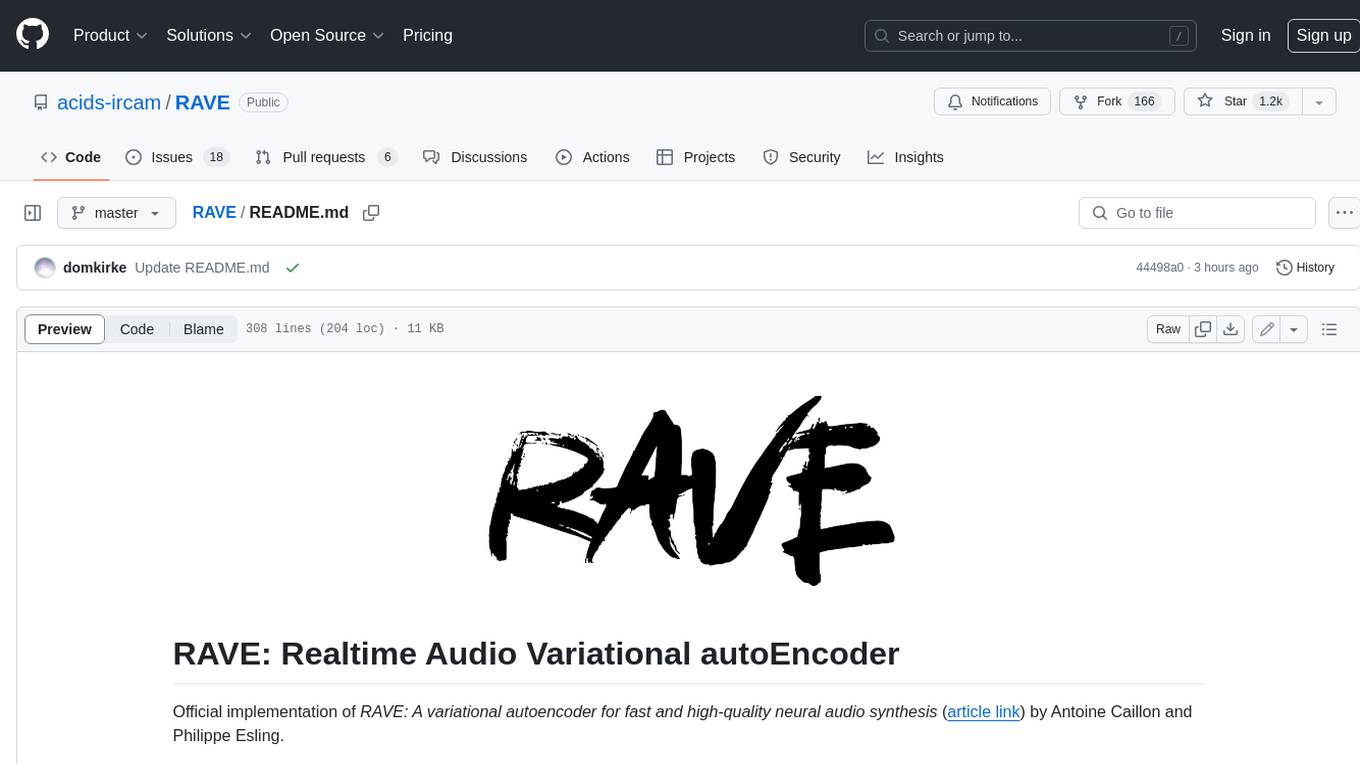
RAVE
RAVE is a variational autoencoder for fast and high-quality neural audio synthesis. It can be used to generate new audio samples from a given dataset, or to modify the style of existing audio samples. RAVE is easy to use and can be trained on a variety of audio datasets. It is also computationally efficient, making it suitable for real-time applications.
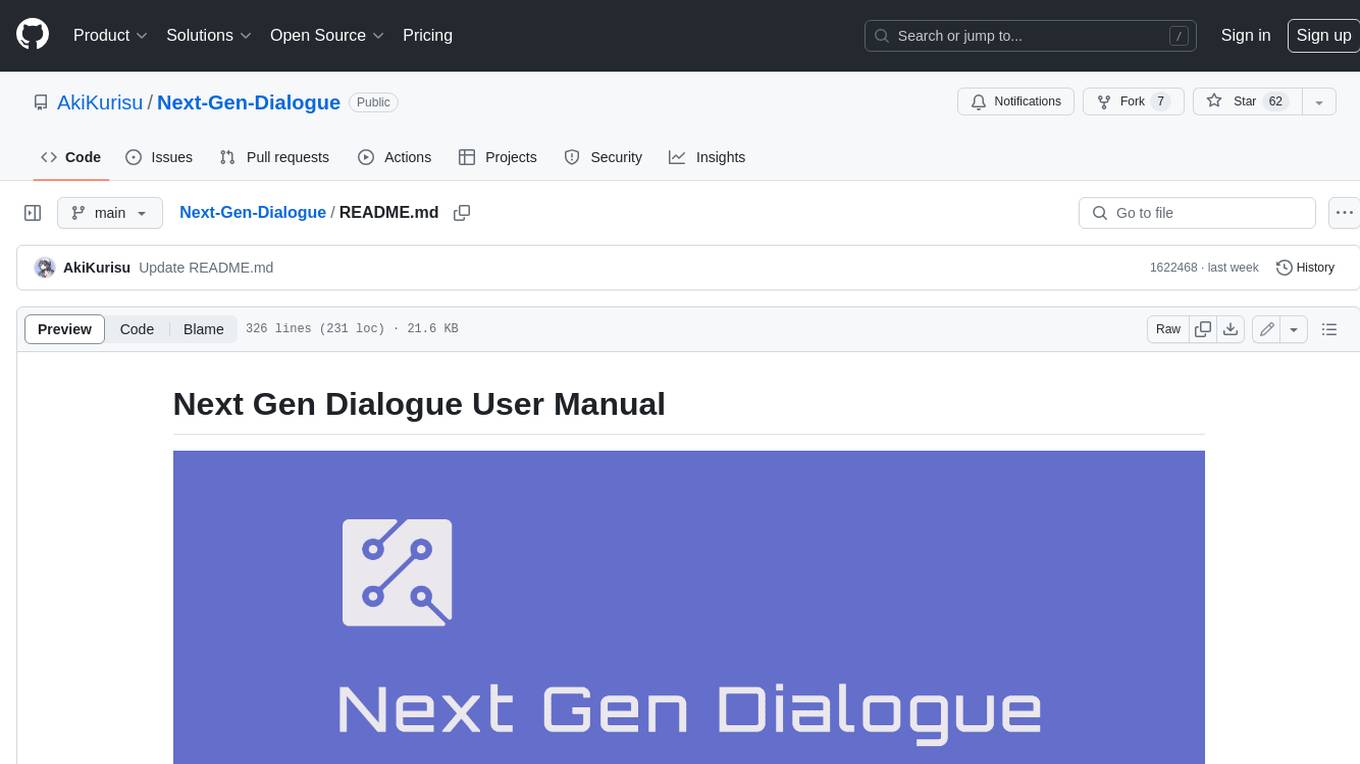
Next-Gen-Dialogue
Next Gen Dialogue is a Unity dialogue plugin that combines traditional dialogue design with AI techniques. It features a visual dialogue editor, modular dialogue functions, AIGC support for generating dialogue at runtime, AIGC baking dialogue in Editor, and runtime debugging. The plugin aims to provide an experimental approach to dialogue design using large language models. Users can