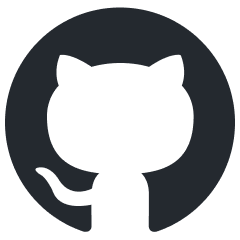
BambooAI
A Python library powered by Language Models (LLMs) for conversational data discovery and analysis.
Stars: 525
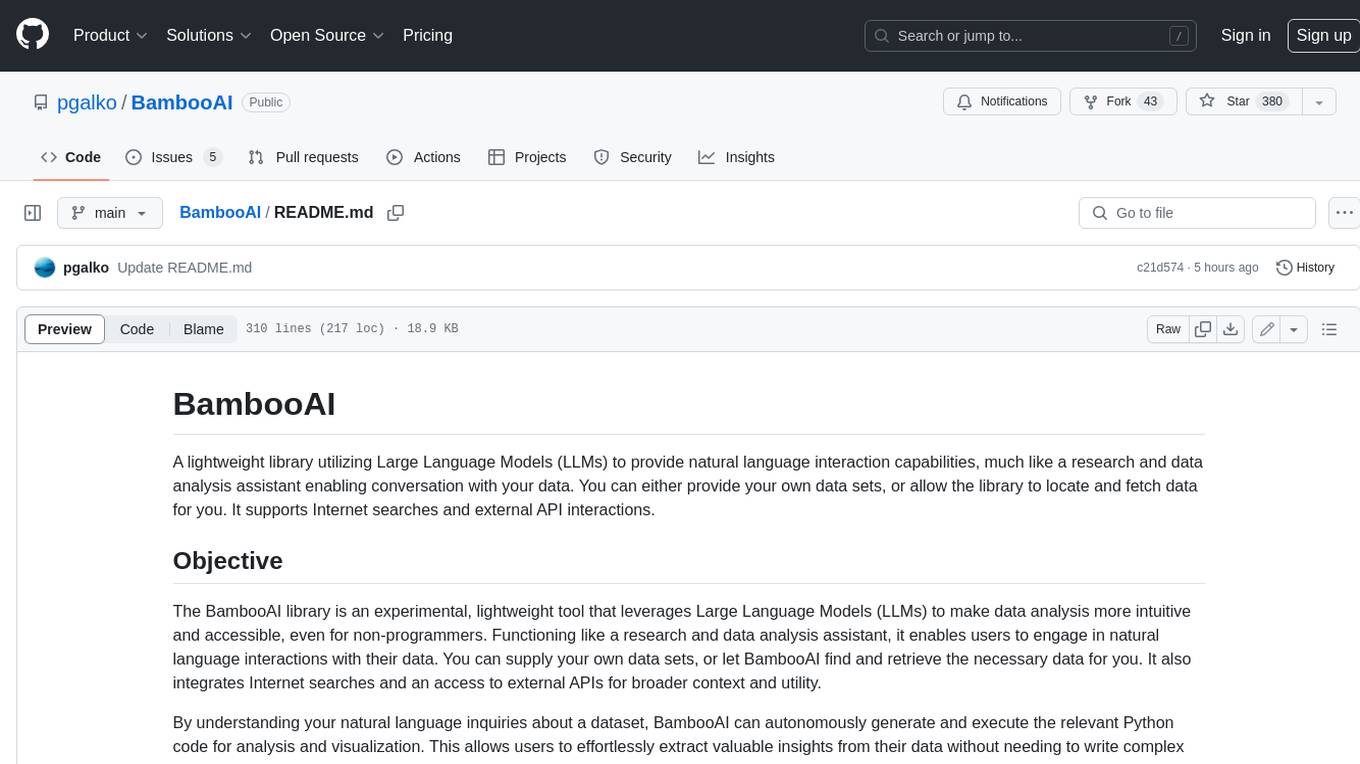
BambooAI is a lightweight library utilizing Large Language Models (LLMs) to provide natural language interaction capabilities, much like a research and data analysis assistant enabling conversation with your data. You can either provide your own data sets, or allow the library to locate and fetch data for you. It supports Internet searches and external API interactions.
README:
An open-source library that lets you analyze and explore data through natural language conversations using LLMs. Works with your local datasets or can fetch data from external sources and APIs.
The BambooAI library is an experimental tool that utilizes Large Language Models (LLMs) to facilitate data analysis, making it more accessible to users, including those without programming expertise. It functions as an assistant for research and data analysis, allowing users to interact with their data through natural language. Users can supply their own datasets or BambooAI can assist in sourcing the necessary data. The tool also integrates internet searches and accesses external APIs to enhance its functionality.
BambooAI processes natural language queries about datasets and can generate and execute Python code for data analysis and visualization. This enables users to derive insights from their data without extensive coding knowledge. Users simply input their dataset, ask questions in simple English, and BambooAI provides the answers, along with visualizations if needed, to help understand the data better.
BambooAI aims to augment the capabilities of data analysts across all levels. It simplifies data analysis and visualization, helping to streamline workflows. The library is designed to be user-friendly, efficient, and adaptable to meet various needs.
Try it out in Google Colab:
A Machine Learning Example using supplied dataframe:
!pip install pandas
!pip install bambooai
import pandas as pd
from bambooai import BambooAI
df = pd.read_csv('titanic.csv')
bamboo = BambooAI(df, debug=False, vector_db=False, search_tool=True)
bamboo.pd_agent_converse()
Jupyter Notebook:
Task: Can you please devise a machine learnig model to predict the survival of passengers on the Titanic? Output the accuracy of the model. Plot the confusion matrix, correlation matrix, and other relevant metrics. Search internet for the best approach to this task.
https://github.com/user-attachments/assets/59ef810c-80d8-4ef1-8edf-82ba64178b85
Web UI:
Task: Various queries related to sports data
https://github.com/user-attachments/assets/5d013ea0-cb44-4044-9cf4-3efa155288b8
The BambooAI agent operates through several key steps to interact with users and generate responses:
1. Initiation
- The user launches the BambooAI agent with a question.
- If no initial question is provided, the agent prompts the user for a question or an 'exit' command to terminate the program.
- The agent then enters a loop where it responds to each question provided, and upon completion, prompts the user for the next question. This loop continues until the user chooses to exit the program.
2. Task Routing
- The agent stores the received question and utilizes the Large Language Model (LLM) to classify it.
- The LLM determines whether the question necessitates a textual response, additional information (Google search: https://serper.dev/), or can be resolved using code.
- Depending on the task evaluation and classification the task is routed to the appropriate agent.
3. Dynamic Prompt Build
- If the question can be resolved by code, the agent determines whether the necessary data is contained within the provided dataset, requires downloading from an external source, or if the question is of a generic nature and data is not required.
- The agent then chooses its approach accordingly. It can formulate a plan to serve as a blueprint for the analysis.
- The original question is modified to align with this algorithm. The agent performs a semantic search against a vector database for similar questions.
- Any matching questions found are appended to the prompt as examples. A selected LLM model is then used to generate code based on the algorithm.
4. Debugging, Execution, and Error Correction
- The code is executed, and if errors occur, the agent logs the error message and refers it back to the LLM for correction.
- This process continues until successful code execution or the error correction limit is reached.
5. Results, Ranking, and Knowledge Base Build
- Post successful execution, user can rank the answer.
- If the rank surpasses a set threshold, the question, answer, code, and rank are stored in the Pinecone vector database.
- Regardless of the rank, the final answer or visualization is formatted and presented to the user.
Throughout this process, the agent continuously solicits user input, stores messages for context, and generates and executes code to ensure optimal results. Various AI models and a vector database are employed in this process to provide accurate and helpful responses to user's questions.
Flow chart (General agent flow):
The library is vendoe agnistic and supports use of various open source or proprietary models, either via API or localy.
API:
- OpenAI - All models
- Google - Gemini Models
- Anthropic - All Models
- Groq - All Models
- Mistral - All Models
Local:
- Ollama - All Models
- A Selection of local models(more info below)
You can specify what vendor/model you want to use for a specific agent by modifying the content of LLM_CONFIG file, replacing the default OpenAI model name with the model and vendor of your choicee. eg. {"agent": "Code Generator", "details": {"model": "open-mixtral-8x22b", "provider":"mistral","max_tokens": 4000, "temperature": 0}}
. The purpose of LLM_CONFIG is described in more detail below.
Installation
pip install bambooai
Usage
- Parameters
df: pd.DataFrame - Dataframe (It will try to source the data from internet, if 'df' is not provided)
max_conversations: int - Number of "user:assistant" conversation pairs to keep in memory for a context. Default=4
debug: bool - If True, the received code is sent back to the LLM for evaluation of its relevance to the user's question, along with code error checking and debugging.
search_tool: bool - If True, the Planner agent will use a "google search API: https://serper.dev/" if the required information is not available or satisfactory. By default it only support HTML sites, but can be enhanced with Selenium if the ChromeDriver exists on the system (details below).
vector_db: bool - If True, each answer will first be ranked from 1 to 10. If the rank surpasses a certain threshold (8), the corresponding question (vectorised), plan, code, and rank (metadata) are all stored in the Pinecone database. Each time a new question is asked, these records will be searched. If the similarity score is above 0.9, they will be offered as examples and included in the prompt (in a one-shot learning scenario)
df_onthology: bool - If True, the onthology defined in the module `df_onthology.py` will be used to inform LLM of the dataframe structure, metrics, record frequency, keys, joins, abstract functions etc. The onthology is custom for each dataframe type, and needs to be defined by the user. Sample onthology is included. This feature signifficantly improves performance, and quality of the solutions.
exploratory: bool - If set to True, the LLM will evaluate the user's question and select an "Expert" that is best suited to address the question (experts: Research Specialist, Data Analyst). In addition, if the task involves code generation/execution, it will generate a task list detailing the steps, which will subsequently be sent to the LLM as a part of the prompt for the next action. This method is particularly effective for vague user prompts, but it might not perform as efficiently with more specific prompts. The default setting is True.
e.g. bamboo = BambooAI(df, debug=True, vector_db=True, search_tool=True, exploratory=True)
bamboo = BambooAI(df,debug=False, vector_db=False, exploratory=True, search_tool=True)
- LLM Config
The agent specific llm configuration is stored in LLM_CONFIG
environment variable, or in the "LLM_CONFIG.json file which needs to be stored in the BambooAI's working directory. The config is in a form of JSON list of dictionaries and specifies model name, provider, temperature and max_tokens for each agent. You can use the provided LLM_CONFIG_sample.json as a starting point, and modify the config to reflect your preferences. If neither "ENV VAR" nor "LLM_CONFIG.json" is present, BambooAI will use the default hardcoded configuration that uses "gpt-3.5-turbo" for all agents.
- Prompt Templates
The BambooAI library uses default hand crafted set of prompt templates for each agent. If you want to experiment with them, you can modify the provided "PROMPT_TEMPLATES_sample.json" file, remove the "_sample from its name and store in the working directory. Subsequently, the content of the modified "PROMPT_TEMPLATES.json" will be used instead of the defaults. You can always revert back to default prompts by removing/renaming the modified "PROMPT_TEMPLATES.json".
- Example usage: Run in a loop
# Run in a loop remembering the conversation history
import pandas as pd
from bambooai import BambooAI
df = pd.read_csv('test_activity_data.csv')
bamboo = BambooAI(df)
bamboo.pd_agent_converse()
- Example Usage: Single execution
# Run programaticaly (Single execution).
import pandas as pd
from bambooai import BambooAI
df = pd.read_csv('test_activity_data.csv')
bamboo = BambooAI(df)
bamboo.pd_agent_converse("Calculate 30, 50, 75 and 90 percentiles of the heart rate column")
Environment Variables
The library requires an OpenAI API account and the API key to connect to OpenAI LLMs. The OpenAI API key needs to be stored in a OPENAI_API_KEY
environment variable.
The key can be obtained from here: https://platform.openai.com/account/api-keys.
In addition to OpenAI models a selection of models from different providers is also supported (Groq, Gemini, Mistral, Anthropic). The API keys needs to be stored in environment variables in the following format <VENDOR_NAME>_API_KEY
.
You need to use GEMINI_API_KEY
for Google Gemini models.
As mentioned above, the llm config can be stored in a string format in the LLM_CONFIG
environment variable. You can use the content of the provided LLM_CONFIG_sample.json as a starting point and modify to your preference, depending on what models you have access to.
The Pincone vector db is optional. If you don want to use it, you dont need to do anything. If you have an account with Pinecone and would like to use the knowledge base and ranking features, you will be required to setup PINECONE_API_KEY
envirooment variable, and set the 'vector_db' parameter to True. The vector db index is created upon first execution.
The Google Search is also optional. If you don want to use it, you dont need to do anything. If you have an account with Serper and would like to use the Google Search functionality, you will be required to setup and account with ": https://serper.dev/", and set SERPER_API_KEY
environment variable, and set the 'search_tool' parameter to True. By default bambooai can only scrape websites with HTML content. However it is also capable of using Selenium with ChromeDriver, which is much more powerfull. To enable this functionality you will need to manualy download a version of ChromeDriver that matches your version of the Chrome browser, store it on the filesystem and create an environment variable SELENIUM_WEBDRIVER_PATH
with a path to your ChromeDriver. BambooAI wil pick it up automaticaly, and use Selenium for all scraping tasks.
Local Open Source Models
The library currently directly supports the following open-source models. I have selected the models that currently score the highest on the HumanEval benchmark.
- WizardCoder(WizardLM): WizardCoder-15B-V1.0, WizardCoder-Python-7B-V1.0, WizardCoder-Python-13B-V1.0, WizardCoder-Python-34B-V1.0
- WizardCoder GPTQ(TheBloke): WizardCoder-15B-1.0-GPTQ, WizardCoder-Python73B-V1.0-GPTQ, WizardCoder-Python-13B-V1.0-GPTQ, WizardCoder-Python-34B-V1.0-GPTQ
- CodeLlama Instruct(TheBloke): CodeLlama-7B-Instruct-fp16, CodeLlama-13B-Instruct-fp16, CodeLlama-34B-Instruct-fp16
- CodeLlama Instruct(Phind): Phind-CodeLlama-34B-v2
- CodeLlama Completion(TheBloke): CodeLlama-7B-Python-fp16, CodeLlama-13B-Python-fp16, CodeLlama-34B-Python-fp16
If you want to use the local model for a specific agent, modify the LLM_CONFIG content replacing the OpenAI model name with the local model name and change the provider value to 'local'. eg. {"agent": "Code Generator", "details": {"model": "Phind-CodeLlama-34B-v2", "provider":"local","max_tokens": 2000, "temperature": 0}}
At present it is recommended to use local models only for code generation tasks, all other tasks like pseudo code generaration, summarisation, error correction and ranking should be still handled by OpenAI models of choice. The model is downloaded from Huggingface and cached localy for subsequent executions. For a reasonable performance it requires CUDA enabled GPU and the pytorch library compatible with the CUDA version. Below are the required libraries that are not included in the package and will need to be installed independently:
pip install torch torchvision torchaudio --index-url https://download.pytorch.org/whl/cu118 (Adjust to match your CUDA version. This library is already included in Colab notebooks)
pip install auto-gptq (Only required if using WizardCoder-15B-1.0-GPTQ model)
pip install accelerate
pip install einops
pip install xformers
pip install bitsandbytes
The settings and parameters for local models are located in local_models.py module and can be adjusted to match your particular configuration or preferences.
Ollama
The library also supports the use of Ollama https://ollama.com/ and all of it's models. If you want to use a local Ollama model for a specific agent, modify the LLM_CONFIG content replacing the OpenAI model name with the Ollama model name and change the provider value to 'ollama'. eg. {"agent": "Code Generator", "details": {"model": "llama3:70b", "provider":"ollama","max_tokens": 2000, "temperature": 0}}
Logging
All LLM interactions (local or via APIs) are logged in the bambooai_consolidated_log.json
file. When the size of the log file reaches 5 MB, a new log file is created. A total of 3 log files are kept on the file system before the oldest file gets overwritten.
The following details are captured:
- Chain ID
- All LLM calls (steps) within the chain, including details of each call eg. agent name, timestamp, model, prompt (context memory), response, token use, cost, tokens per second etc.
- Chain summary, including token use, cost, count of llm calls, tokens per second etc.
- Summary per LLM, including token use, cost, number of calls, tokens per second etc.
Log Structure:
- chain_id: 1695375585
├─ chain_details (LLM Calls)
│ ├─ List of Dictionaries (Multiple Steps)
│ ├─ Call 1
│ │ ├─ agent (String)
│ │ ├─ chain_id (Integer)
│ │ ├─ timestamp (String)
│ │ ├─ model (String)
│ │ ├─ messages (List)
│ │ │ └─ role (String)
│ │ │ └─ content (String)
│ │ └─ Other Fields (content, prompt_tokens, completion_tokens, total_tokens, elapsed_time, tokens_per_second, cost)
│ ├─ Call 2
│ │ └─ ... (Similar Fields)
│ └─ ... (Call 3, Call 4, Call 5 ...)
