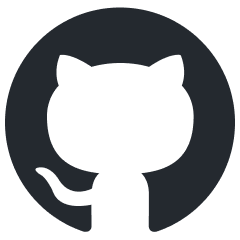
ai-data-science-team
An AI-powered data science team of agents to help you perform common data science tasks 10X faster.
Stars: 1480
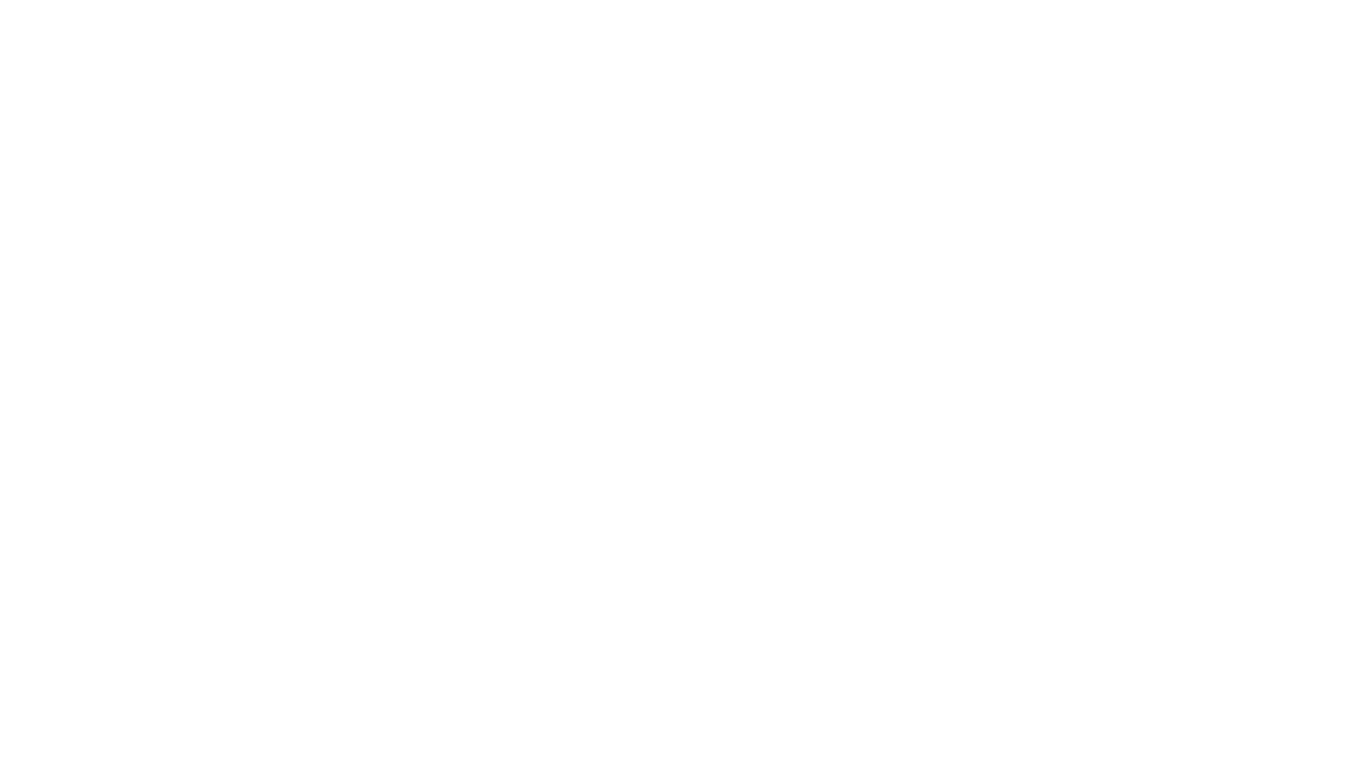
The AI Data Science Team of Copilots is an AI-powered data science team that uses agents to help users perform common data science tasks 10X faster. It includes agents specializing in data cleaning, preparation, feature engineering, modeling, and interpretation of business problems. The project is a work in progress with new data science agents to be released soon. Disclaimer: This project is for educational purposes only and not intended to replace a company's data science team. No warranties or guarantees are provided, and the creator assumes no liability for financial loss.
README:
An AI-powered data science team of agents to help you perform common data science tasks 10X faster.
Please β us on GitHub (it takes 2 seconds and means a lot).
Beta - This Python library is under active development. There may be breaking changes that occur until release of 0.1.0.
The AI Data Science Team of Copilots includes Agents that specialize data cleaning, preparation, feature engineering, modeling (machine learning), and interpretation of various business problems like:
- Churn Modeling
- Employee Attrition
- Lead Scoring
- Insurance Risk
- Credit Card Risk
- And more
- Your AI Data Science Team (πͺ An Army Of Agents)
- Want To Become A Full-Stack Generative AI Data Scientist?
Want to have your own customized enterprise-grade AI Data Science Team and domain-specific AI-powered Apps?
Send inquiries here: https://www.business-science.io/contact.html
If you're an aspiring data scientist who wants to learn how to build AI Agents and AI Apps for your company that performs Data Science, Business Intelligence, Churn Modeling, Time Series Forecasting, and more, then I'd love to help you.
Register for my next Generative AI for Data Scientists workshop here.
This project is a work in progress. New data science agents will be released soon.
π₯ Open Pandas AI Data Analyst: Load an Excel or CSV file and ask it questions. Get data and charts back.
π₯ SQL Database Agent: Connects any SQL Database, generates SQL queries from natural language, and returns data as a downloadable table.
π₯ Exploratory Data Copilot: An AI-powered data science app that performs automated exploratory data analysis (EDA) with EDA Reporting, Missing Data Analysis, Correlation Analysis, and more.
π₯ Pandas Data Analyst Agent: Combines the ability to wrangle, transform, and analyze data with an optional data visualization agent that can create interactive plots.
- NEW Exploratory Data Copilot: An AI-powered data science app that performs automated exploratory data analysis (EDA) with EDA Reporting, Missing Data Analysis, Correlation Analysis, and more. See Application
- SQL Database Agent App: Connects any SQL Database, generates SQL queries from natural language, and returns data as a downloadable table. See Application
- Data Wrangling Agent: Merges, Joins, Preps and Wrangles data into a format that is ready for data analysis. See Example
- Data Visualization Agent: Creates visualizations to help you understand your data. Returns JSON serializable plotly visualizations. See Example
- π₯ Data Cleaning Agent: Performs Data Preparation steps including handling missing values, outliers, and data type conversions. See Example
- Feature Engineering Agent: Converts the prepared data into ML-ready data. Adds features to increase predictive accuracy of ML models. See Example
- π₯ SQL Database Agent: Connects to SQL databases to pull data into the data science environment. Creates pipelines to automate data extraction. Performs Joins, Aggregations, and other SQL Query operations. See Example
- π₯ Data Loader Tools Agent: Loads data from various sources including CSV, Excel, Parquet, and Pickle files. See Example
- π₯ H2O Machine Learning Agent: Builds and logs 100's of high-performance machine learning models. See Example
- π₯ MLflow Tools Agent (MLOps): This agent has 11+ tools for managing models, ML projects, and making production ML predictions with MLflow. See Example
- π₯π₯ EDA Tools Agent: Performs automated exploratory data analysis (EDA) with EDA Reporting, Missing Data Analysis, Correlation Analysis, and more. See Example
- π₯π₯ Pandas Data Analyst Agent: Combines the ability to wrangle, transform, and analyze data with an optional data visualization agent that can create interactive plots. See Example
- π₯π₯ SQL Data Analyst Agent: Connects to SQL databases to pull data into the data science environment. Creates pipelines to automate data extraction. Performs Joins, Aggregations, and other SQL Query operations. Includes a Data Visualization Agent that creates visualizations to help you understand your data. See Example
- Data Analyst: Analyzes data structure, creates exploratory visualizations, and performs correlation analysis to identify relationships.
- Interpretability Agent: Performs Interpretable ML to explain why the model returned predictions including which features were the most important to the model.
- Supervisor: Forms task list. Moderates sub-agents. Returns completed assignment.
This project is for educational purposes only.
- It is not intended to replace your company's data science team
- No warranties or guarantees provided
- Creator assumes no liability for financial loss
- Consult an experienced Generative AI Data Scientist for building your own custom AI Data Science Team
- If you want a custom enterprise-grade AI Data Science Team, send inquiries here.
By using this software, you agree to use it solely for learning purposes.
pip install git+https://github.com/business-science/ai-data-science-team.git --upgrade
feature_engineering_agent = FeatureEngineeringAgent(model = llm)
feature_engineering_agent.invoke_agent(
data_raw = df,
user_instructions = "Make sure to scale and center numeric features",
target_variable = "Churn",
max_retries = 3,
)
---FEATURE ENGINEERING AGENT----
* CREATE FEATURE ENGINEER CODE
* EXECUTING AGENT CODE
* EXPLAIN AGENT CODE
feature_engineering_agent.get_data_engineered()
data_cleaning_agent = DataCleaningAgent(model = llm)
response = data_cleaning_agent.invoke_agent(
data_raw = df,
user_instructions = "Don't remove outliers when cleaning the data.",
max_retries = 3,
)
---DATA CLEANING AGENT----
* CREATE DATA CLEANER CODE
* EXECUTING AGENT CODE
* EXPLAIN AGENT CODE
data_cleaning_agent.get_data_cleaned()
- Fork the repository
- Create a feature branch
- Commit your changes
- Push to the branch
- Create a Pull Request
This project is licensed under the MIT License. See LICENSE file for details.
I teach Generative AI Data Science to help you build AI-powered data science apps. Register for my next Generative AI for Data Scientists workshop here.
For Tasks:
Click tags to check more tools for each tasksFor Jobs:
Alternative AI tools for ai-data-science-team
Similar Open Source Tools
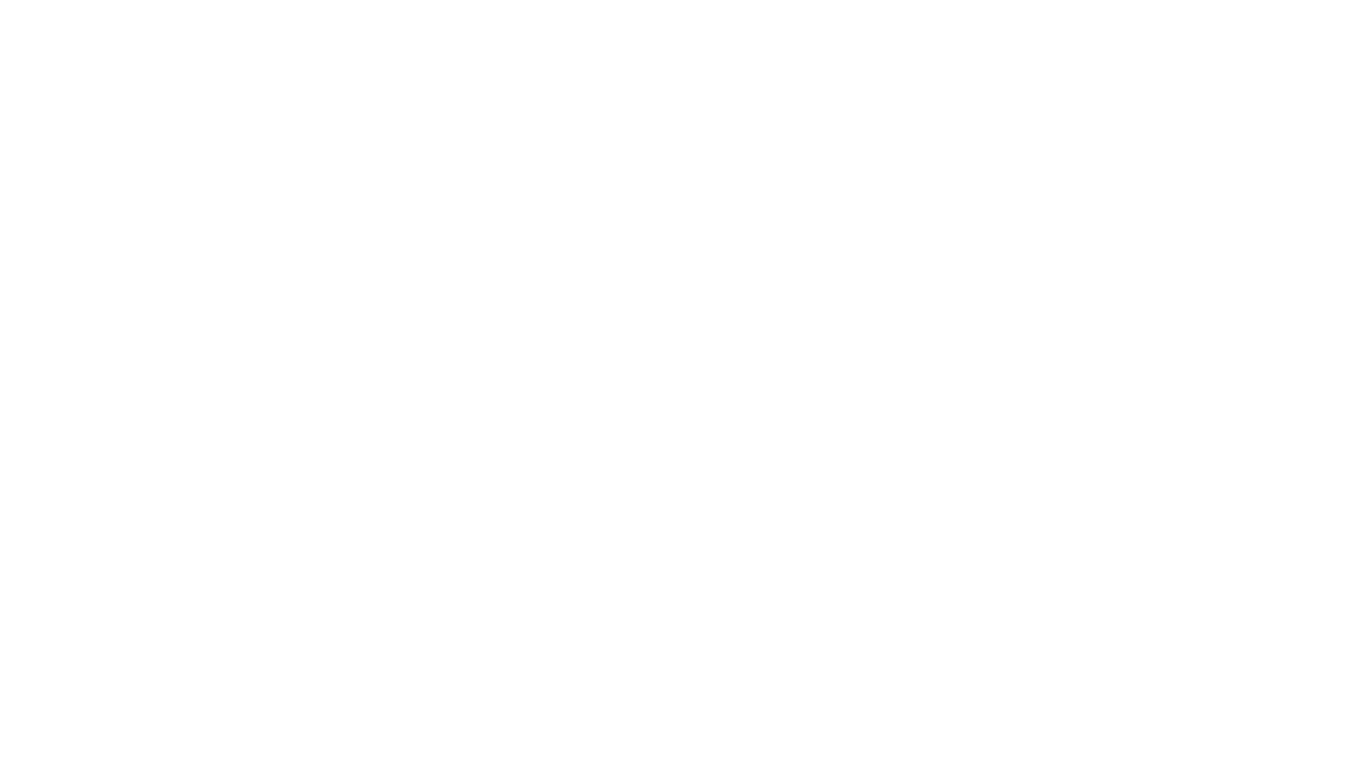
ai-data-science-team
The AI Data Science Team of Copilots is an AI-powered data science team that uses agents to help users perform common data science tasks 10X faster. It includes agents specializing in data cleaning, preparation, feature engineering, modeling, and interpretation of business problems. The project is a work in progress with new data science agents to be released soon. Disclaimer: This project is for educational purposes only and not intended to replace a company's data science team. No warranties or guarantees are provided, and the creator assumes no liability for financial loss.
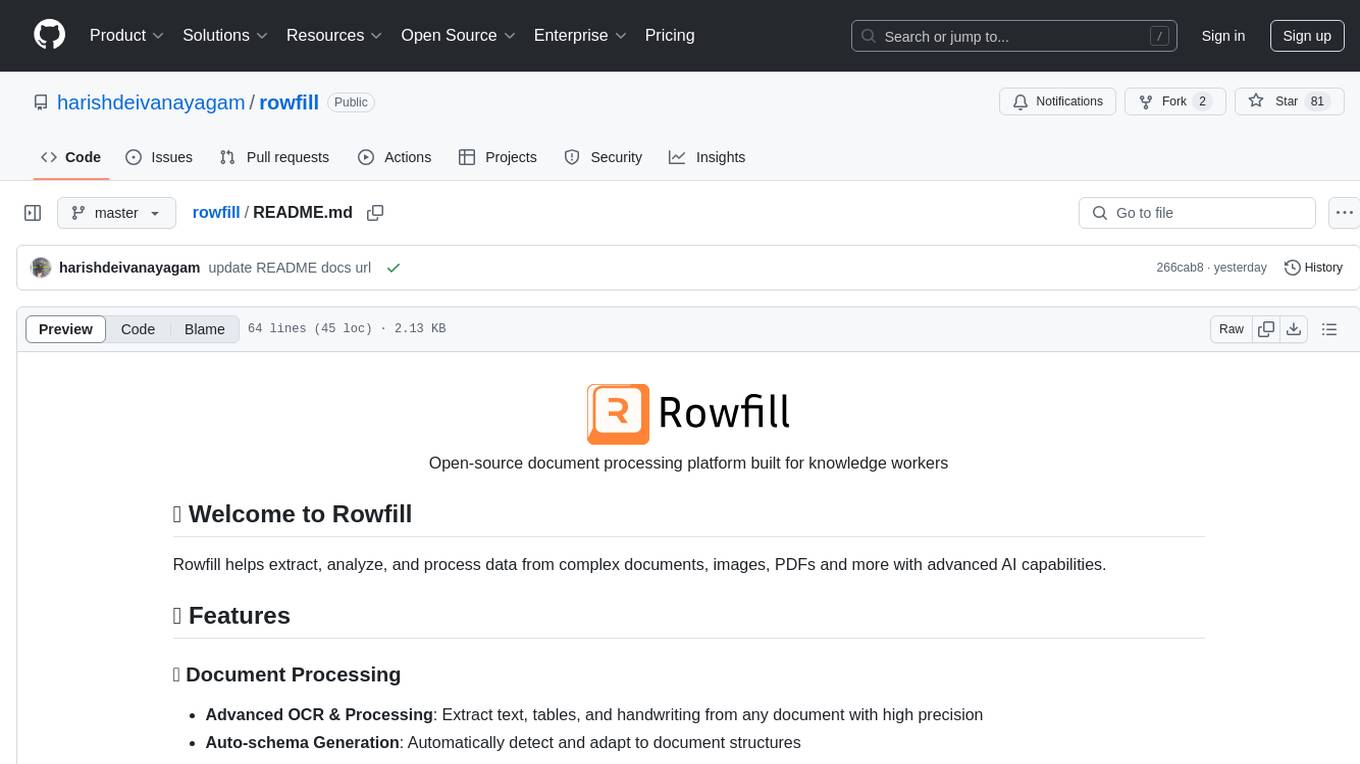
rowfill
Rowfill is an open-source document processing platform designed for knowledge workers. It offers advanced AI capabilities to extract, analyze, and process data from complex documents, images, and PDFs. The platform features advanced OCR and processing functionalities, auto-schema generation, and custom actions for creating tailored workflows. It prioritizes privacy and security by supporting Local LLMs like Llama and Mistral, syncing with company data while maintaining privacy, and being open source with AGPLv3 licensing. Rowfill is a versatile tool that aims to streamline document processing tasks for users in various industries.
