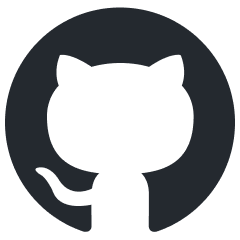
dynamiq
Dynamiq is an orchestration framework for agentic AI and LLM applications
Stars: 73
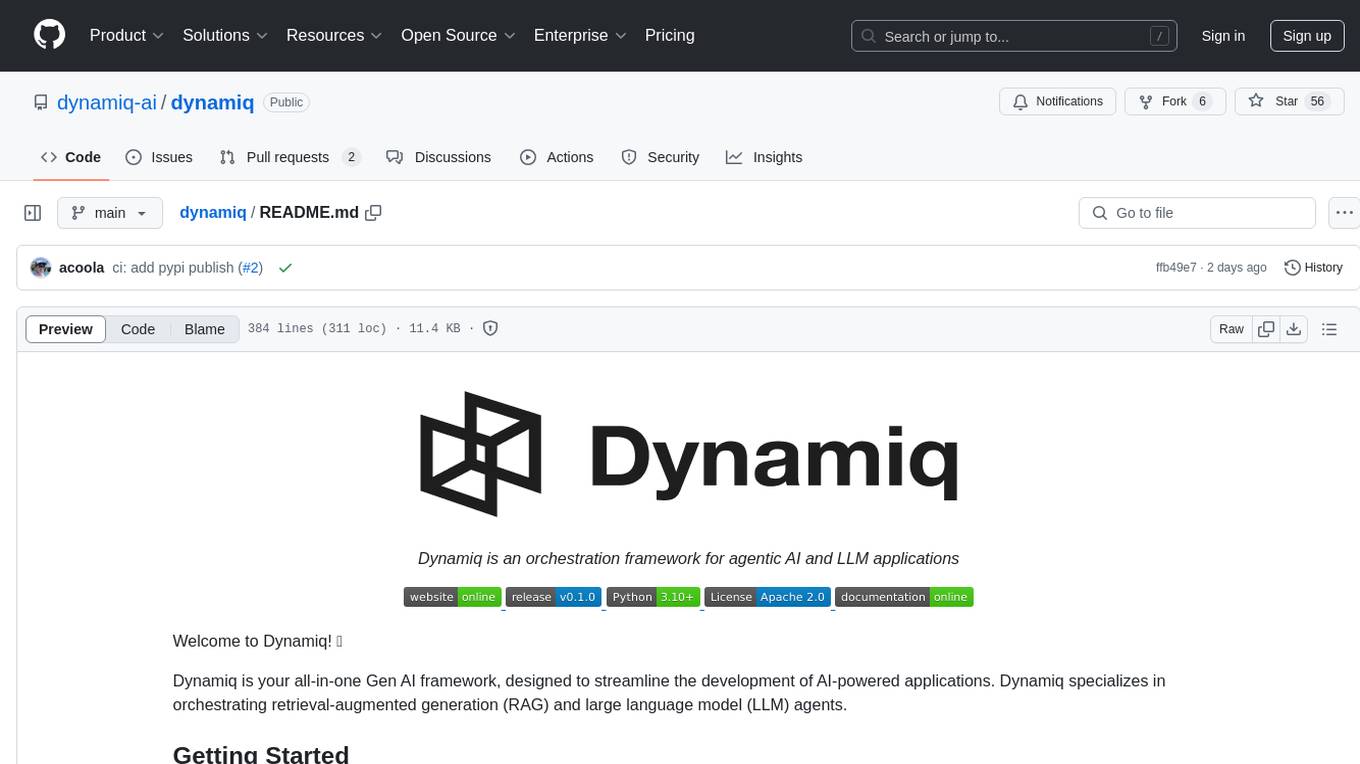
Dynamiq is an orchestration framework designed to streamline the development of AI-powered applications, specializing in orchestrating retrieval-augmented generation (RAG) and large language model (LLM) agents. It provides an all-in-one Gen AI framework for agentic AI and LLM applications, offering tools for multi-agent orchestration, document indexing, and retrieval flows. With Dynamiq, users can easily build and deploy AI solutions for various tasks.
README:
Dynamiq is an orchestration framework for agentic AI and LLM applications
Welcome to Dynamiq! 🤖
Dynamiq is your all-in-one Gen AI framework, designed to streamline the development of AI-powered applications. Dynamiq specializes in orchestrating retrieval-augmented generation (RAG) and large language model (LLM) agents.
Ready to dive in? Here's how you can get started with Dynamiq:
First, let's get Dynamiq installed. You'll need Python, so make sure that's set up on your machine. Then run:
pip install dynamiq
Or build the Python package from the source code:
git clone https://github.com/dynamiq-ai/dynamiq.git
cd dynamiq
poetry install
For more examples and detailed guides, please refer to our documentation.
Here's a simple example to get you started with Dynamiq:
from dynamiq.nodes.llms.openai import OpenAI
from dynamiq.connections import OpenAI as OpenAIConnection
from dynamiq import Workflow
from dynamiq.prompts import Prompt, Message
# Define the prompt template for translation
prompt_template = """
Translate the following text into English: {{ text }}
"""
# Create a Prompt object with the defined template
prompt = Prompt(messages=[Message(content=prompt_template, role="user")])
# Setup your LLM (Large Language Model) Node
llm = OpenAI(
id="openai", # Unique identifier for the node
connection=OpenAIConnection(api_key="$OPENAI_API_KEY"), # Connection using API key
model="gpt-4o", # Model to be used
temperature=0.3, # Sampling temperature for the model
max_tokens=1000, # Maximum number of tokens in the output
prompt=prompt # Prompt to be used for the model
)
# Create a Workflow object
workflow = Workflow()
# Add the LLM node to the workflow
workflow.flow.add_nodes(llm)
# Run the workflow with the input data
result = workflow.run(
input_data={
"text": "Hola Mundo!" # Text to be translated
}
)
# Print the result of the translation
print(result.output)
An agent that has the access to E2B Code Interpreter and is capable of solving complex coding tasks.
from dynamiq.nodes.llms.openai import OpenAI
from dynamiq.connections import OpenAI as OpenAIConnection, E2B as E2BConnection
from dynamiq.nodes.agents.react import ReActAgent
from dynamiq.nodes.tools.e2b_sandbox import E2BInterpreterTool
# Initialize the E2B tool
e2b_tool = E2BInterpreterTool(
connection=E2BConnection(api_key="$API_KEY")
)
# Setup your LLM
llm = OpenAI(
id="openai",
connection=OpenAIConnection(api_key="$API_KEY"),
model="gpt-4o",
temperature=0.3,
max_tokens=1000,
)
# Create the ReAct agent
agent = ReActAgent(
name="react-agent",
llm=llm,
tools=[e2b_tool],
role="Senior Data Scientist",
goal="Provide well-explained final answers to analytical questions",
max_loops=10,
)
# Run the agent with an input
result = agent.run(
input_data={
"input": "Add the first 10 numbers and tell if the result is prime.",
}
)
print(result.output.get("content"))
from dynamiq.connections import (OpenAI as OpenAIConnection,
ScaleSerp as ScaleSerpConnection,
E2B as E2BConnection)
from dynamiq.nodes.llms import OpenAI
from dynamiq.nodes.agents.orchestrators.adaptive import AdaptiveOrchestrator
from dynamiq.nodes.agents.orchestrators.adaptive_manager import AdaptiveAgentManager
from dynamiq.nodes.agents.react import ReActAgent
from dynamiq.nodes.agents.reflection import ReflectionAgent
from dynamiq.nodes.tools.e2b_sandbox import E2BInterpreterTool
from dynamiq.nodes.tools.scale_serp import ScaleSerpTool
# Initialize tools
python_tool = E2BInterpreterTool(
connection=E2BConnection(api_key="$E2B_API_KEY")
)
search_tool = ScaleSerpTool(
connection=ScaleSerpConnection(api_key="$SCALESERP_API_KEY")
)
# Initialize LLM
llm = OpenAI(
connection=OpenAIConnection(api_key="$OPENAI_API_KEY"),
model="gpt-4o",
temperature=0.1,
)
# Define agents
coding_agent = ReActAgent(
name="coding-agent",
llm=llm,
tools=[python_tool],
role="Expert agent with coding skills.",
goal="Provide the solution to the input task using Python software engineering skills.",
max_loops=15,
)
planner_agent = ReflectionAgent(
name="planner-agent",
llm=llm,
role="Expert agent with planning skills.",
goal="Analyze complex requests and provide detailed action plan.",
)
search_agent = ReActAgent(
name="search-agent",
llm=llm,
tools=[search_tool],
role="Expert agent with web search skills.",
goal="Provide the best information available using web browsing and searching skills.",
max_loops=10,
)
# Initialize the adaptive agent manager
agent_manager = AdaptiveAgentManager(llm=llm)
# Create the orchestrator
orchestrator = AdaptiveOrchestrator(
name="adaptive-orchestrator",
agents=[coding_agent, planner_agent, search_agent],
manager=agent_manager,
)
# Define the input task
input_task = (
"Use coding skills to gather data about Nvidia and Intel stock prices for the last 10 years, "
"calculate the average per year for each company, and create a table. Then craft a report "
"and add a conclusion: what would have been better if I had invested $100 ten years ago?"
)
# Run the orchestrator
result = orchestrator.run(
input_data={"input": input_task},
)
# Print the result
print(result.output.get("content"))
Workflow that takes input PDF files, pre-processes them, converts to vector embeddings and stores in Pinecone vector database.
from io import BytesIO
from dynamiq import Workflow
from dynamiq.connections import OpenAI as OpenAIConnection, Pinecone as PineconeConnection
from dynamiq.nodes.converters import PyPDFConverter
from dynamiq.nodes.splitters.document import DocumentSplitter
from dynamiq.nodes.embedders import OpenAIDocumentEmbedder
from dynamiq.nodes.writers import PineconeDocumentWriter
rag_wf = Workflow()
# PyPDF document converter
converter = PyPDFConverter(document_creation_mode="one-doc-per-page")
rag_wf.flow.add_nodes(converter) # add node to the DAG
# Document splitter
document_splitter = (
DocumentSplitter(
split_by="sentence",
split_length=10,
split_overlap=1,
)
.inputs(documents=converter.outputs.documents) # map converter node output to the expected input of the current node
.depends_on(converter)
)
rag_wf.flow.add_nodes(document_splitter)
# OpenAI vector embeddings
embedder = (
OpenAIDocumentEmbedder(
connection=OpenAIConnection(api_key="$OPENAI_API_KEY"),
model="text-embedding-3-small",
)
.inputs(documents=document_splitter.outputs.documents)
.depends_on(document_splitter)
)
rag_wf.flow.add_nodes(embedder)
# Pinecone vector storage
vector_store = (
PineconeDocumentWriter(
connection=PineconeConnection(api_key="$PINECONE_API_KEY"),
index_name="default",
dimension=1536,
)
.inputs(documents=embedder.outputs.documents)
.depends_on(embedder)
)
rag_wf.flow.add_nodes(vector_store)
# Prepare input PDF files
file_paths = ["example.pdf"]
input_data = {
"files": [
BytesIO(open(path, "rb").read()) for path in file_paths
],
"metadata": [
{"filename": path} for path in file_paths
],
}
# Run RAG indexing flow
rag_wf.run(input_data=input_data)
Simple retrieval RAG flow that searches for relevant documents and answers the original user question using retrieved documents.
from dynamiq import Workflow
from dynamiq.nodes import InputTransformer
from dynamiq.connections import OpenAI as OpenAIConnection, Pinecone as PineconeConnection
from dynamiq.nodes.embedders import OpenAITextEmbedder
from dynamiq.nodes.retrievers import PineconeDocumentRetriever
from dynamiq.nodes.llms import OpenAI
from dynamiq.prompts import Message, Prompt
# Initialize the RAG retrieval workflow
retrieval_wf = Workflow()
# Shared OpenAI connection
openai_connection = OpenAIConnection(api_key="$OPENAI_API_KEY")
# OpenAI text embedder for query embedding
embedder = OpenAITextEmbedder(
connection=openai_connection,
model="text-embedding-3-small",
)
retrieval_wf.flow.add_nodes(embedder)
# Pinecone document retriever
document_retriever = (
PineconeDocumentRetriever(
connection=PineconeConnection(api_key="$PINECONE_API_KEY"),
index_name="default",
dimension=1536,
top_k=5,
)
.inputs(embedding=embedder.outputs.embedding)
.depends_on(embedder)
)
retrieval_wf.flow.add_nodes(document_retriever)
# Define the prompt template
prompt_template = """
Please answer the question based on the provided context.
Question: {{ query }}
Context:
{% for document in documents %}
- {{ document.content }}
{% endfor %}
"""
# OpenAI LLM for answer generation
prompt = Prompt(messages=[Message(content=prompt_template, role="user")])
answer_generator = (
OpenAI(
connection=openai_connection,
model="gpt-4o",
prompt=prompt,
)
.inputs(
documents=document_retriever.outputs.documents,
query=embedder.outputs.query,
) # take documents from the vector store node and query from the embedder
.depends_on(document_retriever)
)
retrieval_wf.flow.add_nodes(answer_generator)
# Run the RAG retrieval flow
question = "What are the line intems provided in the invoice?"
result = retrieval_wf.run(input_data={"query": question})
answer = result.output.get(answer_generator.id).get("output", {}).get("content")
print(answer)
We love contributions! Whether it's bug reports, feature requests, or pull requests, head over to our CONTRIBUTING.md to see how you can help.
Dynamiq is open-source and available under the Apache 2 License.
Happy coding! 🚀
For Tasks:
Click tags to check more tools for each tasksFor Jobs:
Alternative AI tools for dynamiq
Similar Open Source Tools
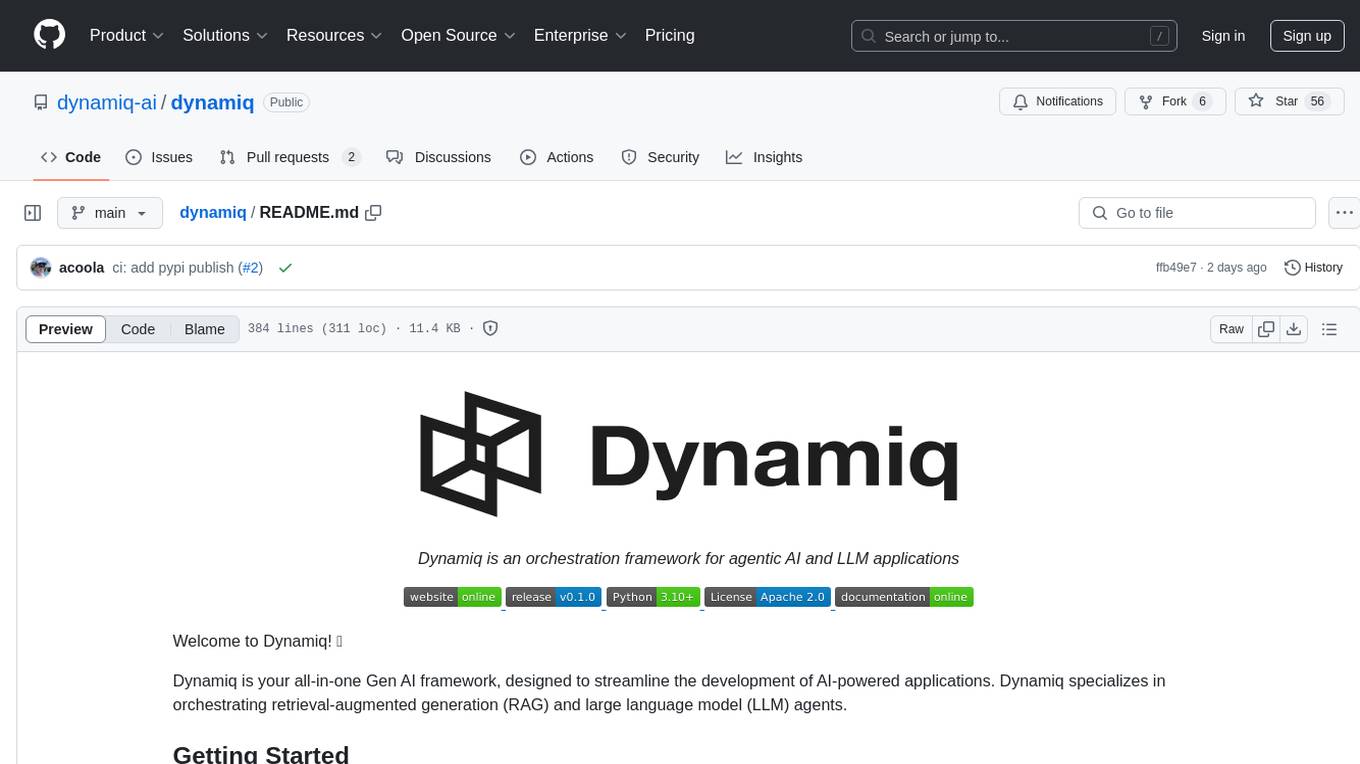
dynamiq
Dynamiq is an orchestration framework designed to streamline the development of AI-powered applications, specializing in orchestrating retrieval-augmented generation (RAG) and large language model (LLM) agents. It provides an all-in-one Gen AI framework for agentic AI and LLM applications, offering tools for multi-agent orchestration, document indexing, and retrieval flows. With Dynamiq, users can easily build and deploy AI solutions for various tasks.
