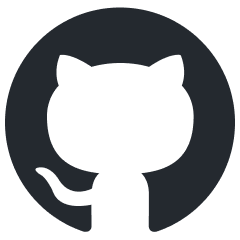
groq-ruby
Groq Cloud runs LLM models fast and cheap. This is a convenience client library for Ruby.
Stars: 82
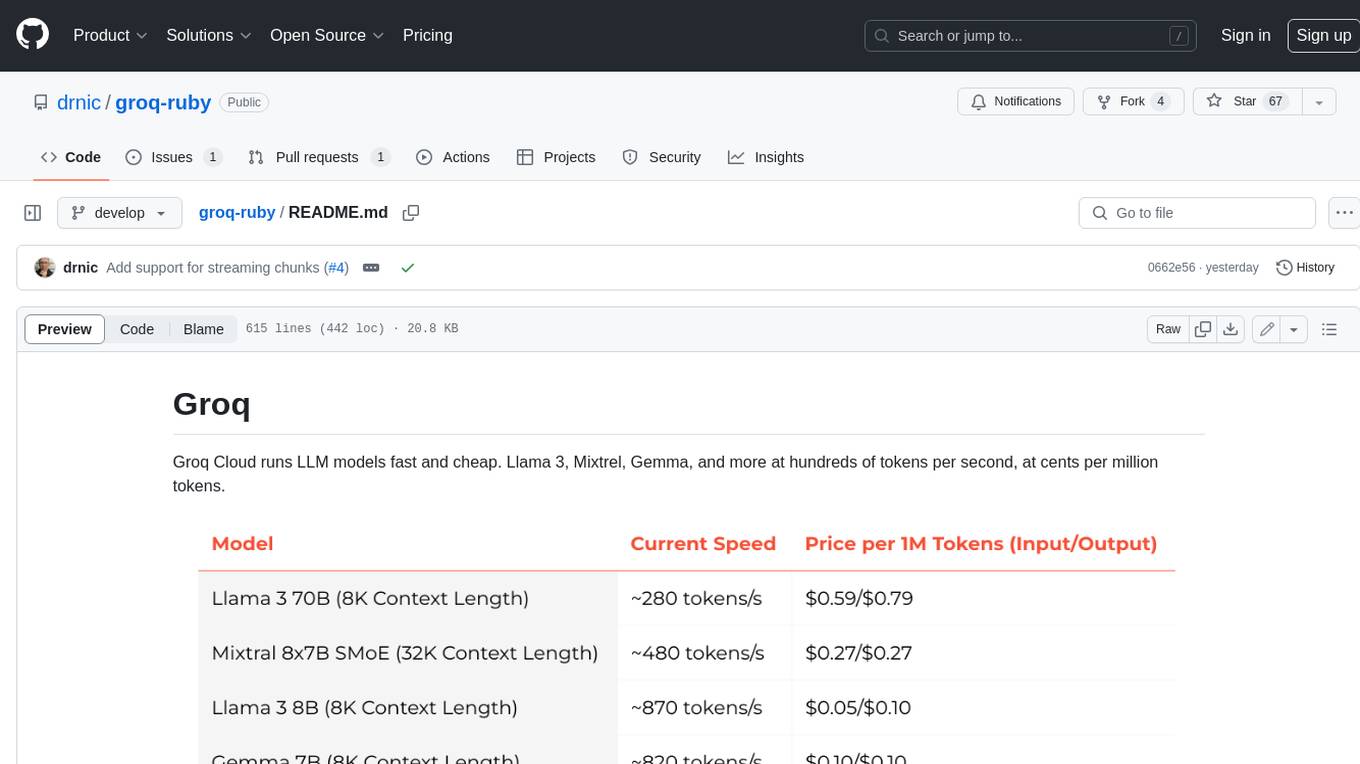
Groq Cloud runs LLM models fast and cheap. Llama 3, Mixtrel, Gemma, and more at hundreds of tokens per second, at cents per million tokens.
README:
Groq Cloud runs LLM models fast and cheap. Llama 3, Mixtrel, Gemma, and more at hundreds of tokens per second, at cents per million tokens.
Speed and pricing at 2024-04-21. Also see their changelog for new models and features.
You can interact with their API using any Ruby HTTP library by following their documentation at https://console.groq.com/docs/quickstart. Also use their Playground and watch the API traffic in the browser's developer tools.
The Groq Cloud API looks to be copying a subset of the OpenAI API. For example, you perform chat completions at https://api.groq.com/openai/v1/chat/completions
with the same POST body schema as OpenAI. The Tools support looks to have the same schema for defining tools/functions.
So you can write your own Ruby client code to interact with the Groq Cloud API.
Or you can use this convenience RubyGem with some nice helpers to get you started.
@client = Groq::Client.new
@client.chat("Hello, world!")
=> {"role"=>"assistant", "content"=>"Hello there! It's great to meet you!"}
include Groq::Helpers
@client.chat([
User("Hi"),
Assistant("Hello back. Ask me anything. I'll reply with 'cat'"),
User("Favourite food?")
])
# => {"role"=>"assistant", "content"=>"Um... CAT"}
# => {"role"=>"assistant", "content"=>"Not a cat! It's a pizza!"}
# => {"role"=>"assistant", "content"=>"Pizza"}
# => {"role"=>"assistant", "content"=>"Cat"}
@client.chat([
System("I am an obedient AI"),
U("Hi"),
A("Hello back. Ask me anything. I'll reply with 'cat'"),
U("Favourite food?")
])
# => {"role"=>"assistant", "content"=>"Cat"}
# => {"role"=>"assistant", "content"=>"cat"}
# => {"role"=>"assistant", "content"=>"Cat"}
JSON mode:
response = @client.chat([
S("Reply with JSON. Use {\"number\": 7} for the answer."),
U("What's 3+4?")
], json: true)
# => {"role"=>"assistant", "content"=>"{\"number\": 7}"}
JSON.parse(response["content"])
# => {"number"=>7}
Install the gem and add to the application's Gemfile by executing:
bundle add groq
If bundler is not being used to manage dependencies, install the gem by executing:
gem install groq
- Get your API key from console.groq.com/keys
- Place in env var
GROQ_API_KEY
, or explicitly pass into configuration below. - Use the
Groq::Client
to interact with Groq and your favourite model.
client = Groq::Client.new # uses ENV["GROQ_API_KEY"] and "llama3-8b-8192"
client = Groq::Client.new(api_key: "...", model_id: "llama3-8b-8192")
Groq.configure do |config|
config.api_key = "..."
config.model_id = "llama3-70b-8192"
end
client = Groq::Client.new
In a Rails application, you can generate a config/initializer/groq.rb
file with:
rails g groq:install
There is a simple chat function to send messages to a model:
# either pass a single message and get a single response
client.chat("Hello, world!")
=> {"role"=>"assistant", "content"=>"Hello there! It's great to meet you!"}
# or pass in a messages array containing multiple messages between user and assistant
client.chat([
{role: "user", content: "What's the next day after Wednesday?"},
{role: "assistant", content: "The next day after Wednesday is Thursday."},
{role: "user", content: "What's the next day after that?"}
])
# => {"role" => "assistant", "content" => "The next day after Thursday is Friday."}
bin/console
This repository has a bin/console
script to start an interactive console to play with the Groq API. The @client
variable is setup using $GROQ_API_KEY
environment variable; and the U
, A
, T
helpers are already included.
@client.chat("Hello, world!")
{"role"=>"assistant",
"content"=>"Hello there! It's great to meet you! Is there something you'd like to talk about or ask? I'm here to listen and help if I can!"}
The remaining examples below will use @client
variable to allow you to copy+paste into bin/console
.
We also have some handy U
, A
, S
, and F
methods to produce the {role:, content:}
hashes:
include Groq::Helpers
@client.chat([
S("I am an obedient AI"),
U("Hi"),
A("Hello back. Ask me anything. I'll reply with 'cat'"),
U("Favourite food?")
])
# => {"role"=>"assistant", "content"=>"Cat"}
The T()
is to provide function/tool responses:
T("tool", tool_call_id: "call_b790", name: "get_weather_report", content: "25 degrees celcius")
# => {"role"=>"function", "tool_call_id"=>"call_b790", "name"=>"get_weather_report", "content"=>"25 degrees celcius"}
There are also aliases for each helper function:
-
U(content)
is alsoUser(content)
-
A(content)
is alsoAssistant(content)
-
S(content)
is alsoSystem(content)
-
T(content, ...)
is alsoTool
,ToolReply
,Function
,F
At the time of writing, Groq Cloud service supports a limited number of models. They've suggested they'll allow uploading custom models in future.
To get the list of known model IDs:
Groq::Model.model_ids
=> ["llama3-8b-8192", "llama3-70b-8192", "llama2-70b-4096", "mixtral-8x7b-32768", "gemma-7b-it"]
To get more data about each model, see Groq::Model::MODELS
.
As above, you can specify the default model to use for all chat()
calls:
client = Groq::Client.new(model_id: "llama3-70b-8192")
# or
Groq.configure do |config|
config.model_id = "llama3-70b-8192"
end
You can also specify the model within the chat()
call:
@client.chat("Hello, world!", model_id: "llama3-70b-8192")
To see all known models reply:
puts "User message: Hello, world!"
Groq::Model.model_ids.each do |model_id|
puts "Assistant reply with model #{model_id}:"
p @client.chat("Hello, world!", model_id: model_id)
end
The output might looks similar to:
> User message: Hello, world!
Assistant reply with model llama3-8b-8192:
Assistant reply with model llama3-70b-8192:
{"role"=>"assistant", "content"=>"The classic \"Hello, world!\" It's great to see you here! Is there something I can help you with, or would you like to just chat?"}
Assistant reply with model llama2-70b-4096:
{"role"=>"assistant", "content"=>"Hello, world!"}
Assistant reply with model mixtral-8x7b-32768:
{"role"=>"assistant", "content"=>"Hello! It's nice to meet you. Is there something specific you would like to know or talk about? I'm here to help answer any questions you have to the best of my ability. I can provide information on a wide variety of topics, so feel free to ask me anything. I'm here to assist you."}
Assistant reply with model gemma-7b-it:
{"role"=>"assistant", "content"=>"Hello to you too! 👋🌎 It's great to hear from you. What would you like to talk about today? 😊"}
JSON mode is a beta feature that guarantees all chat completions are valid JSON.
To use JSON mode:
- Pass
json: true
to thechat()
call - Provide a system message that contains
JSON
in the content, e.g.S("Reply with JSON")
A good idea is to provide an example JSON schema in the system message that you'd prefer to receive.
Other suggestions at JSON mode (beta) Groq docs page.
response = @client.chat([
S("Reply with JSON. Use {\n\"number\": 7\n} for the answer."),
U("What's 3+4?")
], json: true)
# => {"role"=>"assistant", "content"=>"{\n\"number\": 7\n}"}
JSON.parse(response["content"])
# => {"number"=>7}
As a bonus, the S
or System
helper can take a json_schema:
argument and the system message will include the JSON
keyword and the formatted schema in its content.
For example, if you're using dry-schema with its :json_schema
extension you can use Ruby to describe JSON schema.
require "dry-schema"
Dry::Schema.load_extensions(:json_schema)
person_schema_defn = Dry::Schema.JSON do
required(:name).filled(:string)
optional(:age).filled(:integer)
optional(:email).filled(:string)
end
person_schema = person_schema_defn.json_schema
response = @client.chat([
S("You're excellent at extracting personal information", json_schema: person_schema),
U("I'm Dr Nic and I'm almost 50.")
], json: true)
JSON.parse(response["content"])
# => {"name"=>"Dr Nic", "age"=>49}
NOTE: bin/console
already loads the dry-schema
library and the json_schema
extension because its handy.
LLMs are increasingly supporting deferring to tools or functions to fetch data, perform calculations, or store structured data. Groq Cloud in turn then supports their tool implementations through its API.
See the Using Tools documentation for the list of models that currently support tools. Others might support it sometimes and raise errors other times.