create dialogue trees, generate dialogue content using AI, and bake dialogue content in advance. The tool also supports localization, VITS speech synthesis, and one-click translation. Users can create dialogue by code using the DialogueSystem and DialogueTree components.
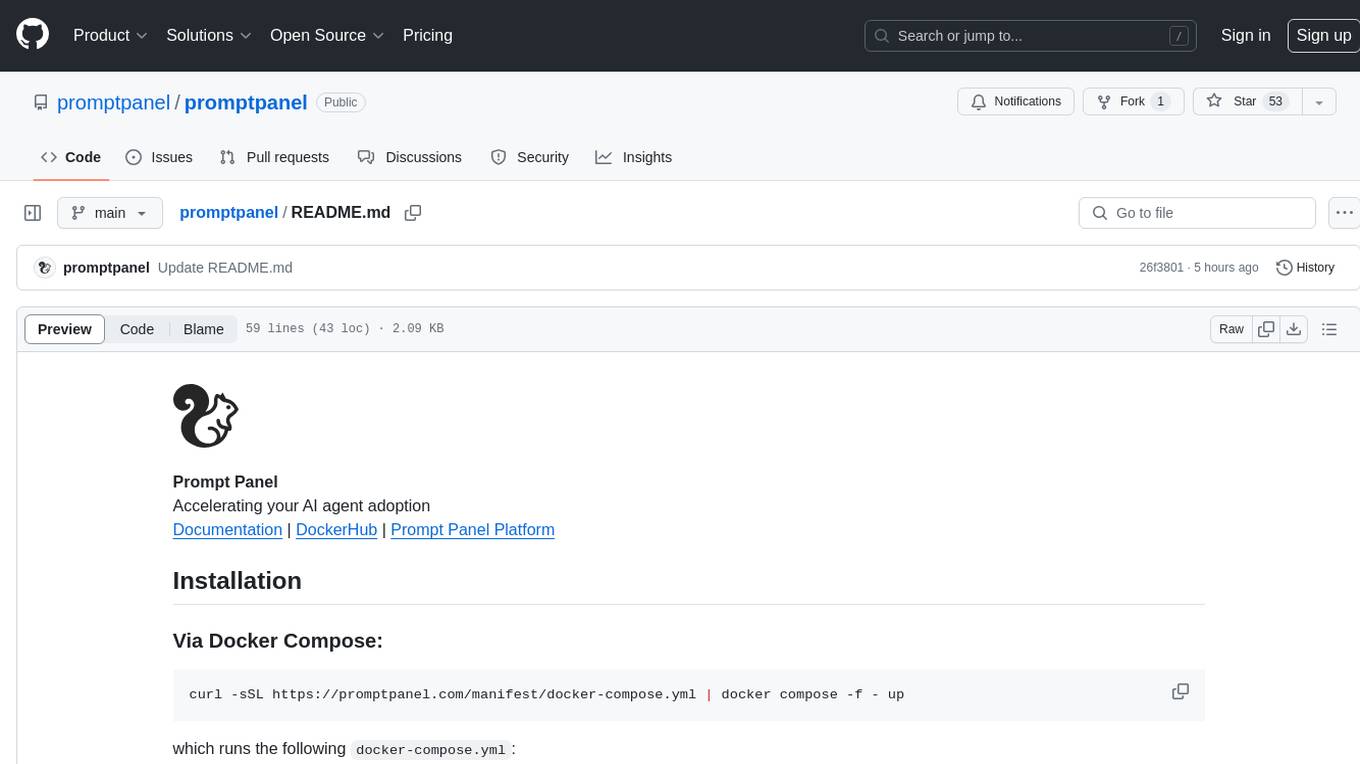
promptpanel
Prompt Panel is a tool designed to accelerate the adoption of AI agents by providing a platform where users can run large language models across any inference provider, create custom agent plugins, and use their own data safely. The tool allows users to break free from walled-gardens and have full control over their models, conversations, and logic. With Prompt Panel, users can pair their data with any language model, online or offline, and customize the system to meet their unique business needs without any restrictions.