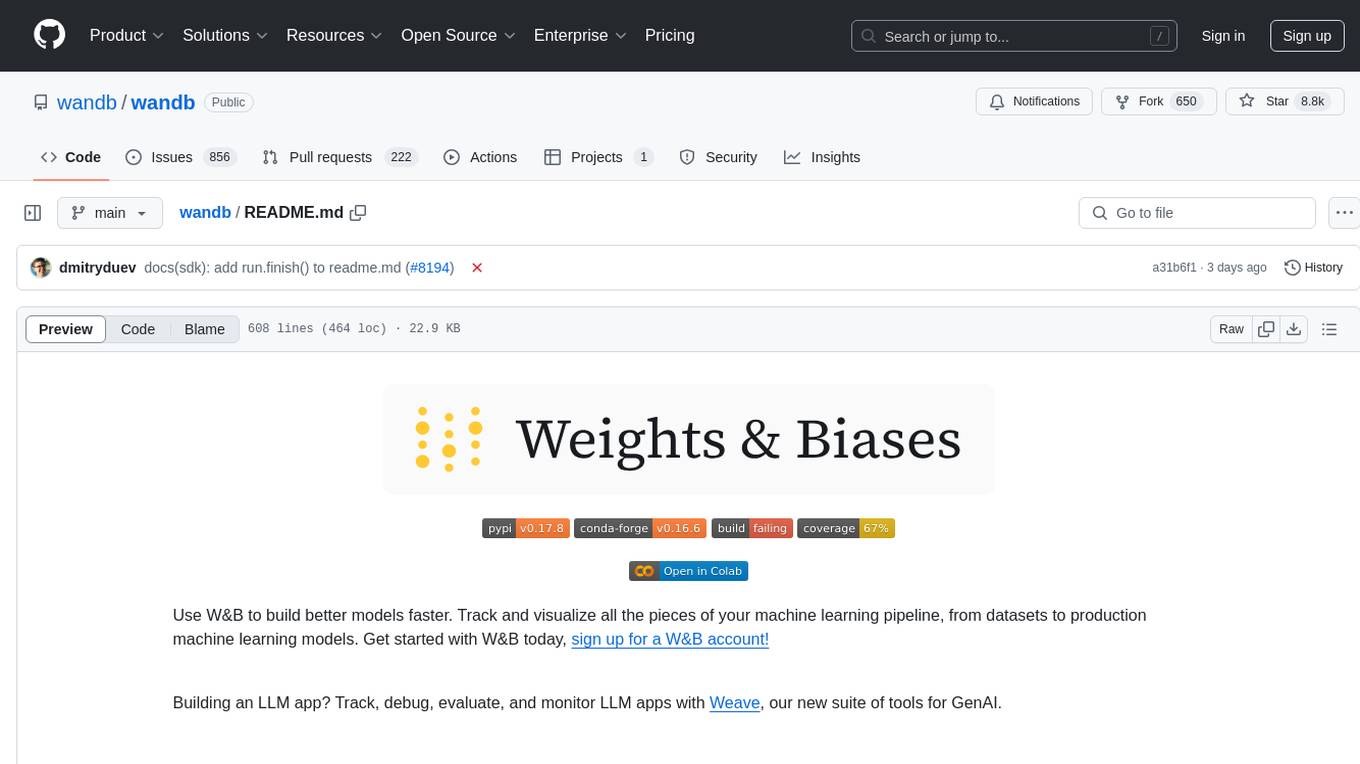
wandb
Weights & Biases (W&B) is a platform that helps users build better machine learning models faster by tracking and visualizing all components of the machine learning pipeline, from datasets to production models. It offers tools for tracking, debugging, evaluating, and monitoring machine learning applications. W&B provides integrations with popular frameworks like PyTorch, TensorFlow/Keras, Hugging Face Transformers, PyTorch Lightning, XGBoost, and Sci-Kit Learn. Users can easily log metrics, visualize performance, and compare experiments using W&B. The platform also supports hosting options in the cloud or on private infrastructure, making it versatile for various deployment needs.
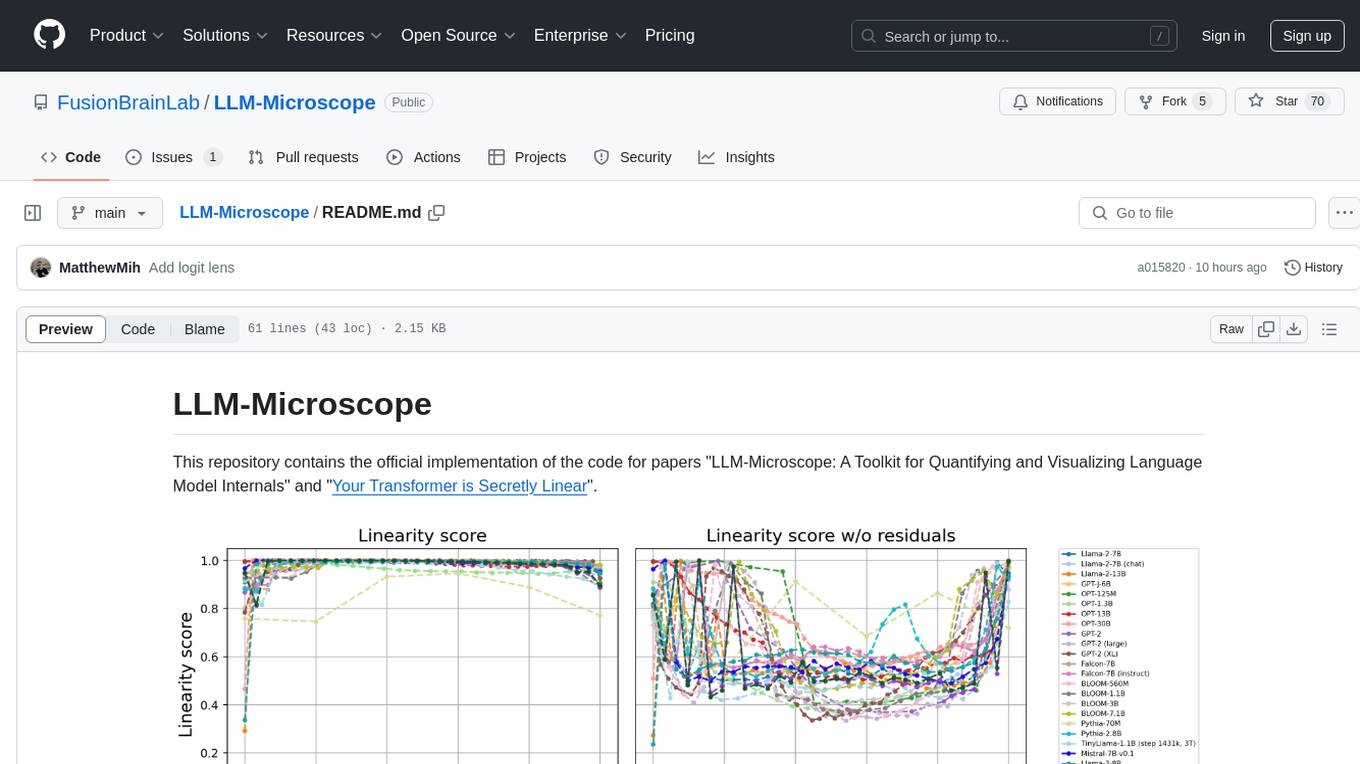
LLM-Microscope
LLM-Microscope is a toolkit designed for quantifying and visualizing language model internals. It provides functions for calculating anisotropy, intrinsic dimension, and linearity score. The toolkit also includes a Logit Lens feature for analyzing model predictions and losses. Users can easily install the toolkit using pip and explore the functionalities through provided examples.
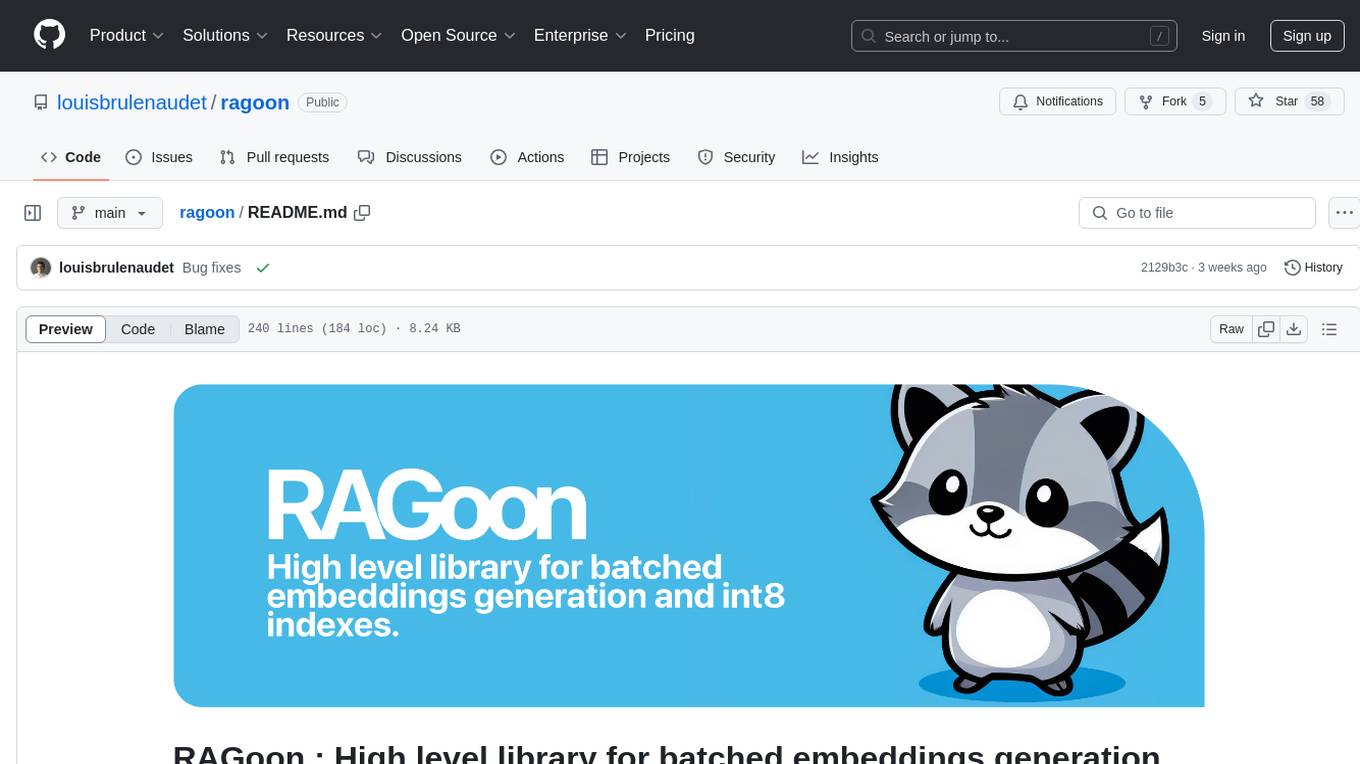
ragoon
RAGoon is a high-level library designed for batched embeddings generation, fast web-based RAG (Retrieval-Augmented Generation) processing, and quantized indexes processing. It provides NLP utilities for multi-model embedding production, high-dimensional vector visualization, and enhancing language model performance through search-based querying, web scraping, and data augmentation techniques.
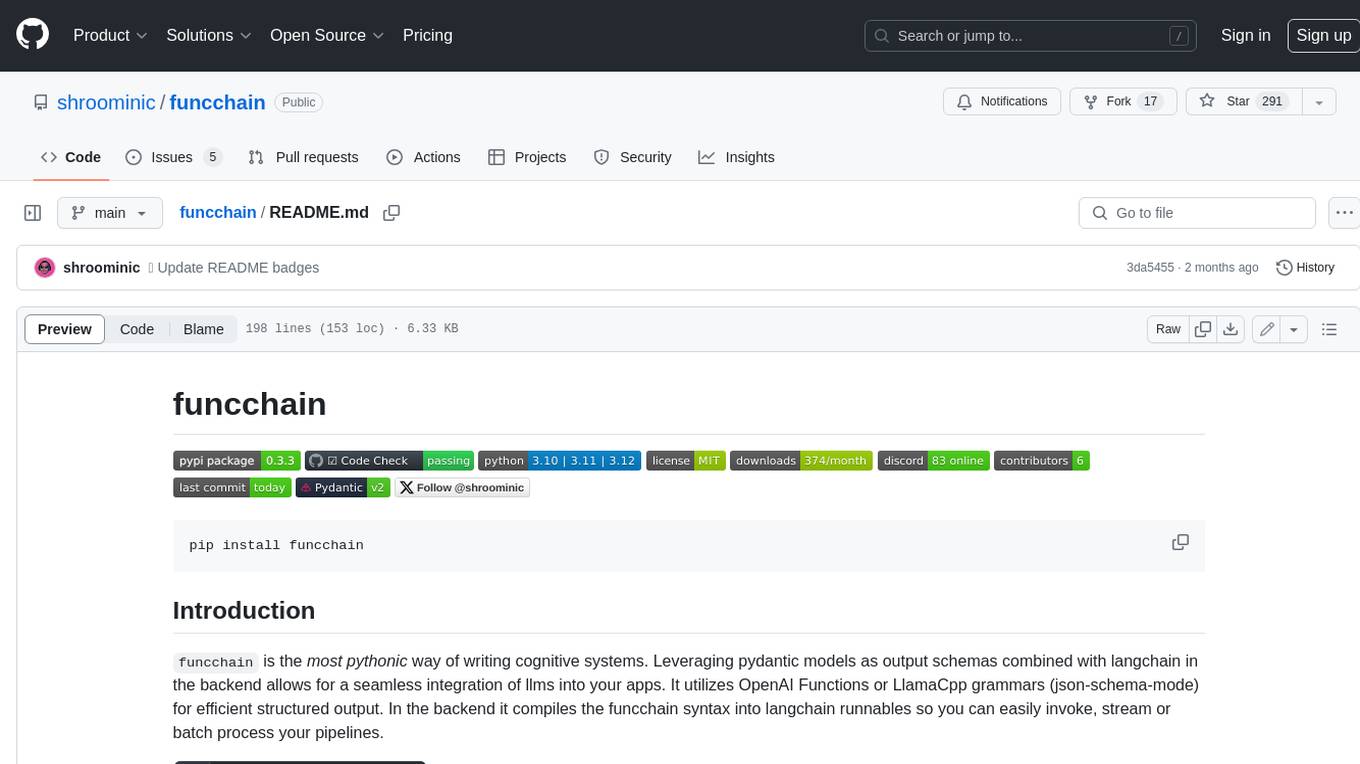
funcchain
Funcchain is a Python library that allows you to easily write cognitive systems by leveraging Pydantic models as output schemas and LangChain in the backend. It provides a seamless integration of LLMs into your apps, utilizing OpenAI Functions or LlamaCpp grammars (json-schema-mode) for efficient structured output. Funcchain compiles the Funcchain syntax into LangChain runnables, enabling you to invoke, stream, or batch process your pipelines effortlessly.
