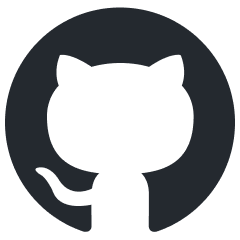
MiniCPM
MiniCPM3-4B: An edge-side LLM that surpasses GPT-3.5-Turbo.
Stars: 6999
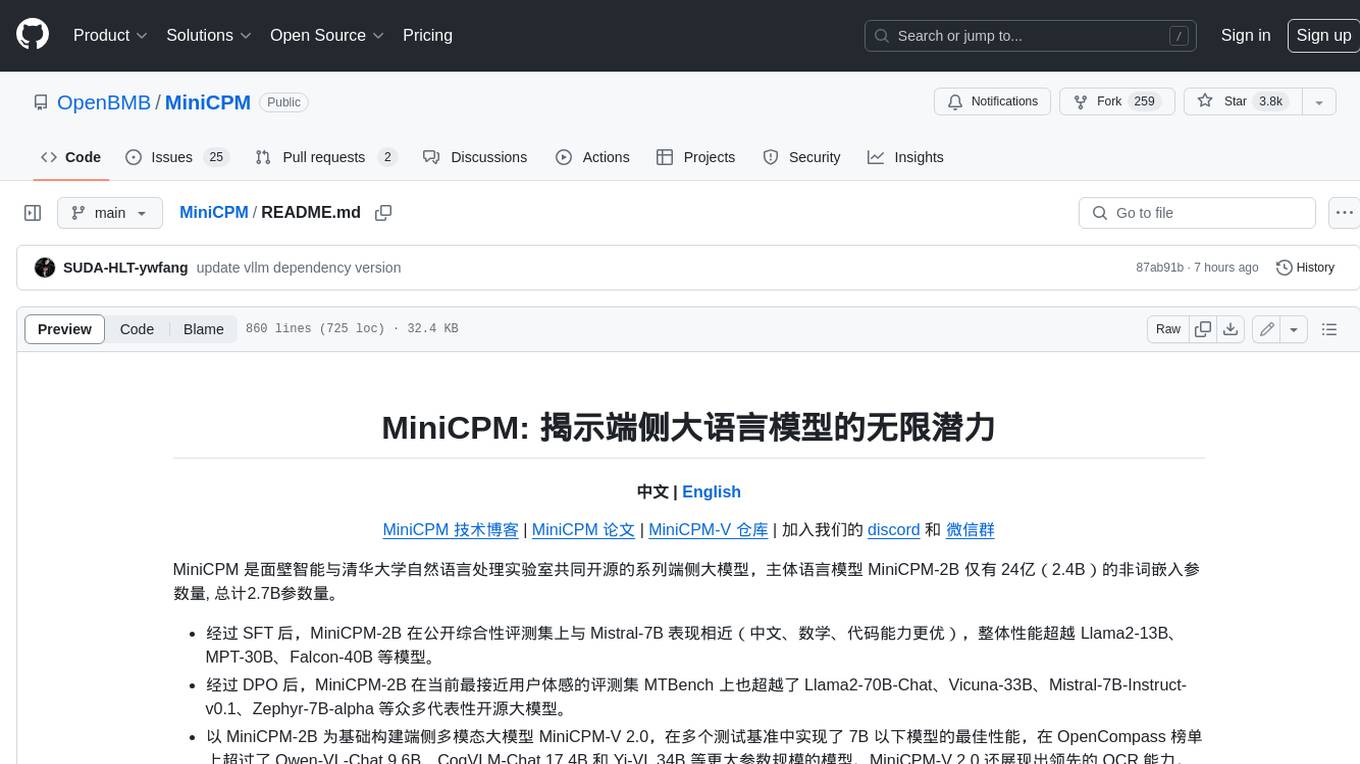
MiniCPM is a series of open-source large models on the client side jointly developed by Face Intelligence and Tsinghua University Natural Language Processing Laboratory. The main language model MiniCPM-2B has only 2.4 billion (2.4B) non-word embedding parameters, with a total of 2.7B parameters. - After SFT, MiniCPM-2B performs similarly to Mistral-7B on public comprehensive evaluation sets (better in Chinese, mathematics, and code capabilities), and outperforms models such as Llama2-13B, MPT-30B, and Falcon-40B overall. - After DPO, MiniCPM-2B also surpasses many representative open-source large models such as Llama2-70B-Chat, Vicuna-33B, Mistral-7B-Instruct-v0.1, and Zephyr-7B-alpha on the current evaluation set MTBench, which is closest to the user experience. - Based on MiniCPM-2B, a multi-modal large model MiniCPM-V 2.0 on the client side is constructed, which achieves the best performance of models below 7B in multiple test benchmarks, and surpasses larger parameter scale models such as Qwen-VL-Chat 9.6B, CogVLM-Chat 17.4B, and Yi-VL 34B on the OpenCompass leaderboard. MiniCPM-V 2.0 also demonstrates leading OCR capabilities, approaching Gemini Pro in scene text recognition capabilities. - After Int4 quantization, MiniCPM can be deployed and inferred on mobile phones, with a streaming output speed slightly higher than human speech speed. MiniCPM-V also directly runs through the deployment of multi-modal large models on mobile phones. - A single 1080/2080 can efficiently fine-tune parameters, and a single 3090/4090 can fully fine-tune parameters. A single machine can continuously train MiniCPM, and the secondary development cost is relatively low.
README:
中文 | English
MiniCPM 技术博客 | MiniCPM 知识库 | MiniCPM 论文 | MiniCPM-V 仓库 | 加入我们的 discord 和 微信群
- [2024.09.28] LLMxMapReduce 开源,支持MiniCPM3-4B,理论上支持无限长文本输入!
- [2024.09.18] SGLang 已经支持 MiniCPM3-4B (推荐使用)!由于 SGLang v0.3 对 MiniCPM3 中使用的 MLA 结构进行了推理优化,吞吐量相比于 vLLM 提高 70%![用法]
- [2024.09.16] llama.cpp 已经官方支持 MiniCPM3-4B![GGUF模型|用法]
- [2024.09.05] 发布 MiniCPM3-4B!该模型的表现超越 Phi-3.5-mini-instruct 和 GPT-3.5-Turbo-0125,并且能够比肩 Llama3.1-8B-Instruct、Qwen2-7B-Instruct、GLM-4-9B-Chat 等多个 7B-9B 参数量的模型。
- [2024.07.09] MiniCPM-2B 已经支持使用 SGLang 推理!
- [2024.07.05] 发布 MiniCPM-S-1B!该模型在保持下游任务性能无损的前提下,FFN 层实现了 87.89% 的平均稀疏度,将 FFN FLOPs 降低了 84%。
- [2024.04.11] 发布 MiniCPM-2B-128k、MiniCPM-MoE-8x2B 和 MiniCPM-1B!点击这里查看技术博客。
- [2024.03.16] MiniCPM-2B 的 30 余个中间检查点开放了!HuggingFace链接
- [2024.02.01] 发布 MiniCPM-2B!该模型在公开评测集上与 Mistral-7B 表现相近(中文、数学、代码能力更优),整体性能超越 Llama2-13B、MPT-30B、Falcon-40B 等模型。
注: 更多模型版本见这里。
MiniCPM 3.0 是一个 4B 参数量的语言模型,相比 MiniCPM1.0/2.0,功能更加全面,综合能力大幅提升,多数评测集上的效果比肩甚至超越众多 7B-9B 模型。
- 支持工具调用🛠️(Function Calling)和代码解释器💻(Code Interpreter):Berkeley Function Calling Leaderboard (BFCL) 上取得 9B 规模以下 SOTA,超越 GLM-4-9B-Chat、Qwen2-7B-Instruct。
- 超强的推理能力🧮:数学能力方面,MathBench 上的效果超越 GPT-3.5-Turbo 以及多个 7B-9B 模型。在非常具有挑战性的 LiveCodeBench 上,效果超越 Llama3.1-8B-Instruct。
- 出色的中英文指令遵循能力🤖:英文指令遵循 IFEval、中文指令遵循 FollowBench-zh 效果超越 GLM-4-9B-Chat、Qwen2-7B-Instruct。
- 长文本能力:原生支持 32k 上下文长度,32k 长度内大海捞针全绿。提出 LLMxMapReduce ,理论可处理的上下文长度达到 +∞,在综合性长文本评测基准 InfiniteBench 平均得分超越GPT-4、KimiChat等标杆模型。
- RAG能力:我们发布了 MiniCPM RAG 套件。基于 MiniCPM 系列模型的 MiniCPM-Embedding、MiniCPM-Reranker 在中文、中英跨语言检索测试中取得 SOTA 表现;针对 RAG 场景的 MiniCPM3-RAG-LoRA 在开放域问答等多项任务上超越 Llama3-8B、Baichuan2-13B 等模型。
评测集 | Qwen2-7B-Instruct | GLM-4-9B-Chat | Gemma2-9B-it | Llama3.1-8B-Instruct | GPT-3.5-Turbo-0125 | Phi-3.5-mini-Instruct(3.8B) | MiniCPM3-4B | |||||||
英文能力 | ||||||||||||||
MMLU | 70.5 | 72.4 | 72.6 | 69.4 | 69.2 | 68.4 | 67.2 | |||||||
BBH | 64.9 | 76.3 | 65.2 | 67.8 | 70.3 | 68.6 | 70.2 | |||||||
MT-Bench | 8.41 | 8.35 | 7.88 | 8.28 | 8.17 | 8.60 | 8.41 | |||||||
IFEVAL (Prompt Strict-Acc.) | 51.0 | 64.5 | 71.9 | 71.5 | 58.8 | 49.4 | 68.4 | |||||||
中文能力 | ||||||||||||||
CMMLU | 80.9 | 71.5 | 59.5 | 55.8 | 54.5 | 46.9 | 73.3 | |||||||
CEVAL | 77.2 | 75.6 | 56.7 | 55.2 | 52.8 | 46.1 | 73.6 | |||||||
AlignBench v1.1 | 7.10 | 6.61 | 7.10 | 5.68 | 5.82 | 5.73 | 6.74 | |||||||
FollowBench-zh (SSR) | 63.0 | 56.4 | 57.0 | 50.6 | 64.6 | 58.1 | 66.8 | |||||||
数学能力 | ||||||||||||||
MATH | 49.6 | 50.6 | 46.0 | 51.9 | 41.8 | 46.4 | 46.6 | |||||||
GSM8K | 82.3 | 79.6 | 79.7 | 84.5 | 76.4 | 82.7 | 81.1 | |||||||
MathBench | 63.4 | 59.4 | 45.8 | 54.3 | 48.9 | 54.9 | 65.6 | |||||||
代码能力 | ||||||||||||||
HumanEval+ | 70.1 | 67.1 | 61.6 | 62.8 | 66.5 | 68.9 | 68.3 | |||||||
MBPP+ | 57.1 | 62.2 | 64.3 | 55.3 | 71.4 | 55.8 | 63.2 | |||||||
LiveCodeBench v3 | 22.2 | 20.2 | 19.2 | 20.4 | 24.0 | 19.6 | 22.6 | |||||||
工具调用能力 | ||||||||||||||
BFCL v2 | 71.6 | 70.1 | 19.2 | 73.3 | 75.4 | 48.4 | 76.0 | |||||||
综合能力 | ||||||||||||||
平均分 | 65.3 | 65.0 | 57.9 | 60.8 | 61.0 | 57.2 | 66.3 |
我们在 Berkeley Function Calling Leaderboard (BFCL) 上测试了模型的工具调用能力,MiniCPM3-4B 在该榜单上的表现超越了多个 7B-9B 参数量的模型,优于 GPT-3.5-Turbo-0125。
模型 | 总体准确率 | AST Summary | Exec Summary | Irrelevance Detection | Relevance Detection |
MiniCPM3-4B | 76.03% | 68.55% | 85.54% | 53.71% | 90.24% |
Llama3.1-8B-Instruct | 73.28% | 64.61% | 86.48% | 43.12% | 85.37% |
Qwen2-7B-Instruct | 71.61% | 65.71% | 79.57% | 44.70% | 90.24% |
GLM-4-9B-Chat | 70.08% | 60.69% | 80.02% | 55.02% | 82.93% |
Phi-3.5-mini-instruct | 48.44% | 38.89% | 54.04% | 46.78% | 65.85% |
Gemma2-9B-it | 19.18% | 5.41% | 18.50% | 88.88% | 7.32% |
在 32k 的上下文长度进行大海捞针测试,结果如下图:
同时我们提出LLMxMapReduce,利用分治的策略,理论上可以处理无限长度的文本。我们在InfiniteBench上测试了模型的长文本处理能力,在LLMxMapReduce框架的加持下,MiniCPM3-4B在这个榜单的平均得分能够超越 GPT-4、KimiChat 等标杆模型。
Context length | Qwen2-70b | Kimi-Chat(2024.06) | GPT-4 (From InfiniteBench) | MiniCPM 3.0 x MR | Qwen2-70b x MR | Llama3-70bx MR | |
---|---|---|---|---|---|---|---|
Math.Find | 87.9k | 59.71% | 18.57% | 60.00% | 83.43% | 54.29% | 91.43% |
Retrieve.KV | 89.9k | 29.00% | 69.20% | 89.00% | 93.80% | 98.80% | 98.89% |
En.Dia | 103.6K | 23.00% | 23.00% | 7.50% | 12.50% | 46.50% | 17.50% |
Code.Debug | 114.7k | 45.43% | 38.32% | 54.31% | 25.63% | 54.82% | 62.94% |
Retrieve.Number | 122.4k | 100.00% | 97.45% | 100.00% | 99.32% | 100.00% | 99.79% |
Retrieve.PassKey | 122.4k | 100.00% | 99.32% | 100.00% | 98.81% | 100.00% | 100.00% |
En.Sum | 171.5K | 31.85% | 29.94% | 14.73% | 25.89% | 32.39% | 30.63% |
En.MC | 184.4k | 81.66% | 79.91% | 68.12% | 66.38% | 83.84% | 82.10% |
En.QA | 192.6k | 21.97% | 18.80% | 22.44% | 28.39% | 23.13% | 34.70% |
Zh.QA | 2068.6k | 21.40% | 19.84% | 25.96% | 23.66% | 19.10% | N/A |
avg w/o Zh.QA | / | 51.92% | 52.96% | 55.33% | 59.29% | 64.98% | 68.64% |
avg | / | 48.86% | 49.65% | 52.39% | 55.55% | 60.39% | N/A |
from transformers import AutoModelForCausalLM, AutoTokenizer
import torch
torch.manual_seed(0)
path = 'openbmb/MiniCPM3-4B'
tokenizer = AutoTokenizer.from_pretrained(path)
model = AutoModelForCausalLM.from_pretrained(path, torch_dtype=torch.bfloat16, device_map='cuda', trust_remote_code=True)
responds, history = model.chat(tokenizer, "请写一篇关于人工智能的文章,详细介绍人工智能的未来发展和隐患。", temperature=0.7, top_p=0.7)
print(responds)
- 安装
参考 SGLang 官方仓库,通过源码安装最新版本。
- 启动推理服务
python -m sglang.launch_server --model openbmb/MiniCPM3-4B --trust-remote-code --port 30000 --chat-template chatml
- 使用示例
from sglang import function, system, user, assistant, gen, set_default_backend, RuntimeEndpoint
@function
def multi_turn_question(s, question_1, question_2):
s += user(question_1)
s += assistant(gen("answer_1", max_tokens=1024))
s += user(question_2)
s += assistant(gen("answer_2", max_tokens=1024))
set_default_backend(RuntimeEndpoint("http://localhost:30000"))
state = multi_turn_question.run(
question_1="介绍一下人工智能",
question_2="写一篇关于它的文章",
)
for m in state.messages():
print(m["role"], ":", m["content"])
- 安装 vllm
pip install "vllm>=0.6.2"
- 推理
from transformers import AutoTokenizer from vllm import LLM, SamplingParams model_name = "openbmb/MiniCPM3-4B" prompt = [{"role": "user", "content": "请写一篇关于人工智能的文章,详细介绍人工智能的未来发展和隐患。"}] tokenizer = AutoTokenizer.from_pretrained(model_name, trust_remote_code=True) input_text = tokenizer.apply_chat_template(prompt, tokenize=False, add_generation_prompt=True) llm = LLM(model=model_name, trust_remote_code=True, tensor_parallel_size=1 ) sampling_params = SamplingParams(top_p=0.7, temperature=0.7, max_tokens=1024) outputs = llm.generate(prompts=input_text, sampling_params=sampling_params) print(outputs[0].outputs[0].text)
我们提供了 MiniCPM3 的 GGUF 版本,可以直接使用 llama.cpp 推理。
- 安装 llama.cpp
git clone https://github.com/ggerganov/llama.cpp cd llama.cpp make
- 推理
./llama-cli -c 1024 -m minicpm3-4b-fp16.gguf -n 1024 --top-p 0.7 --temp 0.7 --prompt "<|im_start|>user\n请写一篇关于人工智能的文章,详细介绍人工智能的未来发展和隐患。<|im_end|>\n<|im_start|>assistant\n"
目前模型微调支持 LLaMA-Factory,使用方法参考 LLaMA-Factory 微调。
对于以下进阶功能,我们的样例代码中使用 vLLM 进行推理。
我们提供了使用 MiniCPM3 调用工具的示例代码:
cd demo/minicpm3/function_call
python function_call.py
如果你想启动一个能够调用工具的推理服务,使用以下代码:
cd demo/minicpm3/function_call
pip install -r requirements.txt
python openai_api_server.py \
--model openbmb/MiniCPM3-4B \
--served-model-name MiniCPM3-4B \
--chat-template chatml.jinja \
--dtype auto \
--api-key token-abc123 \
--tensor-parallel-size 1 \
--trust-remote-code
下面是一个调用搜索工具回答问题的演示:
我们提供了一个 MiniCPM3 使用代码解释器的示例代码:
cd demo/minicpm3/code_interpreter
pip install -r requirements.txt
python code_interpreter.py openbmb/MiniCPM3-4B
下面是一个使用代码解释器生成二维码的演示:
查看 MiniCPM 2.0 的详细信息
MiniCPM 2.0 系列模型对 MiniCPM 进行了多个维度的升级,包括以下模型版本:
- MiniCPM-2B-128k:将 MiniCPM-2B 的上下文长度从 4k 扩展至 128k,在 InfiniteBench 测试集上优于 ChatGLM3-6B-128k、Yi-6B-200k 等更大参数量的模型。
- MiniCPM-MoE-8x2B:基于 MiniCPM-2B 进行 MoE 扩展,综合表现相比于 MiniCPM-2B 平均提高 4.5 个百分点。
- MiniCPM-1B:相比于 MiniCPM-2B 成本下降 60%,综合表现仍然优于 LLaMA2-13B。
- MiniCPM-S-1B:在保持下游任务性能无损的前提下,FFN 层实现了 87.89% 的平均稀疏度,将 FFN FLOPs 降低了 84%。结合 PowerInfer 推理框架,解码速度提升约 2.8 倍。
Model | avg | avg w/o code&math | passkey | number_string | kv_retrieval | longbook_choice_eng | longbook_qa_chn | longbook_qa_eng | longbook_sum_eng | longdialogue_qa_eng | math_calc | math_find | code_debug | code_run |
---|---|---|---|---|---|---|---|---|---|---|---|---|---|---|
LWM-Text-128k | 24.45 | 33.62 | 100 | 97.8 | 0.6 | 28.82 | 15.93 | 14.31 | 9.99 | 1.5 | 0 | 3.43 | 20.05 | 1 |
Yarn-Mistral-7b-128k | 19.84 | 27.36 | 92.71 | 0 | 27.95 | 15.49 | 9.55 | 9.06 | 7.5 | 0 | 17.14 | 0.76 | 1.25 | |
Mistral-7B-Instruct-v0.2(ABF 1000w) | 27.75 | 36.9 | 100 | 78.98 | 3.6 | 37.12 | 11.74 | 17.37 | 21.12 | 9.5 | 0 | 29.43 | 17.51 | 0 |
Yi-6B-200k | 22.15 | 32.54 | 100 | 94.92 | 0 | 36.68 | 15.07 | 9.2 | 0.92 | 3.5 | 0 | 4.29 | 0.51 | 0.75 |
chatglm3-6b-128k | 25.58 | 36.57 | 89.93 | 99.66 | 5.2 | 46.29 | 10.7 | 8.38 | 25.91 | 6.5 | 0 | 8 | 5.33 | 1 |
MiniCPM-2.4B-128k | 27.32 | 37.68 | 98.31 | 99.83 | 9 | 29.69 | 23.06 | 16.33 | 15.73 | 9.5 | 0 | 4.29 | 22.08 | 0 |
Model | BBH | MMLU | CEval | CMMLU | HumanEval | MBPP† | GSM8K | MATH |
---|---|---|---|---|---|---|---|---|
Llama2-34B* | 44.1 | 62.6 | - | - | 22.6 | 33.0 | 42.2 | 6.24 |
Mistral-7B-Instruct-v0.2 | 39.81 | 60.51 | 42.55 | 41.92 | 36.59 | 39.63 | 40.49 | 4.95 |
Gemma-7B* | 55.1 | 64.3 | - | - | 32.3 | 44.4 | 46.4 | 24.3 |
Qwen1.5-7B* | 40.2 | 61 | 74.1 | 73.1 | 36 | 37.4 | 62.5 | 20.3 |
Deepseek-MoE(16B)* | - | 45.0 | 40.6 | 42.5 | 26.8 | 39.2 | 18.8 | 4.3 |
MiniCPM-2.4B | 36.87 | 53.46 | 51.13 | 51.07 | 50.00 | 35.93 | 53.83 | 10.24 |
MiniCPM-MoE-8x2B | 39.22 | 58.90 | 58.11 | 58.80 | 55.49 | 41.68 | 61.56 | 10.52 |
注:* 表示结果取自技术报告。† 表示评测集为MBPP全集。
- 代码生成:在 HumanEval(0-shot)和 MBPP(3-shot)上的平均 pass@1 得分。
- 常识推理:在 PIQA、SIQA、HellaSwag、WinoGrande 和 COPA 上的平均 0-shot 准确率。
- 阅读理解:在 BoolQ、LAMBADA 和 TyDi QA 上的平均 0-shot 准确率。
其他测试集:我们报告在GSM8K(8-shot)、MMLU(5-shot)、BBH(3-shot)和 AGI-Eval(0-shot)上的平均准确率。
Setting | Average Sparsity |
Average Performance |
Code Generation |
Commonsense Reasoning |
Reading Comprehension |
GSM8K | MMLU | BBH | AGI Eval |
---|---|---|---|---|---|---|---|---|---|
LLaMA2-7B | - | 37.96 | 16.37 | 69.59 | 61.87 | 12.96 | 44.45 | 32.96 | 27.53 |
ReluLLaMA-7B | 66.98 | 37.62 | 15.85 | 69.64 | 70.54 | 5.84 | 38.64 | 35.07 | 27.73 |
ProSparse-7B* | 88.11 | 38.31 | 19.47 | 66.29 | 63.33 | 12.74 | 45.21 | 33.59 | 27.55 |
