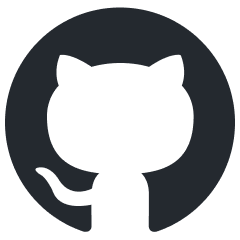
spring-ai
An Application Framework for AI Engineering
Stars: 4447
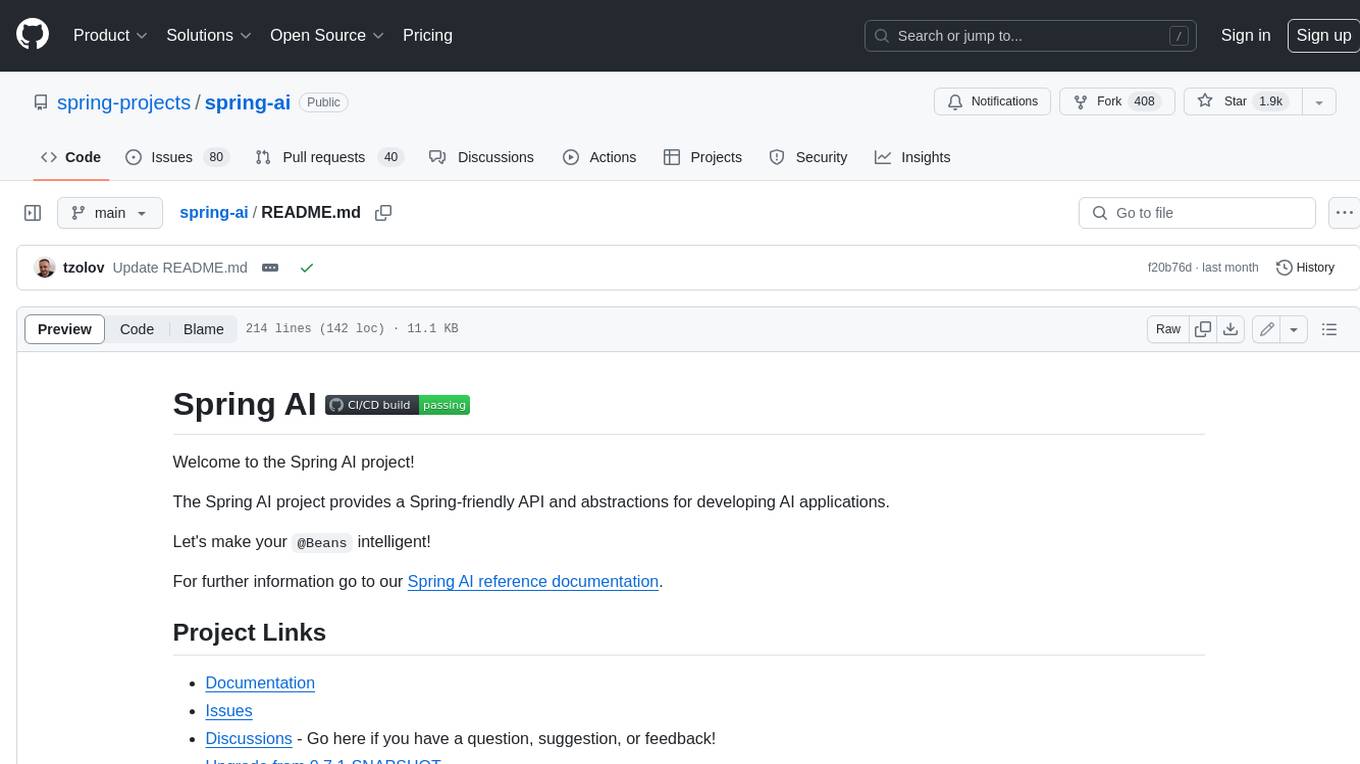
The Spring AI project provides a Spring-friendly API and abstractions for developing AI applications. It offers a portable client API for interacting with generative AI models, enabling developers to easily swap out implementations and access various models like OpenAI, Azure OpenAI, and HuggingFace. Spring AI also supports prompt engineering, providing classes and interfaces for creating and parsing prompts, as well as incorporating proprietary data into generative AI without retraining the model. This is achieved through Retrieval Augmented Generation (RAG), which involves extracting, transforming, and loading data into a vector database for use by AI models. Spring AI's VectorStore abstraction allows for seamless transitions between different vector database implementations.
README:
The Spring AI project provides a Spring-friendly API and abstractions for developing AI applications.
Its goal is to apply to the AI domain Spring ecosystem design principles such as portability and modular design and promote using POJOs as the building blocks of an application to the AI domain.
At its core, Spring AI addresses the fundamental challenge of AI integration: Connecting your enterprise Data and APIs with the AI Models.
The project draws inspiration from notable Python projects, such as LangChain and LlamaIndex, but Spring AI is not a direct port of those projects. The project was founded with the belief that the next wave of Generative AI applications will not be only for Python developers but will be ubiquitous across many programming languages.
You can check out the blog post Why Spring AI for additional motivations.
This is a high level feature overview. You can find more details in the Reference Documentation
- Support for all major AI Model providers such as Anthropic, OpenAI, Microsoft, Amazon, Google, and Ollama. Supported model types include:
- Portable API support across AI providers for both synchronous and streaming options. Access to model-specific features is also available.
- Structured Outputs - Mapping of AI Model output to POJOs.
- Support for all major Vector Database providers such as Apache Cassandra, Azure Vector Search, Chroma, Milvus, MongoDB Atlas, MariaDB, Neo4j, Oracle, PostgreSQL/PGVector, PineCone, Qdrant, Redis, and Weaviate.
- Portable API across Vector Store providers, including a novel SQL-like metadata filter API.
- Tools/Function Calling - permits the model to request the execution of client-side tools and functions, thereby accessing necessary real-time information as required.
- Observability - Provides insights into AI-related operations.
- Document injection ETL framework for Data Engineering.
- AI Model Evaluation - Utilities to help evaluate generated content and protect against hallucinated response.
- ChatClient API - Fluent API for communicating with AI Chat Models, idiomatically similar to the WebClient and RestClient APIs.
- Advisors API - Encapsulates recurring Generative AI patterns, transforms data sent to and from Language Models (LLMs), and provides portability across various models and use cases.
- Support for Chat Conversation Memory and Retrieval Augmented Generation (RAG).
- Spring Boot Auto Configuration and Starters for all AI Models and Vector Stores - use the start.spring.io to select the Model or Vector-store of choice.
Please refer to the Getting Started Guide for instruction on adding your dependencies.
- Awesome Spring AI - A curated list of awesome resources, tools, tutorials, and projects for building generative AI applications using Spring AI
- Spring AI Examples contains example projects that explain specific features in more detail.
- Refer to the upgrade notes to see how to upgrade to 1.0.0.M1 or higher.
This repository contains large model files. To clone it you have to either:
- Ignore the large files (won't affect the spring-ai behaviour) :
GIT_LFS_SKIP_SMUDGE=1 git clone [email protected]:spring-projects/spring-ai.git
. - Or install the Git Large File Storage before cloning the repo.
To build with running unit tests
./mvnw clean package
To build including integration tests.
./mvnw clean verify -Pintegration-tests
Note that you should set API key environment variables for OpenAI or other model providers before running. If the API key isn't set for a specific model provider, the integration test is skipped.
To run a specific integration test allowing for up to two attempts to succeed. This is useful when a hosted service is not reliable or times out.
./mvnw -pl vector-stores/spring-ai-pgvector-store -Pintegration-tests -Dfailsafe.rerunFailingTestsCount=2 -Dit.test=PgVectorStoreIT verify
There are many integration tests ,so it often isn't realistic to run them all at once.
A quick pass through the most important pathways that runs integration tests for
- OpenAI models
- OpenAI autoconfiguration
- PGVector
- Chroma
can be done with the profile -Pci-fast-integration-tests
and is used in the main CI build of this project.
A full integration test is done twice a day in the Spring AI Integration Test Repository
One way to run integration tests on part of the code is to first do a quick compile and install of the project
./mvnw clean install -DskipTests -Dmaven.javadoc.skip=true
Then run the integration test for a specific module using the -pl
option
./mvnw verify -Pintegration-tests -pl spring-ai-spring-boot-testcontainers
To build the docs
./mvnw -pl spring-ai-docs antora
The docs are then in the directory spring-ai-docs/target/antora/site/index.html
To reformat using the java-format plugin
./mvnw spring-javaformat:apply
To update the year on license headers using the license-maven-plugin
./mvnw license:update-file-header -Plicense
To check javadocs using the javadoc:javadoc
./mvnw javadoc:javadoc -Pjavadoc
To build with checkstyles enabled. Checkstyles are currently disabled, but you can enable them by doing the following:
./mvnw clean package -DskipTests -Ddisable.checks=false
For Tasks:
Click tags to check more tools for each tasksFor Jobs:
Alternative AI tools for spring-ai
Similar Open Source Tools
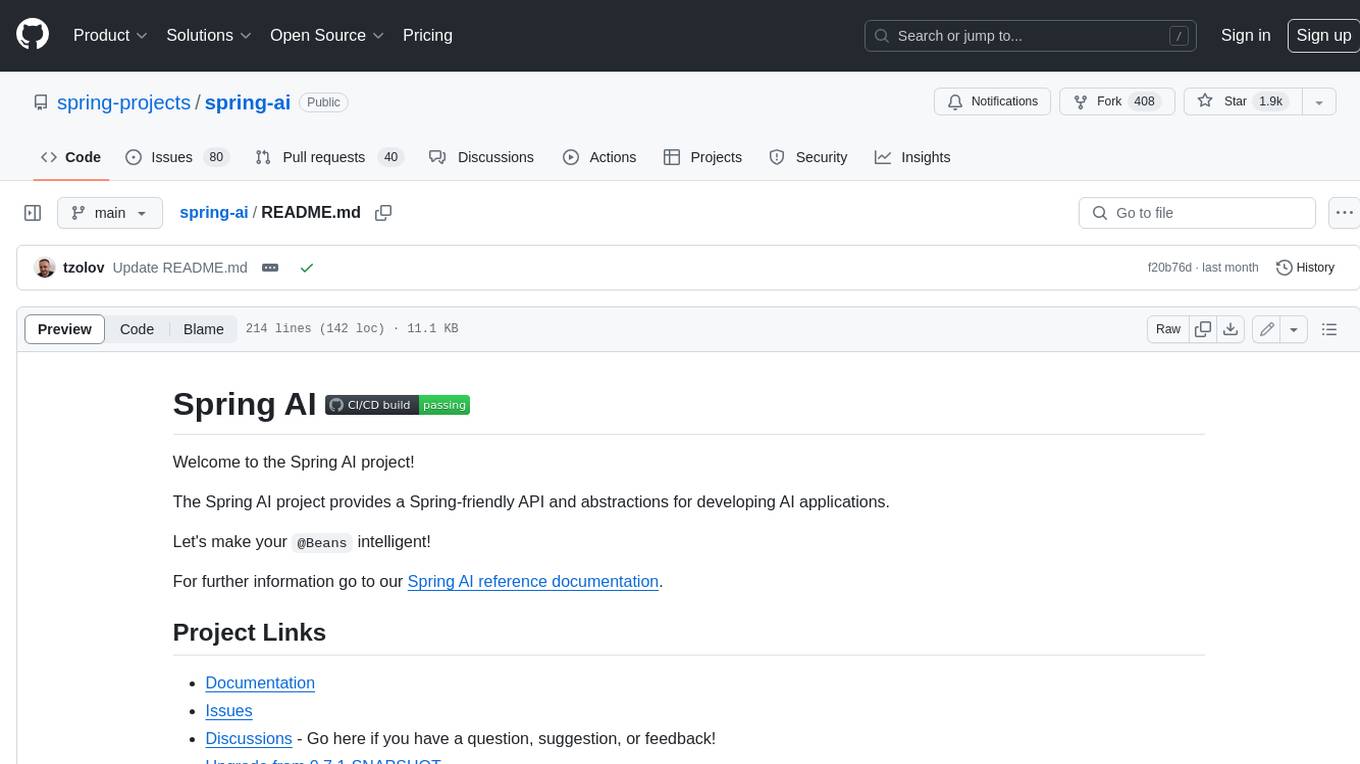
spring-ai
The Spring AI project provides a Spring-friendly API and abstractions for developing AI applications. It offers a portable client API for interacting with generative AI models, enabling developers to easily swap out implementations and access various models like OpenAI, Azure OpenAI, and HuggingFace. Spring AI also supports prompt engineering, providing classes and interfaces for creating and parsing prompts, as well as incorporating proprietary data into generative AI without retraining the model. This is achieved through Retrieval Augmented Generation (RAG), which involves extracting, transforming, and loading data into a vector database for use by AI models. Spring AI's VectorStore abstraction allows for seamless transitions between different vector database implementations.
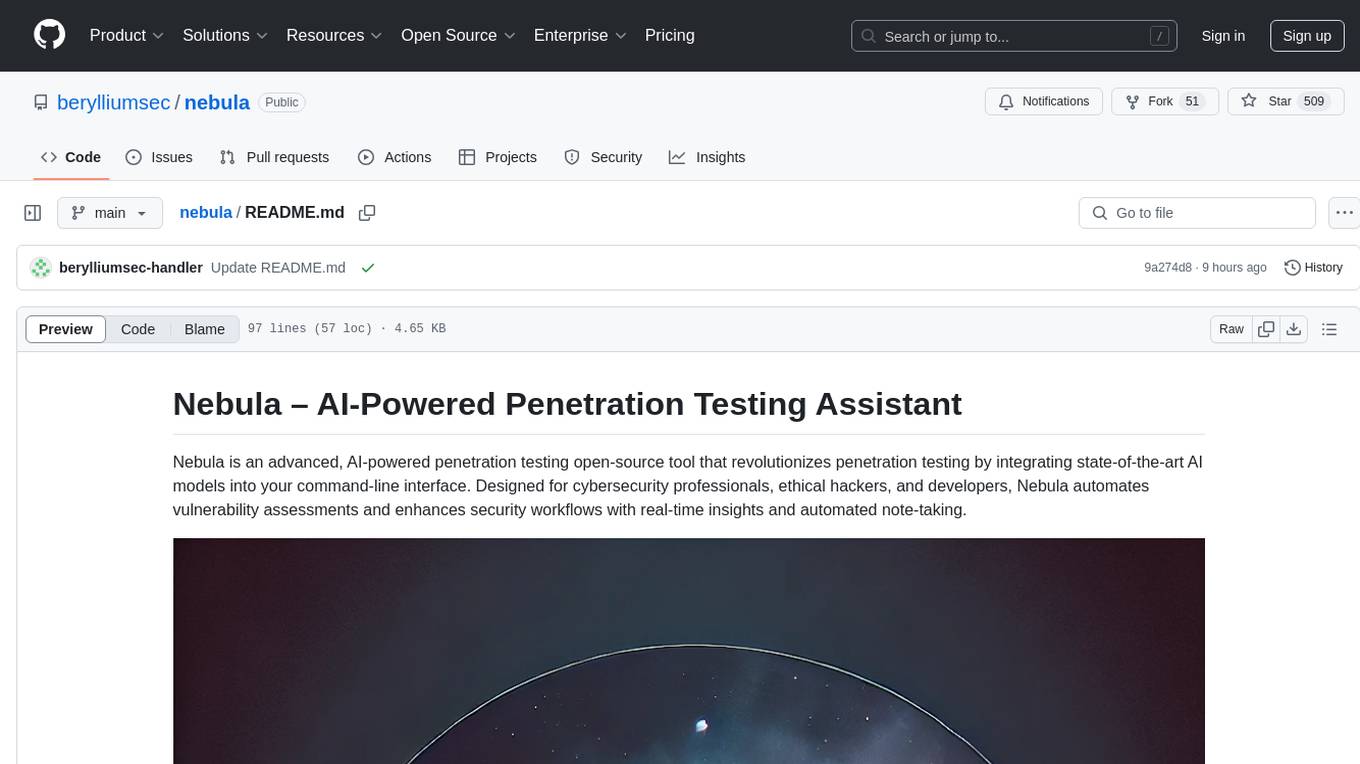
nebula
Nebula is an advanced, AI-powered penetration testing tool designed for cybersecurity professionals, ethical hackers, and developers. It integrates state-of-the-art AI models into the command-line interface, automating vulnerability assessments and enhancing security workflows with real-time insights and automated note-taking. Nebula revolutionizes penetration testing by providing AI-driven insights, enhanced tool integration, AI-assisted note-taking, and manual note-taking features. It also supports any tool that can be invoked from the CLI, making it a versatile and powerful tool for cybersecurity tasks.
