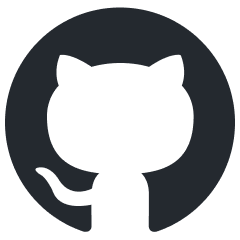
screen-pipe
Library to build personalized AI powered by what you've seen, said, or heard. Works with Ollama. Alternative to Rewind.ai. Open. Secure. You own your data. Rust.
Stars: 1035
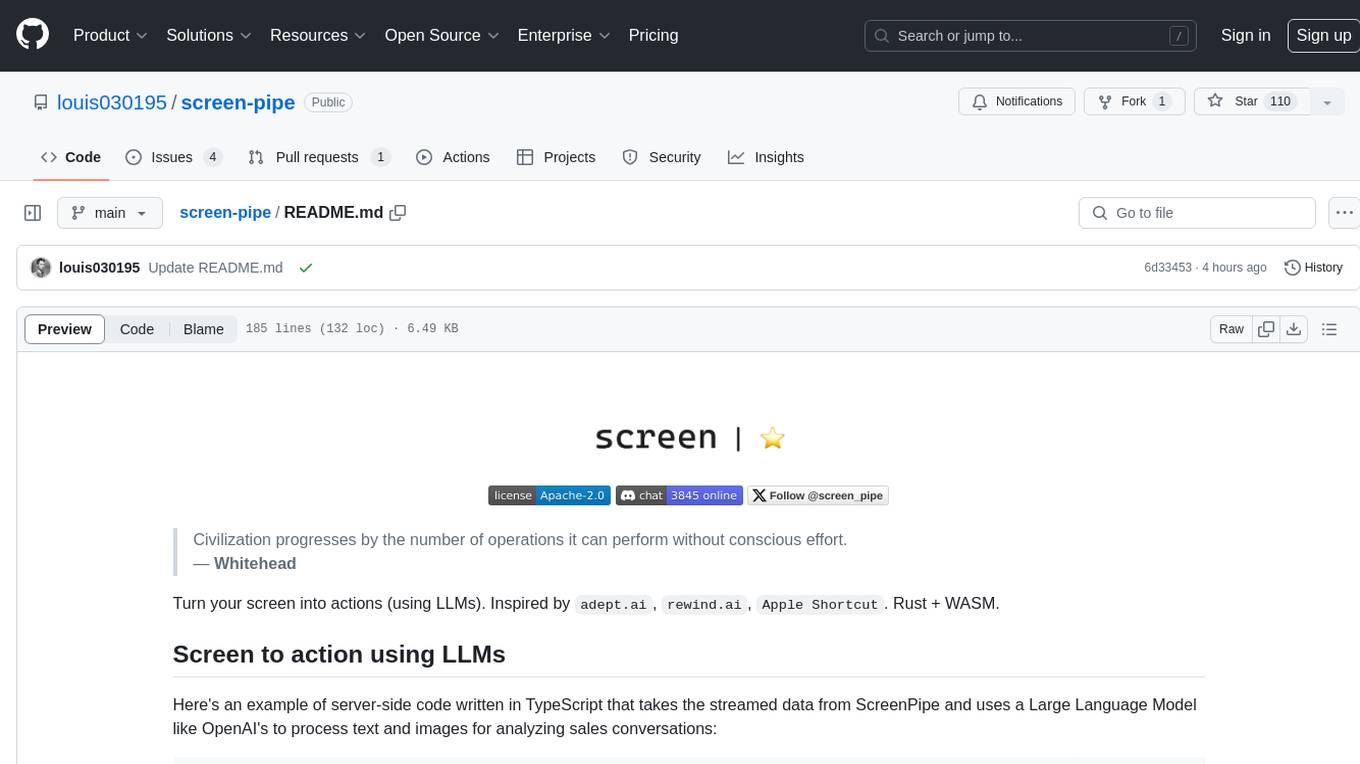
Screen-pipe is a Rust + WASM tool that allows users to turn their screen into actions using Large Language Models (LLMs). It enables users to record their screen 24/7, extract text from frames, and process text and images for tasks like analyzing sales conversations. The tool is still experimental and aims to simplify the process of recording screens, extracting text, and integrating with various APIs for tasks such as filling CRM data based on screen activities. The project is open-source and welcomes contributions to enhance its functionalities and usability.
README:
___ ___ _ __ ___ ___ _ __ _ __ (_)_ __ ___ / __|/ __| '__/ _ \/ _ \ '_ \| '_ \| | '_ \ / _ \ \__ \ (__| | | __/ __/ | | | |_) | | |_) | __/ |___/\___|_| \___|\___|_| |_| .__/|_| .__/ \___| |_| |_|
Latest News 🔥
- [2024/08] We released video embedding. AI gives you links to your video recording in the chat!
- [2024/08] We released the pipe store! Create, share, use plugins that get you the most out of your data in less than 30s, even if you are not technical.
- [2024/08] We released Apple & Windows Native OCR.
- [2024/08] The Linux desktop app is here!.
- [2024/07] The Windows desktop app is here! Get it now!.
- [2024/07] 🎁 Screenpipe won Friends (the AI necklace) hackathon at AGI House (integrations soon)
- [2024/07] We just launched the desktop app! Download now!
Library to build personalized AI powered by what you've seen, said, or heard. Works with Ollama. Alternative to Rewind.ai. Open. Secure. You own your data. Rust.
We are shipping daily, make suggestions, post bugs, give feedback.
Building a reliable stream of audio and screenshot data, where a user simply clicks a button and the script runs in the background 24/7, collecting and extracting data from screen and audio input/output, can be frustrating.
There are numerous use cases that can be built on top of this layer. To simplify life for other developers, we decided to solve this non-trivial problem. It's still in its early stages, but it works end-to-end. We're working on this full-time and would love to hear your feedback and suggestions.
There are multiple ways to install screenpipe:
- as a CLI (continue reading), for rather technical users
- as a paid desktop app with 1 year updates, priority support, and priority features
- as a free forever desktop app (but you need to build it yourself). We're 100% OSS.
- as a free forever desktop app - by sending a PR (example) (or offer free app to a friend)
- as a Rust or WASM library (documentation WIP)
This is the instructions to install the command line interface.
Struggle to get it running? I'll install it with you in a 15 min call.
CLI installation
MacOS
Option I: brew
- Install CLI
brew tap louis030195/screen-pipe https://github.com/louis030195/screen-pipe.git
brew install screenpipe
- Run it:
screenpipe
we just released experimental apple native OCR, to use it:
screenpipe --ocr-engine apple-native
or if you don't want audio to be recorded
screenpipe --disable-audio
if you want to save OCR data to text file in text_json folder in the root of your project (good for testing):
screenpipe --save-text-files
if you want to run screenpipe in debug mode to show more logs in terminal:
screenpipe --debug
by default screenpipe is using whisper-tiny that runs LOCALLY to get better quality or lower compute you can use cloud model (we use Deepgram) via cloud api:
screenpipe -audio-transcription-engine deepgram
by default screenpipe is using a local model for screen capture OCR processing to use the cloud (through unstructured.io) for better performance use this flag:
screenpipe --ocr-engine unstructured
friend wearable integration, in order to link your wearable you need to pass user ID from friend app:
screenpipe --friend-wearable-uid AC...........................F3
you can combine multiple flags if needed
Option II: Install from the source
- Install dependencies:
curl --proto '=https' --tlsv1.2 -sSf https://sh.rustup.rs | sh # takes 5 minutes
brew install pkg-config ffmpeg jq tesseract
- Clone the repo:
git clone https://github.com/louis030195/screen-pipe
This runs a local SQLite DB + an API + screenshot, ocr, mic, stt, mp4 encoding
cd screen-pipe # enter cloned repo
Build the project, takes 5-10 minutes depending on your hardware
# necessary to use apple native OCR
export RUSTFLAGS="-C link-arg=-Wl,-rpath,@executable_path/../../screenpipe-vision/bin -C link-arg=-Wl,-rpath,@loader_path/../../screenpipe-vision/lib"
cargo build --release --features metal # takes 3 minuttes
Then run it
./target/release/screenpipe # add --ocr-engine apple-native to use apple native OCR
# add "--disable-audio" if you don't want audio to be recorded
# "--save-text-files" if you want to save OCR data to text file in text_json folder in the root of your project (good for testing)
# "--debug" if you want to run screenpipe in debug mode to show more logs in terminal
Windows
Currently updating the instructions for Windows which are not straightforward, please feel free to help
Linux
Option I: Install from source
- Install dependencies:
sudo apt-get update
sudo apt-get install -y libavformat-dev libavfilter-dev libavdevice-dev ffmpeg libasound2-dev tesseract-ocr libtesseract-dev
# Install Rust programming language
curl --proto '=https' --tlsv1.2 -sSf https://sh.rustup.rs | sh
- Clone the repo:
git clone https://github.com/louis030195/screen-pipe
cd screen-pipe
- Build and run:
cargo build --release --features cuda # remove "--features cuda" if you do not have a NVIDIA GPU
# then run it
./target/release/screenpipe
Option II: Install through Nix
Choose one of the following methods:
a. Using nix-env
:
nix-env -iA nixpkgs.screen-pipe
b. In your configuration.nix
(for NixOS users):
Add the following to your configuration.nix
:
environment.systemPackages = with pkgs; [
screen-pipe
];
Then rebuild your system with sudo nixos-rebuild switch
.
c. In a Nix shell:
nix-shell -p screen-pipe
d. Using nix run
(for ad-hoc usage):
nix run nixpkgs#screen-pipe
Note: Make sure you're using a recent version of nixpkgs that includes the screen-pipe package.
By default the data is stored in $HOME/.screenpipe
(C:\AppData\Users\<user>\.screenpipe
on Windows) you can change using --data-dir <mydir>
run example vercel/ai chatbot web interface
This example uses OpenAI. If you're looking for ollama example check the examples folder
The desktop app fully support OpenAI & Ollama by default.
To run Vercel chatbot, try this:
git clone https://github.com/louis030195/screen-pipe
Navigate to app directory
cd screen-pipe/examples/typescript/vercel-ai-chatbot
Set up you OPENAI API KEY in .env
echo "OPENAI_API_KEY=XXXXXXXXXXXXXXXXXXXXXXXXXXXXXXXXXXXXXXXXXX" > .env
Install dependencies and run local web server
npm install
npm run dev
You can use terminal commands to query and view your data as shown below. Also, we recommend Tableplus.com to view the database, it has a free tier.
Here's a pseudo code to illustrate how to use screenpipe, after a meeting for example (automatically with our webhooks):
// 1h ago
const startDate = "<some time 1h ago..>"
// 10m ago
const endDate = "<some time 10m ago..>"
// get all the screen & mic data from roughly last hour
const results = fetchScreenpipe(startDate, endDate)
// send it to an LLM and ask for a summary
const summary = fetchOllama("{results} create a summary from these transcriptions")
// or const summary = fetchOpenai(results)
// add the meeting summary to your notes
addToNotion(summary)
// or your favourite note taking app
Or thousands of other usages of all your screen & mic data!
Check which tables you have in the local database
sqlite3 ~/.screenpipe/db.sqlite ".tables"
Print a sample audio_transcriptions from the database
sqlite3 ~/.screenpipe/db.sqlite ".mode json" ".once /dev/stdout" "SELECT * FROM audio_transcriptions ORDER BY id DESC LIMIT 1;" | jq .
Print a sample frame_OCR_text from the database
sqlite3 ~/.screenpipe/db.sqlite ".mode json" ".once /dev/stdout" "SELECT * FROM ocr_text ORDER BY frame_id DESC LIMIT 1;" | jq -r '.[0].text'
Play a sample frame_recording from the database
ffplay "data/2024-07-12_01-14-14.mp4"
Play a sample audio_recording from the database
ffplay "data/Display 1 (output)_2024-07-12_01-14-11.mp4"
Example to query the API
- Basic search query
curl "http://localhost:3030/search?q=Neuralink&limit=5&offset=0&content_type=ocr" | jq
Other Example to query the API
# 2. Search with content type filter (OCR)
curl "http://localhost:3030/search?q=QUERY_HERE&limit=5&offset=0&content_type=ocr"
# 3. Search with content type filter (Audio)
curl "http://localhost:3030/search?q=QUERY_HERE&limit=5&offset=0&content_type=audio"
# 4. Search with pagination
curl "http://localhost:3030/search?q=QUERY_HERE&limit=10&offset=20"
# 6. Search with no query (should return all results)
curl "http://localhost:3030/search?limit=5&offset=0"
# filter by app (wll only return OCR results)
curl "http://localhost:3030/search?app_name=cursor"
Keep in mind that it's still experimental.
https://github.com/user-attachments/assets/edb503d4-6531-4527-9b05-0397fd8b5976
- Search
- Semantic and keyword search. Find information you've forgotten or misplaced
- Playback history of your desktop when searching for a specific info
- Automation:
- Automatically generate documentation
- Populate CRM systems with relevant data
- Synchronize company knowledge across platforms
- Automate repetitive tasks based on screen content
- Analytics:
- Track personal productivity metrics
- Organize and analyze educational materials
- Gain insights into areas for personal improvement
- Analyze work patterns and optimize workflows
- Personal assistant:
- Summarize lengthy documents or videos
- Provide context-aware reminders and suggestions
- Assist with research by aggregating relevant information
- Live captions, translation support
- Collaboration:
- Share and annotate screen captures with team members
- Create searchable archives of meetings and presentations
- Compliance and security:
- Track what your employees are really up to
- Monitor and log system activities for audit purposes
- Detect potential security threats based on screen content
Alpha: runs on my computer Macbook pro m3 32 GB ram
and a $400 Windows laptop, 24/7.
- [ ] Integrations
- [x] ollama
- [x] openai
- [x] Friend wearable
- [x] Fileorganizer2000
- [x] mem0
- [x] Brilliant Frames
- [x] Vercel AI SDK
- [ ] supermemory
- [x] deepgram
- [x] unstructured
- [x] excalidraw
- [x] Obsidian
- [x] Apple shortcut
- [x] multion
- [x] iPhone
- [ ] Android
- [ ] Camera
- [ ] Keyboard
- [x] Browser
- [ ] Pipe Store (a list of "pipes" you can build, share & easily install to get more value out of your screen & mic data without effort). It runs in Deno Typescript engine within screenpipe on your computer
- [x] screenshots + OCR with different engines to optimise privacy, quality, or energy consumption
- [x] tesseract
- [x] Windows native OCR
- [x] Apple native OCR
- [x] unstructured.io
- [ ] screenpipe screen/audio specialised LLM
- [x] audio + STT (works with multi input devices, like your iPhone + mac mic, many STT engines)
- [x] Linux, MacOS, Windows input
- [x] Linux output
- [x] MacOS output
- [ ] Windows output (shipping on 19 august)
- [x] remote capture (run screenpipe on your cloud and it capture your local machine, only tested on Linux) for example when you have low compute laptop
- [x] optimised screen & audio recording (mp4 encoding, estimating 30 gb/m with default settings)
- [x] sqlite local db
- [x] local api
- [x] Cross platform CLI, desktop app (MacOS, Windows, Linux)
- [x] Metal, CUDA
- [ ] TS SDK
- [ ] multimodal embeddings
- [ ] cloud storage options (s3, pgsql, etc.)
- [x] cloud computing options (deepgram for audio, unstructured for OCR)
- [ ] bug-free & stable
- [x] custom storage settings: customizable capture settings (fps, resolution)
- [ ] security
- [x] window specific capture (e.g. can decide to only capture specific tab of cursor, chrome, obsidian, or only specific app)
- [ ] encryption
- [ ] PII removal
- [ ] fast, optimised, energy-efficient modes
- [ ] webhooks/events (for automations)
- [ ] abstractions for multiplayer usage (e.g. aggregate sales team data, company team data, partner, etc.)
Recent breakthroughs in AI have shown that context is the final frontier. AI will soon be able to incorporate the context of an entire human life into its 'prompt', and the technologies that enable this kind of personalisation should be available to all developers to accelerate access to the next stage of our evolution.
Contributions are welcome! If you'd like to contribute, please read CONTRIBUTING.md.
What's the difference with adept.ai and rewind.ai?
- adept.ai is a closed product, focused on automation while we are open and focused on enabling tooling & infra for a wide range of applications like adept
- rewind.ai is a closed product, focused on a single use case (they only focus on meetings now), not customisable, your data is owned by them, and not extendable by developers
Where is the data stored?
- 100% of the data stay local in a SQLite database and mp4/mp3 files. You own your data
Do you encrypt the data?
- Not yet but we're working on it. We want to provide you the highest level of security.
How can I customize capture settings to reduce storage and energy usage?
- You can adjust frame rates and resolution in the configuration. Lower values will reduce storage and energy consumption. We're working on making this more user-friendly in future updates.
What are some practical use cases for screenpipe?
- RAG & question answering
- Automation (write code somewhere else while watching you coding, write docs, fill your CRM, sync company's knowledge, etc.)
- Analytics (track human performance, education, become aware of how you can improve, etc.)
- etc.
- We're constantly exploring new use cases and welcome community input!