ProSparse-7B | 89.32 | 38.46 | 19.42 | 66.27 | 63.50 | 12.13 | 45.48 | 34.99 | 27.46 |
LLaMA2-13B | - | 44.06 | 20.19 | 72.58 | 71.55 | 22.21 | 54.69 | 37.89 | 29.33 |
ReluLLaMA-13B | 71.56 | 42.74 | 20.19 | 70.44 | 73.29 | 18.50 | 50.58 | 37.97 | 28.22 |
ProSparse-13B* | 87.97 | 45.07 | 29.03 | 69.75 | 67.54 | 25.40 | 54.78 | 40.20 | 28.76 |
ProSparse-13B | 88.80 | 44.90 | 28.42 | 69.76 | 66.91 | 26.31 | 54.35 | 39.90 | 28.67 |
MiniCPM-1B | - | 44.44 | 36.85 | 63.67 | 60.90 | 35.48 | 50.44 | 35.03 | 28.71 |
MiniCPM-S-1B* | 86.25 | 44.72 | 41.38 | 64.55 | 60.69 | 34.72 | 49.36 | 34.04 | 28.27 |
MiniCPM-S-1B | 87.89 | 44.72 | 42.04 | 64.37 | 60.73 | 34.57 | 49.51 | 34.08 | 27.77 |
注:
- ReluLLaMA-7B 和 ReluLLaMA-13B 的下载链接分别是 7B and 13B。"ProSparse-7B*"、"ProSparse-13B*" 和 "MiniCPM-S-1B*" 代表没有激活阈值偏移的 ProSparse 版本。
- 对于 PIQA、SIQA、HellaSwag、WinoGrande、COPA、BoolQ、LAMBADA、TyDi QA 和 AGI-Eval,我们根据各个选项的 PPL 来进行答案选择。对于 GSM8K、MMLU 和 BBH,我们直接生成答案。
参考 MiniCPM 1.0 中的模型推理部分。
针对 MiniCPM-S-1B 模型,我们可以使用 Powerinfer 进行推理加速,使用方法如下:
- 保证cmake版本3.17以上,如果已经安装过,则跳过此步骤
# 下载安装包
sudo wget https://cmake.org/files/v3.23/cmake-3.23.0.tar.gz
# 解压安装包
sudo tar -zxvf cmake-3.23.0.tar.gz
# 配置安装环境
sudo ./configure
sudo make -j8
# 编译安装
sudo make install
# 查看安装后版本
cmake --version
# 返回版本号则安装成功
#cmake version 3.23.0
- 安装powerinfer:
git clone https://github.com/SJTU-IPADS/PowerInfer
cd PowerInfer
pip install -r requirements.txt # install Python helpers' dependencies
- cpu版本powerinfer编译,如果你的机器只有cpu,或者只想使用cpu进行推理,则运行以下命令:
cmake -S . -B build
cmake --build build --config Release
- gpu版本powerinfer编译,如果你的机器有gpu,则可以运行以下命令:
cmake -S . -B build -DLLAMA_CUBLAS=ON
cmake --build build --config Release
- 获取稀疏模型
git clone https://huggingface.co/openbmb/MiniCPM-S-1B-sft-gguf/tree/main
#or
git clone https://modelscope.cn/models/OpenBMB/MiniCPM-S-1B-sft-gguf
- 模型推理:
cd PowerInfer
# 以下是命令模版,output_token_count为最大输出tokens,thread_num 为线程数,prompt为输入prompt字符
#./build/bin/main -m /PATH/TO/MODEL -n $output_token_count -t $thread_num -p $prompt
# 以下是示例
./build/bin/main -m /root/ld/ld_model_pretrain/1b-s-minicpm/MiniCPM-S-1B-sft.gguf -n 2048 -t 8 -p '<用户>hello,tell me a story please.<AI>'
查看 MiniCPM 1.0 的详细信息
MiniCPM-2B 语言模型有 24亿(2.4B)的非词嵌入参数量, 总计 2.7B 参数量。
- 经过 SFT 后,MiniCPM-2B 在公开评测集上与 Mistral-7B 表现相近(中文、数学、代码能力更优),整体性能超越 Llama2-13B、MPT-30B、Falcon-40B 等模型。
- 经过 DPO 后,MiniCPM-2B 在 MTBench 上也超越了 Llama2-70B-Chat、Vicuna-33B、Mistral-7B-Instruct-v0.1、Zephyr-7B-alpha 等众多代表性开源大模型。
注意:为了保证在学术研究用途上模型的通用性,我们未对 MiniCPM-2B 进行任何身份认同训练。同时由于我们用 ShareGPT 开源语料作为部分训练数据,模型可能会输出类似 GPT 系列模型的身份认同信息。
- 由于大模型评测难以统一,且大量评测也没有公开的prompt和测试代码,对于具体评测方式,我们只能尽量做到适合各类模型。
- 整体而言,我们测试时采用统一的prompt输入,并按照各模型对应的模板进行输入调整。
-
评测脚本及prompt已开源在我们的Github仓库中,也欢迎更多开发者来不断改进我们的评测方式。
- 文本评测部分,采用了我们的开源大模型能力评测框架UltraEval。以下为开源模型复现流程:
- 安装UltraEval
git clone https://github.com/OpenBMB/UltraEval.git cd UltraEval pip install -e .
- 下载相关数据并解压处理
wget -O RawData.zip "https://cloud.tsinghua.edu.cn/f/71b5232264ae4833a4d0/?dl=1" unzip RawData.zip python data_process.py
- 执行评测脚本(提供了模板,可自定义)
bash run_eval.sh
- 安装UltraEval
- 文本评测部分,采用了我们的开源大模型能力评测框架UltraEval。以下为开源模型复现流程:
- 因为MiniCPM采用Mup的结构,与现有模型在具体计算上有细微差别,我们是基于vllm=0.2.2版本进行了我们模型的实现。
- 对于非MiniCPM模型,我们采用了vllm=0.2.7的最新版本进行推理。
- 对于QA任务(选择题任务),我们选用两种方式进行测试:
- PPL:将选项作为题目生成的延续,并根据各个选项的PPL来进行答案选择;
- 第二种是直接生成答案选项。
- 对于不同模型,这两种方式得到的结果差异较大。MiniCPM两种模式上的结果较为接近,而Mistral-7B-v0.1等模型在PPL上表现较好,直接生成上效果较差。
- 在具体评测时,我们以两种评测方式得分的最高者为最终结果,以此保证对比的公平性(以下表格中*号表示采用PPL)。
越级比较:
模型 | 平均分 | 英文均分 | 中文均分 | C-Eval | CMMLU | MMLU | HumanEval | MBPP | GSM8K | MATH | BBH | ARC-E | ARC-C | HellaSwag |
---|---|---|---|---|---|---|---|---|---|---|---|---|---|---|
Llama2-7B | 35.40 | 36.21 | 31.765 | 32.42 | 31.11 | 44.32 | 12.2 | 27.17 | 13.57 | 1.8 | 33.23 | 75.25 | 42.75 | 75.62* |
Qwen-7B | 49.46 | 47.19 | 59.655 | 58.96 | 60.35 | 57.65 | 17.07 | 42.15 | 41.24 | 5.34 | 37.75 | 83.42 | 64.76 | 75.32* |
Deepseek-7B | 39.96 | 39.15 | 43.64 | 42.82 | 44.45 | 47.82 | 20.12 | 41.45 | 15.85 | 1.53 | 33.38 | 74.58* | 42.15* | 75.45* |
Mistral-7B | 48.97 | 49.96 | 44.54 | 46.12 | 42.96 | 62.69 | 27.44 | 45.2 | 33.13 | 5.0 | 41.06 | 83.92 | 70.73 | 80.43* |
Llama2-13B | 41.48 | 42.44 | 37.19 | 37.32 | 37.06 | 54.71 | 17.07 | 32.55 | 21.15 | 2.25 | 37.92 | 78.87* | 58.19 | 79.23* |
MPT-30B | 38.17 | 39.82 | 30.72 | 29.34 | 32.09 | 46.56 | 21.95 | 35.36 | 10.31 | 1.56 | 38.22 | 78.66* | 46.08* | 79.72* |
Falcon-40B | 43.62 | 44.21 | 40.93 | 40.29 | 41.57 | 53.53 | 24.39 | 36.53 | 22.44 | 1.92 | 36.24 | 81.94* | 57.68 | 83.26* |
MiniCPM-2B | 52.33 | 52.6 | 51.1 | 51.13 | 51.07 | 53.46 | 50.00 | 47.31 | 53.83 | 10.24 | 36.87 | 85.44 | 68.00 | 68.25 |
同级比较:
模型 | 平均分 | 英文均分 | 中文均分 | C-Eval | CMMLU | MMLU | HumanEval | MBPP | GSM8K | MATH | BBH | ARC-E | ARC-C | HellaSwag |
---|---|---|---|---|---|---|---|---|---|---|---|---|---|---|
TinyLlama-1.1B | 25.36 | 25.55 | 24.525 | 25.02 | 24.03 | 24.3 | 6.71 | 19.91 | 2.27 | 0.74 | 28.78 | 60.77* | 28.15* | 58.33* |
Qwen-1.8B | 34.72 | 31.87 | 47.57 | 49.81 | 45.32 | 43.37 | 7.93 | 17.80 | 19.26 | 2.42 | 29.07 | 63.97* | 43.69 | 59.28* |
Gemini Nano-3B | - | - | - | - | - | - | - | 27.2(report) | 22.8(report) | - | 42.4(report) | - | - | - |
StableLM-Zephyr-3B | 43.46 | 46.31 | 30.62 | 30.34 | 30.89 | 45.9 | 35.37 | 31.85 | 52.54 | 12.49 | 37.68 | 73.78 | 55.38 | 71.87* |
Phi-2-2B | 48.84 | 54.41 | 23.78 | 23.37 | 24.18 | 52.66 | 47.56 | 55.04 | 57.16 | 3.5 | 43.39 | 86.11 | 71.25 | 73.07* |
MiniCPM-2B | 52.33 | 52.6 | 51.10 | 51.13 | 51.07 | 53.46 | 50.00 | 47.31 | 53.83 | 10.24 | 36.87 | 85.44 | 68.00 | 68.25 |
Chat模型比较:
模型 | 平均分 | 英文均分 | 中文均分 | C-Eval | CMMLU | MMLU | HumanEval | MBPP | GSM8K | MATH | BBH | ARC-E | ARC-C | HellaSwag |
---|---|---|---|---|---|---|---|---|---|---|---|---|---|---|
ChatGLM2-6B | 37.98 | 35.17 | 50.63 | 52.05 | 49.21 | 45.77 | 10.37 | 9.38 | 22.74 | 5.96 | 32.6 | 74.45 | 56.82 | 58.48* |
Mistral-7B-Instruct-v0.1 | 44.36 | 45.89 | 37.51 | 38.06 | 36.96 | 53.56 | 29.27 | 39.34 | 28.73 | 3.48 | 39.52 | 81.61 | 63.99 | 73.47* |
Mistral-7B-Instruct-v0.2 | 50.91 | 52.83 | 42.235 | 42.55 | 41.92 | 60.51 | 36.59 | 48.95 | 40.49 | 4.95 | 39.81 | 86.28 | 73.38 | 84.55* |
Qwen-7B-Chat | 44.93 | 42.05 | 57.9 | 58.57 | 57.23 | 56.03 | 15.85 | 40.52 | 42.23 | 8.3 | 37.34 | 64.44* | 39.25* | 74.52* |
Yi-6B-Chat | 50.46 | 45.89 | 70.995 | 70.88 | 71.11 | 62.95 | 14.02 | 28.34 | 36.54 | 3.88 | 37.43 | 84.89 | 70.39 | 74.6* |
Baichuan2-7B-Chat | 44.68 | 42.74 | 53.39 | 53.28 | 53.5 | 53 | 21.34 | 32.32 | 25.25 | 6.32 | 37.46 | 79.63 | 60.15 | 69.23* |
Deepseek-7B-chat | 49.34 | 49.56 | 48.335 | 46.95 | 49.72 | 51.67 | 40.85 | 48.48 | 48.52 | 4.26 | 35.7 | 76.85 | 63.05 | 76.68* |
Llama2-7B-Chat | 38.16 | 39.17 | 33.59 | 34.54 | 32.64 | 47.64 | 14.02 | 27.4 | 21.15 | 2.08 | 35.54 | 74.28 | 54.78 | 75.65* |
MiniCPM-2B | 52.33 | 52.6 | 51.10 | 51.13 | 51.07 | 53.46 | 50.00 | 47.31 | 53.83 | 10.24 | 36.87 | 85.44 | 68.00 | 68.25 |
DPO后模型比较:
模型 | MT-bench |
---|---|
GPT-4-turbo | 9.32 |
GPT-3.5-turbo | 8.39 |
Mistral-8*7b-Instruct-v0.1 | 8.30 |
Claude-2.1 | 8.18 |
Zephyr-7B-beta | 7.34 |
MiniCPM-2B | 7.25 |
Vicuna-33B | 7.12 |
Zephyr-7B-alpha | 6.88 |
LLaMA-2-70B-chat | 6.86 |
Mistral-7B-Instruct-v0.1 | 6.84 |
MPT-34B-instruct | 6.39 |
- 使用如下命令启动基于Gradio的网页版demo:
# generation powered by vllm
python demo/minicpm/vllm_based_demo.py --model_path <vllmcpm_repo_path>
# generation powered by huggingface
python demo/minicpm/hf_based_demo.py --model_path <hf_repo_path>
安装transformers>=4.36.0
以及accelerate
后,运行以下代码:
from transformers import AutoModelForCausalLM, AutoTokenizer
import torch
torch.manual_seed(0)
path = 'openbmb/MiniCPM-2B-dpo-bf16'
tokenizer = AutoTokenizer.from_pretrained(path)
model = AutoModelForCausalLM.from_pretrained(path, torch_dtype=torch.bfloat16, device_map='cuda', trust_remote_code=True)
responds, history = model.chat(tokenizer, "山东省最高的山是哪座山, 它比黄山高还是矮?差距多少?", temperature=0.5, top_p=0.8, repetition_penalty=1.02)
print(responds)
我们将MiniCPM的模型权重转化成了Llama代码可以直接调用的格式,以便大家尝试:
import torch
from transformers import LlamaTokenizerFast, LlamaForCausalLM
model_path = "openbmb/MiniCPM-2B-dpo-bf16-llama-format"
tokenizer = LlamaTokenizerFast.from_pretrained(model_path)
model = LlamaForCausalLM.from_pretrained(model_path, torch_dtype=torch.bfloat16, device_map='cuda', trust_remote_code=True)
prompt="Now you act like a terminal situated within a beginner's C++ practice repository folder, please provide the output for the command: `ls -l`"
input_ids = tokenizer.encode("<用户>{}<AI>".format(prompt), return_tensors='pt', add_special_tokens=True).cuda()
responds = model.generate(input_ids, temperature=0.3, top_p=0.8, repetition_penalty=1.02, max_length=1024)
responds = tokenizer.decode(responds[0], skip_special_tokens=True)
print(responds)
安装 vLLM。
pip install "vllm>=0.4.1"
具体推理代码见这里。
安装 SGLang。
- 首先需要启动一个服务:
python -m sglang.launch_server --model-path openbmb/MiniCPM-2B-dpo-fp16 --trust-remote-code --port 30000
- 下面是一个推理代码的样例:
from sglang import function, gen, set_default_backend, RuntimeEndpoint
@function
def text_qa(s, question):
s += "<用户>" + question + "<AI>"
s += gen("answer", max_tokens=1024, temperature=0.7, top_p=0.7)
set_default_backend(RuntimeEndpoint("http://localhost:30000"))
state = text_qa.run(
question="What is the capital of China?",
)
print(state["answer"])
MiniCPM支持llama.cpp 、ollama、fastllm、mlx_lm推理。感谢@runfuture对llama.cpp和ollama的适配。
请参考 MiniCPM 知识库中的边端部署教程。
请参考 MiniCPM 知识库中的量化指南。
- 一张 1080/2080 可实现高效参数微调:代码
- mlx 微调:教程
- xtuner: MiniCPM高效率微调的不二选择
- LLaMA-Factory:MiniCPM微调一键式解决方案
- 本仓库中代码依照 Apache-2.0 协议开源
- MiniCPM 模型权重的使用则需要遵循 MiniCPM 模型商用许可协议。
- MiniCPM 模型权重对学术研究完全开放,在填写问卷进行登记后亦允许免费商业使用。
- 作为一个语言模型,MiniCPM 通过学习大量的文本来生成内容,但它无法理解、表达个人观点或价值判断,它所输出的任何内容都不代表模型开发者的观点和立场。
- 因此用户在使用 MiniCPM 生成的内容时,应自行负责对其进行评估和验证。
- 如果由于使用 MiniCPM 开源模型而导致的任何问题,包括但不限于数据安全问题、公共舆论风险,或模型被误导、滥用、传播或不当利用所带来的任何风险和问题,我们将不承担任何责任。
本项目由以下机构共同开发:
- 如果觉得MiniCPM有助于您的工作,请引用我们的论文
@article{hu2024minicpm,
title={MiniCPM: Unveiling the Potential of Small Language Models with Scalable Training Strategies},
author={Hu, Shengding and Tu, Yuge and Han, Xu and He, Chaoqun and Cui, Ganqu and Long, Xiang and Zheng, Zhi and Fang, Yewei and Huang, Yuxiang and Zhao, Weilin and others},
journal={arXiv preprint arXiv:2404.06395},
year={2024}
}
For Tasks:
Click tags to check more tools for each tasksFor Jobs:
Alternative AI tools for MiniCPM
Similar Open Source Tools
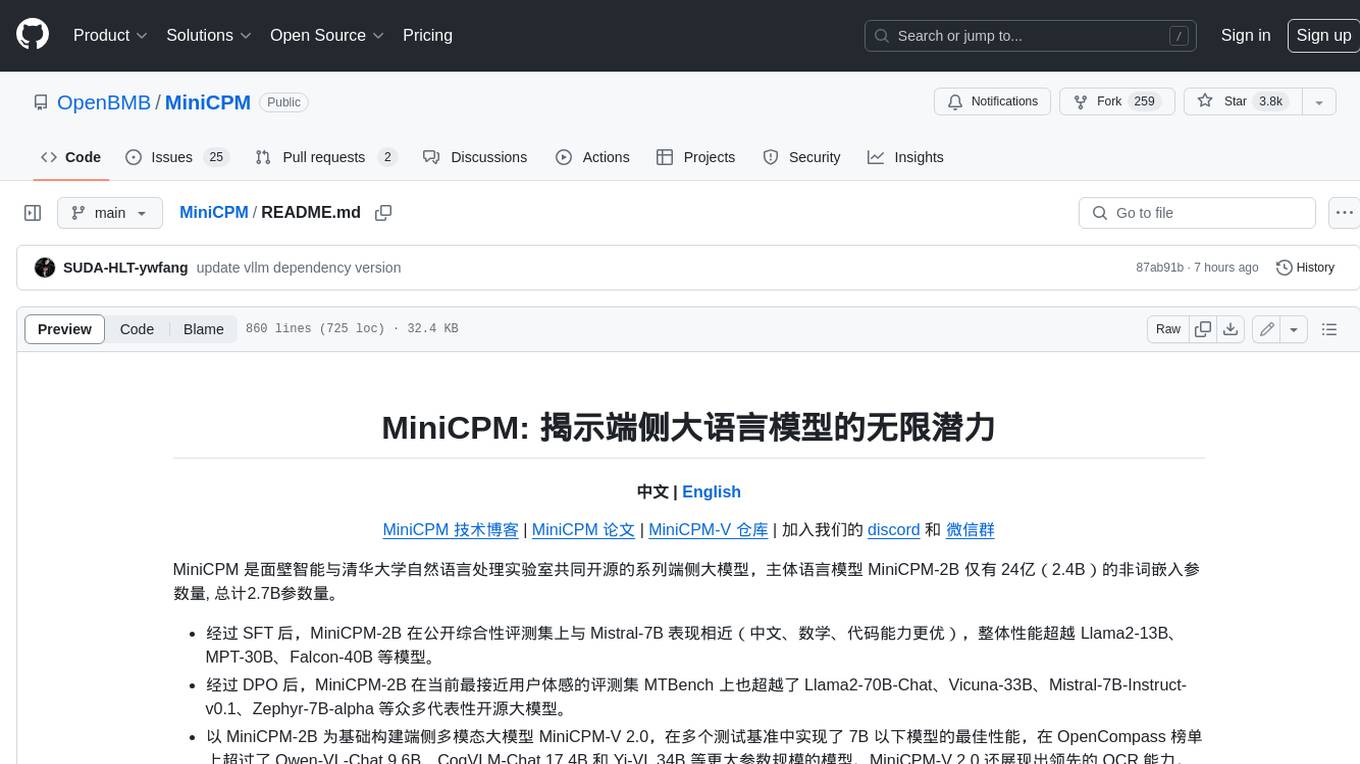
MiniCPM
MiniCPM is a series of open-source large models on the client side jointly developed by Face Intelligence and Tsinghua University Natural Language Processing Laboratory. The main language model MiniCPM-2B has only 2.4 billion (2.4B) non-word embedding parameters, with a total of 2.7B parameters. - After SFT, MiniCPM-2B performs similarly to Mistral-7B on public comprehensive evaluation sets (better in Chinese, mathematics, and code capabilities), and outperforms models such as Llama2-13B, MPT-30B, and Falcon-40B overall. - After DPO, MiniCPM-2B also surpasses many representative open-source large models such as Llama2-70B-Chat, Vicuna-33B, Mistral-7B-Instruct-v0.1, and Zephyr-7B-alpha on the current evaluation set MTBench, which is closest to the user experience. - Based on MiniCPM-2B, a multi-modal large model MiniCPM-V 2.0 on the client side is constructed, which achieves the best performance of models below 7B in multiple test benchmarks, and surpasses larger parameter scale models such as Qwen-VL-Chat 9.6B, CogVLM-Chat 17.4B, and Yi-VL 34B on the OpenCompass leaderboard. MiniCPM-V 2.0 also demonstrates leading OCR capabilities, approaching Gemini Pro in scene text recognition capabilities. - After Int4 quantization, MiniCPM can be deployed and inferred on mobile phones, with a streaming output speed slightly higher than human speech speed. MiniCPM-V also directly runs through the deployment of multi-modal large models on mobile phones. - A single 1080/2080 can efficiently fine-tune parameters, and a single 3090/4090 can fully fine-tune parameters. A single machine can continuously train MiniCPM, and the secondary development cost is relatively low.