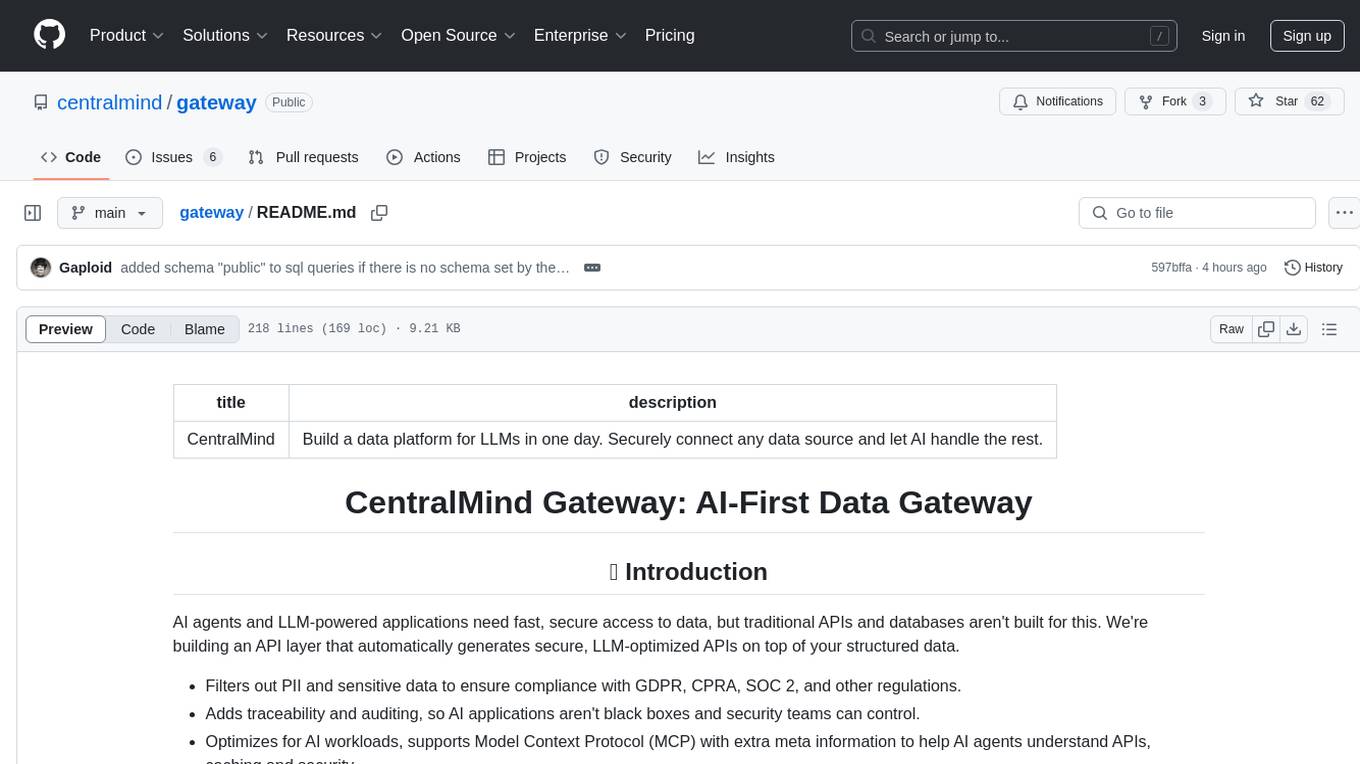
gateway
CentralMind Gateway is an AI-first data gateway that securely connects any data source and automatically generates secure, LLM-optimized APIs. It filters out sensitive data, adds traceability, and optimizes for AI workloads. Suitable for companies deploying AI agents for customer support and analytics.
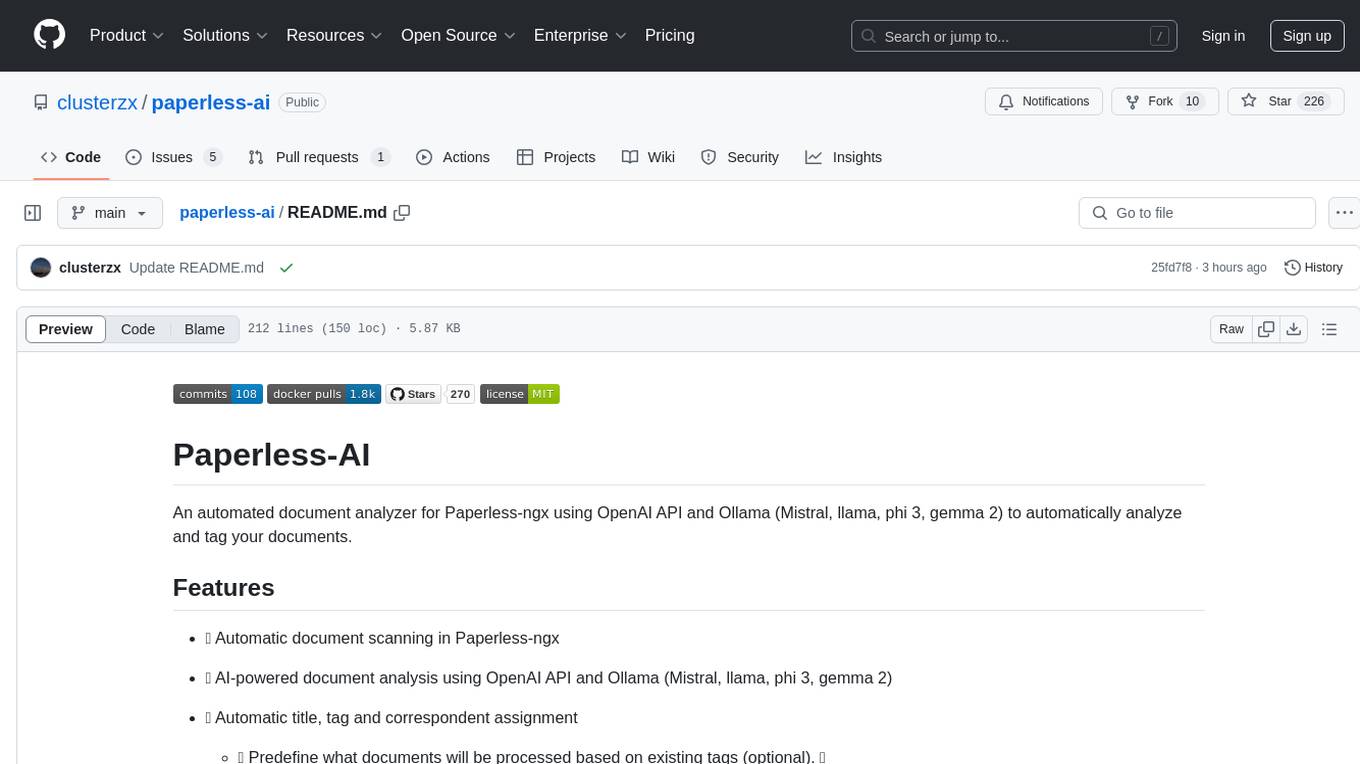
paperless-ai
Paperless-AI is an automated document analyzer tool designed for Paperless-ngx users. It utilizes the OpenAI API and Ollama (Mistral, llama, phi 3, gemma 2) to automatically scan, analyze, and tag documents. The tool offers features such as automatic document scanning, AI-powered document analysis, automatic title and tag assignment, manual mode for analyzing documents, easy setup through a web interface, document processing dashboard, error handling, and Docker support. Users can configure the tool through a web interface and access a debug interface for monitoring and troubleshooting. Paperless-AI aims to streamline document organization and analysis processes for users with access to Paperless-ngx and AI capabilities.
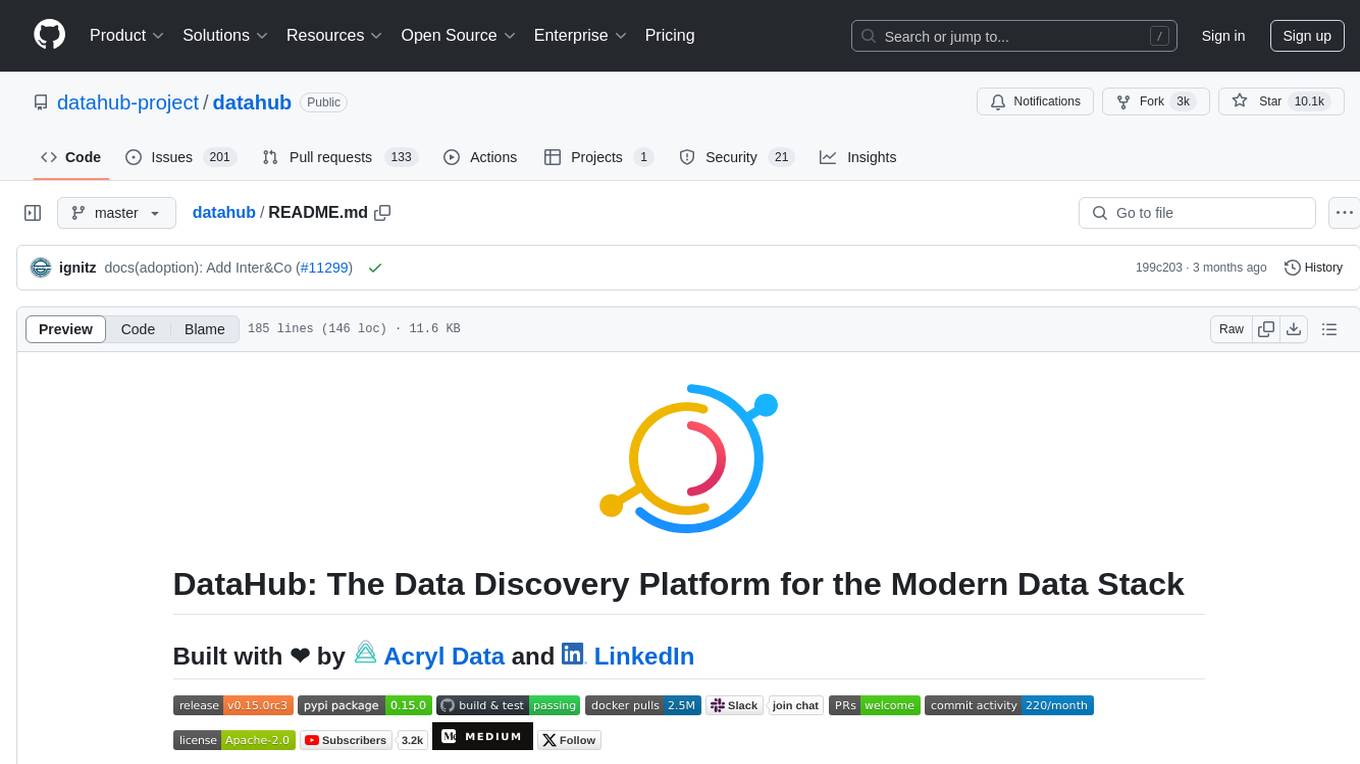
datahub
DataHub is an open-source data catalog designed for the modern data stack. It provides a platform for managing metadata, enabling users to discover, understand, and collaborate on data assets within their organization. DataHub offers features such as data lineage tracking, data quality monitoring, and integration with various data sources. It is built with contributions from Acryl Data and LinkedIn, aiming to streamline data management processes and enhance data discoverability across different teams and departments.
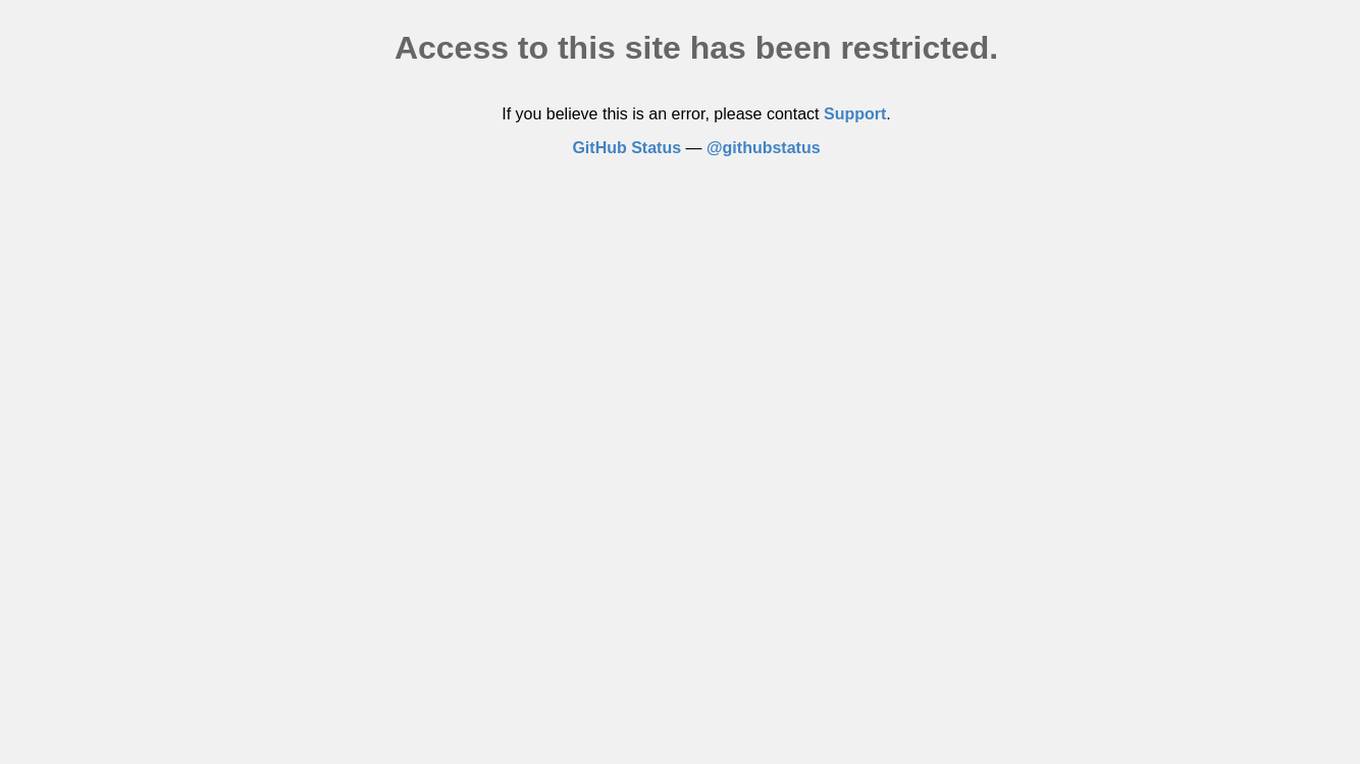
Open-Medical-Reasoning-Tasks
Open Life Science AI: Medical Reasoning Tasks is a collaborative hub for developing cutting-edge reasoning tasks for Large Language Models (LLMs) in the medical, healthcare, and clinical domains. The repository aims to advance AI capabilities in healthcare by fostering accurate diagnoses, personalized treatments, and improved patient outcomes. It offers a diverse range of medical reasoning challenges such as Diagnostic Reasoning, Treatment Planning, Medical Image Analysis, Clinical Data Interpretation, Patient History Analysis, Ethical Decision Making, Medical Literature Comprehension, and Drug Interaction Assessment. Contributors can join the community of healthcare professionals, AI researchers, and enthusiasts to contribute to the repository by creating new tasks or improvements following the provided guidelines. The repository also provides resources including a task list, evaluation metrics, medical AI papers, and healthcare datasets for training and evaluation.