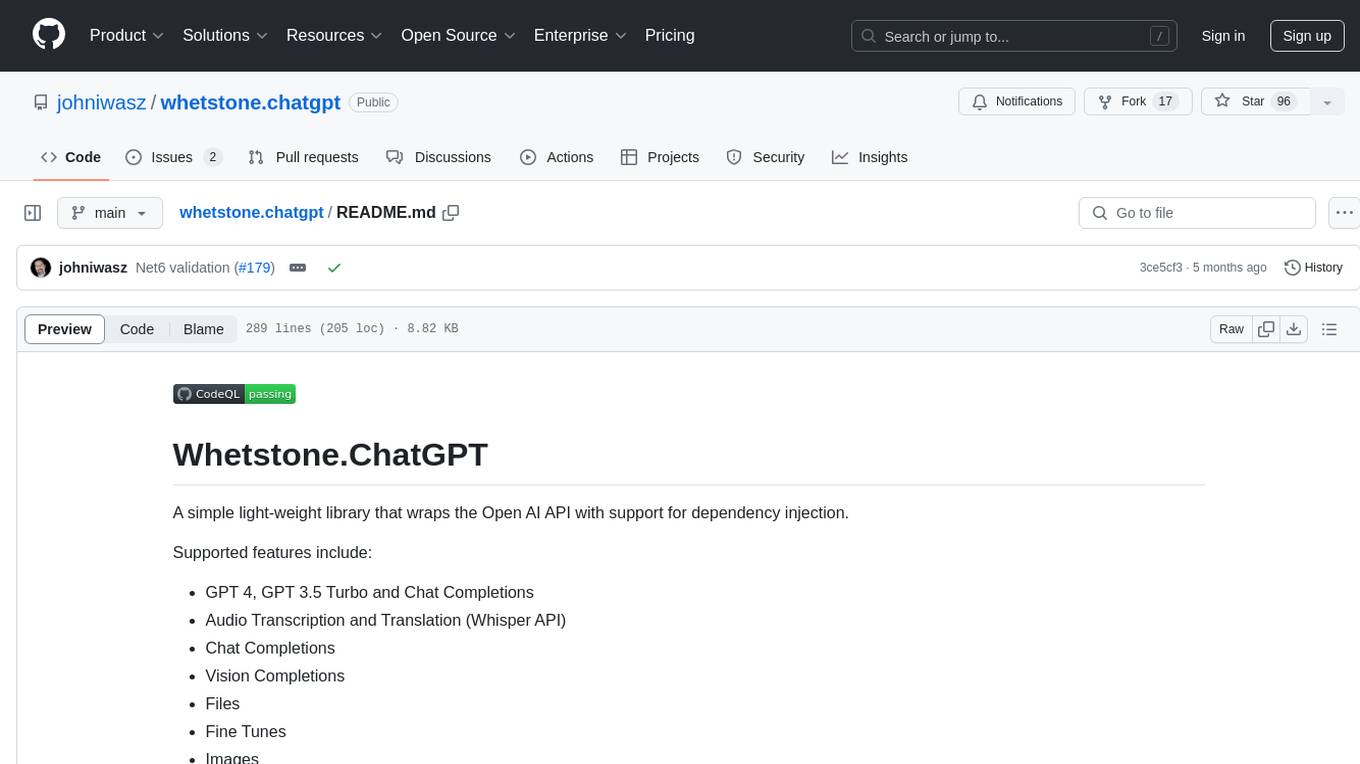
whetstone.chatgpt
Whetstone.ChatGPT is a simple light-weight library that wraps the Open AI API with support for dependency injection. It supports features like GPT 4, GPT 3.5 Turbo, chat completions, audio transcription and translation, vision completions, files, fine tunes, images, embeddings, moderations, and response streaming. The library provides a video walkthrough of a Blazor web app built on it and includes examples such as a command line bot. It offers quickstarts for dependency injection, chat completions, completions, file handling, fine tuning, image generation, and audio transcription.
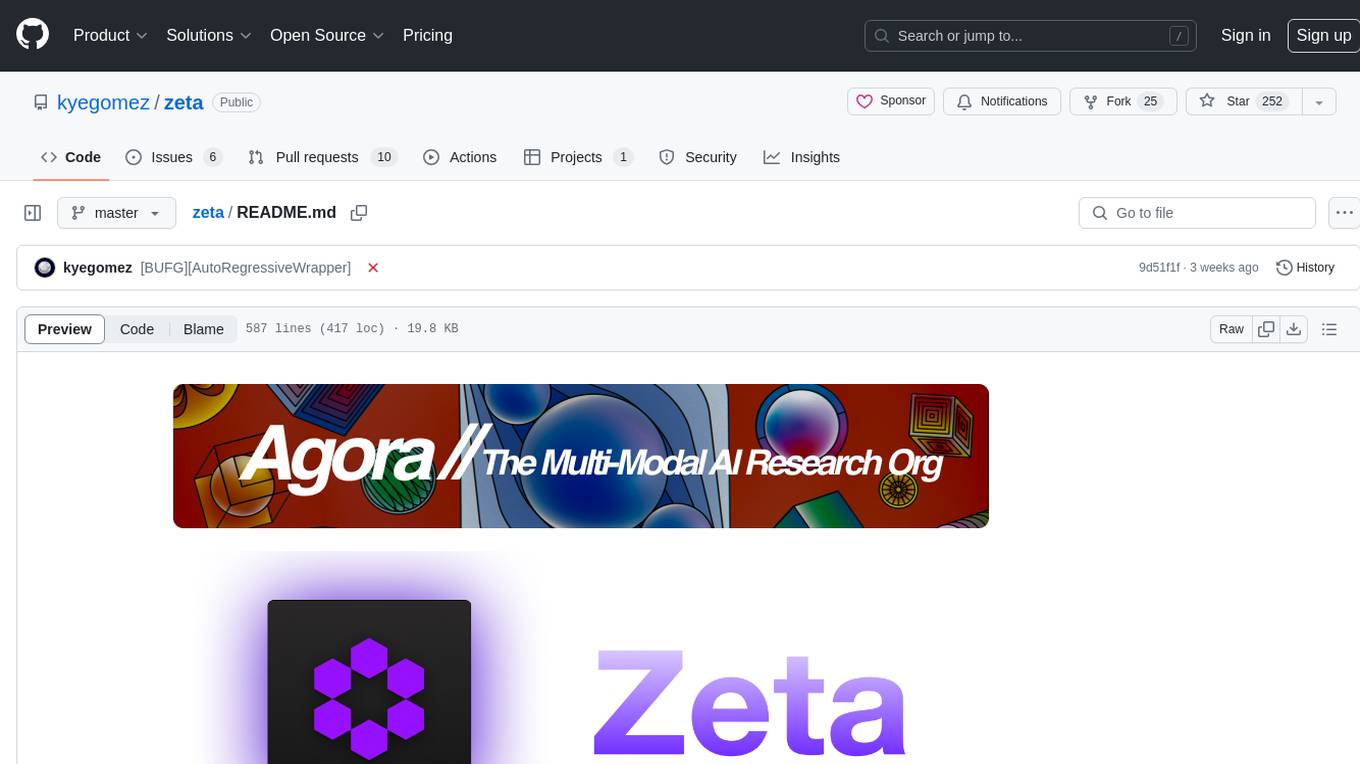
zeta
Zeta is a tool designed to build state-of-the-art AI models faster by providing modular, high-performance, and scalable building blocks. It addresses the common issues faced while working with neural nets, such as chaotic codebases, lack of modularity, and low performance modules. Zeta emphasizes usability, modularity, and performance, and is currently used in hundreds of models across various GitHub repositories. It enables users to prototype, train, optimize, and deploy the latest SOTA neural nets into production. The tool offers various modules like FlashAttention, SwiGLUStacked, RelativePositionBias, FeedForward, BitLinear, PalmE, Unet, VisionEmbeddings, niva, FusedDenseGELUDense, FusedDropoutLayerNorm, MambaBlock, Film, hyper_optimize, DPO, and ZetaCloud for different tasks in AI model development.
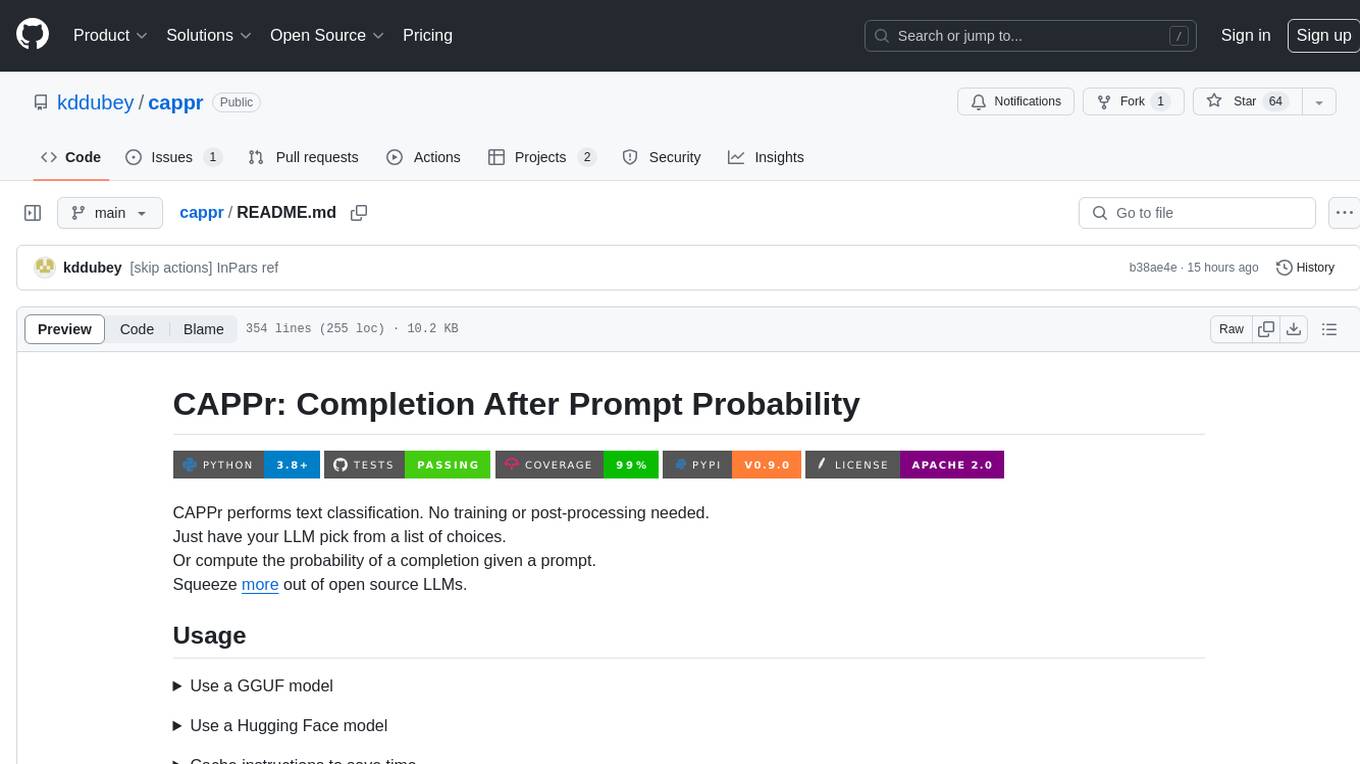
cappr
CAPPr is a tool for text classification that does not require training or post-processing. It allows users to have their language models pick from a list of choices or compute the probability of a completion given a prompt. The tool aims to help users get more out of open source language models by simplifying the text classification process. CAPPr can be used with GGUF models, Hugging Face models, models from the OpenAI API, and for tasks like caching instructions, extracting final answers from step-by-step completions, and running predictions in batches with different sets of completions.
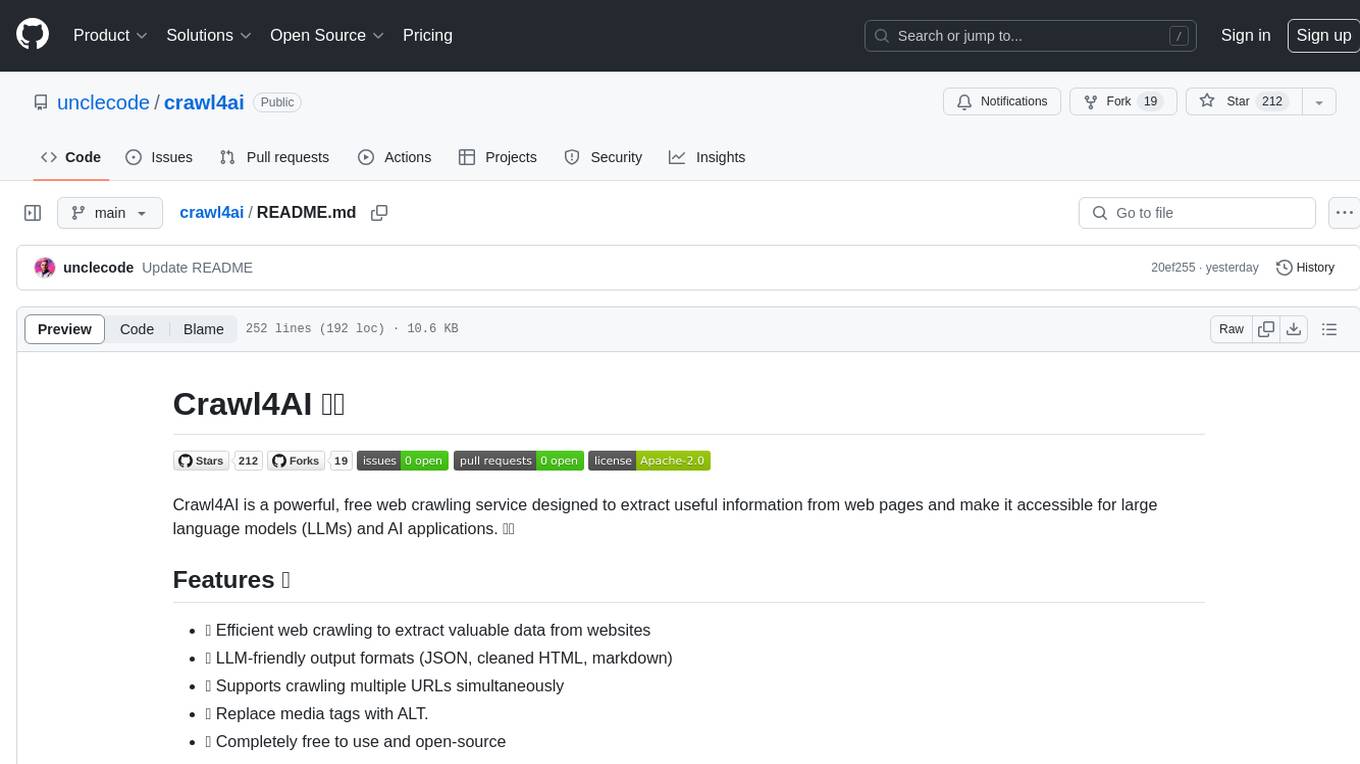
crawl4ai
Crawl4AI is a powerful and free web crawling service that extracts valuable data from websites and provides LLM-friendly output formats. It supports crawling multiple URLs simultaneously, replaces media tags with ALT, and is completely free to use and open-source. Users can integrate Crawl4AI into Python projects as a library or run it as a standalone local server. The tool allows users to crawl and extract data from specified URLs using different providers and models, with options to include raw HTML content, force fresh crawls, and extract meaningful text blocks. Configuration settings can be adjusted in the `crawler/config.py` file to customize providers, API keys, chunk processing, and word thresholds. Contributions to Crawl4AI are welcome from the open-source community to enhance its value for AI enthusiasts and developers.
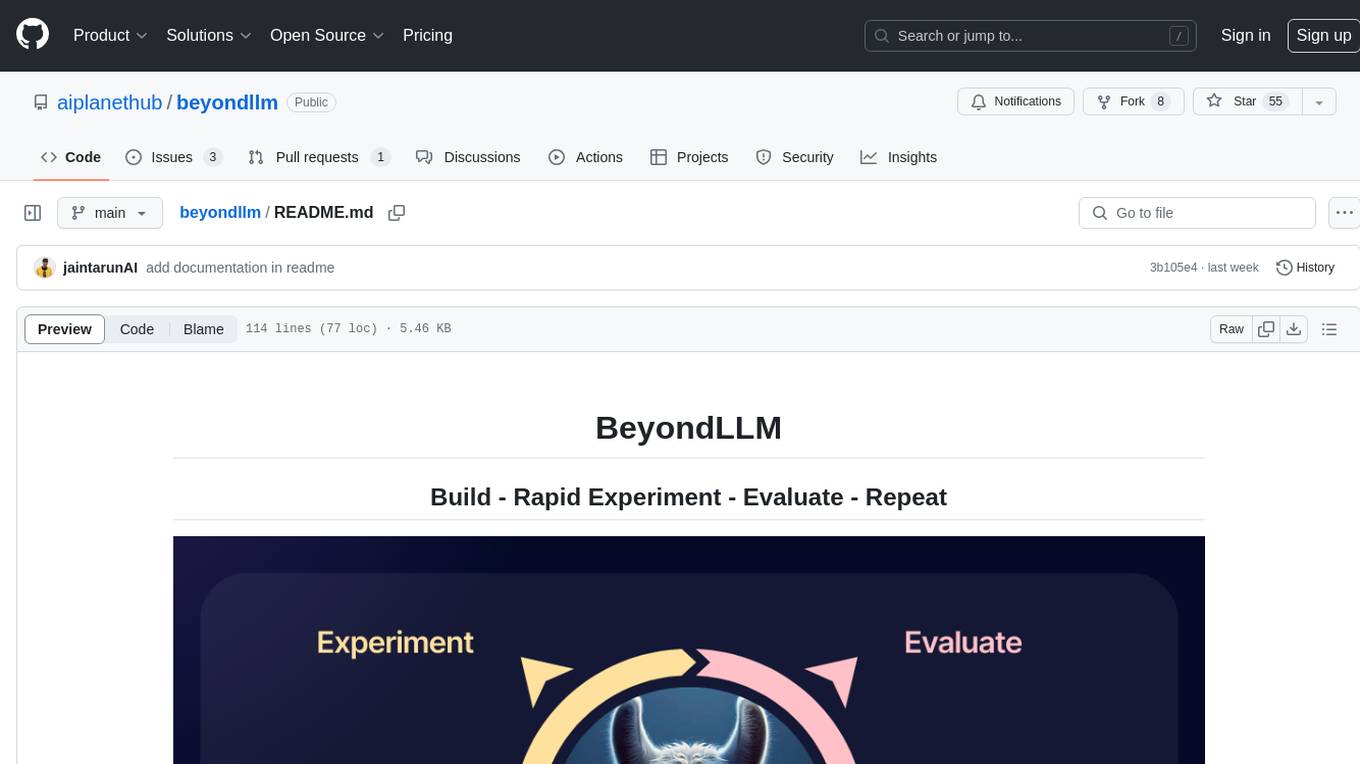
beyondllm
Beyond LLM offers an all-in-one toolkit for experimentation, evaluation, and deployment of Retrieval-Augmented Generation (RAG) systems. It simplifies the process with automated integration, customizable evaluation metrics, and support for various Large Language Models (LLMs) tailored to specific needs. The aim is to reduce LLM hallucination risks and enhance reliability.
