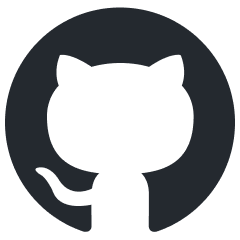
intelligence-toolkit
Interactive workflows for creating AI intelligence reports from real-world data sources
Stars: 66
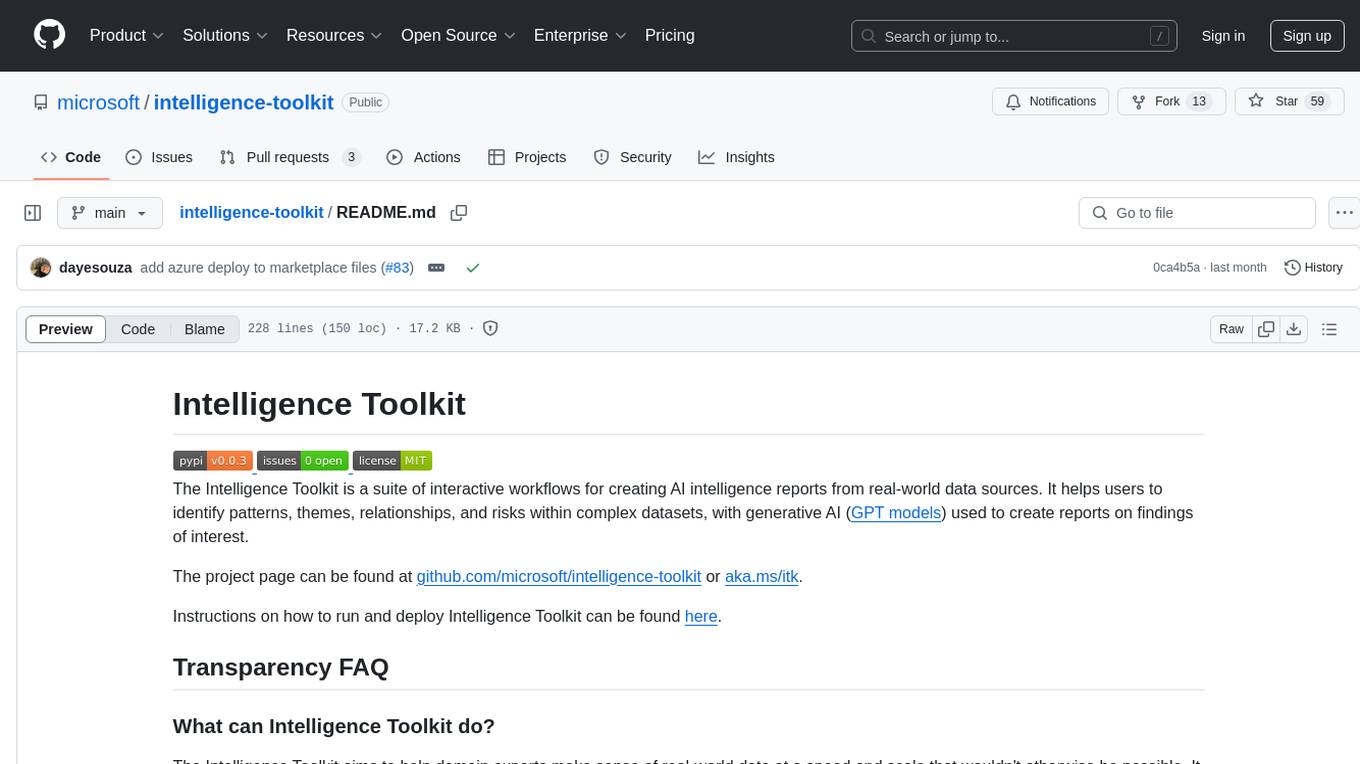
The Intelligence Toolkit is a suite of interactive workflows designed to help domain experts make sense of real-world data by identifying patterns, themes, relationships, and risks within complex datasets. It utilizes generative AI (GPT models) to create reports on findings of interest. The toolkit supports analysis of case, entity, and text data, providing various interactive workflows for different intelligence tasks. Users are expected to evaluate the quality of data insights and AI interpretations before taking action. The system is designed for moderate-sized datasets and responsible use of personal case data. It uses the GPT-4 model from OpenAI or Azure OpenAI APIs for generating reports and insights.
README:
The Intelligence Toolkit is a suite of interactive workflows for creating AI intelligence reports from real-world data sources. It helps users to identify patterns, themes, relationships, and risks within complex datasets, with generative AI (GPT models) used to create reports on findings of interest.
The project page can be found at github.com/microsoft/intelligence-toolkit or aka.ms/itk.
Instructions on how to run and deploy Intelligence Toolkit can be found here.
The Intelligence Toolkit aims to help domain experts make sense of real-world data at a speed and scale that wouldn't otherwise be possible. It was specifically designed for analysis of case, entity, and text data:
-
Case Data
- Units are structured records describing individual people.
- Analysis aims to inform policy while preserving privacy.
-
Entity Data
- Units are structured records describing real-world entities.
- Analysis aims to understand risks represented by relationships.
-
Text Data
- Units are collections or instances of unstructured text documents.
- Analysis aims to retrieve information and summarize themes.
The Intelligence Toolkit is designed to be used by domain experts who are familiar with the data and the intelligence they want to derive from it. Users should be independently capable of evaluating the quality of data insights and AI interpretations before taking action, e.g., sharing intelligence outputs or making decisions informed by these outputs.
It supports a variety of interactive workflows, each designed to address a specific type of intelligence task:
-
Case Intelligence Workflows
-
Anonymize Case Data
generates differentially-private datasets and summaries from sensitive case records. -
Detect Case Patterns
generates reports on patterns of attribute values detected in streams of case records. -
Compare Case Groups
generates reports by defining and comparing groups of case records.
-
-
Entity Intelligence Workflows
-
Match Entity Records
generates fuzzy record matches across different entity datasets. -
Detect Entity Networks
generates reports on risk exposure for networks of related entities.
-
-
Text Intelligence Workflows
-
Query Text Data
generates reports from a collection of text documents. -
Extract Record Data
generate schema-aligned JSON objects and CSV records from unstructured text. -
Generate Mock Data
generates mock records and texts from a JSON schema defined or uploaded by the user.
-
All tutorial data and examples used in Intelligence Toolkit were created for this purpose using the Generate Mock Data
workflow.
Use the diagram to identify an appropriate workflow, which can be opened from the left sidebar while running the application.
%%{init: {
"flowchart": {"htmlLabels": true}} }%%
flowchart TD
NoData["<b>Input</b>: None"] --> |"<b>Generate Mock Data</b><br/>workflow"| MockData["AI-Generated Records"]
NoData["<b>Input</b>: None"] --> |"<b>Generate Mock Data</b><br/>workflow"| MockText["AI-Generated Texts"]
MockText["AI-Generated Texts"] --> TextDocs["<b>Input:</b> Text Data"]
MockData["AI-Generated Records"] --> PersonalData["<b>Input</b>: Personal Case Records"]
MockData["AI-Generated Records"] --> CaseRecords["<b>Input</b>: Case Records"]
MockData["AI-Generated Records"] --> EntityData["<b>Input</b>: Entity Records"]
PersonalData["<b>Input</b>: Personal Case Records"] ----> |"<b>Anonymize Case Data</b><br/>workflow"| AnonData["Anonymous Case Records"]
CaseRecords["<b>Input</b>: Case Records"] ---> HasTime{"Time<br/>Attributes?"}
HasTime{"Time<br/>Attributes?"} --> |"<b>Detect Case Patterns</b><br/>workflow"| CasePatterns["AI Pattern Reports"]
CaseRecords["<b>Input</b>: Case Records"] ---> HasGroups{"Grouping<br/>Attributes?"}
HasGroups{"Grouping<br/>Attributes?"} --> |"<b>Compare Case Groups</b><br/>workflow"| MatchedEntities["AI Group Reports"]
EntityData["<b>Input</b>: Entity Records"] ---> HasInconsistencies{"Inconsistent<br/>Attributes?"} --> |"<b>Match Entity Records</b><br/>workflow"| RecordLinking["AI-Matched Records"]
EntityData["<b>Input</b>: Entity Records"] ---> HasIdentifiers{"Identifying<br/>Attributes?"} --> |"<b>Detect Entity Networks</b><br/>workflow"| NetworkAnalysis["AI Network Reports"]
TextDocs["<b>Input:</b> Text Data"] ---> NeedRecords{"Need<br/>Records?"} --> |"<b>Extract Record Data</b><br/>workflow"| ExtractedRecords["AI-Extracted Records"]
TextDocs["<b>Input:</b> Text Data"] ---> NeedAnswers{"Need<br/>Answers?"} --> |"<b>Query Text Data</b><br/>workflow"| AnswerReports["AI Answer Reports"]
The Intelligence Toolkit was designed, refined, and evaluated in the context of the Tech Against Trafficking (TAT) accelerator program with Issara Institute and Polaris (2023-2024). It includes and builds on prior accelerator outputs developed with Unseen (2021-2022) and IOM/CTDC (2019-2020). See this launch blog for more information.
Additionally, a comprehensive system evaluation was performed from the standpoint of Responsible Artificial Intelligence (RAI). This evaluation was carried out utilizing the GPT-4 model. It is important to note that the choice of model plays a significant role in the evaluation process. Consequently, employing a model different from GPT-4 may yield varying results, as each model possesses unique characteristics and processing methodologies that can influence the outcome of the evaluation. Please refer to this Overview of Responsible AI practices for more information.
What are the limitations of Intelligence Toolkit? How can users minimize the impact of these limitations when using the system?
- The Intelligence toolkit aims to detect and explain patterns, relationships, and risks in data provided by the user. It is not designed to make decisions or take actions based on these findings.
- The statistical "insights" that it detects may not be insightful or useful in practice, and will inherit any biases, errors, or omissions present in the data collecting/generating process. These may be further amplified by the AI interpretations and reports generated by the toolkit.
- The generative AI model may itself introduce additional statistical or societal biases, or fabricate information not present in its grounding data, as a consequence of its training and design.
- Users should be experts in their domain, familiar with the data, and both able and willing to evaluate the quality of the insights and AI interpretations before taking action.
- The system was designed and tested for the processing of English language data and the creation of English language outputs. Performance in other languages may vary and should be assessed by someone who is both an expert on the data and a native speaker of that language.
What operational factors and settings allow for effective and responsible use of Intelligence Toolkit?
- The Intelligence Toolkit is designed for moderate-sized datasets (e.g., 100s of thousands of records, 100s of PDF documents). Larger datasets will require longer to process and may exceed the memory limits of the execution environment.
- Responsible use of personal case data requires that the data be deidentified prior to uploading and then converted into anonymous data using the Anonymize Case Data workflow. Any subsequent analysis of the case data should be done using the anonym case data, not the original (sensitive/personal) case data.
- It is the user's responsibility to ensure that any data sent to generative AI models is not personal/sensitive/secret/confidential, that use of generative AI models is consistent with the terms of service of the model provider, and that such use incurs per-token costs charged to the account linked to the user-provided API key. Understanding usage costs (OpenAI, Azure) and setting a billing cap (OpenAI) or budget (Azure) is recommended.
Intelligence Toolkit may be deployed as a desktop application or a cloud service. The application supports short, end-to-end workflows from input data to output reports. As such, it stores no data beyond the use of a caching mechanism for text embeddings that avoids unnecessary recomputation costs. No data is collected by Microsoft or sent to any other service other than the selected AI model API.
The system uses the GPT-4 model from OpenAI, either via OpenAI or Azure OpenAI APIs. See the GPT-4 System Card to understand the capabilities and limitations of this model. For models hosted on Azure OpenAI, also see the accompanying Transparency Note.
- Intelligence Toolkit is an AI system that generates text.
- System performance may vary by workflow, dataset, query, and response.
- Outputs may include factual errors, fabrication, or speculation.
- Users are responsible for determining the accuracy of generated content.
- System outputs do not represent the opinions of Microsoft.
- All decisions leveraging outputs of the system should be made with human oversight and not be based solely on system outputs.
- The system is only intended to be used for analysis by domain experts capable of evaluating the quality of data insights it generates.
- Use of the system must comply with all applicable laws, regulations, and policies, including those pertaining to privacy and security.
- The system should not be used in highly regulated domains where inaccurate outputs could suggest actions that lead to injury or negatively impact an individual's legal, financial, or life opportunities.
- Intelligence Toolkit is meant to be used to evaluate populations and entities, not individuals, identifying areas for further investigation by human experts.
- Intelligence Toolkit is not meant to be used as per se evidence of a crime or to establish criminal activity.
All use of Intelligence Toolkit should be consistent with this documentation. In addition, using the system in any of the following ways is strictly prohibited:
- Pursuing any illegal purpose.
- Identifying or evaluating individuals.
- Establishing criminal activity.
You can start using the Intelligence Toolkit as either a web application (in Azure or locally with a tool called Docker) or a Python package (via PyPI). Choose one of the options below based on your needs.
Option 1: Using Intelligence Toolkit in Azure
Non-profit organizations can apply for an annual Azure credit grant of $2,000, which can be used to set up and run an instance of the intelligence-toolkit app for your organization.
Read more about eligibility and registration here
See instructions on how to.
Option 2: Using Intelligence Toolkit as a Web Application (via Docker)
To use the Intelligence Toolkit as a web application, you can download and run it using Docker.
1. Install Docker:
Download and install Docker Desktop from docker.com.
