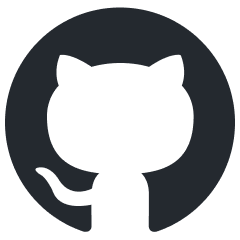
DISC-LawLLM
[中文法律大模型] DISC-LawLLM: an intelligent legal system powered by large language models (LLMs) to provide a wide range of legal services.
Stars: 590
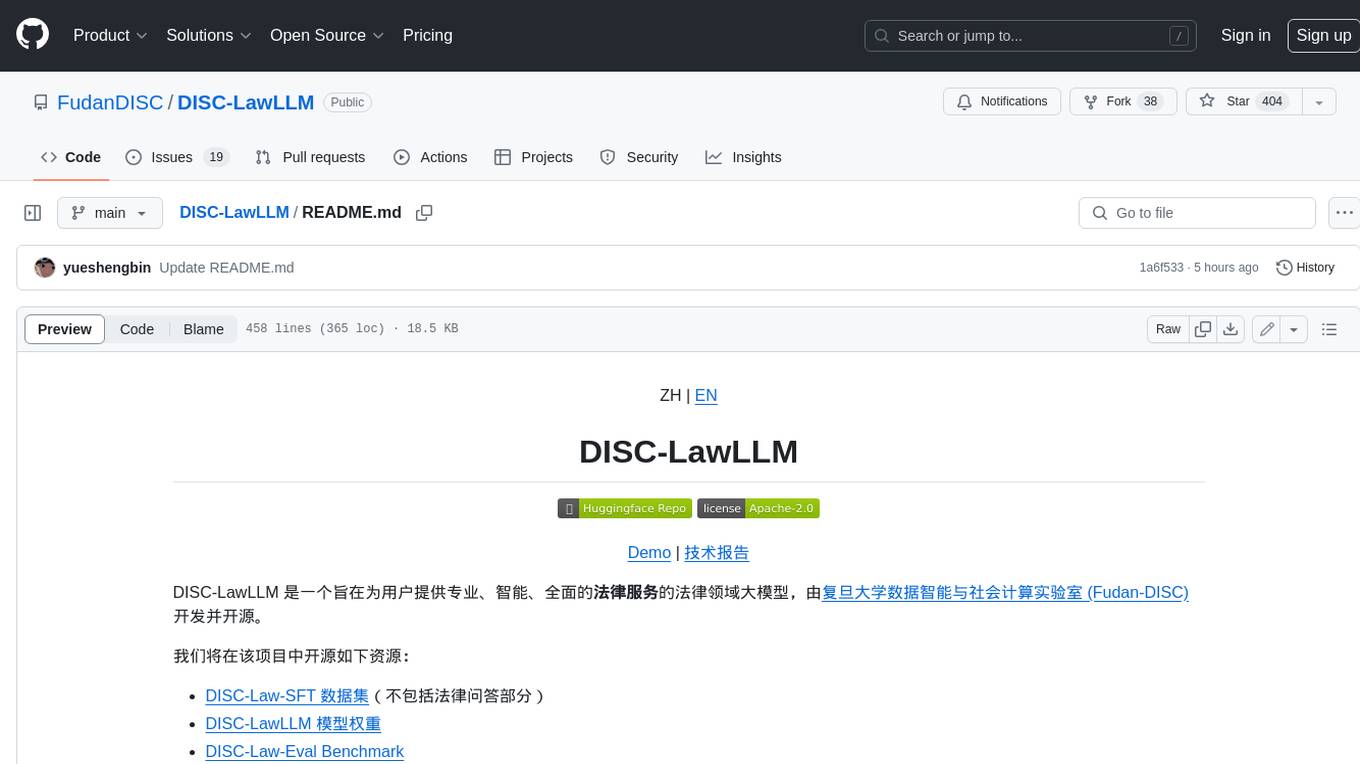
DISC-LawLLM is a legal domain large model that aims to provide professional, intelligent, and comprehensive **legal services** to users. It is developed and open-sourced by the Data Intelligence and Social Computing Lab (Fudan-DISC) at Fudan University.
README:
DISC-LawLLM 是一个旨在为用户提供专业、智能、全面的法律服务的法律领域大模型,由复旦大学数据智能与社会计算实验室 (Fudan-DISC) 开发并开源。
我们将在该项目中开源如下资源:
您可以通过访问这个链接来在线体验我们的 DISC-LawLLM。
[2024/10/15] 🎉 我们开源了DISC-Law-SFT 数据集中的法律问答部分(DISC-Law-SFT-Pair-QA-released.jsonl和DISC-Law-SFT-Triplet-QA-released.jsonl)
[2024/03/15] 🎉🥳✨我们的论文 “LawLLM: Intelligent Legal System with Legal Reasoning and Verifiable Retrieval” 被 DASFAA 2024 (CCF-B) 的 Research Track 录用为长文.✨
[2023/12/20] 🎉 我们在最新的法律评测Benchmark Lawbench 上的评测了DISC-LawLLM,结果仅次于GPT-4,超出了GPT3.5和目前所有的法律大模型。
[2023/11/20] 🎉 我们开源了 DISC-Law-Eval Benchmark 的评测代码,更多详情请在此处查看。
[2023/10/19] 我们开源了 DISC-Law-Eval Benchmark 中的评测数据集(包括标准答案)。
[2023/09/26] DISC-LawLLM v1.0 已正式发布,开源 DISC-LawLLM-13B 模型 和 DISC-Law-SFT 数据集。
DISC-LawLLM 是一个具有法律推理和知识检索能力的智能法律系统,它面向不同群体,能在不同应用场景下提供帮助,主要有以下几个特点:
- 法律文本处理能力: 针对法律语言的理解与生成能力,包括信息抽取、文本摘要等,我们基于现有的 NLP 司法任务公开数据和真实世界的法律相关文本进行了微调数据的构建。
- 法律推理思维能力: 针对智慧司法领域任务的需求,我们使用法律三段论这一法理推理理论设计了指令数据,有效地提高了模型的法理推理能力。
- 司法领域知识遵循能力: 我们为智能法律处理系统配备了检索增强的模块,增强了系统对于背景知识的的检索和遵循能力。
除此之外,我们的研究过程还包括了如下贡献:
- 高质量的训练数据集和普遍有效的训练范式
- 完备的法律模型测评框架和测评数据集
DISC-LawLLM在Lawbench上的评测结果仅次于GPT-4,超出了目前所有的法律大模型。以下是DISC-LawLLM和其他模型在Lawbench上Zero-shot、One-shot的平均分数排序:
不同场景下的法律智能应用通常需要结合法律文本理解和生成的多种基本能力。为此,我们构建了一个高质量的监督微调数据集 DISC-Law-SFT,包括法律信息提取、判决预测、文档摘要和法律问题解答,确保覆盖不同司法应用场景。DISC-Law-SFT 包括两个子集,即 DISC-Law-SFT-Pair 和 DISC-Law-SFT-Triplet。前者旨在为 LLM 引入法律推理能力,后者则有助于提高模型利用外部知识的能力,具体的构建细节请参照我们的技术报告。数据集的分布如下所示:
数据集 | 对应任务/来源 | 样本量 | 对应情境 |
---|---|---|---|
DISC-Law-SFT-Pair | 司法要素提取 | 32K | 法律专业人员助手 |
司法事件检测 | 27K | ||
案件分类 | 20K | ||
判决预测 | 11K | ||
类案匹配 | 8K | ||
司法摘要 | 9K | ||
舆情摘要 | 6K | ||
法律问答 | 93K | 法律咨询服务 | |
司法阅读理解 | 38K | 法律考试助手 | |
法律考试 | 12K | ||
DISC-Law-SFT-Triplet | 判决预测 | 16K | 法律专业人员助手 |
法律问答 | 23K | 法律咨询服务 | |
General | Alpaca-GPT4 | 48K | 通用场景 |
Firefly | 60K | ||
总计 | 403K |
我们总共发布了近30万条训练数据,其中包括 DISC-Law-SFT-Pair 和 DISC-Law-SFT-Triplet。您可以访问这个链接下载数据集。
我们在 DISC-LawLLM 的基础上增加了一个基于开源检索框架 Langchain-Chatchat 的检索模块。我们的知识库目前包括法条库和法考题库。
- 法条库包含 800 多部国家地方法律、条例和规定,其中包括《宪法》、《刑法》、《行政诉讼法》、《保险法》、《劳动法》、《著作权法》、《民法典》、《专利法》、《专属经济区和大陆架法》、《中国人民解放军选举全国人民代表大会和县级以上地方各级人民代表大会代表的办法》、《反分裂国家法》、《出境入境边防检查条例》、《国务院关于鼓励台湾同胞投资的规定》、《境内外国人宗教活动管理规定》等。
- 法考题库包含 2.4 万道法律相关的考试题目。
在未来,我们会增加更加丰富的知识库。我们还将进一步深入探索检索增强的 DISC-LawLLM,包括但不限于检索器与 LLM 的联合训练机制,各位有兴趣可以与我们一起交流。
开源版本的 DISC-LawLLM 是基于 Baichuan-13B-Base 进行微调训练得到的。您可以直接从 Hugging Face 上下载我们的模型权重,或者根据下面的代码样例自动获取。推理前请安装依赖:
pip install -r requirements.txt
import torch
from transformers import AutoModelForCausalLM, AutoTokenizer
from transformers.generation.utils import GenerationConfig
model_path = "ShengbinYue/DISC-LawLLM"
model = AutoModelForCausalLM.from_pretrained(
model_path, torch_dtype=torch.float16, device_map="auto", trust_remote_code=True
)
model.generation_config = GenerationConfig.from_pretrained(model_path)
tokenizer = AutoTokenizer.from_pretrained(
model_path, use_fast=False, trust_remote_code=True,
)
messages = [
{"role": "user", "content": "生产销售假冒伪劣商品罪如何判刑?"},
]
response = model.chat(tokenizer, messages)
python cli_demo.py
依靠 streamlit 工具运行以下命令,会在本地启动一个 web 服务,把控制台给出的地址输入浏览器即可访问:
streamlit run web_demo.py --server.port 8888
此外,目前版本的 DISC-LawLLM 是以 Baichuan-13B 作为基座的,您可以参照 Baichuan-13B 的介绍来进行 int8 或 int4 量化推理部署以及 CPU 部署。
开发者可以对 DISC-LawLLM 进行微调使用。在此可以参照与 DISC-LawLLM 兼容的微调工具 LLaMA Efficient Tuning 或是我们的 DISC-MedLLM 医疗大模型。我们以 LLaMA Efficient Tuning 为例给出全量和 LoRA 两种微调示例。
首先,下载 LLaMA Efficient Tuning 并按其要求安装依赖。注意训练数据按照项目中的要求进行处理。下面我们给出两种微调场景下的脚本样例。
我们在 8 * Nvidia A800 80 GB + deepspeed 的环境下进行了全量微调测试。训练启动脚本示例如下:
deepspeed --num_gpus=8 src/train_bash.py \
--stage sft \
--model_name_or_path S heng bin \
--do_train \
--dataset alpaca_gpt4_zh \
--template baichuan \
--finetuning_type full \
--output_dir path_to_your_sft_checkpoint \
--overwrite_cache \
--per_device_train_batch_size 4 \
--per_device_eval_batch_size 4 \
--gradient_accumulation_steps 8 \
--preprocessing_num_workers 8 \
--lr_scheduler_type cosine \
--logging_steps 10 \
--save_steps 100 \
--eval_steps 100 \
--learning_rate 5e-5 \
--max_grad_norm 0.5 \
--num_train_epochs 2.0 \
--dev_ratio 0.01 \
--evaluation_strategy steps \
--load_best_model_at_end \
--plot_loss \
--fp16 \
--deepspeed deepspeed.json
deep_speed.json
配置示例如下:
{
"train_micro_batch_size_per_gpu": "auto",
"zero_allow_untested_optimizer": true,
"fp16": {
"enabled": "auto",
"loss_scale": 0,
"initial_scale_power": 16,
"loss_scale_window": 1000,
"hysteresis": 2,
"min_loss_scale": 1
},
"zero_optimization": {
"stage": 2,
"allgather_partitions": true,
"allgather_bucket_size": 5e8,
"overlap_comm": false,
"reduce_scatter": true,
"reduce_bucket_size": 5e8,
"contiguous_gradients" : true
}
}
我们在 4 * Nvidia A800 80G 显卡上进行了 LoRA 微调测试。训练启动脚本示例如下:
torchrun --nproc_per_node 4 src/train_bash.py \
--stage sft \
--model_name_or_path ShengbinYue/DISC-LawLLM \