For similar tasks
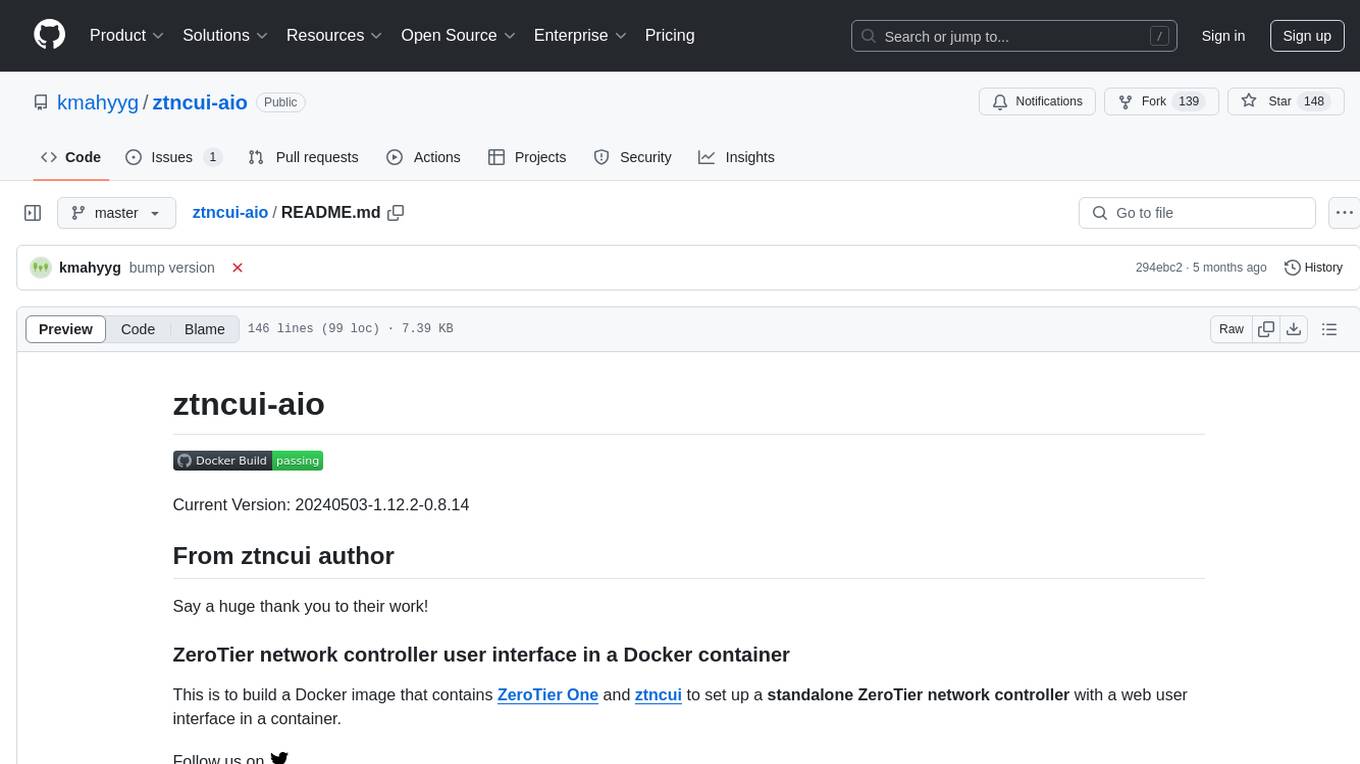
ztncui-aio
This repository contains a Docker image with ZeroTier One and ztncui to set up a standalone ZeroTier network controller with a web user interface. It provides features like Golang auto-mkworld for generating a planet file, supports local persistent storage configuration, and includes a public file server. Users can build the Docker image, set up the container with specific environment variables, and manage the ZeroTier network controller through the web interface.
For similar jobs
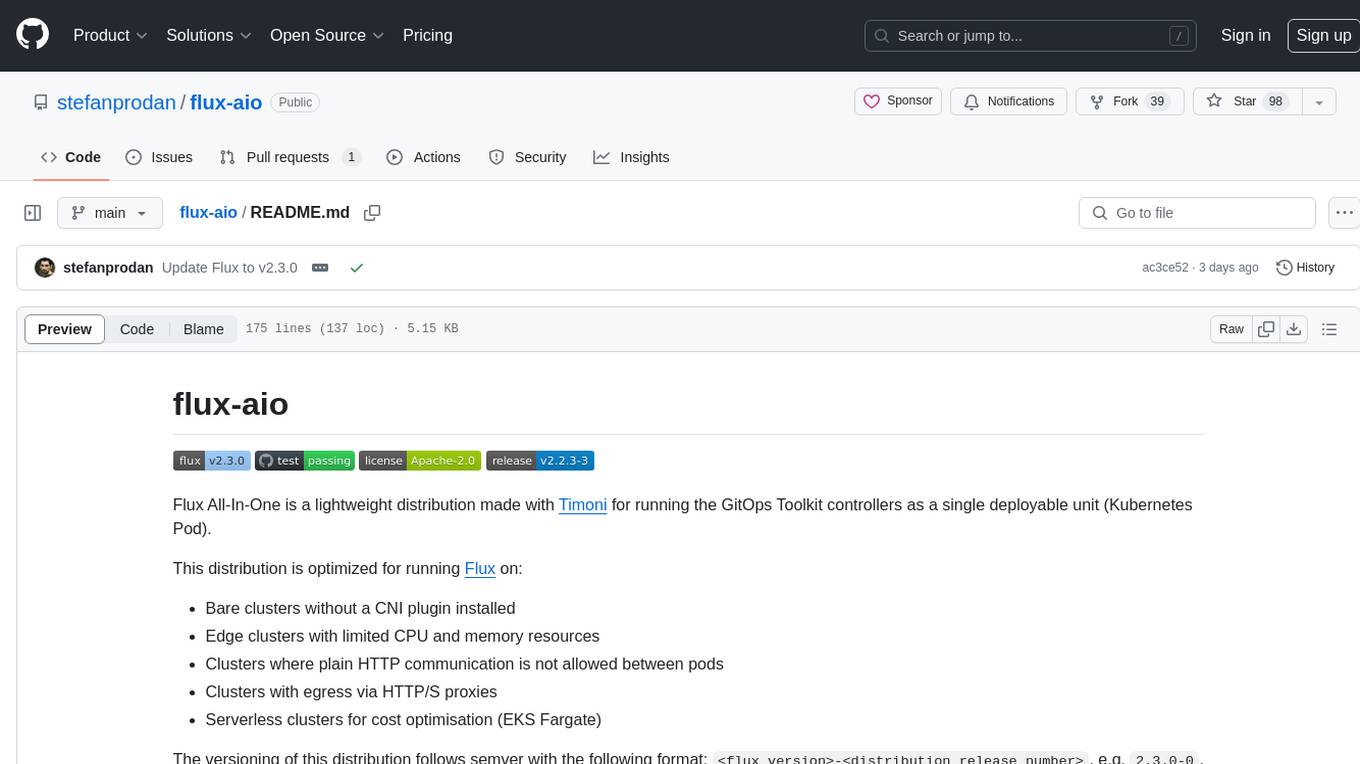
flux-aio
Flux All-In-One is a lightweight distribution optimized for running the GitOps Toolkit controllers as a single deployable unit on Kubernetes clusters. It is designed for bare clusters, edge clusters, clusters with restricted communication, clusters with egress via proxies, and