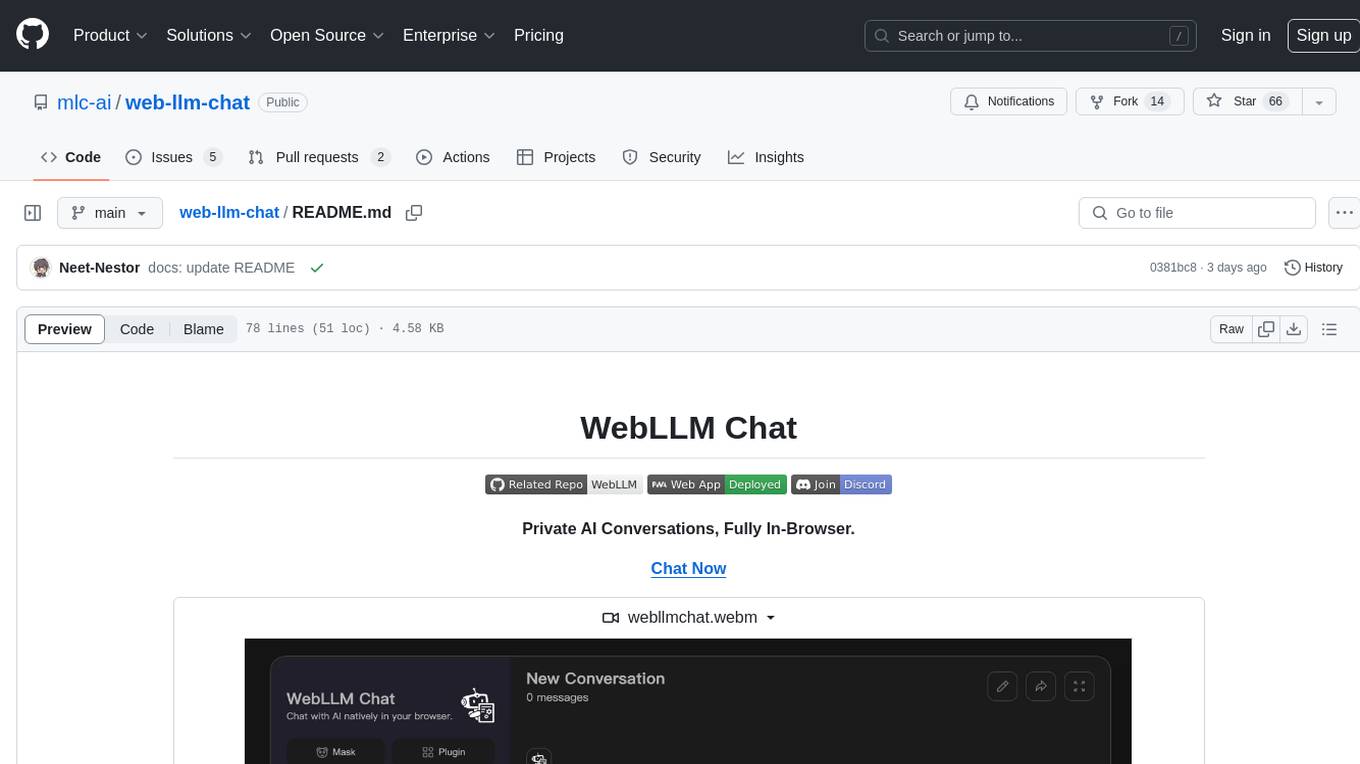
web-llm-chat
WebLLM Chat is a private AI chat interface that combines WebLLM with a user-friendly design, leveraging WebGPU to run large language models natively in your browser. It offers browser-native AI experience with WebGPU acceleration, guaranteed privacy as all data processing happens locally, offline accessibility, user-friendly interface with markdown support, and open-source customization. The project aims to democratize AI technology by making powerful tools accessible directly to end-users, enhancing the chatting experience and broadening the scope for deployment of self-hosted and customizable language models.
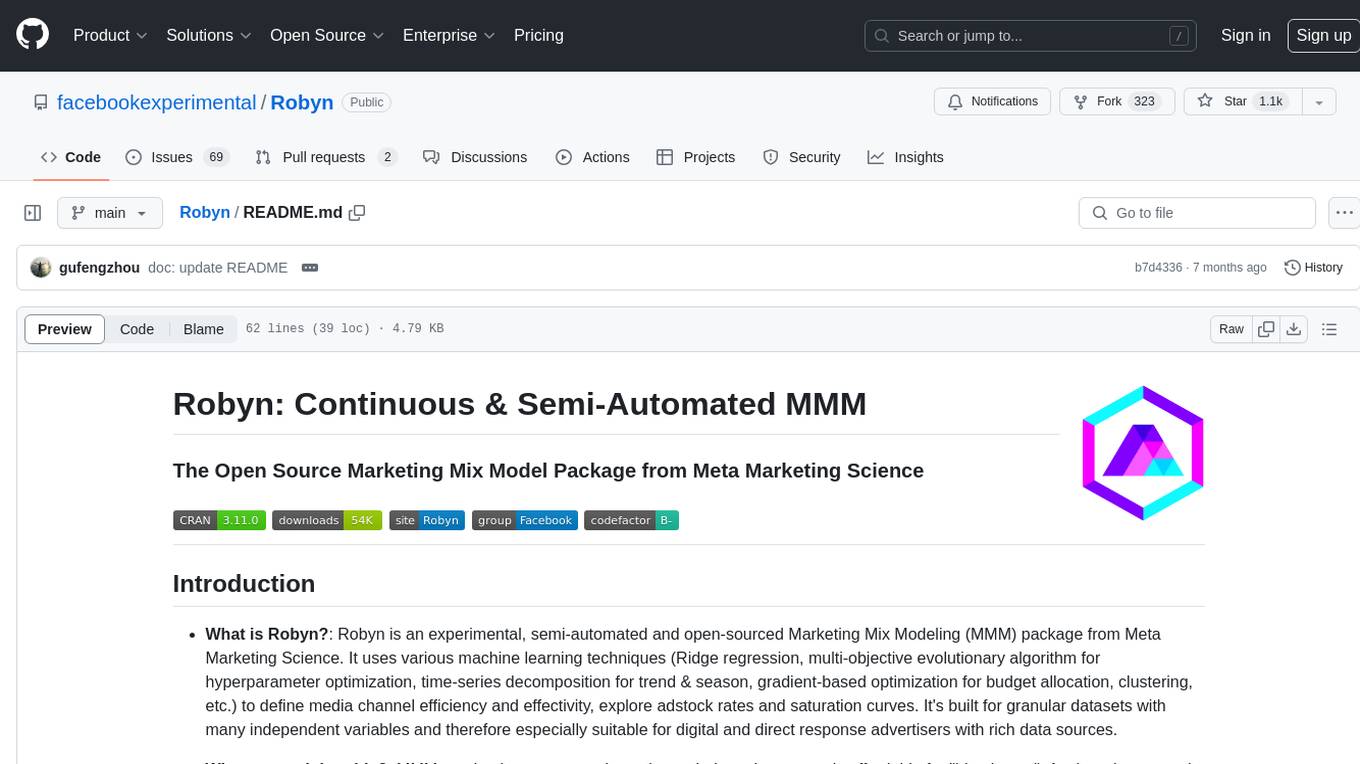
Robyn
Robyn is an experimental, semi-automated and open-sourced Marketing Mix Modeling (MMM) package from Meta Marketing Science. It uses various machine learning techniques to define media channel efficiency and effectivity, explore adstock rates and saturation curves. Built for granular datasets with many independent variables, especially suitable for digital and direct response advertisers with rich data sources. Aiming to democratize MMM, make it accessible for advertisers of all sizes, and contribute to the measurement landscape.
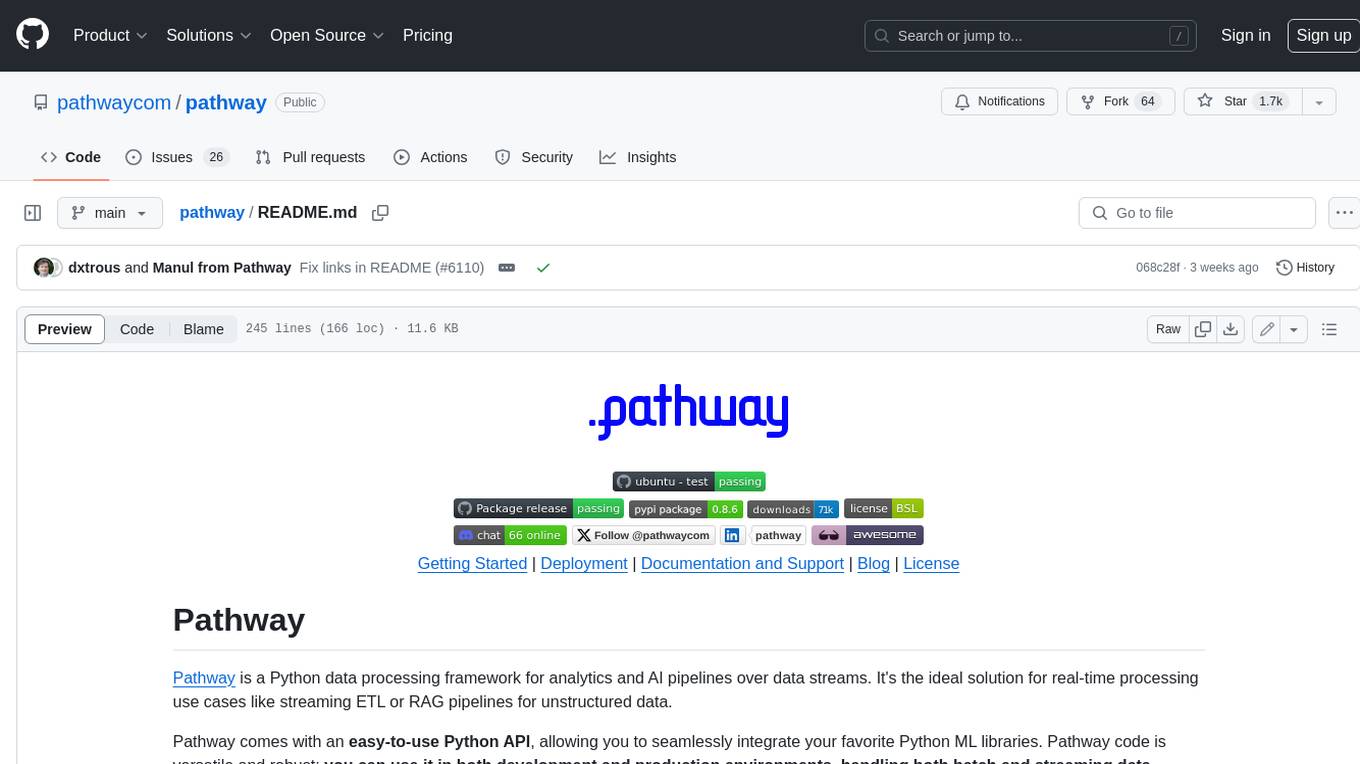
pathway
Pathway is a Python data processing framework for analytics and AI pipelines over data streams. It's the ideal solution for real-time processing use cases like streaming ETL or RAG pipelines for unstructured data. Pathway comes with an **easy-to-use Python API** , allowing you to seamlessly integrate your favorite Python ML libraries. Pathway code is versatile and robust: **you can use it in both development and production environments, handling both batch and streaming data effectively**. The same code can be used for local development, CI/CD tests, running batch jobs, handling stream replays, and processing data streams. Pathway is powered by a **scalable Rust engine** based on Differential Dataflow and performs incremental computation. Your Pathway code, despite being written in Python, is run by the Rust engine, enabling multithreading, multiprocessing, and distributed computations. All the pipeline is kept in memory and can be easily deployed with **Docker and Kubernetes**. You can install Pathway with pip: `pip install -U pathway` For any questions, you will find the community and team behind the project on Discord.
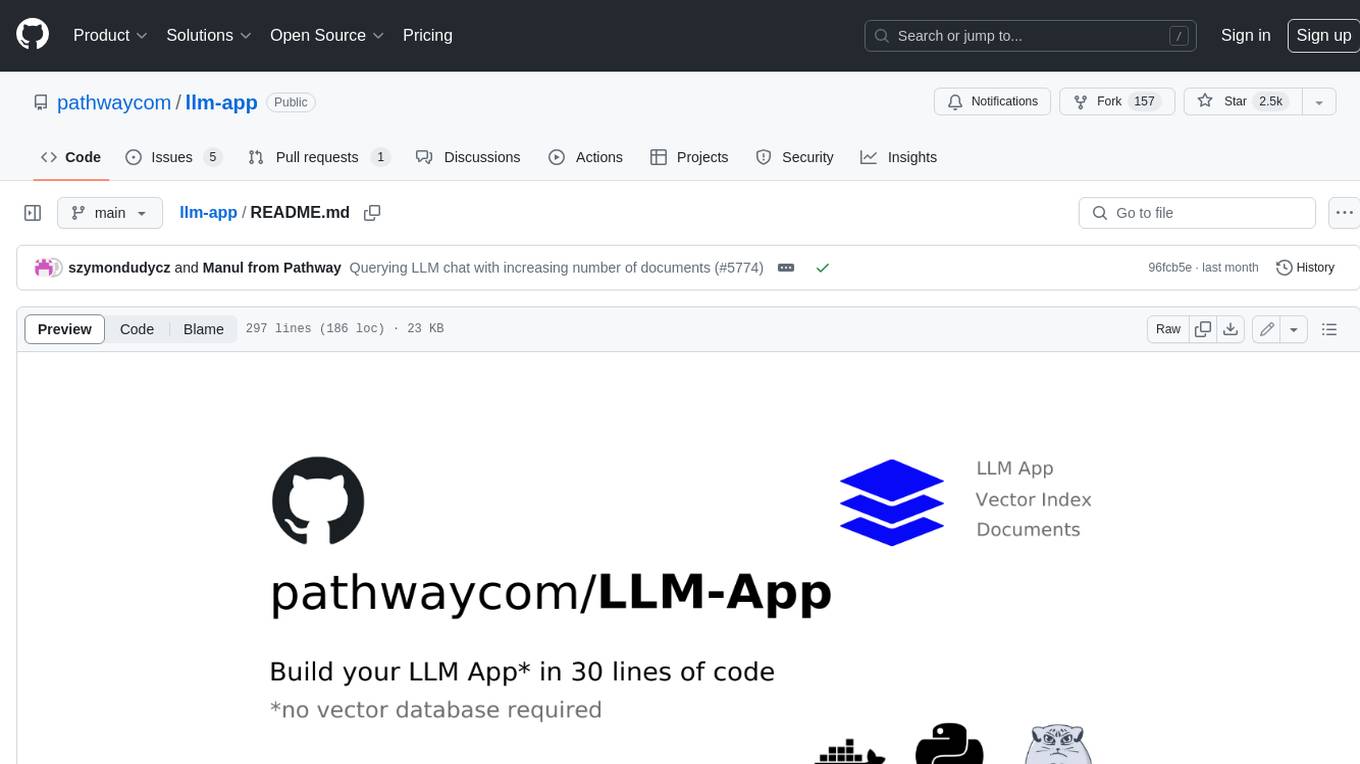
llm-app
Pathway's LLM (Large Language Model) Apps provide a platform to quickly deploy AI applications using the latest knowledge from data sources. The Python application examples in this repository are Docker-ready, exposing an HTTP API to the frontend. These apps utilize the Pathway framework for data synchronization, API serving, and low-latency data processing without the need for additional infrastructure dependencies. They connect to document data sources like S3, Google Drive, and Sharepoint, offering features like real-time data syncing, easy alert setup, scalability, monitoring, security, and unification of application logic.