Start the Docker Desktop app and make sure it’s running before proceeding.
2. Open Terminal:
Open a terminal according to your OS:
-
If you are using Windows, search for and open the app
Windows Powershell
in the Windows start menu. -
If you are using Linux or Mac, search for and open
Terminal
.
3. Pull the Docker Container:
Download a copy of the Intelligence Toolkit application from GitHub:
docker pull ghcr.io/microsoft/intelligence-toolkit:latest
Note: The image is approximately 2GB, so the download may take some time depending on your internet speed.
4. Run the Docker Container:
Once the download is finished, run the Intelligence Toolkit application using Docker by pasting the following command into your terminal and pressing enter:
docker run -d --name intelligence-toolkit -p 80:80 ghcr.io/microsoft/intelligence-toolkit:latest
5. Access the Web Application:
Open http://localhost:80 in your web browser to start using Intelligence Toolkit.
Note: Docker Desktop App may enter sleep mode if inactive. In this case, open Docker Desktop, select Container in the left menu, then press play on intelligence-toolkit.
6. Setting up the AI model:
Intelligence Toolkit can be used with either OpenAI or Azure OpenAI as the generative AI API.
The Generate Mock Data
and Extract Record Data
workflows additionally use OpenAI's Structured Outputs API, which requires a gpt-4o model as follows:
gpt-4o-mini
gpt-4o
You can access the Settings
page on the left sidebar when running the application:
-
For OpenAI, you will need an active OpenAI account (create here) and API key (create here).
-
For Azure OpenAI, you will need an active Azure account (create here), endpoint, key and version for the AI Service (create here).
Option 3: Using Intelligence Toolkit as a Python Package (via PyPI)
If you prefer to use Intelligence Toolkit as a Python package, install it directly from PyPI:
pip install intelligence-toolkit
After installation, explore the examples in the example_notebooks folder to get started with various functionalities.
- To start developing, see DEVELOPING.md.
- To instructions on how to deploy, see DEPLOYING.md.
- To learn about our contribution guidelines, see CONTRIBUTING.md.
- For license details, see LICENSE.md.
If you have any questions or need further assistance, you can reach out to the project maintainers at [email protected].
- This project may contain trademarks or logos for projects, products, or services.
- Authorized use of Microsoft trademarks or logos is subject to and must follow Microsoft's Trademark & Brand Guidelines.
- Use of Microsoft trademarks or logos in modified versions of this project must not cause confusion or imply Microsoft sponsorship.
- Any use of third-party trademarks or logos are subject to those third-party's policies.
For Tasks:
Click tags to check more tools for each tasksFor Jobs:
Alternative AI tools for intelligence-toolkit
Similar Open Source Tools
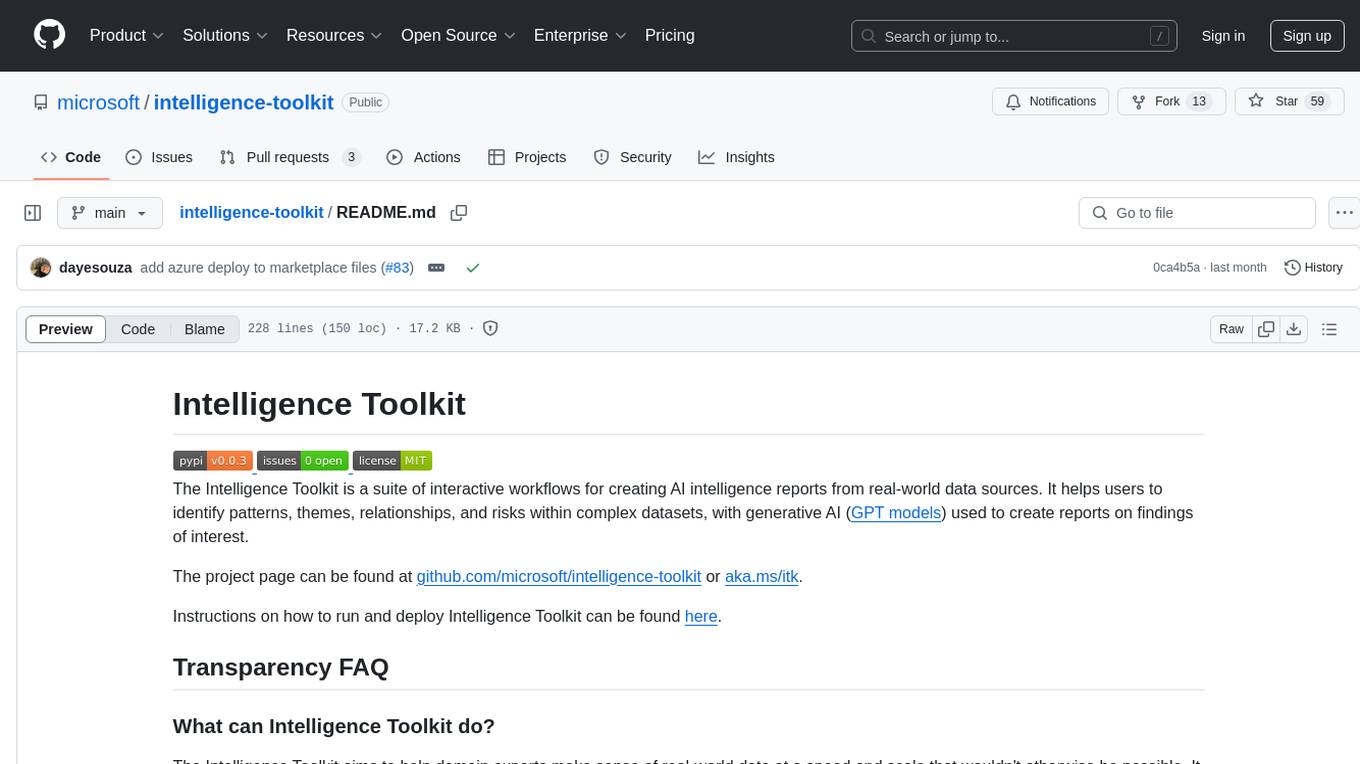
intelligence-toolkit
The Intelligence Toolkit is a suite of interactive workflows designed to help domain experts make sense of real-world data by identifying patterns, themes, relationships, and risks within complex datasets. It utilizes generative AI (GPT models) to create reports on findings of interest. The toolkit supports analysis of case, entity, and text data, providing various interactive workflows for different intelligence tasks. Users are expected to evaluate the quality of data insights and AI interpretations before taking action. The system is designed for moderate-sized datasets and responsible use of personal case data. It uses the GPT-4 model from OpenAI or Azure OpenAI APIs for generating reports and insights.
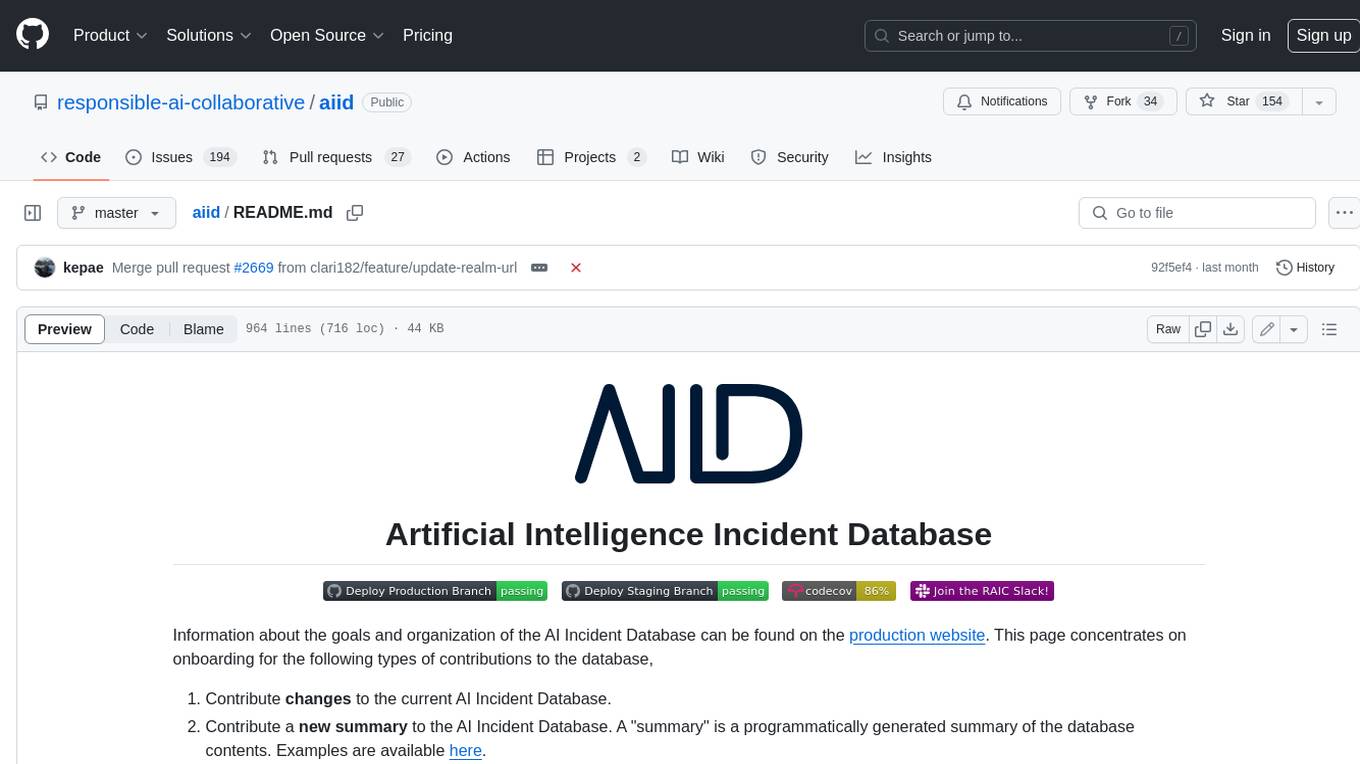
aiid
The Artificial Intelligence Incident Database (AIID) is a collection of incidents involving the development and use of artificial intelligence (AI). The database is designed to help researchers, policymakers, and the public understand the potential risks and benefits of AI, and to inform the development of policies and practices to mitigate the risks and promote the benefits of AI. The AIID is a collaborative project involving researchers from the University of California, Berkeley, the University of Washington, and the University of Toronto.
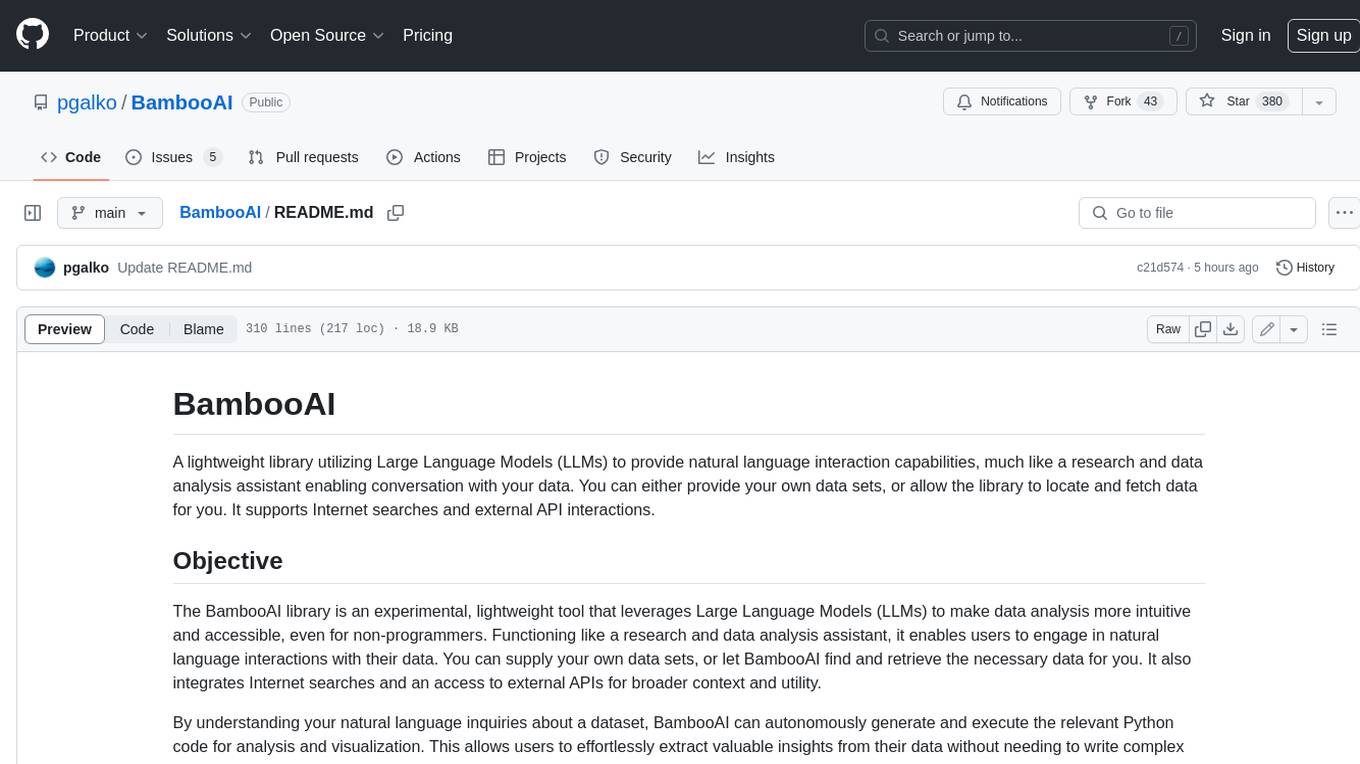
BambooAI
BambooAI is a lightweight library utilizing Large Language Models (LLMs) to provide natural language interaction capabilities, much like a research and data analysis assistant enabling conversation with your data. You can either provide your own data sets, or allow the library to locate and fetch data for you. It supports Internet searches and external API interactions.