For Tasks:
Click tags to check more tools for each tasksFor Jobs:
Alternative AI tools for screen-pipe
Similar Open Source Tools
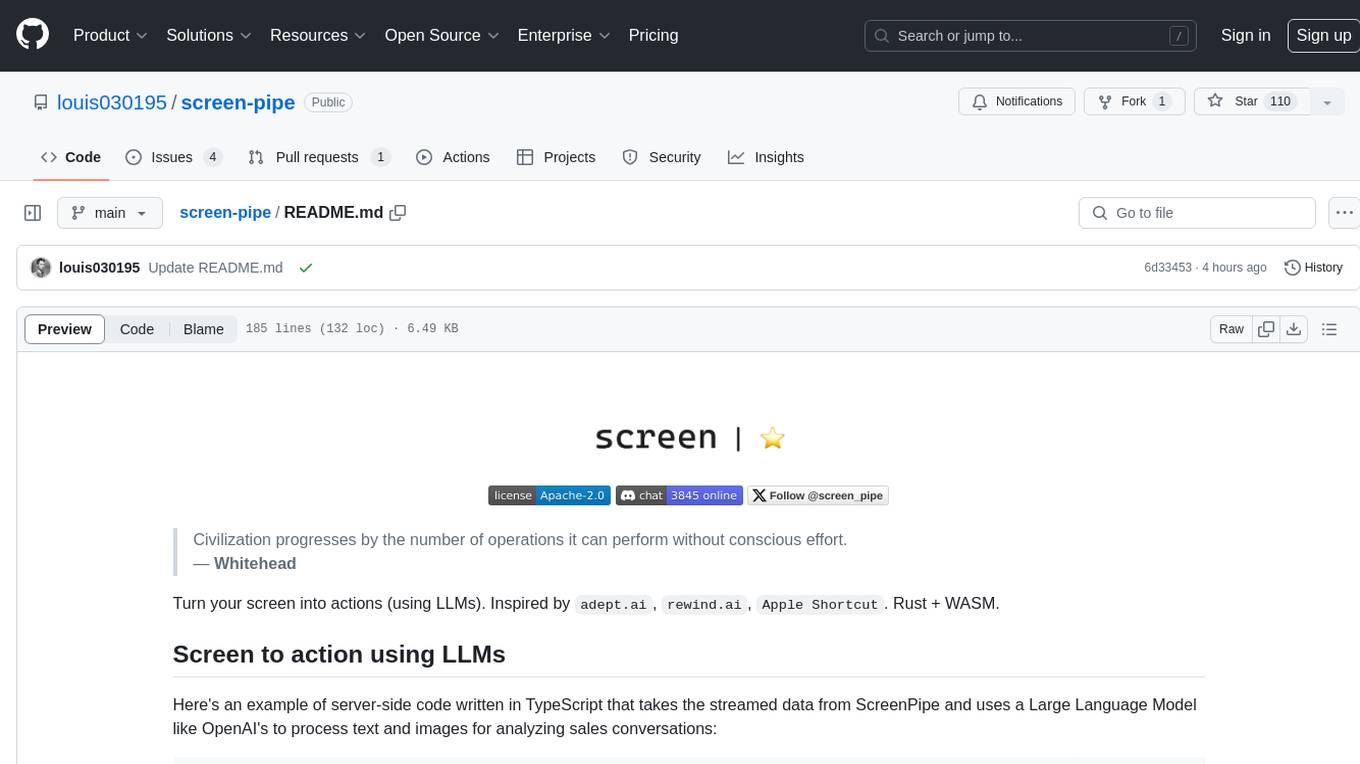
screen-pipe
Screen-pipe is a Rust + WASM tool that allows users to turn their screen into actions using Large Language Models (LLMs). It enables users to record their screen 24/7, extract text from frames, and process text and images for tasks like analyzing sales conversations. The tool is still experimental and aims to simplify the process of recording screens, extracting text, and integrating with various APIs for tasks such as filling CRM data based on screen activities. The project is open-source and welcomes contributions to enhance its functionalities and usability.
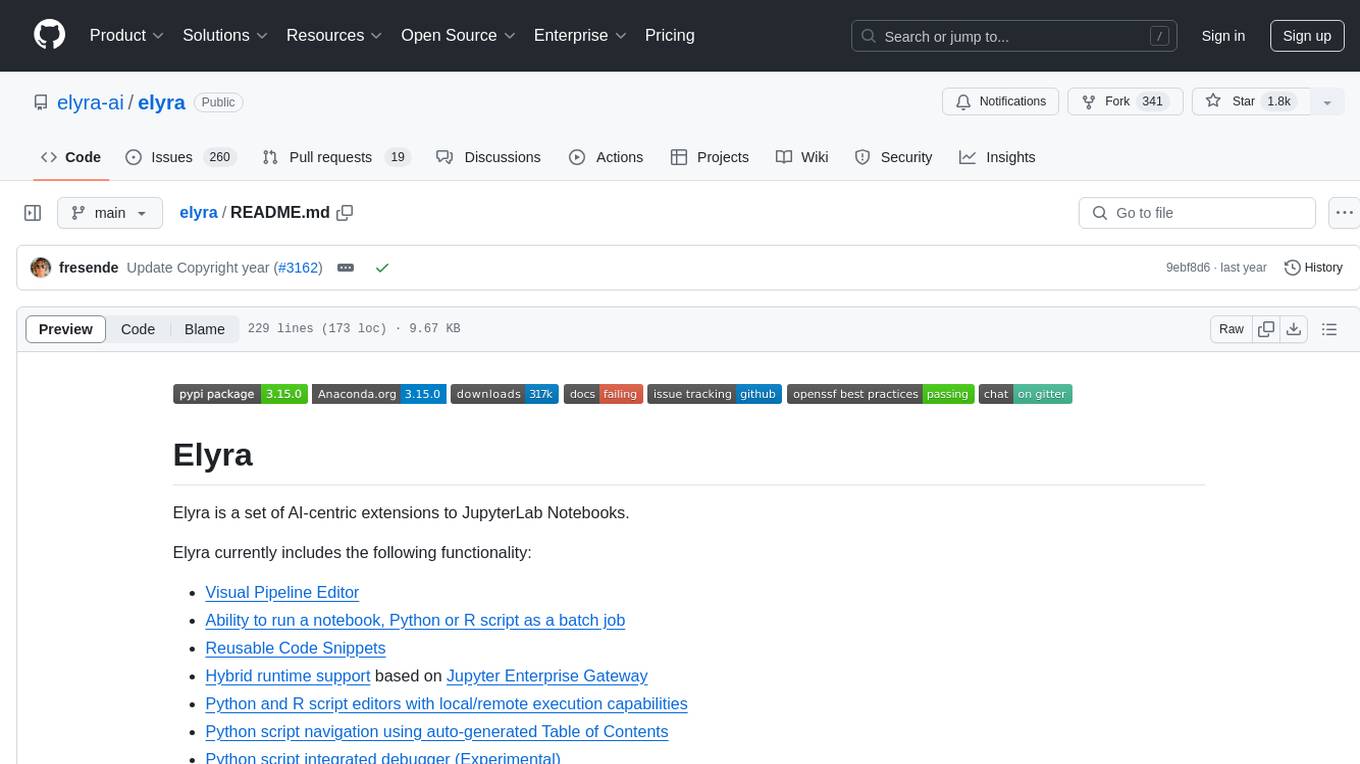
elyra
Elyra is a set of AI-centric extensions to JupyterLab Notebooks that includes features like Visual Pipeline Editor, running notebooks/scripts as batch jobs, reusable code snippets, hybrid runtime support, script editors with execution capabilities, debugger, version control using Git, and more. It provides a comprehensive environment for data scientists and AI practitioners to develop, test, and deploy machine learning models and workflows efficiently.
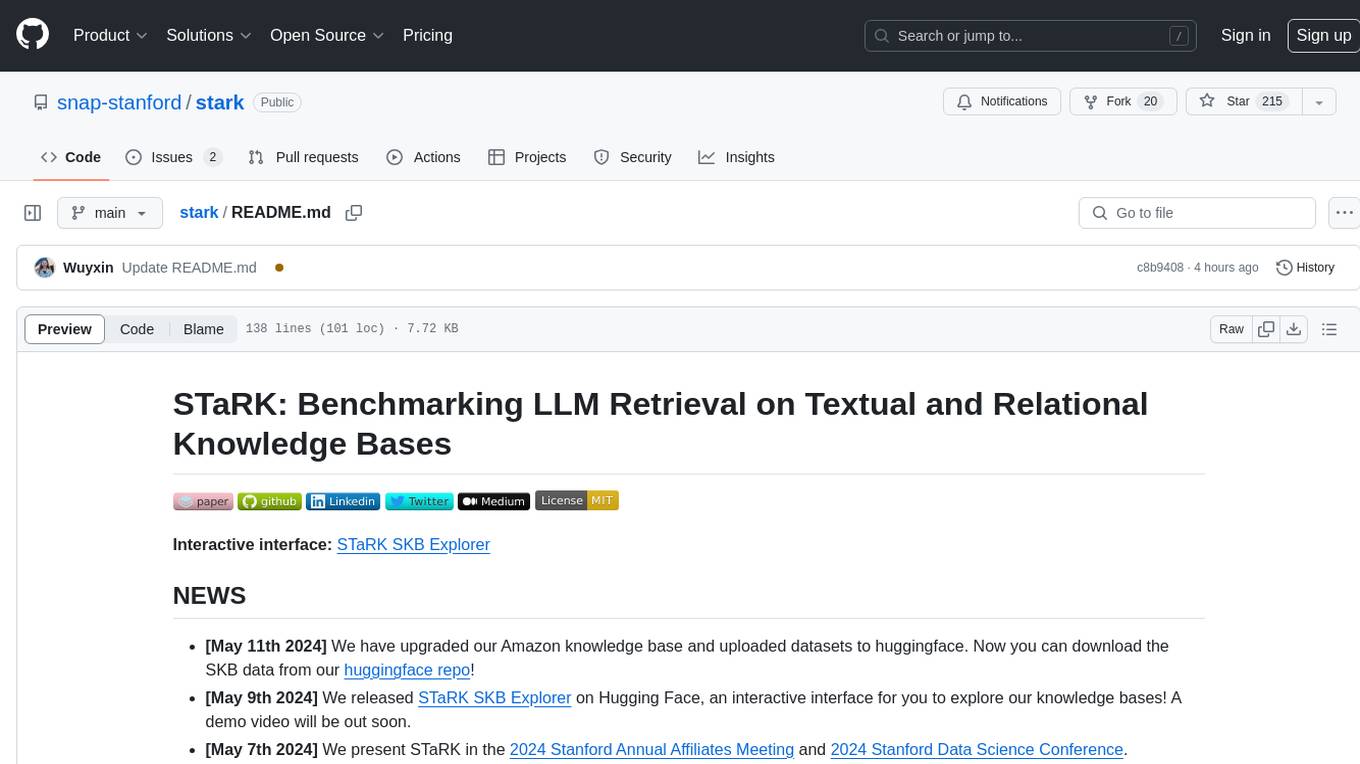
stark
STaRK is a large-scale semi-structure retrieval benchmark on Textual and Relational Knowledge Bases. It provides natural-sounding and practical queries crafted to incorporate rich relational information and complex textual properties, closely mirroring real-life scenarios. The benchmark aims to assess how effectively large language models can handle the interplay between textual and relational requirements in queries, using three diverse knowledge bases constructed from public sources.
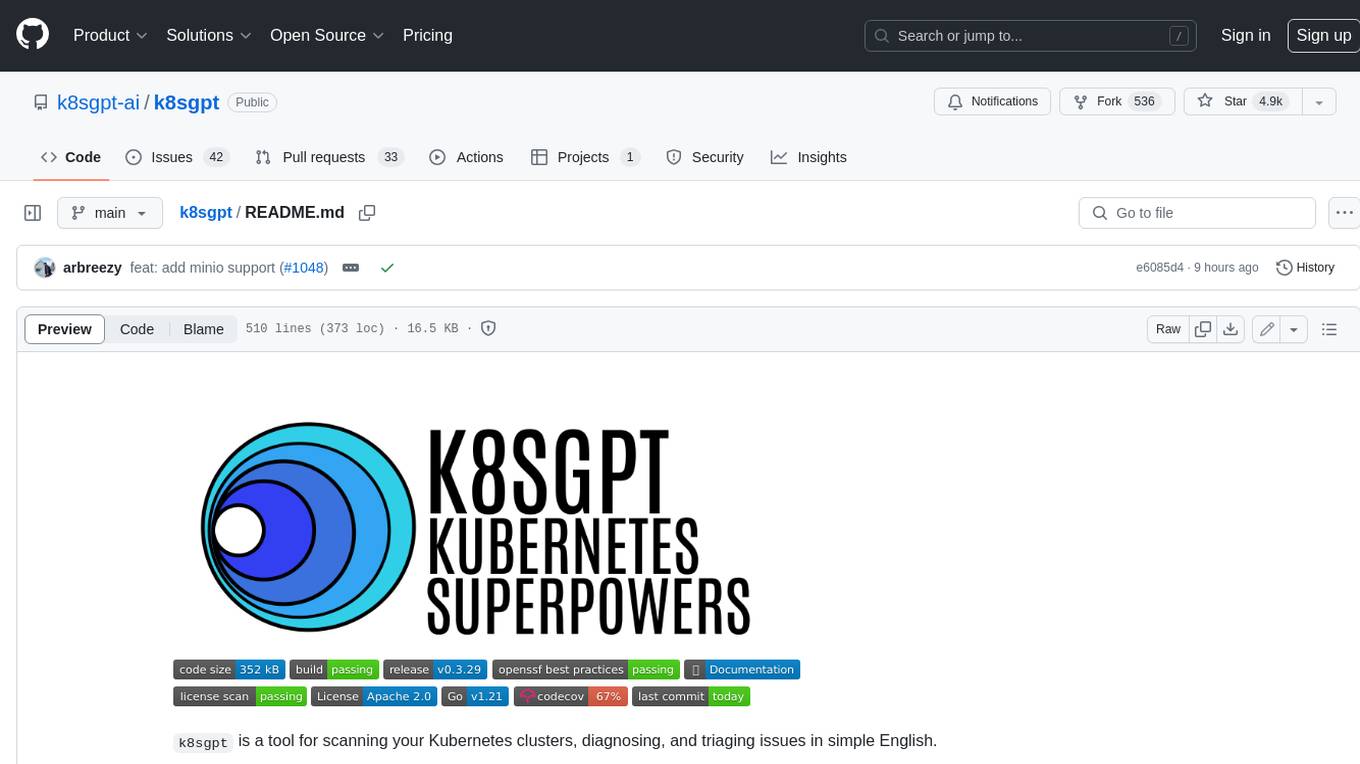
k8sgpt
K8sGPT is a tool for scanning your Kubernetes clusters, diagnosing, and triaging issues in simple English. It has SRE experience codified into its analyzers and helps to pull out the most relevant information to enrich it with AI.
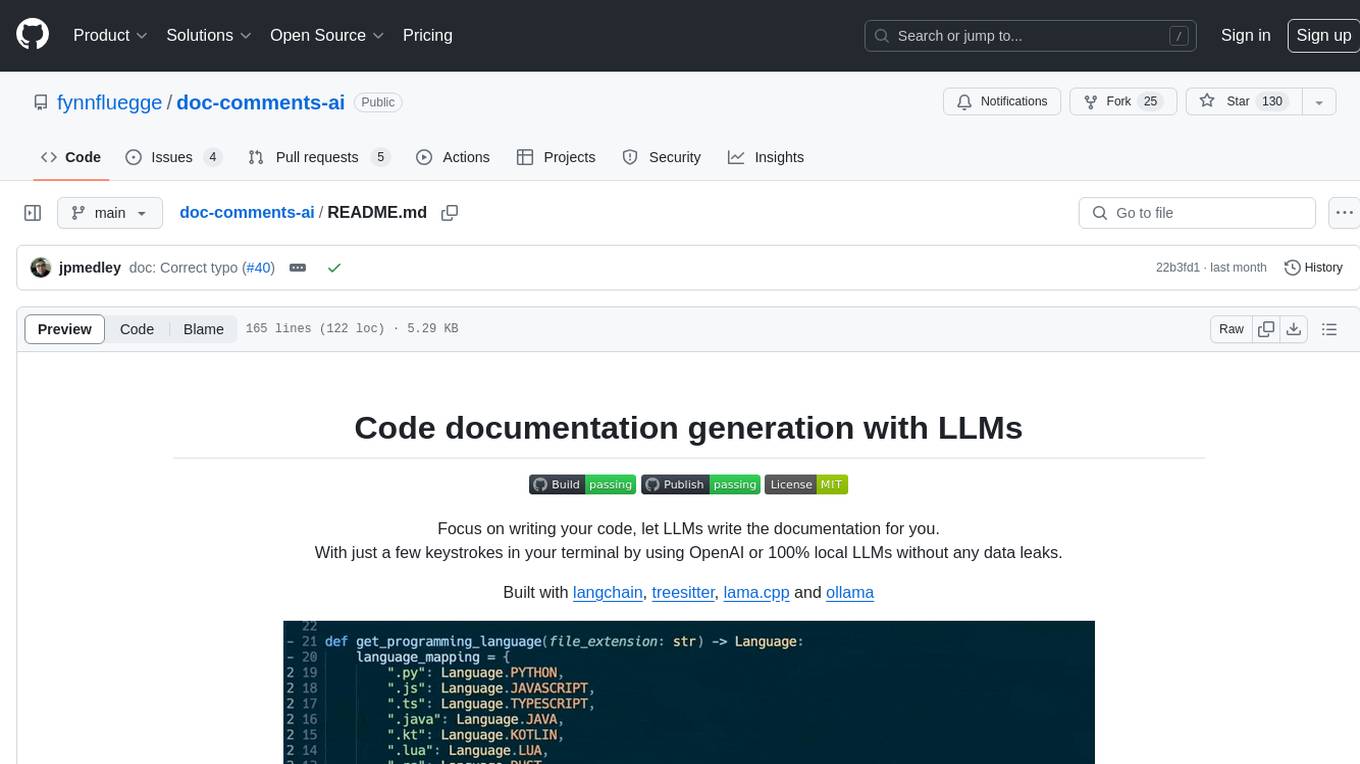
doc-comments-ai
doc-comments-ai is a tool designed to automatically generate code documentation using language models. It allows users to easily create documentation comment blocks for methods in various programming languages such as Python, Typescript, Javascript, Java, Rust, and more. The tool supports both OpenAI and local LLMs, ensuring data privacy and security. Users can generate documentation comments for methods in files, inline comments in method bodies, and choose from different models like GPT-3.5-Turbo, GPT-4, and Azure OpenAI. Additionally, the tool provides support for Treesitter integration and offers guidance on selecting the appropriate model for comprehensive documentation needs.
