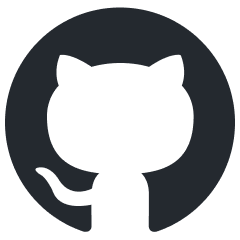
Open-Medical-Reasoning-Tasks
A comprehensive repository of reasoning tasks for Medical LLMs (and beyond)
Stars: 80
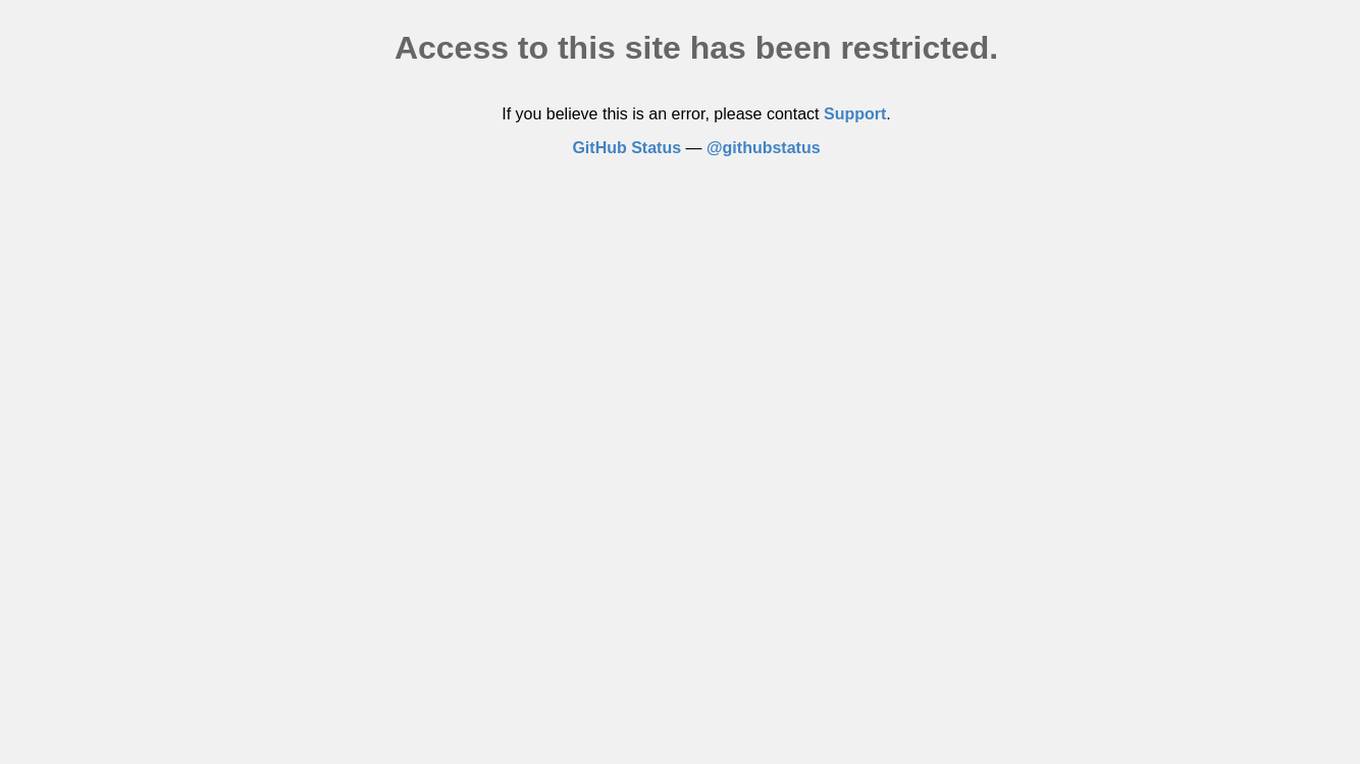
Open Life Science AI: Medical Reasoning Tasks is a collaborative hub for developing cutting-edge reasoning tasks for Large Language Models (LLMs) in the medical, healthcare, and clinical domains. The repository aims to advance AI capabilities in healthcare by fostering accurate diagnoses, personalized treatments, and improved patient outcomes. It offers a diverse range of medical reasoning challenges such as Diagnostic Reasoning, Treatment Planning, Medical Image Analysis, Clinical Data Interpretation, Patient History Analysis, Ethical Decision Making, Medical Literature Comprehension, and Drug Interaction Assessment. Contributors can join the community of healthcare professionals, AI researchers, and enthusiasts to contribute to the repository by creating new tasks or improvements following the provided guidelines. The repository also provides resources including a task list, evaluation metrics, medical AI papers, and healthcare datasets for training and evaluation.
README:
Advancing AI in Healthcare through Collaborative Task Development
Image Source: Can large language models reason about medical questions?
Welcome to the frontier of medical AI! This repository is a collaborative hub for developing cutting-edge reasoning tasks for Large Language Models (LLMs) in the medical, healthcare, and clinical domains.
Our Mission: To push the boundaries of AI capabilities in healthcare, fostering more accurate diagnoses, personalized treatments, and improved patient outcomes.
Explore our diverse range of medical reasoning challenges (Not limited to):
- Diagnostic Reasoning
- Treatment Planning
- Medical Image Analysis
- Clinical Data Interpretation
- Patient History Analysis
- Ethical Decision Making
- Medical Literature Comprehension
- Drug Interaction Assessment
- More to come
We believe in the power of collective intelligence. Join our community of healthcare professionals, AI researchers, and enthusiasts!
To contribute, please use GitHub and follow these guidelines. If you're not familiar with GitHub, don't worry! You can use this Google form to submit your tasks. Make sure to check the example provided to ensure all information is filled out correctly.
With Github:
- Fork this repository
- Create a new branch for your task or improvement
- Add your contribution following our guidelines
- Submit a pull request
Detailed instructions available in our Contribution Guide.
- π Task List: Comprehensive list of medical reasoning tasks
- π Evaluation Metrics: Assessing LLM performance in healthcare
- π Medical AI Papers: Curated list of groundbreaking research
- ποΈ Healthcare Datasets: High-quality data for training and evaluation
/
βββ π tasks/
β βββ π¬ diagnostic-reasoning/
β βββ π treatment-planning/
β βββ πΌοΈ medical-image-analysis/
β βββ ...
βββ π examples/
βββ π resources/
βββ π guidelines/
βββ π evaluation/
βββ π LICENSE
βββ π README.md
This project is licensed under the MIT License.
If our work contributes to your research, please cite us:
@misc{medicalreasoningtasks,
title = {Open Medical Reasoning Tasks: A Comprehensive Collection of LLM Reasoning Tasks in Healthcare},
author = {Pal, Ankit and Open Life Science AI team},
url = {https://github.com/OpenLifeScienceAI/Medical-Reasoning-Tasks},
year = {2024},
}
Join us in revolutionizing healthcare with AI!
For Tasks:
Click tags to check more tools for each tasksFor Jobs:
Alternative AI tools for Open-Medical-Reasoning-Tasks
Similar Open Source Tools
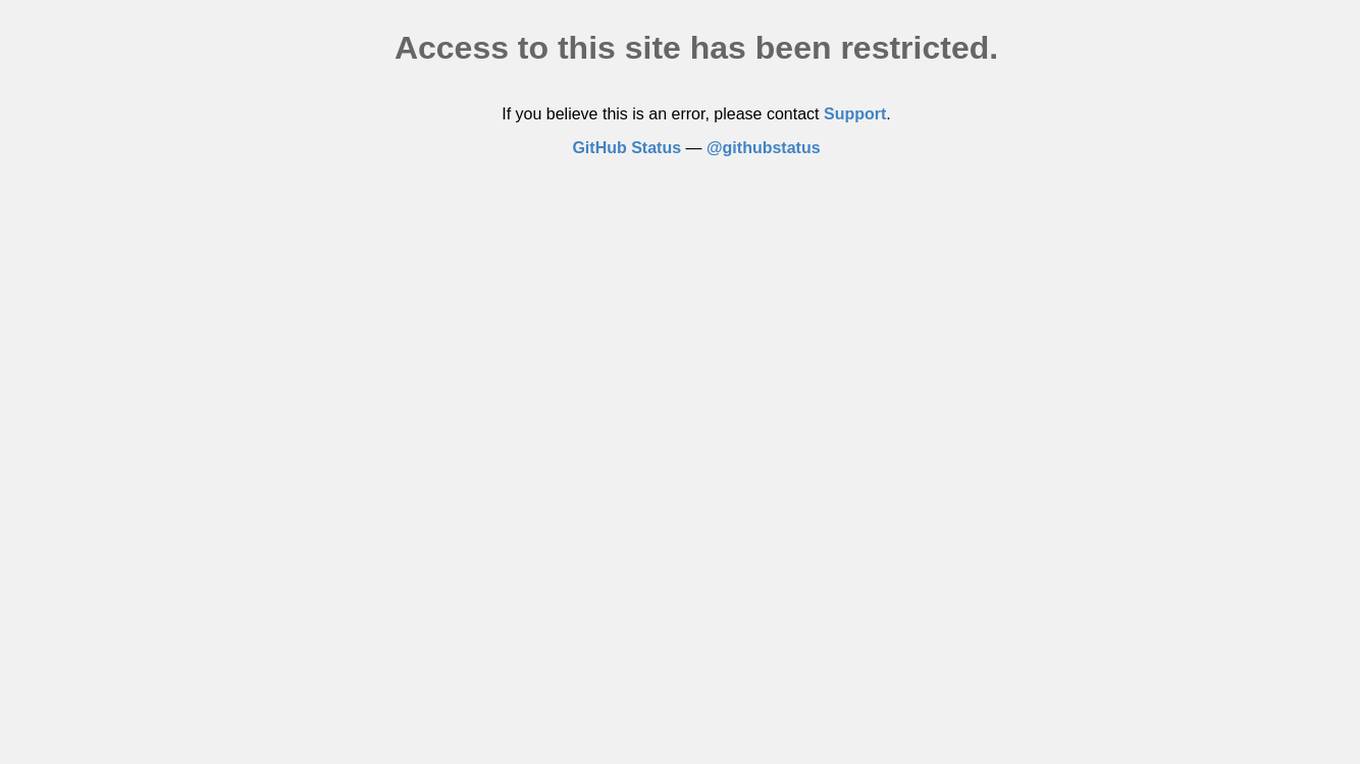
Open-Medical-Reasoning-Tasks
Open Life Science AI: Medical Reasoning Tasks is a collaborative hub for developing cutting-edge reasoning tasks for Large Language Models (LLMs) in the medical, healthcare, and clinical domains. The repository aims to advance AI capabilities in healthcare by fostering accurate diagnoses, personalized treatments, and improved patient outcomes. It offers a diverse range of medical reasoning challenges such as Diagnostic Reasoning, Treatment Planning, Medical Image Analysis, Clinical Data Interpretation, Patient History Analysis, Ethical Decision Making, Medical Literature Comprehension, and Drug Interaction Assessment. Contributors can join the community of healthcare professionals, AI researchers, and enthusiasts to contribute to the repository by creating new tasks or improvements following the provided guidelines. The repository also provides resources including a task list, evaluation metrics, medical AI papers, and healthcare datasets for training and evaluation.