@client = Groq::Client.new(model_id: "mixtral-8x7b-32768")
The Groq/OpenAI schema for defining a tool/function (which differs from the Anthropic/Claude3 schema) is:
tools = [{
type: "function",
function: {
name: "get_weather_report",
description: "Get the weather report for a city",
parameters: {
type: "object",
properties: {
city: {
type: "string",
description: "The city or region to get the weather report for"
}
},
required: ["city"]
}
}
}]
Pass the tools
array into the chat()
call:
@client = Groq::Client.new(model_id: "mixtral-8x7b-32768")
include Groq::Helpers
messages = [U("What's the weather in Paris?")]
response = @client.chat(messages, tools: tools)
# => {"role"=>"assistant", "tool_calls"=>[{"id"=>"call_b790", "type"=>"function", "function"=>{"name"=>"get_weather_report", "arguments"=>"{\"city\":\"Paris\"}"}}]}
You'd then invoke the Ruby implementation of get_weather_report
to return the weather report for Paris as the next message in the chat.
messages << response
tool_call_id = response["tool_calls"].first["id"]
messages << T("25 degrees celcius", tool_call_id: tool_call_id, name: "get_weather_report")
@client.chat(messages)
# => {"role"=>"assistant", "content"=> "I'm glad you called the function!\n\nAs of your current location, the weather in Paris is indeed 25°C (77°F)..."}
Max tokens is the maximum number of tokens that the model can process in a single response. This limits ensures computational efficiency and resource management.
Temperature setting for each API call controls randomness of responses. A lower temperature leads to more predictable outputs while a higher temperature results in more varies and sometimes more creative outputs. The range of values is 0 to 2.
Each API call includes a max_token:
and temperature:
value.
The defaults are:
@client.max_tokens
=> 1024
@client.temperature
=> 1
You can override them in the Groq.configure
block, or with each chat()
call:
Groq.configure do |config|
config.max_tokens = 512
config.temperature = 0.5
end
# or
@client.chat("Hello, world!", max_tokens: 512, temperature: 0.5)
The underlying HTTP library being used is faraday, and you can enabled debugging, or configure other faraday internals by passing a block to the Groq::Client.new
constructor.
require 'logger'
# Create a logger instance
logger = Logger.new(STDOUT)
logger.level = Logger::DEBUG
@client = Groq::Client.new do |faraday|
# Log request and response bodies
faraday.response :logger, logger, bodies: true
end
If you pass --debug
to bin/console
you will have this logger setup for you.
bin/console --debug
If your AI assistant responses are being telecast live to a human, then that human might want some progressive responses. The Groq API supports streaming responses.
Pass a block to chat()
with either one or two arguments.
- The first argument is the string content chunk of the response.
- The optional second argument is the full response object from the API containing extra metadata.
The final block call will be the last chunk of the response:
- The first argument will be
nil
- The optional second argument, the full response object, contains a summary of the Groq API usage, such as prompt tokens, prompt time, etc.
puts "🍕 "
messages = [
S("You are a pizza sales person."),
U("What do you sell?")
]
@client.chat(messages) do |content|
print content
end
puts
Each chunk of the response will be printed to the console as it is received. It will look pretty.
The default llama3-7b-8192
model is very very fast and you might not see any streaming. Try a slower model like llama3-70b-8192
or mixtral-8x7b-32768
.
@client = Groq::Client.new(model_id: "llama3-70b-8192")
@client.chat("Write a long poem about patience") do |content|
print content
end
puts
You can pass in a second argument to get the full response JSON object:
@client.chat("Write a long poem about patience") do |content, response|
pp content
pp response
end
Alternately, you can pass a Proc
or any object that responds to call
via a stream:
keyword argument:
@client.chat("Write a long poem about patience", stream: ->(content) { print content })
You could use a class with a call
method with either one or two arguments, like the Proc
discussion above.
class MessageBits
def initialize(emoji)
print "#{emoji} "
@bits = []
end
def call(content)
if content.nil?
puts
else
print(content)
@bits << content
end
end
def to_s
@bits.join("")
end
def to_assistant_message
Assistant(to_s)
end
end
bits = MessageBits.new("🍕")
@client.chat("Write a long poem about pizza", stream: bits)
Here are some example uses of Groq, of the groq
gem and its syntax.
Also, see the examples/
folder for more example apps.
Talking with a pizzeria.
Our pizzeria agent can be as simple as a function that combines a system message and the current messages array:
@agent_message = <<~EOS
You are an employee at a pizza store.
You sell hawaiian, and pepperoni pizzas; in small and large sizes for $10, and $20 respectively.
Pick up only in. Ready in 10 mins. Cash on pickup.
EOS
def chat_pizza_agent(messages)
@client.chat([
System(@agent_message),
*messages
])
end
Now for our first interaction:
messages = [U("Is this the pizza shop? Do you sell hawaiian?")]
response = chat_pizza_agent(messages)
puts response["content"]
The output might be:
Yeah! This is the place! Yes, we sell Hawaiian pizzas here! We've got both small and large sizes available for you. The small Hawaiian pizza is $10, and the large one is $20. Plus, because we're all about getting you your pizza fast, our pick-up time is only 10 minutes! So, what can I get for you today? Would you like to order a small or large Hawaiian pizza?
Continue with user's reply.
Note, we build the messages
array with the previous user and assistant messages and the new user message:
messages << response << U("Yep, give me a large.")
response = chat_pizza_agent(messages)
puts response["content"]
Response:
I'll get that ready for you. So, to confirm, you'd like to order a large Hawaiian pizza for $20, and I'll have it ready for you in 10 minutes. When you come to pick it up, please have the cash ready as we're a cash-only transaction. See you in 10!
Making a change:
messages << response << U("Actually, make it two smalls.")
response = chat_pizza_agent(messages)
puts response["content"]
Response:
I've got it! Two small Hawaiian pizzas on the way! That'll be $20 for two small pizzas. Same deal, come back in 10 minutes to pick them up, and bring cash for the payment. See you soon!
Oh my. Let's also have an agent that represents the customer.
@customer_message = <<~EOS
You are a customer at a pizza store.
You want to order a pizza. You can ask about the menu, prices, sizes, and pickup times.
You'll agree with the price and terms of the pizza order.
You'll make a choice of the available options.
If you're first in the conversation, you'll say hello and ask about the menu.
EOS
def chat_pizza_customer(messages)
@client.chat([
System(@customer_message),
*messages
])
end
First interaction starts with no user or assistant messages. We're generating the customer's first message:
customer_messages = []
response = chat_pizza_customer(customer_messages)
puts response["content"]
Customer's first message:
Hello! I'd like to order a pizza. Could you tell me more about the menu and prices? What kind of pizzas do you have available?
Now we need to pass this to the pizzeria agent:
customer_message = response["content"]
pizzeria_messages = [U(customer_message)]
response = chat_pizza_agent(pizzeria_messages)
puts response["content"]
Pizzeria agent response:
Hi there! Yeah, sure thing! We've got two delicious options to choose from: Hawaiian and Pepperoni. Both come in small and large sizes. The small pizzas are $10 and the large pizzas are $20.
Our Hawaiian pizza features fresh ham and pineapple on a bed of melted mozzarella. And if you're in the mood for something classic, our Pepperoni pizza is loaded with plenty of sliced pepperoni and melted mozzarella cheese.
Now let's add this response to the customer agent's message array, and generate the customer's next response to the pizzera:
customer_messages << U(response["content"])
response = chat_pizza_customer(customer_messages)
puts response["content"]
Customer agent response:
Wow, those both sound delicious! I'm intrigued by the Hawaiian combo, I never thought of putting ham and pineapple on a pizza before. How would you recommend I customize it? Can I add any extra toppings or keep it as is? And do you have any recommendations for the size? Small or large?
Add this to the pizzeria agent's message array, and generate the pizzeria's response:
pizzeria_messages << U(response["content"])
response = chat_pizza_agent(pizzeria_messages)
puts response["content"]
Pizzeria agent response:
The Hawaiian pizza is definitely a unique twist on traditional toppings! You can definitely customize it to your liking. We allow two extra toppings of your choice for an additional $1 each. If you want to add any other toppings beyond that, it's $2 per topping.
As for recommends, I'd say the small size is a great starting point, especially if you're trying something new like the Hawaiian pizza. The small size is $10 and it's a great bite-sized portion. But if you're looking for a bigger pie, the large size is $20 and would be a great option if you're feeding a crowd or want leftovers.
Keep in mind that our pizzas are cooked fresh in 10 minutes, so it's ready when it's ready! Would you like to place an order now?
Will the customer actually buy anything now?
I think I'd like to go with the Hawaiian pizza in the small size, so the total would be $10. And I'll take advantage of the extra topping option. I think I'll add some mushrooms to it. So, that's an extra $1 for the mushroom topping. Would that be $11 total? And do you have a pickup time available soon?
OMG, the customer bought something.
Pizzeria agent response:
That sounds like a great choice! Yeah, the total would be $11, the small Hawaiian pizza with mushrooms. And yes, we do have pickup available shortly. It'll be ready in about 10 minutes. Cash on pickup, okay? Would you like to pay when you pick up your pizza?
Maybe these two do not know how to stop talking. The Halting Problem exists in pizza shops too.
After checking out the repo, run bin/setup
to install dependencies. Then, run rake test
to run the tests. You can also run bin/console
for an interactive prompt that will allow you to experiment.
To install this gem onto your local machine, run bundle exec rake install
. To release a new version, update the version number in version.rb
, and then run bundle exec rake release
, which will create a git tag for the version, push git commits and the created tag, and push the .gem
file to rubygems.org.
Bug reports and pull requests are welcome on GitHub at https://github.com/drnic/groq-ruby. This project is intended to be a safe, welcoming space for collaboration, and contributors are expected to adhere to the code of conduct.
The gem is available as open source under the terms of the MIT License.
Everyone interacting in the Groq project's codebases, issue trackers, chat rooms and mailing lists is expected to follow the code of conduct.
For Tasks:
Click tags to check more tools for each tasksFor Jobs:
Alternative AI tools for groq-ruby
Similar Open Source Tools
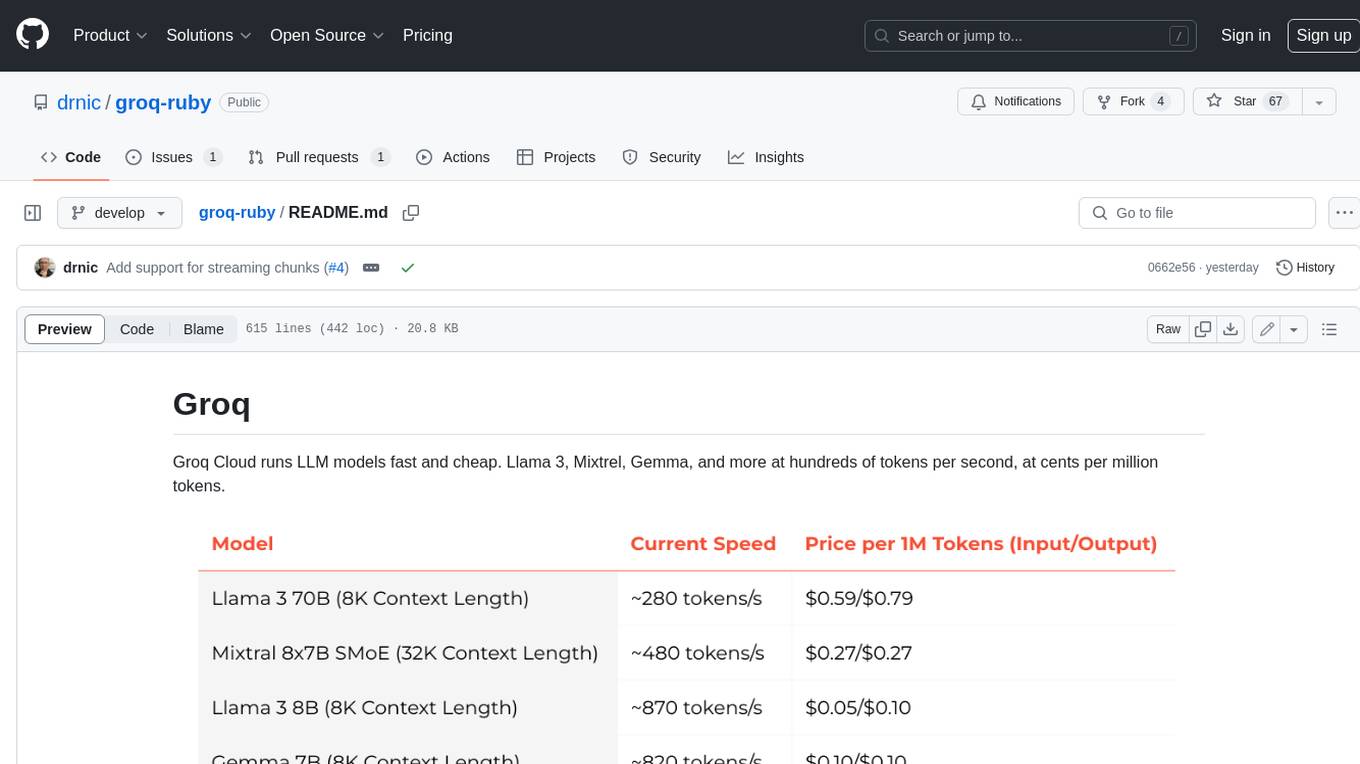
groq-ruby
Groq Cloud runs LLM models fast and cheap. Llama 3, Mixtrel, Gemma, and more at hundreds of tokens per second, at cents per million tokens.