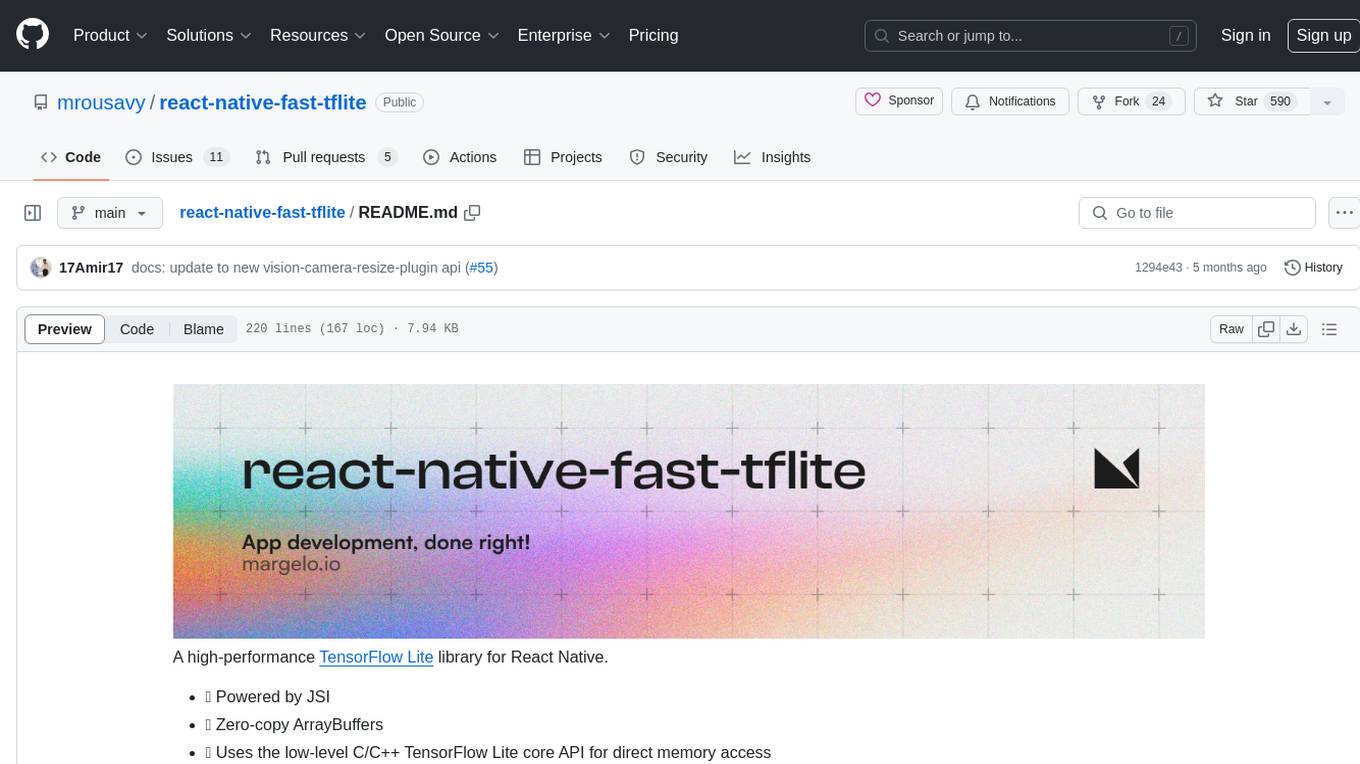
react-native-fast-tflite
A high-performance TensorFlow Lite library for React Native that utilizes JSI for power, zero-copy ArrayBuffers for efficiency, and low-level C/C++ TensorFlow Lite core API for direct memory access. It supports swapping out TensorFlow Models at runtime and GPU-accelerated delegates like CoreML/Metal/OpenGL. Easy VisionCamera integration allows for seamless usage. Users can load TensorFlow Lite models, interpret input and output data, and utilize GPU Delegates for faster computation. The library is suitable for real-time object detection, image classification, and other machine learning tasks in React Native applications.
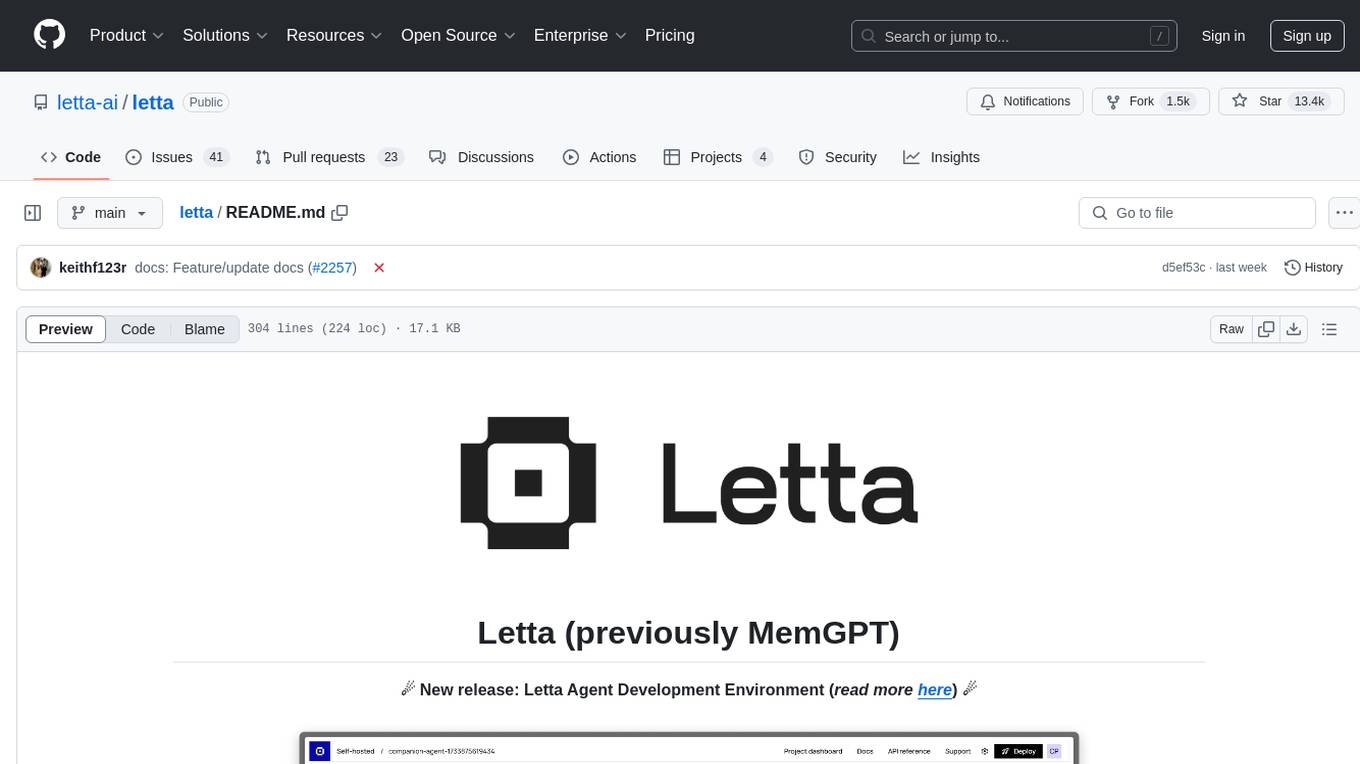
letta
Letta is an open source framework for building stateful LLM applications. It allows users to build stateful agents with advanced reasoning capabilities and transparent long-term memory. The framework is white box and model-agnostic, enabling users to connect to various LLM API backends. Letta provides a graphical interface, the Letta ADE, for creating, deploying, interacting, and observing with agents. Users can access Letta via REST API, Python, Typescript SDKs, and the ADE. Letta supports persistence by storing agent data in a database, with PostgreSQL recommended for data migrations. Users can install Letta using Docker or pip, with Docker defaulting to PostgreSQL and pip defaulting to SQLite. Letta also offers a CLI tool for interacting with agents. The project is open source and welcomes contributions from the community.
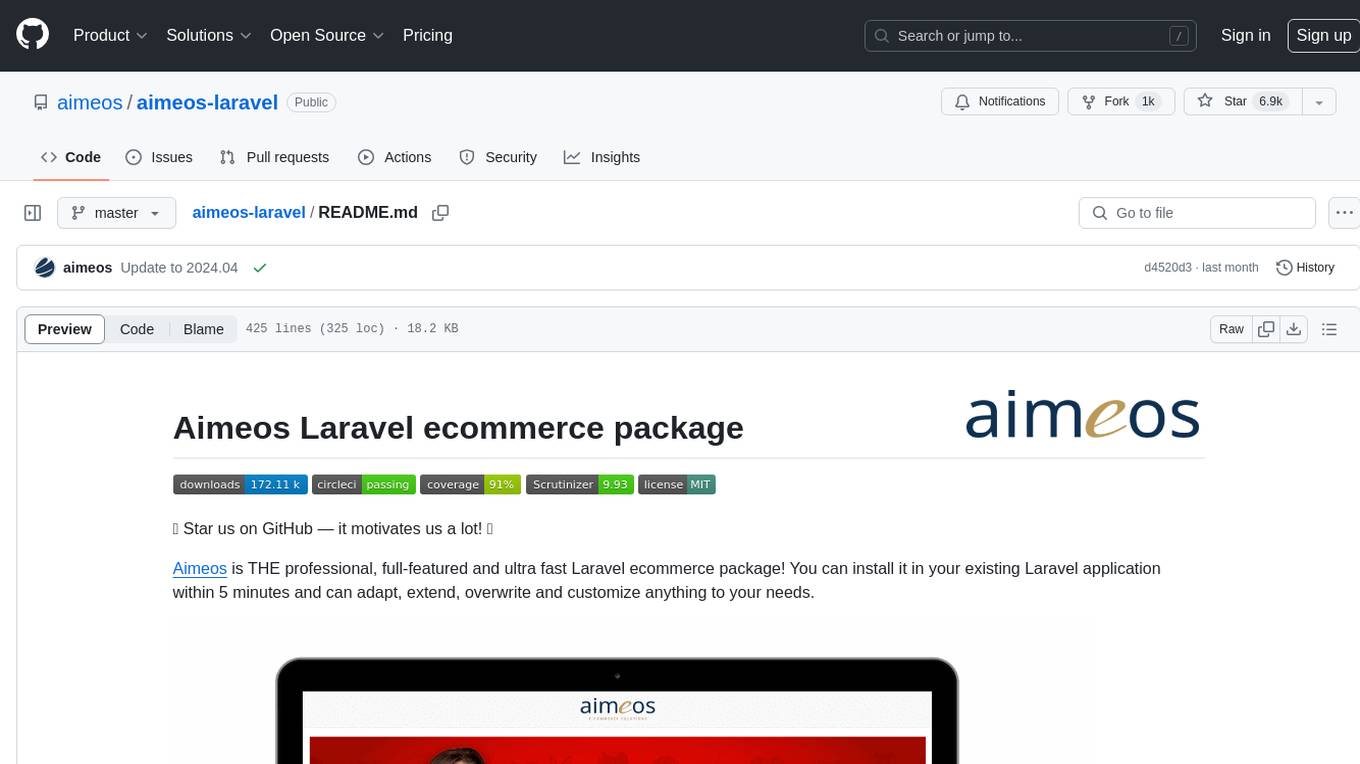
aimeos-laravel
Aimeos Laravel is a professional, full-featured, and ultra-fast Laravel ecommerce package that can be easily integrated into existing Laravel applications. It offers a wide range of features including multi-vendor, multi-channel, and multi-warehouse support, fast performance, support for various product types, subscriptions with recurring payments, multiple payment gateways, full RTL support, flexible pricing options, admin backend, REST and GraphQL APIs, modular structure, SEO optimization, multi-language support, AI-based text translation, mobile optimization, and high-quality source code. The package is highly configurable and extensible, making it suitable for e-commerce SaaS solutions, marketplaces, and online shops with millions of vendors.
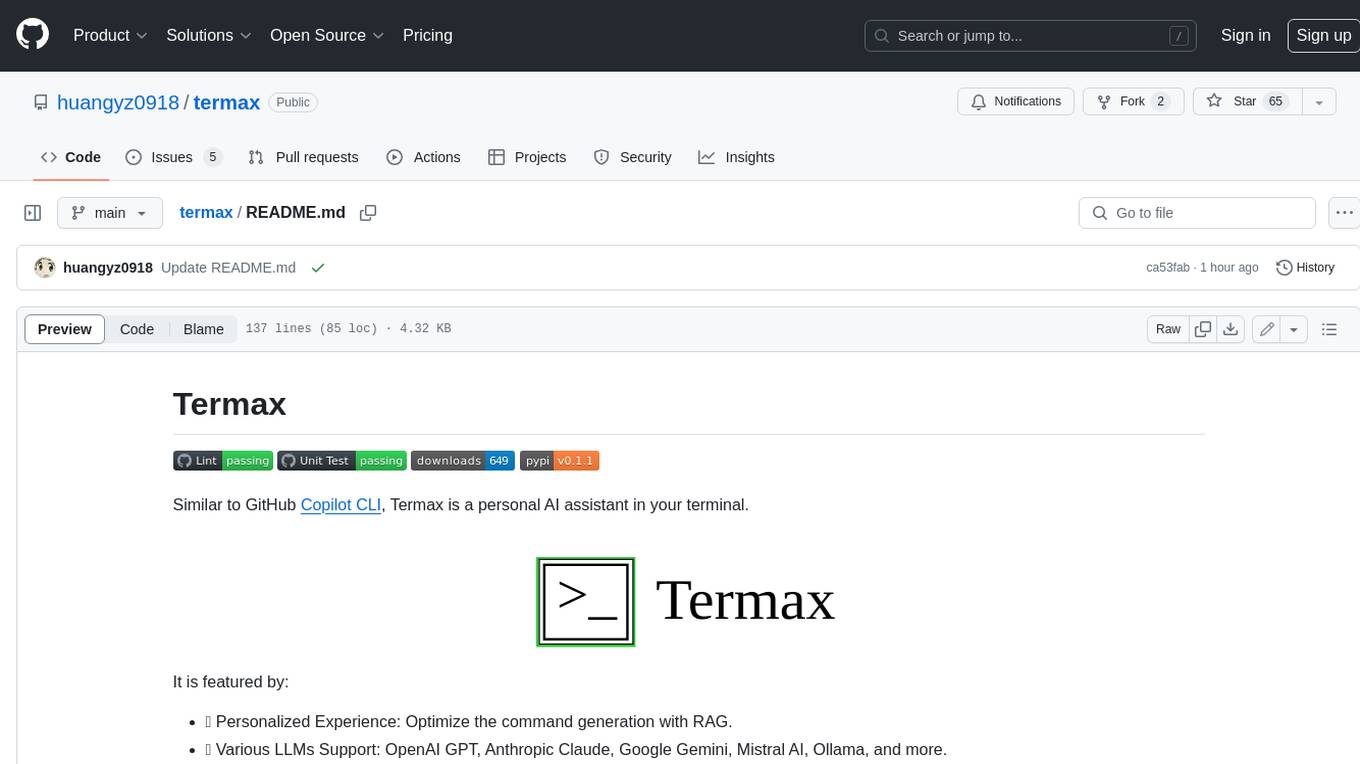
termax
Termax is an LLM agent in your terminal that converts natural language to commands. It is featured by: - Personalized Experience: Optimize the command generation with RAG. - Various LLMs Support: OpenAI GPT, Anthropic Claude, Google Gemini, Mistral AI, and more. - Shell Extensions: Plugin with popular shells like `zsh`, `bash` and `fish`. - Cross Platform: Able to run on Windows, macOS, and Linux.