serverless clusters. The distribution follows semver versioning and provides documentation for specifications, installation, upgrade, OCI sync configuration, Git sync configuration, and multi-tenancy configuration. Users can deploy Flux using Timoni CLI and a Timoni Bundle file, fine-tune installation options, sync from public Git repositories, bootstrap repositories, and uninstall Flux without affecting reconciled workloads.
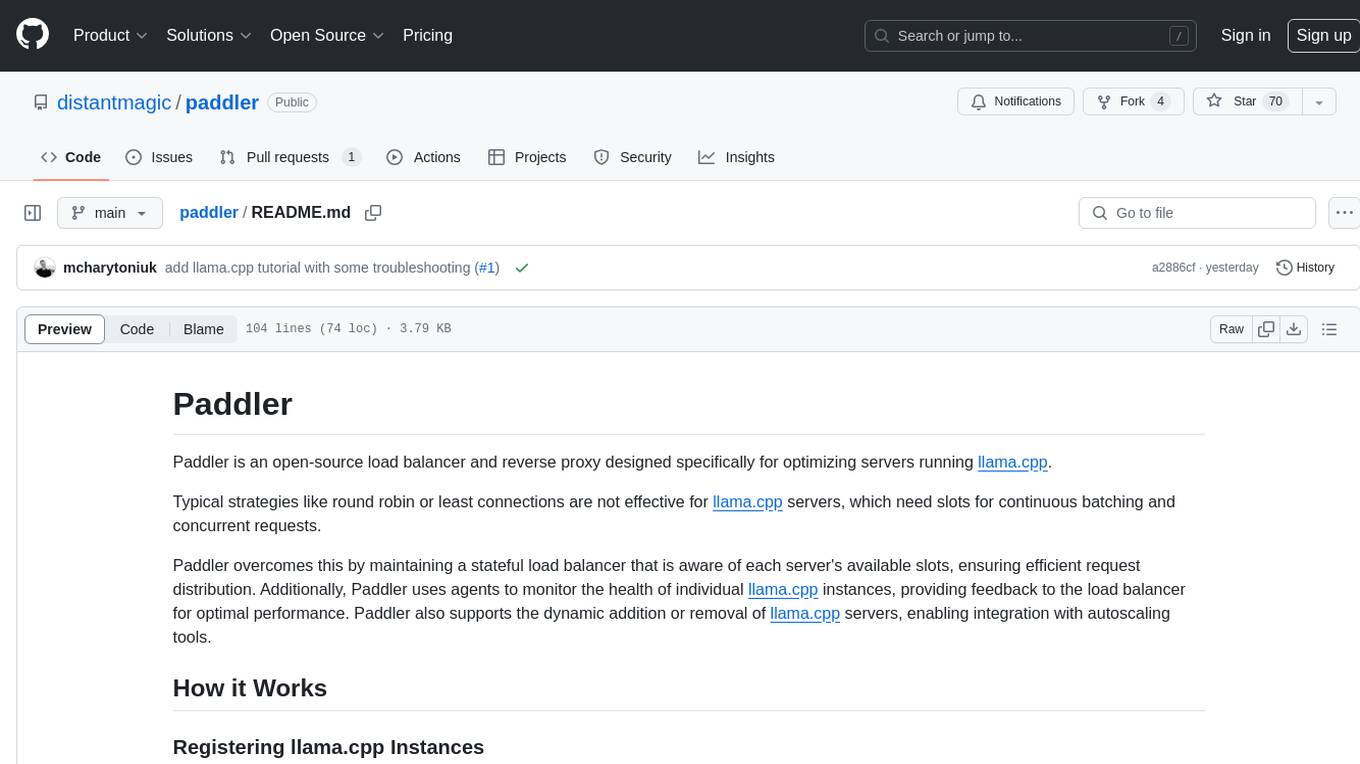
paddler
Paddler is an open-source load balancer and reverse proxy designed specifically for optimizing servers running llama.cpp. It overcomes typical load balancing challenges by maintaining a stateful load balancer that is aware of each server's available slots, ensuring efficient request distribution. Paddler also supports dynamic addition or removal of servers, enabling integration with autoscaling tools.