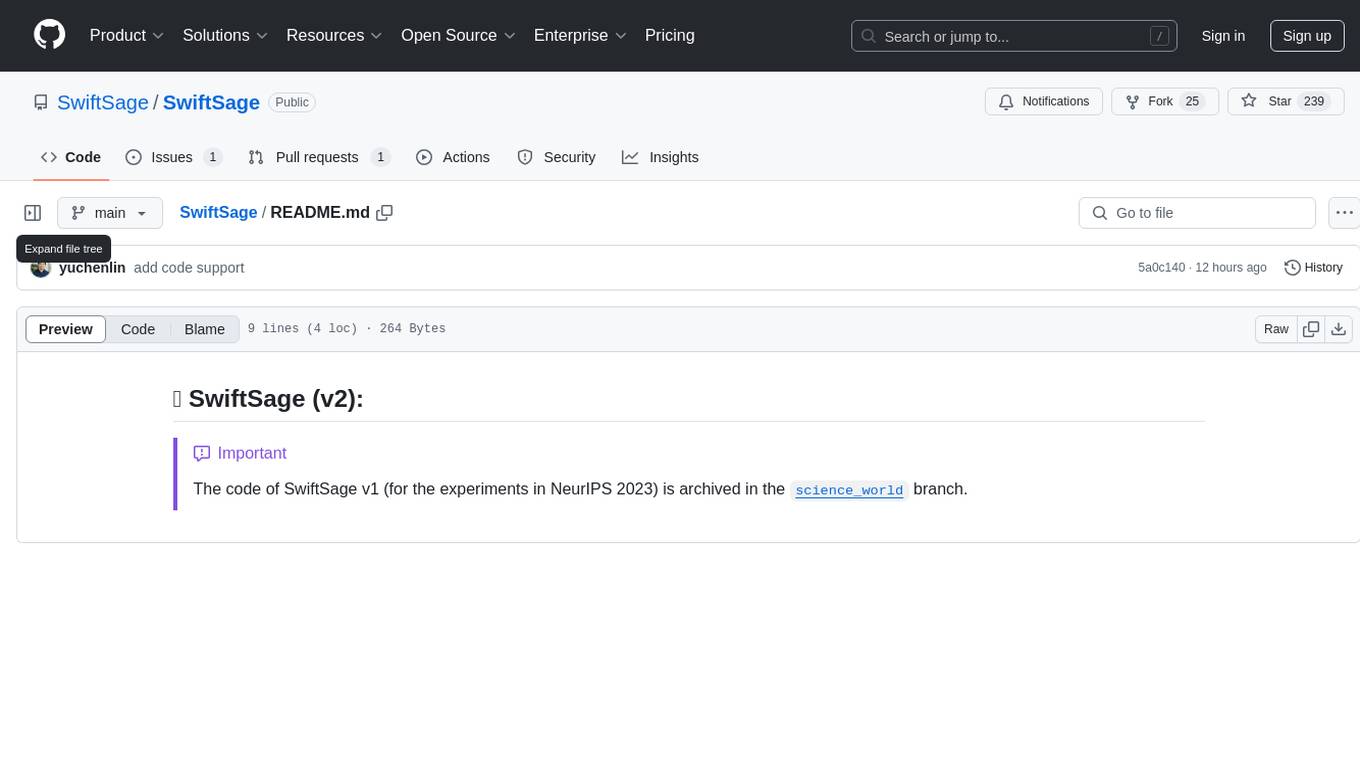
SwiftSage
SwiftSage is a tool designed for conducting experiments in the field of machine learning and artificial intelligence. It provides a platform for researchers and developers to implement and test various algorithms and models. The tool is particularly useful for exploring new ideas and conducting experiments in a controlled environment. SwiftSage aims to streamline the process of developing and testing machine learning models, making it easier for users to iterate on their ideas and achieve better results. With its user-friendly interface and powerful features, SwiftSage is a valuable tool for anyone working in the field of AI and ML.
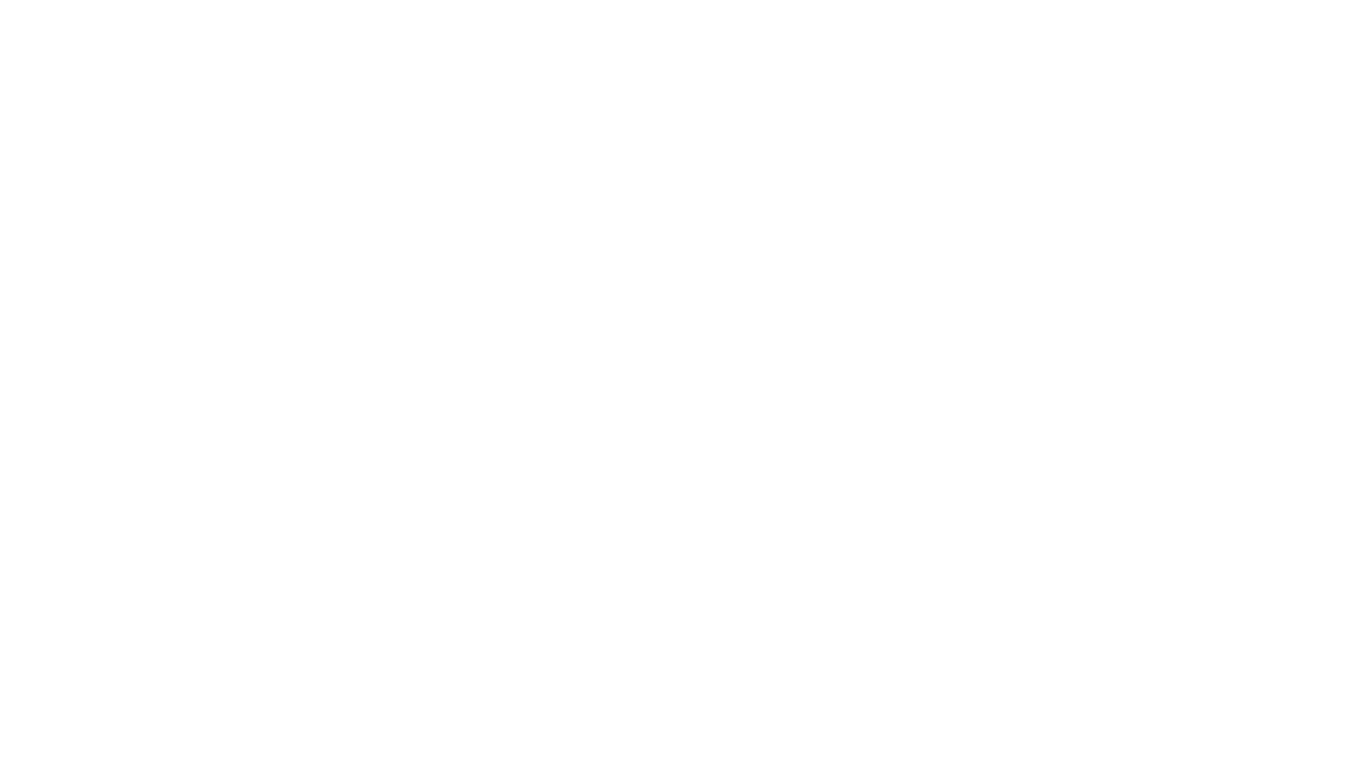
project_alice
Alice is an agentic workflow framework that integrates task execution and intelligent chat capabilities. It provides a flexible environment for creating, managing, and deploying AI agents for various purposes, leveraging a microservices architecture with MongoDB for data persistence. The framework consists of components like APIs, agents, tasks, and chats that interact to produce outputs through files, messages, task results, and URL references. Users can create, test, and deploy agentic solutions in a human-language framework, making it easy to engage with by both users and agents. The tool offers an open-source option, user management, flexible model deployment, and programmatic access to tasks and chats.
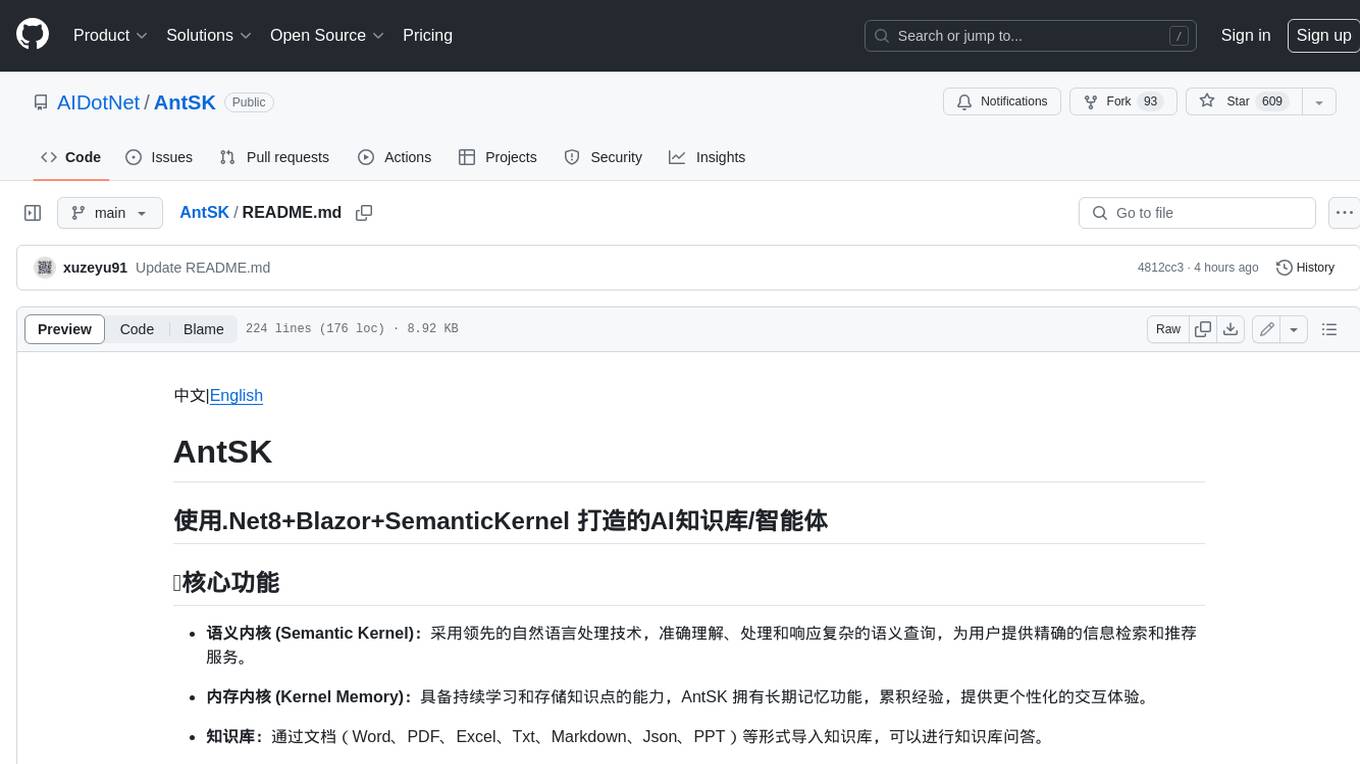
AntSK
AntSK is an AI knowledge base/agent built with .Net8+Blazor+SemanticKernel. It features a semantic kernel for accurate natural language processing, a memory kernel for continuous learning and knowledge storage, a knowledge base for importing and querying knowledge from various document formats, a text-to-image generator integrated with StableDiffusion, GPTs generation for creating personalized GPT models, API interfaces for integrating AntSK into other applications, an open API plugin system for extending functionality, a .Net plugin system for integrating business functions, real-time information retrieval from the internet, model management for adapting and managing different models from different vendors, support for domestic models and databases for operation in a trusted environment, and planned model fine-tuning based on llamafactory.