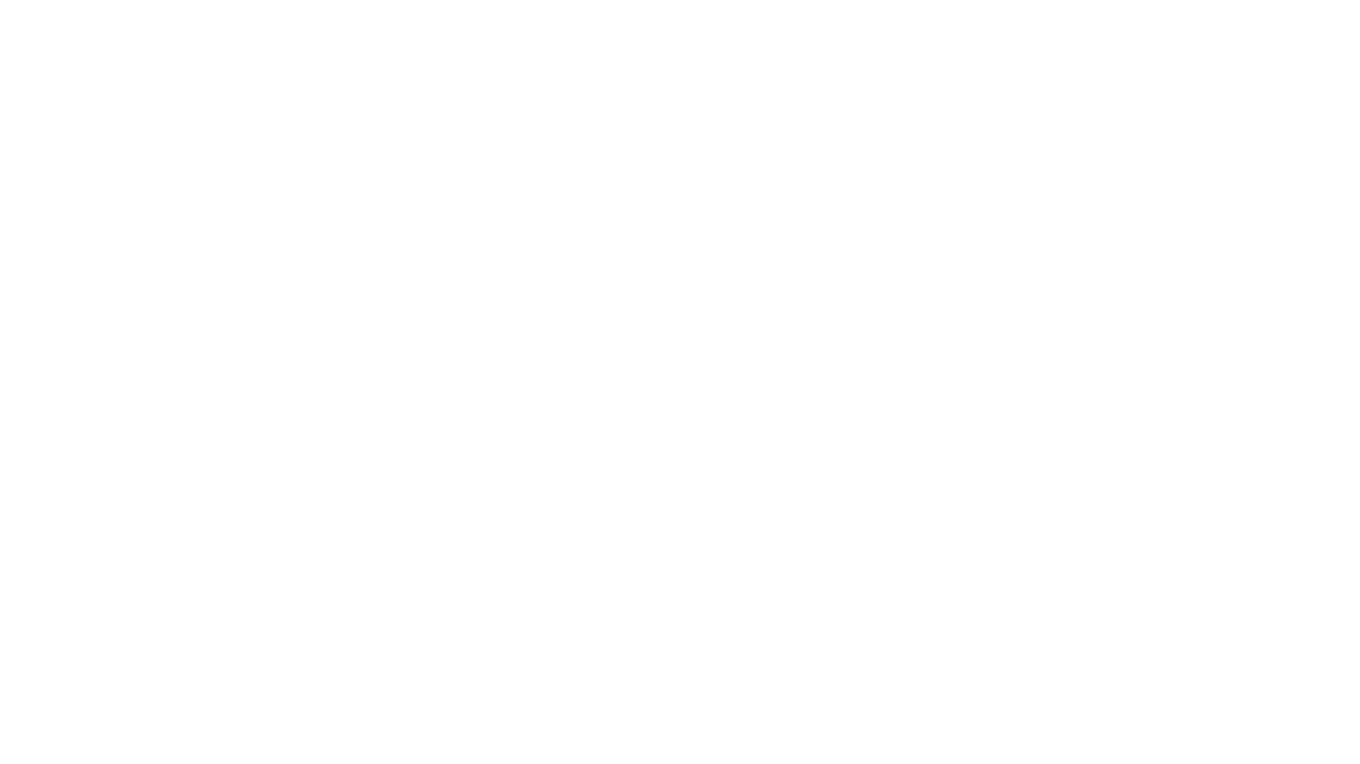
motia
Motia is an AI agent framework designed for software engineers to create, test, and deploy production-ready AI agents quickly. It provides a code-first approach, allowing developers to write agent logic in familiar languages and visualize execution in real-time. With Motia, developers can focus on business logic rather than infrastructure, offering zero infrastructure headaches, multi-language support, composable steps, built-in observability, instant APIs, and full control over AI logic. Ideal for building sophisticated agents and intelligent automations, Motia's event-driven architecture and modular steps enable the creation of GenAI-powered workflows, decision-making systems, and data processing pipelines.
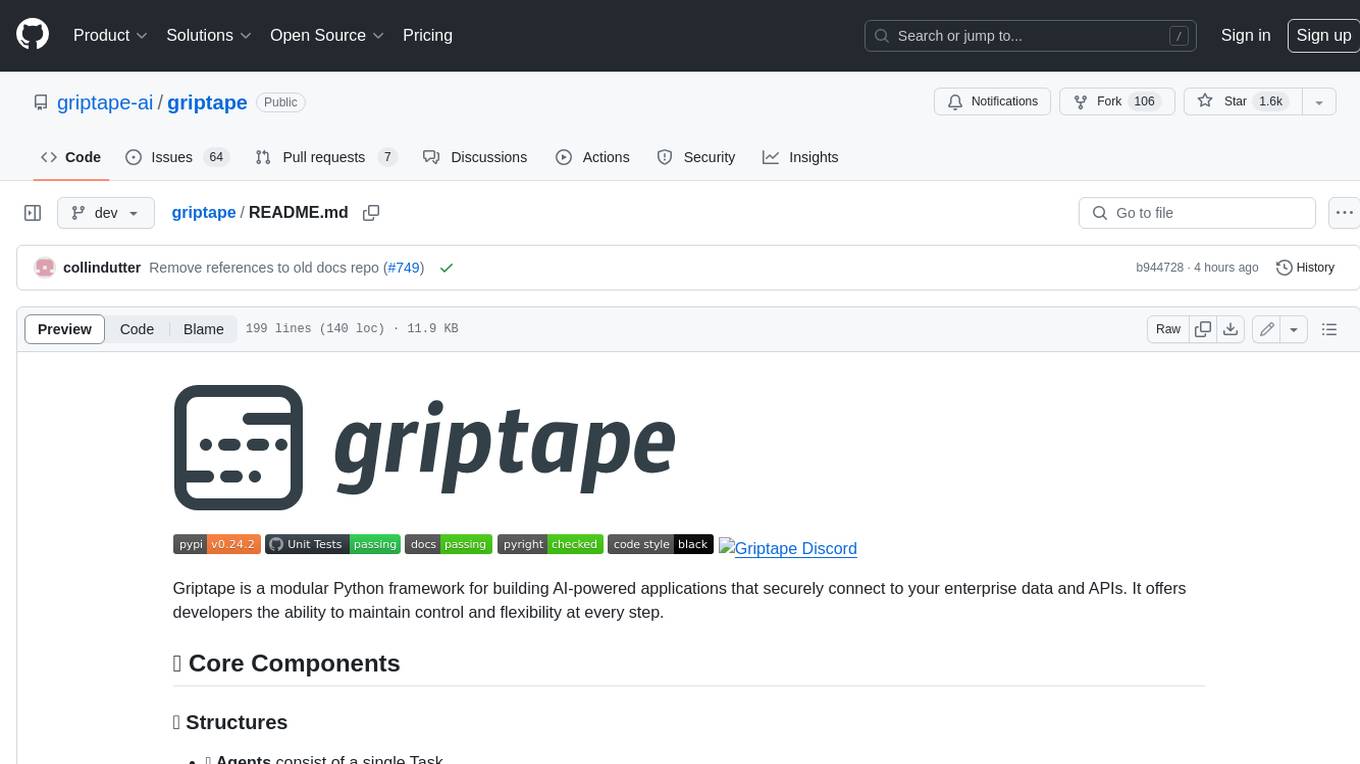
griptape
Griptape is a modular Python framework for building AI-powered applications that securely connect to your enterprise data and APIs. It offers developers the ability to maintain control and flexibility at every step. Griptape's core components include Structures (Agents, Pipelines, and Workflows), Tasks, Tools, Memory (Conversation Memory, Task Memory, and Meta Memory), Drivers (Prompt and Embedding Drivers, Vector Store Drivers, Image Generation Drivers, Image Query Drivers, SQL Drivers, Web Scraper Drivers, and Conversation Memory Drivers), Engines (Query Engines, Extraction Engines, Summary Engines, Image Generation Engines, and Image Query Engines), and additional components (Rulesets, Loaders, Artifacts, Chunkers, and Tokenizers). Griptape enables developers to create AI-powered applications with ease and efficiency.
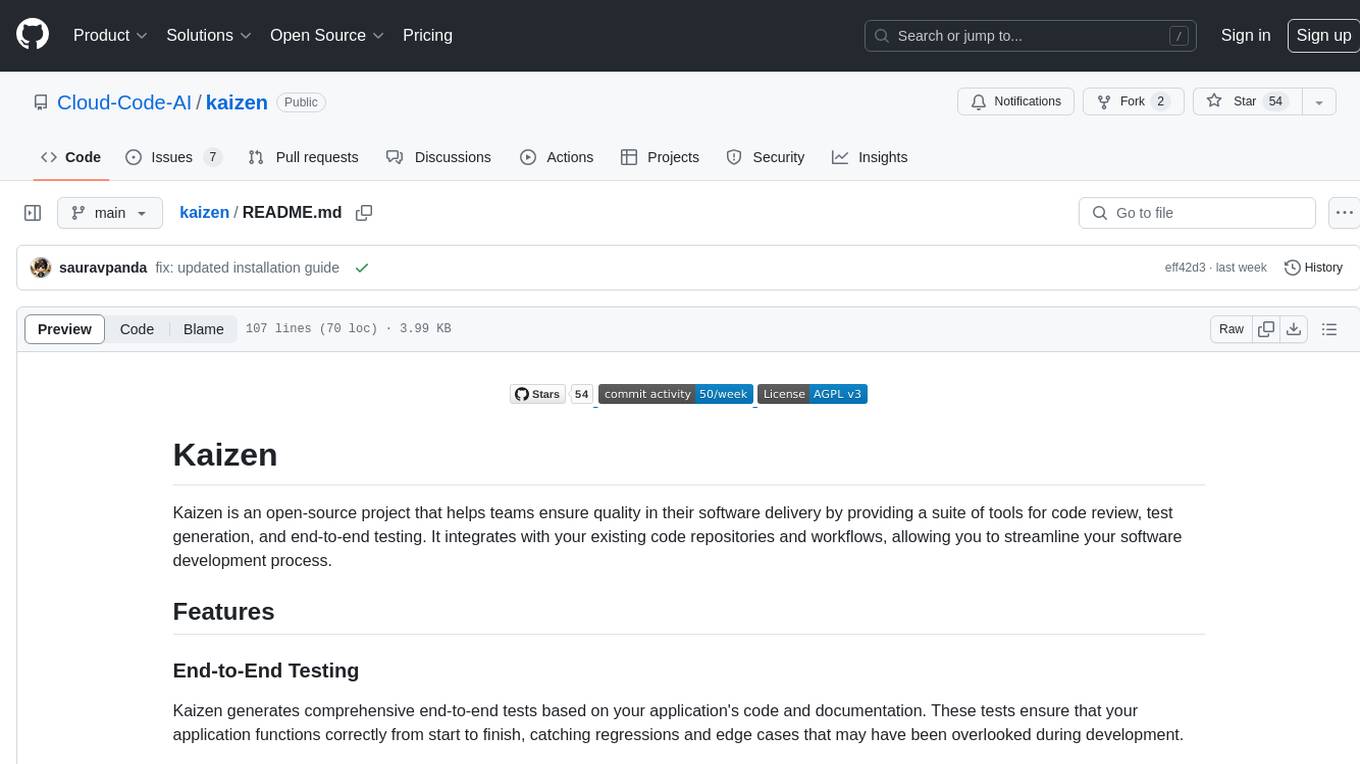
kaizen
Kaizen is an open-source project that helps teams ensure quality in their software delivery by providing a suite of tools for code review, test generation, and end-to-end testing. It integrates with your existing code repositories and workflows, allowing you to streamline your software development process. Kaizen generates comprehensive end-to-end tests, provides UI testing and review, and automates code review with insightful feedback. The file structure includes components for API server, logic, actors, generators, LLM integrations, documentation, and sample code. Getting started involves installing the Kaizen package, generating tests for websites, and executing tests. The tool also runs an API server for GitHub App actions. Contributions are welcome under the AGPL License.
