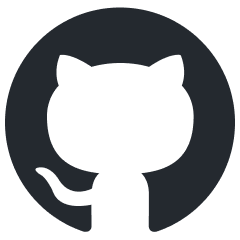
elyra
Elyra extends JupyterLab with an AI centric approach.
Stars: 1888
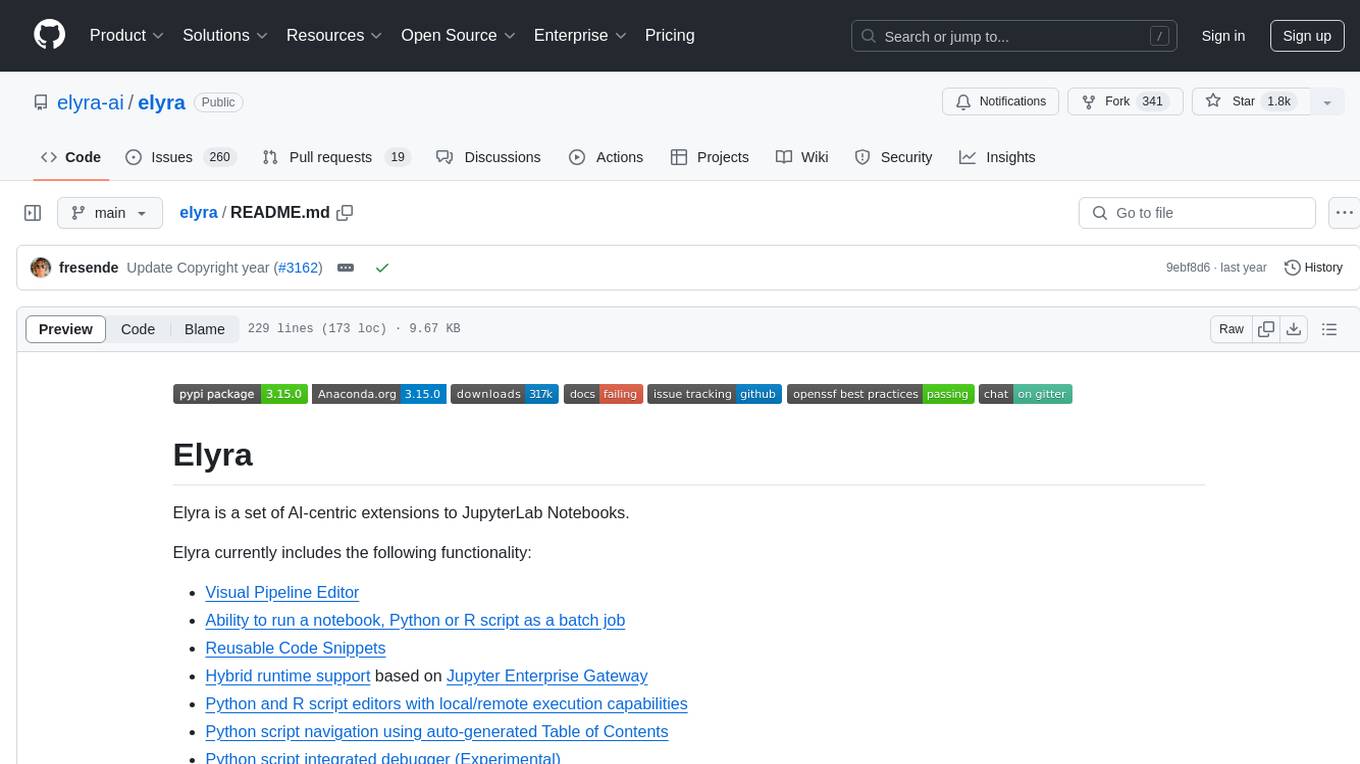
Elyra is a set of AI-centric extensions to JupyterLab Notebooks that includes features like Visual Pipeline Editor, running notebooks/scripts as batch jobs, reusable code snippets, hybrid runtime support, script editors with execution capabilities, debugger, version control using Git, and more. It provides a comprehensive environment for data scientists and AI practitioners to develop, test, and deploy machine learning models and workflows efficiently.
README:
Elyra is a set of AI-centric extensions to JupyterLab Notebooks.
Elyra currently includes the following functionality:
- Visual Pipeline Editor
- Ability to run a notebook, Python or R script as a batch job
- Reusable Code Snippets
- Hybrid runtime support based on Jupyter Enterprise Gateway
- Python and R script editors with local/remote execution capabilities
- Python script navigation using auto-generated Table of Contents
- Python script integrated debugger (Experimental)
- Notebook navigation using auto-generated outlines using Table of Contents
- Language Server Protocol integration
- Version control using Git integration
The Elyra Getting Started Guide includes more details on these features. A version-specific summary of new features is located on the releases page.
You can also try Elyra by running one of the container images from Docker Hub or quay.io:
-
elyra/elyra:latest
has the latest released version installed. -
elyra/elyra:x.y.z
has a specific version installed.
Note: You can also build a container image from the main
branch ("dev build") to try out features that have not been released yet.
To run one of the container images, issue the following command, specifying a tag of your choice.
docker run -it -p 8888:8888 elyra/elyra:dev jupyter lab --debug
To make a local directory containing your Notebooks (e.g. ${HOME}/opensource/jupyter-notebooks/) available in your docker container, you can use a mount command similar to the following:
docker run -it -p 8888:8888 -v ${HOME}/opensource/jupyter-notebooks/:/home/jovyan/work -w /home/jovyan/work elyra/elyra:dev jupyter lab --debug
These should produce output similar to that below, where you can then find the URL to be used to access Elyra in your local browser.
To access the notebook, open this file in a browser:
file:///home/jovyan/.local/share/jupyter/runtime/nbserver-6-open.html
Or copy and paste one of these URLs:
http://4d17829ecd4c:8888/?token=d690bde267ec75d6f88c64a39825f8b05b919dd084451f82
or http://127.0.0.1:8888/?token=d690bde267ec75d6f88c64a39825f8b05b919dd084451f82
Refer to the installation documentation for details.
For detailed information refer to the installation documentation.
- Node.js 22
- Python 3.9+
- Miniconda / Micromamba (optional)
The current release version is displayed at the top of this page.
-
Install from PyPI
pip3 install --upgrade "elyra[all]"
-
Install from conda-forge
conda install -c conda-forge "elyra[all]"
Installation instructions and JupyterLab support vary by release. Note that a JupyterLab build is required. Installation instructions are located in the release-specific documentation, which can be accessed by selecting a specific version.
Elyra 4.x (JupyterLab 4.2.5+)
-
Install from PyPI
pip3 install --upgrade "elyra[all]"
-
Install from conda-forge
conda install -c conda-forge "elyra[all]"
Elyra 3.7 < 4.0 (JupyterLab 3.x)
-
Install from PyPI
pip3 install --upgrade "elyra[all]<4.0.0"
-
Install from conda-forge
conda install -c conda-forge "elyra[all]<4.0.0"
Elyra 3.1 < 3.7 (JupyterLab 3.x)
-
Install from PyPI
pip3 install --upgrade "elyra[all]>=3.1.0" && jupyter lab build
-
Install from conda-forge
conda install -c conda-forge "elyra[all]>=3.1.0" && jupyter lab build
Elyra 2.0 < 3.1 (JupyterLab 3.x)
-
Install from PyPI
pip3 install --upgrade "elyra>=2.0.1" && jupyter lab build
-
Install from conda-forge
conda install -c conda-forge "elyra>=2.0.1" && jupyter lab build
Run the following commands to verify the installation. Note that in the example output below the [version]
placeholder is displayed instead of an actual version identifier, which might change with every release.
jupyter server extension list
Should output:
Config dir: /.../.jupyter
Config dir: /.../etc/jupyter
elyra enabled
- Validating elyra...
elyra OK
jupyter_lsp enabled
- Validating jupyter_lsp...
jupyter_lsp [version] OK
jupyter_resource_usage enabled
- Validating jupyter_resource_usage...
jupyter_resource_usage [version] OK
jupyter_server_mathjax enabled
- Validating jupyter_server_mathjax...
jupyter_server_mathjax OK
jupyterlab enabled
- Validating jupyterlab...
jupyterlab [version] OK
jupyterlab_git enabled
- Validating jupyterlab_git...
jupyterlab_git [version] OK
nbclassic enabled
- Validating nbclassic...
nbclassic OK
nbdime enabled
- Validating nbdime...
nbdime [version] OK
Config dir: /.../etc/jupyter
NOTE: If you don't see the Elyra server extension enabled, you may need to explicitly enable
it with jupyter server extension enable elyra
jupyter labextension list
Should output:
JupyterLab [version]
/.../share/jupyter/labextensions
nbdime-jupyterlab [version] enabled OK
@jupyter-server/resource-usage [version] enabled OK (python, jupyter-resource-usage)
@krassowski/jupyterlab-lsp [version] enabled OK (python, jupyterlab_lsp)
@elyra/code-snippet-extension [version] enabled OK
@elyra/code-viewer-extension [version] enabled OK
@elyra/metadata-extension [version] enabled OK
@elyra/pipeline-editor-extension [version] enabled OK
@elyra/python-editor-extension [version] enabled OK
@elyra/scala-editor-extension [version] enabled OK
@elyra/r-editor-extension [version] enabled OK
@elyra/theme-extension [version] enabled OK
@jupyterlab/git [version] enabled OK (python, jupyterlab-git)
Other labextensions (built into JupyterLab)
app dir: /.../share/jupyter/lab
After verifying Elyra has been installed, start Elyra with:
jupyter lab
We welcome your questions, ideas, and feedback. Check the Getting Help
section in the Getting Started guide
to learn more about the channels you can use to get in touch with us.
If you are interested in helping make Elyra better, we encourage you to take a look at our Contributing page, Development Workflow documentation, and invite you to attend our weekly dev community meetings.
Our daily and weekly community meeting schedule can be found here.
For Tasks:
Click tags to check more tools for each tasksFor Jobs:
Alternative AI tools for elyra
Similar Open Source Tools
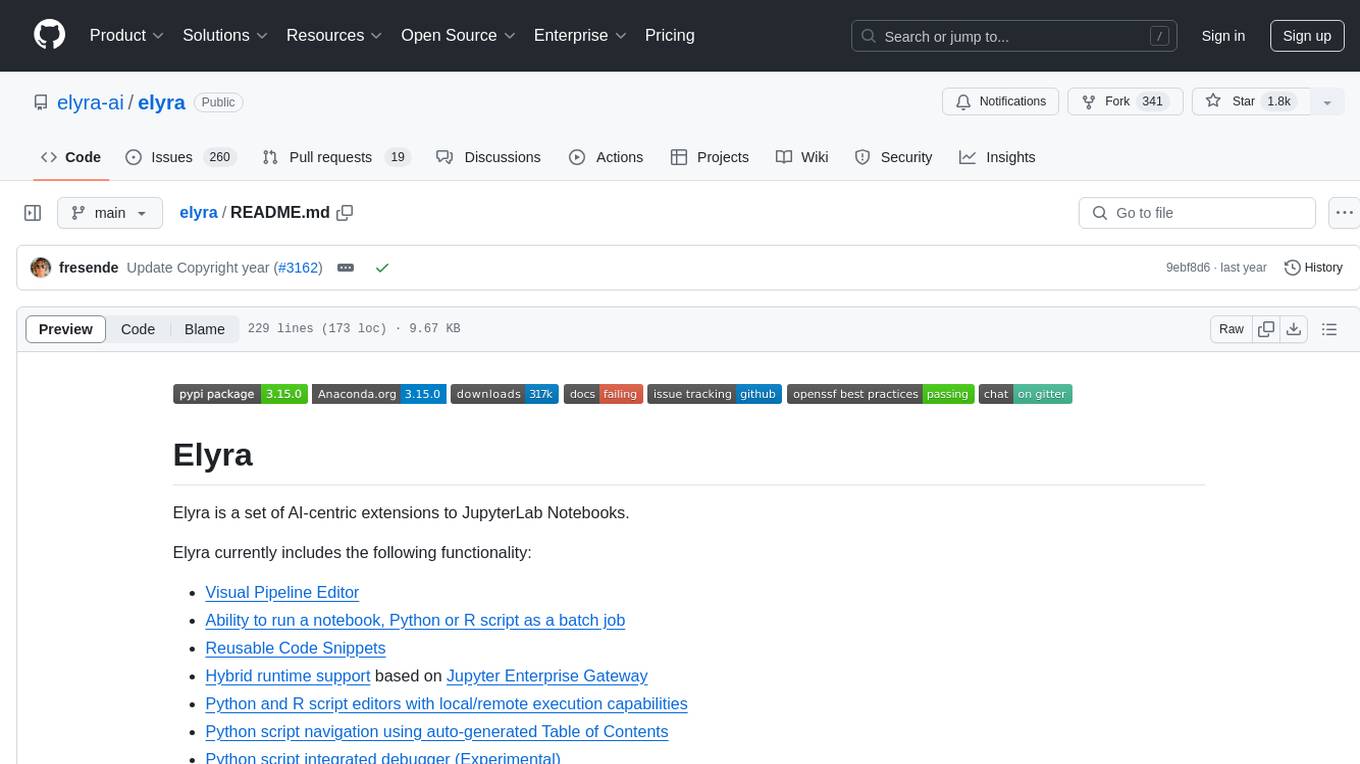
elyra
Elyra is a set of AI-centric extensions to JupyterLab Notebooks that includes features like Visual Pipeline Editor, running notebooks/scripts as batch jobs, reusable code snippets, hybrid runtime support, script editors with execution capabilities, debugger, version control using Git, and more. It provides a comprehensive environment for data scientists and AI practitioners to develop, test, and deploy machine learning models and workflows efficiently.
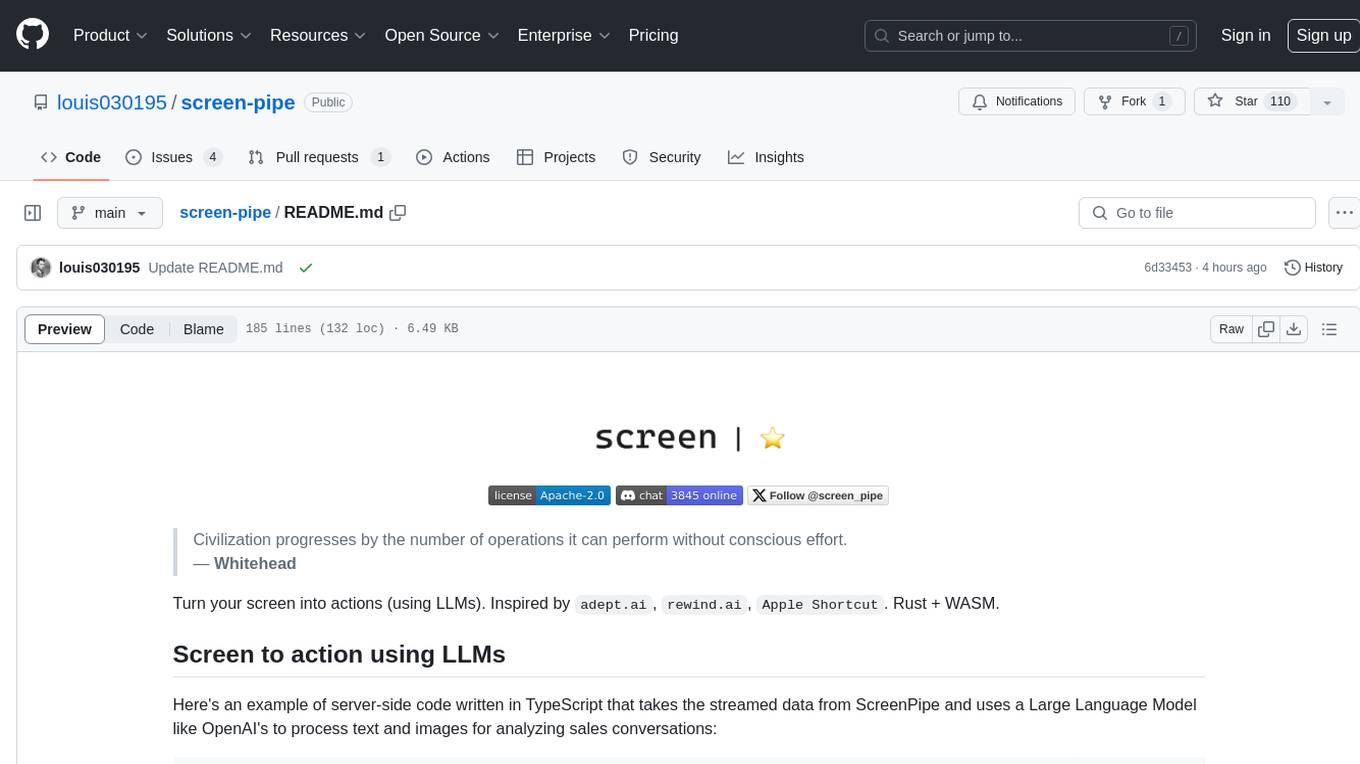
screen-pipe
Screen-pipe is a Rust + WASM tool that allows users to turn their screen into actions using Large Language Models (LLMs). It enables users to record their screen 24/7, extract text from frames, and process text and images for tasks like analyzing sales conversations. The tool is still experimental and aims to simplify the process of recording screens, extracting text, and integrating with various APIs for tasks such as filling CRM data based on screen activities. The project is open-source and welcomes contributions to enhance its functionalities and usability.