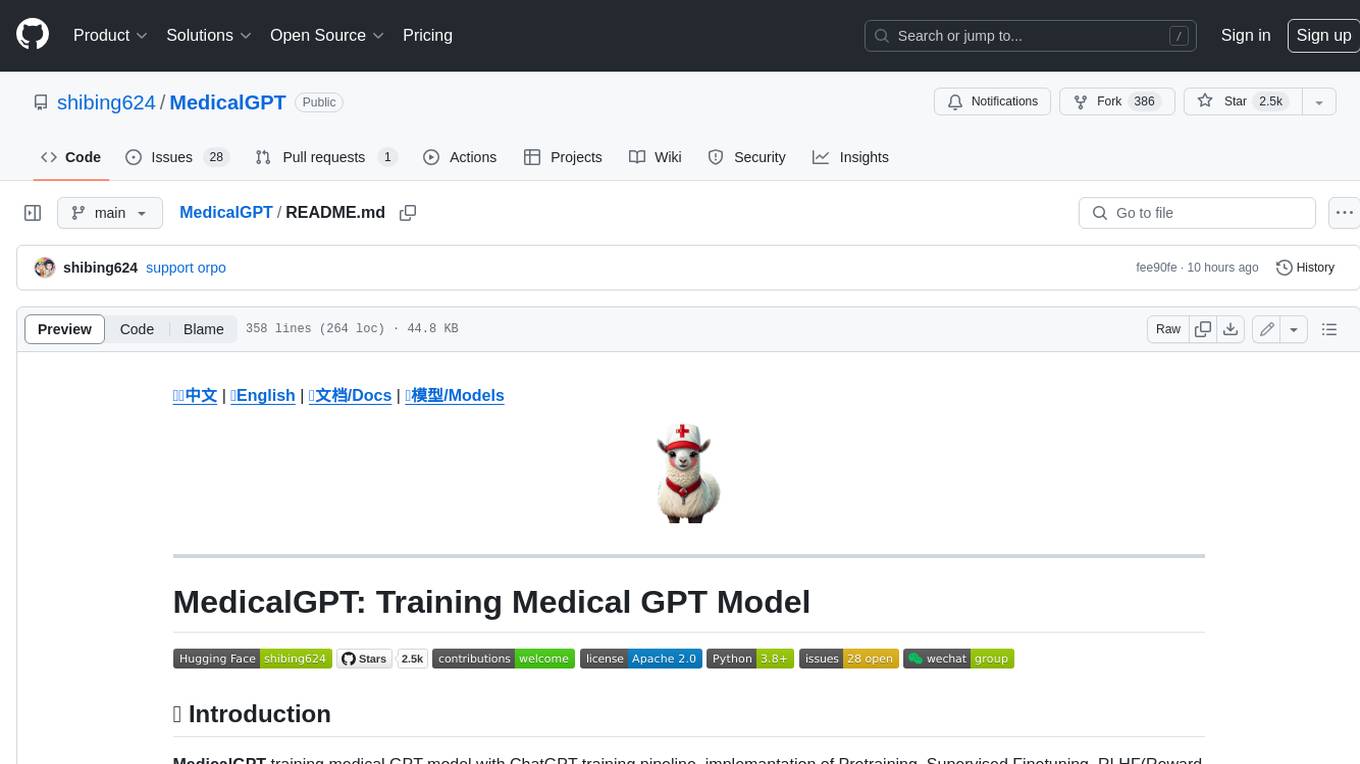
MedicalGPT
MedicalGPT is a training medical GPT model with ChatGPT training pipeline, implement of Pretraining, Supervised Finetuning, RLHF(Reward Modeling and Reinforcement Learning) and DPO(Direct Preference Optimization).
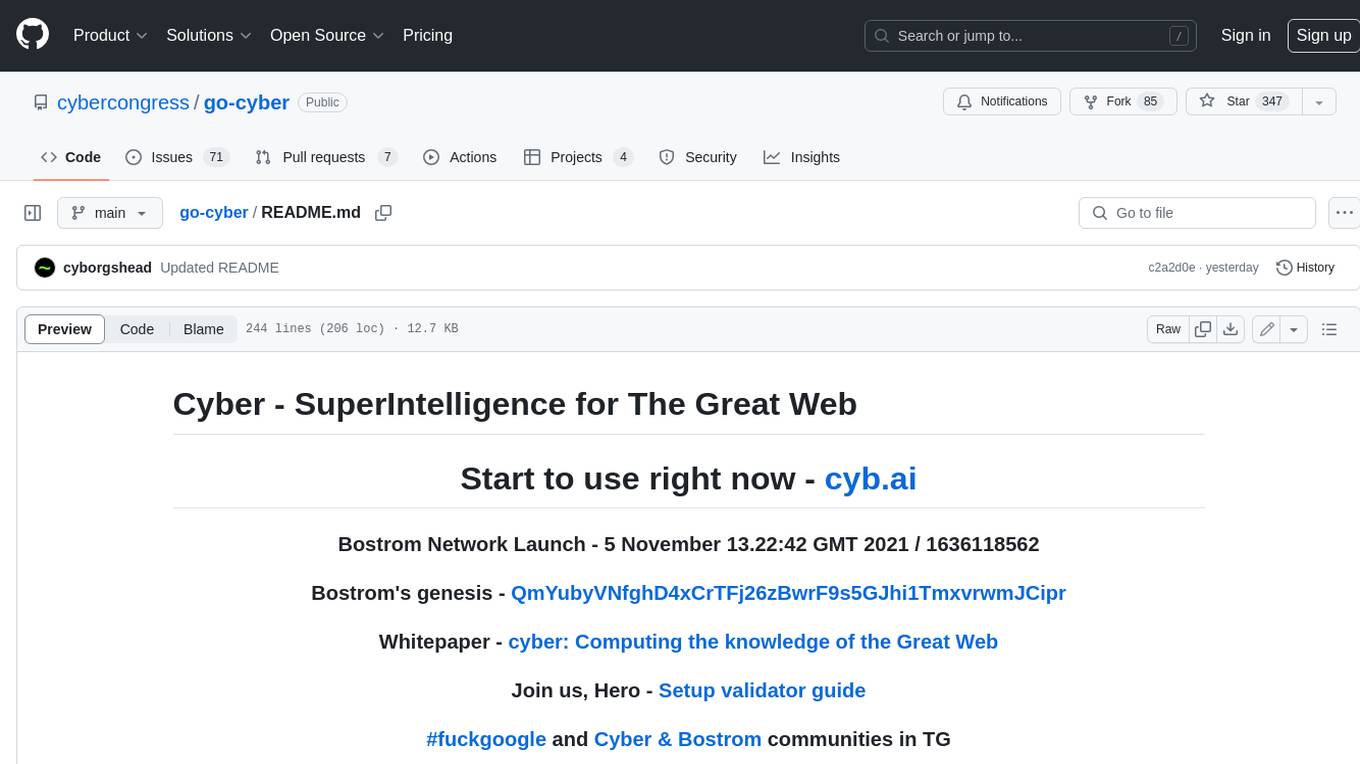
go-cyber
Cyber is a superintelligence protocol that aims to create a decentralized and censorship-resistant internet. It uses a novel consensus mechanism called CometBFT and a knowledge graph to store and process information. Cyber is designed to be scalable, secure, and efficient, and it has the potential to revolutionize the way we interact with the internet.
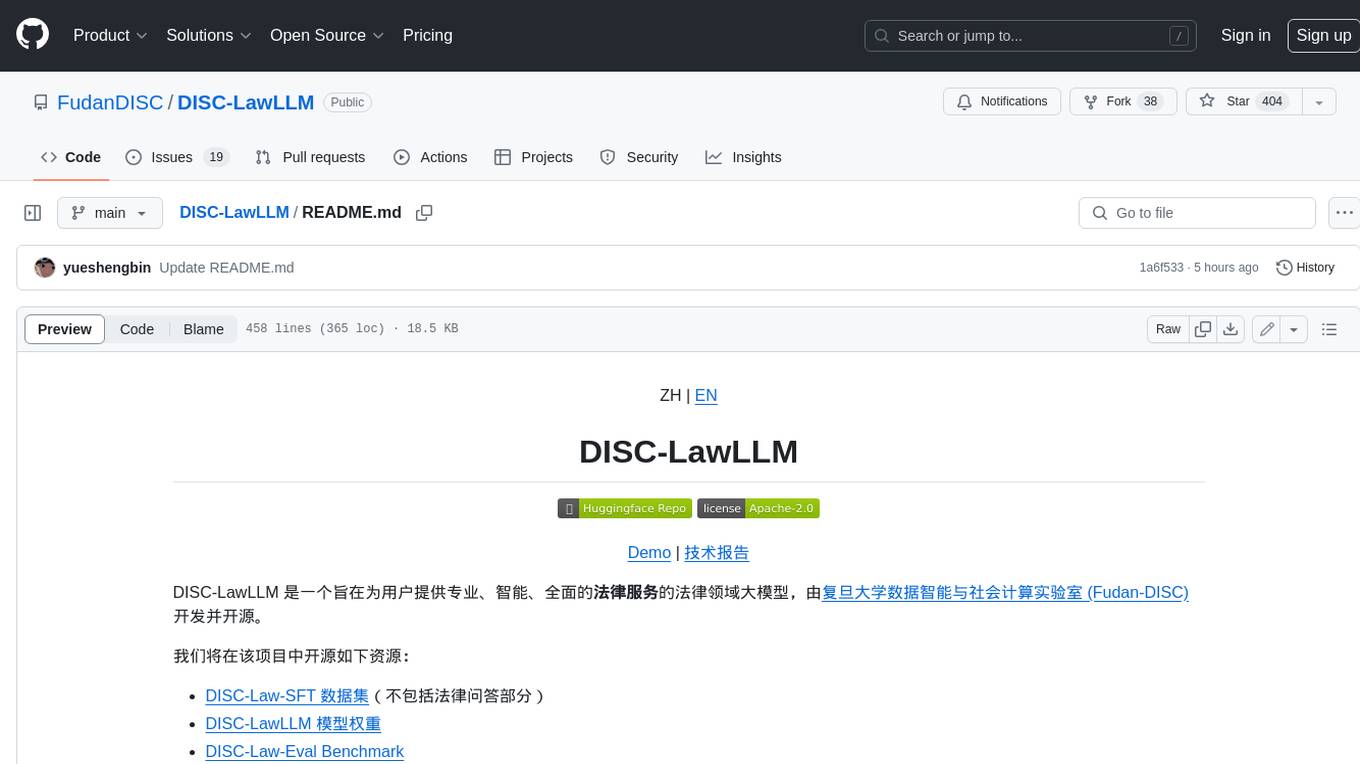
DISC-LawLLM
DISC-LawLLM is a legal domain large model that aims to provide professional, intelligent, and comprehensive **legal services** to users. It is developed and open-sourced by the Data Intelligence and Social Computing Lab (Fudan-DISC) at Fudan University.
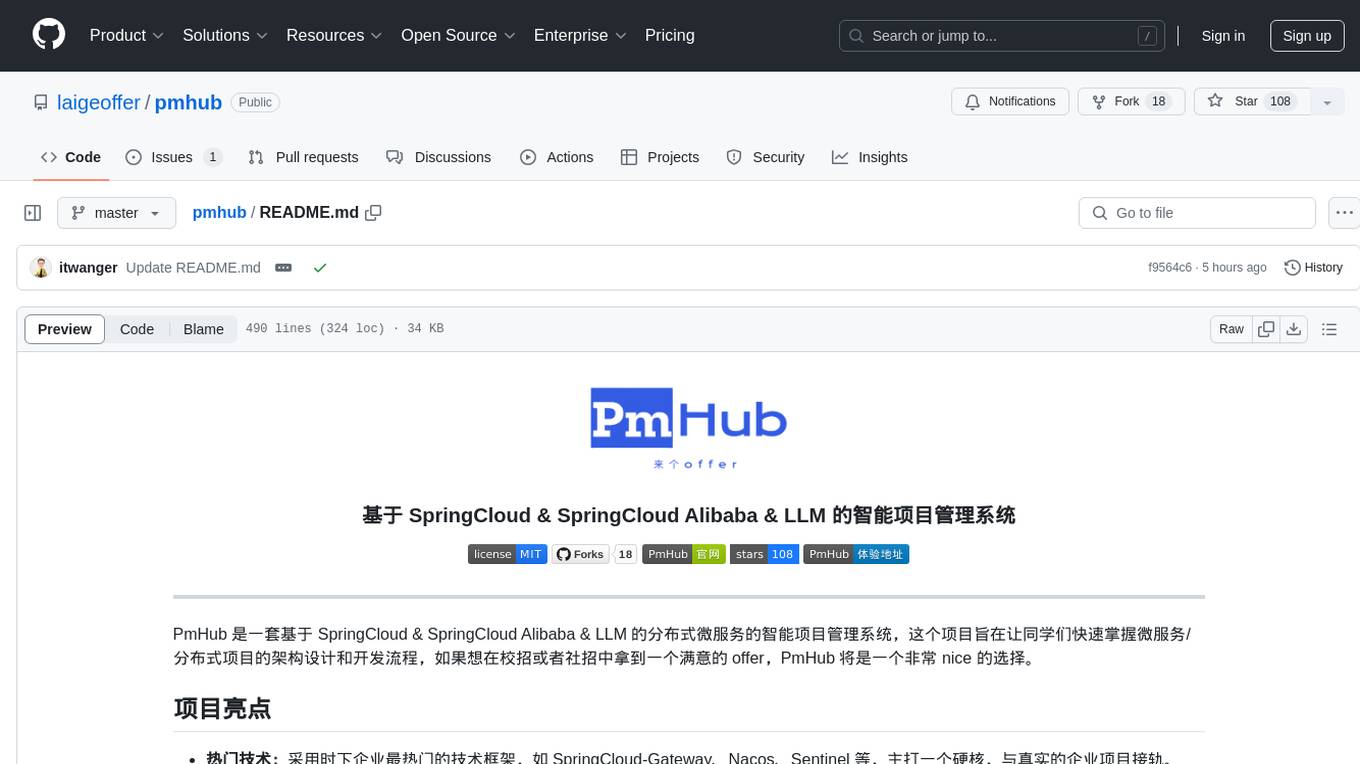
pmhub
PmHub is a smart project management system based on SpringCloud, SpringCloud Alibaba, and LLM. It aims to help students quickly grasp the architecture design and development process of microservices/distributed projects. PmHub provides a platform for students to experience the transformation from monolithic to microservices architecture, understand the pros and cons of both architectures, and prepare for job interviews. It offers popular technologies like SpringCloud-Gateway, Nacos, Sentinel, and provides high-quality code, continuous integration, product design documents, and an enterprise workflow system. PmHub is suitable for beginners and advanced learners who want to master core knowledge of microservices/distributed projects.