│
├─ chain_summary
│ ├─ Dictionary
│ ├─ Total LLM Calls (Integer)
│ ├─ Prompt Tokens (Integer)
│ ├─ Completion Tokens (Integer)
│ ├─ Total Tokens (Integer)
│ ├─ Total Time (Float)
│ ├─ Tokens per Second (Float)
│ ├─ Total Cost (Float)
│
├─ summary_per_model
├─ Dictionary
├─ LLM 1 (Dictionary)
│ ├─ LLM Calls (Integer)
│ ├─ Prompt Tokens (Integer)
│ ├─ Completion Tokens (Integer)
│ ├─ Total Tokens (Integer)
│ ├─ Total Time (Float)
│ ├─ Tokens per Second (Float)
│ ├─ Total Cost (Float)
├─ LLM 2
| └─ ... (Similar Fields)
└─ ... (LLM 3, LLM 4, LLM 5 ...)
Task: Devise a machine learning model to predict the survival of passengers on the Titanic. The output should include the accuracy of the model and visualizations of the confusion matrix, correlation matrix, and other relevant metrics.
Dataset: Titanic.csv
Model: GPT-4-Turbo
-
Result:
-
Confusion Matrix:
- True Negative (TN): 90 passengers were correctly predicted as not surviving.
- True Positive (TP): 56 passengers were correctly predicted as surviving.
- False Negative (FN): 18 passengers were incorrectly predicted as not surviving.
- False Positive (FP): 15 passengers were incorrectly predicted as surviving.
-
Confusion Matrix:
Metric | Value |
---|---|
Execution Time | 77.12 seconds |
Input Tokens | 7128 |
Output Tokens | 1215 |
Total Cost | $0.1077 |
-
Result:
-
Confusion Matrix:
- True Negative (TN): 92 passengers were correctly predicted as not surviving.
- True Positive (TP): 55 passengers were correctly predicted as surviving.
- False Negative (FN): 19 passengers were incorrectly predicted as not surviving.
- False Positive (FP): 13 passengers were incorrectly predicted as surviving.
-
Confusion Matrix:
Metric | Value |
---|---|
Execution Time | 47.39 seconds |
Input Tokens | 722 |
Output Tokens | 931 |
Total Cost | $0.0353 |
Objective Assessment of AI Tools for Sports Data Analytics_ Maxwell-v2 vs. Generic LLMs.pdf
- The library currently suports models from various vendors OpenAI, Anthropic, Google, Mistral, Groq served through their APIs. It also supports all Ollama models.
- The library executes LLM generated Python code, this can be bad if the LLM generated Python code is harmful. Use cautiously.
- Be sure to monitor your token usage. The cummulative summary is displayed after each interaction.*
Contributions are welcome; please feel free to open a pull request. Keep in mind that our goal is to maintain a concise codebase with high readability.
- A lot :-)
For Tasks:
Click tags to check more tools for each tasksFor Jobs:
Alternative AI tools for BambooAI
Similar Open Source Tools
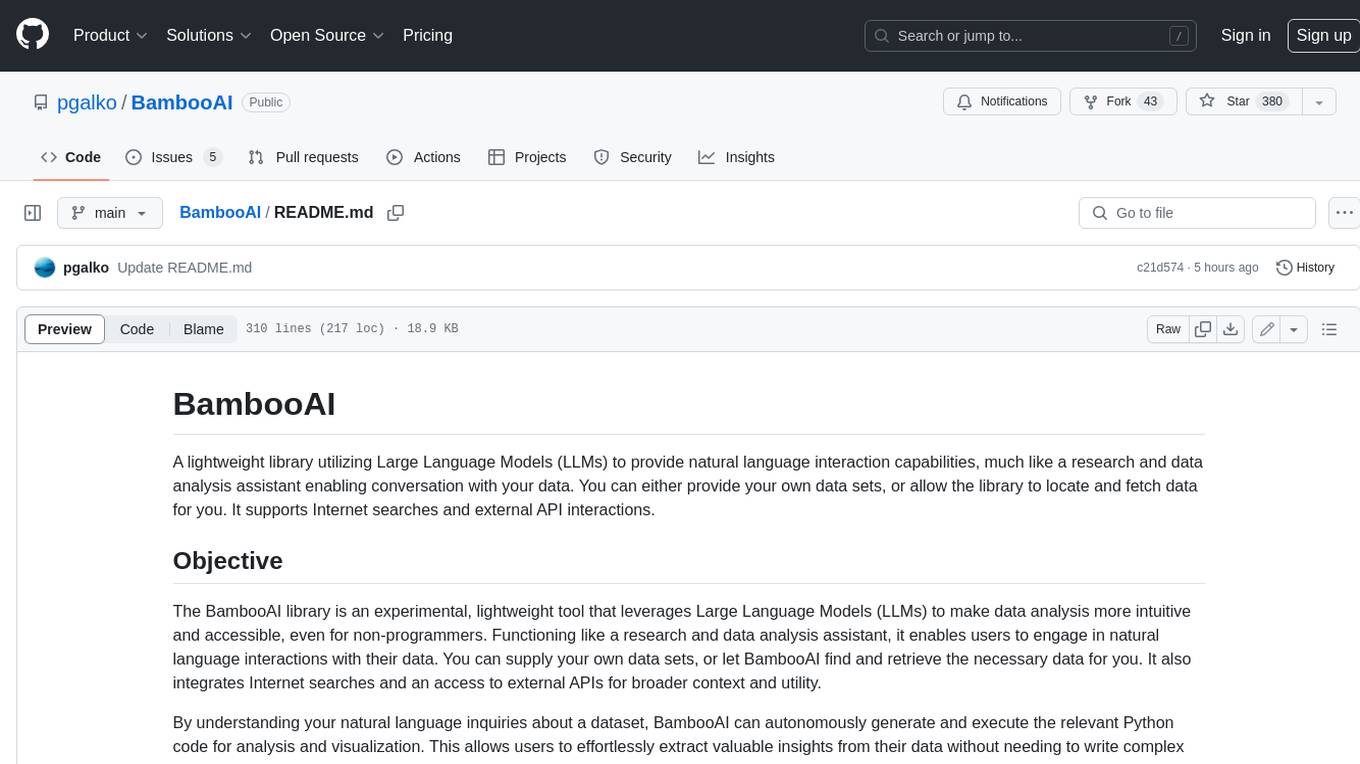
BambooAI
BambooAI is a lightweight library utilizing Large Language Models (LLMs) to provide natural language interaction capabilities, much like a research and data analysis assistant enabling conversation with your data. You can either provide your own data sets, or allow the library to locate and fetch data for you. It supports Internet searches and external API interactions.
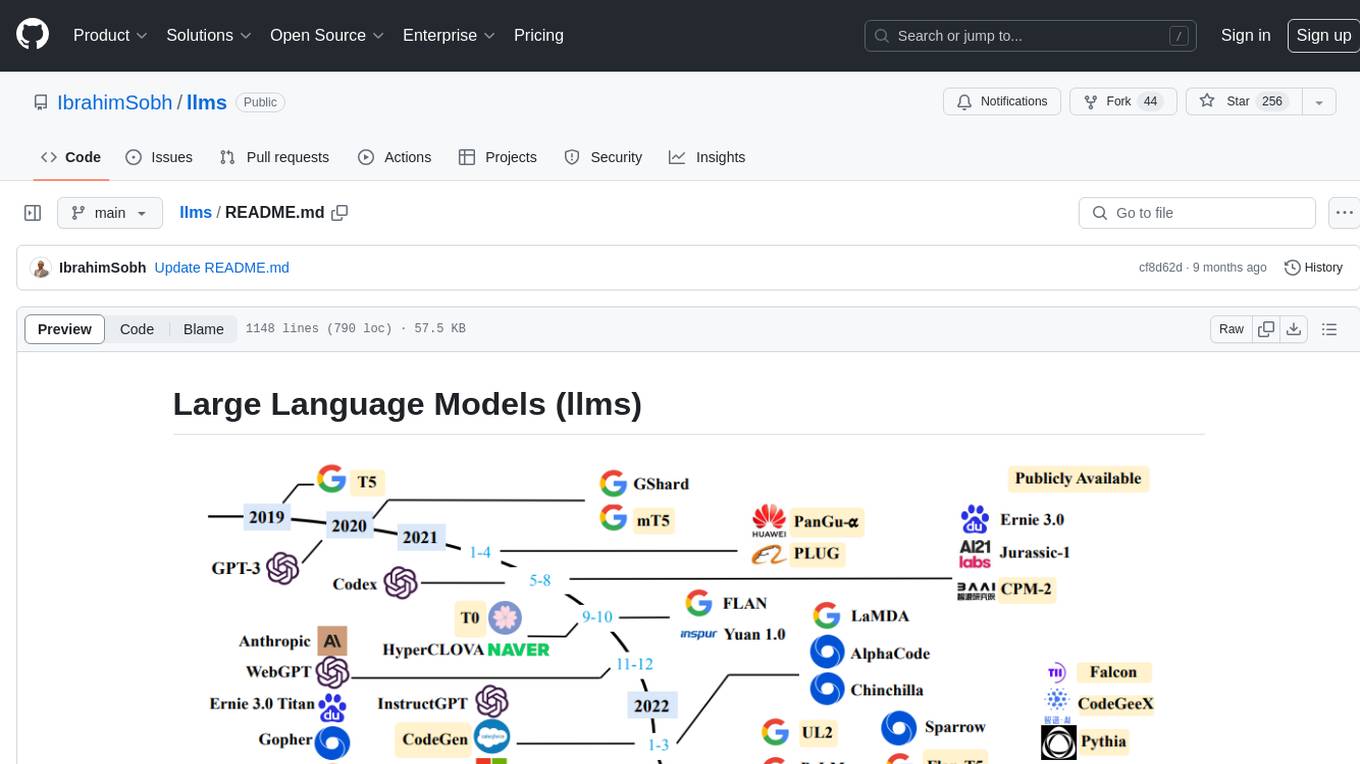
llms
The 'llms' repository is a comprehensive guide on Large Language Models (LLMs), covering topics such as language modeling, applications of LLMs, statistical language modeling, neural language models, conditional language models, evaluation methods, transformer-based language models, practical LLMs like GPT and BERT, prompt engineering, fine-tuning LLMs, retrieval augmented generation, AI agents, and LLMs for computer vision. The repository provides detailed explanations, examples, and tools for working with LLMs.
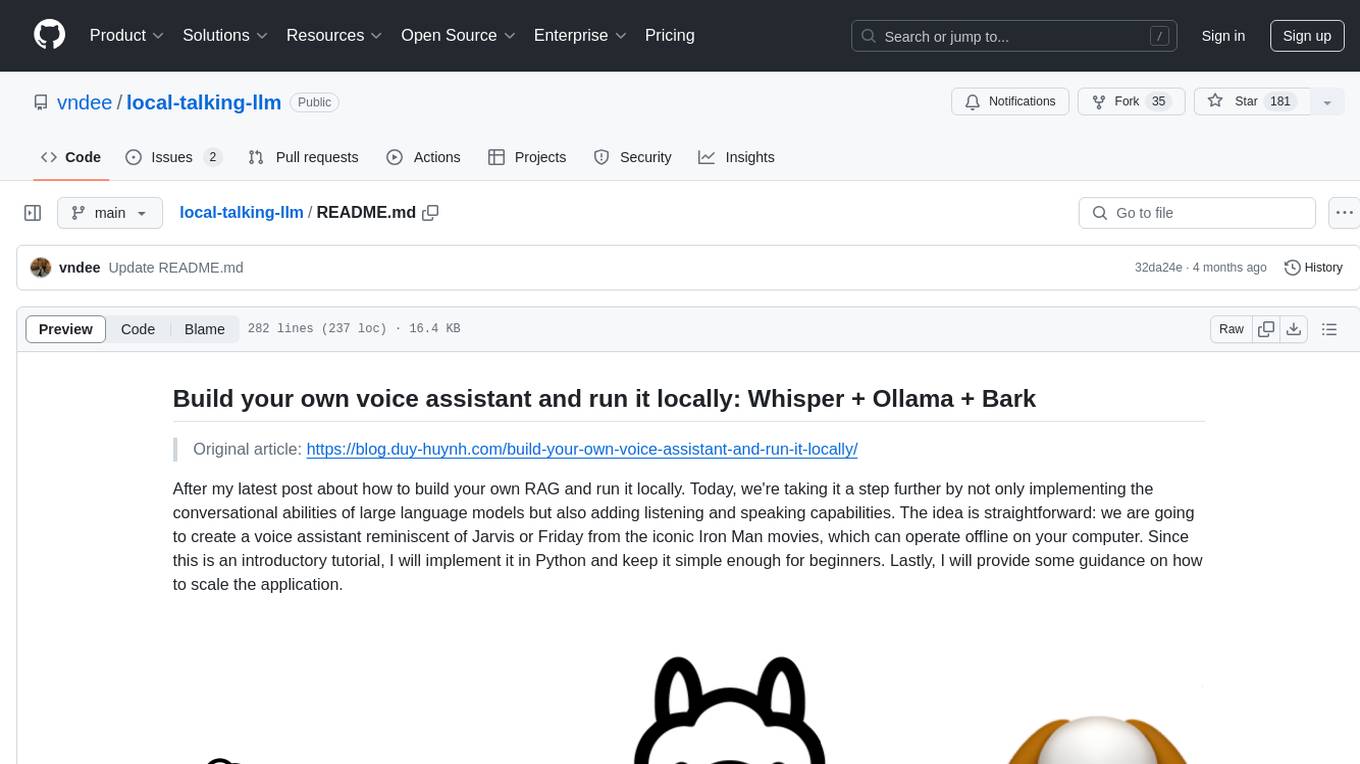
local-talking-llm
The 'local-talking-llm' repository provides a tutorial on building a voice assistant similar to Jarvis or Friday from Iron Man movies, capable of offline operation on a computer. The tutorial covers setting up a Python environment, installing necessary libraries like rich, openai-whisper, suno-bark, langchain, sounddevice, pyaudio, and speechrecognition. It utilizes Ollama for Large Language Model (LLM) serving and includes components for speech recognition, conversational chain, and speech synthesis. The implementation involves creating a TextToSpeechService class for Bark, defining functions for audio recording, transcription, LLM response generation, and audio playback. The main application loop guides users through interactive voice-based conversations with the assistant.
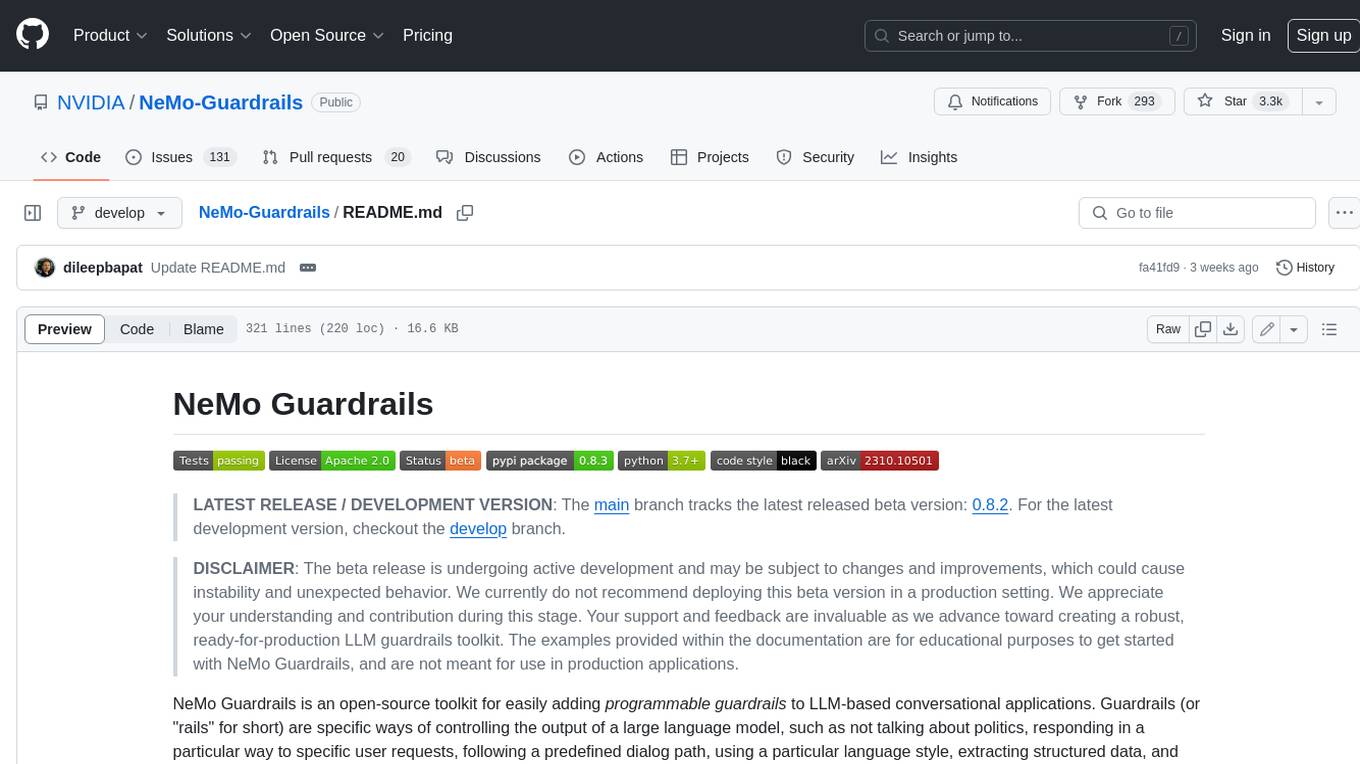
NeMo-Guardrails
NeMo Guardrails is an open-source toolkit for easily adding _programmable guardrails_ to LLM-based conversational applications. Guardrails (or "rails" for short) are specific ways of controlling the output of a large language model, such as not talking about politics, responding in a particular way to specific user requests, following a predefined dialog path, using a particular language style, extracting structured data, and more.