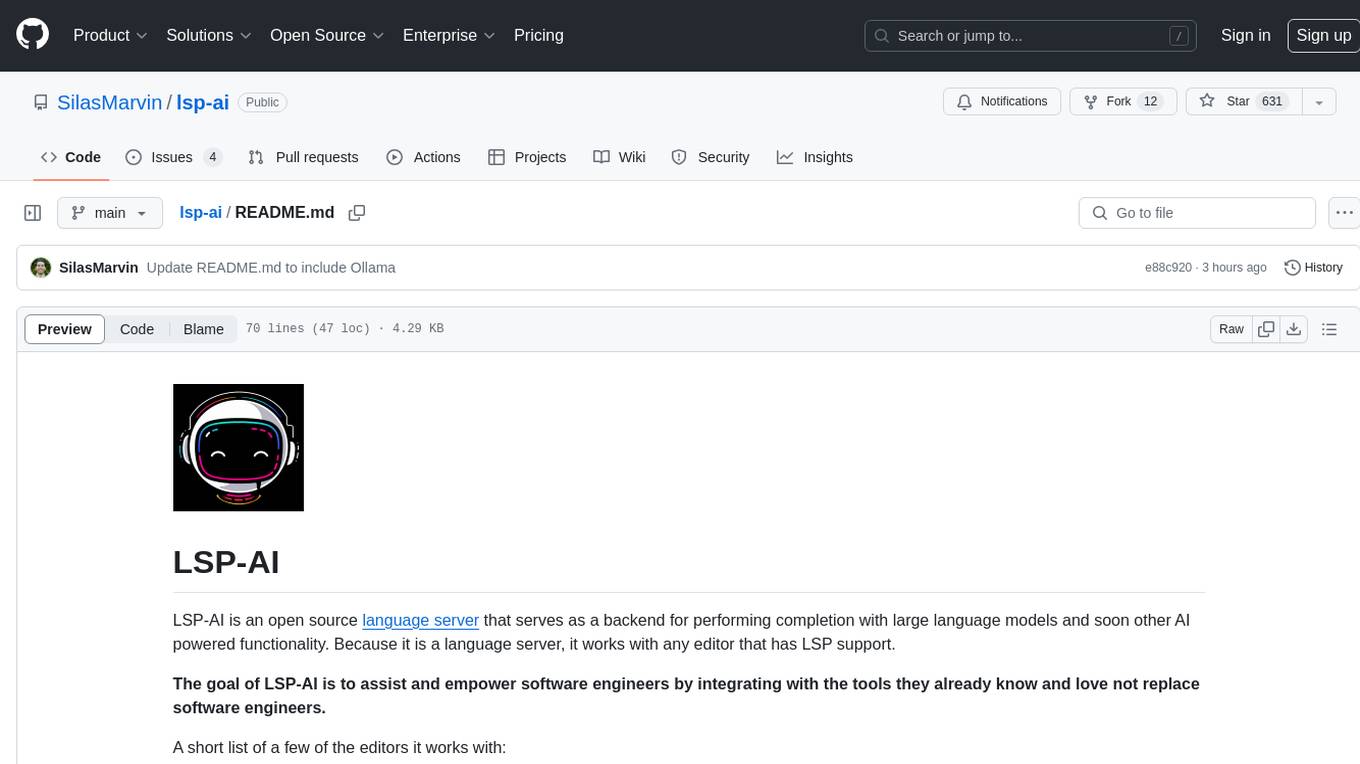
lsp-ai
LSP-AI is an open source language server designed to enhance software engineers' productivity by integrating AI-powered functionality into various text editors. It serves as a backend for completion with large language models and offers features like unified AI capabilities, simplified plugin development, enhanced collaboration, broad compatibility with editors supporting Language Server Protocol, flexible LLM backend support, and commitment to staying updated with the latest advancements in LLM-driven software development. The tool aims to centralize open-source development work, provide a collaborative platform for developers, and offer a future-ready solution for AI-powered assistants in text editors.
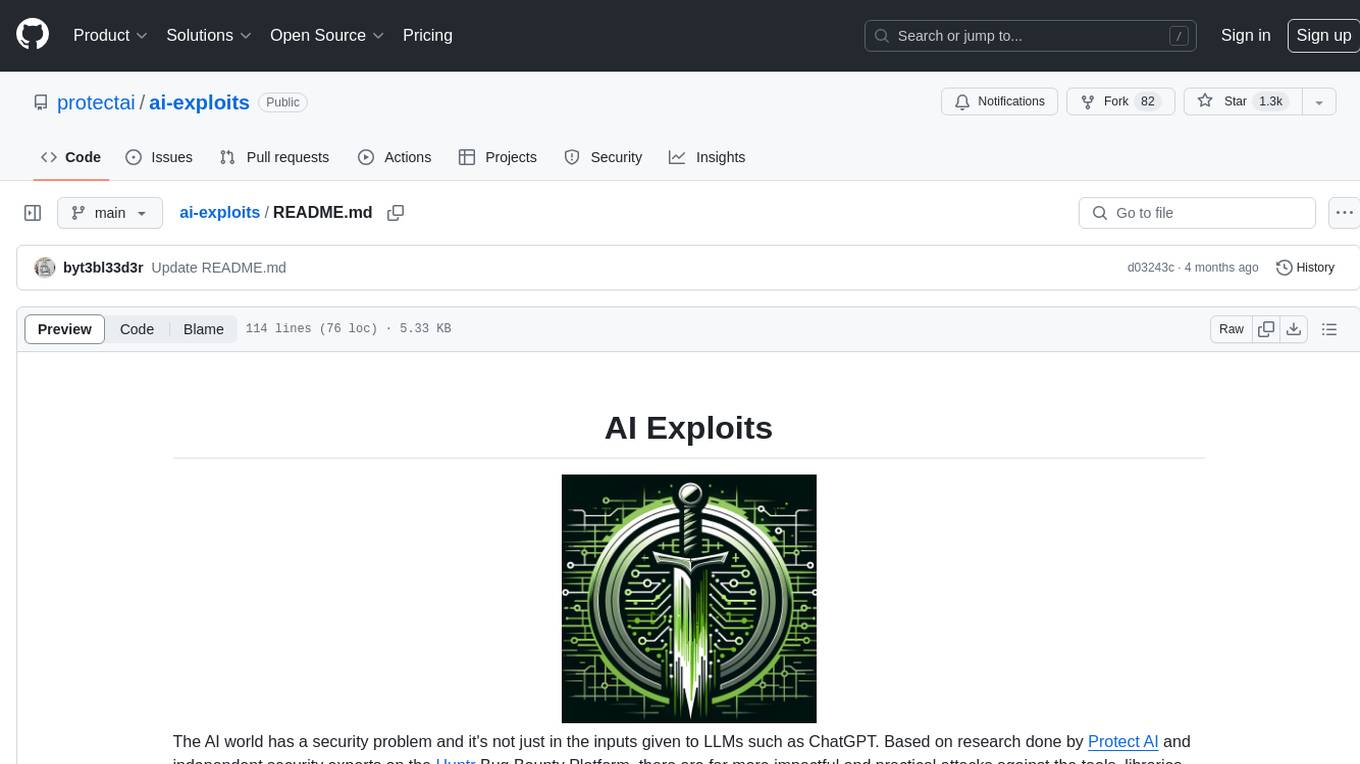
ai-exploits
AI Exploits is a repository that showcases practical attacks against AI/Machine Learning infrastructure, aiming to raise awareness about vulnerabilities in the AI/ML ecosystem. It contains exploits and scanning templates for responsibly disclosed vulnerabilities affecting machine learning tools, including Metasploit modules, Nuclei templates, and CSRF templates. Users can use the provided Docker image to easily run the modules and templates. The repository also provides guidelines for using Metasploit modules, Nuclei templates, and CSRF templates to exploit vulnerabilities in machine learning tools.
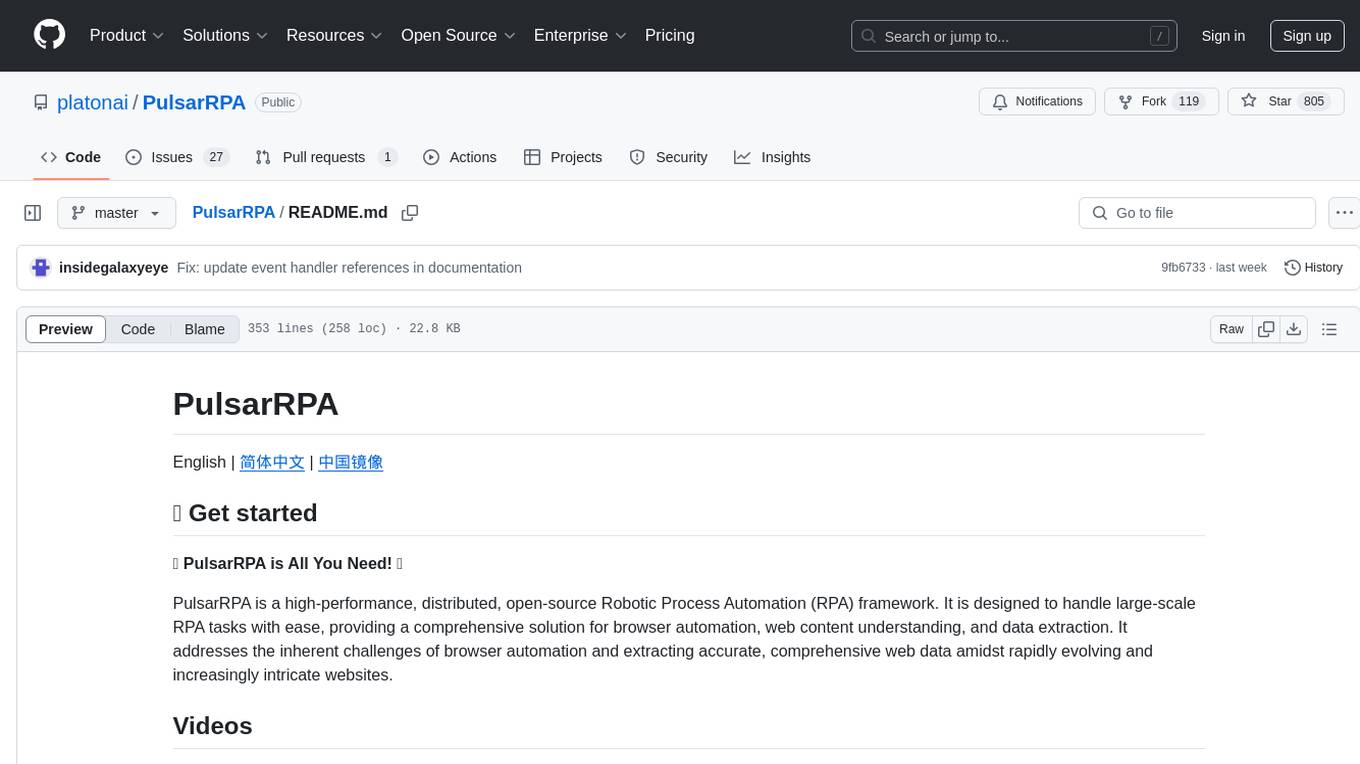
PulsarRPA
PulsarRPA is a high-performance, distributed, open-source Robotic Process Automation (RPA) framework designed to handle large-scale RPA tasks with ease. It provides a comprehensive solution for browser automation, web content understanding, and data extraction. PulsarRPA addresses challenges of browser automation and accurate web data extraction from complex and evolving websites. It incorporates innovative technologies like browser rendering, RPA, intelligent scraping, advanced DOM parsing, and distributed architecture to ensure efficient, accurate, and scalable web data extraction. The tool is open-source, customizable, and supports cutting-edge information extraction technology, making it a preferred solution for large-scale web data extraction.
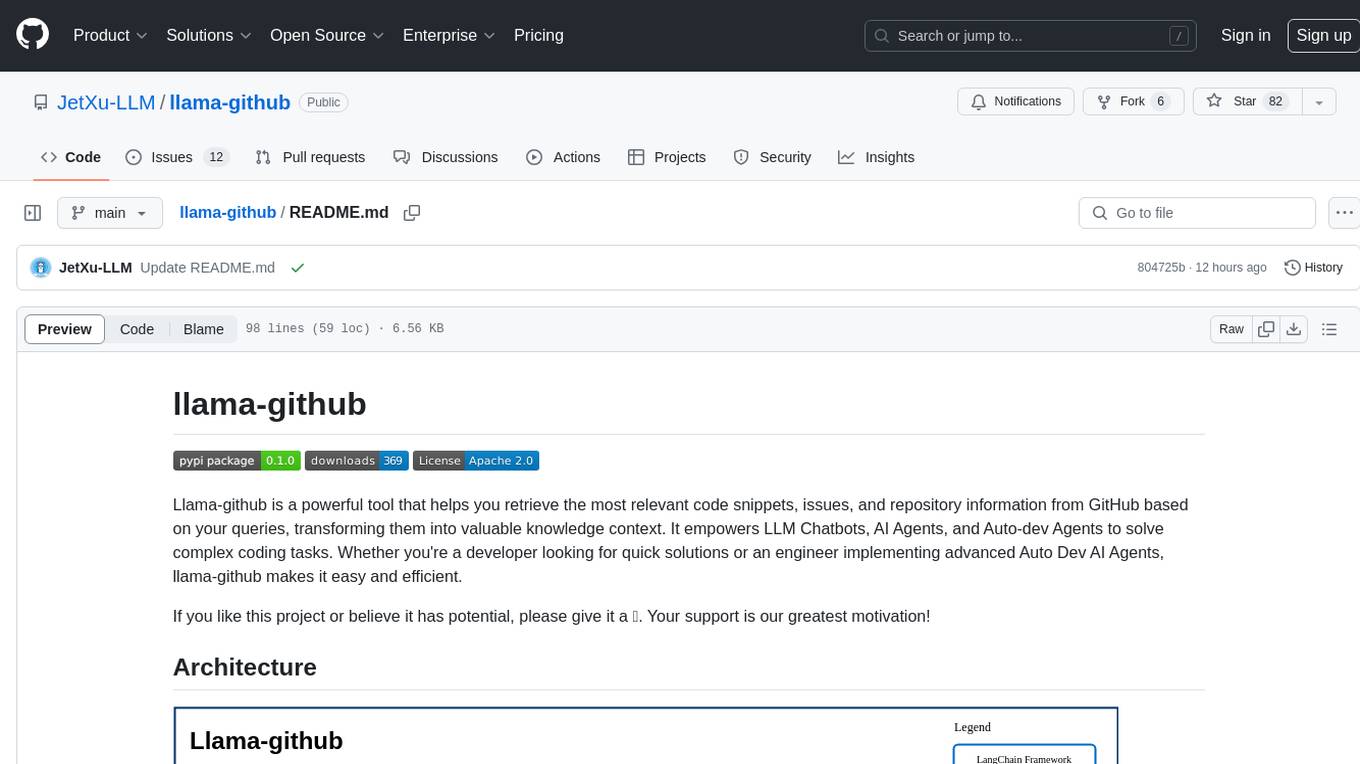
llama-github
Llama-github is a powerful tool that helps retrieve relevant code snippets, issues, and repository information from GitHub based on queries. It empowers AI agents and developers to solve coding tasks efficiently. With features like intelligent GitHub retrieval, repository pool caching, LLM-powered question analysis, and comprehensive context generation, llama-github excels at providing valuable knowledge context for development needs. It supports asynchronous processing, flexible LLM integration, robust authentication options, and logging/error handling for smooth operations and troubleshooting. The vision is to seamlessly integrate with GitHub for AI-driven development solutions, while the roadmap focuses on empowering LLMs to automatically resolve complex coding tasks.