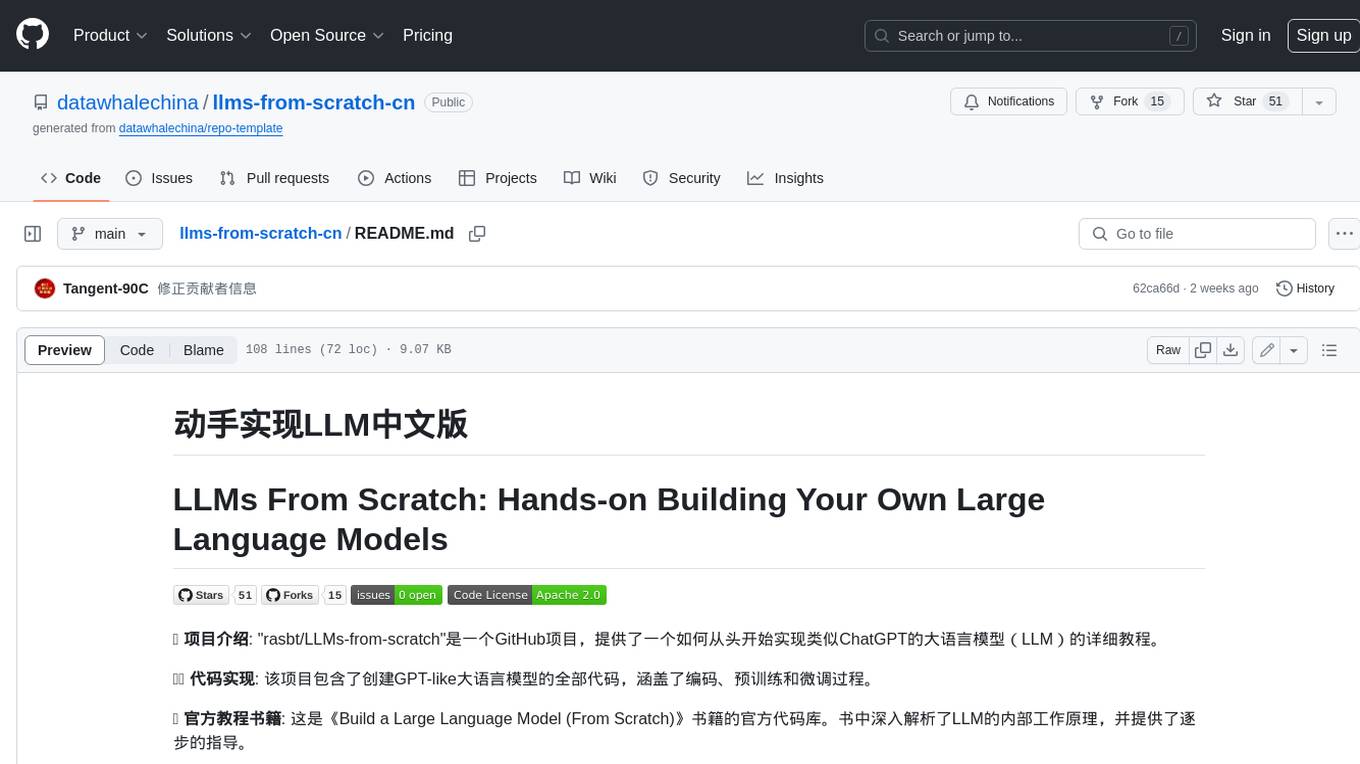
llms-from-scratch-cn
This repository provides a detailed tutorial on how to build your own large language model (LLM) from scratch. It includes all the code necessary to create a GPT-like LLM, covering the encoding, pre-training, and fine-tuning processes. The tutorial is written in a clear and concise style, with plenty of examples and illustrations to help you understand the concepts involved. It is suitable for developers and researchers with some programming experience who are interested in learning more about LLMs and how to build them.
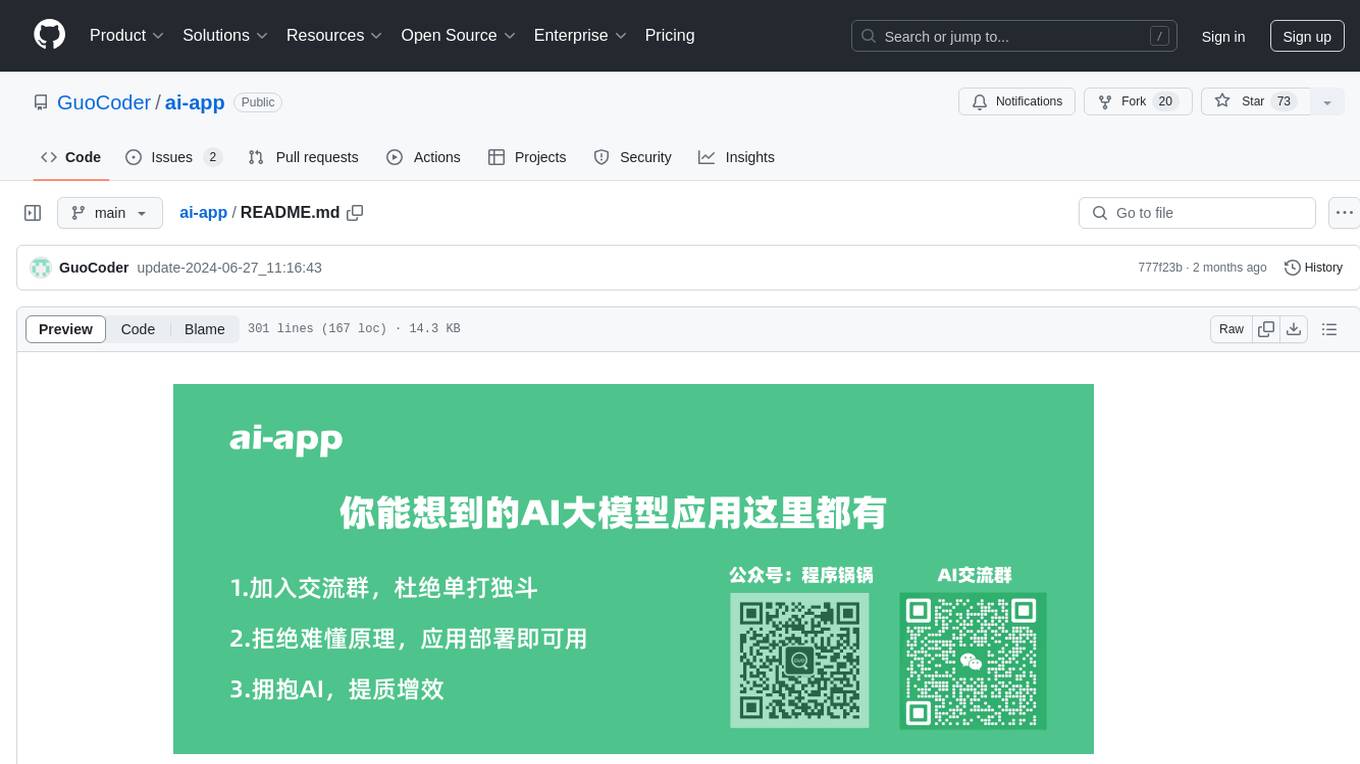
ai-app
The 'ai-app' repository is a comprehensive collection of tools and resources related to artificial intelligence, focusing on topics such as server environment setup, PyCharm and Anaconda installation, large model deployment and training, Transformer principles, RAG technology, vector databases, AI image, voice, and music generation, and AI Agent frameworks. It also includes practical guides and tutorials on implementing various AI applications. The repository serves as a valuable resource for individuals interested in exploring different aspects of AI technology.
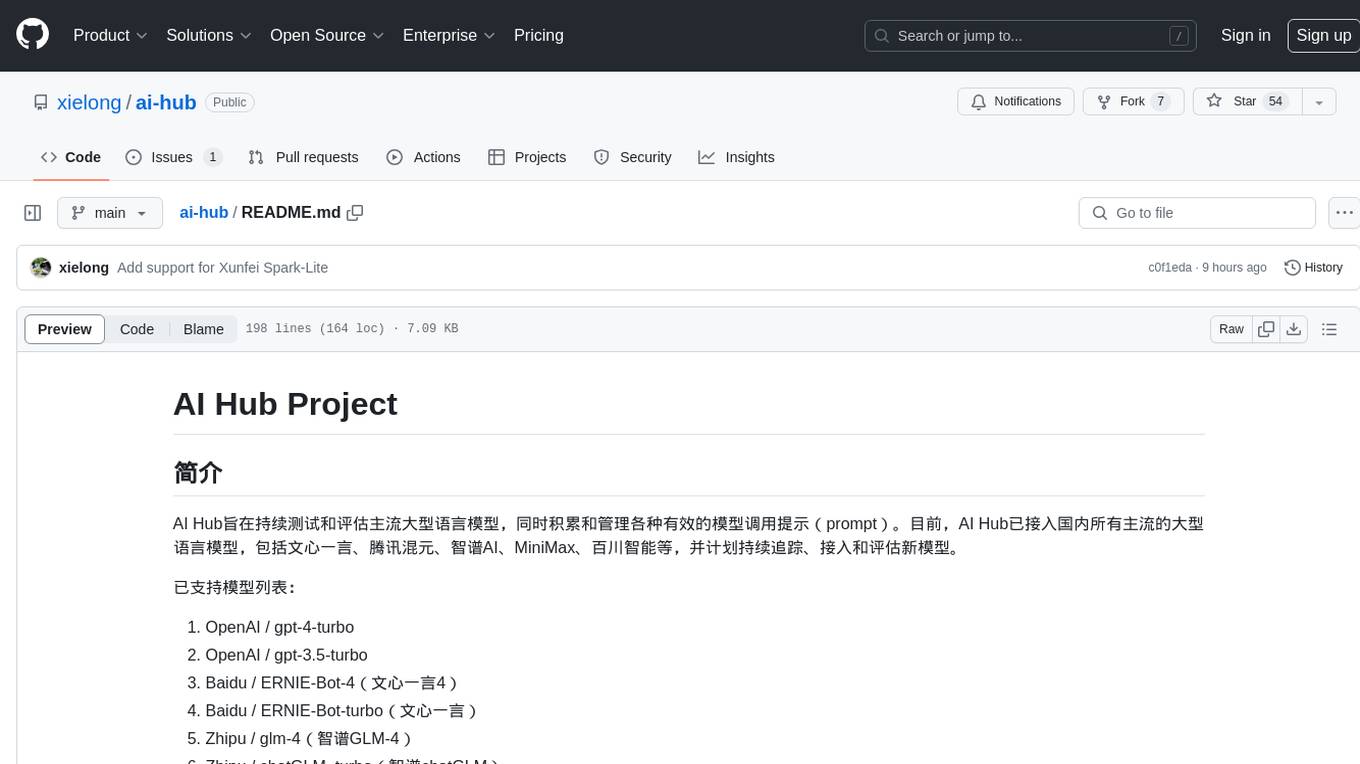
ai-hub
AI Hub Project aims to continuously test and evaluate mainstream large language models, while accumulating and managing various effective model invocation prompts. It has integrated all mainstream large language models in China, including OpenAI GPT-4 Turbo, Baidu ERNIE-Bot-4, Tencent ChatPro, MiniMax abab5.5-chat, and more. The project plans to continuously track, integrate, and evaluate new models. Users can access the models through REST services or Java code integration. The project also provides a testing suite for translation, coding, and benchmark testing.
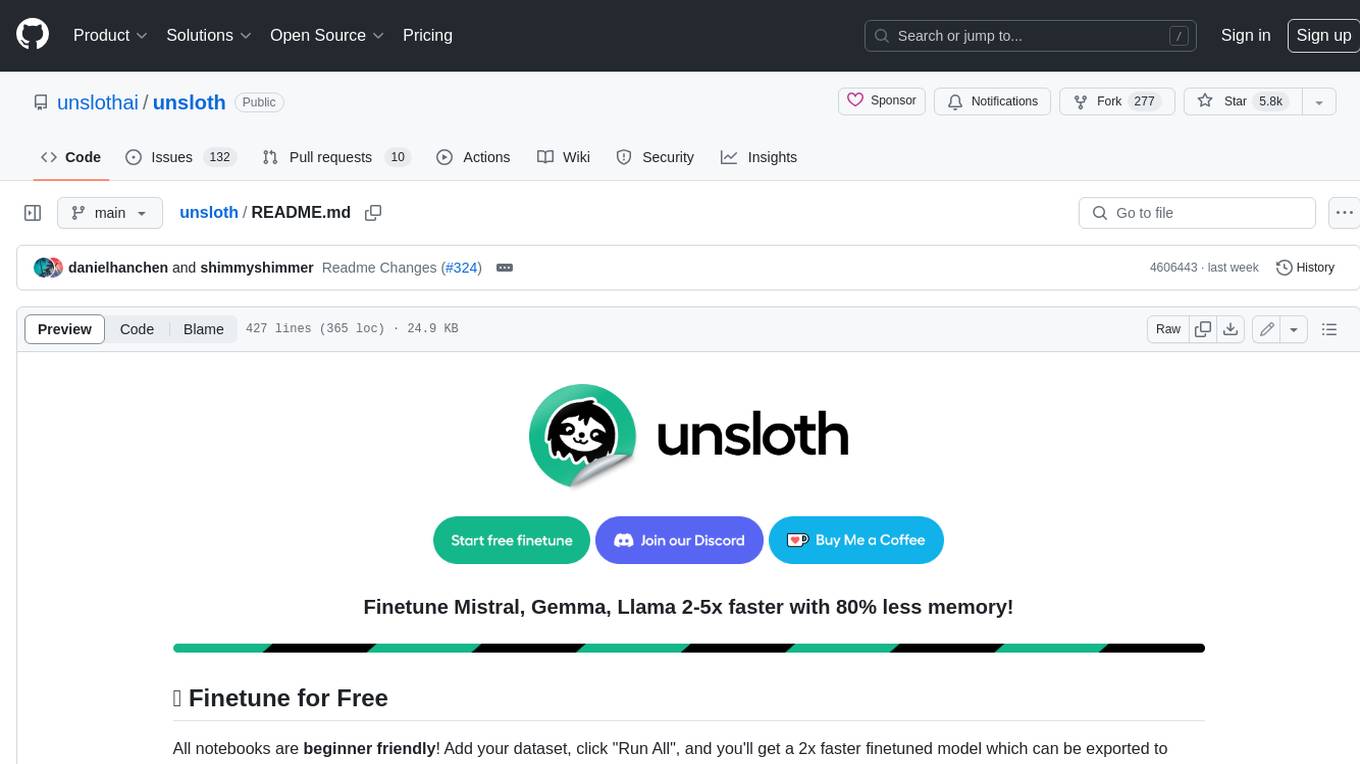
unsloth
Unsloth is a tool that allows users to fine-tune large language models (LLMs) 2-5x faster with 80% less memory. It is a free and open-source tool that can be used to fine-tune LLMs such as Gemma, Mistral, Llama 2-5, TinyLlama, and CodeLlama 34b. Unsloth supports 4-bit and 16-bit QLoRA / LoRA fine-tuning via bitsandbytes. It also supports DPO (Direct Preference Optimization), PPO, and Reward Modelling. Unsloth is compatible with Hugging Face's TRL, Trainer, Seq2SeqTrainer, and Pytorch code. It is also compatible with NVIDIA GPUs since 2018+ (minimum CUDA Capability 7.0).
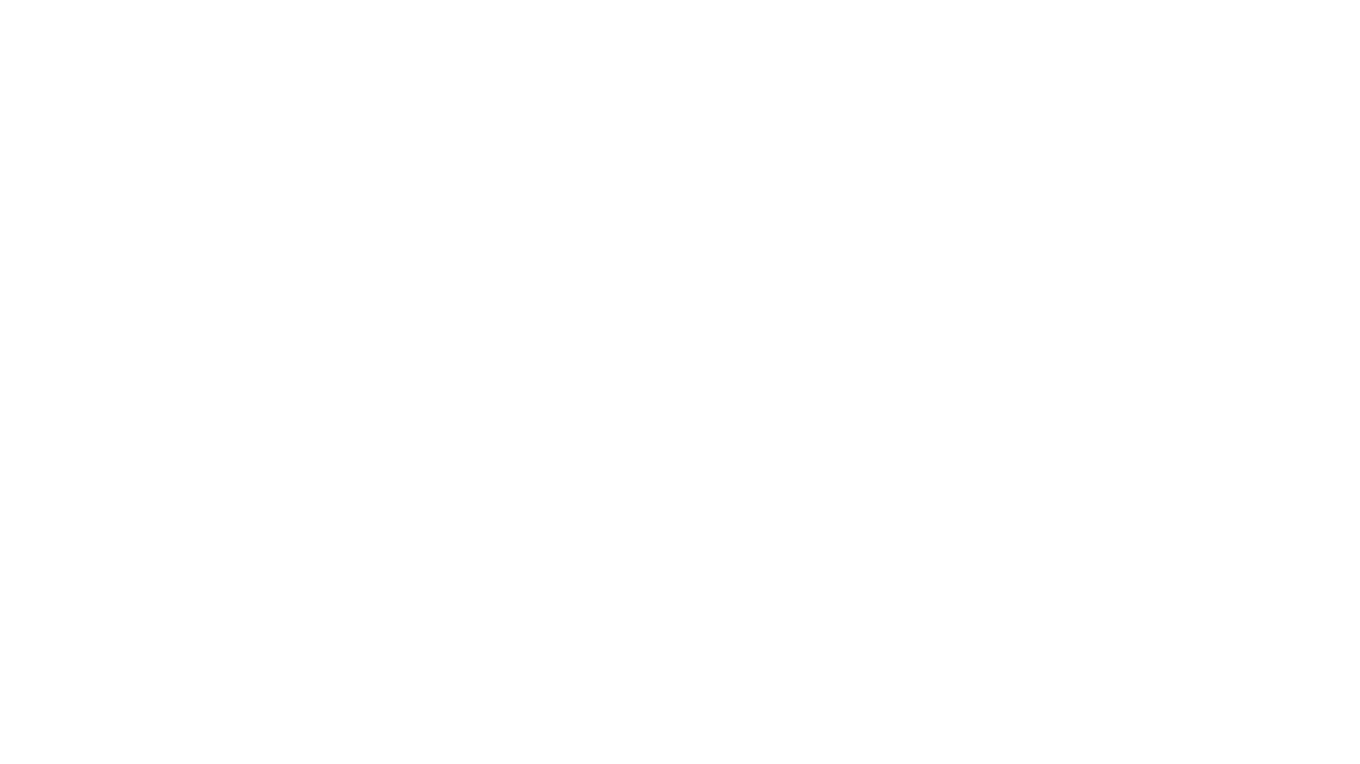
Chinese-LLaMA-Alpaca-2
Chinese-LLaMA-Alpaca-2 is a large Chinese language model developed by Meta AI. It is based on the Llama-2 model and has been further trained on a large dataset of Chinese text. Chinese-LLaMA-Alpaca-2 can be used for a variety of natural language processing tasks, including text generation, question answering, and machine translation. Here are some of the key features of Chinese-LLaMA-Alpaca-2: * It is the largest Chinese language model ever trained, with 13 billion parameters. * It is trained on a massive dataset of Chinese text, including books, news articles, and social media posts. * It can be used for a variety of natural language processing tasks, including text generation, question answering, and machine translation. * It is open-source and available for anyone to use. Chinese-LLaMA-Alpaca-2 is a powerful tool that can be used to improve the performance of a wide range of natural language processing tasks. It is a valuable resource for researchers and developers working in the field of artificial intelligence.
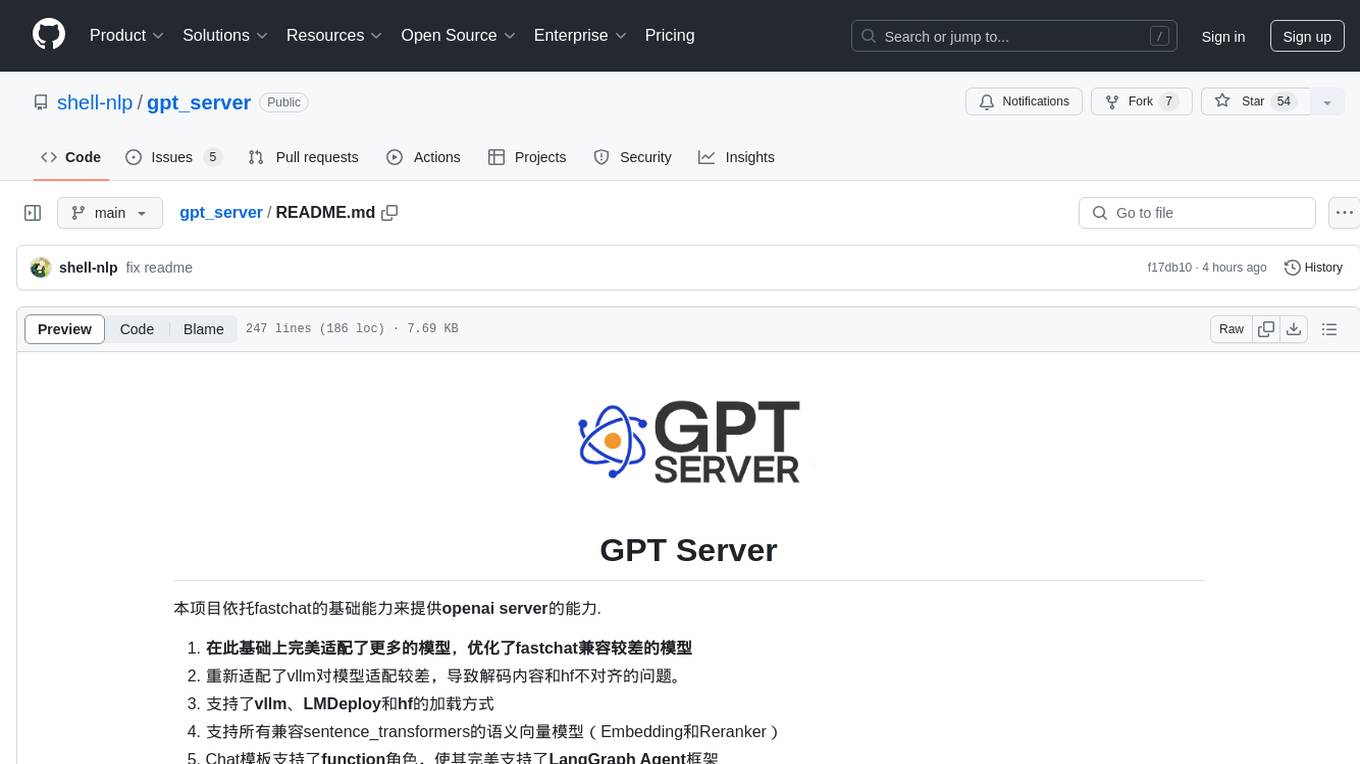
gpt_server
The GPT Server project leverages the basic capabilities of FastChat to provide the capabilities of an openai server. It perfectly adapts more models, optimizes models with poor compatibility in FastChat, and supports loading vllm, LMDeploy, and hf in various ways. It also supports all sentence_transformers compatible semantic vector models, including Chat templates with function roles, Function Calling (Tools) capability, and multi-modal large models. The project aims to reduce the difficulty of model adaptation and project usage, making it easier to deploy the latest models with minimal code changes.
