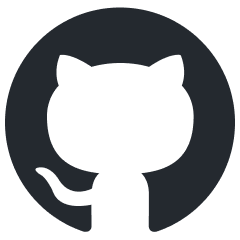
MedicalGPT
MedicalGPT: Training Your Own Medical GPT Model with ChatGPT Training Pipeline. 训练医疗大模型,实现了包括增量预训练(PT)、有监督微调(SFT)、RLHF、DPO、ORPO、GRPO。
Stars: 3616
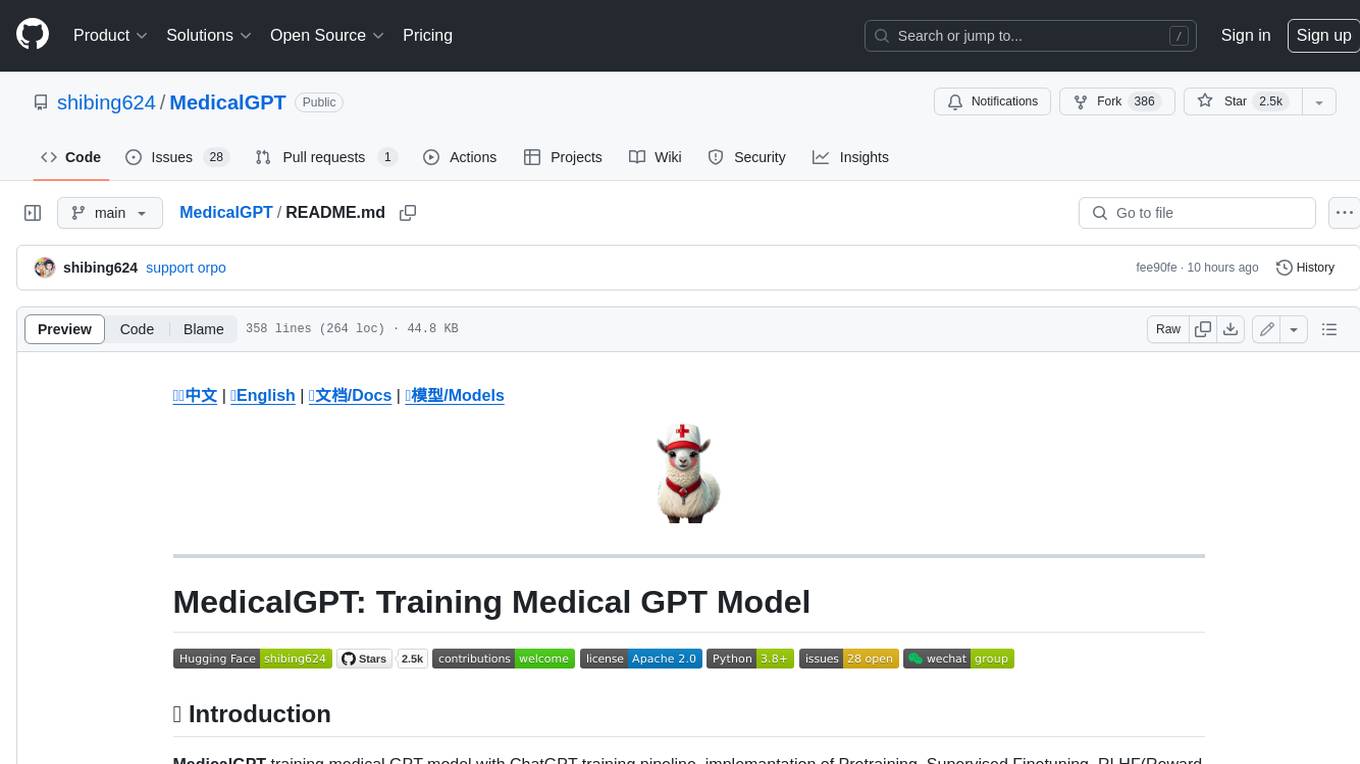
MedicalGPT is a training medical GPT model with ChatGPT training pipeline, implement of Pretraining, Supervised Finetuning, RLHF(Reward Modeling and Reinforcement Learning) and DPO(Direct Preference Optimization).
README:
🇨🇳中文 | 🌐English | 📖文档/Docs | 🤖模型/Models
MedicalGPT training medical GPT model with ChatGPT training pipeline, implemantation of Pretraining, Supervised Finetuning, RLHF(Reward Modeling and Reinforcement Learning) and DPO(Direct Preference Optimization).
MedicalGPT 训练医疗大模型,实现了包括增量预训练、有监督微调、RLHF(奖励建模、强化学习训练)和DPO(直接偏好优化)。
- RLHF training pipeline来自Andrej Karpathy的演讲PDF State of GPT,视频 Video
- DPO方法来自论文Direct Preference Optimization:Your Language Model is Secretly a Reward Model
- ORPO方法来自论文ORPO: Monolithic Preference Optimization without Reference Model
[2025/02/17] v2.4版本:新增GRPO训练方法,GRPO通过纯RL方法可以体验aha moment
,支持了 DeepSeek-R1 模型,详见Release-v2.4
[2024/09/21] v2.3版本:支持了 Qwen-2.5 系列模型,详见Release-v2.3
[2024/08/02] v2.2版本:支持了角色扮演模型训练,新增了医患对话SFT数据生成脚本role_play_data,详见Release-v2.2
展开日志
[2024/06/11] v2.1版本:支持了 Qwen-2 系列模型,详见Release-v2.1
[2024/04/24] v2.0版本:支持了 Llama-3 系列模型,详见Release-v2.0
[2024/04/17] v1.9版本:支持了 ORPO,详细用法请参照 run_orpo.sh
。详见Release-v1.9
[2024/01/26] v1.8版本:支持微调Mixtral混合专家MoE模型 Mixtral 8x7B。详见Release-v1.8
[2024/01/14] v1.7版本:新增检索增强生成(RAG)的基于文件问答ChatPDF功能,代码chatpdf.py
,可以基于微调后的LLM结合知识库文件问答提升行业问答准确率。详见Release-v1.7
[2023/10/23] v1.6版本:新增RoPE插值来扩展GPT模型的上下文长度;针对LLaMA模型支持了FlashAttention-2和LongLoRA 提出的 $S^2$-Attn;支持了NEFTune给embedding加噪训练方法。详见Release-v1.6
[2023/08/28] v1.5版本: 新增DPO(直接偏好优化)方法,DPO通过直接优化语言模型来实现对其行为的精确控制,可以有效学习到人类偏好。详见Release-v1.5
[2023/08/08] v1.4版本: 发布基于ShareGPT4数据集微调的中英文Vicuna-13B模型shibing624/vicuna-baichuan-13b-chat,和对应的LoRA模型shibing624/vicuna-baichuan-13b-chat-lora,详见Release-v1.4
[2023/08/02] v1.3版本: 新增LLaMA, LLaMA2, Bloom, ChatGLM, ChatGLM2, Baichuan模型的多轮对话微调训练;新增领域词表扩充功能;新增中文预训练数据集和中文ShareGPT微调训练集,详见Release-v1.3
[2023/07/13] v1.1版本: 发布中文医疗LLaMA-13B模型shibing624/ziya-llama-13b-medical-merged,基于Ziya-LLaMA-13B-v1模型,SFT微调了一版医疗模型,医疗问答效果有提升,发布微调后的完整模型权重,详见Release-v1.1
[2023/06/15] v1.0版本: 发布中文医疗LoRA模型shibing624/ziya-llama-13b-medical-lora,基于Ziya-LLaMA-13B-v1模型,SFT微调了一版医疗模型,医疗问答效果有提升,发布微调后的LoRA权重,详见Release-v1.0
[2023/06/05] v0.2版本: 以医疗为例,训练领域大模型,实现了四阶段训练:包括二次预训练、有监督微调、奖励建模、强化学习训练。详见Release-v0.2
基于ChatGPT Training Pipeline,本项目实现了领域模型--医疗行业语言大模型的训练:
- 第一阶段:PT(Continue PreTraining)增量预训练,在海量领域文档数据上二次预训练GPT模型,以适应领域数据分布(可选)
- 第二阶段:SFT(Supervised Fine-tuning)有监督微调,构造指令微调数据集,在预训练模型基础上做指令精调,以对齐指令意图,并注入领域知识
- 第三阶段
- RLHF(Reinforcement Learning from Human Feedback)基于人类反馈对语言模型进行强化学习,分为两步:
- RM(Reward Model)奖励模型建模,构造人类偏好排序数据集,训练奖励模型,用来建模人类偏好,主要是"HHH"原则,具体是"helpful, honest, harmless"
- RL(Reinforcement Learning)强化学习,用奖励模型来训练SFT模型,生成模型使用奖励或惩罚来更新其策略,以便生成更高质量、更符合人类偏好的文本
- DPO(Direct Preference Optimization)直接偏好优化方法,DPO通过直接优化语言模型来实现对其行为的精确控制,而无需使用复杂的强化学习,也可以有效学习到人类偏好,DPO相较于RLHF更容易实现且易于训练,效果更好
- ORPO比值比偏好优化,不需要参考模型(ref_model)的优化方法,通过ORPO,LLM可以同时学习SFT和对齐,将两个过程整合为单一步骤,缓解模型灾难性遗忘问题
- RLHF(Reinforcement Learning from Human Feedback)基于人类反馈对语言模型进行强化学习,分为两步:
Model | Base Model | Introduction |
---|---|---|
shibing624/ziya-llama-13b-medical-lora | IDEA-CCNL/Ziya-LLaMA-13B-v1 | 在240万条中英文医疗数据集shibing624/medical上SFT微调了一版Ziya-LLaMA-13B模型,医疗问答效果有提升,发布微调后的LoRA权重(单轮对话) |
shibing624/ziya-llama-13b-medical-merged | IDEA-CCNL/Ziya-LLaMA-13B-v1 | 在240万条中英文医疗数据集shibing624/medical上SFT微调了一版Ziya-LLaMA-13B模型,医疗问答效果有提升,发布微调后的完整模型权重(单轮对话) |
shibing624/vicuna-baichuan-13b-chat-lora | baichuan-inc/Baichuan-13B-Chat | 在10万条多语言ShareGPT GPT4多轮对话数据集shibing624/sharegpt_gpt4 和 医疗数据集shibing624/medical 上SFT微调了一版baichuan-13b-chat多轮问答模型,日常问答和医疗问答效果有提升,发布微调后的LoRA权重 |
shibing624/vicuna-baichuan-13b-chat | baichuan-inc/Baichuan-13B-Chat | 在10万条多语言ShareGPT GPT4多轮对话数据集shibing624/sharegpt_gpt4 和 医疗数据集shibing624/medical 上SFT微调了一版baichuan-13b-chat多轮问答模型,日常问答和医疗问答效果有提升,发布微调后的完整模型权重 |
shibing624/llama-3-8b-instruct-262k-chinese | Llama-3-8B-Instruct-262k | 在2万条中英文偏好数据集shibing624/DPO-En-Zh-20k-Preference上使用ORPO方法微调得到的超长文本多轮对话模型,适用于RAG、多轮对话 |
演示shibing624/vicuna-baichuan-13b-chat模型效果:
具体case见Inference Examples
我们提供了一个简洁的基于gradio的交互式web界面,启动服务后,可通过浏览器访问,输入问题,模型会返回答案。
启动服务,命令如下:
CUDA_VISIBLE_DEVICES=0 python gradio_demo.py --model_type base_model_type --base_model path_to_llama_hf_dir --lora_model path_to_lora_dir
参数说明:
-
--model_type {base_model_type}
:预训练模型类型,如llama、bloom、chatglm等 -
--base_model {base_model}
:存放HF格式的LLaMA模型权重和配置文件的目录,也可使用HF Model Hub模型调用名称 -
--lora_model {lora_model}
:LoRA文件所在目录,也可使用HF Model Hub模型调用名称。若lora权重已经合并到预训练模型,则删除--lora_model参数 -
--tokenizer_path {tokenizer_path}
:存放对应tokenizer的目录。若不提供此参数,则其默认值与--base_model相同 -
--template_name
:模板名称,如vicuna
、alpaca
等。若不提供此参数,则其默认值是vicuna -
--only_cpu
: 仅使用CPU进行推理 -
--resize_emb
:是否调整embedding大小,若不调整,则使用预训练模型的embedding大小,默认不调整
requirements.txt
会不时更新以适配最新功能,使用以下命令更新依赖:
git clone https://github.com/shibing624/MedicalGPT
cd MedicalGPT
pip install -r requirements.txt --upgrade
* 估算值
训练方法 | 精度 | 7B | 13B | 30B | 70B | 110B | 8x7B | 8x22B |
---|---|---|---|---|---|---|---|---|
全参数 | AMP(自动混合精度) | 120GB | 240GB | 600GB | 1200GB | 2000GB | 900GB | 2400GB |
全参数 | 16 | 60GB | 120GB | 300GB | 600GB | 900GB | 400GB | 1200GB |
LoRA | 16 | 16GB | 32GB | 64GB | 160GB | 240GB | 120GB | 320GB |
QLoRA | 8 | 10GB | 20GB | 40GB | 80GB | 140GB | 60GB | 160GB |
QLoRA | 4 | 6GB | 12GB | 24GB | 48GB | 72GB | 30GB | 96GB |
QLoRA | 2 | 4GB | 8GB | 16GB | 24GB | 48GB | 18GB | 48GB |
Training Stage:
Stage | Introduction | Python script | Shell script |
---|---|---|---|
Continue Pretraining | 增量预训练 | pretraining.py | run_pt.sh |
Supervised Fine-tuning | 有监督微调 | supervised_finetuning.py | run_sft.sh |
Direct Preference Optimization | 直接偏好优化 | dpo_training.py | run_dpo.sh |
Reward Modeling | 奖励模型建模 | reward_modeling.py | run_rm.sh |
Reinforcement Learning | 强化学习 | ppo_training.py | run_ppo.sh |
ORPO | 概率偏好优化 | orpo_training.py | run_orpo.sh |
- 提供完整PT+SFT+DPO全阶段串起来训练的pipeline:run_training_dpo_pipeline.ipynb ,其对应的colab:
,运行完大概需要15分钟
- 提供完整PT+SFT+RLHF全阶段串起来训练的pipeline:run_training_ppo_pipeline.ipynb ,其对应的colab:
,运行完大概需要20分钟
- 提供基于知识库文件的LLM问答功能(RAG):chatpdf.py
- 训练参数说明 | 训练参数说明wiki
- 数据集 | 数据集wiki
- 扩充词表 | 扩充词表wiki
- FAQ | FAQ_wiki
Model Name | Model Size | Target Modules | Template |
---|---|---|---|
Baichuan | 7B/13B | W_pack | baichuan |
Baichuan2 | 7B/13B | W_pack | baichuan2 |
BLOOMZ | 560M/1.1B/1.7B/3B/7.1B/176B | query_key_value | vicuna |
ChatGLM | 6B | query_key_value | chatglm |
ChatGLM2 | 6B | query_key_value | chatglm2 |
ChatGLM3 | 6B | query_key_value | chatglm3 |
Cohere | 104B | q_proj,v_proj | cohere |
DeepSeek | 7B/16B/67B | q_proj,v_proj | deepseek |
DeepSeek3 | 671B | q_proj,v_proj | deepseek3 |
InternLM2 | 7B/20B | wqkv | intern2 |
LLaMA | 7B/13B/33B/65B | q_proj,v_proj | alpaca |
LLaMA2 | 7B/13B/70B | q_proj,v_proj | llama2 |
LLaMA3 | 8B/70B | q_proj,v_proj | llama3 |
Mistral | 7B/8x7B | q_proj,v_proj | mistral |
Orion | 14B | q_proj,v_proj | orion |
Qwen | 1.8B/7B/14B/72B | c_attn | qwen |
Qwen1.5 | 0.5B/1.8B/4B/14B/32B/72B/110B | q_proj,v_proj | qwen |
Qwen2 | 0.5B/1.5B/7B/72B | q_proj,v_proj | qwen |
Qwen2.5 | 0.5B/1.8B/4B/14B/72B | q_proj,v_proj | qwen |
XVERSE | 13B | query_key_value | xverse |
Yi | 6B/34B | q_proj,v_proj | yi |
训练完成后,现在我们加载训练好的模型,验证模型生成文本的效果。
CUDA_VISIBLE_DEVICES=0 python inference.py \
--model_type base_model_type \
