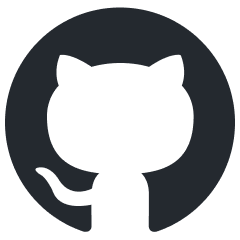
app-builder
appbuilder-sdk, 千帆AppBuilder-SDK帮助开发者灵活、快速的搭建AI原生应用
Stars: 503
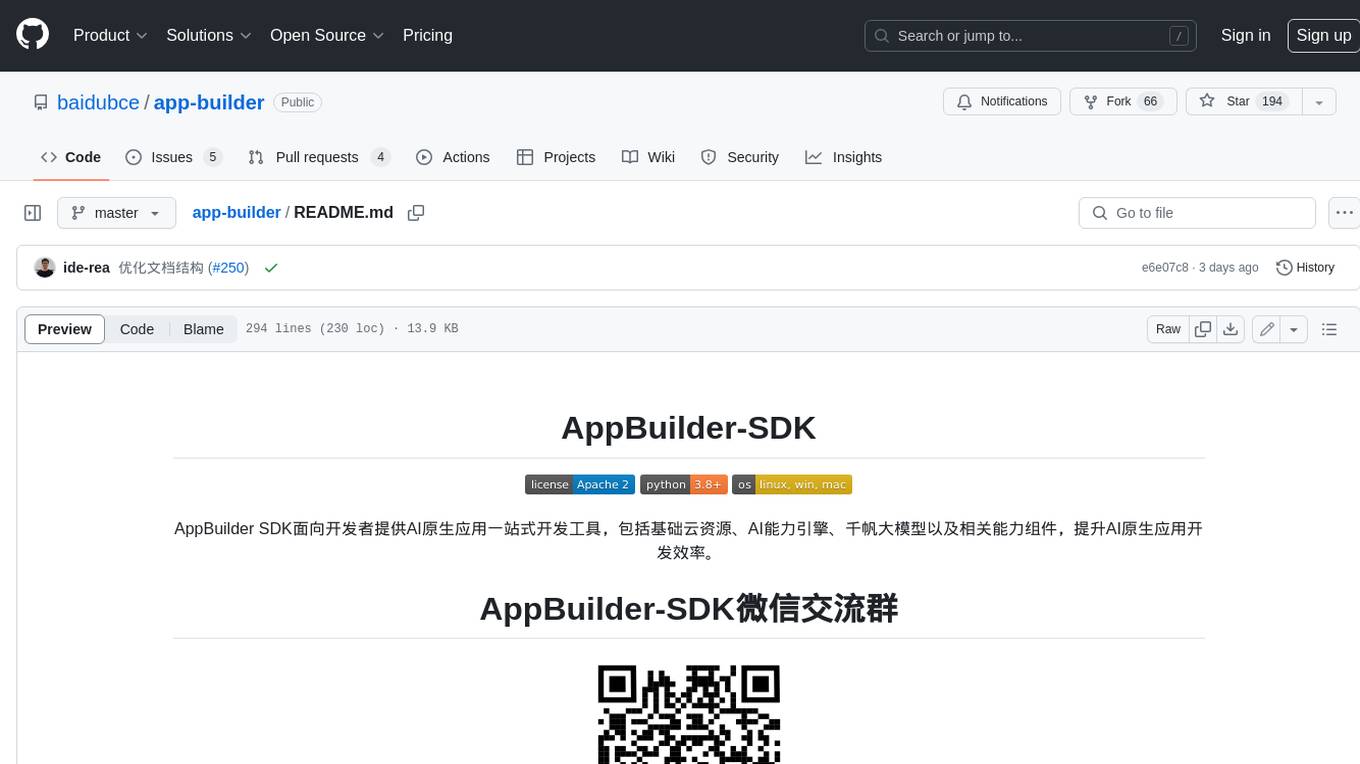
AppBuilder SDK is a one-stop development tool for AI native applications, providing basic cloud resources, AI capability engine, Qianfan large model, and related capability components to improve the development efficiency of AI native applications.
README:
百度智能云千帆AppBuilder-SDK是百度智能云千帆AppBuilder面向AI原生应用开发者提供的一站式开发平台的客户端SDK。
百度智能云千帆AppBuilder-SDK提供了以下AI应用开发者的必备功能:
-
调用
- 调用大模型,可自由调用您在百度智能云千帆大模型平台的模型,开发并调优prompt
- 调用能力组件,提供40+个源于百度生态的优质组件,赋能Agent应用
- 调用AI原生应用,通过AppBuilderClient可访问并管理在百度智能云千帆AppBuilder网页端发布的AI原生应用,并可注册本地函数联动端云组件
-
编排
- 配置知识库,通过KnowledgeBase管理知识库,进行文档及知识切片的增删改查,配合网页端开发产业级的
RAG
应用 - 编排工作流,提供了
Message
、Component
、AgentRuntime
多级工作流抽象,实现工作流编排,并可与LangChain、OpenAI等业界生态能力打通
- 配置知识库,通过KnowledgeBase管理知识库,进行文档及知识切片的增删改查,配合网页端开发产业级的
-
监控
- 提供了可视化Tracing、详细DebugLog等监控工具,助力开发者在生产环境应用
-
部署
-
AgentRuntime
支持部署为基于Flask
与gunicorn
的API服务 -
AgentRuntime
支持部署为基于Chainlit
的对话框交互前端 - 提供了
appbuilder_bce_deploy
工具,可快速部署程序到百度云,提供公网API服务,联动AppBuilder工作流
-
AppBuilder-SDK提供多类型组件,覆盖以下构建产业级RAG
应用的完整步骤:
- 文档解析(Parser)
- 文档切片(Chunker)
- 切片向量化(Embedding)
- 索引构建(Indexing)
- 切片召回(Retrieval)
- 答案生成(Answer Generation)
AppBuilder-SDK不仅提供了百度智能云提供的基础能力组件,同时提供经过深度优化的大模型高级能力组件,可以组合下表提供的原子能力组件,构建个性化的RAG应用RAG 原子能力 CookBook:
阶段 | 组件名称 | 组件类型 | 组件链接 |
---|---|---|---|
文档解析 | 文档矫正增强 (DocCropEnhance) | 基础能力组件 | 链接 |
文档解析 | 文档格式转换 (DocFormatConverter) | 基础能力组件 | 链接 |
文档解析 | 文档解析(DocParser) | 基础能力组件 | 链接 |
文档解析 | 表格抽取组件(ExtractTableFromDoc) | 基础能力组件 | 链接 |
文档解析 | 通用文字识别-高精度版(GeneralOCR) | 基础能力组件 | 链接 |
文档切片 | 文档切分(DocSplitter) | 基础能力组件 | 链接 |
切片向量化 | 向量计算(Embedding) | 基础能力组件 | 链接 |
索引构建及切片召回 | 向量检索-VectorDB(BaiduVectorDBRetriever) | 基础能力组件 | 链接 |
索引构建及切片召回 | 向量检索-BES(BaiduElasticSearchRetriever) | 基础能力组件 | 链接 |
文档切片及答案生成 | 问答对挖掘(QAPairMining) | 高级能力组件 | 链接 |
文档切片及答案生成 | 相似问生成(SimilarQuestion) | 高级能力组件 | 链接 |
答案生成 | 标签抽取(TagExtraction) | 高级能力组件 | 链接 |
答案生成 | 复杂Query判定(IsComplexQuery) | 高级能力组件 | 链接 |
答案生成 | 复杂Query分解(QueryDecomposition) | 高级能力组件 | 链接 |
答案生成 | 多轮改写 (QueryRewrite) | 高级能力组件 | 链接 |
答案生成 | 阅读理解问答(MRC) | 高级能力组件 | 链接 |
答案生成 | 幻觉检测(Hallucination Detection) | 高级能力组件 | 链接 |
功能预告:在AppBuiler-SDK 1.0.0版本中,AppBuilder-SDK可联动AppBuilder平台,自定义离线与在线处理的能力及Pipeline,构建更加灵活、可沉淀、可复用的产业级
RAG
应用,敬请期待
百度智能云千帆AppBuilder-SDK 更新记录&最新特性请查阅我们的版本说明
-
Python
版本安装,要求Python版本 >=3.9
python3 -m pip install --upgrade appbuilder-sdk
-
Java
及Go
版本安装,以及通过Docker
镜像使用,请查阅安装说明
- 请在
>=3.9
的Python环境安装appbuilder-sdk
后使用该端到端应用示例- 示例中提供了试用Token,访问和QPS受限,正式使用请替换为您的个人Token
- 使用
Playground
组件可自由调用,您在百度智能云千帆大模型平台有权限的任何模型,并可自定义prompt
模板 与 模型参数
import appbuilder
import os
# 设置环境中的TOKEN,以下TOKEN为访问和QPS受限的试用TOKEN,正式使用请替换为您的个人TOKEN
os.environ["APPBUILDER_TOKEN"] = "bce-v3/ALTAK-n5AYUIUJMarF7F7iFXVeK/1bf65eed7c8c7efef9b11388524fa1087f90ea58"
# 定义prompt模板
template_str = "你扮演{role}, 请回答我的问题。\n\n问题:{question}。\n\n回答:"
# 定义输入,调用playground组件
input = appbuilder.Message({"role": "java工程师", "question": "请简要回答java语言的内存回收机制是什么,要求100字以内"})
playground = appbuilder.Playground(prompt_template=template_str, model="Qianfan-Agent-Speed-8K")
# 以打字机的方式,流式展示大模型回答内容
output = playground(input, stream=True, temperature=1e-10)
for stream_message in output.content:
print(stream_message)
# 流式输出结束后,可再次打印完整的大模型对话结果,除回答内容外,还包括token的用量情况
print(output.model_dump_json(indent=4))
Java语言的
内存回收机制是通过垃圾回收器(Garbage Collector)来实现的。
垃圾回收器会自动检测不再使用的对象,并释放其占用的内存空间,从而确保系统的内存不会被耗尽。
Java提供了多种垃圾回收器,如串行回收器、并行回收器、CMS回收器和G1回收器等,以满足不同场景下的性能需求
。
{
"content": "Java语言的内存回收机制是通过垃圾回收器(Garbage Collector)来实现的。垃圾回收器会自动检测不再使用的对象,并释放其占用的内存空间,从而确保系统的内存不会被耗尽。Java提供了多种垃圾回收器,如串行回收器、并行回收器、CMS回收器和G1回收器等,以满足不同场景下的性能需求。",
"name": "msg",
"mtype": "dict",
"id": "2bbee989-40e3-45e4-9802-e144cdc829a9",
"extra": {},
"token_usage": {
"prompt_tokens": 35,
"completion_tokens": 70,
"total_tokens": 105
}
}
- SDK提供了40+个源于百度生态的优质组件,列表可见组件列表, 调用前需要申领免费试用额度
- 示例中的组件为
RAG with Baidu Search增强版
, 结合百度搜索的搜索引擎技术和ERNIE模型的语义理解能力,可以更准确地理解用户的搜索意图,并提供与搜索查询相关性更高的搜索结果
import appbuilder
import os
# 设置环境中的TOKEN,以下TOKEN为访问和QPS受限的试用TOKEN,正式使用请替换为您的个人TOKEN
os.environ["APPBUILDER_TOKEN"] = "bce-v3/ALTAK-n5AYUIUJMarF7F7iFXVeK/1bf65eed7c8c7efef9b11388524fa1087f90ea58"
rag_with_baidu_search_pro = appbuilder.RagWithBaiduSearchPro(model="Qianfan-Agent-Speed-8K")
input = appbuilder.Message("9.11和9.8哪个大")
result = rag_with_baidu_search_pro.run(
message=input,
instruction=appbuilder.Message("你是专业知识助手"))
# 输出运行结果
print(result.model_dump_json(indent=4))
{
"content": "9.11小于9.8。在比较两个小数的大小时,需要逐位比较它们的数值,包括整数部分和小数部分。对于9.11和9.8,整数部分都是9,所以需要在小数部分进行比较。小数点后的第一位是1和8,显然1小于8,所以9.11小于9.8。",
"name": "msg",
"mtype": "dict",
"id": "eb31b7de-dd6a-485f-adb9-1f7921a6f4bf",
"extra": {
"search_baidu": [
{
"content": "大模型‘智商’受质疑:9.11 vs 9...",
"icon": "https://appbuilder.bj.bcebos.com/baidu-search-rag-pro/icon/souhu.ico",
"url": "https://m.sohu.com/a/793754123_121924584/",
"ref_id": "2",
"site_name": "搜狐网",
"title": "大模型‘智商’受质疑:9.11 vs 9.8的比较揭示AI理解能力的..."
},
{
"content": "究竟|9.11比9.8大?大模型们为何会...",
"icon": "https://appbuilder.bj.bcebos.com/baidu-search-rag-pro/icon/tencent.svg.png",
"url": "https://new.qq.com/rain/a/20240717A07JLV00",
"ref_id": "4",
"site_name": "腾讯网",
"title": "究竟|9.11比9.8大?大模型们为何会在小学数学题上集体..."
},
...
]
},
"token_usage": {
"completion_tokens": 77,
"prompt_tokens": 2008,
"total_tokens": 2085
}
}
- 示例中的应用为:说唱导师,点击该连接在网页端试用
import appbuilder
import os
# 设置环境中的TOKEN,以下TOKEN为访问和QPS受限的试用TOKEN,正式使用请替换为您的个人TOKEN
os.environ["APPBUILDER_TOKEN"] = "bce-v3/ALTAK-n5AYUIUJMarF7F7iFXVeK/1bf65eed7c8c7efef9b11388524fa1087f90ea58"
# 从AppBuilder网页获取并传入应用ID,以下为说唱导师应用ID
app_id = "4678492a-5864-472e-810a-654538d3503c"
app_builder_client = appbuilder.AppBuilderClient(app_id)
conversation_id = app_builder_client.create_conversation()
answer = app_builder_client.run(conversation_id, "以“上班狼狈却又追逐梦想“为主题进行一首说唱创作,保持押韵, 控制在200字以内")
print(answer.content.answer)
好的,我们来以“上班狼狈却又追逐梦想”为主题,进行一段简短的说唱创作。这里是一个简单的示例,你可以根据自己的感觉进行调整:
Intro:
朝九晚五,生活重压,
狼狈上班,却心怀梦想,
每一天,都是新的挑战,
为了那未来,我奋发向前。
Verse 1:
穿上西装,打好领带,
步入人群,去追逐名利,
虽然狼狈,却不曾言败,
因为心中,有梦想在激励。
Hook:
上班狼狈,却不曾放弃,
追逐梦想,是我心中的火炬,
照亮前路,指引我前行,
无论多难,我都要坚持到底。
这首小曲儿以“上班狼狈却又追逐梦想”为主题,通过押韵的方式表达了上班族虽然生活艰辛,但依然怀揣梦想,勇往直前的精神。希望你喜欢!