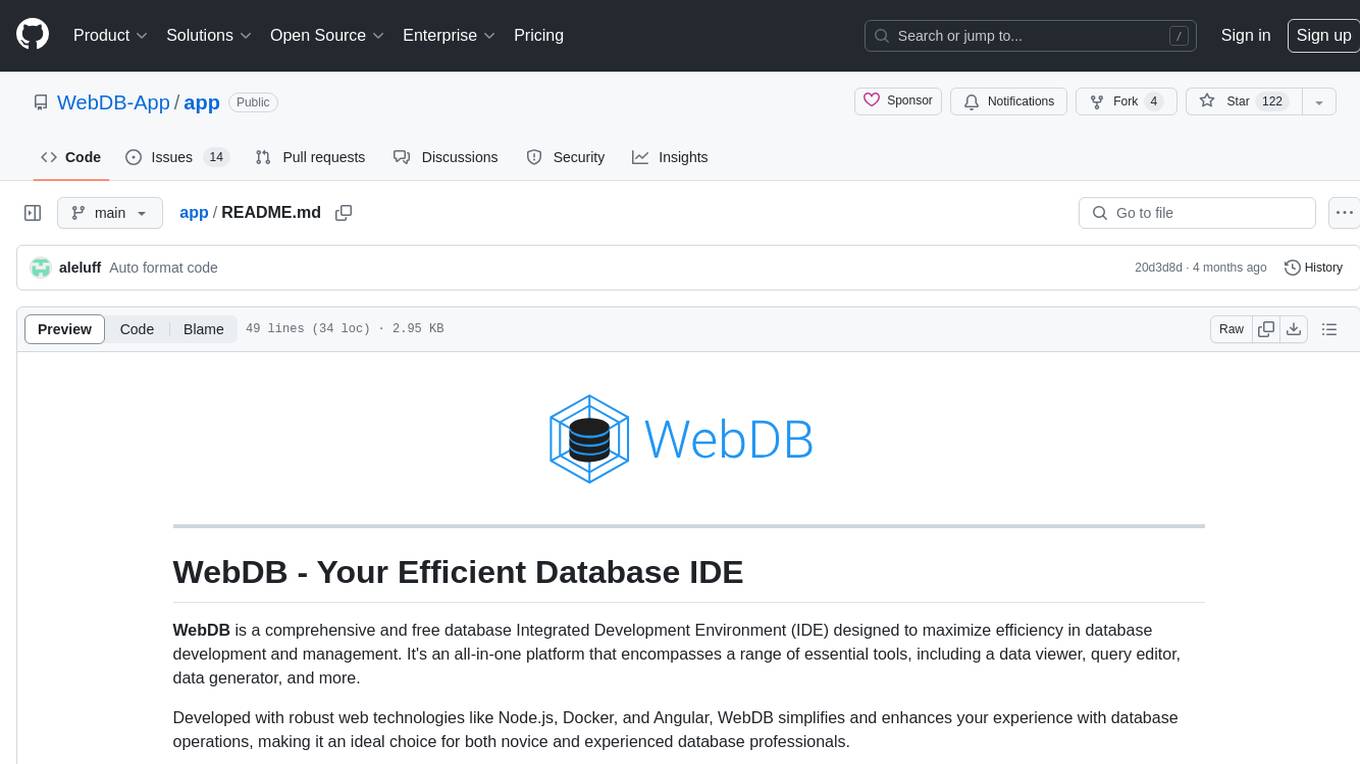
app
WebDB is a comprehensive and free database Integrated Development Environment (IDE) designed to maximize efficiency in database development and management. It simplifies and enhances database operations with features like DBMS discovery, query editor, time machine, NoSQL structure inferring, modern ERD visualization, and intelligent data generator. Developed with robust web technologies, WebDB is suitable for both novice and experienced database professionals.
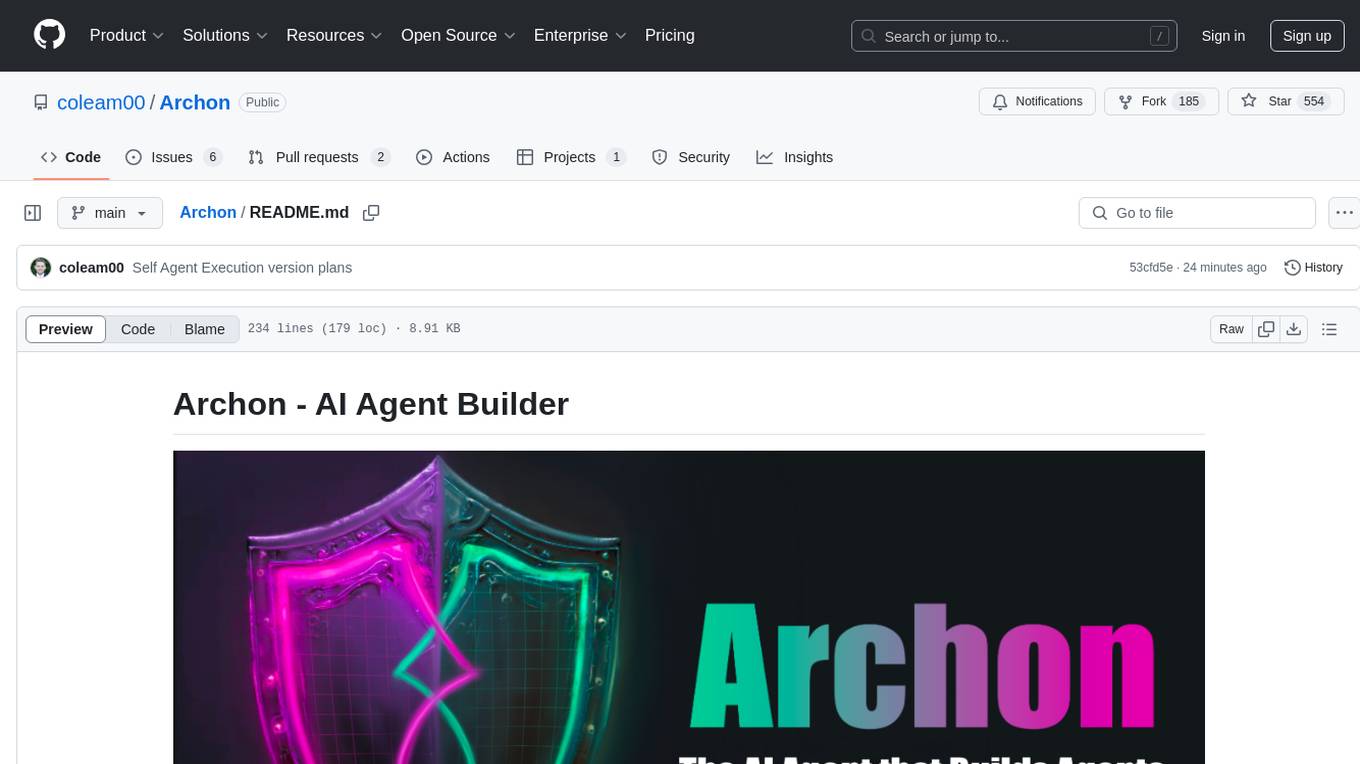
Archon
Archon is an AI meta-agent designed to autonomously build, refine, and optimize other AI agents. It serves as a practical tool for developers and an educational framework showcasing the evolution of agentic systems. Through iterative development, Archon demonstrates the power of planning, feedback loops, and domain-specific knowledge in creating robust AI agents.
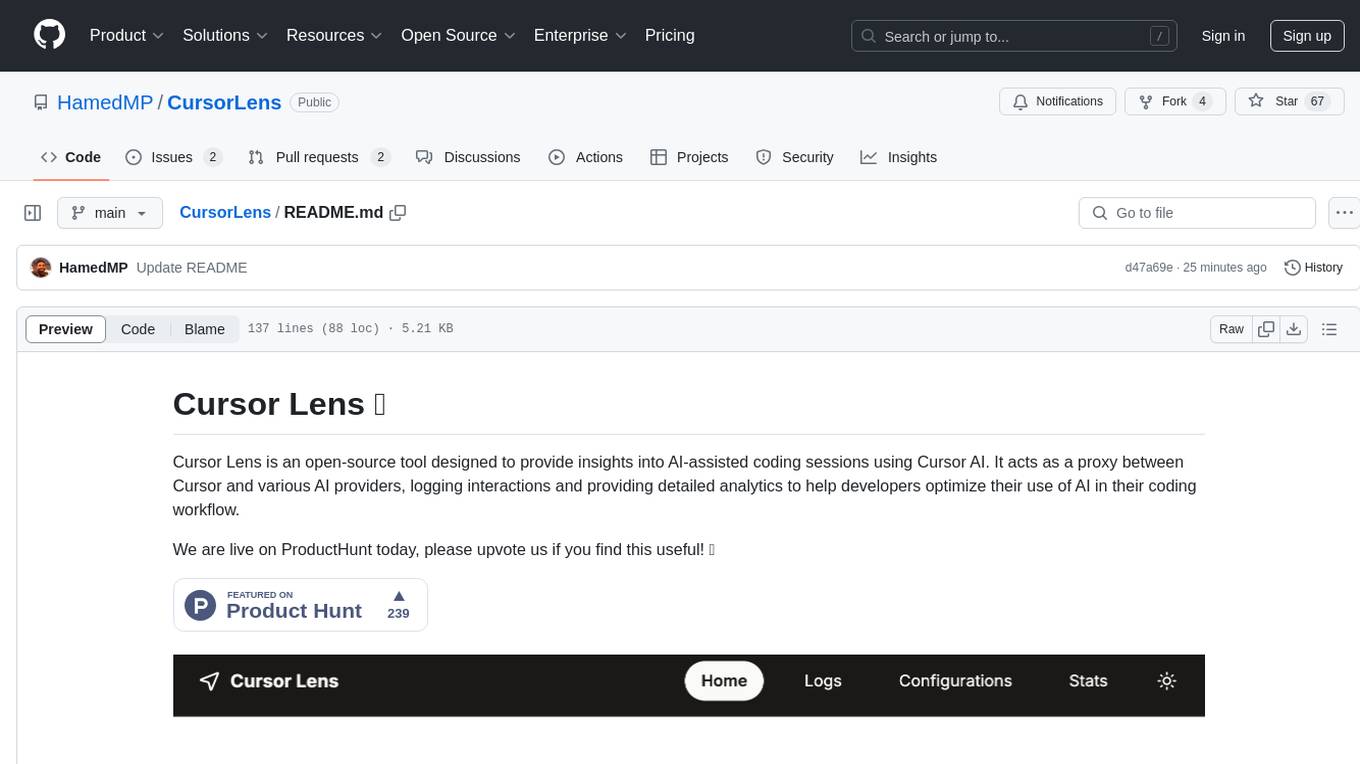
CursorLens
Cursor Lens is an open-source tool that acts as a proxy between Cursor and various AI providers, logging interactions and providing detailed analytics to help developers optimize their use of AI in their coding workflow. It supports multiple AI providers, captures and logs all requests, provides visual analytics on AI usage, allows users to set up and switch between different AI configurations, offers real-time monitoring of AI interactions, tracks token usage, estimates costs based on token usage and model pricing. Built with Next.js, React, PostgreSQL, Prisma ORM, Vercel AI SDK, Tailwind CSS, and shadcn/ui components.
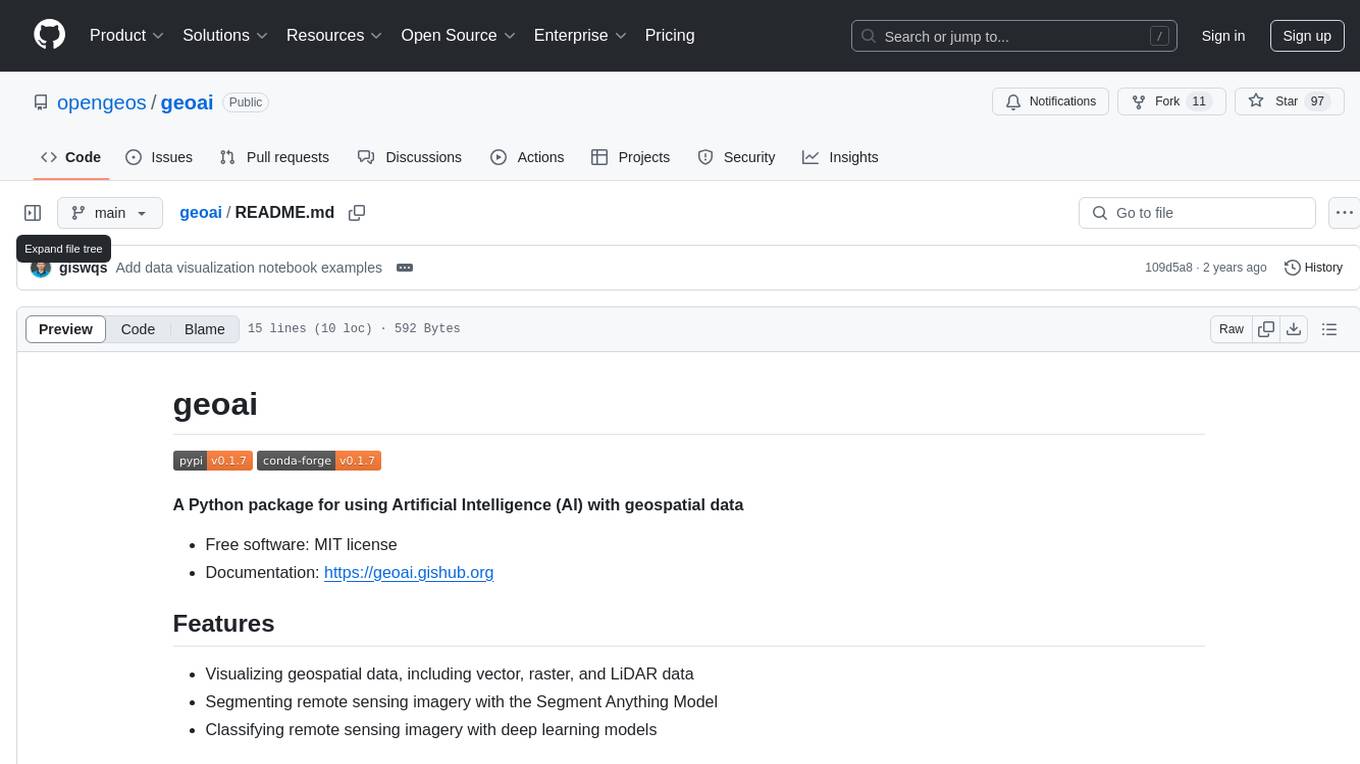
geoai
geoai is a Python package designed for utilizing Artificial Intelligence (AI) in the context of geospatial data. It allows users to visualize various types of geospatial data such as vector, raster, and LiDAR data. Additionally, the package offers functionalities for segmenting remote sensing imagery using the Segment Anything Model and classifying remote sensing imagery with deep learning models. With a focus on geospatial AI applications, geoai provides a versatile tool for processing and analyzing spatial data with the power of AI.
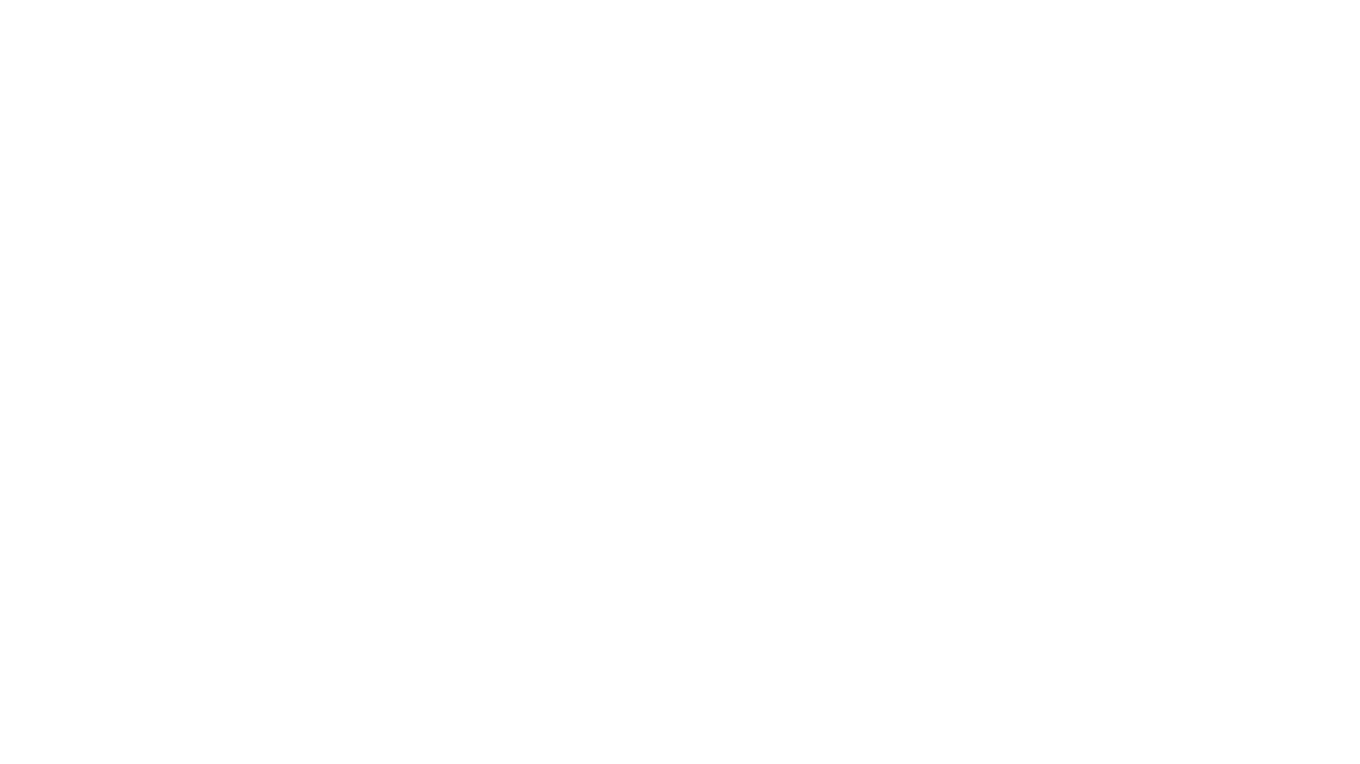
DevDocs
DevDocs is a platform designed to simplify the process of digesting technical documentation for software engineers and developers. It automates the extraction and conversion of web content into markdown format, making it easier for users to access and understand the information. By crawling through child pages of a given URL, DevDocs provides a streamlined approach to gathering relevant data and integrating it into various tools for software development. The tool aims to save time and effort by eliminating the need for manual research and content extraction, ultimately enhancing productivity and efficiency in the development process.