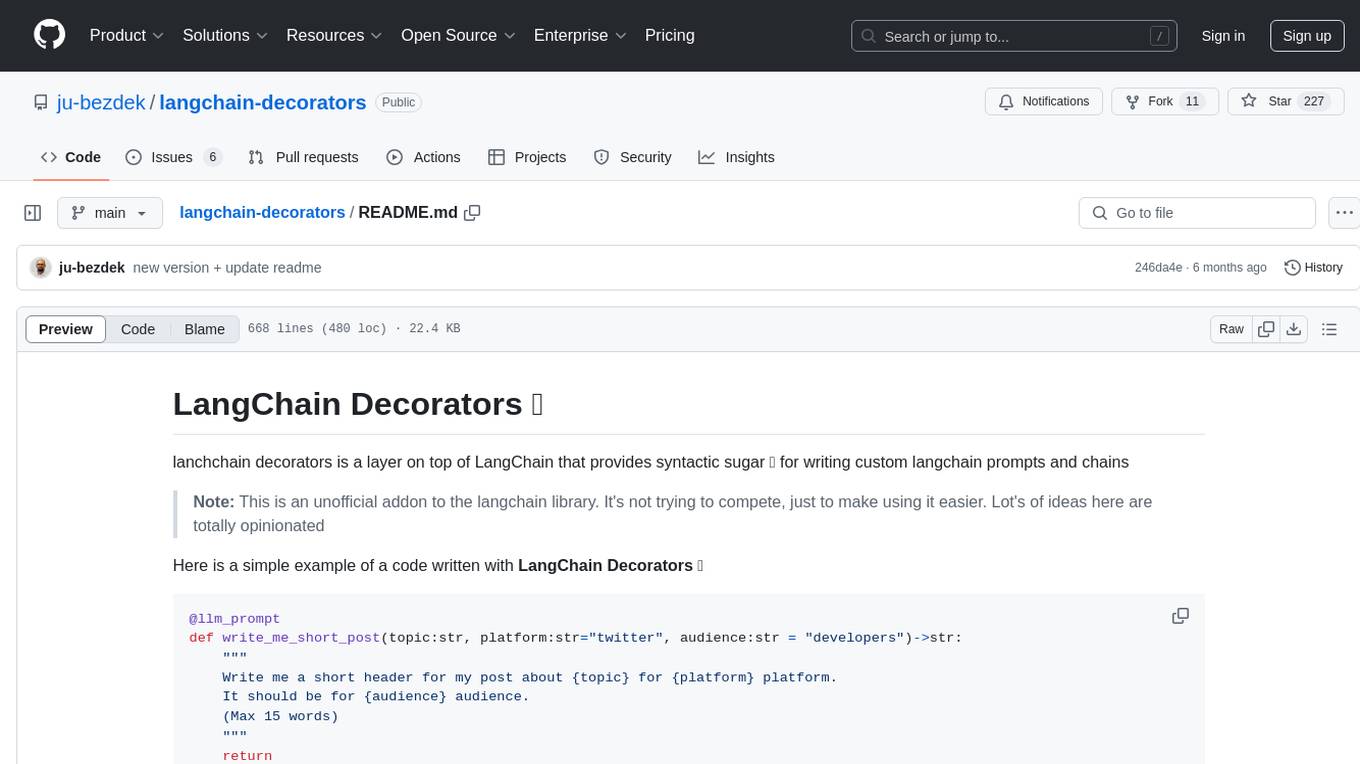
langchain-decorators
LangChain Decorators is a layer on top of LangChain that provides syntactic sugar for writing custom langchain prompts and chains. It offers a more pythonic way of writing code, multiline prompts without breaking code flow, IDE support for hinting and type checking, leveraging LangChain ecosystem, support for optional parameters, and sharing parameters between prompts. It simplifies streaming, automatic LLM selection, defining custom settings, debugging, and passing memory, callback, stop, etc. It also provides functions provider, dynamic function schemas, binding prompts to objects, defining custom settings, and debugging options. The project aims to enhance the LangChain library by making it easier to use and more efficient for writing custom prompts and chains.
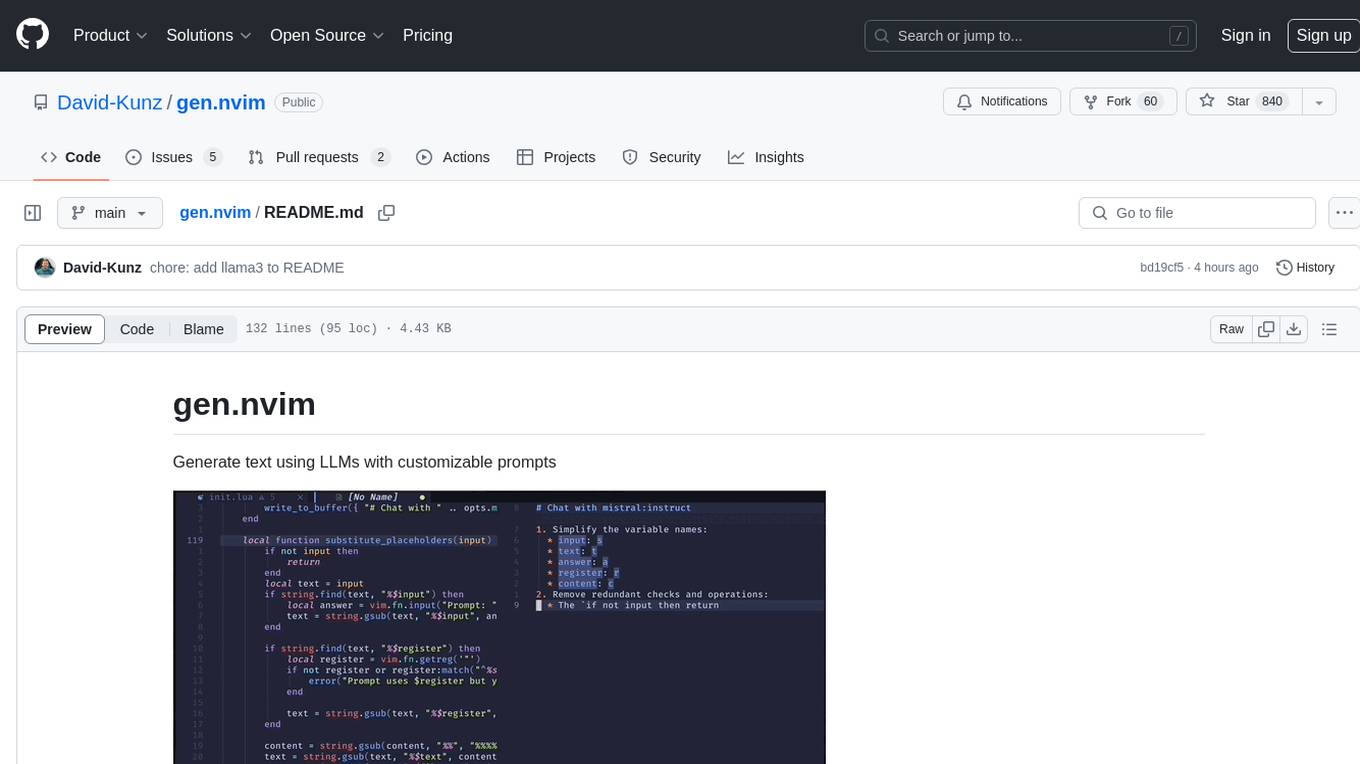
gen.nvim
gen.nvim is a tool that allows users to generate text using Language Models (LLMs) with customizable prompts. It requires Ollama with models like `llama3`, `mistral`, or `zephyr`, along with Curl for installation. Users can use the `Gen` command to generate text based on predefined or custom prompts. The tool provides key maps for easy invocation and allows for follow-up questions during conversations. Additionally, users can select a model from a list of installed models and customize prompts as needed.
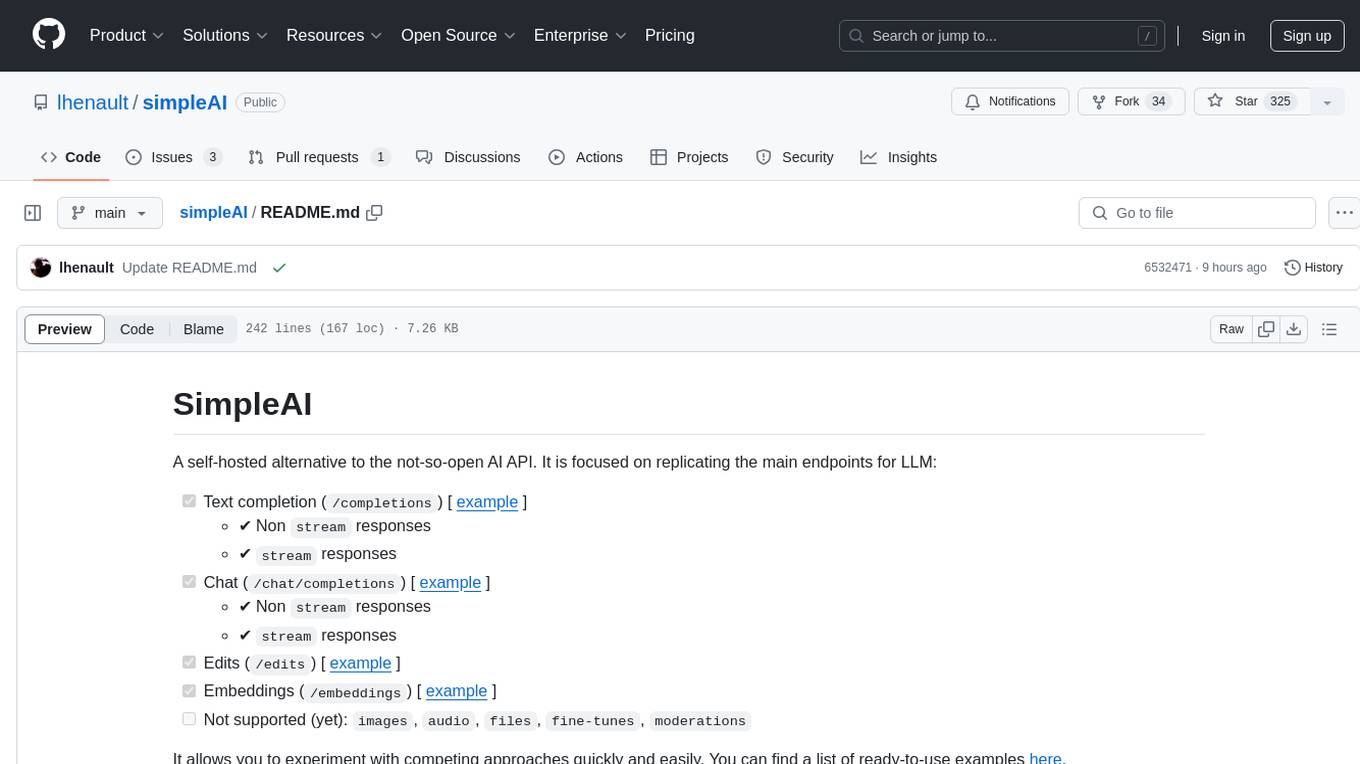
simpleAI
SimpleAI is a self-hosted alternative to the not-so-open AI API, focused on replicating main endpoints for LLM such as text completion, chat, edits, and embeddings. It allows quick experimentation with different models, creating benchmarks, and handling specific use cases without relying on external services. Users can integrate and declare models through gRPC, query endpoints using Swagger UI or API, and resolve common issues like CORS with FastAPI middleware. The project is open for contributions and welcomes PRs, issues, documentation, and more.