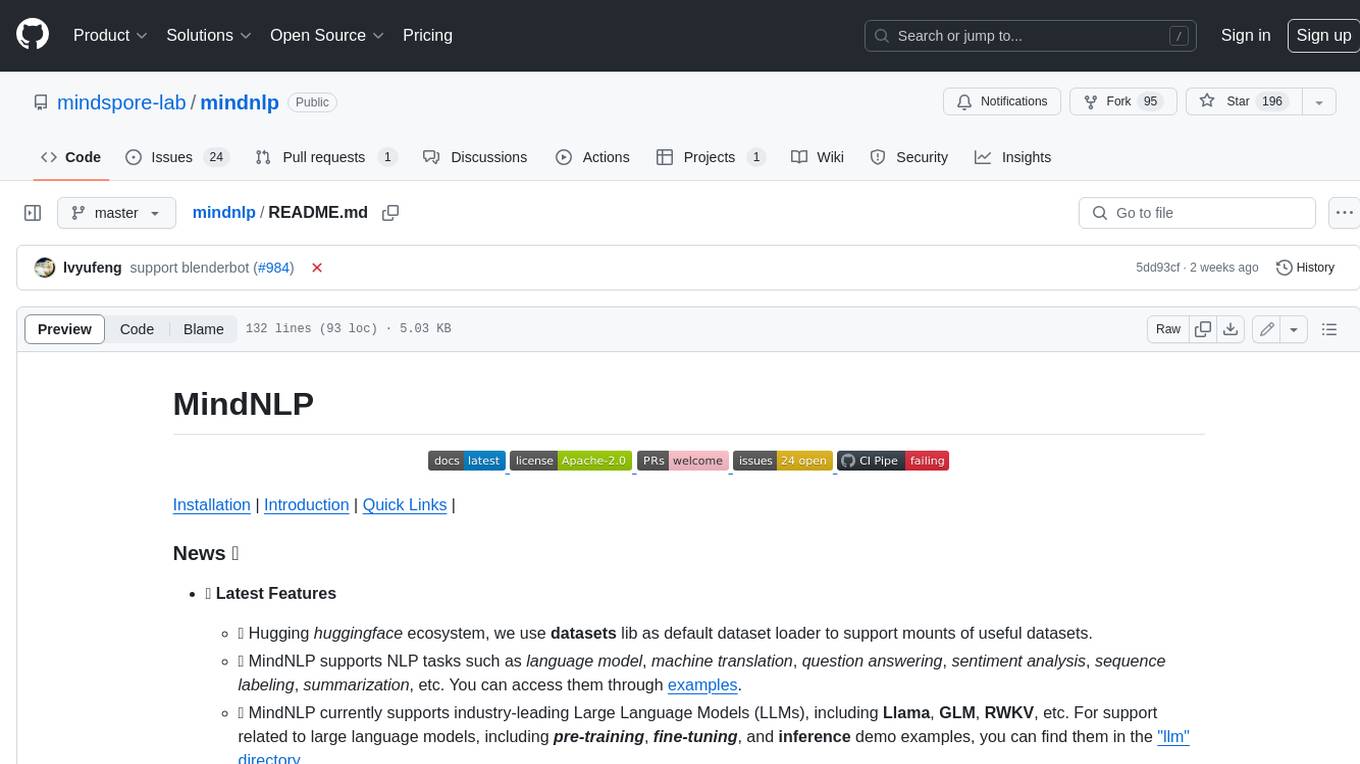
mindnlp
MindNLP is an open-source NLP library based on MindSpore. It provides a platform for solving natural language processing tasks, containing many common approaches in NLP. It can help researchers and developers to construct and train models more conveniently and rapidly. Key features of MindNLP include: * Comprehensive data processing: Several classical NLP datasets are packaged into a friendly module for easy use, such as Multi30k, SQuAD, CoNLL, etc. * Friendly NLP model toolset: MindNLP provides various configurable components. It is friendly to customize models using MindNLP. * Easy-to-use engine: MindNLP simplified complicated training process in MindSpore. It supports Trainer and Evaluator interfaces to train and evaluate models easily. MindNLP supports a wide range of NLP tasks, including: * Language modeling * Machine translation * Question answering * Sentiment analysis * Sequence labeling * Summarization MindNLP also supports industry-leading Large Language Models (LLMs), including Llama, GLM, RWKV, etc. For support related to large language models, including pre-training, fine-tuning, and inference demo examples, you can find them in the "llm" directory. To install MindNLP, you can either install it from Pypi, download the daily build wheel, or install it from source. The installation instructions are provided in the documentation. MindNLP is released under the Apache 2.0 license. If you find this project useful in your research, please consider citing the following paper: @misc{mindnlp2022, title={{MindNLP}: a MindSpore NLP library}, author={MindNLP Contributors}, howpublished = {\url{https://github.com/mindlab-ai/mindnlp}}, year={2022} }
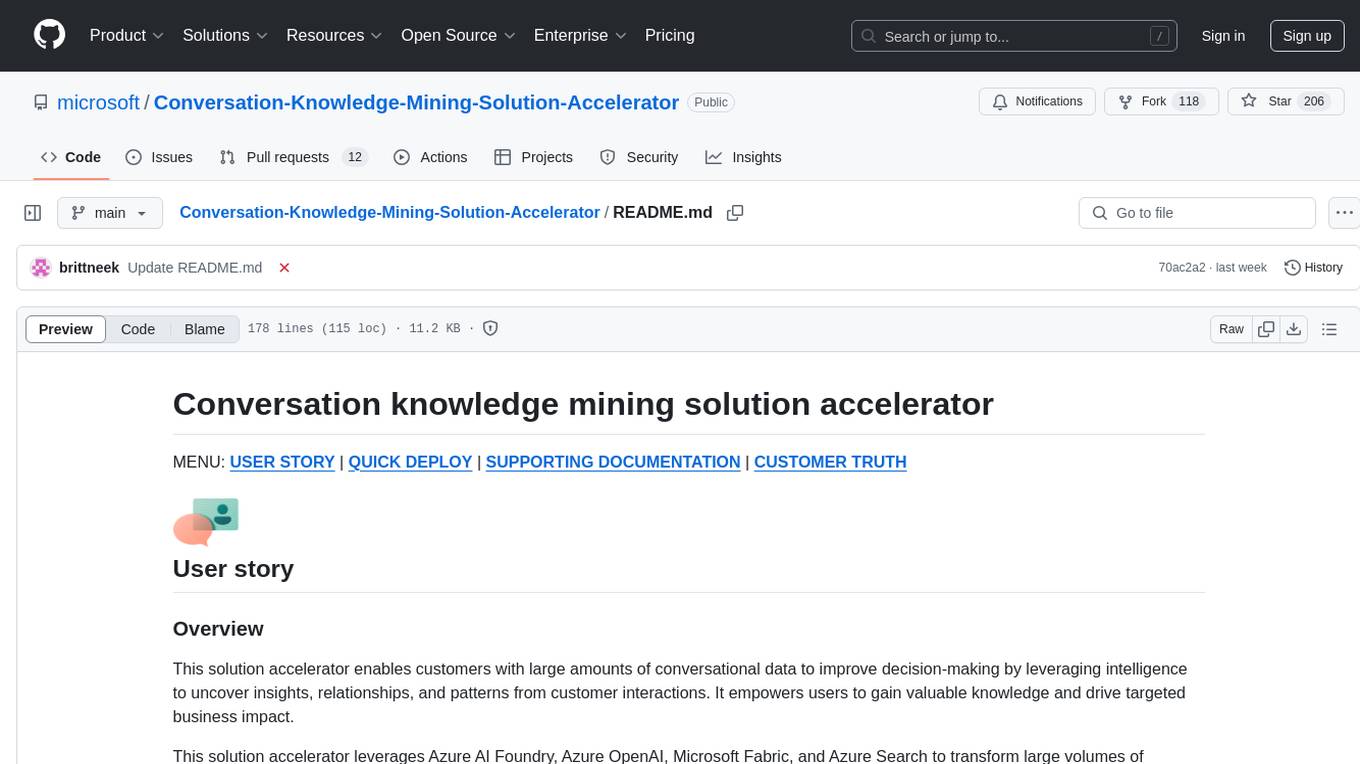
Conversation-Knowledge-Mining-Solution-Accelerator
The Conversation Knowledge Mining Solution Accelerator enables customers to leverage intelligence to uncover insights, relationships, and patterns from conversational data. It empowers users to gain valuable knowledge and drive targeted business impact by utilizing Azure AI Foundry, Azure OpenAI, Microsoft Fabric, and Azure Search for topic modeling, key phrase extraction, speech-to-text transcription, and interactive chat experiences.
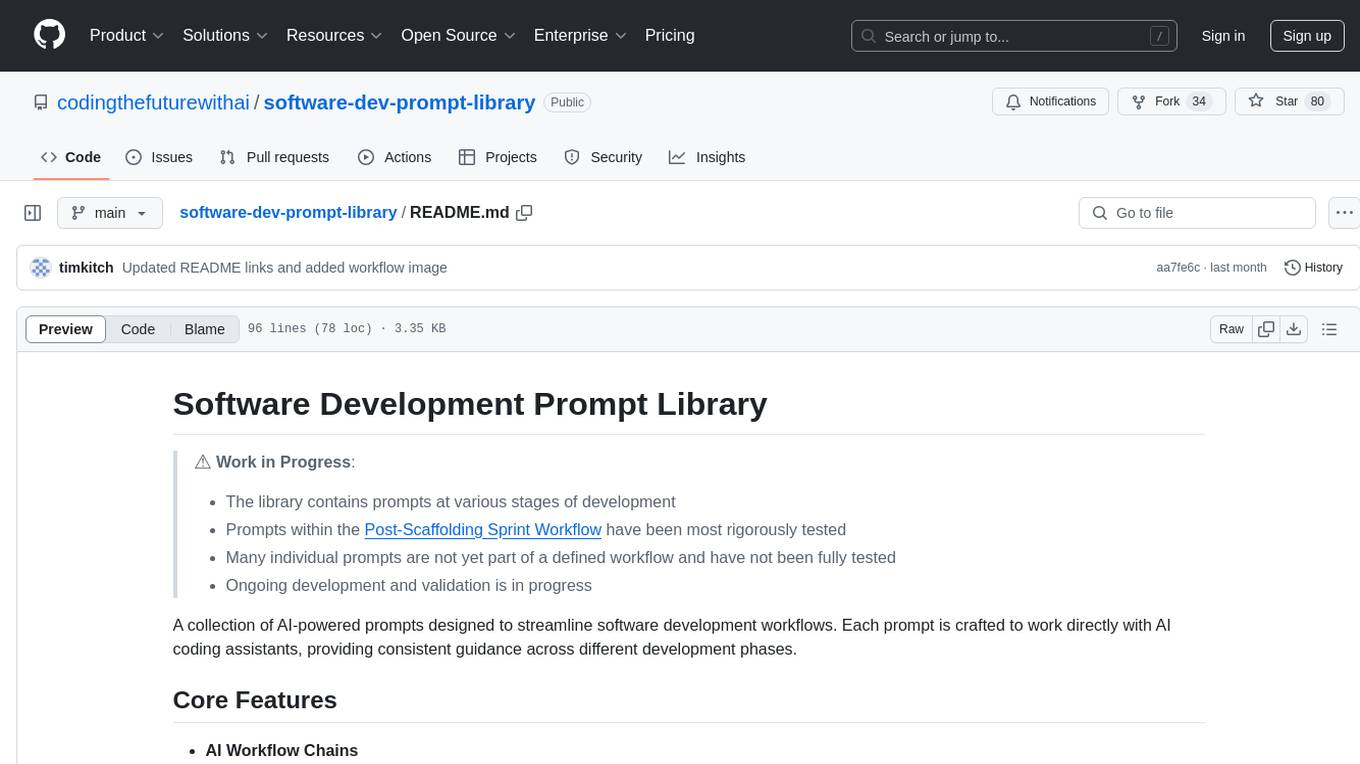
software-dev-prompt-library
A collection of AI-powered prompts designed to streamline software development workflows. The library contains prompts at various stages of development, with structured sequences of connected prompts, project initialization support, development assistance, and documentation generation. It aims to provide consistent guidance across different development phases, promote systematic development processes, and enable progress tracking and validation.