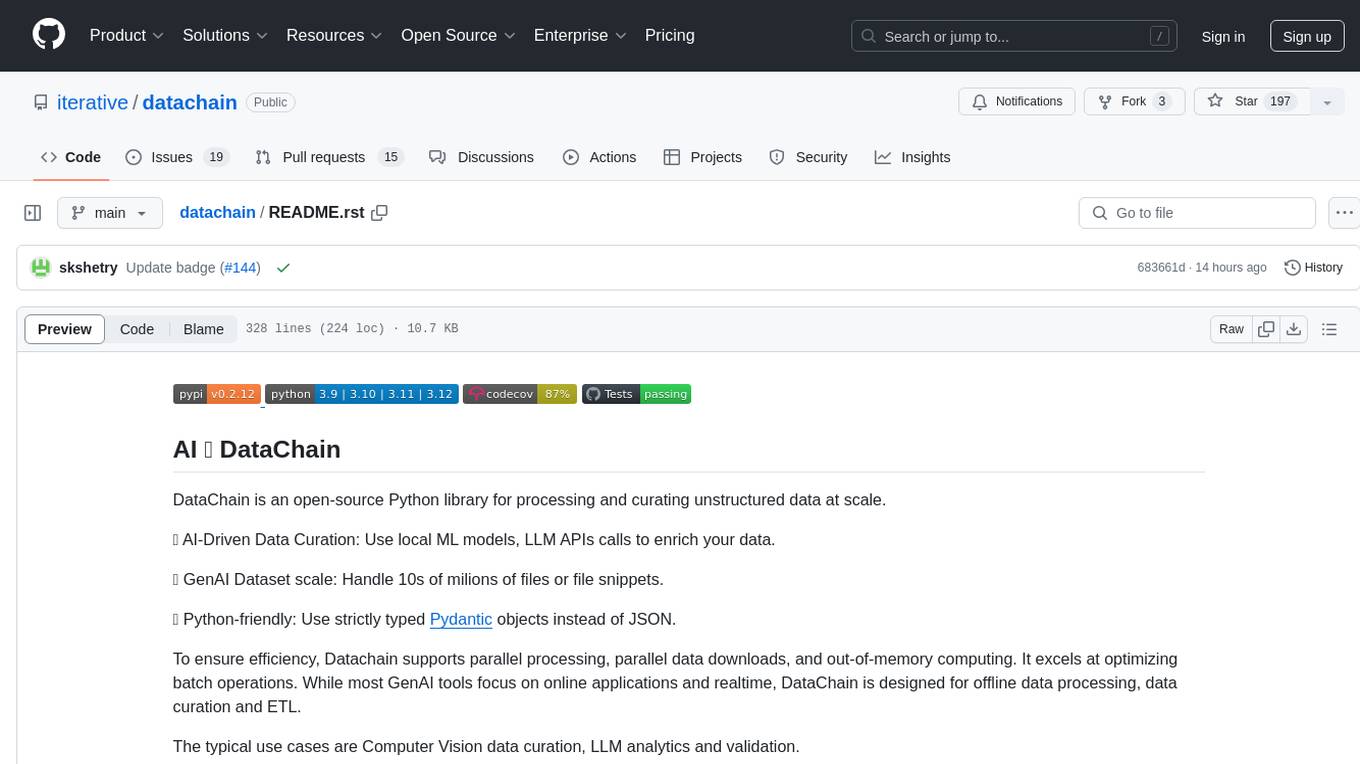
datachain
DataChain is an open-source Python library for processing and curating unstructured data at scale. It supports AI-driven data curation using local ML models and LLM APIs, handles large datasets, and is Python-friendly with Pydantic objects. It excels at optimizing batch operations and is designed for offline data processing, curation, and ETL. Typical use cases include Computer Vision data curation, LLM analytics, and validation.
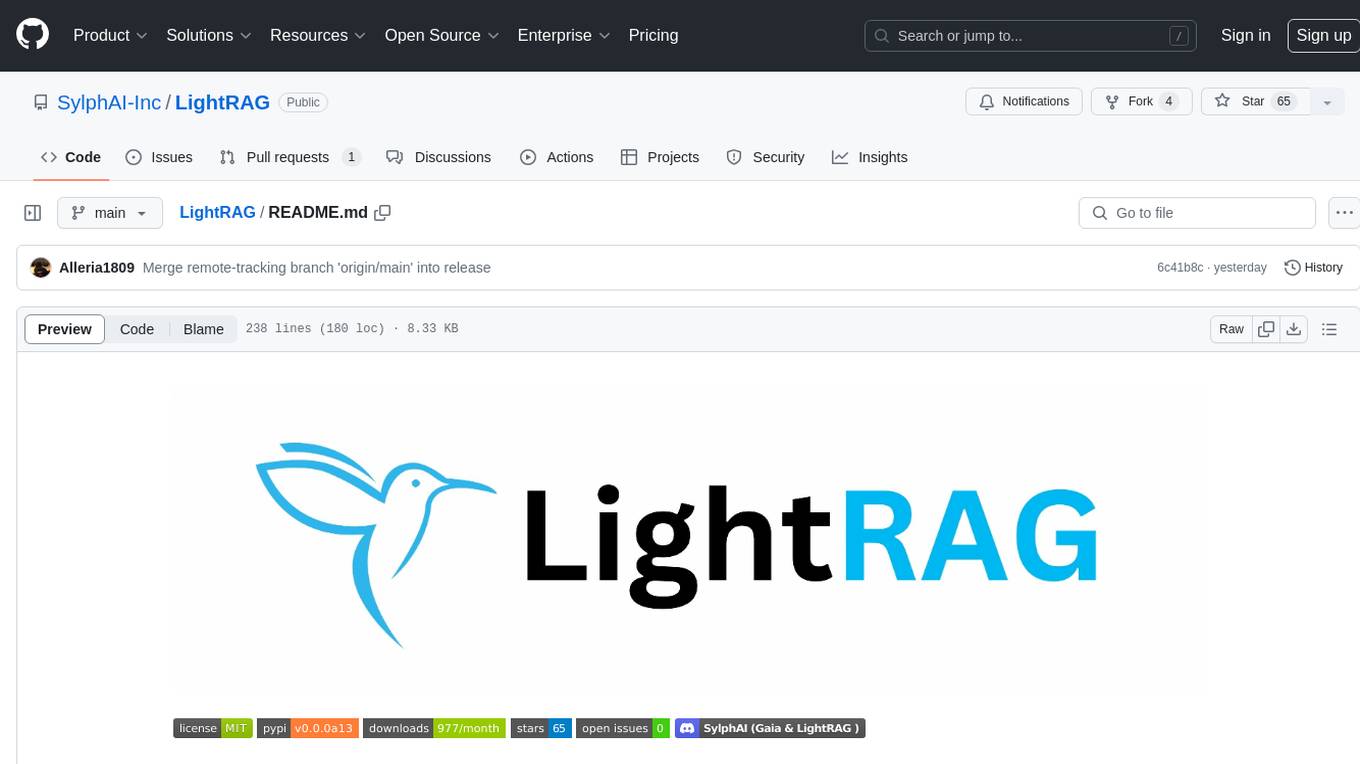
LightRAG
LightRAG is a PyTorch library designed for building and optimizing Retriever-Agent-Generator (RAG) pipelines. It follows principles of simplicity, quality, and optimization, offering developers maximum customizability with minimal abstraction. The library includes components for model interaction, output parsing, and structured data generation. LightRAG facilitates tasks like providing explanations and examples for concepts through a question-answering pipeline.
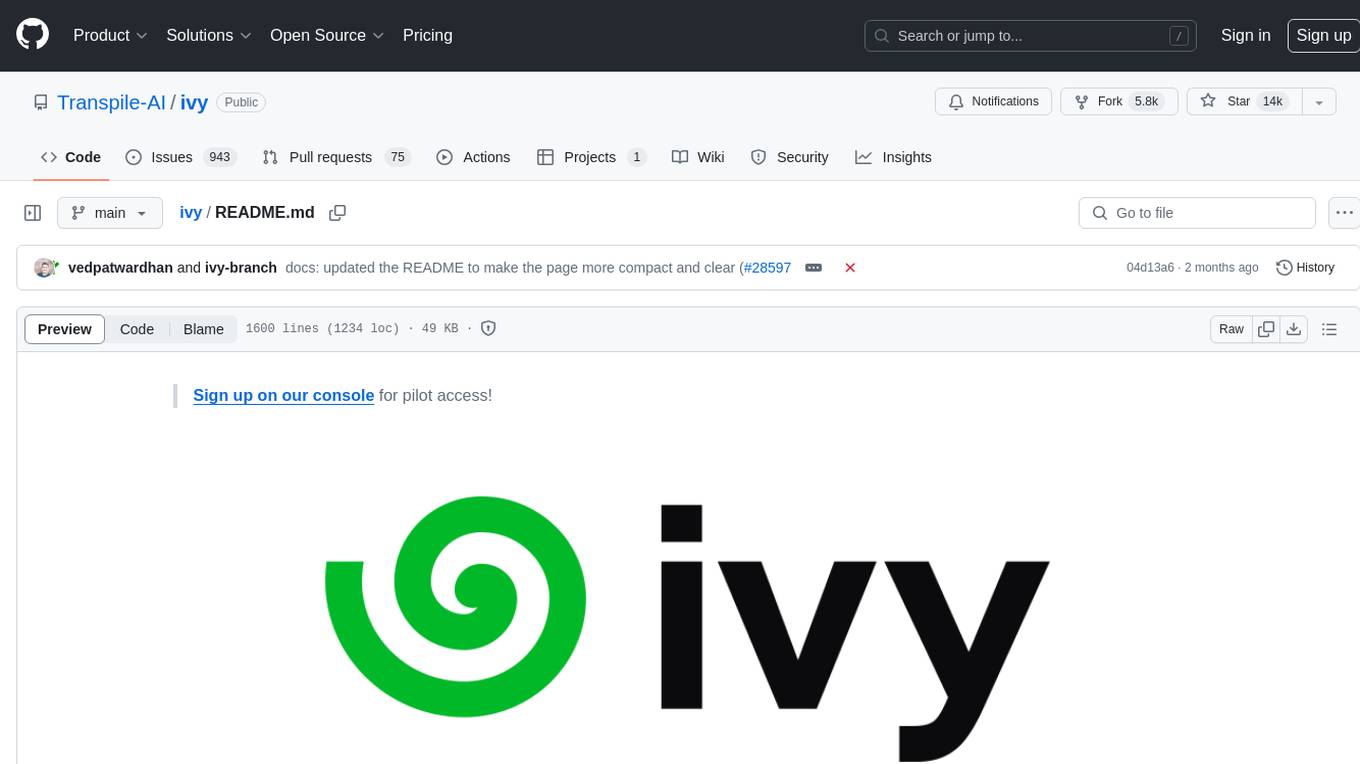
ivy
Ivy is an open-source machine learning framework that enables users to convert code between different ML frameworks and write framework-agnostic code. It allows users to transpile code from one framework to another, making it easy to use building blocks from different frameworks in a single project. Ivy also serves as a flexible framework that breaks free from framework limitations, allowing users to publish code that is interoperable with various frameworks and future frameworks. Users can define trainable modules and layers using Ivy's stateful API, making it easy to build and train models across different backends.
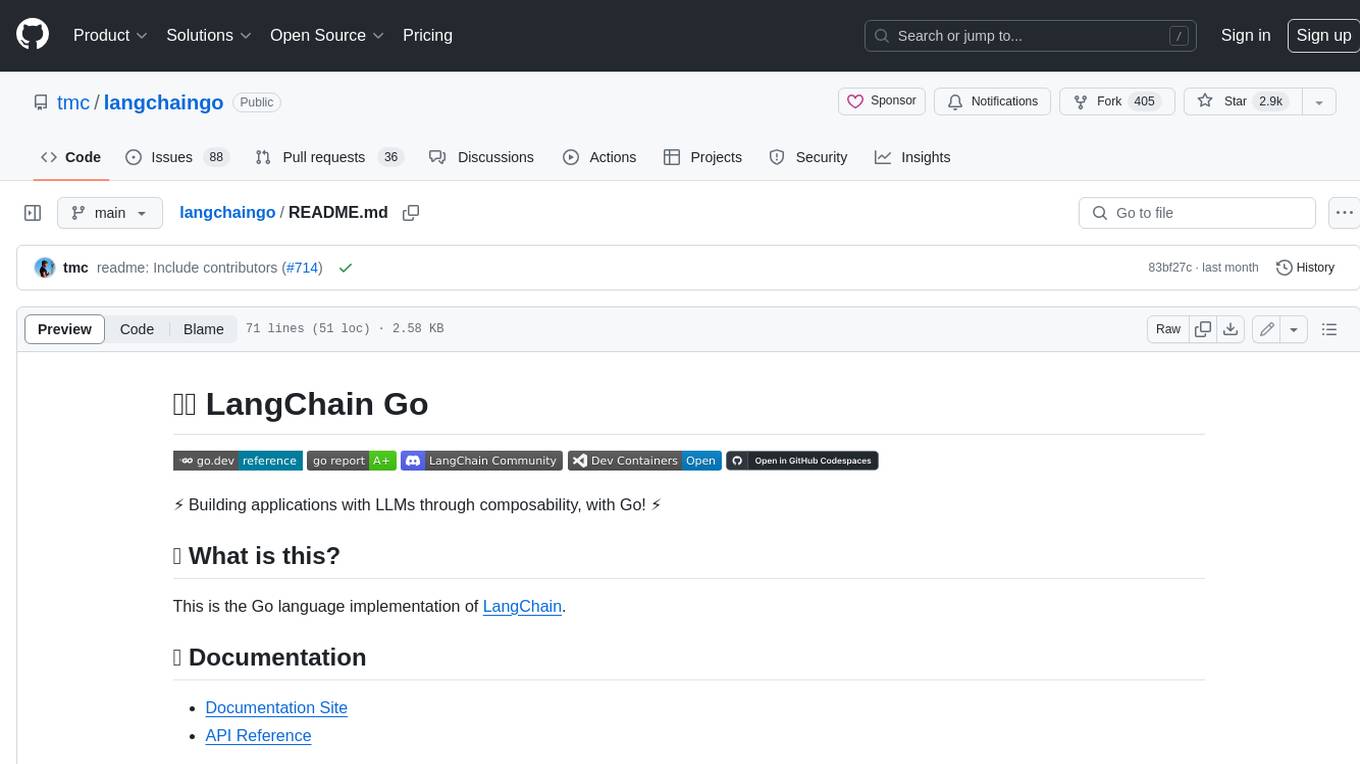
langchaingo
LangChain Go is a Go language implementation of LangChain, a framework for building applications with LLMs through composability. It provides a simple and easy-to-use API for interacting with LLMs, making it easy to add language-based features to your applications.