应用类型 | 应用链接 | 推荐理由 |
---|---|---|
基础能力组件 | 通用文字识别 | 体验百度AI开放平台提供的通用文字识别-高精度版的精准识别结果 |
基础能力组件 | 基础组件服务化 | 基础组件可通过flask实现服务化部署 或 通过chainlit实现可交互的前端部署,集成到您的系统中 |
流程编排 | Assistant SDK | 学习如何纯代码态搭建一个Agent应用,并实现自定义工作流程及FunctionCall |
端到端应用 | AppBuilder Client SDK | 使用AppBuilder网页端创建并发布一个Agent应用后,通过AppBuilderClient SDK集成到你的系统中 |
端到端应用 | Agent应用-工作流Agent | 使用AppBuilder网页端创建并发布一个工作流Agent应用后,通过AppBuilderClient SDK集成到你的系统中 |
端到端应用 | 通过AppBuilder-ToolCall功能实现端云组件联动的Agent | 学习Agent、FunctionCall的知识,并构造调用本地组件的Agent |
端到端应用 | 简历筛选小助手 | 通过对本地简历库的简历进行解析、切片、创建索引,实现基于JD进行简历筛选,并对筛选的Top1简历进行总结 |
端到端应用 | 企业级问答系统 | 学习如何通过SDK与网页平台搭配,实现离线知识库生产与在线问答 |
进阶应用 | 使用appbuilder_bce_deploy部署公有云服务 | 一键将自己的服务部署到百度智能云,部署后可以自动生成公网ip,联动工作流的API节点 |
进阶应用 | 使用appbuilder_trace_server实现对使用状态的跟踪 | 使用Appbuilder-SDK Trace功能实现对组件、应用调用情况的追踪 |
-
首页
- 快速上手:
- 开始你的第一个AI原生应用:
- 产业实践应用范例:
- SDK当前支持的编程语言
- 基础:
- 模型:
- 组件
- 监控:
- 部署:
- 平台:
- 应用:
- 知识库:
- 自定义组件:
- 应用:
- Agent:
- RAG:
- Workflow:
- 开发者指南:
- 快速上手:
-
Github Issue: 提交安装/使用问题、报告bug、建议新特性、沟通开发计划等
AppBuilder-SDK遵循Apache-2.0开源协议。
For Tasks:
Click tags to check more tools for each tasksFor Jobs:
Alternative AI tools for app-builder
Similar Open Source Tools
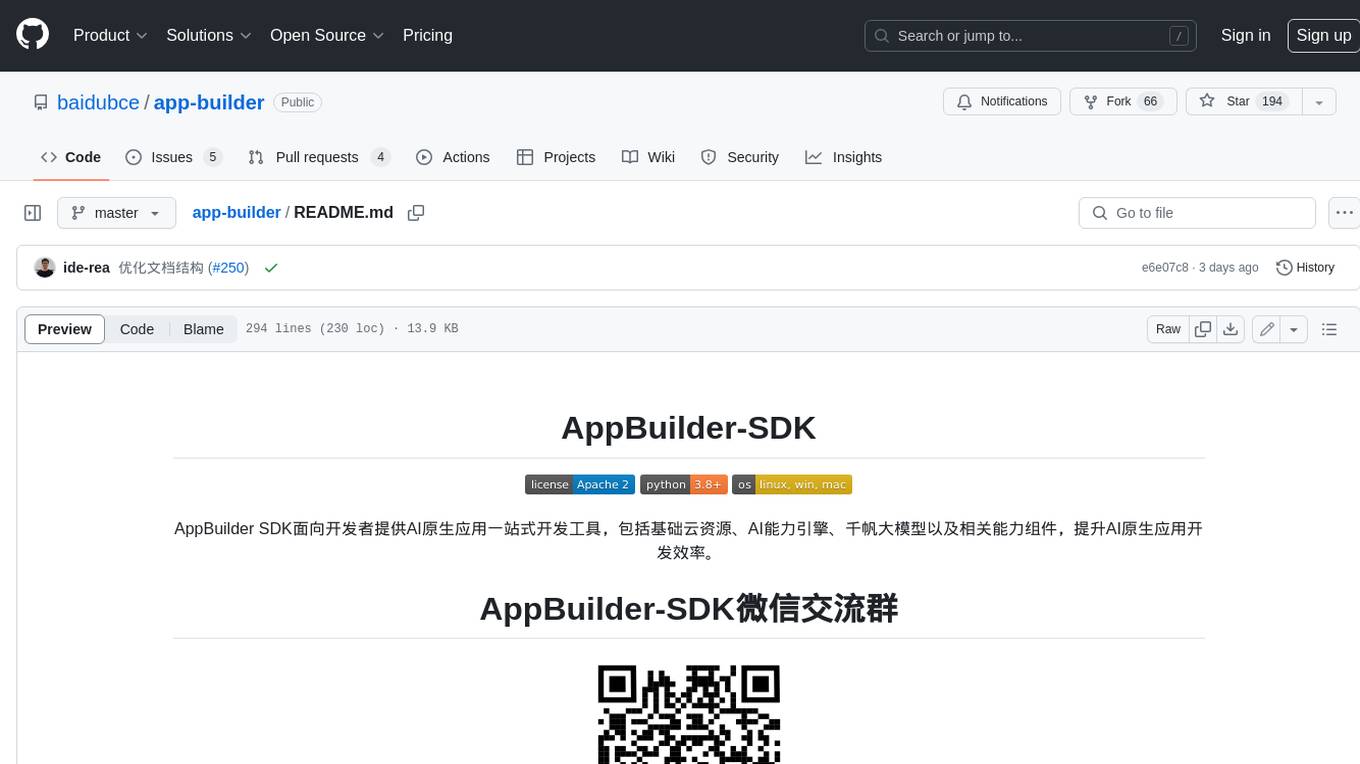
app-builder
AppBuilder SDK is a one-stop development tool for AI native applications, providing basic cloud resources, AI capability engine, Qianfan large model, and related capability components to improve the development efficiency of AI native applications.
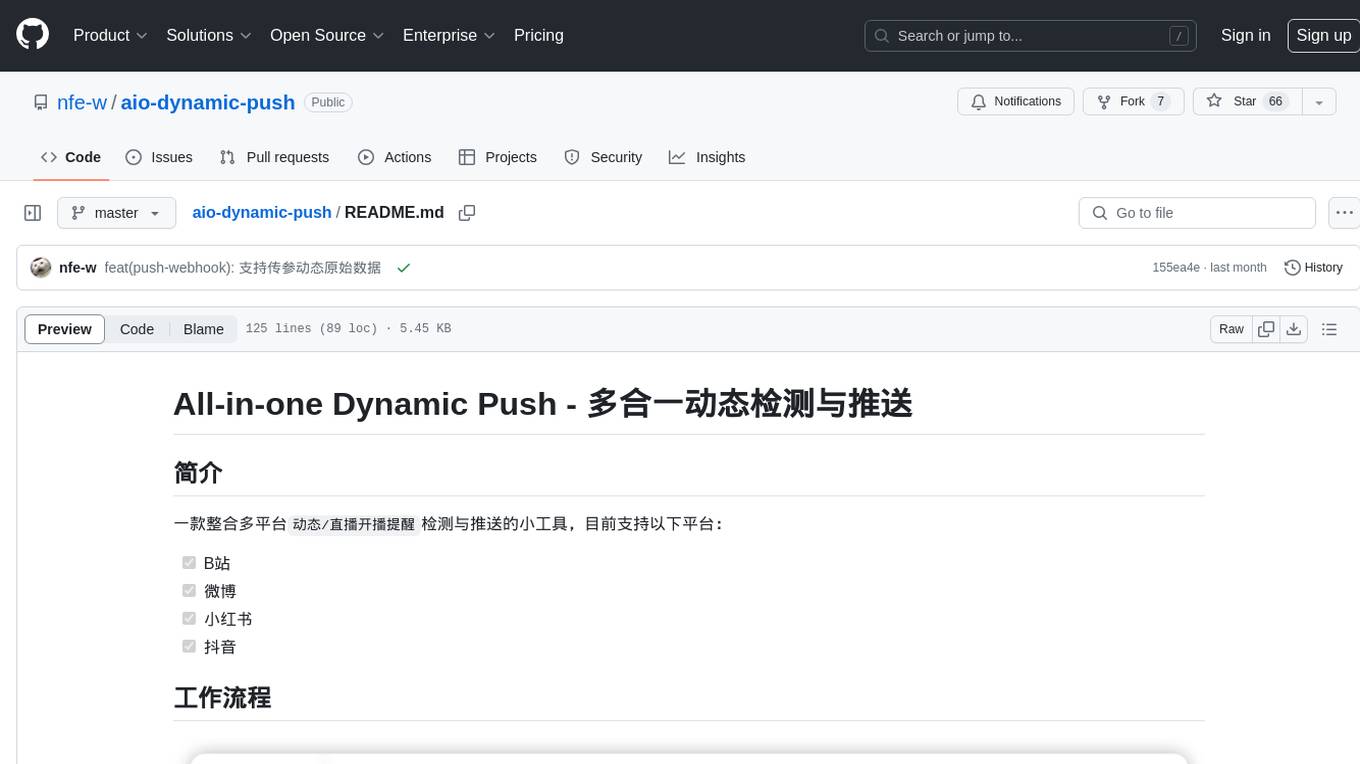
aio-dynamic-push
Aio-dynamic-push is a tool that integrates multiple platforms for dynamic/live streaming alerts detection and push notifications. It currently supports platforms such as Bilibili, Weibo, Xiaohongshu, and Douyin. Users can configure different tasks and push channels in the config file to receive notifications. The tool is designed to simplify the process of monitoring and receiving alerts from various social media platforms, making it convenient for users to stay updated on their favorite content creators or accounts.
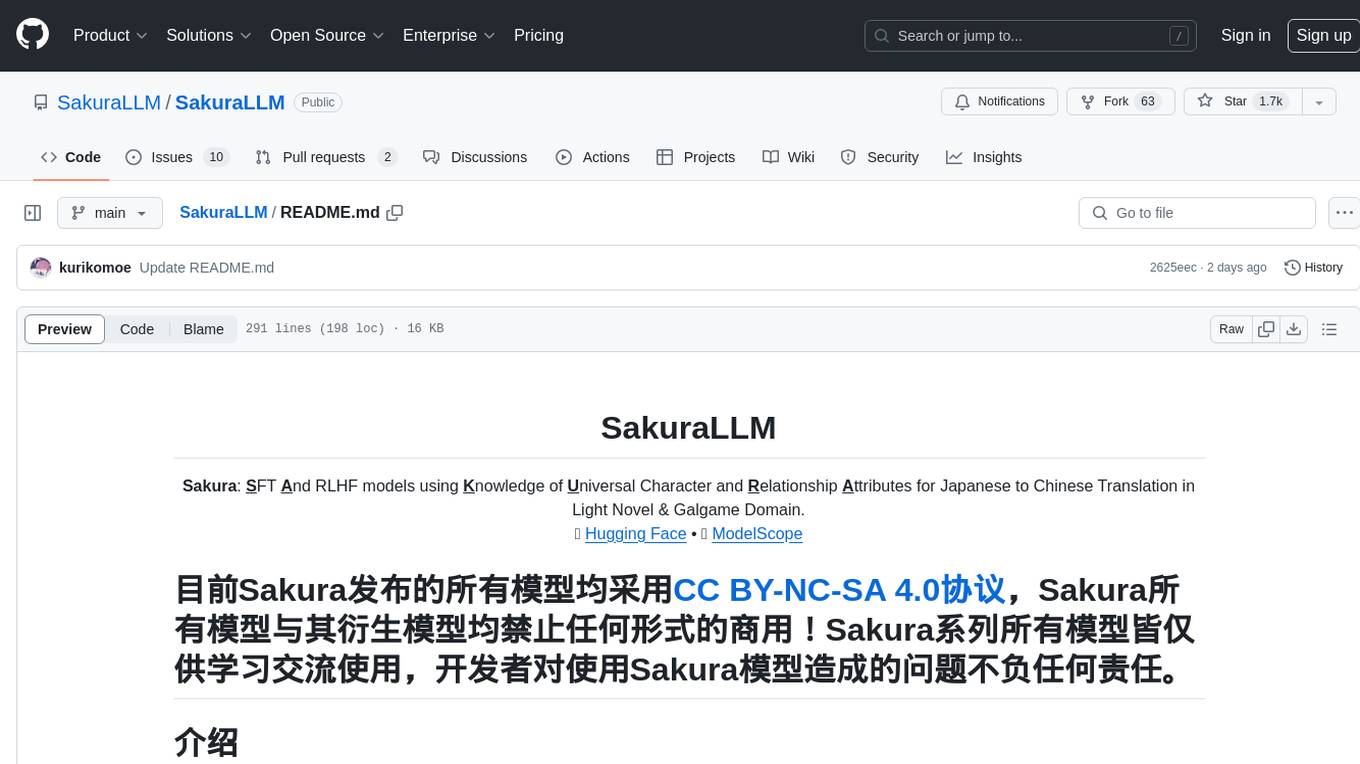
SakuraLLM
SakuraLLM is a project focused on building large language models for Japanese to Chinese translation in the light novel and galgame domain. The models are based on open-source large models and are pre-trained and fine-tuned on general Japanese corpora and specific domains. The project aims to provide high-performance language models for galgame/light novel translation that are comparable to GPT3.5 and can be used offline. It also offers an API backend for running the models, compatible with the OpenAI API format. The project is experimental, with version 0.9 showing improvements in style, fluency, and accuracy over GPT-3.5.
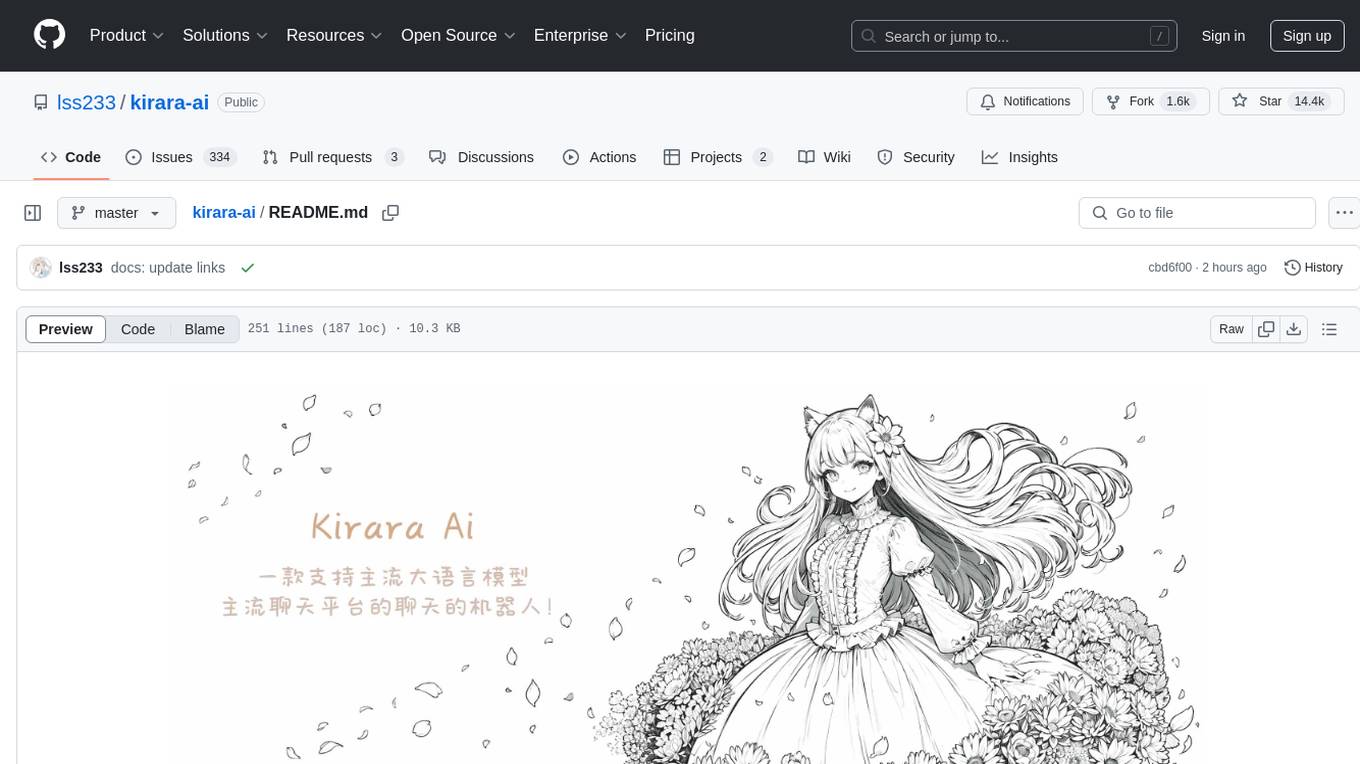
kirara-ai
Kirara AI is a chatbot that supports mainstream large language models and chat platforms. It provides features such as image sending, keyword-triggered replies, multi-account support, personality settings, and support for various chat platforms like QQ, Telegram, Discord, and WeChat. The tool also supports HTTP server for Web API, popular large models like OpenAI and DeepSeek, plugin mechanism, conditional triggers, admin commands, drawing models, voice replies, multi-turn conversations, cross-platform message sending, custom workflows, web management interface, and built-in Frpc intranet penetration.