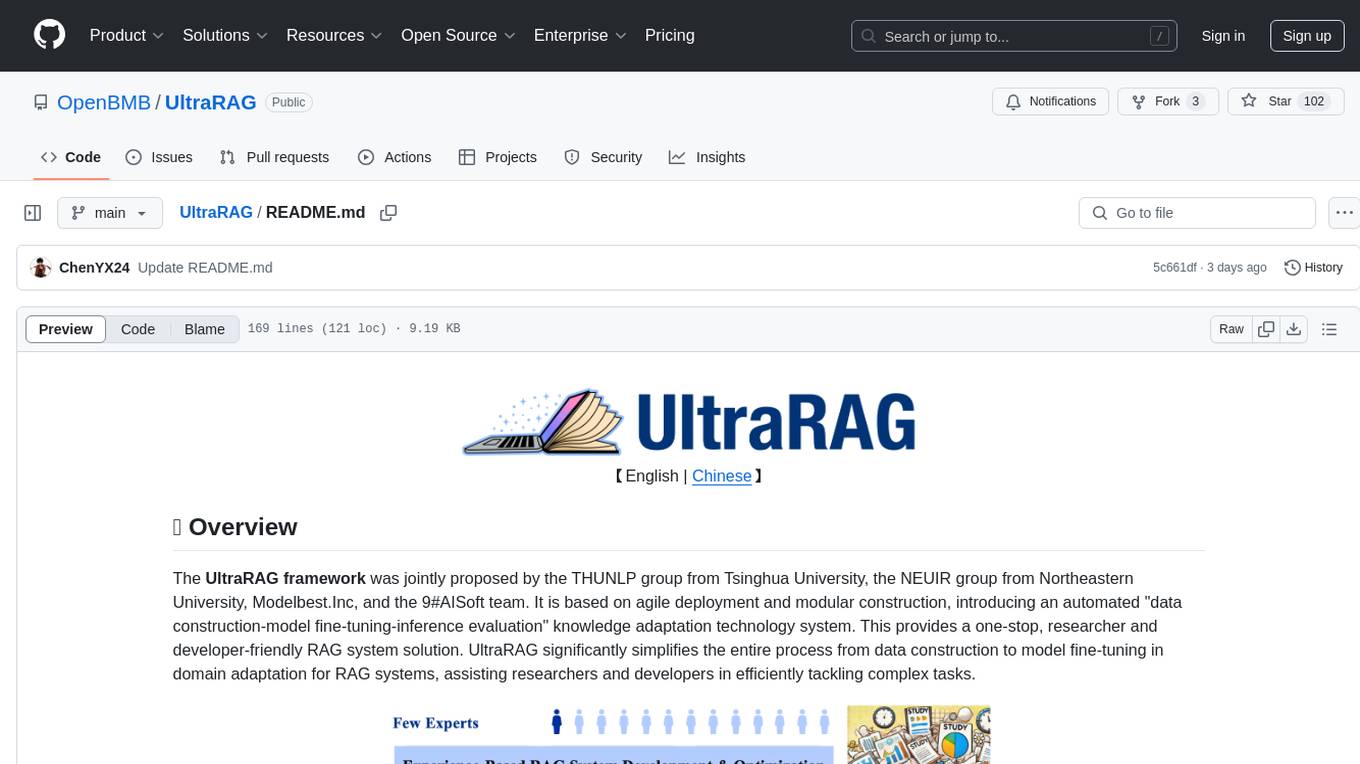
UltraRAG
The UltraRAG framework is a researcher and developer-friendly RAG system solution that simplifies the process from data construction to model fine-tuning in domain adaptation. It introduces an automated knowledge adaptation technology system, supporting no-code programming, one-click synthesis and fine-tuning, multidimensional evaluation, and research-friendly exploration work integration. The architecture consists of Frontend, Service, and Backend components, offering flexibility in customization and optimization. Performance evaluation in the legal field shows improved results compared to VanillaRAG, with specific metrics provided. The repository is licensed under Apache-2.0 and encourages citation for support.
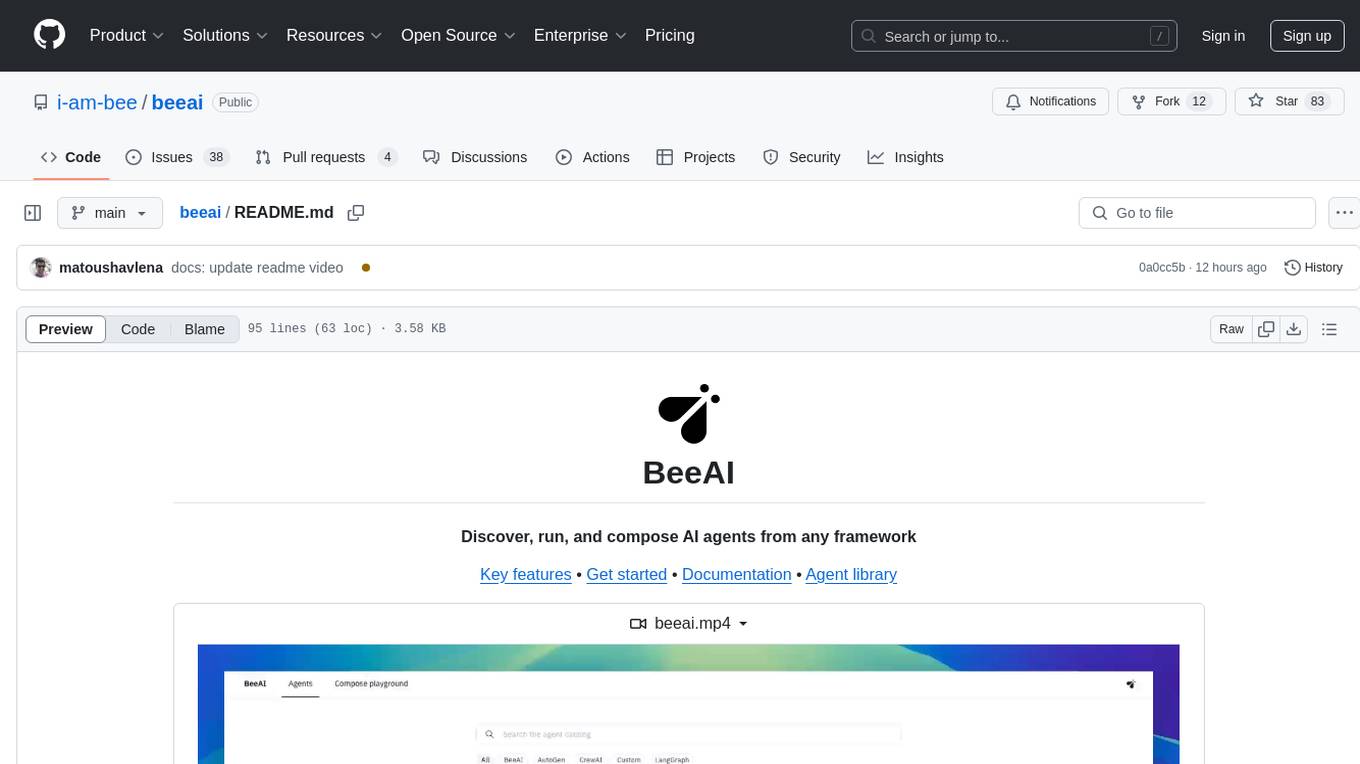
beeai
BeeAI is an open platform that helps users discover, run, and compose AI agents from any framework and language. It offers a framework-agnostic approach, allowing seamless integration of AI agents regardless of the language or platform. Users can build complex workflows using simple building blocks, explore a catalog of powerful agents with integrated search, and benefit from the BeeAI ecosystem with first-class support for Python and TypeScript agent developers.
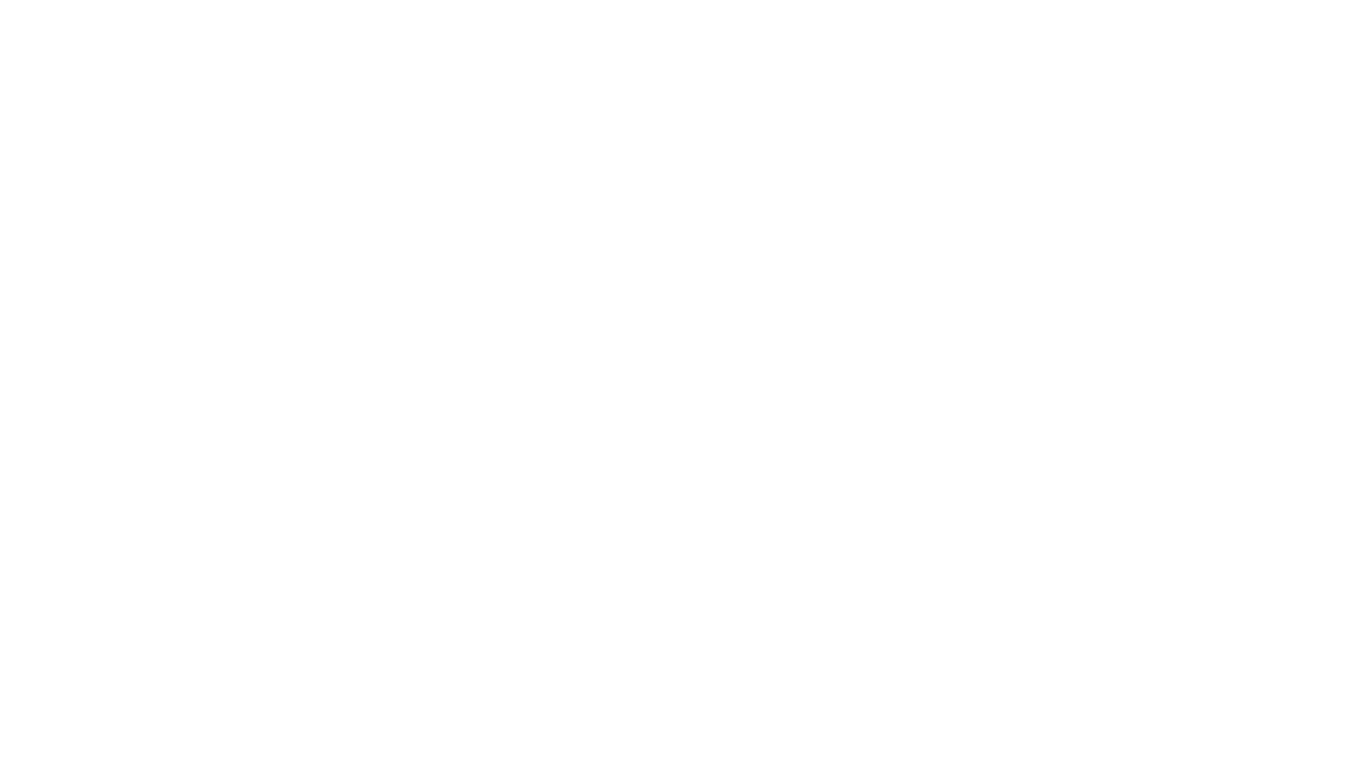
ai-data-science-team
The AI Data Science Team of Copilots is an AI-powered data science team that uses agents to help users perform common data science tasks 10X faster. It includes agents specializing in data cleaning, preparation, feature engineering, modeling, and interpretation of business problems. The project is a work in progress with new data science agents to be released soon. Disclaimer: This project is for educational purposes only and not intended to replace a company's data science team. No warranties or guarantees are provided, and the creator assumes no liability for financial loss.