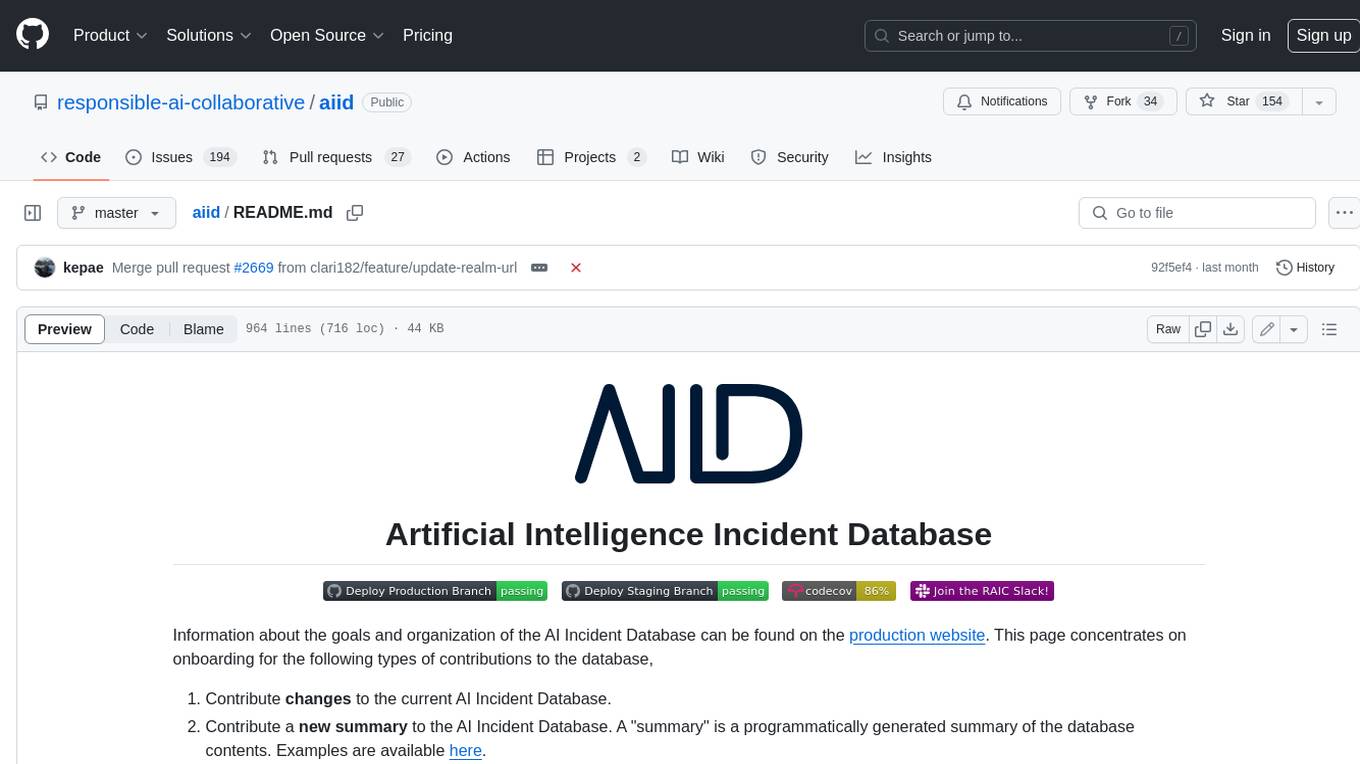
aiid
The Artificial Intelligence Incident Database (AIID) is a collection of incidents involving the development and use of artificial intelligence (AI). The database is designed to help researchers, policymakers, and the public understand the potential risks and benefits of AI, and to inform the development of policies and practices to mitigate the risks and promote the benefits of AI. The AIID is a collaborative project involving researchers from the University of California, Berkeley, the University of Washington, and the University of Toronto.
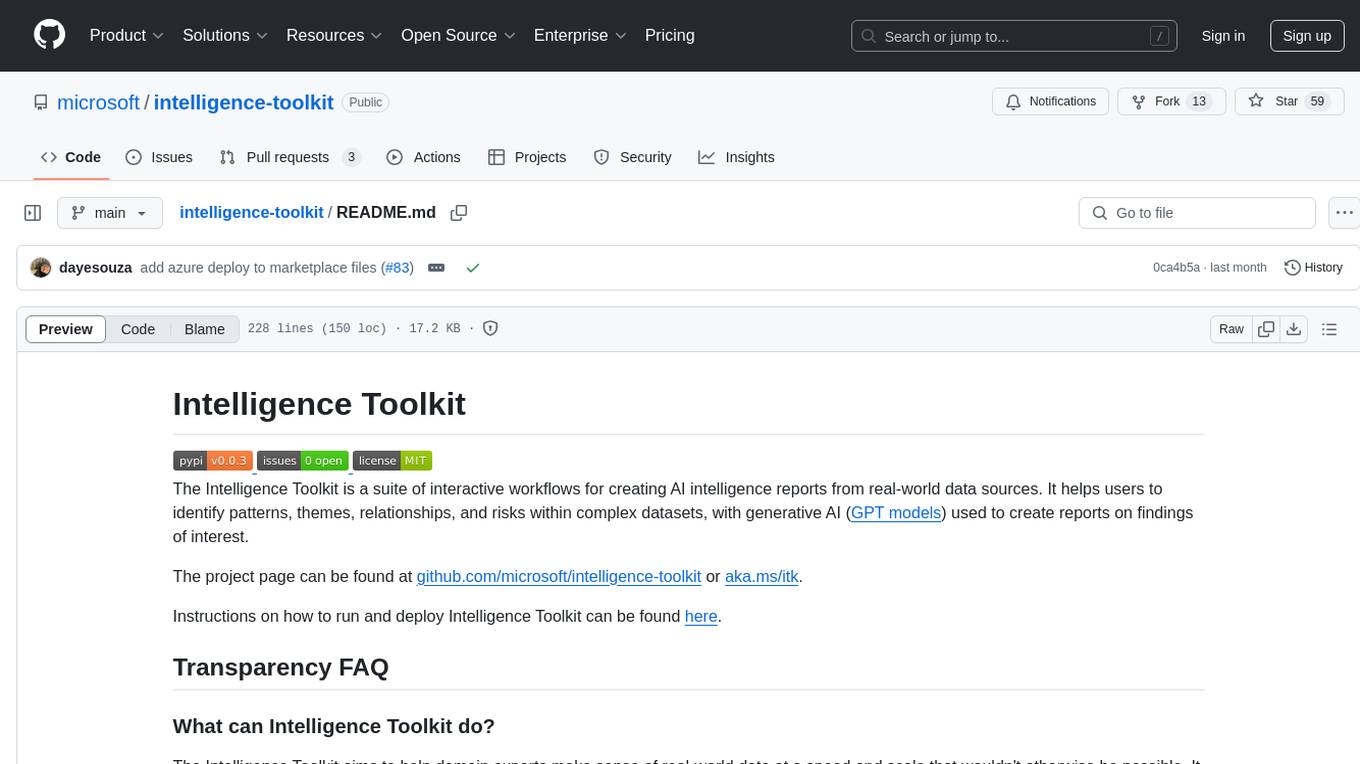
intelligence-toolkit
The Intelligence Toolkit is a suite of interactive workflows designed to help domain experts make sense of real-world data by identifying patterns, themes, relationships, and risks within complex datasets. It utilizes generative AI (GPT models) to create reports on findings of interest. The toolkit supports analysis of case, entity, and text data, providing various interactive workflows for different intelligence tasks. Users are expected to evaluate the quality of data insights and AI interpretations before taking action. The system is designed for moderate-sized datasets and responsible use of personal case data. It uses the GPT-4 model from OpenAI or Azure OpenAI APIs for generating reports and insights.
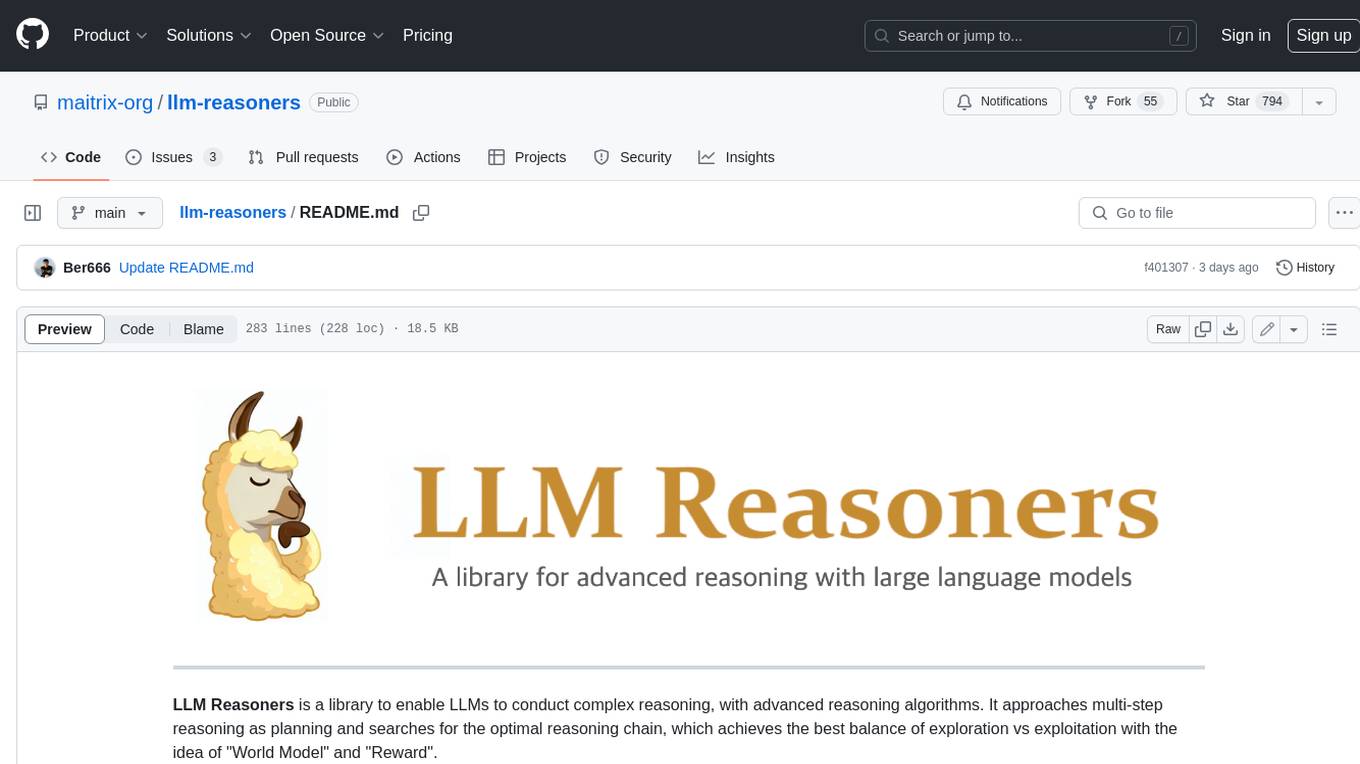
llm-reasoners
LLM Reasoners is a library that enables LLMs to conduct complex reasoning, with advanced reasoning algorithms. It approaches multi-step reasoning as planning and searches for the optimal reasoning chain, which achieves the best balance of exploration vs exploitation with the idea of "World Model" and "Reward". Given any reasoning problem, simply define the reward function and an optional world model (explained below), and let LLM reasoners take care of the rest, including Reasoning Algorithms, Visualization, LLM calling, and more!
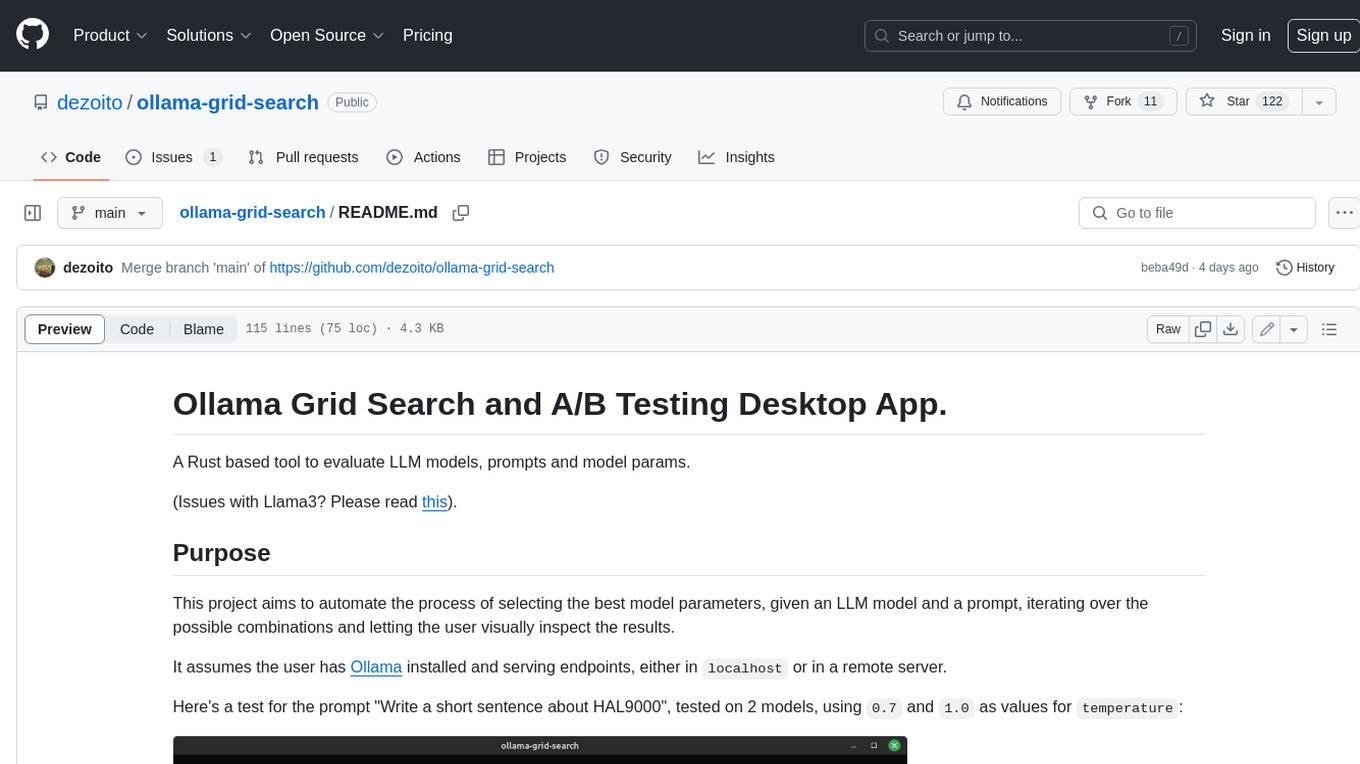
ollama-grid-search
A Rust based tool to evaluate LLM models, prompts and model params. It automates the process of selecting the best model parameters, given an LLM model and a prompt, iterating over the possible combinations and letting the user visually inspect the results. The tool assumes the user has Ollama installed and serving endpoints, either in `localhost` or in a remote server. Key features include: * Automatically fetches models from local or remote Ollama servers * Iterates over different models and params to generate inferences * A/B test prompts on different models simultaneously * Allows multiple iterations for each combination of parameters * Makes synchronous inference calls to avoid spamming servers * Optionally outputs inference parameters and response metadata (inference time, tokens and tokens/s) * Refetching of individual inference calls * Model selection can be filtered by name * List experiments which can be downloaded in JSON format * Configurable inference timeout * Custom default parameters and system prompts can be defined in settings
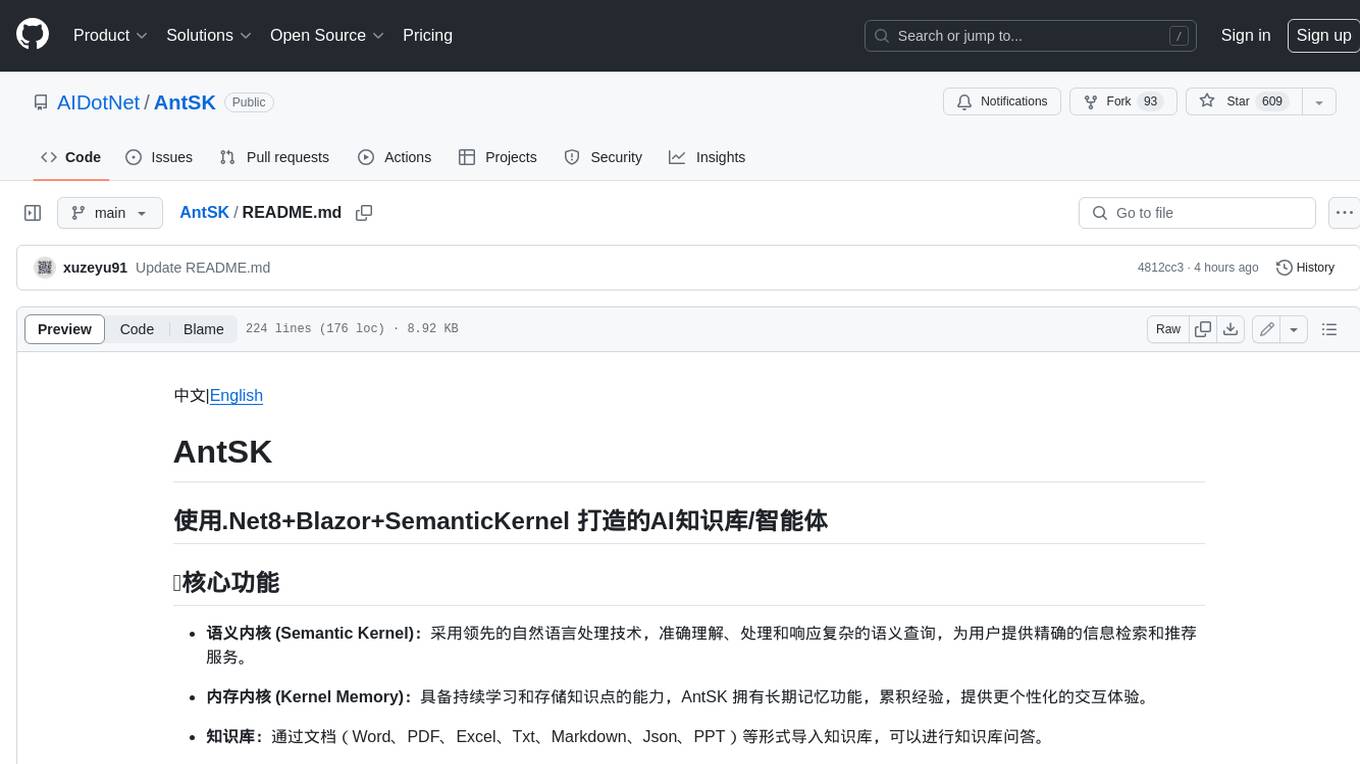
AntSK
AntSK is an AI knowledge base/agent built with .Net8+Blazor+SemanticKernel. It features a semantic kernel for accurate natural language processing, a memory kernel for continuous learning and knowledge storage, a knowledge base for importing and querying knowledge from various document formats, a text-to-image generator integrated with StableDiffusion, GPTs generation for creating personalized GPT models, API interfaces for integrating AntSK into other applications, an open API plugin system for extending functionality, a .Net plugin system for integrating business functions, real-time information retrieval from the internet, model management for adapting and managing different models from different vendors, support for domestic models and databases for operation in a trusted environment, and planned model fine-tuning based on llamafactory.