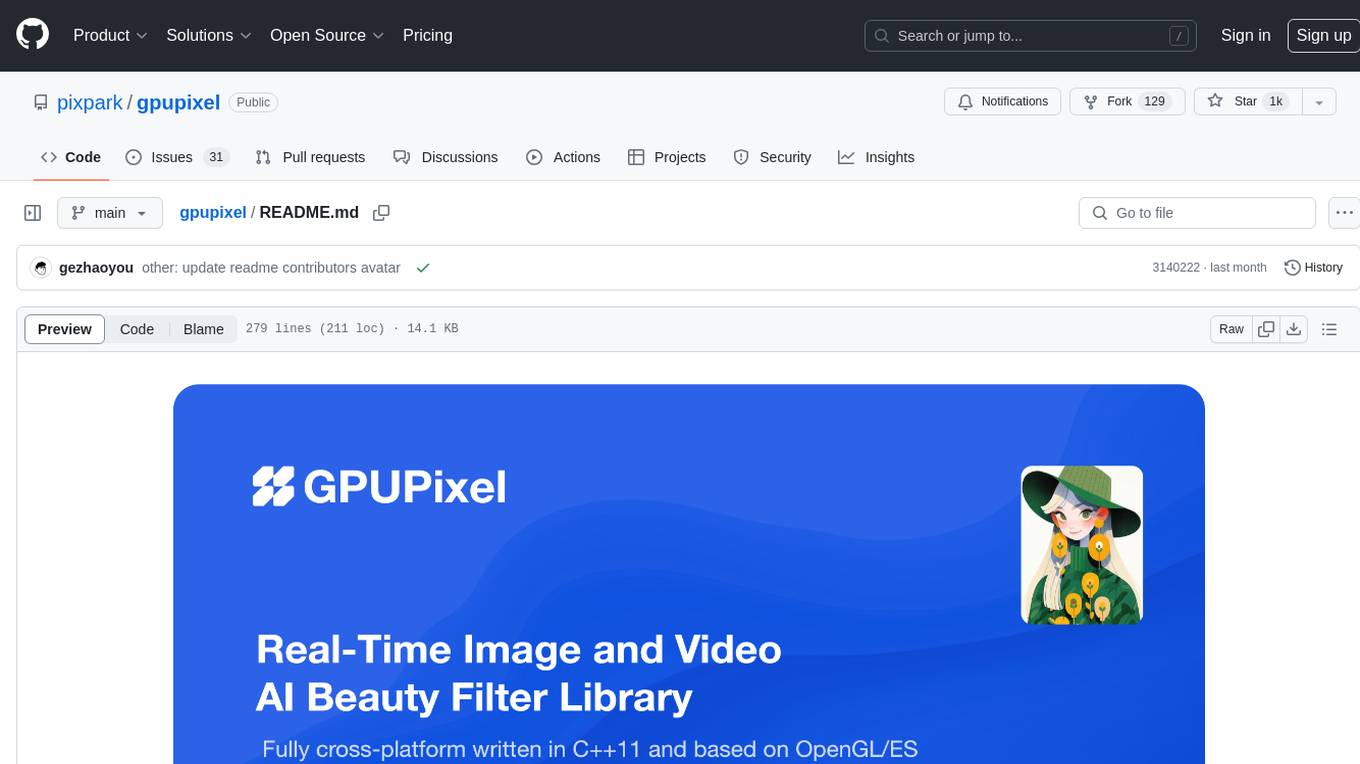
gpupixel
GPUPixel is a real-time, high-performance image and video filter library written in C++11 and based on OpenGL/ES. It incorporates a built-in beauty face filter that achieves commercial-grade beauty effects. The library is extremely easy to compile and integrate with a small size, supporting platforms including iOS, Android, Mac, Windows, and Linux. GPUPixel provides various filters like skin smoothing, whitening, face slimming, big eyes, lipstick, and blush. It supports input formats like YUV420P, RGBA, JPEG, PNG, and output formats like RGBA and YUV420P. The library's performance on devices like iPhone and Android is optimized, with low CPU usage and fast processing times. GPUPixel's lib size is compact, making it suitable for mobile and desktop applications.
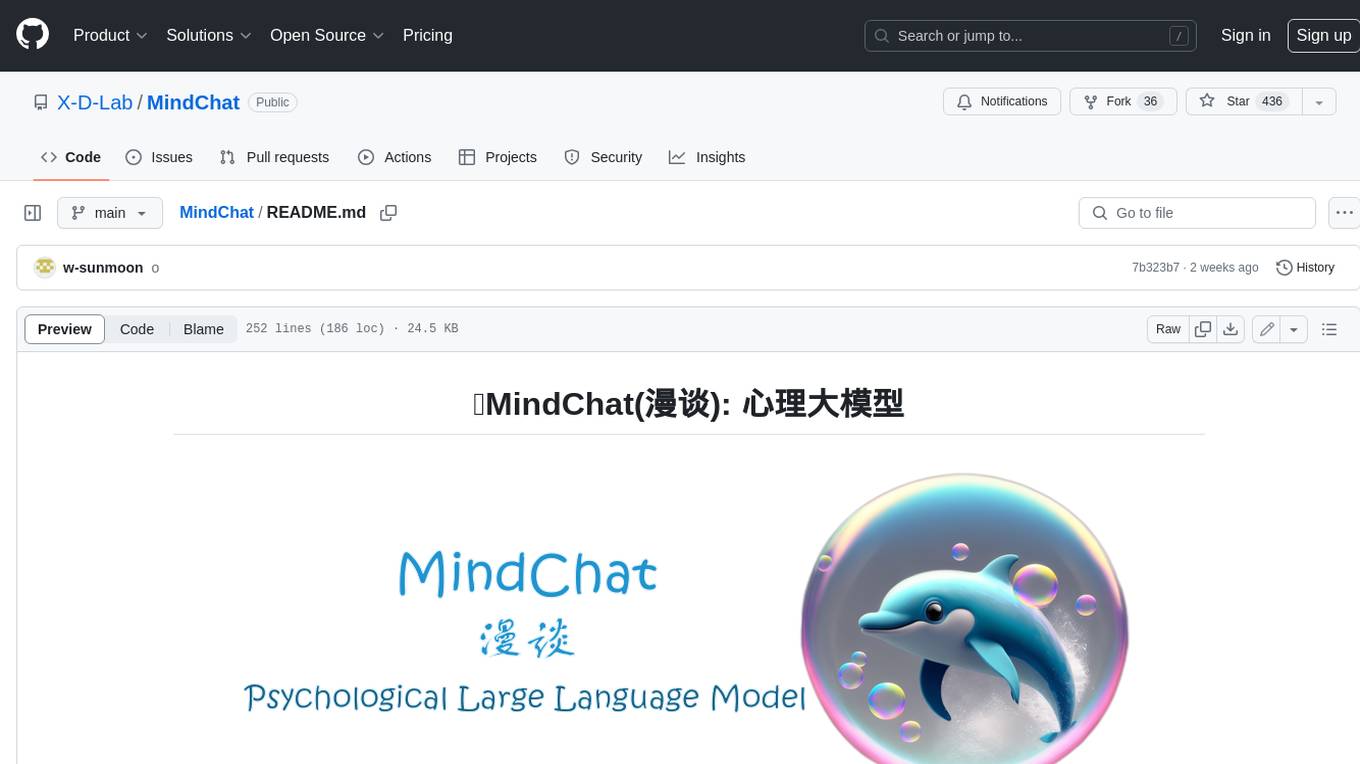
MindChat
MindChat is a psychological large language model designed to help individuals relieve psychological stress and solve mental confusion, ultimately improving mental health. It aims to provide a relaxed and open conversation environment for users to build trust and understanding. MindChat offers privacy, warmth, safety, timely, and convenient conversation settings to help users overcome difficulties and challenges, achieve self-growth, and development. The tool is suitable for both work and personal life scenarios, providing comprehensive psychological support and therapeutic assistance to users while strictly protecting user privacy. It combines psychological knowledge with artificial intelligence technology to contribute to a healthier, more inclusive, and equal society.
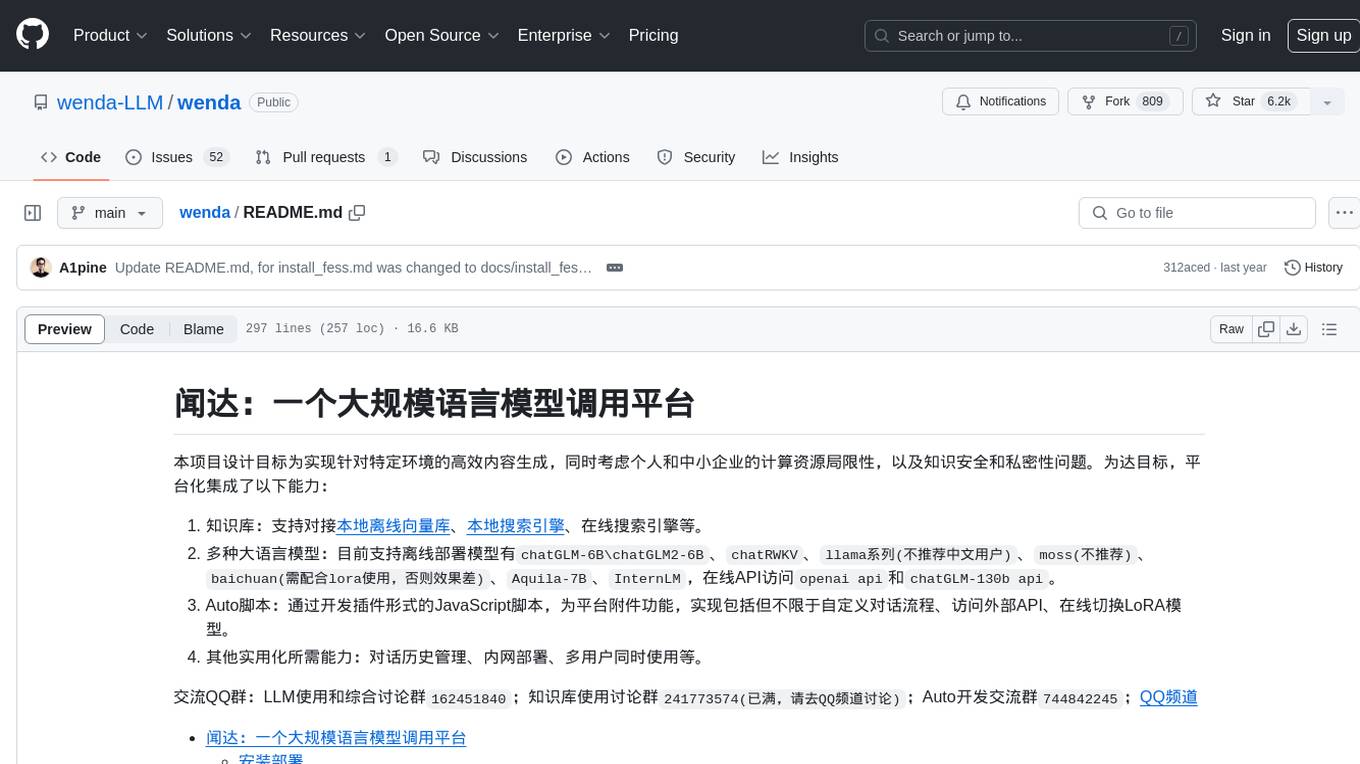
wenda
Wenda is a platform for large-scale language model invocation designed to efficiently generate content for specific environments, considering the limitations of personal and small business computing resources, as well as knowledge security and privacy issues. The platform integrates capabilities such as knowledge base integration, multiple large language models for offline deployment, auto scripts for additional functionality, and other practical capabilities like conversation history management and multi-user simultaneous usage.
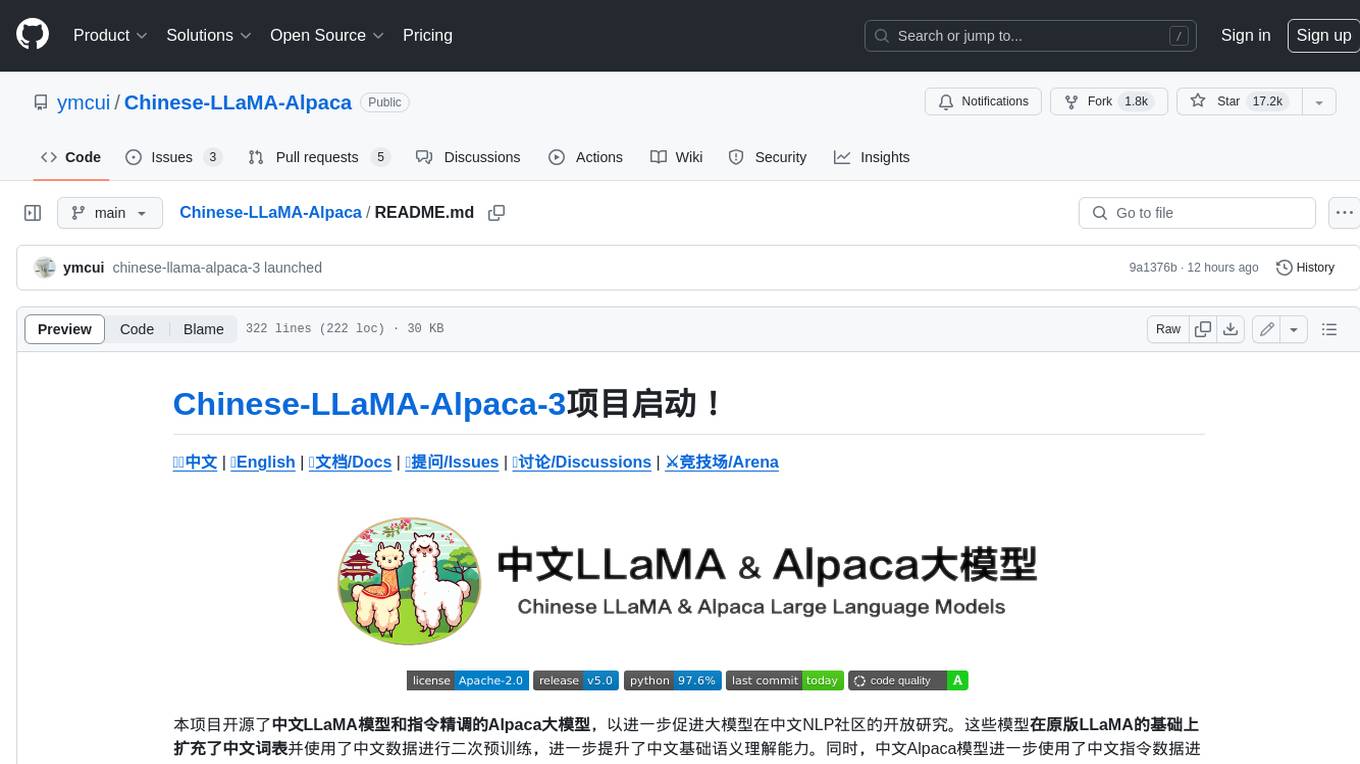
Chinese-LLaMA-Alpaca
This project open sources the **Chinese LLaMA model and the Alpaca large model fine-tuned with instructions**, to further promote the open research of large models in the Chinese NLP community. These models **extend the Chinese vocabulary based on the original LLaMA** and use Chinese data for secondary pre-training, further enhancing the basic Chinese semantic understanding ability. At the same time, the Chinese Alpaca model further uses Chinese instruction data for fine-tuning, significantly improving the model's understanding and execution of instructions.
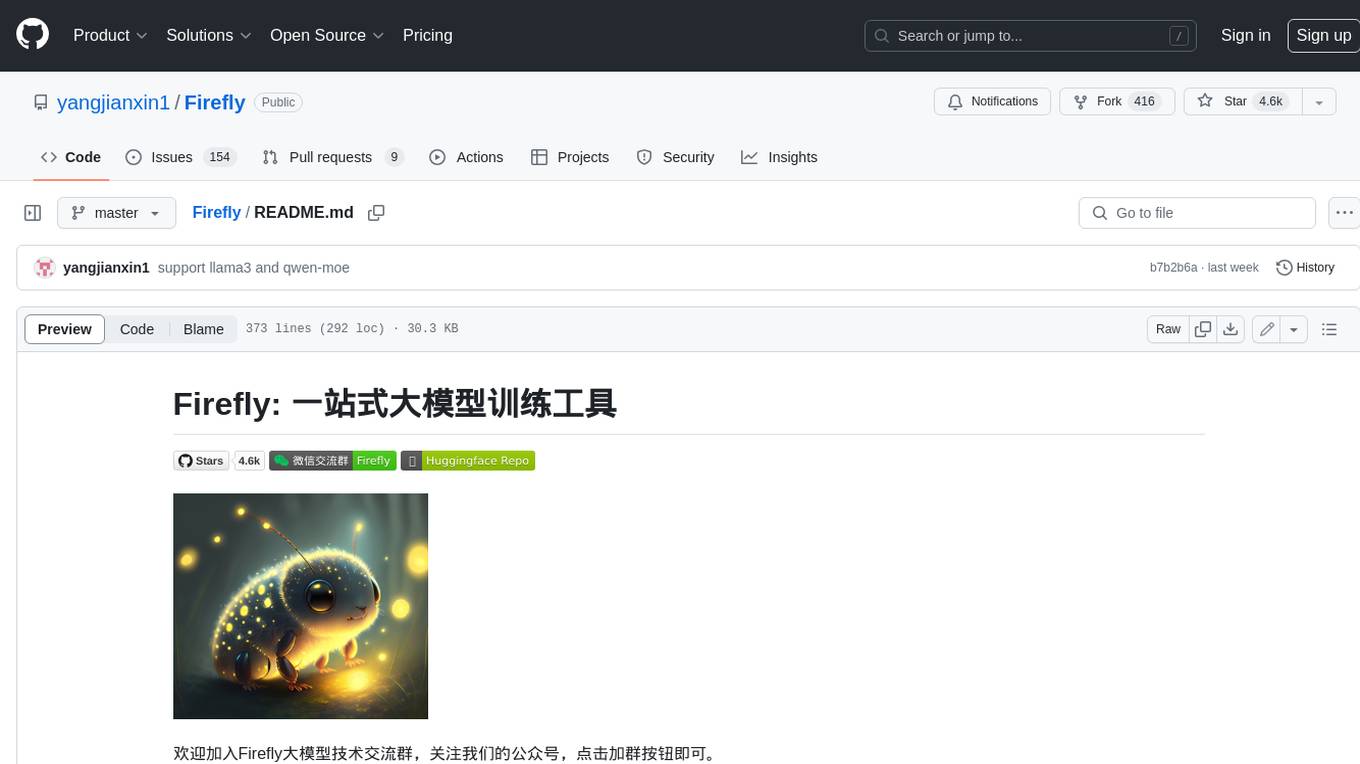
Firefly
Firefly is an open-source large model training project that supports pre-training, fine-tuning, and DPO of mainstream large models. It includes models like Llama3, Gemma, Qwen1.5, MiniCPM, Llama, InternLM, Baichuan, ChatGLM, Yi, Deepseek, Qwen, Orion, Ziya, Xverse, Mistral, Mixtral-8x7B, Zephyr, Vicuna, Bloom, etc. The project supports full-parameter training, LoRA, QLoRA efficient training, and various tasks such as pre-training, SFT, and DPO. Suitable for users with limited training resources, QLoRA is recommended for fine-tuning instructions. The project has achieved good results on the Open LLM Leaderboard with QLoRA training process validation. The latest version has significant updates and adaptations for different chat model templates.