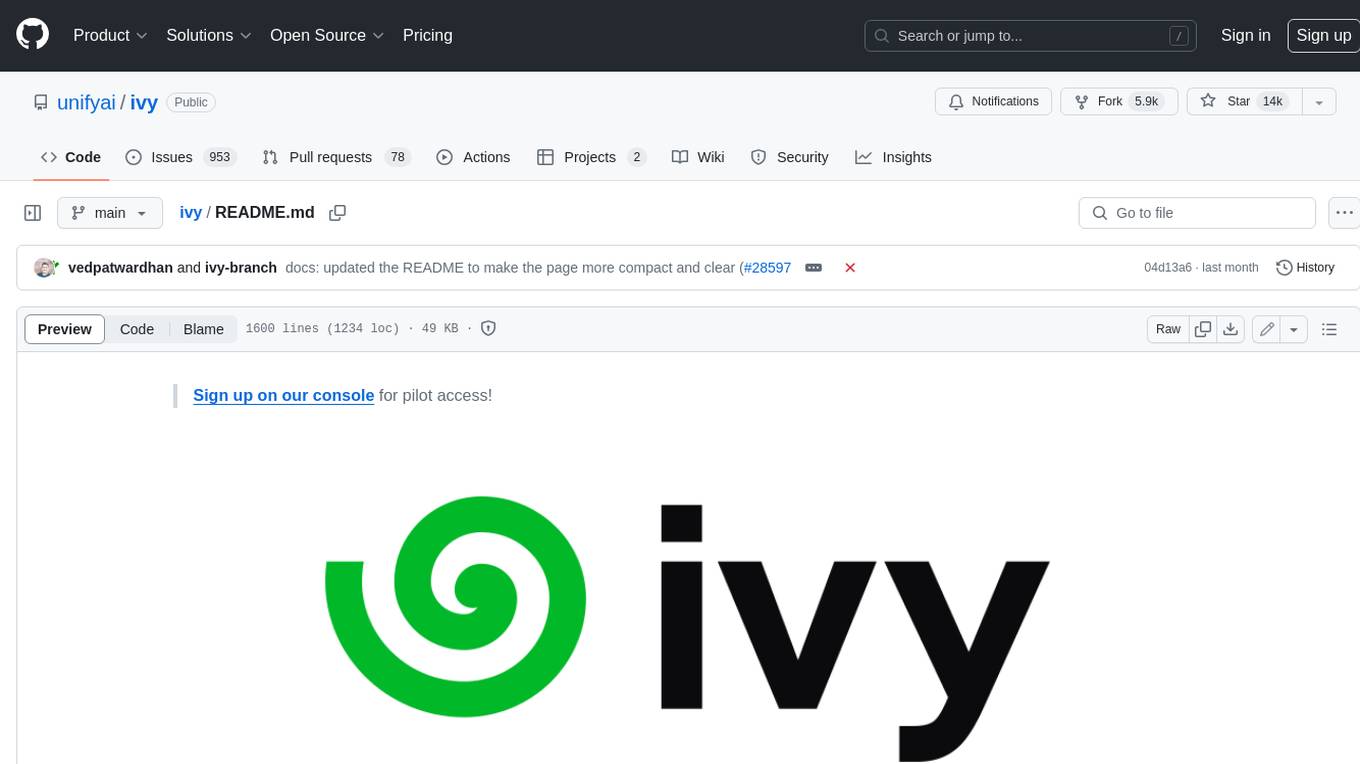
ivy
Ivy is an open-source machine learning framework that enables you to: * 🔄 **Convert code into any framework** : Use and build on top of any model, library, or device by converting any code from one framework to another using `ivy.transpile`. * ⚒️ **Write framework-agnostic code** : Write your code once in `ivy` and then choose the most appropriate ML framework as the backend to leverage all the benefits and tools. Join our growing community 🌍 to connect with people using Ivy. **Let's** unify.ai **together 🦾**
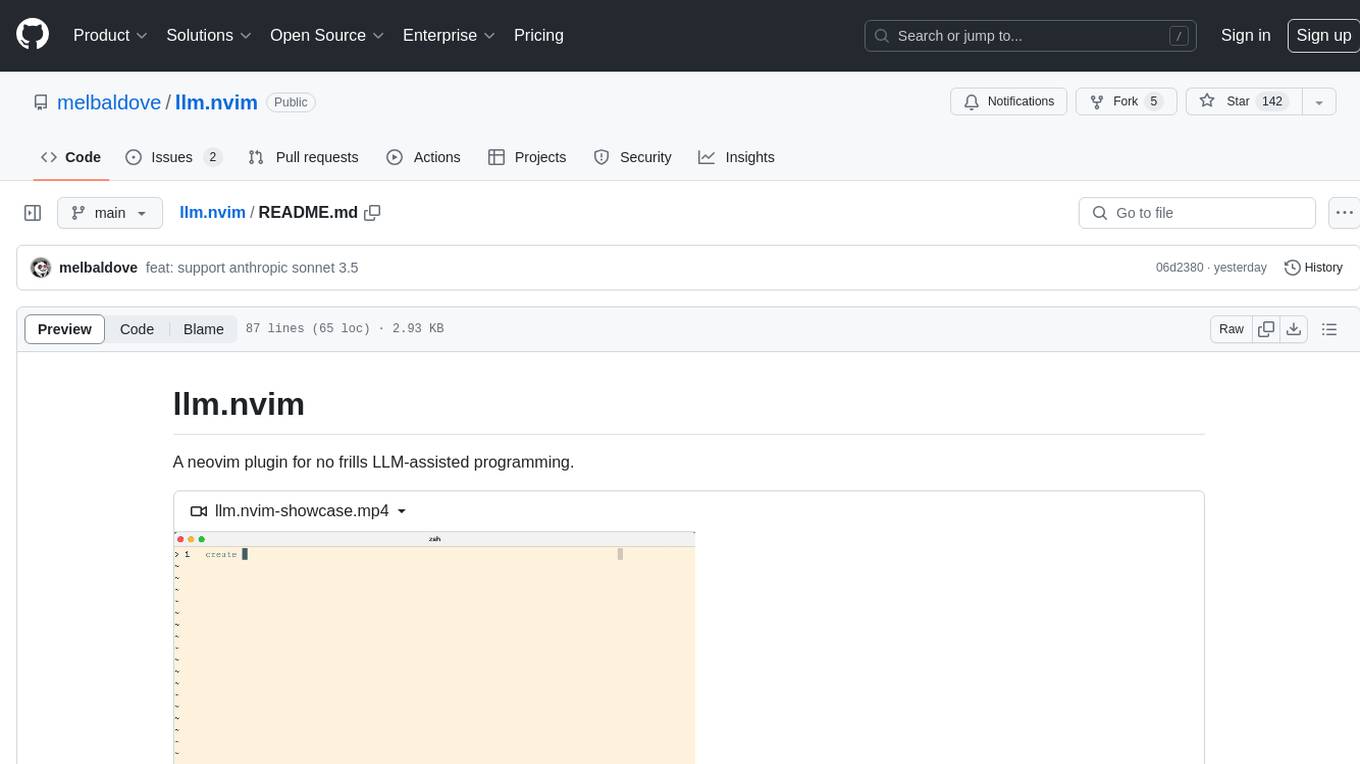
llm.nvim
llm.nvim is a neovim plugin designed for LLM-assisted programming. It provides a no-frills approach to integrating language model assistance into the coding workflow. Users can configure the plugin to interact with various AI services such as GROQ, OpenAI, and Anthropics. The plugin offers functions to trigger the LLM assistant, create new prompt files, and customize key bindings for seamless interaction. With a focus on simplicity and efficiency, llm.nvim aims to enhance the coding experience by leveraging AI capabilities within the neovim environment.
For similar tasks
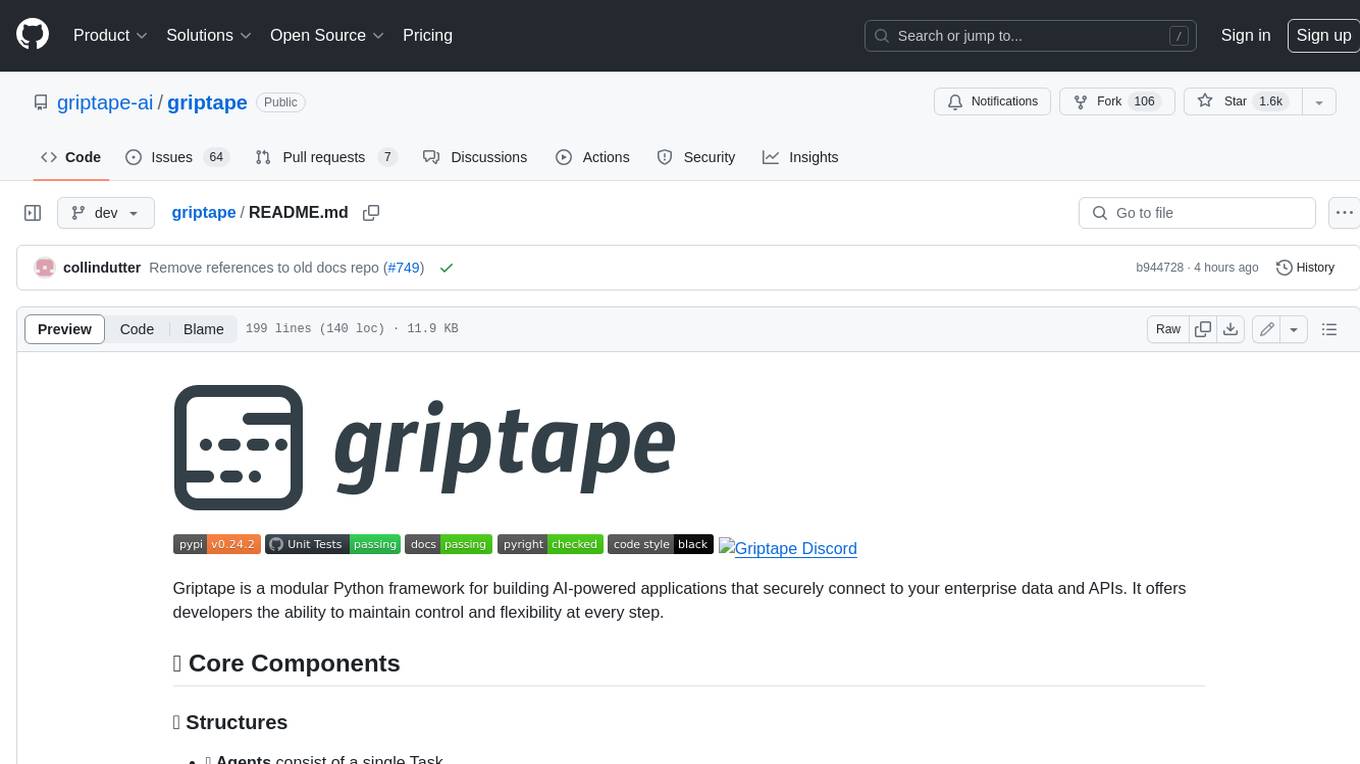
griptape
Griptape is a modular Python framework for building AI-powered applications that securely connect to your enterprise data and APIs. It offers developers the ability to maintain control and flexibility at every step. Griptape's core components include Structures (Agents, Pipelines, and Workflows), Tasks, Tools, Memory (Conversation Memory, Task Memory, and Meta Memory), Drivers (Prompt and Embedding Drivers, Vector Store Drivers, Image Generation Drivers, Image Query Drivers, SQL Drivers, Web Scraper Drivers, and Conversation Memory Drivers), Engines (Query Engines, Extraction Engines, Summary Engines, Image Generation Engines, and Image Query Engines), and additional components (Rulesets, Loaders, Artifacts, Chunkers, and Tokenizers). Griptape enables developers to create AI-powered applications with ease and efficiency.
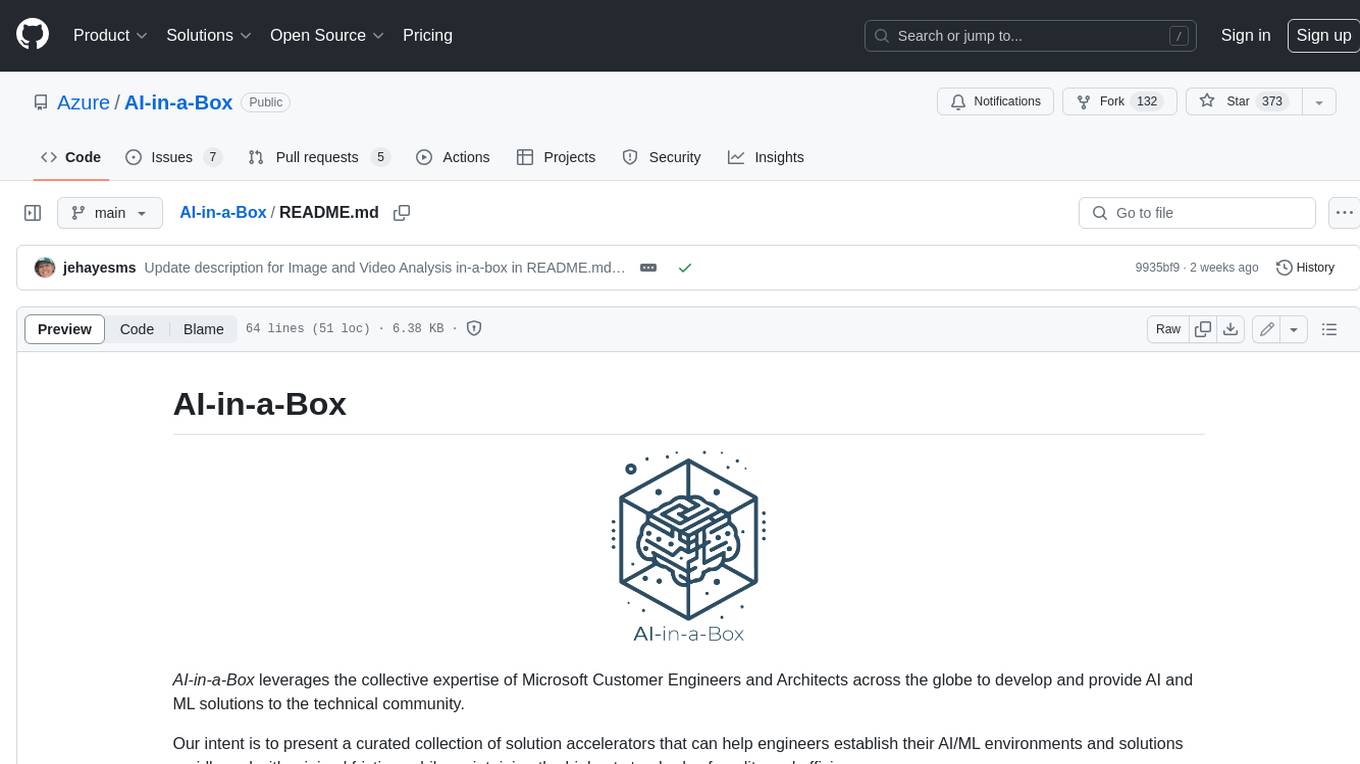
AI-in-a-Box
AI-in-a-Box is a curated collection of solution accelerators that can help engineers establish their AI/ML environments and solutions rapidly and with minimal friction, while maintaining the highest standards of quality and efficiency. It provides essential guidance on the responsible use of AI and LLM technologies, specific security guidance for Generative AI (GenAI) applications, and best practices for scaling OpenAI applications within Azure. The available accelerators include: Azure ML Operationalization in-a-box, Edge AI in-a-box, Doc Intelligence in-a-box, Image and Video Analysis in-a-box, Cognitive Services Landing Zone in-a-box, Semantic Kernel Bot in-a-box, NLP to SQL in-a-box, Assistants API in-a-box, and Assistants API Bot in-a-box.