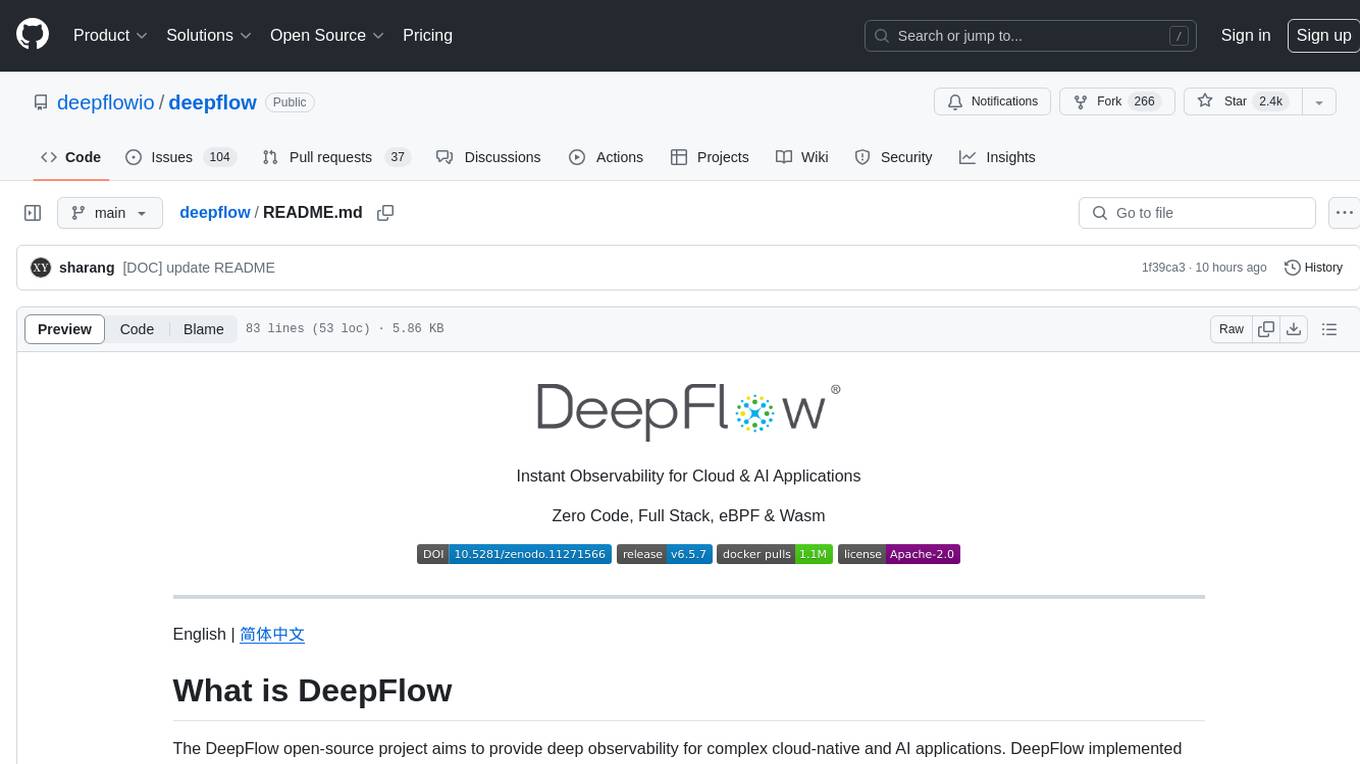
deepflow
DeepFlow is an open-source project that provides deep observability for complex cloud-native and AI applications. It offers Zero Code data collection with eBPF for metrics, distributed tracing, request logs, and function profiling. DeepFlow is integrated with SmartEncoding to achieve Full Stack correlation and efficient access to all observability data. With DeepFlow, cloud-native and AI applications automatically gain deep observability, removing the burden of developers continually instrumenting code and providing monitoring and diagnostic capabilities covering everything from code to infrastructure for DevOps/SRE teams.
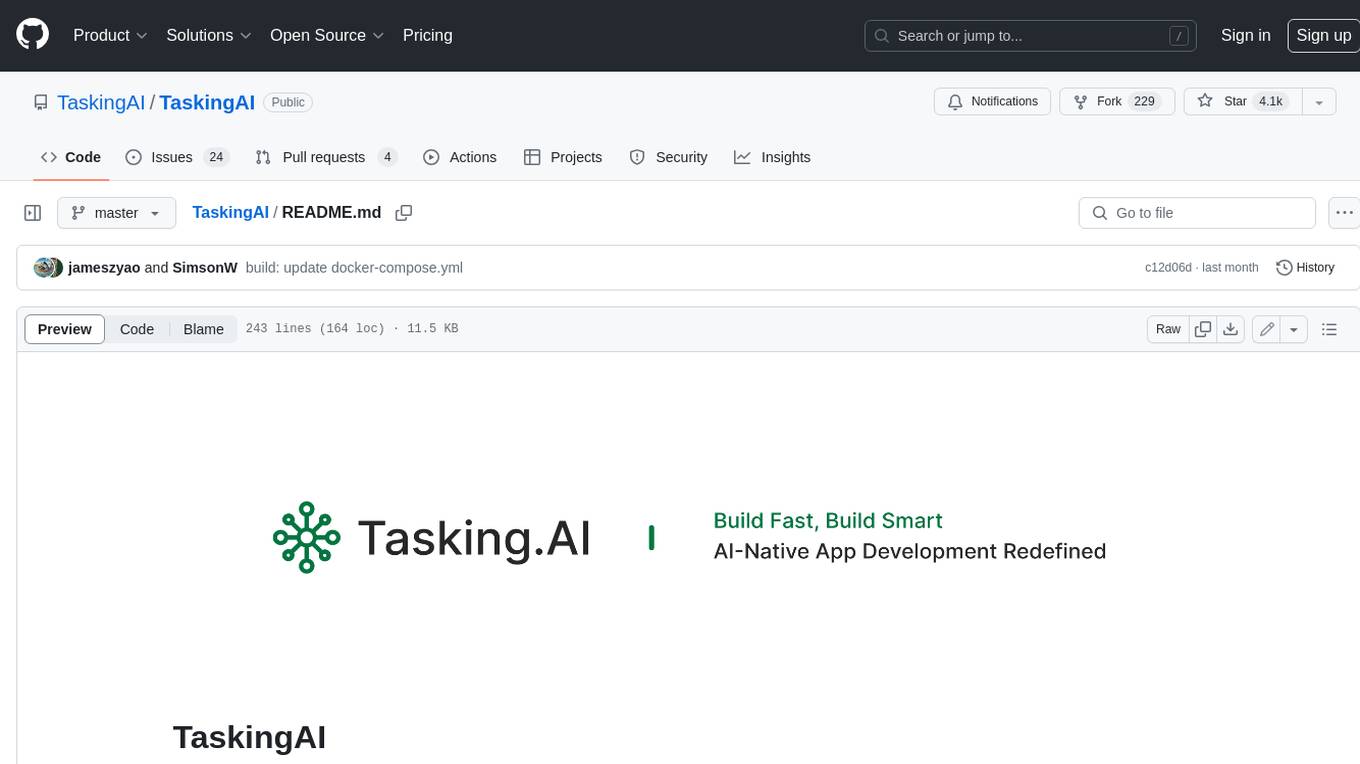
TaskingAI
TaskingAI brings Firebase's simplicity to **AI-native app development**. The platform enables the creation of GPTs-like multi-tenant applications using a wide range of LLMs from various providers. It features distinct, modular functions such as Inference, Retrieval, Assistant, and Tool, seamlessly integrated to enhance the development process. TaskingAIβs cohesive design ensures an efficient, intelligent, and user-friendly experience in AI application development.
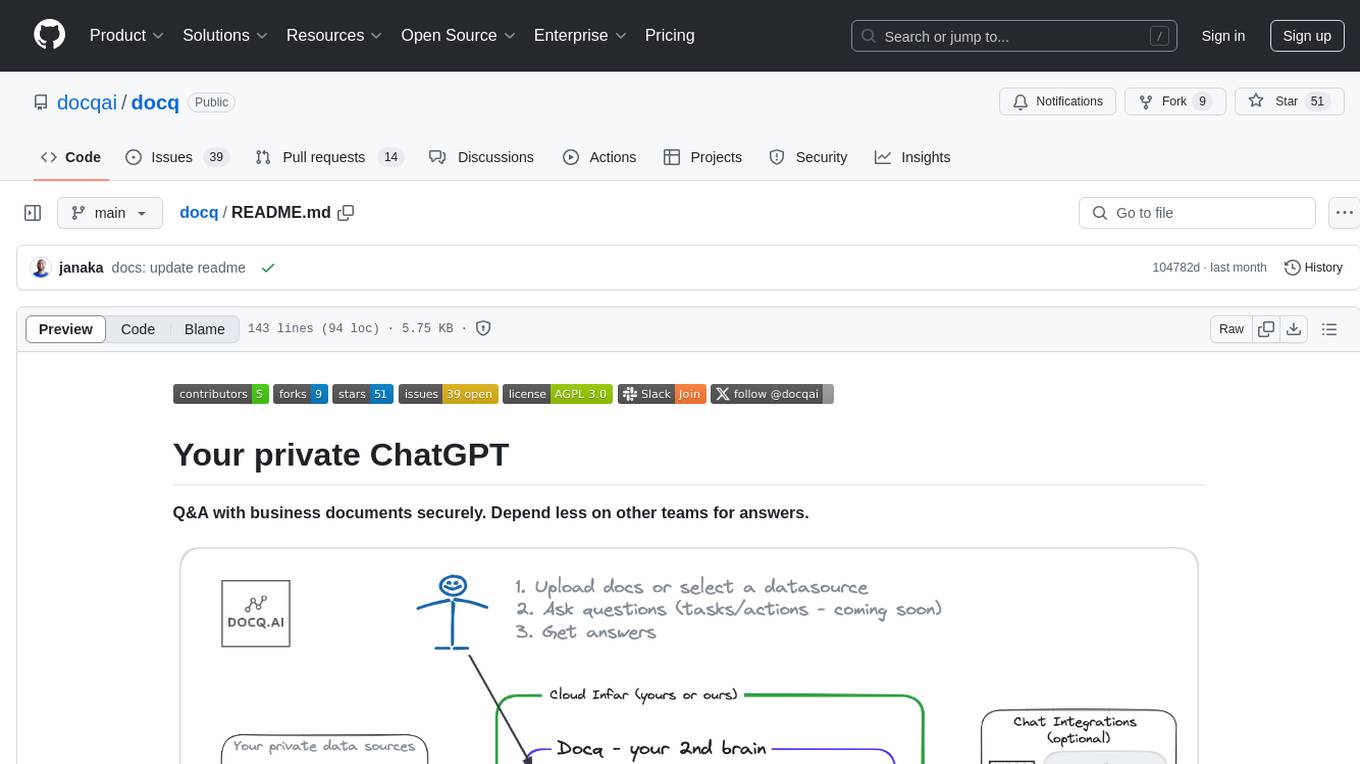
docq
Docq is a private and secure GenAI tool designed to extract knowledge from business documents, enabling users to find answers independently. It allows data to stay within organizational boundaries, supports self-hosting with various cloud vendors, and offers multi-model and multi-modal capabilities. Docq is extensible, open-source (AGPLv3), and provides commercial licensing options. The tool aims to be a turnkey solution for organizations to adopt AI innovation safely, with plans for future features like more data ingestion options and model fine-tuning.