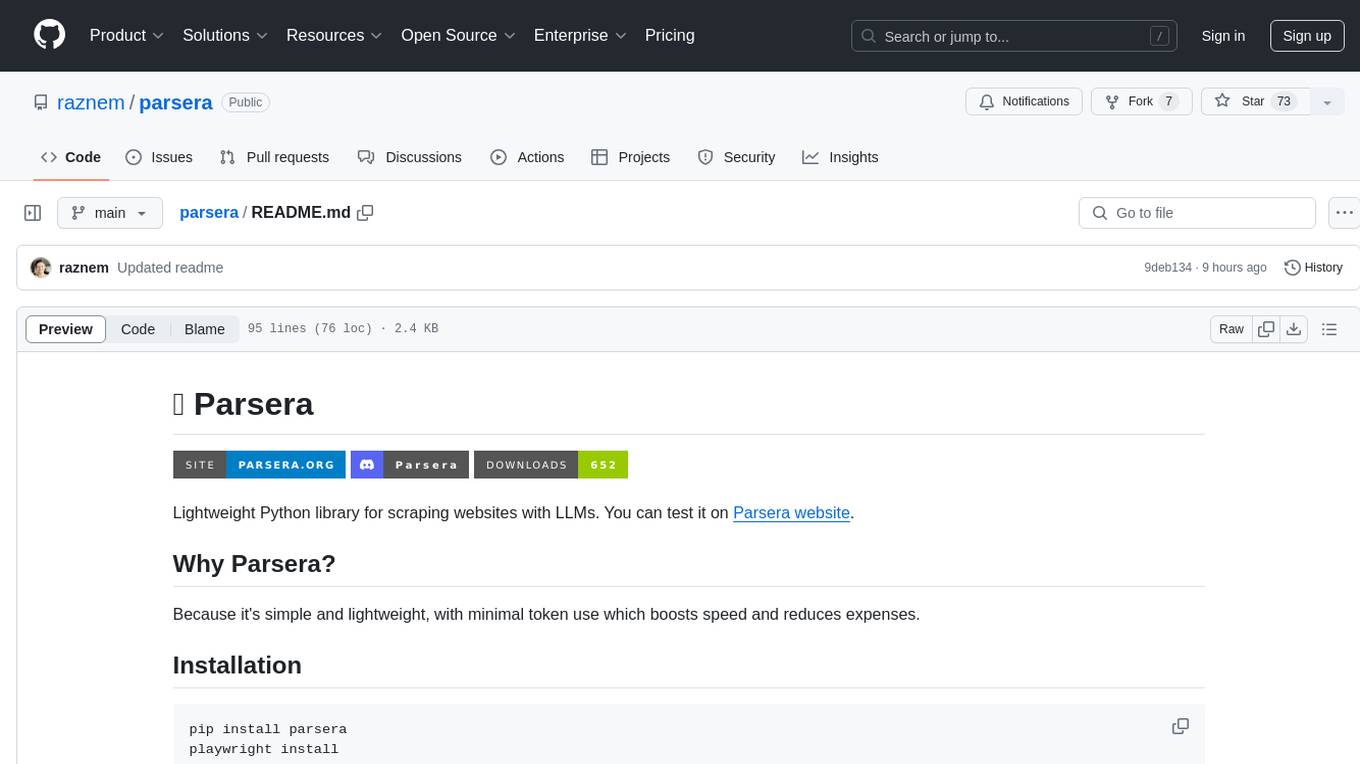
parsera
Parsera is a lightweight Python library designed for scraping websites using LLMs. It offers simplicity and efficiency by minimizing token usage, enhancing speed, and reducing costs. Users can easily set up and run the tool to extract specific elements from web pages, generating JSON output with relevant data. Additionally, Parsera supports integration with various chat models, such as Azure, expanding its functionality and customization options for web scraping tasks.
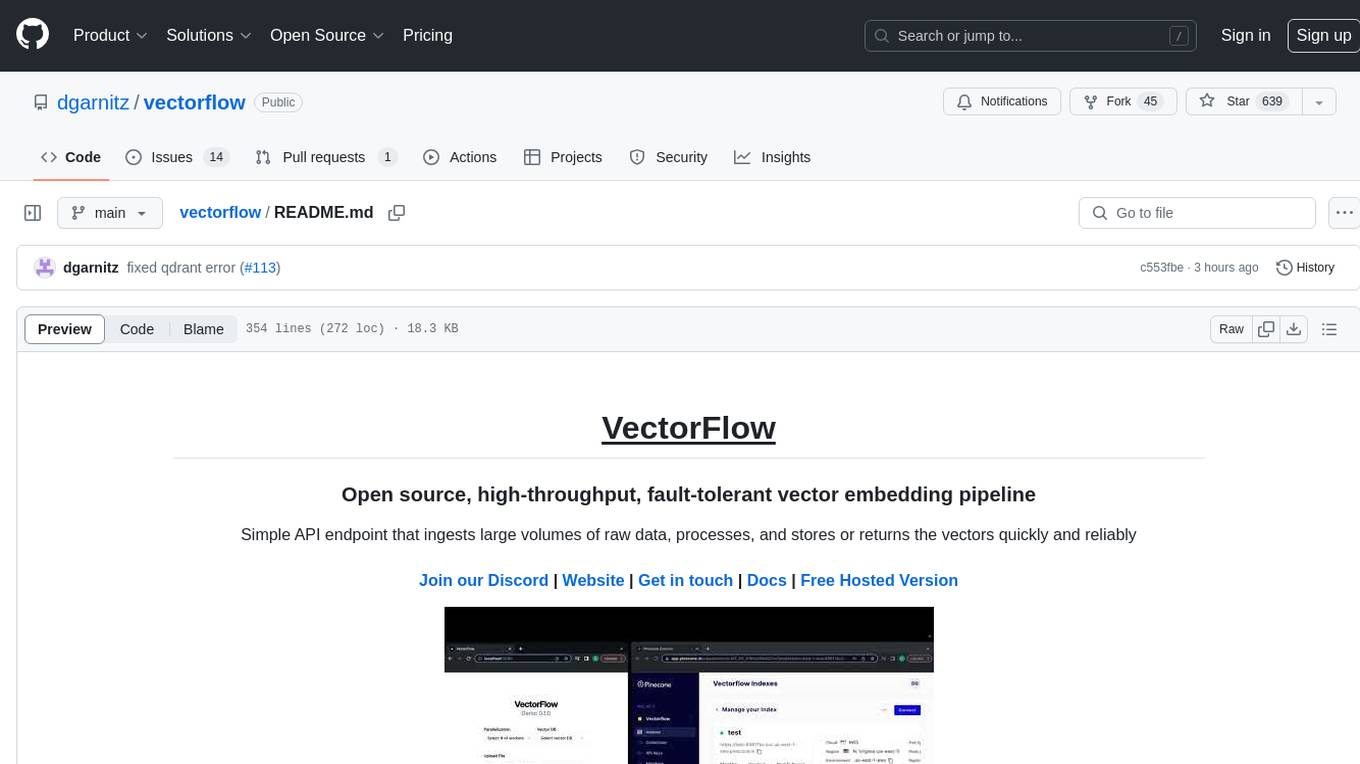
vectorflow
VectorFlow is an open source, high throughput, fault tolerant vector embedding pipeline. It provides a simple API endpoint for ingesting large volumes of raw data, processing, and storing or returning the vectors quickly and reliably. The tool supports text-based files like TXT, PDF, HTML, and DOCX, and can be run locally with Kubernetes in production. VectorFlow offers functionalities like embedding documents, running chunking schemas, custom chunking, and integrating with vector databases like Pinecone, Qdrant, and Weaviate. It enforces a standardized schema for uploading data to a vector store and supports features like raw embeddings webhook, chunk validation webhook, S3 endpoint, and telemetry. The tool can be used with the Python client and provides detailed instructions for running and testing the functionalities.
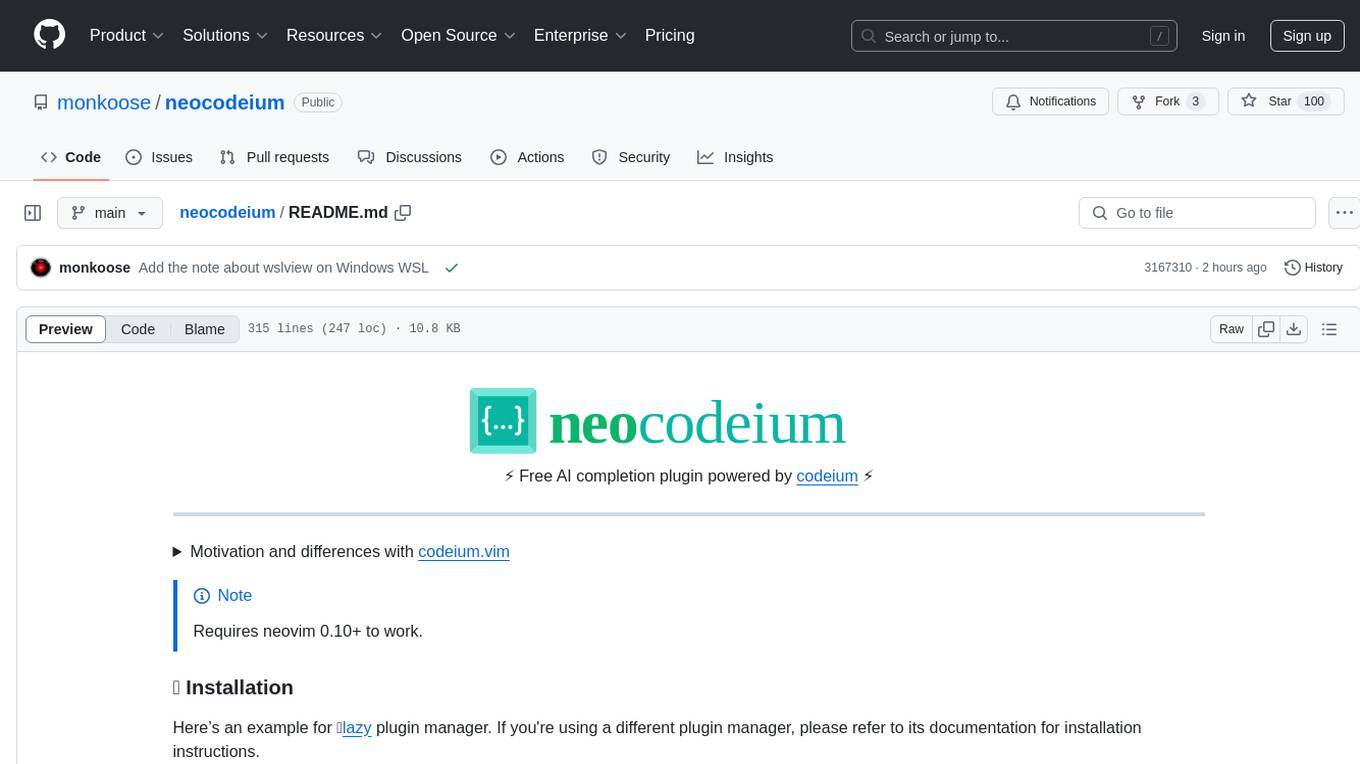
neocodeium
NeoCodeium is a free AI completion plugin powered by Codeium, designed for Neovim users. It aims to provide a smoother experience by eliminating flickering suggestions and allowing for repeatable completions using the `.` key. The plugin offers performance improvements through cache techniques, displays suggestion count labels, and supports Lua scripting. Users can customize keymaps, manage suggestions, and interact with the AI chat feature. NeoCodeium enhances code completion in Neovim, making it a valuable tool for developers seeking efficient coding assistance.