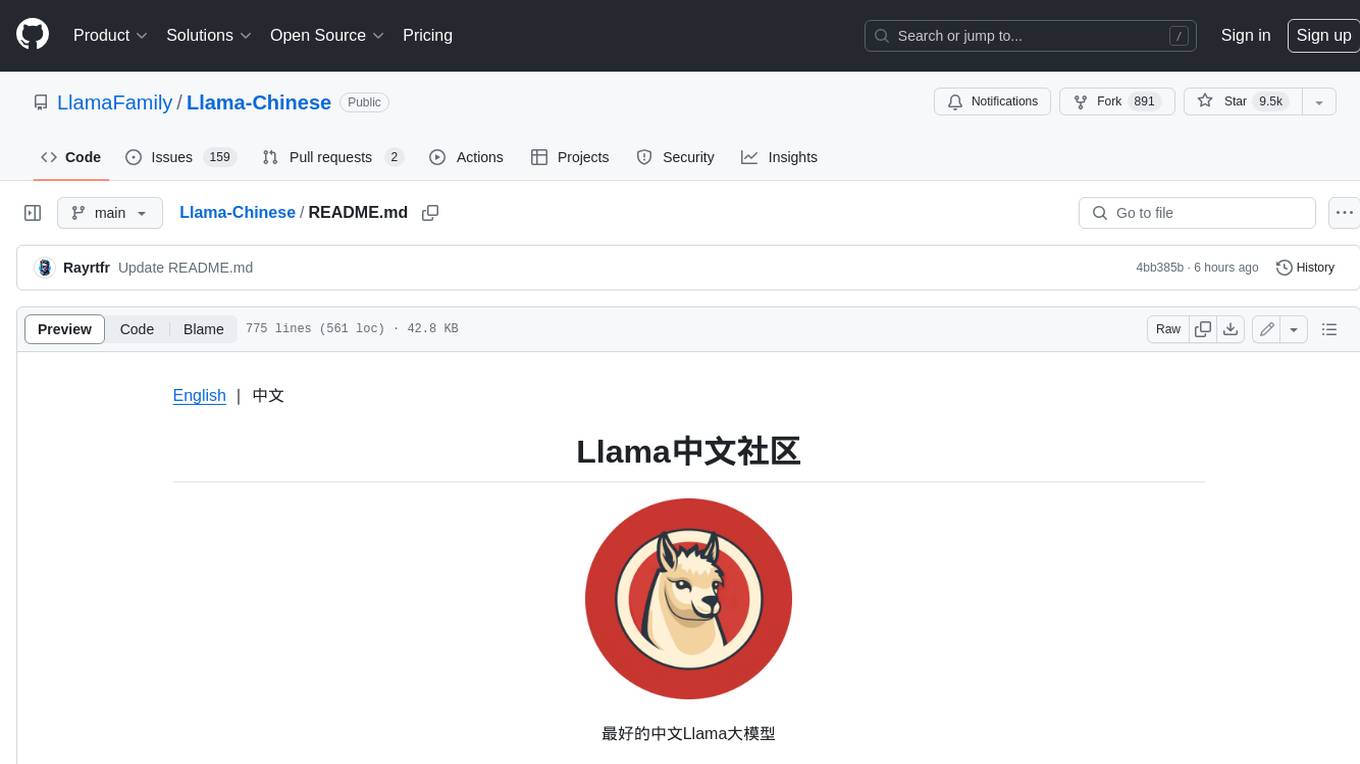
Llama-Chinese
Llama中文社区是一个专注于Llama模型在中文方面的优化和上层建设的高级技术社区。 **已经基于大规模中文数据,从预训练开始对Llama2模型进行中文能力的持续迭代升级【Done】**。**正在对Llama3模型进行中文能力的持续迭代升级【Doing】** 我们热忱欢迎对大模型LLM充满热情的开发者和研究者加入我们的行列。
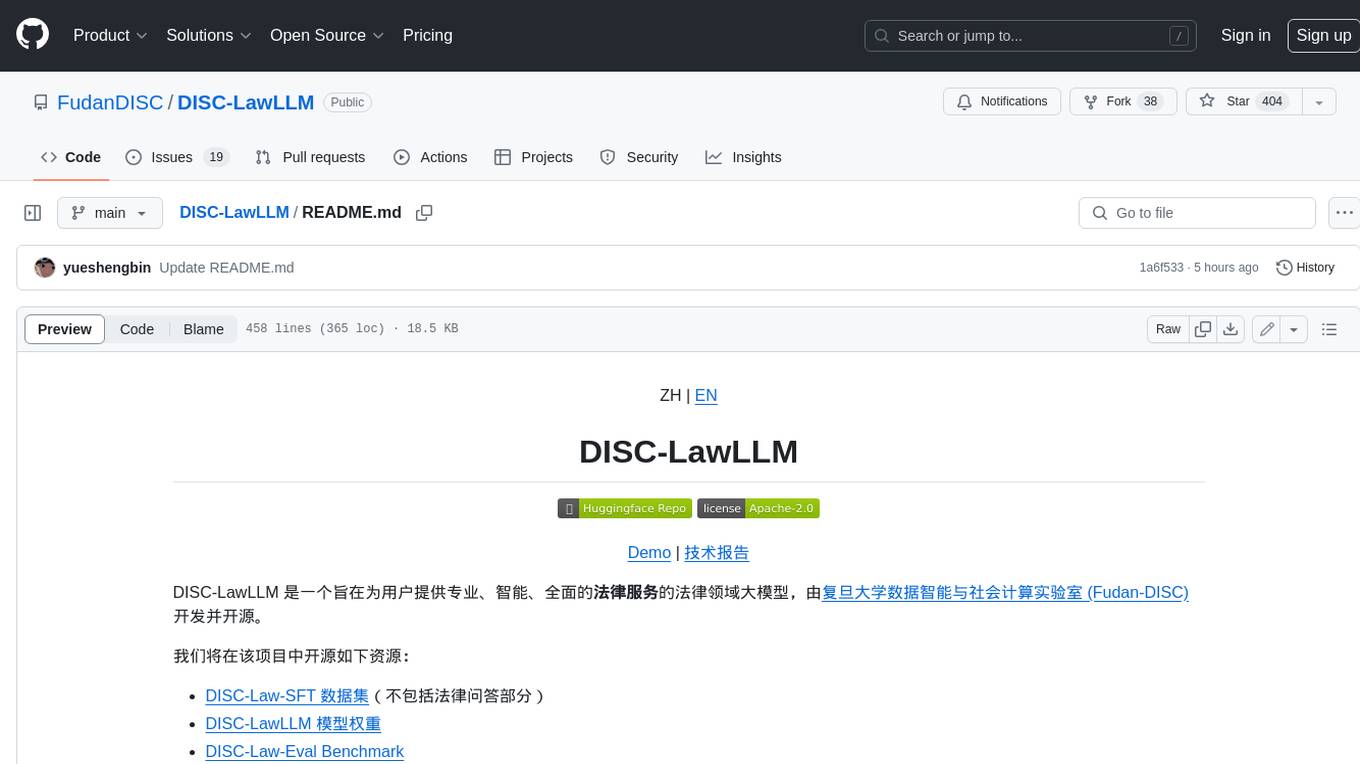
DISC-LawLLM
DISC-LawLLM is a legal domain large model that aims to provide professional, intelligent, and comprehensive **legal services** to users. It is developed and open-sourced by the Data Intelligence and Social Computing Lab (Fudan-DISC) at Fudan University.
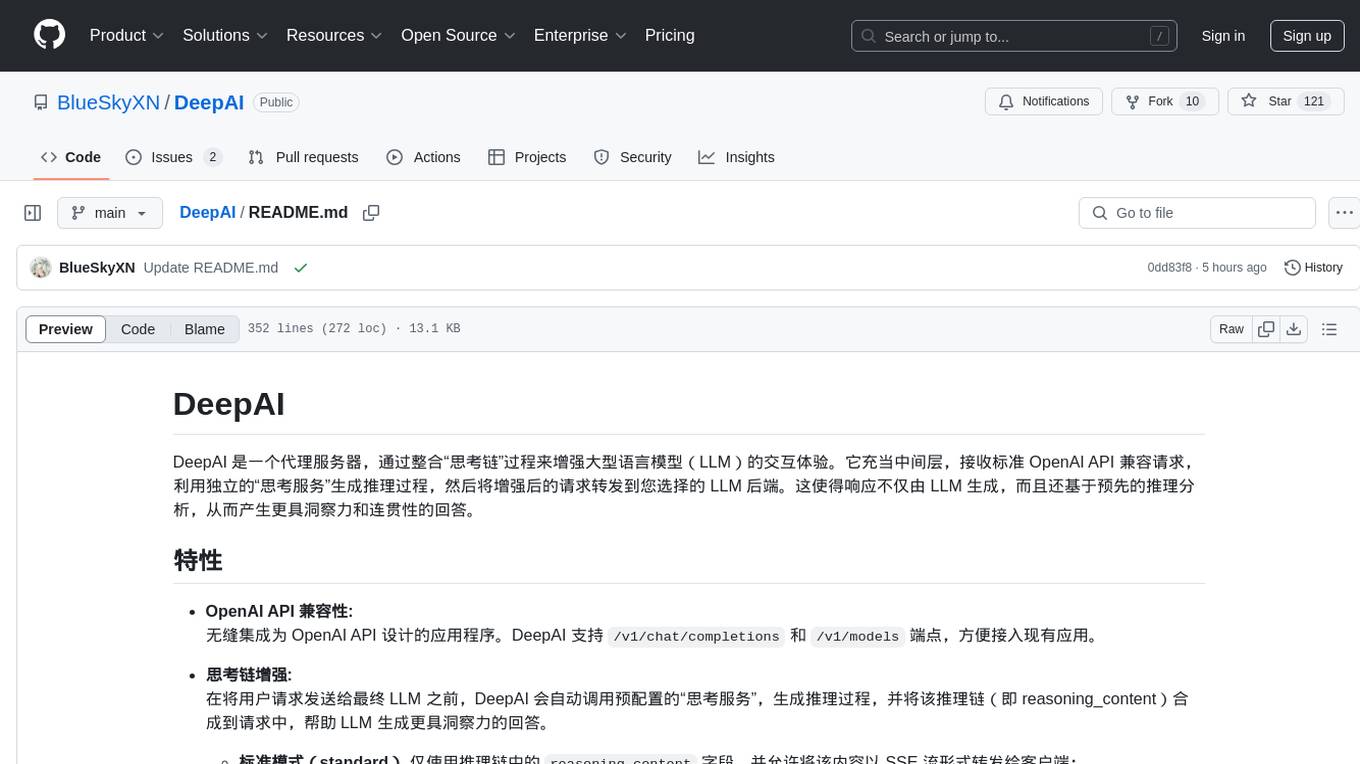
DeepAI
DeepAI is a proxy server that enhances the interaction experience of large language models (LLMs) by integrating the 'thinking chain' process. It acts as an intermediary layer, receiving standard OpenAI API compatible requests, using independent 'thinking services' to generate reasoning processes, and then forwarding the enhanced requests to the LLM backend of your choice. This ensures that responses are not only generated by the LLM but also based on pre-inference analysis, resulting in more insightful and coherent answers. DeepAI supports seamless integration with applications designed for the OpenAI API, providing endpoints for '/v1/chat/completions' and '/v1/models', making it easy to integrate into existing applications. It offers features such as reasoning chain enhancement, flexible backend support, API key routing, weighted random selection, proxy support, comprehensive logging, and graceful shutdown.
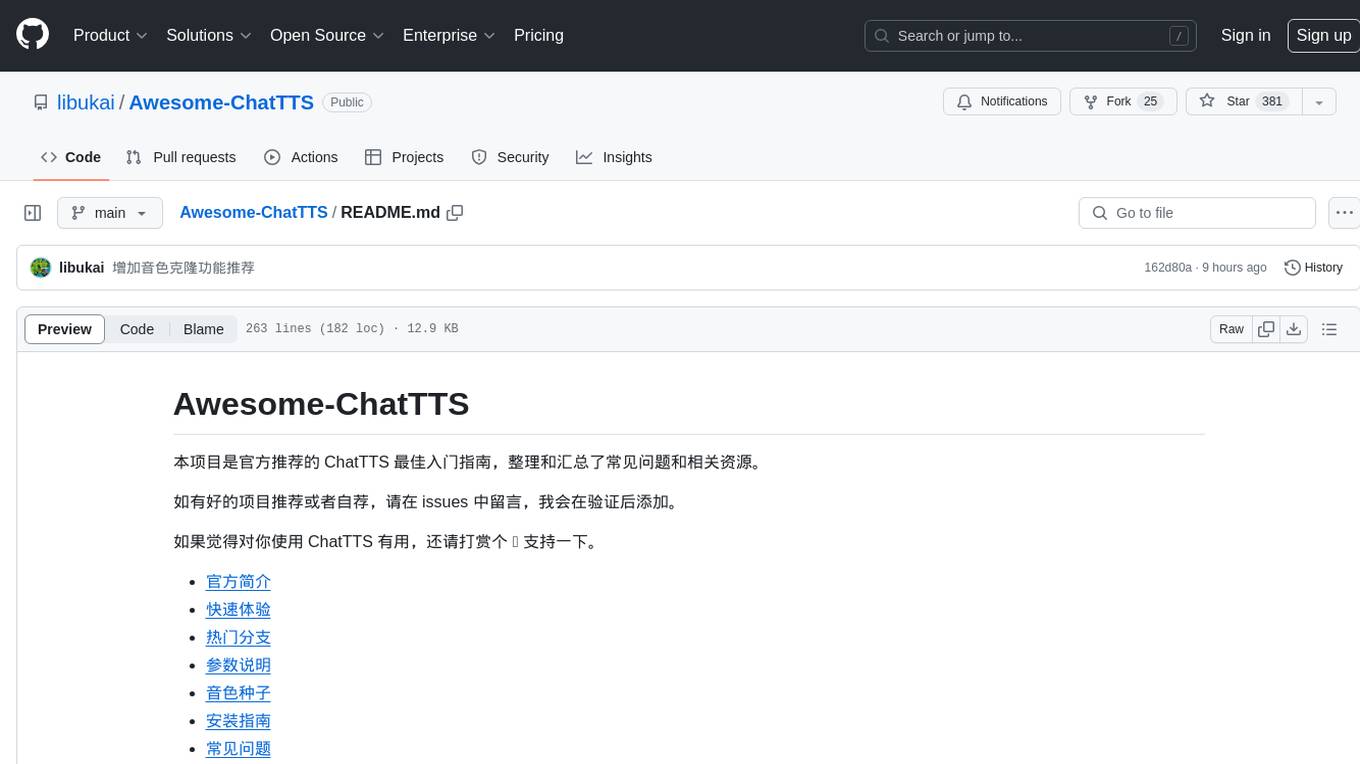
Awesome-ChatTTS
Awesome-ChatTTS is an official recommended guide for ChatTTS beginners, compiling common questions and related resources. It provides a comprehensive overview of the project, including official introduction, quick experience options, popular branches, parameter explanations, voice seed details, installation guides, FAQs, and error troubleshooting. The repository also includes video tutorials, discussion community links, and project trends analysis. Users can explore various branches for different functionalities and enhancements related to ChatTTS.
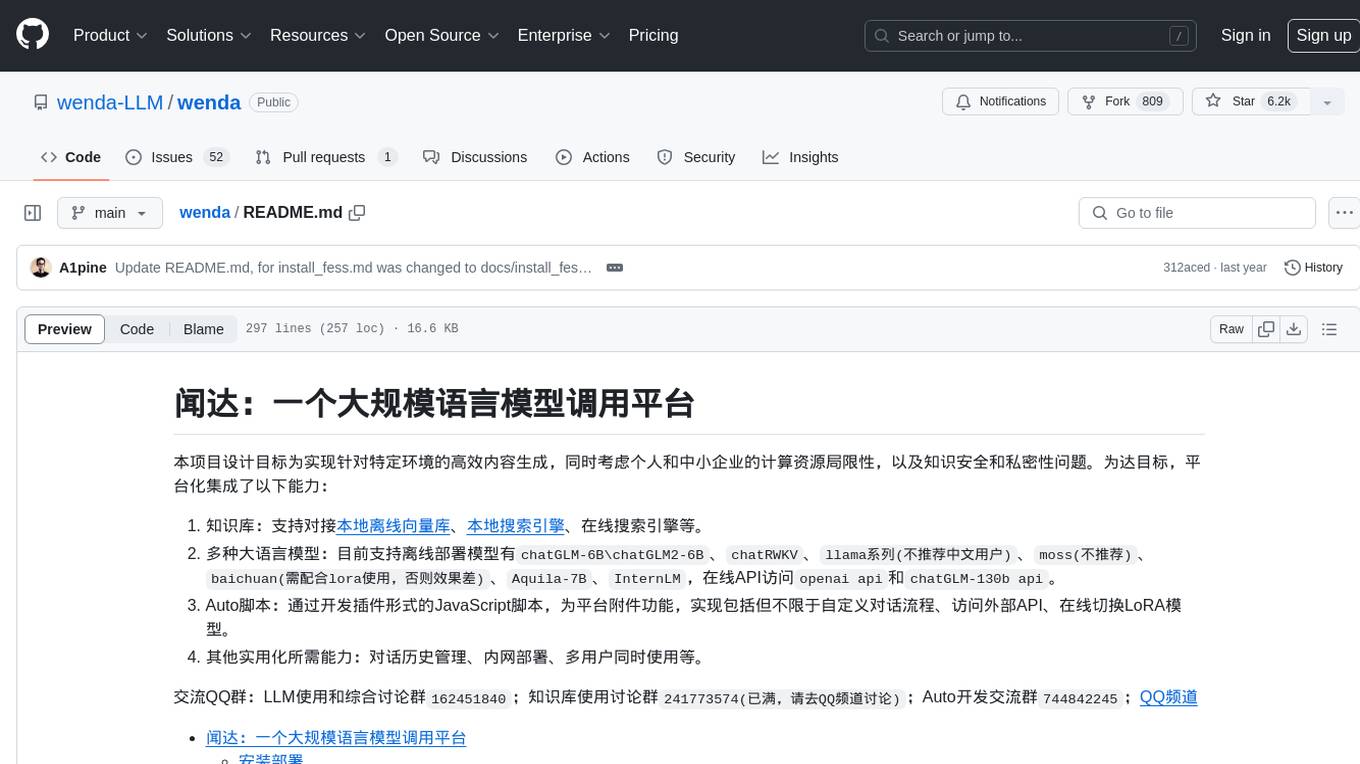
wenda
Wenda is a platform for large-scale language model invocation designed to efficiently generate content for specific environments, considering the limitations of personal and small business computing resources, as well as knowledge security and privacy issues. The platform integrates capabilities such as knowledge base integration, multiple large language models for offline deployment, auto scripts for additional functionality, and other practical capabilities like conversation history management and multi-user simultaneous usage.