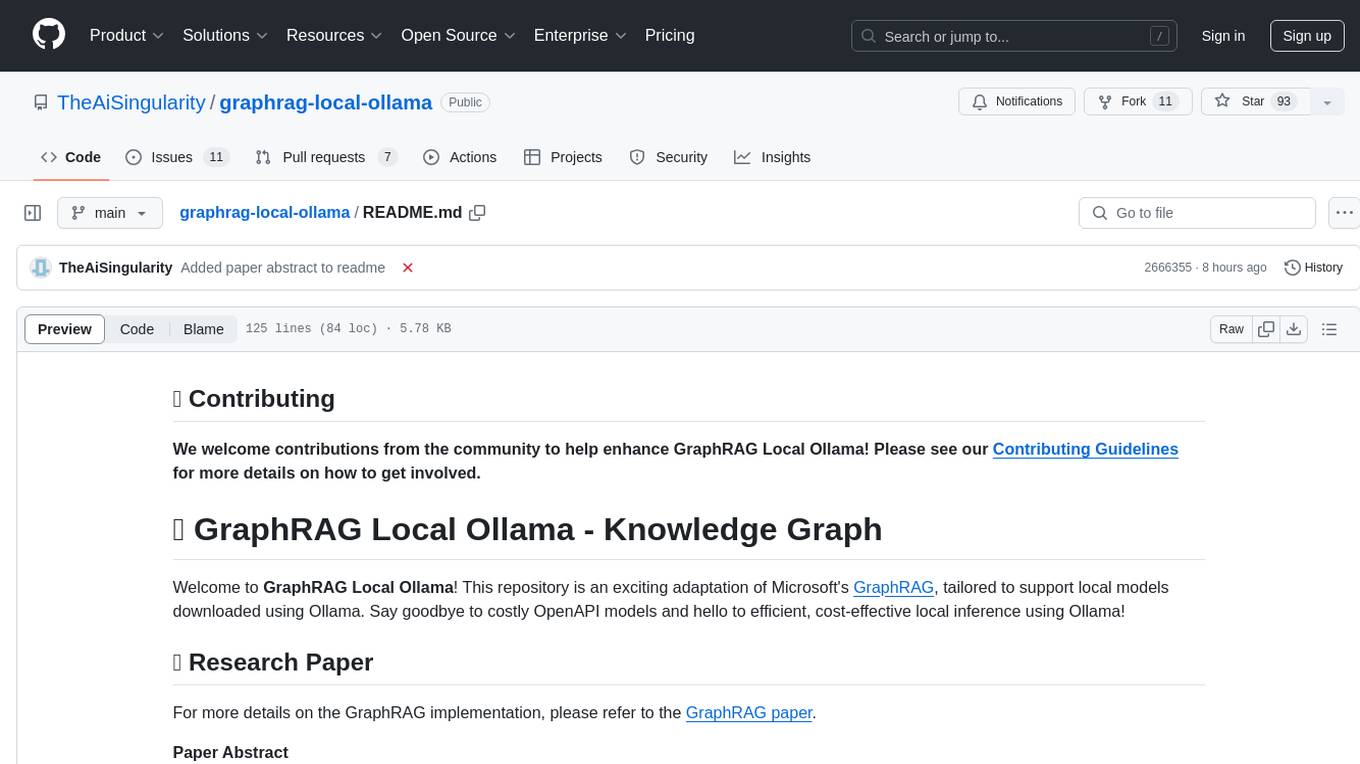
graphrag-local-ollama
GraphRAG Local Ollama is a repository that offers an adaptation of Microsoft's GraphRAG, customized to support local models downloaded using Ollama. It enables users to leverage local models with Ollama for large language models (LLMs) and embeddings, eliminating the need for costly OpenAPI models. The repository provides a simple setup process and allows users to perform question answering over private text corpora by building a graph-based text index and generating community summaries for closely-related entities. GraphRAG Local Ollama aims to improve the comprehensiveness and diversity of generated answers for global sensemaking questions over datasets.
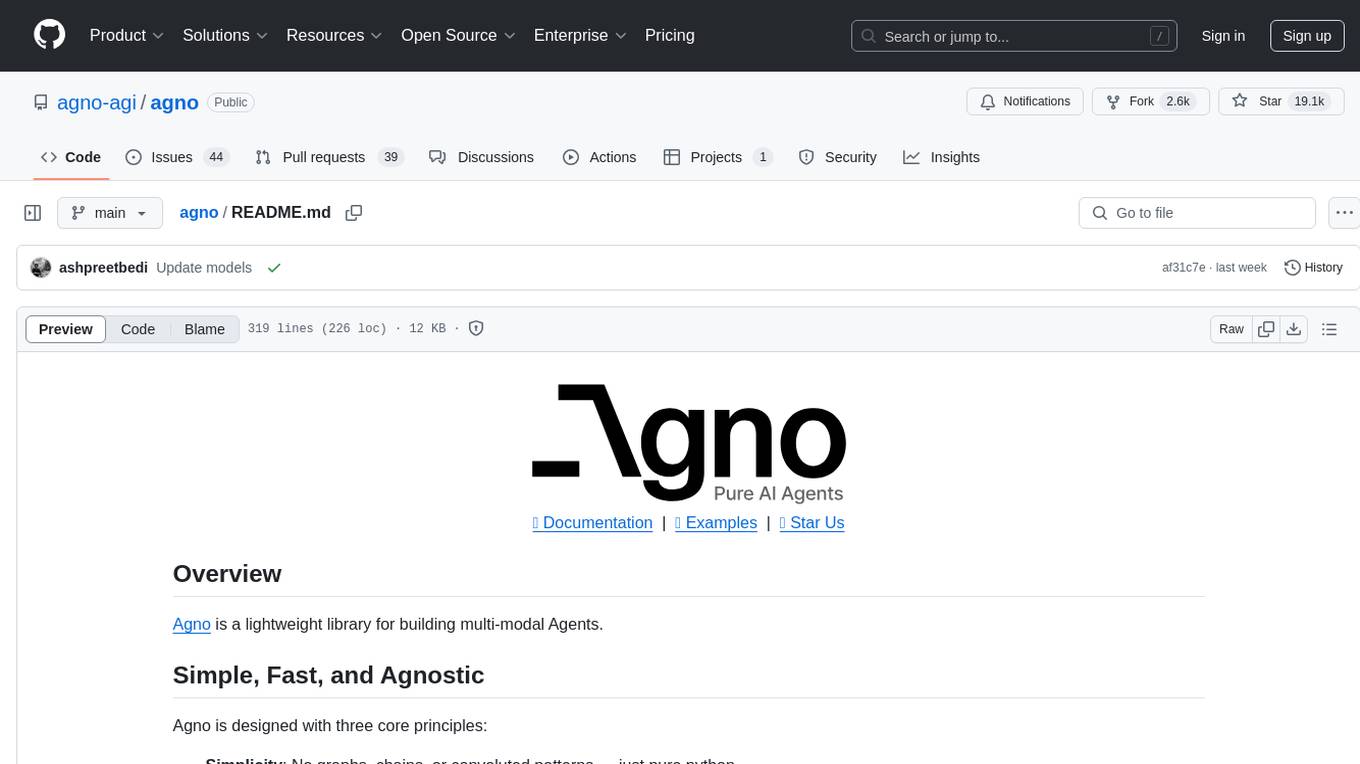
agno
Agno is a lightweight library for building multi-modal Agents. It is designed with core principles of simplicity, uncompromising performance, and agnosticism, allowing users to create blazing fast agents with minimal memory footprint. Agno supports any model, any provider, and any modality, making it a versatile container for AGI. Users can build agents with lightning-fast agent creation, model agnostic capabilities, native support for text, image, audio, and video inputs and outputs, memory management, knowledge stores, structured outputs, and real-time monitoring. The library enables users to create autonomous programs that use language models to solve problems, improve responses, and achieve tasks with varying levels of agency and autonomy.
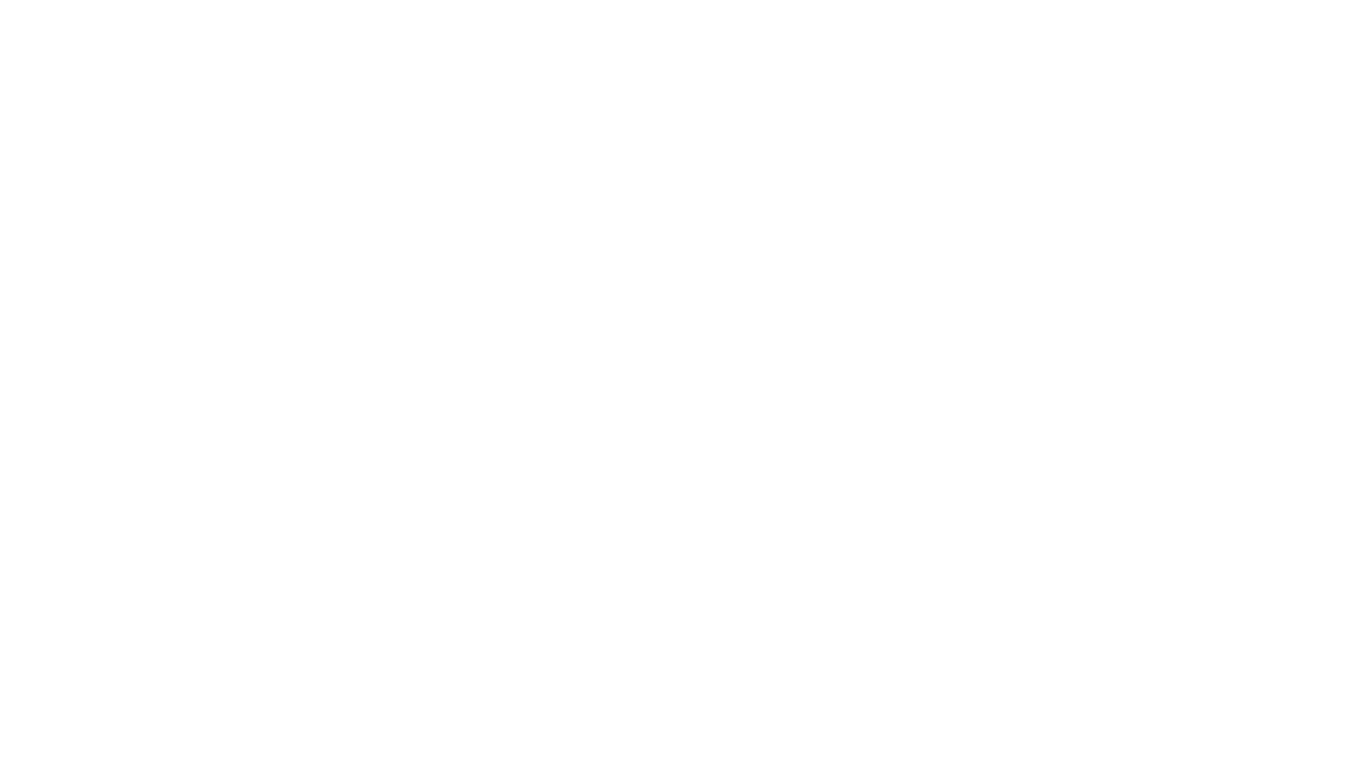
project_alice
Alice is an agentic workflow framework that integrates task execution and intelligent chat capabilities. It provides a flexible environment for creating, managing, and deploying AI agents for various purposes, leveraging a microservices architecture with MongoDB for data persistence. The framework consists of components like APIs, agents, tasks, and chats that interact to produce outputs through files, messages, task results, and URL references. Users can create, test, and deploy agentic solutions in a human-language framework, making it easy to engage with by both users and agents. The tool offers an open-source option, user management, flexible model deployment, and programmatic access to tasks and chats.
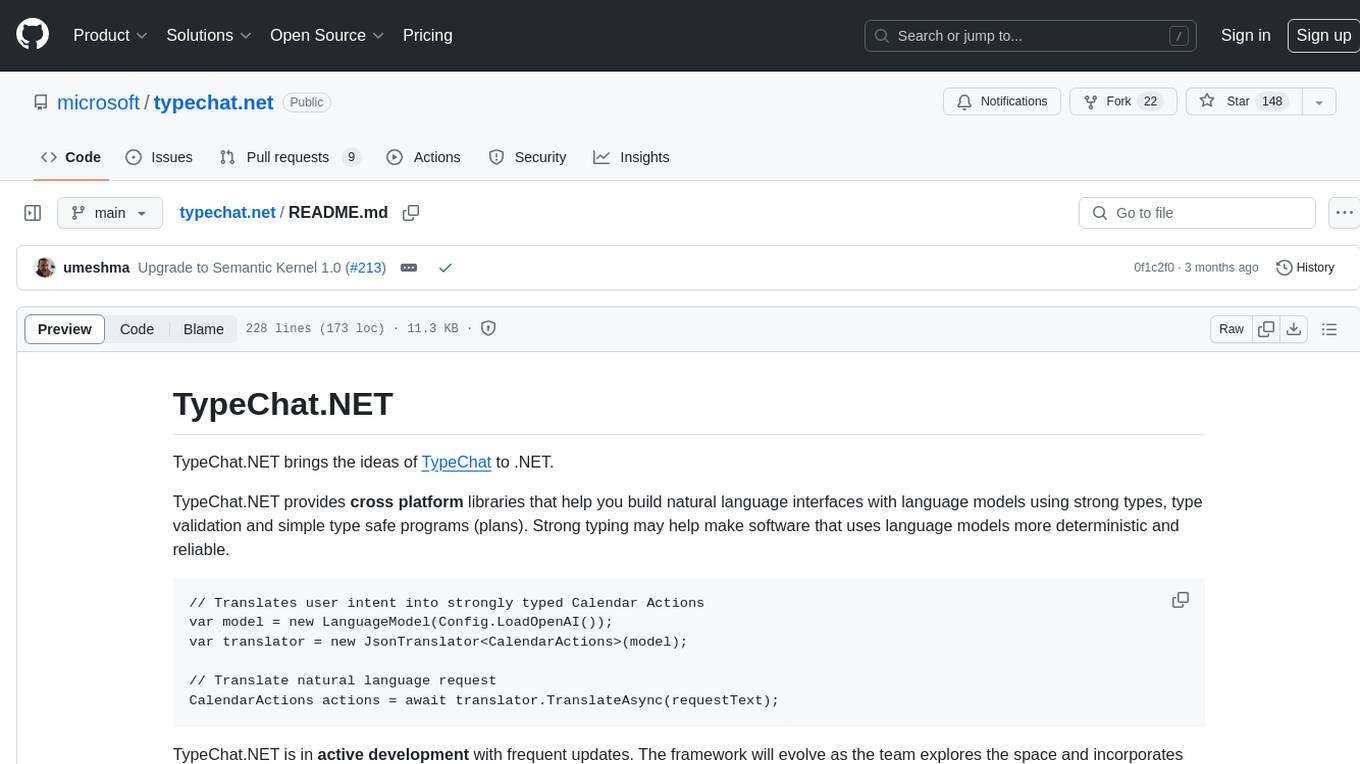
typechat.net
TypeChat.NET is a framework that provides cross-platform libraries for building natural language interfaces with language models using strong types, type validation, and simple type-safe programs. It translates user intent into strongly typed objects and JSON programs, with support for schema export, extensibility, and common scenarios. The framework is actively developed with frequent updates, evolving based on exploration and feedback. It consists of assemblies for translating user intent, synthesizing JSON programs, and integrating with Microsoft Semantic Kernel. TypeChat.NET requires familiarity with and access to OpenAI language models for its examples and scenarios.