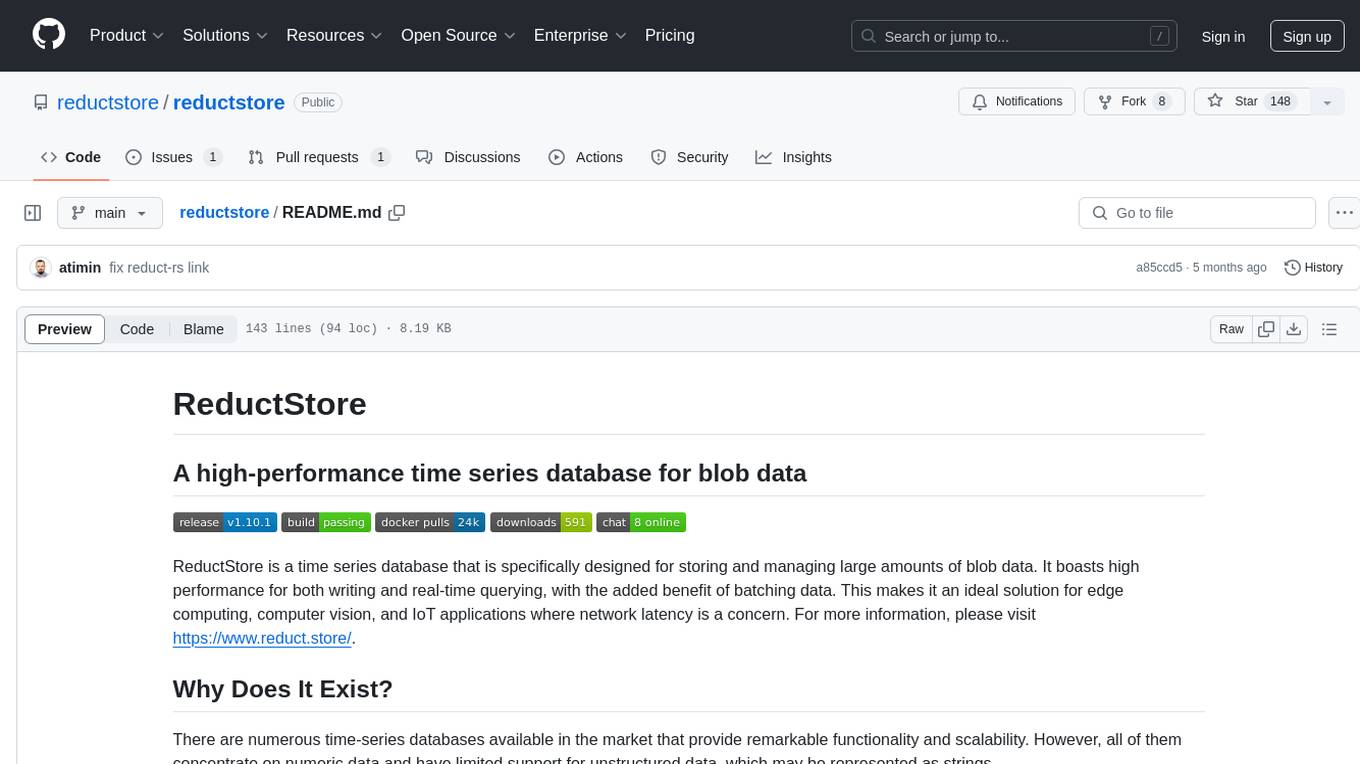
reductstore
ReductStore is a high-performance time series database designed for storing and managing large amounts of unstructured blob data. It offers features such as real-time querying, batching data, and HTTP(S) API for edge computing, computer vision, and IoT applications. The database ensures data integrity, implements retention policies, and provides efficient data access, making it a cost-effective solution for applications requiring unstructured data storage and access at specific time intervals.
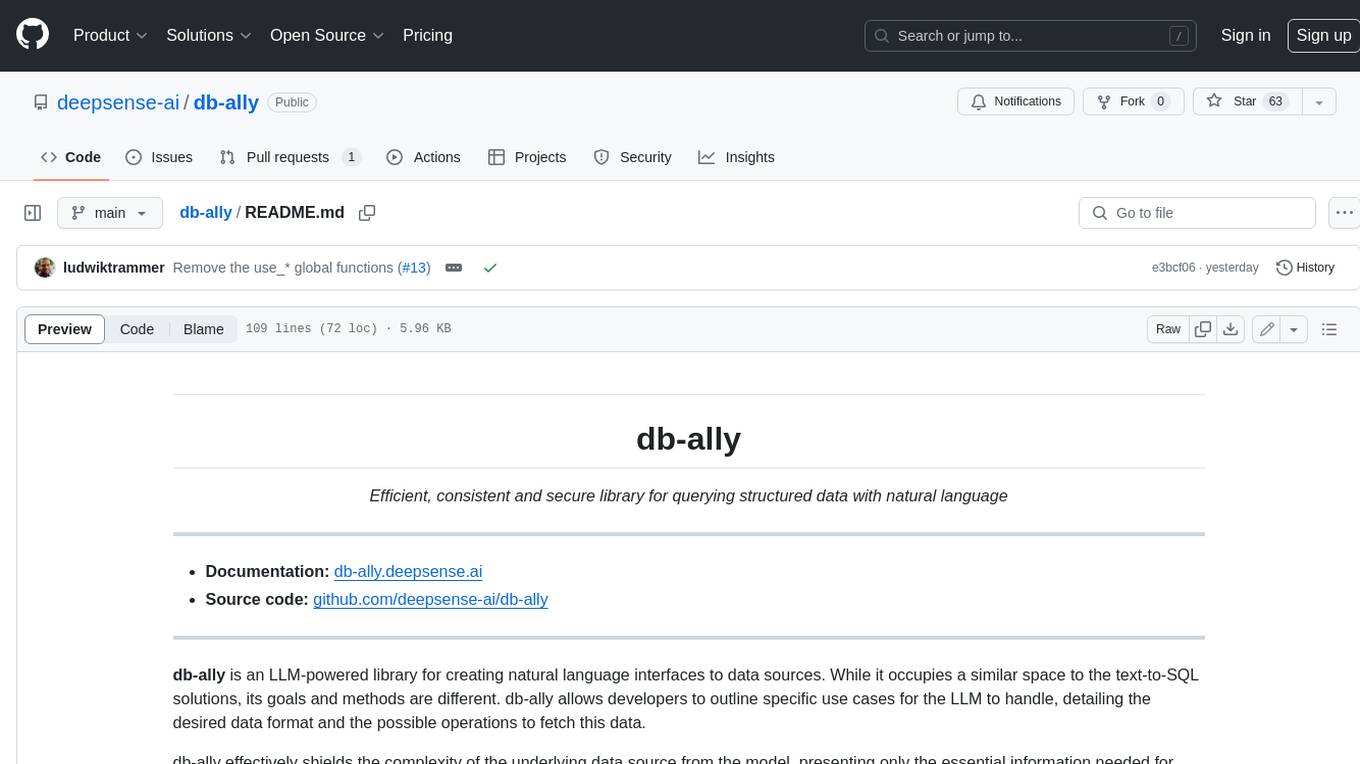
db-ally
db-ally is a library for creating natural language interfaces to data sources. It allows developers to outline specific use cases for a large language model (LLM) to handle, detailing the desired data format and the possible operations to fetch this data. db-ally effectively shields the complexity of the underlying data source from the model, presenting only the essential information needed for solving the specific use cases. Instead of generating arbitrary SQL, the model is asked to generate responses in a simplified query language.
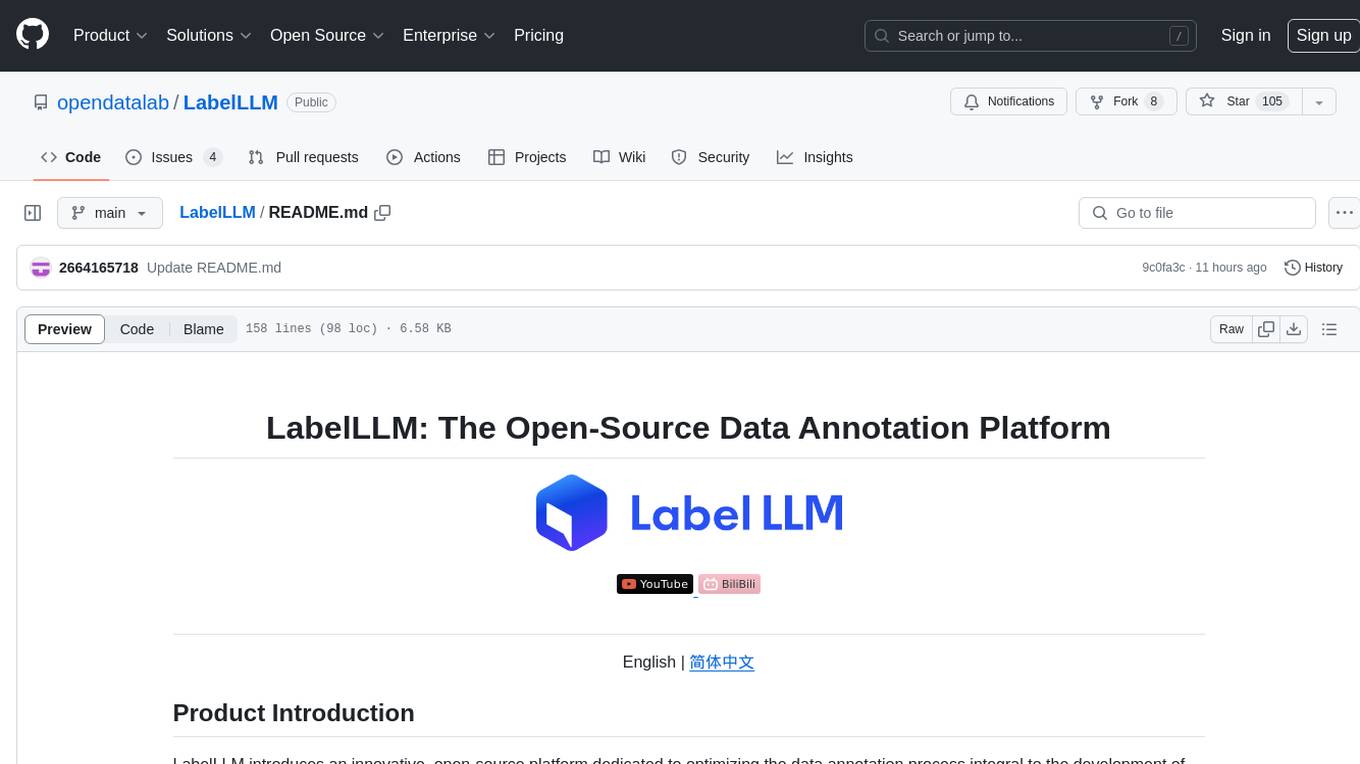
LabelLLM
LabelLLM is an open-source data annotation platform designed to optimize the data annotation process for LLM development. It offers flexible configuration, multimodal data support, comprehensive task management, and AI-assisted annotation. Users can access a suite of annotation tools, enjoy a user-friendly experience, and enhance efficiency. The platform allows real-time monitoring of annotation progress and quality control, ensuring data integrity and timeliness.
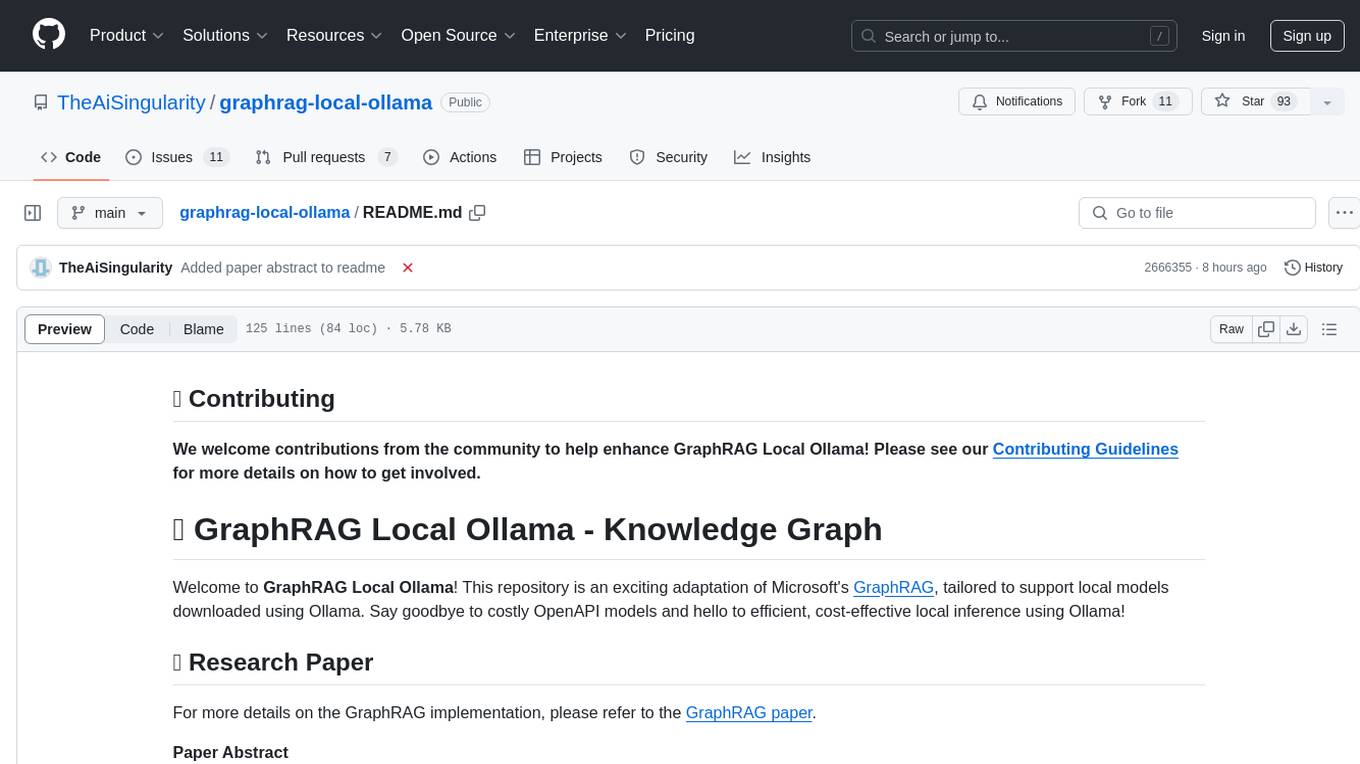
graphrag-local-ollama
GraphRAG Local Ollama is a repository that offers an adaptation of Microsoft's GraphRAG, customized to support local models downloaded using Ollama. It enables users to leverage local models with Ollama for large language models (LLMs) and embeddings, eliminating the need for costly OpenAPI models. The repository provides a simple setup process and allows users to perform question answering over private text corpora by building a graph-based text index and generating community summaries for closely-related entities. GraphRAG Local Ollama aims to improve the comprehensiveness and diversity of generated answers for global sensemaking questions over datasets.