For similar tasks
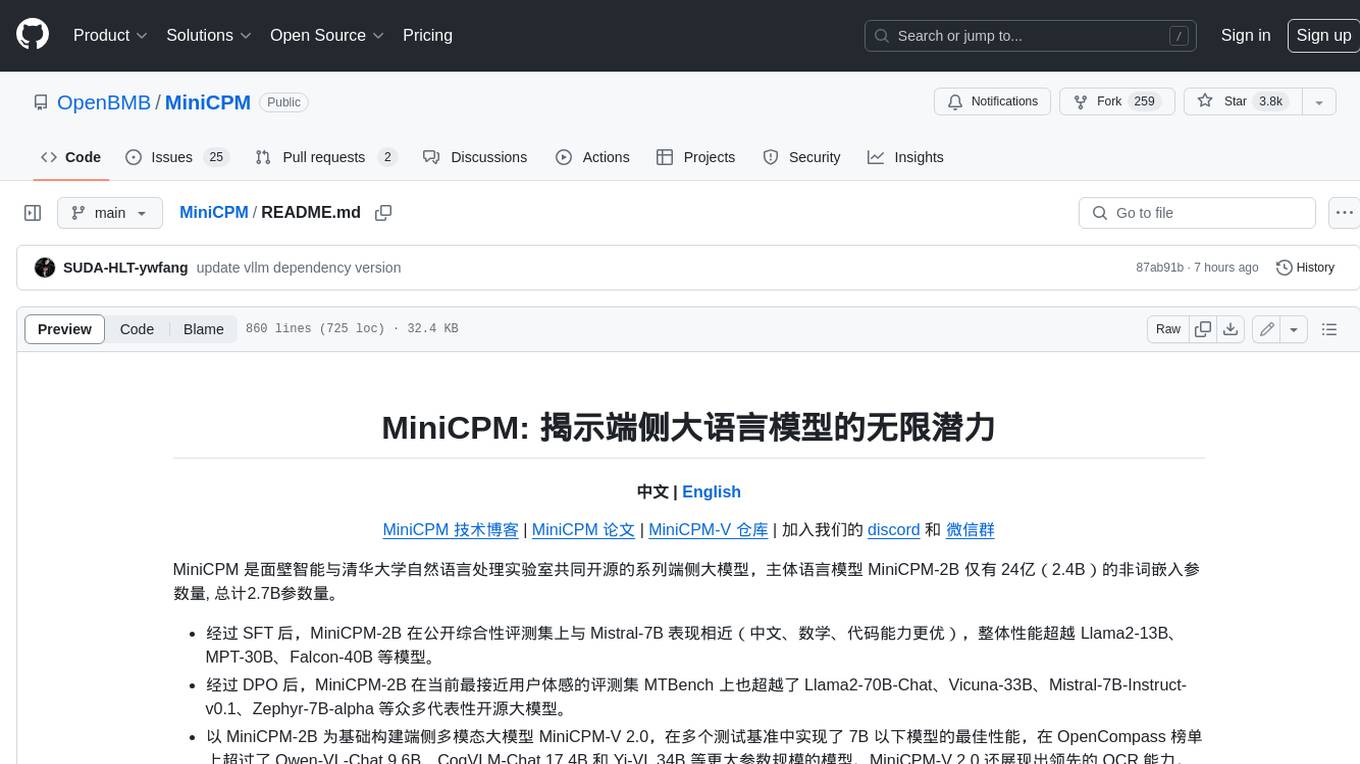
MiniCPM
MiniCPM is a series of open-source large models on the client side jointly developed by Face Intelligence and Tsinghua University Natural Language Processing Laboratory. The main language model MiniCPM-2B has only 2.4 billion (2.4B) non-word embedding parameters, with a total of 2.7B parameters. - After SFT, MiniCPM-2B performs similarly to Mistral-7B on public comprehensive evaluation sets (better in Chinese, mathematics, and code capabilities), and outperforms models such as Llama2-13B, MPT-30B, and Falcon-40B overall. - After DPO, MiniCPM-2B also surpasses many representative open-source large models such as Llama2-70B-Chat, Vicuna-33B, Mistral-7B-Instruct-v0.1, and Zephyr-7B-alpha on the current evaluation set MTBench, which is closest to the user experience. - Based on MiniCPM-2B, a multi-modal large model MiniCPM-V 2.0 on the client side is constructed, which achieves the best performance of models below 7B in multiple test benchmarks, and surpasses larger parameter scale models such as Qwen-VL-Chat 9.6B, CogVLM-Chat 17.4B, and Yi-VL 34B on the OpenCompass leaderboard. MiniCPM-V 2.0 also demonstrates leading OCR capabilities, approaching Gemini Pro in scene text recognition capabilities. - After Int4 quantization, MiniCPM can be deployed and inferred on mobile phones, with a streaming output speed slightly higher than human speech speed. MiniCPM-V also directly runs through the deployment of multi-modal large models on mobile phones. - A single 1080/2080 can efficiently fine-tune parameters, and a single 3090/4090 can fully fine-tune parameters. A single machine can continuously train MiniCPM, and the secondary development cost is relatively low.
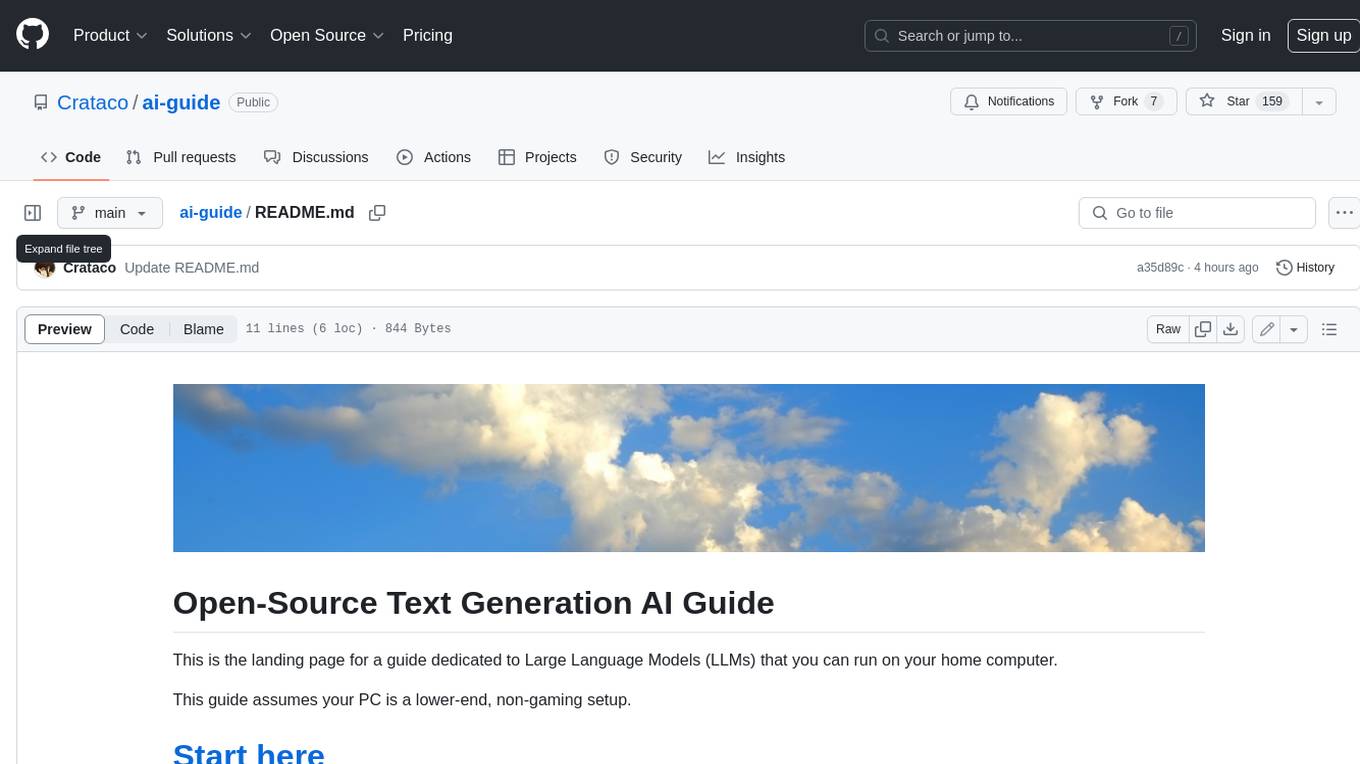
ai-guide
This guide is dedicated to Large Language Models (LLMs) that you can run on your home computer. It assumes your PC is a lower-end, non-gaming setup.
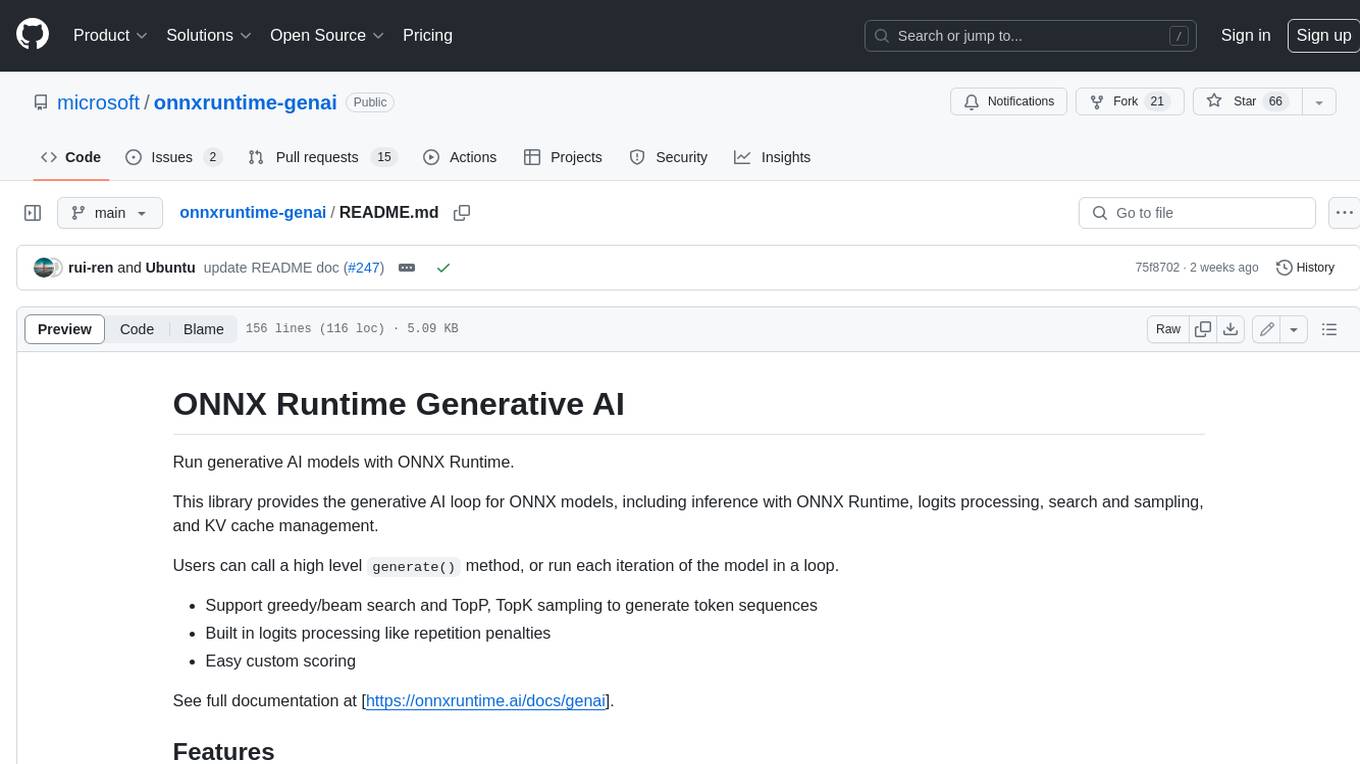
onnxruntime-genai
ONNX Runtime Generative AI is a library that provides the generative AI loop for ONNX models, including inference with ONNX Runtime, logits processing, search and sampling, and KV cache management. Users can call a high level `generate()` method, or run each iteration of the model in a loop. It supports greedy/beam search and TopP, TopK sampling to generate token sequences, has built in logits processing like repetition penalties, and allows for easy custom scoring.
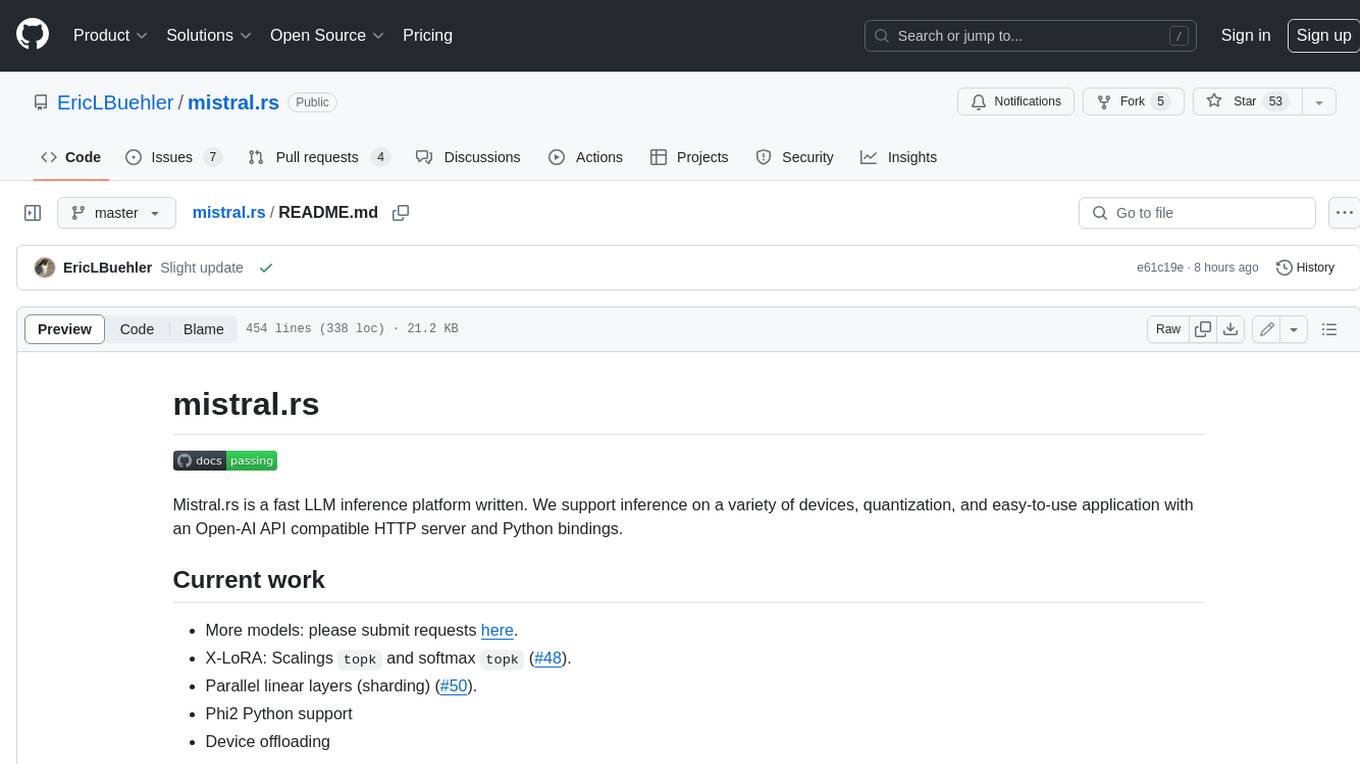
mistral.rs
Mistral.rs is a fast LLM inference platform written in Rust. We support inference on a variety of devices, quantization, and easy-to-use application with an Open-AI API compatible HTTP server and Python bindings.
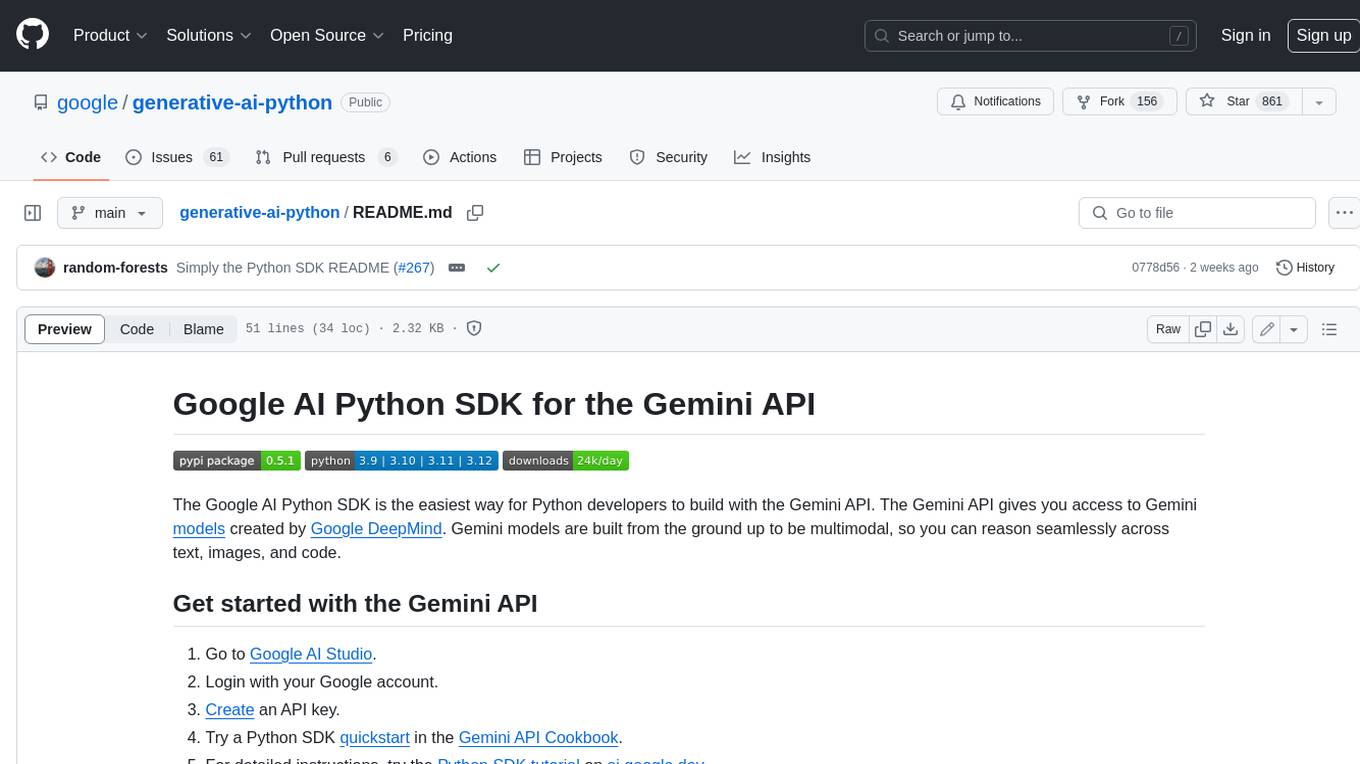
generative-ai-python
The Google AI Python SDK is the easiest way for Python developers to build with the Gemini API. The Gemini API gives you access to Gemini models created by Google DeepMind. Gemini models are built from the ground up to be multimodal, so you can reason seamlessly across text, images, and code.