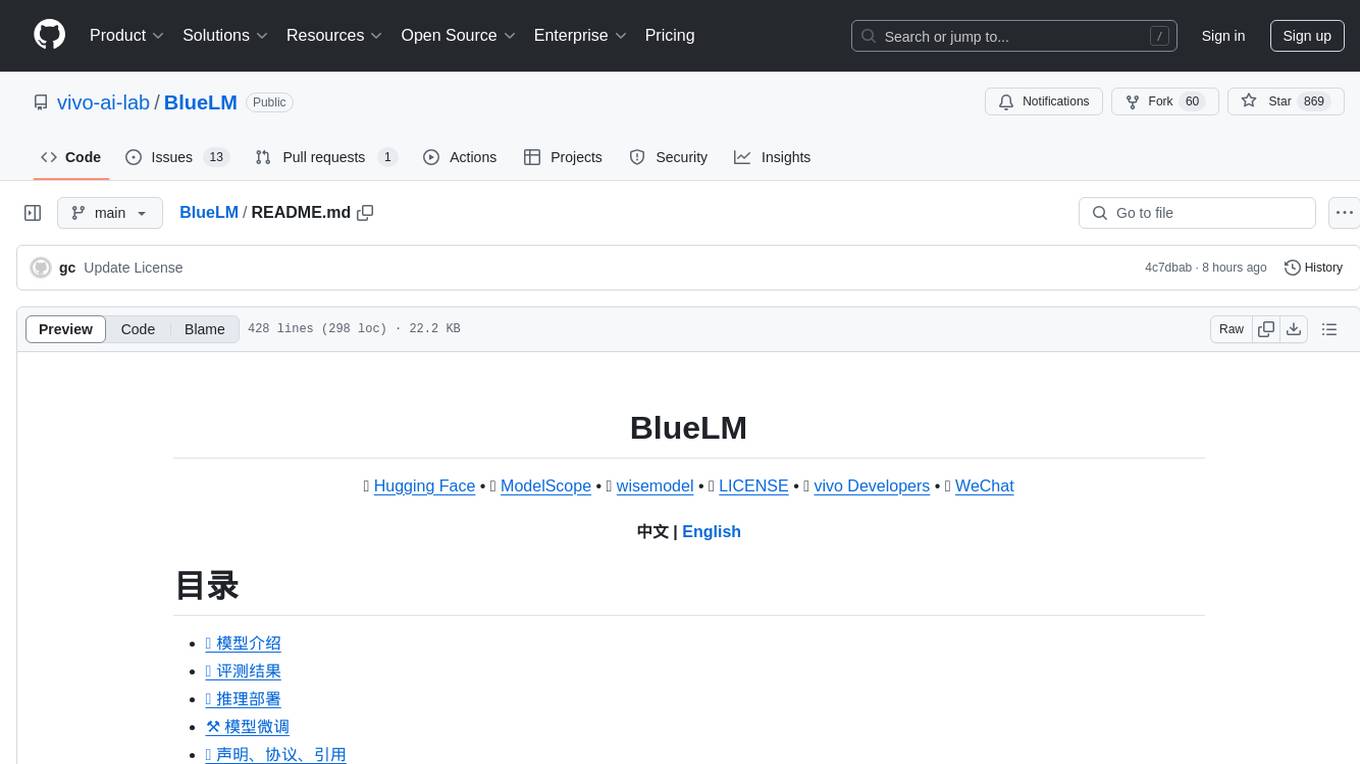
BlueLM
BlueLM is a large-scale pre-trained language model developed by vivo AI Global Research Institute, featuring 7B base and chat models. It includes high-quality training data with a token scale of 26 trillion, supporting both Chinese and English languages. BlueLM-7B-Chat excels in C-Eval and CMMLU evaluations, providing strong competition among open-source models of similar size. The models support 32K long texts for better context understanding while maintaining base capabilities. BlueLM welcomes developers for academic research and commercial applications.
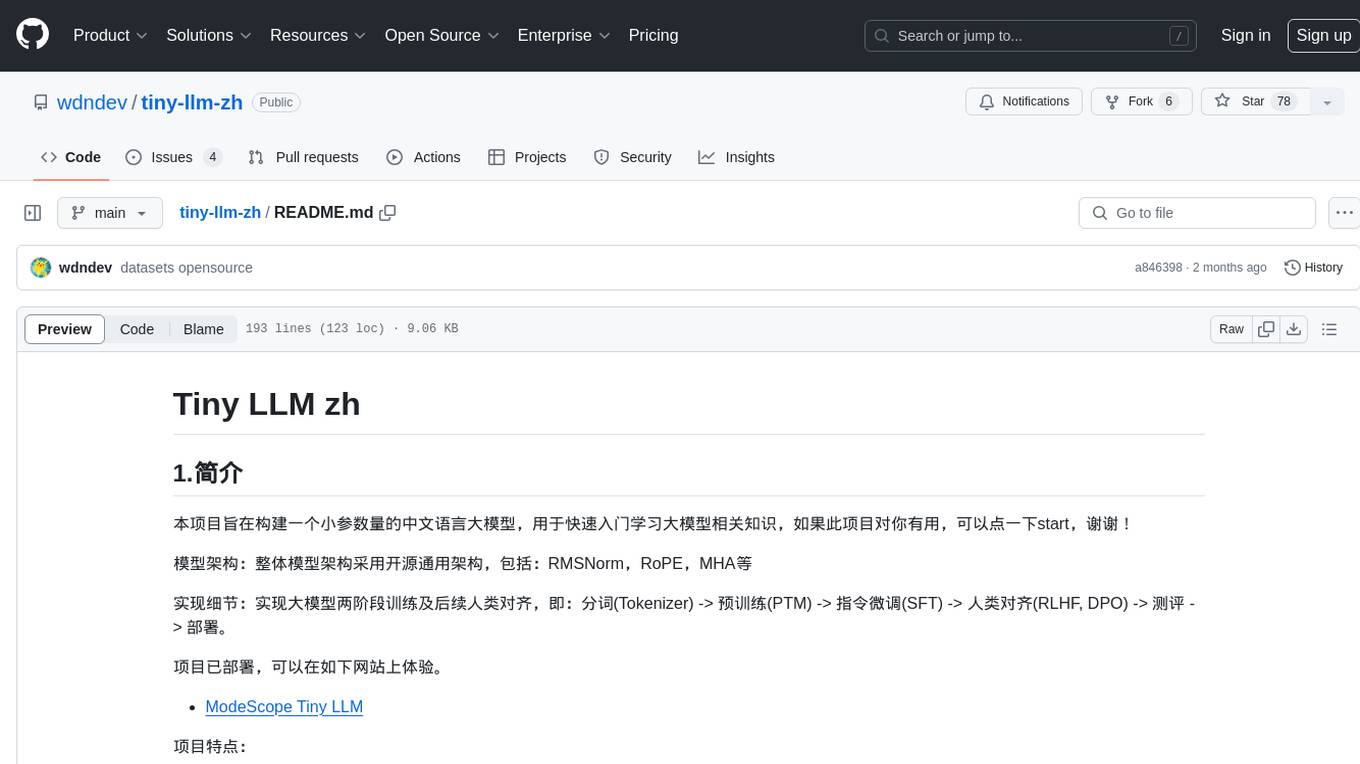
tiny-llm-zh
Tiny LLM zh is a project aimed at building a small-parameter Chinese language large model for quick entry into learning large model-related knowledge. The project implements a two-stage training process for large models and subsequent human alignment, including tokenization, pre-training, instruction fine-tuning, human alignment, evaluation, and deployment. It is deployed on ModeScope Tiny LLM website and features open access to all data and code, including pre-training data and tokenizer. The project trains a tokenizer using 10GB of Chinese encyclopedia text to build a Tiny LLM vocabulary. It supports training with Transformers deepspeed, multiple machine and card support, and Zero optimization techniques. The project has three main branches: llama2_torch, main tiny_llm, and tiny_llm_moe, each with specific modifications and features.
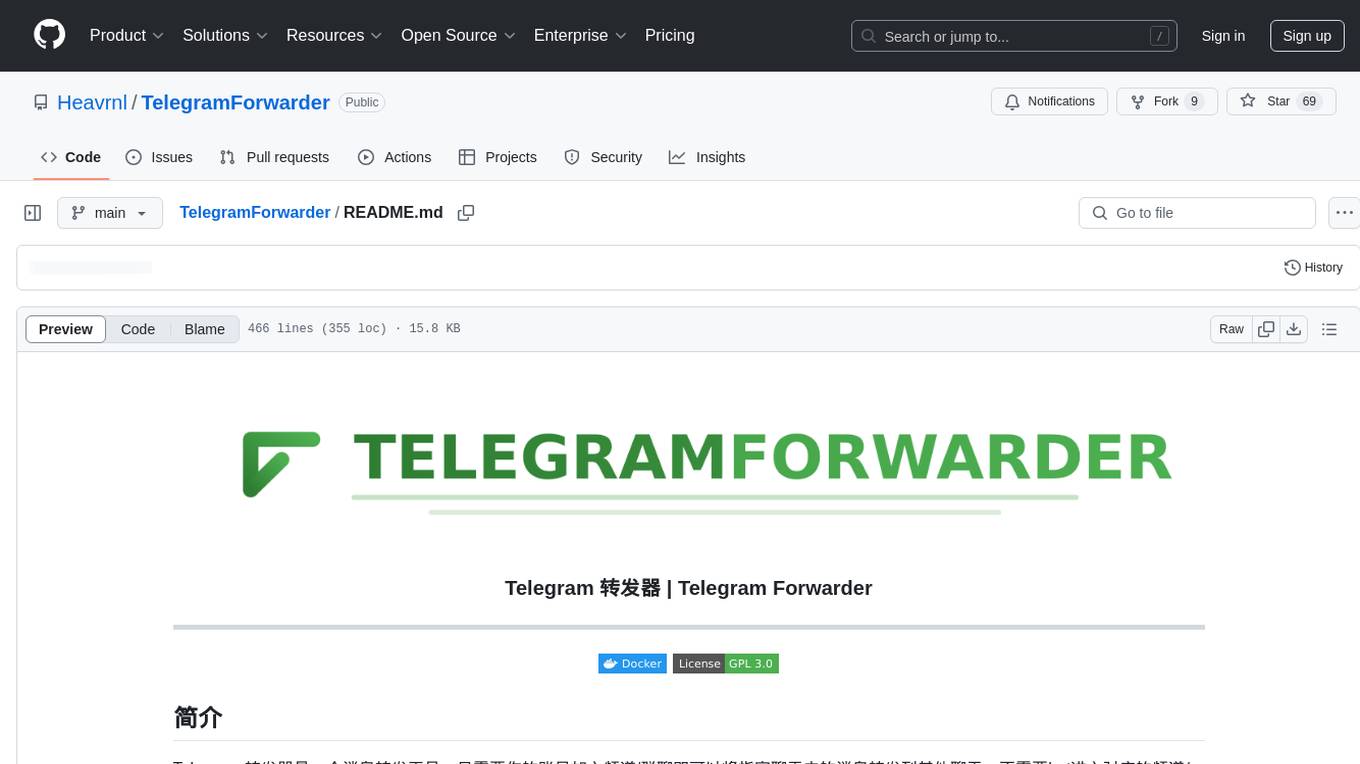
TelegramForwarder
Telegram Forwarder is a message forwarding tool that allows you to forward messages from specified chats to other chats without the need for a bot to enter the corresponding channels/groups to listen. It can be used for information stream integration filtering, message reminders, content archiving, and more. The tool supports multiple sources forwarding, keyword filtering in whitelist and blacklist modes, regular expression matching, message content modification, AI processing using major vendors' AI interfaces, media file filtering, and synchronization with a universal forum blocking plugin to achieve three-end blocking.
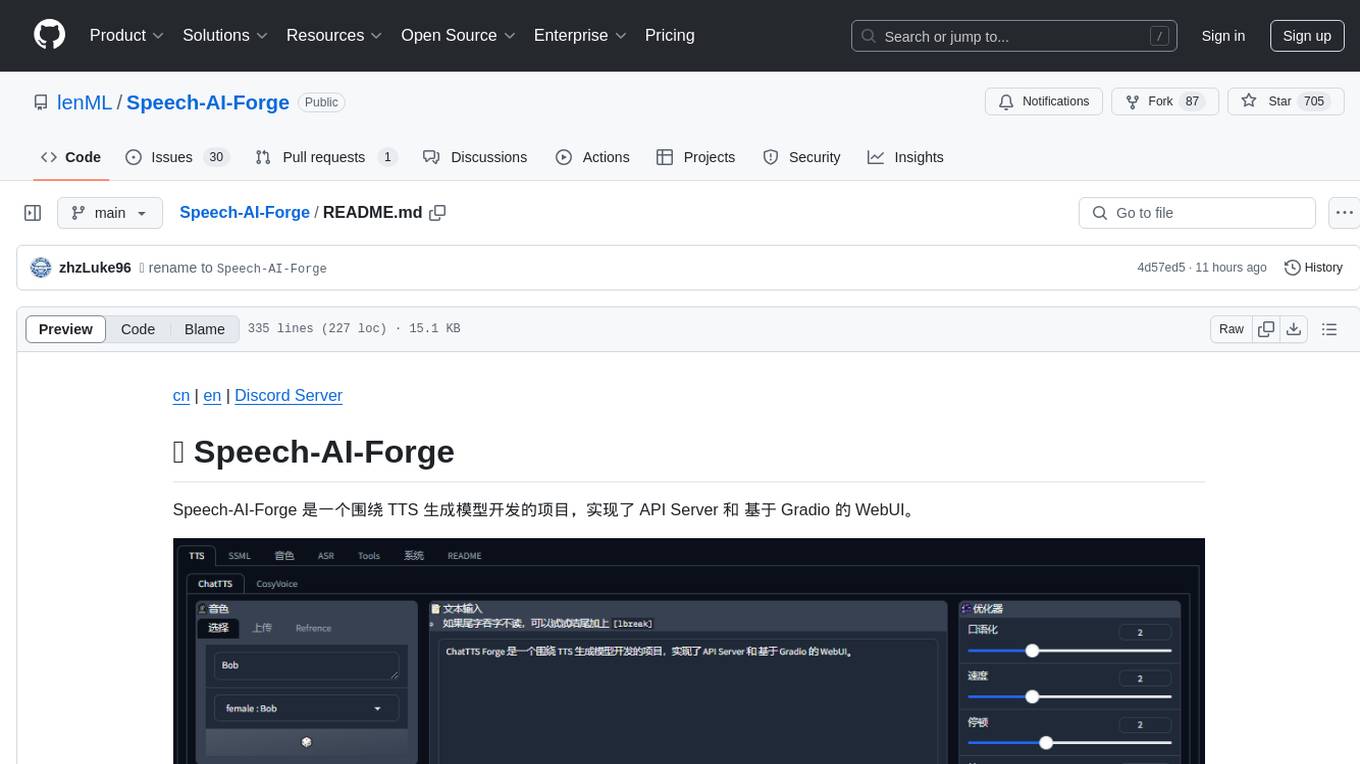
Speech-AI-Forge
Speech-AI-Forge is a project developed around TTS generation models, implementing an API Server and a WebUI based on Gradio. The project offers various ways to experience and deploy Speech-AI-Forge, including online experience on HuggingFace Spaces, one-click launch on Colab, container deployment with Docker, and local deployment. The WebUI features include TTS model functionality, speaker switch for changing voices, style control, long text support with automatic text segmentation, refiner for ChatTTS native text refinement, various tools for voice control and enhancement, support for multiple TTS models, SSML synthesis control, podcast creation tools, voice creation, voice testing, ASR tools, and post-processing tools. The API Server can be launched separately for higher API throughput. The project roadmap includes support for various TTS models, ASR models, voice clone models, and enhancer models. Model downloads can be manually initiated using provided scripts. The project aims to provide inference services and may include training-related functionalities in the future.