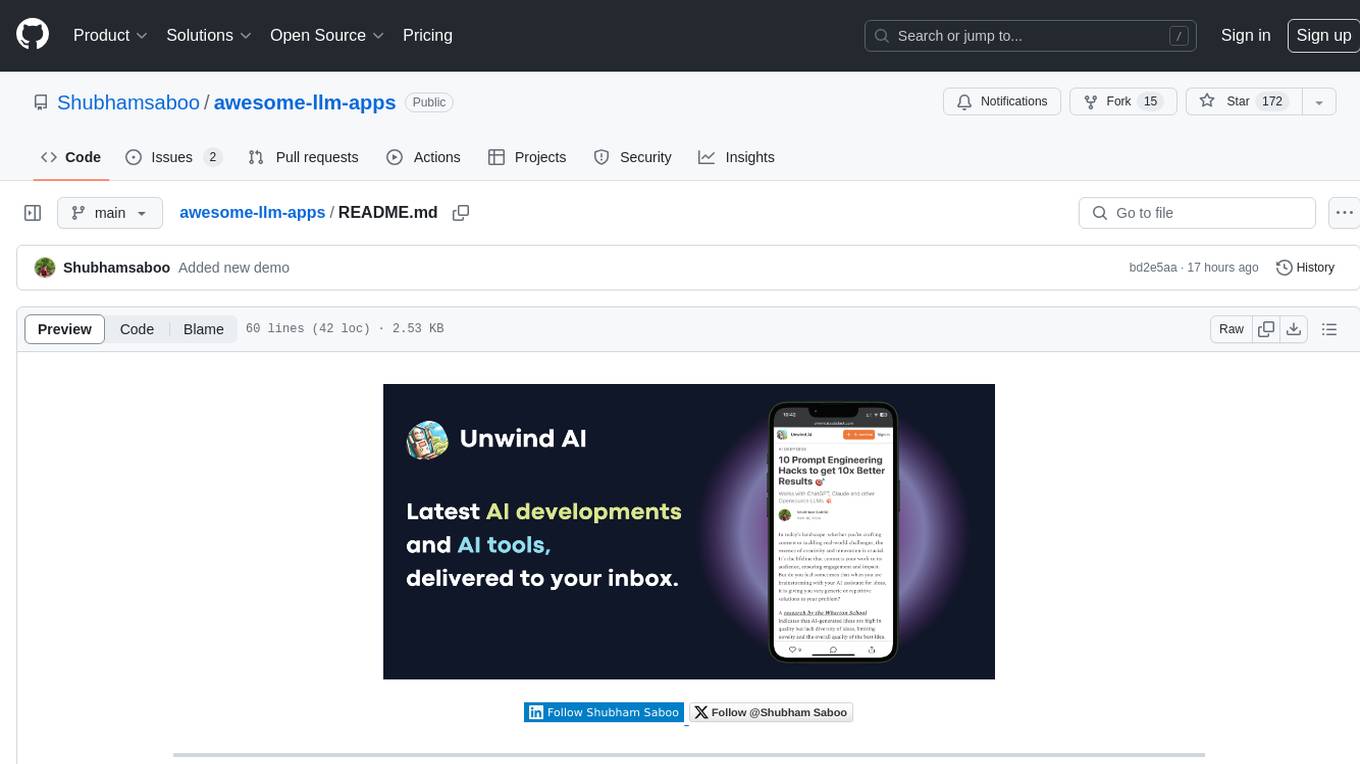
awesome-llm-apps
Awesome LLM Apps is a curated collection of applications that leverage RAG with OpenAI, Anthropic, Gemini, and open-source models. The repository contains projects such as Local Llama-3 with RAG for chatting with webpages locally, Chat with Gmail for interacting with Gmail using natural language, Chat with Substack Newsletter for conversing with Substack newsletters using GPT-4, Chat with PDF for intelligent conversation based on PDF documents, and Chat with YouTube Videos for engaging with YouTube video content through natural language. Users can clone the repository, navigate to specific project directories, install dependencies, and follow project-specific instructions to set up and run the apps. Contributions are encouraged, and new app ideas or improvements can be submitted via pull requests.
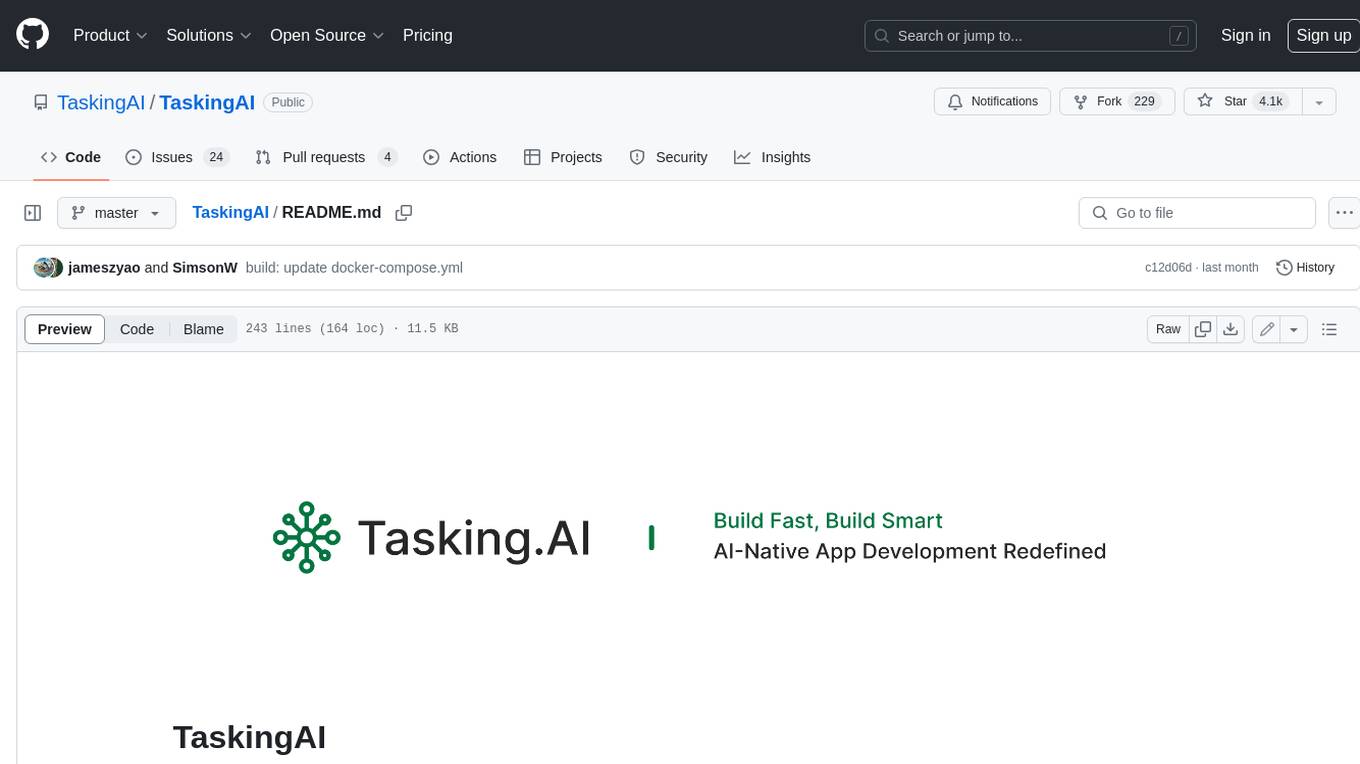
TaskingAI
TaskingAI brings Firebase's simplicity to **AI-native app development**. The platform enables the creation of GPTs-like multi-tenant applications using a wide range of LLMs from various providers. It features distinct, modular functions such as Inference, Retrieval, Assistant, and Tool, seamlessly integrated to enhance the development process. TaskingAIβs cohesive design ensures an efficient, intelligent, and user-friendly experience in AI application development.
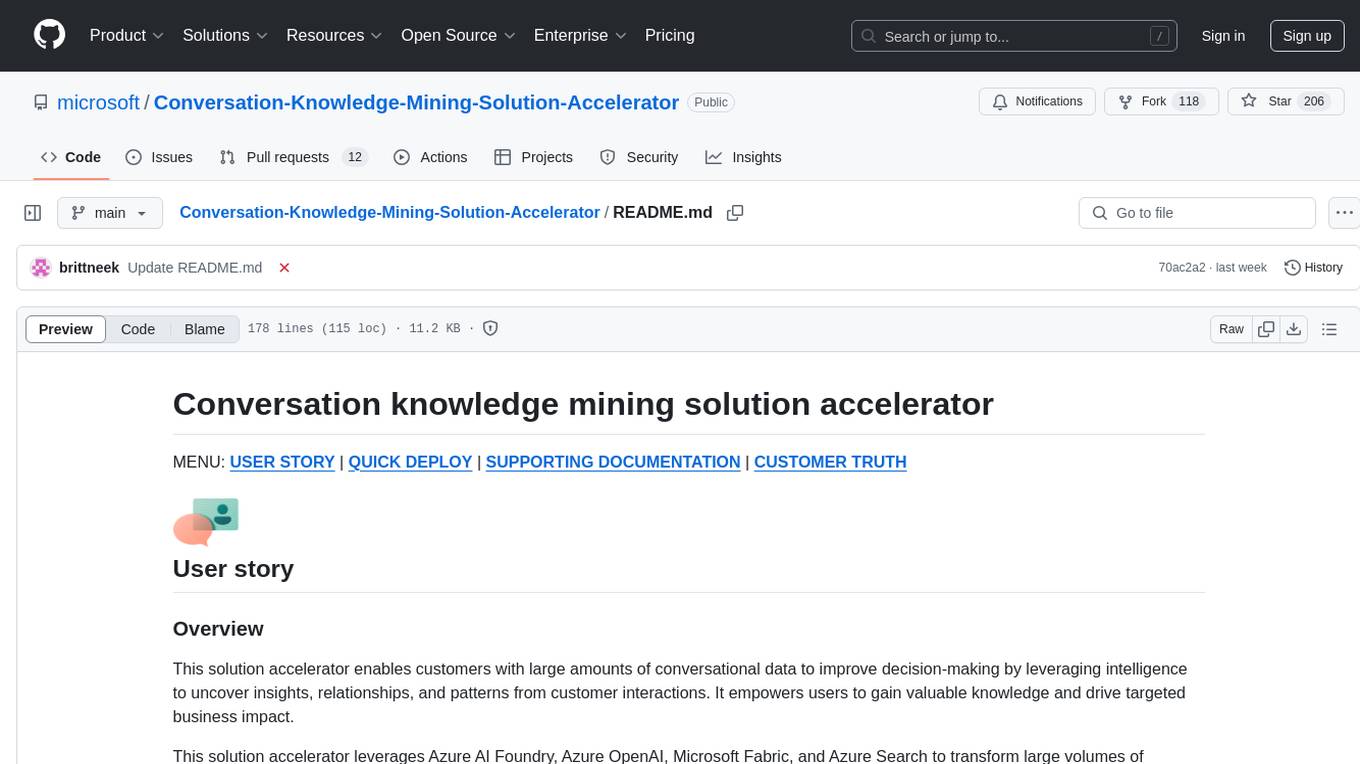
Conversation-Knowledge-Mining-Solution-Accelerator
The Conversation Knowledge Mining Solution Accelerator enables customers to leverage intelligence to uncover insights, relationships, and patterns from conversational data. It empowers users to gain valuable knowledge and drive targeted business impact by utilizing Azure AI Foundry, Azure OpenAI, Microsoft Fabric, and Azure Search for topic modeling, key phrase extraction, speech-to-text transcription, and interactive chat experiences.
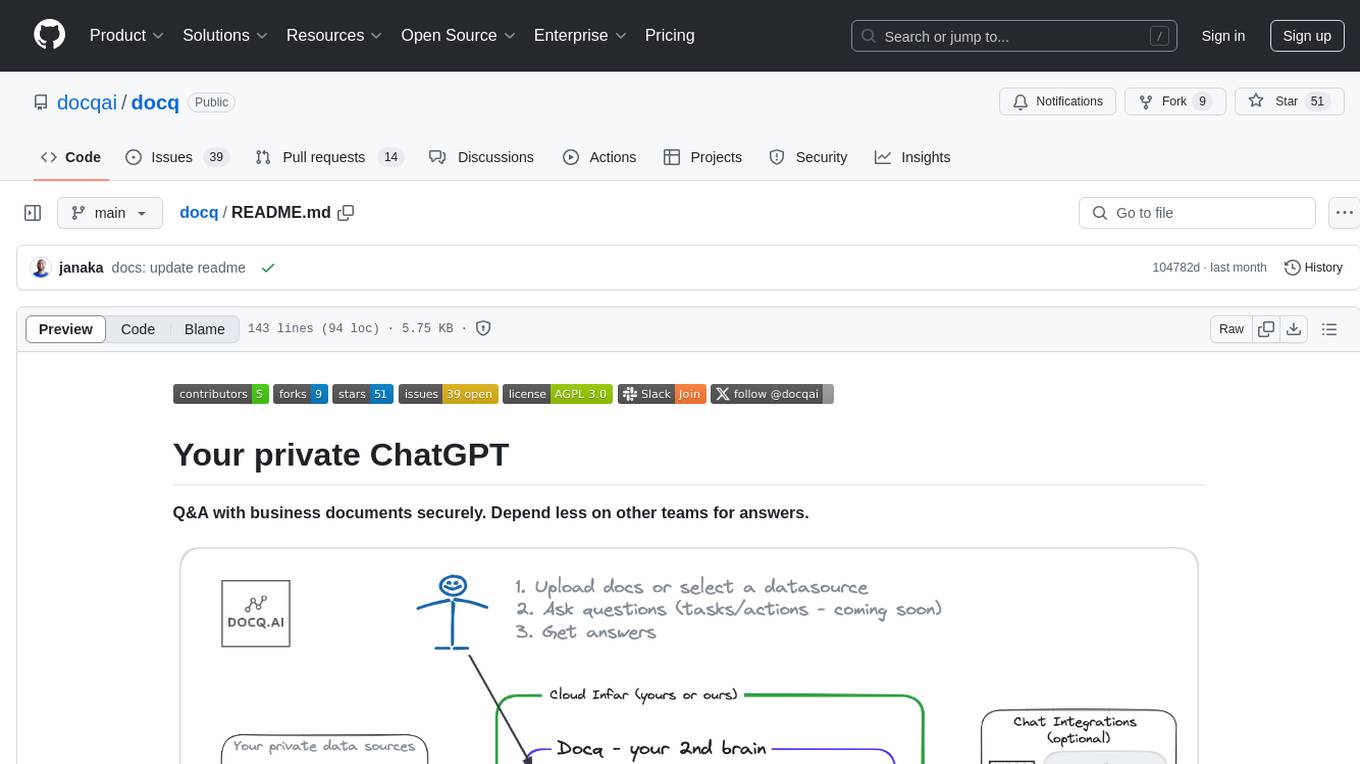
docq
Docq is a private and secure GenAI tool designed to extract knowledge from business documents, enabling users to find answers independently. It allows data to stay within organizational boundaries, supports self-hosting with various cloud vendors, and offers multi-model and multi-modal capabilities. Docq is extensible, open-source (AGPLv3), and provides commercial licensing options. The tool aims to be a turnkey solution for organizations to adopt AI innovation safely, with plans for future features like more data ingestion options and model fine-tuning.