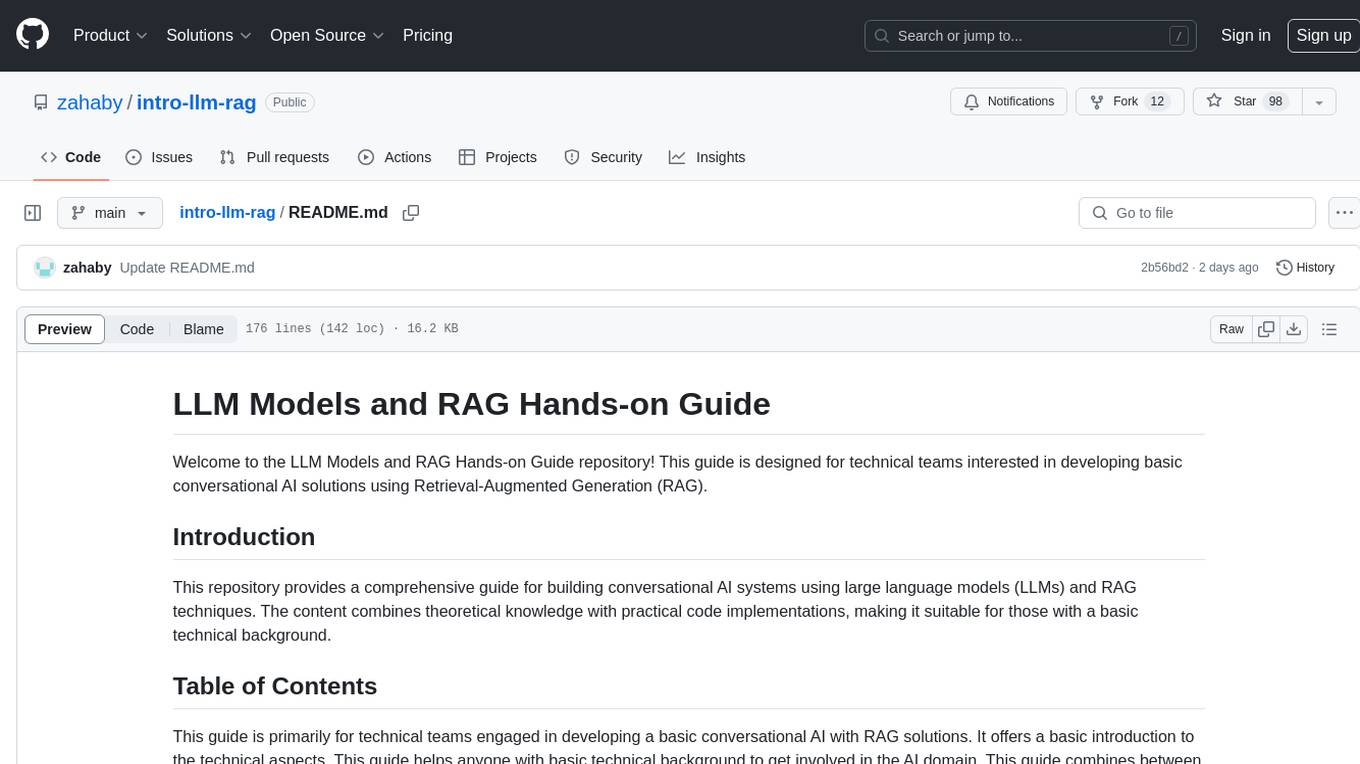
intro-llm-rag
This repository serves as a comprehensive guide for technical teams interested in developing conversational AI solutions using Retrieval-Augmented Generation (RAG) techniques. It covers theoretical knowledge and practical code implementations, making it suitable for individuals with a basic technical background. The content includes information on large language models (LLMs), transformers, prompt engineering, embeddings, vector stores, and various other key concepts related to conversational AI. The repository also provides hands-on examples for two different use cases, along with implementation details and performance analysis.
For similar tasks
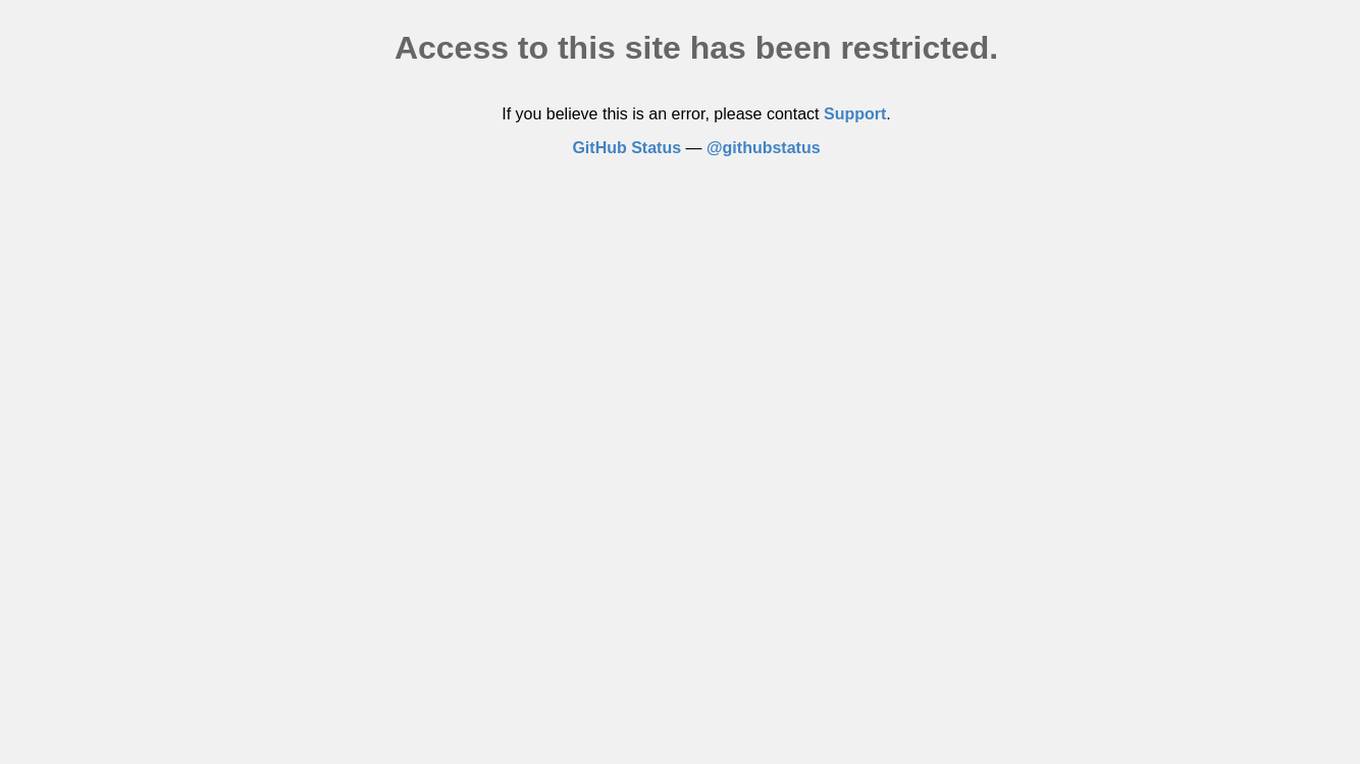
Open-Medical-Reasoning-Tasks
Open Life Science AI: Medical Reasoning Tasks is a collaborative hub for developing cutting-edge reasoning tasks for Large Language Models (LLMs) in the medical, healthcare, and clinical domains. The repository aims to advance AI capabilities in healthcare by fostering accurate diagnoses, personalized treatments, and improved patient outcomes. It offers a diverse range of medical reasoning challenges such as Diagnostic Reasoning, Treatment Planning, Medical Image Analysis, Clinical Data Interpretation, Patient History Analysis, Ethical Decision Making, Medical Literature Comprehension, and Drug Interaction Assessment. Contributors can join the community of healthcare professionals, AI researchers, and enthusiasts to contribute to the repository by creating new tasks or improvements following the provided guidelines. The repository also provides resources including a task list, evaluation metrics, medical AI papers, and healthcare datasets for training and evaluation.
For similar jobs
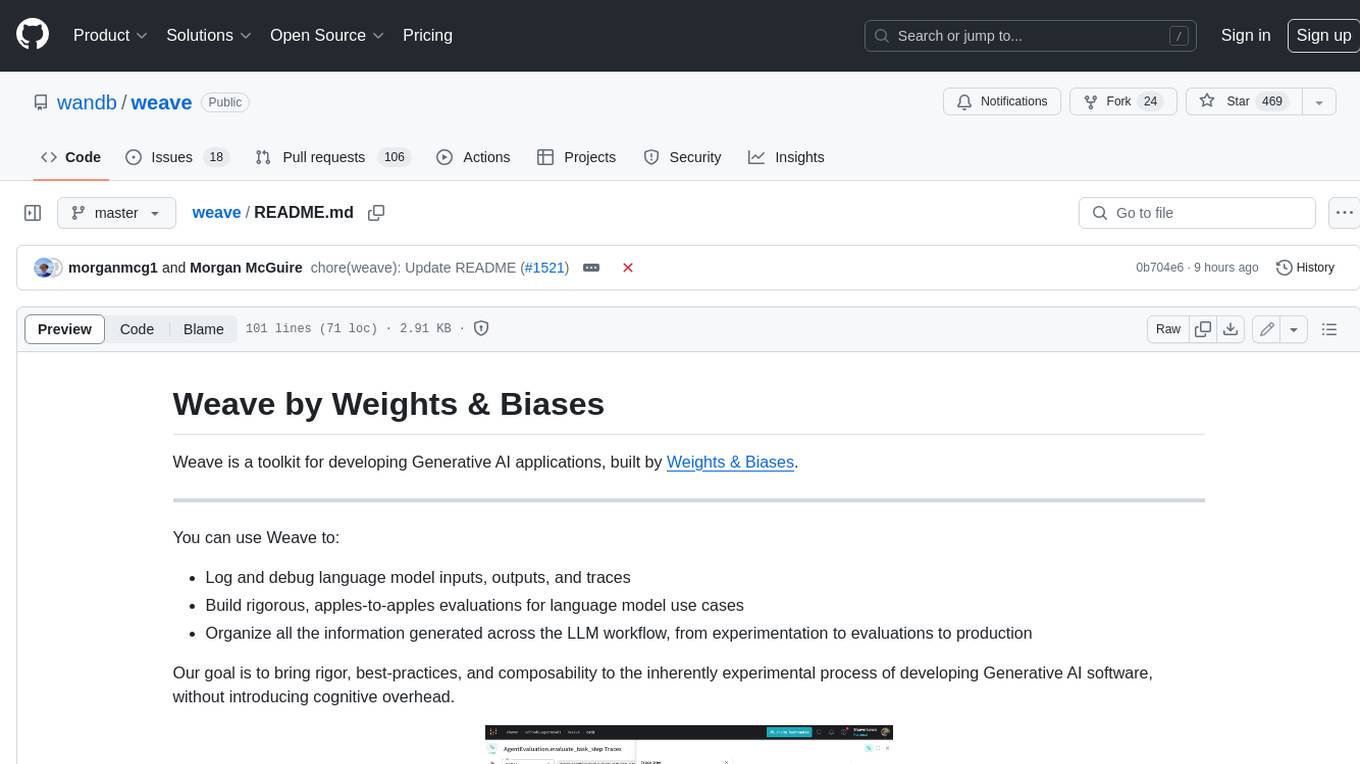
weave
Weave is a toolkit for developing Generative AI applications, built by Weights & Biases. With Weave, you can log and debug language model inputs, outputs, and traces; build rigorous, apples-to-apples evaluations for language model use cases; and organize all the information generated across the LLM workflow, from experimentation to evaluations to production. Weave aims to bring rigor, best-practices, and composability to the inherently experimental process of developing Generative AI software, without introducing cognitive overhead.
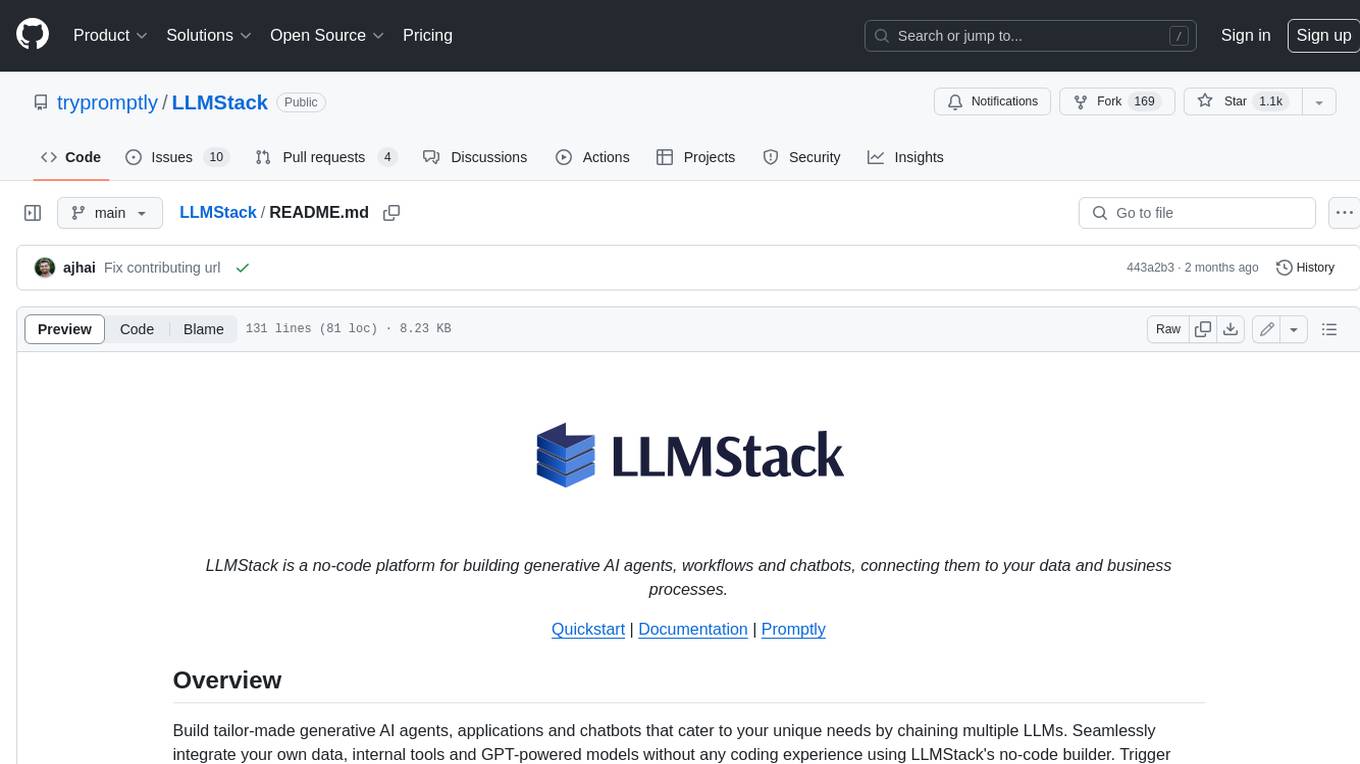
LLMStack
LLMStack is a no-code platform for building generative AI agents, workflows, and chatbots. It allows users to connect their own data, internal tools, and GPT-powered models without any coding experience. LLMStack can be deployed to the cloud or on-premise and can be accessed via HTTP API or triggered from Slack or Discord.