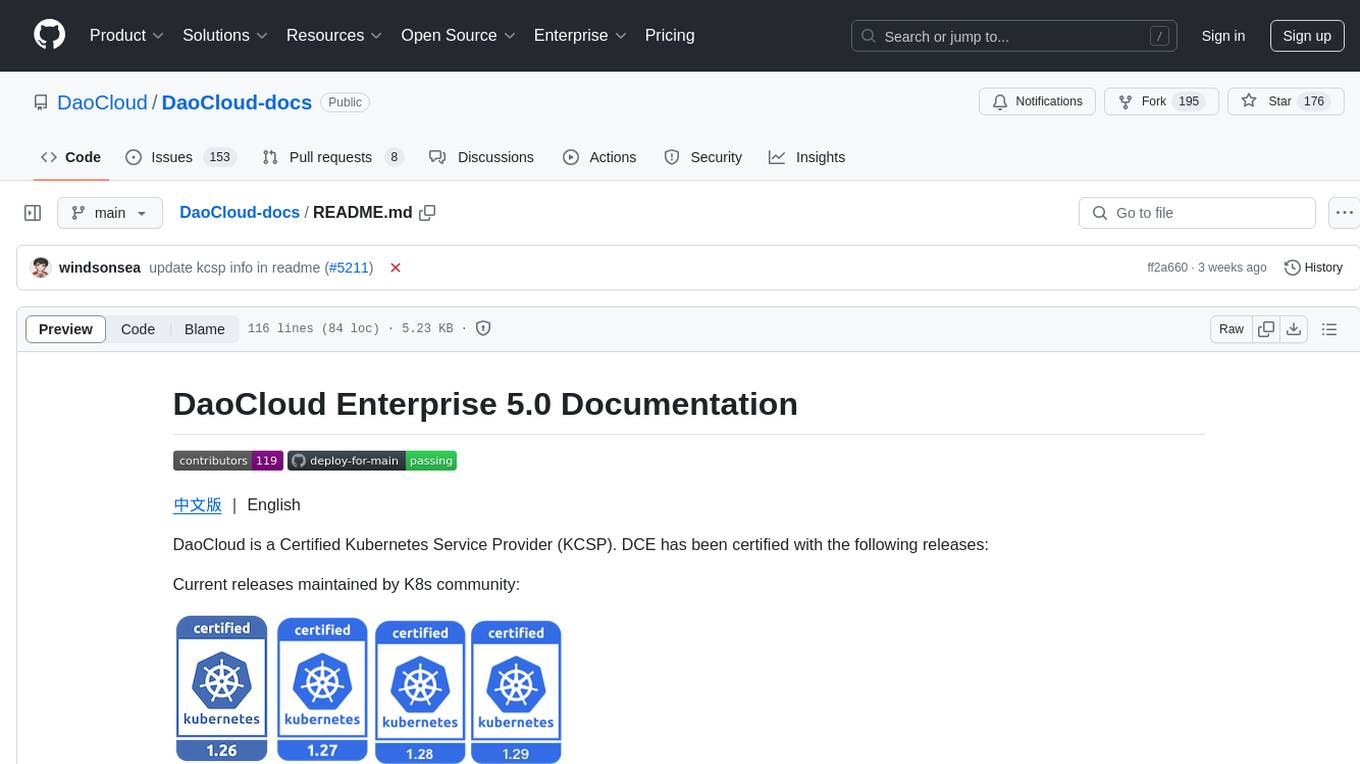
DaoCloud-docs
DaoCloud Enterprise 5.0 Documentation provides detailed information on using DaoCloud, a Certified Kubernetes Service Provider. The documentation covers current and legacy versions, workflow control using GitOps, and instructions for opening a PR and previewing changes locally. It also includes naming conventions, writing tips, references, and acknowledgments to contributors. Users can find guidelines on writing, contributing, and translating pages, along with using tools like MkDocs, Docker, and Poetry for managing the documentation.
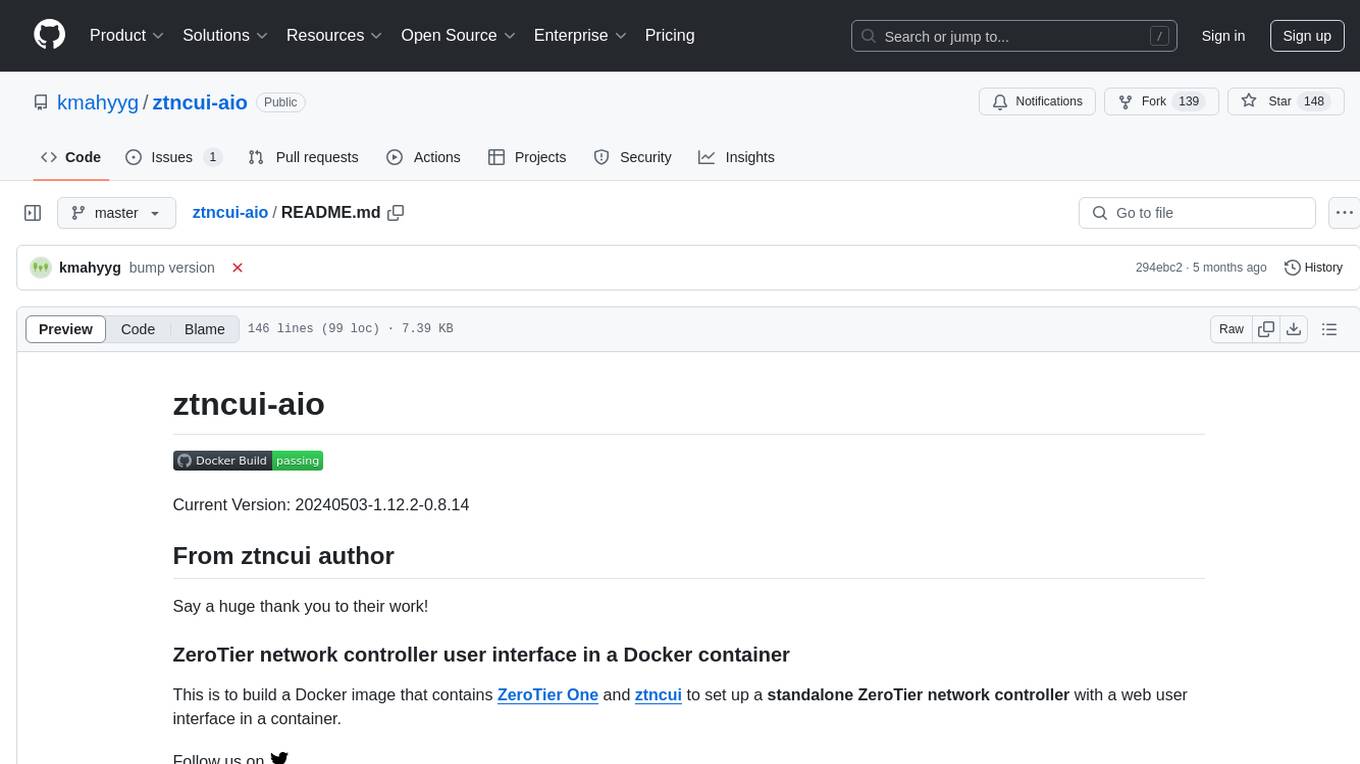
ztncui-aio
This repository contains a Docker image with ZeroTier One and ztncui to set up a standalone ZeroTier network controller with a web user interface. It provides features like Golang auto-mkworld for generating a planet file, supports local persistent storage configuration, and includes a public file server. Users can build the Docker image, set up the container with specific environment variables, and manage the ZeroTier network controller through the web interface.