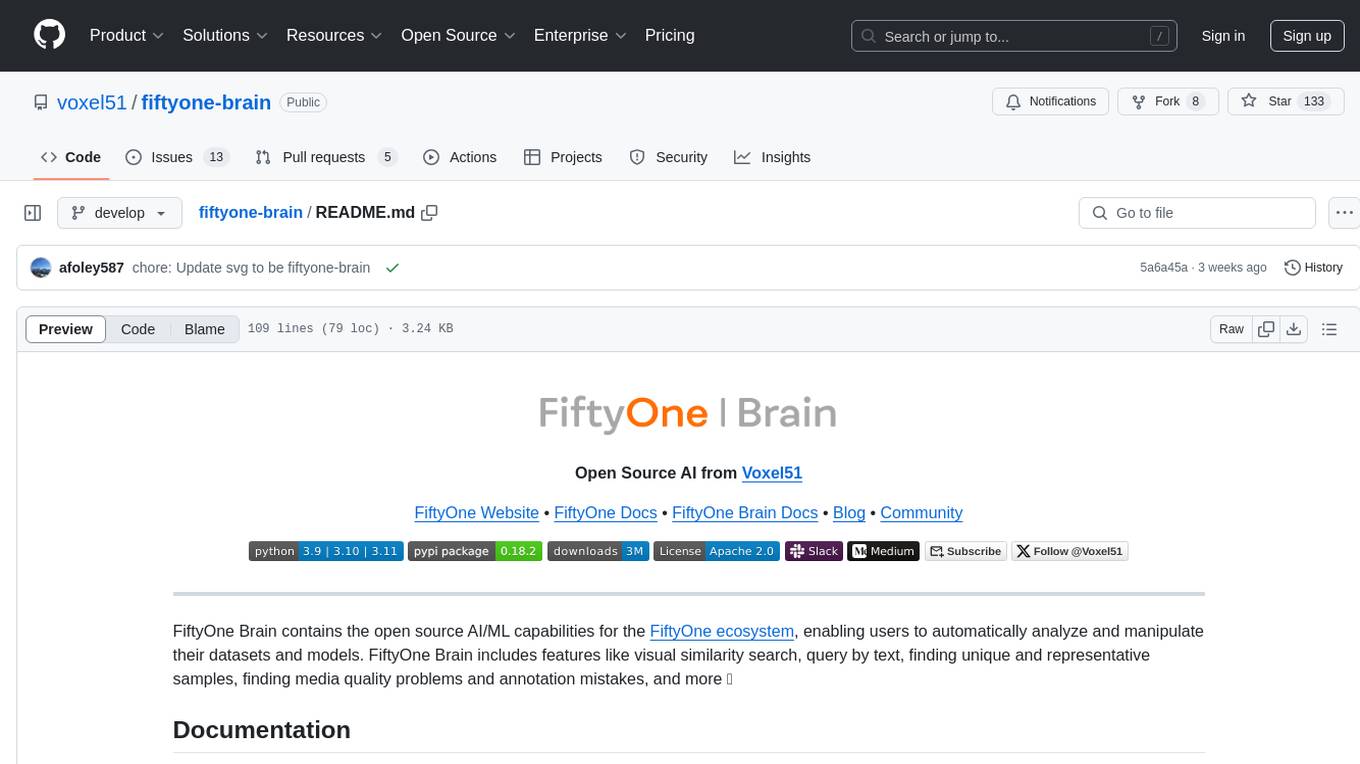
fiftyone-brain
FiftyOne Brain contains the open source AI/ML capabilities for the FiftyOne ecosystem, enabling users to automatically analyze and manipulate their datasets and models. Features include visual similarity search, query by text, finding unique and representative samples, finding media quality problems and annotation mistakes, and more.
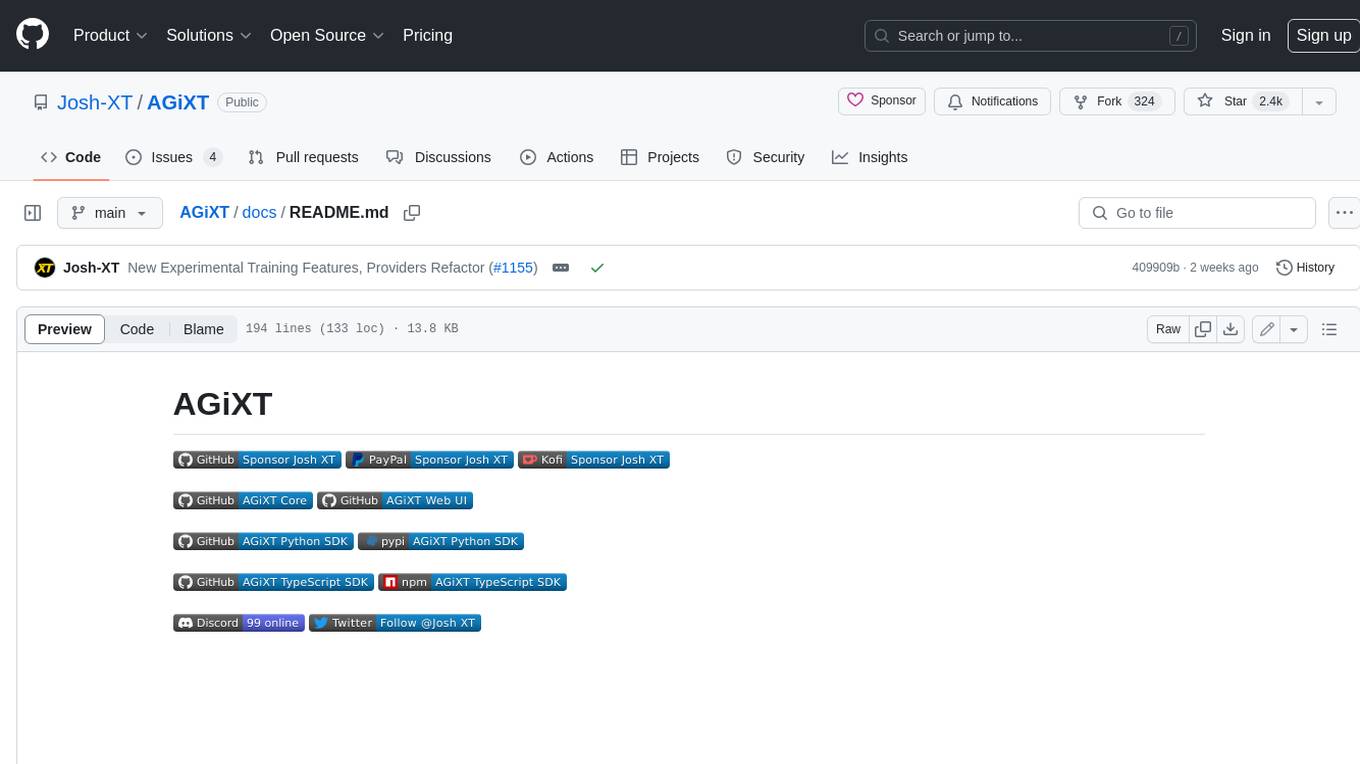
AGiXT
AGiXT is a dynamic Artificial Intelligence Automation Platform engineered to orchestrate efficient AI instruction management and task execution across a multitude of providers. Our solution infuses adaptive memory handling with a broad spectrum of commands to enhance AI's understanding and responsiveness, leading to improved task completion. The platform's smart features, like Smart Instruct and Smart Chat, seamlessly integrate web search, planning strategies, and conversation continuity, transforming the interaction between users and AI. By leveraging a powerful plugin system that includes web browsing and command execution, AGiXT stands as a versatile bridge between AI models and users. With an expanding roster of AI providers, code evaluation capabilities, comprehensive chain management, and platform interoperability, AGiXT is consistently evolving to drive a multitude of applications, affirming its place at the forefront of AI technology.
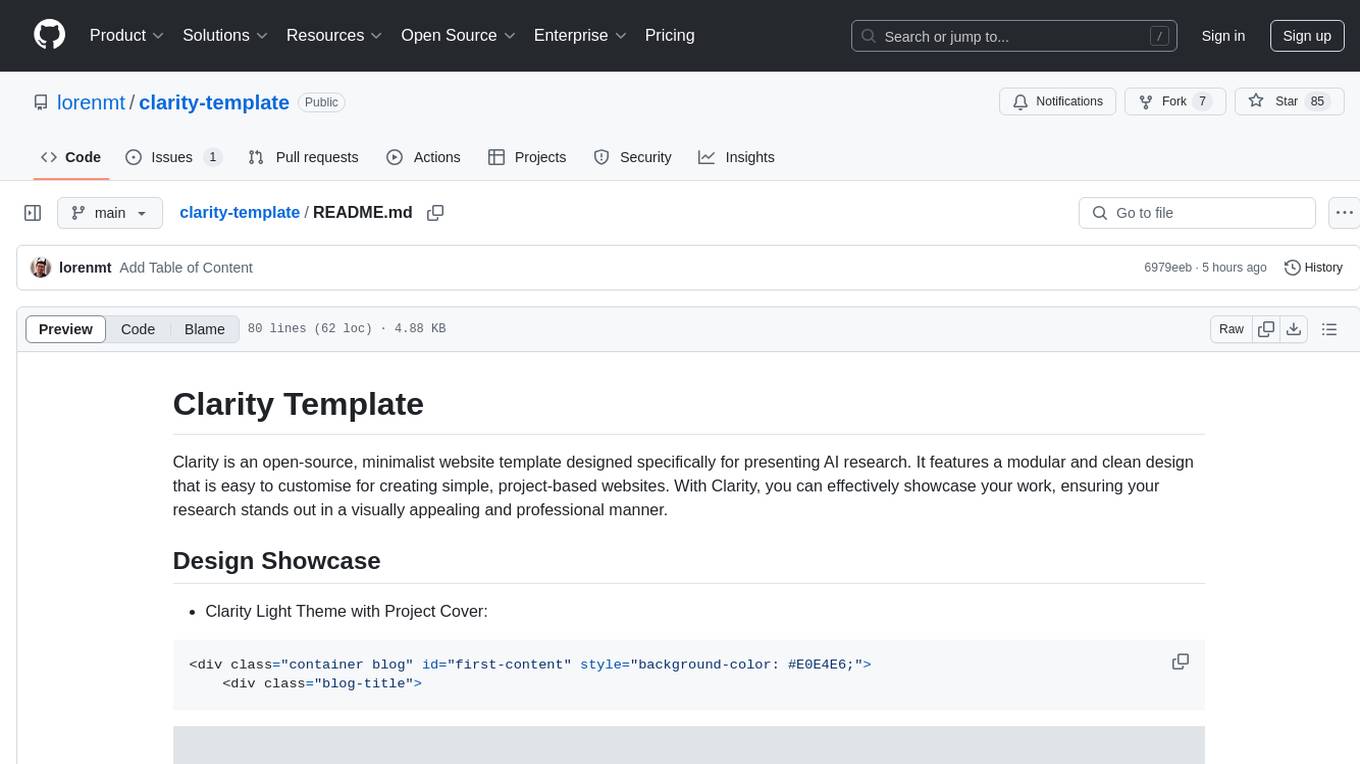
clarity-template
Clarity Template is an open-source, minimalist website template tailored for presenting AI research. It offers a modular and clean design that can be easily customized for creating simple, project-based websites. With Clarity, users can effectively showcase their work in a visually appealing and professional manner. The template includes various design themes and features like navbar design, slideshow display, title page layout, and comparison display. Users can follow the visual guidelines provided in the Clarity blog post or clarity.html file to optimize visual layouts and design. Clarity offers two font options: free fonts (Charter and Poppins) and licensed fonts (Tiempos Text and Athletics) with trial licenses available for non-commercial use. Users can switch between font options by downloading and placing font files in the assets/fonts directory, updating font settings in the stylesheet, and ensuring compatibility across different browsers using a web font converter.
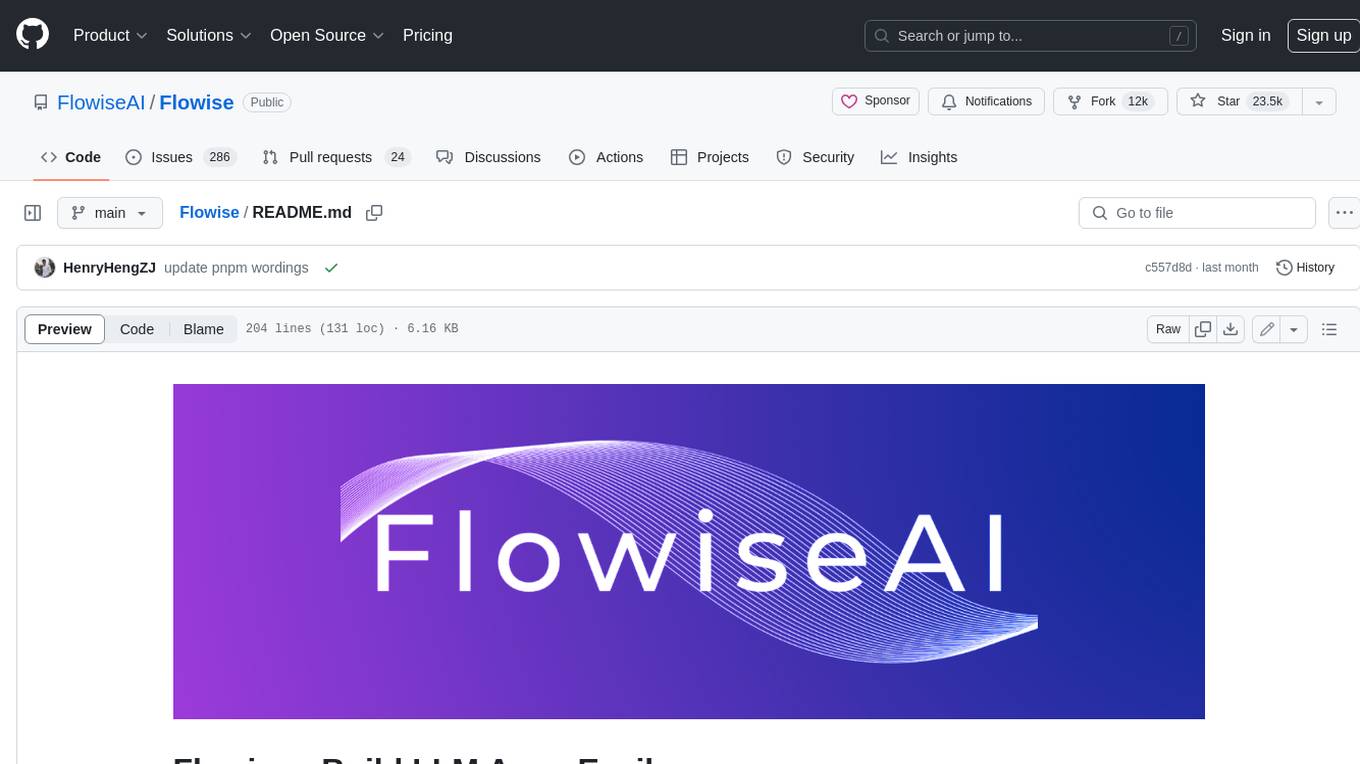
Flowise
Flowise is a tool that allows users to build customized LLM flows with a drag-and-drop UI. It is open-source and self-hostable, and it supports various deployments, including AWS, Azure, Digital Ocean, GCP, Railway, Render, HuggingFace Spaces, Elestio, Sealos, and RepoCloud. Flowise has three different modules in a single mono repository: server, ui, and components. The server module is a Node backend that serves API logics, the ui module is a React frontend, and the components module contains third-party node integrations. Flowise supports different environment variables to configure your instance, and you can specify these variables in the .env file inside the packages/server folder.