--base_model path_to_model_hf_dir \
--tokenizer_path path_to_model_hf_dir \
--lora_model path_to_lora \
--interactive
参数说明:
-
--model_type {base_model_type}
:预训练模型类型,如llama、bloom、chatglm等 -
--base_model {base_model}
:存放HF格式的LLaMA模型权重和配置文件的目录 -
--tokenizer_path {base_model}
:存放HF格式的LLaMA模型权重和配置文件的目录 -
--lora_model {lora_model}
:LoRA解压后文件所在目录,也可使用HF Model Hub模型调用名称。如果已经合并了LoRA权重到预训练模型,则可以不提供此参数 -
--tokenizer_path {tokenizer_path}
:存放对应tokenizer的目录。若不提供此参数,则其默认值与--base_model相同 -
--template_name
:模板名称,如vicuna
、alpaca
等。若不提供此参数,则其默认值是vicuna -
--interactive
:以交互方式启动多轮问答,使用流式推理 -
--data_file {file_name}
:非交互方式启动下,读取file_name中的的内容进行batch预测 -
--output_file {file_name}
:非交互式方式下,将预测的结果以jsonl格式写入file_name -
--resize_emb
:是否调整embedding大小,若不调整,则使用预训练模型的embedding大小,默认不调整 -
--only_cpu
:仅使用CPU进行推理 -
--gpus {gpu_ids}
:指定使用的GPU设备编号,默认为0。如使用多张GPU,以逗号分隔,如0,1,2
多卡数据并行,batch推理
CUDA_VISIBLE_DEVICES=0,1 torchrun --nproc_per_node 2 inference_multigpu_demo.py --model_type baichuan --base_model shibing624/vicuna-baichuan-13b-chat
bash run_Multi-GPUs-deployment.sh
shibing624/vicuna-baichuan-13b-chat inference examples:
Model output case
Human | Assitant |
---|---|
小孩发烧怎么办 | 发烧是身体对感染或其他疾病的自然反应。以下是一些可以帮助缓解发烧症状的建议: 1. 保持身体水分充足:发烧时,身体会失去水分,因此需要保持水分充足。 2. 使用冰袋:将冰袋放在额头或颈部可以帮助降低体温。 3. 使用退烧药:退烧药可以帮助降低体温。 4. 保持身体温度:保持身体温度可以帮助降低体温。 5. 使用冷水或温水擦拭身体:使用冷水或温水擦拭身体可以帮助降低体温。 6. 使用退烧药:退烧药可以帮助降低体温。 7. 保持身体温度:保持身体温度可以帮助降低体温。 8. 使用冷水或温水擦拭身体:使用冷水或温水擦拭身体可以帮助降低体温。 9. 使用退烧药:退烧药可以帮助降低体温。 10. 保持身体温度:保持身体温度可以帮助降低体温。 |
一岁宝宝发烧能吃啥药? | 1岁宝宝发烧时,可以考虑以下几种药物: 1. 退烧药:如布洛芬(Ibuprofen)或对乙酰氨基酚(Paracetamol)。这些药物可以帮助降低体温,缓解发烧症状。 2. 抗生素:如果发烧是由细菌感染引起的,可以考虑使用抗生素。但请务必在医生的建议下使用。 3. 抗病毒药物:如果发烧是由病毒感染引起的,可以考虑使用抗病毒药物。但请务必在医生的建议下使用。 4. 解热镇痛药:如阿司匹林(Aspirin)或对乙酰氨基酚(Paracetamol)。这些药物可以帮助缓解发烧症状。 5. 抗组胺药:如苯海拉明(Diphenhydramine)或非索非那定(Naproxen)。这些药物可以帮助缓解发烧症状。 请务必在医生的建议下使用这些药物,并遵循药物说明书上的用量和用法。同时,保持宝宝充足的水分摄入,并确保宝宝有足够的休息。 |
肛门病变可能是什么疾病的症状 | 肛门病变可能由多种原因引起,包括感染、炎症、肿瘤或其他疾病。一些常见的肛门病变包括: 1. 肛裂:这是一种肛门皮肤撕裂,通常由便秘或其他压力因素引起。 2. 肛瘘:这是一种肛门感染,导致肛门皮肤和直肠之间形成瘘管。 3. 肛门疣:这是一种肛门皮肤上形成的疣状病变,通常由人类乳头状瘤病毒(HPV)引起。 4. 肛门脓肿:这是一种肛门感染,导致肛门皮肤下形成脓肿。 5. 肛门肿瘤:这是一种肛门皮肤或直肠内形成的肿瘤。 6. 肛门溃疡:这是一种肛门皮肤上形成的溃疡,通常由感染或炎症引起。 7. 肛门瘙痒:这是一种肛门皮肤瘙痒的症状,可能由感染、炎症或其他疾病引起。 如果您有肛门病变的症状,请咨询医疗保健专业人员以获得适当的诊断和治疗。 |
医生,您好白带增多泛黄怎么回事? | 您好,白带增多和发黄可能是由多种原因引起的,包括感染、荷尔蒙变化、阴道干燥或其他妇科问题。以下是一些可能的原因: 1. 感染:白带增多和发黄可能是由阴道或尿道感染引起的。感染可能由细菌、病毒或真菌引起。 2. 荷尔蒙变化:荷尔蒙变化,如月经周期或更年期,可能会导致白带增多和发黄。 3. 阴道干燥:阴道干燥可能会导致白带增多和发黄。 4. 其他妇科问题:其他妇科问题,如子宫颈炎、子宫内膜炎或子宫内膜异位症,也可能导致白带增多和发黄。 如果您担心自己的白带,建议您咨询妇科医生进行检查和诊断。医生可以根据您的症状和检查结果确定原因并提供适当的治疗。 |
药流后有巧克力白带是没流干净吗 | 药流后出现巧克力白带并不一定意味着没有流干净。巧克力白带是由于子宫内膜脱落和出血引起的,通常在药流后的一段时间内会出现。 药流后出现巧克力白带的原因可能包括: 1. 子宫内膜脱落:药流后,子宫内膜会脱落,导致出血。这种出血可能表现为巧克力白带。 2. 感染:药流后,子宫内膜和宫颈口可能存在创伤,容易受到细菌感染。感染可能导致巧克力白带。 3. 激素水平变化:药流后,激素水平可能发生变化,导致巧克力白带。 如果巧克力白带持续时间较长,或者伴有其他症状,如腹痛、发热、分泌物异味等,建议及时就诊,进行相关检查,如妇科检查、B超等,以确定是否存在感染或其他问题。在医生的指导下进行相应的治疗。 |
维胺酯维E乳膏能治理什么疾病 | 维胺酯维E乳膏是一种外用药物,主要用于治疗痤疮、脂溢性皮炎等皮肤病。它可以帮助减少皮肤油脂分泌,减轻炎症,促进皮肤修复。 需要注意的是,维胺酯维E乳膏并非万能药,对于某些皮肤病可能并不适用。在使用前,建议咨询医生或药师,了解药物的适应症、禁忌症、用法用量等信息。同时,使用药物时应遵循医嘱,避免自行滥用。 |
痤疮是什么原因导致的? | 痤疮,也称为粉刺,是由皮肤毛孔堵塞引起的。堵塞毛孔的原因有很多,包括: 1. 皮脂腺过度活跃:皮脂腺产生过多油脂,导致毛孔堵塞。 2. 毛孔堵塞:皮肤表面的死皮细胞、油脂和细菌堵塞毛孔。 3. 炎症:痤疮通常伴随着炎症,这可能是由于皮肤感染或免疫系统对痤疮的反应引起的。 4. 遗传因素:某些人可能更容易患痤疮,这可能是由于遗传因素引起的。 5. 荷尔蒙变化:痤疮在青春期和月经期间更为常见,这可能是由于荷尔蒙变化引起的。 6. 生活方式因素:某些生活方式因素,如压力、不良饮食和缺乏运动,也可能导致痤疮。 7. 化妆品和护肤品:某些化妆品和护肤品可能导致痤疮,特别是那些含有刺激性成分的产品。 8. 药物:某些药物,如口服避孕药,也可能导致痤疮。 要预防痤疮,可以采取一些措施,如保持皮肤清洁、使用温和的护肤品、避免过度使用化妆品和护肤品、保持健康的生活方式和饮食习惯、避免压力和荷尔蒙变化。如果痤疮严重,可能需要寻求皮肤科医生的帮助。 |
脸上长青春痘怎么办,能吃啥药,需要注意啥 | 青春痘,又称痤疮,是一种常见的皮肤病。青春痘的治疗需要综合考虑饮食、生活习惯、药物等多个方面。以下是一些建议: 1. 饮食: * 避免高糖、高脂、高油的食物,如快餐、甜点、油炸食品等。 * 多吃新鲜蔬菜、水果、全谷类食物,以增加纤维摄入,促进肠道蠕动。 * 避免摄入过多的咖啡因、酒精、辛辣食物等刺激性食物。 2. 生活习惯: * 保持良好的作息时间,保证充足的睡眠。 * 避免长时间使用电脑、手机等电子产品,以免辐射对皮肤造成刺激。 * 保持皮肤清洁,每天早晚使用温和的洁面产品清洁皮肤。 * 避免挤压青春痘,以免感染和留下疤痕。 3. 药物: * 外用药物:可以使用含有水杨酸、苯甲酸、壬二酸等成分的护肤品,帮助去除皮肤表面的角质,减少痘痘的形成。 * 口服药物:如果青春痘严重,可以考虑口服抗生素、维生素B族、锌等药物,但需在医生指导下使用。 4. 注意: * 不要随意使用化妆品、护肤品,以免刺激皮肤。 * 不要过度清洁皮肤,以免破坏皮肤屏障。 * 保持心情舒畅,避免焦虑、抑郁等负面情绪。 总之,青春痘的治疗需要综合考虑饮食、生活习惯、药物等多个方面。在治疗过程中,要保持耐心和信心,遵循医生的建议,逐步改善皮肤状况。 |
由于输精管堵塞会不会导致男性不育?,医生您好,我同事这几天不开心,我问她怎么了,她说她老公输精管堵塞,所以一直没孩子,不是不想要,请问输精管堵塞会不会导致男性不育? | 输精管堵塞可能会导致男性不育。输精管是连接睾丸和尿道的一条管道,负责将精子从睾丸输送到尿道。如果输精管堵塞,精子就无法通过输精管到达尿道,从而导致不育。 输精管堵塞的原因有很多,包括先天性发育异常、炎症、肿瘤、外伤等。输精管堵塞的治疗方法包括手术疏通、药物治疗等。如果输精管堵塞严重,可能需要进行手术疏通。 需要注意的是,输精管堵塞并不一定导致不育,因为还有其他途径可以将精子输送到女性体内。例如,精子可以通过附睾、前列腺等组织到达女性体内。因此,如果输精管堵塞,建议及时就医,寻求专业医生的帮助。 |
心肌梗塞能治疗吗? | 心肌梗死是一种严重的疾病,如果及时治疗,可以挽救生命并防止长期残疾。治疗心肌梗死的关键是尽早识别和治疗。 心肌梗死的治疗通常包括以下步骤: 1. 立即就医:如果出现胸痛、呼吸急促或晕厥等症状,立即就医。 2. 药物治疗:医生可能会开处方药物,如硝酸甘油或阿司匹林,以帮助缓解症状并防止进一步的损伤。 3. 溶栓治疗:如果患者在到达医院前出现心肌梗死,医生可能会进行溶栓治疗,通过注射溶解血栓的药物来帮助恢复血流。 4. 手术治疗:如果药物治疗或溶栓治疗无效,医生可能会建议进行手术,如冠状动脉搭桥手术或心脏支架植入术。 5. 康复和预防:心肌梗死的治疗通常包括康复计划,以帮助患者恢复身体功能并预防未来的心脏事件。此外,患者还应遵循医生的建议,以降低患心脏病的风险。 需要注意的是,心肌梗死的治疗取决于患者的具体情况和症状。因此,如果您或您认识的人出现心肌梗死症状,请立即就医。 |
怎么让自己精力充沛,列5点建议 | 1. 保持充足的睡眠:睡眠对于保持精力充沛至关重要。确保每晚获得7-9小时的高质量睡眠。 2. 均衡饮食:均衡饮食对于保持能量水平至关重要。确保摄入足够的蛋白质、碳水化合物和健康脂肪。 3. 定期锻炼:定期锻炼可以帮助提高能量水平。尝试每天进行至少30分钟的有氧运动,如散步、跑步或骑自行车。 4. 管理压力:压力会消耗能量,因此管理压力对于保持精力充沛至关重要。尝试找到放松和减压的方法,如冥想、瑜伽或深呼吸练习。 5. 保持水分:脱水会降低能量水平。确保每天喝足够的水,并根据需要补充水分。 |
写个春天的诗 | 春天, 是大地苏醒的季节, 是万物复苏的季节, 是生命绽放的季节。 春天, 是阳光明媚的季节, 是鸟语花香的季节, 是绿意盎然的季节, 是希望无限的季节。 春天, 是人们欢笑的季节, 是孩子们嬉戏的季节, 是恋人们相拥的季节, 是人们追求梦想的季节。 |
南极洲为啥没有北极熊? | 南极洲没有北极熊,因为北极熊生活在北极地区,而南极洲位于南半球,气候和环境与北极地区有很大不同。南极洲的气候非常寒冷,常年被冰雪覆盖,而北极熊则生活在北极地区,那里有丰富的海洋生物和陆地哺乳动物。此外,南极洲的生态系统也与北极地区不同,没有北极熊所需的生存条件。 |
- 240万条中文医疗数据集(包括预训练、指令微调和奖励数据集):shibing624/medical
- 22万条中文医疗对话数据集(华佗项目):shibing624/huatuo_medical_qa_sharegpt 【本项目支持格式】
- 16GB中英文无监督、平行语料Linly-AI/Chinese-pretraining-dataset
- 524MB中文维基百科语料wikipedia-cn-20230720-filtered
- 10万条多语言ShareGPT GPT4多轮对话数据集:shibing624/sharegpt_gpt4 【本项目支持格式】
- 9万条英文ShareGPT多轮对话数集:anon8231489123/ShareGPT_Vicuna_unfiltered 【本项目支持格式】
- 50万条中文ChatGPT指令Belle数据集:BelleGroup/train_0.5M_CN
- 100万条中文ChatGPT指令Belle数据集:BelleGroup/train_1M_CN
- 5万条英文ChatGPT指令Alpaca数据集:50k English Stanford Alpaca dataset
- 2万条中文ChatGPT指令Alpaca数据集:shibing624/alpaca-zh
- 69万条中文指令Guanaco数据集(Belle50万条+Guanaco19万条):Chinese-Vicuna/guanaco_belle_merge_v1.0
- 5万条英文ChatGPT多轮对话数据集:RyokoAI/ShareGPT52K
- 80万条中文ChatGPT多轮对话数据集:BelleGroup/multiturn_chat_0.8M
- 116万条中文ChatGPT多轮对话数据集:fnlp/moss-002-sft-data
- 3.8万条中文ShareGPT多轮对话数据集:FreedomIntelligence/ShareGPT-CN
- 130万条中文微调数据集(汇总):zhuangxialie/Llama3-Chinese-Dataset 【本项目支持格式】
- 7千条中文角色扮演多轮对话数据集:shibing624/roleplay-zh-sharegpt-gpt4-data 【本项目支持格式】
- 2万条中英文偏好数据集:shibing624/DPO-En-Zh-20k-Preference 【本项目支持格式】
- 原版的oasst1数据集:OpenAssistant/oasst1
- 2万条多语言oasst1的reward数据集:tasksource/oasst1_pairwise_rlhf_reward
- 11万条英文hh-rlhf的reward数据集:Dahoas/full-hh-rlhf
- 9万条英文reward数据集(来自Anthropic's Helpful Harmless dataset):Dahoas/static-hh
- 7万条英文reward数据集(来源同上):Dahoas/rm-static
- 7万条繁体中文的reward数据集(翻译自rm-static)liswei/rm-static-m2m100-zh
- 7万条英文Reward数据集:yitingxie/rlhf-reward-datasets
- 3千条中文知乎问答偏好数据集:liyucheng/zhihu_rlhf_3k
- Issue(建议)
:
- 邮件我:xuming: [email protected]
- 微信我: 加我微信号:xuming624, 备注:姓名-公司名-NLP 进NLP交流群(加我拉你进群)。
本项目仅可应用于研究目的,项目开发者不承担任何因使用本项目(包含但不限于数据、模型、代码等)导致的危害或损失。详细请参考免责声明。
MedicalGPT项目代码的授权协议为 The Apache License 2.0,代码可免费用做商业用途,模型权重和数据只能用于研究目的。请在产品说明中附加MedicalGPT的链接和授权协议。
如果你在研究中使用了MedicalGPT,请按如下格式引用:
@misc{MedicalGPT,
title={MedicalGPT: Training Medical GPT Model},
author={Ming Xu},
year={2023},
howpublished={\url{https://github.com/shibing624/MedicalGPT}},
}
项目代码还很粗糙,如果大家对代码有所改进,欢迎提交回本项目,在提交之前,注意以下两点:
- 在
tests
添加相应的单元测试 - 使用
python -m pytest
来运行所有单元测试,确保所有单测都是通过的
之后即可提交PR。
- Direct Preference Optimization:Your Language Model is Secretly a Reward Model
- tloen/alpaca-lora
- ymcui/Chinese-LLaMA-Alpaca
- hiyouga/LLaMA-Factory
- dvlab-research/LongLoRA
Thanks for their great work!