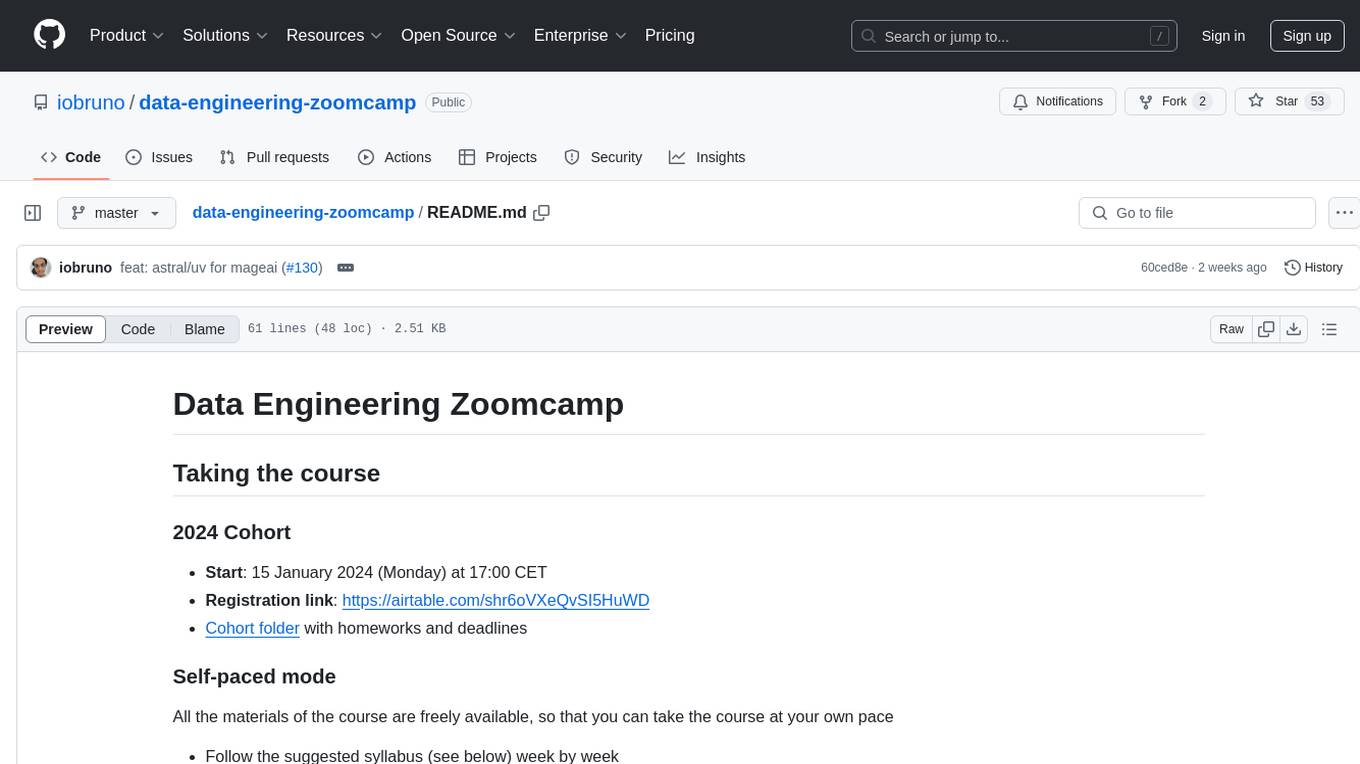
data-engineering-zoomcamp
Data Engineering Zoomcamp is a comprehensive course covering various aspects of data engineering, including data ingestion, workflow orchestration, data warehouse, analytics engineering, batch processing, and stream processing. The course provides hands-on experience with tools like Python, Rust, Terraform, Airflow, BigQuery, dbt, PySpark, Kafka, and more. Students will learn how to work with different data technologies to build scalable and efficient data pipelines for analytics and processing. The course is designed for individuals looking to enhance their data engineering skills and gain practical experience in working with big data technologies.
For similar tasks
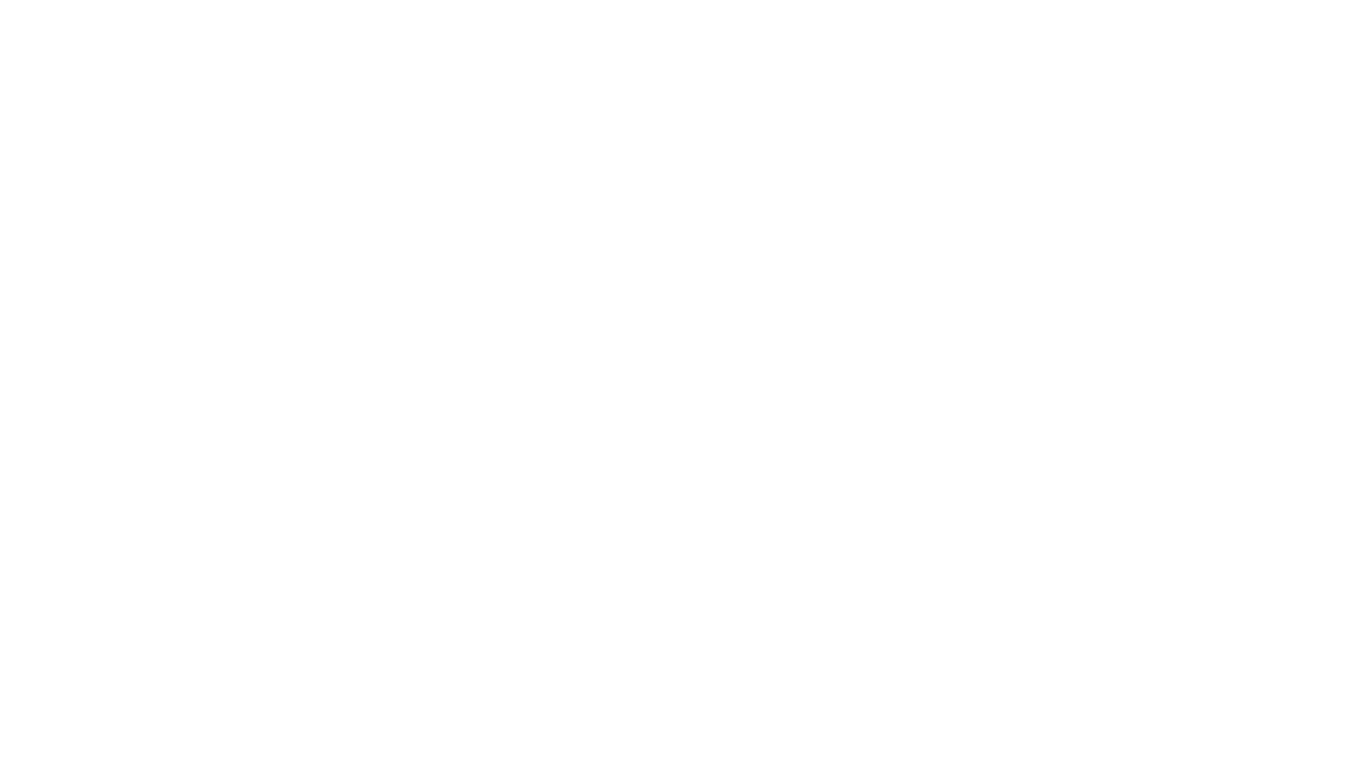
ai-data-science-team
The AI Data Science Team of Copilots is an AI-powered data science team that uses agents to help users perform common data science tasks 10X faster. It includes agents specializing in data cleaning, preparation, feature engineering, modeling, and interpretation of business problems. The project is a work in progress with new data science agents to be released soon. Disclaimer: This project is for educational purposes only and not intended to replace a company's data science team. No warranties or guarantees are provided, and the creator assumes no liability for financial loss.
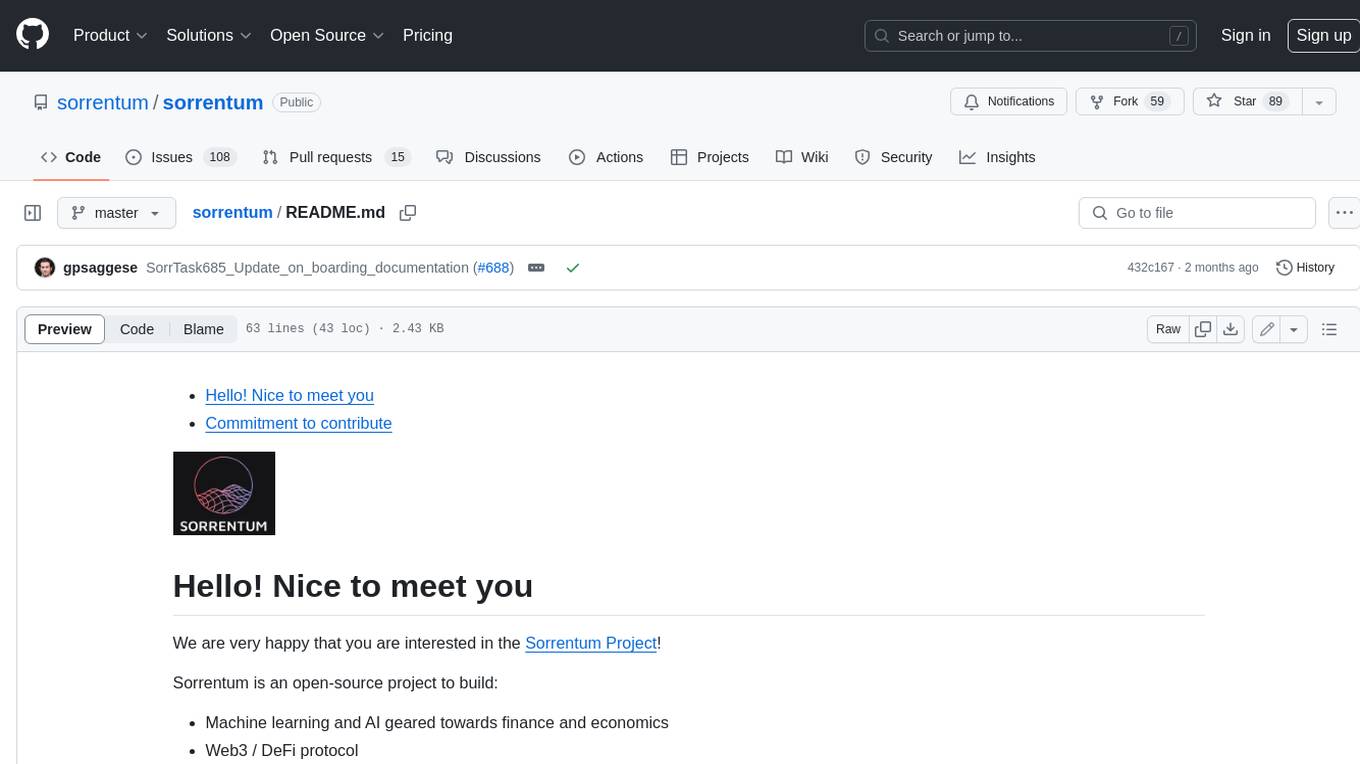
sorrentum
Sorrentum is an open-source project that aims to combine open-source development, startups, and brilliant students to build machine learning, AI, and Web3 / DeFi protocols geared towards finance and economics. The project provides opportunities for internships, research assistantships, and development grants, as well as the chance to work on cutting-edge problems, learn about startups, write academic papers, and get internships and full-time positions at companies working on Sorrentum applications.
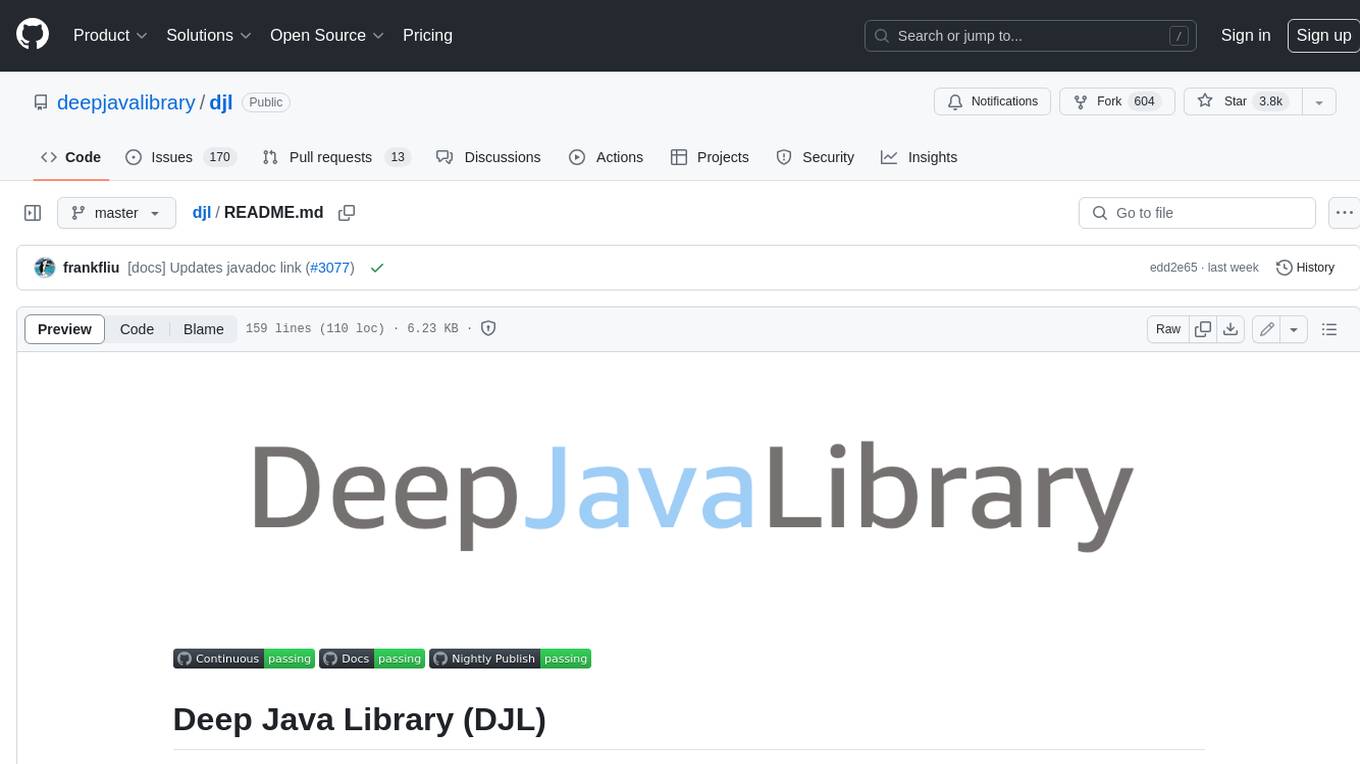
djl
Deep Java Library (DJL) is an open-source, high-level, engine-agnostic Java framework for deep learning. It is designed to be easy to get started with and simple to use for Java developers. DJL provides a native Java development experience and allows users to integrate machine learning and deep learning models with their Java applications. The framework is deep learning engine agnostic, enabling users to switch engines at any point for optimal performance. DJL's ergonomic API interface guides users with best practices to accomplish deep learning tasks, such as running inference and training neural networks.