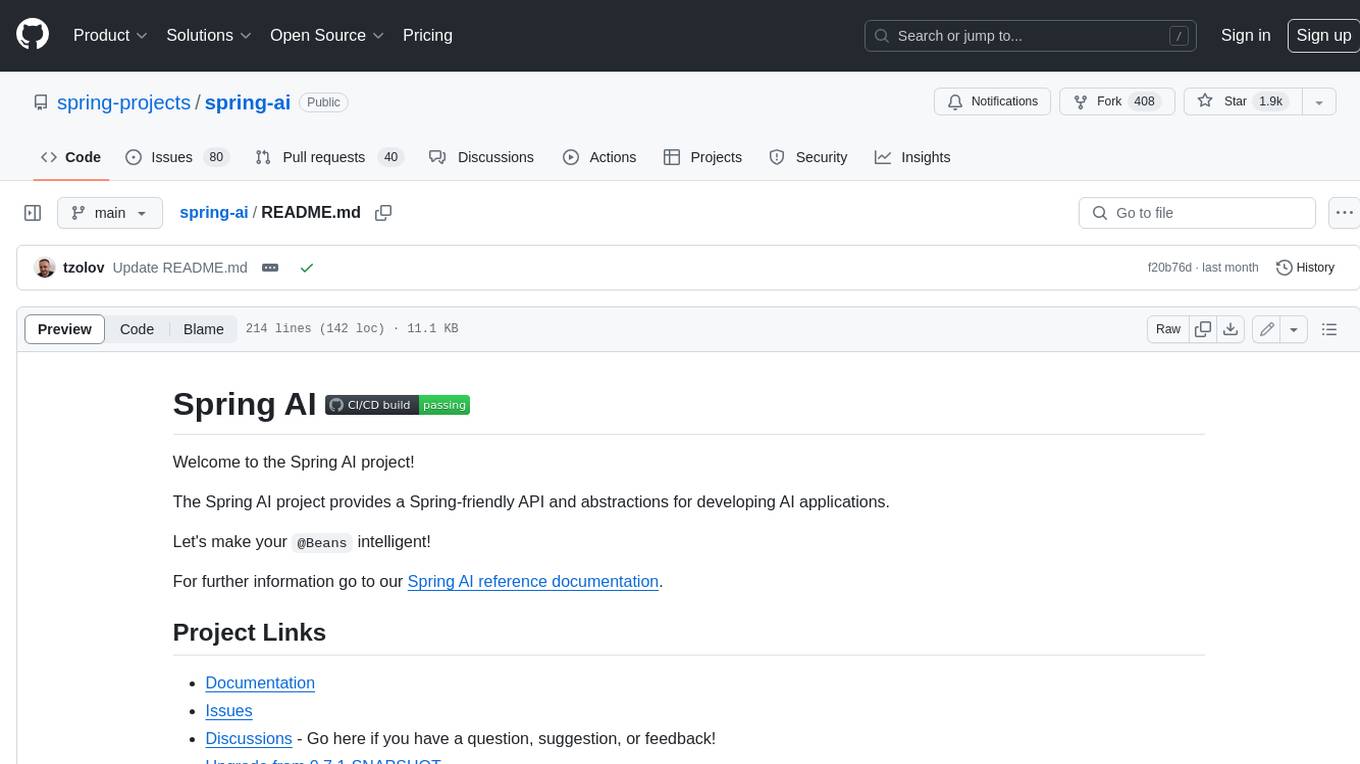
spring-ai
The Spring AI project provides a Spring-friendly API and abstractions for developing AI applications. It offers a portable client API for interacting with generative AI models, enabling developers to easily swap out implementations and access various models like OpenAI, Azure OpenAI, and HuggingFace. Spring AI also supports prompt engineering, providing classes and interfaces for creating and parsing prompts, as well as incorporating proprietary data into generative AI without retraining the model. This is achieved through Retrieval Augmented Generation (RAG), which involves extracting, transforming, and loading data into a vector database for use by AI models. Spring AI's VectorStore abstraction allows for seamless transitions between different vector database implementations.
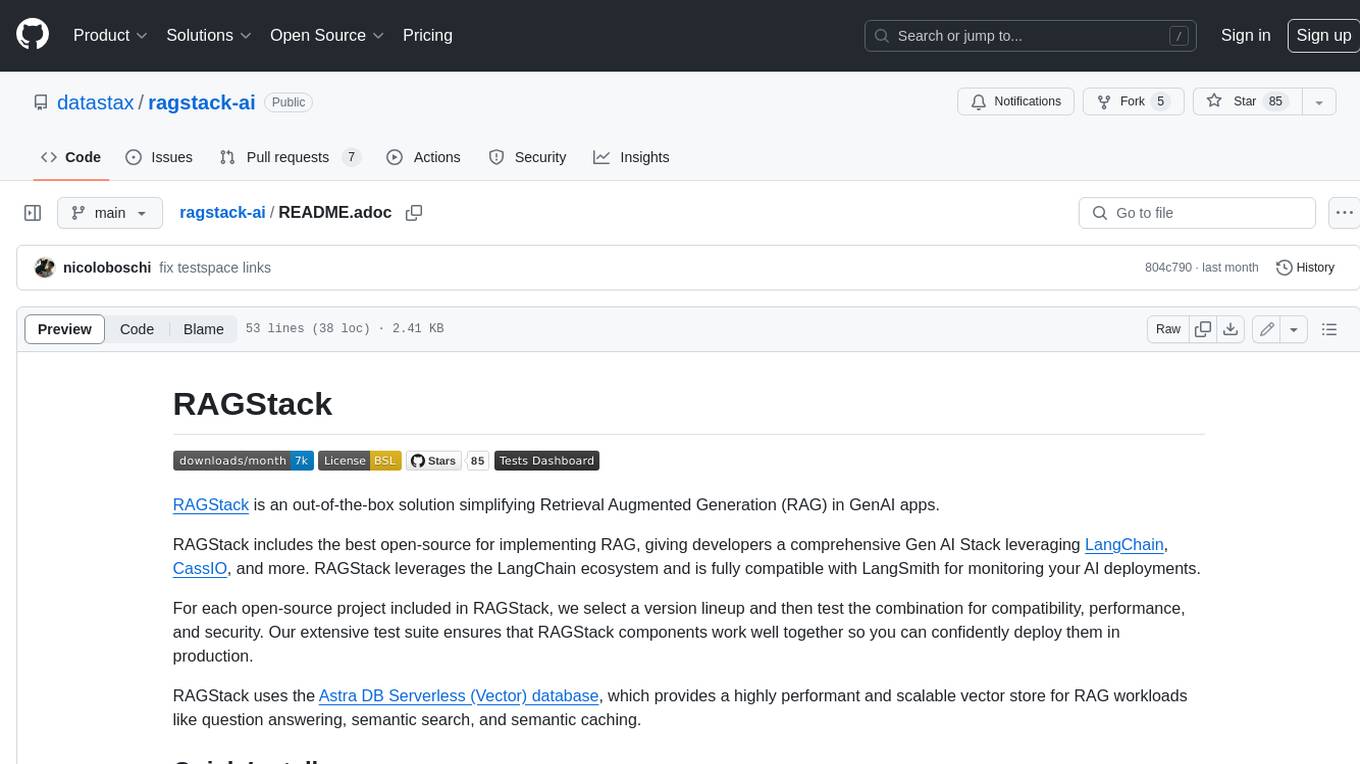
ragstack-ai
RAGStack is an out-of-the-box solution simplifying Retrieval Augmented Generation (RAG) in GenAI apps. RAGStack includes the best open-source for implementing RAG, giving developers a comprehensive Gen AI Stack leveraging LangChain, CassIO, and more. RAGStack leverages the LangChain ecosystem and is fully compatible with LangSmith for monitoring your AI deployments.
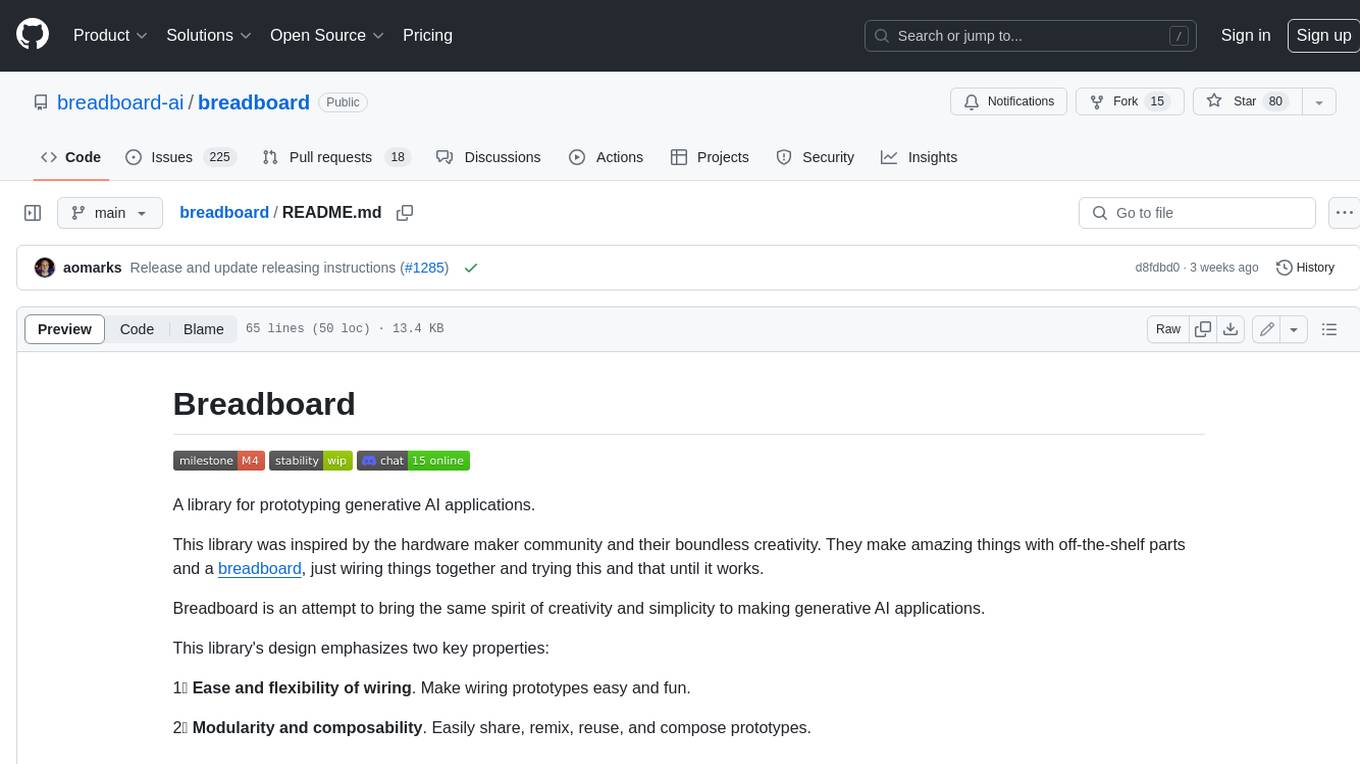
breadboard
Breadboard is a library for prototyping generative AI applications. It is inspired by the hardware maker community and their boundless creativity. Breadboard makes it easy to wire prototypes and share, remix, reuse, and compose them. The library emphasizes ease and flexibility of wiring, as well as modularity and composability.
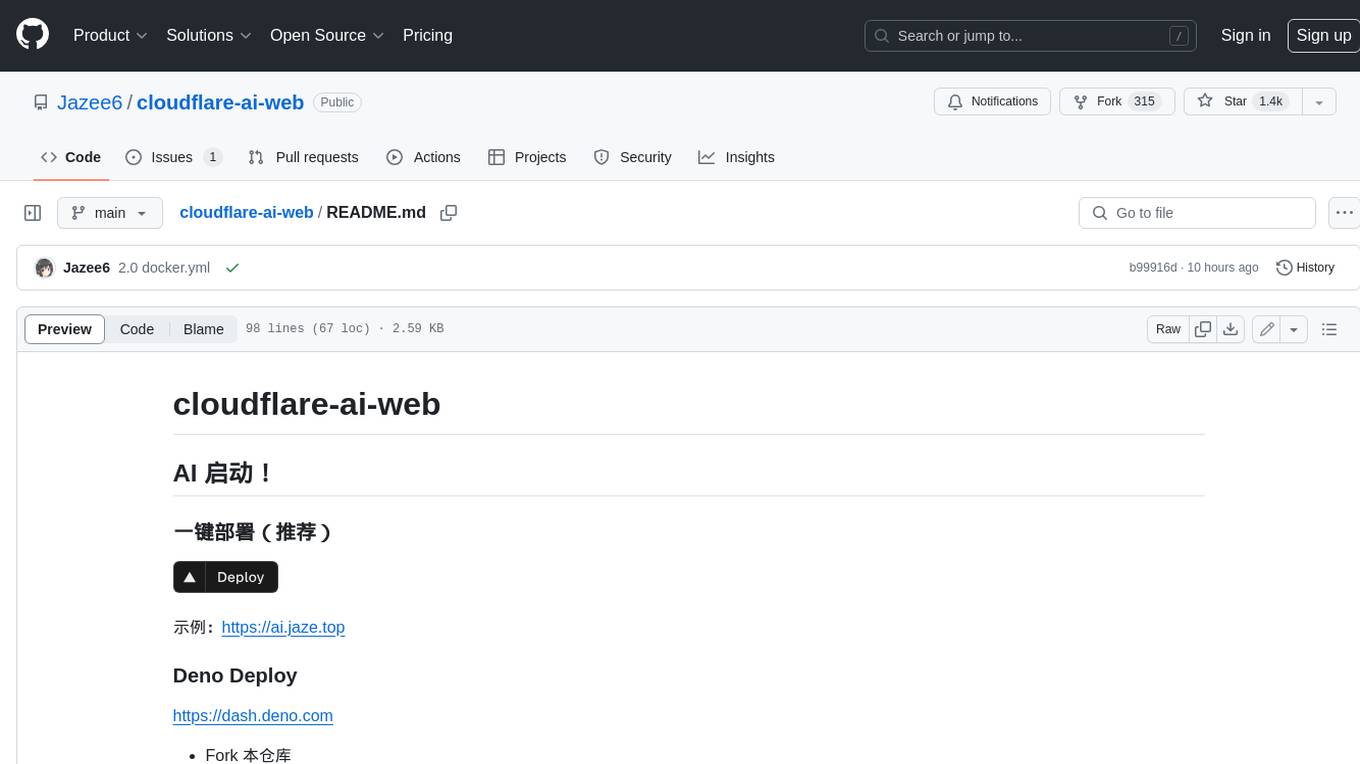
cloudflare-ai-web
Cloudflare-ai-web is a lightweight and easy-to-use tool that allows you to quickly deploy a multi-modal AI platform using Cloudflare Workers AI. It supports serverless deployment, password protection, and local storage of chat logs. With a size of only ~638 kB gzip, it is a great option for building AI-powered applications without the need for a dedicated server.
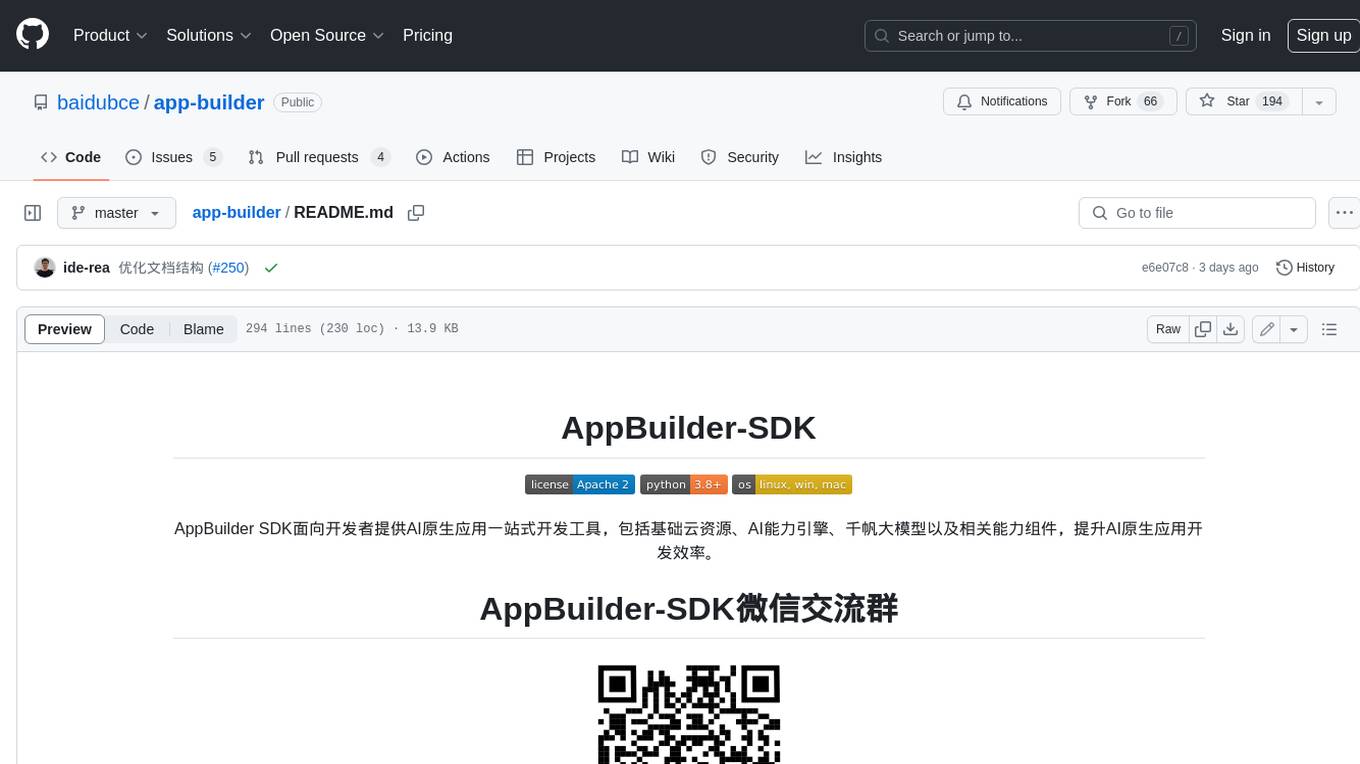
app-builder
AppBuilder SDK is a one-stop development tool for AI native applications, providing basic cloud resources, AI capability engine, Qianfan large model, and related capability components to improve the development efficiency of AI native applications.