- shibing624/ChatPilot:给 LLM Agent(包括RAG、在线搜索、Code interpreter) 提供一个简单好用的Web UI界面
For Tasks:
Click tags to check more tools for each tasksFor Jobs:
Alternative AI tools for MedicalGPT
Similar Open Source Tools
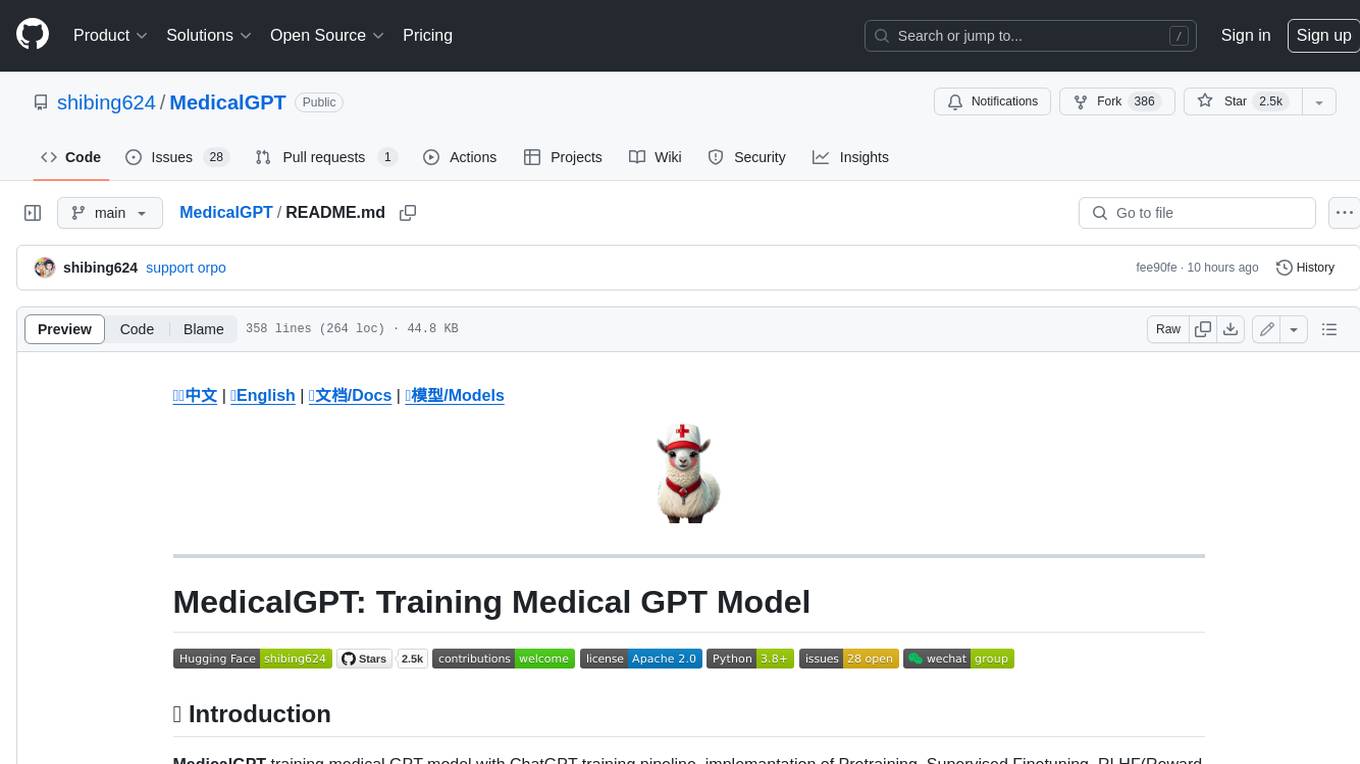
MedicalGPT
MedicalGPT is a training medical GPT model with ChatGPT training pipeline, implement of Pretraining, Supervised Finetuning, RLHF(Reward Modeling and Reinforcement Learning) and DPO(Direct Preference Optimization).
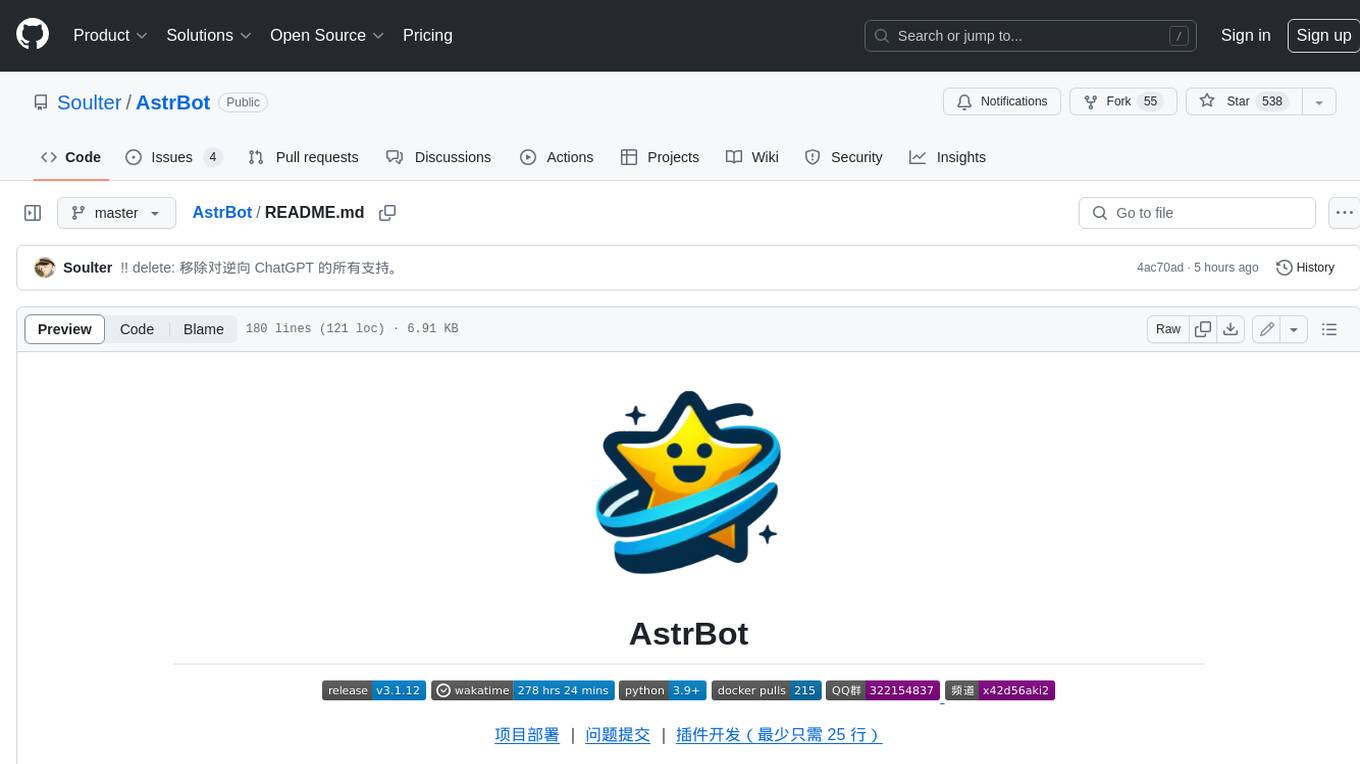
AstrBot
AstrBot is a powerful and versatile tool that leverages the capabilities of large language models (LLMs) like GPT-3, GPT-3.5, and GPT-4 to enhance communication and automate tasks. It seamlessly integrates with popular messaging platforms such as QQ, QQ Channel, and Telegram, enabling users to harness the power of AI within their daily conversations and workflows.
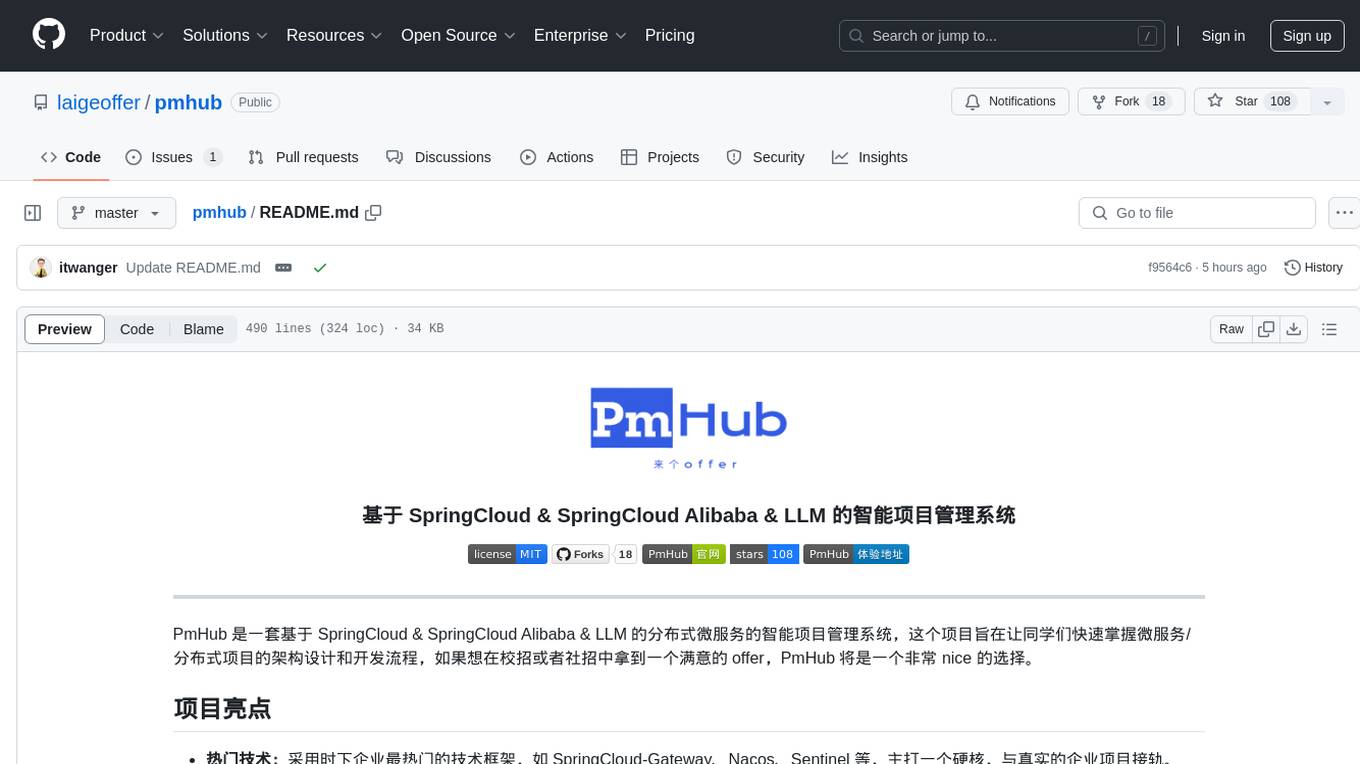
pmhub
PmHub is a smart project management system based on SpringCloud, SpringCloud Alibaba, and LLM. It aims to help students quickly grasp the architecture design and development process of microservices/distributed projects. PmHub provides a platform for students to experience the transformation from monolithic to microservices architecture, understand the pros and cons of both architectures, and prepare for job interviews. It offers popular technologies like SpringCloud-Gateway, Nacos, Sentinel, and provides high-quality code, continuous integration, product design documents, and an enterprise workflow system. PmHub is suitable for beginners and advanced learners who want to master core knowledge of microservices/distributed projects.
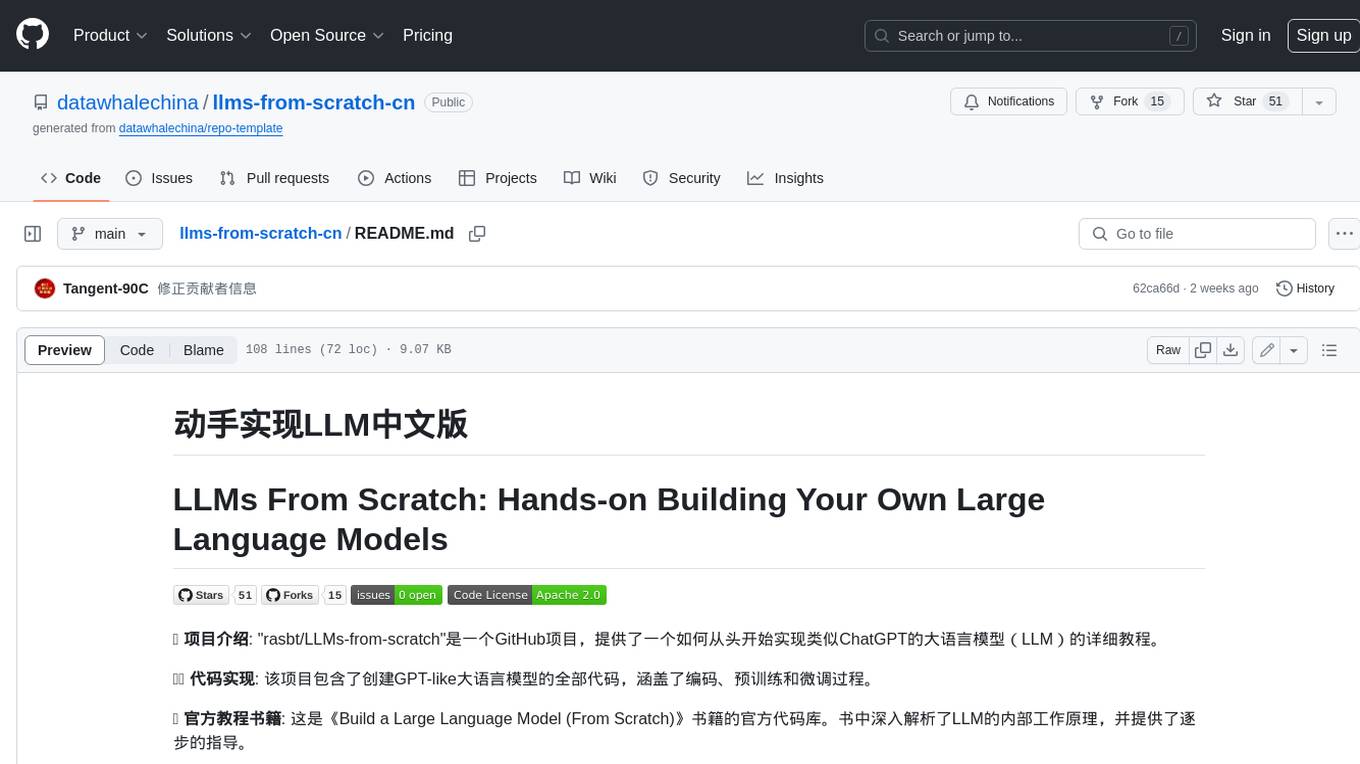
llms-from-scratch-cn
This repository provides a detailed tutorial on how to build your own large language model (LLM) from scratch. It includes all the code necessary to create a GPT-like LLM, covering the encoding, pre-training, and fine-tuning processes. The tutorial is written in a clear and concise style, with plenty of examples and illustrations to help you understand the concepts involved. It is suitable for developers and researchers with some programming experience who are interested in learning more about LLMs and how to build them.
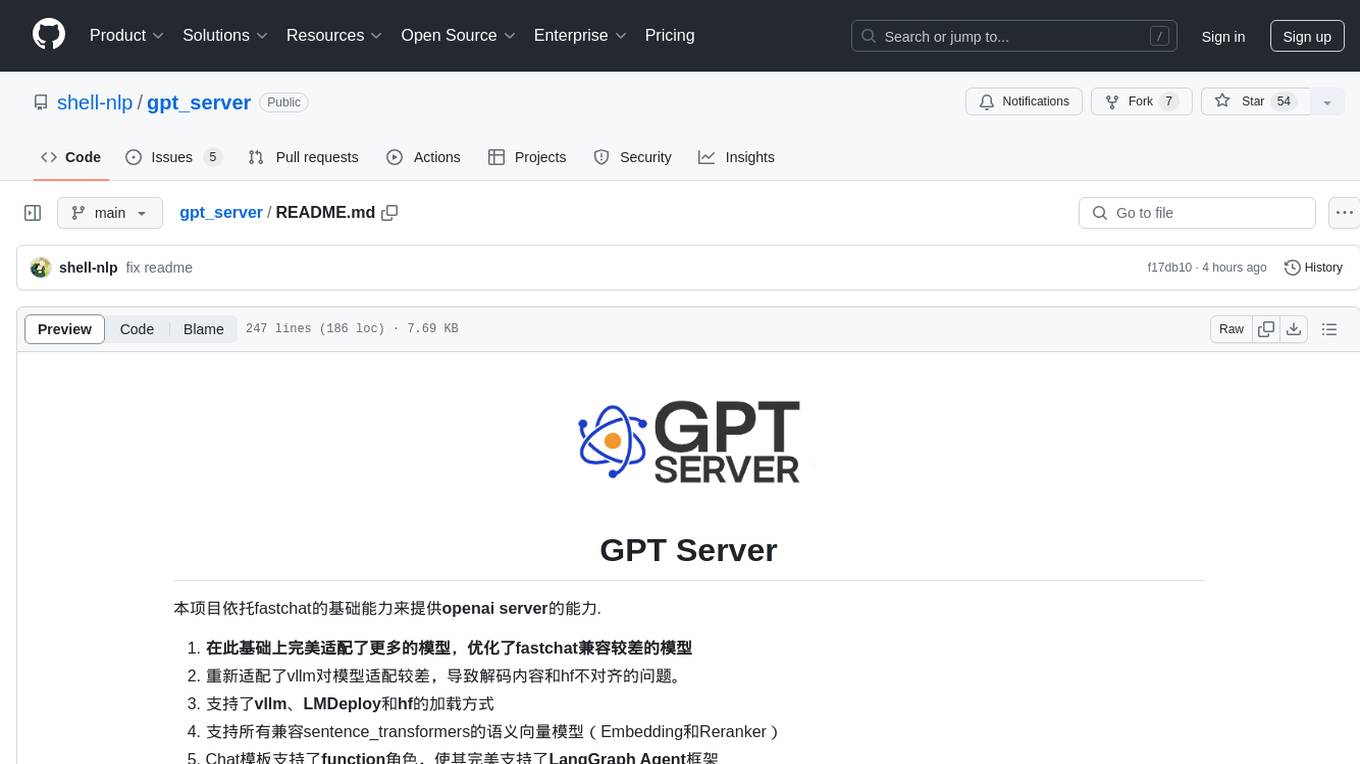
gpt_server
The GPT Server project leverages the basic capabilities of FastChat to provide the capabilities of an openai server. It perfectly adapts more models, optimizes models with poor compatibility in FastChat, and supports loading vllm, LMDeploy, and hf in various ways. It also supports all sentence_transformers compatible semantic vector models, including Chat templates with function roles, Function Calling (Tools) capability, and multi-modal large models. The project aims to reduce the difficulty of model adaptation and project usage, making it easier to deploy the latest models with minimal code changes.