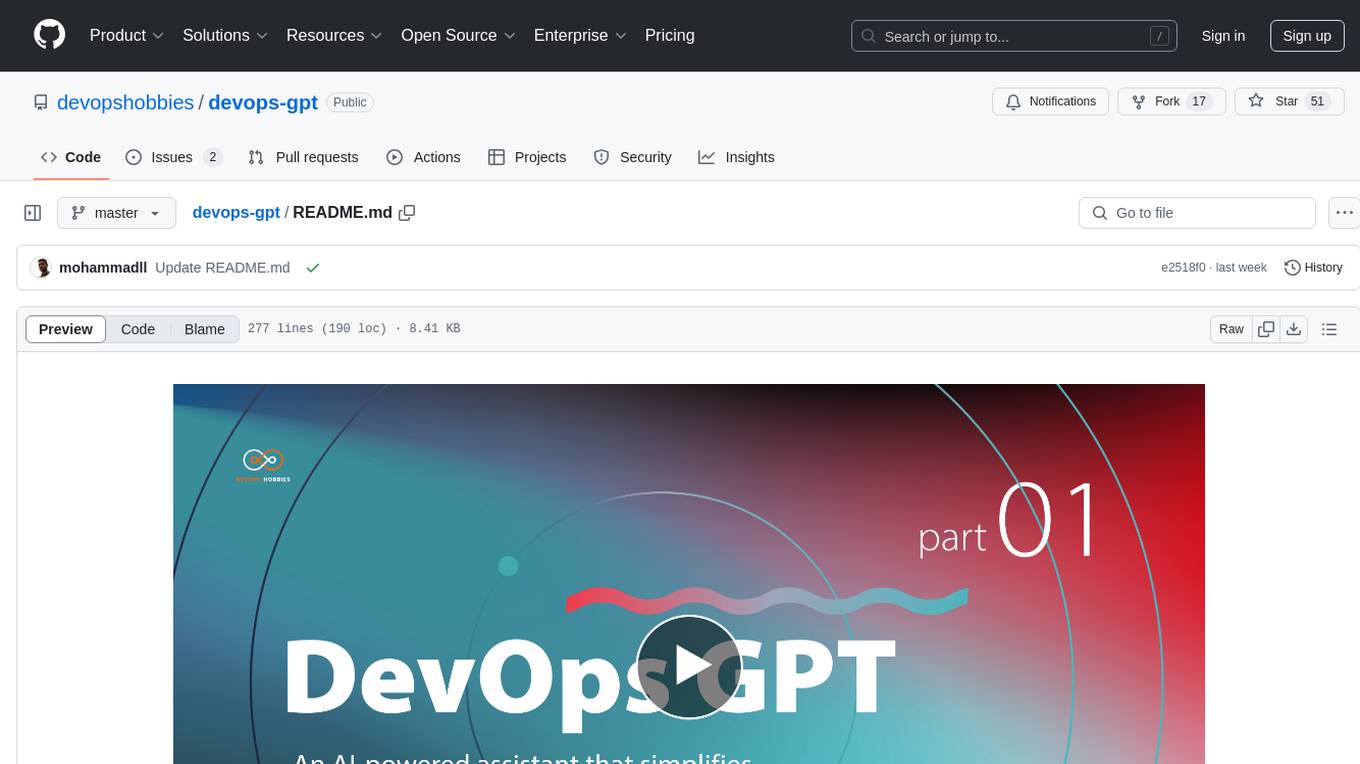
devops-gpt
DevOpsGPT is a revolutionary tool designed to streamline your workflow and empower you to build systems and automate tasks with ease. Tired of spending hours on repetitive DevOps tasks? DevOpsGPT is here to help! Whether you're setting up infrastructure, speeding up deployments, or tackling any other DevOps challenge, our app can make your life easier and more productive. With DevOpsGPT, you can expect faster task completion, simplified workflows, and increased efficiency. Ready to experience the DevOpsGPT difference? Visit our website, sign in or create an account, start exploring the features, and share your feedback to help us improve. DevOpsGPT will become an essential tool in your DevOps toolkit.