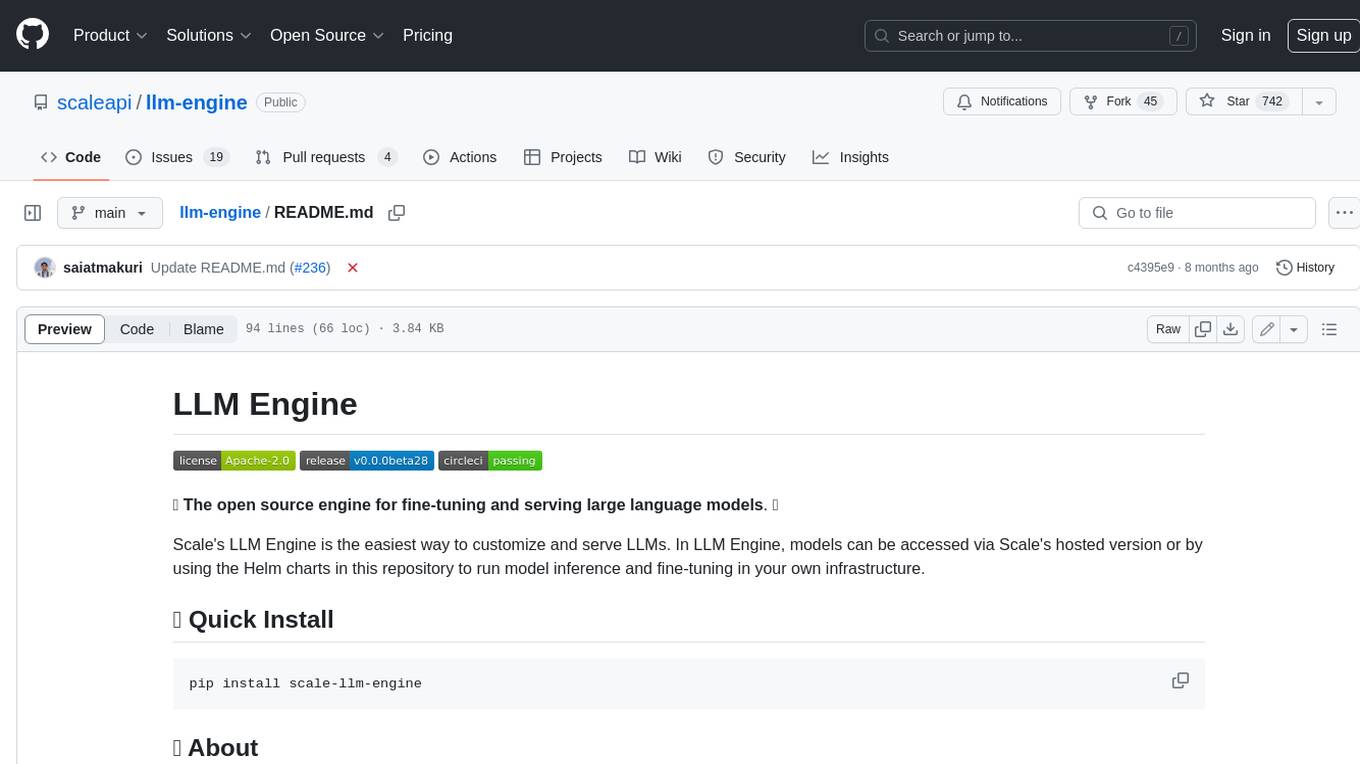
llm-engine
Scale's LLM Engine is an open-source Python library, CLI, and Helm chart that provides everything you need to serve and fine-tune foundation models, whether you use Scale's hosted infrastructure or do it in your own cloud infrastructure using Kubernetes.
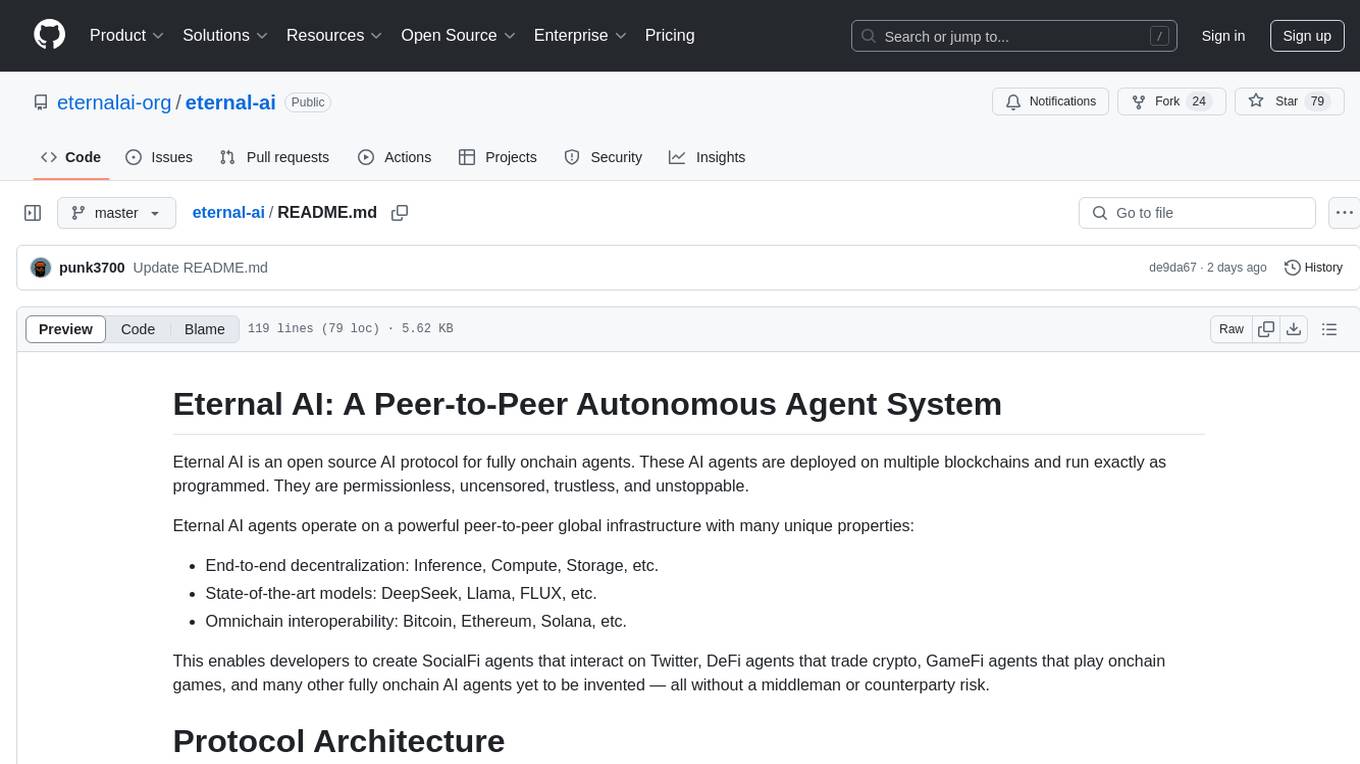
eternal-ai
Eternal AI is an open source AI protocol for fully onchain agents, enabling developers to create various types of onchain AI agents without middlemen. It operates on a decentralized infrastructure with state-of-the-art models and omnichain interoperability. The protocol architecture includes components like ai-kernel, decentralized-agents, decentralized-inference, decentralized-compute, agent-as-a-service, and agent-studio. Ongoing research projects include cuda-evm, nft-ai, and physical-ai. The system requires Node.js, npm, Docker Desktop, Go, and Ollama for installation. The 'eai' CLI tool allows users to create agents, fetch agent info, list agents, and chat with agents. Design principles focus on decentralization, trustlessness, production-grade quality, and unified agent interface. Featured integrations can be quickly implemented, and governance will be overseen by EAI holders in the future.
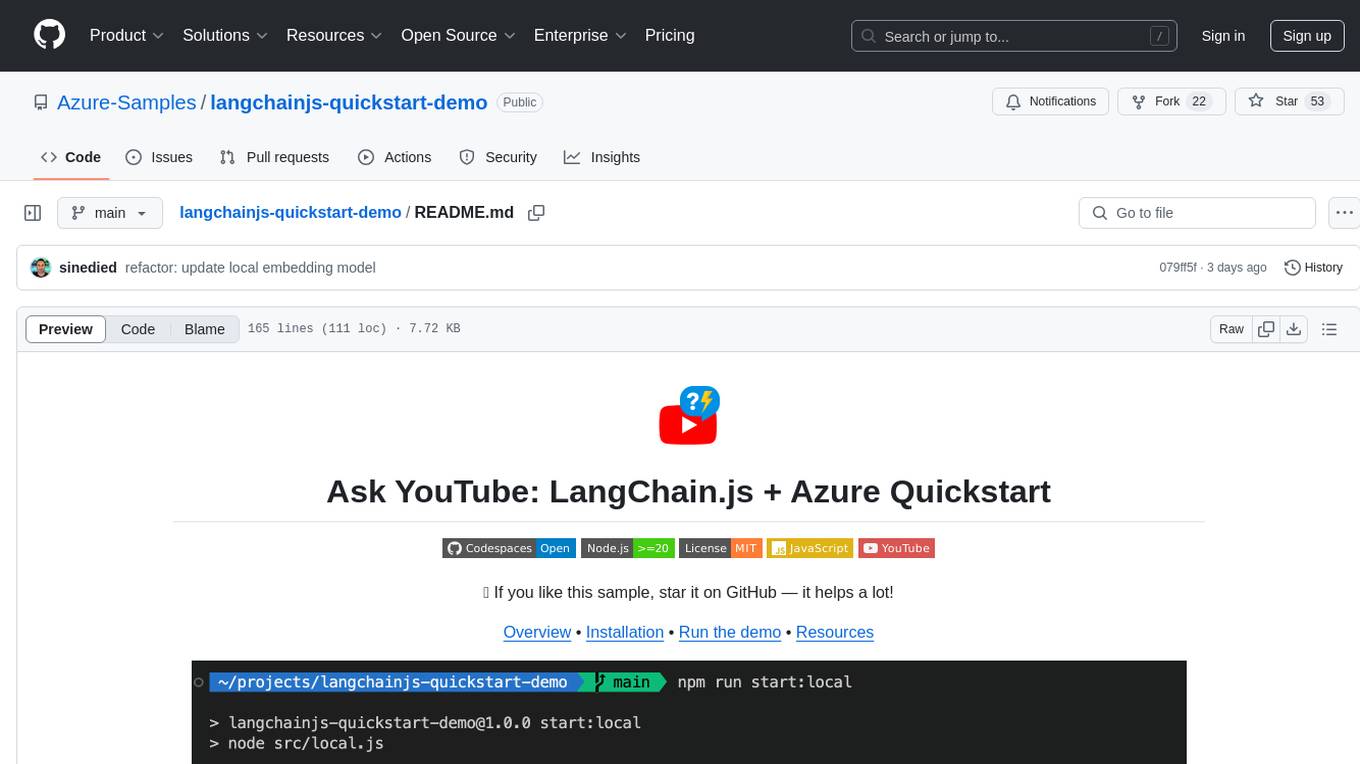
langchainjs-quickstart-demo
Discover the journey of building a generative AI application using LangChain.js and Azure. This demo explores the development process from idea to production, using a RAG-based approach for a Q&A system based on YouTube video transcripts. The application allows to ask text-based questions about a YouTube video and uses the transcript of the video to generate responses. The code comes in two versions: local prototype using FAISS and Ollama with LLaMa3 model for completion and all-minilm-l6-v2 for embeddings, and Azure cloud version using Azure AI Search and GPT-4 Turbo model for completion and text-embedding-3-large for embeddings. Either version can be run as an API using the Azure Functions runtime.
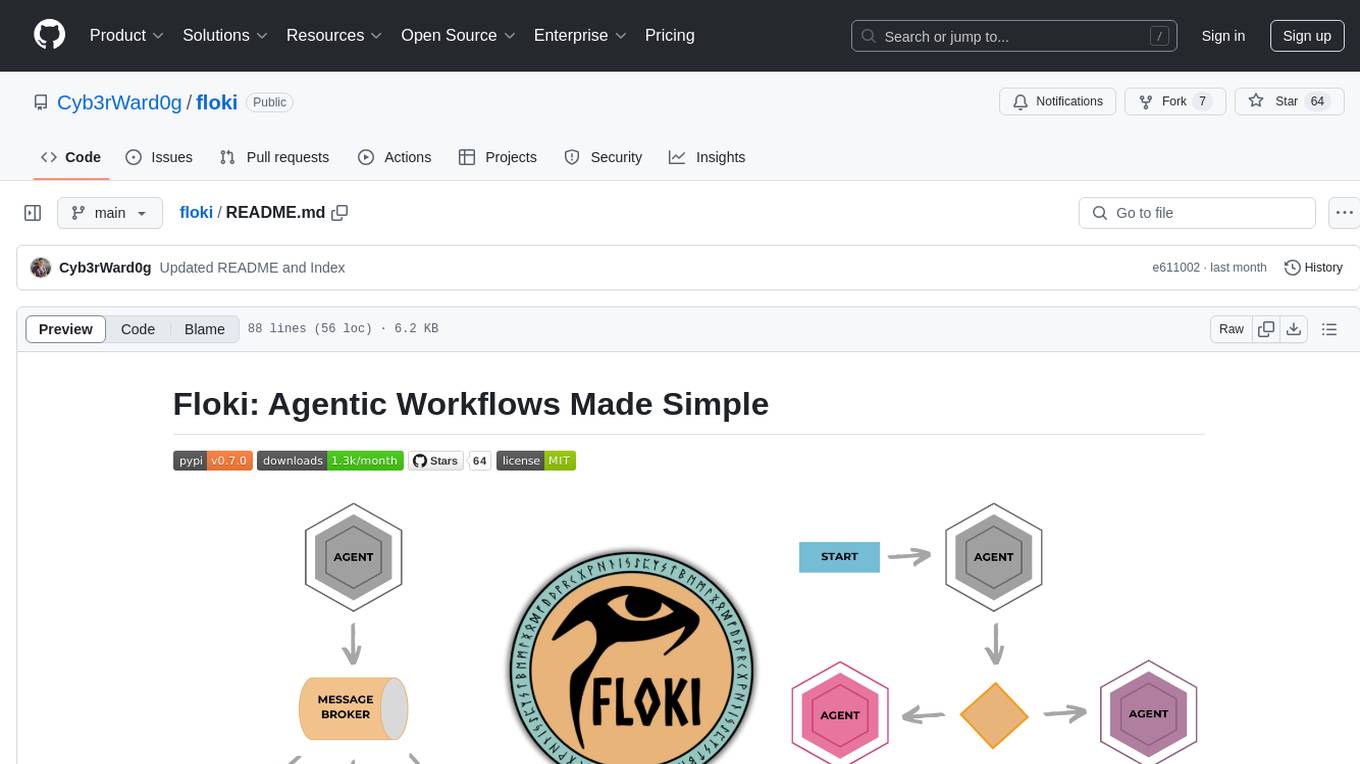
floki
Floki is an open-source framework for researchers and developers to experiment with LLM-based autonomous agents. It provides tools to create, orchestrate, and manage agents while seamlessly connecting to LLM inference APIs. Built on Dapr, Floki leverages a unified programming model that simplifies microservices and supports both deterministic workflows and event-driven interactions. By bringing together these features, Floki provides a powerful way to explore agentic workflows and the components that enable multi-agent systems to collaborate and scale, all powered by Dapr.
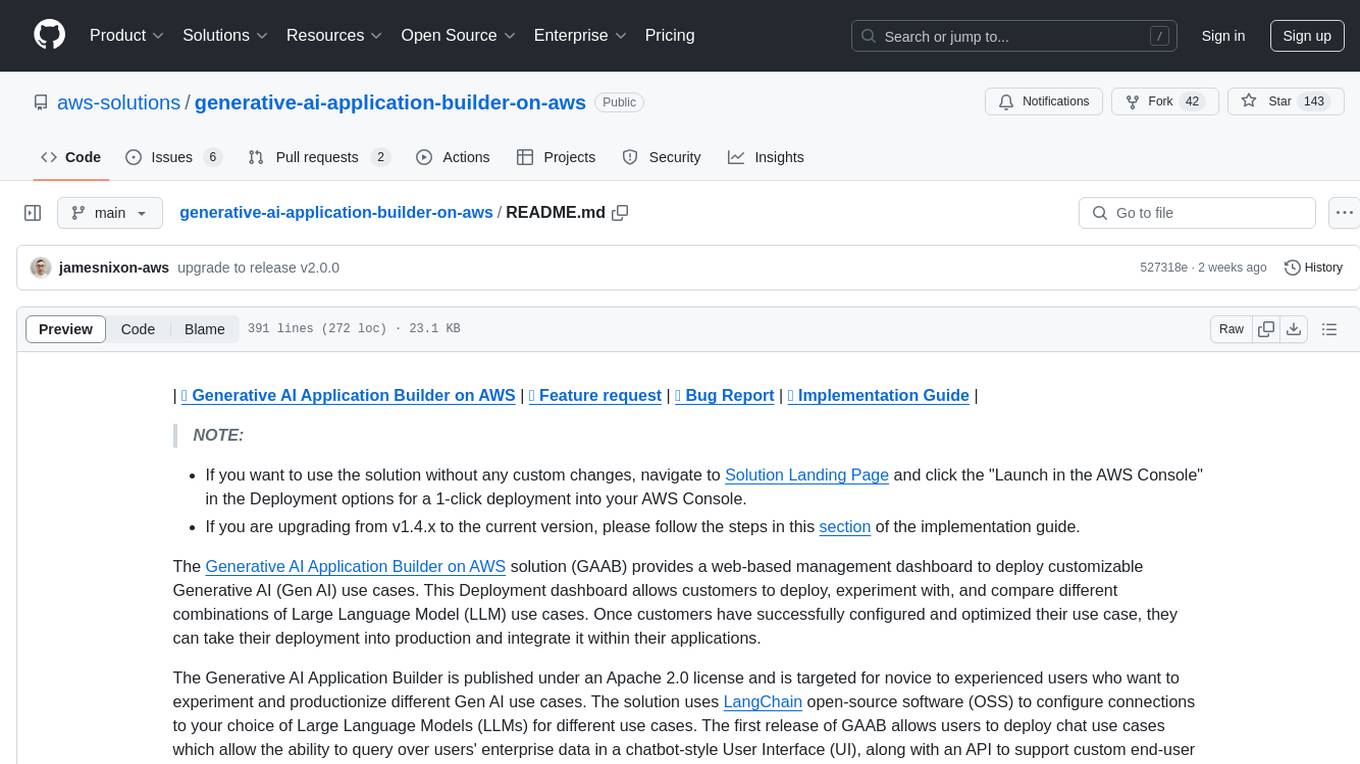
generative-ai-application-builder-on-aws
The Generative AI Application Builder on AWS (GAAB) is a solution that provides a web-based management dashboard for deploying customizable Generative AI (Gen AI) use cases. Users can experiment with and compare different combinations of Large Language Model (LLM) use cases, configure and optimize their use cases, and integrate them into their applications for production. The solution is targeted at novice to experienced users who want to experiment and productionize different Gen AI use cases. It uses LangChain open-source software to configure connections to Large Language Models (LLMs) for various use cases, with the ability to deploy chat use cases that allow querying over users' enterprise data in a chatbot-style User Interface (UI) and support custom end-user implementations through an API.