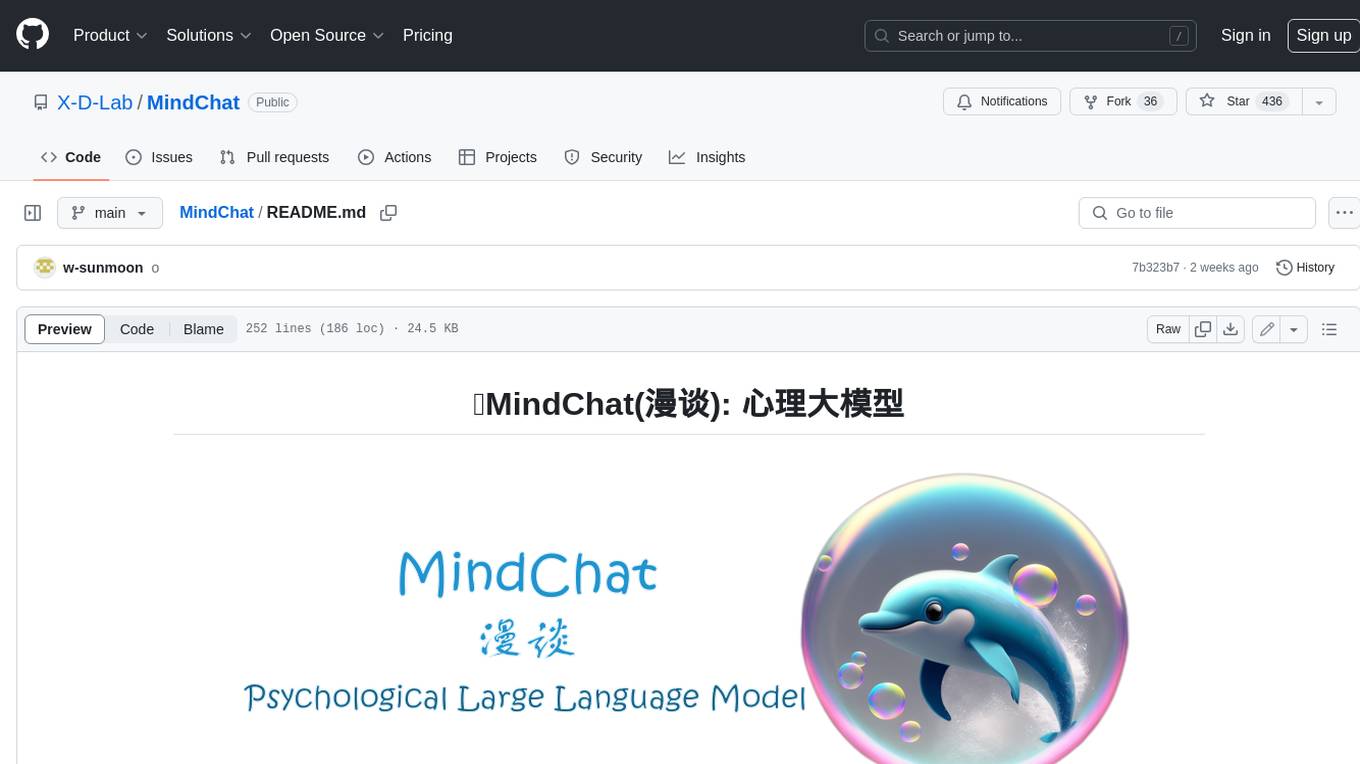
MindChat
MindChat is a psychological large language model designed to help individuals relieve psychological stress and solve mental confusion, ultimately improving mental health. It aims to provide a relaxed and open conversation environment for users to build trust and understanding. MindChat offers privacy, warmth, safety, timely, and convenient conversation settings to help users overcome difficulties and challenges, achieve self-growth, and development. The tool is suitable for both work and personal life scenarios, providing comprehensive psychological support and therapeutic assistance to users while strictly protecting user privacy. It combines psychological knowledge with artificial intelligence technology to contribute to a healthier, more inclusive, and equal society.
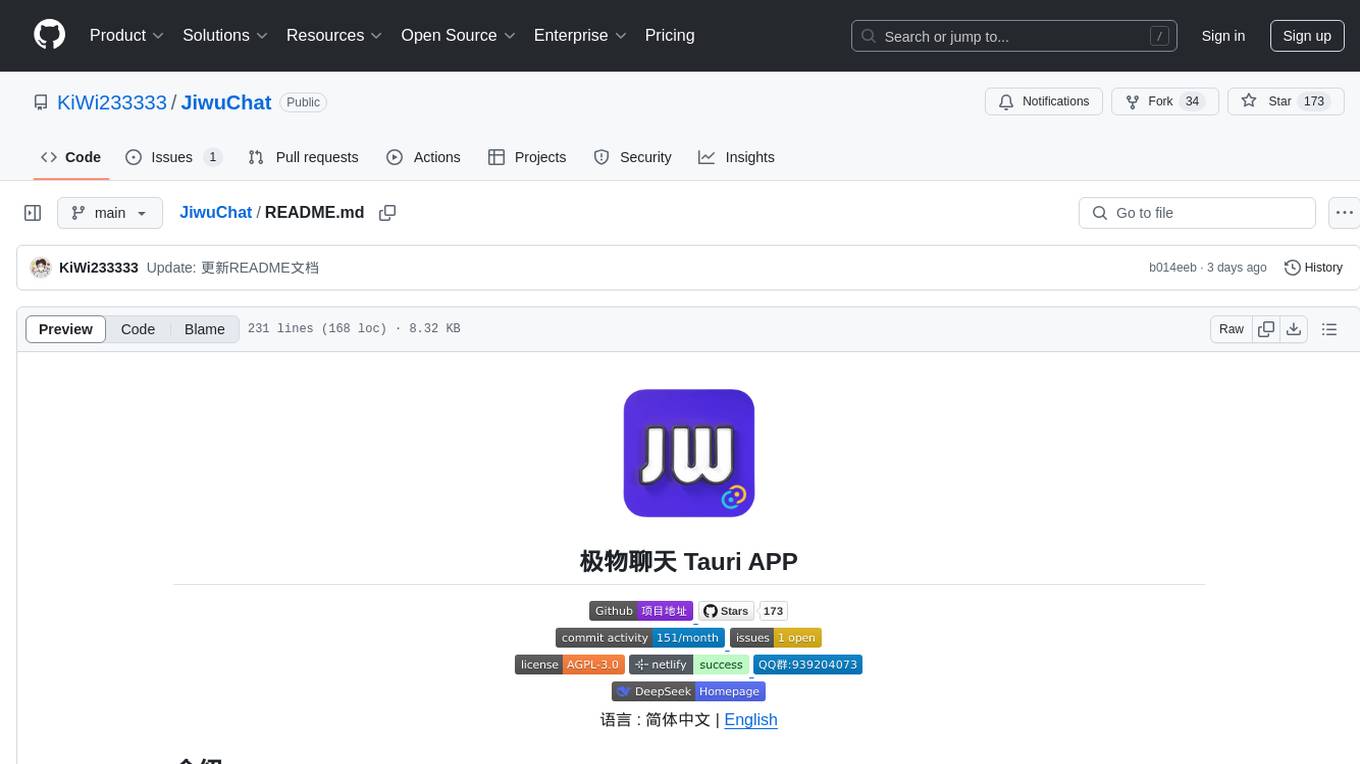
JiwuChat
JiwuChat is a lightweight multi-platform chat application built on Tauri2 and Nuxt3, with various real-time messaging features, AI group chat bots (such as 'iFlytek Spark', 'KimiAI' etc.), WebRTC audio-video calling, screen sharing, and AI shopping functions. It supports seamless cross-device communication, covering text, images, files, and voice messages, also supporting group chats and customizable settings. It provides light/dark mode for efficient social networking.
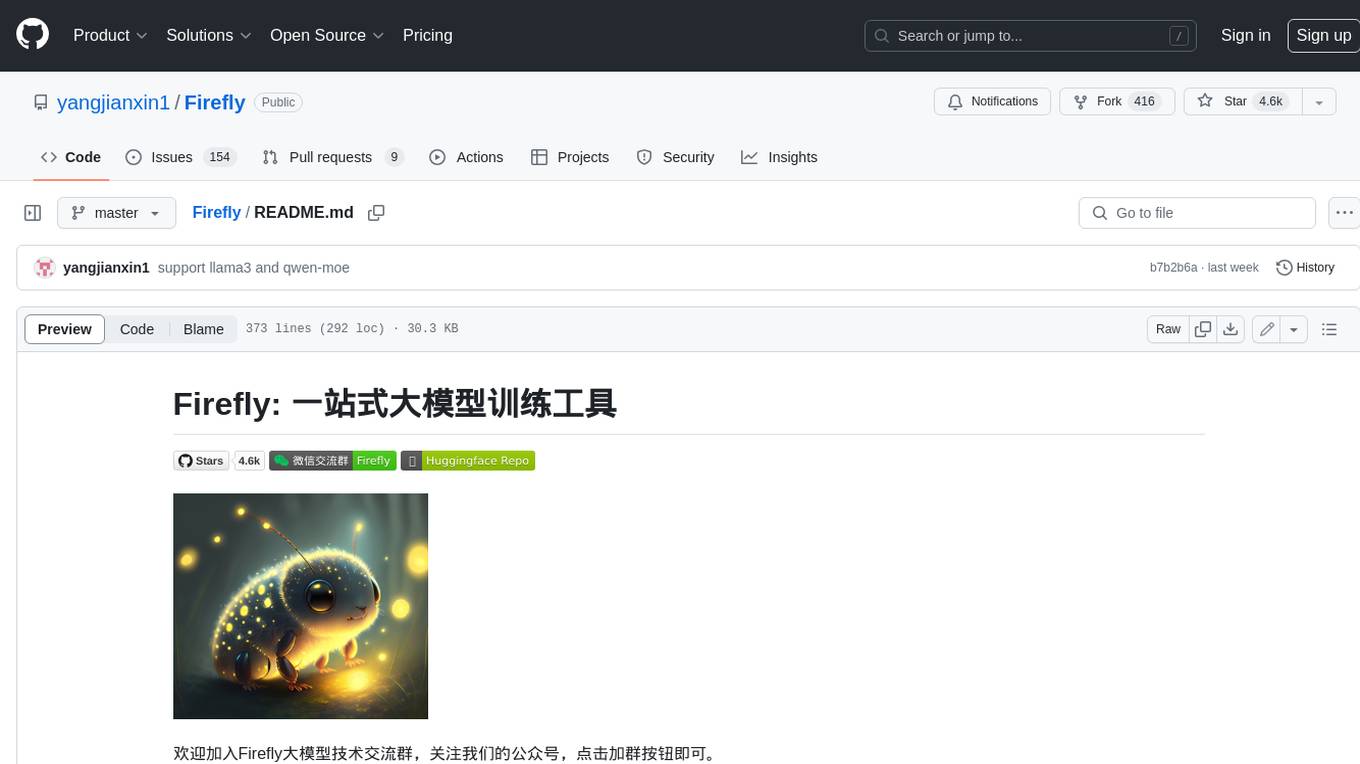
Firefly
Firefly is an open-source large model training project that supports pre-training, fine-tuning, and DPO of mainstream large models. It includes models like Llama3, Gemma, Qwen1.5, MiniCPM, Llama, InternLM, Baichuan, ChatGLM, Yi, Deepseek, Qwen, Orion, Ziya, Xverse, Mistral, Mixtral-8x7B, Zephyr, Vicuna, Bloom, etc. The project supports full-parameter training, LoRA, QLoRA efficient training, and various tasks such as pre-training, SFT, and DPO. Suitable for users with limited training resources, QLoRA is recommended for fine-tuning instructions. The project has achieved good results on the Open LLM Leaderboard with QLoRA training process validation. The latest version has significant updates and adaptations for different chat model templates.
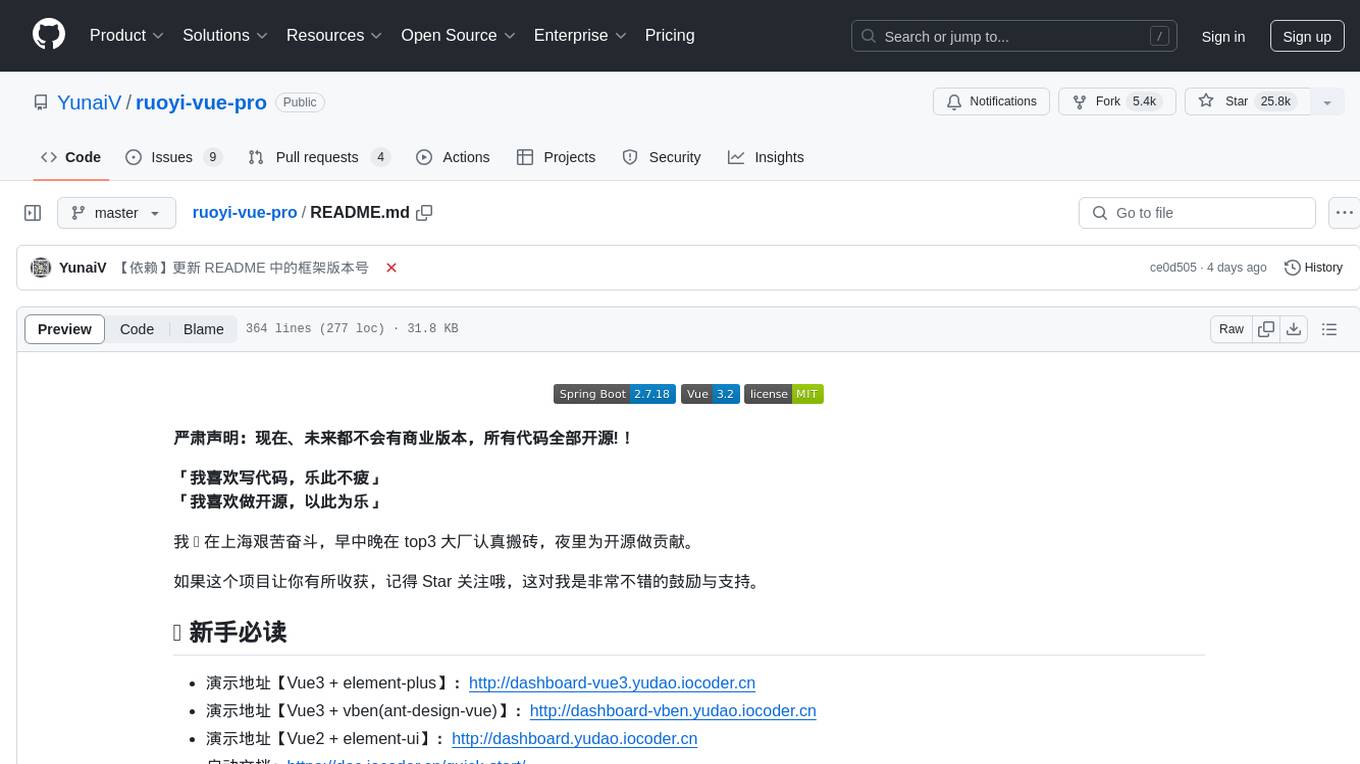
ruoyi-vue-pro
The ruoyi-vue-pro repository is an open-source project that provides a comprehensive development platform with various functionalities such as system features, infrastructure, member center, data reports, workflow, payment system, mall system, ERP system, CRM system, and AI big model. It is built using Java backend with Spring Boot framework and Vue frontend with different versions like Vue3 with element-plus, Vue3 with vben(ant-design-vue), and Vue2 with element-ui. The project aims to offer a fast development platform for developers and enterprises, supporting features like dynamic menu loading, button-level access control, SaaS multi-tenancy, code generator, real-time communication, integration with third-party services like WeChat, Alipay, and cloud services, and more.