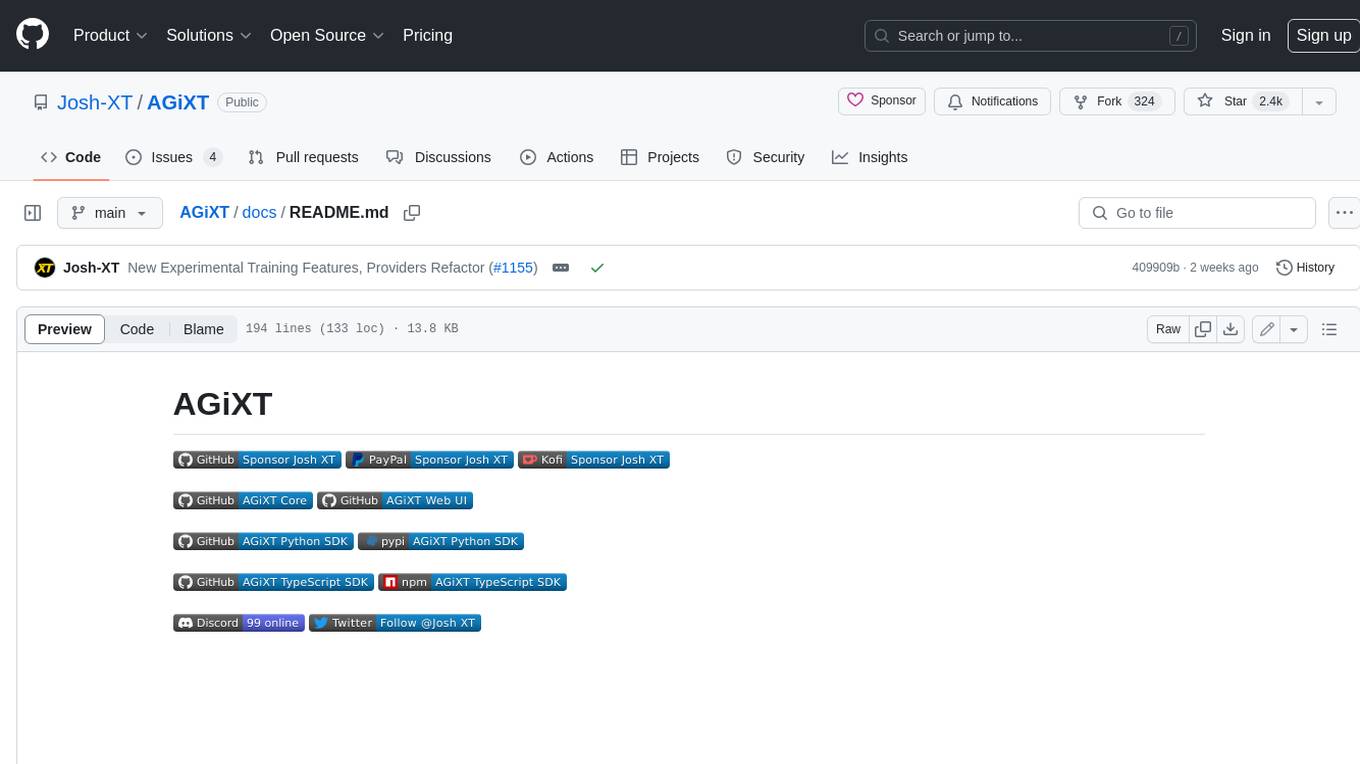
AGiXT
AGiXT is a dynamic Artificial Intelligence Automation Platform engineered to orchestrate efficient AI instruction management and task execution across a multitude of providers. Our solution infuses adaptive memory handling with a broad spectrum of commands to enhance AI's understanding and responsiveness, leading to improved task completion. The platform's smart features, like Smart Instruct and Smart Chat, seamlessly integrate web search, planning strategies, and conversation continuity, transforming the interaction between users and AI. By leveraging a powerful plugin system that includes web browsing and command execution, AGiXT stands as a versatile bridge between AI models and users. With an expanding roster of AI providers, code evaluation capabilities, comprehensive chain management, and platform interoperability, AGiXT is consistently evolving to drive a multitude of applications, affirming its place at the forefront of AI technology.
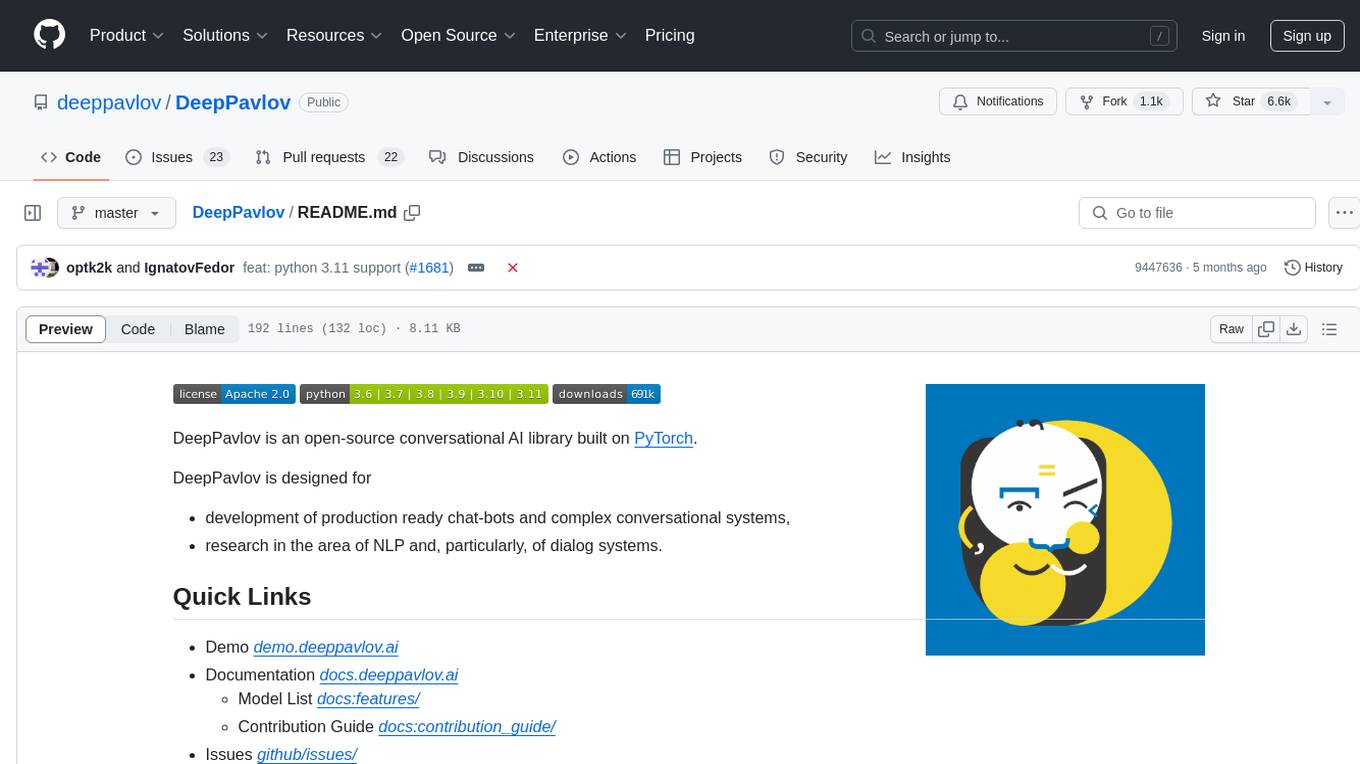
DeepPavlov
DeepPavlov is an open-source conversational AI library built on PyTorch. It is designed for the development of production-ready chatbots and complex conversational systems, as well as for research in the area of NLP and dialog systems. The library offers a wide range of models for tasks such as Named Entity Recognition, Intent/Sentence Classification, Question Answering, Sentence Similarity/Ranking, Syntactic Parsing, and more. DeepPavlov also provides embeddings like BERT, ELMo, and FastText for various languages, along with AutoML capabilities and integrations with REST API, Socket API, and Amazon AWS.
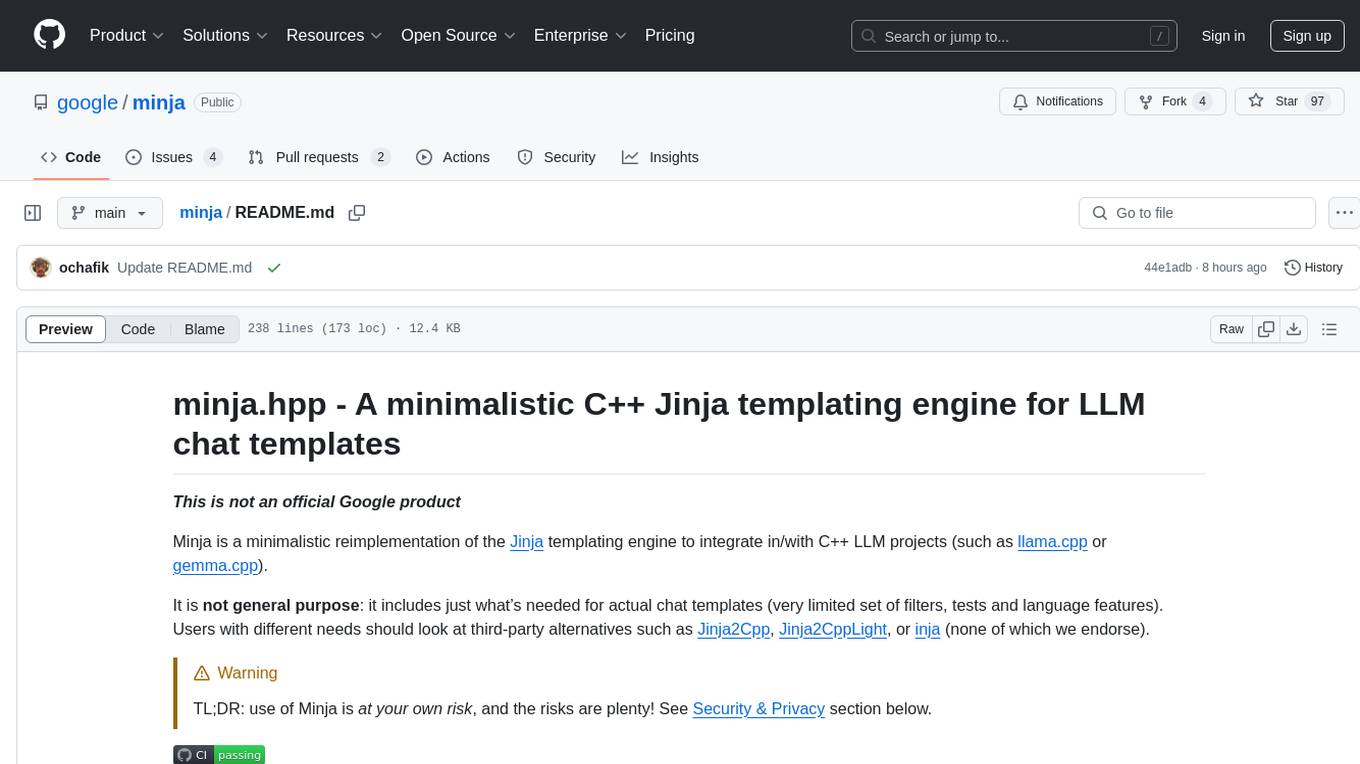
minja
Minja is a minimalistic C++ Jinja templating engine designed specifically for integration with C++ LLM projects, such as llama.cpp or gemma.cpp. It is not a general-purpose tool but focuses on providing a limited set of filters, tests, and language features tailored for chat templates. The library is header-only, requires C++17, and depends only on nlohmann::json. Minja aims to keep the codebase small, easy to understand, and offers decent performance compared to Python. Users should be cautious when using Minja due to potential security risks, and it is not intended for producing HTML or JavaScript output.
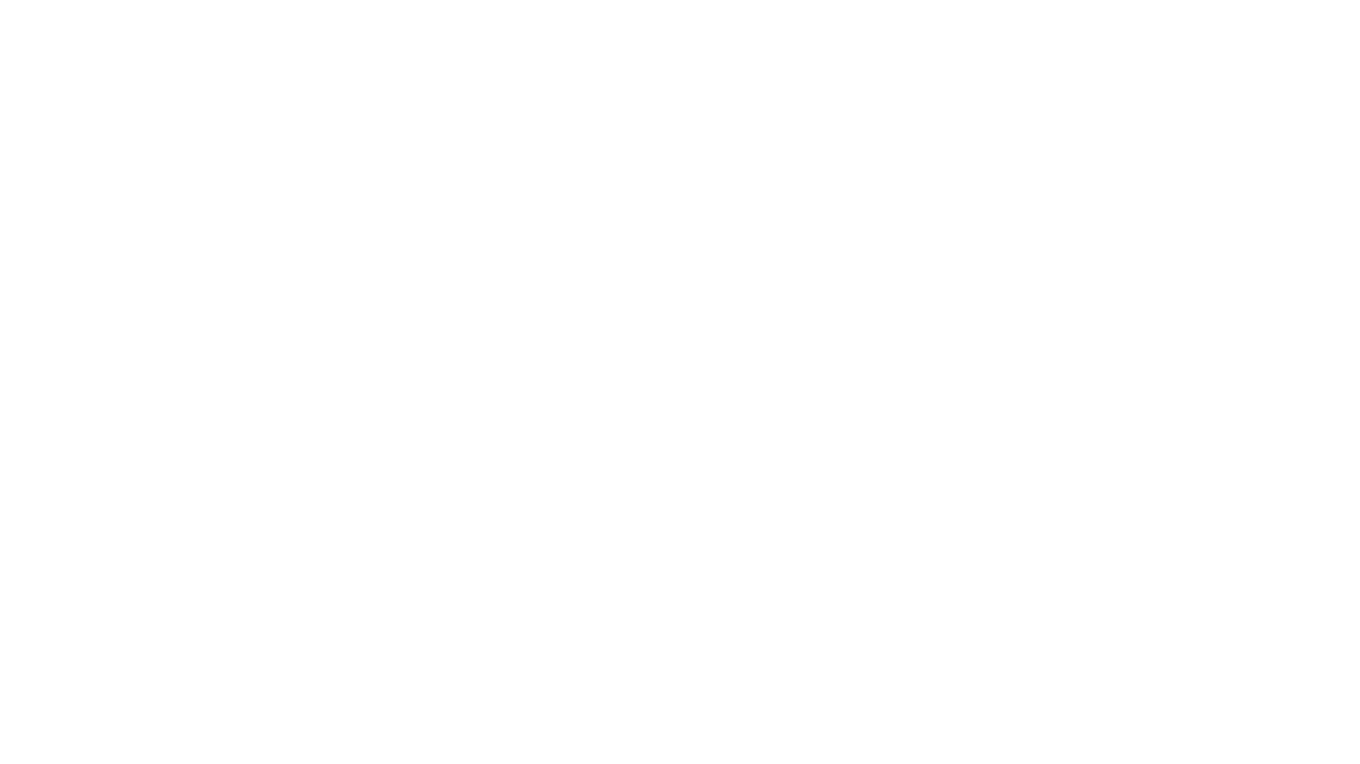
Gemini-API
Gemini-API is a reverse-engineered asynchronous Python wrapper for Google Gemini web app (formerly Bard). It provides features like persistent cookies, ImageFx support, extension support, classified outputs, official flavor, and asynchronous operation. The tool allows users to generate contents from text or images, have conversations across multiple turns, retrieve images in response, generate images with ImageFx, save images to local files, use Gemini extensions, check and switch reply candidates, and control log level.
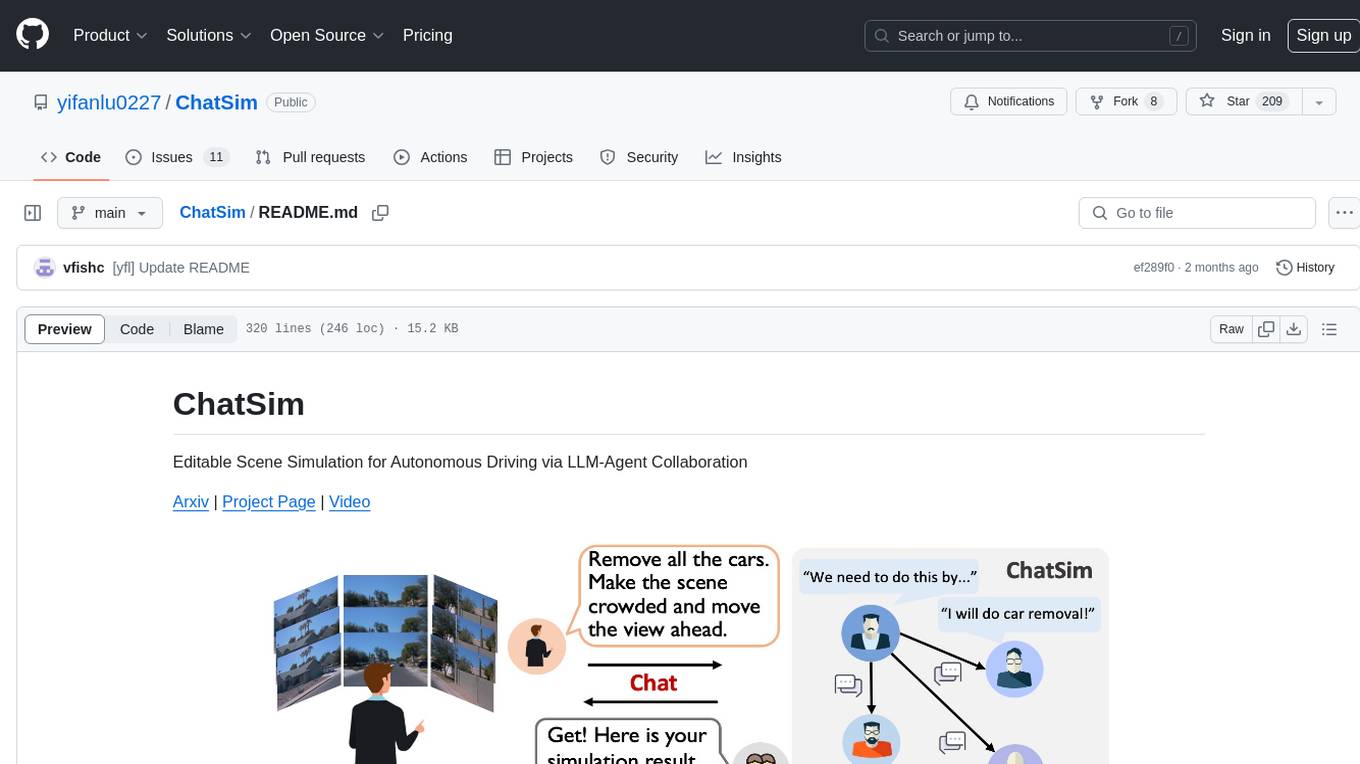
ChatSim
ChatSim is a tool designed for editable scene simulation for autonomous driving via LLM-Agent collaboration. It provides functionalities for setting up the environment, installing necessary dependencies like McNeRF and Inpainting tools, and preparing data for simulation. Users can train models, simulate scenes, and track trajectories for smoother and more realistic results. The tool integrates with Blender software and offers options for training McNeRF models and McLight's skydome estimation network. It also includes a trajectory tracking module for improved trajectory tracking. ChatSim aims to facilitate the simulation of autonomous driving scenarios with collaborative LLM-Agents.