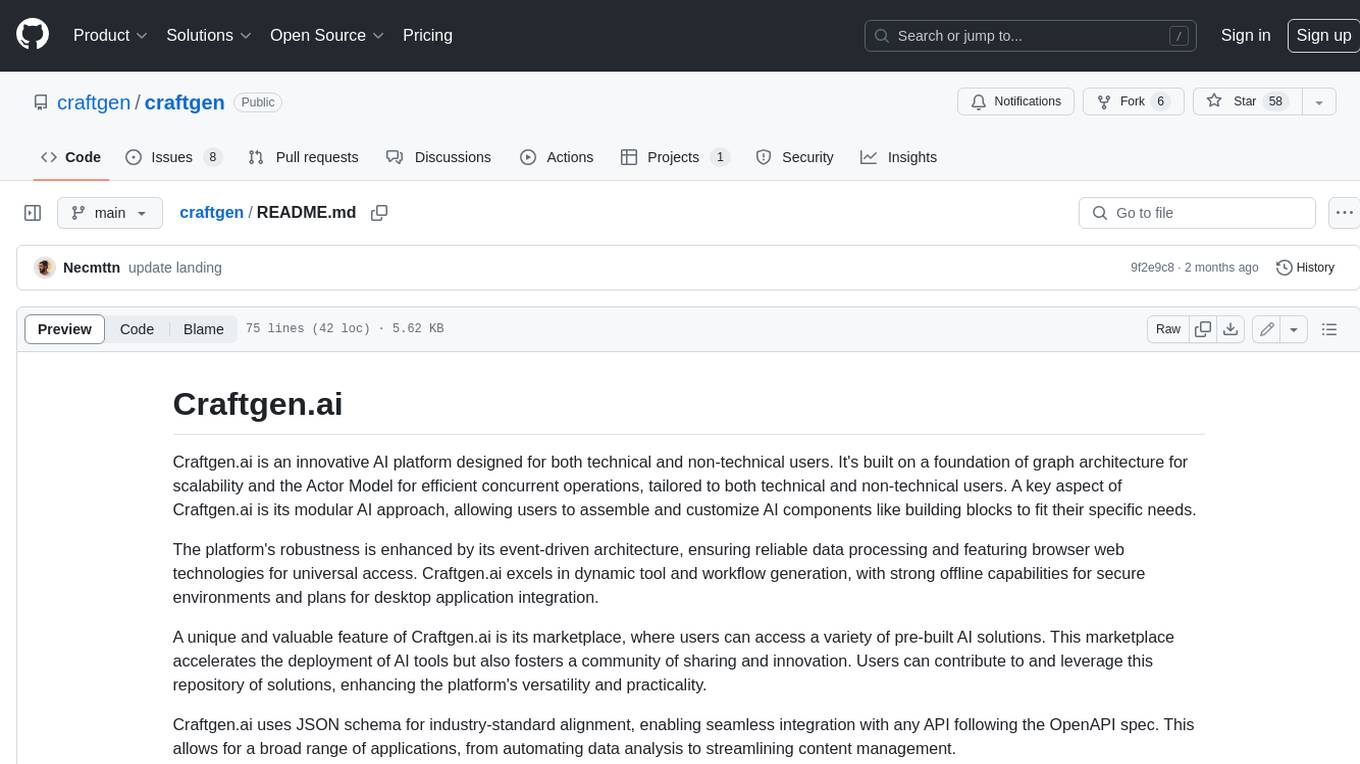
craftgen
Craftgen.ai is an innovative AI platform designed for both technical and non-technical users. It's built on a foundation of graph architecture for scalability and the Actor Model for efficient concurrent operations, tailored to both technical and non-technical users. A key aspect of Craftgen.ai is its modular AI approach, allowing users to assemble and customize AI components like building blocks to fit their specific needs. The platform's robustness is enhanced by its event-driven architecture, ensuring reliable data processing and featuring browser web technologies for universal access. Craftgen.ai excels in dynamic tool and workflow generation, with strong offline capabilities for secure environments and plans for desktop application integration. A unique and valuable feature of Craftgen.ai is its marketplace, where users can access a variety of pre-built AI solutions. This marketplace accelerates the deployment of AI tools but also fosters a community of sharing and innovation. Users can contribute to and leverage this repository of solutions, enhancing the platform's versatility and practicality. Craftgen.ai uses JSON schema for industry-standard alignment, enabling seamless integration with any API following the OpenAPI spec. This allows for a broad range of applications, from automating data analysis to streamlining content management. The platform is designed to bridge the gap between advanced AI technology and practical usability. It's a flexible, secure, and intuitive platform that empowers users, from developers seeking to create custom AI solutions to businesses looking to automate routine tasks. Craftgen.ai's goal is to make AI technology an integral, seamless part of everyday problem-solving and innovation, providing a platform where modular AI and a thriving marketplace converge to meet the diverse needs of its users.
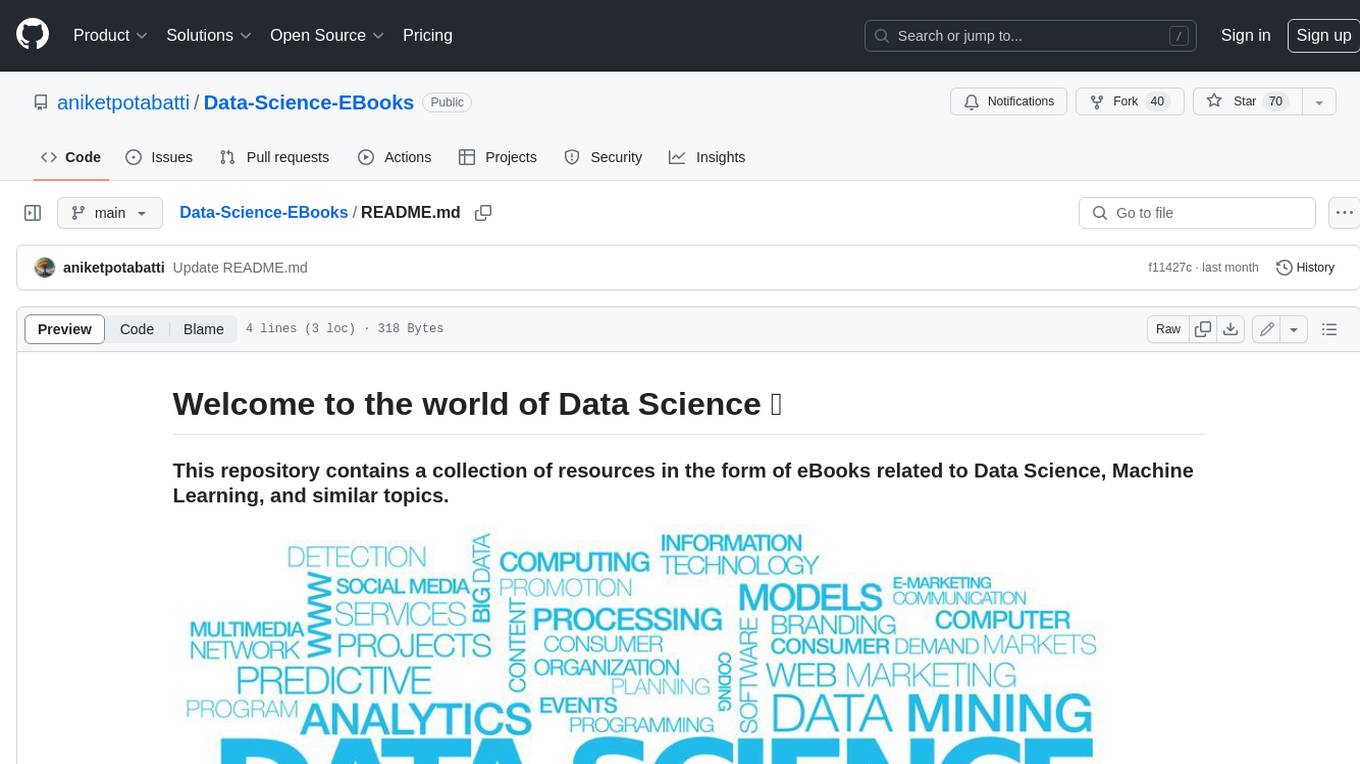
Data-Science-EBooks
This repository contains a collection of resources in the form of eBooks related to Data Science, Machine Learning, and similar topics.
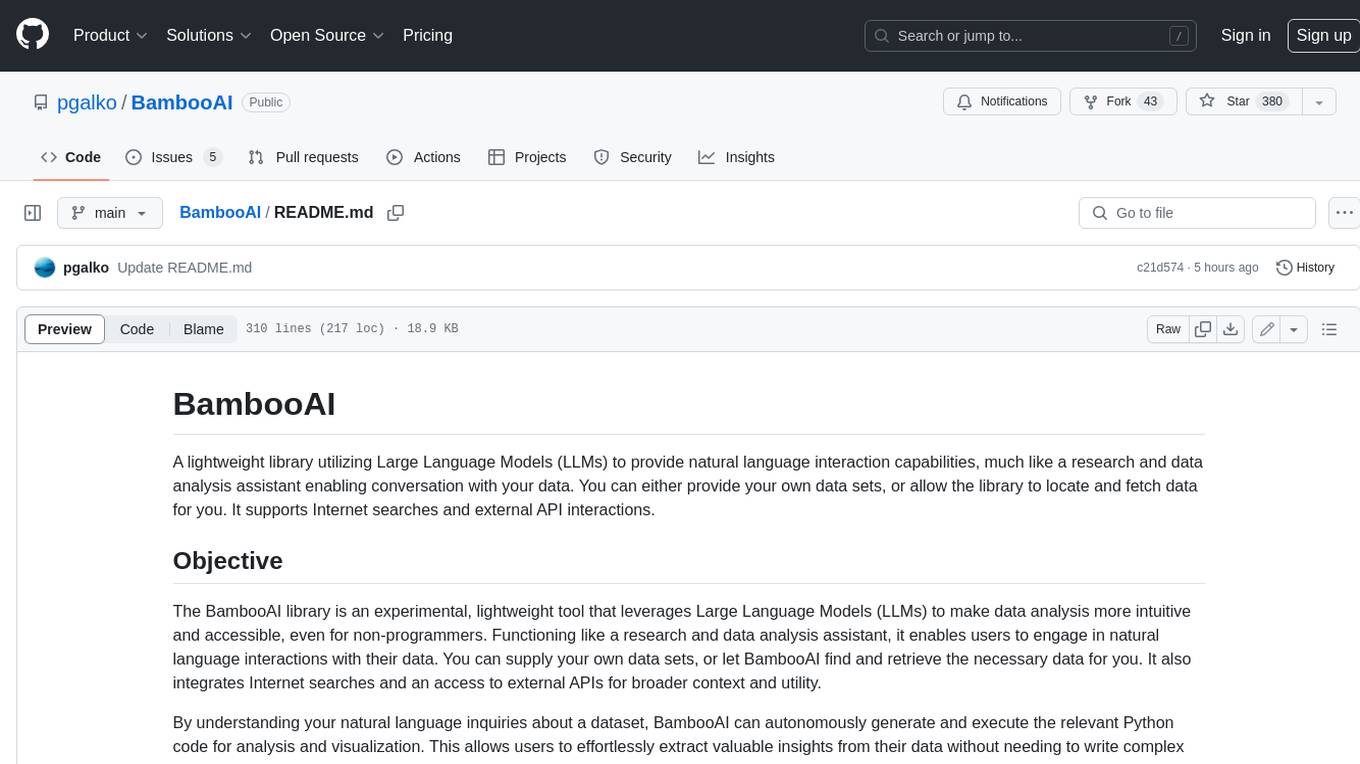
BambooAI
BambooAI is a lightweight library utilizing Large Language Models (LLMs) to provide natural language interaction capabilities, much like a research and data analysis assistant enabling conversation with your data. You can either provide your own data sets, or allow the library to locate and fetch data for you. It supports Internet searches and external API interactions.
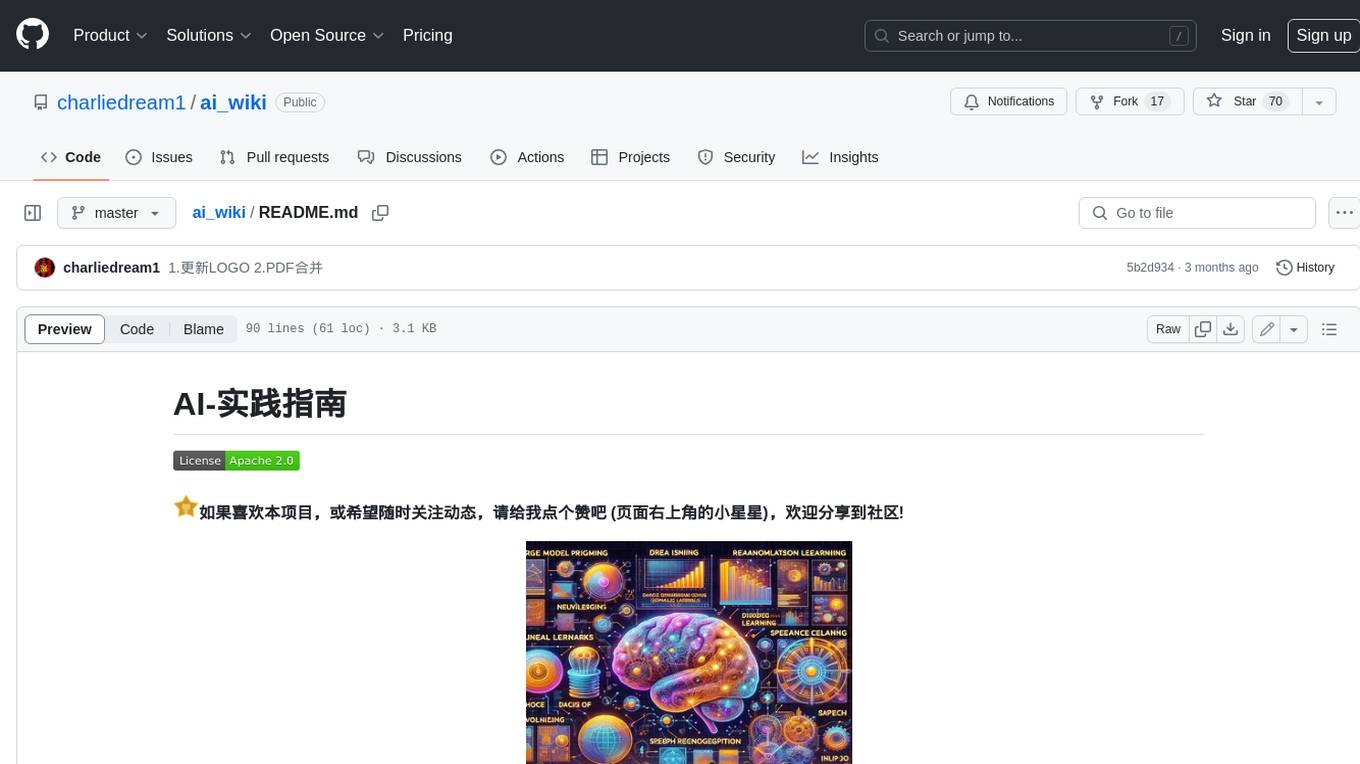
ai_wiki
This repository provides a comprehensive collection of resources, open-source tools, and knowledge related to quantitative analysis. It serves as a valuable knowledge base and navigation guide for individuals interested in various aspects of quantitative investing, including platforms, programming languages, mathematical foundations, machine learning, deep learning, and practical applications. The repository is well-structured and organized, with clear sections covering different topics. It includes resources on system platforms, programming codes, mathematical foundations, algorithm principles, machine learning, deep learning, reinforcement learning, graph networks, model deployment, and practical applications. Additionally, there are dedicated sections on quantitative trading and investment, as well as large models. The repository is actively maintained and updated, ensuring that users have access to the latest information and resources.