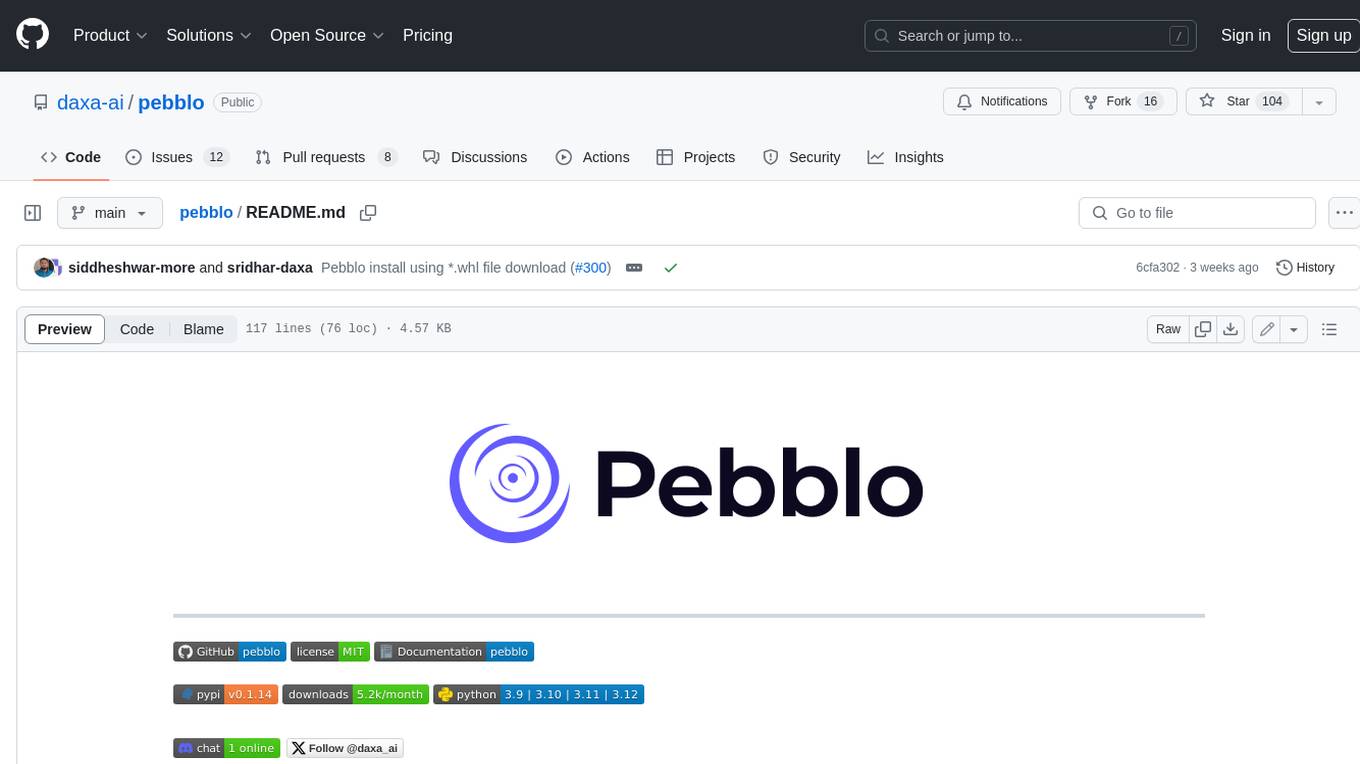
pebblo
Pebblo enables developers to safely load data and promote their Gen AI app to deployment without worrying about the organization’s compliance and security requirements. The project identifies semantic topics and entities found in the loaded data and summarizes them on the UI or a PDF report.
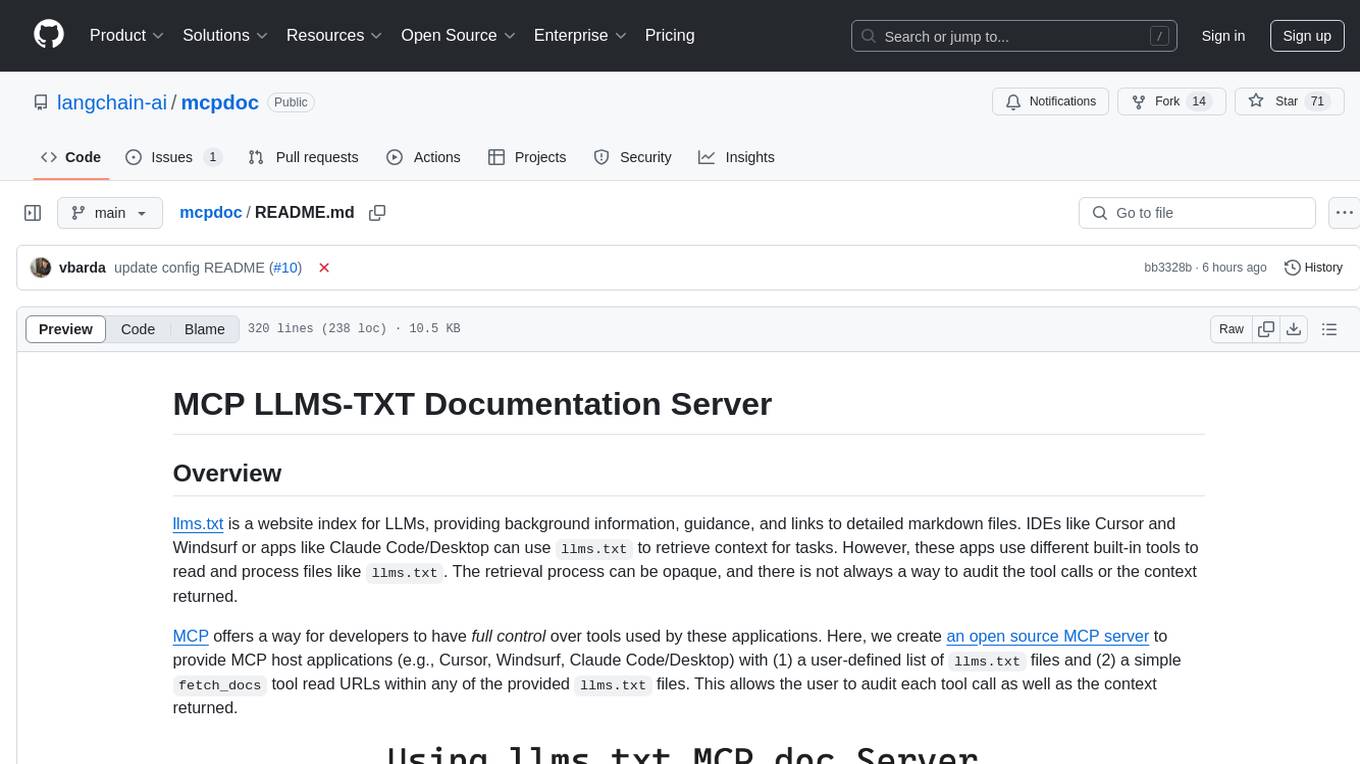
mcpdoc
The MCP LLMS-TXT Documentation Server is an open-source server that provides developers full control over tools used by applications like Cursor, Windsurf, and Claude Code/Desktop. It allows users to create a user-defined list of `llms.txt` files and use a `fetch_docs` tool to read URLs within these files, enabling auditing of tool calls and context returned. The server supports various applications and provides a way to connect to them, configure rules, and test tool calls for tasks related to documentation retrieval and processing.
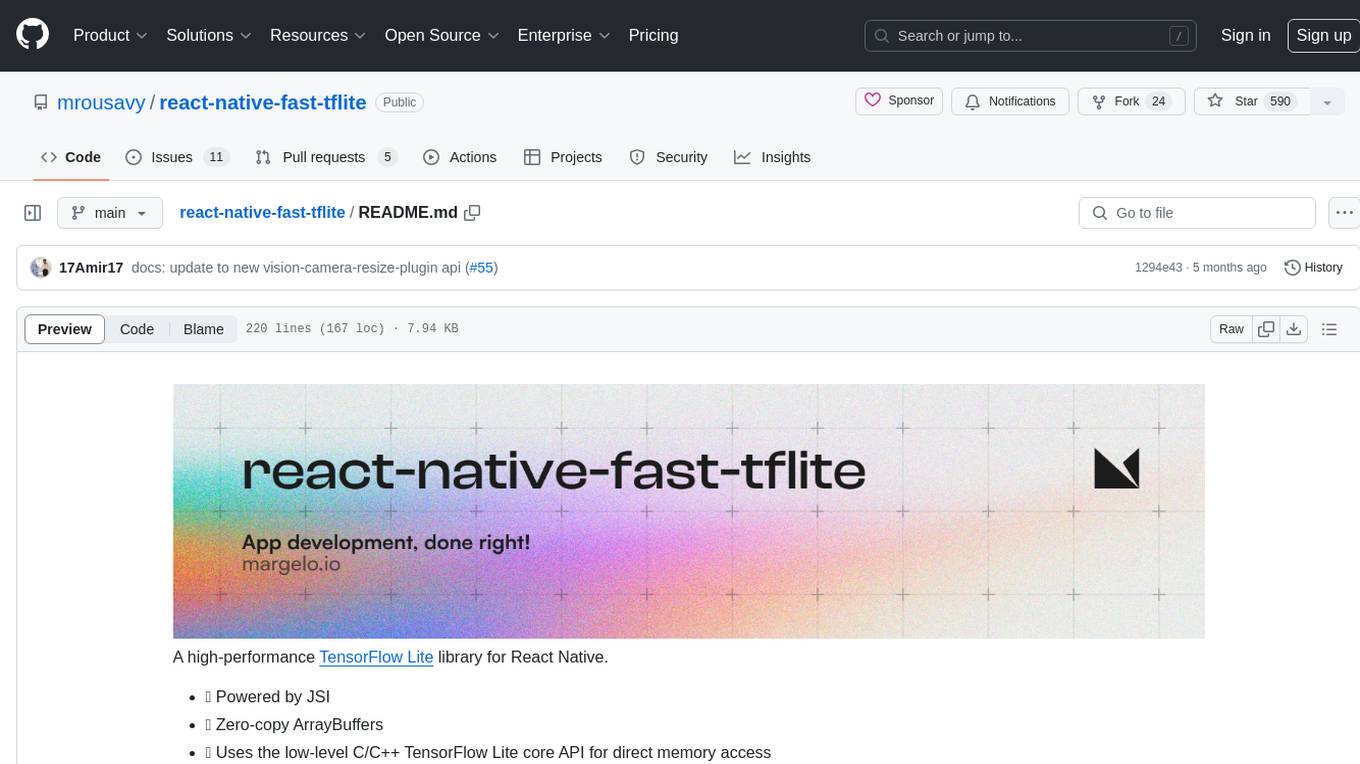
react-native-fast-tflite
A high-performance TensorFlow Lite library for React Native that utilizes JSI for power, zero-copy ArrayBuffers for efficiency, and low-level C/C++ TensorFlow Lite core API for direct memory access. It supports swapping out TensorFlow Models at runtime and GPU-accelerated delegates like CoreML/Metal/OpenGL. Easy VisionCamera integration allows for seamless usage. Users can load TensorFlow Lite models, interpret input and output data, and utilize GPU Delegates for faster computation. The library is suitable for real-time object detection, image classification, and other machine learning tasks in React Native applications.
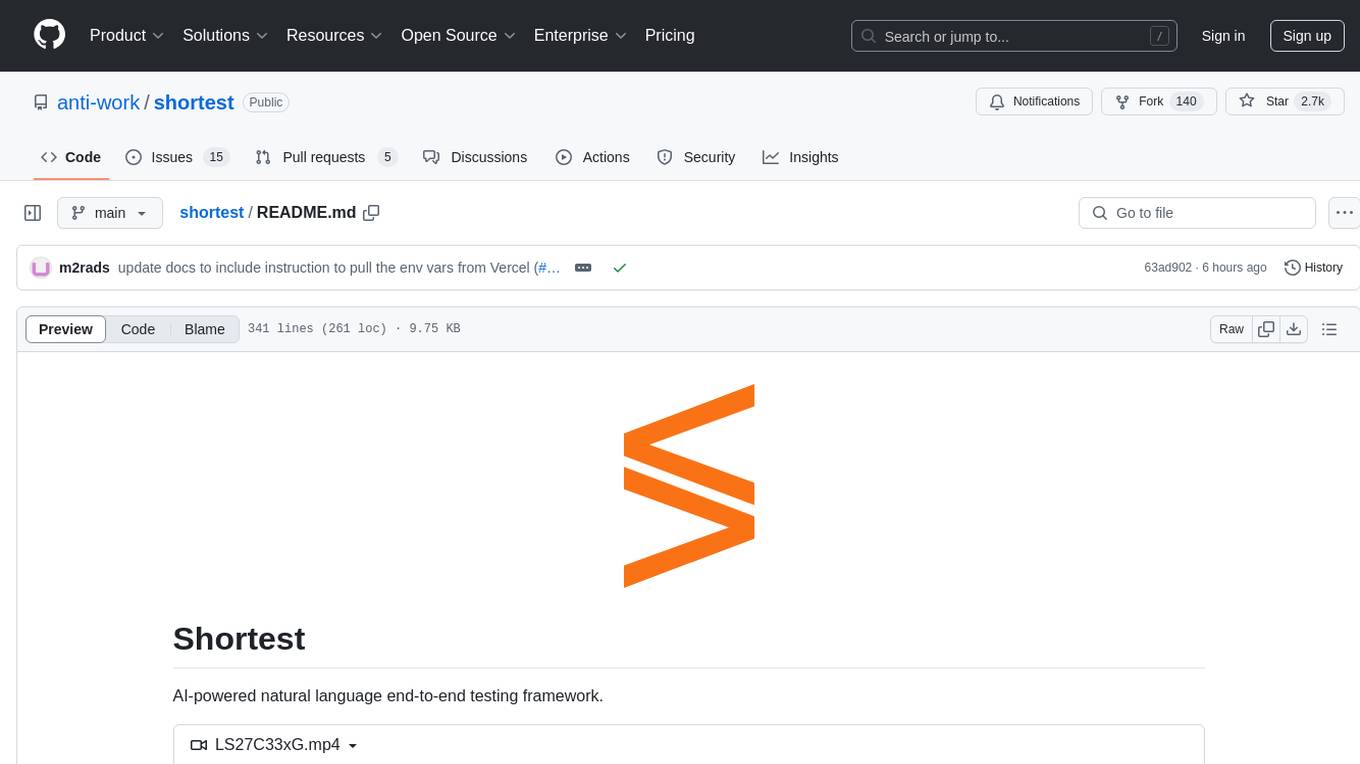
shortest
Shortest is an AI-powered natural language end-to-end testing framework built on Playwright. It provides a seamless testing experience by allowing users to write tests in natural language and execute them using Anthropic Claude API. The framework also offers GitHub integration with 2FA support, making it suitable for testing web applications with complex authentication flows. Shortest simplifies the testing process by enabling users to run tests locally or in CI/CD pipelines, ensuring the reliability and efficiency of web applications.