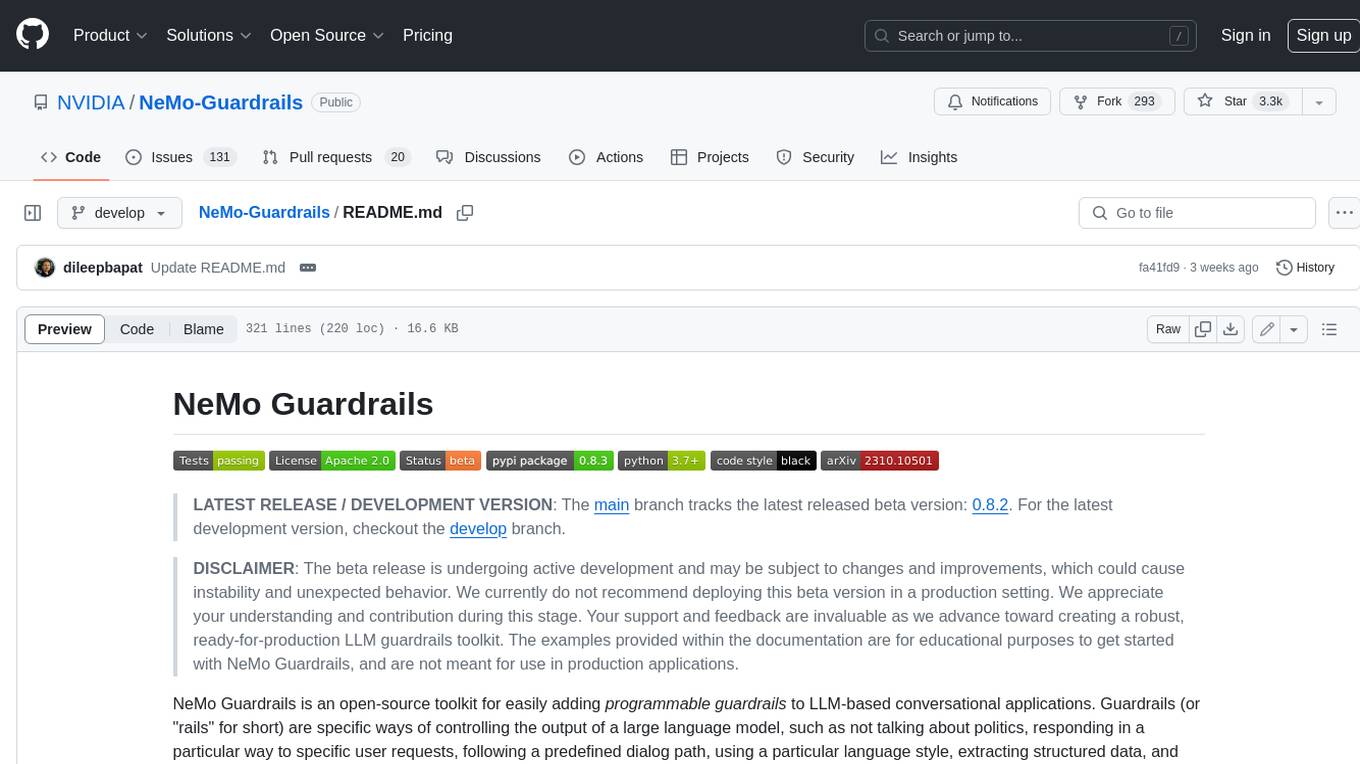
NeMo-Guardrails
NeMo Guardrails is an open-source toolkit for easily adding _programmable guardrails_ to LLM-based conversational applications. Guardrails (or "rails" for short) are specific ways of controlling the output of a large language model, such as not talking about politics, responding in a particular way to specific user requests, following a predefined dialog path, using a particular language style, extracting structured data, and more.
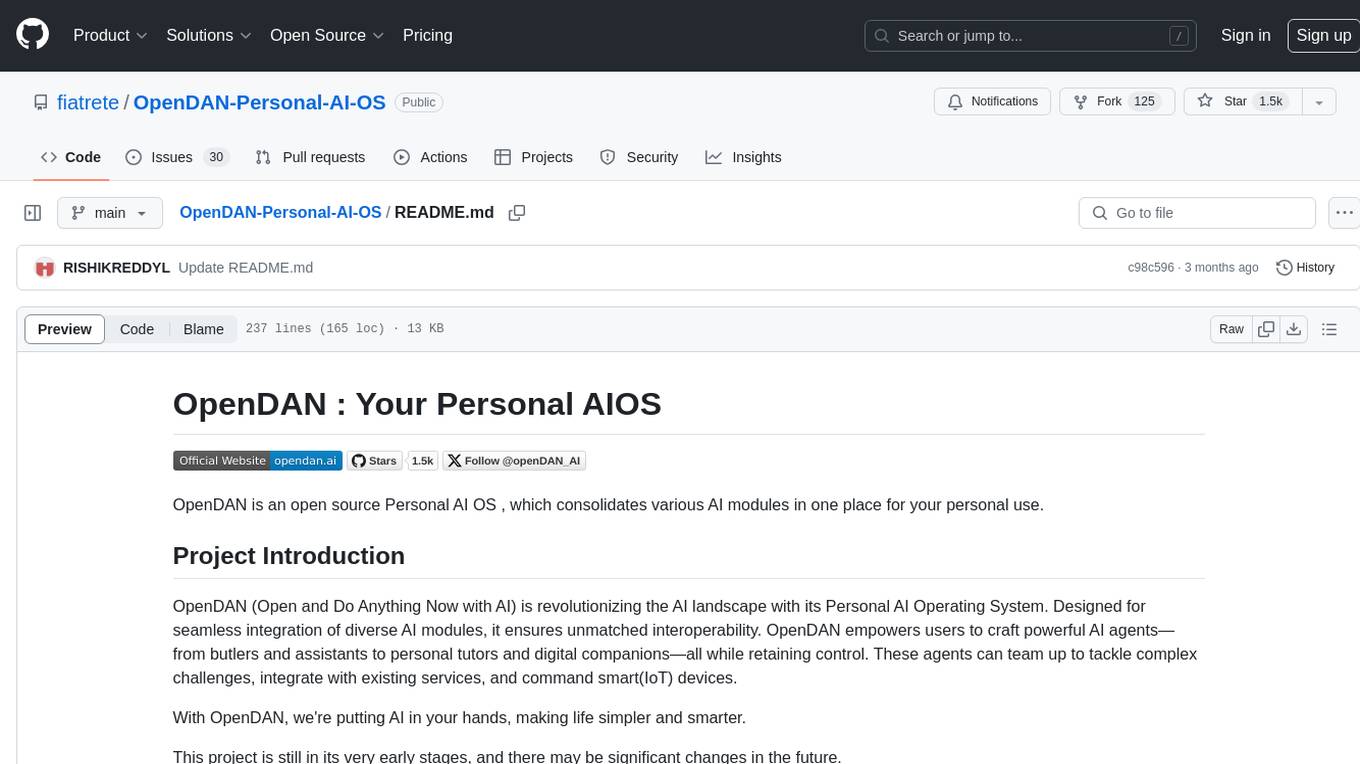
OpenDAN-Personal-AI-OS
OpenDAN is an open source Personal AI OS that consolidates various AI modules for personal use. It empowers users to create powerful AI agents like assistants, tutors, and companions. The OS allows agents to collaborate, integrate with services, and control smart devices. OpenDAN offers features like rapid installation, AI agent customization, connectivity via Telegram/Email, building a local knowledge base, distributed AI computing, and more. It aims to simplify life by putting AI in users' hands. The project is in early stages with ongoing development and future plans for user and kernel mode separation, home IoT device control, and an official OpenDAN SDK release.
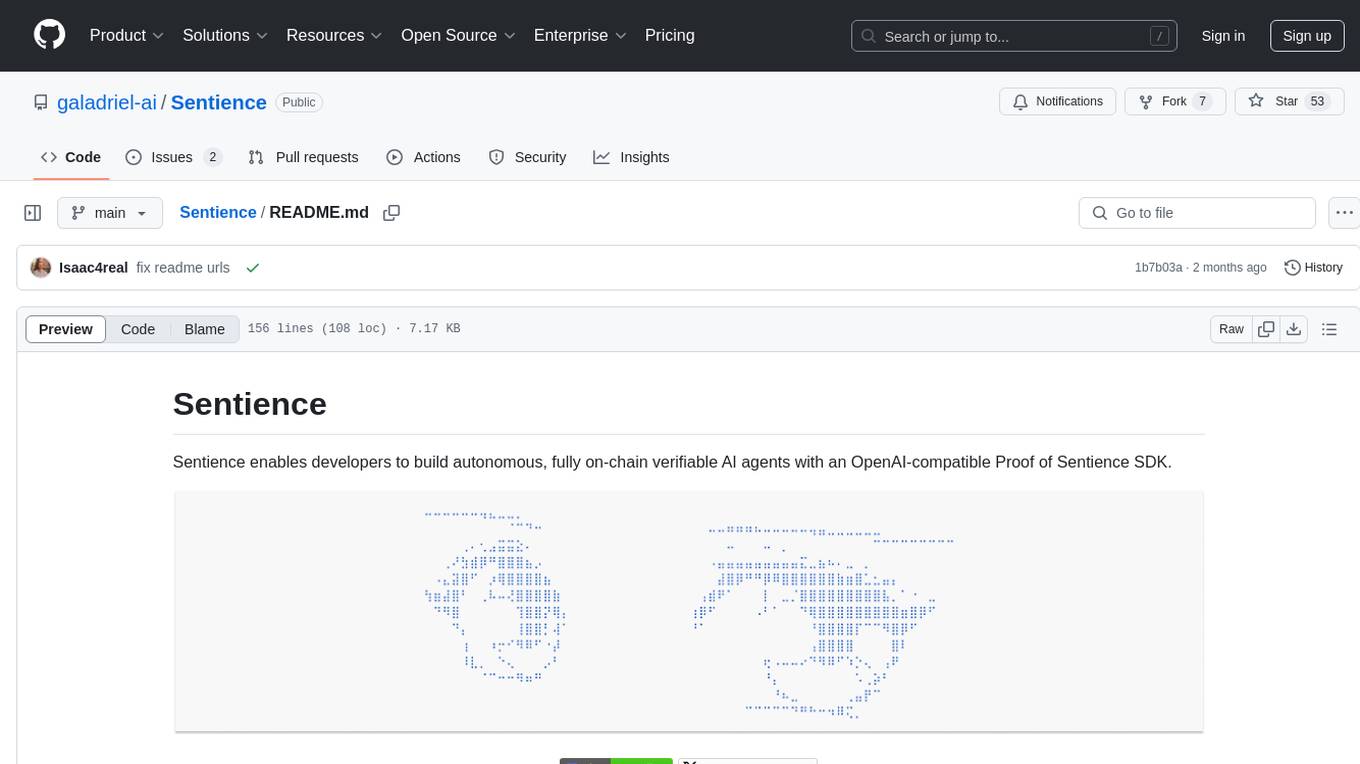
Sentience
Sentience is a tool that allows developers to create autonomous AI agents on-chain with verifiable proofs. It leverages a Trusted Execution Environment (TEE) architecture to ensure secure execution of AI calls and provides transparency through cryptographic attestations posted on Solana's blockchain. The tool enhances market potential by transforming agents into cryptographically verifiable entities, addressing the need for trust in AI development. Sentience offers features like OpenAI compatibility, on-chain verifiability, an explorer for agent history, and an easy-to-use developer experience. The repository includes SDKs for Python and JavaScript, along with components for verified inference and instructions for verifying the TEE architecture.