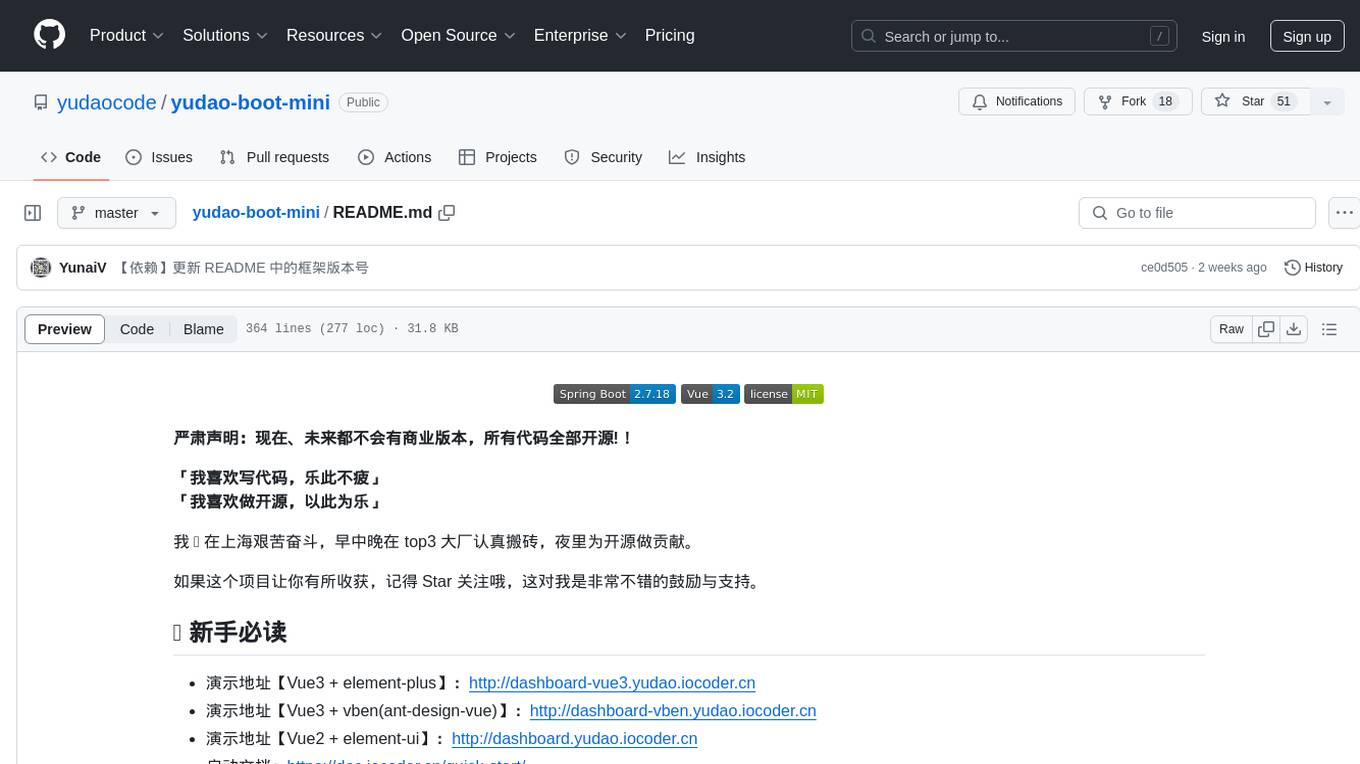
yudao-boot-mini
yudao-boot-mini is an open-source project focused on developing a rapid development platform for developers in China. It includes features like system functions, infrastructure, member center, data reports, workflow, mall system, WeChat official account, CRM, ERP, etc. The project is based on Spring Boot with Java backend and Vue for frontend. It offers various functionalities such as user management, role management, menu management, department management, workflow management, payment system, code generation, API documentation, database documentation, file service, WebSocket integration, message queue, Java monitoring, and more. The project is licensed under the MIT License, allowing both individuals and enterprises to use it freely without restrictions.
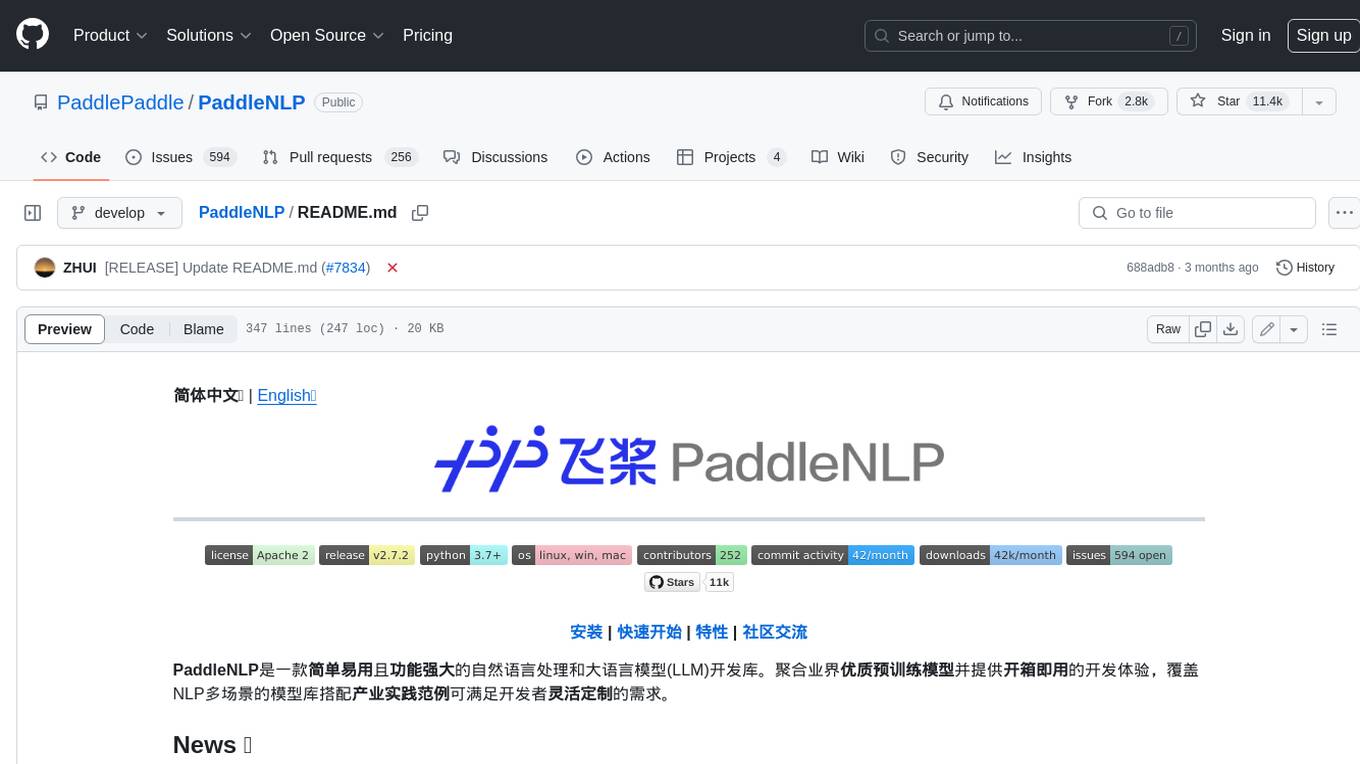
PaddleNLP
PaddleNLP is an easy-to-use and high-performance NLP library. It aggregates high-quality pre-trained models in the industry and provides out-of-the-box development experience, covering a model library for multiple NLP scenarios with industry practice examples to meet developers' flexible customization needs.
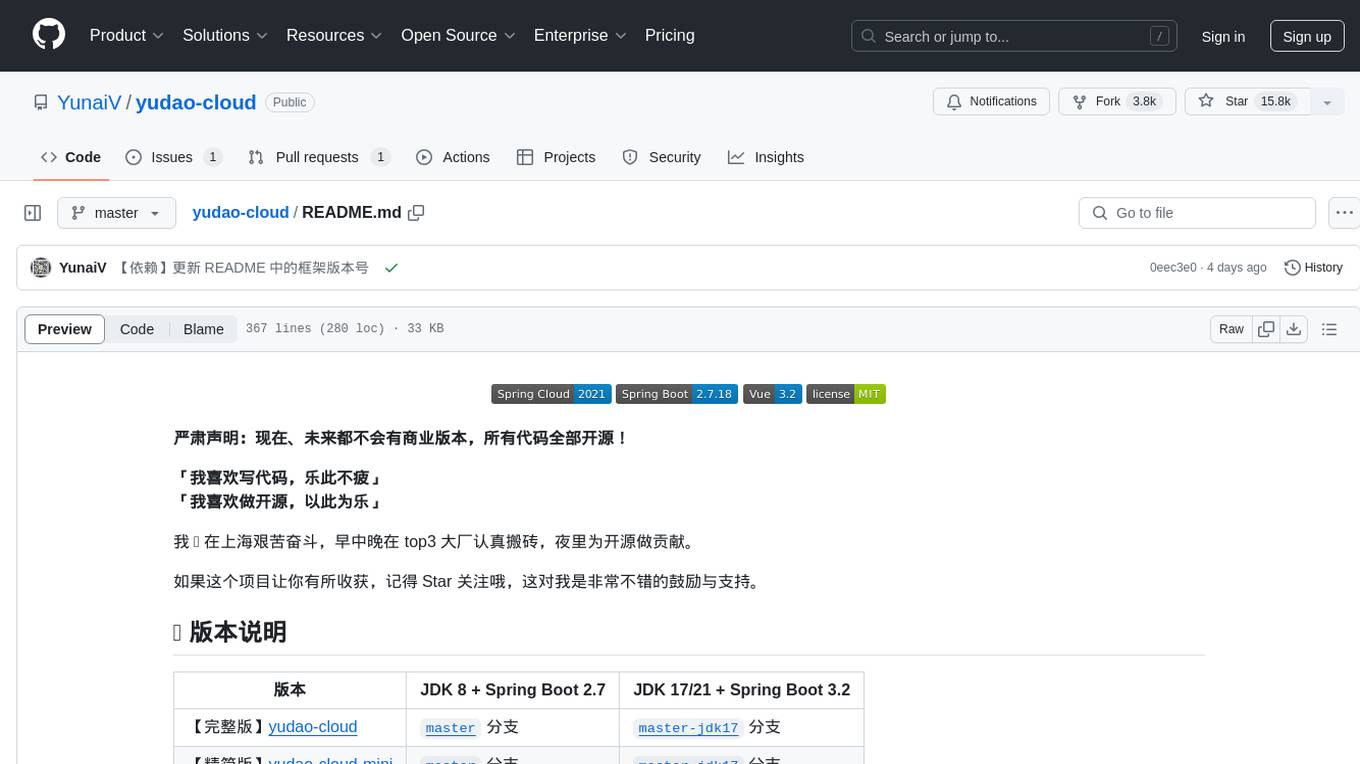
yudao-cloud
Yudao-cloud is an open-source project designed to provide a fast development platform for developers in China. It includes various system functions, infrastructure, member center, data reports, workflow, mall system, WeChat public account, CRM, ERP, etc. The project is based on Java backend with Spring Boot and Spring Cloud Alibaba microservices architecture. It supports multiple databases, message queues, authentication systems, dynamic menu loading, SaaS multi-tenant system, code generator, real-time communication, integration with third-party services like WeChat, Alipay, and more. The project is well-documented and follows the Alibaba Java development guidelines, ensuring clean code and architecture.
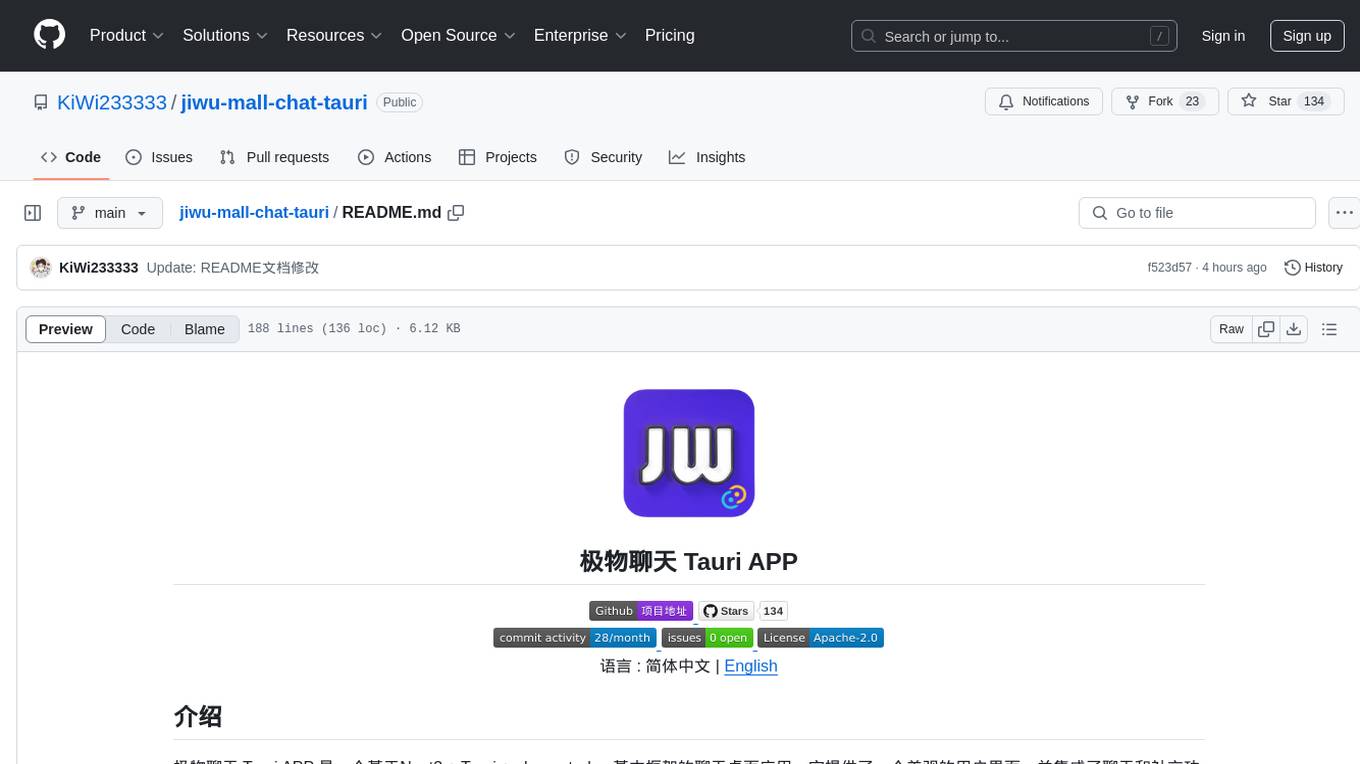
jiwu-mall-chat-tauri
Jiwu Chat Tauri APP is a desktop chat application based on Nuxt3 + Tauri + Element Plus framework. It provides a beautiful user interface with integrated chat and social functions. It also supports AI shopping chat and global dark mode. Users can engage in real-time chat, share updates, and interact with AI customer service through this application.
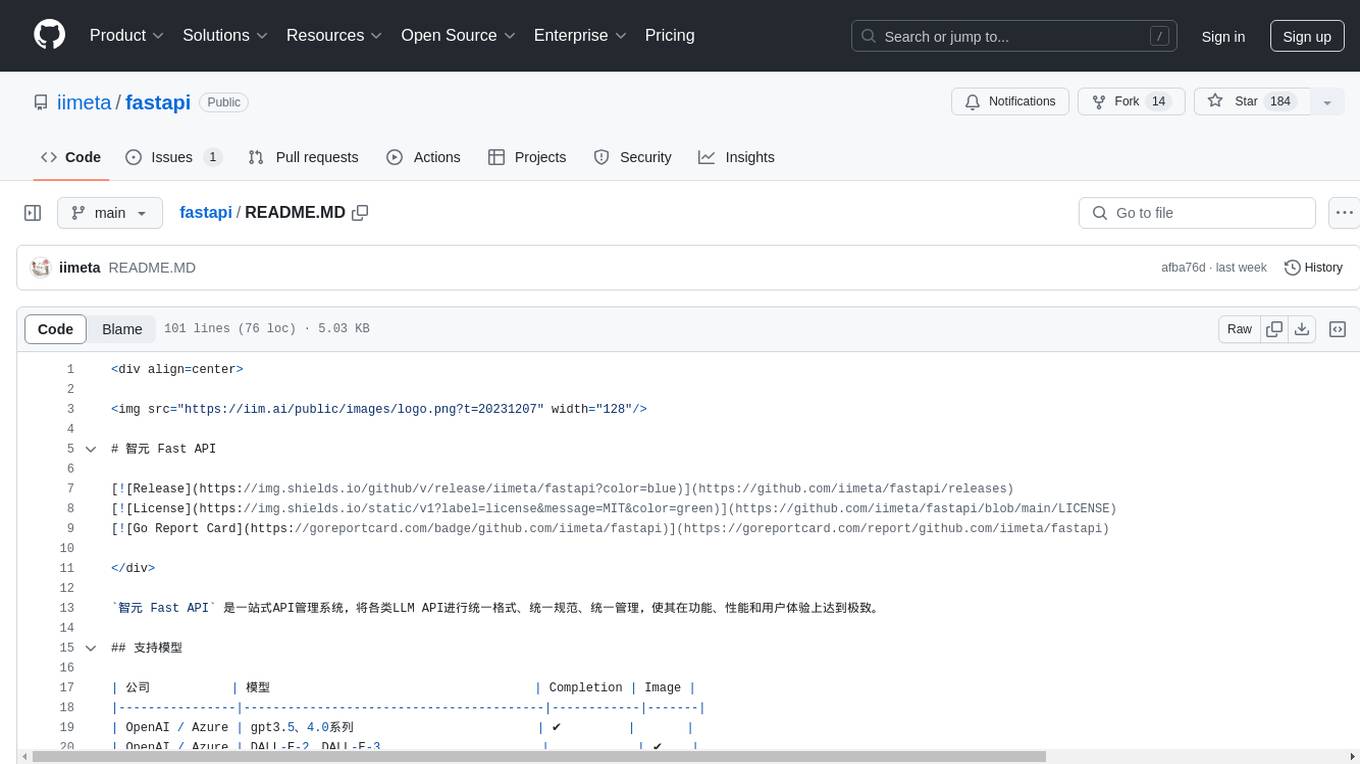
fastapi
智元 Fast API is a one-stop API management system that unifies various LLM APIs in terms of format, standards, and management, achieving the ultimate in functionality, performance, and user experience. It supports various models from companies like OpenAI, Azure, Baidu, Keda Xunfei, Alibaba Cloud, Zhifu AI, Google, DeepSeek, 360 Brain, and Midjourney. The project provides user and admin portals for preview, supports cluster deployment, multi-site deployment, and cross-zone deployment. It also offers Docker deployment, a public API site for registration, and screenshots of the admin and user portals. The API interface is similar to OpenAI's interface, and the project is open source with repositories for API, web, admin, and SDK on GitHub and Gitee.