--do_train \
--dataset alpaca_gpt4_zh \
--template baichuan \
--finetuning_type lora \
--lora_rank 8 \
--lora_target W_pack \
--output_dir path_to_your_sft_checkpoint \
--overwrite_cache \
--per_device_train_batch_size 4 \
--per_device_eval_batch_size 4 \
--gradient_accumulation_steps 8 \
--preprocessing_num_workers 16 \
--lr_scheduler_type cosine \
--logging_steps 10 \
--save_steps 100 \
--eval_steps 100 \
--learning_rate 1e-5 \
--max_grad_norm 0.5 \
--num_train_epochs 2.0 \
--dev_ratio 0.01 \
--evaluation_strategy steps \
--load_best_model_at_end \
--plot_loss \
--fp16
受司法考试构成的启发,我们开发了一个公平的评估框架 —— DISC-Law-Eval Benchmark,从客观和主观两个角度对法律大语言模型的性能进行评估,以考察模型在中国法律领域的性能。您可以点击在这里查看 DISC-Law-Eval Benchmark 的更多详情。我们还开发了名为 ml3m 的 Python 套件,取自 Multilevel Legal LLM。您可以在这里查看其技术文档。
为了客观、定量地评估智能法律系统的法律知识和推理能力,客观的评价数据集由一系列中国法律标准化考试和知识竞赛的单项和多项选择题组成,并根据内容复杂性和演绎难度,将问题分为困难、中等和简单三个层次。它可以提供一个更具挑战性和可靠的方法来衡量模型是否可以利用其知识来推理正确的答案。我们通过一系列正则表达式来匹配模型回复中所选择的选项,并将其与标准答案比对,最终通过计算模型回答争取的题目的百分比来衡量模型的客观题答题性能。你可以在这里查看我们的客观评测集。数据集具体构成如下:
科目 | 难度等级 | 单选题数量 | 多选题数量 | 总数 |
---|---|---|---|---|
NJE:国家统一法律职业资格考试 | 困难 | 537 | 463 | 1000 |
PAE:专利代理人考试 | 118 | 276 | 394 | |
CPA:注册会计师资格考试 | 197 | 120 | 317 | |
UNGEE:法学专硕全国统考试题 | 中等 | 320 | 87 | 407 |
LBK:法律基础知识题库 | 简单 | 275 | - | 275 |
PFE:事业编、公务员考试法律试题 | 170 | - | 170 |
在主观评测部分,我们采用问答题形式进行评估,模拟主观考试问题的过程。我们从法律咨询、在线论坛、与司法相关的出版物和法律文件中手工构建了一个高质量的测试集。我们用 GPT-3.5 Turbo 作为裁判模型来评估模型的输出,并基于标准答案用准确性、完整性和清晰度这三个标准提供 1-5 的评分。详见 ml3m 文档。
主观题数据集从来源于法律咨询、网上发帖、司法相关出版物和法律文书中手动构建的一个高质量的测试集,其中包括 300 个示例,涵盖了法律知识问答、法律咨询和判决预测等场景。你可以在这里查看我们的主观评测集。
客观题评测采用 few-shot 方式,结果(%)如下:
模型 | NJE 单选 | NJE 多选 | PAE 单选 | PAE 多选 | CPA 单选 | CPA 多选 | UNGEE 单选 | UNGEE 多选 | PFE 单选 | LBK 单选 | 平均 |
---|---|---|---|---|---|---|---|---|---|---|---|
ChatGLM | 31.66 | 1.08 | 27.97 | 2.90 | 37.06 | 13.33 | 39.69 | 20.69 | 37.65 | 42.91 | 24.66 |
Baichuan-Chat | 31.47 | 10.15 | 29.66 | 8.70 | 35.53 | 19.17 | 50.00 | 27.59 | 53.12 | 53.45 | 30.78 |
Chinese-Alpaca-2 | 25.70 | 10.15 | 30.51 | 11.59 | 32.99 | 19.17 | 40.94 | 21.84 | 44.12 | 43.27 | 26.73 |
GPT-3.5-turbo | 36.50 | 10.58 | 37.29 | 17.03 | 42.13 | 21.67 | 51.25 | 28.74 | 53.53 | 54.18 | 34.10 |
LexiLaw | 20.11 | 7.56 | 23.73 | 10.14 | 24.87 | 19.17 | 31.56 | 16.09 | 31.76 | 40.36 | 21.50 |
LawGPT | 22.91 | 6.26 | 31.36 | 7.61 | 25.38 | 16.67 | 30.31 | 13.79 | 34.71 | 29.09 | 20.60 |
Lawyer LLaMa | 35.75 | 5.62 | 32.20 | 6.52 | 29.95 | 13.33 | 32.50 | 14.94 | 39.41 | 39.64 | 25.05 |
ChatLaw | 27.56 | 7.99 | 31.36 | 9.42 | 35.53 | 11.67 | 35.62 | 17.24 | 42.35 | 41.09 | 25.20 |
DISC-LawLLM | 42.09 | 19.87 | 40.68 | 18.48 | 39.59 | 19.17 | 50.94 | 25.29 | 57.06 | 54.91 | 37.10 |
主观题评测分数为 1-5,结果如下:
模型 | 准确性 | 完整性 | 清晰性 | 平均 |
---|---|---|---|---|
ChatGLM | 2.64 | 2.75 | 3.23 | 2.87 |
Baichuan-Chat | 3.22 | 3.34 | 3.18 | 3.25 |
Chinese-Alpaca-2 | 3.13 | 3.23 | 3.17 | 3.17 |
LexiLaw | 3.06 | 2.62 | 3.00 | 2.90 |
LawGPT | 3.02 | 2.58 | 2.96 | 2.86 |
Lawyer LLaMa | 3.13 | 2.83 | 3.35 | 3.10 |
ChatLaw | 3.31 | 2.90 | 3.35 | 3.19 |
DISC-LawLLM | 3.46 | 3.12 | 3.59 | 3.39 |
本项目基于如下开源项目展开,在此对相关项目和开发人员表示诚挚的感谢:
同样感谢其他限于篇幅未能列举的为本项目提供了重要帮助的工作。
DISC-LawLLM 有着目前大语言模型尚无法克服的问题和缺陷,尽管它能够在许多任务和情境上提供法律服务,但模型应当仅供用户参考使用,并不能替代专业律师和法律专家,我们希望 DISC-LawLLM 的用户以批判性的眼光去评估模型。我们不对因使用 DISC-LawLLM 所引发的任何问题、风险或不良后果承担责任。
如果我们的项目对您的研究和工作有帮助,请如下引用我们的项目:
@misc{yue2023disclawllm,
title={DISC-LawLLM: Fine-tuning Large Language Models for Intelligent Legal Services},
author={Shengbin Yue and Wei Chen and Siyuan Wang and Bingxuan Li and Chenchen Shen and Shujun Liu and Yuxuan Zhou and Yao Xiao and Song Yun and Xuanjing Huang and Zhongyu Wei},
year={2023},
eprint={2309.11325},
archivePrefix={arXiv},
primaryClass={cs.CL}
}
@inproceedings{yue2024lawllm,
title={LawLLM: Intelligent Legal System with Legal Reasoning and Verifiable Retrieval},
author={Yue, Shengbin and Liu, Shujun and Zhou, Yuxuan and Shen, Chenchen and Wang, Siyuan and Xiao, Yao and Li, Bingxuan and Song, Yun and Shen, Xiaoyu and Chen, Wei and others},
booktitle={International Conference on Database Systems for Advanced Applications},
pages={304--321},
year={2024},
organization={Springer}
}
DISC-LawLLM 可在 Apache 许可证下使用。请查看 LICENSE 文件获取更多信息。
For Tasks:
Click tags to check more tools for each tasksFor Jobs:
Alternative AI tools for DISC-LawLLM
Similar Open Source Tools
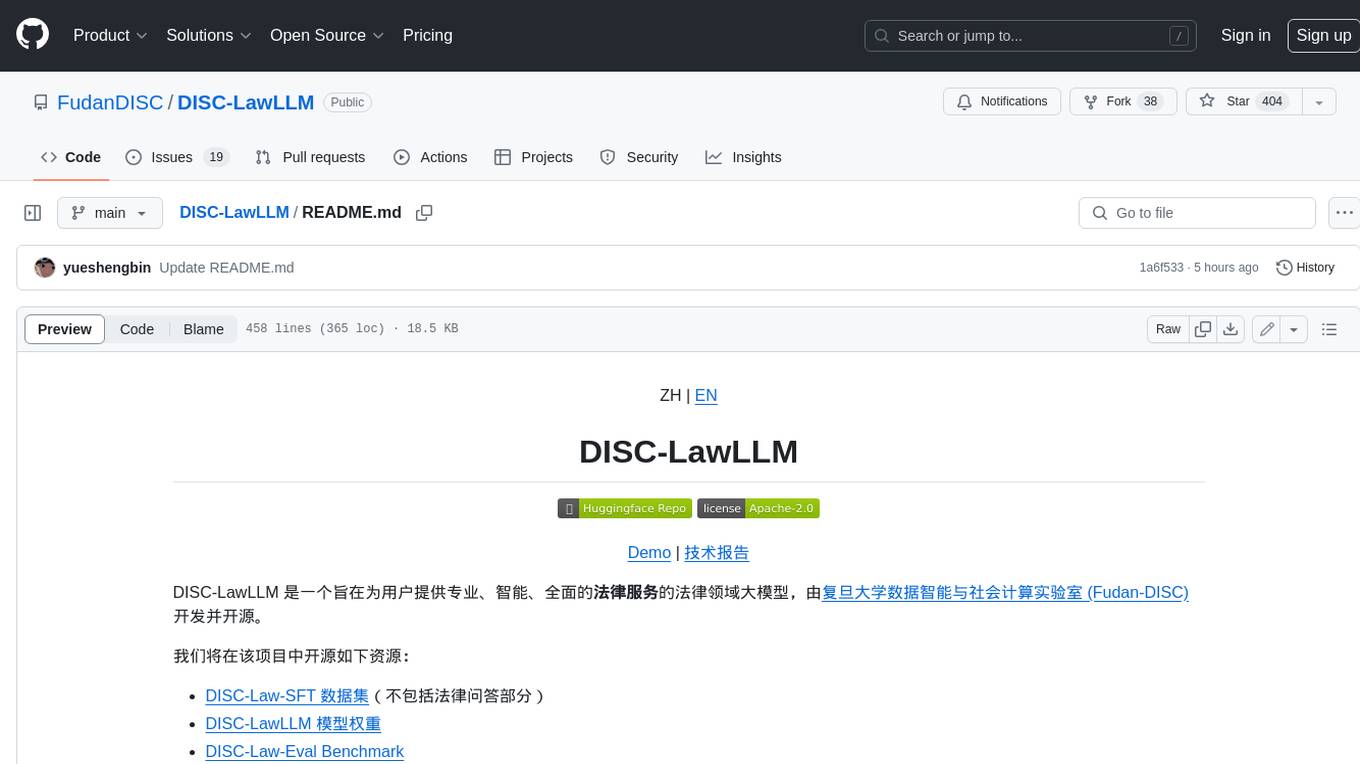
DISC-LawLLM
DISC-LawLLM is a legal domain large model that aims to provide professional, intelligent, and comprehensive **legal services** to users. It is developed and open-sourced by the Data Intelligence and Social Computing Lab (Fudan-DISC) at Fudan University.
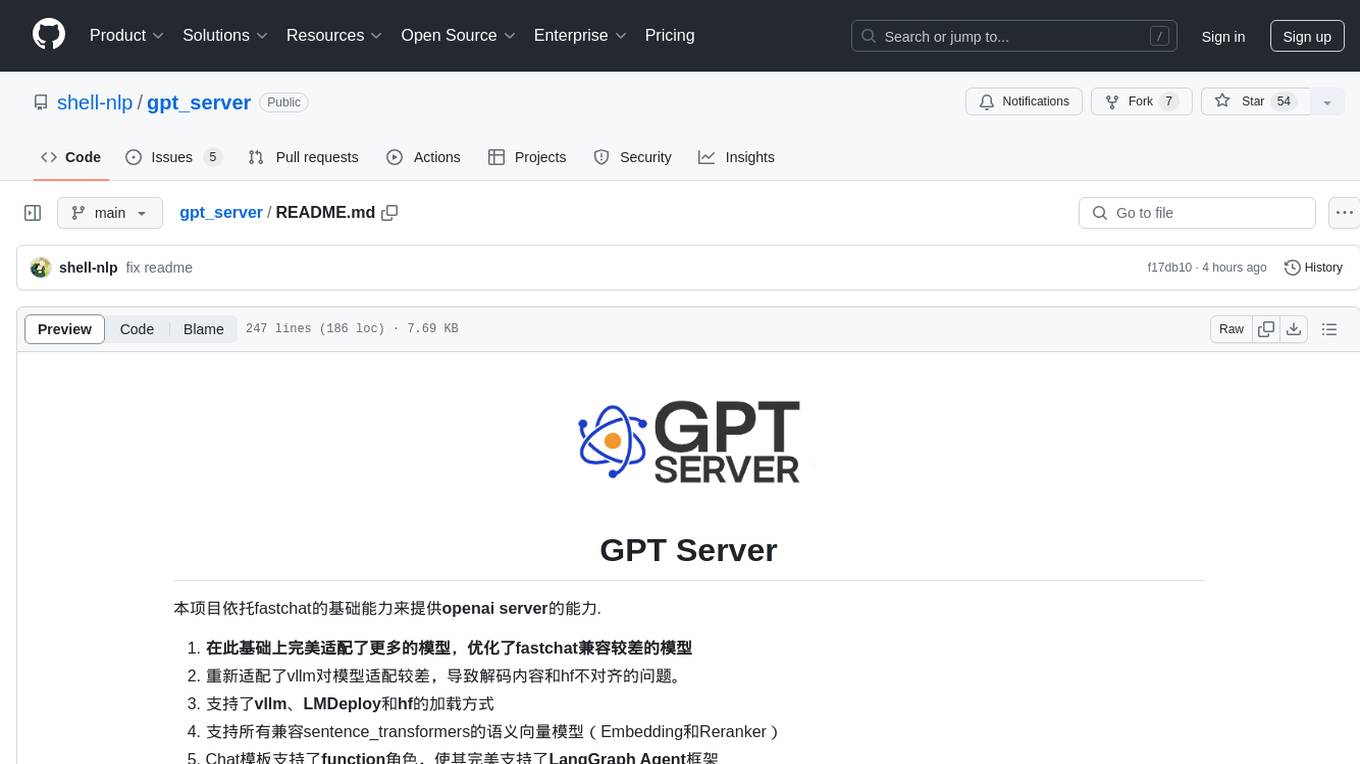
gpt_server
The GPT Server project leverages the basic capabilities of FastChat to provide the capabilities of an openai server. It perfectly adapts more models, optimizes models with poor compatibility in FastChat, and supports loading vllm, LMDeploy, and hf in various ways. It also supports all sentence_transformers compatible semantic vector models, including Chat templates with function roles, Function Calling (Tools) capability, and multi-modal large models. The project aims to reduce the difficulty of model adaptation and project usage, making it easier to deploy the latest models with minimal code changes.