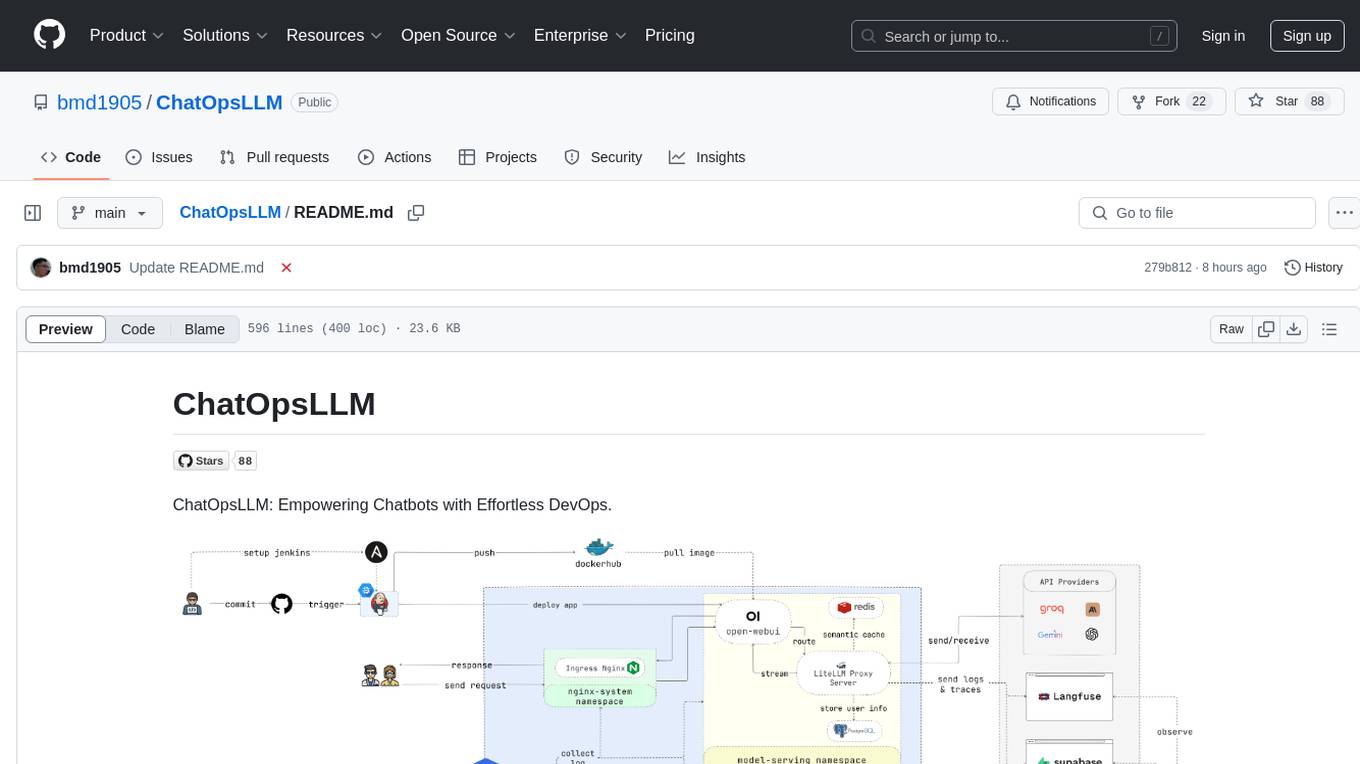
ChatOpsLLM
ChatOpsLLM is a project designed to empower chatbots with effortless DevOps capabilities. It provides an intuitive interface and streamlined workflows for managing and scaling language models. The project incorporates robust MLOps practices, including CI/CD pipelines with Jenkins and Ansible, monitoring with Prometheus and Grafana, and centralized logging with the ELK stack. Developers can find detailed documentation and instructions on the project's website.
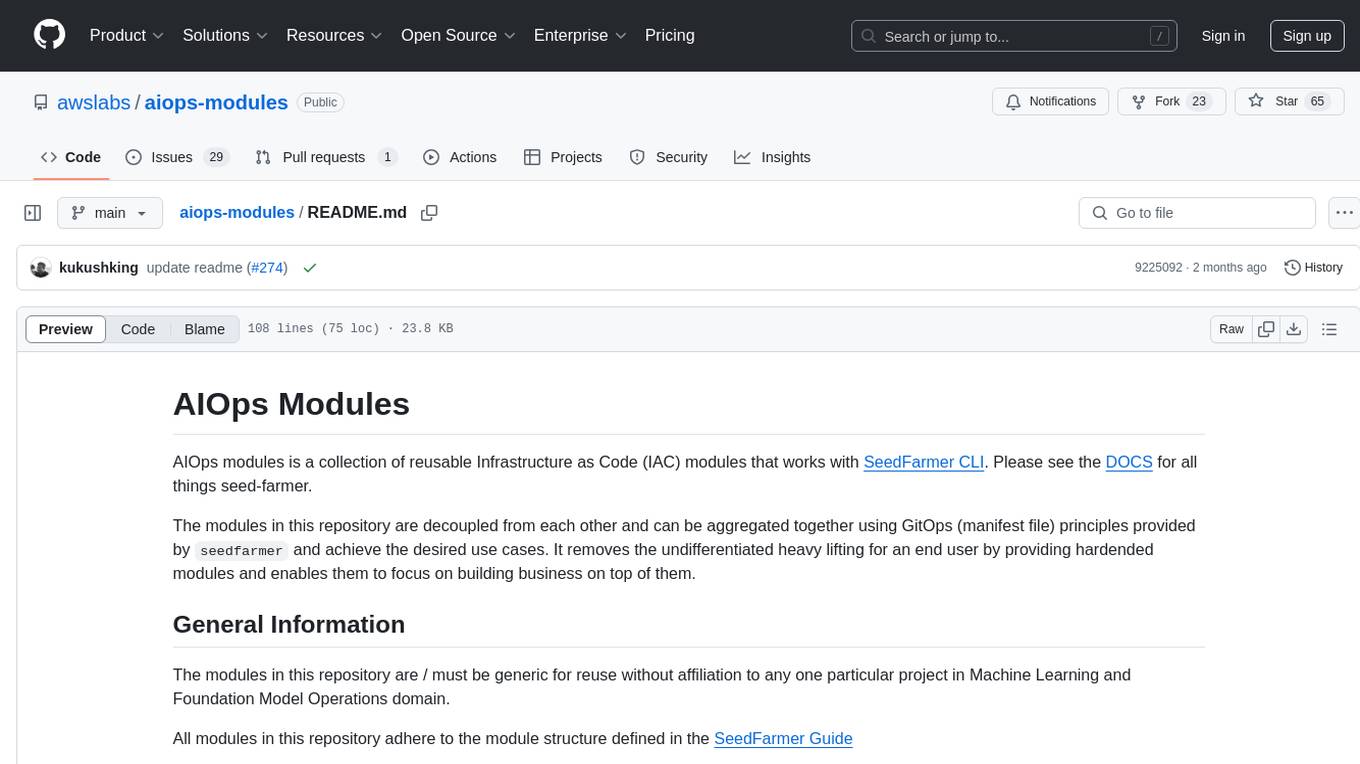
aiops-modules
AIOps Modules is a collection of reusable Infrastructure as Code (IAC) modules that work with SeedFarmer CLI. The modules are decoupled and can be aggregated using GitOps principles to achieve desired use cases, removing heavy lifting for end users. They must be generic for reuse in Machine Learning and Foundation Model Operations domain, adhering to SeedFarmer Guide structure. The repository includes deployment steps, project manifests, and various modules for