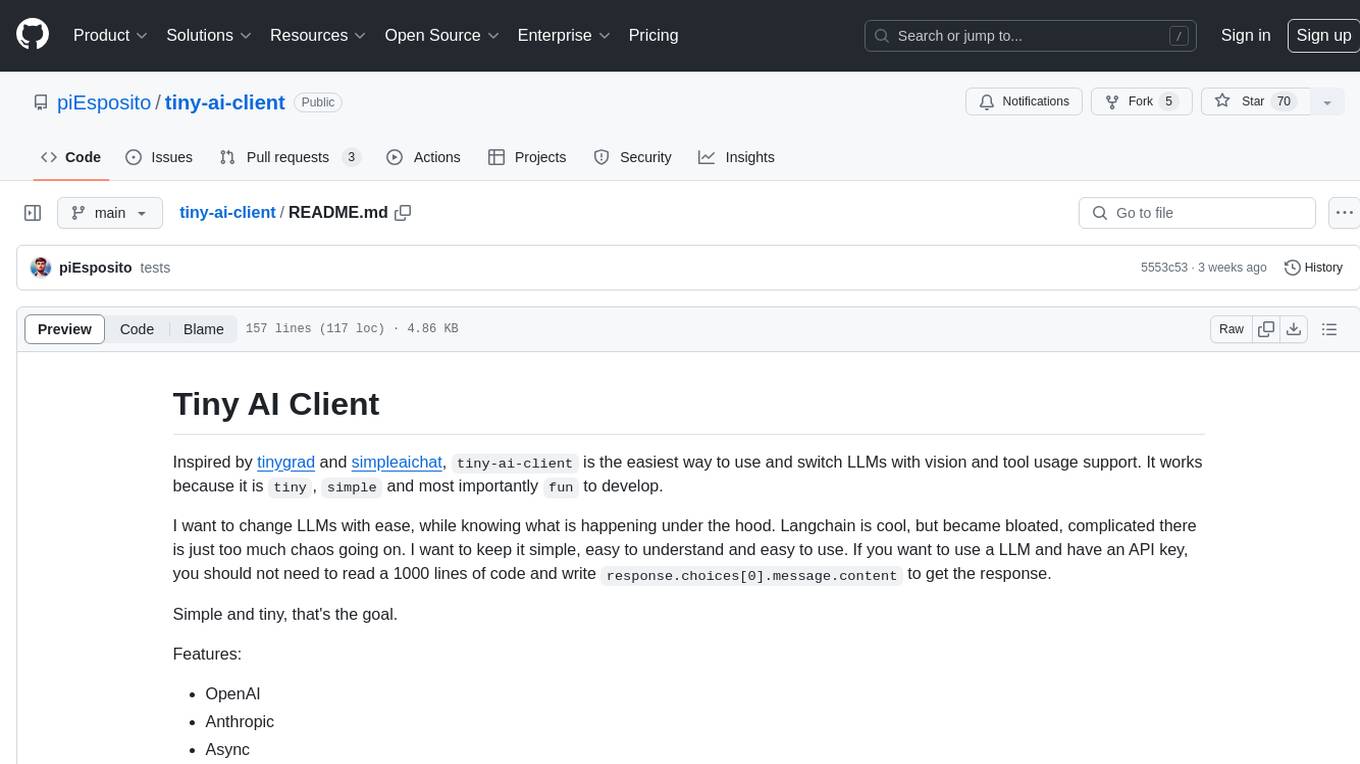
tiny-ai-client
Tiny AI Client is a lightweight tool designed for easy usage and switching of Language Model Models (LLMs) with support for vision and tool usage. It aims to provide a simple and intuitive interface for interacting with various LLMs, allowing users to easily set, change models, send messages, use tools, and handle vision tasks. The core logic of the tool is kept minimal and easy to understand, with separate modules for vision and tool usage utilities. Users can interact with the tool through simple Python scripts, passing model names, messages, tools, and images as required.
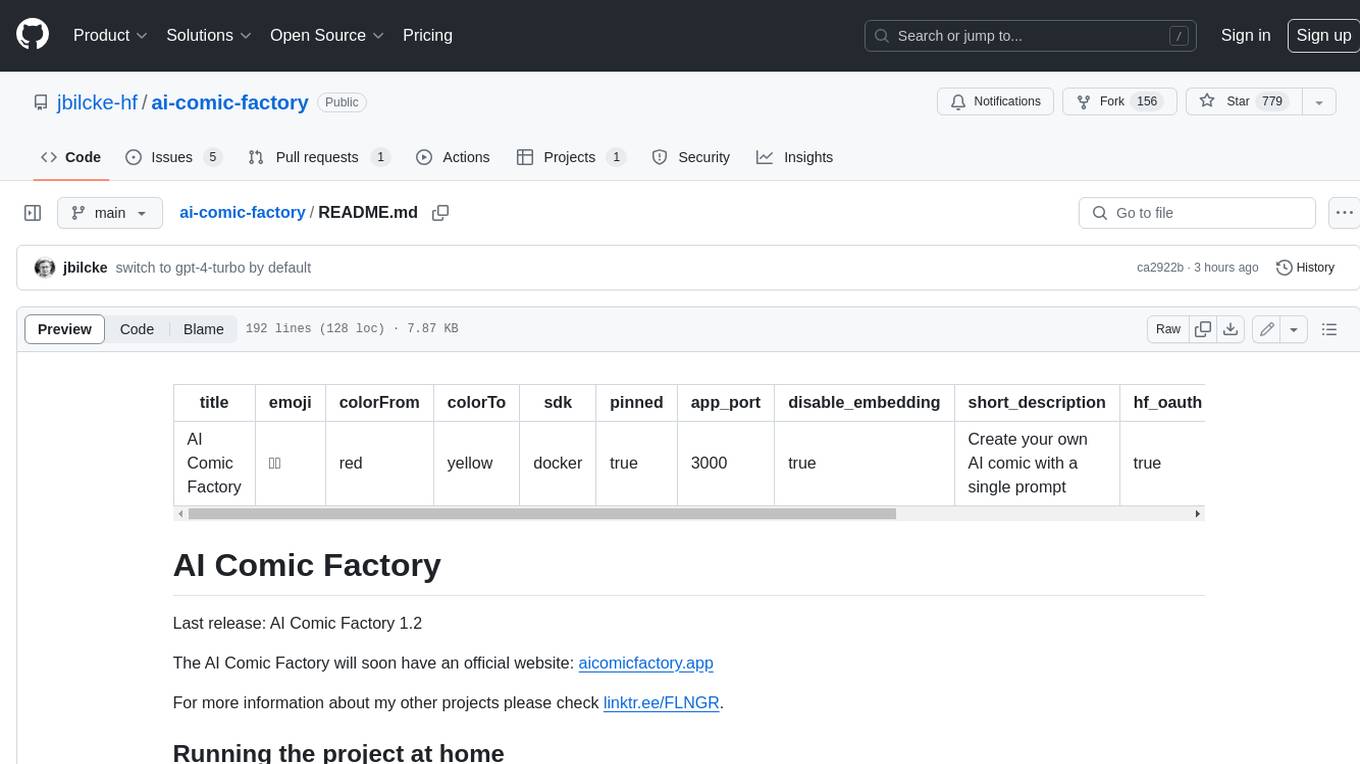
ai-comic-factory
The AI Comic Factory is a tool that allows you to create your own AI comics with a single prompt. It uses a large language model (LLM) to generate the story and dialogue, and a rendering API to generate the panel images. The AI Comic Factory is open-source and can be run on your own website or computer. It is a great tool for anyone who wants to create their own comics, or for anyone who is interested in the potential of AI for storytelling.
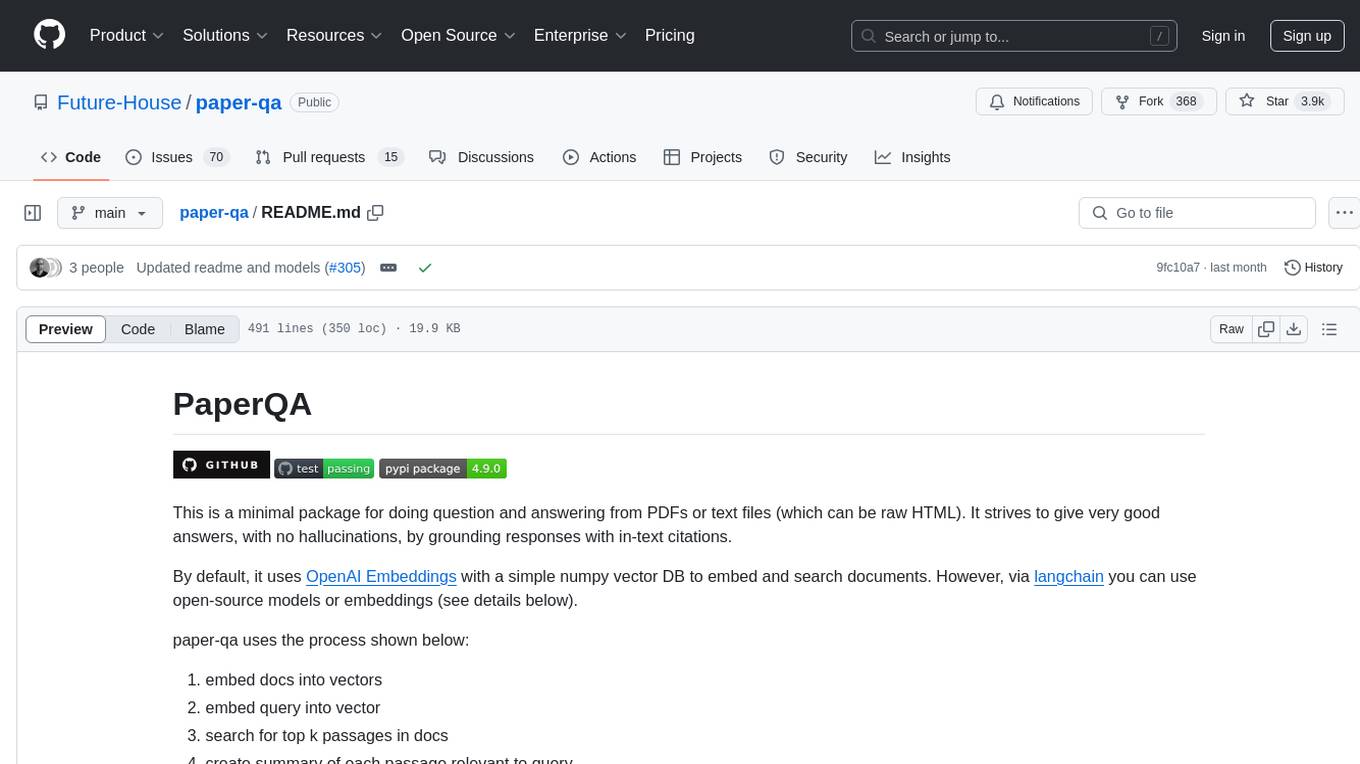
paper-qa
PaperQA is a minimal package for question and answering from PDFs or text files, providing very good answers with in-text citations. It uses OpenAI Embeddings to embed and search documents, and includes a process of embedding docs, queries, searching for top passages, creating summaries, using an LLM to re-score and select relevant summaries, putting summaries into prompt, and generating answers. The tool can be used to answer specific questions related to scientific research by leveraging citations and relevant passages from documents.
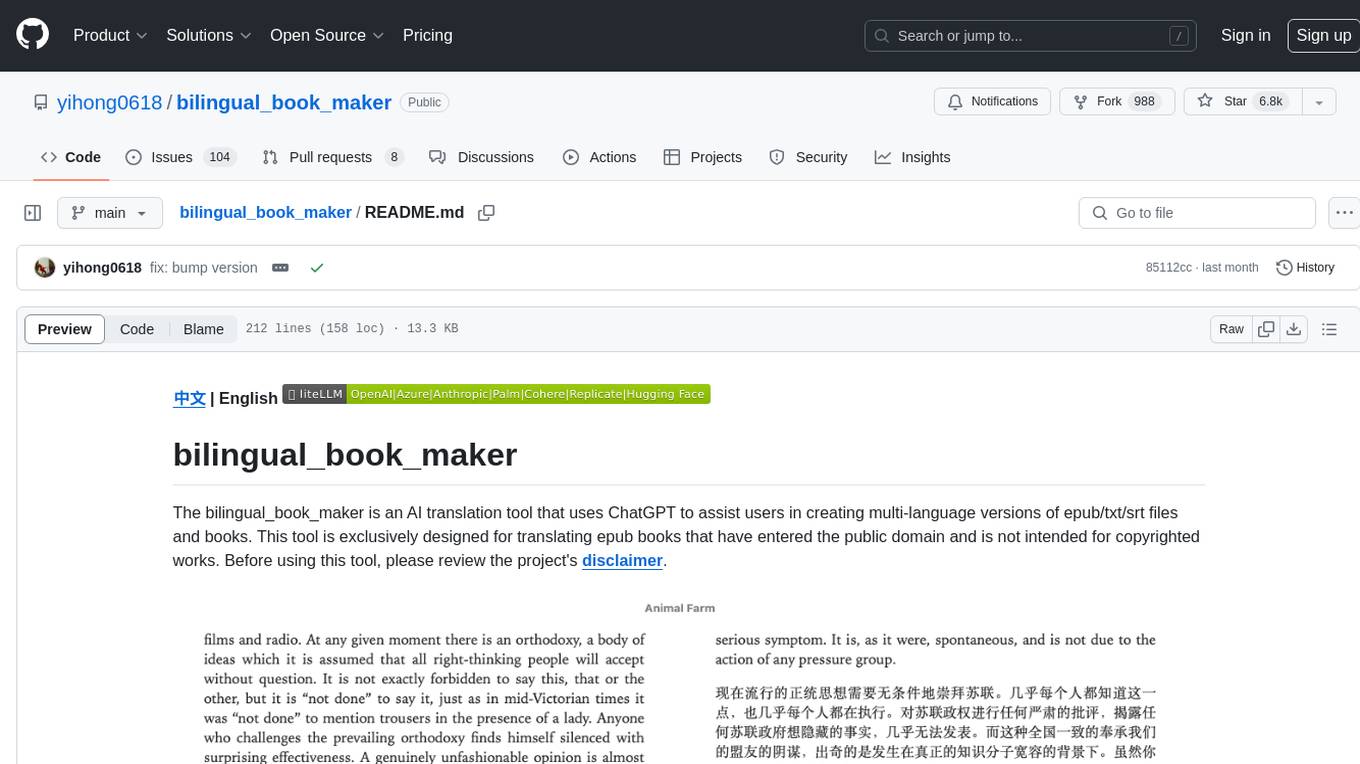
bilingual_book_maker
The bilingual_book_maker is an AI translation tool that uses ChatGPT to assist users in creating multi-language versions of epub/txt/srt files and books. It supports various models like gpt-4, gpt-3.5-turbo, claude-2, palm, llama-2, azure-openai, command-nightly, and gemini. Users need ChatGPT or OpenAI token, epub/txt books, internet access, and Python 3.8+. The tool provides options to specify OpenAI API key, model selection, target language, proxy server, context addition, translation style, and more. It generates bilingual books in epub format after translation. Users can test translations, set batch size, tweak prompts, and use different models like DeepL, Google Gemini, Tencent TranSmart, and more. The tool also supports retranslation, translating specific tags, and e-reader type specification. Docker usage is available for easy setup.