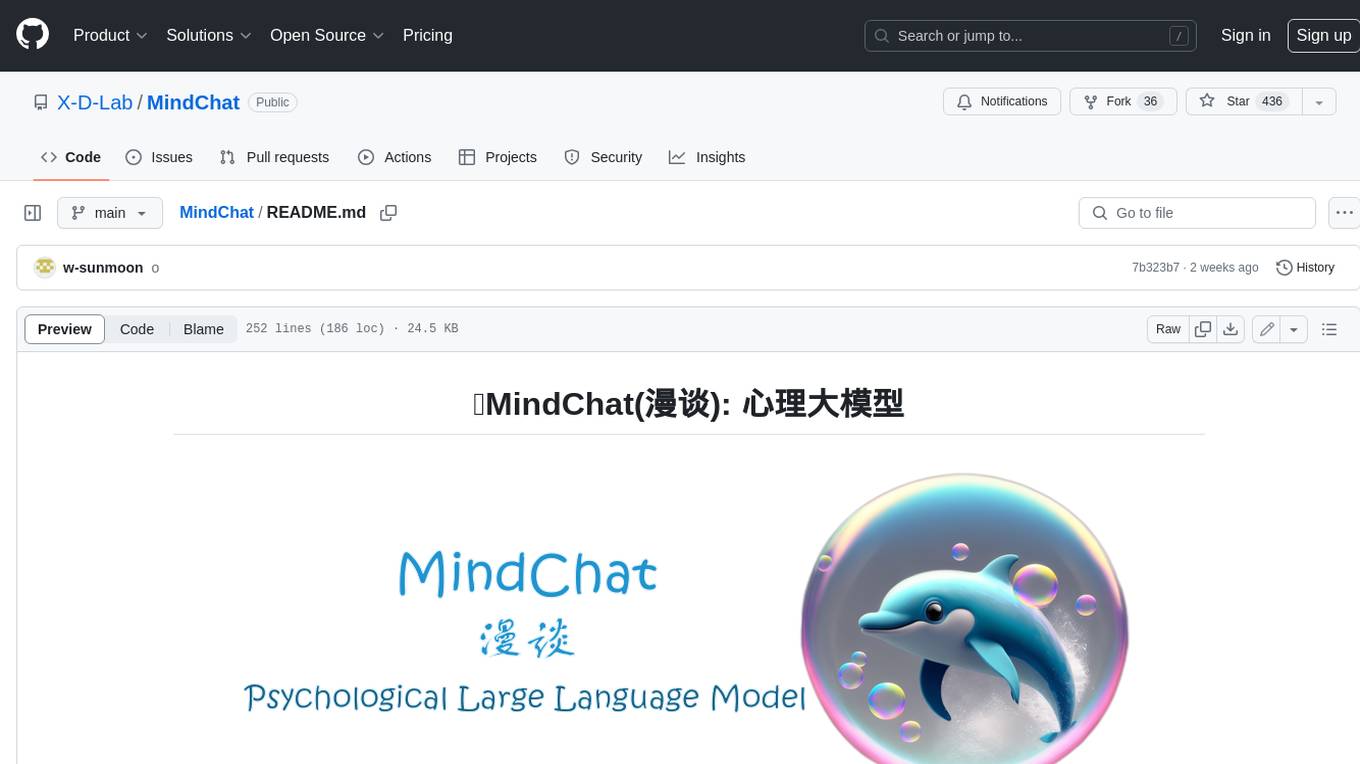
MindChat
MindChat is a psychological large language model designed to help individuals relieve psychological stress and solve mental confusion, ultimately improving mental health. It aims to provide a relaxed and open conversation environment for users to build trust and understanding. MindChat offers privacy, warmth, safety, timely, and convenient conversation settings to help users overcome difficulties and challenges, achieve self-growth, and development. The tool is suitable for both work and personal life scenarios, providing comprehensive psychological support and therapeutic assistance to users while strictly protecting user privacy. It combines psychological knowledge with artificial intelligence technology to contribute to a healthier, more inclusive, and equal society.
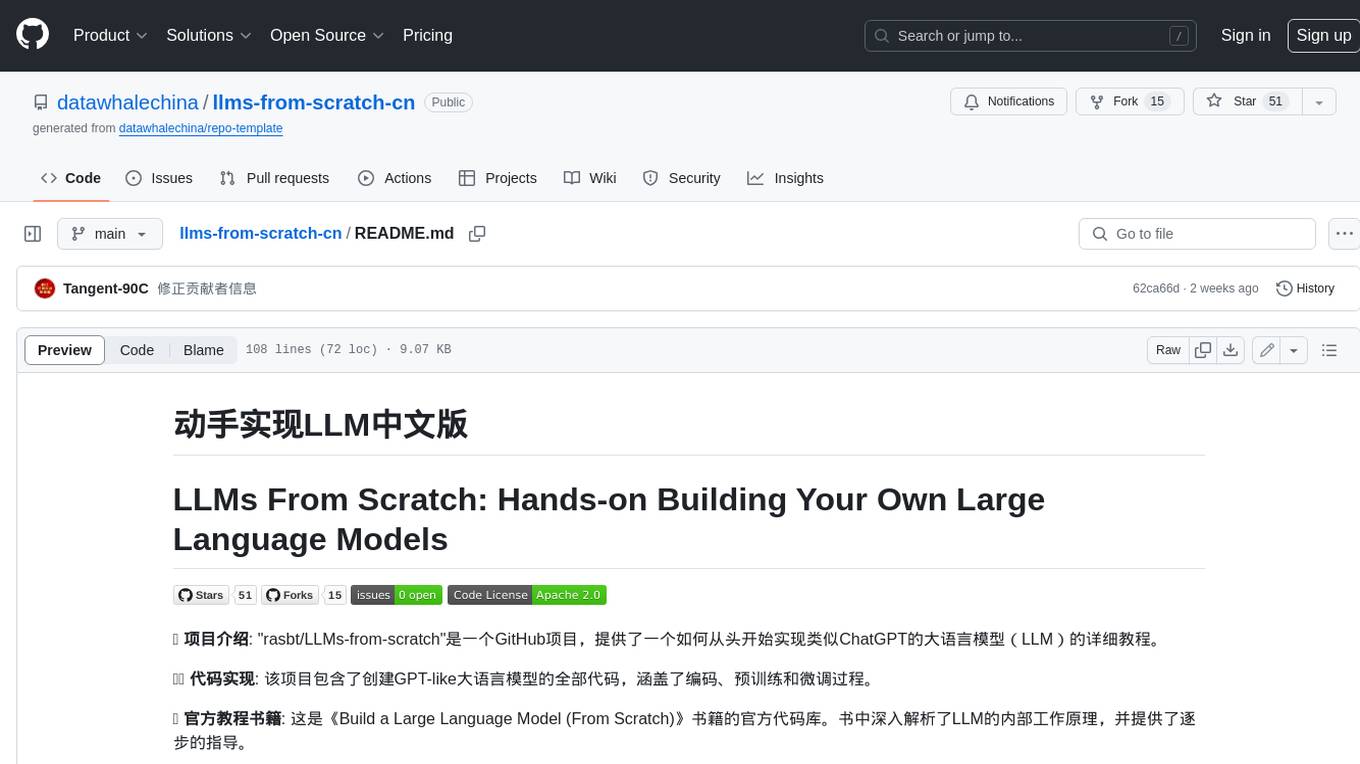
llms-from-scratch-cn
This repository provides a detailed tutorial on how to build your own large language model (LLM) from scratch. It includes all the code necessary to create a GPT-like LLM, covering the encoding, pre-training, and fine-tuning processes. The tutorial is written in a clear and concise style, with plenty of examples and illustrations to help you understand the concepts involved. It is suitable for developers and researchers with some programming experience who are interested in learning more about LLMs and how to build them.
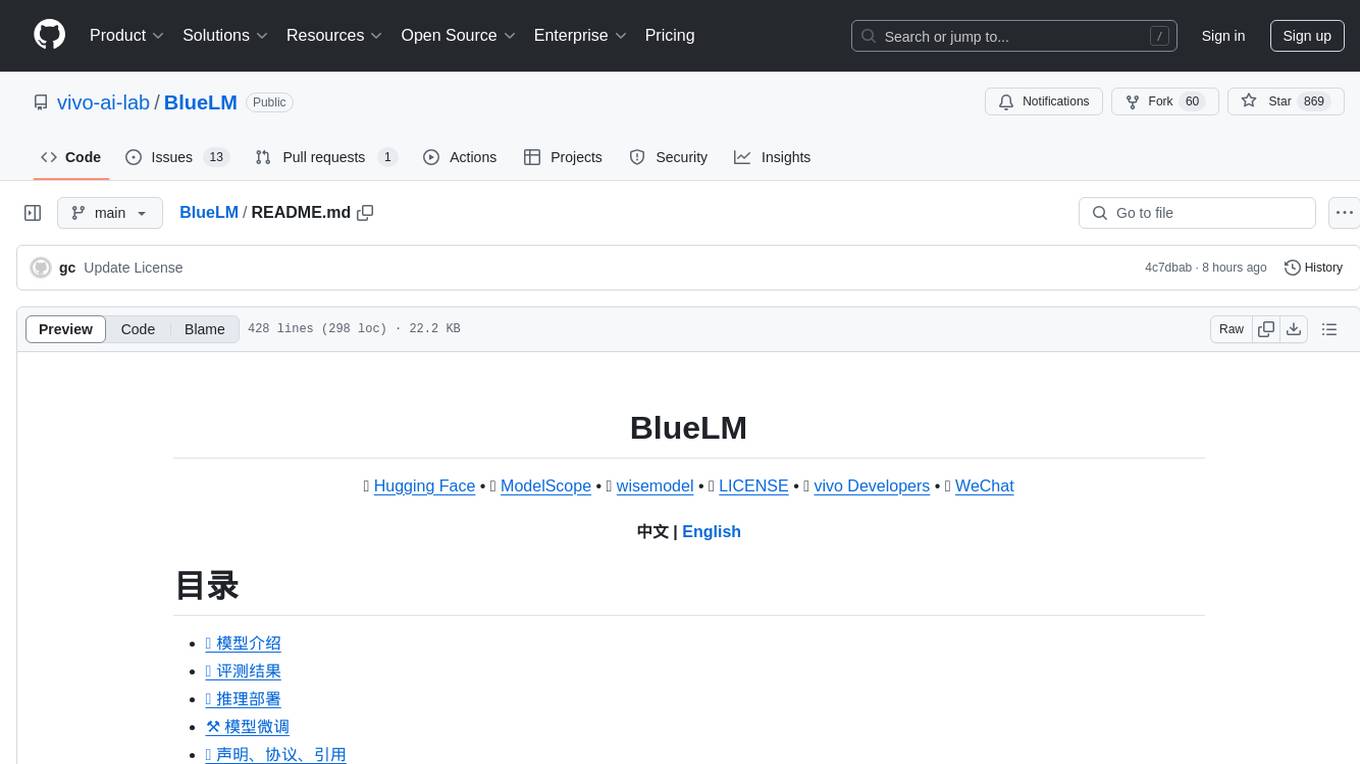
BlueLM
BlueLM is a large-scale pre-trained language model developed by vivo AI Global Research Institute, featuring 7B base and chat models. It includes high-quality training data with a token scale of 26 trillion, supporting both Chinese and English languages. BlueLM-7B-Chat excels in C-Eval and CMMLU evaluations, providing strong competition among open-source models of similar size. The models support 32K long texts for better context understanding while maintaining base capabilities. BlueLM welcomes developers for academic research and commercial applications.