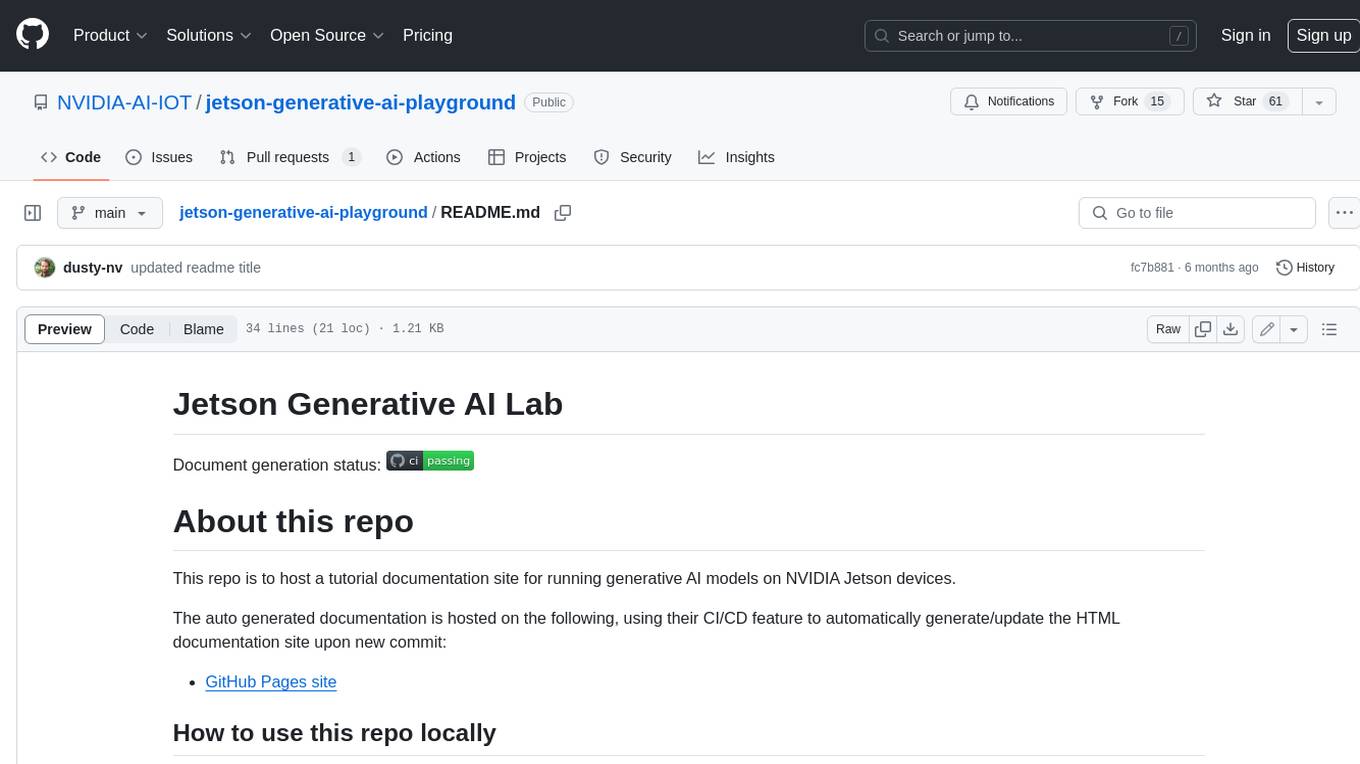
jetson-generative-ai-playground
This repo hosts tutorial documentation for running generative AI models on NVIDIA Jetson devices. The documentation is auto-generated and hosted on GitHub Pages using their CI/CD feature to automatically generate/update the HTML documentation site upon new commits.
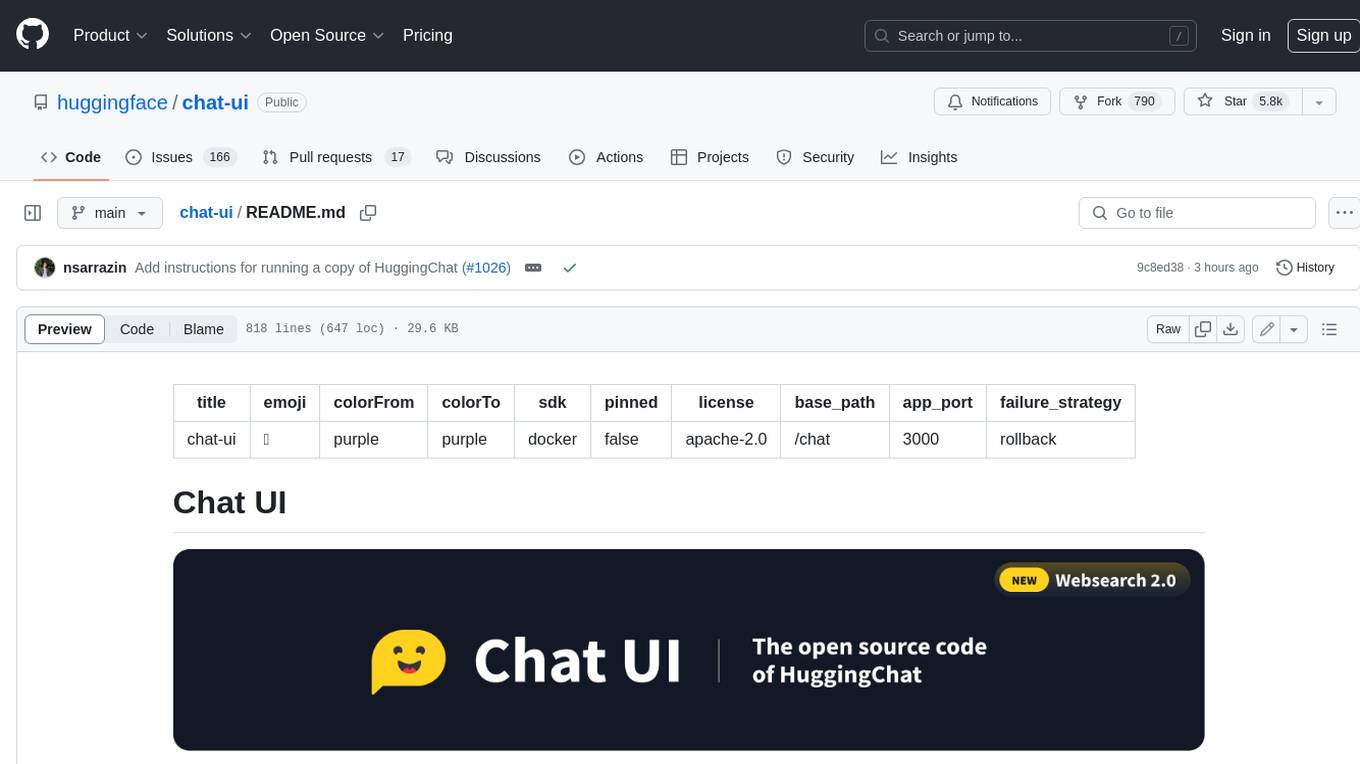
chat-ui
A chat interface using open source models, eg OpenAssistant or Llama. It is a SvelteKit app and it powers the HuggingChat app on hf.co/chat.
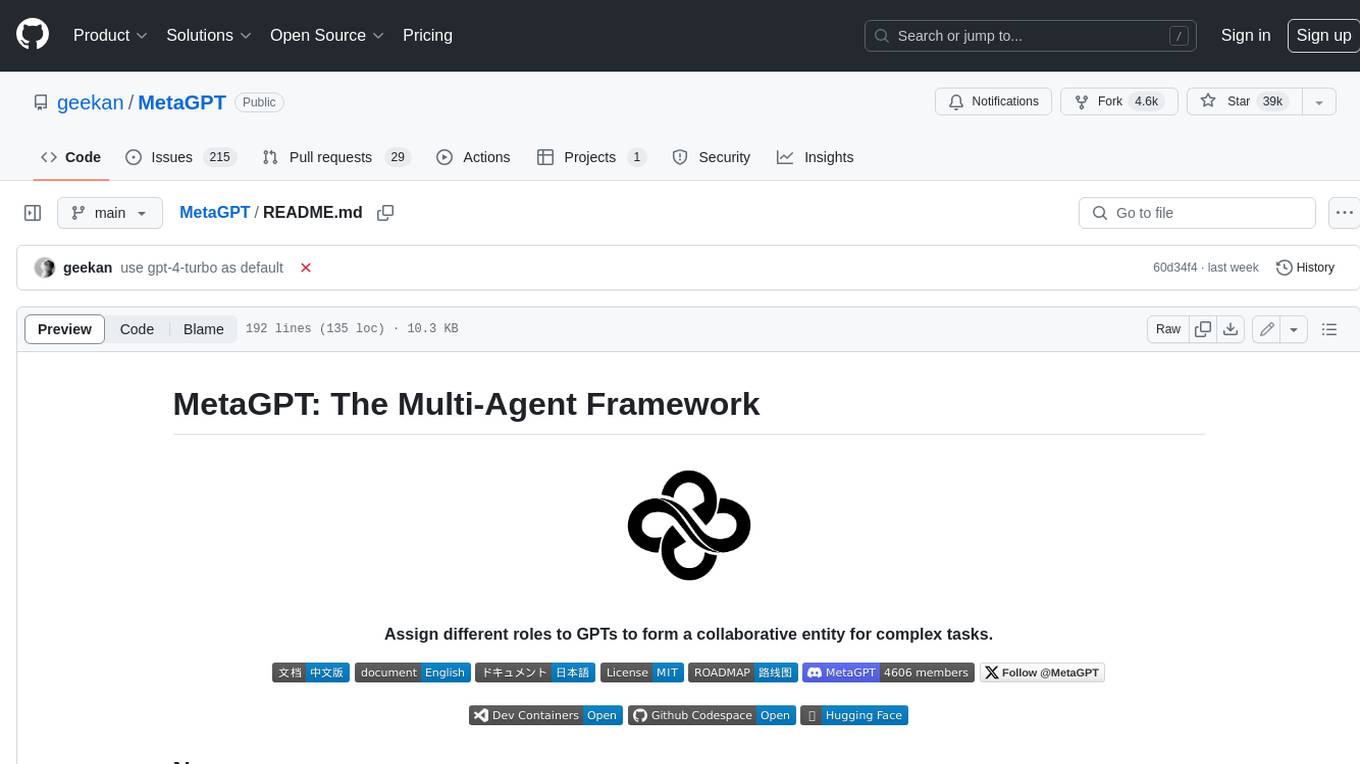
MetaGPT
MetaGPT is a multi-agent framework that enables GPT to work in a software company, collaborating to tackle more complex tasks. It assigns different roles to GPTs to form a collaborative entity for complex tasks. MetaGPT takes a one-line requirement as input and outputs user stories, competitive analysis, requirements, data structures, APIs, documents, etc. Internally, MetaGPT includes product managers, architects, project managers, and engineers. It provides the entire process of a software company along with carefully orchestrated SOPs. MetaGPT's core philosophy is "Code = SOP(Team)", materializing SOP and applying it to teams composed of LLMs.
For similar jobs
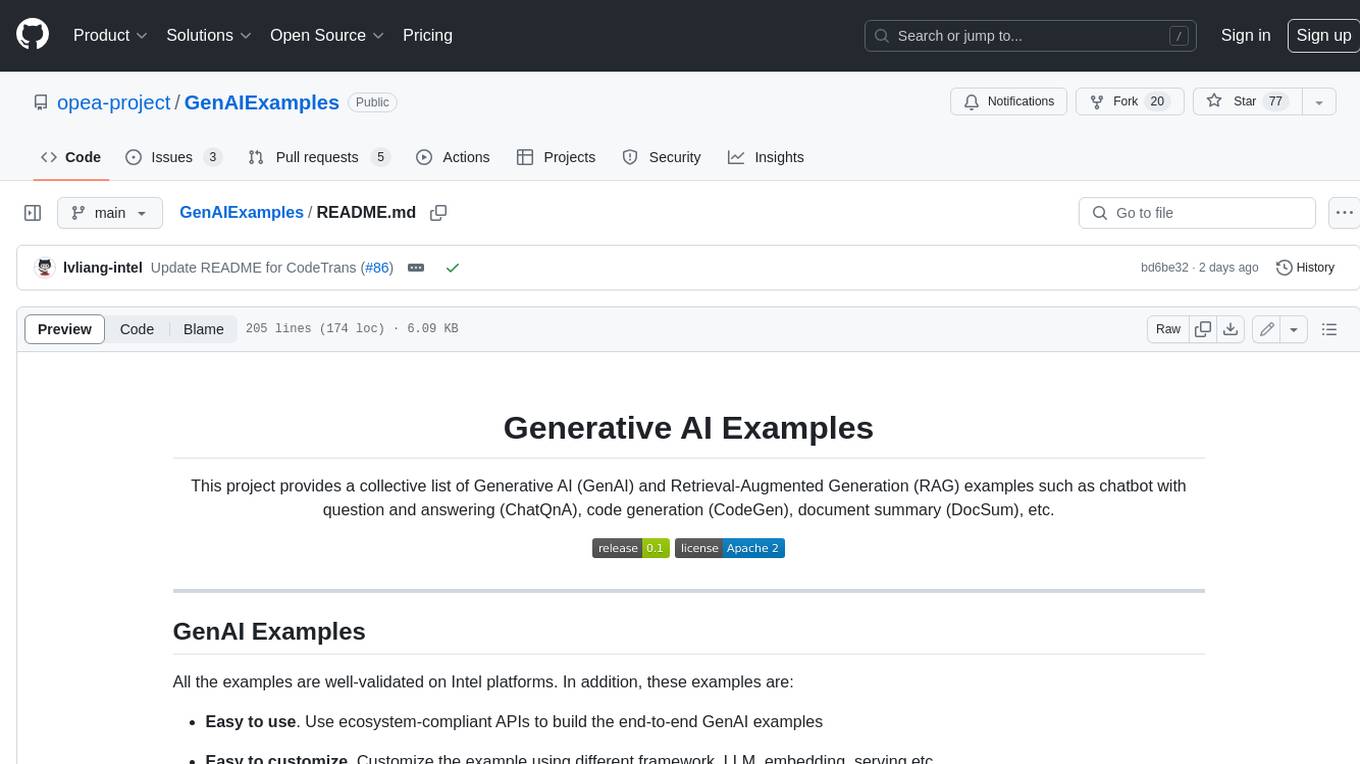
GenAIExamples
This project provides a collective list of Generative AI (GenAI) and Retrieval-Augmented Generation (RAG) examples such as chatbot with question and answering (ChatQnA), code generation (CodeGen), document summary (DocSum), etc.
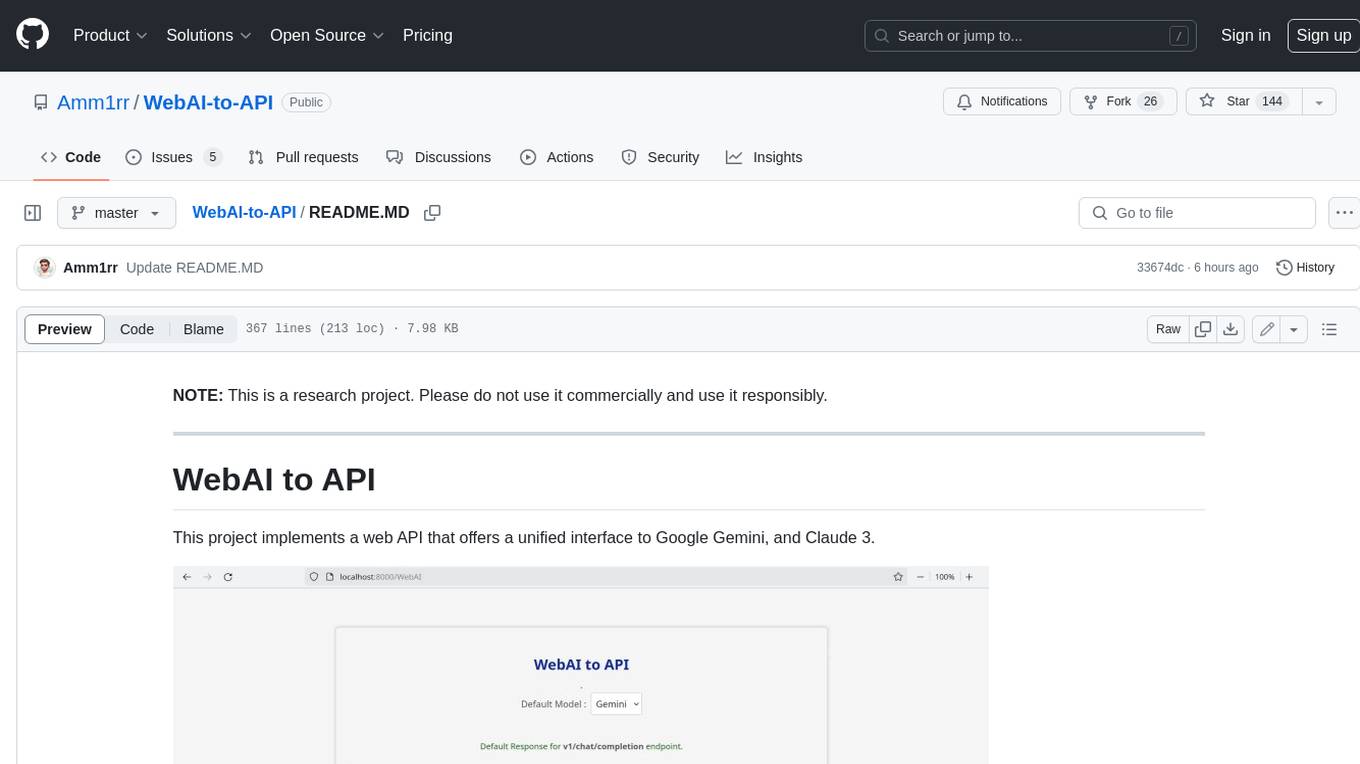
WebAI-to-API
This project implements a web API that offers a unified interface to Google Gemini and Claude 3. It provides a self-hosted, lightweight, and scalable solution for accessing these AI models through a streaming API. The API supports both Claude and Gemini models, allowing users to interact with them in real-time. The project includes a user-friendly web UI for configuration and documentation, making it easy to get started and explore the capabilities of the API.