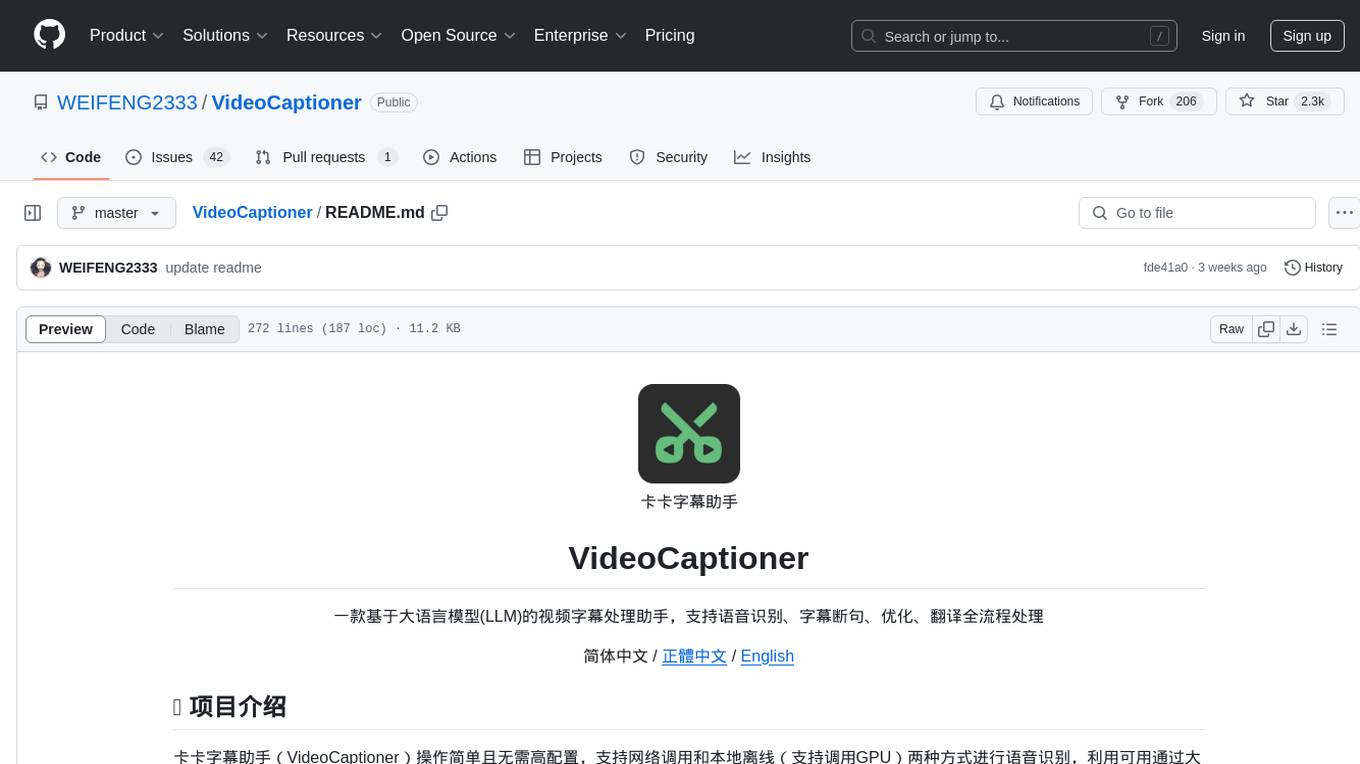
VideoCaptioner
VideoCaptioner is a video subtitle processing assistant based on a large language model (LLM), supporting speech recognition, subtitle segmentation, optimization, translation, and full-process handling. It is user-friendly and does not require high configuration, supporting both network calls and local offline (GPU-enabled) speech recognition. It utilizes a large language model for intelligent subtitle segmentation, correction, and translation, providing stunning subtitles for videos. The tool offers features such as accurate subtitle generation without GPU, intelligent segmentation and sentence splitting based on LLM, AI subtitle optimization and translation, batch video subtitle synthesis, intuitive subtitle editing interface with real-time preview and quick editing, and low model token consumption with built-in basic LLM model for easy use.
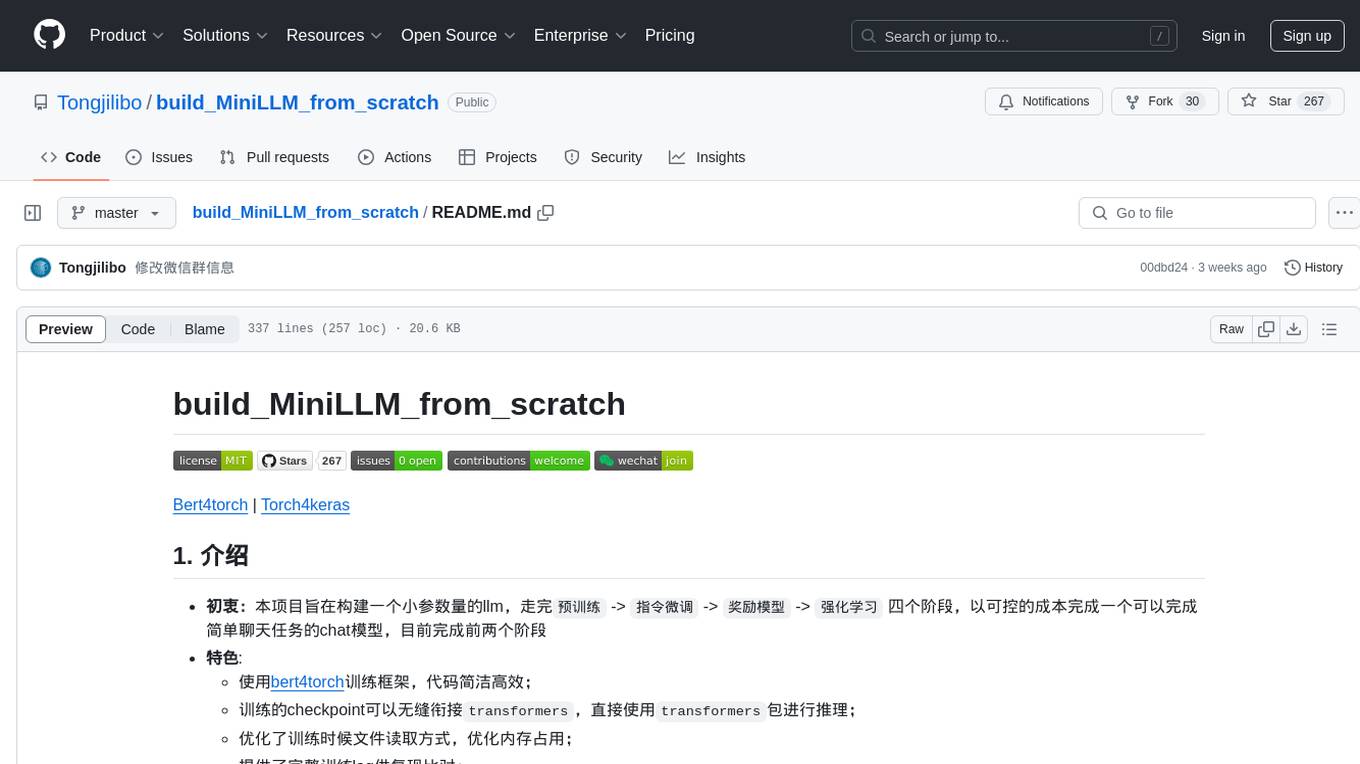
build_MiniLLM_from_scratch
This repository aims to build a low-parameter LLM model through pretraining, fine-tuning, model rewarding, and reinforcement learning stages to create a chat model capable of simple conversation tasks. It features using the bert4torch training framework, seamless integration with transformers package for inference, optimized file reading during training to reduce memory usage, providing complete training logs for reproducibility, and the ability to customize robot attributes. The chat model supports multi-turn conversations. The trained model currently only supports basic chat functionality due to limitations in corpus size, model scale, SFT corpus size, and quality.
For similar tasks
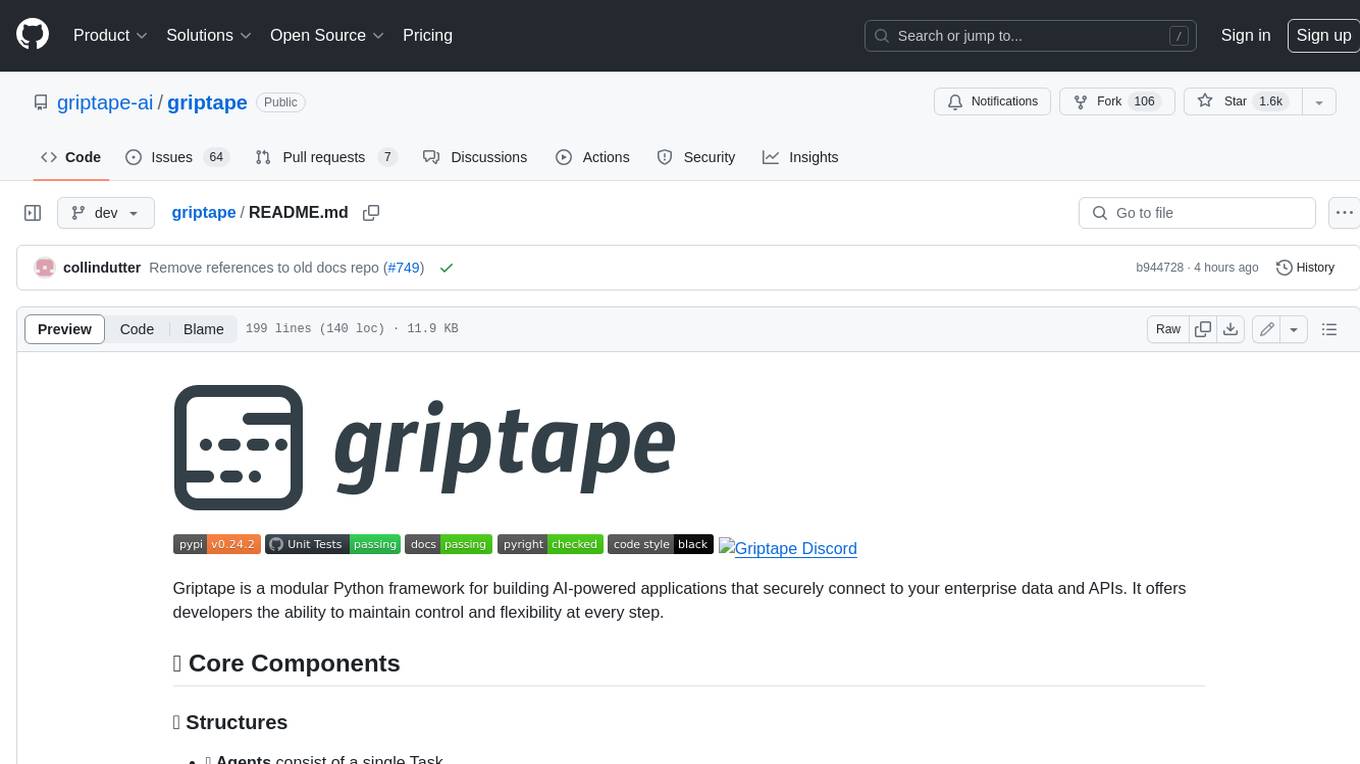
griptape
Griptape is a modular Python framework for building AI-powered applications that securely connect to your enterprise data and APIs. It offers developers the ability to maintain control and flexibility at every step. Griptape's core components include Structures (Agents, Pipelines, and Workflows), Tasks, Tools, Memory (Conversation Memory, Task Memory, and Meta Memory), Drivers (Prompt and Embedding Drivers, Vector Store Drivers, Image Generation Drivers, Image Query Drivers, SQL Drivers, Web Scraper Drivers, and Conversation Memory Drivers), Engines (Query Engines, Extraction Engines, Summary Engines, Image Generation Engines, and Image Query Engines), and additional components (Rulesets, Loaders, Artifacts, Chunkers, and Tokenizers). Griptape enables developers to create AI-powered applications with ease and efficiency.
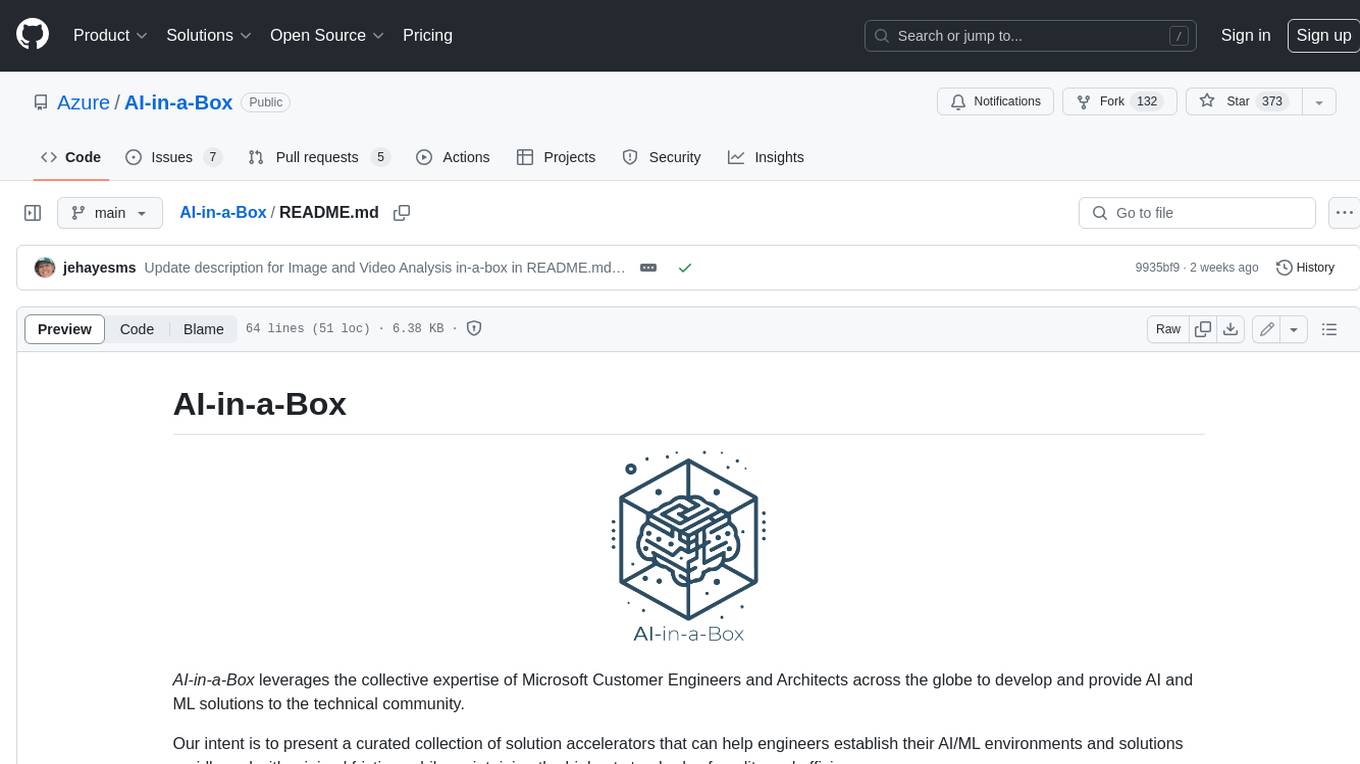
AI-in-a-Box
AI-in-a-Box is a curated collection of solution accelerators that can help engineers establish their AI/ML environments and solutions rapidly and with minimal friction, while maintaining the highest standards of quality and efficiency. It provides essential guidance on the responsible use of AI and LLM technologies, specific security guidance for Generative AI (GenAI) applications, and best practices for scaling OpenAI applications within Azure. The available accelerators include: Azure ML Operationalization in-a-box, Edge AI in-a-box, Doc Intelligence in-a-box, Image and Video Analysis in-a-box, Cognitive Services Landing Zone in-a-box, Semantic Kernel Bot in-a-box, NLP to SQL in-a-box, Assistants API in-a-box, and Assistants API Bot in-a-box.