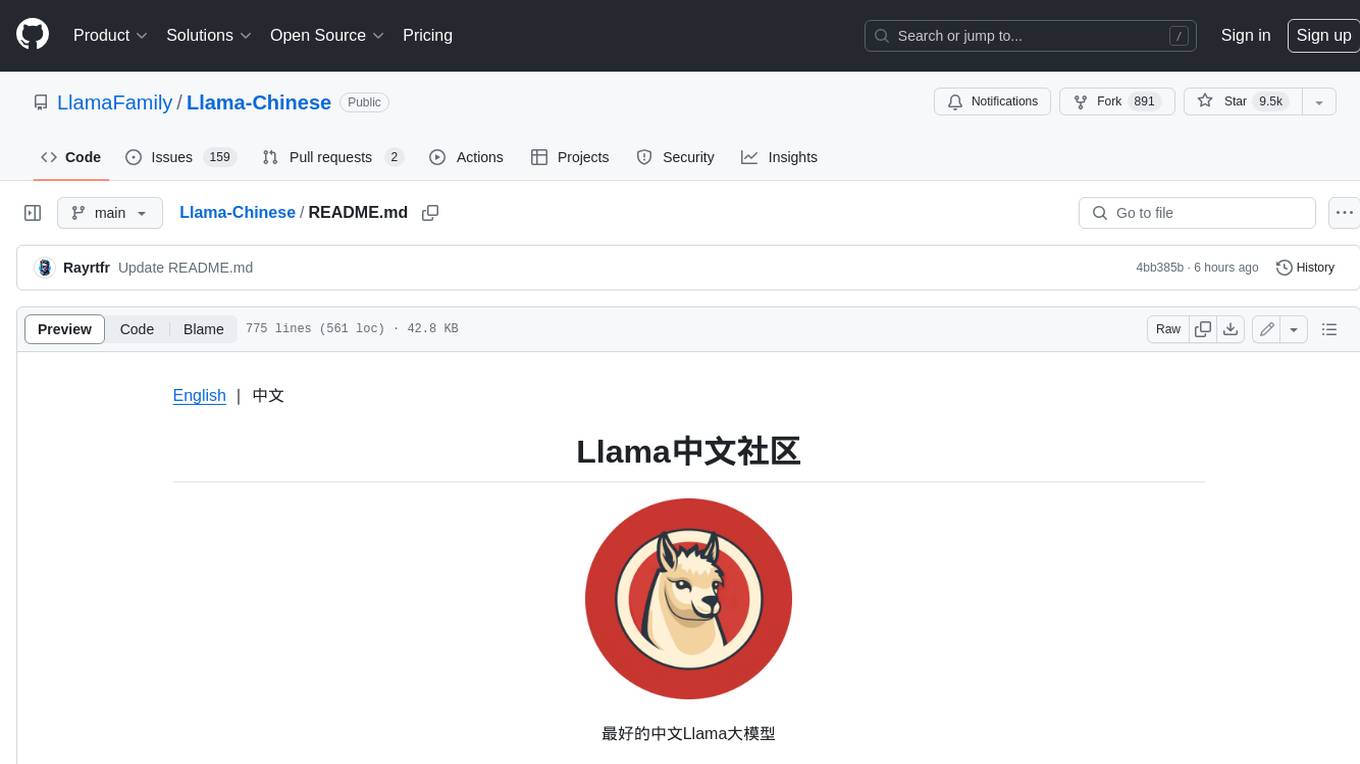
Llama-Chinese
Llama中文社区是一个专注于Llama模型在中文方面的优化和上层建设的高级技术社区。 **已经基于大规模中文数据,从预训练开始对Llama2模型进行中文能力的持续迭代升级【Done】**。**正在对Llama3模型进行中文能力的持续迭代升级【Doing】** 我们热忱欢迎对大模型LLM充满热情的开发者和研究者加入我们的行列。
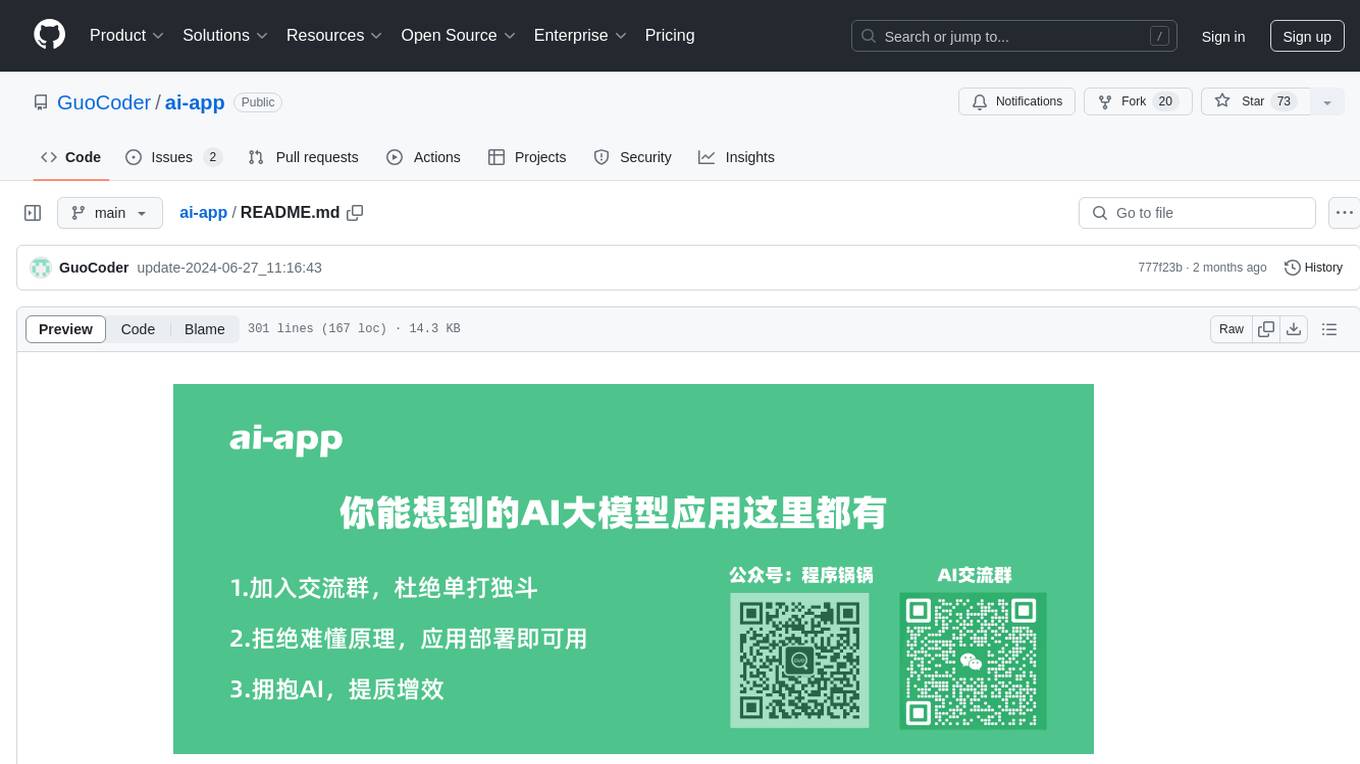
ai-app
The 'ai-app' repository is a comprehensive collection of tools and resources related to artificial intelligence, focusing on topics such as server environment setup, PyCharm and Anaconda installation, large model deployment and training, Transformer principles, RAG technology, vector databases, AI image, voice, and music generation, and AI Agent frameworks. It also includes practical guides and tutorials on implementing various AI applications. The repository serves as a valuable resource for individuals interested in exploring different aspects of AI technology.
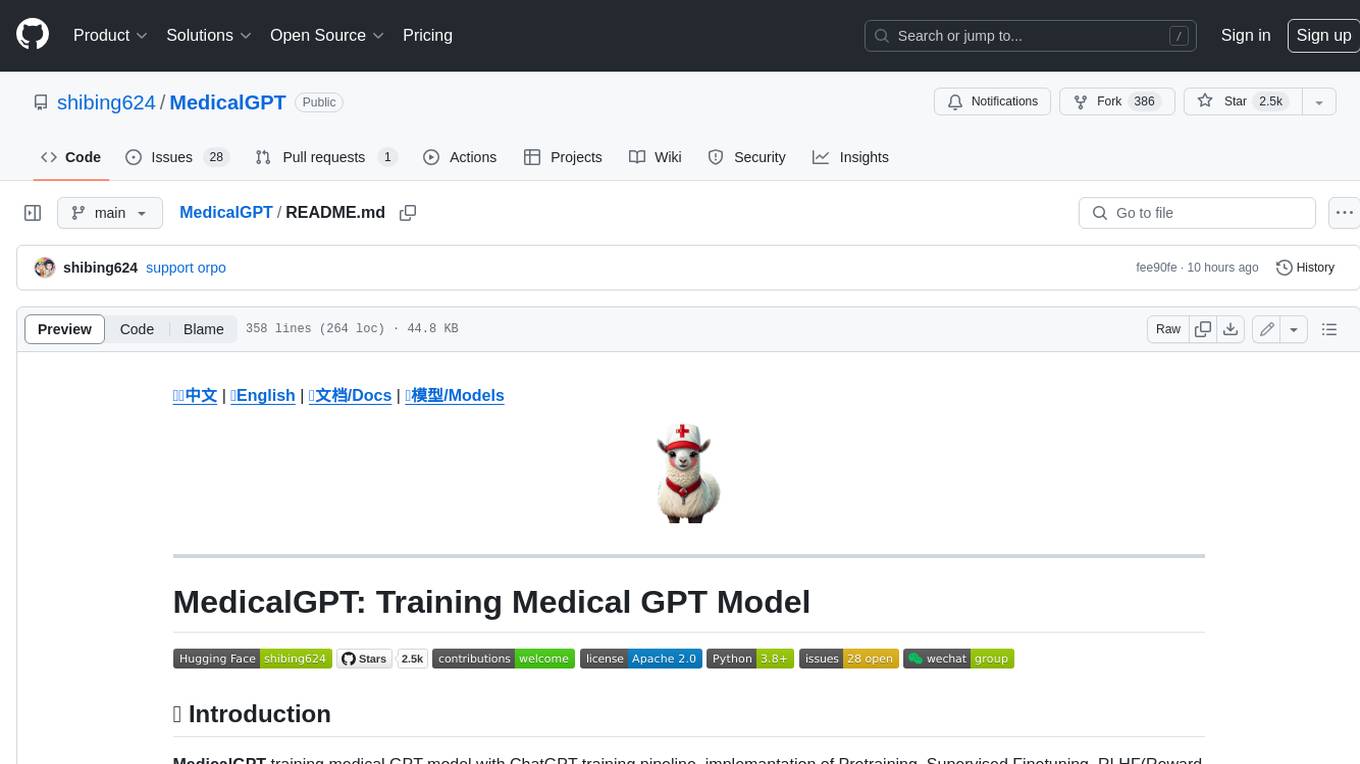
MedicalGPT
MedicalGPT is a training medical GPT model with ChatGPT training pipeline, implement of Pretraining, Supervised Finetuning, RLHF(Reward Modeling and Reinforcement Learning) and DPO(Direct Preference Optimization).
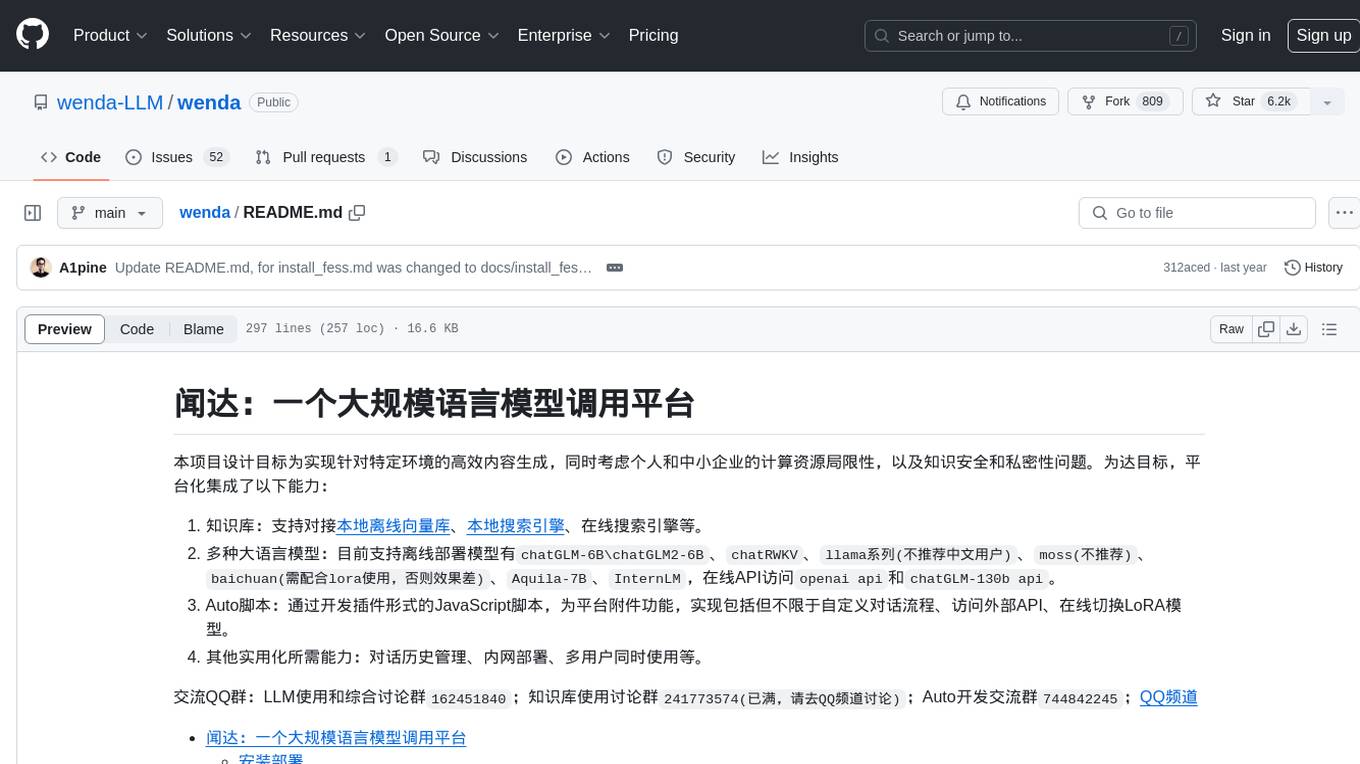
wenda
Wenda is a platform for large-scale language model invocation designed to efficiently generate content for specific environments, considering the limitations of personal and small business computing resources, as well as knowledge security and privacy issues. The platform integrates capabilities such as knowledge base integration, multiple large language models for offline deployment, auto scripts for additional functionality, and other practical capabilities like conversation history management and multi-user simultaneous usage.
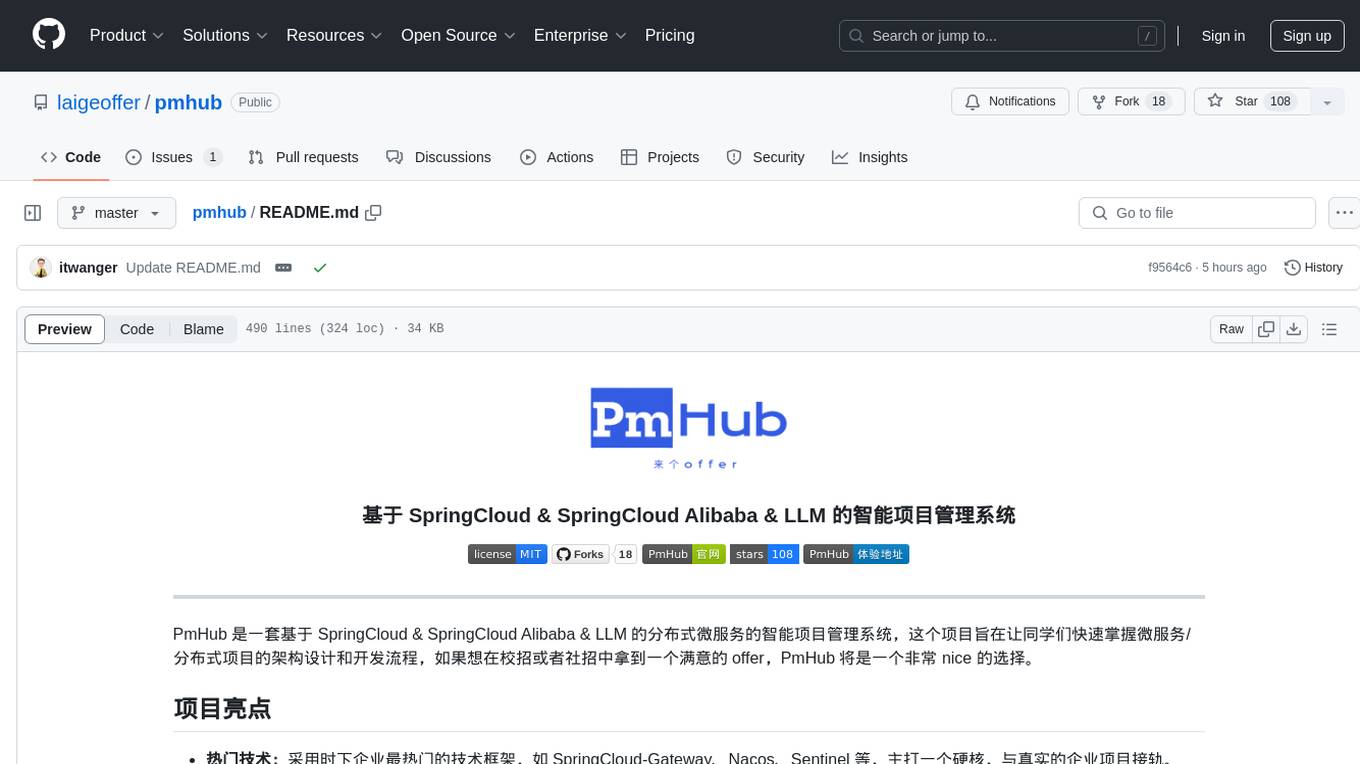
pmhub
PmHub is a smart project management system based on SpringCloud, SpringCloud Alibaba, and LLM. It aims to help students quickly grasp the architecture design and development process of microservices/distributed projects. PmHub provides a platform for students to experience the transformation from monolithic to microservices architecture, understand the pros and cons of both architectures, and prepare for job interviews. It offers popular technologies like SpringCloud-Gateway, Nacos, Sentinel, and provides high-quality code, continuous integration, product design documents, and an enterprise workflow system. PmHub is suitable for beginners and advanced learners who want to master core knowledge of microservices/distributed projects.