For similar tasks
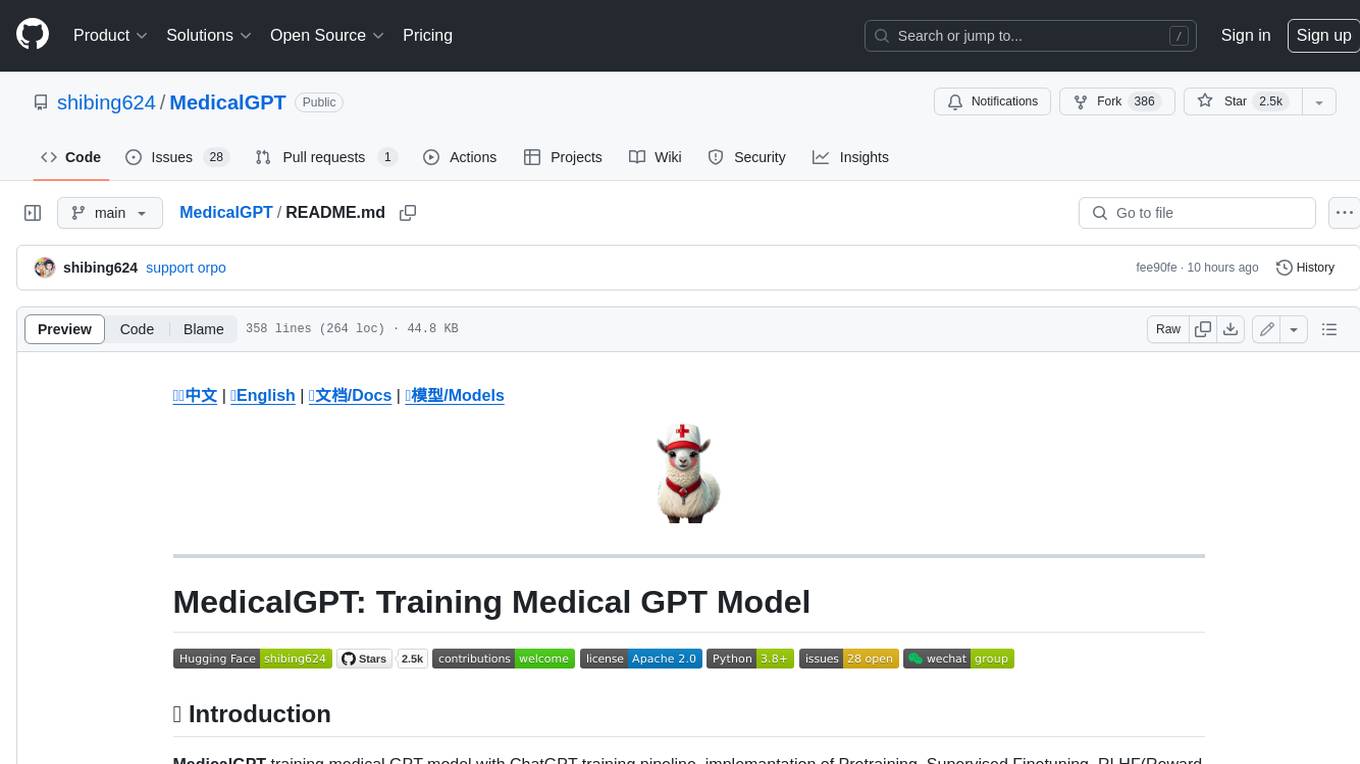
MedicalGPT
MedicalGPT is a training medical GPT model with ChatGPT training pipeline, implement of Pretraining, Supervised Finetuning, RLHF(Reward Modeling and Reinforcement Learning) and DPO(Direct Preference Optimization).
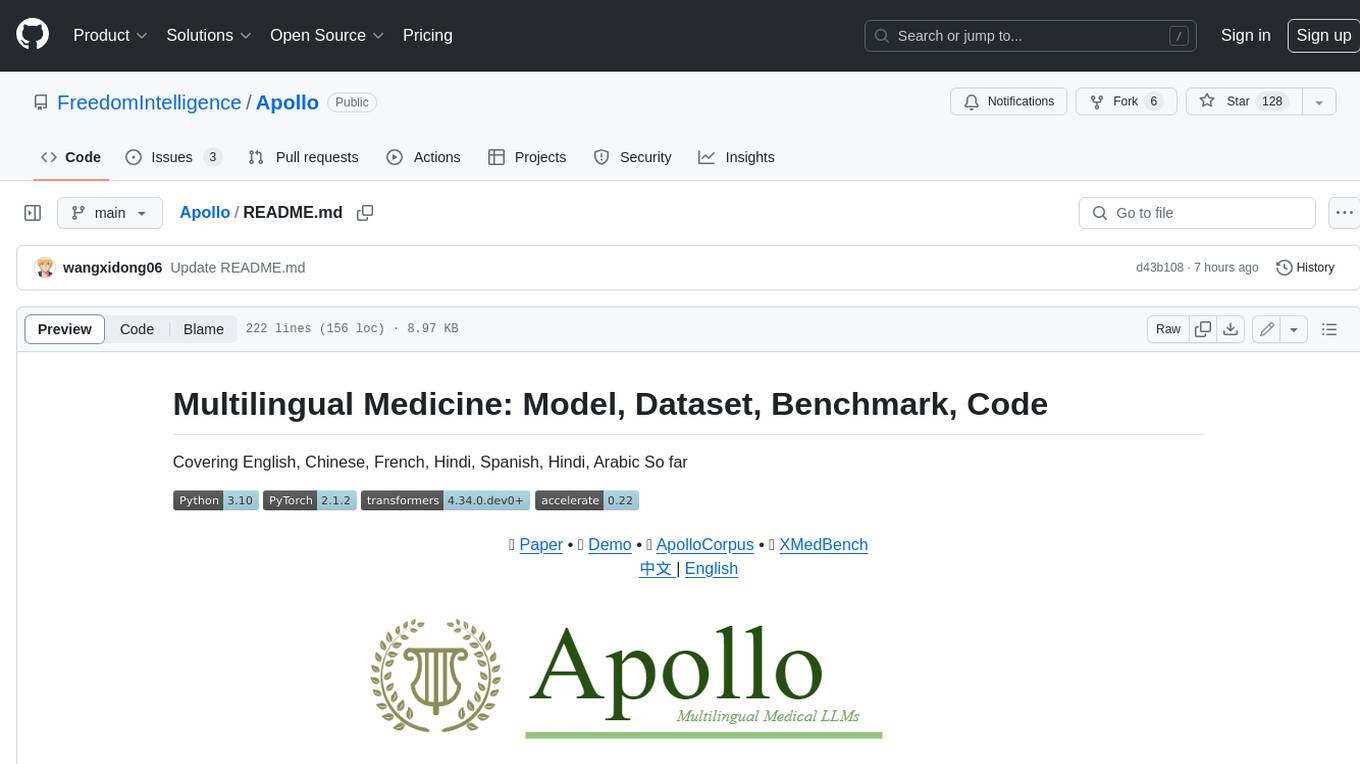
Apollo
Apollo is a multilingual medical LLM that covers English, Chinese, French, Hindi, Spanish, Hindi, and Arabic. It is designed to democratize medical AI to 6B people. Apollo has achieved state-of-the-art results on a variety of medical NLP tasks, including question answering, medical dialogue generation, and medical text classification. Apollo is easy to use and can be integrated into a variety of applications, making it a valuable tool for healthcare professionals and researchers.
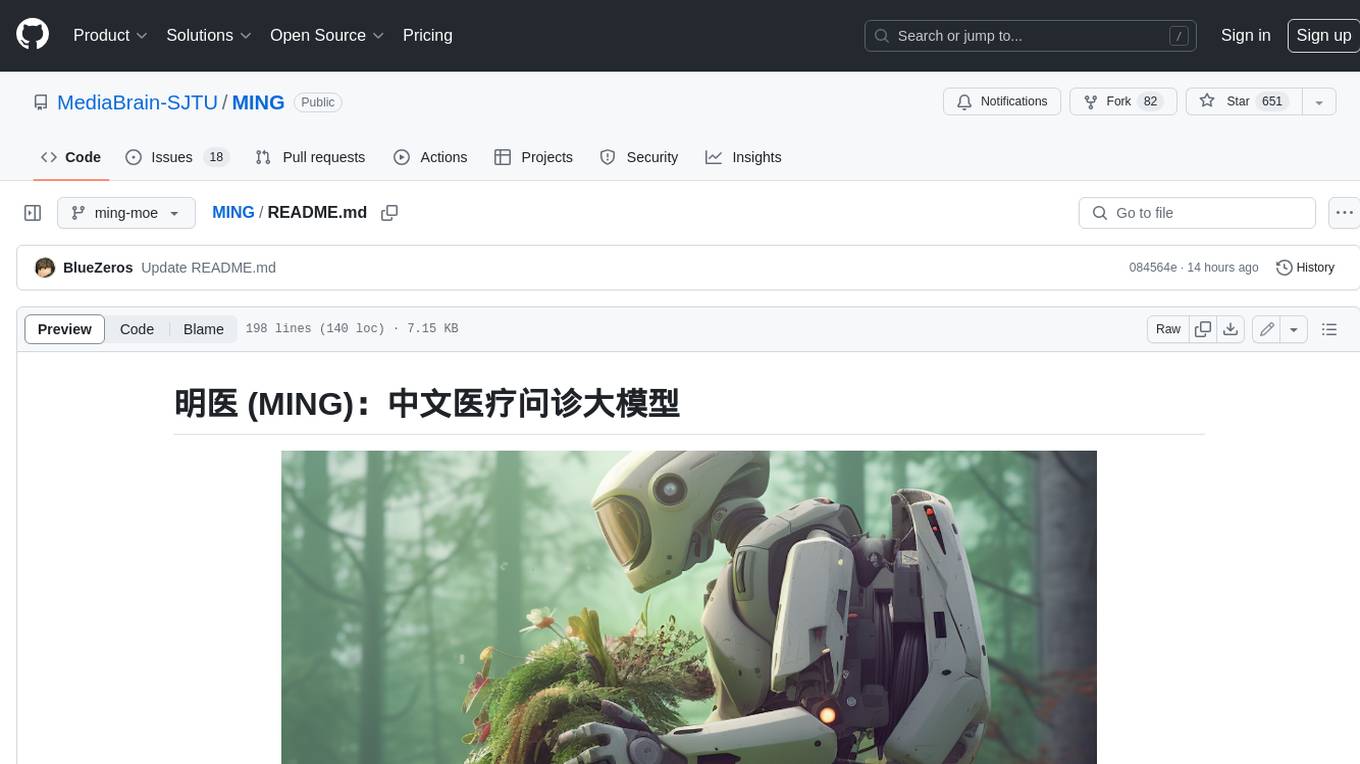
MING
MING is an open-sourced Chinese medical consultation model fine-tuned based on medical instructions. The main functions of the model are as follows: Medical Q&A: answering medical questions and analyzing cases. Intelligent consultation: giving diagnosis results and suggestions after multiple rounds of consultation.
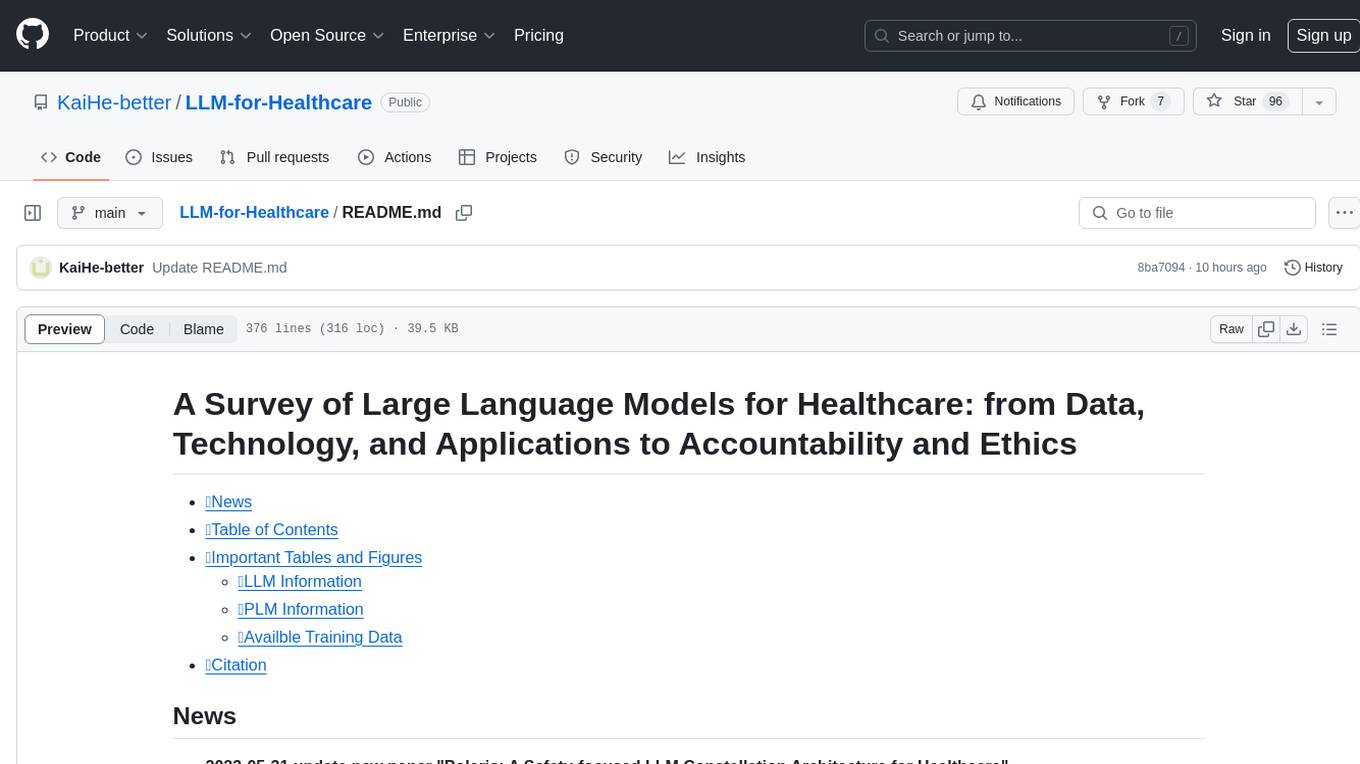
LLM-for-Healthcare
The repository 'LLM-for-Healthcare' provides a comprehensive survey of large language models (LLMs) for healthcare, covering data, technology, applications, and accountability and ethics. It includes information on various LLM models, training data, evaluation methods, and computation costs. The repository also discusses tasks such as NER, text classification, question answering, dialogue systems, and generation of medical reports from images in the healthcare domain.