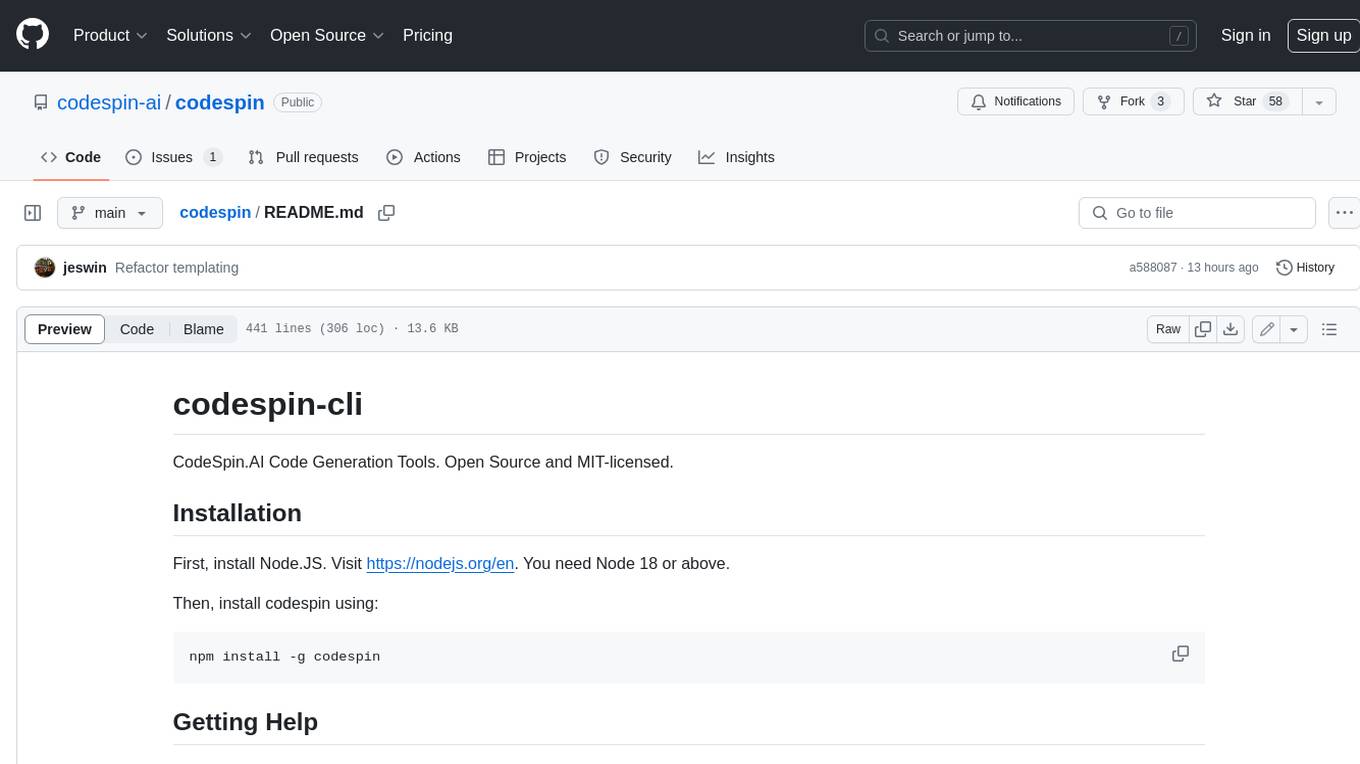
codespin
CodeSpin.AI is a set of open-source code generation tools that leverage large language models (LLMs) to automate coding tasks. With CodeSpin, you can generate code in various programming languages, including Python, JavaScript, Java, and C++, by providing natural language prompts. CodeSpin offers a range of features to enhance code generation, such as custom templates, inline prompting, and the ability to use ChatGPT as an alternative to API keys. Additionally, CodeSpin provides options for regenerating code, executing code in prompt files, and piping data into the LLM for processing. By utilizing CodeSpin, developers can save time and effort in coding tasks, improve code quality, and explore new possibilities in code generation.
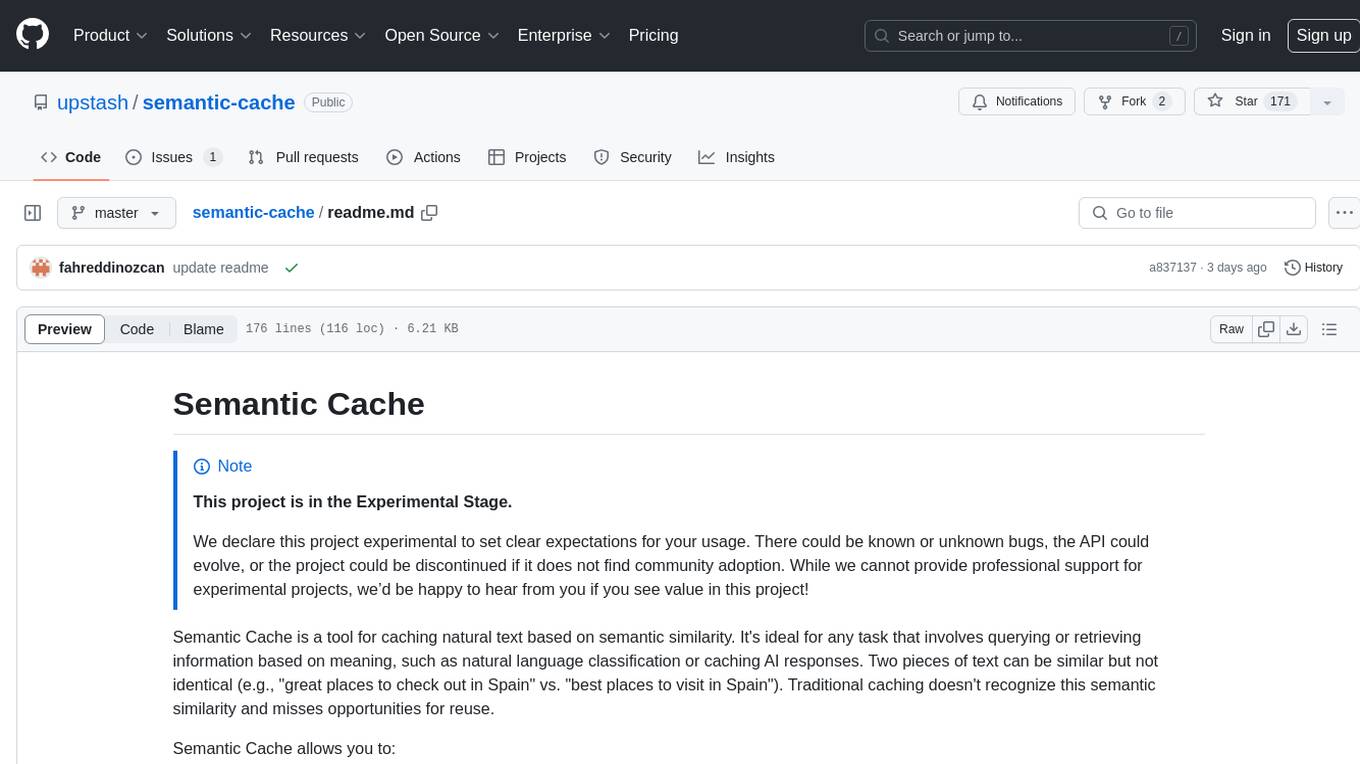
semantic-cache
Semantic Cache is a tool for caching natural text based on semantic similarity. It allows for classifying text into categories, caching AI responses, and reducing API latency by responding to similar queries with cached values. The tool stores cache entries by meaning, handles synonyms, supports multiple languages, understands complex queries, and offers easy integration with Node.js applications. Users can set a custom proximity threshold for filtering results. The tool is ideal for tasks involving querying or retrieving information based on meaning, such as natural language classification or caching AI responses.
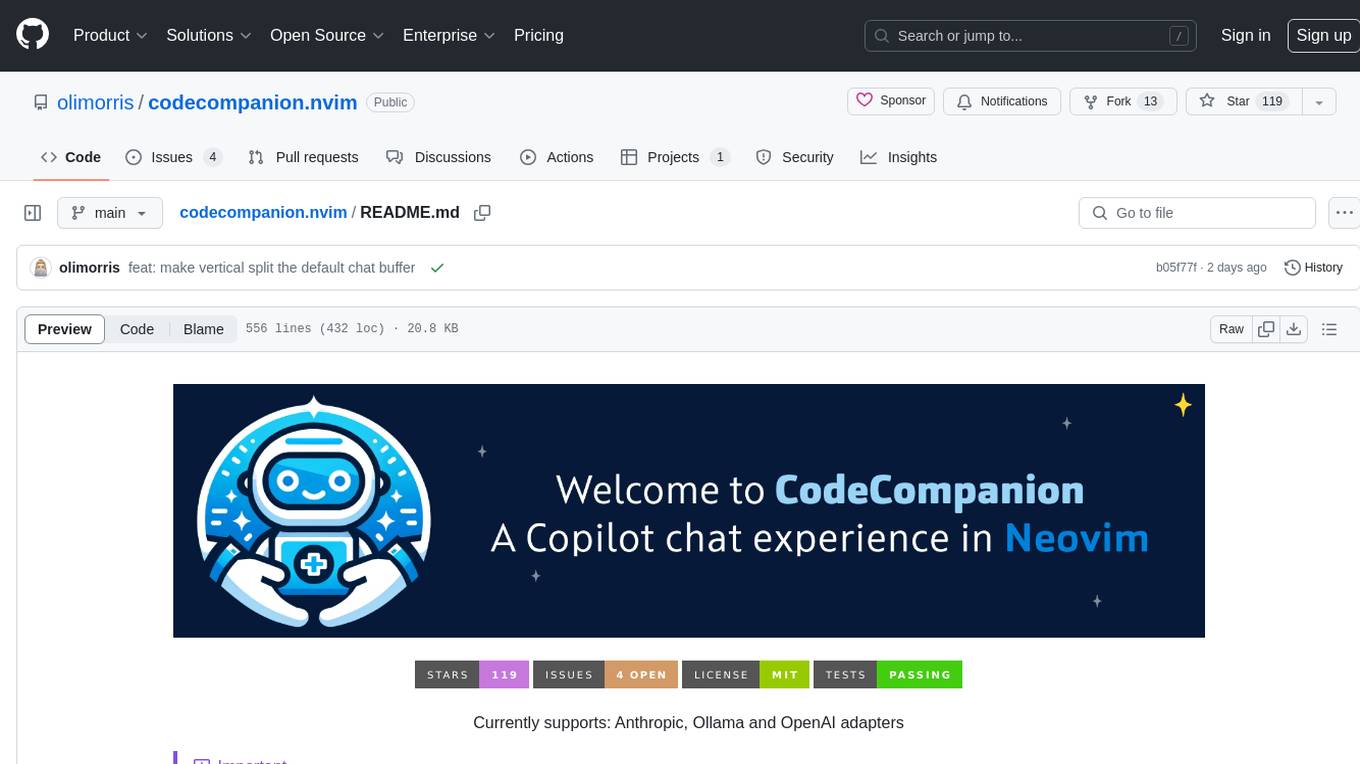
codecompanion.nvim
CodeCompanion.nvim is a Neovim plugin that provides a Copilot Chat experience, adapter support for various LLMs, agentic workflows, inline code creation and modification, built-in actions for language prompts and error fixes, custom actions creation, async execution, and more. It supports Anthropic, Ollama, and OpenAI adapters. The plugin is primarily developed for personal workflows with no guarantees of regular updates or support. Users can customize the plugin to their needs by forking the project.