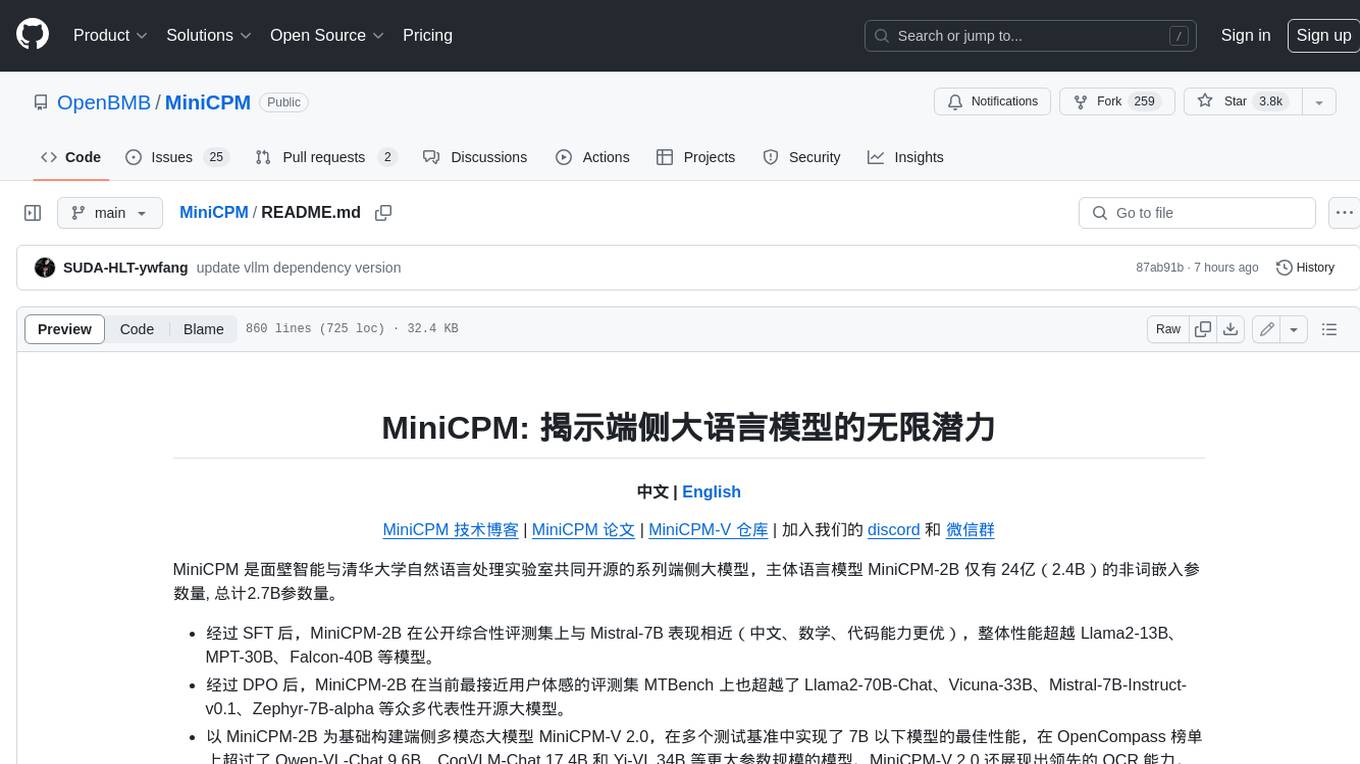
MiniCPM
MiniCPM is a series of open-source large models on the client side jointly developed by Face Intelligence and Tsinghua University Natural Language Processing Laboratory. The main language model MiniCPM-2B has only 2.4 billion (2.4B) non-word embedding parameters, with a total of 2.7B parameters. - After SFT, MiniCPM-2B performs similarly to Mistral-7B on public comprehensive evaluation sets (better in Chinese, mathematics, and code capabilities), and outperforms models such as Llama2-13B, MPT-30B, and Falcon-40B overall. - After DPO, MiniCPM-2B also surpasses many representative open-source large models such as Llama2-70B-Chat, Vicuna-33B, Mistral-7B-Instruct-v0.1, and Zephyr-7B-alpha on the current evaluation set MTBench, which is closest to the user experience. - Based on MiniCPM-2B, a multi-modal large model MiniCPM-V 2.0 on the client side is constructed, which achieves the best performance of models below 7B in multiple test benchmarks, and surpasses larger parameter scale models such as Qwen-VL-Chat 9.6B, CogVLM-Chat 17.4B, and Yi-VL 34B on the OpenCompass leaderboard. MiniCPM-V 2.0 also demonstrates leading OCR capabilities, approaching Gemini Pro in scene text recognition capabilities. - After Int4 quantization, MiniCPM can be deployed and inferred on mobile phones, with a streaming output speed slightly higher than human speech speed. MiniCPM-V also directly runs through the deployment of multi-modal large models on mobile phones. - A single 1080/2080 can efficiently fine-tune parameters, and a single 3090/4090 can fully fine-tune parameters. A single machine can continuously train MiniCPM, and the secondary development cost is relatively low.
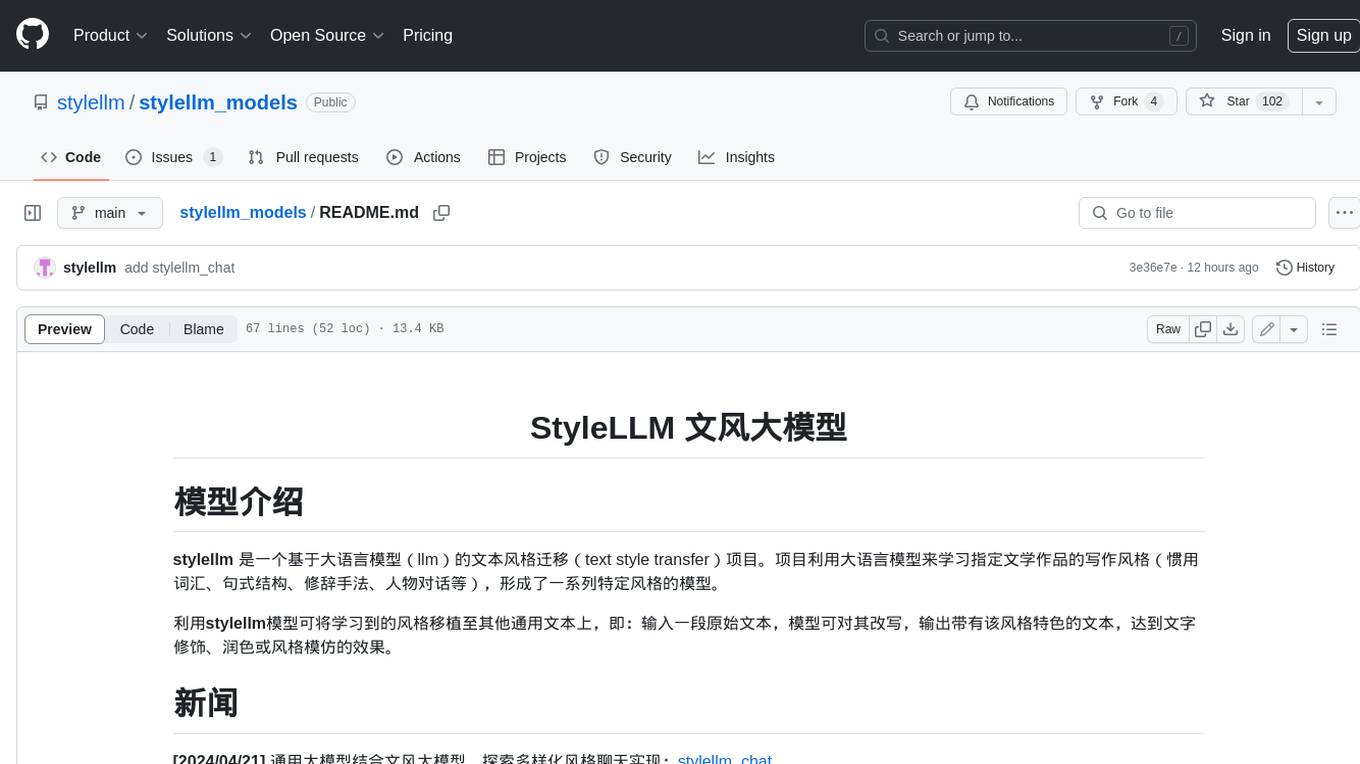
stylellm_models
**stylellm** is a text style transfer project based on large language models (llms). The project utilizes large language models to learn the writing style of specific literary works (commonly used vocabulary, sentence structure, rhetoric, character dialogue, etc.), forming a series of specific style models. Using the **stylellm** model, the learned style can be transferred to other general texts, that is: input a piece of original text, the model can rewrite it, output text with the characteristics of that style, achieving the effect of text modification,润色or style imitation.
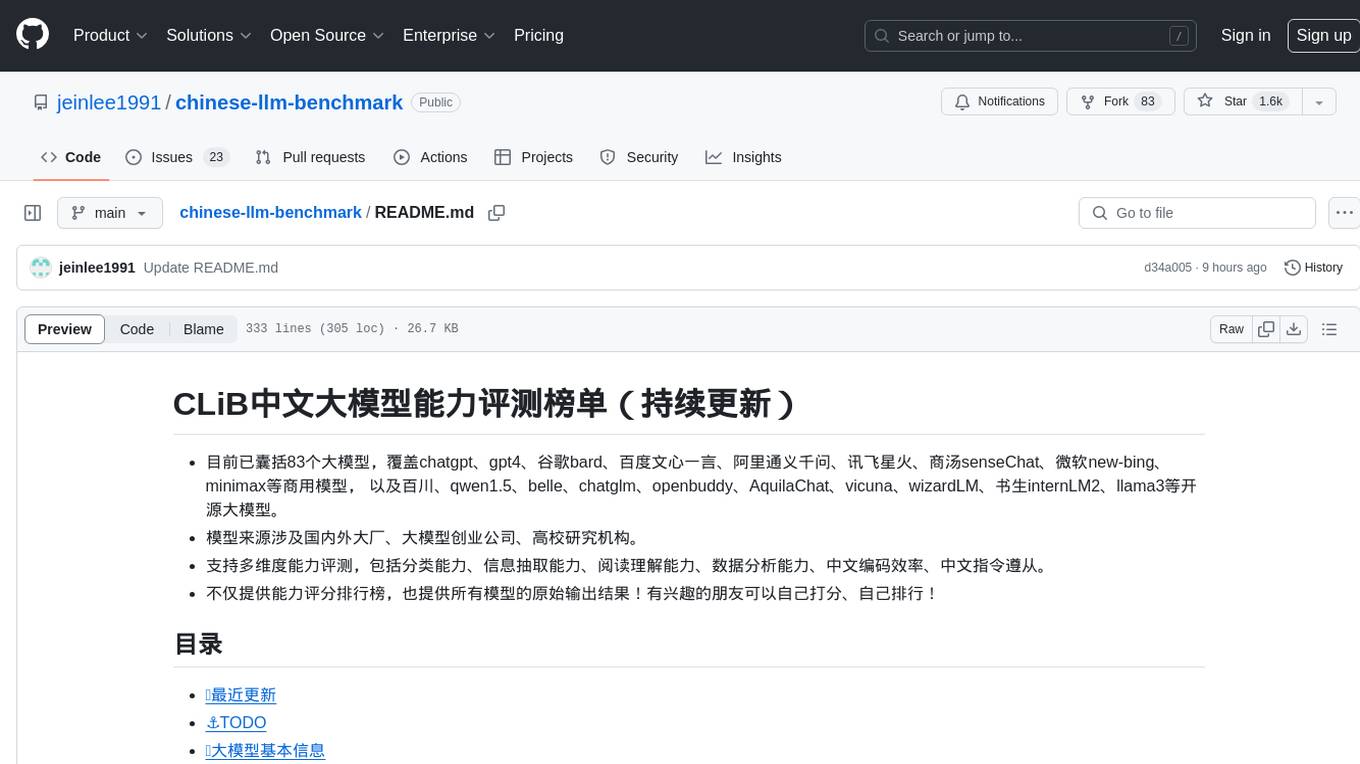
chinese-llm-benchmark
The Chinese LLM Benchmark is a continuous evaluation list of large models in CLiB, covering a wide range of commercial and open-source models from various companies and research institutions. It supports multidimensional evaluation of capabilities including classification, information extraction, reading comprehension, data analysis, Chinese encoding efficiency, and Chinese instruction compliance. The benchmark not only provides capability score rankings but also offers the original output results of all models for interested individuals to score and rank themselves.