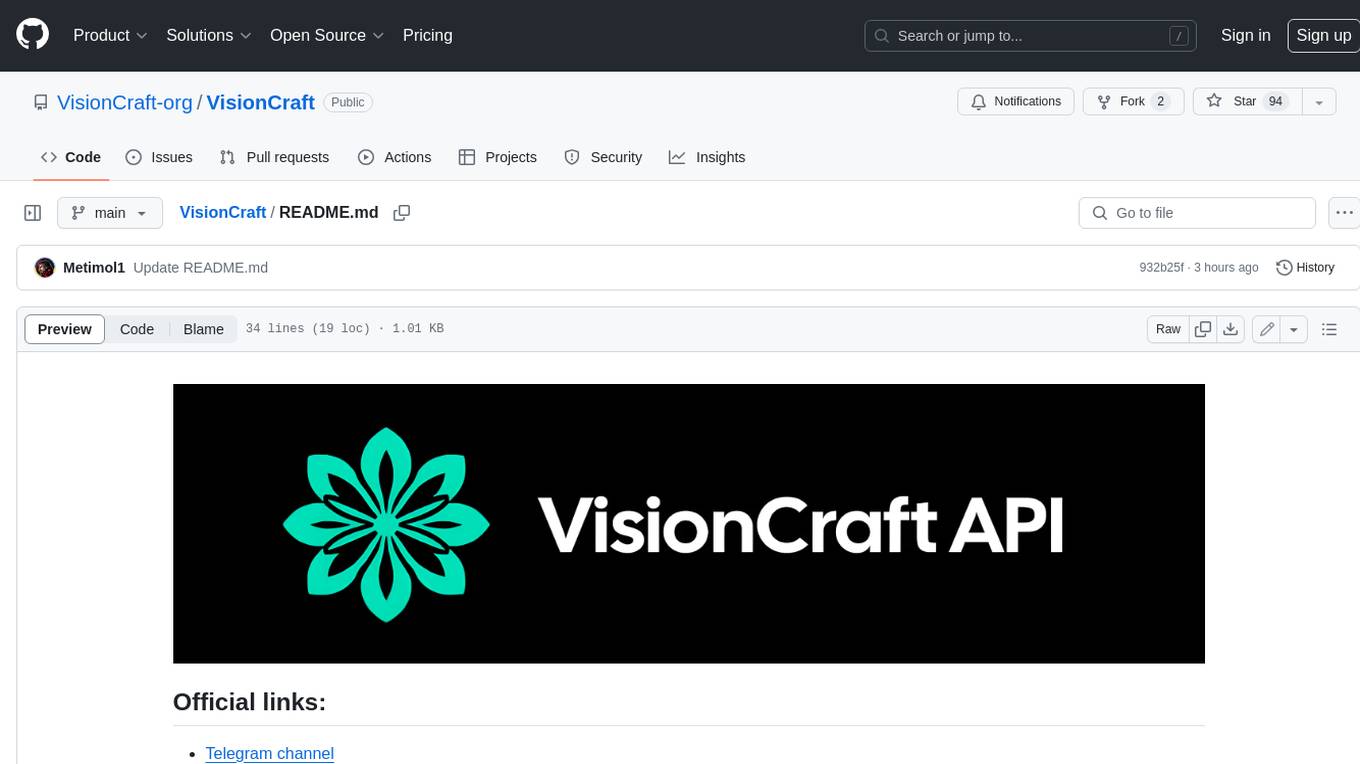
VisionCraft
The VisionCraft API is a free API for using over 100 different AI models. From images to sound.
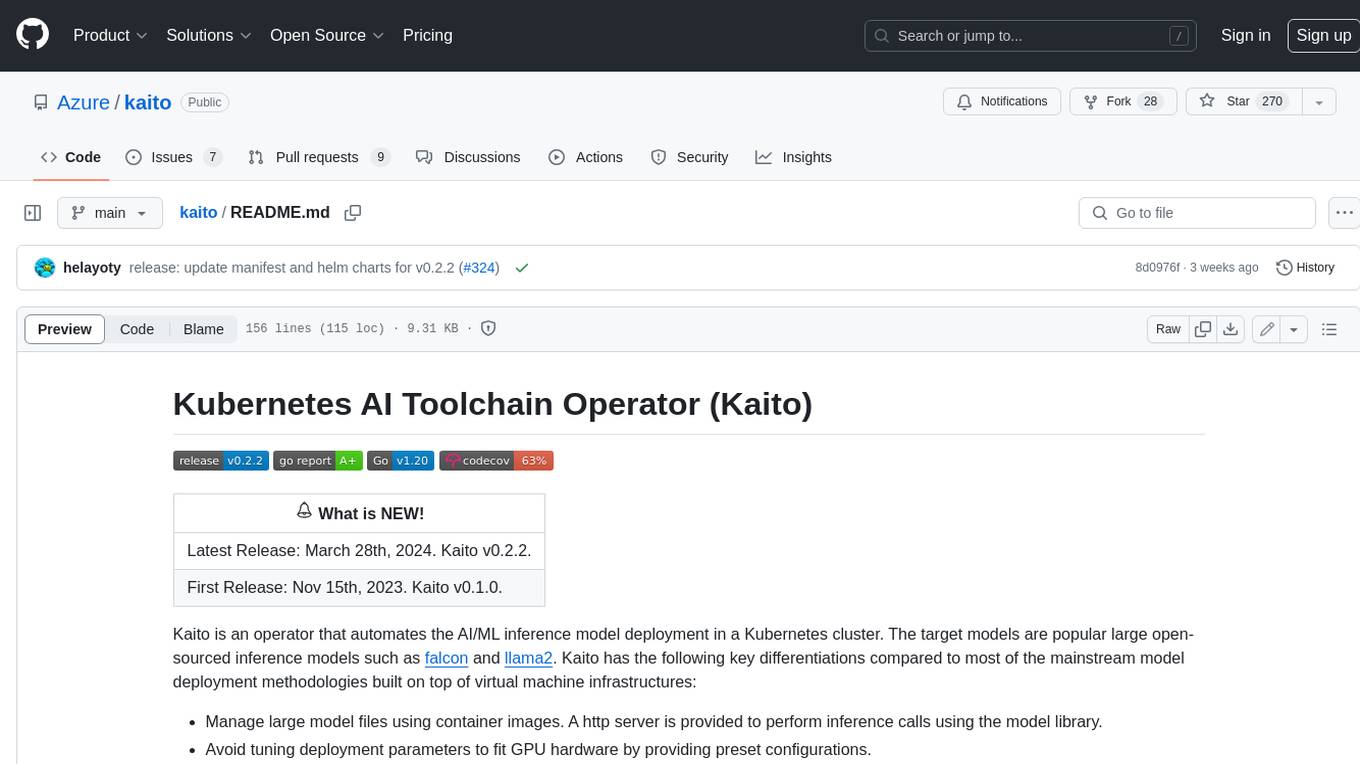
kaito
Kaito is an operator that automates the AI/ML inference model deployment in a Kubernetes cluster. It manages large model files using container images, avoids tuning deployment parameters to fit GPU hardware by providing preset configurations, auto-provisions GPU nodes based on model requirements, and hosts large model images in the public Microsoft Container Registry (MCR) if the license allows. Using Kaito, the workflow of onboarding large AI inference models in Kubernetes is largely simplified.
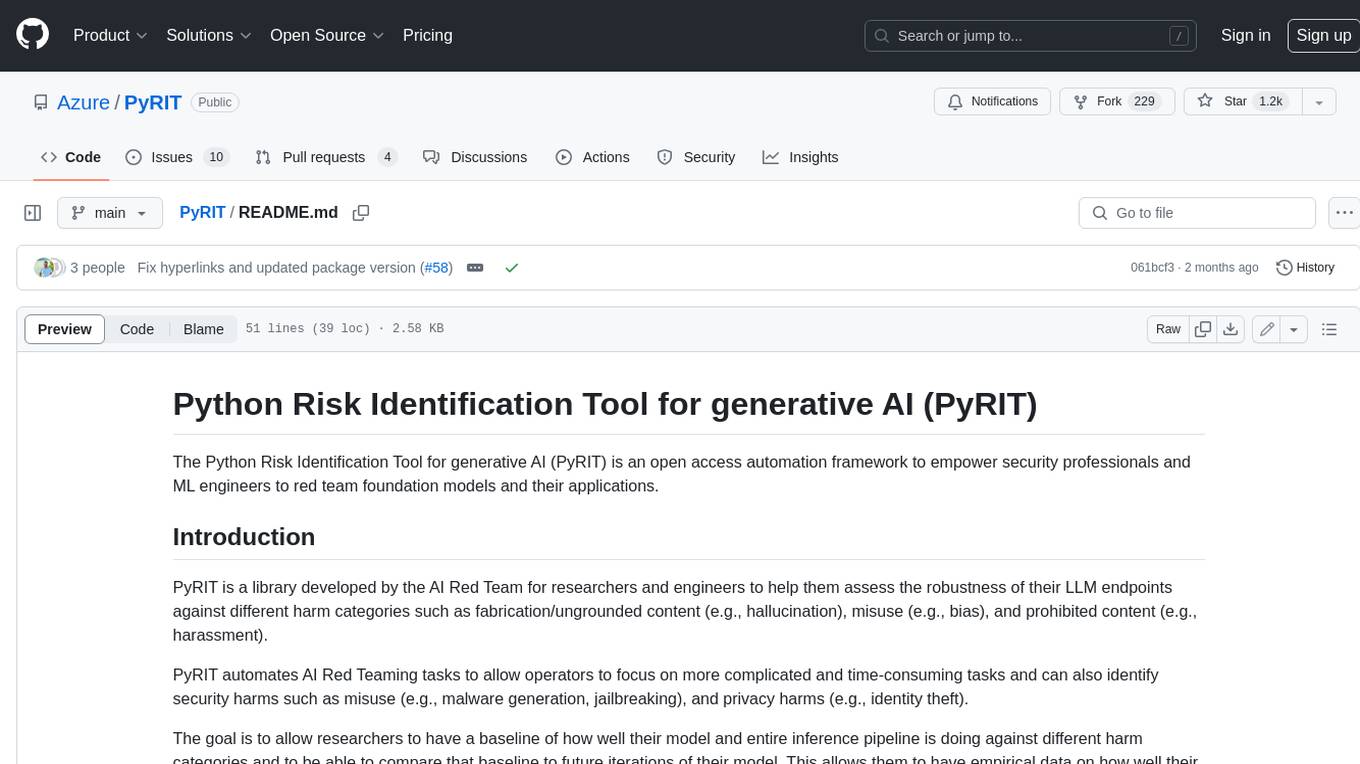
PyRIT
PyRIT is an open access automation framework designed to empower security professionals and ML engineers to red team foundation models and their applications. It automates AI Red Teaming tasks to allow operators to focus on more complicated and time-consuming tasks and can also identify security harms such as misuse (e.g., malware generation, jailbreaking), and privacy harms (e.g., identity theft). The goal is to allow researchers to have a baseline of how well their model and entire inference pipeline is doing against different harm categories and to be able to compare that baseline to future iterations of their model. This allows them to have empirical data on how well their model is doing today, and detect any degradation of performance based on future improvements.