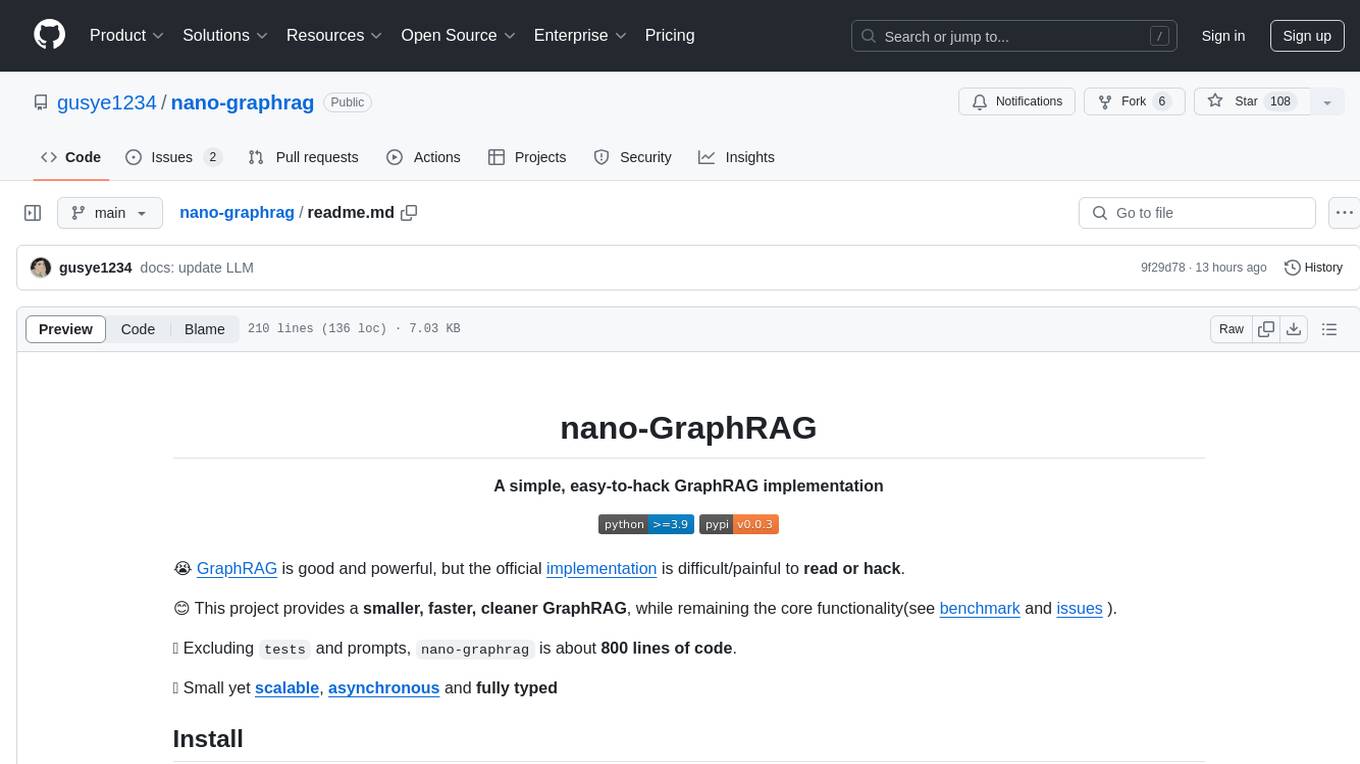
nano-graphrag
nano-GraphRAG is a simple, easy-to-hack implementation of GraphRAG that provides a smaller, faster, and cleaner version of the official implementation. It is about 800 lines of code, small yet scalable, asynchronous, and fully typed. The tool supports incremental insert, async methods, and various parameters for customization. Users can replace storage components and LLM functions as needed. It also allows for embedding function replacement and comes with pre-defined prompts for entity extraction and community reports. However, some features like covariates and global search implementation differ from the original GraphRAG. Future versions aim to address issues related to data source ID, community description truncation, and add new components.
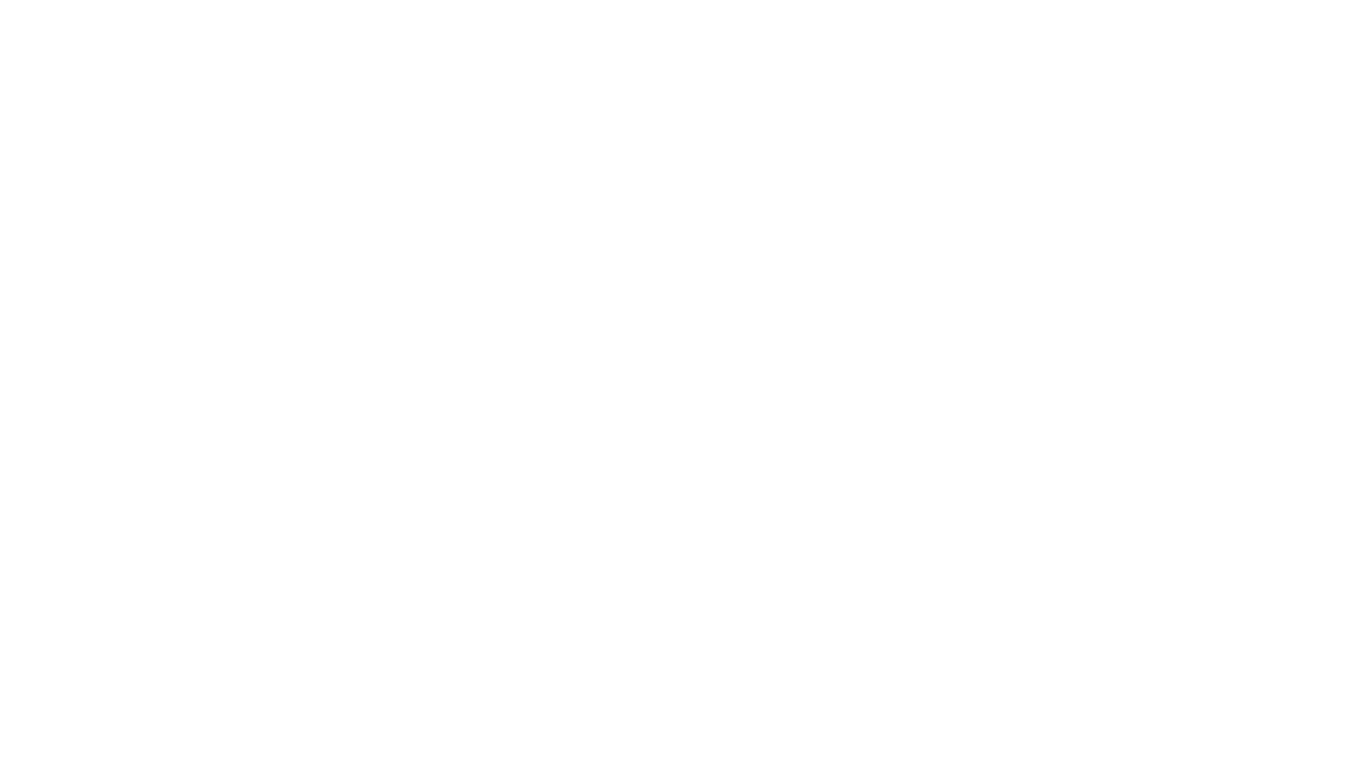
shellChatGPT
ShellChatGPT is a shell wrapper for OpenAI's ChatGPT, DALL-E, Whisper, and TTS, featuring integration with LocalAI, Ollama, Gemini, Mistral, Groq, and GitHub Models. It provides text and chat completions, vision, reasoning, and audio models, voice-in and voice-out chatting mode, text editor interface, markdown rendering support, session management, instruction prompt manager, integration with various service providers, command line completion, file picker dialogs, color scheme personalization, stdin and text file input support, and compatibility with Linux, FreeBSD, MacOS, and Termux for a responsive experience.
For similar tasks
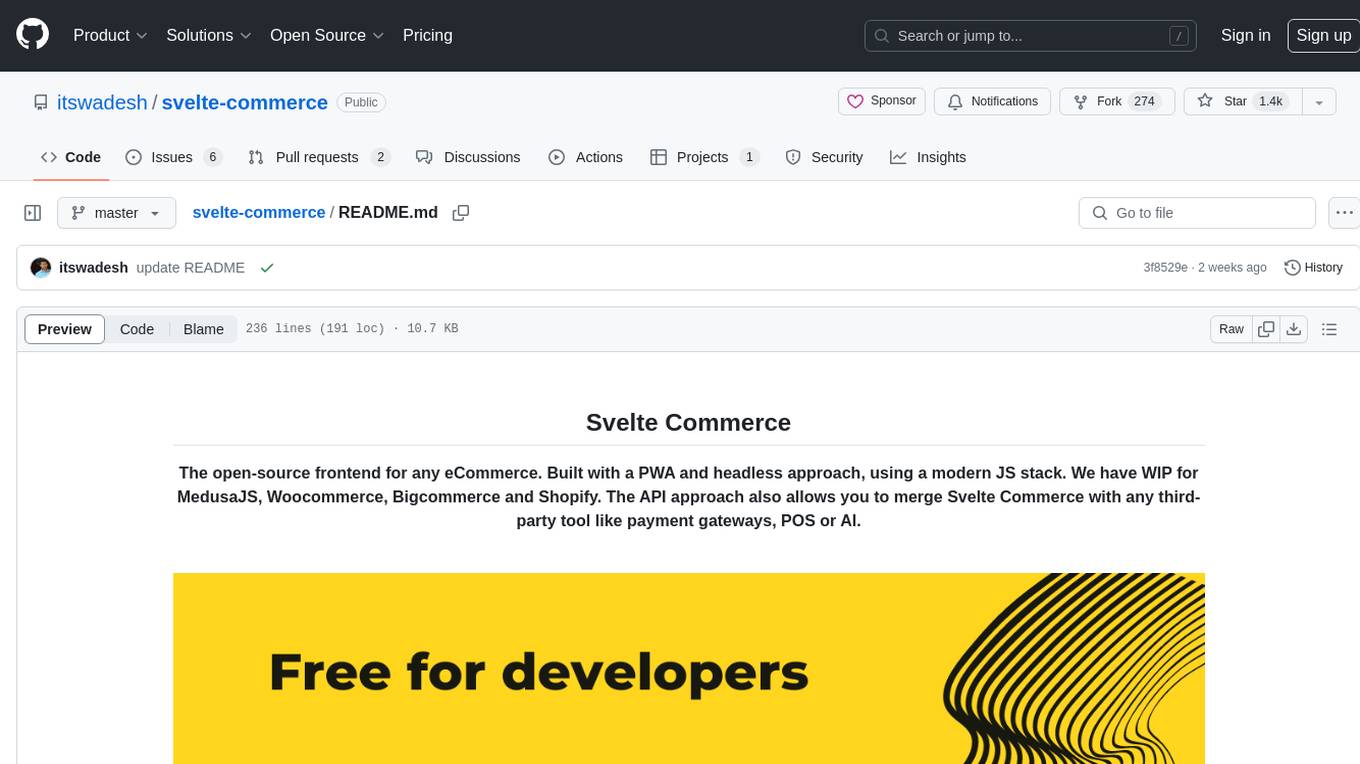
svelte-commerce
Svelte Commerce is an open-source frontend for eCommerce, utilizing a PWA and headless approach with a modern JS stack. It supports integration with various eCommerce backends like MedusaJS, Woocommerce, Bigcommerce, and Shopify. The API flexibility allows seamless connection with third-party tools such as payment gateways, POS systems, and AI services. Svelte Commerce offers essential eCommerce features, is both SSR and SPA, superfast, and free to download and modify. Users can easily deploy it on Netlify or Vercel with zero configuration. The tool provides features like headless commerce, authentication, cart & checkout, TailwindCSS styling, server-side rendering, proxy + API integration, animations, lazy loading, search functionality, faceted filters, and more.
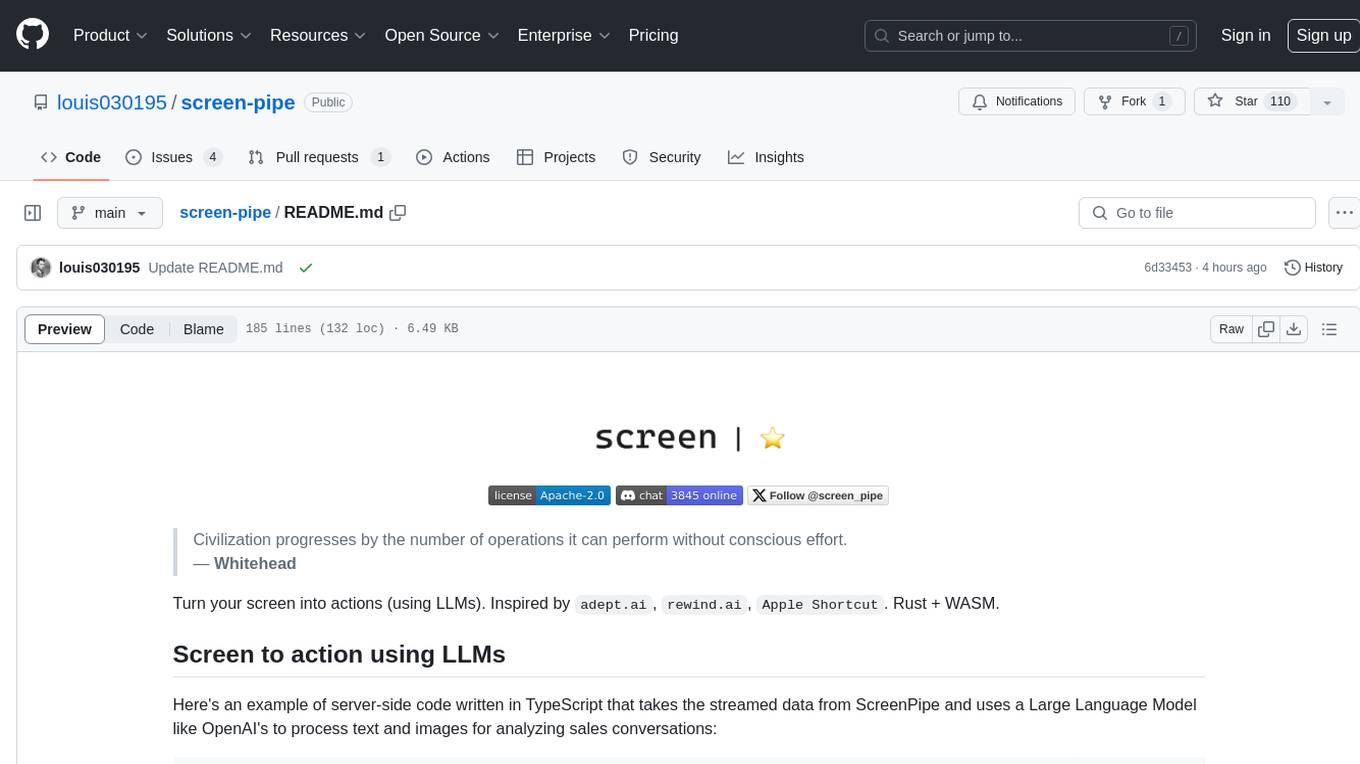
screen-pipe
Screen-pipe is a Rust + WASM tool that allows users to turn their screen into actions using Large Language Models (LLMs). It enables users to record their screen 24/7, extract text from frames, and process text and images for tasks like analyzing sales conversations. The tool is still experimental and aims to simplify the process of recording screens, extracting text, and integrating with various APIs for tasks such as filling CRM data based on screen activities. The project is open-source and welcomes contributions to enhance its functionalities and usability.