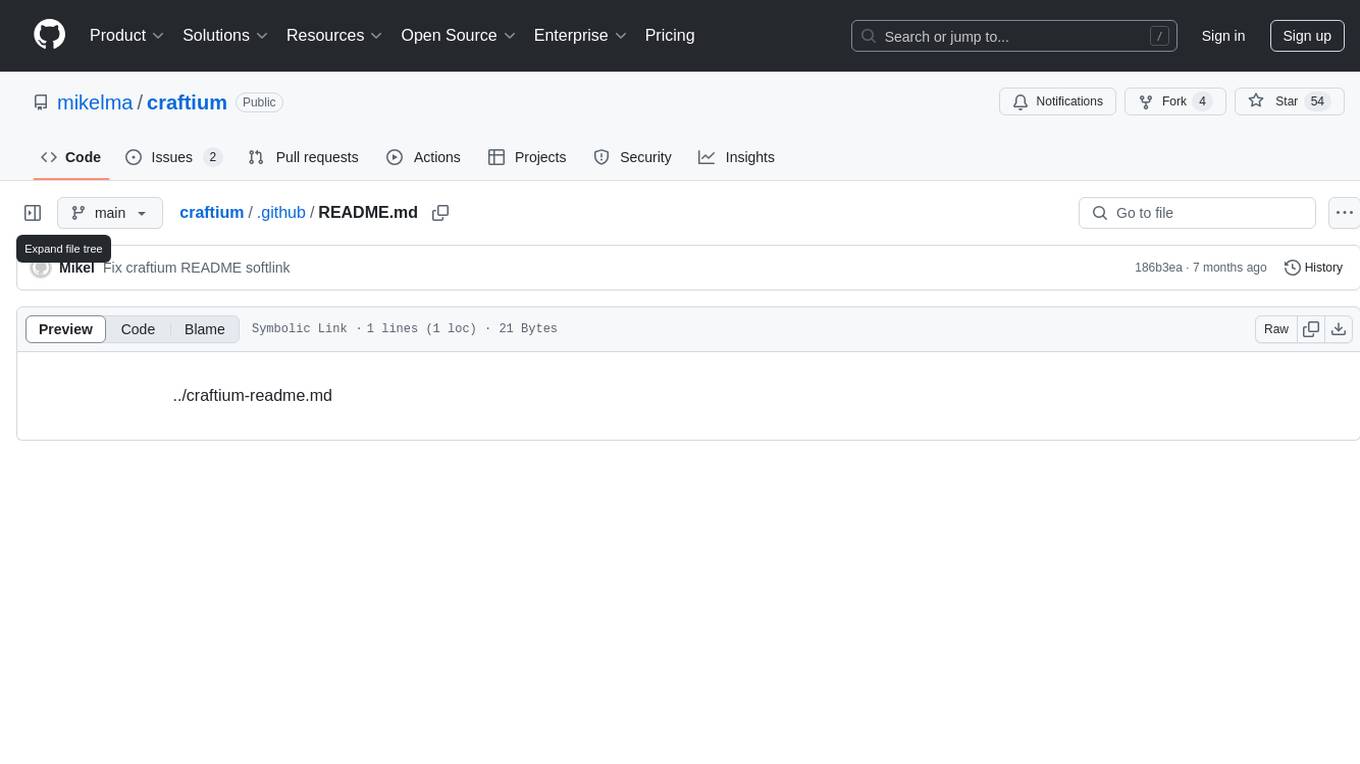
craftium
Craftium is an open-source platform based on the Minetest voxel game engine and the Gymnasium and PettingZoo APIs, designed for creating fast, rich, and diverse single and multi-agent environments. It allows for connecting to Craftium's Python process, executing actions as keyboard and mouse controls, extending the Lua API for creating RL environments and tasks, and supporting client/server synchronization for slow agents. Craftium is fully extensible, extensively documented, modern RL API compatible, fully open source, and eliminates the need for Java. It offers a variety of environments for research and development in reinforcement learning.
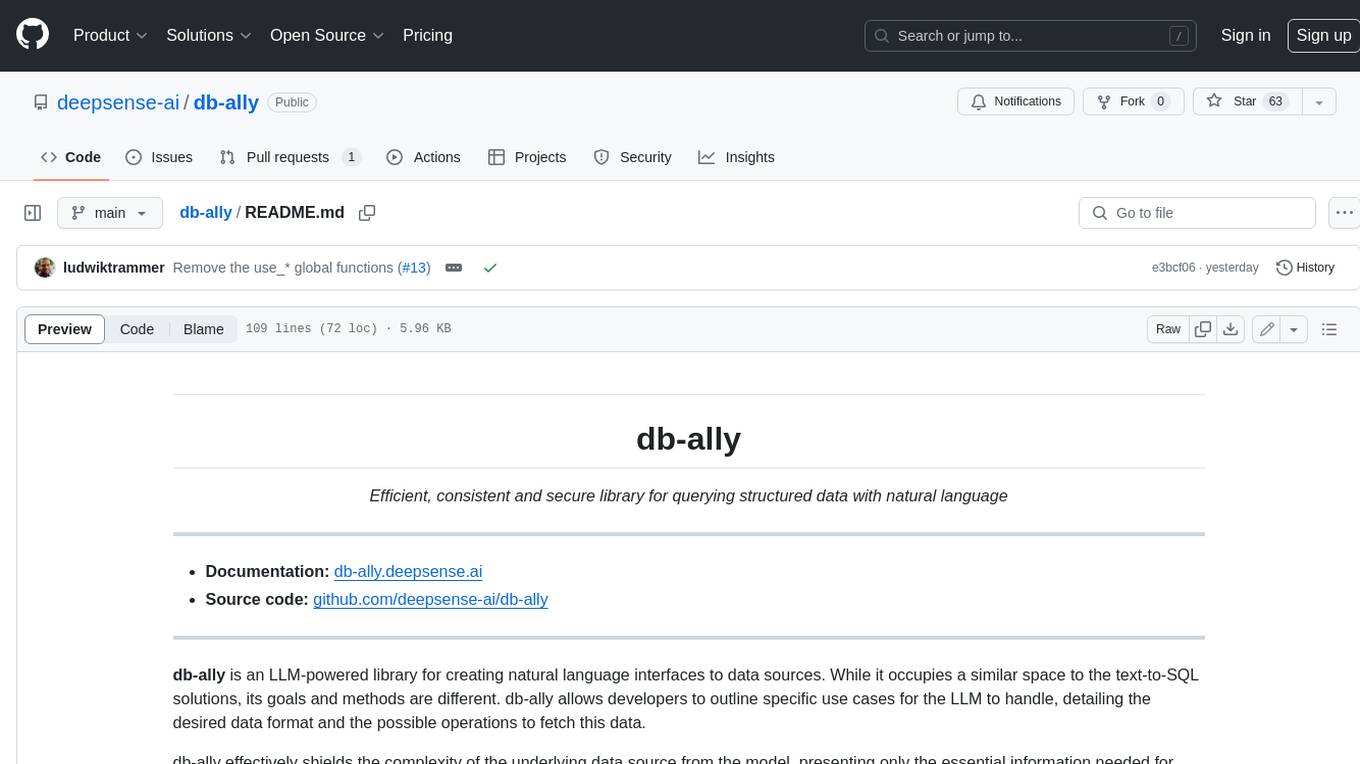
db-ally
db-ally is a library for creating natural language interfaces to data sources. It allows developers to outline specific use cases for a large language model (LLM) to handle, detailing the desired data format and the possible operations to fetch this data. db-ally effectively shields the complexity of the underlying data source from the model, presenting only the essential information needed for solving the specific use cases. Instead of generating arbitrary SQL, the model is asked to generate responses in a simplified query language.
For similar tasks
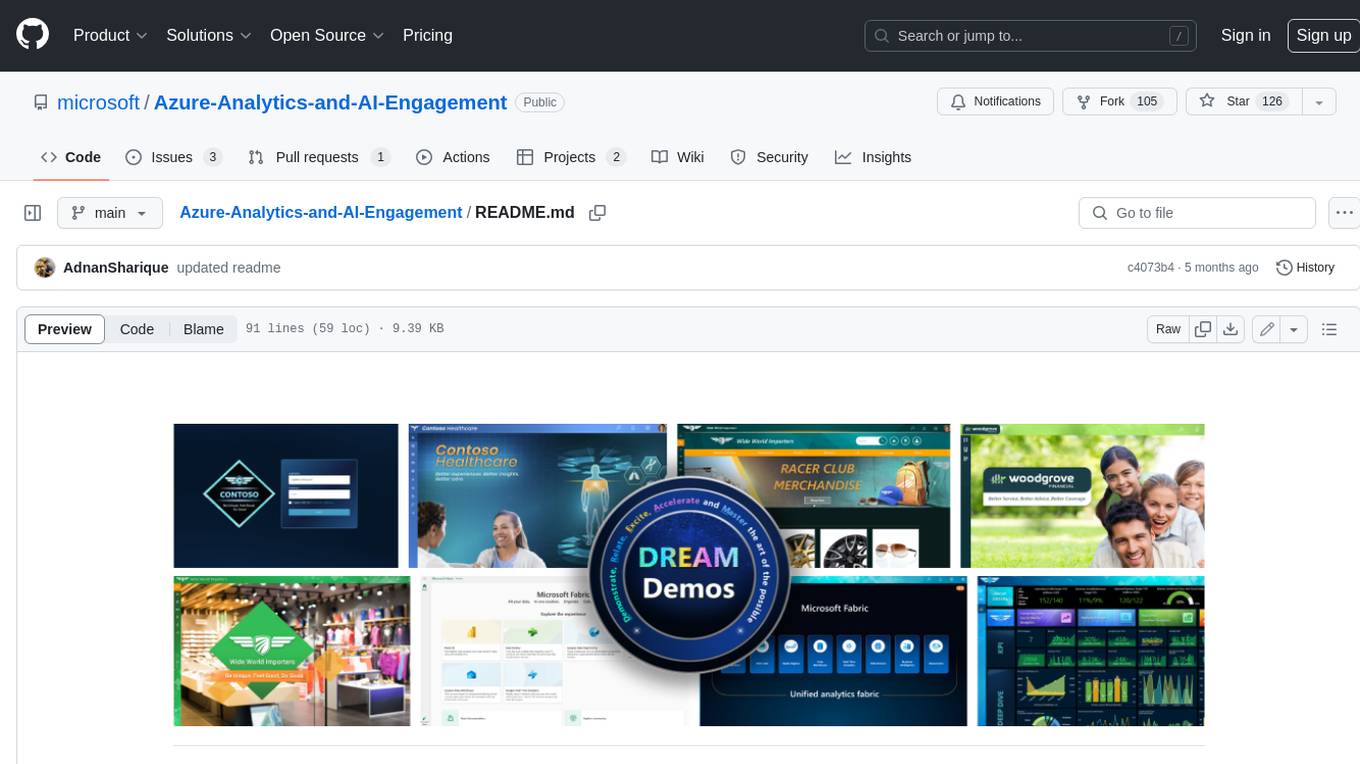
Azure-Analytics-and-AI-Engagement
The Azure-Analytics-and-AI-Engagement repository provides packaged Industry Scenario DREAM Demos with ARM templates (Containing a demo web application, Power BI reports, Synapse resources, AML Notebooks etc.) that can be deployed in a customer’s subscription using the CAPE tool within a matter of few hours. Partners can also deploy DREAM Demos in their own subscriptions using DPoC.
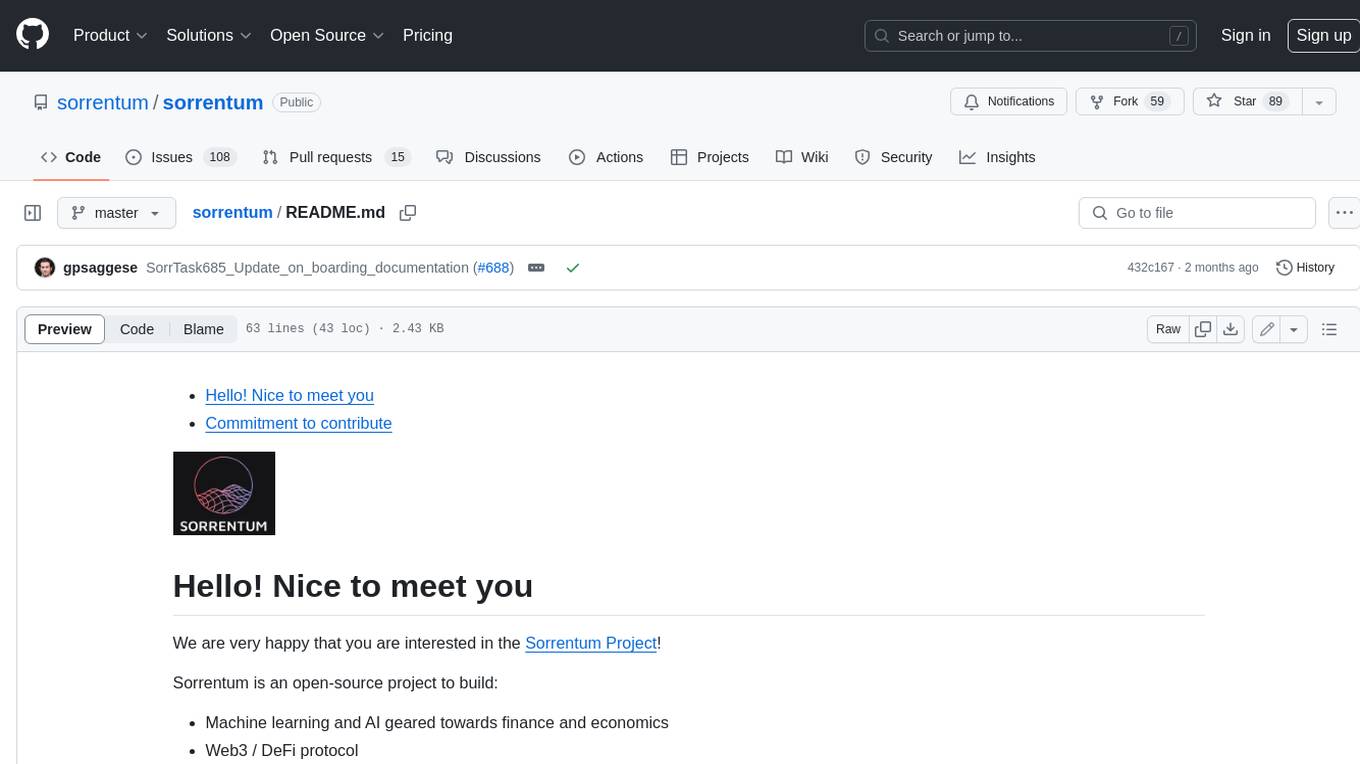
sorrentum
Sorrentum is an open-source project that aims to combine open-source development, startups, and brilliant students to build machine learning, AI, and Web3 / DeFi protocols geared towards finance and economics. The project provides opportunities for internships, research assistantships, and development grants, as well as the chance to work on cutting-edge problems, learn about startups, write academic papers, and get internships and full-time positions at companies working on Sorrentum applications.