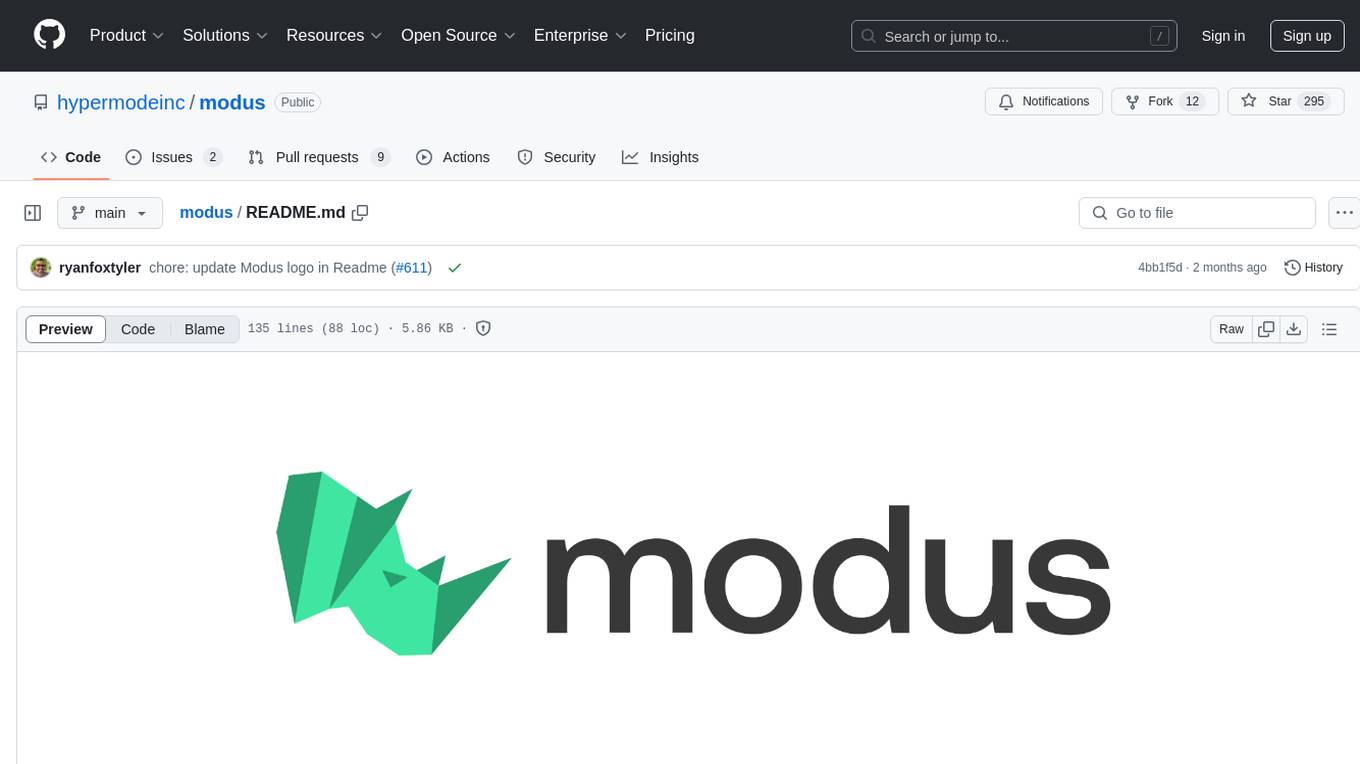
modus
Modus is an open-source, serverless framework for building APIs powered by WebAssembly. It simplifies integrating AI models, data, and business logic with sandboxed execution. Modus extracts metadata, compiles code with optimizations, caches compiled modules, prepares invocation plans, generates API schema, and activates endpoints. Users query the endpoint, and Modus loads compiled code into a sandboxed environment, runs code securely, queries data and AI models, and responds via API. It provides a production-ready scalable endpoint for AI-enabled apps, optimized for sub-second response times. Modus supports programming languages like AssemblyScript and Go, and can be hosted on Hypermode or any platform. Developed by Hypermode as an open-source project, Modus welcomes external contributions.
For similar tasks
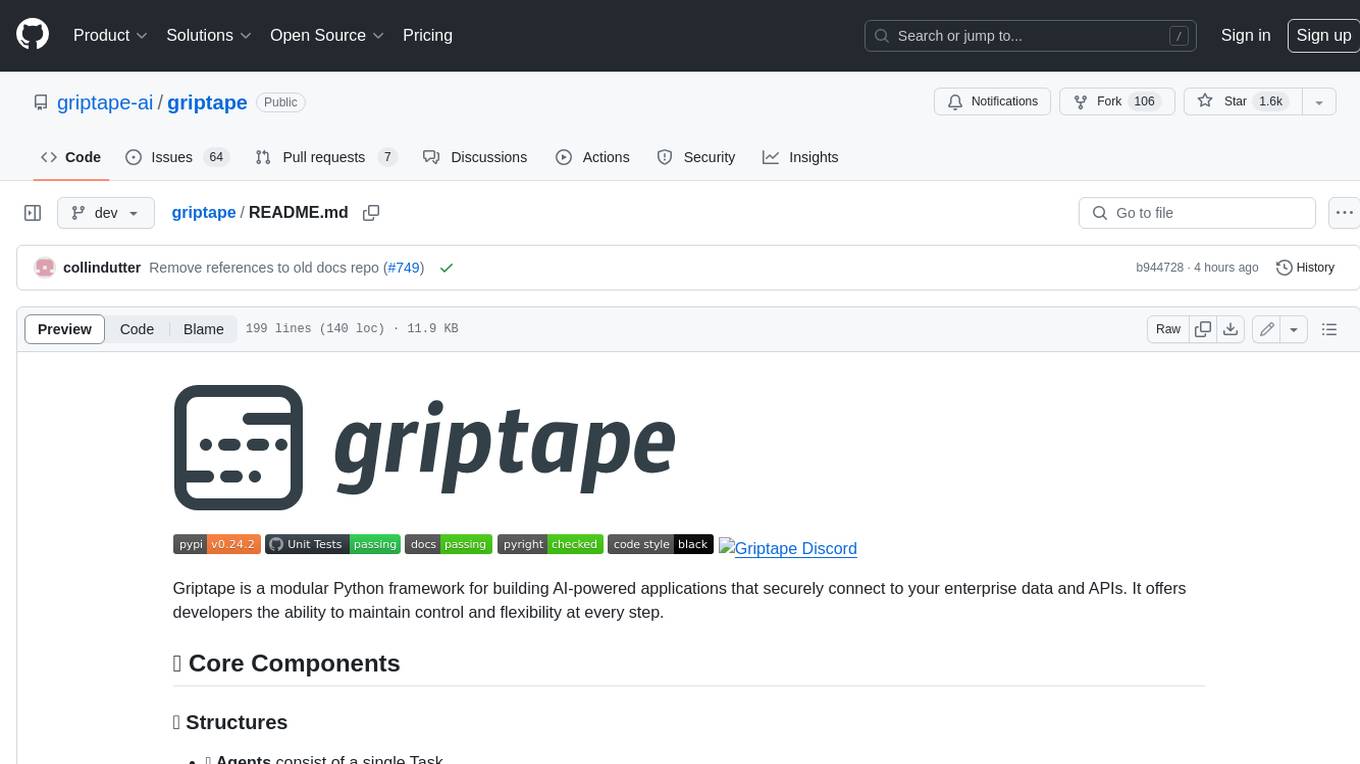
griptape
Griptape is a modular Python framework for building AI-powered applications that securely connect to your enterprise data and APIs. It offers developers the ability to maintain control and flexibility at every step. Griptape's core components include Structures (Agents, Pipelines, and Workflows), Tasks, Tools, Memory (Conversation Memory, Task Memory, and Meta Memory), Drivers (Prompt and Embedding Drivers, Vector Store Drivers, Image Generation Drivers, Image Query Drivers, SQL Drivers, Web Scraper Drivers, and Conversation Memory Drivers), Engines (Query Engines, Extraction Engines, Summary Engines, Image Generation Engines, and Image Query Engines), and additional components (Rulesets, Loaders, Artifacts, Chunkers, and Tokenizers). Griptape enables developers to create AI-powered applications with ease and efficiency.
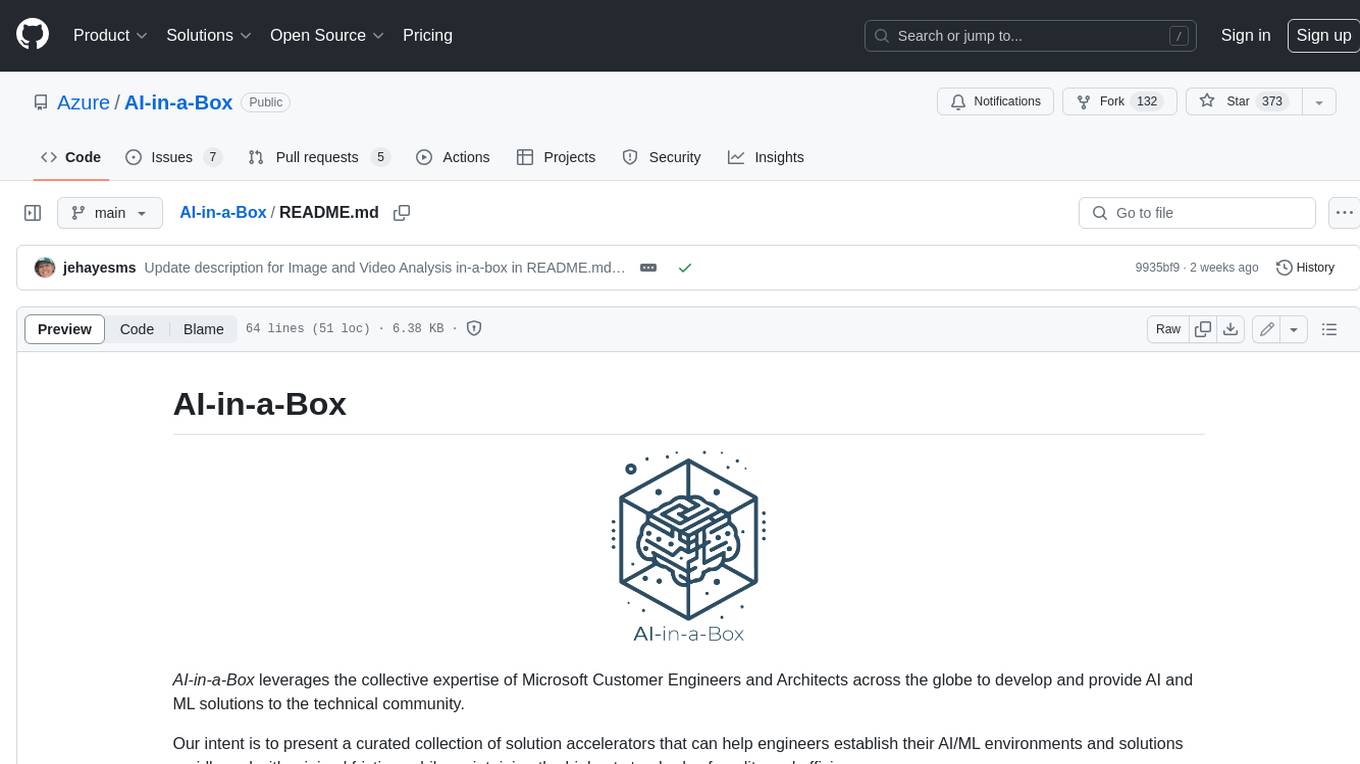
AI-in-a-Box
AI-in-a-Box is a curated collection of solution accelerators that can help engineers establish their AI/ML environments and solutions rapidly and with minimal friction, while maintaining the highest standards of quality and efficiency. It provides essential guidance on the responsible use of AI and LLM technologies, specific security guidance for Generative AI (GenAI) applications, and best practices for scaling OpenAI applications within Azure. The available accelerators include: Azure ML Operationalization in-a-box, Edge AI in-a-box, Doc Intelligence in-a-box, Image and Video Analysis in-a-box, Cognitive Services Landing Zone in-a-box, Semantic Kernel Bot in-a-box, NLP to SQL in-a-box, Assistants API in-a-box, and Assistants API Bot in-a-box.
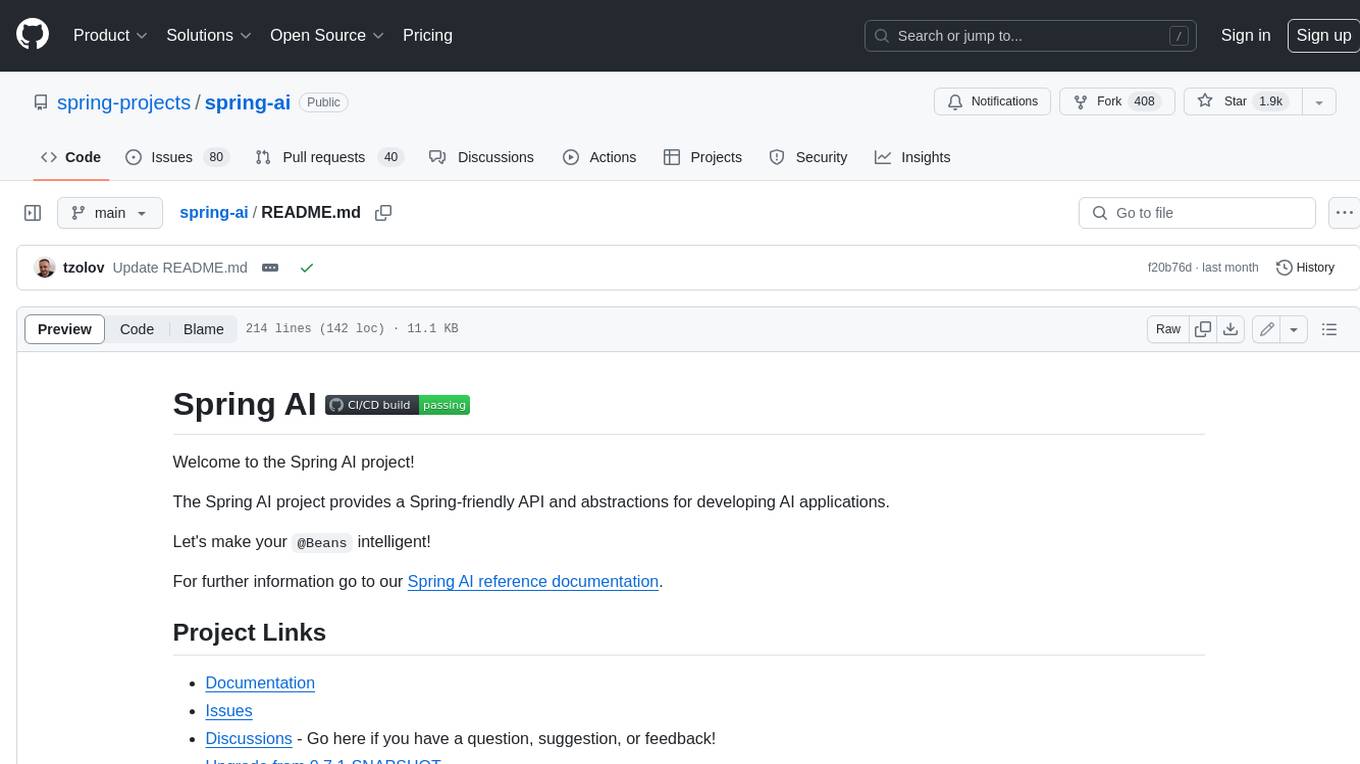
spring-ai
The Spring AI project provides a Spring-friendly API and abstractions for developing AI applications. It offers a portable client API for interacting with generative AI models, enabling developers to easily swap out implementations and access various models like OpenAI, Azure OpenAI, and HuggingFace. Spring AI also supports prompt engineering, providing classes and interfaces for creating and parsing prompts, as well as incorporating proprietary data into generative AI without retraining the model. This is achieved through Retrieval Augmented Generation (RAG), which involves extracting, transforming, and loading data into a vector database for use by AI models. Spring AI's VectorStore abstraction allows for seamless transitions between different vector database implementations.