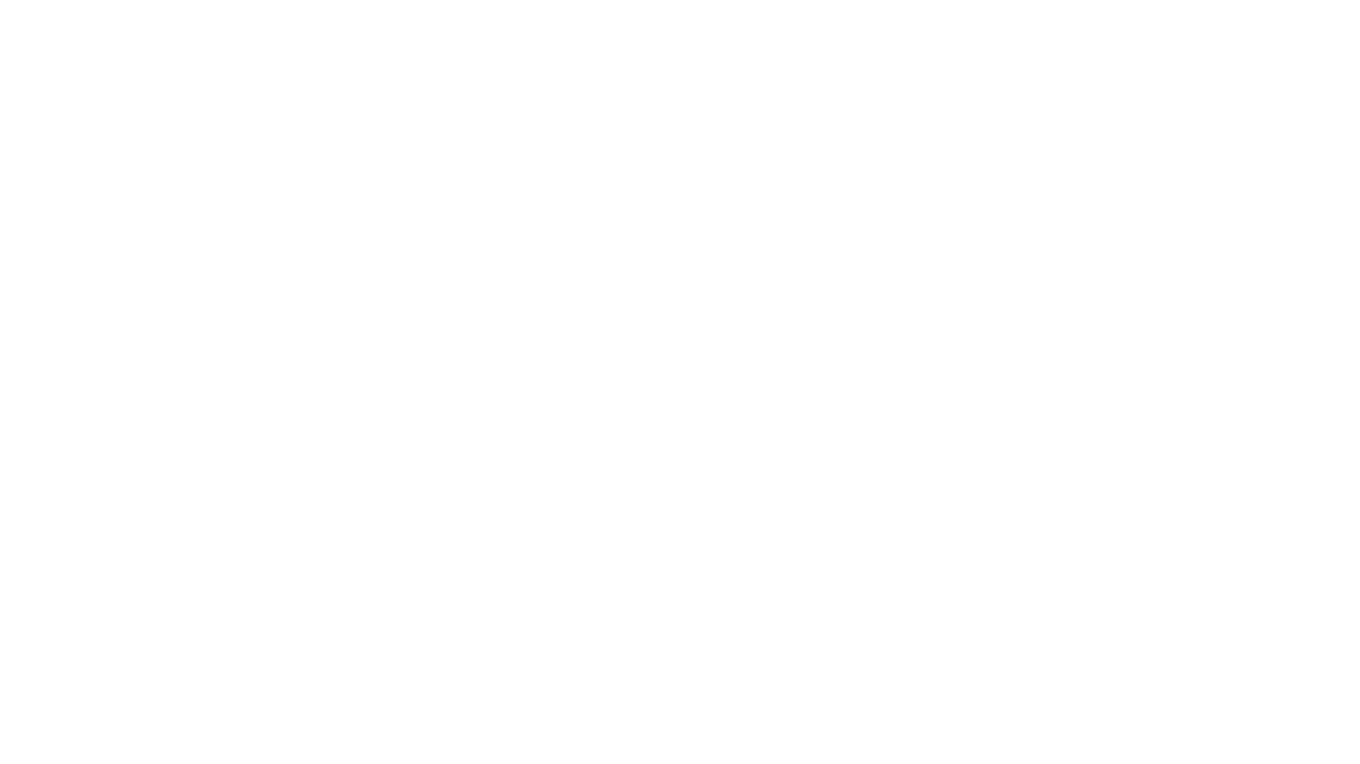
Me-LLaMA
Me LLaMA introduces a suite of open-source medical Large Language Models (LLMs), including Me LLaMA 13B/70B and their chat-enhanced versions. Developed through innovative continual pre-training and instruction tuning, these models leverage a vast medical corpus comprising PubMed papers, medical guidelines, and general domain data. Me LLaMA sets new benchmarks on medical reasoning tasks, making it a significant asset for medical NLP applications and research. The models are intended for computational linguistics and medical research, not for clinical decision-making without validation and regulatory approval.
For similar jobs
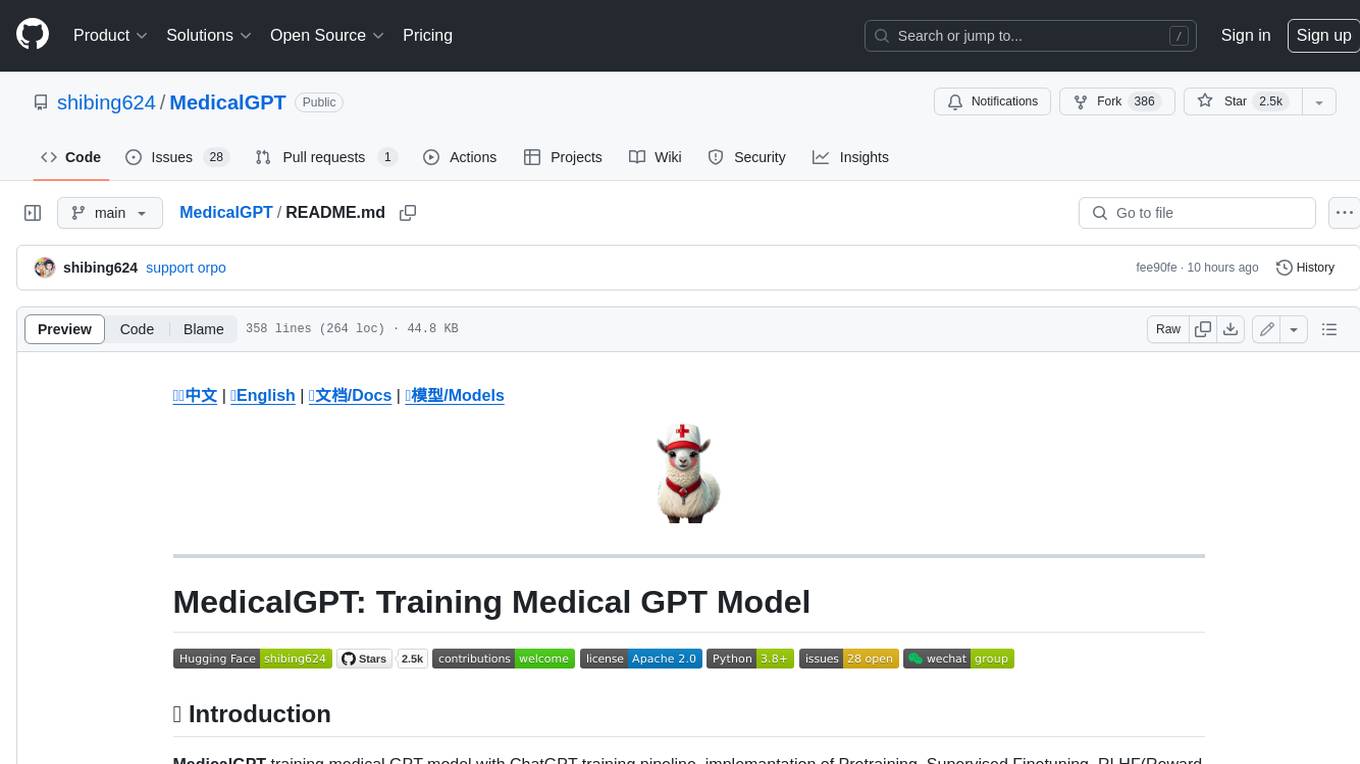
MedicalGPT
MedicalGPT is a training medical GPT model with ChatGPT training pipeline, implement of Pretraining, Supervised Finetuning, RLHF(Reward Modeling and Reinforcement Learning) and DPO(Direct Preference Optimization).
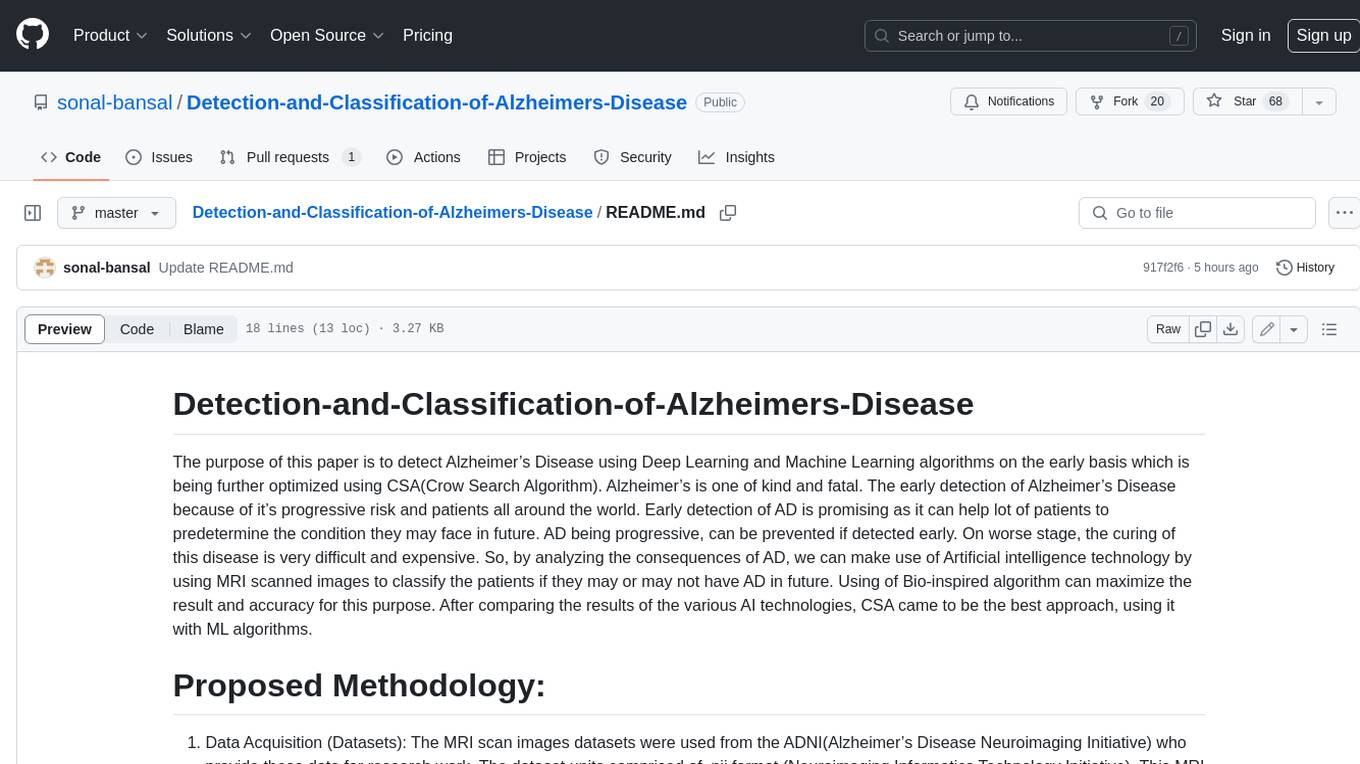
Detection-and-Classification-of-Alzheimers-Disease
This tool is designed to detect and classify Alzheimer's Disease using Deep Learning and Machine Learning algorithms on an early basis, which is further optimized using the Crow Search Algorithm (CSA). Alzheimer's is a fatal disease, and early detection is crucial for patients to predetermine their condition and prevent its progression. By analyzing MRI scanned images using Artificial Intelligence technology, this tool can classify patients who may or may not develop AD in the future. The CSA algorithm, combined with ML algorithms, has proven to be the most effective approach for this purpose.
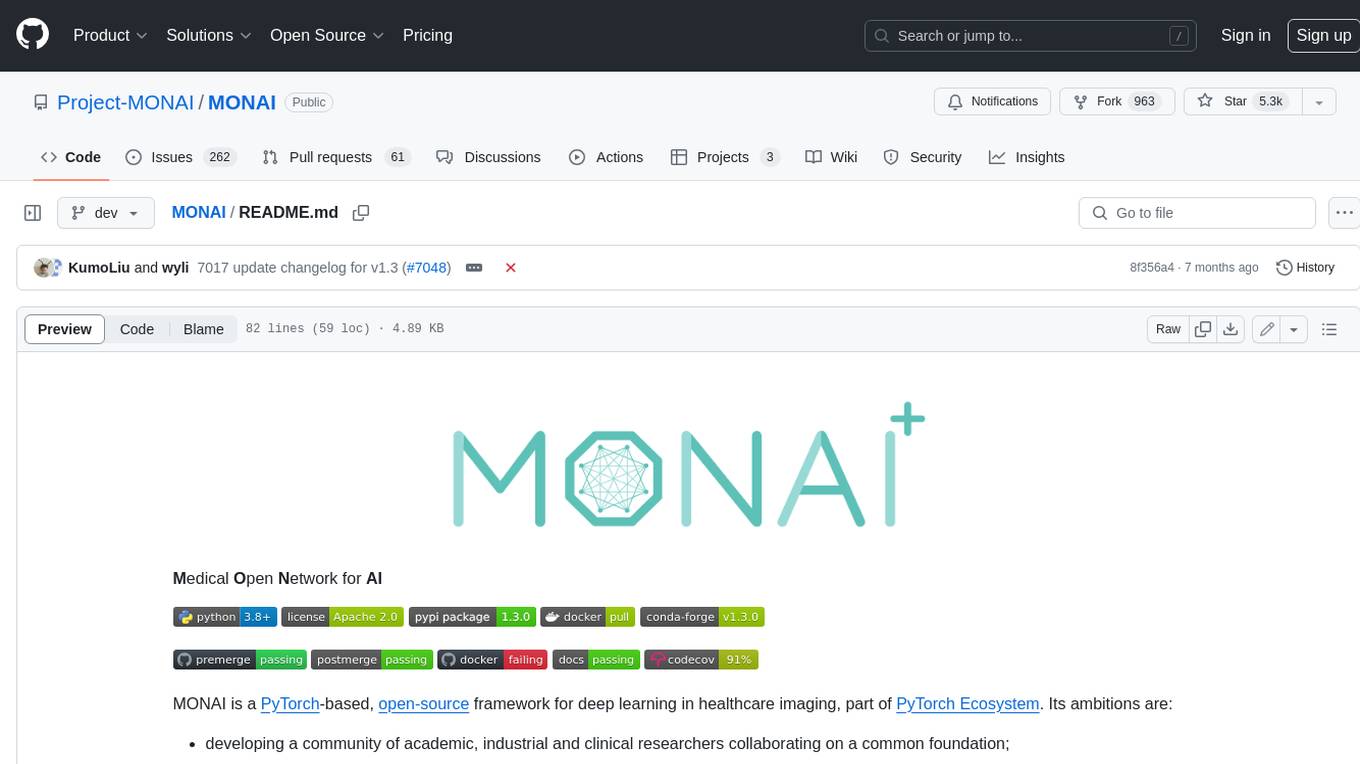
MONAI
MONAI is a PyTorch-based, open-source framework for deep learning in healthcare imaging. It provides a comprehensive set of tools for medical image analysis, including data preprocessing, model training, and evaluation. MONAI is designed to be flexible and easy to use, making it a valuable resource for researchers and developers in the field of medical imaging.
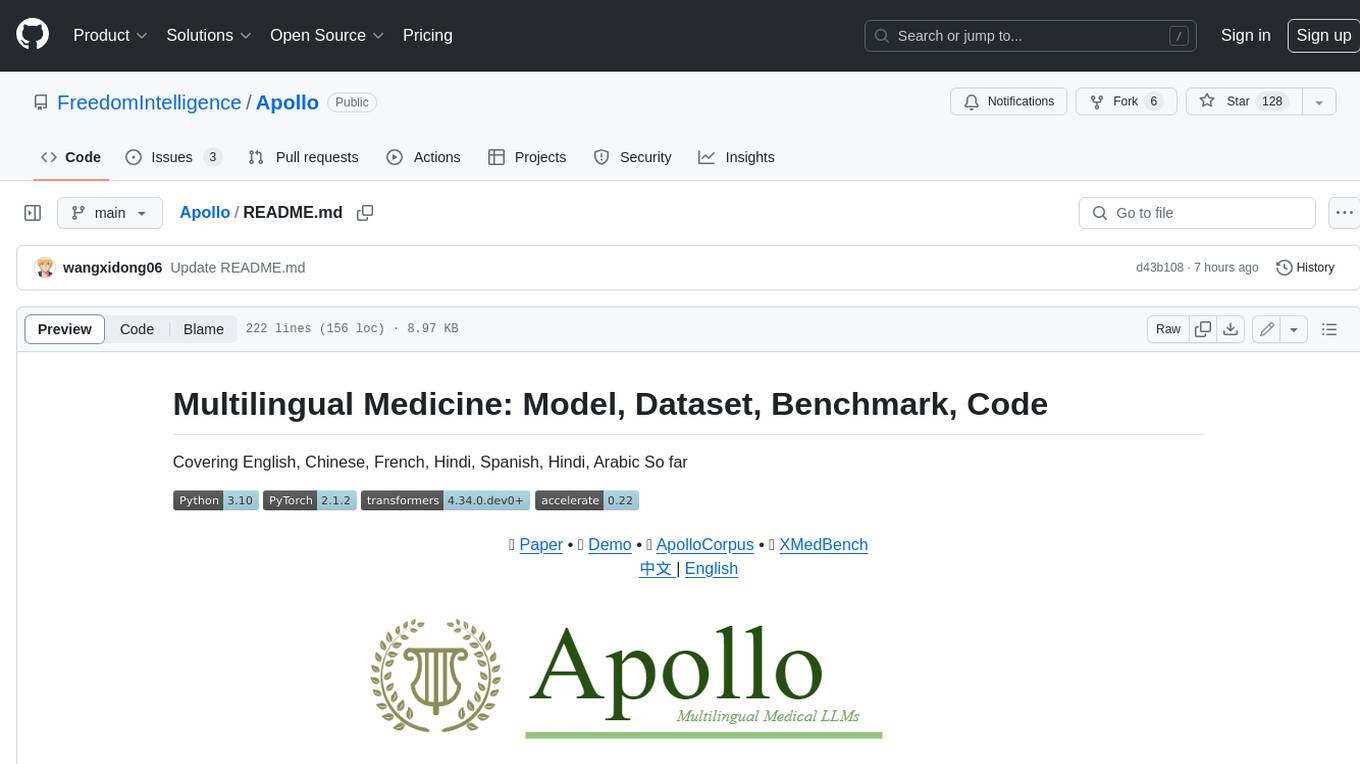
Apollo
Apollo is a multilingual medical LLM that covers English, Chinese, French, Hindi, Spanish, Hindi, and Arabic. It is designed to democratize medical AI to 6B people. Apollo has achieved state-of-the-art results on a variety of medical NLP tasks, including question answering, medical dialogue generation, and medical text classification. Apollo is easy to use and can be integrated into a variety of applications, making it a valuable tool for healthcare professionals and researchers.