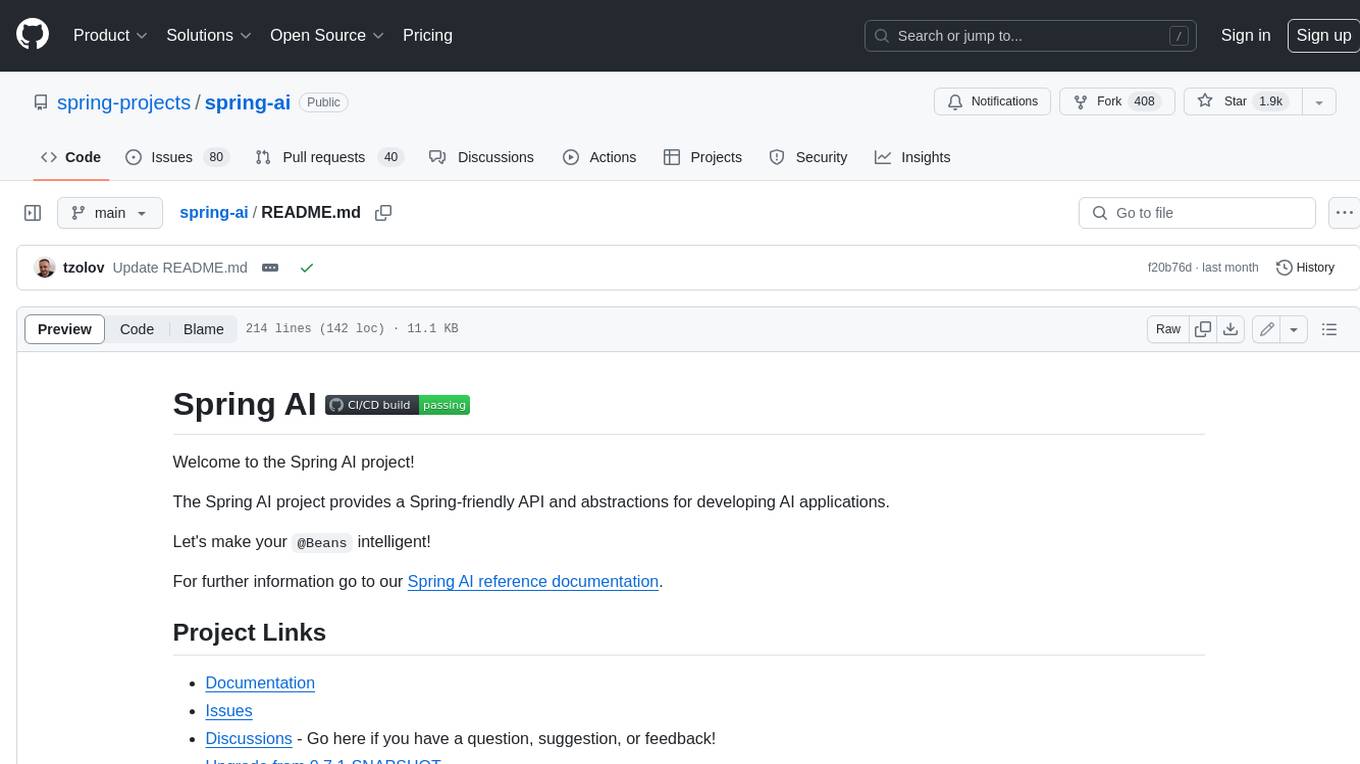
spring-ai
The Spring AI project provides a Spring-friendly API and abstractions for developing AI applications. It offers a portable client API for interacting with generative AI models, enabling developers to easily swap out implementations and access various models like OpenAI, Azure OpenAI, and HuggingFace. Spring AI also supports prompt engineering, providing classes and interfaces for creating and parsing prompts, as well as incorporating proprietary data into generative AI without retraining the model. This is achieved through Retrieval Augmented Generation (RAG), which involves extracting, transforming, and loading data into a vector database for use by AI models. Spring AI's VectorStore abstraction allows for seamless transitions between different vector database implementations.
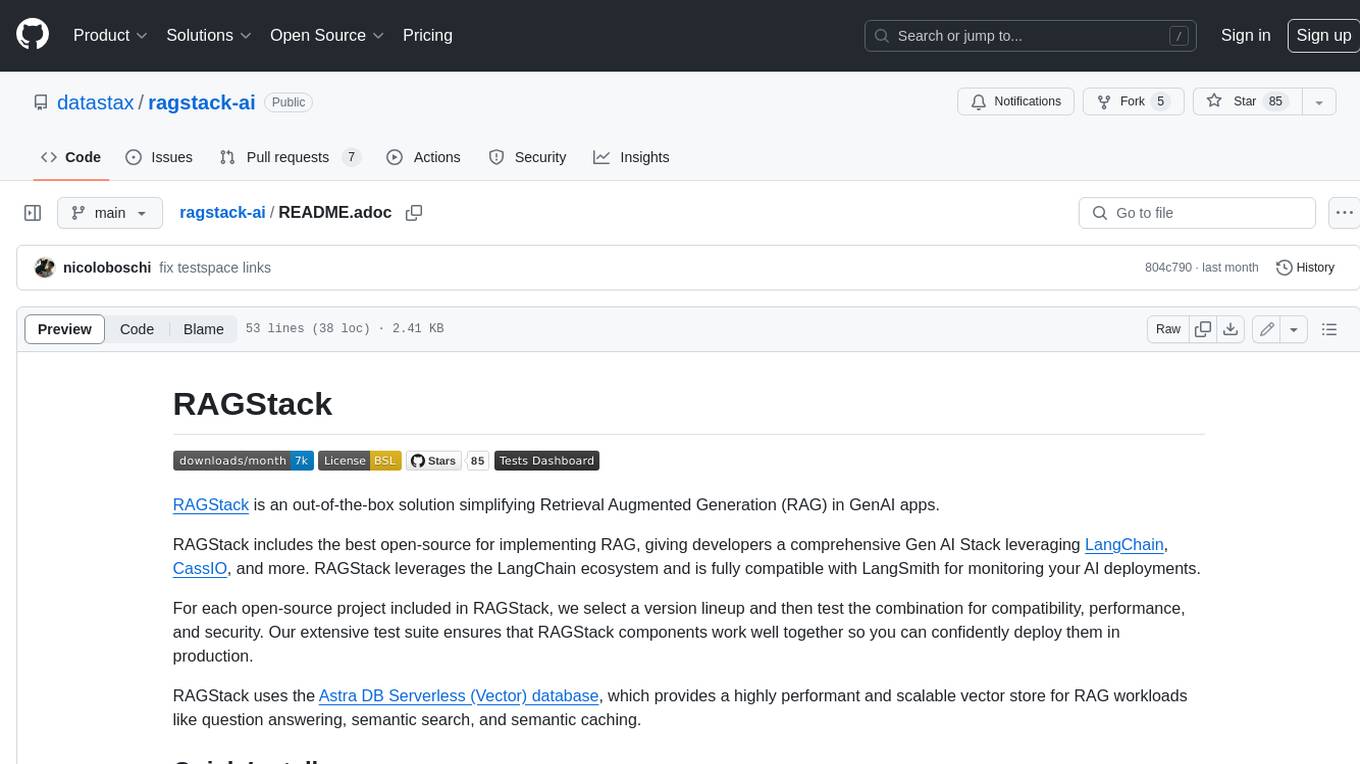
ragstack-ai
RAGStack is an out-of-the-box solution simplifying Retrieval Augmented Generation (RAG) in GenAI apps. RAGStack includes the best open-source for implementing RAG, giving developers a comprehensive Gen AI Stack leveraging LangChain, CassIO, and more. RAGStack leverages the LangChain ecosystem and is fully compatible with LangSmith for monitoring your AI deployments.
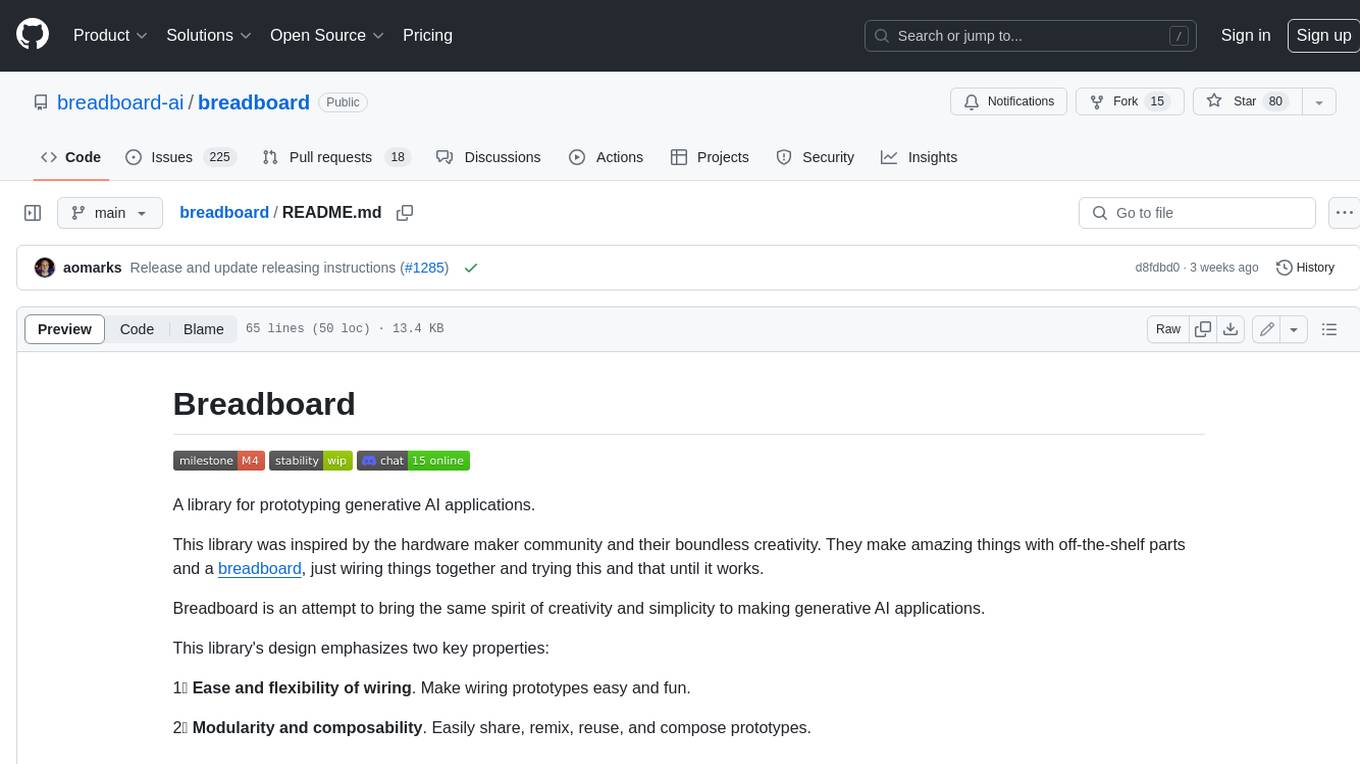
breadboard
Breadboard is a library for prototyping generative AI applications. It is inspired by the hardware maker community and their boundless creativity. Breadboard makes it easy to wire prototypes and share, remix, reuse, and compose them. The library emphasizes ease and flexibility of wiring, as well as modularity and composability.
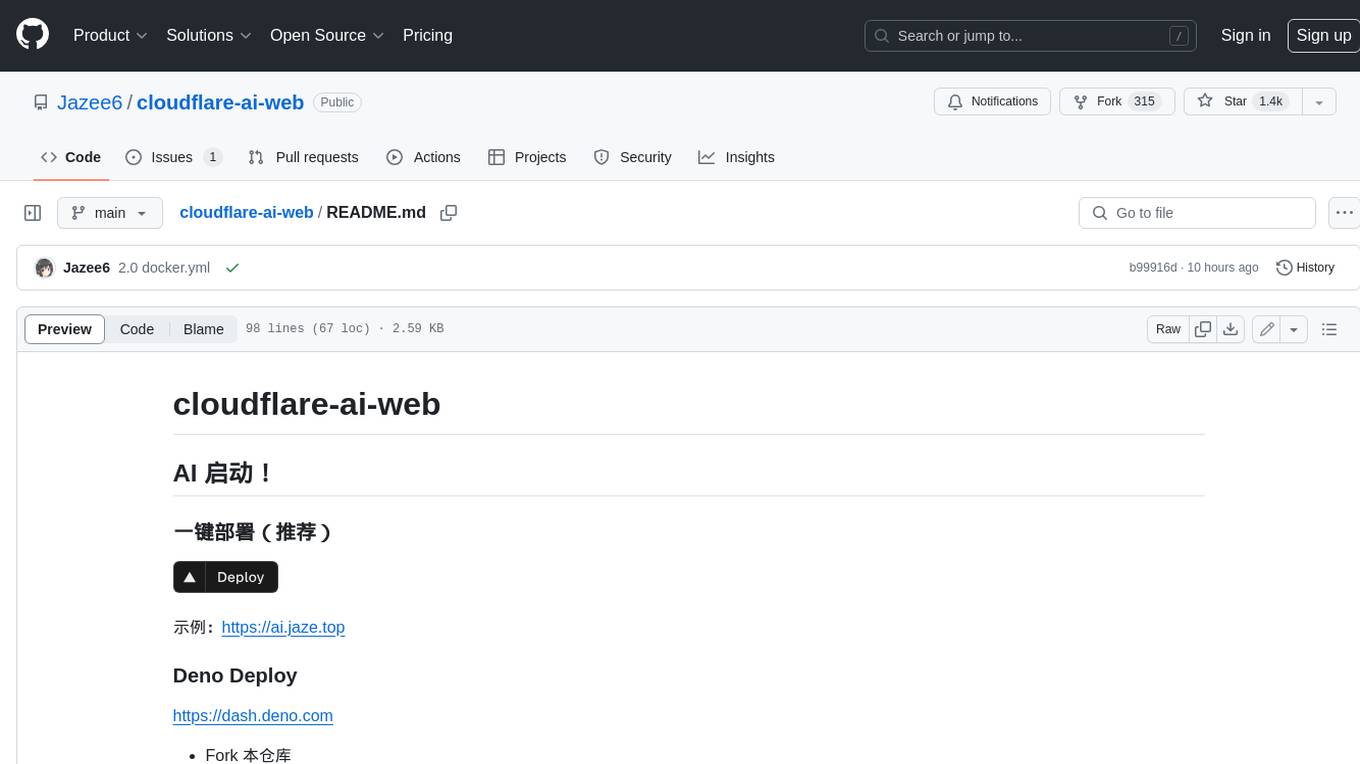
cloudflare-ai-web
Cloudflare-ai-web is a lightweight and easy-to-use tool that allows you to quickly deploy a multi-modal AI platform using Cloudflare Workers AI. It supports serverless deployment, password protection, and local storage of chat logs. With a size of only ~638 kB gzip, it is a great option for building AI-powered applications without the need for a dedicated server.
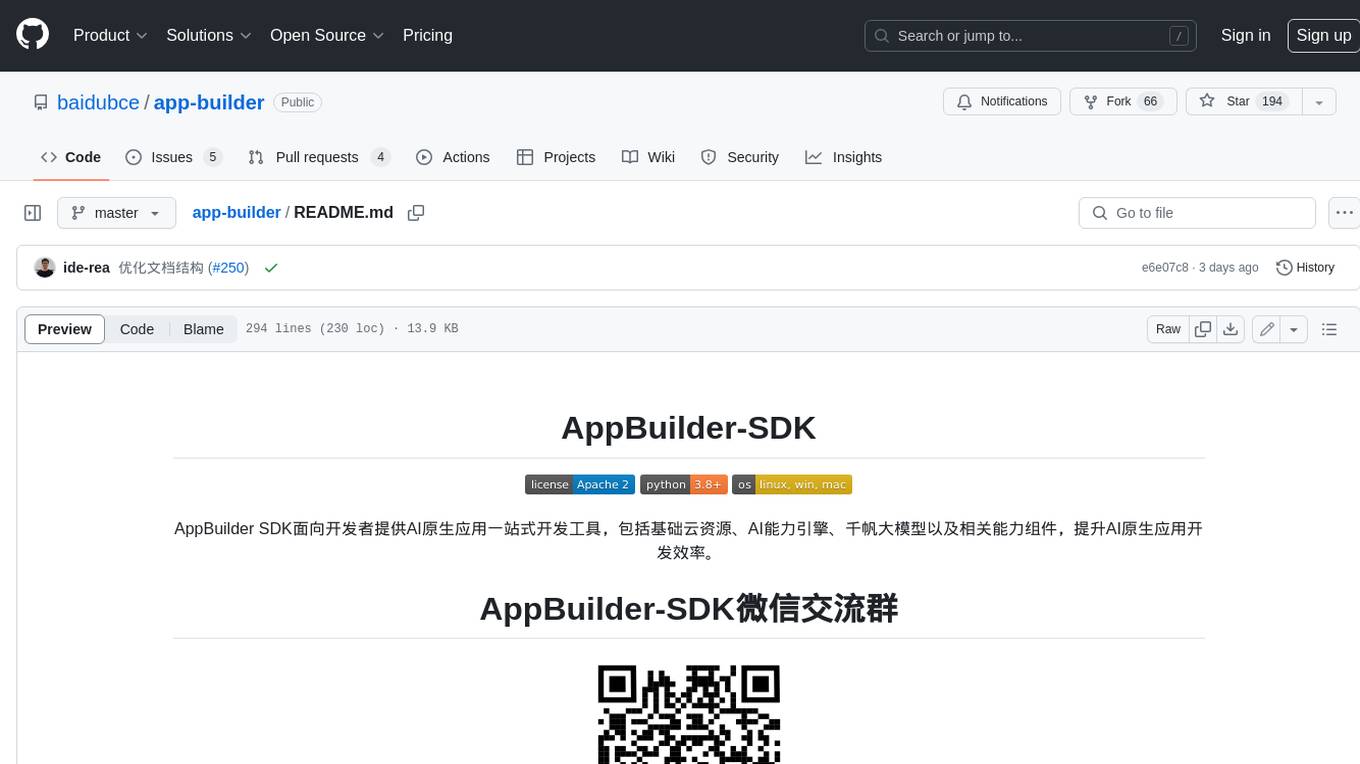
app-builder
AppBuilder SDK is a one-stop development tool for AI native applications, providing basic cloud resources, AI capability engine, Qianfan large model, and related capability components to improve the development efficiency of AI native applications.