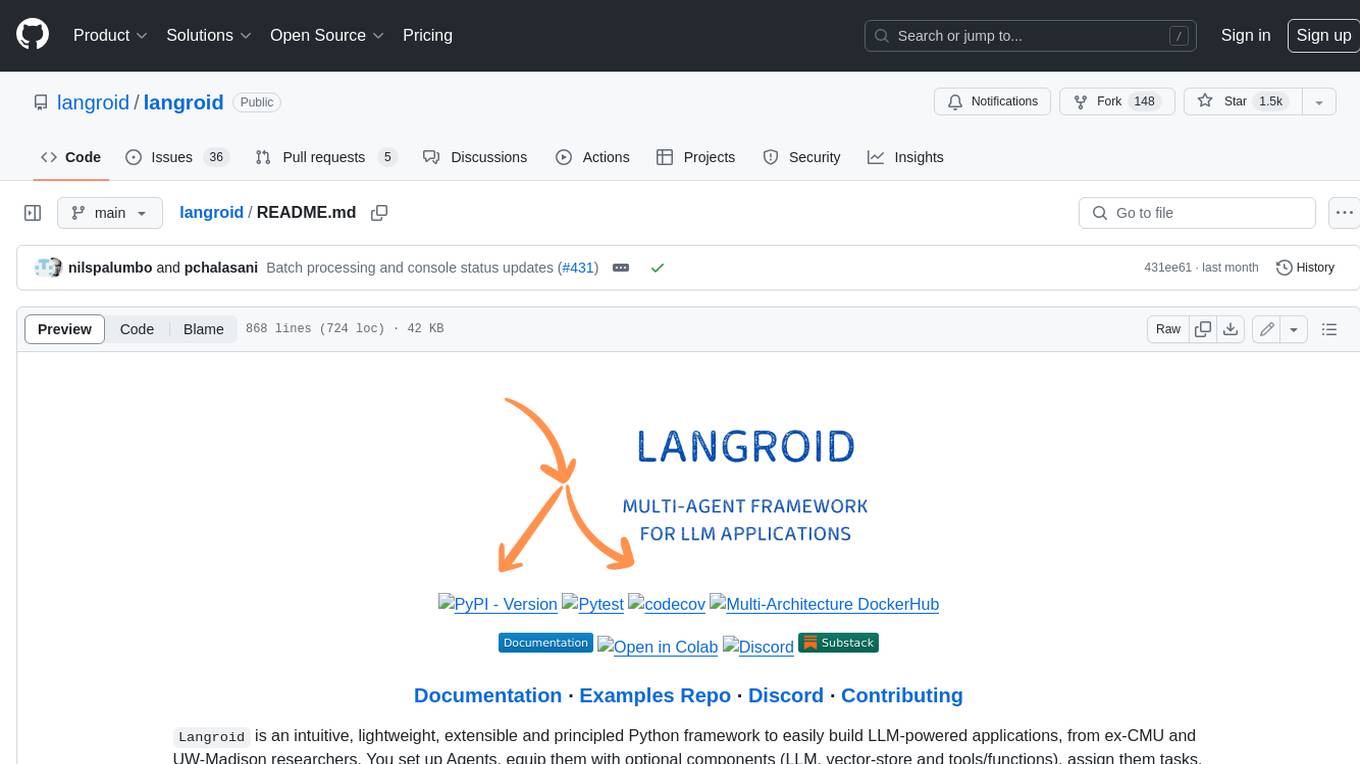
langroid
Langroid is a Python framework that makes it easy to build LLM-powered applications. It uses a multi-agent paradigm inspired by the Actor Framework, where you set up Agents, equip them with optional components (LLM, vector-store and tools/functions), assign them tasks, and have them collaboratively solve a problem by exchanging messages. Langroid is a fresh take on LLM app-development, where considerable thought has gone into simplifying the developer experience; it does not use Langchain.
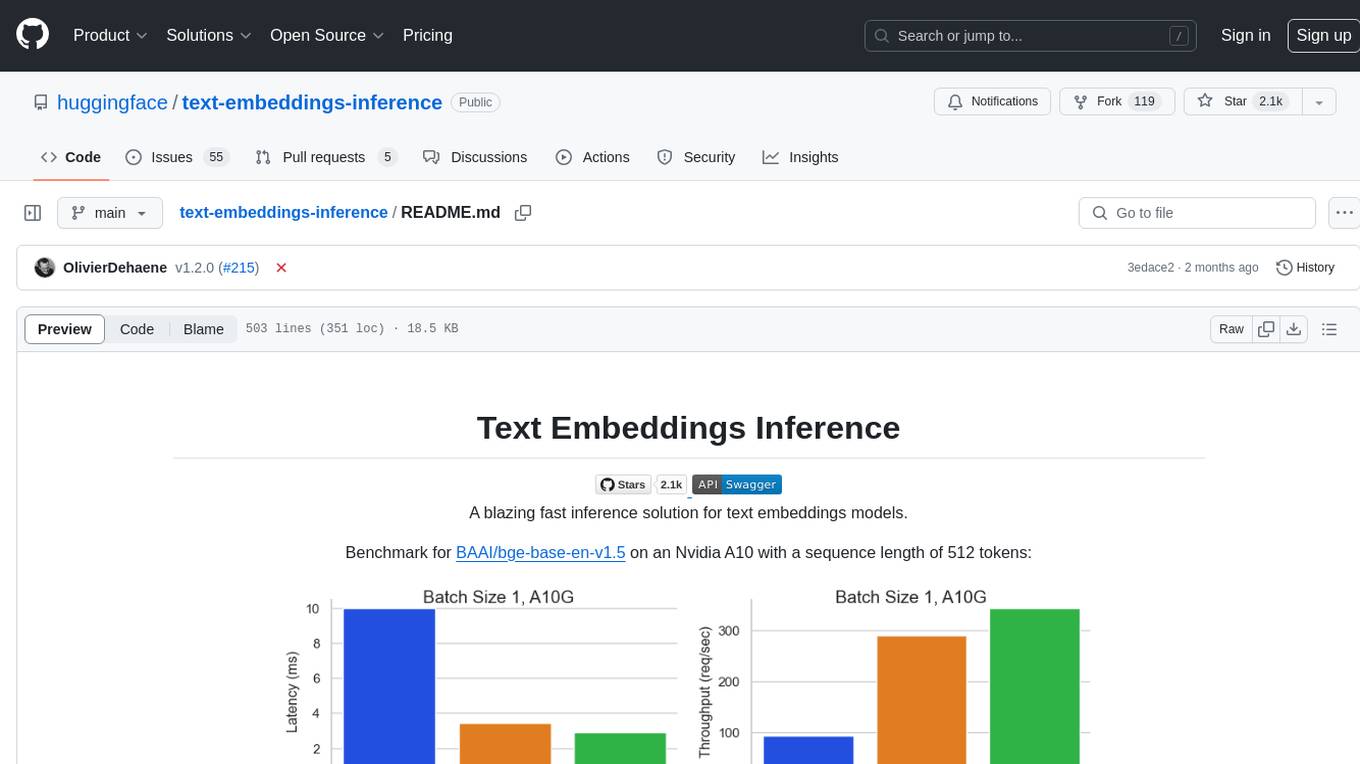
text-embeddings-inference
Text Embeddings Inference (TEI) is a toolkit for deploying and serving open source text embeddings and sequence classification models. TEI enables high-performance extraction for popular models like FlagEmbedding, Ember, GTE, and E5. It implements features such as no model graph compilation step, Metal support for local execution on Macs, small docker images with fast boot times, token-based dynamic batching, optimized transformers code for inference using Flash Attention, Candle, and cuBLASLt, Safetensors weight loading, and production-ready features like distributed tracing with Open Telemetry and Prometheus metrics.
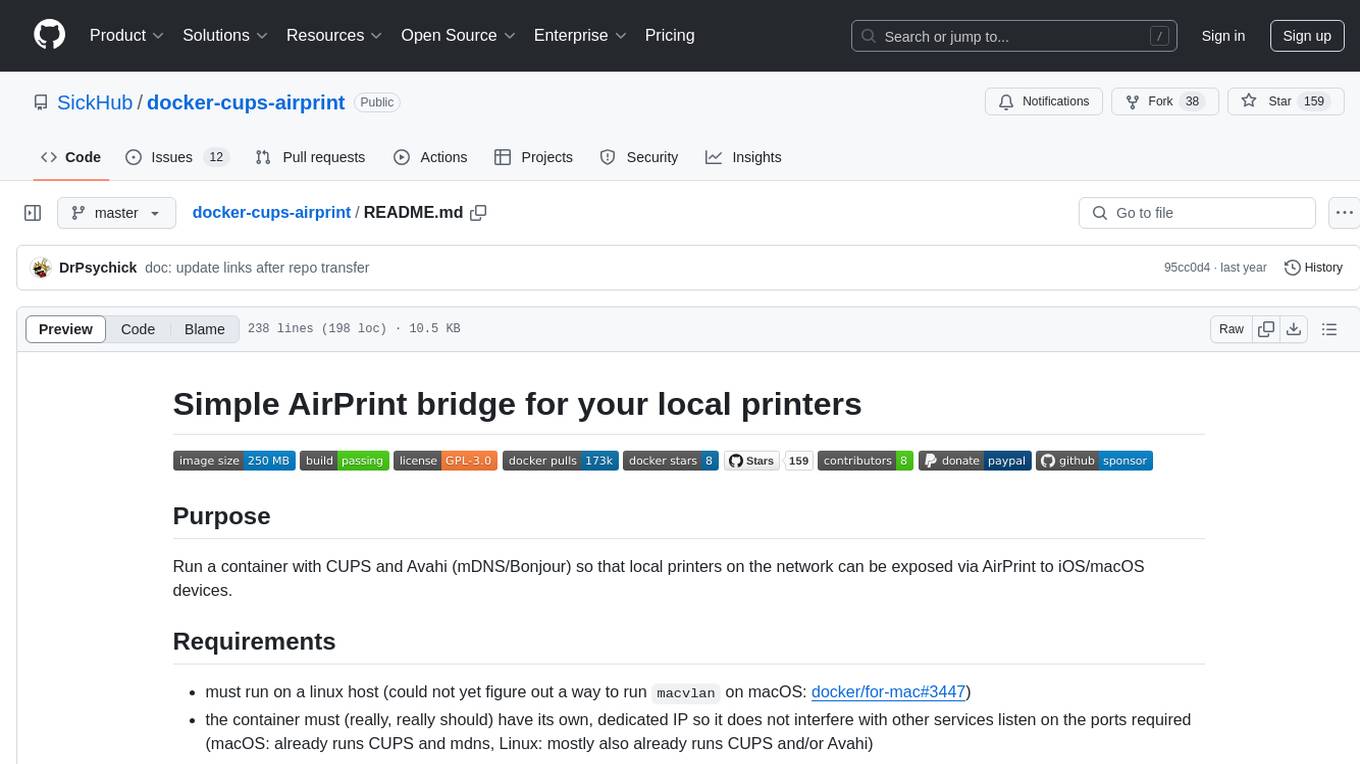
docker-cups-airprint
This repository provides a Docker image that acts as an AirPrint bridge for local printers, allowing them to be exposed to iOS/macOS devices. It runs a container with CUPS and Avahi to facilitate this functionality. Users must have CUPS drivers available for their printers. The tool requires a Linux host and a dedicated IP for the container to avoid interference with other services. It supports setting up printers through environment variables and offers options for automated configuration via command line, web interface, or files. The repository includes detailed instructions on setting up and testing the AirPrint bridge.
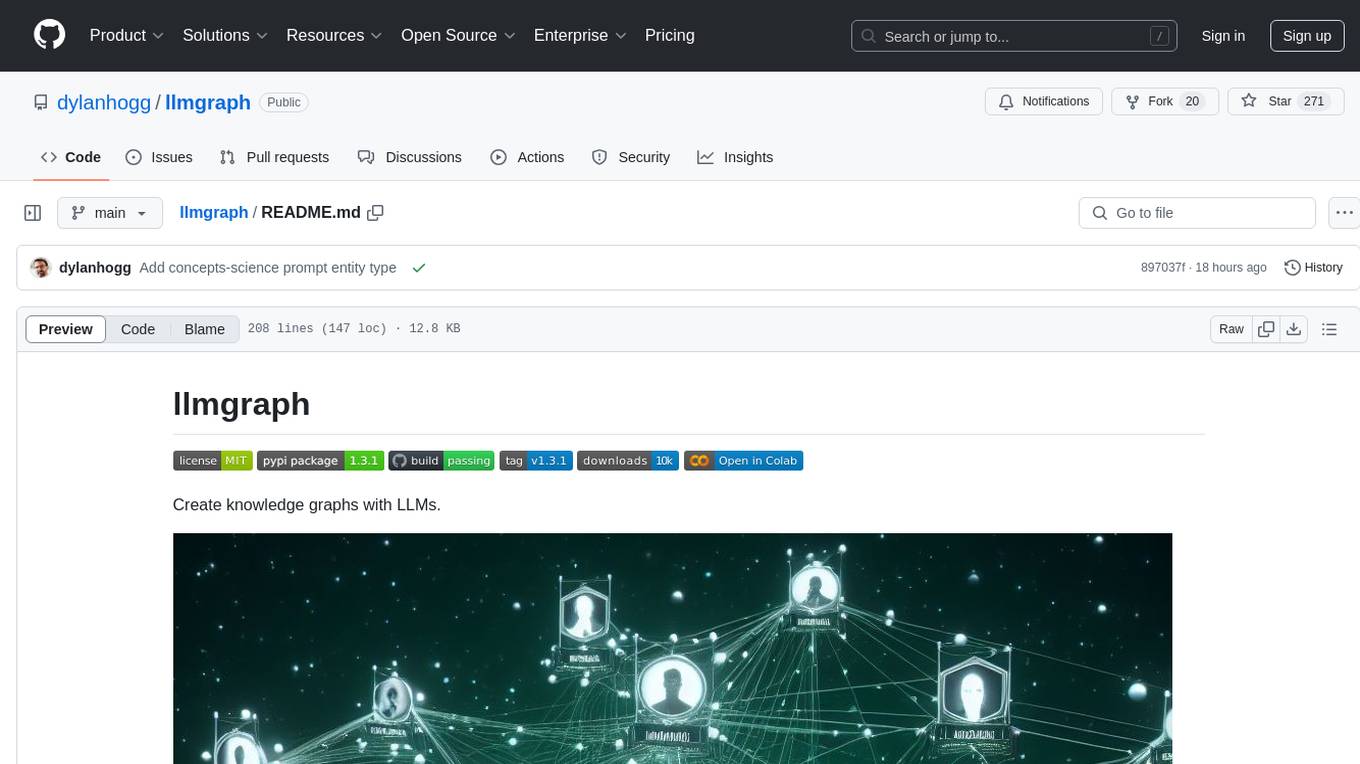
llmgraph
llmgraph is a tool that enables users to create knowledge graphs in GraphML, GEXF, and HTML formats by extracting world knowledge from large language models (LLMs) like ChatGPT. It supports various entity types and relationships, offers cache support for efficient graph growth, and provides insights into LLM costs. Users can customize the model used and interact with different LLM providers. The tool allows users to generate interactive graphs based on a specified entity type and Wikipedia link, making it a valuable resource for knowledge graph creation and exploration.