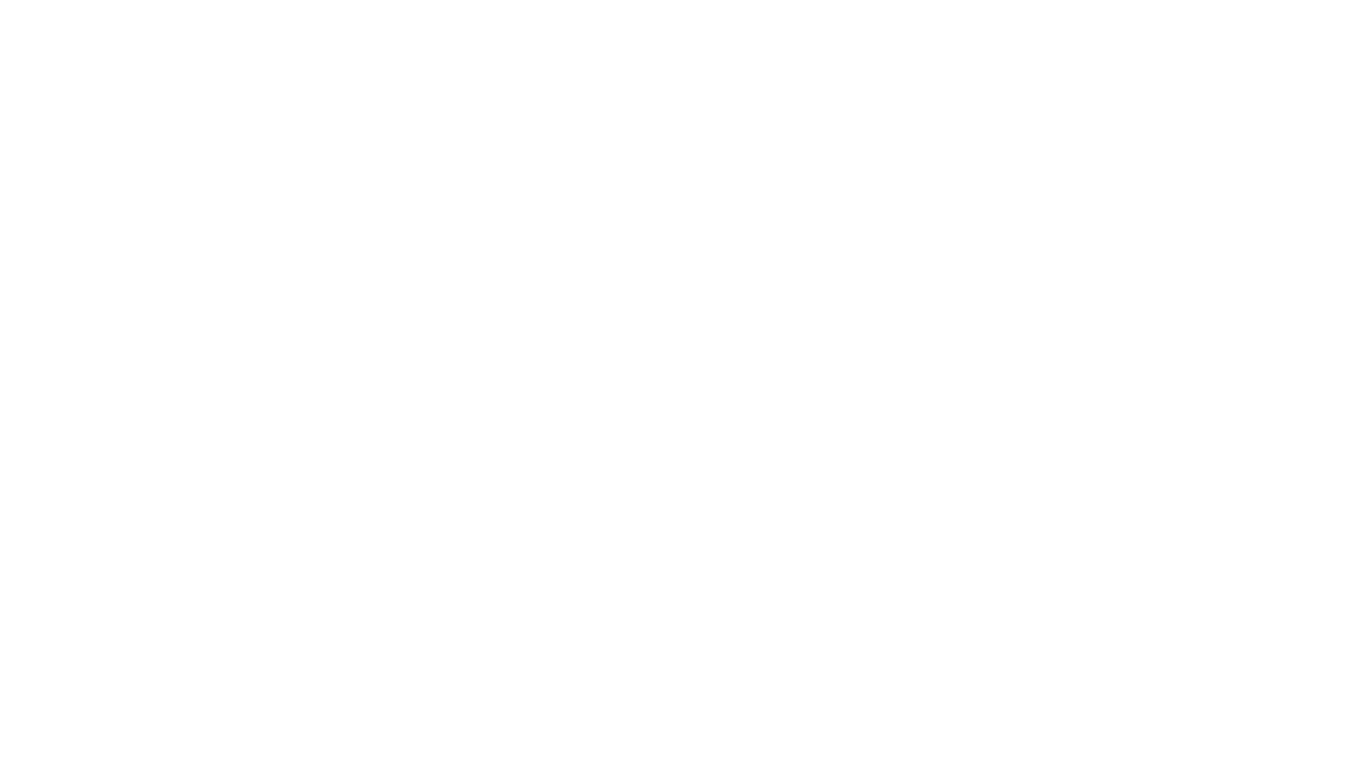
free-for-life
A massive list including a huge amount of products and services that are completely free! β Star on GitHub β’ π€ Contribute # Table of Contents * APIs, Data & ML * Artificial Intelligence * BaaS * Code Editors * Code Generation * DNS * Databases * Design & UI * Domains * Email * Font * For Students * Forms * Linux Distributions * Messaging & Streaming * PaaS * Payments & Billing * SSL
For similar jobs
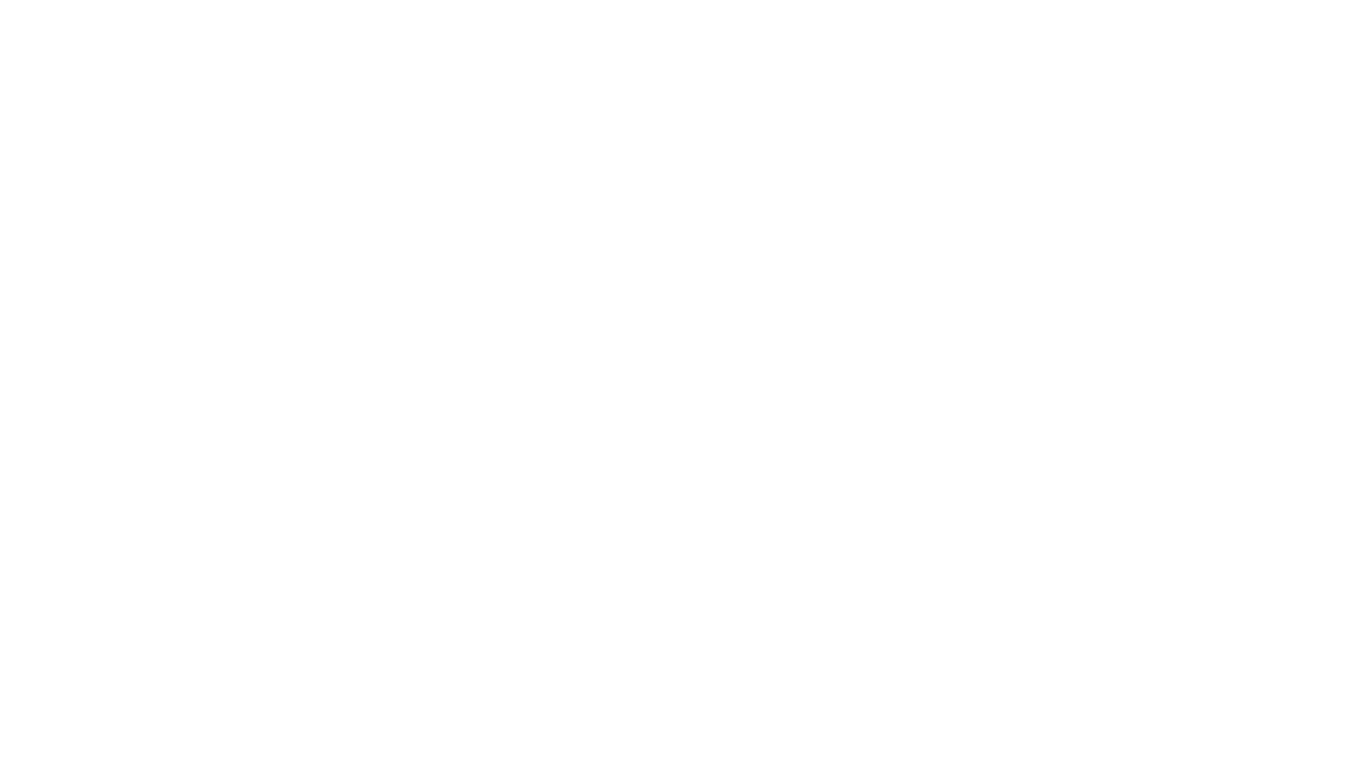
ai-data-science-team
The AI Data Science Team of Copilots is an AI-powered data science team that uses agents to help users perform common data science tasks 10X faster. It includes agents specializing in data cleaning, preparation, feature engineering, modeling, and interpretation of business problems. The project is a work in progress with new data science agents to be released soon. Disclaimer: This project is for educational purposes only and not intended to replace a company's data science team. No warranties or guarantees are provided, and the creator assumes no liability for financial loss.
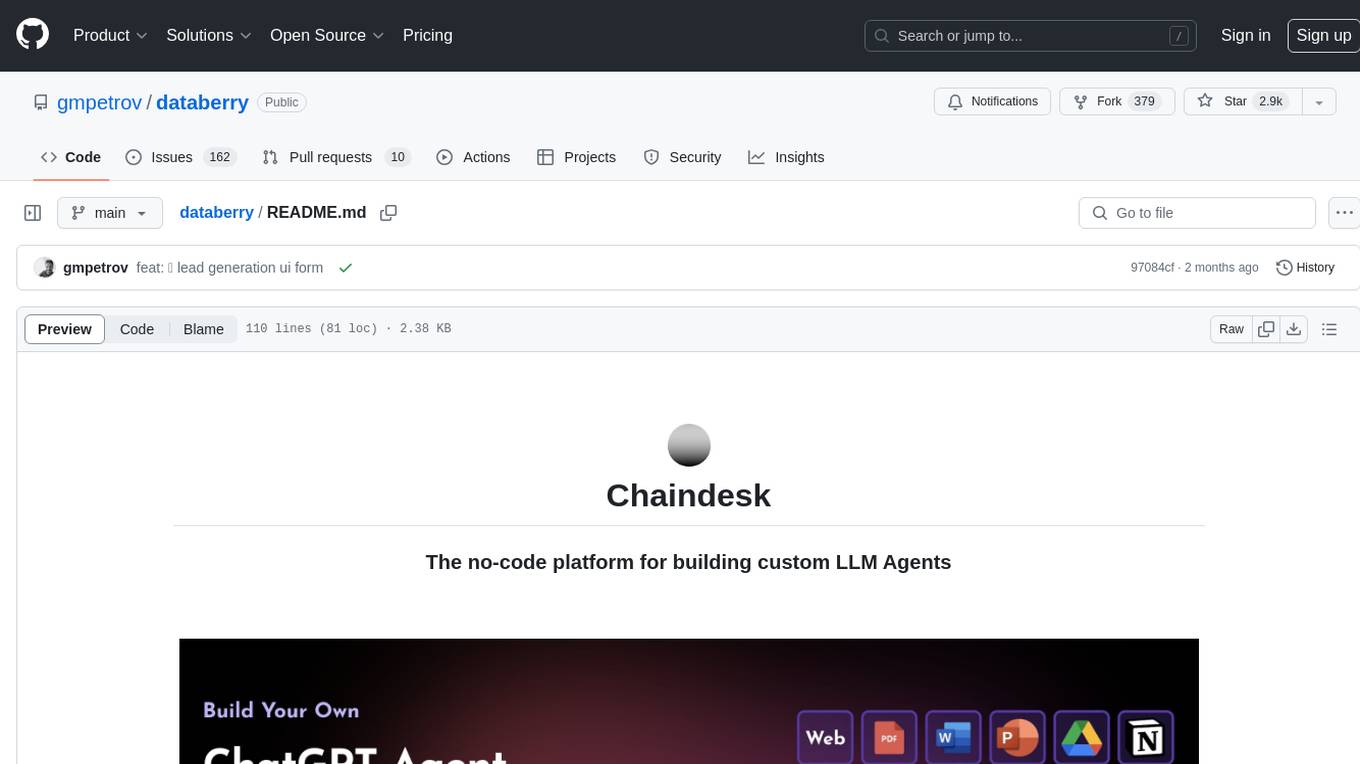
databerry
Chaindesk is a no-code platform that allows users to easily set up a semantic search system for personal data without technical knowledge. It supports loading data from various sources such as raw text, web pages, files (Word, Excel, PowerPoint, PDF, Markdown, Plain Text), and upcoming support for web sites, Notion, and Airtable. The platform offers a user-friendly interface for managing datastores, querying data via a secure API endpoint, and auto-generating ChatGPT Plugins for each datastore. Chaindesk utilizes a Vector Database (Qdrant), Openai's text-embedding-ada-002 for embeddings, and has a chunk size of 1024 tokens. The technology stack includes Next.js, Joy UI, LangchainJS, PostgreSQL, Prisma, and Qdrant, inspired by the ChatGPT Retrieval Plugin.
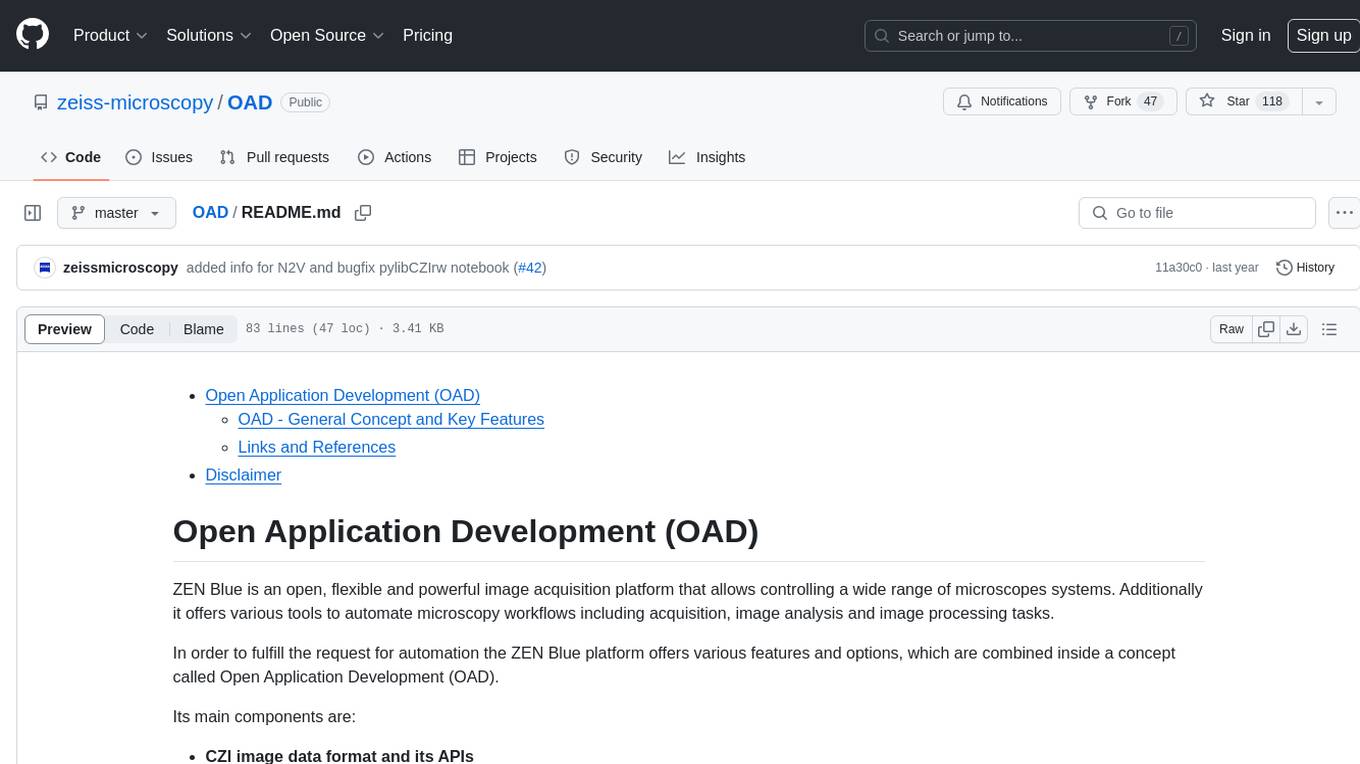
OAD
OAD is a powerful open-source tool for analyzing and visualizing data. It provides a user-friendly interface for exploring datasets, generating insights, and creating interactive visualizations. With OAD, users can easily import data from various sources, clean and preprocess data, perform statistical analysis, and create customizable visualizations to communicate findings effectively. Whether you are a data scientist, analyst, or researcher, OAD can help you streamline your data analysis workflow and uncover valuable insights from your data.
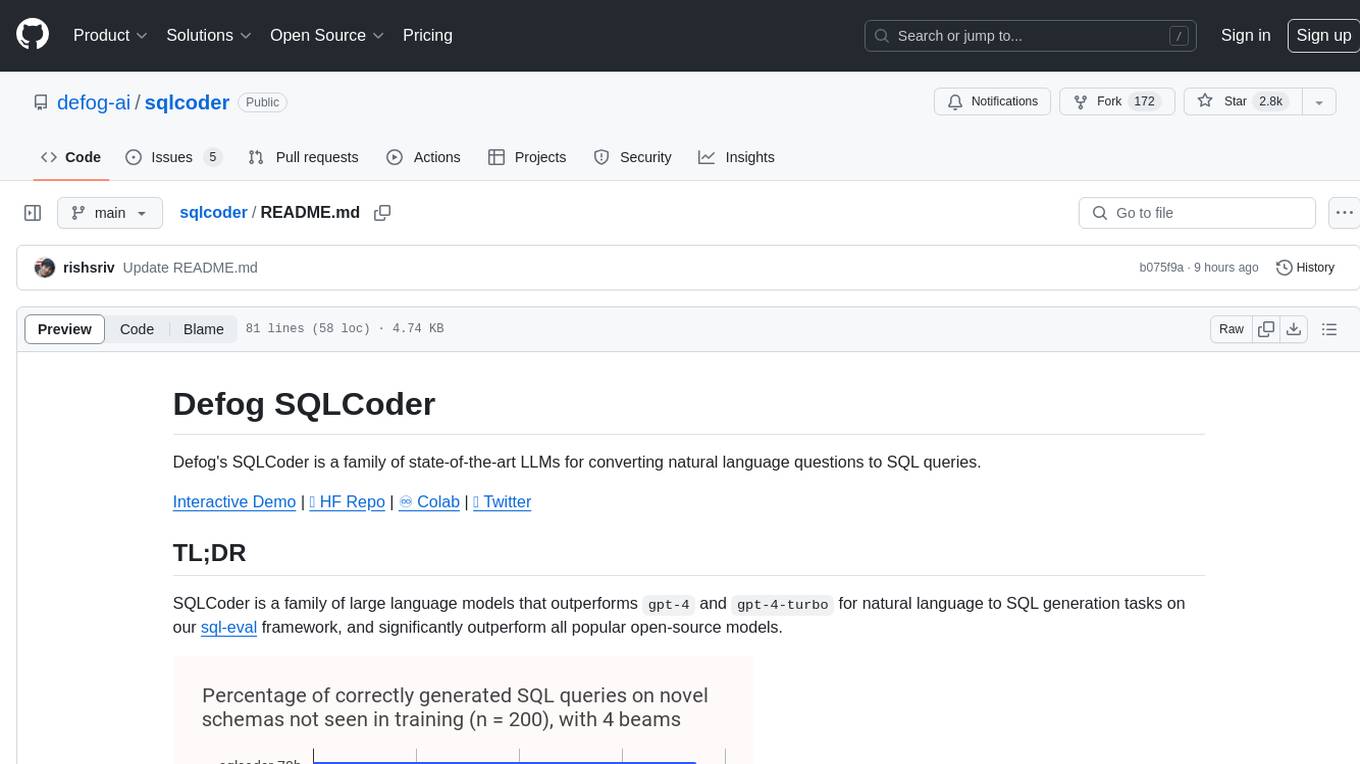
sqlcoder
Defog's SQLCoder is a family of state-of-the-art large language models (LLMs) designed for converting natural language questions into SQL queries. It outperforms popular open-source models like gpt-4 and gpt-4-turbo on SQL generation tasks. SQLCoder has been trained on more than 20,000 human-curated questions based on 10 different schemas, and the model weights are licensed under CC BY-SA 4.0. Users can interact with SQLCoder through the 'transformers' library and run queries using the 'sqlcoder launch' command in the terminal. The tool has been tested on NVIDIA GPUs with more than 16GB VRAM and Apple Silicon devices with some limitations. SQLCoder offers a demo on their website and supports quantized versions of the model for consumer GPUs with sufficient memory.