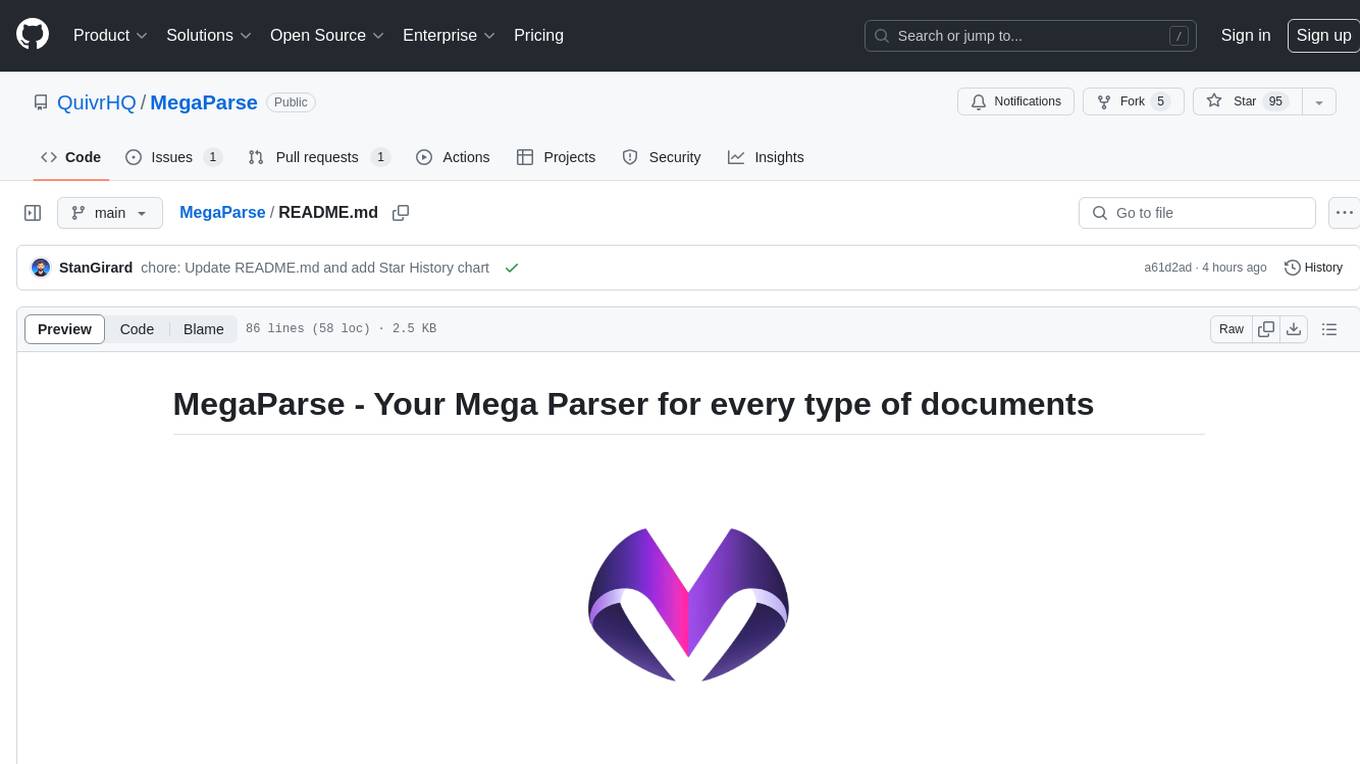
MegaParse
MegaParse is a powerful and versatile parser designed to handle various types of documents such as text, PDFs, Powerpoint presentations, and Word documents with no information loss. It is fast, efficient, and open source, supporting a wide range of file formats. MegaParse ensures compatibility with tables, table of contents, headers, footers, and images, making it a comprehensive solution for document parsing.
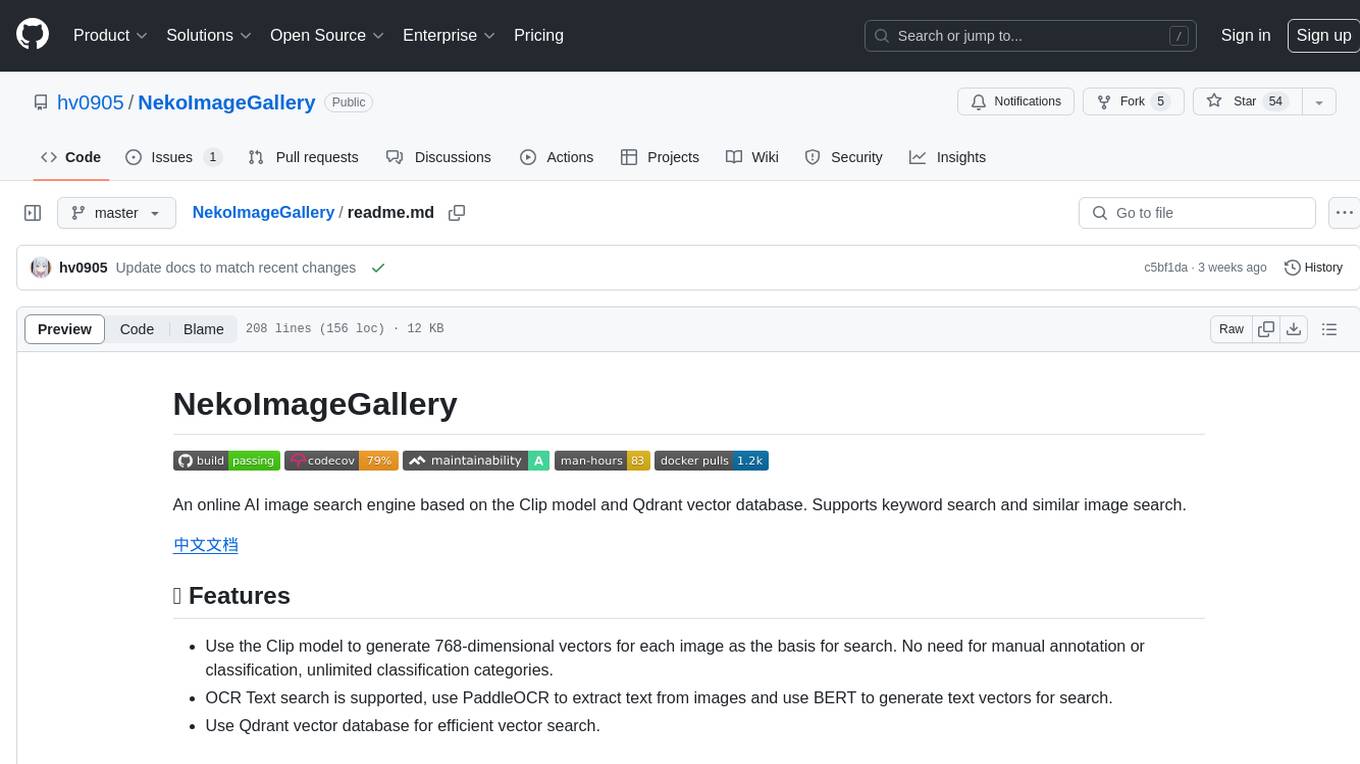
NekoImageGallery
NekoImageGallery is an online AI image search engine that utilizes the Clip model and Qdrant vector database. It supports keyword search and similar image search. The tool generates 768-dimensional vectors for each image using the Clip model, supports OCR text search using PaddleOCR, and efficiently searches vectors using the Qdrant vector database. Users can deploy the tool locally or via Docker, with options for metadata storage using Qdrant database or local file storage. The tool provides API documentation through FastAPI's built-in Swagger UI and can be used for tasks like image search, text extraction, and vector search.
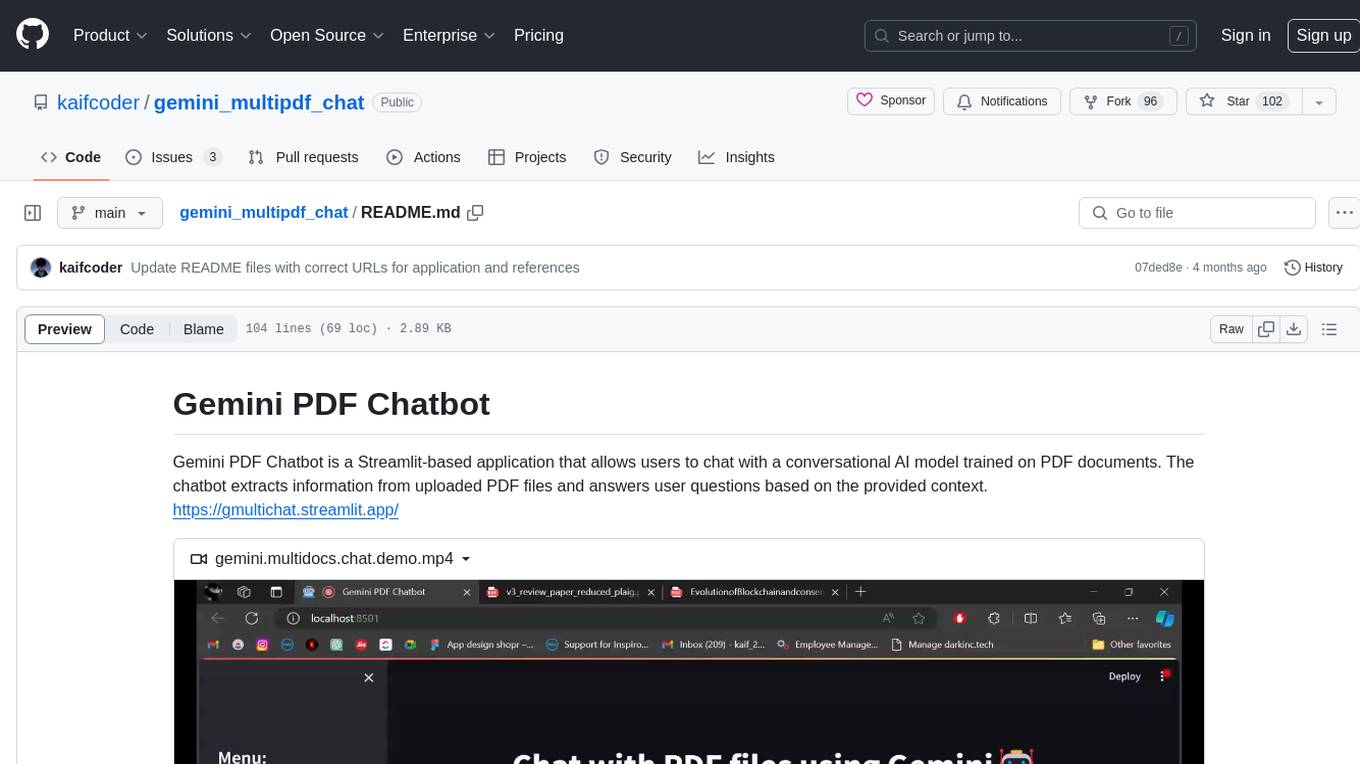
gemini_multipdf_chat
Gemini PDF Chatbot is a Streamlit-based application that allows users to chat with a conversational AI model trained on PDF documents. The chatbot extracts information from uploaded PDF files and answers user questions based on the provided context. It features PDF upload, text extraction, conversational AI using the Gemini model, and a chat interface. Users can deploy the application locally or to the cloud, and the project structure includes main application script, environment variable file, requirements, and documentation. Dependencies include PyPDF2, langchain, Streamlit, google.generativeai, and dotenv.
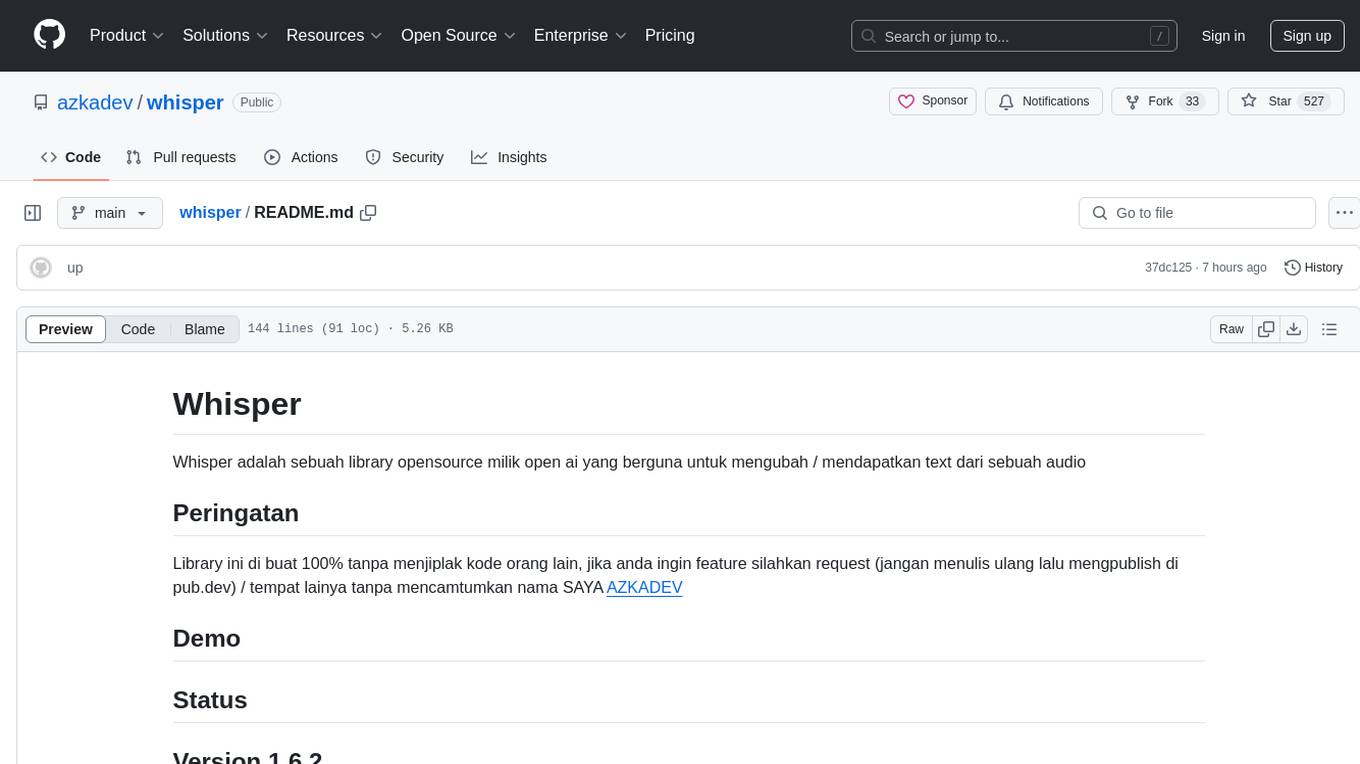
whisper
Whisper is an open-source library by Open AI that converts/extracts text from audio. It is a cross-platform tool that supports real-time transcription of various types of audio/video without manual conversion to WAV format. The library is designed to run on Linux and Android platforms, with plans for expansion to other platforms. Whisper utilizes three frameworks to function: DART for CLI execution, Flutter for mobile app integration, and web/WASM for web application deployment. The tool aims to provide a flexible and easy-to-use solution for transcription tasks across different programs and platforms.
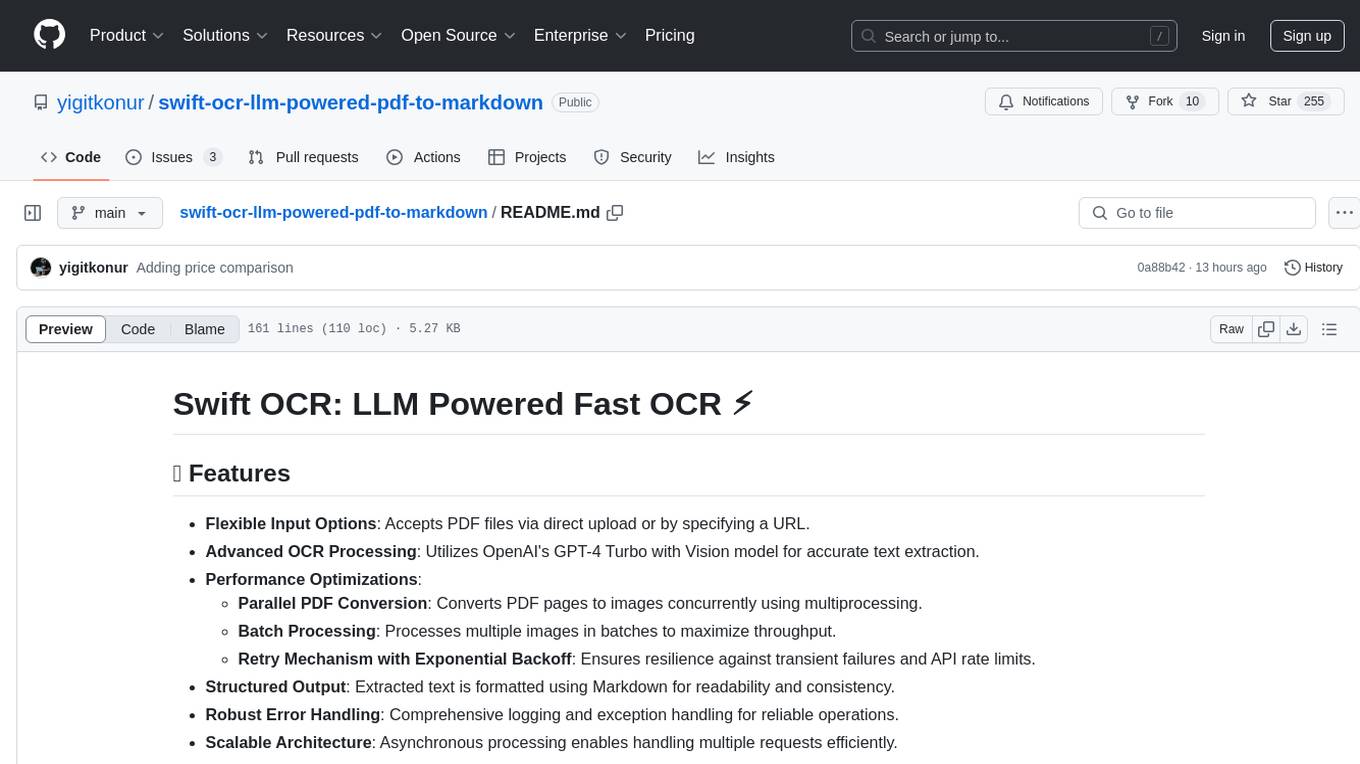
swift-ocr-llm-powered-pdf-to-markdown
Swift OCR is a powerful tool for extracting text from PDF files using OpenAI's GPT-4 Turbo with Vision model. It offers flexible input options, advanced OCR processing, performance optimizations, structured output, robust error handling, and scalable architecture. The tool ensures accurate text extraction, resilience against failures, and efficient handling of multiple requests.