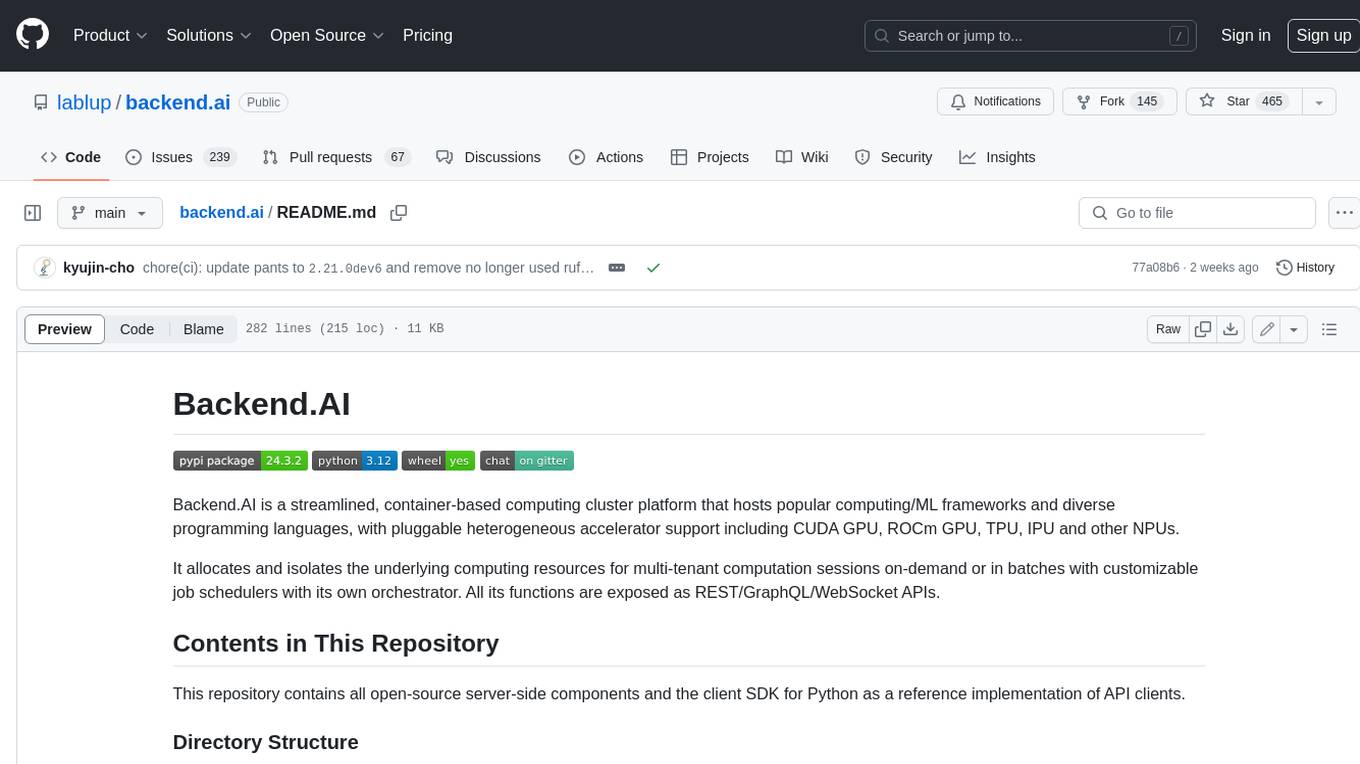
backend.ai
Backend.AI is a streamlined, container-based computing cluster platform that hosts popular computing/ML frameworks and diverse programming languages, with pluggable heterogeneous accelerator support including CUDA GPU, ROCm GPU, TPU, IPU and other NPUs. It allocates and isolates the underlying computing resources for multi-tenant computation sessions on-demand or in batches with customizable job schedulers with its own orchestrator. All its functions are exposed as REST/GraphQL/WebSocket APIs.
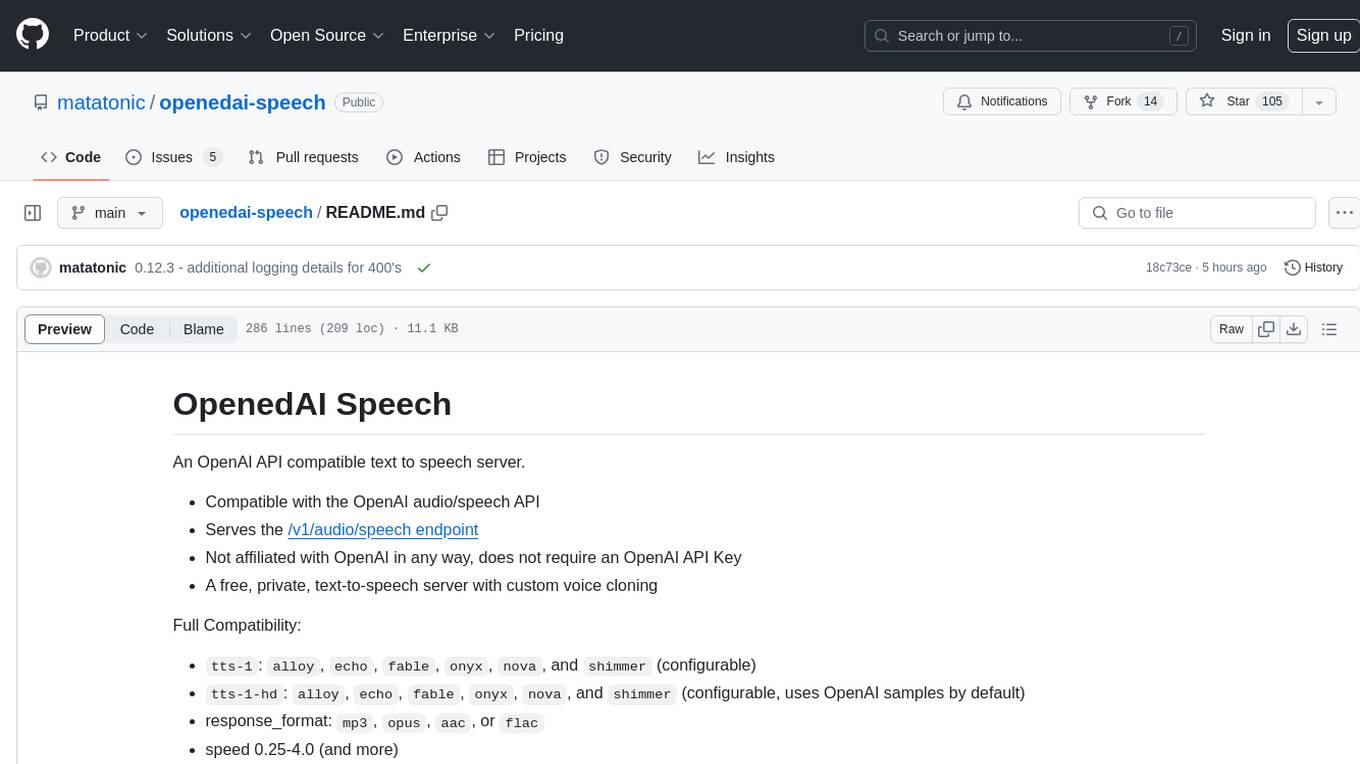
openedai-speech
OpenedAI Speech is a free, private text-to-speech server compatible with the OpenAI audio/speech API. It offers custom voice cloning and supports various models like tts-1 and tts-1-hd. Users can map their own piper voices and create custom cloned voices. The server provides multilingual support with XTTS voices and allows fixing incorrect sounds with regex. Recent changes include bug fixes, improved error handling, and updates for multilingual support. Installation can be done via Docker or manual setup, with usage instructions provided. Custom voices can be created using Piper or Coqui XTTS v2, with guidelines for preparing audio files. The tool is suitable for tasks like generating speech from text, creating custom voices, and multilingual text-to-speech applications.
For similar tasks
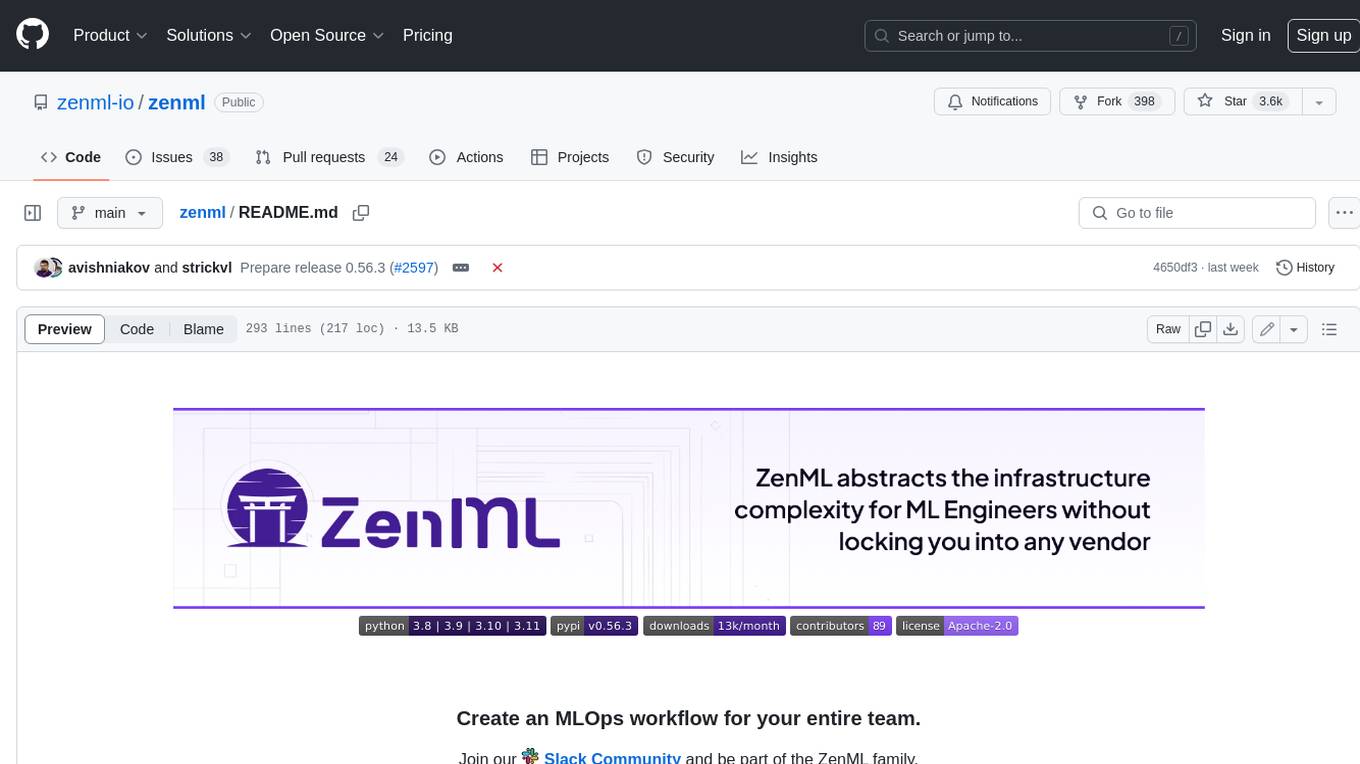
zenml
ZenML is an extensible, open-source MLOps framework for creating portable, production-ready machine learning pipelines. By decoupling infrastructure from code, ZenML enables developers across your organization to collaborate more effectively as they develop to production.
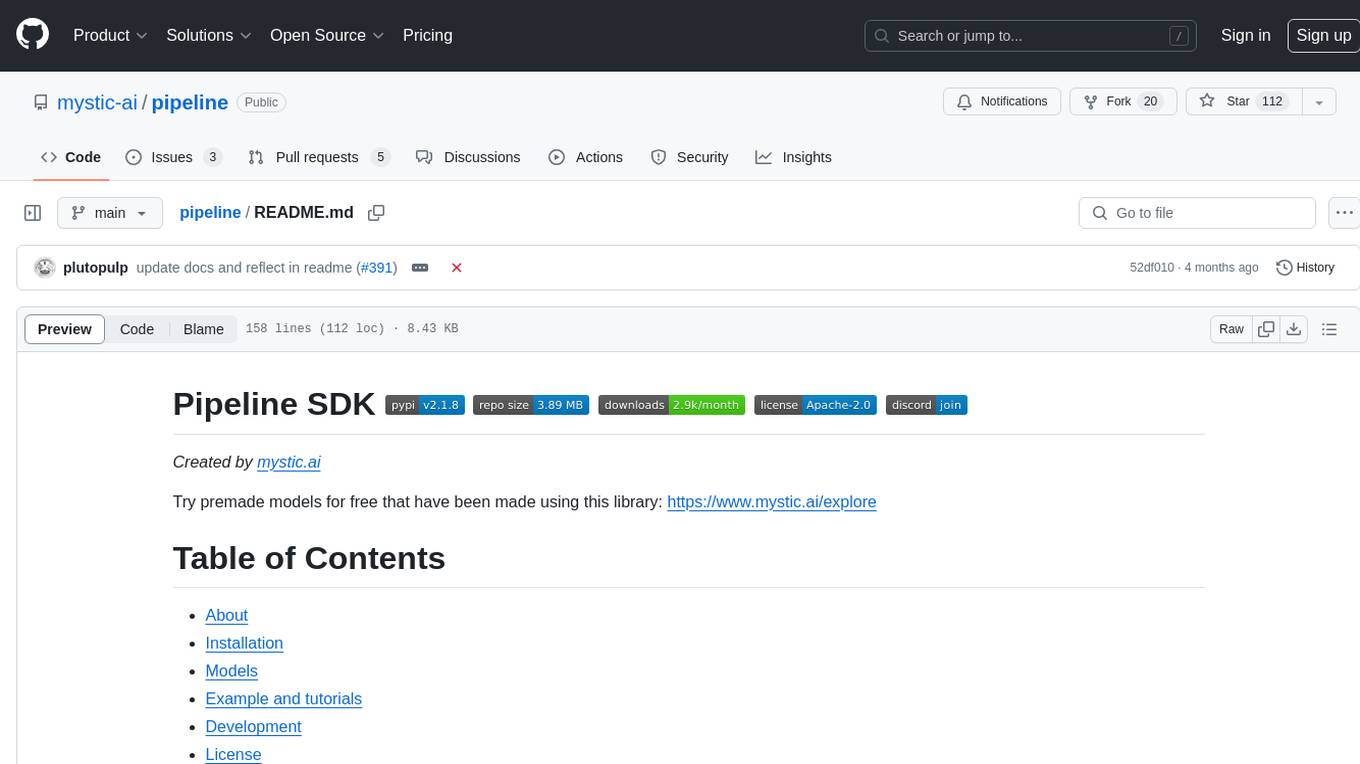
pipeline
Pipeline is a Python library designed for constructing computational flows for AI/ML models. It supports both development and production environments, offering capabilities for inference, training, and finetuning. The library serves as an interface to Mystic, enabling the execution of pipelines at scale and on enterprise GPUs. Users can also utilize this SDK with Pipeline Core on a private hosted cluster. The syntax for defining AI/ML pipelines is reminiscent of sessions in Tensorflow v1 and Flows in Prefect.
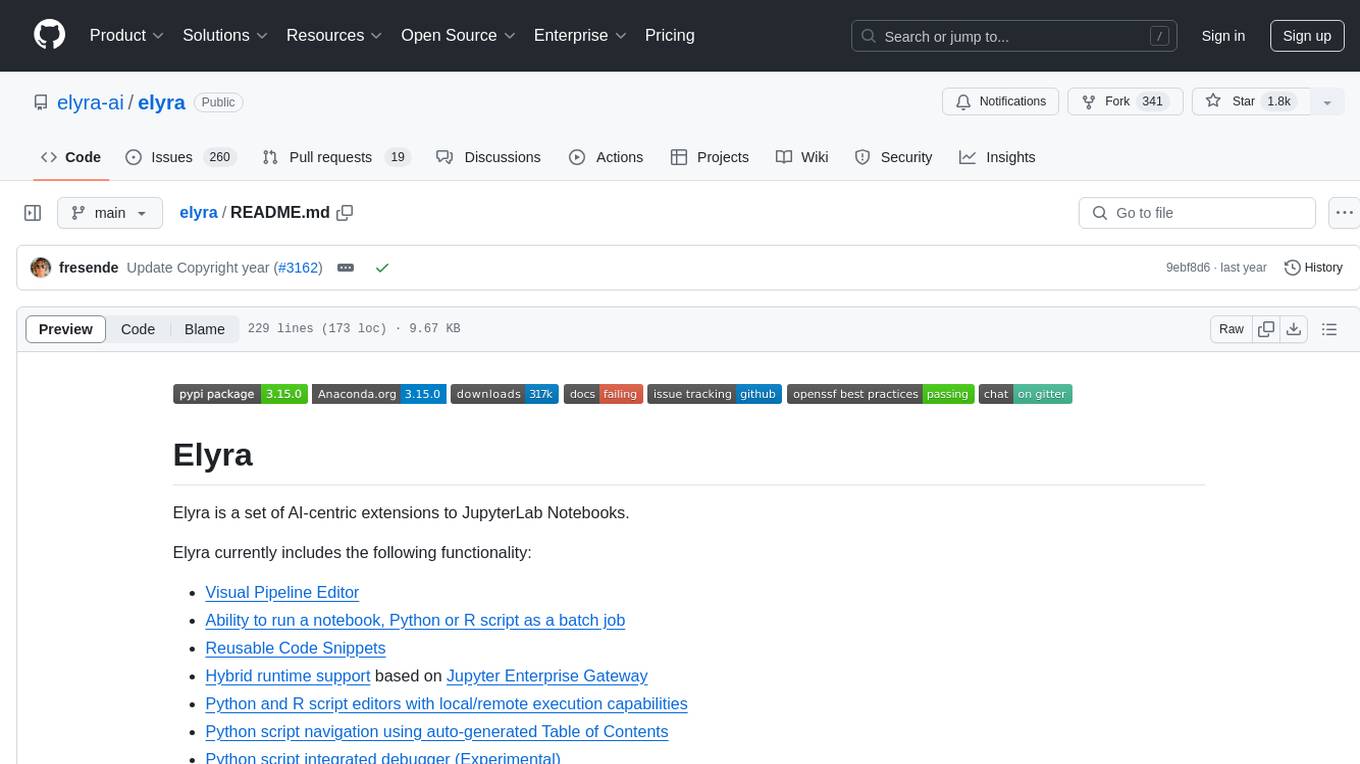
elyra
Elyra is a set of AI-centric extensions to JupyterLab Notebooks that includes features like Visual Pipeline Editor, running notebooks/scripts as batch jobs, reusable code snippets, hybrid runtime support, script editors with execution capabilities, debugger, version control using Git, and more. It provides a comprehensive environment for data scientists and AI practitioners to develop, test, and deploy machine learning models and workflows efficiently.