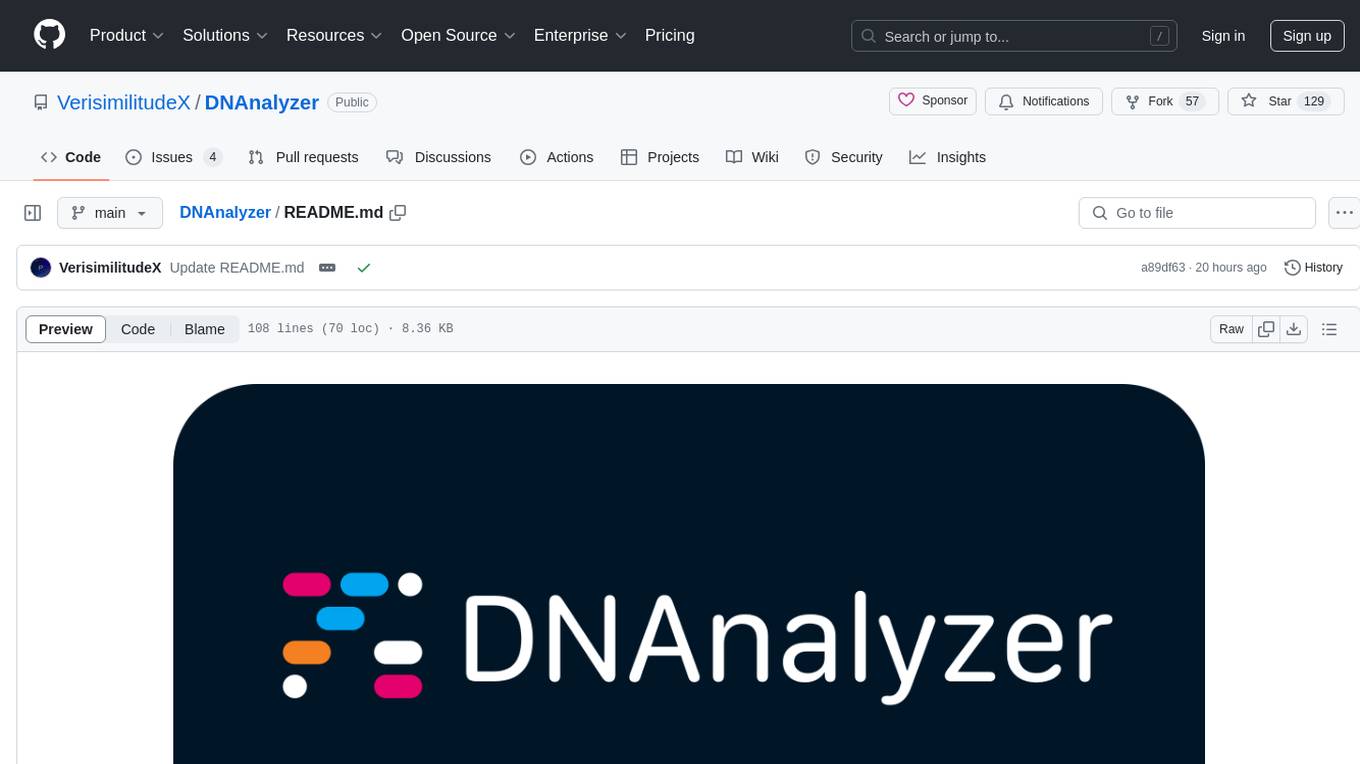
DNAnalyzer
DNAnalyzer is a nonprofit organization dedicated to revolutionizing DNA analysis through AI-powered tools. It aims to democratize access to DNA analysis for a deeper understanding of human health and disease. The tool provides innovative AI-powered analysis and interpretive tools to empower geneticists, physicians, and researchers to gain deep insights into DNA sequences, revolutionizing how we understand human health and disease.
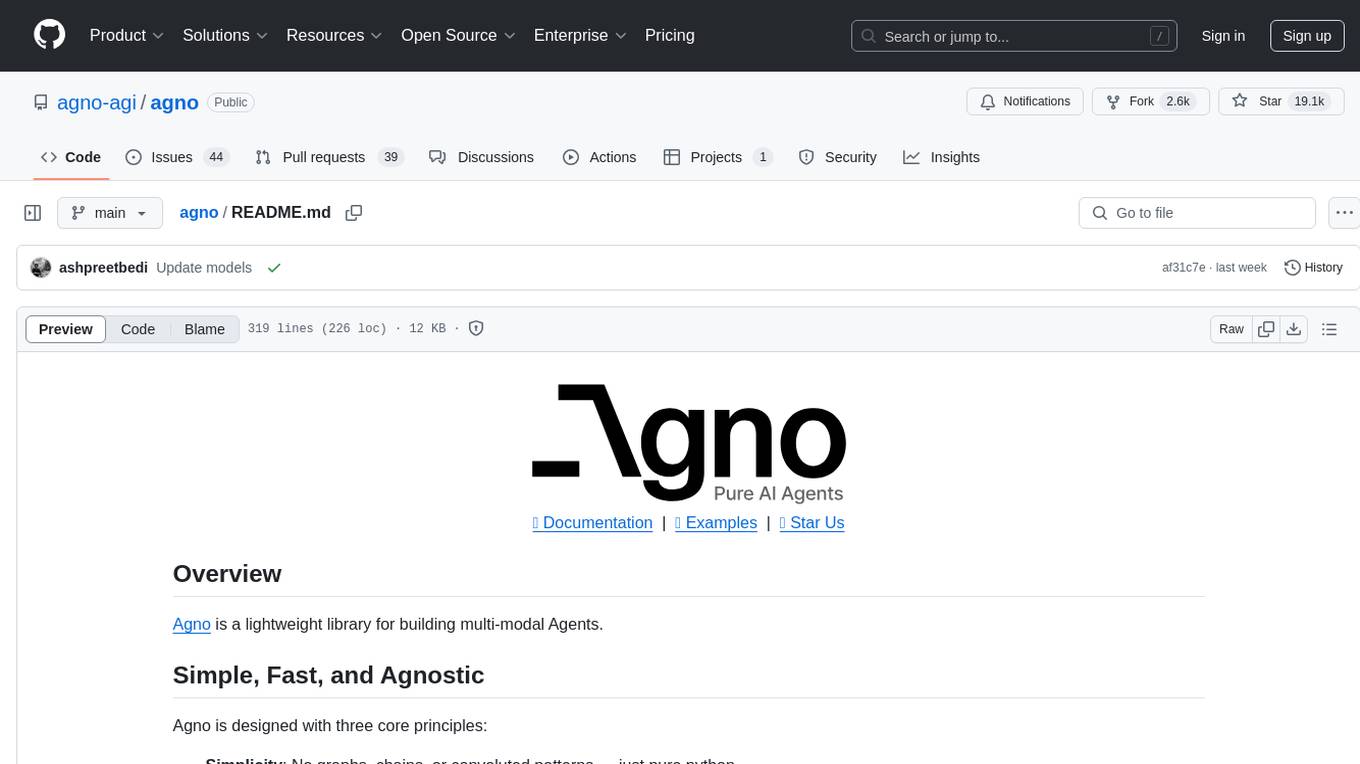
agno
Agno is a lightweight library for building multi-modal Agents. It is designed with core principles of simplicity, uncompromising performance, and agnosticism, allowing users to create blazing fast agents with minimal memory footprint. Agno supports any model, any provider, and any modality, making it a versatile container for AGI. Users can build agents with lightning-fast agent creation, model agnostic capabilities, native support for text, image, audio, and video inputs and outputs, memory management, knowledge stores, structured outputs, and real-time monitoring. The library enables users to create autonomous programs that use language models to solve problems, improve responses, and achieve tasks with varying levels of agency and autonomy.
For similar tasks
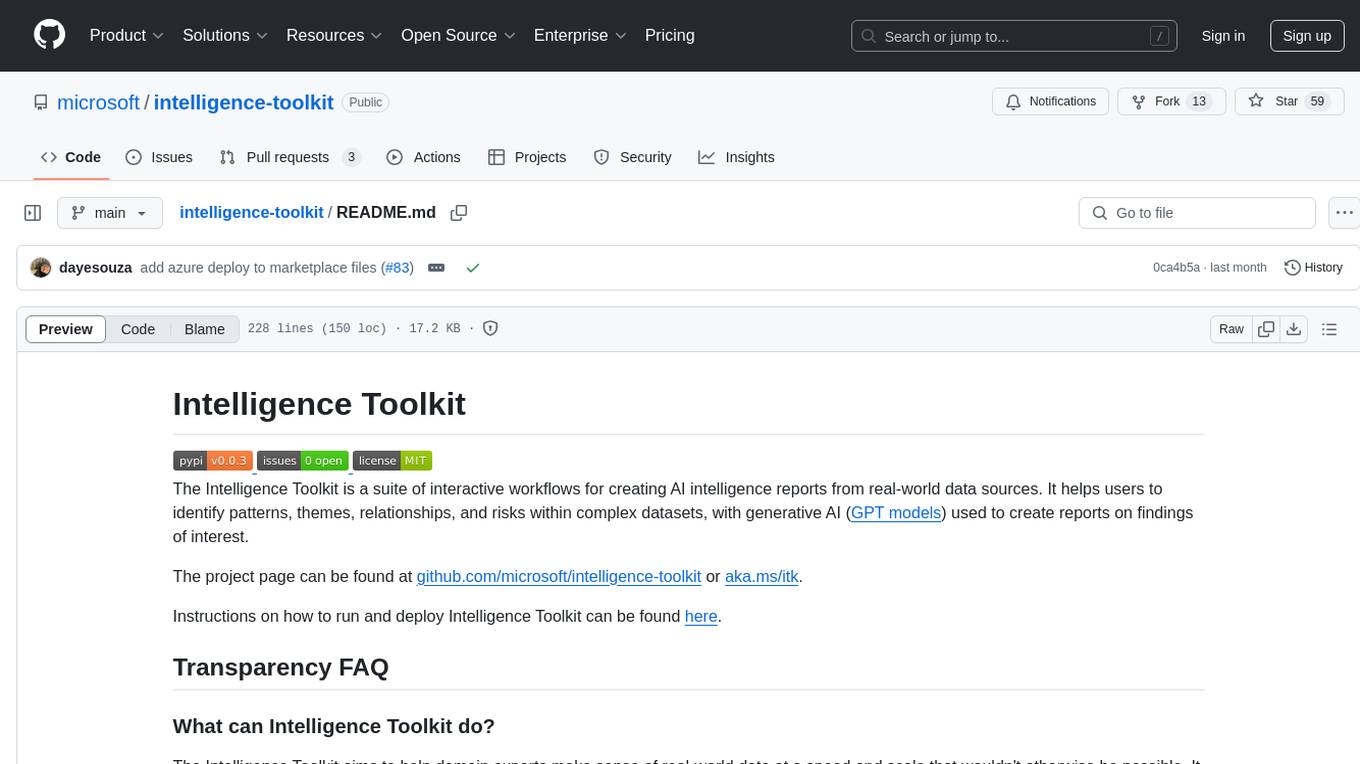
intelligence-toolkit
The Intelligence Toolkit is a suite of interactive workflows designed to help domain experts make sense of real-world data by identifying patterns, themes, relationships, and risks within complex datasets. It utilizes generative AI (GPT models) to create reports on findings of interest. The toolkit supports analysis of case, entity, and text data, providing various interactive workflows for different intelligence tasks. Users are expected to evaluate the quality of data insights and AI interpretations before taking action. The system is designed for moderate-sized datasets and responsible use of personal case data. It uses the GPT-4 model from OpenAI or Azure OpenAI APIs for generating reports and insights.
For similar jobs
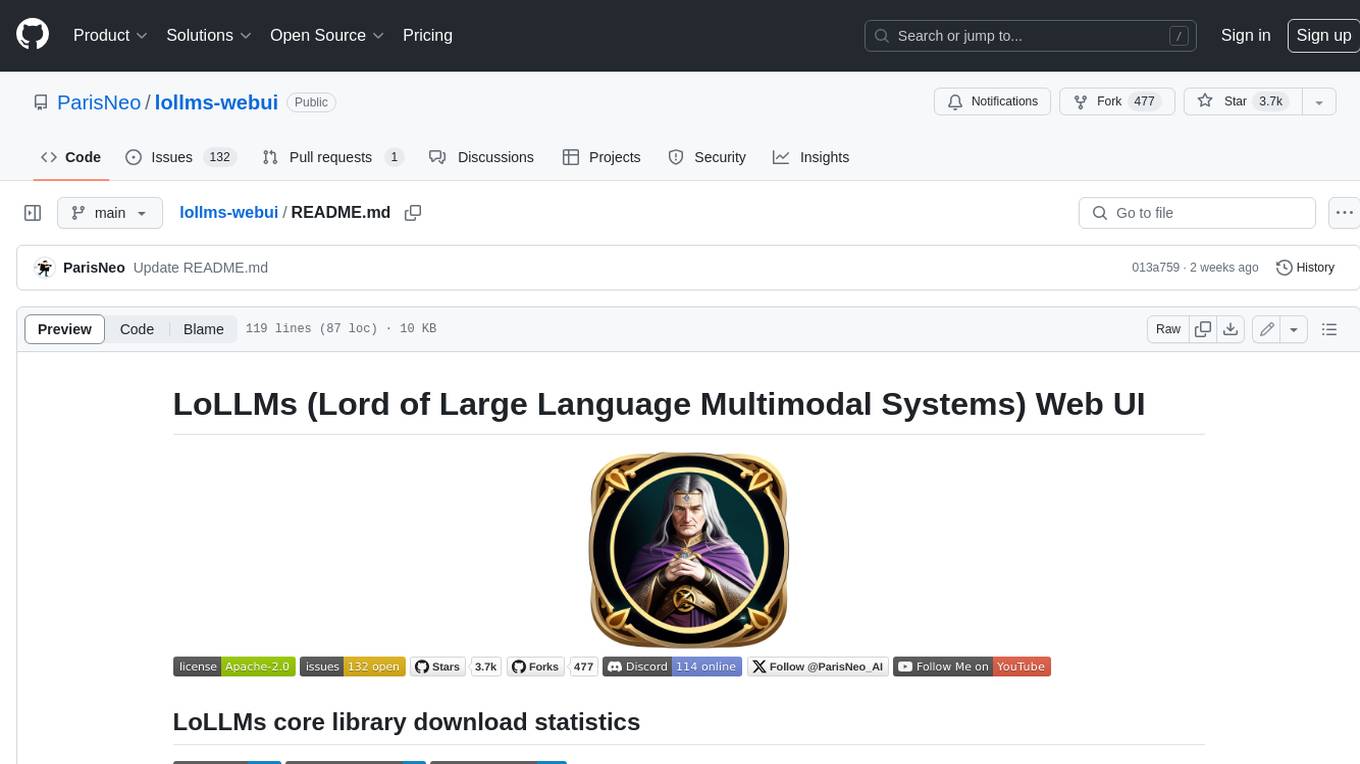
lollms-webui
LoLLMs WebUI (Lord of Large Language Multimodal Systems: One tool to rule them all) is a user-friendly interface to access and utilize various LLM (Large Language Models) and other AI models for a wide range of tasks. With over 500 AI expert conditionings across diverse domains and more than 2500 fine tuned models over multiple domains, LoLLMs WebUI provides an immediate resource for any problem, from car repair to coding assistance, legal matters, medical diagnosis, entertainment, and more. The easy-to-use UI with light and dark mode options, integration with GitHub repository, support for different personalities, and features like thumb up/down rating, copy, edit, and remove messages, local database storage, search, export, and delete multiple discussions, make LoLLMs WebUI a powerful and versatile tool.