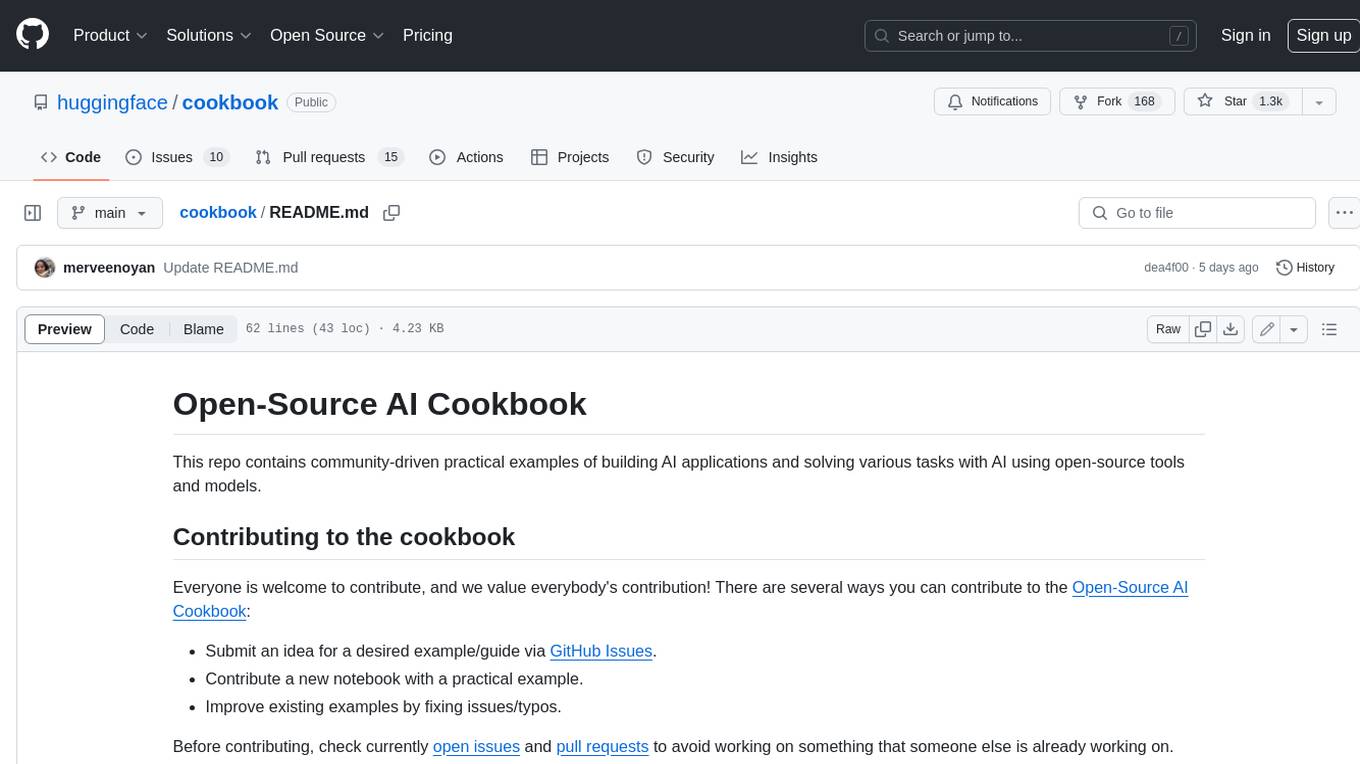
cookbook
This repository contains community-driven practical examples of building AI applications and solving various tasks with AI using open-source tools and models. Everyone is welcome to contribute, and we value everybody's contribution! There are several ways you can contribute to the Open-Source AI Cookbook: Submit an idea for a desired example/guide via GitHub Issues. Contribute a new notebook with a practical example. Improve existing examples by fixing issues/typos. Before contributing, check currently open issues and pull requests to avoid working on something that someone else is already working on.
For similar jobs
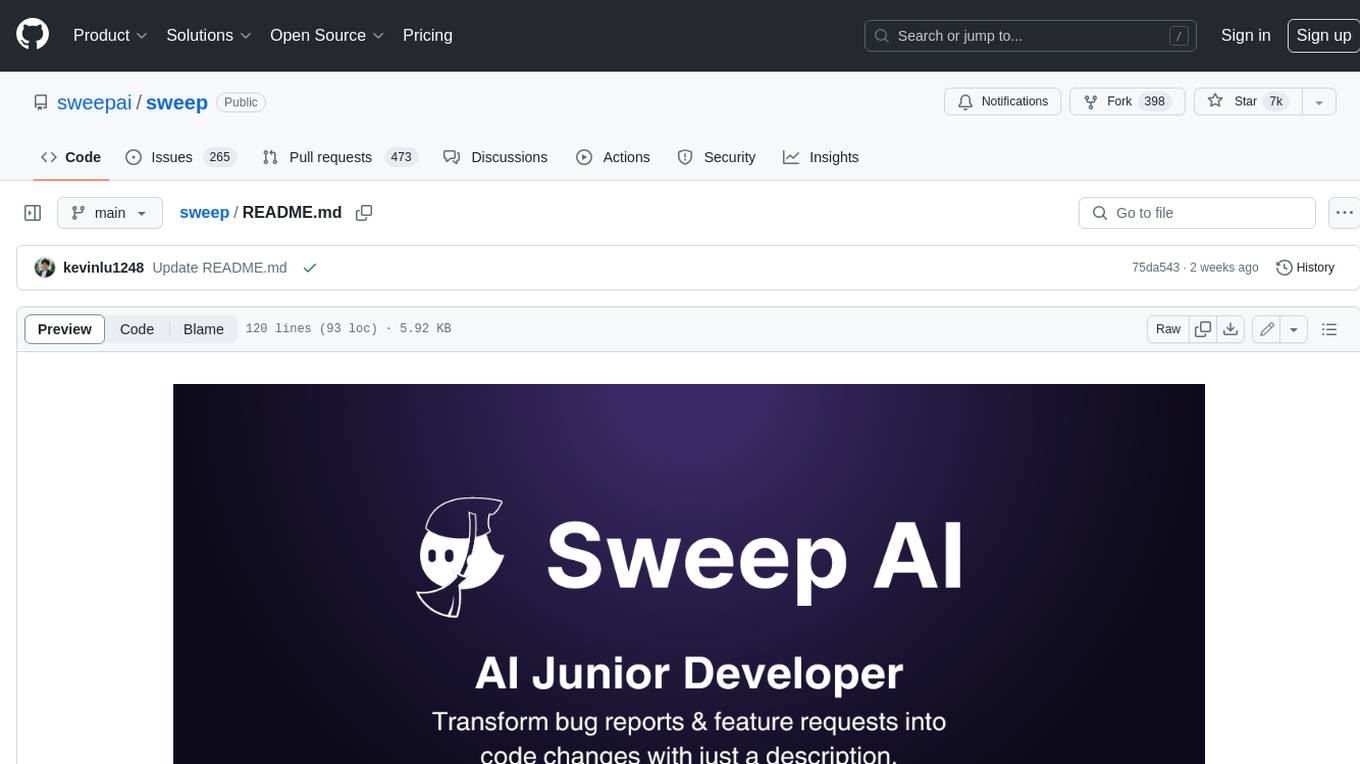
sweep
Sweep is an AI junior developer that turns bugs and feature requests into code changes. It automatically handles developer experience improvements like adding type hints and improving test coverage.
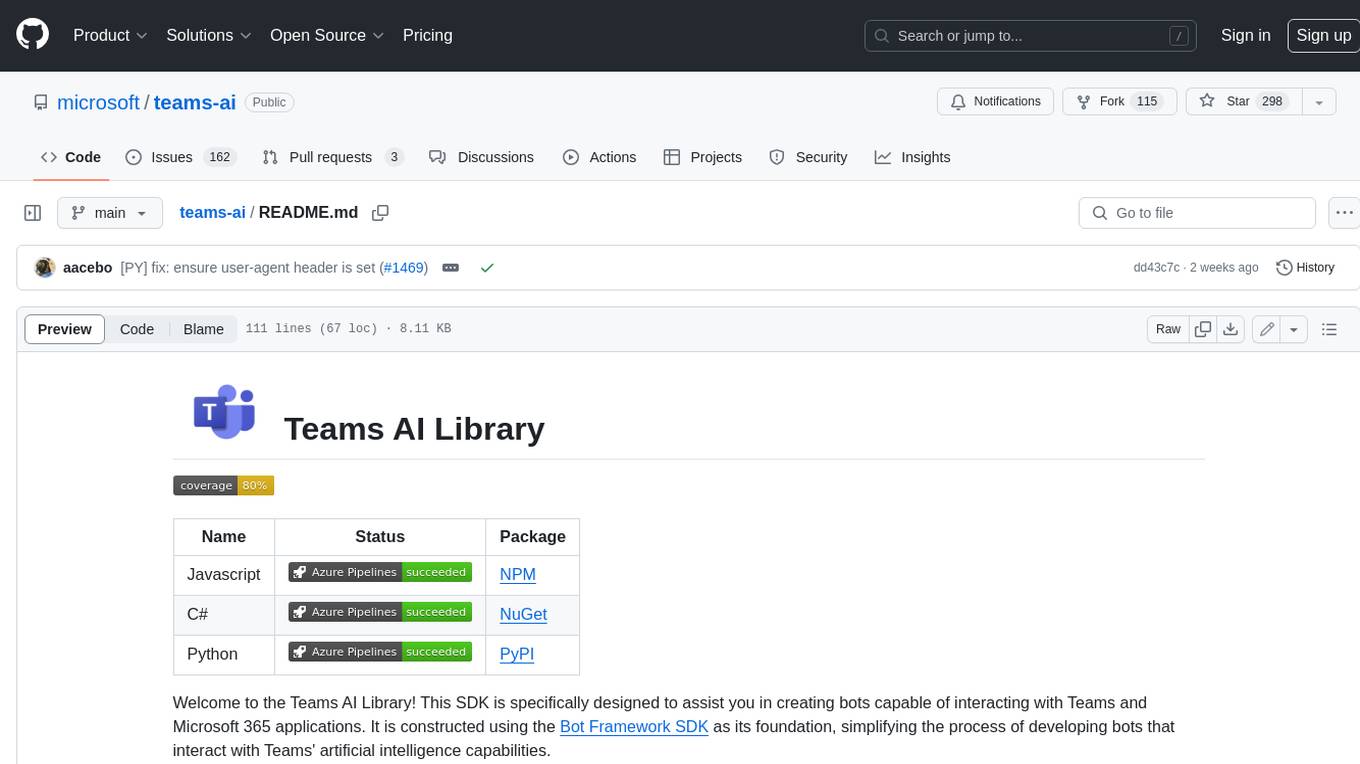
teams-ai
The Teams AI Library is a software development kit (SDK) that helps developers create bots that can interact with Teams and Microsoft 365 applications. It is built on top of the Bot Framework SDK and simplifies the process of developing bots that interact with Teams' artificial intelligence capabilities. The SDK is available for JavaScript/TypeScript, .NET, and Python.
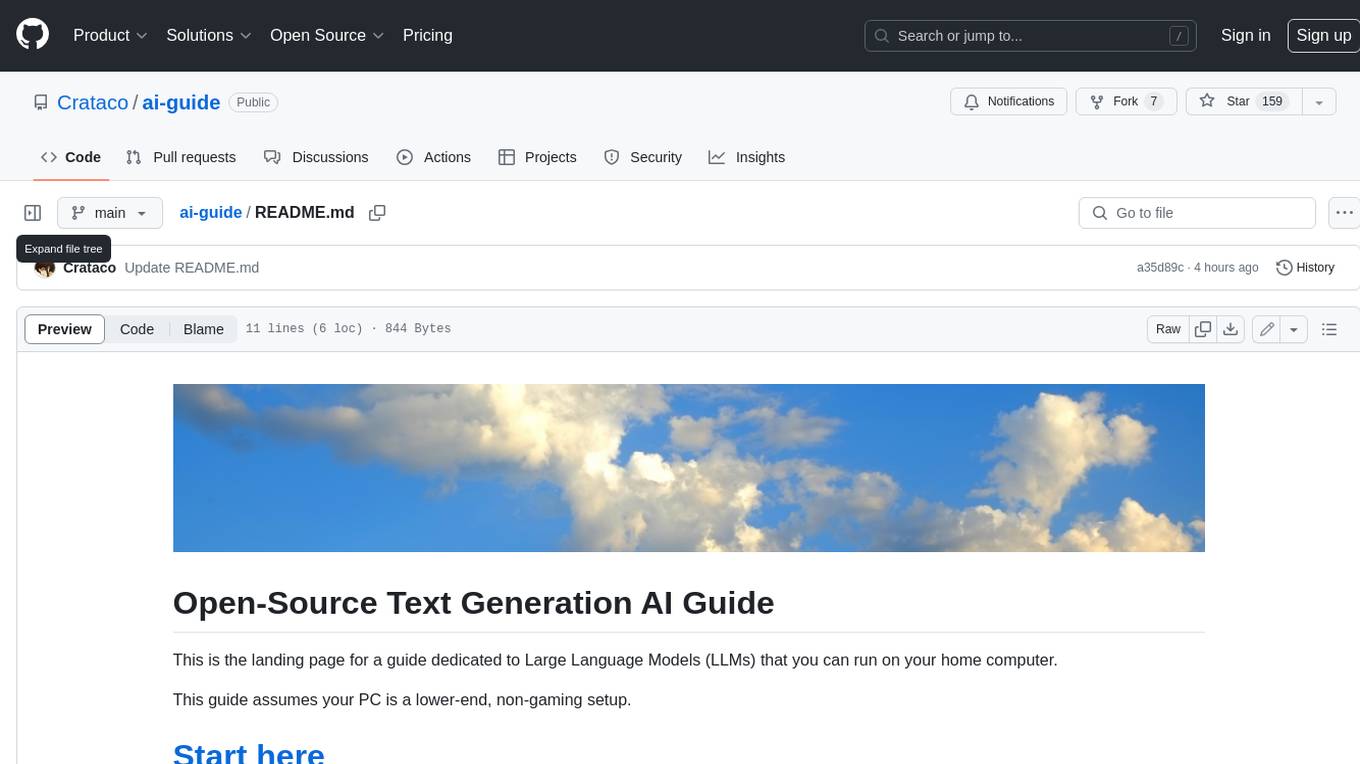
ai-guide
This guide is dedicated to Large Language Models (LLMs) that you can run on your home computer. It assumes your PC is a lower-end, non-gaming setup.
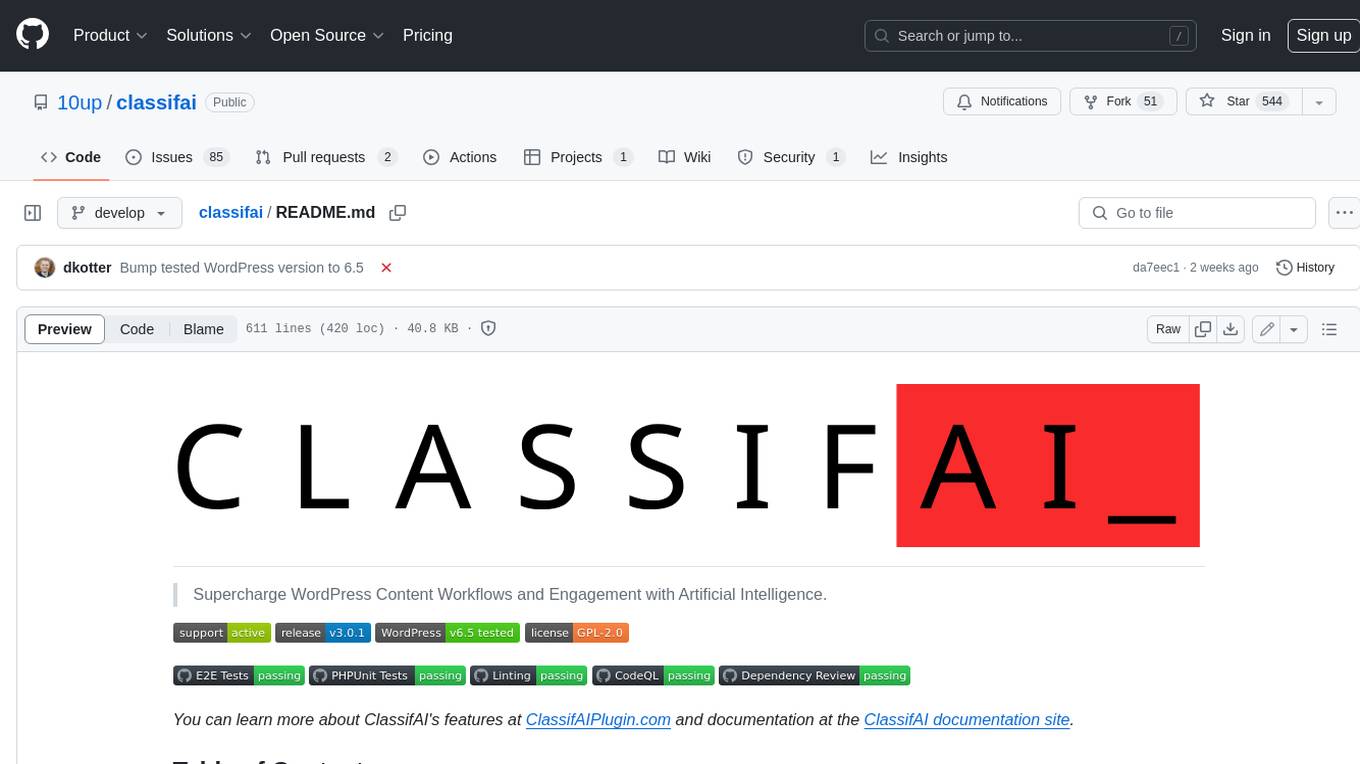
classifai
Supercharge WordPress Content Workflows and Engagement with Artificial Intelligence. Tap into leading cloud-based services like OpenAI, Microsoft Azure AI, Google Gemini and IBM Watson to augment your WordPress-powered websites. Publish content faster while improving SEO performance and increasing audience engagement. ClassifAI integrates Artificial Intelligence and Machine Learning technologies to lighten your workload and eliminate tedious tasks, giving you more time to create original content that matters.