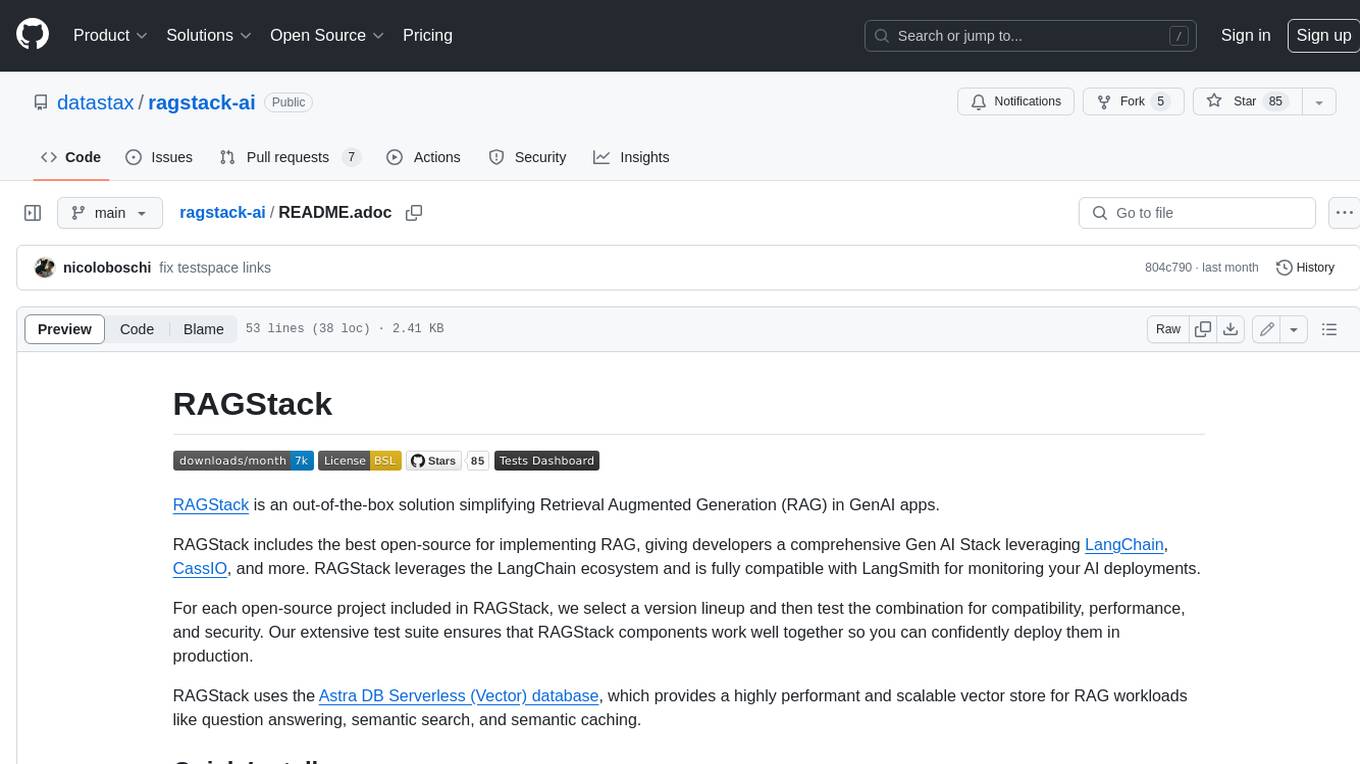
ragstack-ai
RAGStack is an out-of-the-box solution simplifying Retrieval Augmented Generation (RAG) in GenAI apps. RAGStack includes the best open-source for implementing RAG, giving developers a comprehensive Gen AI Stack leveraging LangChain, CassIO, and more. RAGStack leverages the LangChain ecosystem and is fully compatible with LangSmith for monitoring your AI deployments.
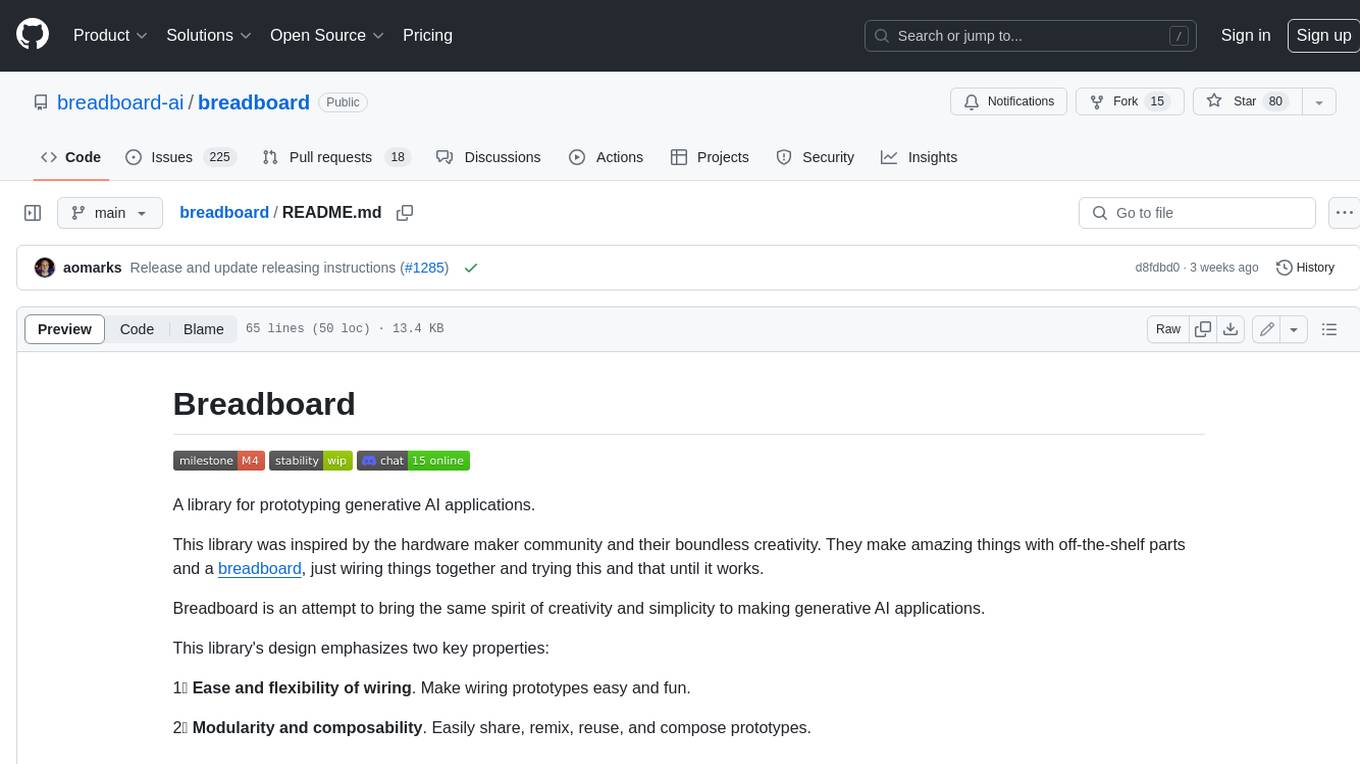
breadboard
Breadboard is a library for prototyping generative AI applications. It is inspired by the hardware maker community and their boundless creativity. Breadboard makes it easy to wire prototypes and share, remix, reuse, and compose them. The library emphasizes ease and flexibility of wiring, as well as modularity and composability.
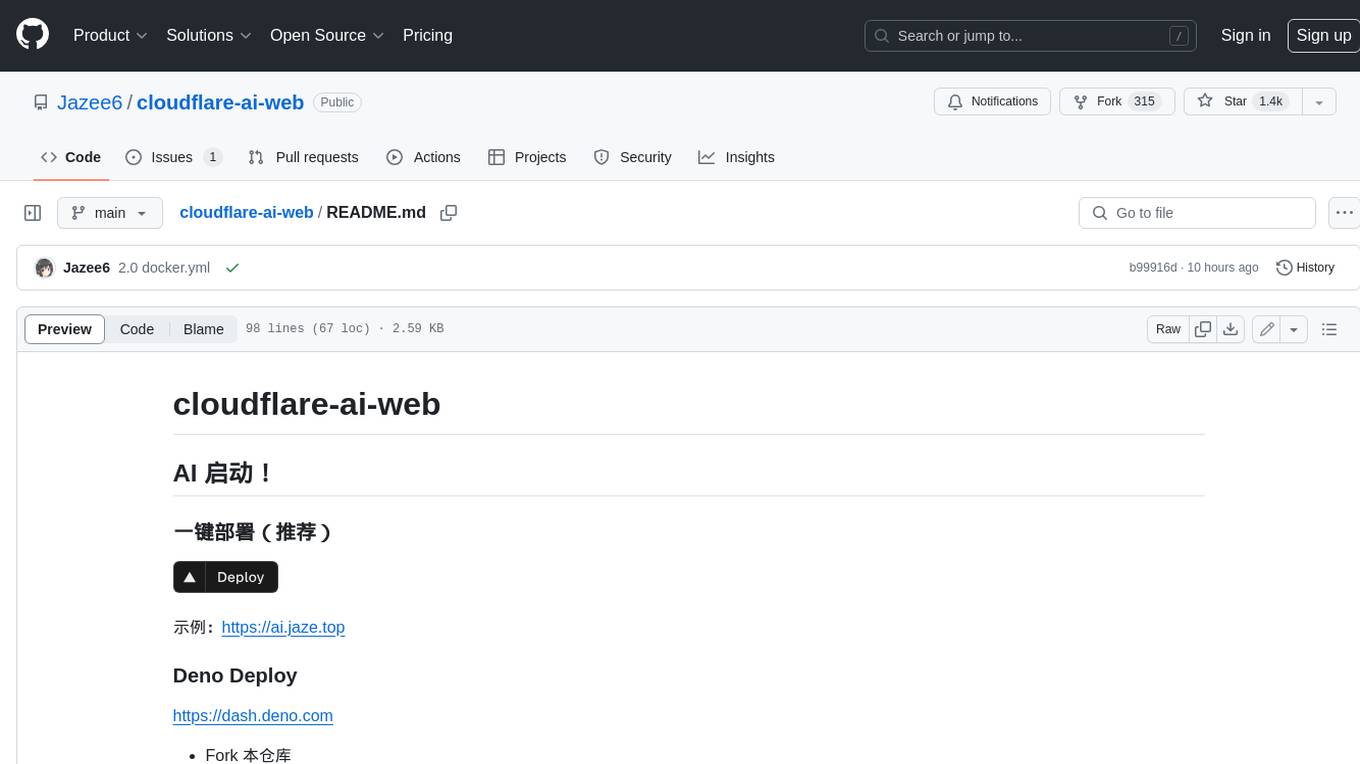
cloudflare-ai-web
Cloudflare-ai-web is a lightweight and easy-to-use tool that allows you to quickly deploy a multi-modal AI platform using Cloudflare Workers AI. It supports serverless deployment, password protection, and local storage of chat logs. With a size of only ~638 kB gzip, it is a great option for building AI-powered applications without the need for a dedicated server.
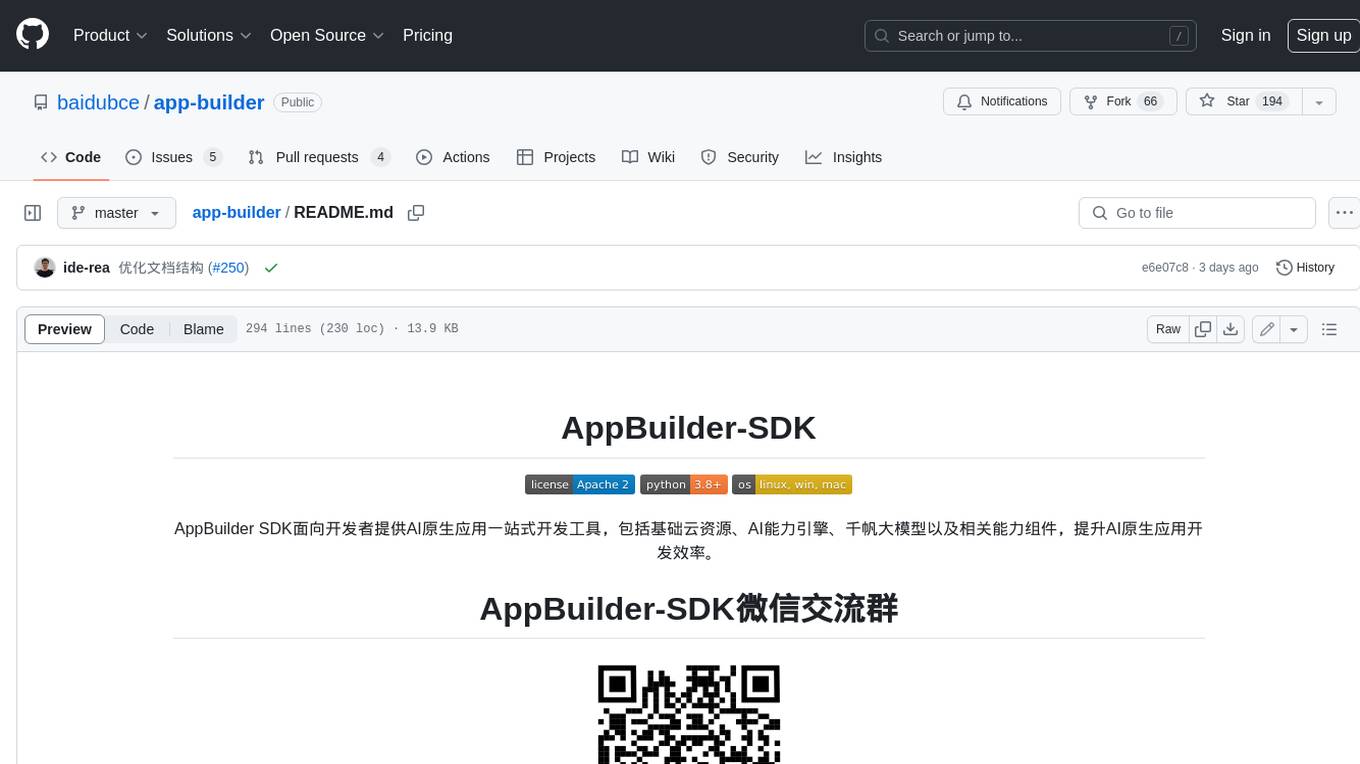
app-builder
AppBuilder SDK is a one-stop development tool for AI native applications, providing basic cloud resources, AI capability engine, Qianfan large model, and related capability components to improve the development efficiency of AI native applications.