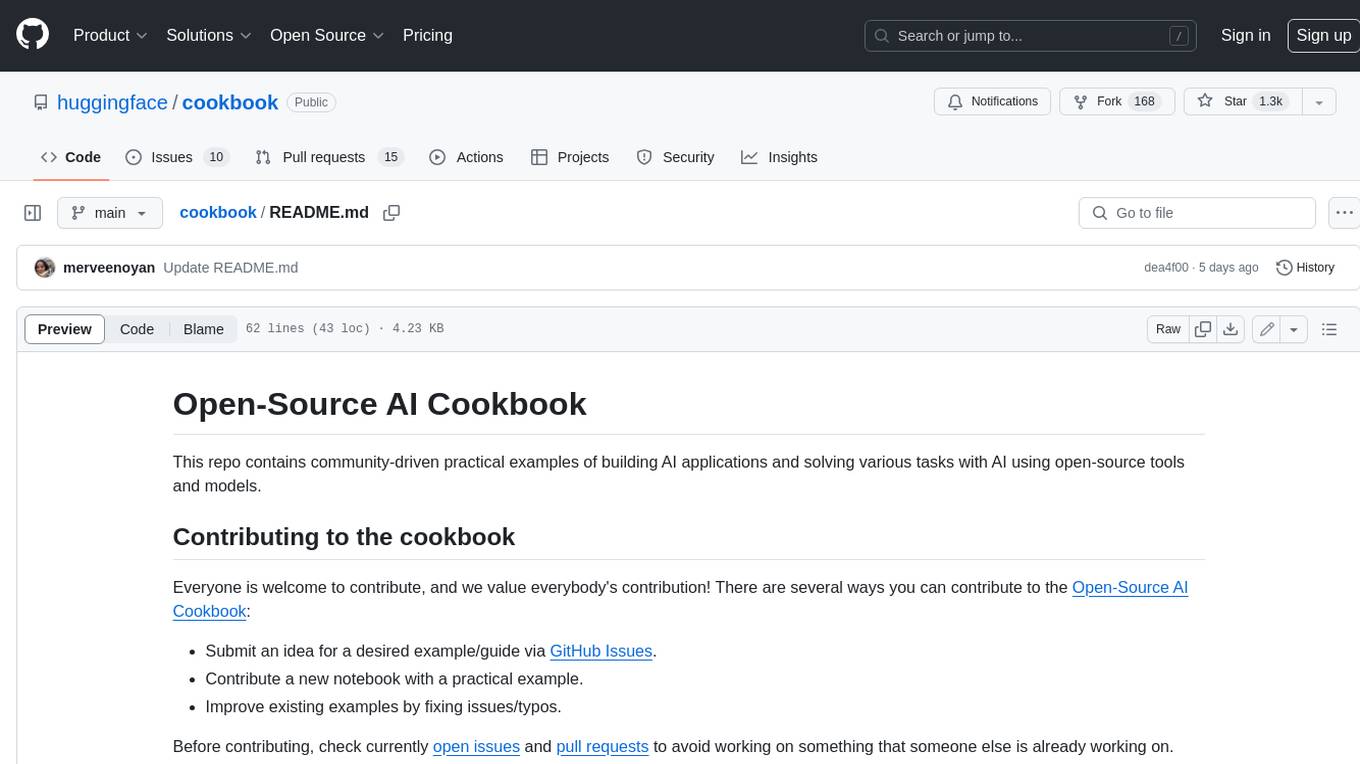
cookbook
This repository contains community-driven practical examples of building AI applications and solving various tasks with AI using open-source tools and models. Everyone is welcome to contribute, and we value everybody's contribution! There are several ways you can contribute to the Open-Source AI Cookbook: Submit an idea for a desired example/guide via GitHub Issues. Contribute a new notebook with a practical example. Improve existing examples by fixing issues/typos. Before contributing, check currently open issues and pull requests to avoid working on something that someone else is already working on.
For similar jobs
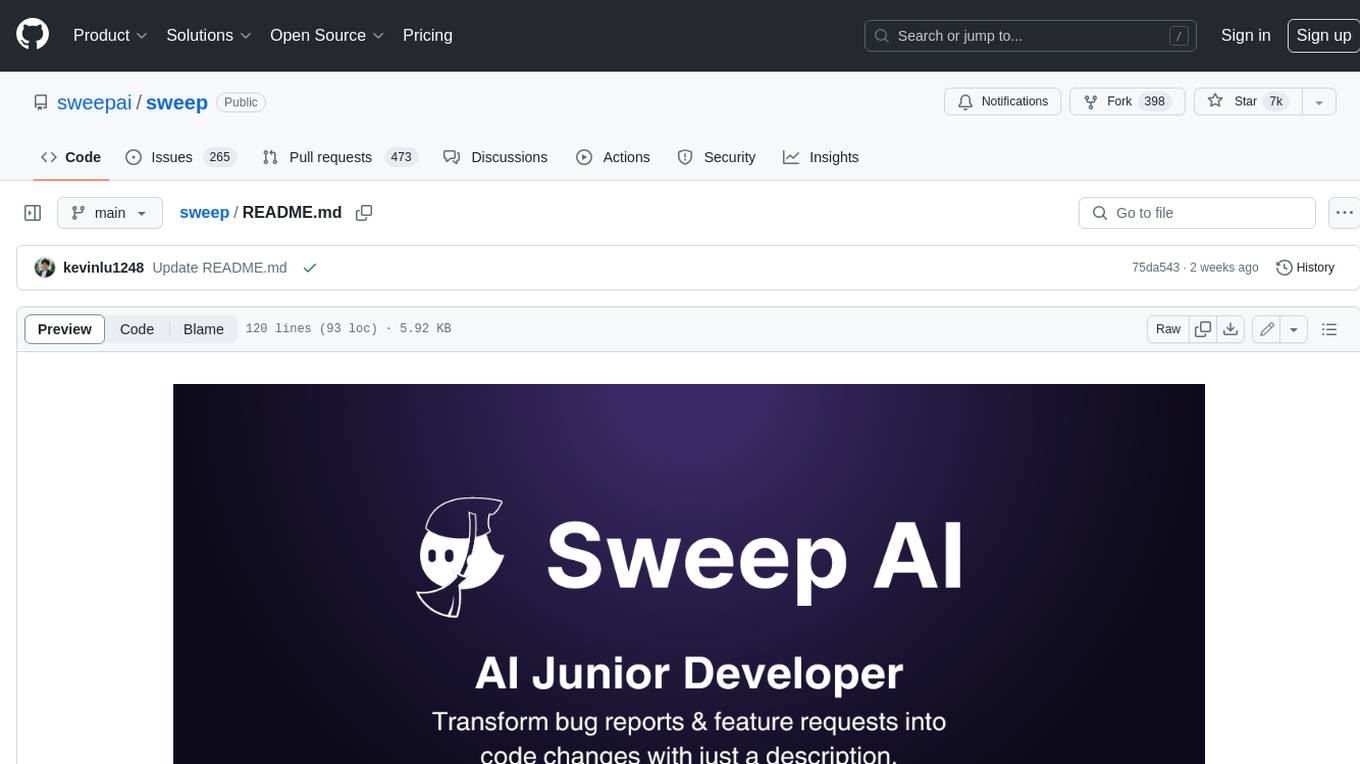
sweep
Sweep is an AI junior developer that turns bugs and feature requests into code changes. It automatically handles developer experience improvements like adding type hints and improving test coverage.
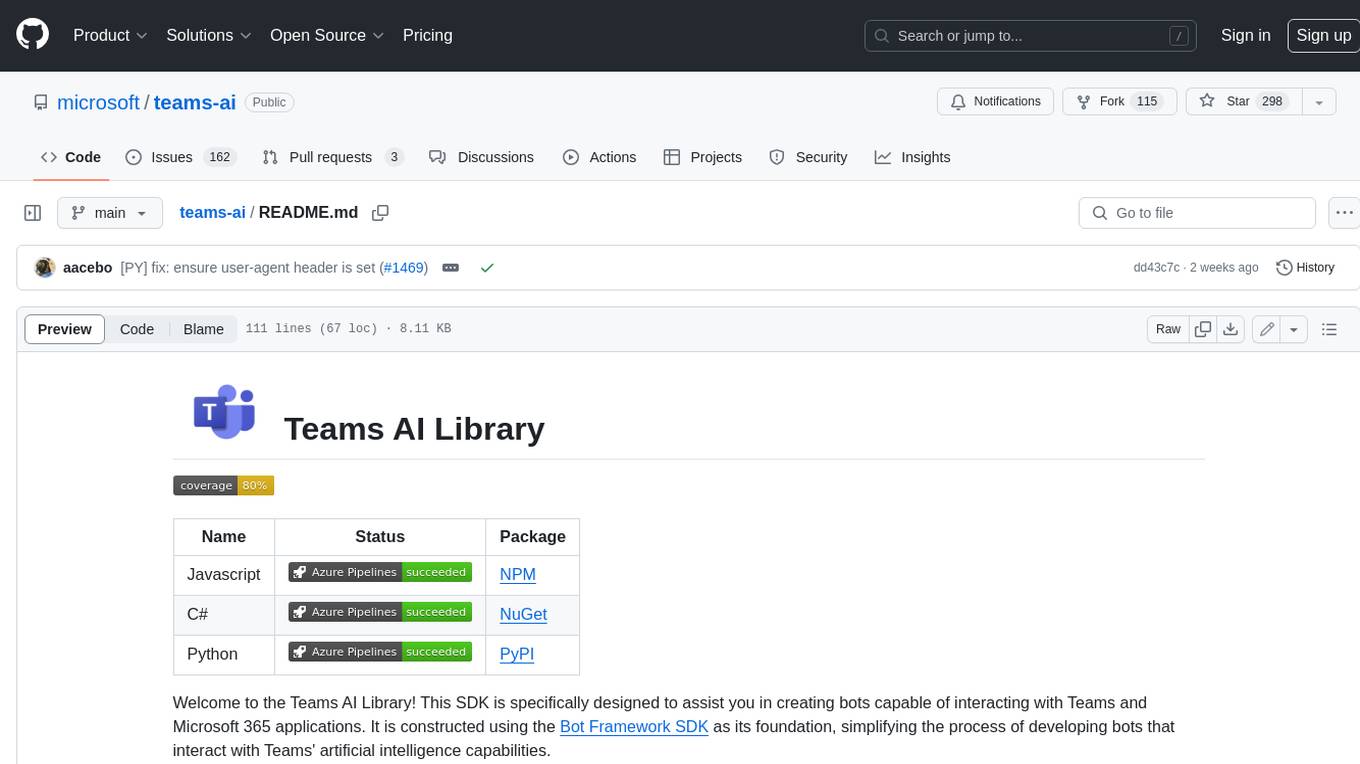
teams-ai
The Teams AI Library is a software development kit (SDK) that helps developers create bots that can interact with Teams and Microsoft 365 applications. It is built on top of the Bot Framework SDK and simplifies the process of developing bots that interact with Teams' artificial intelligence capabilities. The SDK is available for JavaScript/TypeScript, .NET, and Python.
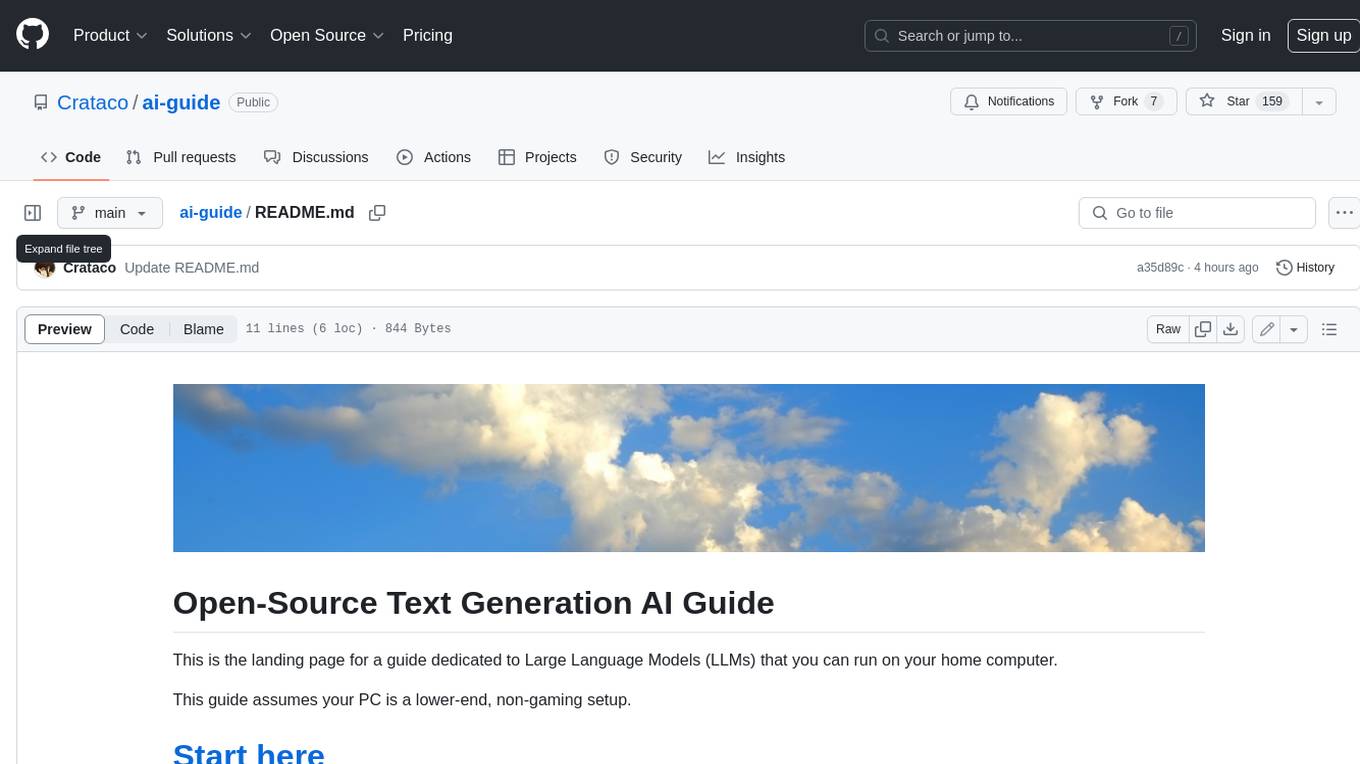
ai-guide
This guide is dedicated to Large Language Models (LLMs) that you can run on your home computer. It assumes your PC is a lower-end, non-gaming setup.
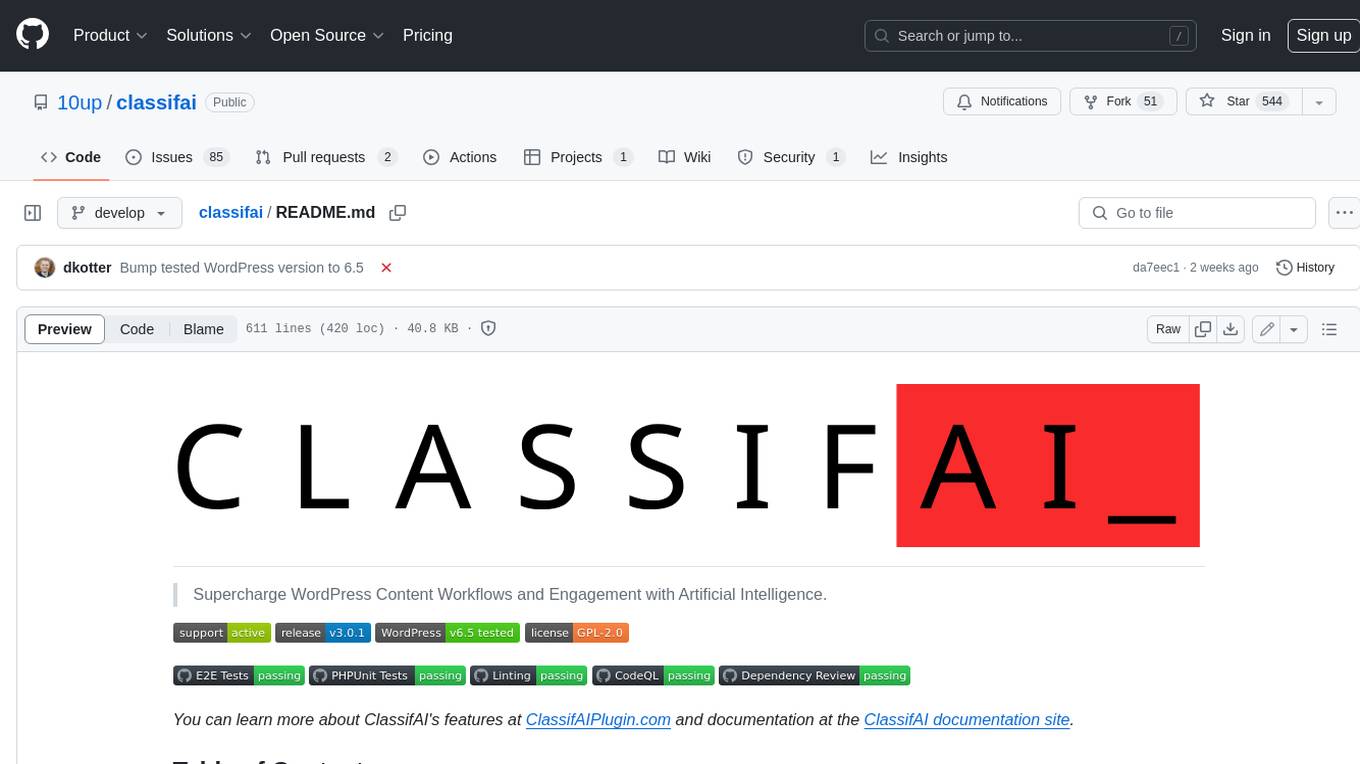
classifai
Supercharge WordPress Content Workflows and Engagement with Artificial Intelligence. Tap into leading cloud-based services like OpenAI, Microsoft Azure AI, Google Gemini and IBM Watson to augment your WordPress-powered websites. Publish content faster while improving SEO performance and increasing audience engagement. ClassifAI integrates Artificial Intelligence and Machine Learning technologies to lighten your workload and eliminate tedious tasks, giving you more time to create original content that matters.