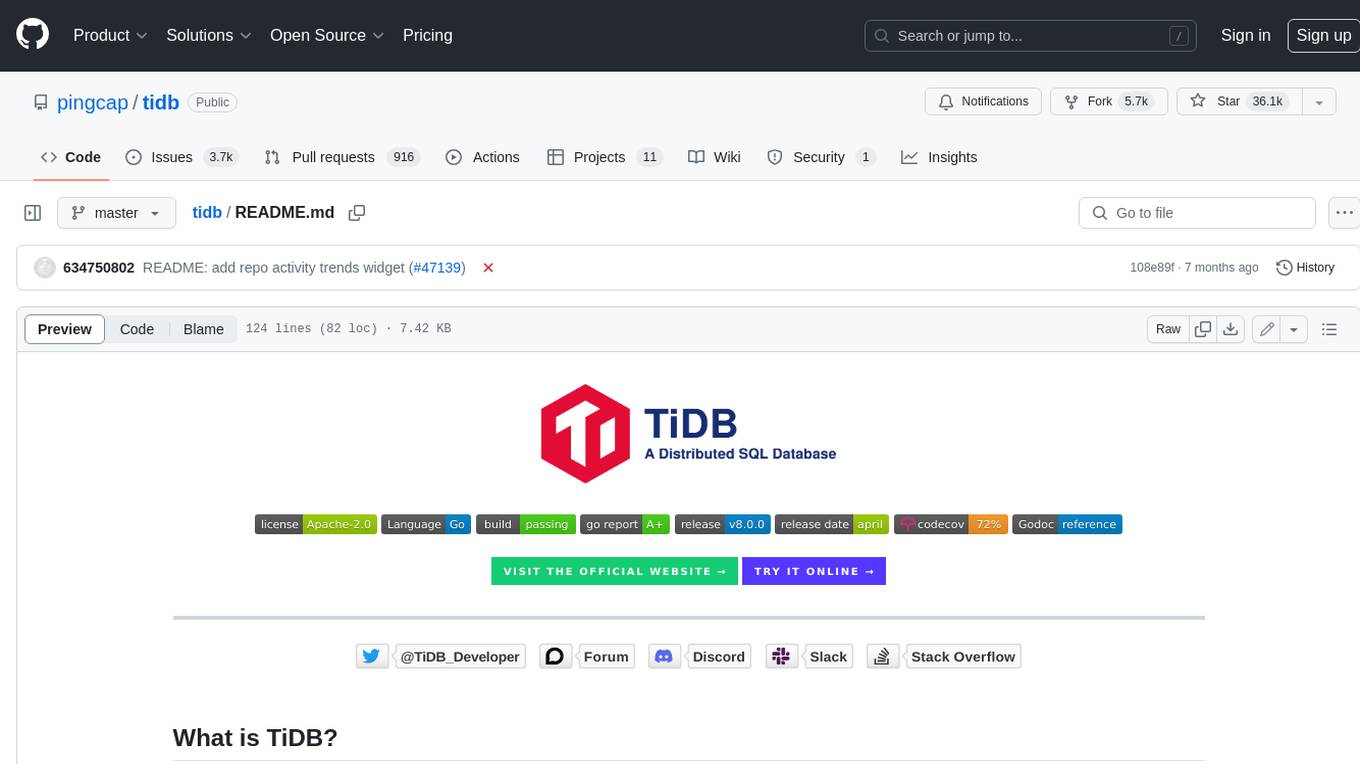
tidb
TiDB is an open-source distributed SQL database that supports Hybrid Transactional and Analytical Processing (HTAP) workloads. It is MySQL compatible and features horizontal scalability, strong consistency, and high availability.
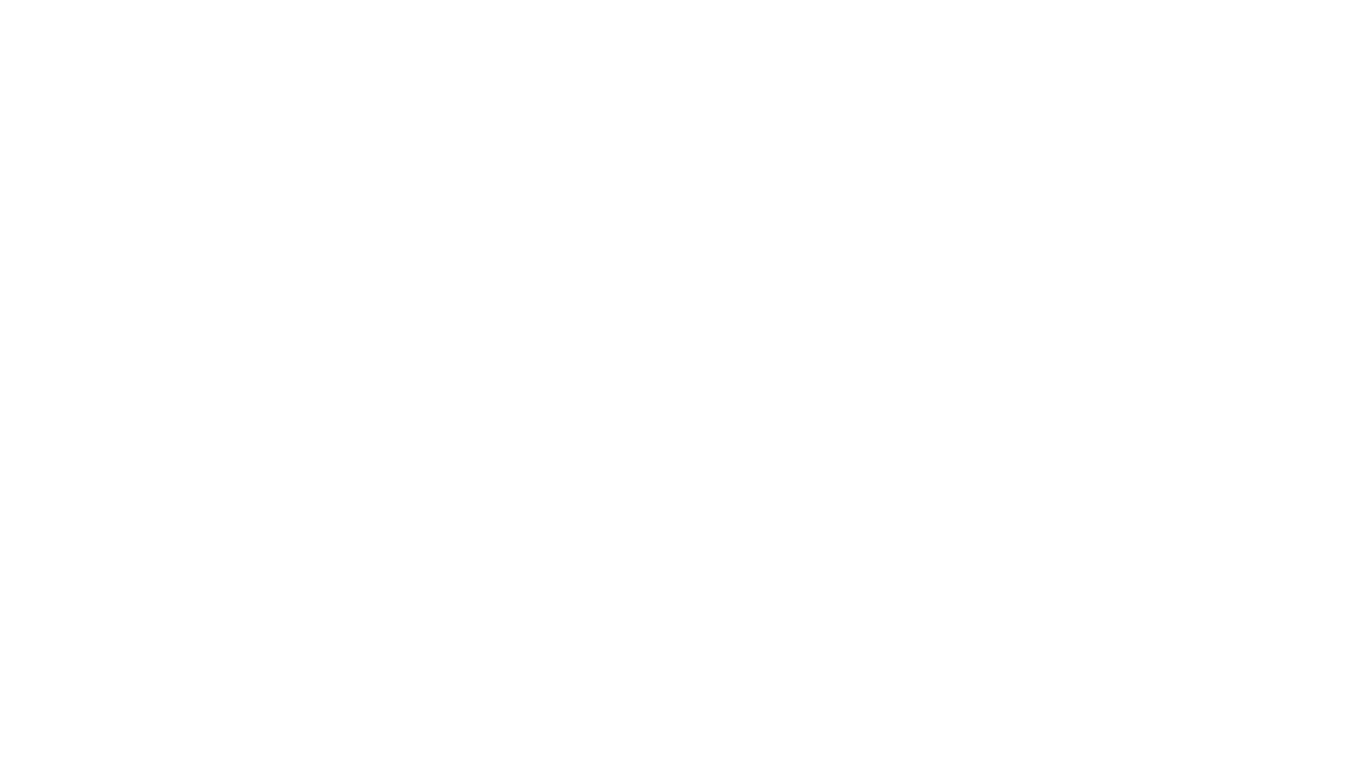
zep-python
Zep is an open-source platform for building and deploying large language model (LLM) applications. It provides a suite of tools and services that make it easy to integrate LLMs into your applications, including chat history memory, embedding, vector search, and data enrichment. Zep is designed to be scalable, reliable, and easy to use, making it a great choice for developers who want to build LLM-powered applications quickly and easily.
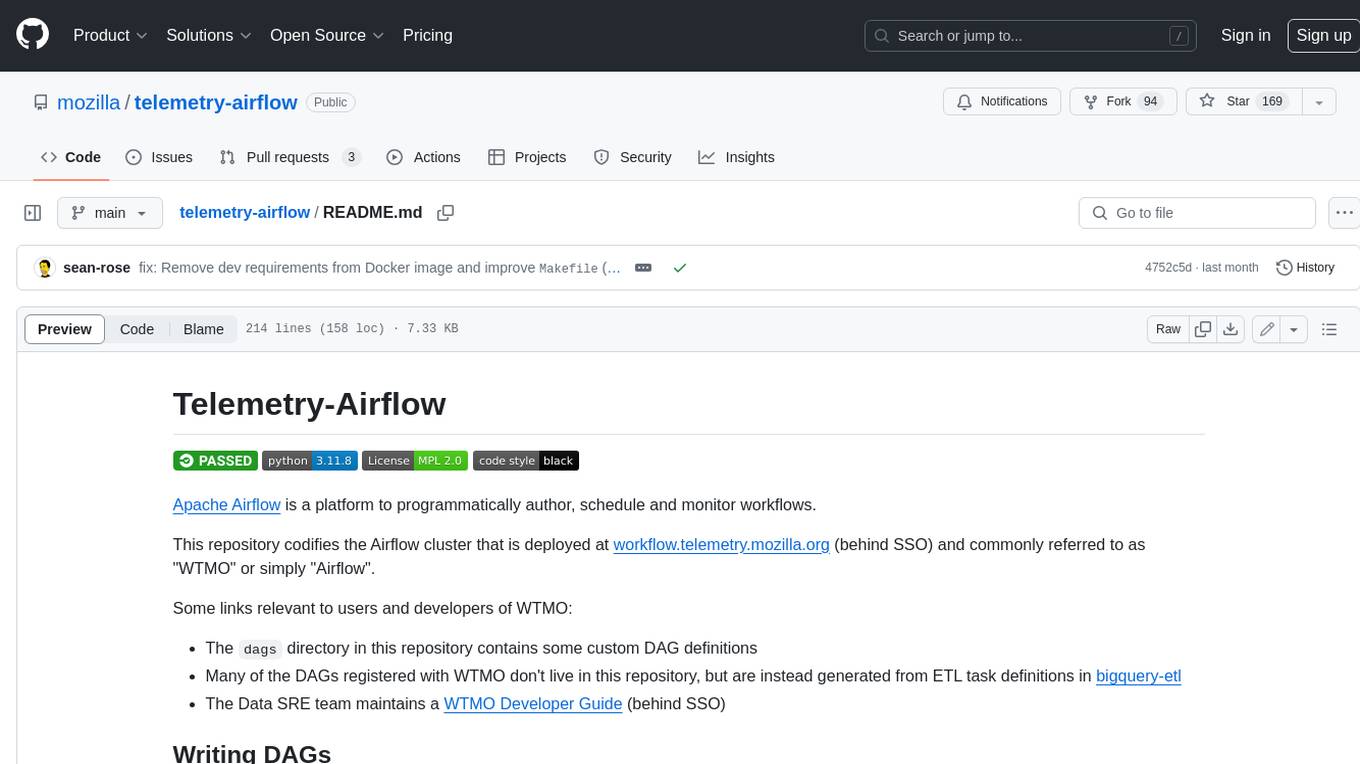
telemetry-airflow
This repository codifies the Airflow cluster that is deployed at workflow.telemetry.mozilla.org (behind SSO) and commonly referred to as "WTMO" or simply "Airflow". Some links relevant to users and developers of WTMO: * The `dags` directory in this repository contains some custom DAG definitions * Many of the DAGs registered with WTMO don't live in this repository, but are instead generated from ETL task definitions in bigquery-etl * The Data SRE team maintains a WTMO Developer Guide (behind SSO)
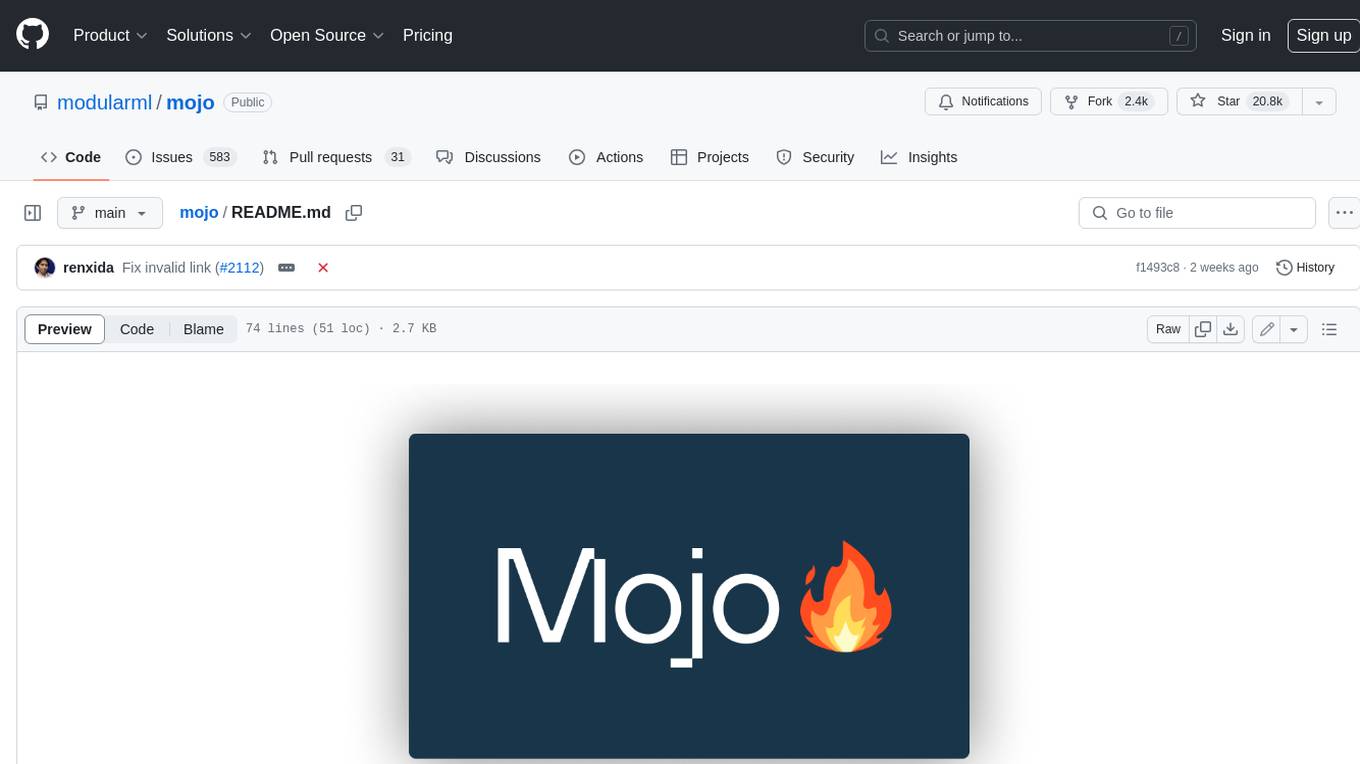
mojo
Mojo is a new programming language that bridges the gap between research and production by combining Python syntax and ecosystem with systems programming and metaprogramming features. Mojo is still young, but it is designed to become a superset of Python over time.
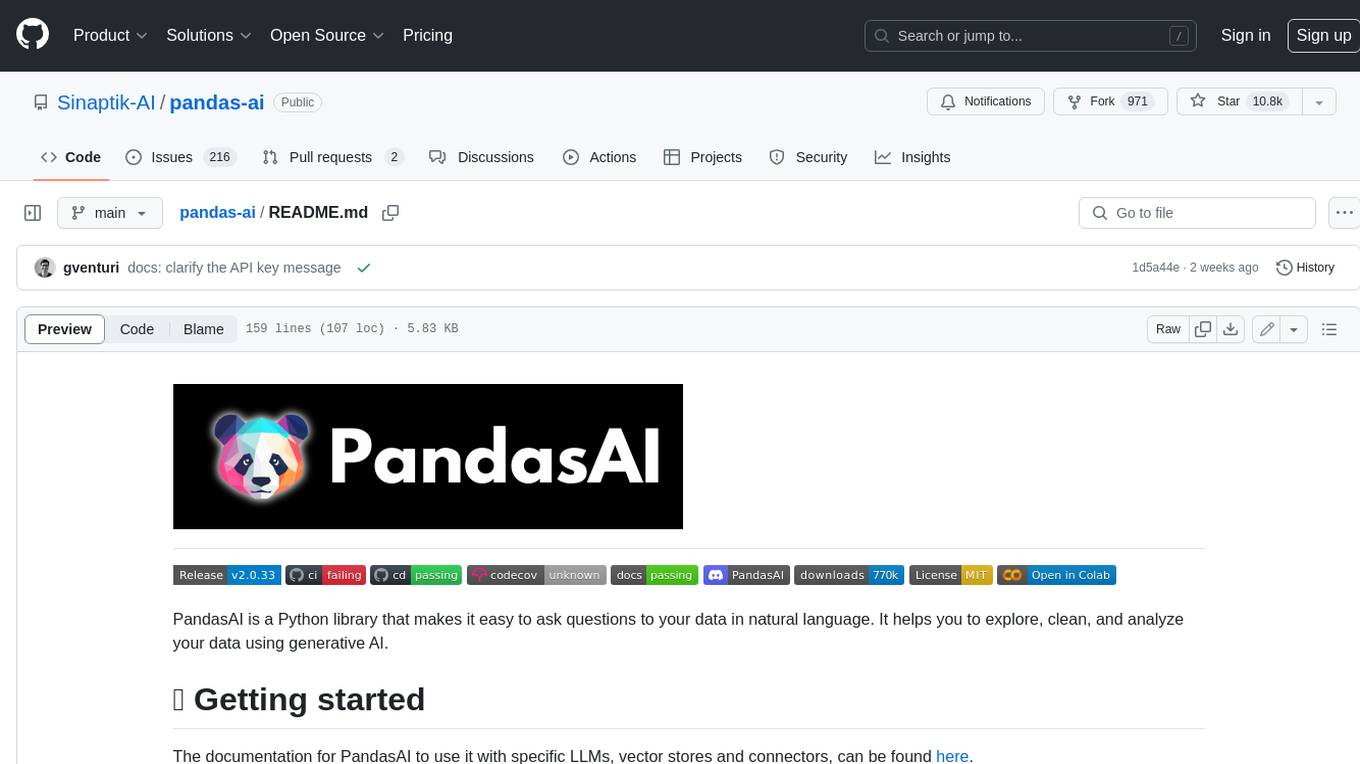
pandas-ai
PandasAI is a Python library that makes it easy to ask questions to your data in natural language. It helps you to explore, clean, and analyze your data using generative AI.