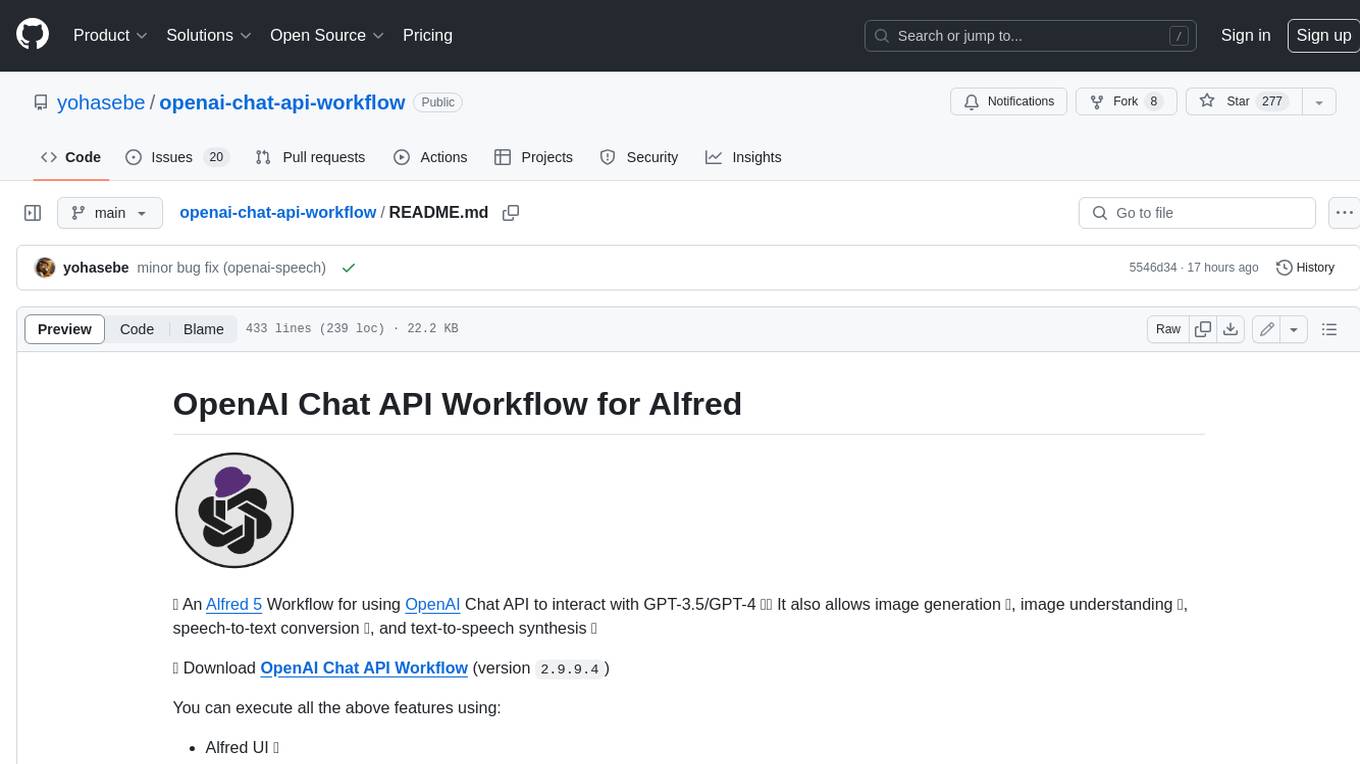
openai-chat-api-workflow
**OpenAI Chat API Workflow for Alfred** An Alfred 5 Workflow for using OpenAI Chat API to interact with GPT-3.5/GPT-4 🤖💬 It also allows image generation 🖼️, image understanding 👀, speech-to-text conversion 🎤, and text-to-speech synthesis 🔈 **Features:** * Execute all features using Alfred UI, selected text, or a dedicated web UI * Web UI is constructed by the workflow and runs locally on your Mac 💻 * API call is made directly between the workflow and OpenAI, ensuring your chat messages are not shared online with anyone other than OpenAI 🔒 * OpenAI does not use the data from the API Platform for training 🚫 * Export chat data to a simple JSON format external file 📄 * Continue the chat by importing the exported data later 🔄
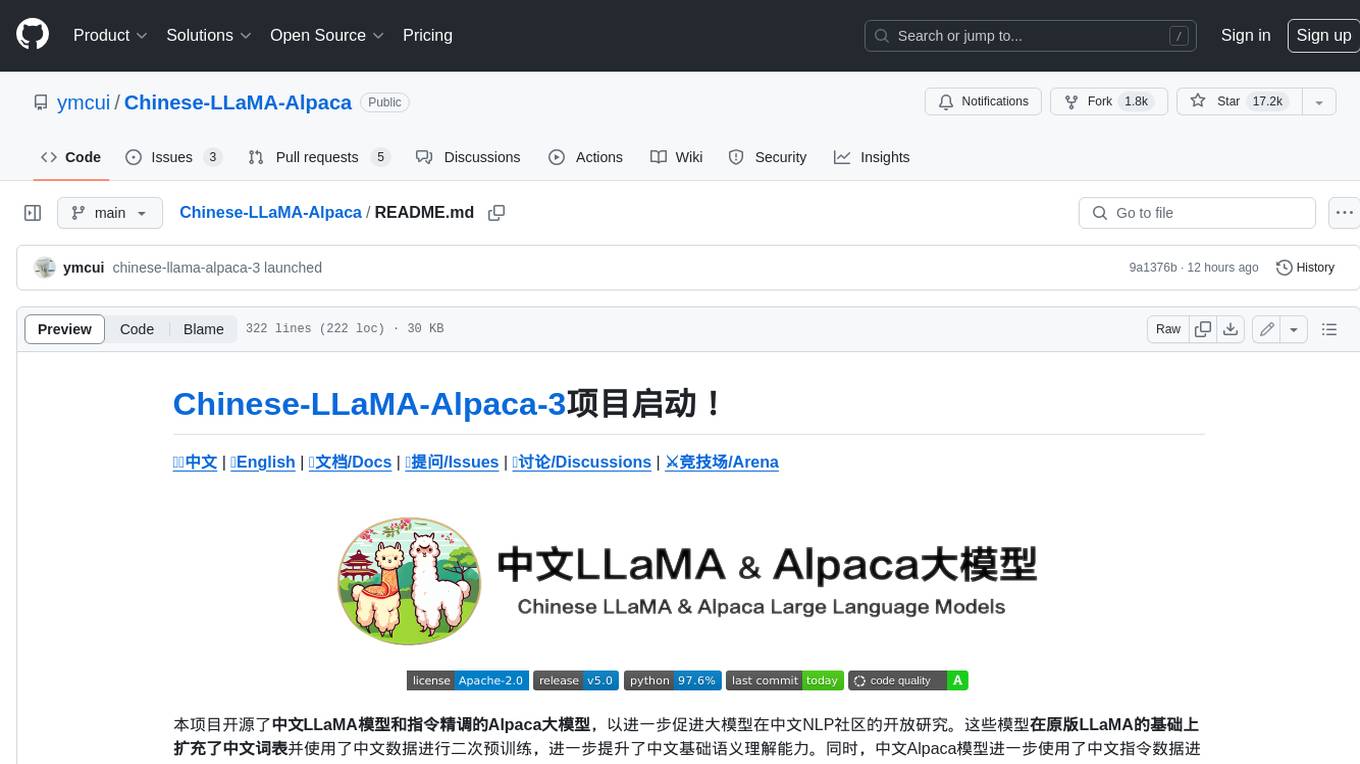
Chinese-LLaMA-Alpaca
This project open sources the **Chinese LLaMA model and the Alpaca large model fine-tuned with instructions**, to further promote the open research of large models in the Chinese NLP community. These models **extend the Chinese vocabulary based on the original LLaMA** and use Chinese data for secondary pre-training, further enhancing the basic Chinese semantic understanding ability. At the same time, the Chinese Alpaca model further uses Chinese instruction data for fine-tuning, significantly improving the model's understanding and execution of instructions.
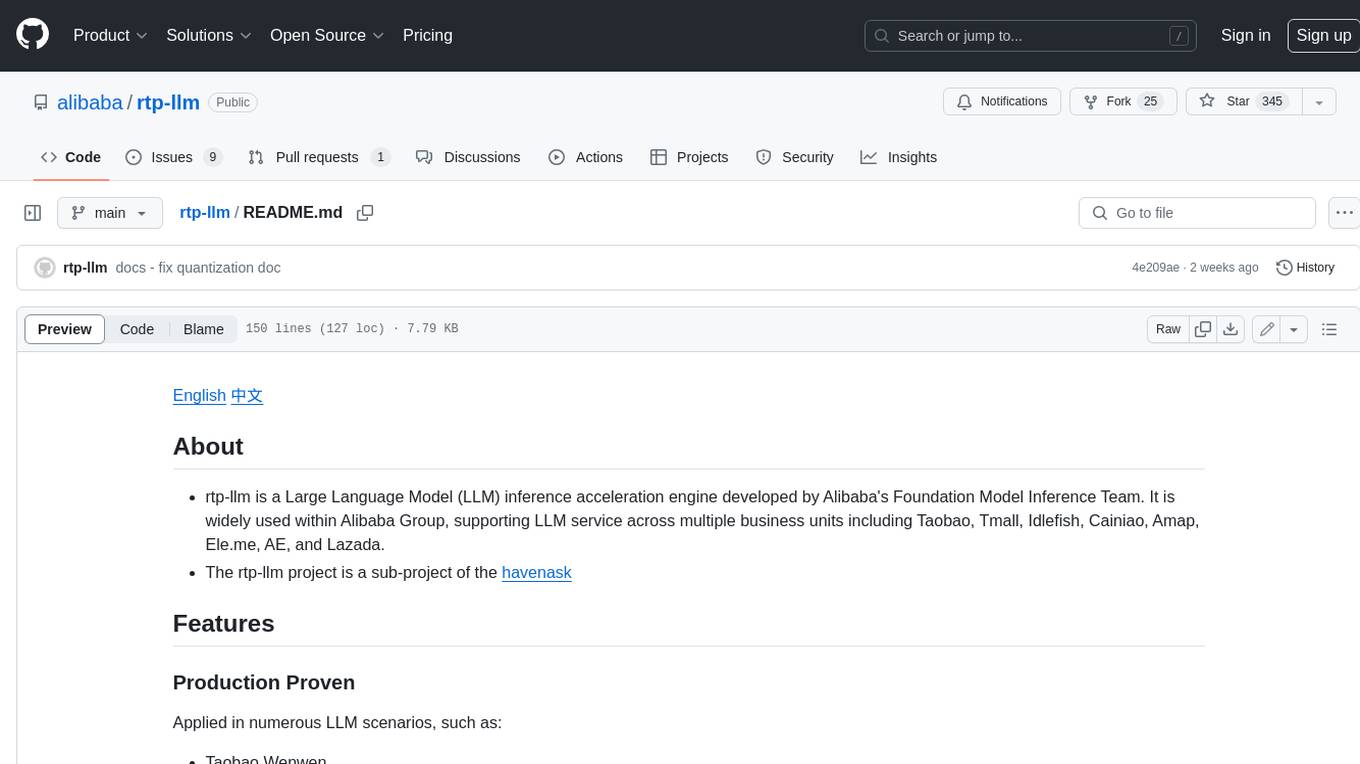
rtp-llm
**rtp-llm** is a Large Language Model (LLM) inference acceleration engine developed by Alibaba's Foundation Model Inference Team. It is widely used within Alibaba Group, supporting LLM service across multiple business units including Taobao, Tmall, Idlefish, Cainiao, Amap, Ele.me, AE, and Lazada. The rtp-llm project is a sub-project of the havenask.
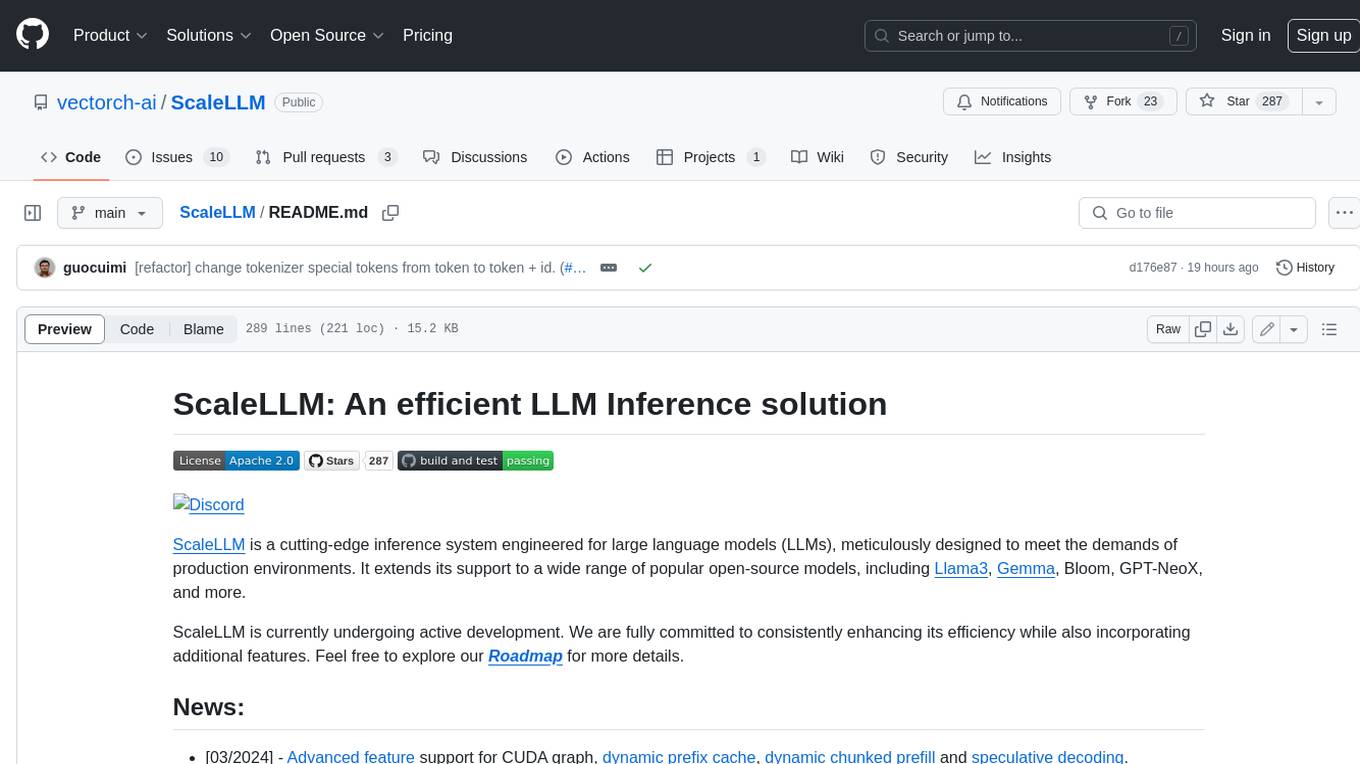
ScaleLLM
ScaleLLM is a cutting-edge inference system engineered for large language models (LLMs), meticulously designed to meet the demands of production environments. It extends its support to a wide range of popular open-source models, including Llama3, Gemma, Bloom, GPT-NeoX, and more. ScaleLLM is currently undergoing active development. We are fully committed to consistently enhancing its efficiency while also incorporating additional features. Feel free to explore our **_Roadmap_** for more details. ## Key Features * High Efficiency: Excels in high-performance LLM inference, leveraging state-of-the-art techniques and technologies like Flash Attention, Paged Attention, Continuous batching, and more. * Tensor Parallelism: Utilizes tensor parallelism for efficient model execution. * OpenAI-compatible API: An efficient golang rest api server that compatible with OpenAI. * Huggingface models: Seamless integration with most popular HF models, supporting safetensors. * Customizable: Offers flexibility for customization to meet your specific needs, and provides an easy way to add new models. * Production Ready: Engineered with production environments in mind, ScaleLLM is equipped with robust system monitoring and management features to ensure a seamless deployment experience.
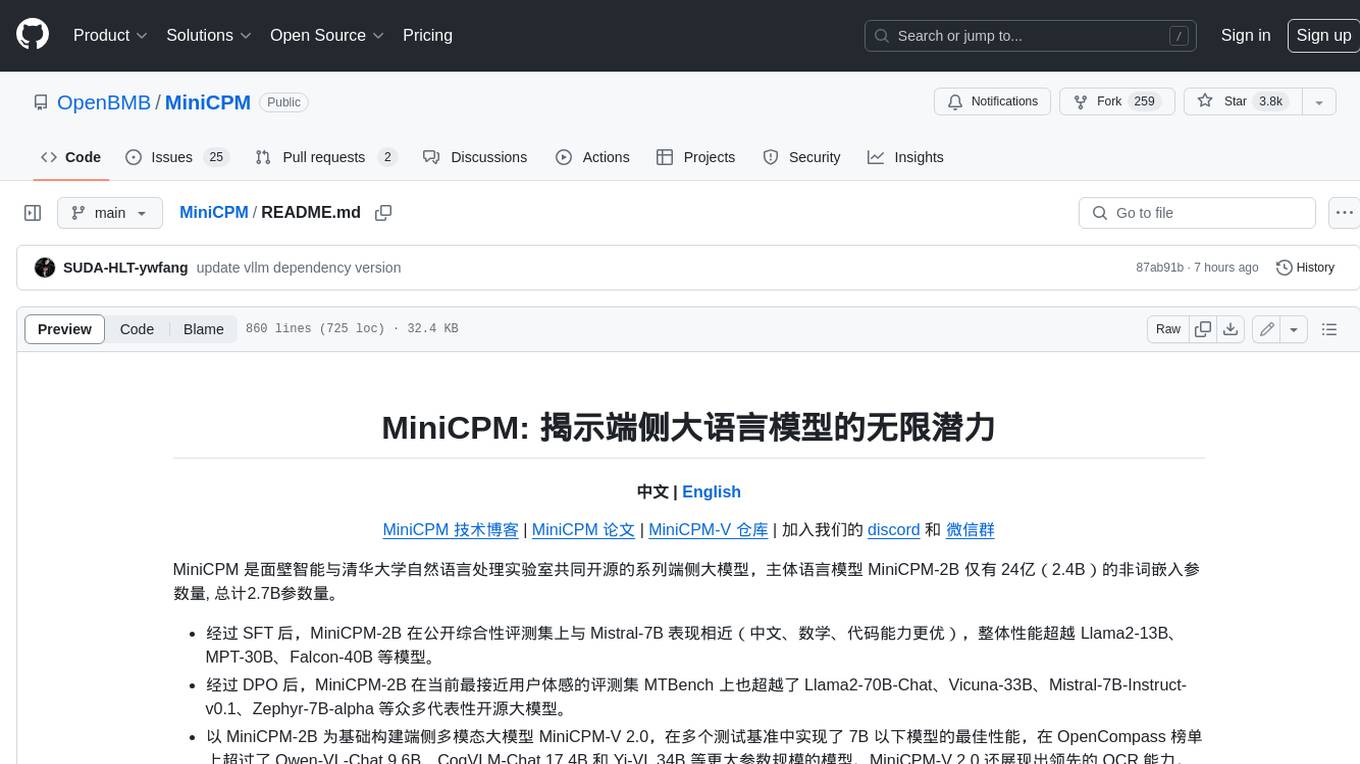
MiniCPM
MiniCPM is a series of open-source large models on the client side jointly developed by Face Intelligence and Tsinghua University Natural Language Processing Laboratory. The main language model MiniCPM-2B has only 2.4 billion (2.4B) non-word embedding parameters, with a total of 2.7B parameters. - After SFT, MiniCPM-2B performs similarly to Mistral-7B on public comprehensive evaluation sets (better in Chinese, mathematics, and code capabilities), and outperforms models such as Llama2-13B, MPT-30B, and Falcon-40B overall. - After DPO, MiniCPM-2B also surpasses many representative open-source large models such as Llama2-70B-Chat, Vicuna-33B, Mistral-7B-Instruct-v0.1, and Zephyr-7B-alpha on the current evaluation set MTBench, which is closest to the user experience. - Based on MiniCPM-2B, a multi-modal large model MiniCPM-V 2.0 on the client side is constructed, which achieves the best performance of models below 7B in multiple test benchmarks, and surpasses larger parameter scale models such as Qwen-VL-Chat 9.6B, CogVLM-Chat 17.4B, and Yi-VL 34B on the OpenCompass leaderboard. MiniCPM-V 2.0 also demonstrates leading OCR capabilities, approaching Gemini Pro in scene text recognition capabilities. - After Int4 quantization, MiniCPM can be deployed and inferred on mobile phones, with a streaming output speed slightly higher than human speech speed. MiniCPM-V also directly runs through the deployment of multi-modal large models on mobile phones. - A single 1080/2080 can efficiently fine-tune parameters, and a single 3090/4090 can fully fine-tune parameters. A single machine can continuously train MiniCPM, and the secondary development cost is relatively low.
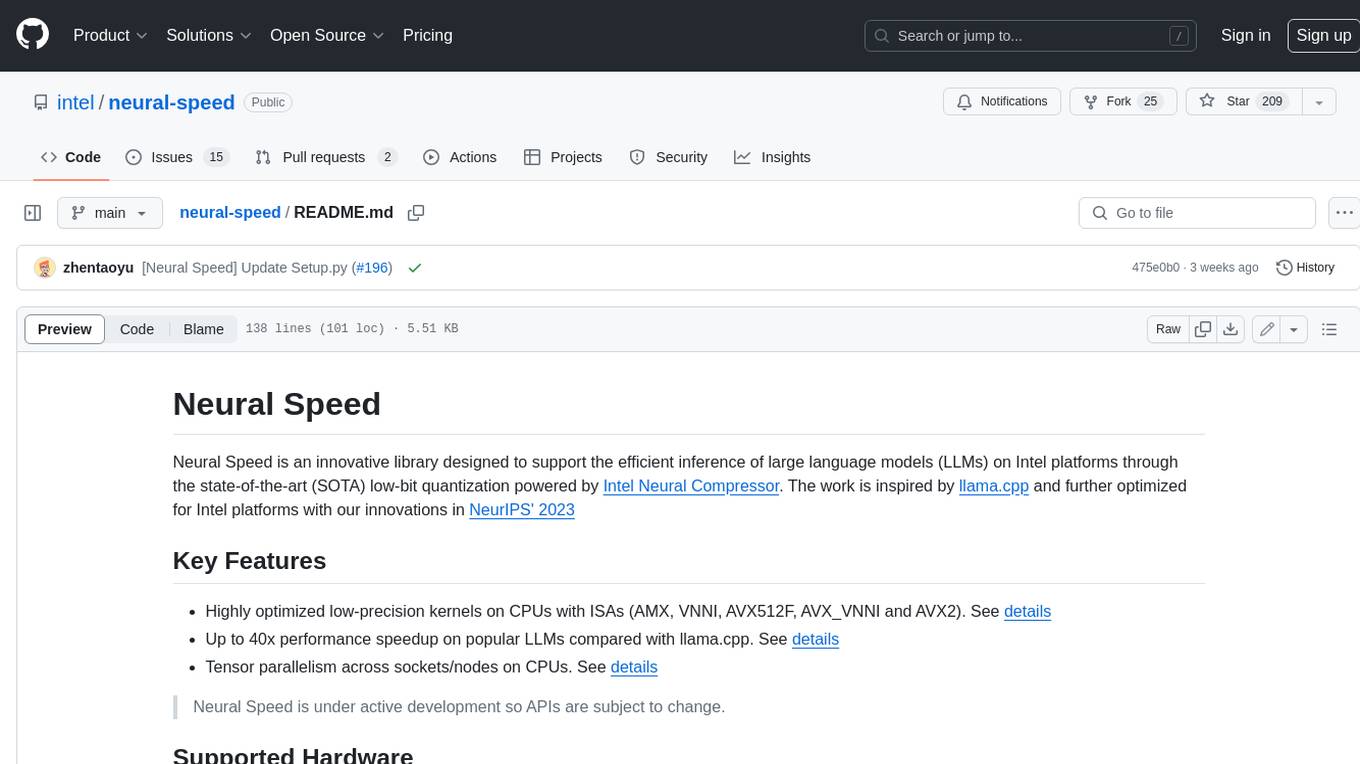
neural-speed
Neural Speed is an innovative library designed to support the efficient inference of large language models (LLMs) on Intel platforms through the state-of-the-art (SOTA) low-bit quantization powered by Intel Neural Compressor. The work is inspired by llama.cpp and further optimized for Intel platforms with our innovations in NeurIPS' 2023