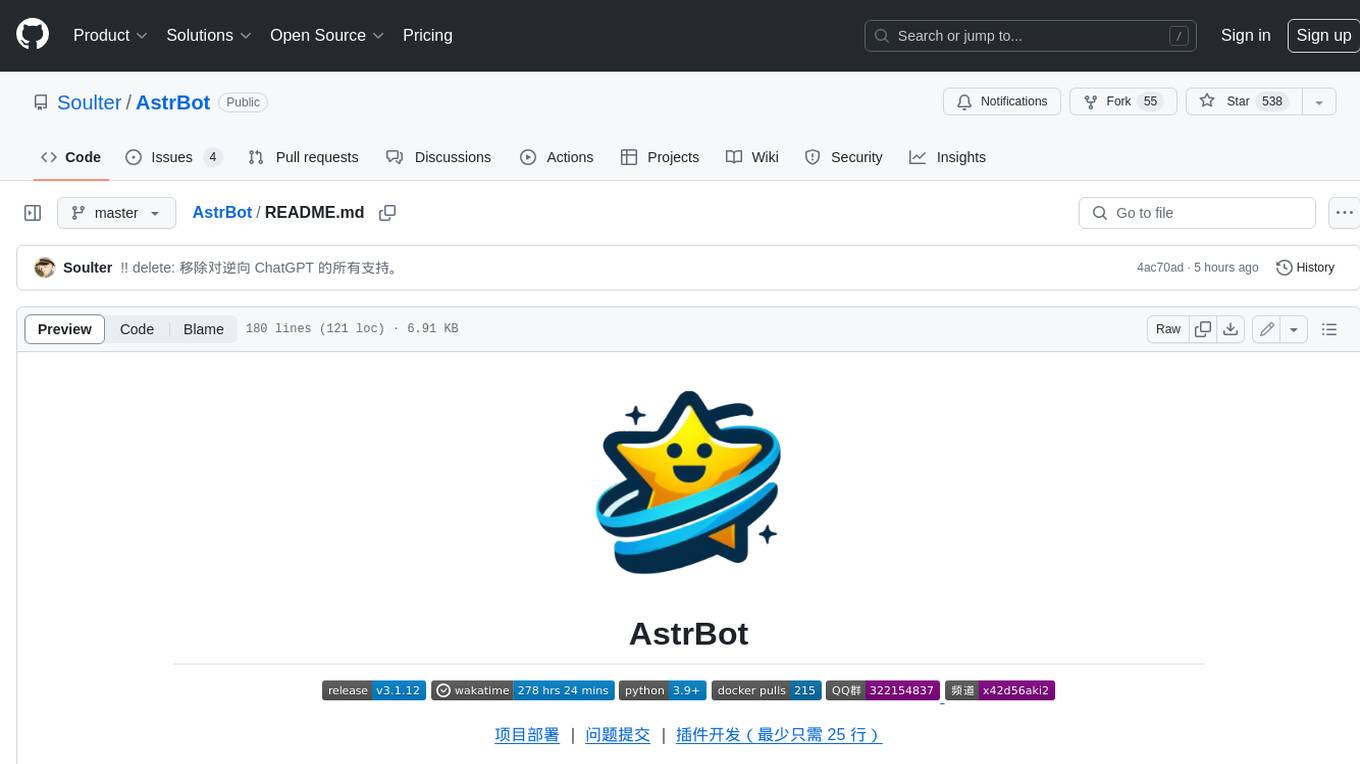
AstrBot
AstrBot is a powerful and versatile tool that leverages the capabilities of large language models (LLMs) like GPT-3, GPT-3.5, and GPT-4 to enhance communication and automate tasks. It seamlessly integrates with popular messaging platforms such as QQ, QQ Channel, and Telegram, enabling users to harness the power of AI within their daily conversations and workflows.
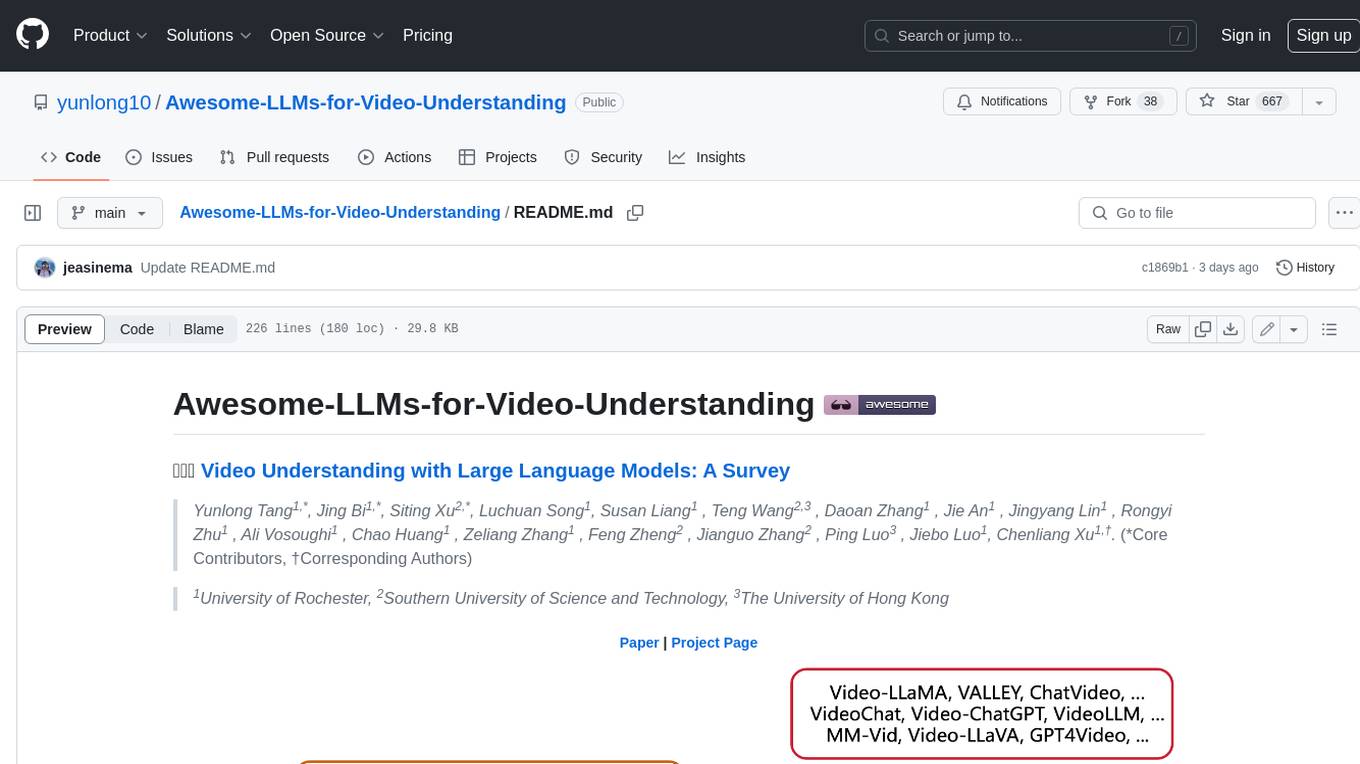
Awesome-LLMs-for-Video-Understanding
Awesome-LLMs-for-Video-Understanding is a repository dedicated to exploring Video Understanding with Large Language Models. It provides a comprehensive survey of the field, covering models, pretraining, instruction tuning, and hybrid methods. The repository also includes information on tasks, datasets, and benchmarks related to video understanding. Contributors are encouraged to add new papers, projects, and materials to enhance the repository.
For similar tasks
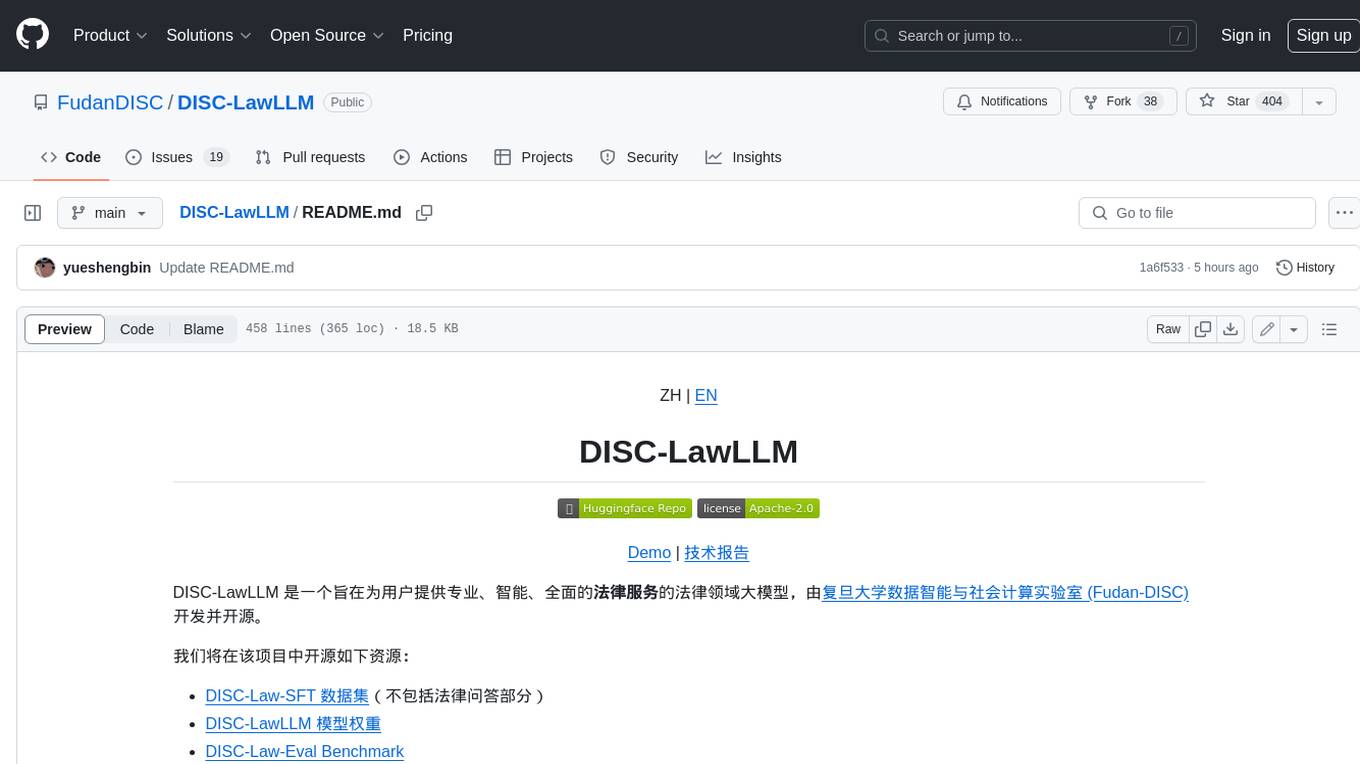
DISC-LawLLM
DISC-LawLLM is a legal domain large model that aims to provide professional, intelligent, and comprehensive **legal services** to users. It is developed and open-sourced by the Data Intelligence and Social Computing Lab (Fudan-DISC) at Fudan University.
For similar jobs
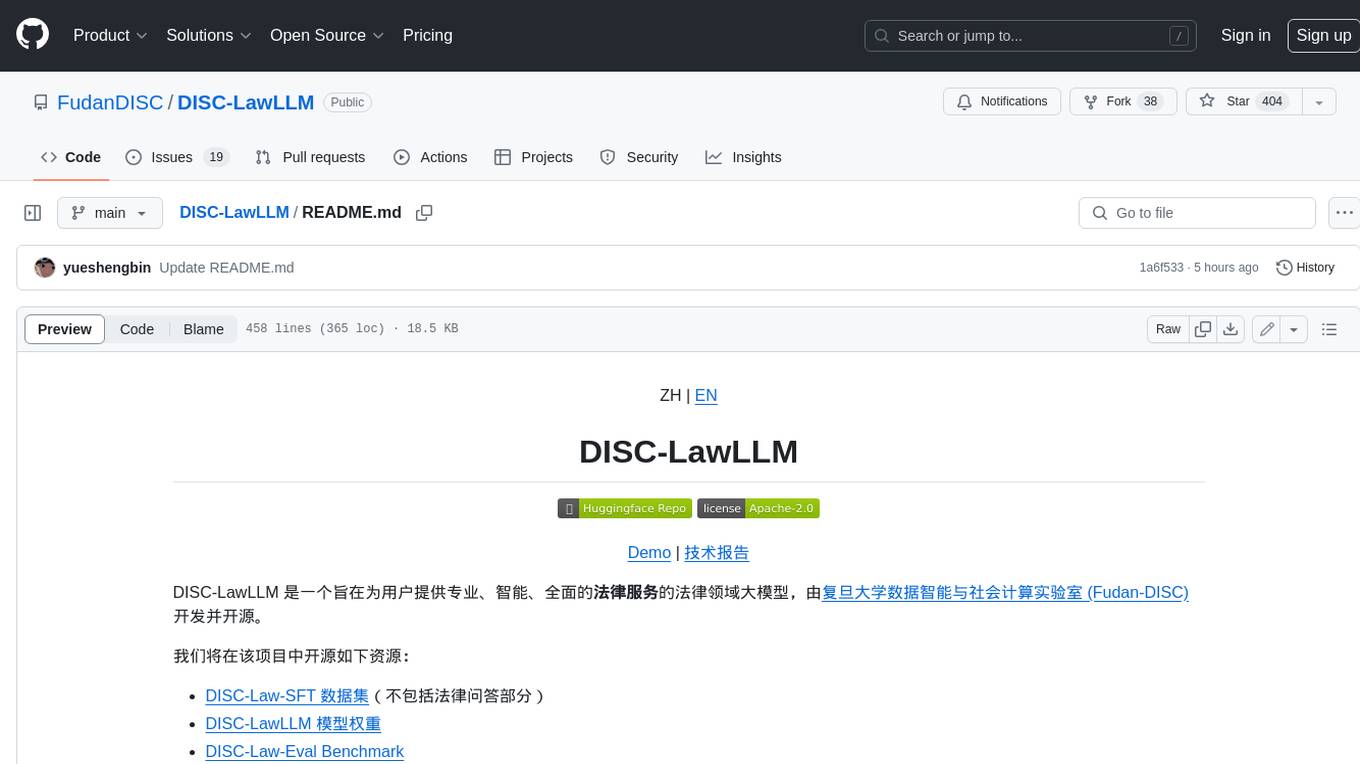
DISC-LawLLM
DISC-LawLLM is a legal domain large model that aims to provide professional, intelligent, and comprehensive **legal services** to users. It is developed and open-sourced by the Data Intelligence and Social Computing Lab (Fudan-DISC) at Fudan University.