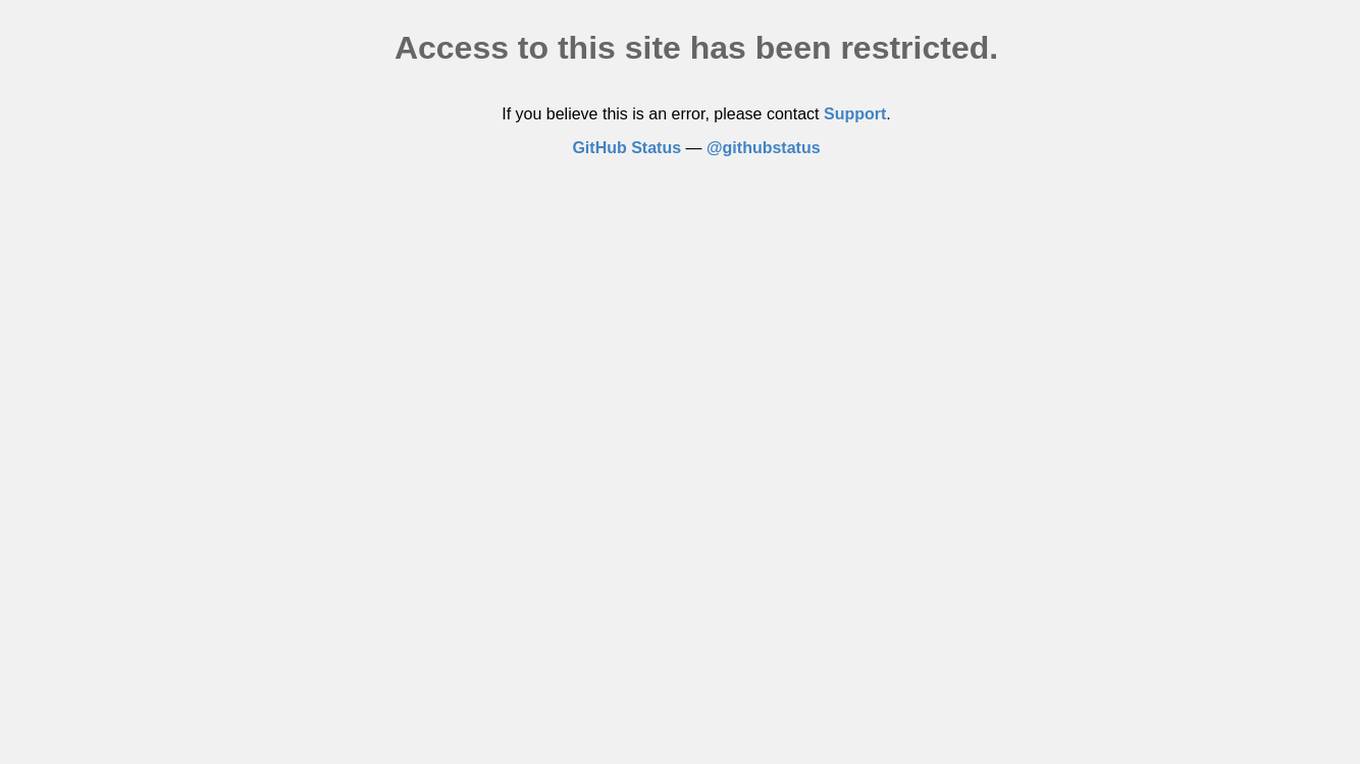
Open-Medical-Reasoning-Tasks
Open Life Science AI: Medical Reasoning Tasks is a collaborative hub for developing cutting-edge reasoning tasks for Large Language Models (LLMs) in the medical, healthcare, and clinical domains. The repository aims to advance AI capabilities in healthcare by fostering accurate diagnoses, personalized treatments, and improved patient outcomes. It offers a diverse range of medical reasoning challenges such as Diagnostic Reasoning, Treatment Planning, Medical Image Analysis, Clinical Data Interpretation, Patient History Analysis, Ethical Decision Making, Medical Literature Comprehension, and Drug Interaction Assessment. Contributors can join the community of healthcare professionals, AI researchers, and enthusiasts to contribute to the repository by creating new tasks or improvements following the provided guidelines. The repository also provides resources including a task list, evaluation metrics, medical AI papers, and healthcare datasets for training and evaluation.
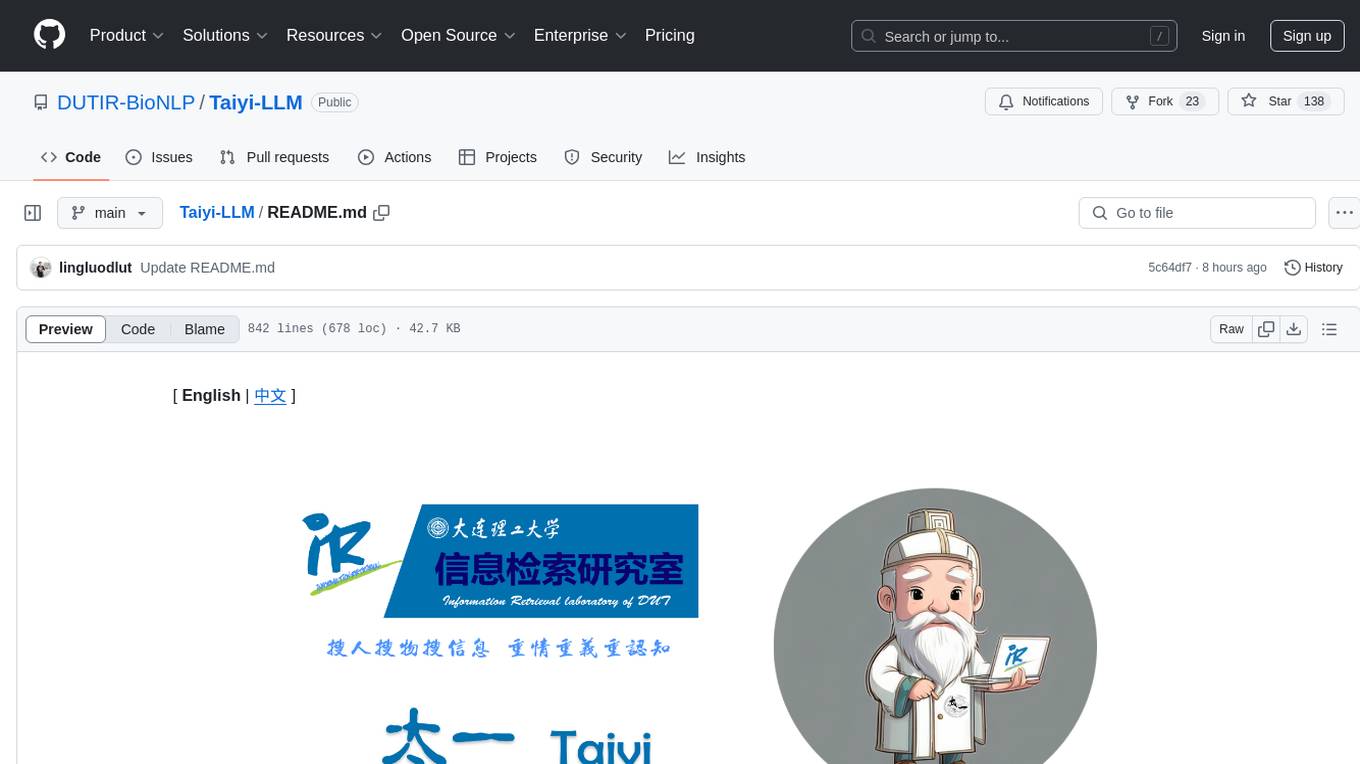
Taiyi-LLM
Taiyi (太一) is a bilingual large language model fine-tuned for diverse biomedical tasks. It aims to facilitate communication between healthcare professionals and patients, provide medical information, and assist in diagnosis, biomedical knowledge discovery, drug development, and personalized healthcare solutions. The model is based on the Qwen-7B-base model and has been fine-tuned using rich bilingual instruction data. It covers tasks such as question answering, biomedical dialogue, medical report generation, biomedical information extraction, machine translation, title generation, text classification, and text semantic similarity. The project also provides standardized data formats, model training details, model inference guidelines, and overall performance metrics across various BioNLP tasks.
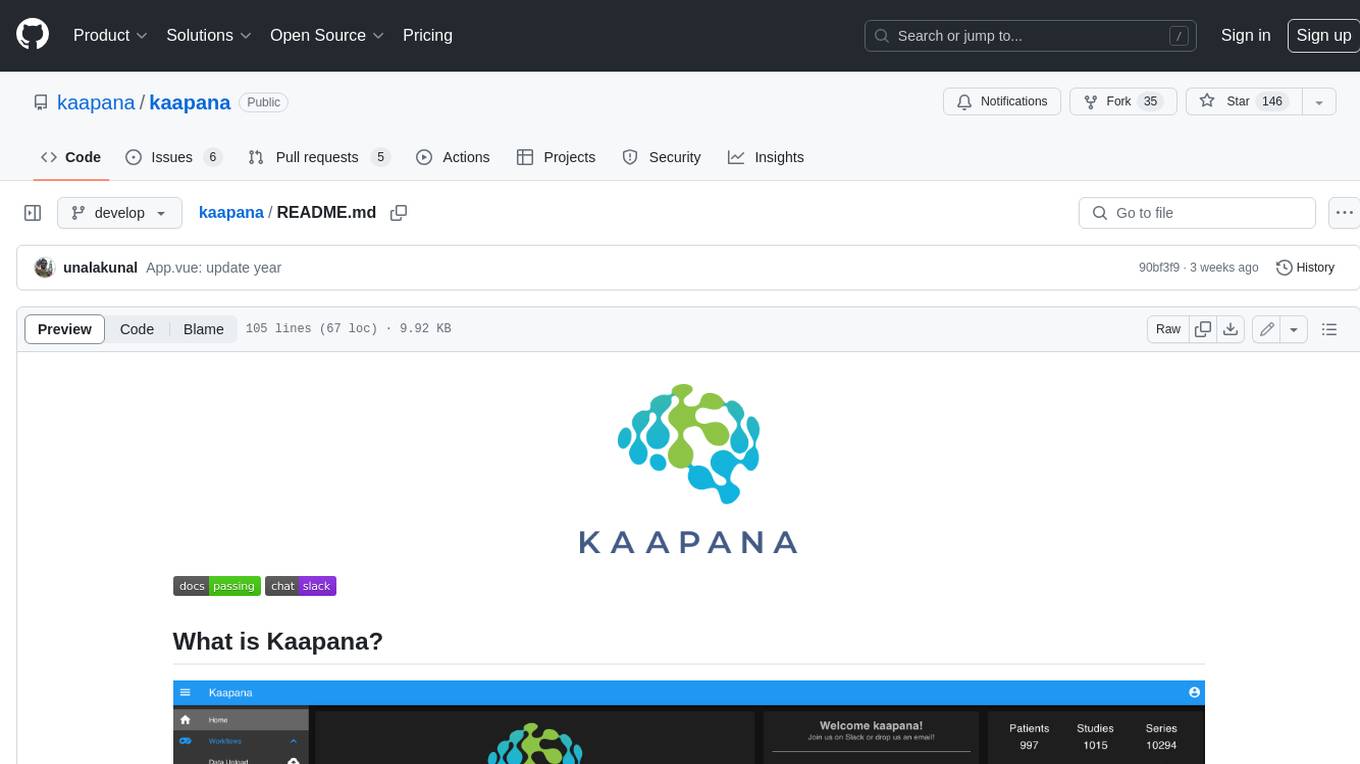
kaapana
Kaapana is an open-source toolkit for state-of-the-art platform provisioning in the field of medical data analysis. The applications comprise AI-based workflows and federated learning scenarios with a focus on radiological and radiotherapeutic imaging. Obtaining large amounts of medical data necessary for developing and training modern machine learning methods is an extremely challenging effort that often fails in a multi-center setting, e.g. due to technical, organizational and legal hurdles. A federated approach where the data remains under the authority of the individual institutions and is only processed on-site is, in contrast, a promising approach ideally suited to overcome these difficulties. Following this federated concept, the goal of Kaapana is to provide a framework and a set of tools for sharing data processing algorithms, for standardized workflow design and execution as well as for performing distributed method development. This will facilitate data analysis in a compliant way enabling researchers and clinicians to perform large-scale multi-center studies. By adhering to established standards and by adopting widely used open technologies for private cloud development and containerized data processing, Kaapana integrates seamlessly with the existing clinical IT infrastructure, such as the Picture Archiving and Communication System (PACS), and ensures modularity and easy extensibility.
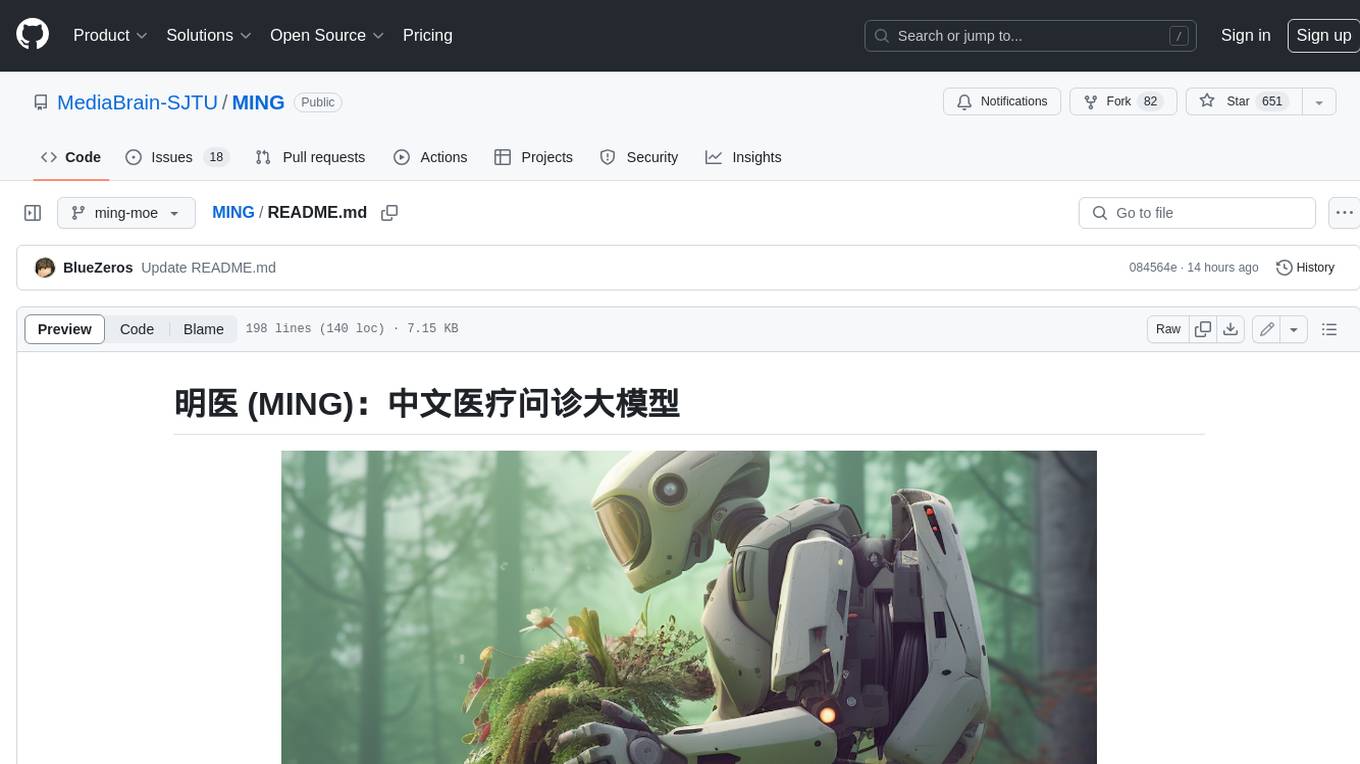
MING
MING is an open-sourced Chinese medical consultation model fine-tuned based on medical instructions. The main functions of the model are as follows: Medical Q&A: answering medical questions and analyzing cases. Intelligent consultation: giving diagnosis results and suggestions after multiple rounds of consultation.