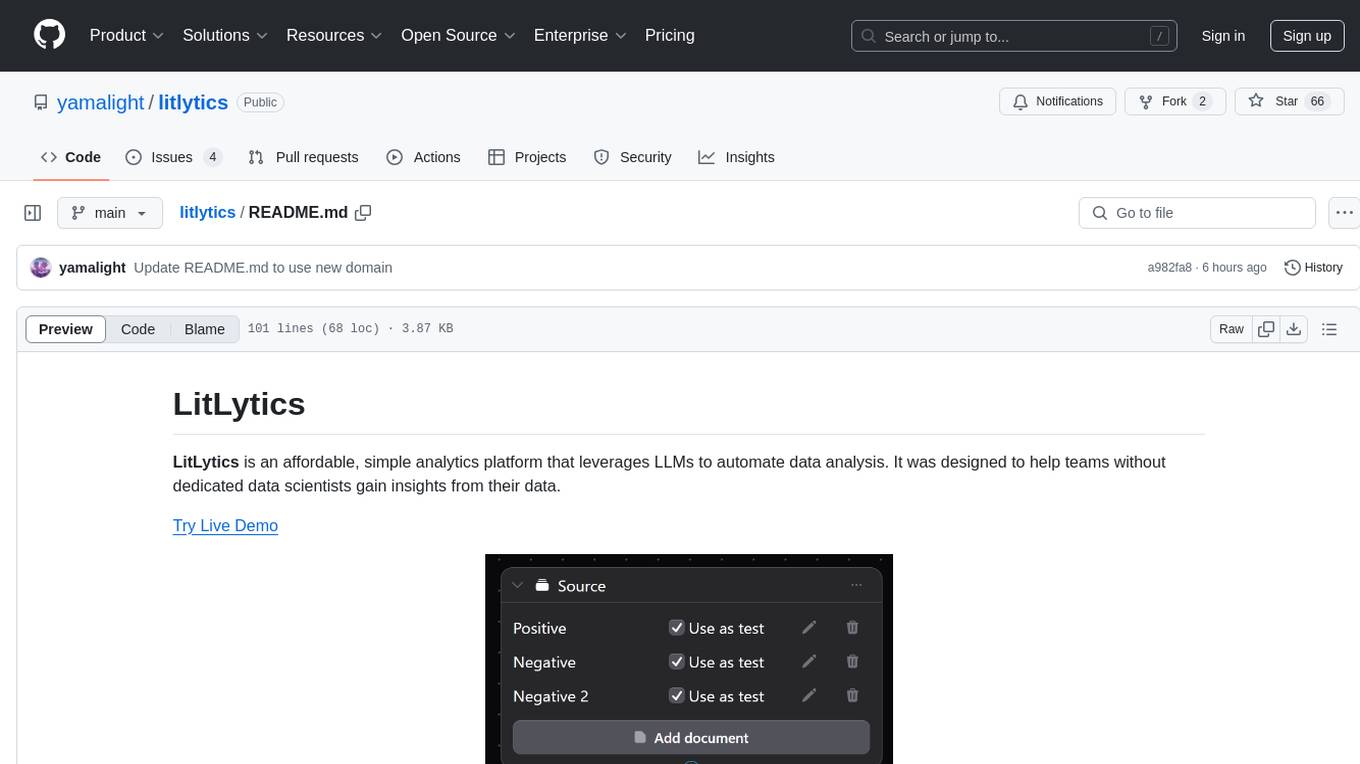
litlytics
LitLytics is an affordable analytics platform leveraging LLMs for automated data analysis. It simplifies analytics for teams without data scientists, generates custom pipelines, and allows customization. Cost-efficient with low data processing costs. Scalable and flexible, works with CSV, PDF, and plain text data formats.
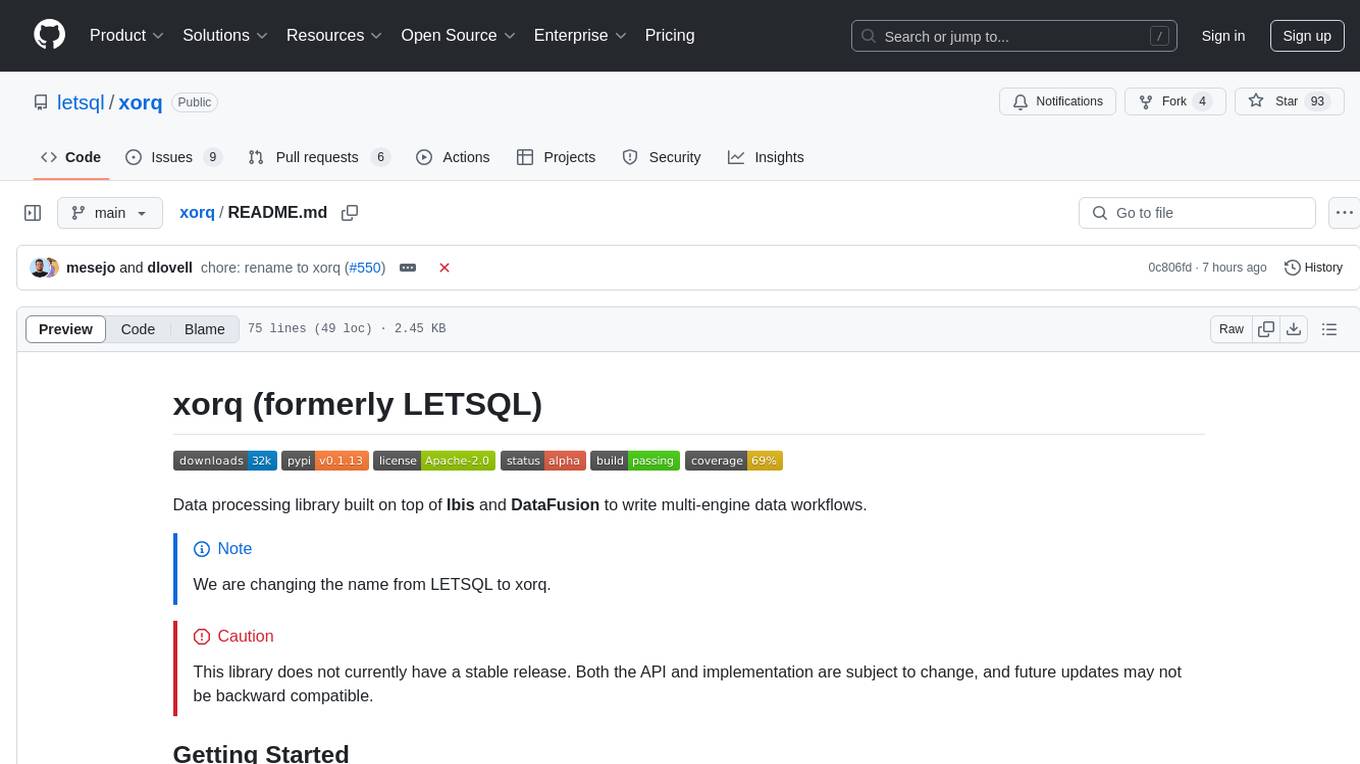
xorq
Xorq (formerly LETSQL) is a data processing library built on top of Ibis and DataFusion to write multi-engine data workflows. It provides a flexible and powerful tool for processing and analyzing data from various sources, enabling users to create complex data pipelines and perform advanced data transformations.
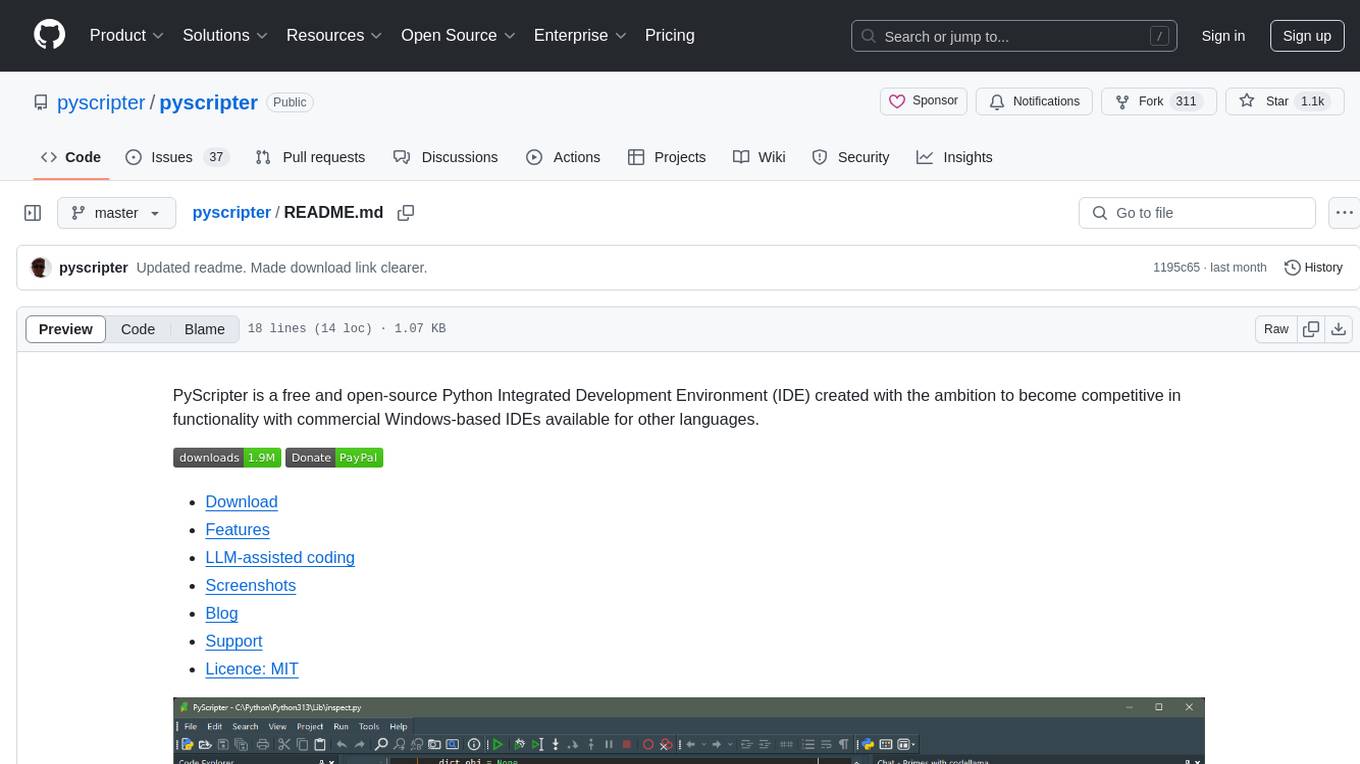
pyscripter
PyScripter is a free and open-source Python Integrated Development Environment (IDE) aiming to compete with commercial Windows-based IDEs for other languages. It offers features like LLM-assisted coding and provides support for Python development projects. The tool is designed to enhance the coding experience for Python developers by providing a user-friendly interface and a range of functionalities to streamline the development process.
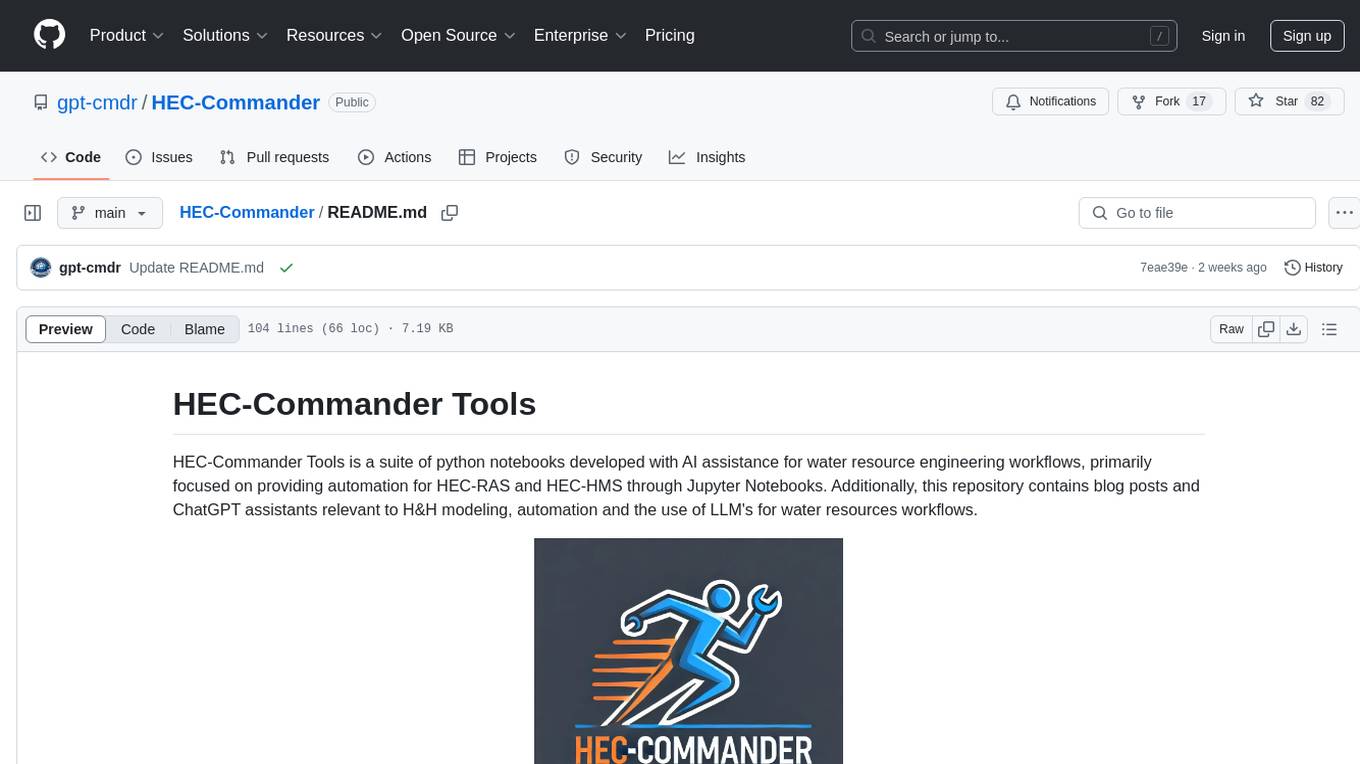
HEC-Commander
HEC-Commander Tools is a suite of python notebooks developed with AI assistance for water resource engineering workflows, focused on providing automation for HEC-RAS and HEC-HMS through Jupyter Notebooks. It contains automation scripts for HEC-HMS and HEC-RAS, tools for plotting results, and miscellaneous scripts for workflow assistance. The repository also includes blog posts, ChatGPT assistants, and presentations related to H&H modeling and the use of LLM's for water resources workflows.