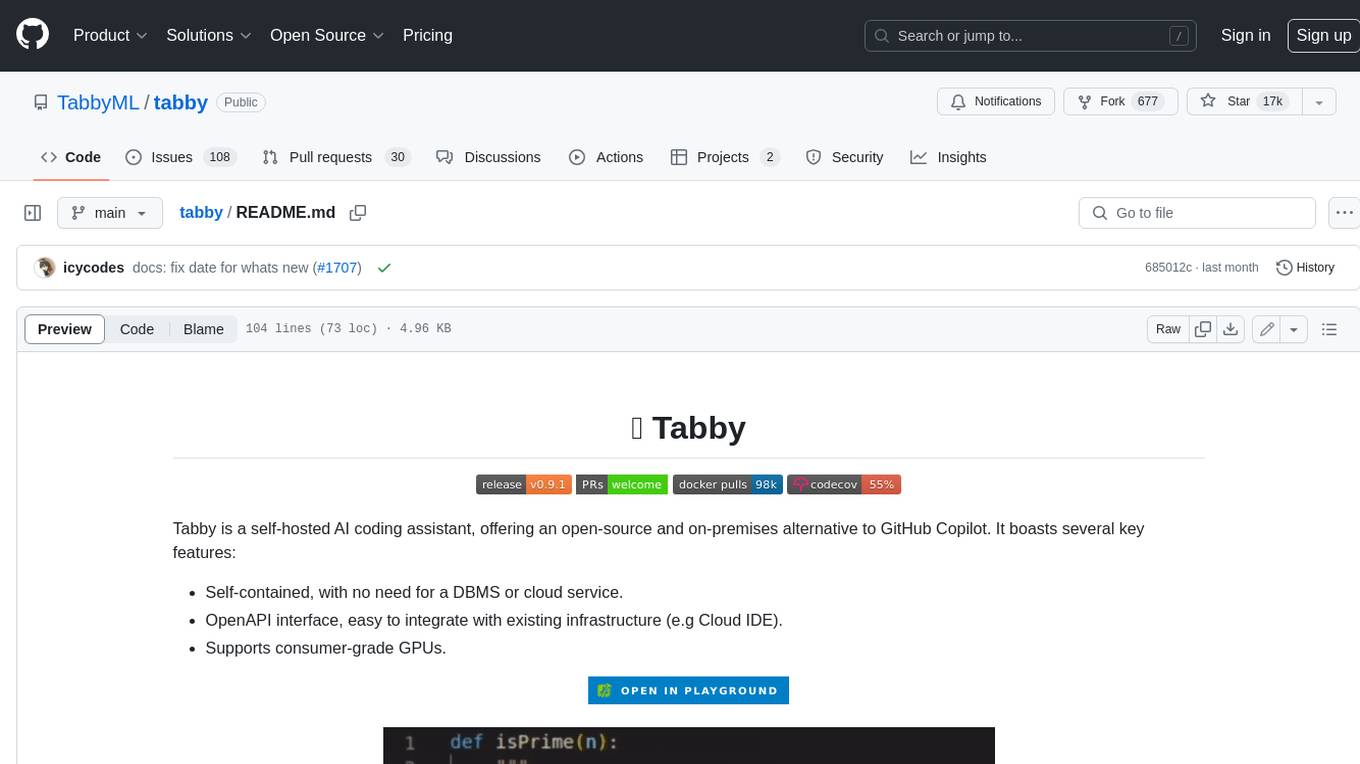
tabby
Tabby is a self-hosted AI coding assistant, offering an open-source and on-premises alternative to GitHub Copilot. It boasts several key features: * Self-contained, with no need for a DBMS or cloud service. * OpenAPI interface, easy to integrate with existing infrastructure (e.g Cloud IDE). * Supports consumer-grade GPUs.
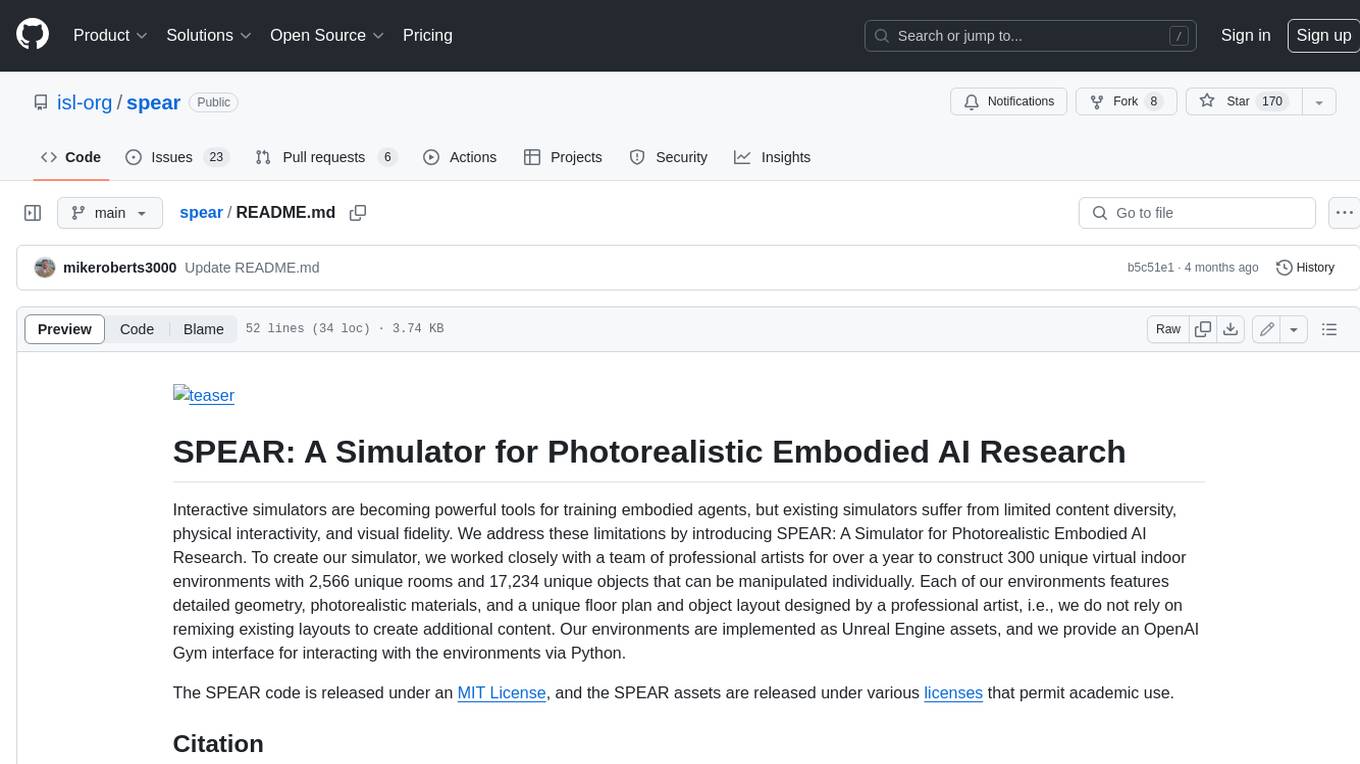
spear
SPEAR (Simulator for Photorealistic Embodied AI Research) is a powerful tool for training embodied agents. It features 300 unique virtual indoor environments with 2,566 unique rooms and 17,234 unique objects that can be manipulated individually. Each environment is designed by a professional artist and features detailed geometry, photorealistic materials, and a unique floor plan and object layout. SPEAR is implemented as Unreal Engine assets and provides an OpenAI Gym interface for interacting with the environments via Python.
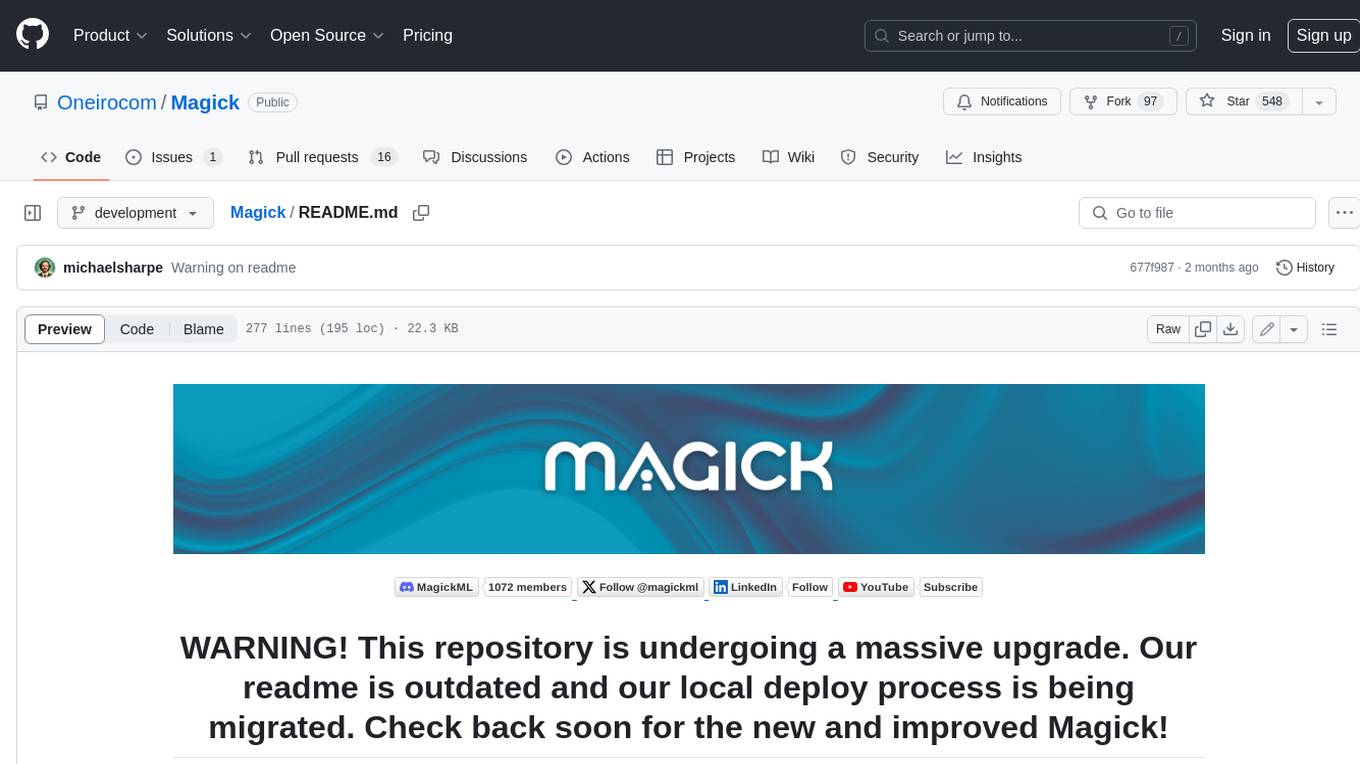
Magick
Magick is a groundbreaking visual AIDE (Artificial Intelligence Development Environment) for no-code data pipelines and multimodal agents. Magick can connect to other services and comes with nodes and templates well-suited for intelligent agents, chatbots, complex reasoning systems and realistic characters.