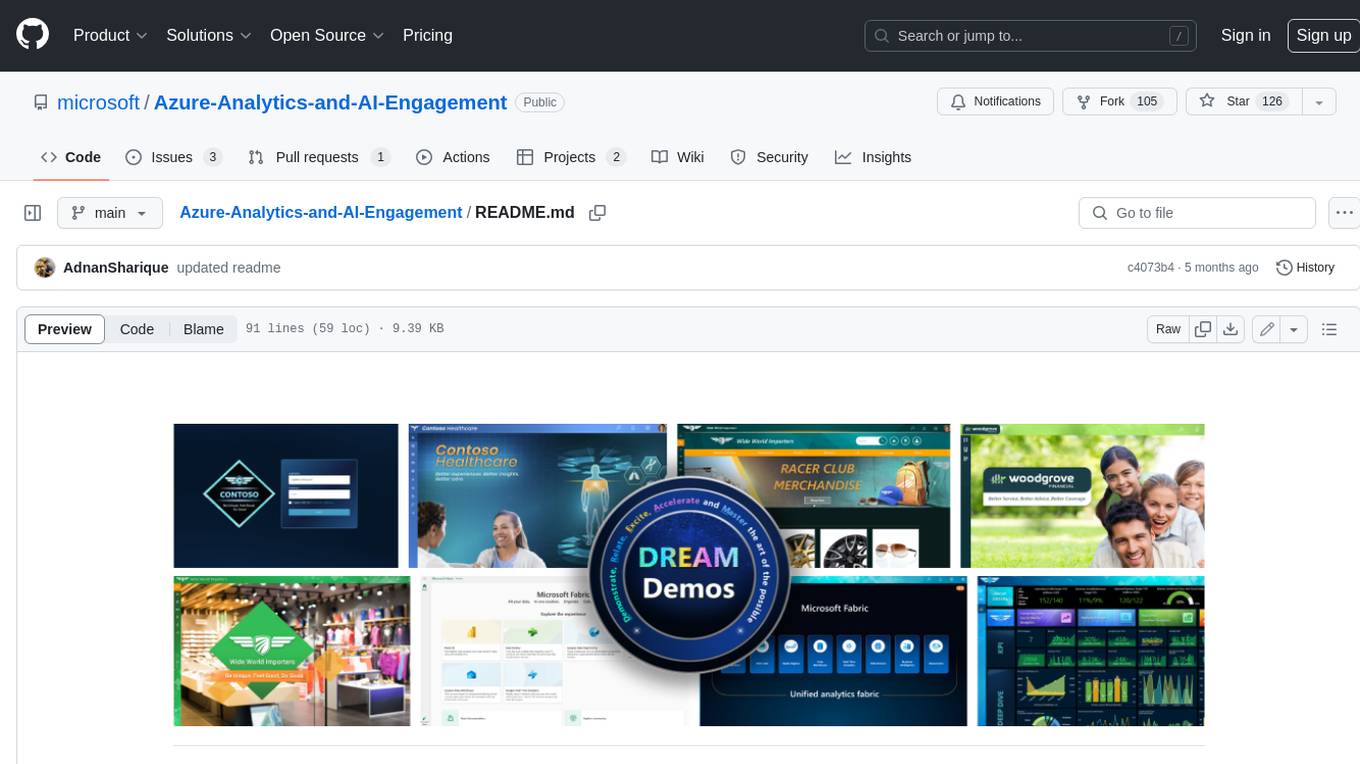
Azure-Analytics-and-AI-Engagement
The Azure-Analytics-and-AI-Engagement repository provides packaged Industry Scenario DREAM Demos with ARM templates (Containing a demo web application, Power BI reports, Synapse resources, AML Notebooks etc.) that can be deployed in a customer’s subscription using the CAPE tool within a matter of few hours. Partners can also deploy DREAM Demos in their own subscriptions using DPoC.
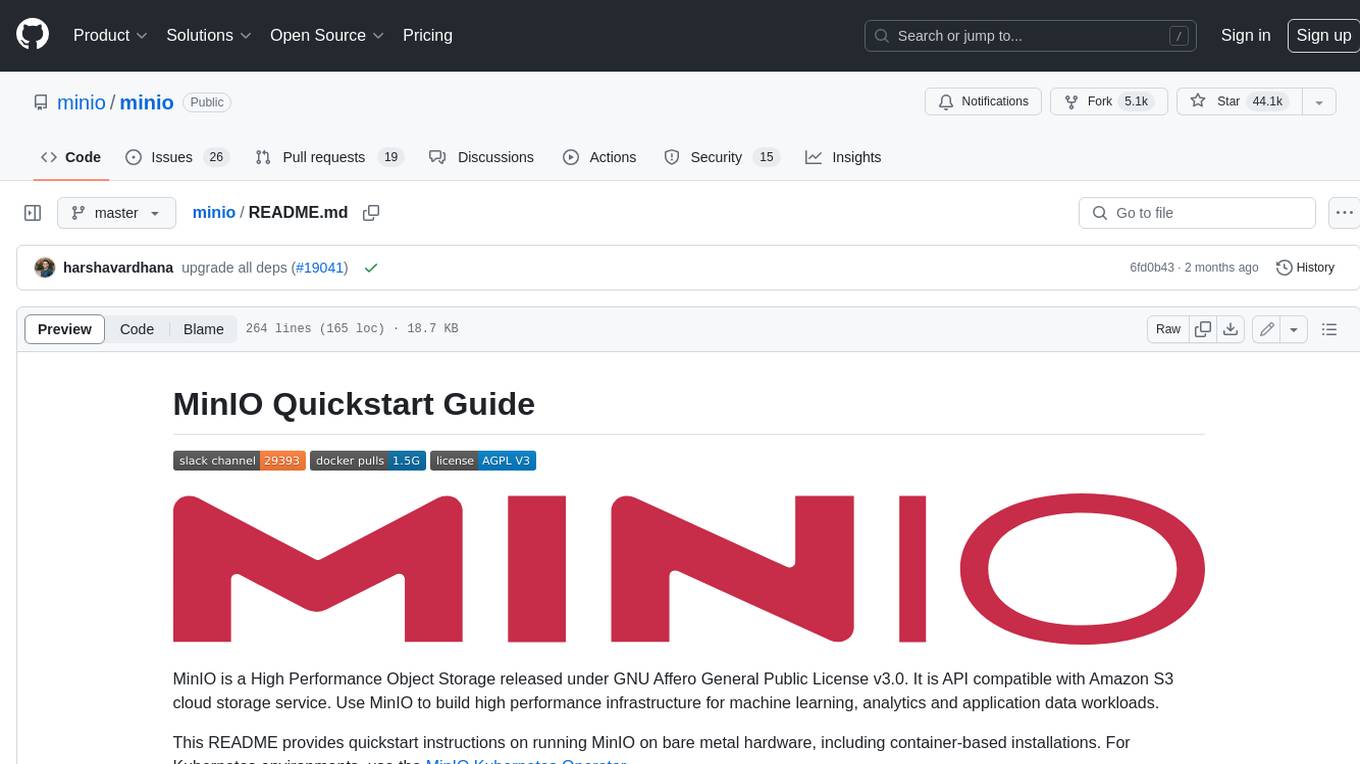
minio
MinIO is a High Performance Object Storage released under GNU Affero General Public License v3.0. It is API compatible with Amazon S3 cloud storage service. Use MinIO to build high performance infrastructure for machine learning, analytics and application data workloads.
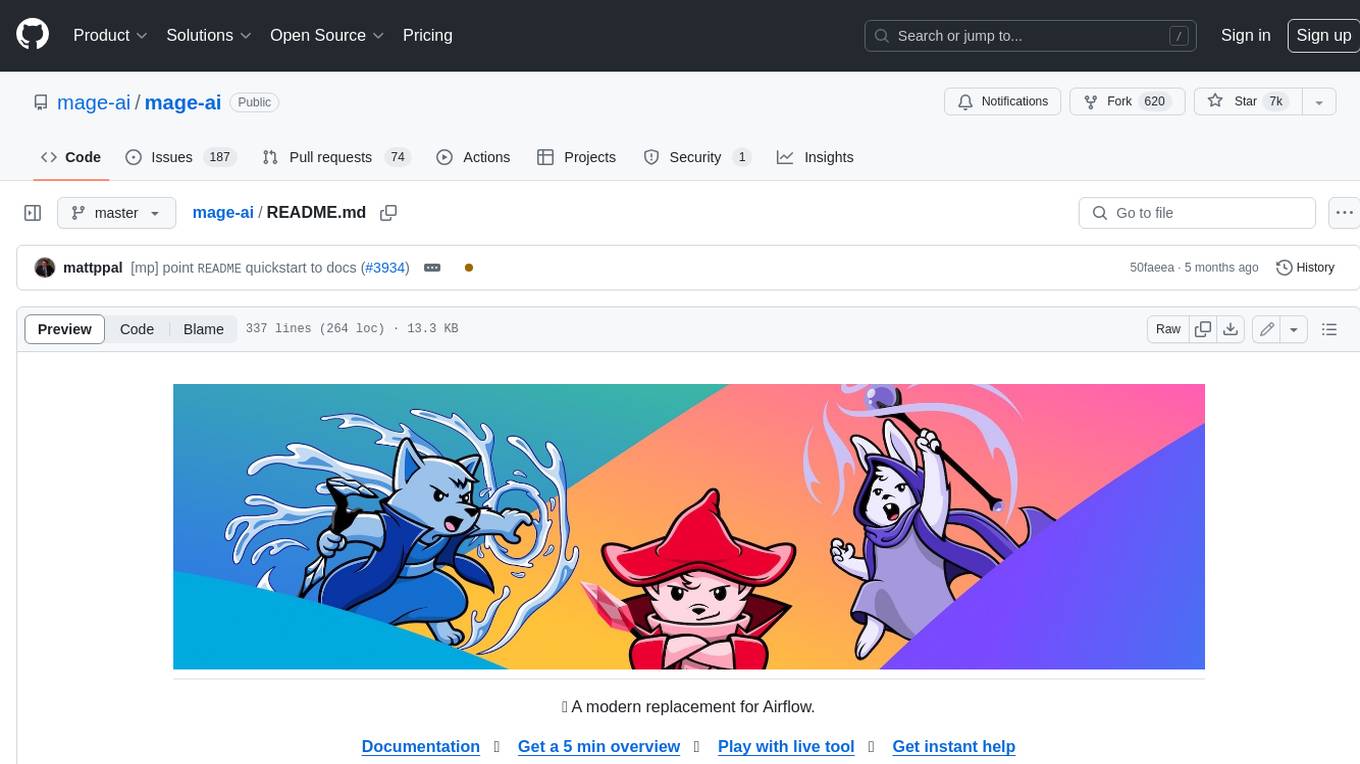
mage-ai
Mage is an open-source data pipeline tool for transforming and integrating data. It offers an easy developer experience, engineering best practices built-in, and data as a first-class citizen. Mage makes it easy to build, preview, and launch data pipelines, and provides observability and scaling capabilities. It supports data integrations, streaming pipelines, and dbt integration.