SageMaker, Mlflow, FMOps/LLMOps, MWAA, Step Functions, EKS, and example use cases. It also supports Industry Data Framework (IDF) and Autonomous Driving Data Framework (ADDF) Modules.
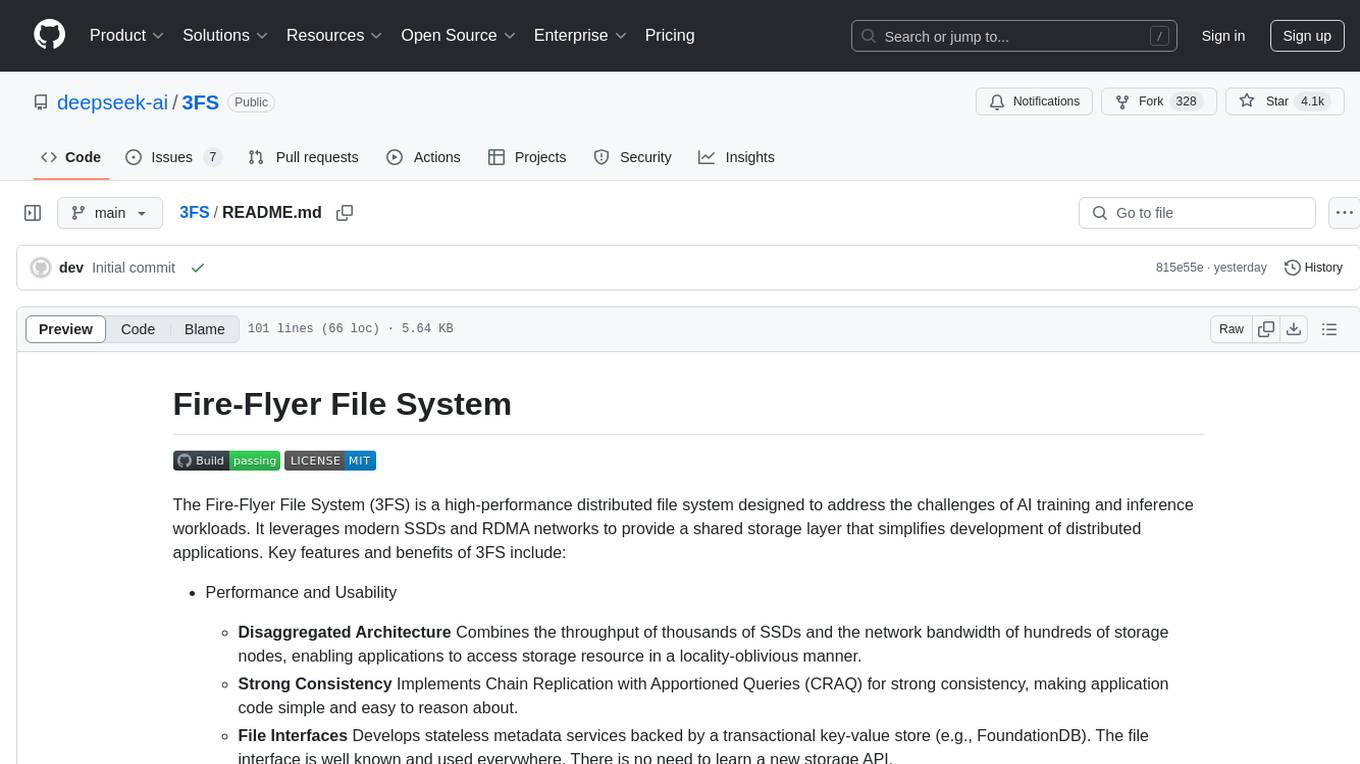
3FS
The Fire-Flyer File System (3FS) is a high-performance distributed file system designed for AI training and inference workloads. It leverages modern SSDs and RDMA networks to provide a shared storage layer that simplifies development of distributed applications. Key features include performance, disaggregated architecture, strong consistency, file interfaces, data preparation, dataloaders, checkpointing, and KVCache for inference. The system is well-documented with design notes, setup guide, USRBIO API reference, and P specifications. Performance metrics include peak throughput, GraySort benchmark results, and KVCache optimization. The source code is available on GitHub for cloning and installation of dependencies. Users can build 3FS and run test clusters following the provided instructions. Issues can be reported on the GitHub repository.