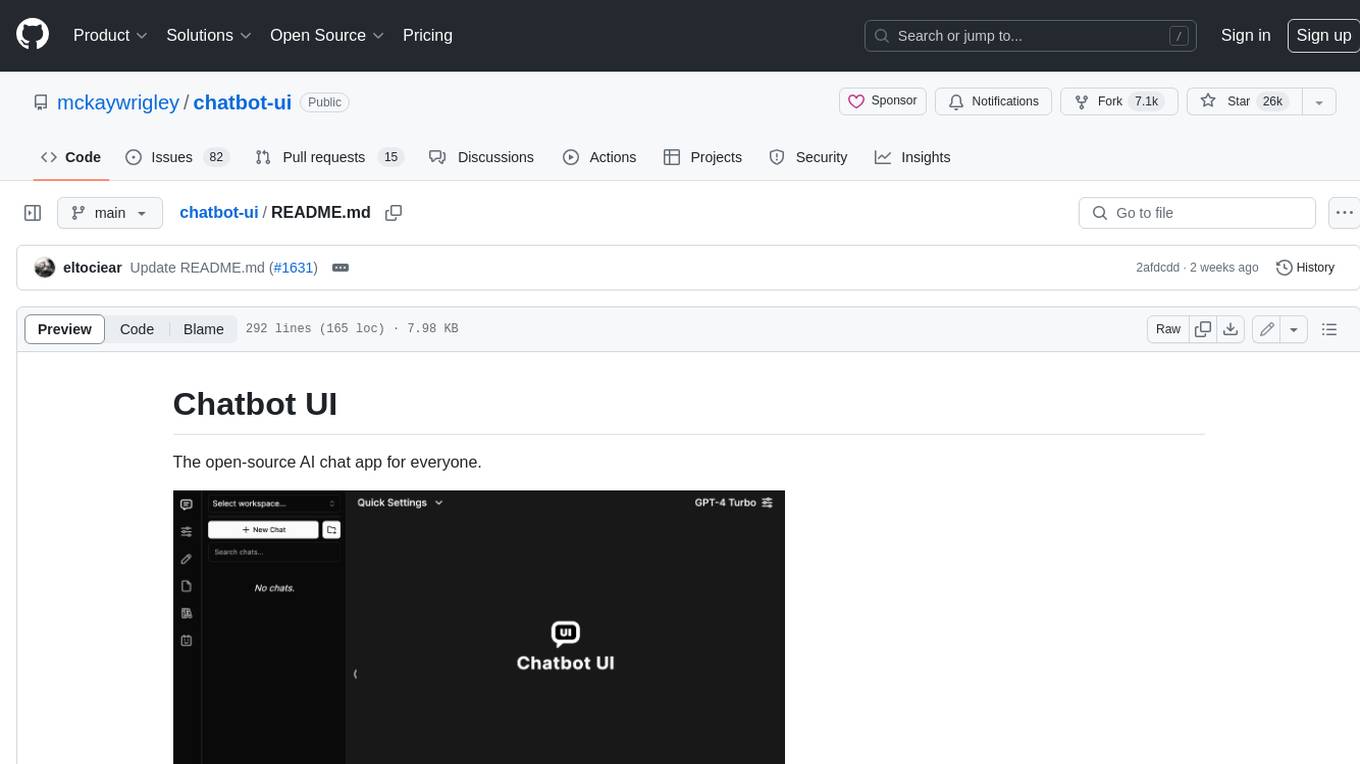
chatbot-ui
Chatbot UI is an open-source AI chat app that allows users to create and deploy their own AI chatbots. It is easy to use and can be customized to fit any need. Chatbot UI is perfect for businesses, developers, and anyone who wants to create a chatbot.
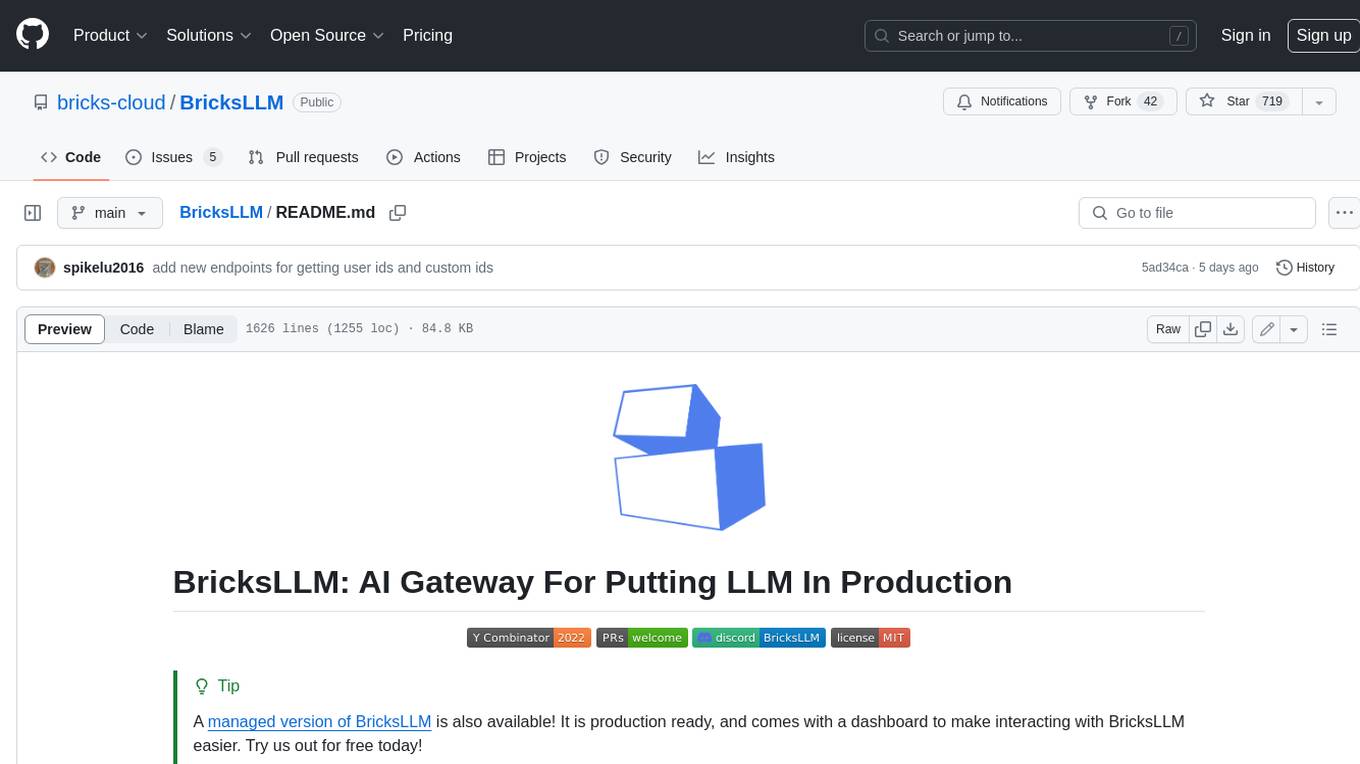
BricksLLM
BricksLLM is a cloud native AI gateway written in Go. Currently, it provides native support for OpenAI, Anthropic, Azure OpenAI and vLLM. BricksLLM aims to provide enterprise level infrastructure that can power any LLM production use cases. Here are some use cases for BricksLLM: * Set LLM usage limits for users on different pricing tiers * Track LLM usage on a per user and per organization basis * Block or redact requests containing PIIs * Improve LLM reliability with failovers, retries and caching * Distribute API keys with rate limits and cost limits for internal development/production use cases * Distribute API keys with rate limits and cost limits for students
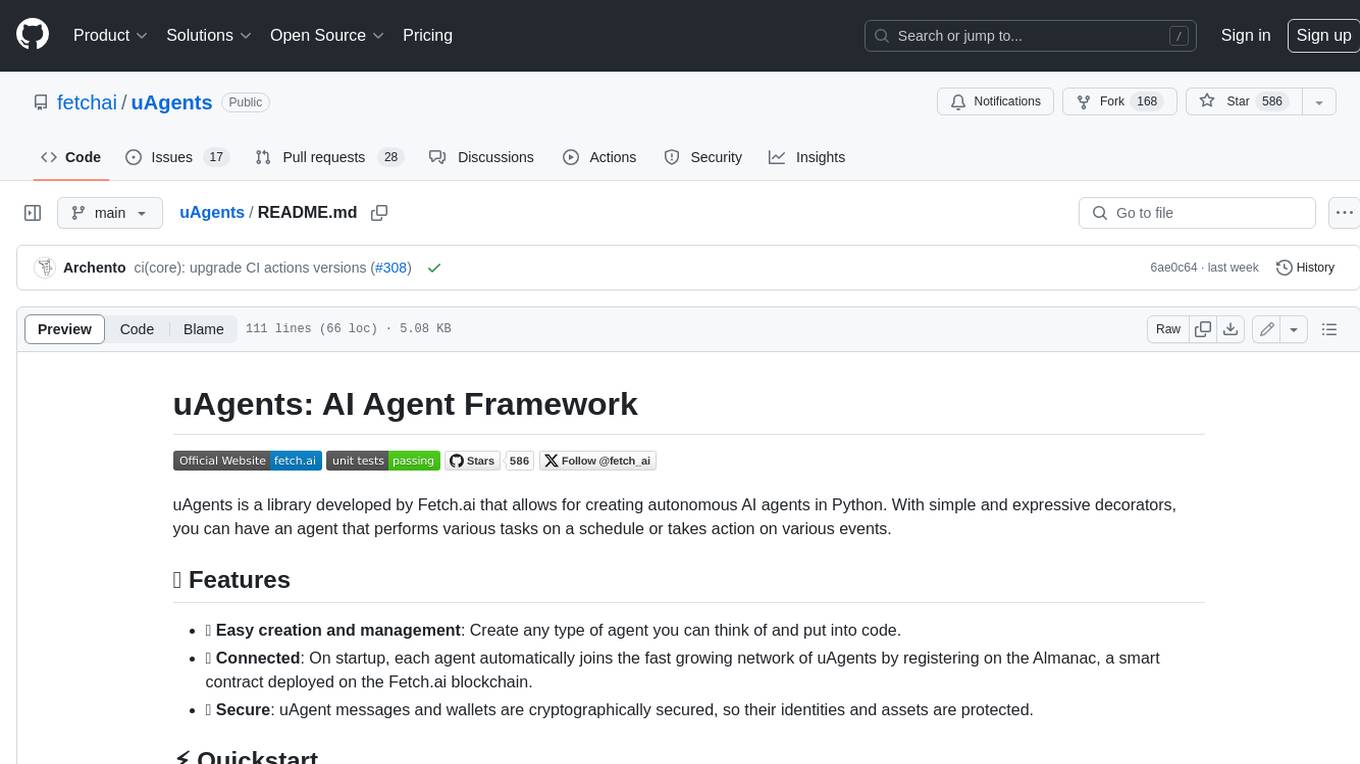
uAgents
uAgents is a Python library developed by Fetch.ai that allows for the creation of autonomous AI agents. These agents can perform various tasks on a schedule or take action on various events. uAgents are easy to create and manage, and they are connected to a fast-growing network of other uAgents. They are also secure, with cryptographically secured messages and wallets.
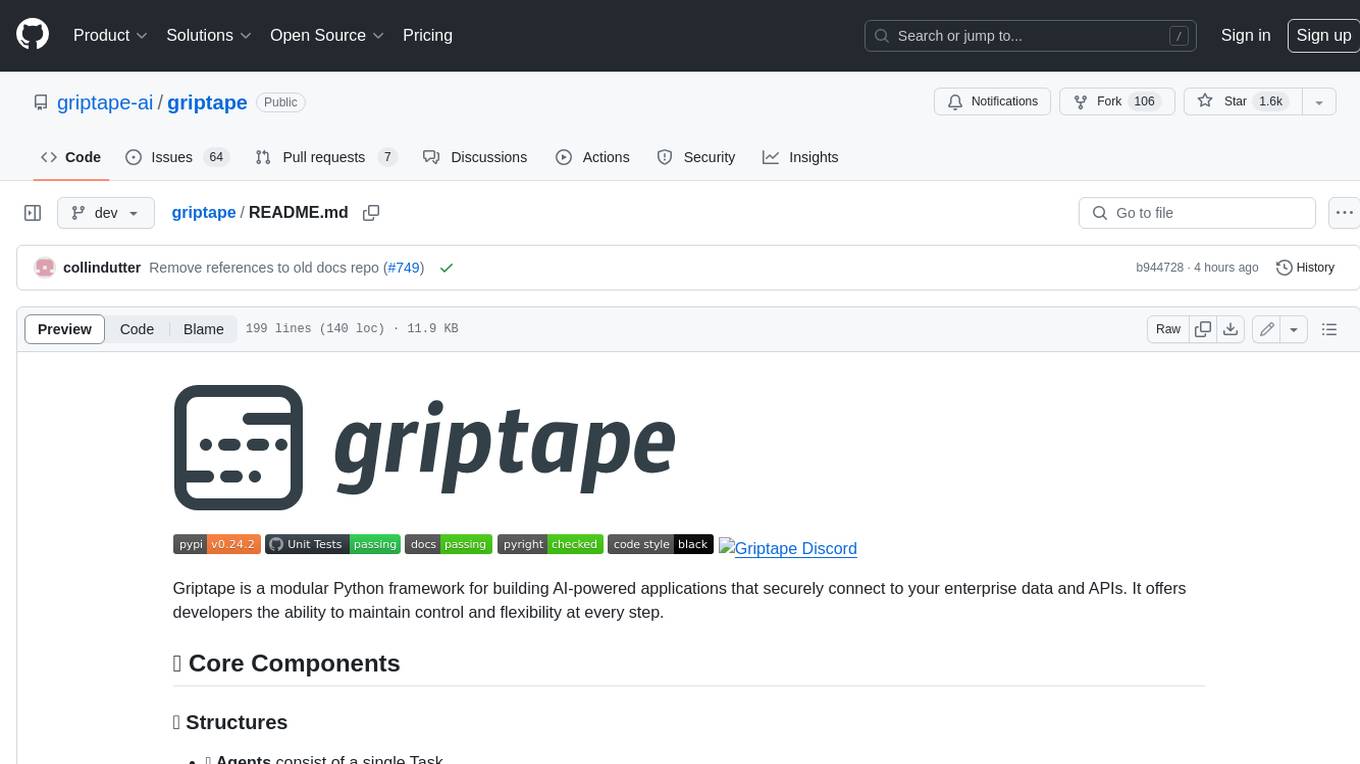
griptape
Griptape is a modular Python framework for building AI-powered applications that securely connect to your enterprise data and APIs. It offers developers the ability to maintain control and flexibility at every step. Griptape's core components include Structures (Agents, Pipelines, and Workflows), Tasks, Tools, Memory (Conversation Memory, Task Memory, and Meta Memory), Drivers (Prompt and Embedding Drivers, Vector Store Drivers, Image Generation Drivers, Image Query Drivers, SQL Drivers, Web Scraper Drivers, and Conversation Memory Drivers), Engines (Query Engines, Extraction Engines, Summary Engines, Image Generation Engines, and Image Query Engines), and additional components (Rulesets, Loaders, Artifacts, Chunkers, and Tokenizers). Griptape enables developers to create AI-powered applications with ease and efficiency.