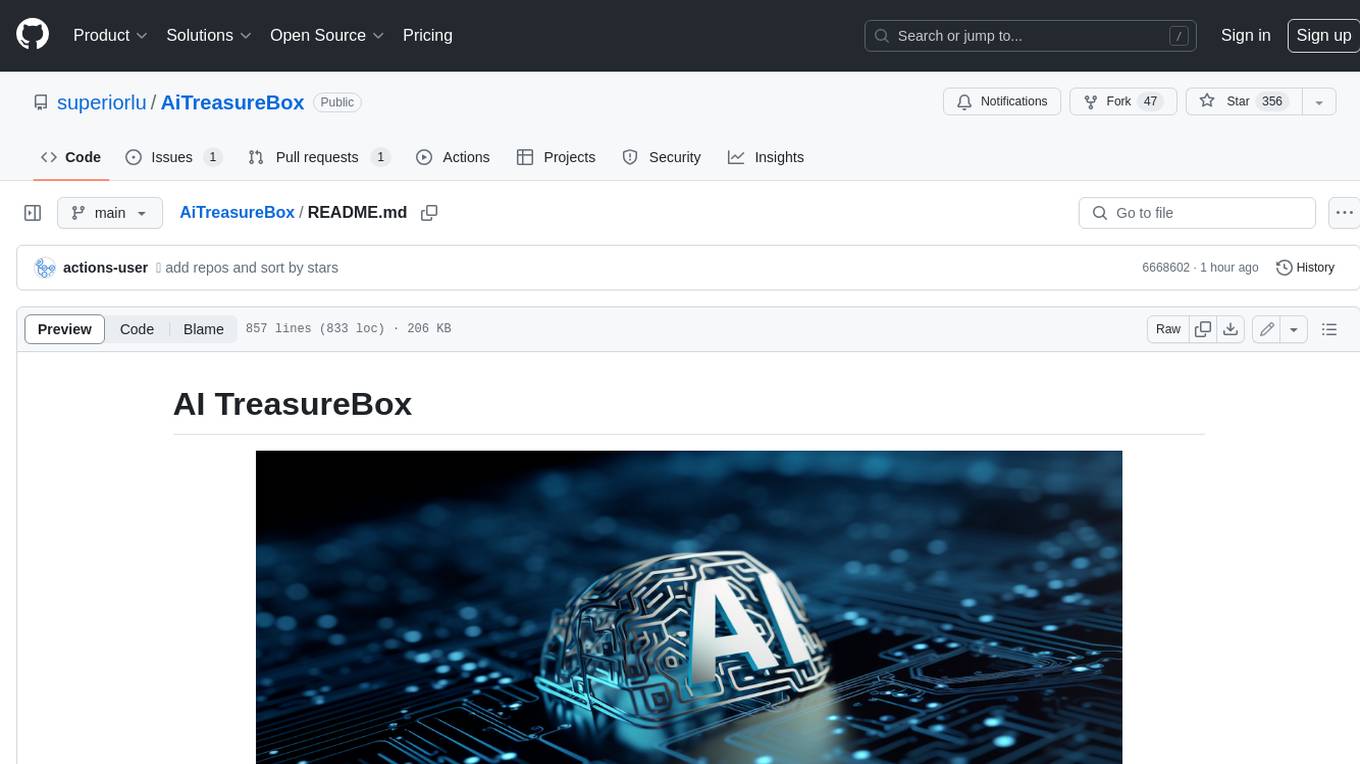
AiTreasureBox
AiTreasureBox is a versatile AI tool that provides a collection of pre-trained models and algorithms for various machine learning tasks. It simplifies the process of implementing AI solutions by offering ready-to-use components that can be easily integrated into projects. With AiTreasureBox, users can quickly prototype and deploy AI applications without the need for extensive knowledge in machine learning or deep learning. The tool covers a wide range of tasks such as image classification, text generation, sentiment analysis, object detection, and more. It is designed to be user-friendly and accessible to both beginners and experienced developers, making AI development more efficient and accessible to a wider audience.
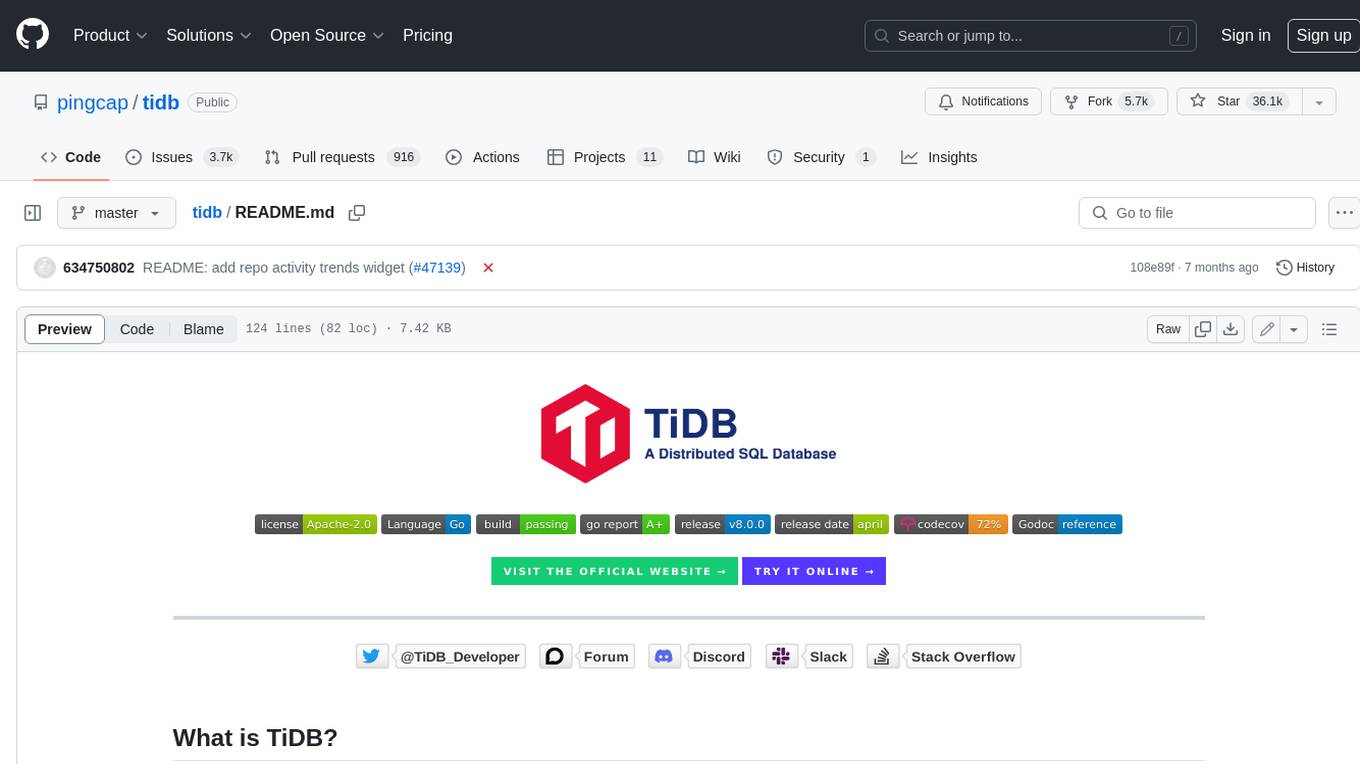
tidb
TiDB is an open-source distributed SQL database that supports Hybrid Transactional and Analytical Processing (HTAP) workloads. It is MySQL compatible and features horizontal scalability, strong consistency, and high availability.
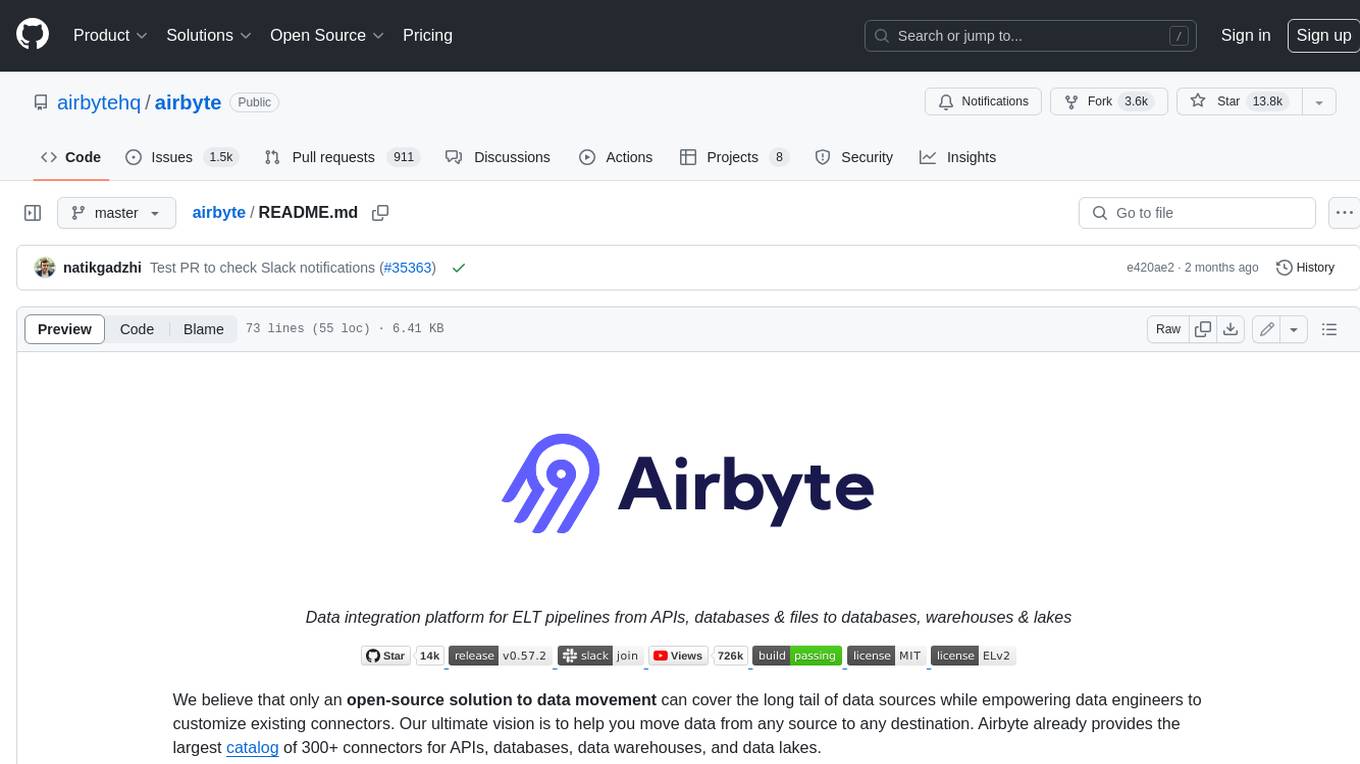
airbyte
Airbyte is an open-source data integration platform that makes it easy to move data from any source to any destination. With Airbyte, you can build and manage data pipelines without writing any code. Airbyte provides a library of pre-built connectors that make it easy to connect to popular data sources and destinations. You can also create your own connectors using Airbyte's no-code Connector Builder or low-code CDK. Airbyte is used by data engineers and analysts at companies of all sizes to build and manage their data pipelines.
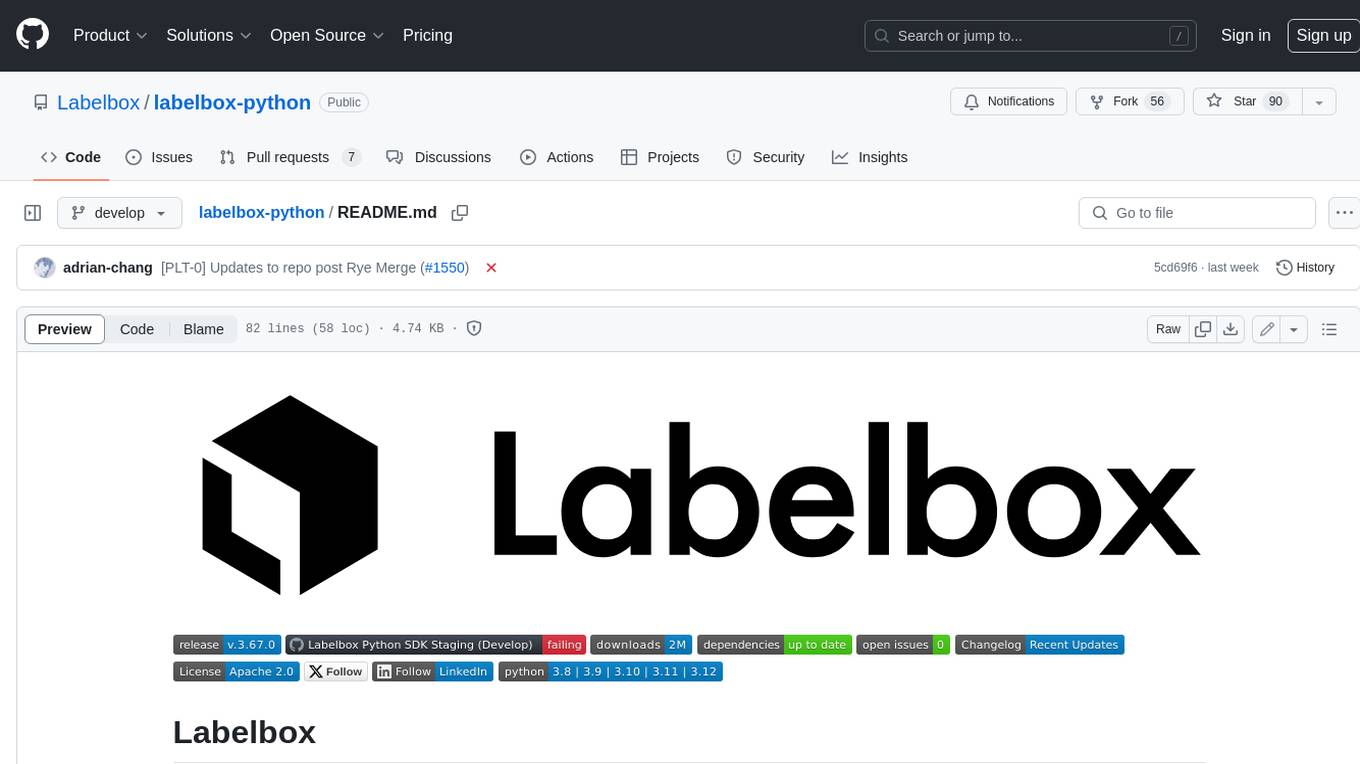
labelbox-python
Labelbox is a data-centric AI platform for enterprises to develop, optimize, and use AI to solve problems and power new products and services. Enterprises use Labelbox to curate data, generate high-quality human feedback data for computer vision and LLMs, evaluate model performance, and automate tasks by combining AI and human-centric workflows. The academic & research community uses Labelbox for cutting-edge AI research.