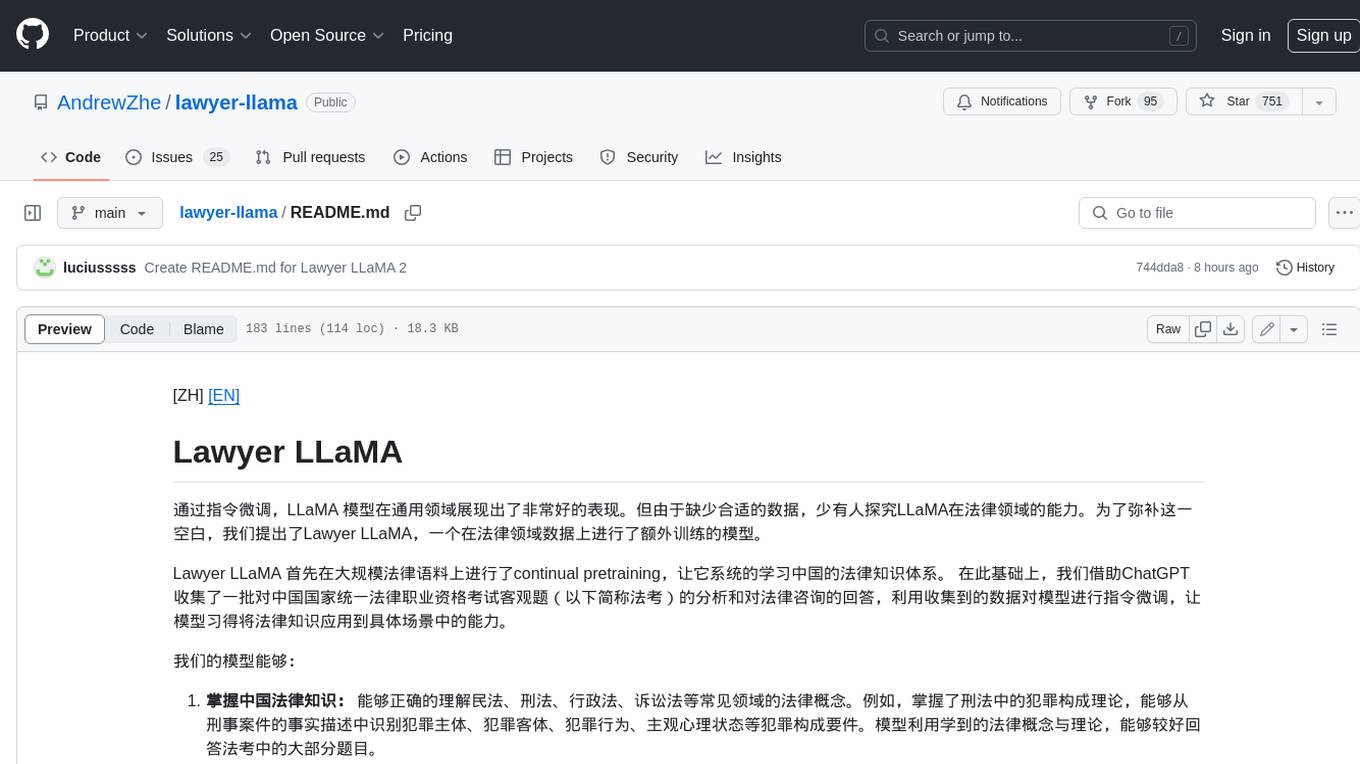
lawyer-llama
Lawyer LLaMA is a large language model that has been specifically trained on legal data, including Chinese laws, regulations, and case documents. It has been fine-tuned on a large dataset of legal questions and answers, enabling it to understand and respond to legal inquiries in a comprehensive and informative manner. Lawyer LLaMA is designed to assist legal professionals and individuals with a variety of law-related tasks, including: * **Legal research:** Quickly and efficiently search through vast amounts of legal information to find relevant laws, regulations, and case precedents. * **Legal analysis:** Analyze legal issues, identify potential legal risks, and provide insights on how to proceed. * **Document drafting:** Draft legal documents, such as contracts, pleadings, and legal opinions, with accuracy and precision. * **Legal advice:** Provide general legal advice and guidance on a wide range of legal matters, helping users understand their rights and options. Lawyer LLaMA is a powerful tool that can significantly enhance the efficiency and effectiveness of legal research, analysis, and decision-making. It is an invaluable resource for lawyers, paralegals, law students, and anyone else who needs to navigate the complexities of the legal system.
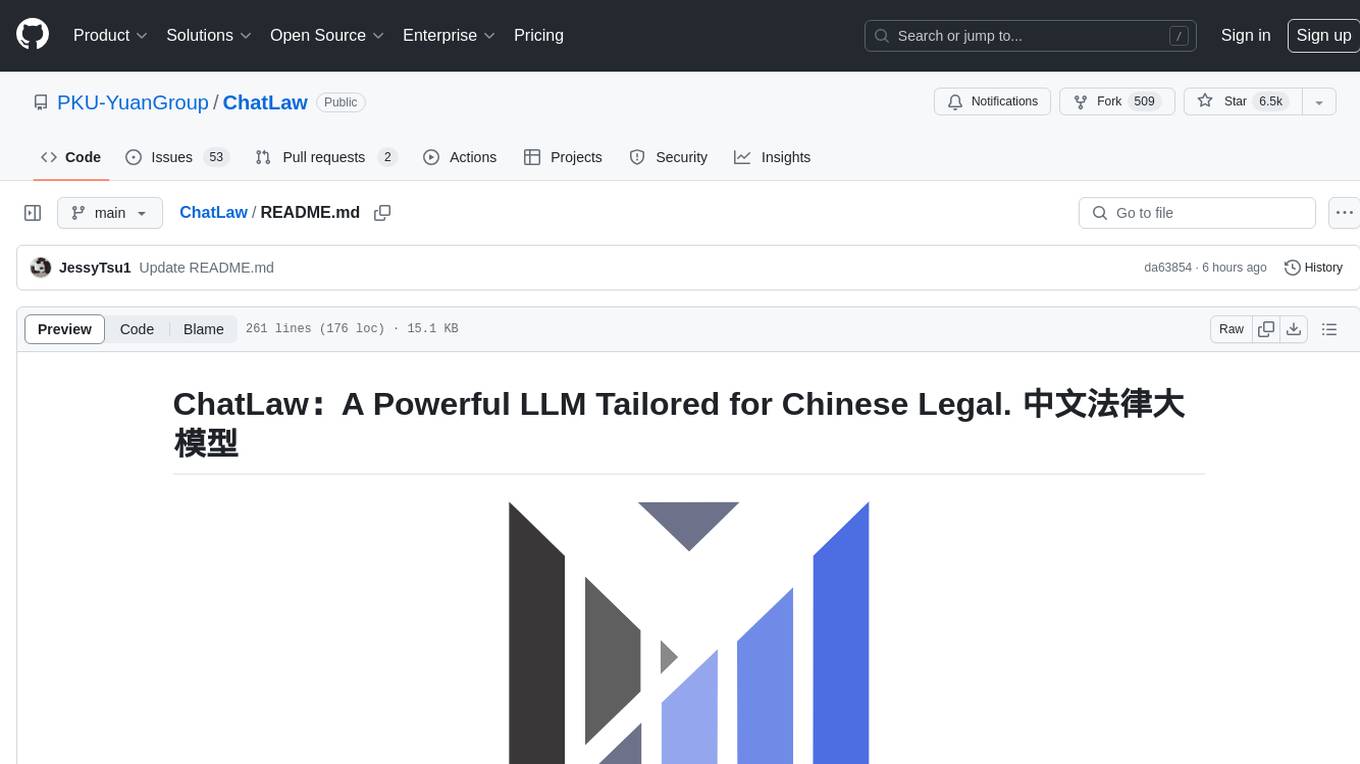
ChatLaw
ChatLaw is an open-source legal large language model tailored for Chinese legal scenarios. It aims to combine LLM and knowledge bases to provide solutions for legal scenarios. The models include ChatLaw-13B and ChatLaw-33B, trained on various legal texts to construct dialogue data. The project focuses on improving logical reasoning abilities and plans to train models with parameters exceeding 30B for better performance. The dataset consists of forum posts, news, legal texts, judicial interpretations, legal consultations, exam questions, and court judgments, cleaned and enhanced to create dialogue data. The tool is designed to assist in legal tasks requiring complex logical reasoning, with a focus on accuracy and reliability.
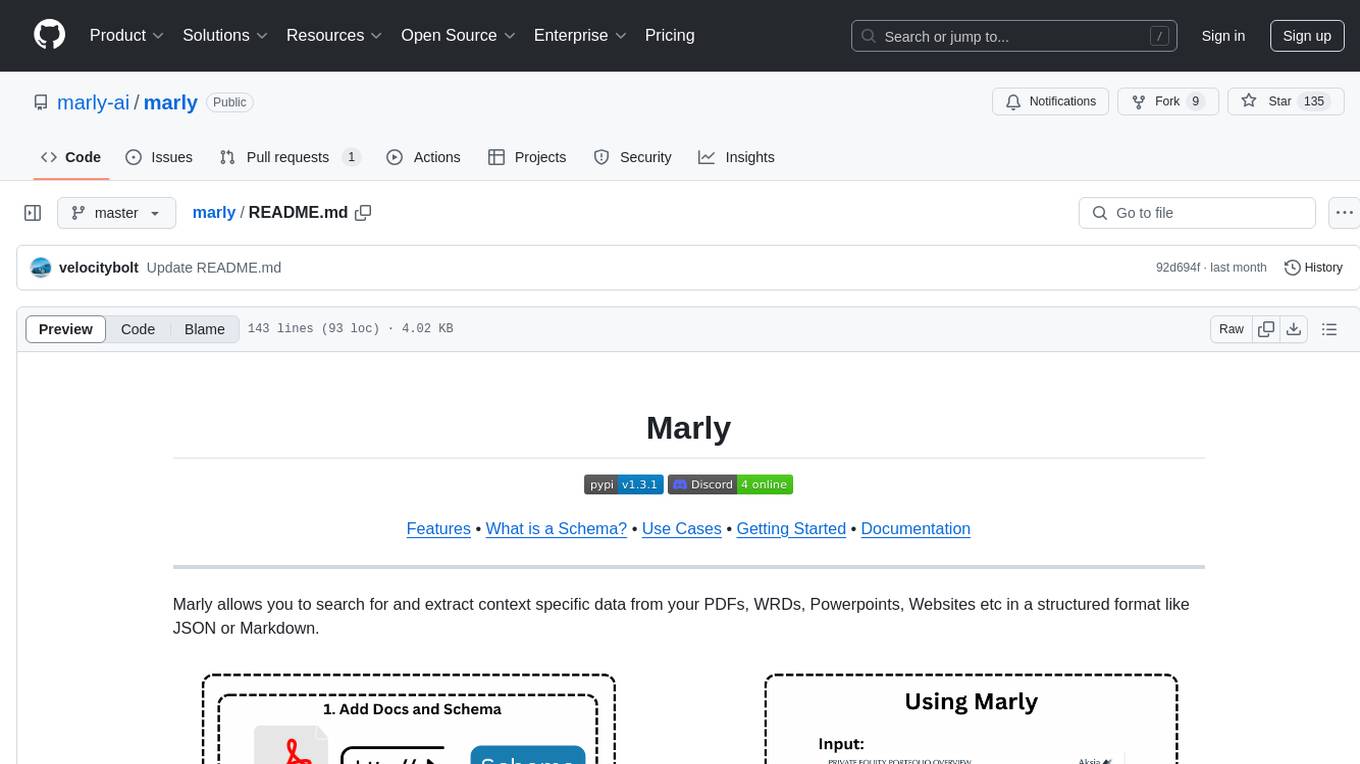
marly
Marly is a tool that allows users to search for and extract context-specific data from various types of documents such as PDFs, Word files, Powerpoints, and websites. It provides the ability to extract data in structured formats like JSON or Markdown, making it easy to integrate into workflows. Marly supports multi-schema and multi-document extraction, offers built-in caching for rapid repeat extractions, and ensures no vendor lock-in by allowing flexibility in choosing model providers.