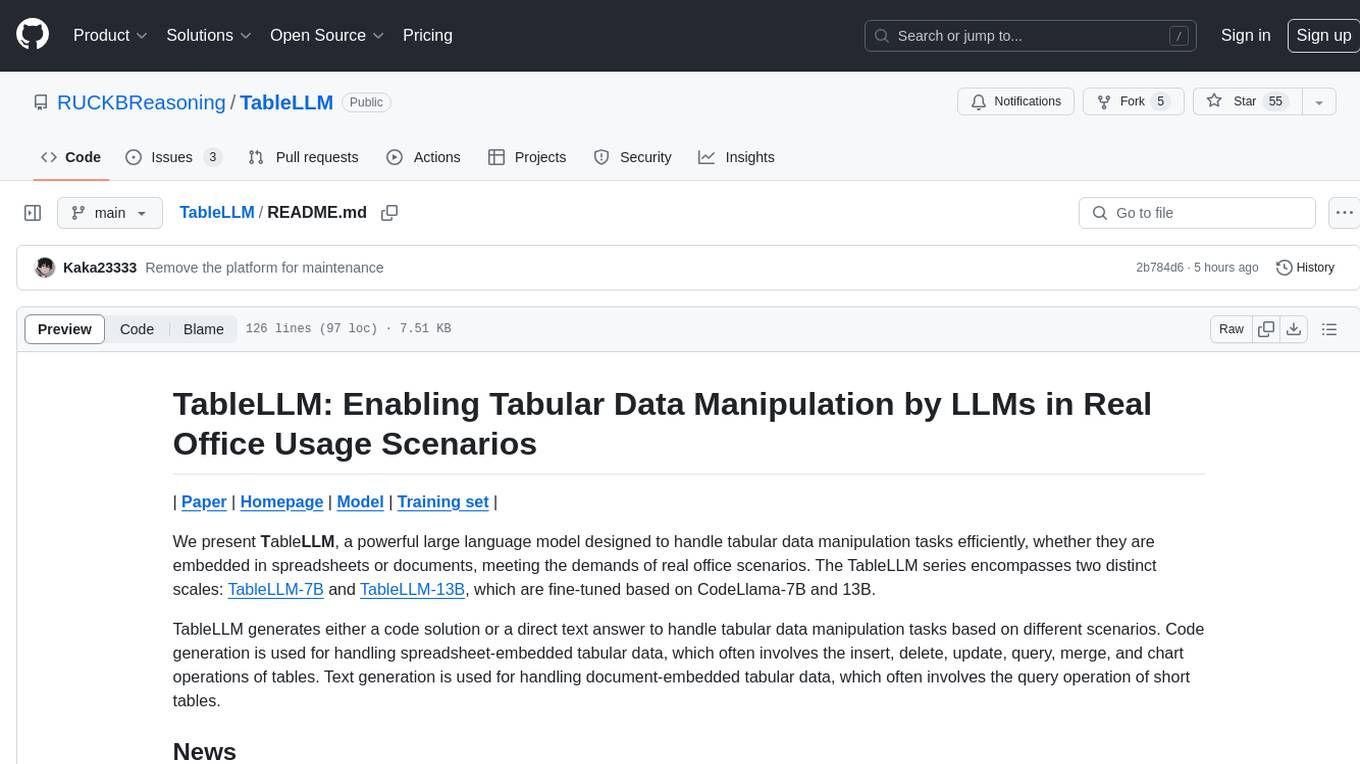
TableLLM
TableLLM is a large language model designed for efficient tabular data manipulation tasks in real office scenarios. It can generate code solutions or direct text answers for tasks like insert, delete, update, query, merge, and chart operations on tables embedded in spreadsheets or documents. The model has been fine-tuned based on CodeLlama-7B and 13B, offering two scales: TableLLM-7B and TableLLM-13B. Evaluation results show its performance on benchmarks like WikiSQL, Spider, and self-created table operation benchmark. Users can use TableLLM for code and text generation tasks on tabular data.
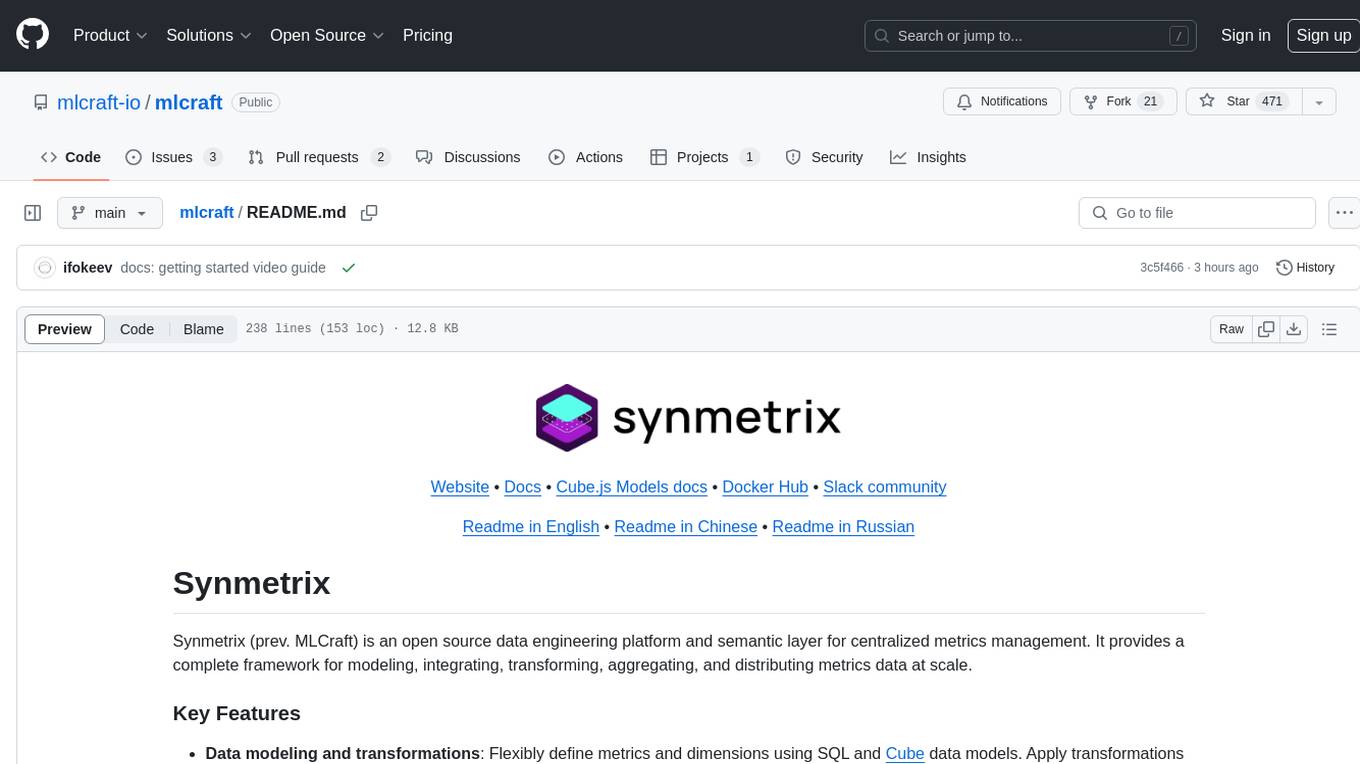
mlcraft
Synmetrix (prev. MLCraft) is an open source data engineering platform and semantic layer for centralized metrics management. It provides a complete framework for modeling, integrating, transforming, aggregating, and distributing metrics data at scale. Key features include data modeling and transformations, semantic layer for unified data model, scheduled reports and alerts, versioning, role-based access control, data exploration, caching, and collaboration on metrics modeling. Synmetrix leverages Cube (Cube.js) for flexible data models that consolidate metrics from various sources, enabling downstream distribution via a SQL API for integration into BI tools, reporting, dashboards, and data science. Use cases include data democratization, business intelligence, embedded analytics, and enhancing accuracy in data handling and queries. The tool speeds up data-driven workflows from metrics definition to consumption by combining data engineering best practices with self-service analytics capabilities.
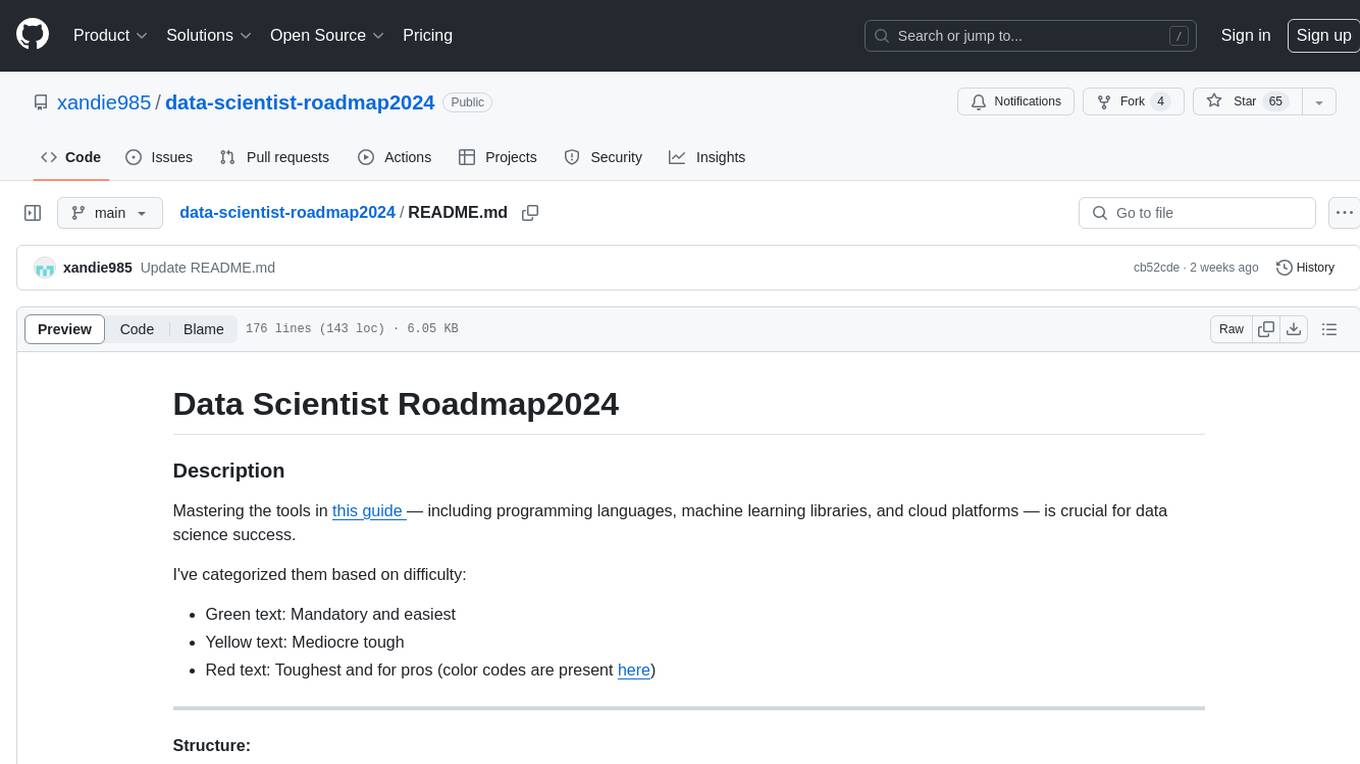
data-scientist-roadmap2024
The Data Scientist Roadmap2024 provides a comprehensive guide to mastering essential tools for data science success. It includes programming languages, machine learning libraries, cloud platforms, and concepts categorized by difficulty. The roadmap covers a wide range of topics from programming languages to machine learning techniques, data visualization tools, and DevOps/MLOps tools. It also includes web development frameworks and specific concepts like supervised and unsupervised learning, NLP, deep learning, reinforcement learning, and statistics. Additionally, it delves into DevOps tools like Airflow and MLFlow, data visualization tools like Tableau and Matplotlib, and other topics such as ETL processes, optimization algorithms, and financial modeling.
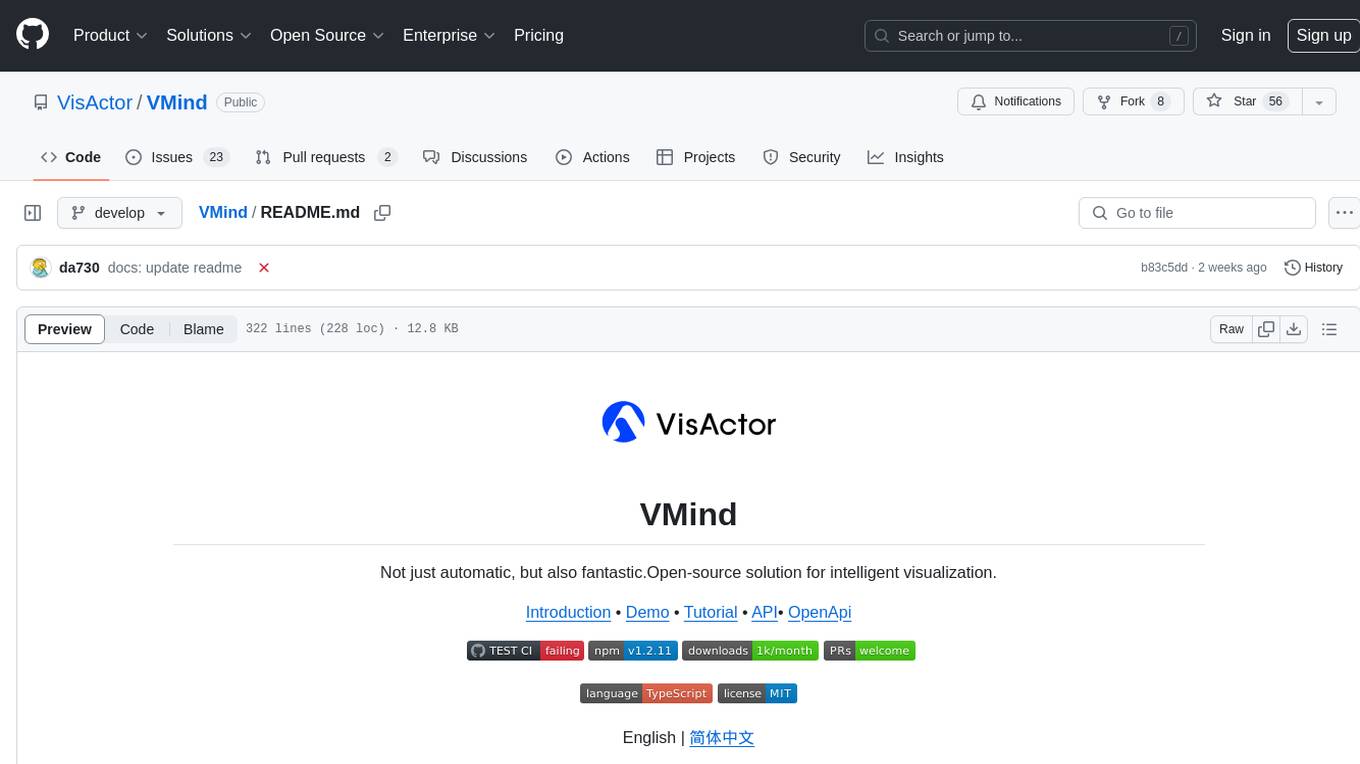
VMind
VMind is an open-source solution for intelligent visualization, providing an intelligent chart component based on LLM by VisActor. It allows users to create chart narrative works with natural language interaction, edit charts through dialogue, and export narratives as videos or GIFs. The tool is easy to use, scalable, supports various chart types, and offers one-click export functionality. Users can customize chart styles, specify themes, and aggregate data using LLM models. VMind aims to enhance efficiency in creating data visualization works through dialogue-based editing and natural language interaction.