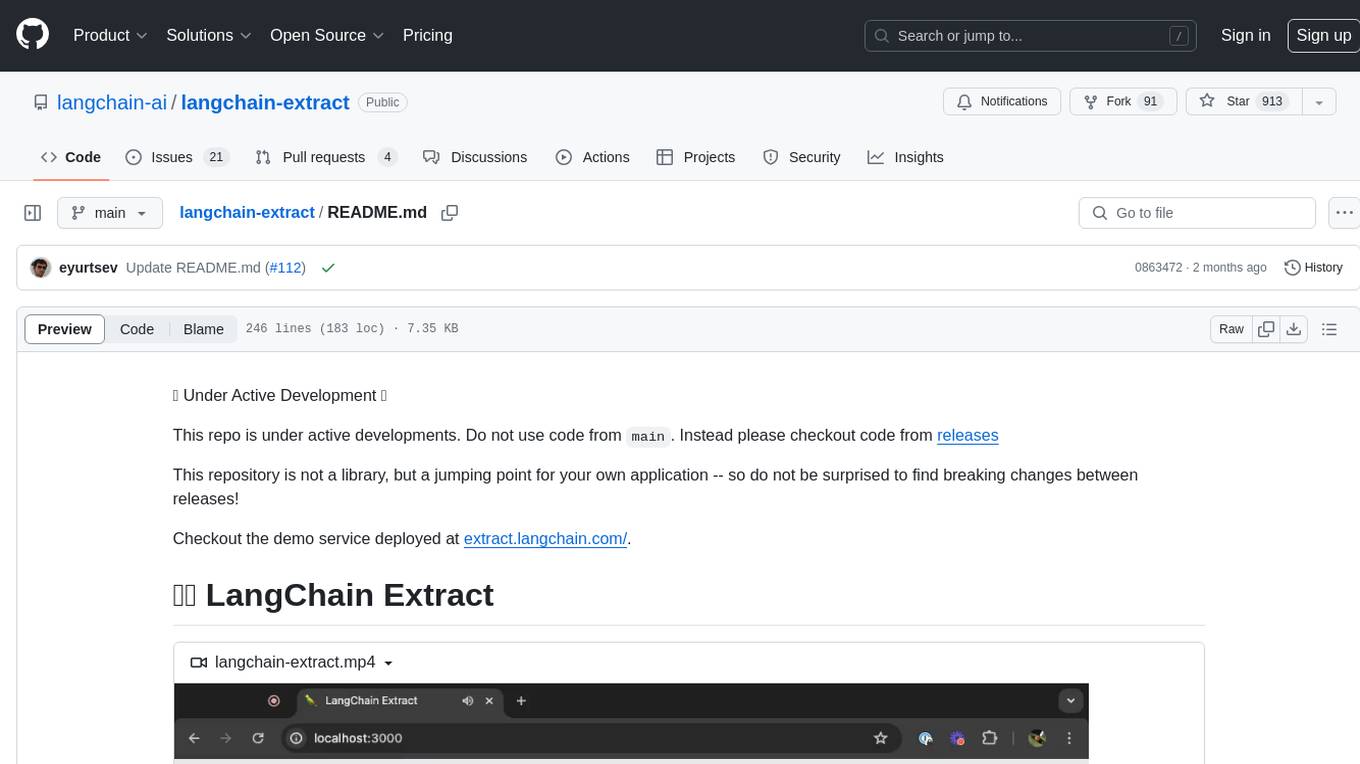
langchain-extract
LangChain Extract is a simple web server that allows you to extract information from text and files using LLMs. It is built using FastAPI, LangChain, and Postgresql. The backend closely follows the extraction use-case documentation and provides a reference implementation of an app that helps to do extraction over data using LLMs. This repository is meant to be a starting point for building your own extraction application which may have slightly different requirements or use cases.
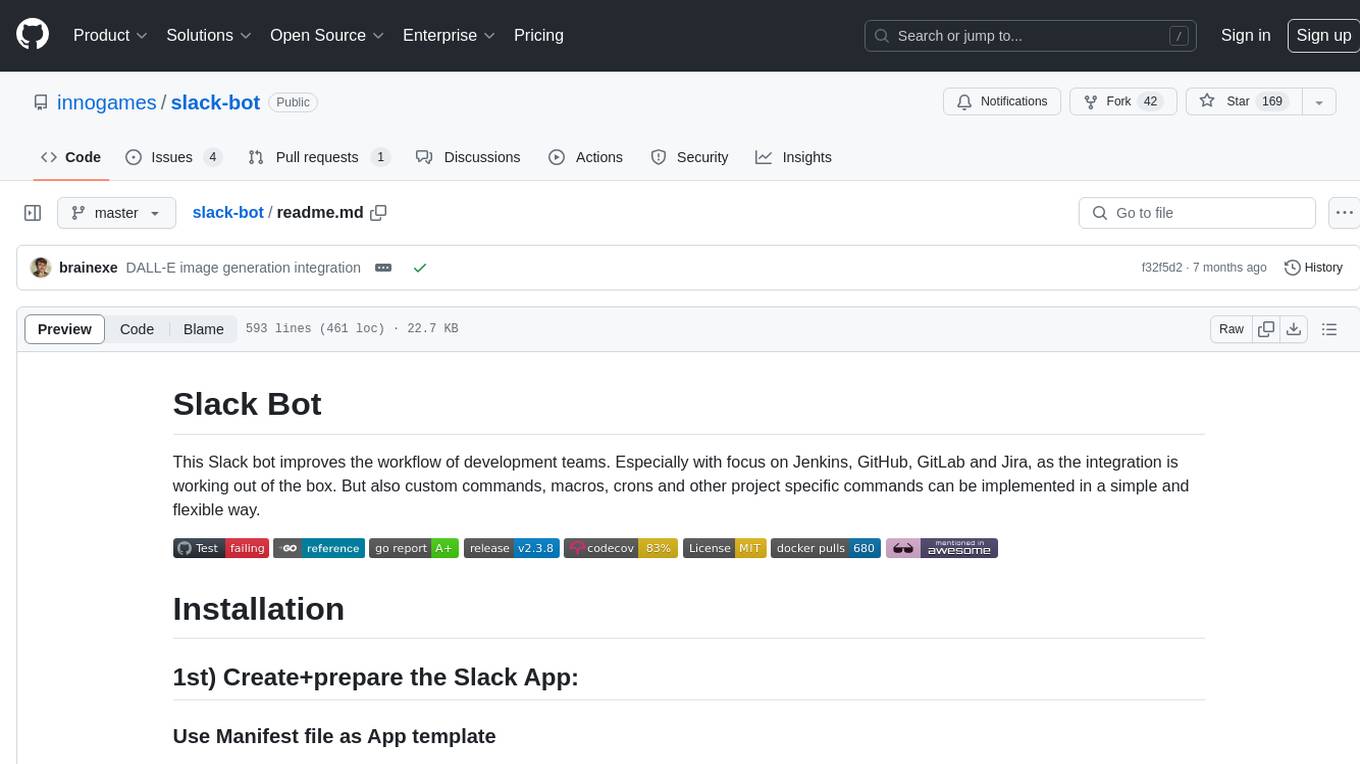
slack-bot
The Slack Bot is a tool designed to enhance the workflow of development teams by integrating with Jenkins, GitHub, GitLab, and Jira. It allows for custom commands, macros, crons, and project-specific commands to be implemented easily. Users can interact with the bot through Slack messages, execute commands, and monitor job progress. The bot supports features like starting and monitoring Jenkins jobs, tracking pull requests, querying Jira information, creating buttons for interactions, generating images with DALL-E, playing quiz games, checking weather, defining custom commands, and more. Configuration is managed via YAML files, allowing users to set up credentials for external services, define custom commands, schedule cron jobs, and configure VCS systems like Bitbucket for automated branch lookup in Jenkins triggers.
For similar tasks
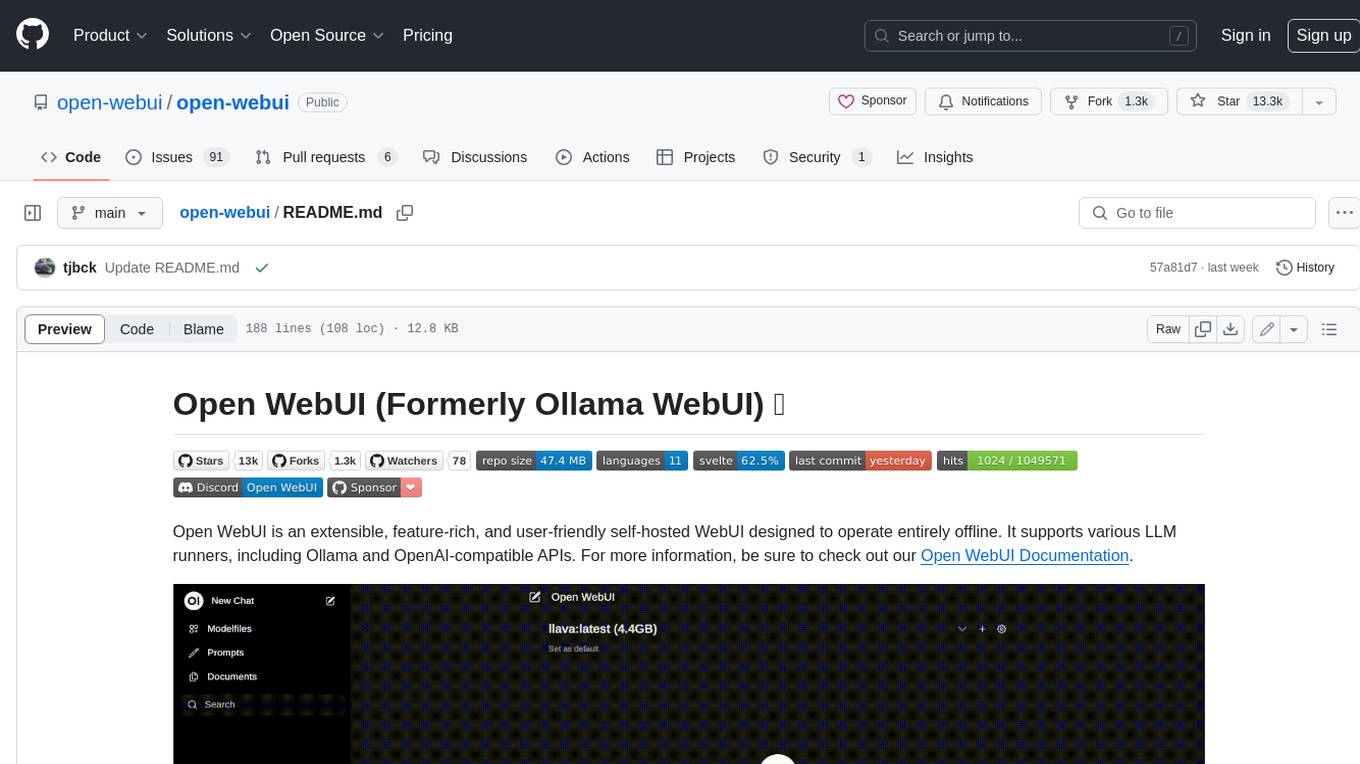
open-webui
Open WebUI is an extensible, feature-rich, and user-friendly self-hosted WebUI designed to operate entirely offline. It supports various LLM runners, including Ollama and OpenAI-compatible APIs. For more information, be sure to check out our Open WebUI Documentation.