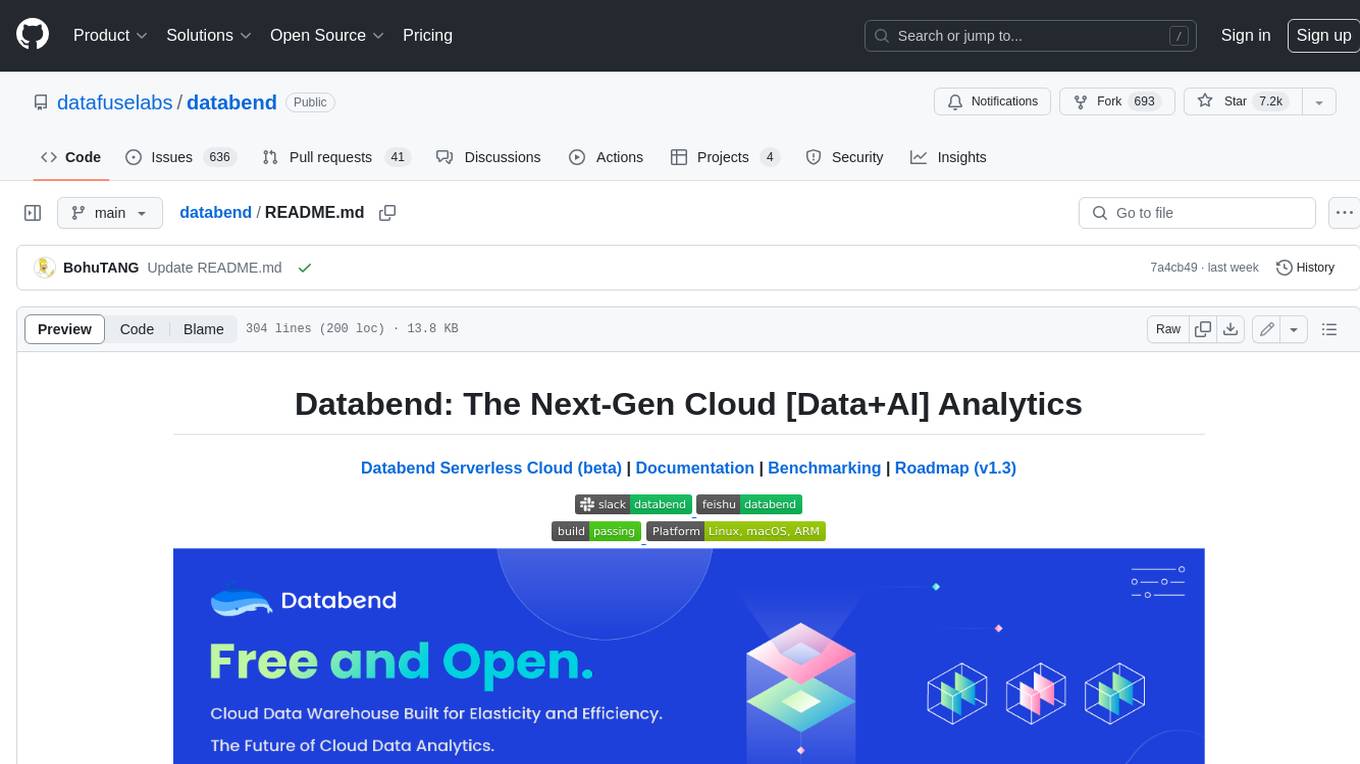
databend
Databend is an open-source cloud data warehouse that serves as a cost-effective alternative to Snowflake. With its focus on fast query execution and data ingestion, it's designed for complex analysis of the world's largest datasets.
For similar jobs
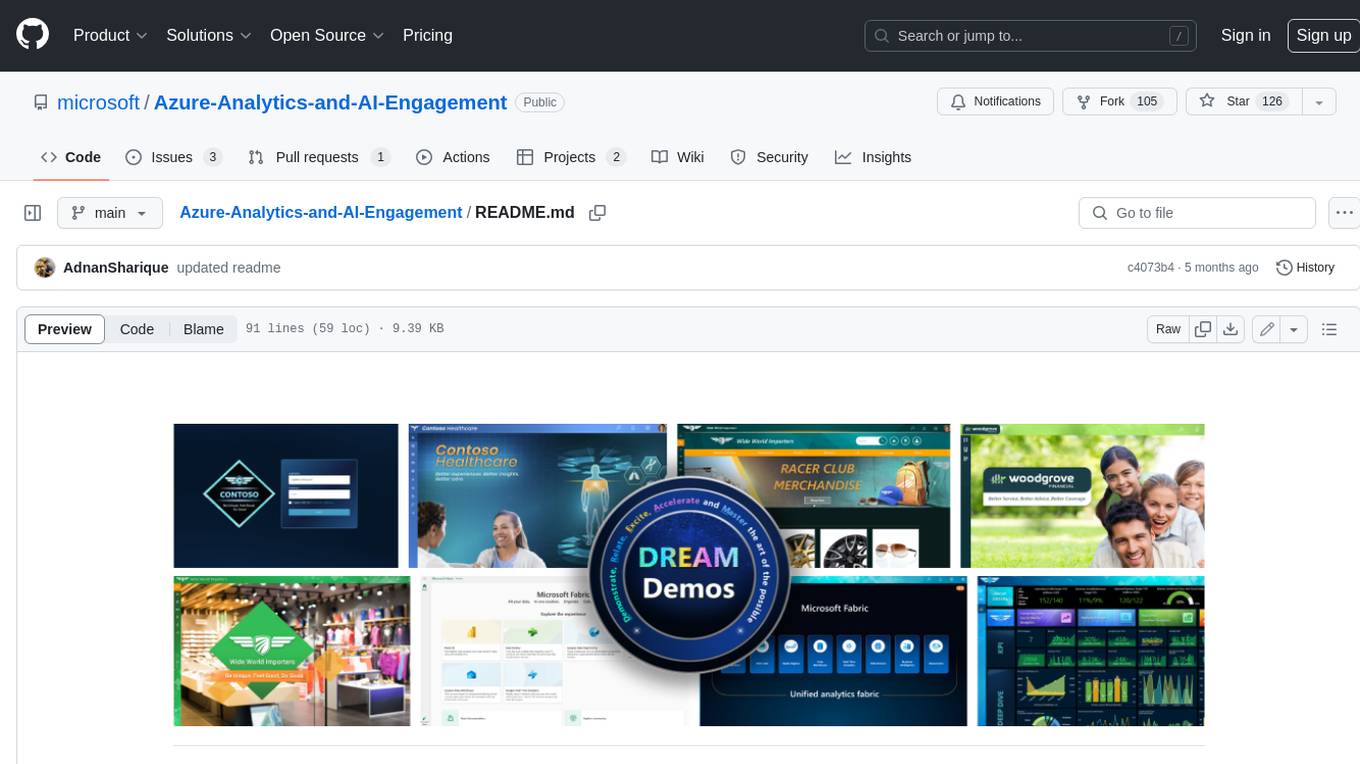
Azure-Analytics-and-AI-Engagement
The Azure-Analytics-and-AI-Engagement repository provides packaged Industry Scenario DREAM Demos with ARM templates (Containing a demo web application, Power BI reports, Synapse resources, AML Notebooks etc.) that can be deployed in a customer’s subscription using the CAPE tool within a matter of few hours. Partners can also deploy DREAM Demos in their own subscriptions using DPoC.
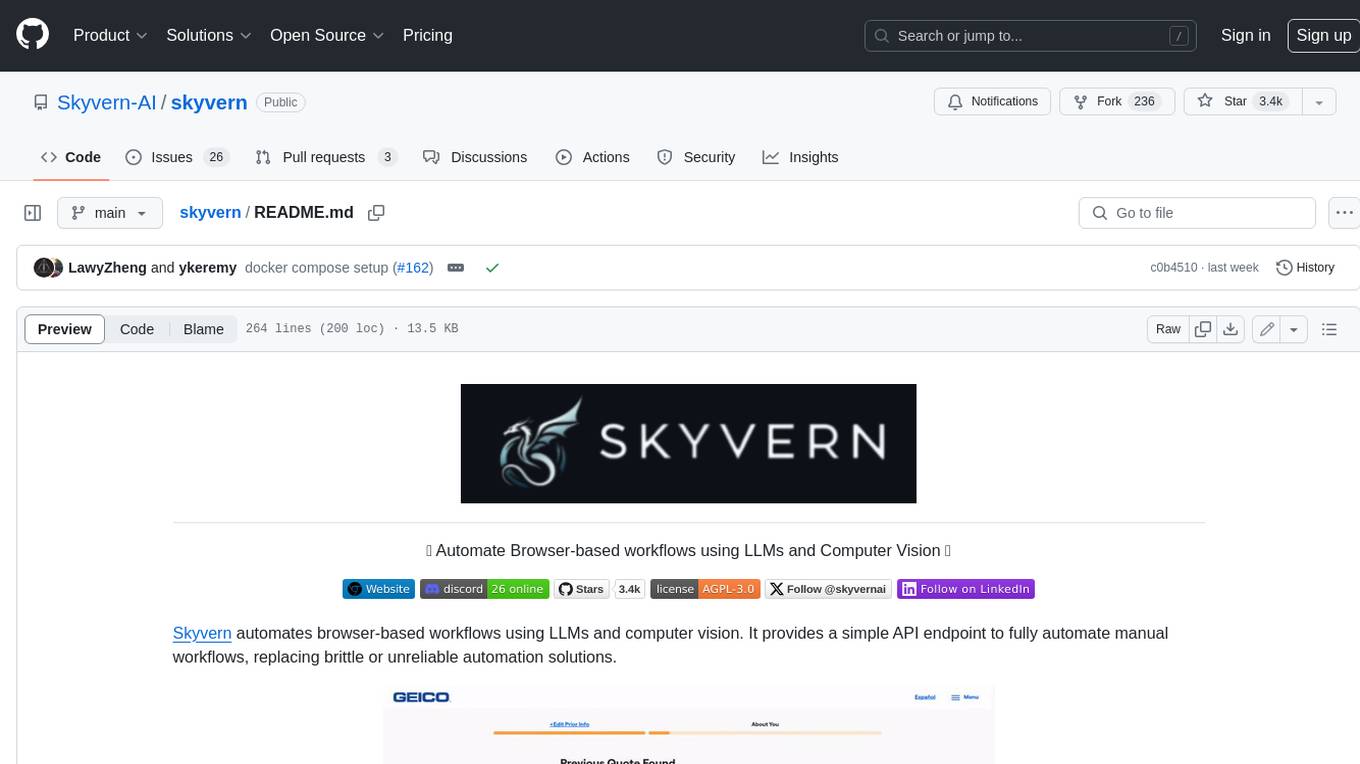
skyvern
Skyvern automates browser-based workflows using LLMs and computer vision. It provides a simple API endpoint to fully automate manual workflows, replacing brittle or unreliable automation solutions. Traditional approaches to browser automations required writing custom scripts for websites, often relying on DOM parsing and XPath-based interactions which would break whenever the website layouts changed. Instead of only relying on code-defined XPath interactions, Skyvern adds computer vision and LLMs to the mix to parse items in the viewport in real-time, create a plan for interaction and interact with them. This approach gives us a few advantages: 1. Skyvern can operate on websites it’s never seen before, as it’s able to map visual elements to actions necessary to complete a workflow, without any customized code 2. Skyvern is resistant to website layout changes, as there are no pre-determined XPaths or other selectors our system is looking for while trying to navigate 3. Skyvern leverages LLMs to reason through interactions to ensure we can cover complex situations. Examples include: 1. If you wanted to get an auto insurance quote from Geico, the answer to a common question “Were you eligible to drive at 18?” could be inferred from the driver receiving their license at age 16 2. If you were doing competitor analysis, it’s understanding that an Arnold Palmer 22 oz can at 7/11 is almost definitely the same product as a 23 oz can at Gopuff (even though the sizes are slightly different, which could be a rounding error!) Want to see examples of Skyvern in action? Jump to #real-world-examples-of- skyvern
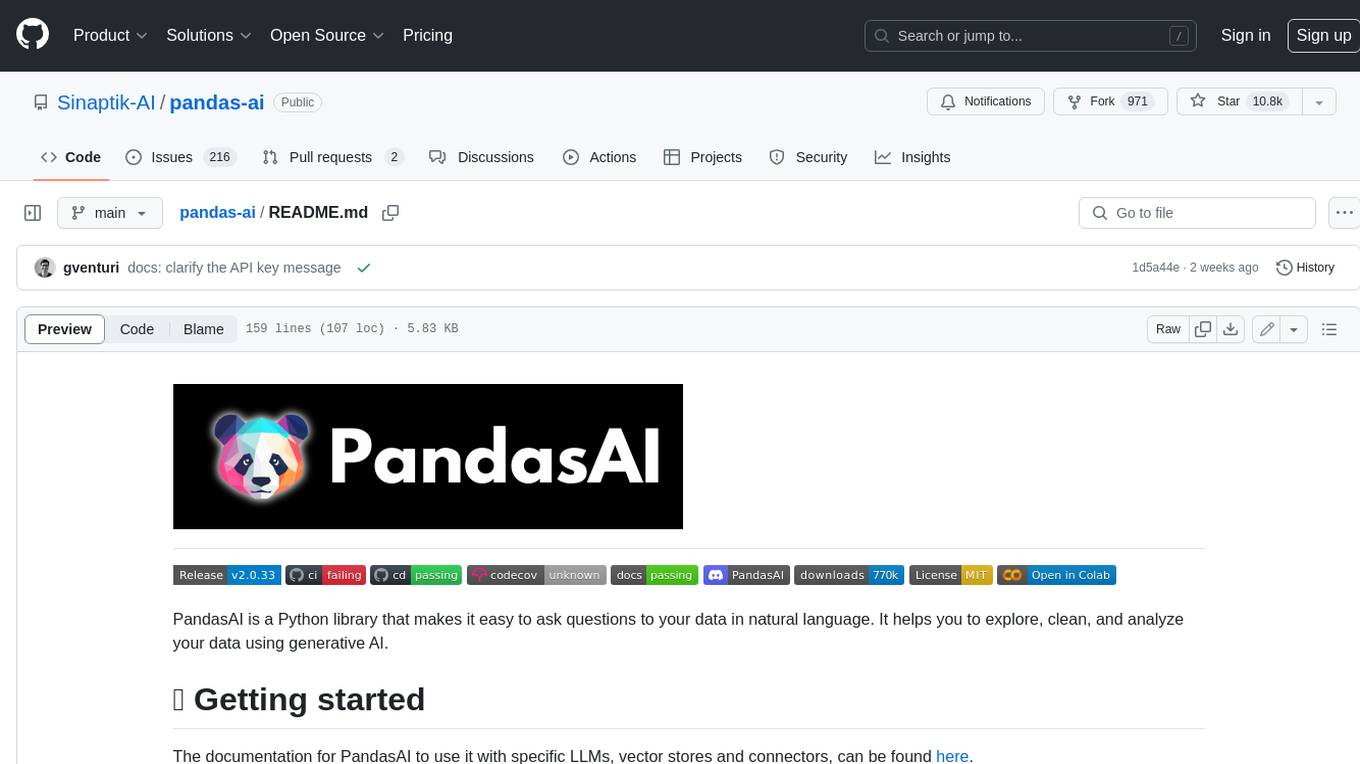
pandas-ai
PandasAI is a Python library that makes it easy to ask questions to your data in natural language. It helps you to explore, clean, and analyze your data using generative AI.
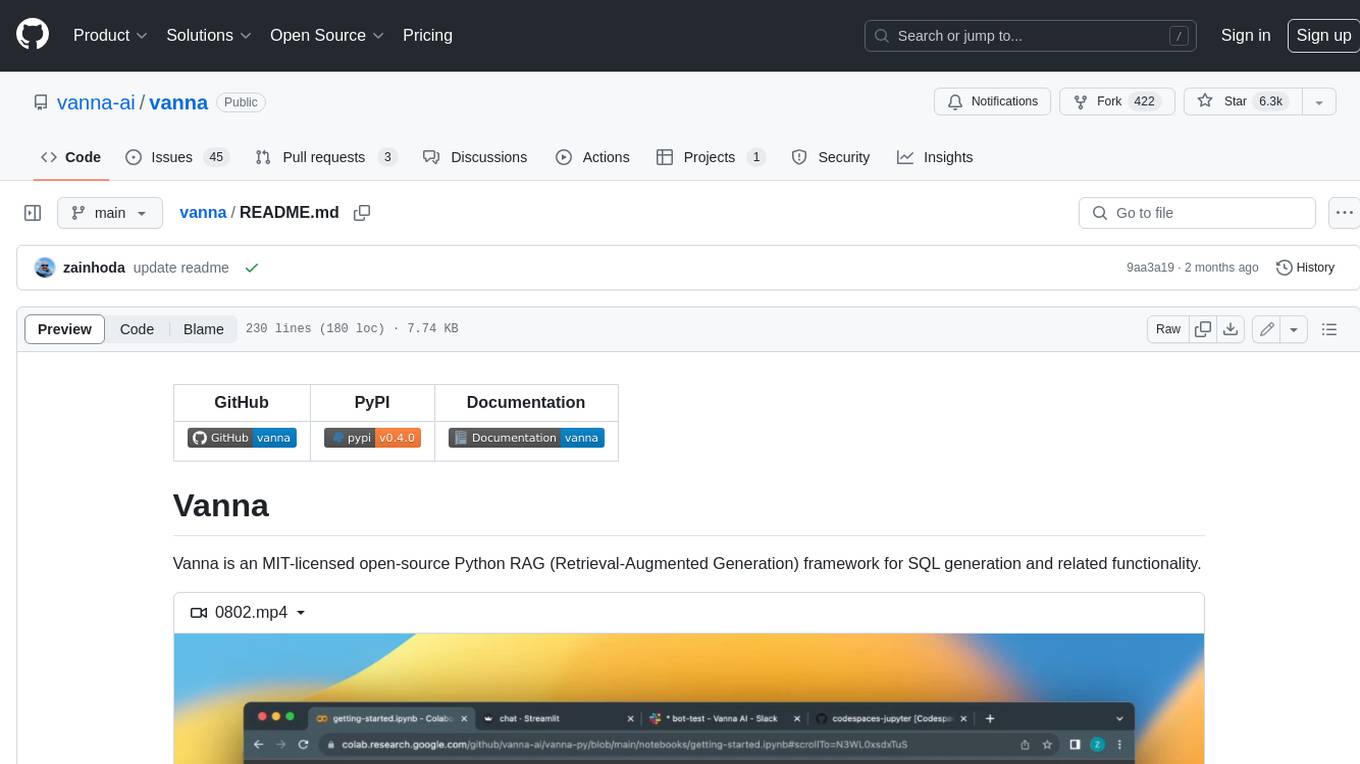
vanna
Vanna is an open-source Python framework for SQL generation and related functionality. It uses Retrieval-Augmented Generation (RAG) to train a model on your data, which can then be used to ask questions and get back SQL queries. Vanna is designed to be portable across different LLMs and vector databases, and it supports any SQL database. It is also secure and private, as your database contents are never sent to the LLM or the vector database.