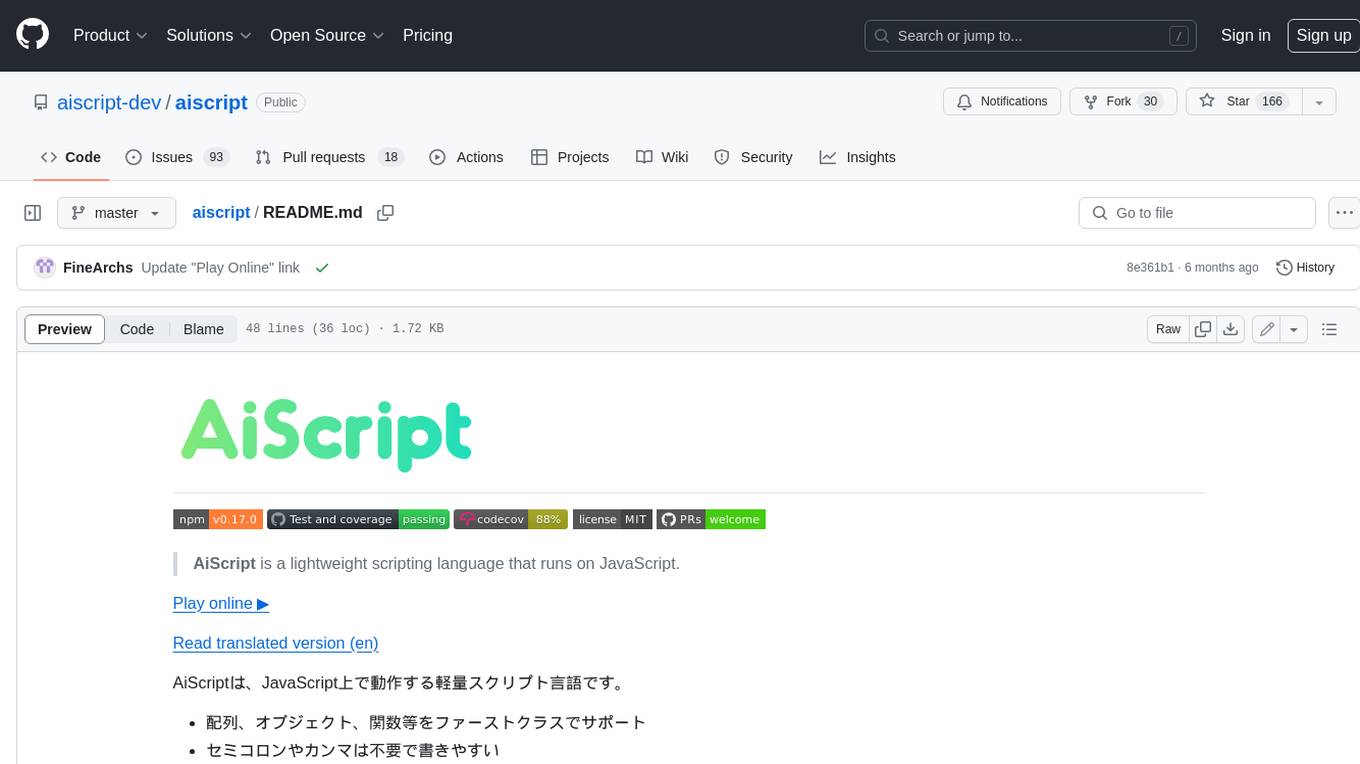
aiscript
AiScript is a lightweight scripting language that runs on JavaScript. It supports arrays, objects, and functions as first-class citizens, and is easy to write without the need for semicolons or commas. AiScript runs in a secure sandbox environment, preventing infinite loops from freezing the host. It also allows for easy provision of variables and functions from the host.
For similar jobs
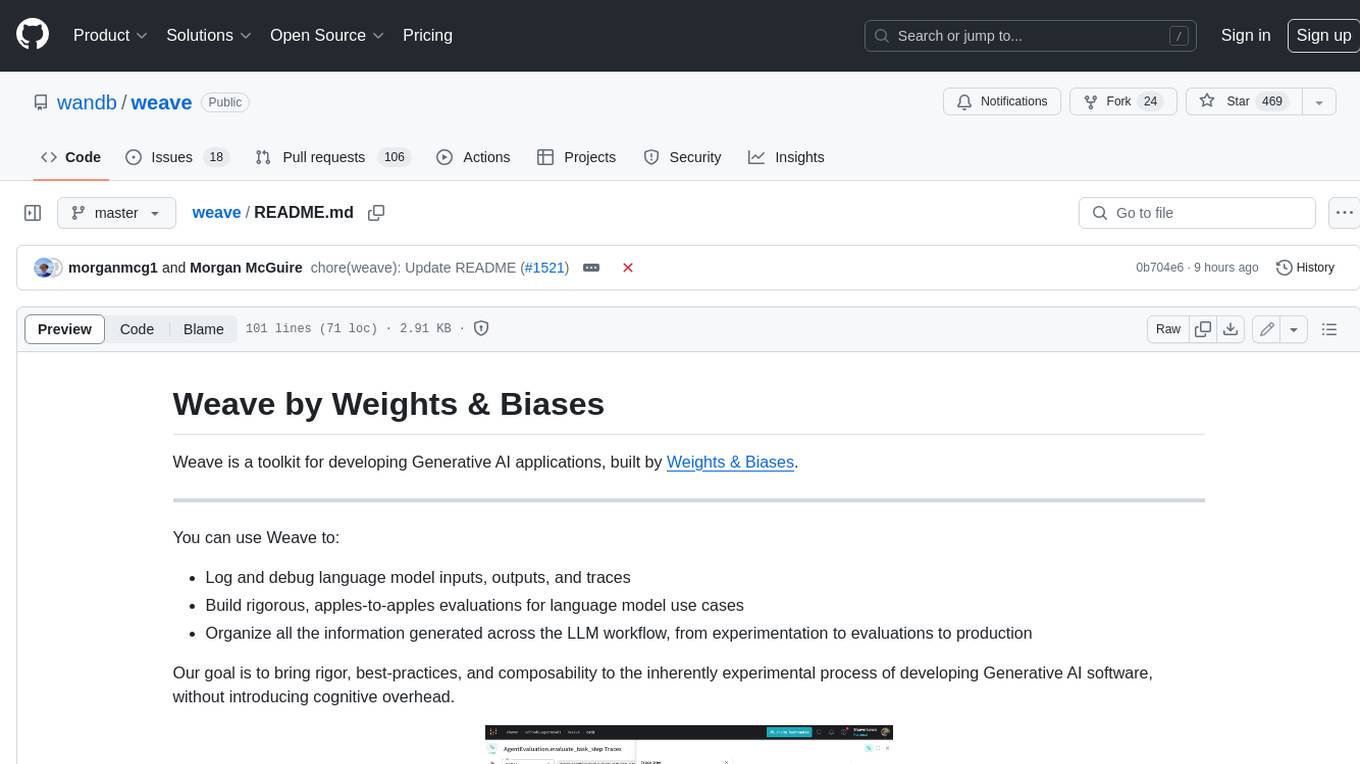
weave
Weave is a toolkit for developing Generative AI applications, built by Weights & Biases. With Weave, you can log and debug language model inputs, outputs, and traces; build rigorous, apples-to-apples evaluations for language model use cases; and organize all the information generated across the LLM workflow, from experimentation to evaluations to production. Weave aims to bring rigor, best-practices, and composability to the inherently experimental process of developing Generative AI software, without introducing cognitive overhead.
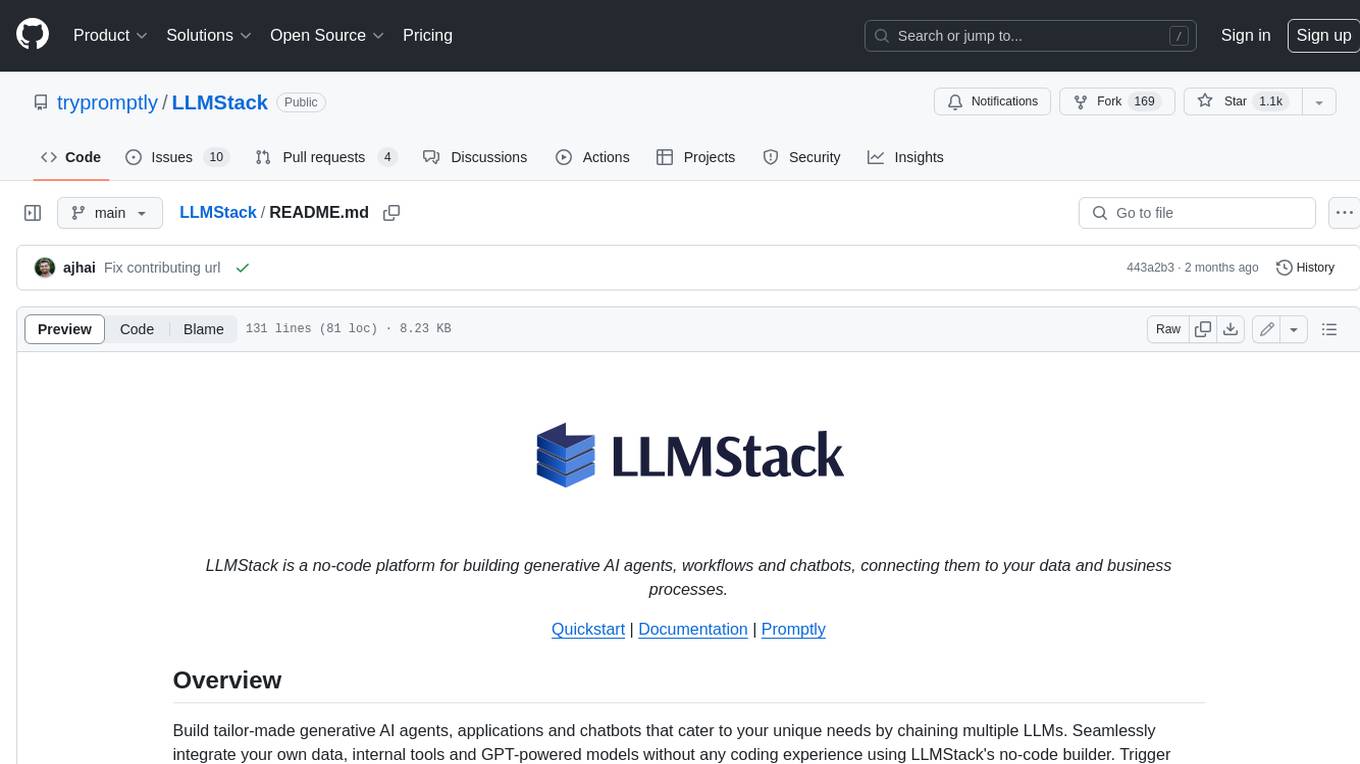
LLMStack
LLMStack is a no-code platform for building generative AI agents, workflows, and chatbots. It allows users to connect their own data, internal tools, and GPT-powered models without any coding experience. LLMStack can be deployed to the cloud or on-premise and can be accessed via HTTP API or triggered from Slack or Discord.
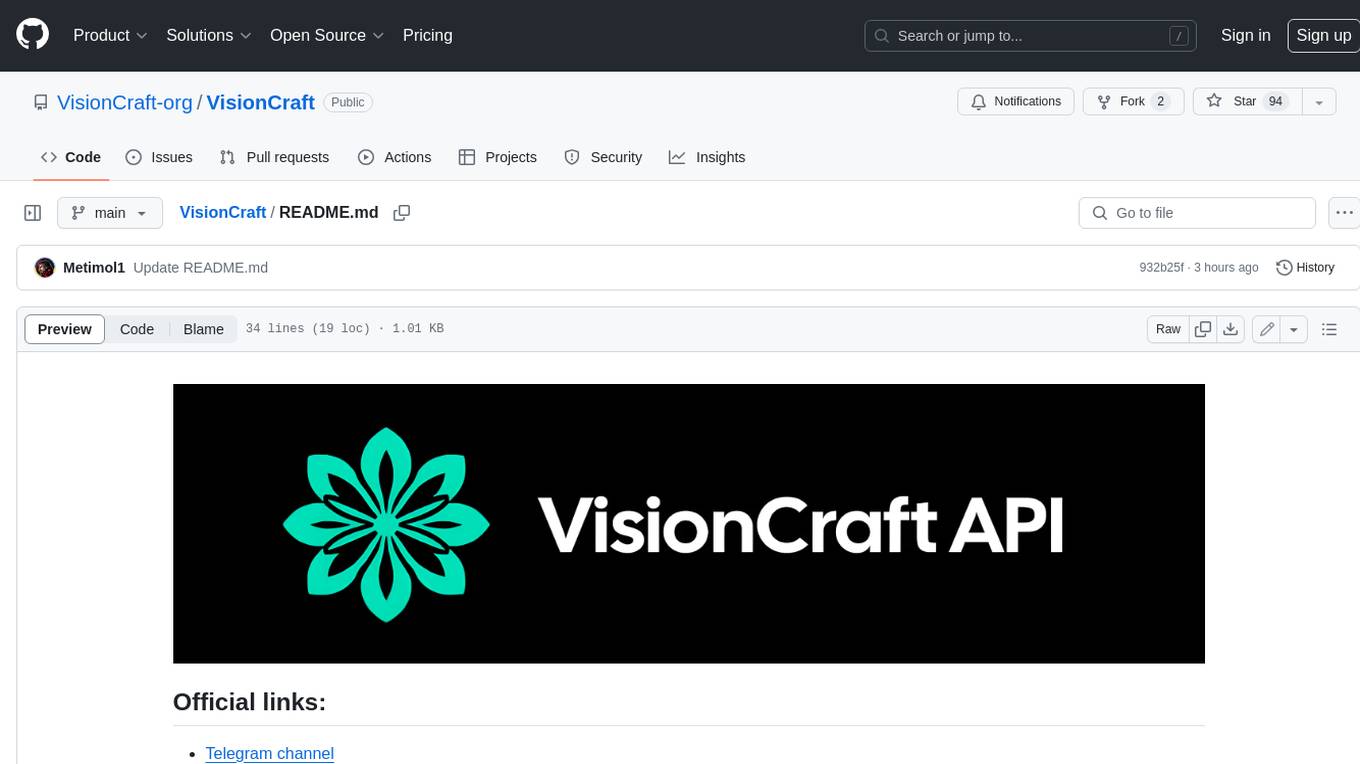
VisionCraft
The VisionCraft API is a free API for using over 100 different AI models. From images to sound.
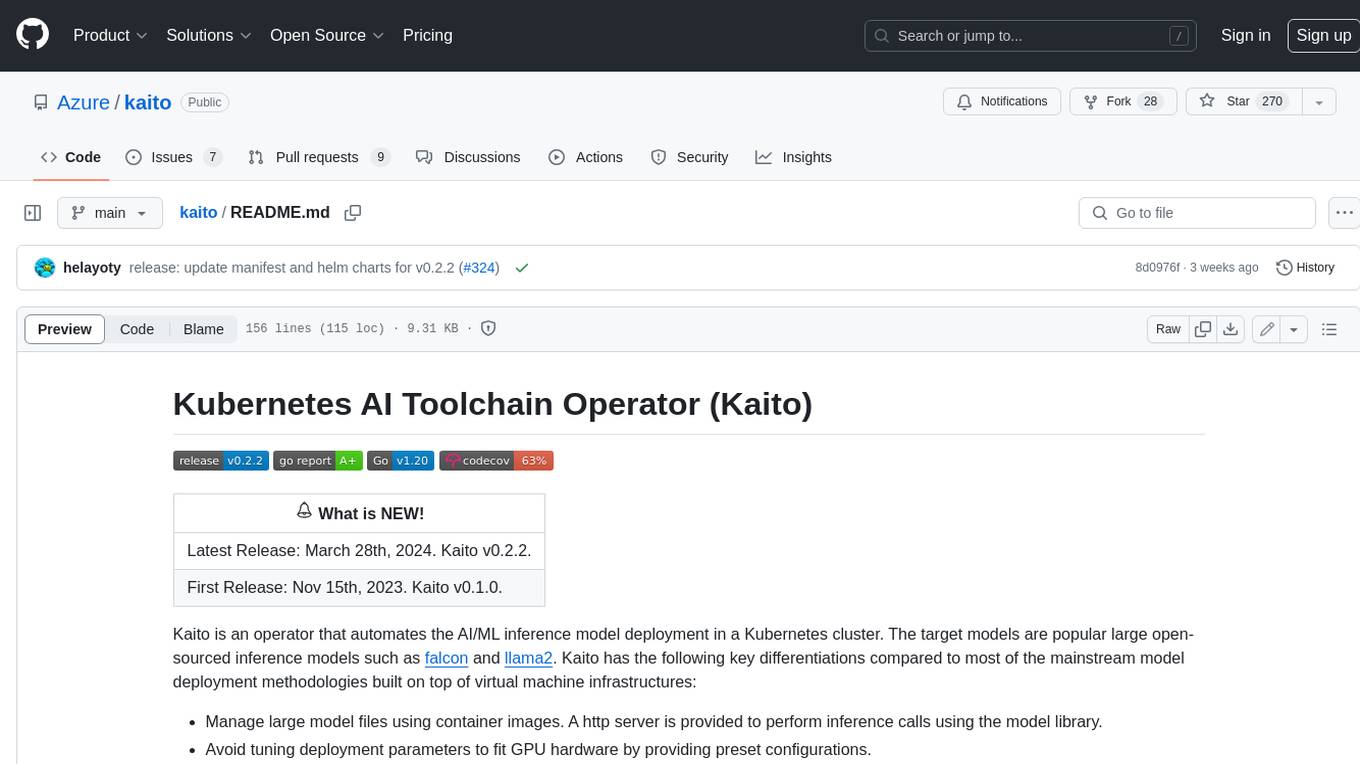
kaito
Kaito is an operator that automates the AI/ML inference model deployment in a Kubernetes cluster. It manages large model files using container images, avoids tuning deployment parameters to fit GPU hardware by providing preset configurations, auto-provisions GPU nodes based on model requirements, and hosts large model images in the public Microsoft Container Registry (MCR) if the license allows. Using Kaito, the workflow of onboarding large AI inference models in Kubernetes is largely simplified.