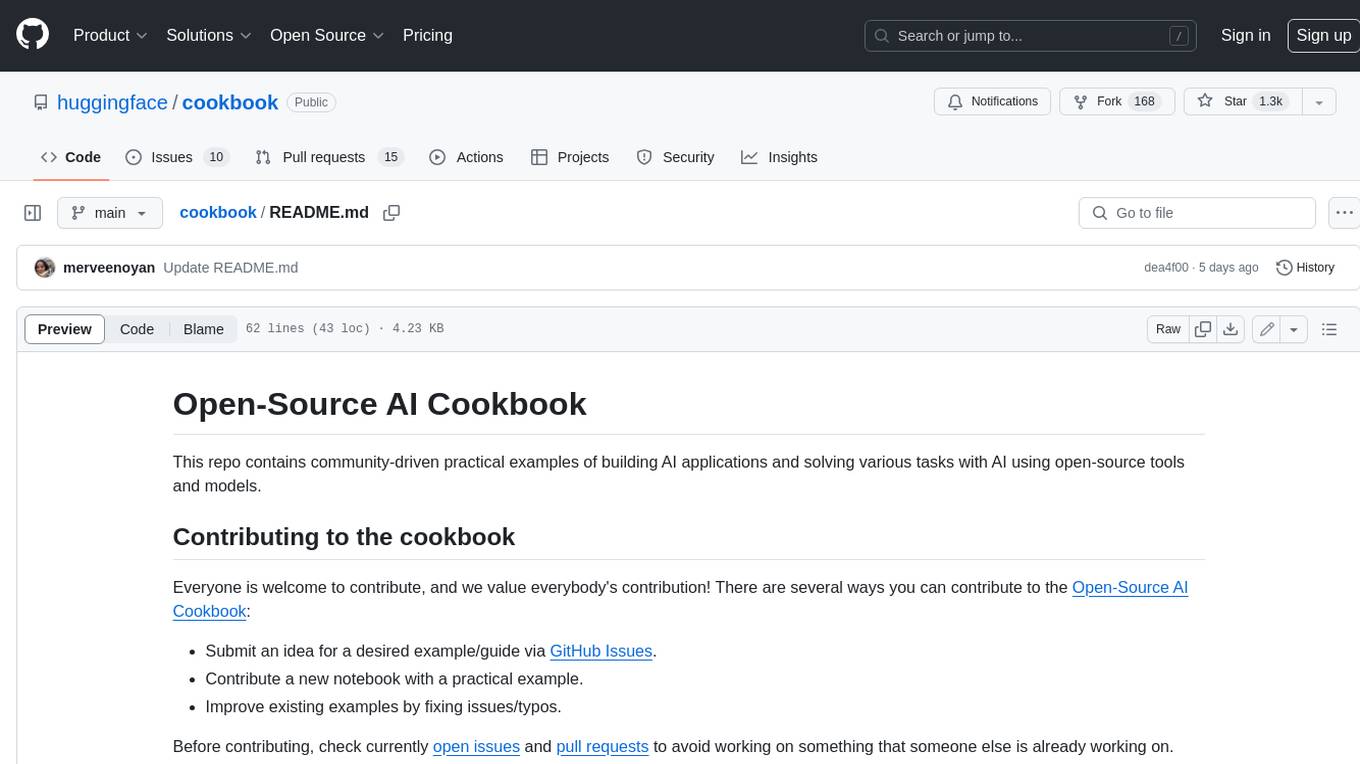
cookbook
This repository contains community-driven practical examples of building AI applications and solving various tasks with AI using open-source tools and models. Everyone is welcome to contribute, and we value everybody's contribution! There are several ways you can contribute to the Open-Source AI Cookbook: Submit an idea for a desired example/guide via GitHub Issues. Contribute a new notebook with a practical example. Improve existing examples by fixing issues/typos. Before contributing, check currently open issues and pull requests to avoid working on something that someone else is already working on.
For similar jobs
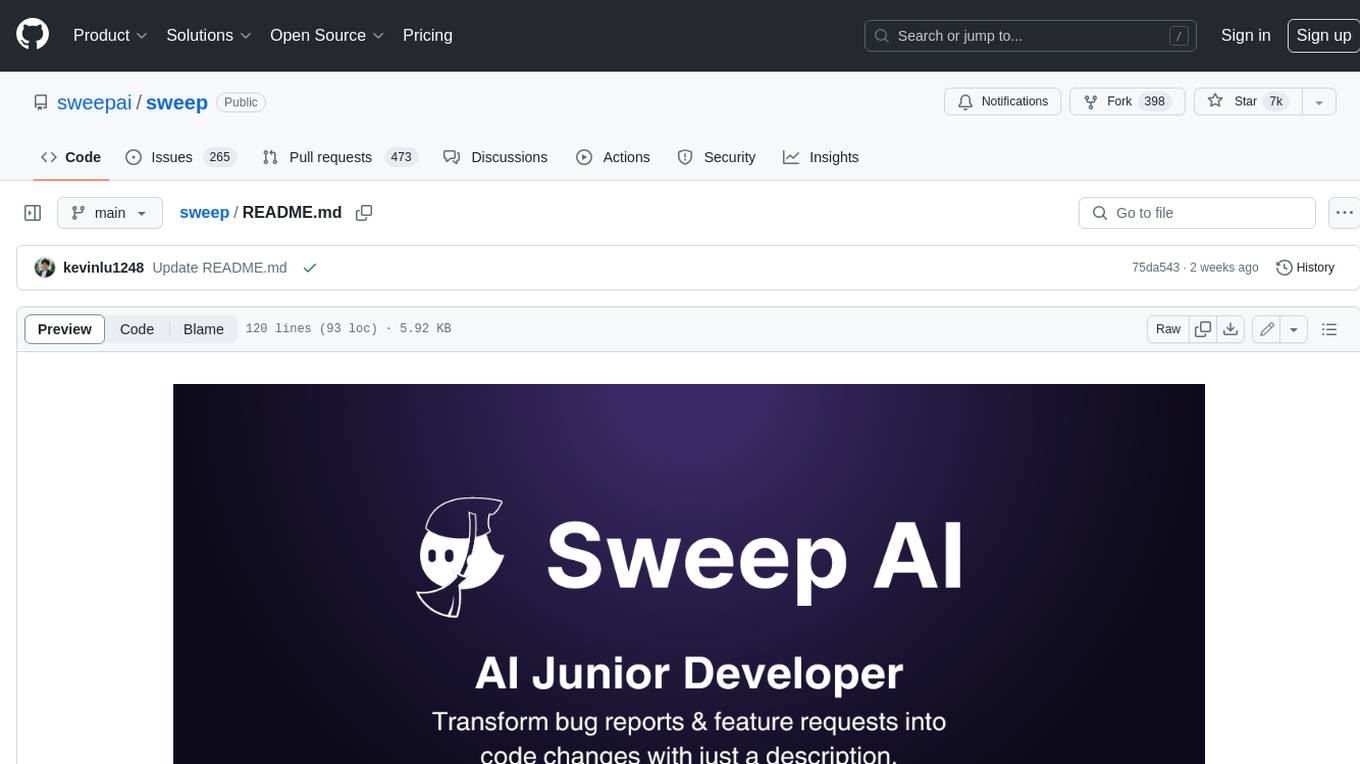
sweep
Sweep is an AI junior developer that turns bugs and feature requests into code changes. It automatically handles developer experience improvements like adding type hints and improving test coverage.
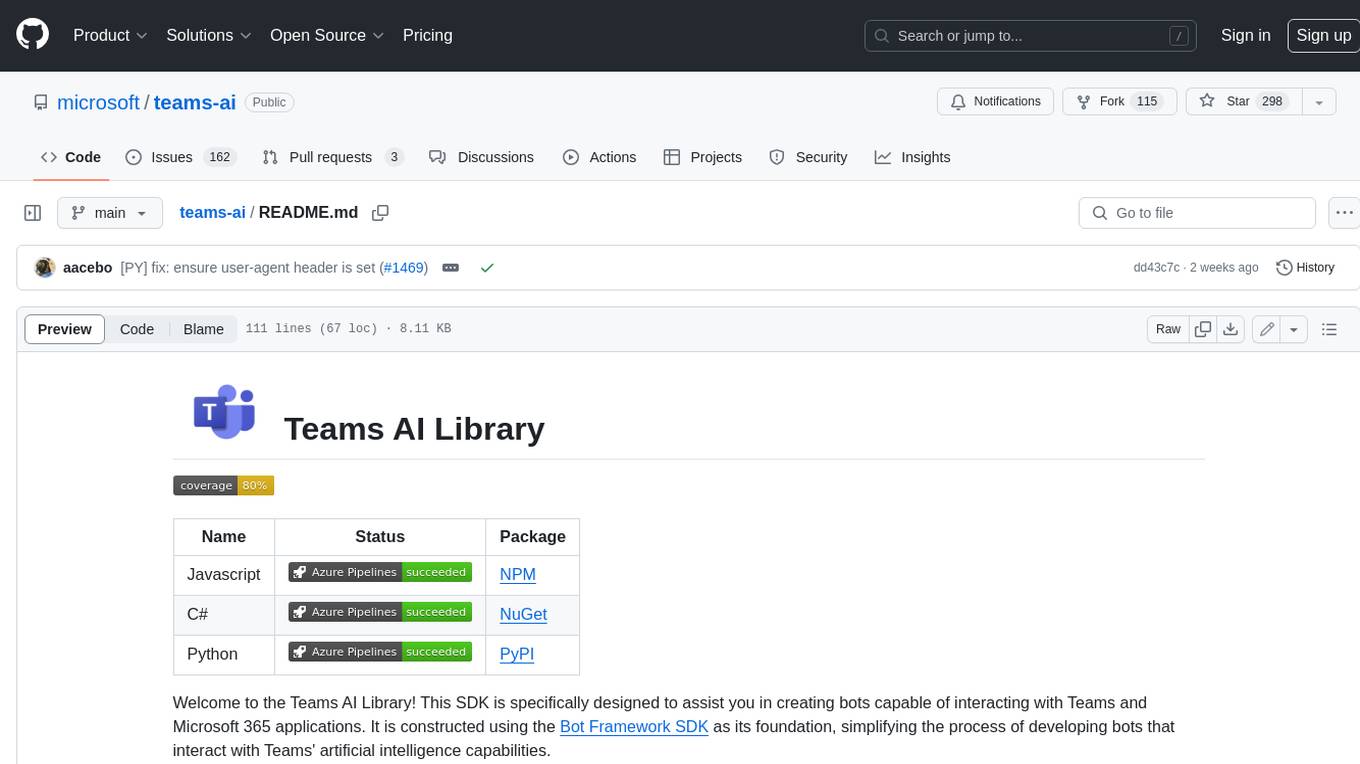
teams-ai
The Teams AI Library is a software development kit (SDK) that helps developers create bots that can interact with Teams and Microsoft 365 applications. It is built on top of the Bot Framework SDK and simplifies the process of developing bots that interact with Teams' artificial intelligence capabilities. The SDK is available for JavaScript/TypeScript, .NET, and Python.
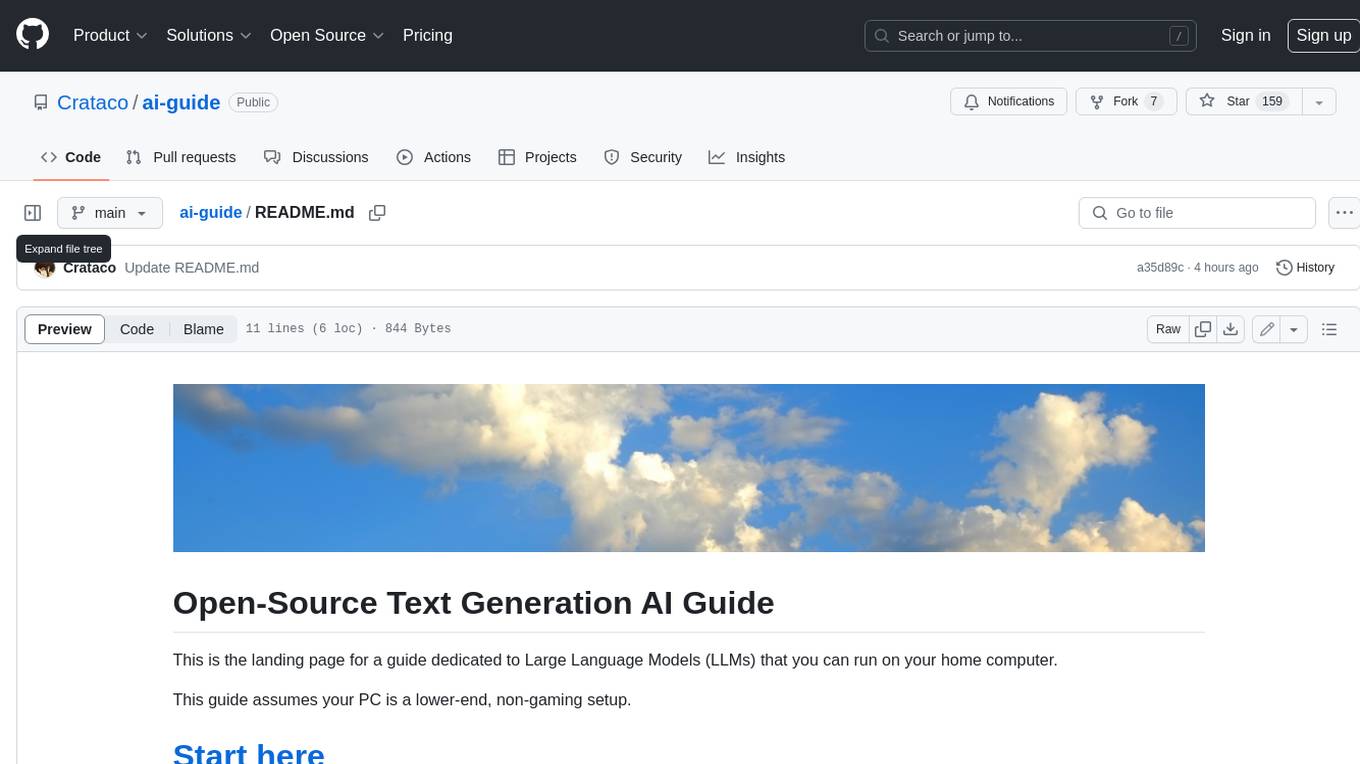
ai-guide
This guide is dedicated to Large Language Models (LLMs) that you can run on your home computer. It assumes your PC is a lower-end, non-gaming setup.
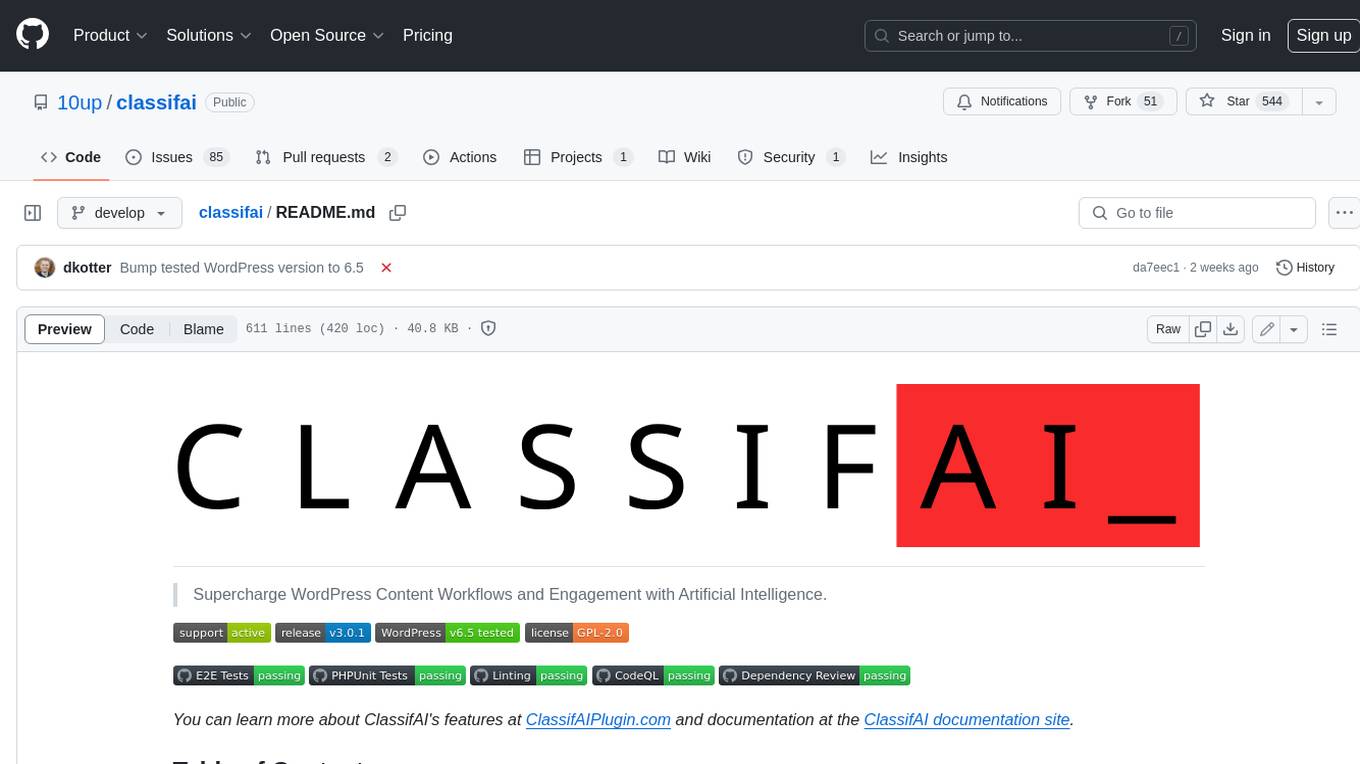
classifai
Supercharge WordPress Content Workflows and Engagement with Artificial Intelligence. Tap into leading cloud-based services like OpenAI, Microsoft Azure AI, Google Gemini and IBM Watson to augment your WordPress-powered websites. Publish content faster while improving SEO performance and increasing audience engagement. ClassifAI integrates Artificial Intelligence and Machine Learning technologies to lighten your workload and eliminate tedious tasks, giving you more time to create original content that matters.