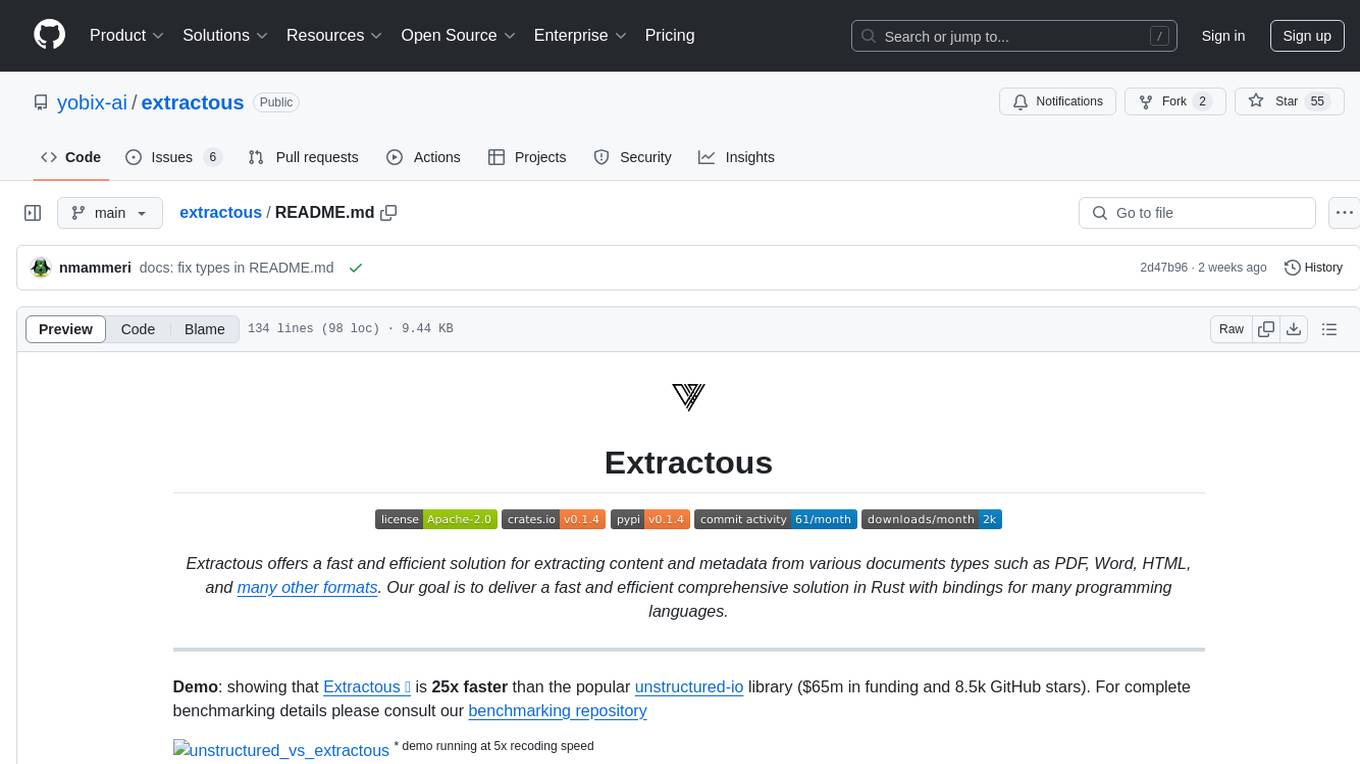
extractous
Extractous offers a fast and efficient solution for extracting content and metadata from various document types such as PDF, Word, HTML, and many other formats. It is built with Rust, providing high performance, memory safety, and multi-threading capabilities. The tool eliminates the need for external services or APIs, making data processing pipelines faster and more efficient. It supports multiple file formats, including Microsoft Office, OpenOffice, PDF, spreadsheets, web documents, e-books, text files, images, and email formats. Extractous provides a clear and simple API for extracting text and metadata content, with upcoming support for JavaScript/TypeScript. It is free for commercial use under the Apache 2.0 License.
For similar jobs
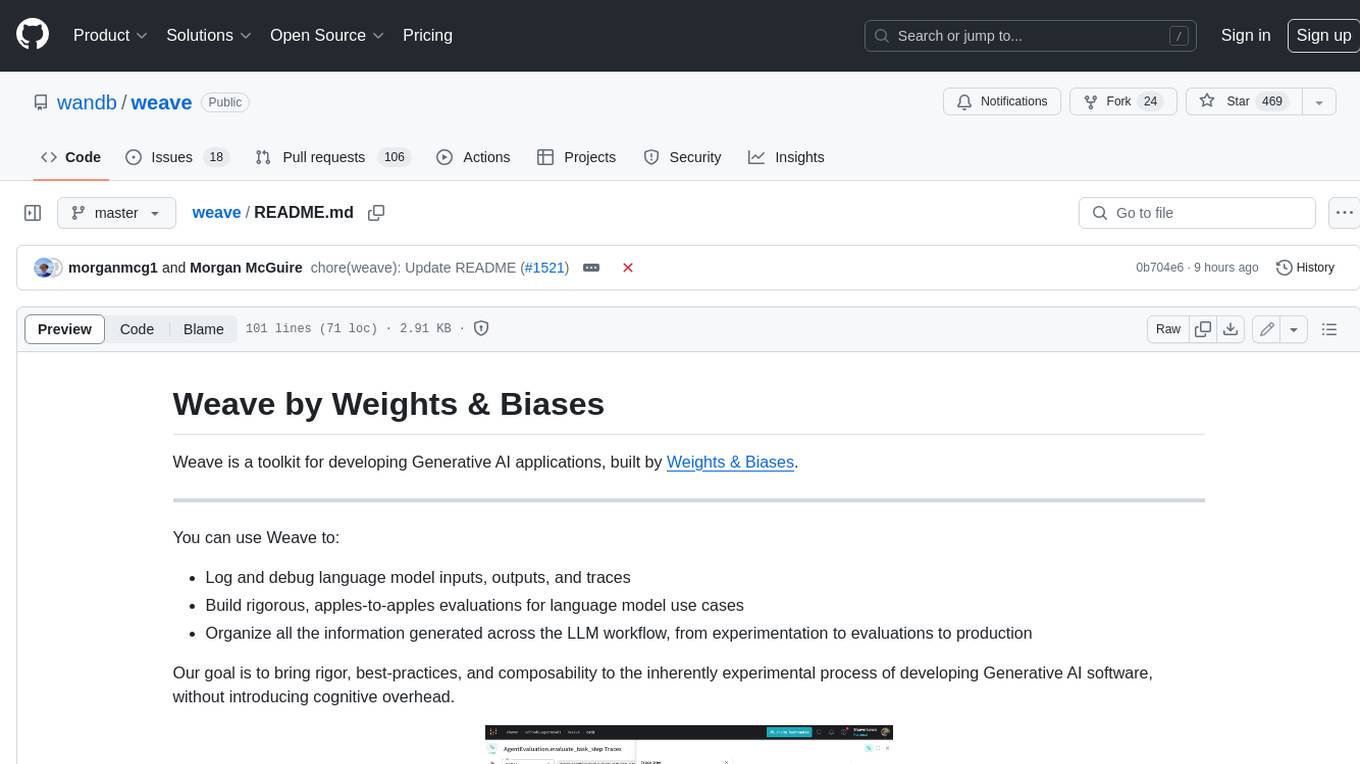
weave
Weave is a toolkit for developing Generative AI applications, built by Weights & Biases. With Weave, you can log and debug language model inputs, outputs, and traces; build rigorous, apples-to-apples evaluations for language model use cases; and organize all the information generated across the LLM workflow, from experimentation to evaluations to production. Weave aims to bring rigor, best-practices, and composability to the inherently experimental process of developing Generative AI software, without introducing cognitive overhead.
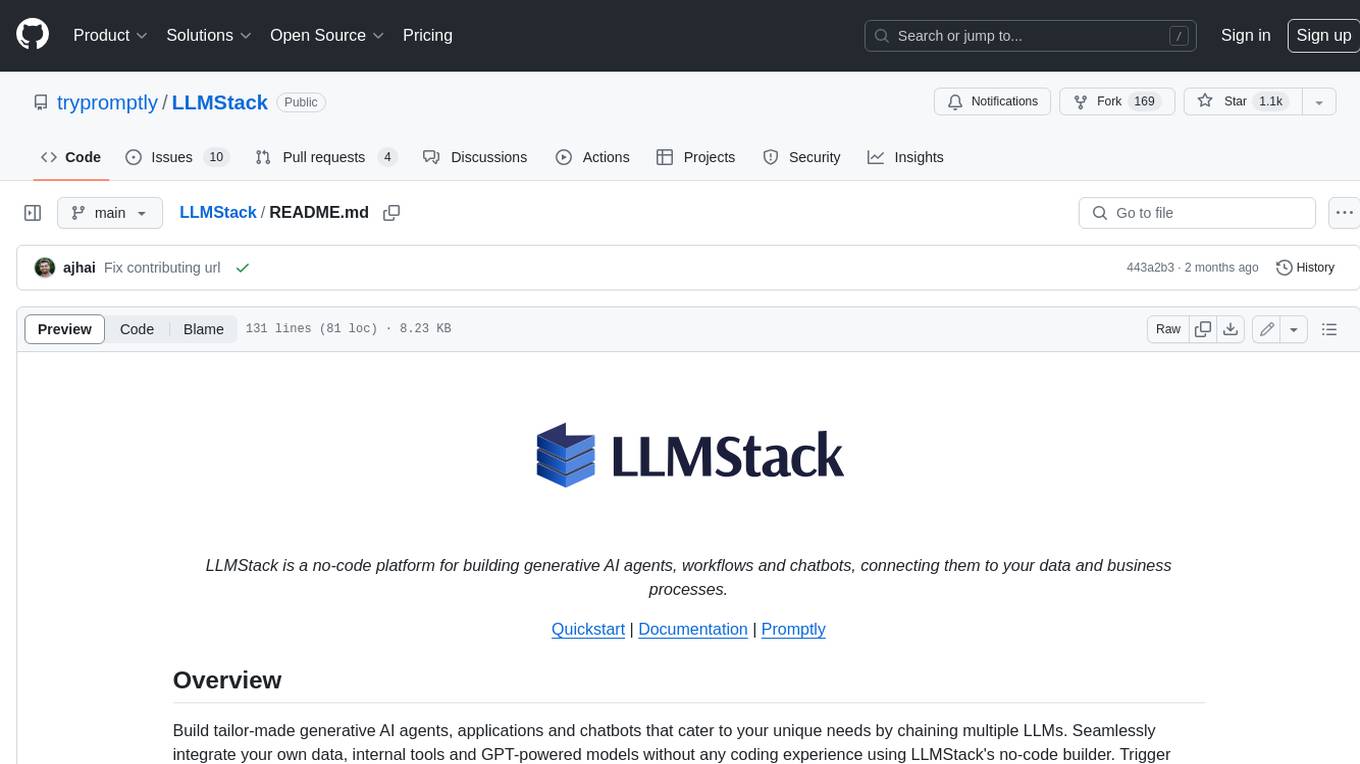
LLMStack
LLMStack is a no-code platform for building generative AI agents, workflows, and chatbots. It allows users to connect their own data, internal tools, and GPT-powered models without any coding experience. LLMStack can be deployed to the cloud or on-premise and can be accessed via HTTP API or triggered from Slack or Discord.
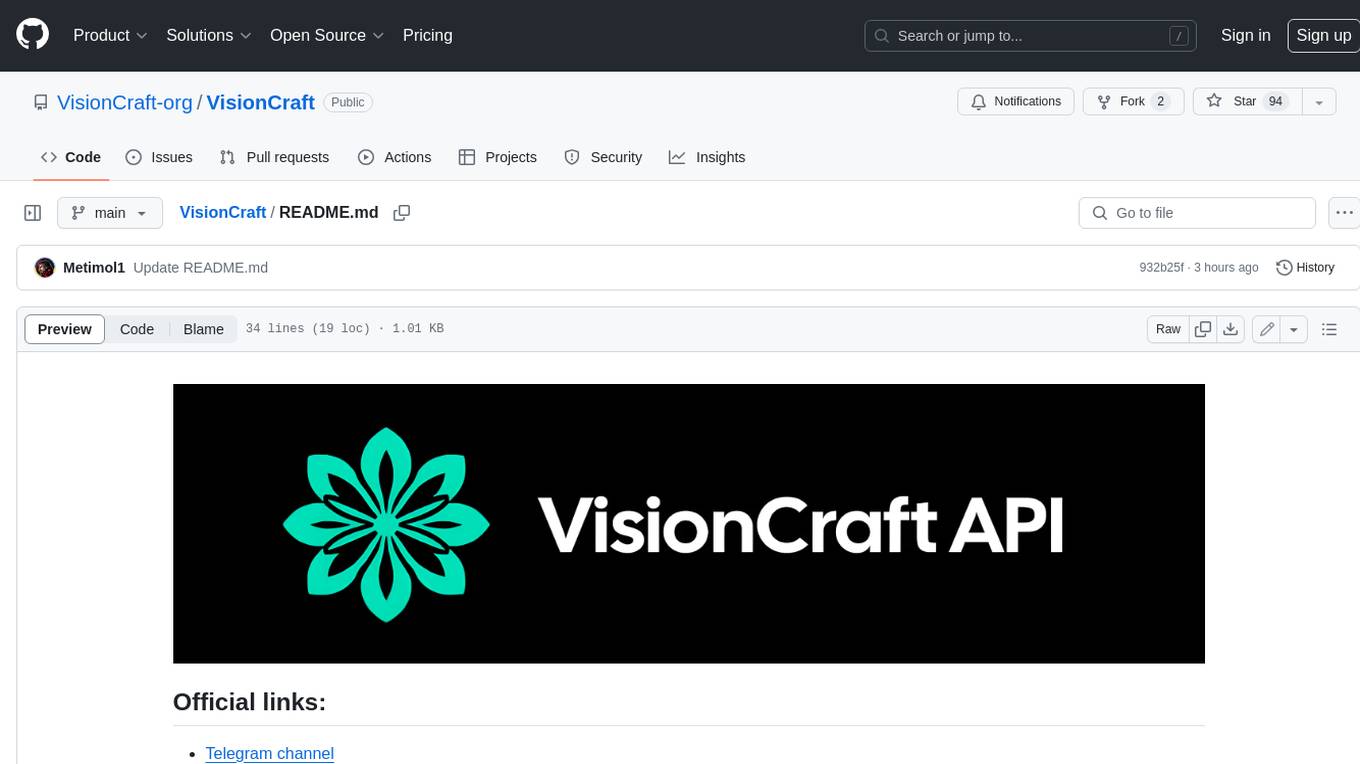
VisionCraft
The VisionCraft API is a free API for using over 100 different AI models. From images to sound.