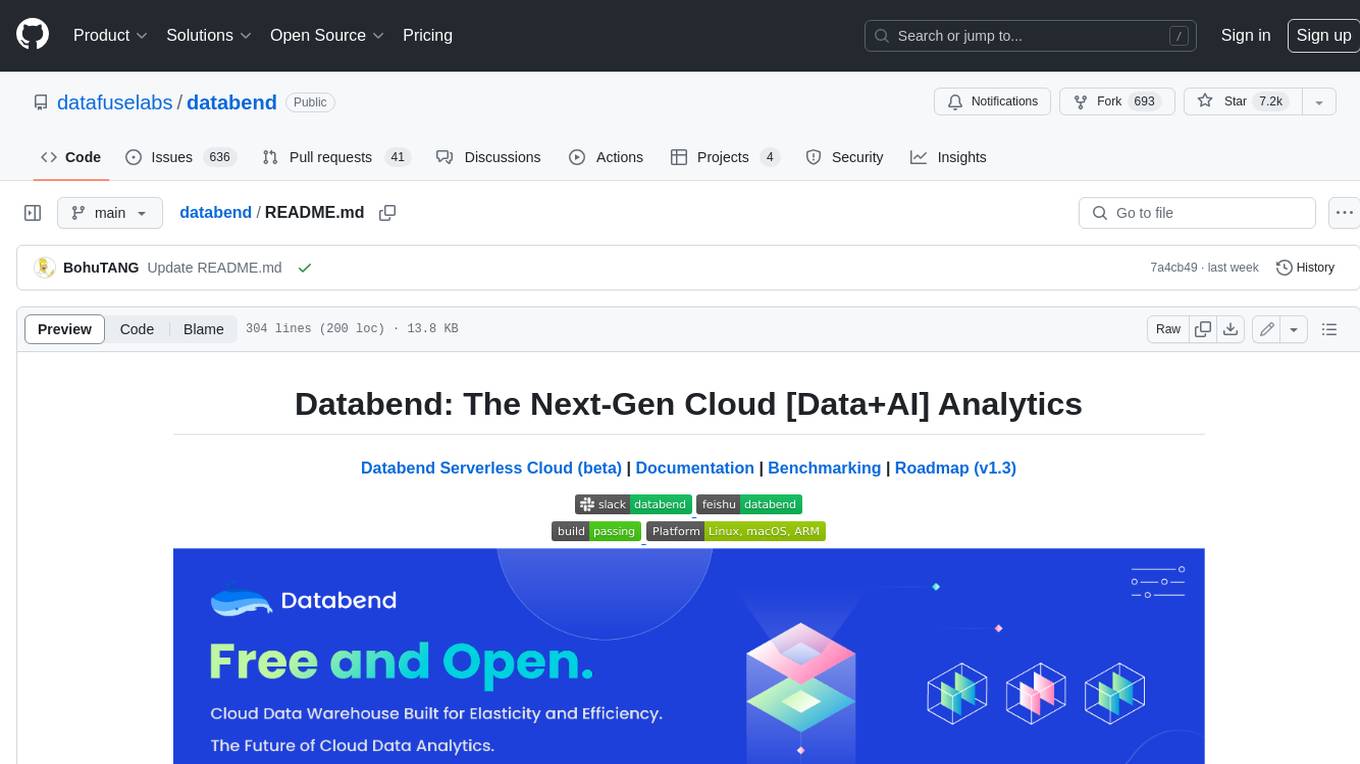
databend
Databend is an open-source cloud data warehouse that serves as a cost-effective alternative to Snowflake. With its focus on fast query execution and data ingestion, it's designed for complex analysis of the world's largest datasets.
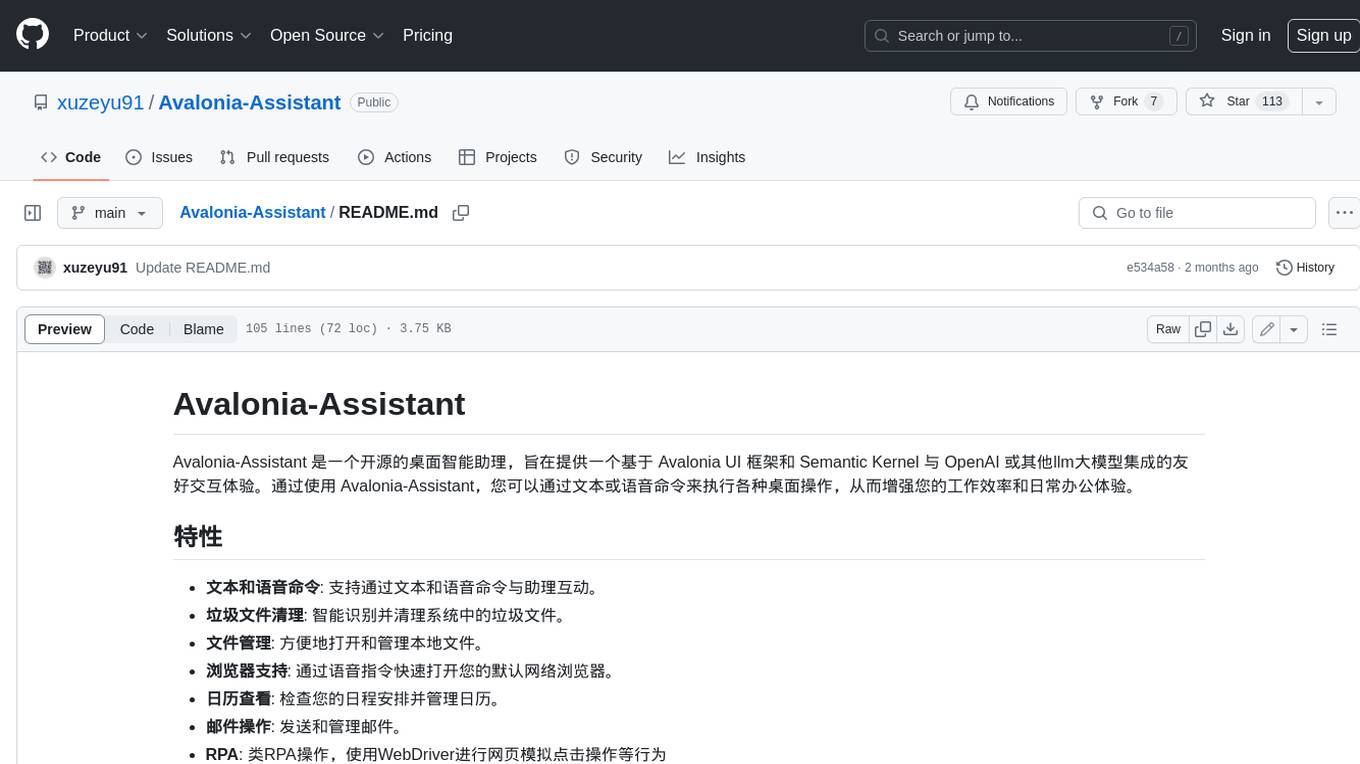
Avalonia-Assistant
Avalonia-Assistant is an open-source desktop intelligent assistant that aims to provide a user-friendly interactive experience based on the Avalonia UI framework and the integration of Semantic Kernel with OpenAI or other large LLM models. By utilizing Avalonia-Assistant, you can perform various desktop operations through text or voice commands, enhancing your productivity and daily office experience.
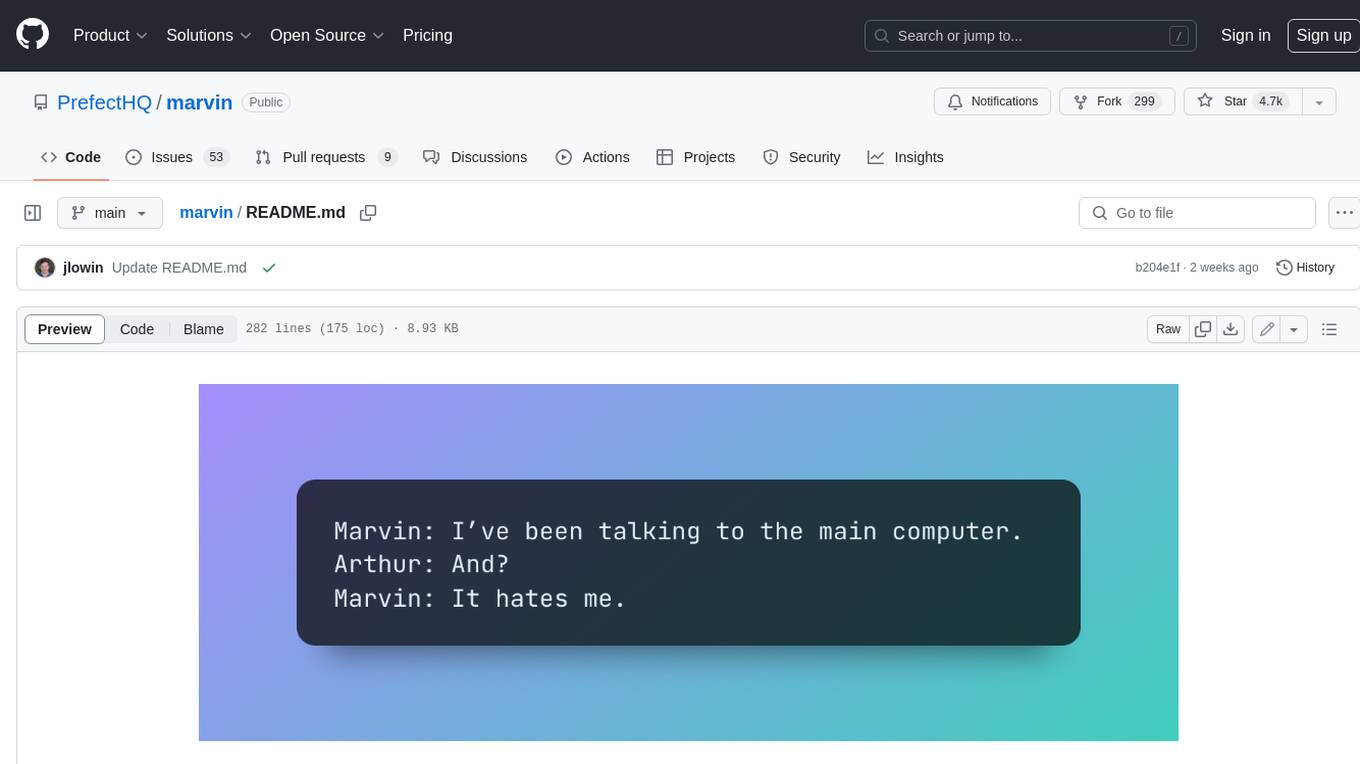
marvin
Marvin is a lightweight AI toolkit for building natural language interfaces that are reliable, scalable, and easy to trust. Each of Marvin's tools is simple and self-documenting, using AI to solve common but complex challenges like entity extraction, classification, and generating synthetic data. Each tool is independent and incrementally adoptable, so you can use them on their own or in combination with any other library. Marvin is also multi-modal, supporting both image and audio generation as well using images as inputs for extraction and classification. Marvin is for developers who care more about _using_ AI than _building_ AI, and we are focused on creating an exceptional developer experience. Marvin users should feel empowered to bring tightly-scoped "AI magic" into any traditional software project with just a few extra lines of code. Marvin aims to merge the best practices for building dependable, observable software with the best practices for building with generative AI into a single, easy-to-use library. It's a serious tool, but we hope you have fun with it. Marvin is open-source, free to use, and made with 💙 by the team at Prefect.
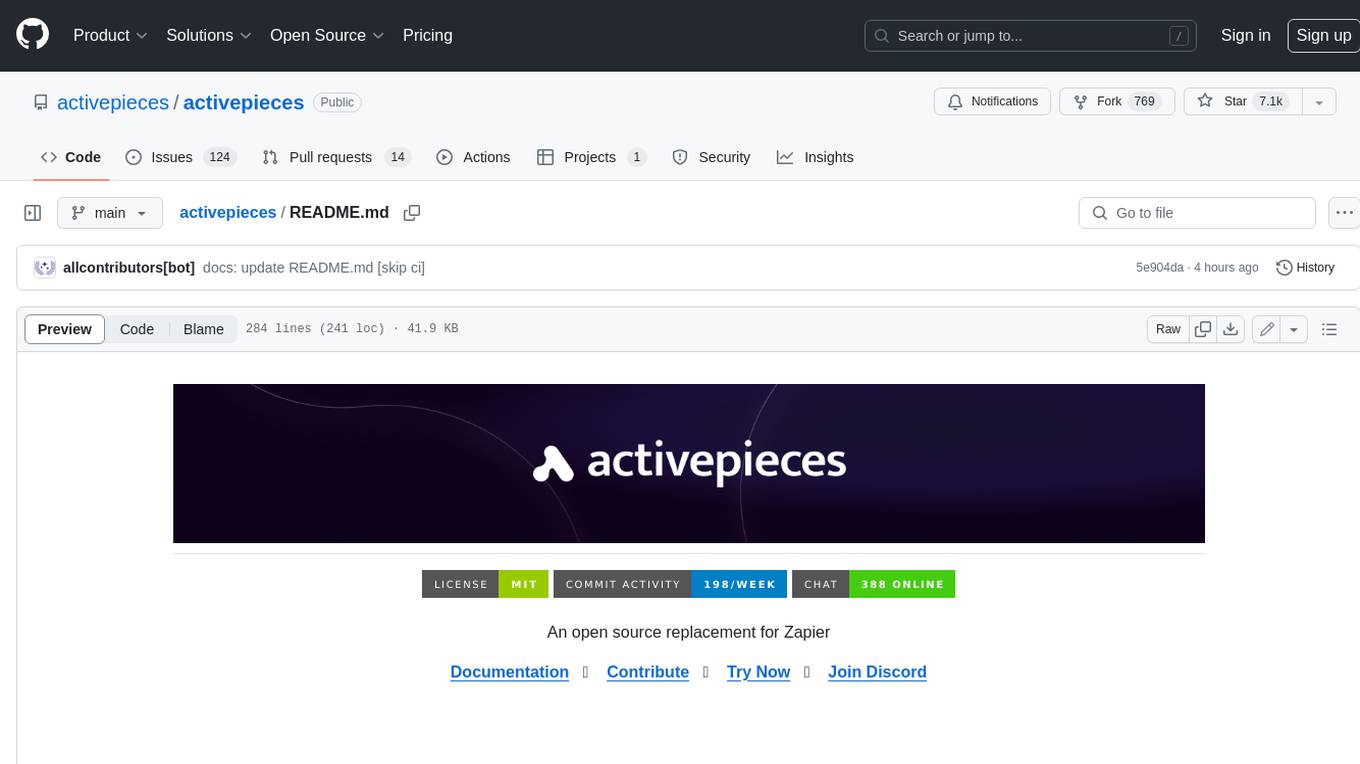
activepieces
Activepieces is an open source replacement for Zapier, designed to be extensible through a type-safe pieces framework written in Typescript. It features a user-friendly Workflow Builder with support for Branches, Loops, and Drag and Drop. Activepieces integrates with Google Sheets, OpenAI, Discord, and RSS, along with 80+ other integrations. The list of supported integrations continues to grow rapidly, thanks to valuable contributions from the community. Activepieces is an open ecosystem; all piece source code is available in the repository, and they are versioned and published directly to npmjs.com upon contributions. If you cannot find a specific piece on the pieces roadmap, please submit a request by visiting the following link: Request Piece Alternatively, if you are a developer, you can quickly build your own piece using our TypeScript framework. For guidance, please refer to the following guide: Contributor's Guide