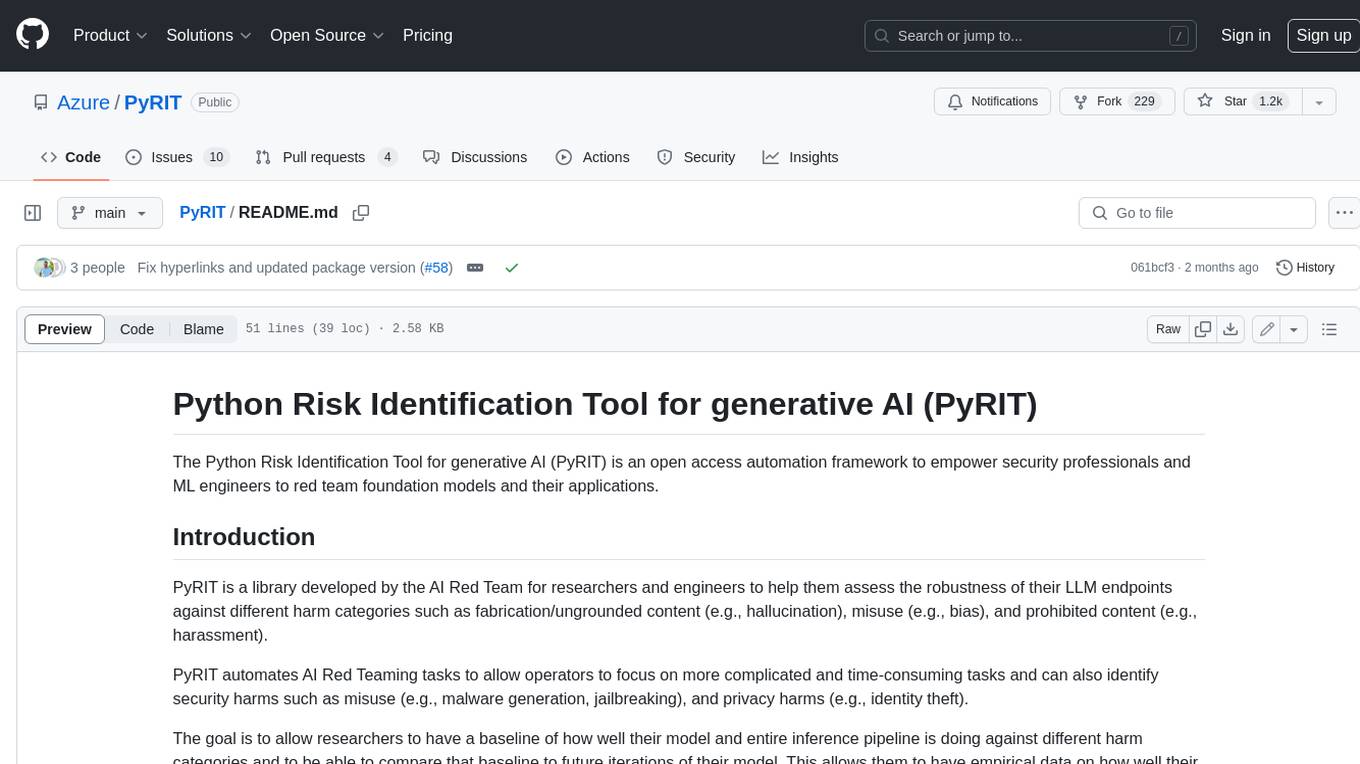
PyRIT
PyRIT is an open access automation framework designed to empower security professionals and ML engineers to red team foundation models and their applications. It automates AI Red Teaming tasks to allow operators to focus on more complicated and time-consuming tasks and can also identify security harms such as misuse (e.g., malware generation, jailbreaking), and privacy harms (e.g., identity theft). The goal is to allow researchers to have a baseline of how well their model and entire inference pipeline is doing against different harm categories and to be able to compare that baseline to future iterations of their model. This allows them to have empirical data on how well their model is doing today, and detect any degradation of performance based on future improvements.
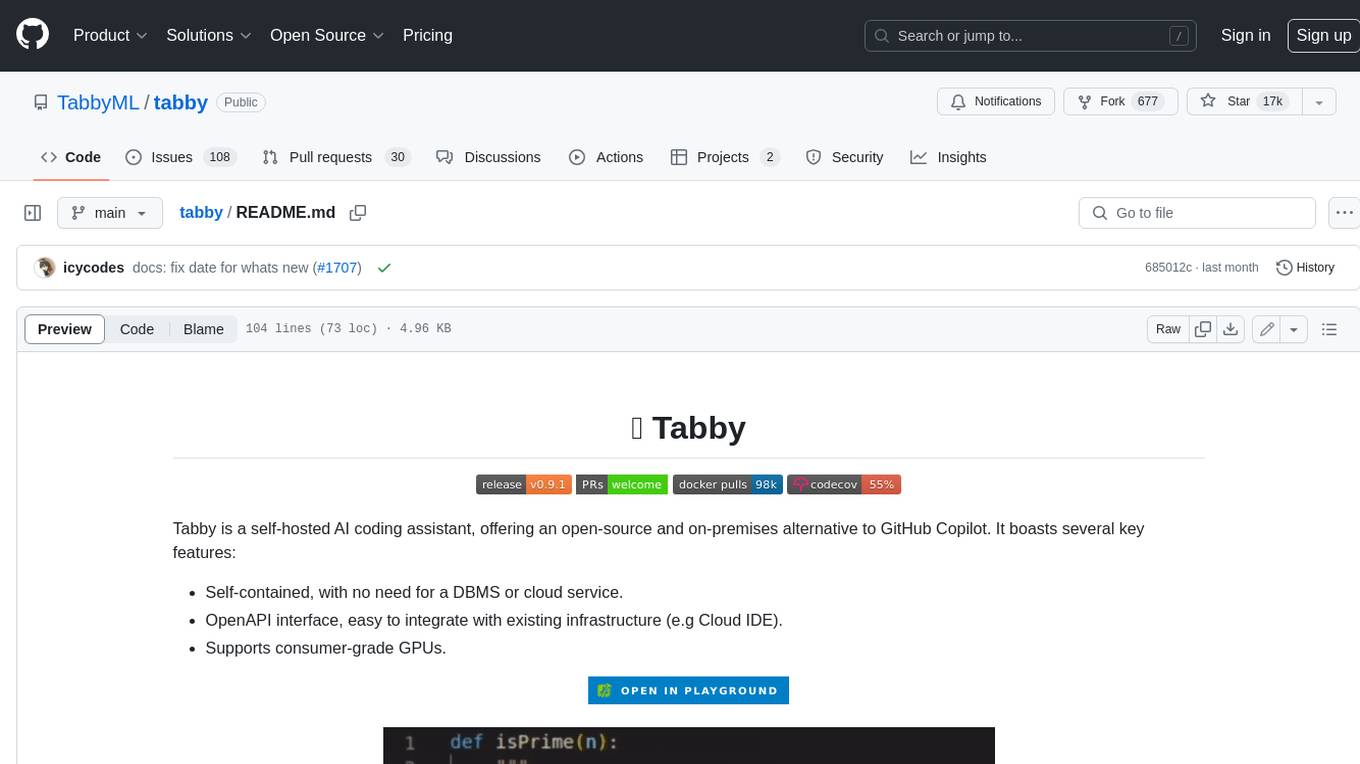
tabby
Tabby is a self-hosted AI coding assistant, offering an open-source and on-premises alternative to GitHub Copilot. It boasts several key features: * Self-contained, with no need for a DBMS or cloud service. * OpenAPI interface, easy to integrate with existing infrastructure (e.g Cloud IDE). * Supports consumer-grade GPUs.
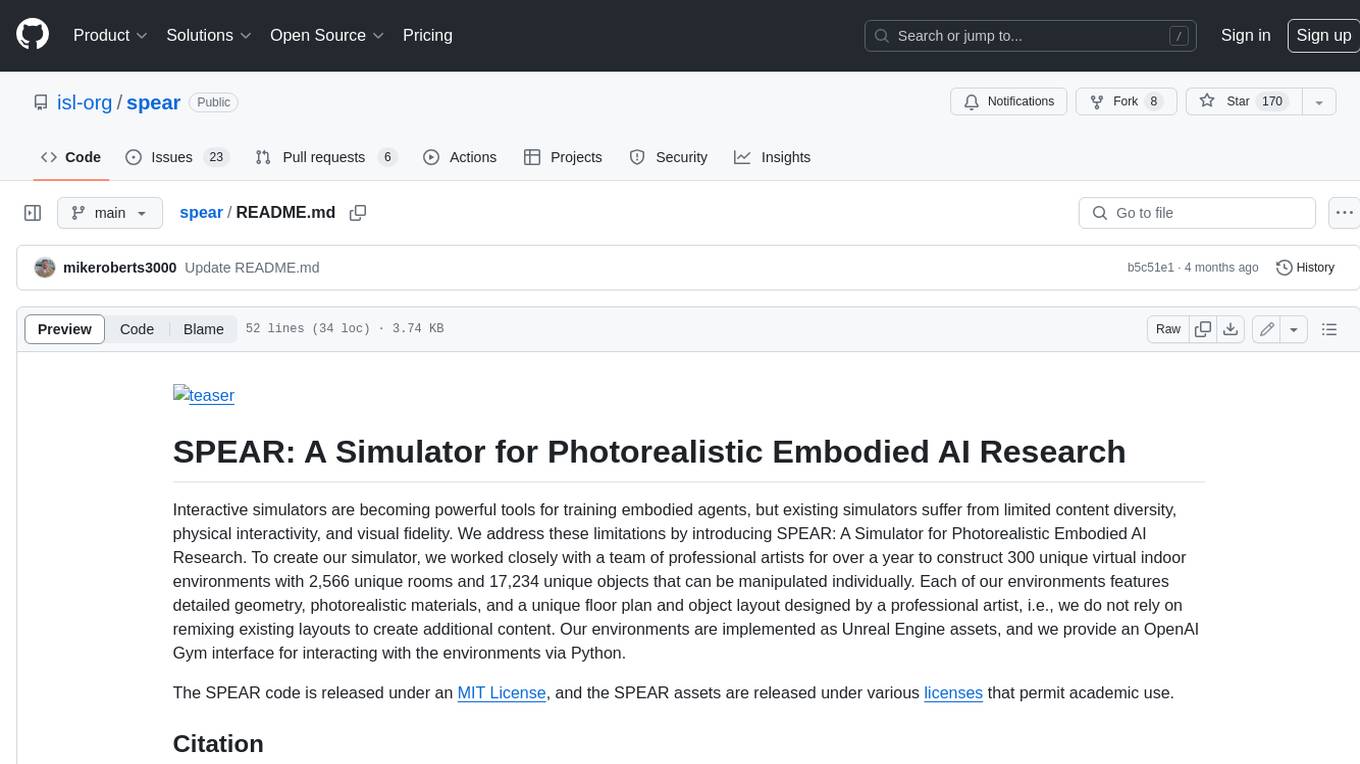
spear
SPEAR (Simulator for Photorealistic Embodied AI Research) is a powerful tool for training embodied agents. It features 300 unique virtual indoor environments with 2,566 unique rooms and 17,234 unique objects that can be manipulated individually. Each environment is designed by a professional artist and features detailed geometry, photorealistic materials, and a unique floor plan and object layout. SPEAR is implemented as Unreal Engine assets and provides an OpenAI Gym interface for interacting with the environments via Python.
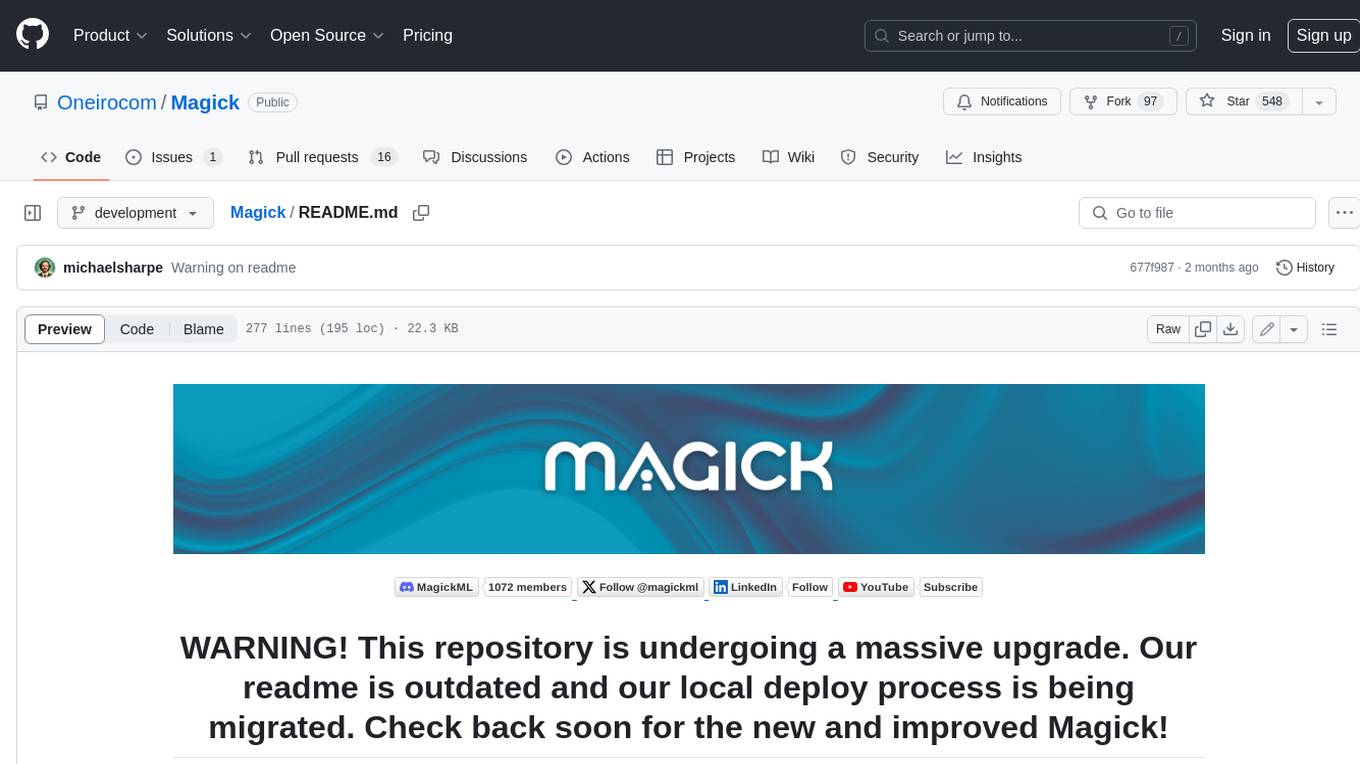
Magick
Magick is a groundbreaking visual AIDE (Artificial Intelligence Development Environment) for no-code data pipelines and multimodal agents. Magick can connect to other services and comes with nodes and templates well-suited for intelligent agents, chatbots, complex reasoning systems and realistic characters.