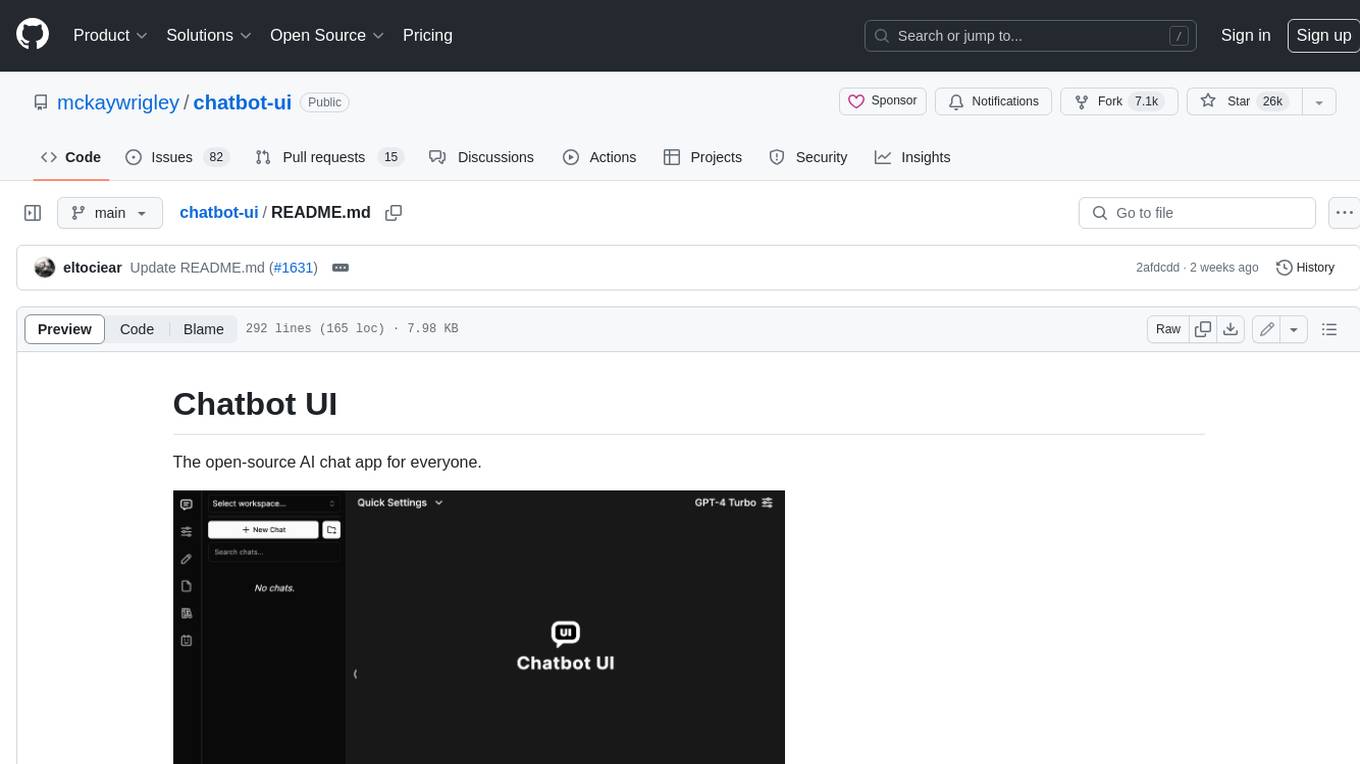
chatbot-ui
Chatbot UI is an open-source AI chat app that allows users to create and deploy their own AI chatbots. It is easy to use and can be customized to fit any need. Chatbot UI is perfect for businesses, developers, and anyone who wants to create a chatbot.
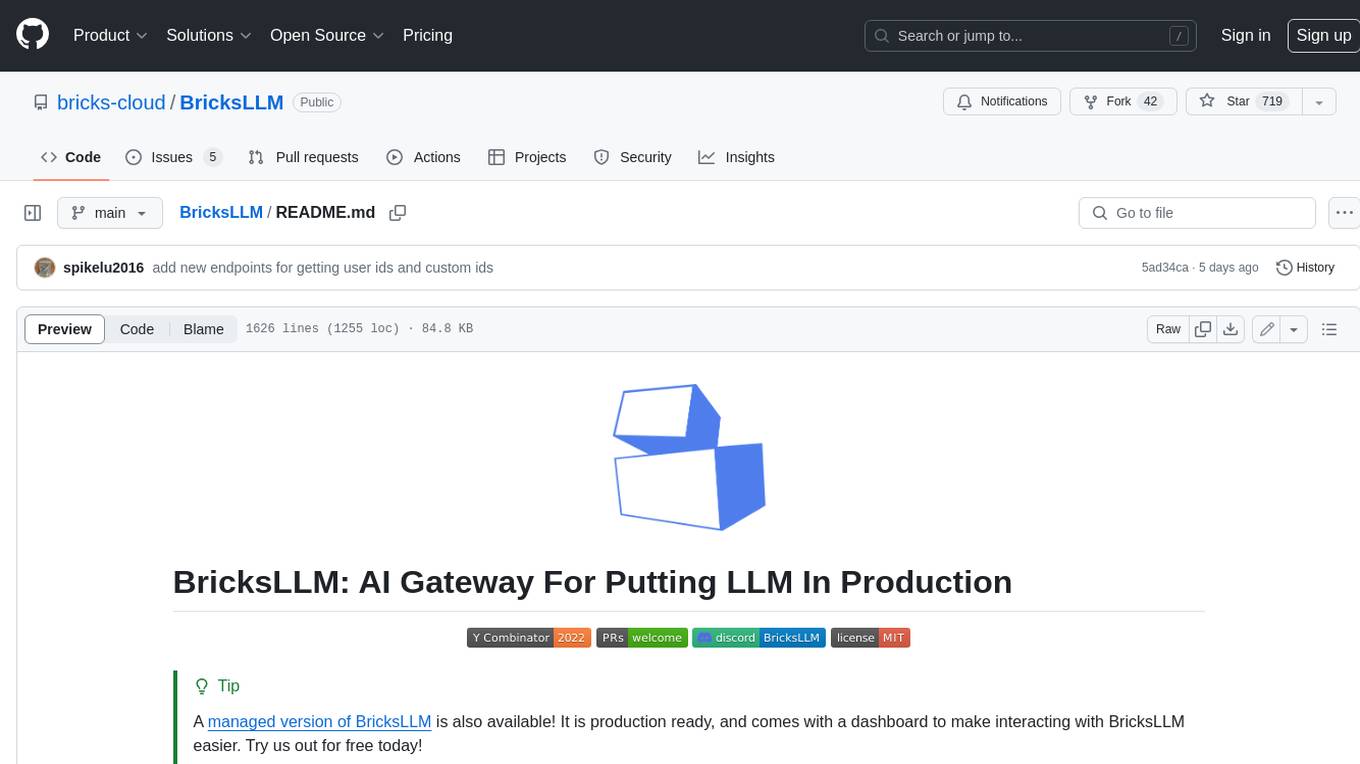
BricksLLM
BricksLLM is a cloud native AI gateway written in Go. Currently, it provides native support for OpenAI, Anthropic, Azure OpenAI and vLLM. BricksLLM aims to provide enterprise level infrastructure that can power any LLM production use cases. Here are some use cases for BricksLLM: * Set LLM usage limits for users on different pricing tiers * Track LLM usage on a per user and per organization basis * Block or redact requests containing PIIs * Improve LLM reliability with failovers, retries and caching * Distribute API keys with rate limits and cost limits for internal development/production use cases * Distribute API keys with rate limits and cost limits for students
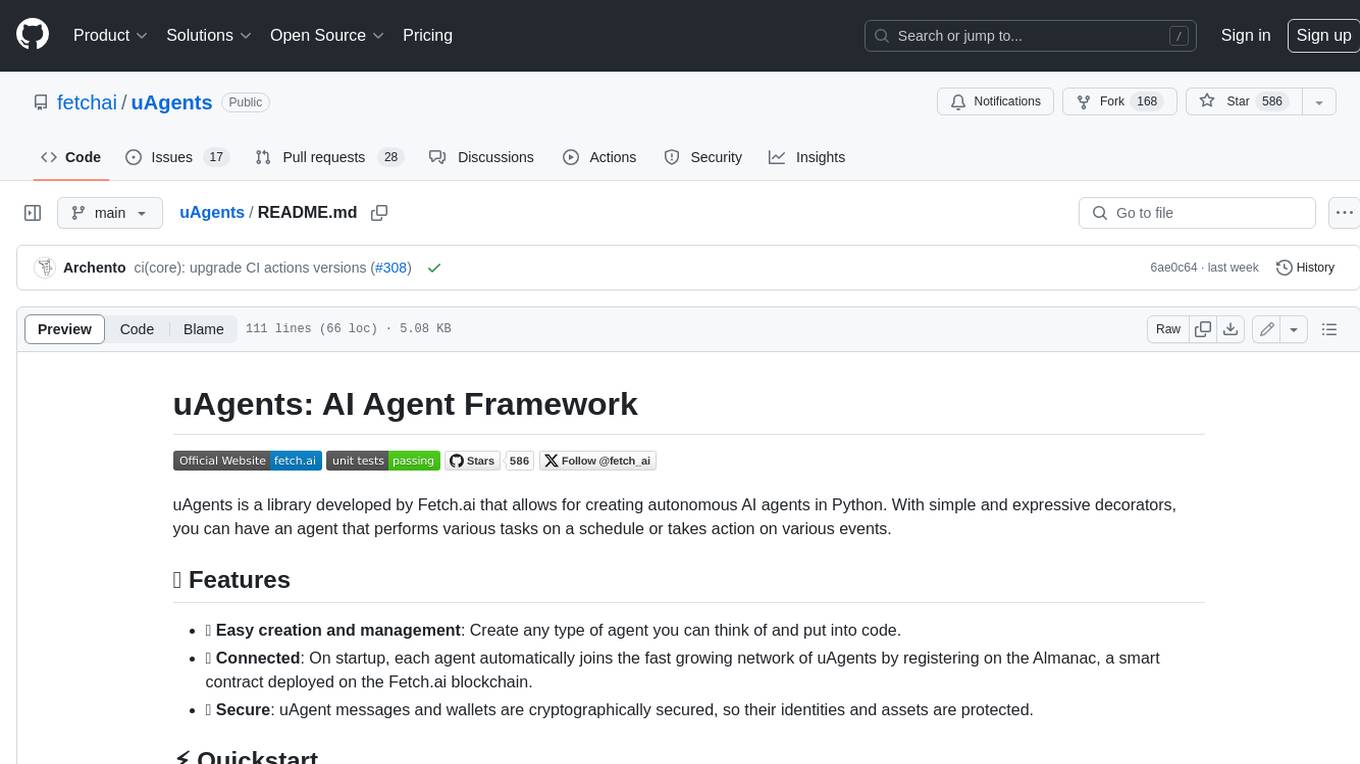
uAgents
uAgents is a Python library developed by Fetch.ai that allows for the creation of autonomous AI agents. These agents can perform various tasks on a schedule or take action on various events. uAgents are easy to create and manage, and they are connected to a fast-growing network of other uAgents. They are also secure, with cryptographically secured messages and wallets.
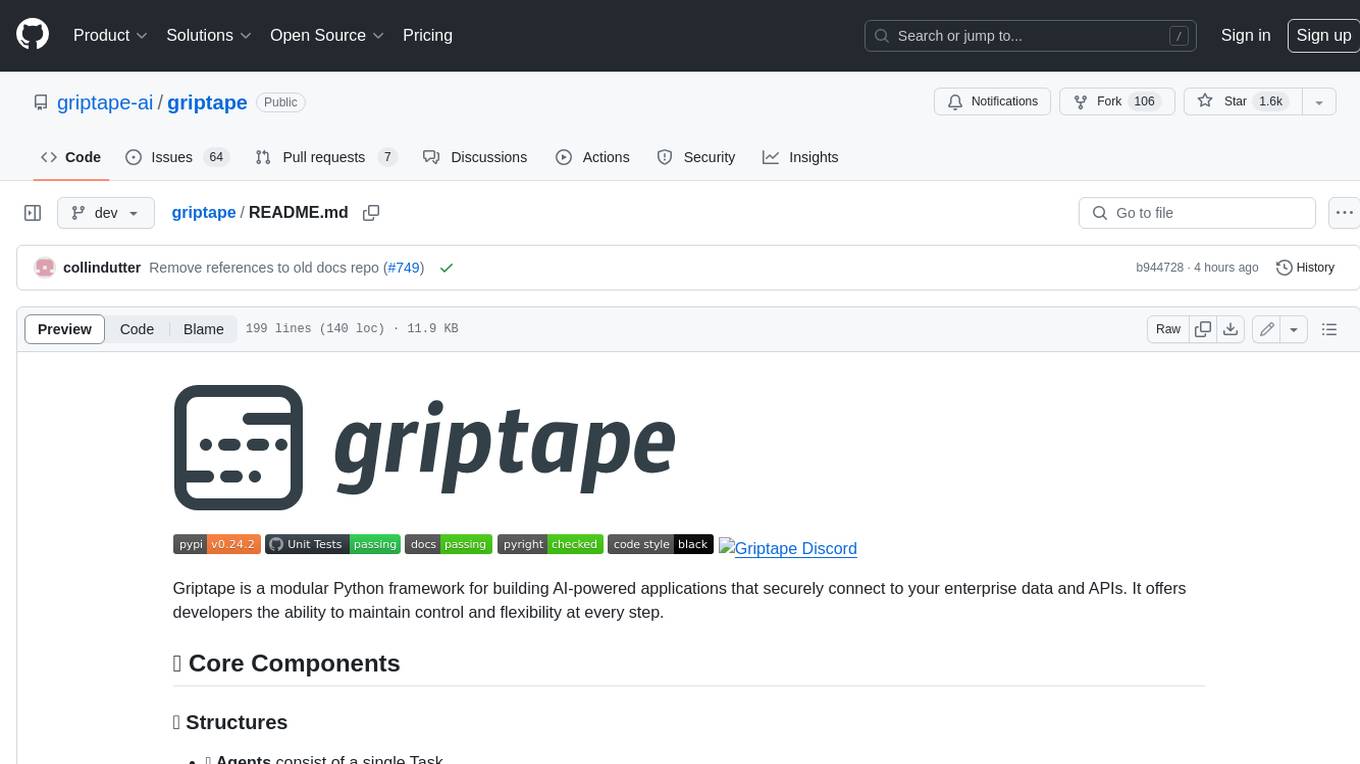
griptape
Griptape is a modular Python framework for building AI-powered applications that securely connect to your enterprise data and APIs. It offers developers the ability to maintain control and flexibility at every step. Griptape's core components include Structures (Agents, Pipelines, and Workflows), Tasks, Tools, Memory (Conversation Memory, Task Memory, and Meta Memory), Drivers (Prompt and Embedding Drivers, Vector Store Drivers, Image Generation Drivers, Image Query Drivers, SQL Drivers, Web Scraper Drivers, and Conversation Memory Drivers), Engines (Query Engines, Extraction Engines, Summary Engines, Image Generation Engines, and Image Query Engines), and additional components (Rulesets, Loaders, Artifacts, Chunkers, and Tokenizers). Griptape enables developers to create AI-powered applications with ease and efficiency.