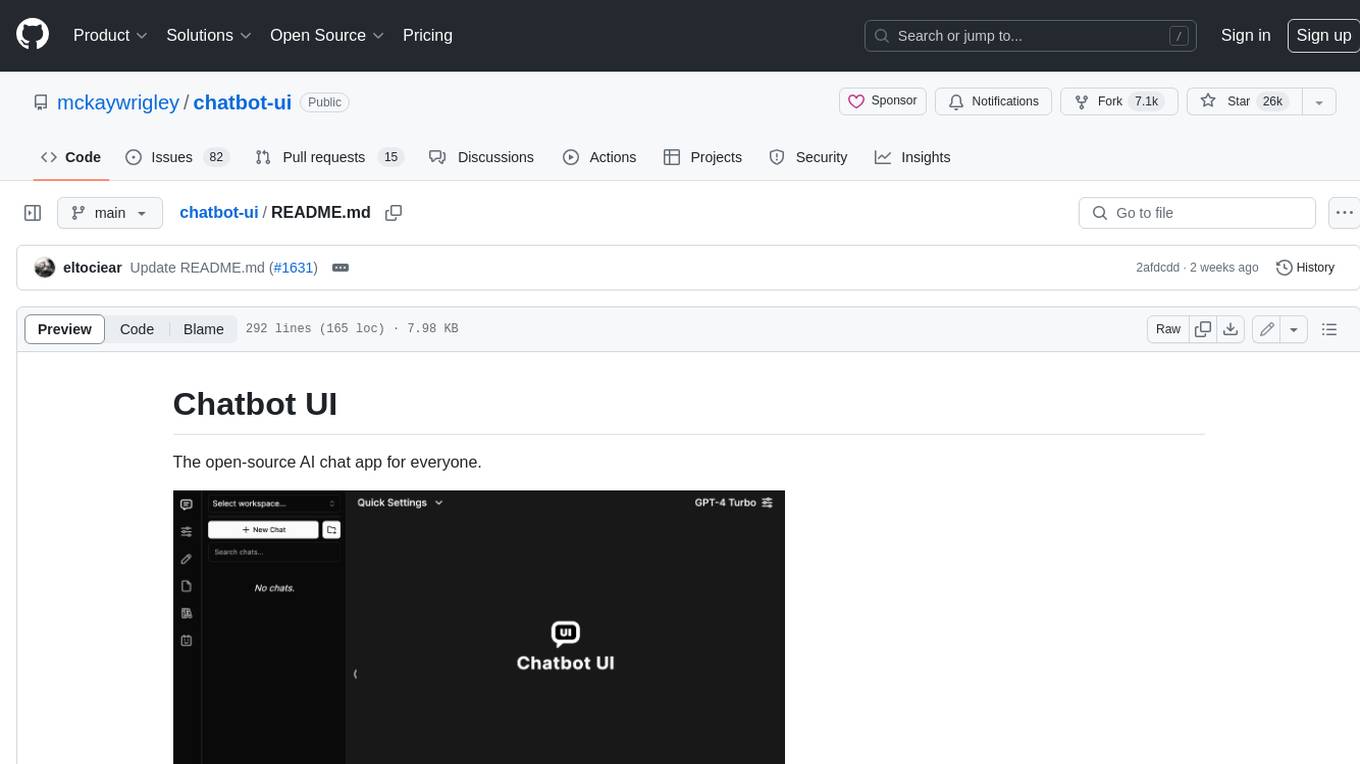
chatbot-ui
Chatbot UI is an open-source AI chat app that allows users to create and deploy their own AI chatbots. It is easy to use and can be customized to fit any need. Chatbot UI is perfect for businesses, developers, and anyone who wants to create a chatbot.
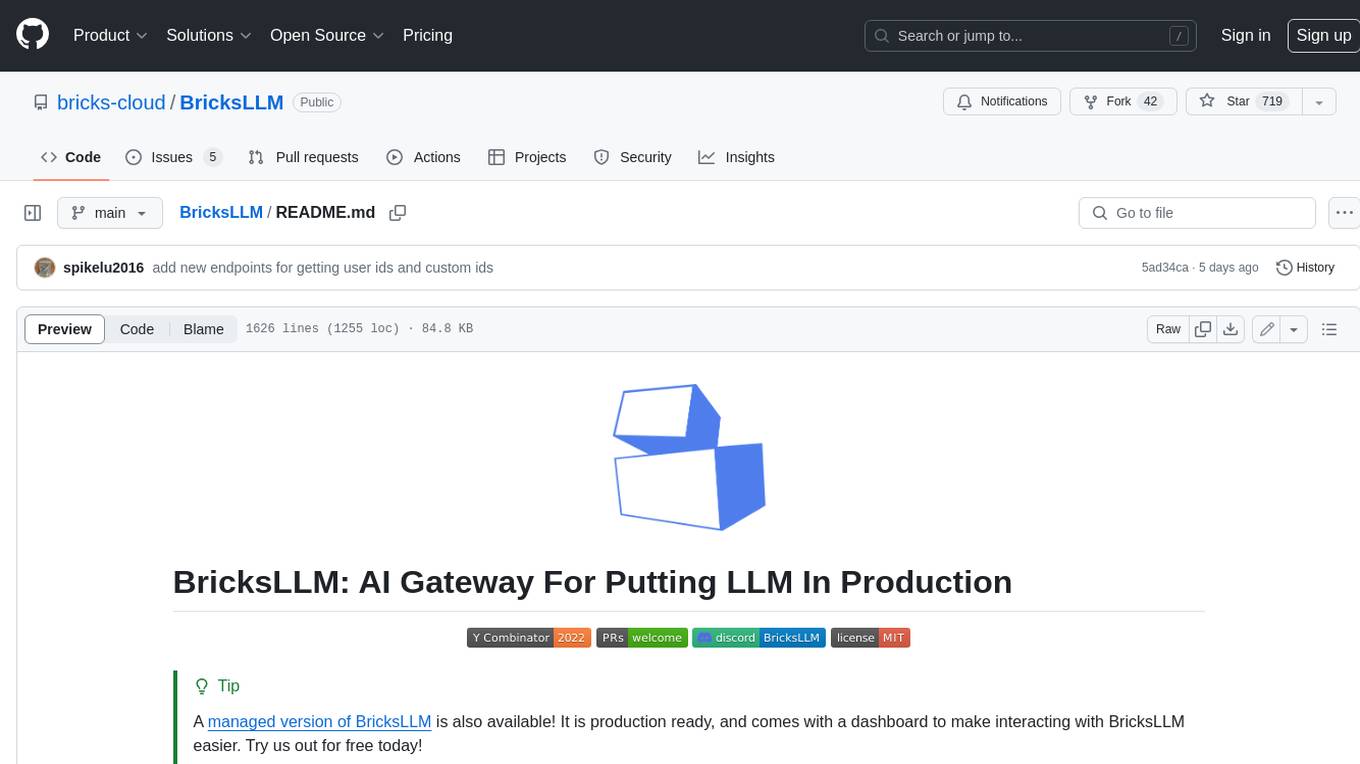
BricksLLM
BricksLLM is a cloud native AI gateway written in Go. Currently, it provides native support for OpenAI, Anthropic, Azure OpenAI and vLLM. BricksLLM aims to provide enterprise level infrastructure that can power any LLM production use cases. Here are some use cases for BricksLLM: * Set LLM usage limits for users on different pricing tiers * Track LLM usage on a per user and per organization basis * Block or redact requests containing PIIs * Improve LLM reliability with failovers, retries and caching * Distribute API keys with rate limits and cost limits for internal development/production use cases * Distribute API keys with rate limits and cost limits for students
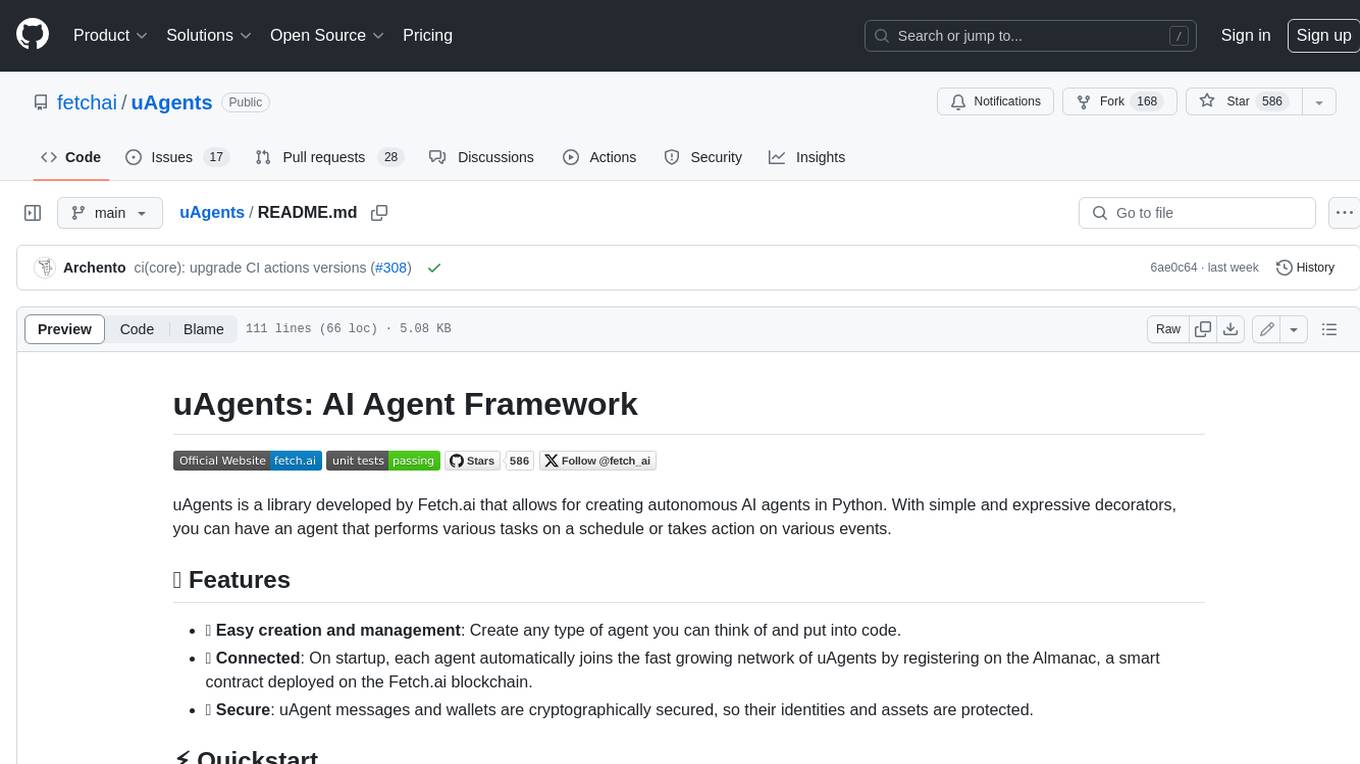
uAgents
uAgents is a Python library developed by Fetch.ai that allows for the creation of autonomous AI agents. These agents can perform various tasks on a schedule or take action on various events. uAgents are easy to create and manage, and they are connected to a fast-growing network of other uAgents. They are also secure, with cryptographically secured messages and wallets.
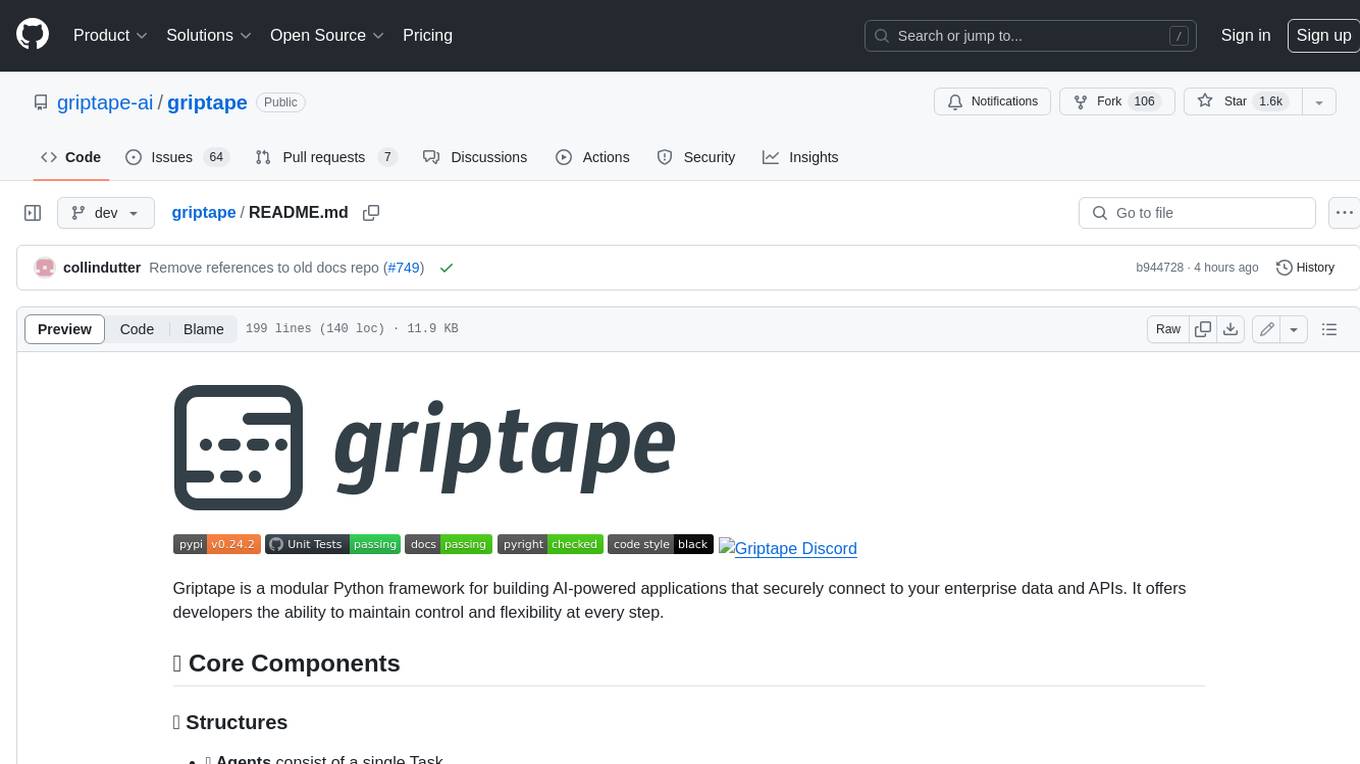
griptape
Griptape is a modular Python framework for building AI-powered applications that securely connect to your enterprise data and APIs. It offers developers the ability to maintain control and flexibility at every step. Griptape's core components include Structures (Agents, Pipelines, and Workflows), Tasks, Tools, Memory (Conversation Memory, Task Memory, and Meta Memory), Drivers (Prompt and Embedding Drivers, Vector Store Drivers, Image Generation Drivers, Image Query Drivers, SQL Drivers, Web Scraper Drivers, and Conversation Memory Drivers), Engines (Query Engines, Extraction Engines, Summary Engines, Image Generation Engines, and Image Query Engines), and additional components (Rulesets, Loaders, Artifacts, Chunkers, and Tokenizers). Griptape enables developers to create AI-powered applications with ease and efficiency.