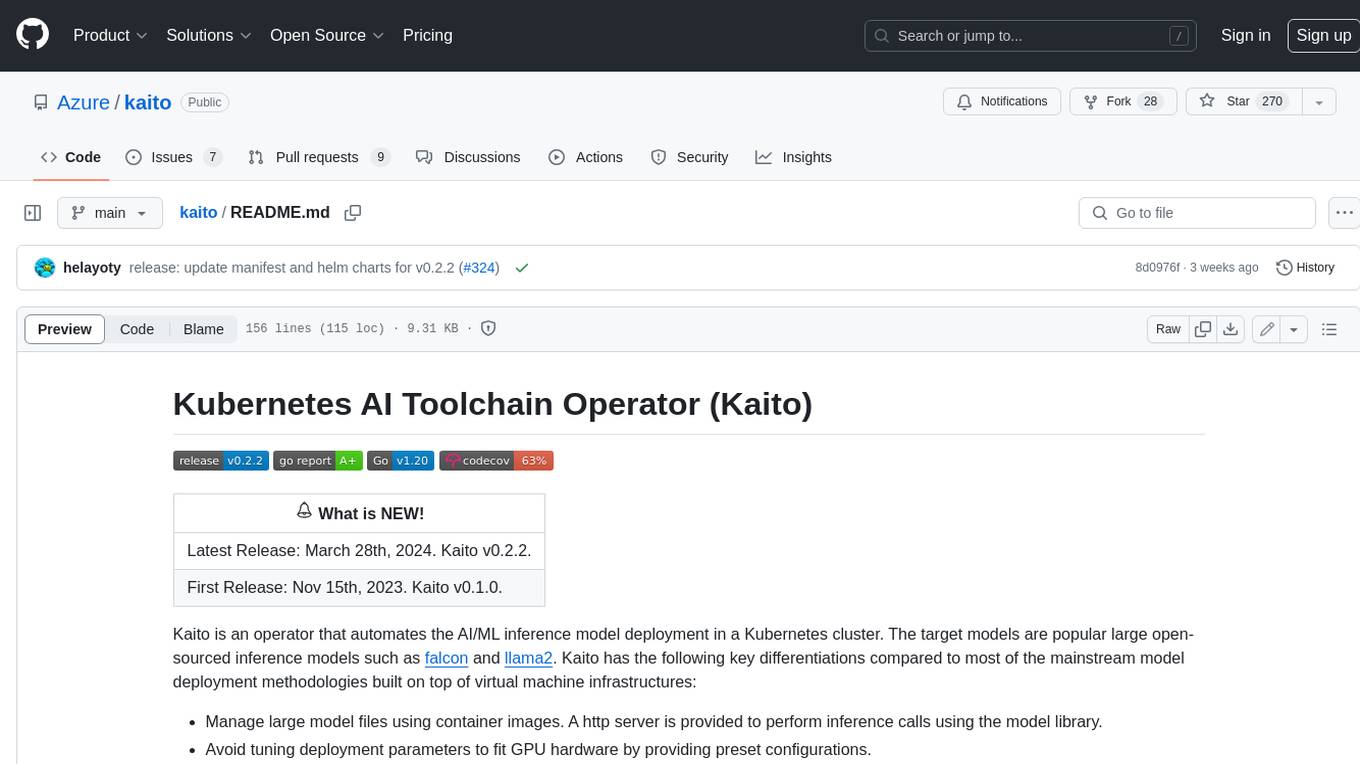
kaito
Kaito is an operator that automates the AI/ML inference model deployment in a Kubernetes cluster. It manages large model files using container images, avoids tuning deployment parameters to fit GPU hardware by providing preset configurations, auto-provisions GPU nodes based on model requirements, and hosts large model images in the public Microsoft Container Registry (MCR) if the license allows. Using Kaito, the workflow of onboarding large AI inference models in Kubernetes is largely simplified.
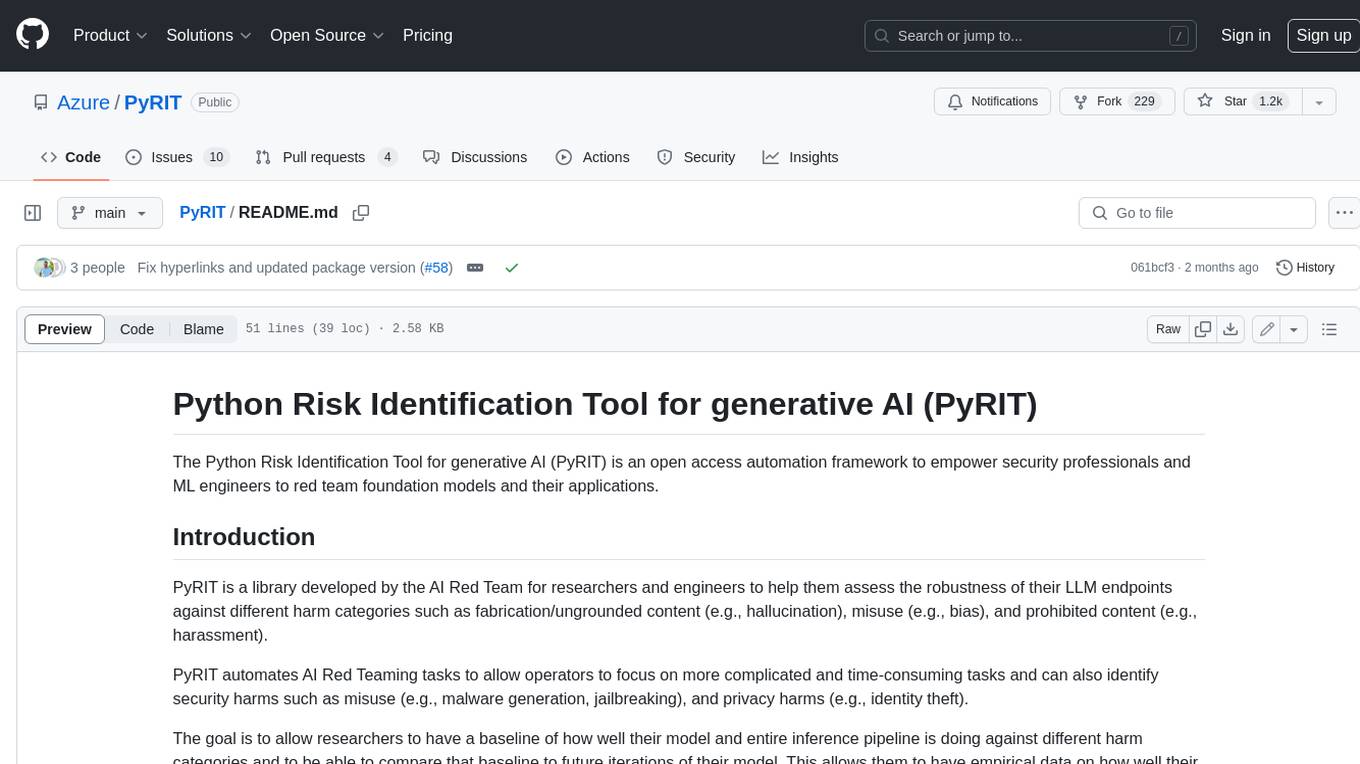
PyRIT
PyRIT is an open access automation framework designed to empower security professionals and ML engineers to red team foundation models and their applications. It automates AI Red Teaming tasks to allow operators to focus on more complicated and time-consuming tasks and can also identify security harms such as misuse (e.g., malware generation, jailbreaking), and privacy harms (e.g., identity theft). The goal is to allow researchers to have a baseline of how well their model and entire inference pipeline is doing against different harm categories and to be able to compare that baseline to future iterations of their model. This allows them to have empirical data on how well their model is doing today, and detect any degradation of performance based on future improvements.
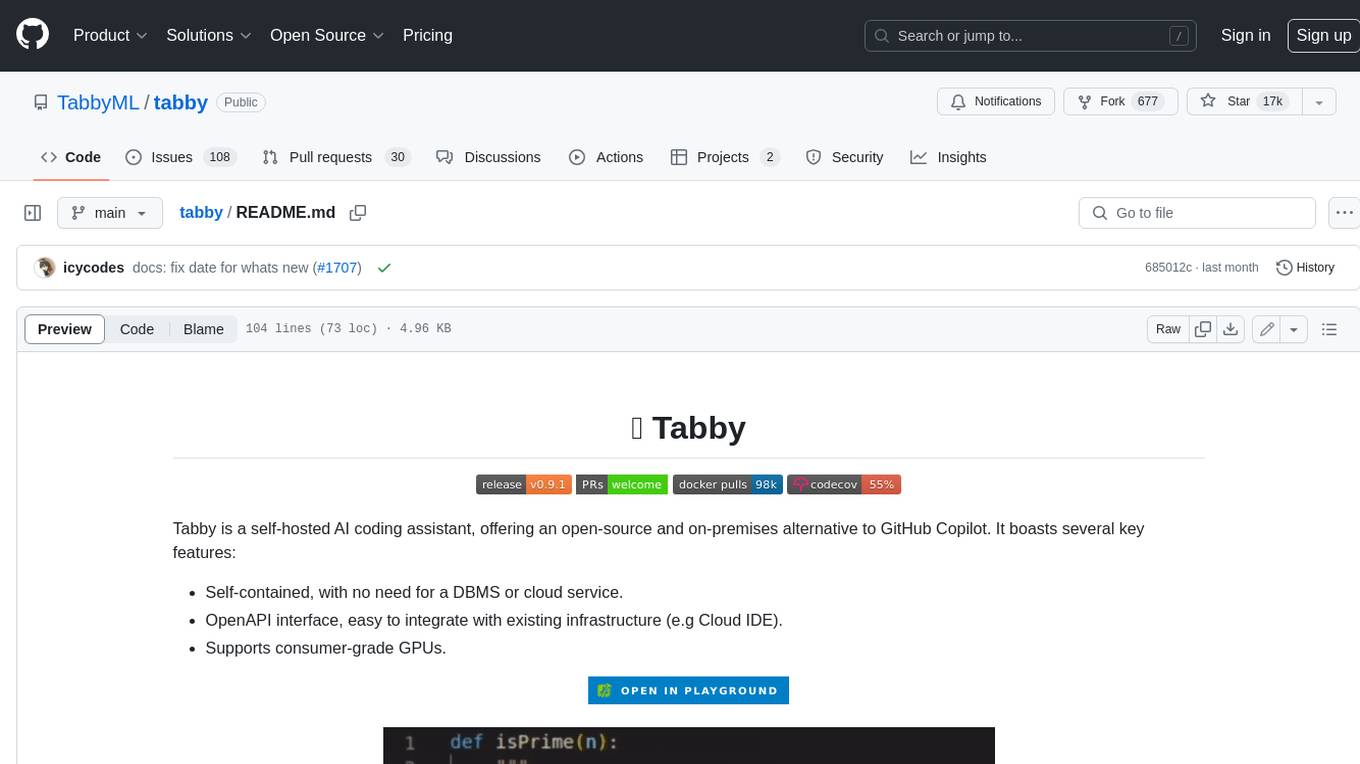
tabby
Tabby is a self-hosted AI coding assistant, offering an open-source and on-premises alternative to GitHub Copilot. It boasts several key features: * Self-contained, with no need for a DBMS or cloud service. * OpenAPI interface, easy to integrate with existing infrastructure (e.g Cloud IDE). * Supports consumer-grade GPUs.
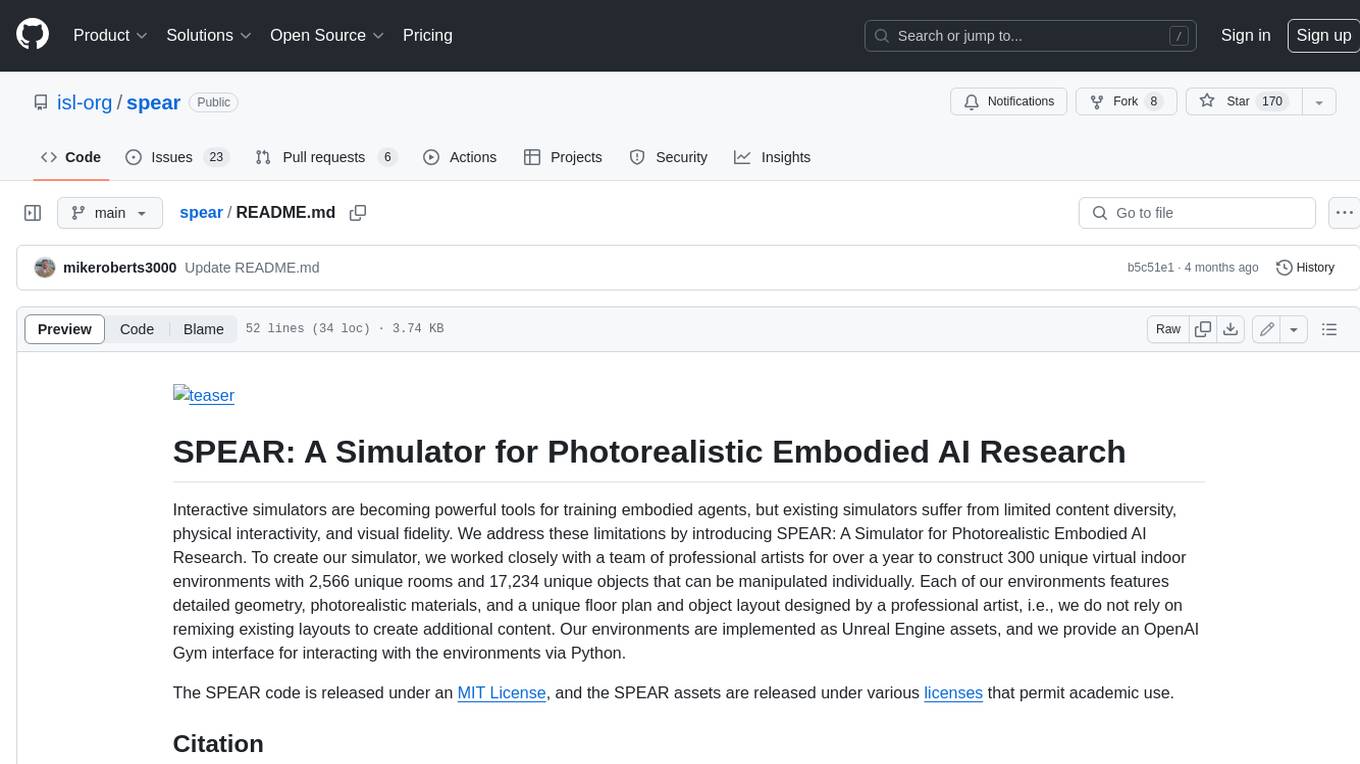
spear
SPEAR (Simulator for Photorealistic Embodied AI Research) is a powerful tool for training embodied agents. It features 300 unique virtual indoor environments with 2,566 unique rooms and 17,234 unique objects that can be manipulated individually. Each environment is designed by a professional artist and features detailed geometry, photorealistic materials, and a unique floor plan and object layout. SPEAR is implemented as Unreal Engine assets and provides an OpenAI Gym interface for interacting with the environments via Python.
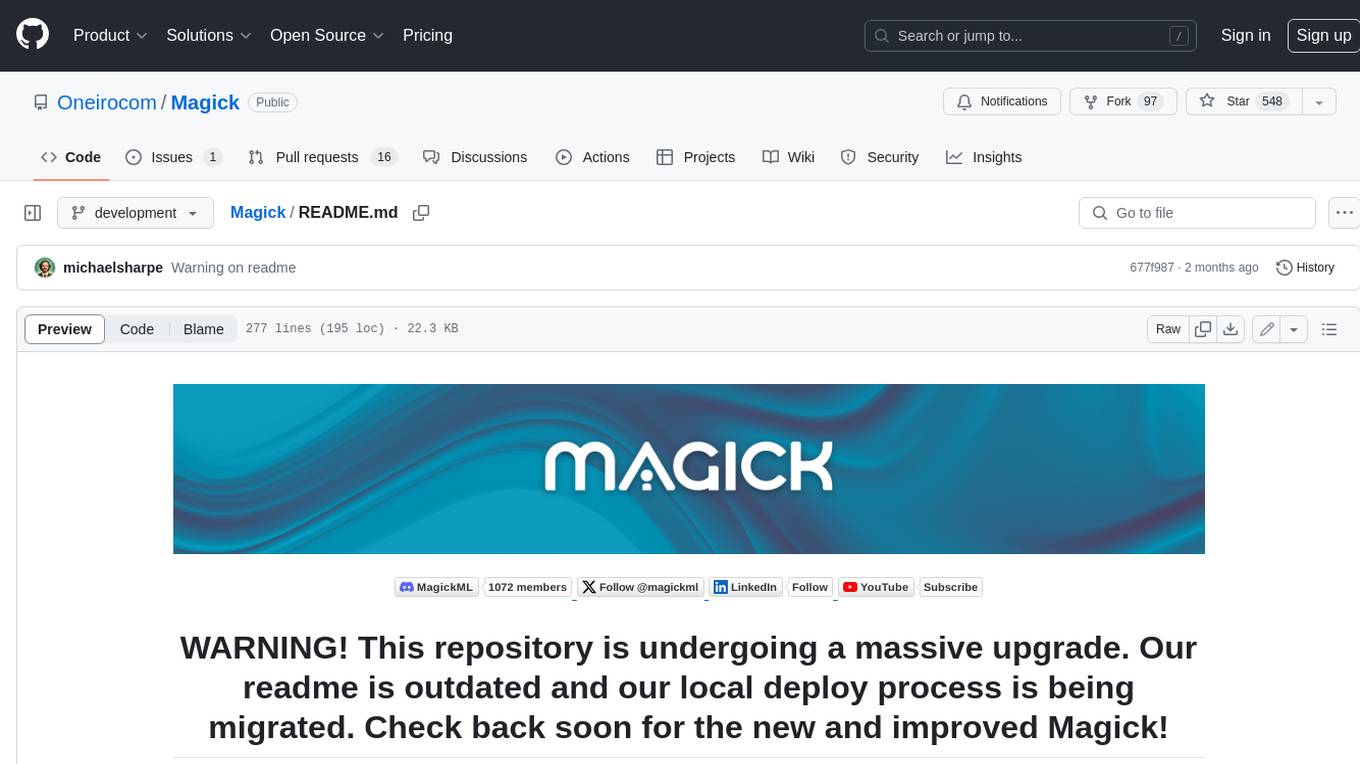
Magick
Magick is a groundbreaking visual AIDE (Artificial Intelligence Development Environment) for no-code data pipelines and multimodal agents. Magick can connect to other services and comes with nodes and templates well-suited for intelligent agents, chatbots, complex reasoning systems and realistic characters.