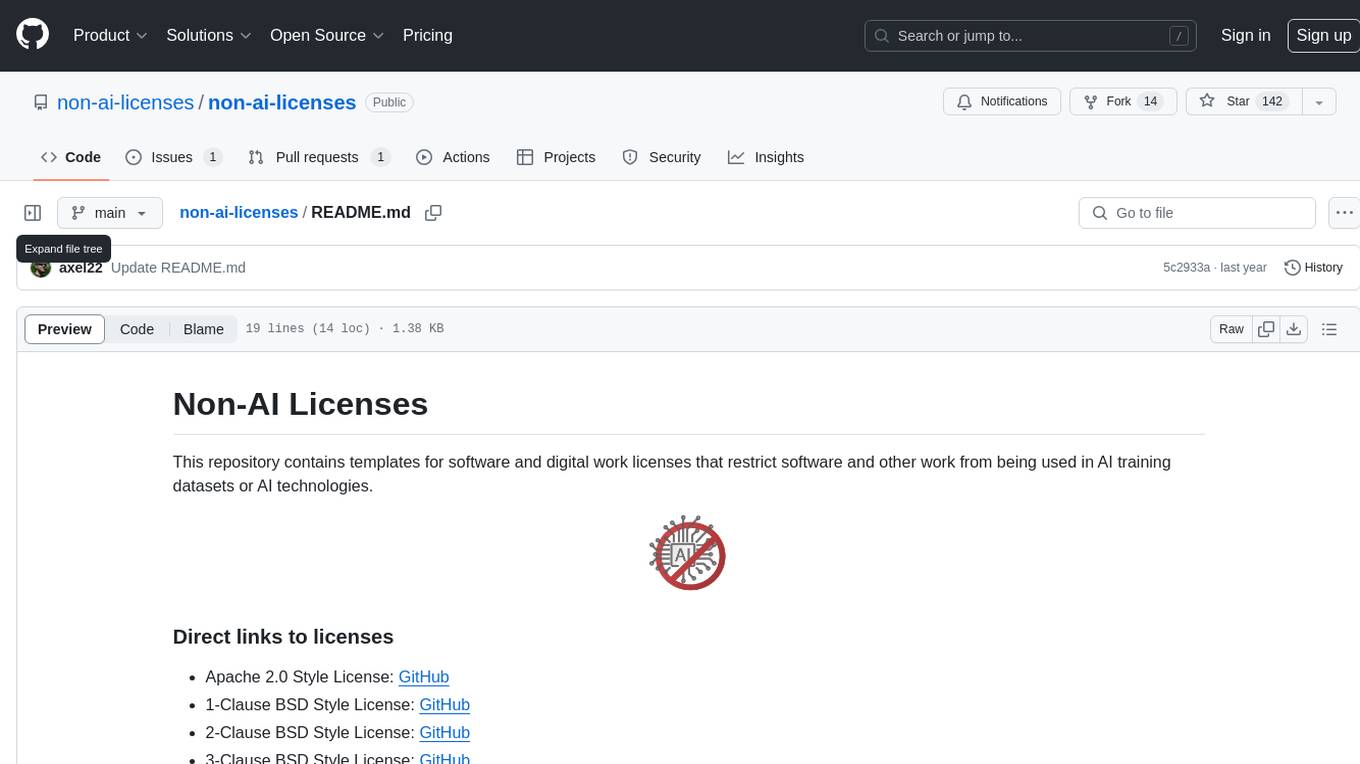
non-ai-licenses
This repository provides templates for software and digital work licenses that restrict usage in AI training datasets or AI technologies. It includes various license styles such as Apache, BSD, MIT, UPL, ISC, CC0, and MPL-2.0.
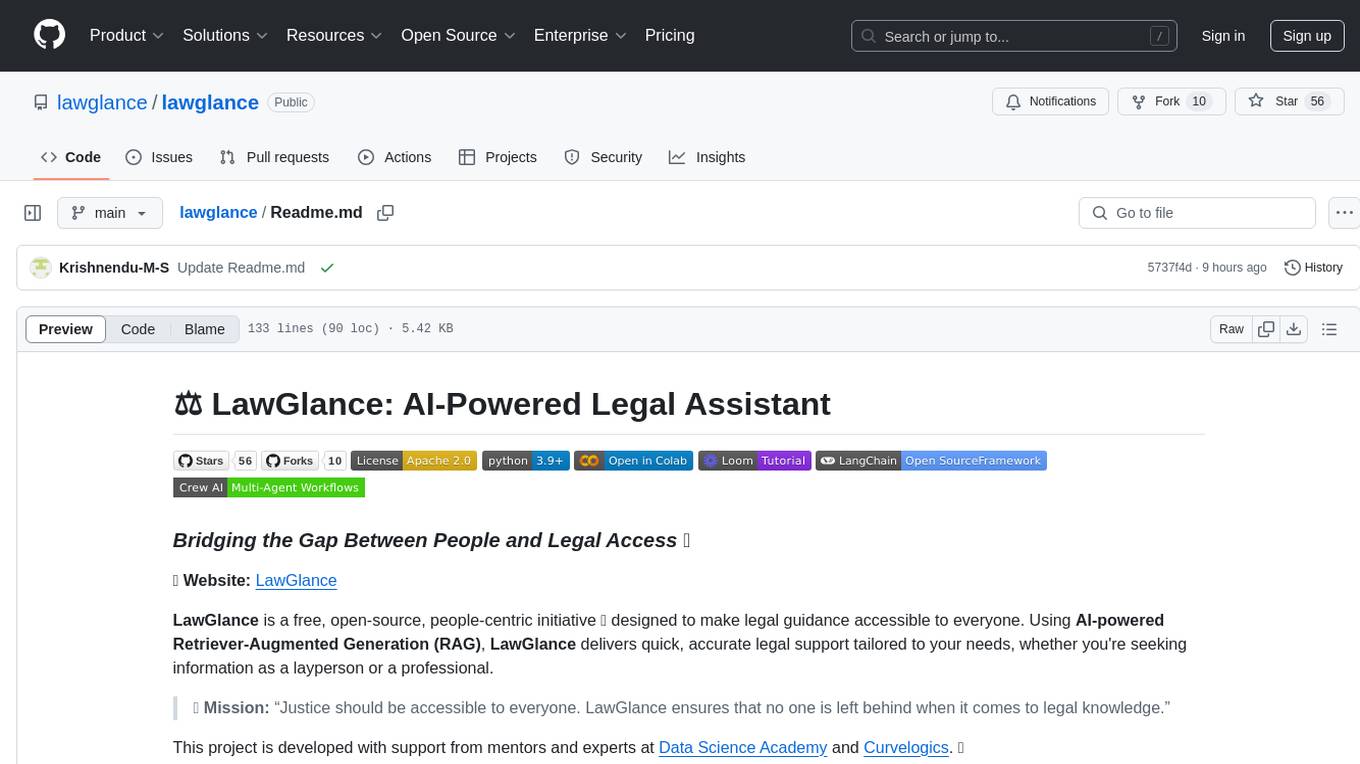
lawglance
LawGlance is an AI-powered legal assistant that aims to bridge the gap between people and legal access. It is a free, open-source initiative designed to provide quick and accurate legal support tailored to individual needs. The project covers various laws, with plans for international expansion in the future. LawGlance utilizes AI-powered Retriever-Augmented Generation (RAG) to deliver legal guidance accessible to both laypersons and professionals. The tool is developed with support from mentors and experts at Data Science Academy and Curvelogics.
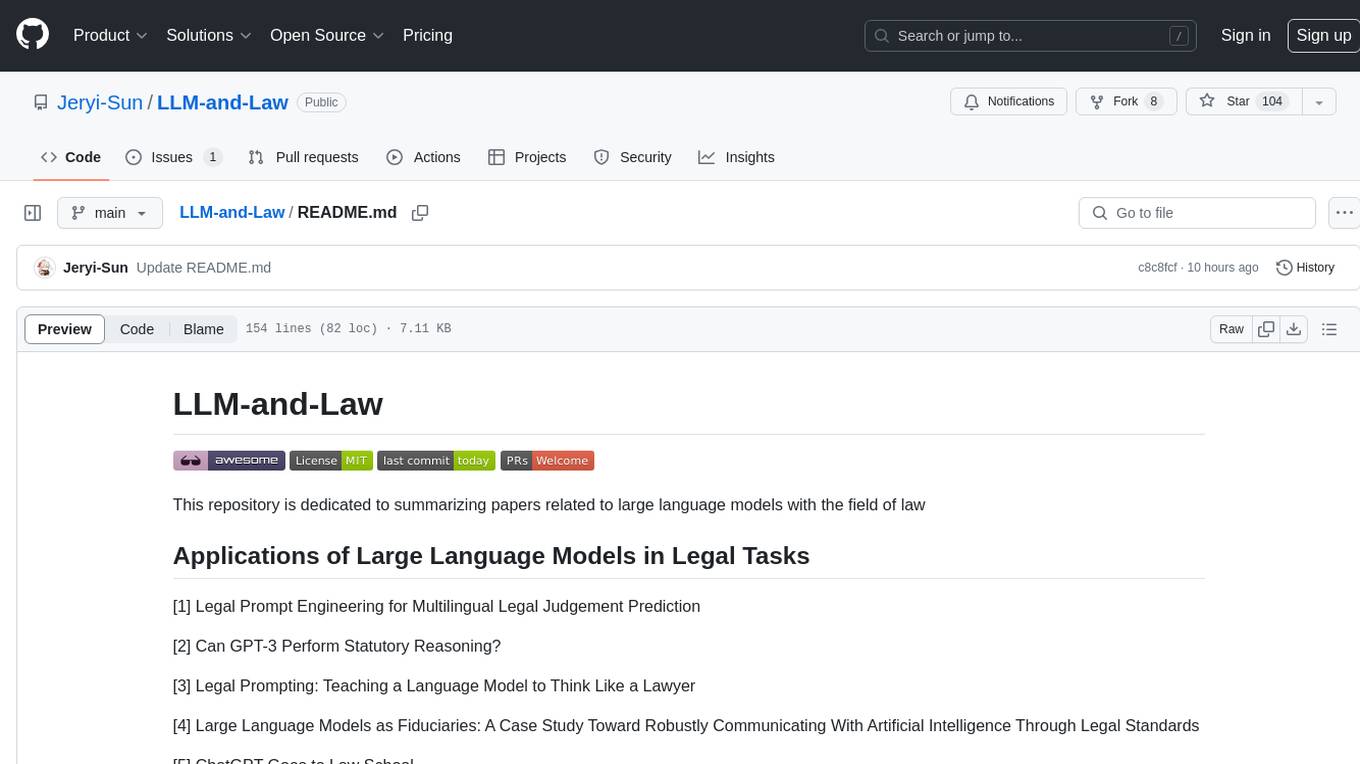
LLM-and-Law
This repository is dedicated to summarizing papers related to large language models with the field of law. It includes applications of large language models in legal tasks, legal agents, legal problems of large language models, data resources for large language models in law, law LLMs, and evaluation of large language models in the legal domain.
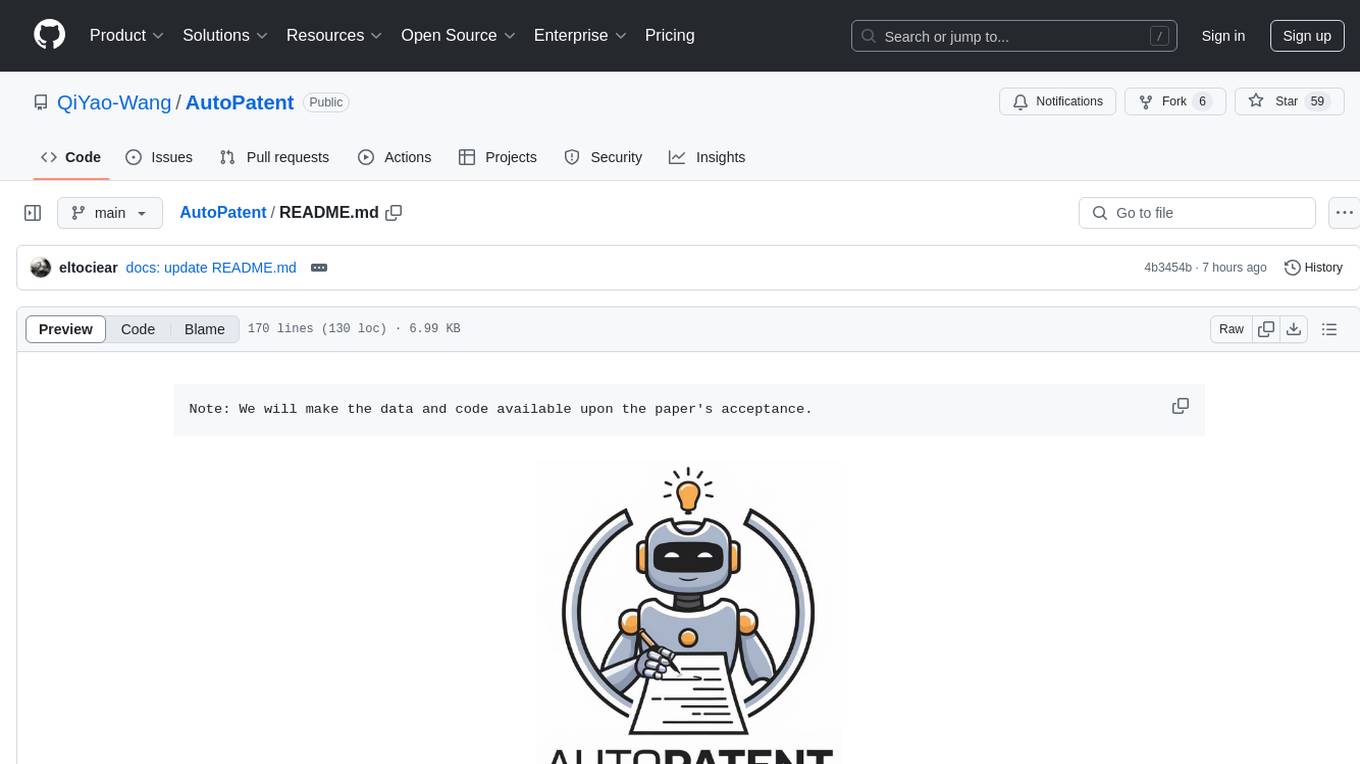
AutoPatent
AutoPatent is a multi-agent framework designed for automatic patent generation. It challenges large language models to generate full-length patents based on initial drafts. The framework leverages planner, writer, and examiner agents along with PGTree and RRAG to craft lengthy, intricate, and high-quality patent documents. It introduces a new metric, IRR (Inverse Repetition Rate), to measure sentence repetition within patents. The tool aims to streamline the patent generation process by automating the creation of detailed and specialized patent documents.