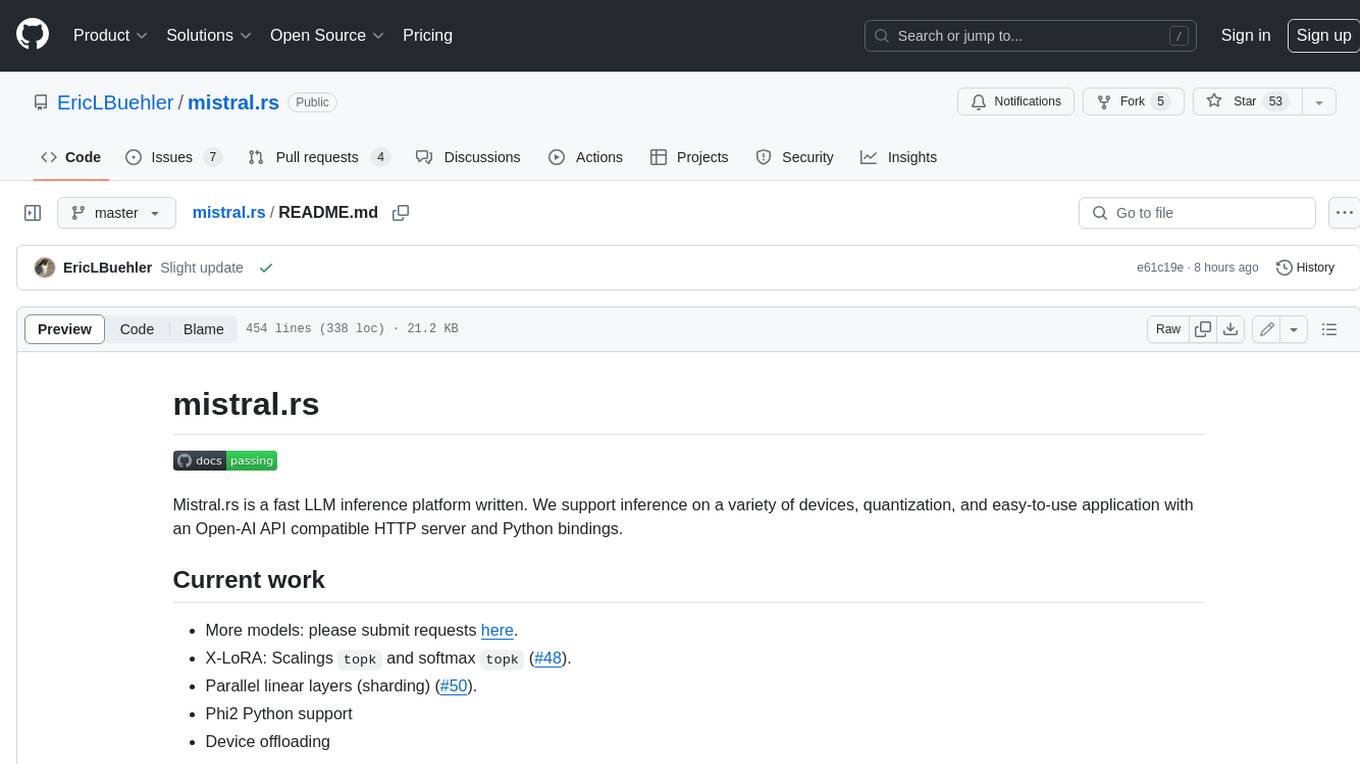
mistral.rs
Mistral.rs is a fast LLM inference platform written in Rust. We support inference on a variety of devices, quantization, and easy-to-use application with an Open-AI API compatible HTTP server and Python bindings.
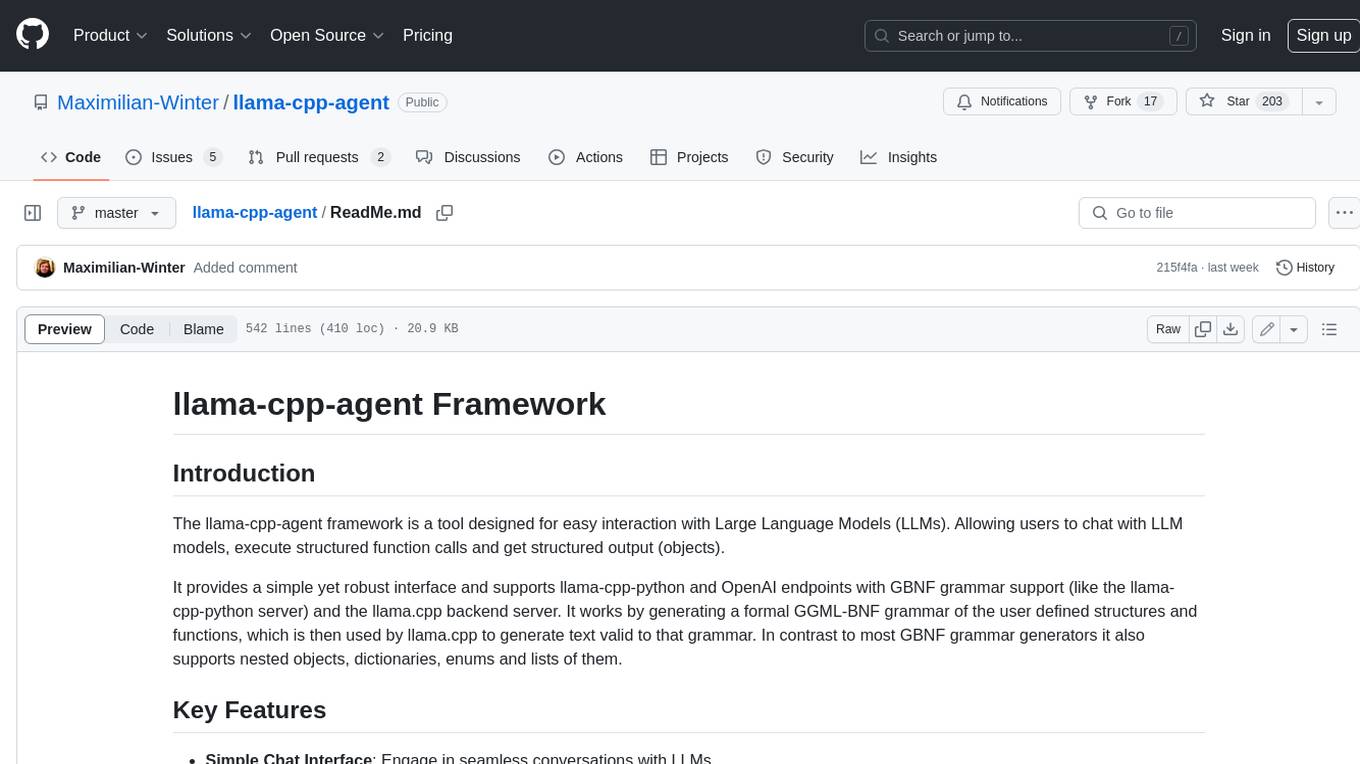
llama-cpp-agent
The llama-cpp-agent framework is a tool designed for easy interaction with Large Language Models (LLMs). Allowing users to chat with LLM models, execute structured function calls and get structured output (objects). It provides a simple yet robust interface and supports llama-cpp-python and OpenAI endpoints with GBNF grammar support (like the llama-cpp-python server) and the llama.cpp backend server. It works by generating a formal GGML-BNF grammar of the user defined structures and functions, which is then used by llama.cpp to generate text valid to that grammar. In contrast to most GBNF grammar generators it also supports nested objects, dictionaries, enums and lists of them.
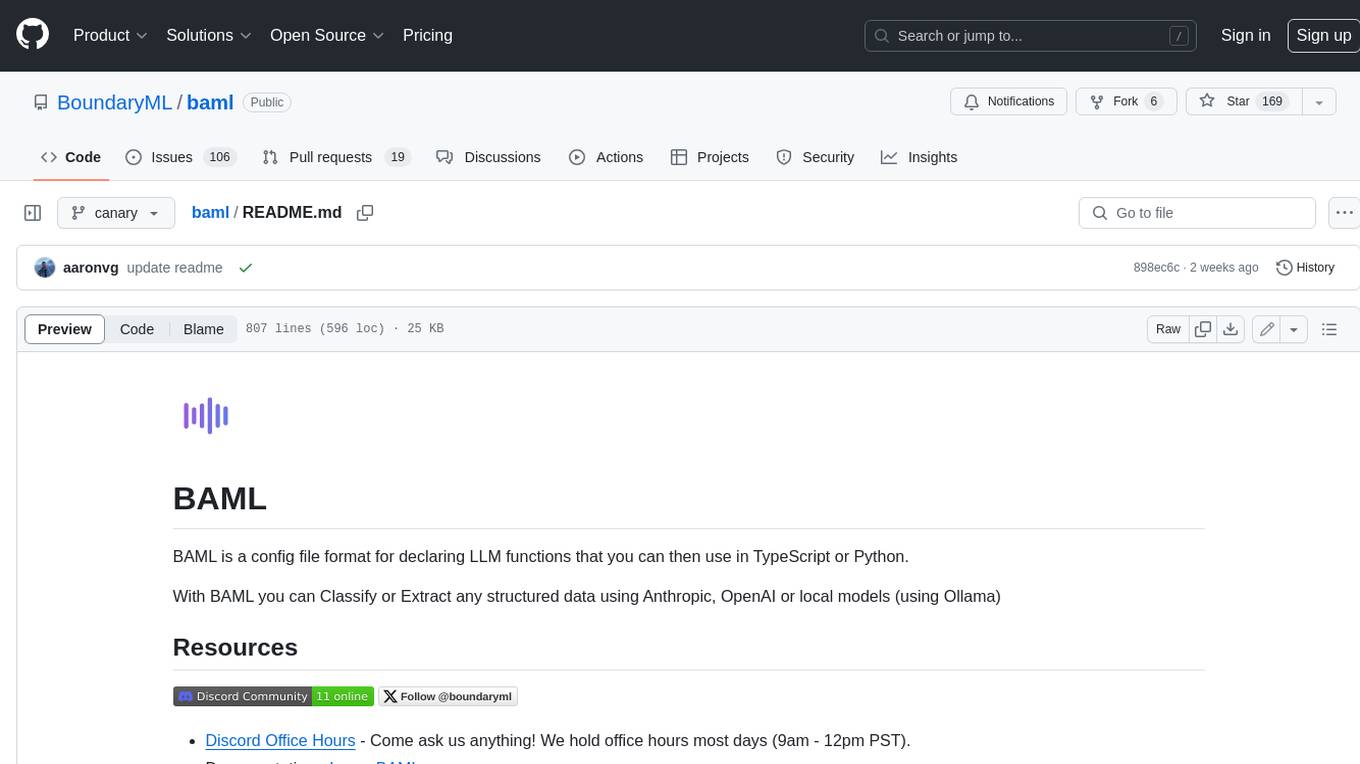
baml
BAML is a config file format for declaring LLM functions that you can then use in TypeScript or Python. With BAML you can Classify or Extract any structured data using Anthropic, OpenAI or local models (using Ollama) ## Resources  [Discord Community](https://discord.gg/boundaryml)  [Follow us on Twitter](https://twitter.com/boundaryml) * Discord Office Hours - Come ask us anything! We hold office hours most days (9am - 12pm PST). * Documentation - Learn BAML * Documentation - BAML Syntax Reference * Documentation - Prompt engineering tips * Boundary Studio - Observability and more #### Starter projects * BAML + NextJS 14 * BAML + FastAPI + Streaming ## Motivation Calling LLMs in your code is frustrating: * your code uses types everywhere: classes, enums, and arrays * but LLMs speak English, not types BAML makes calling LLMs easy by taking a type-first approach that lives fully in your codebase: 1. Define what your LLM output type is in a .baml file, with rich syntax to describe any field (even enum values) 2. Declare your prompt in the .baml config using those types 3. Add additional LLM config like retries or redundancy 4. Transpile the .baml files to a callable Python or TS function with a type-safe interface. (VSCode extension does this for you automatically). We were inspired by similar patterns for type safety: protobuf and OpenAPI for RPCs, Prisma and SQLAlchemy for databases. BAML guarantees type safety for LLMs and comes with tools to give you a great developer experience:  Jump to BAML code or how Flexible Parsing works without additional LLM calls. | BAML Tooling | Capabilities | | ----------------------------------------------------------------------------------------- | ---------------------------------------------------------------------------------------------------------------------------------------------------------------------------------------------------------------------------------------------------------------------------------------------------------------------------------- | | BAML Compiler install | Transpiles BAML code to a native Python / Typescript library (you only need it for development, never for releases) Works on Mac, Windows, Linux  | | VSCode Extension install | Syntax highlighting for BAML files Real-time prompt preview Testing UI | | Boundary Studio open (not open source) | Type-safe observability Labeling |
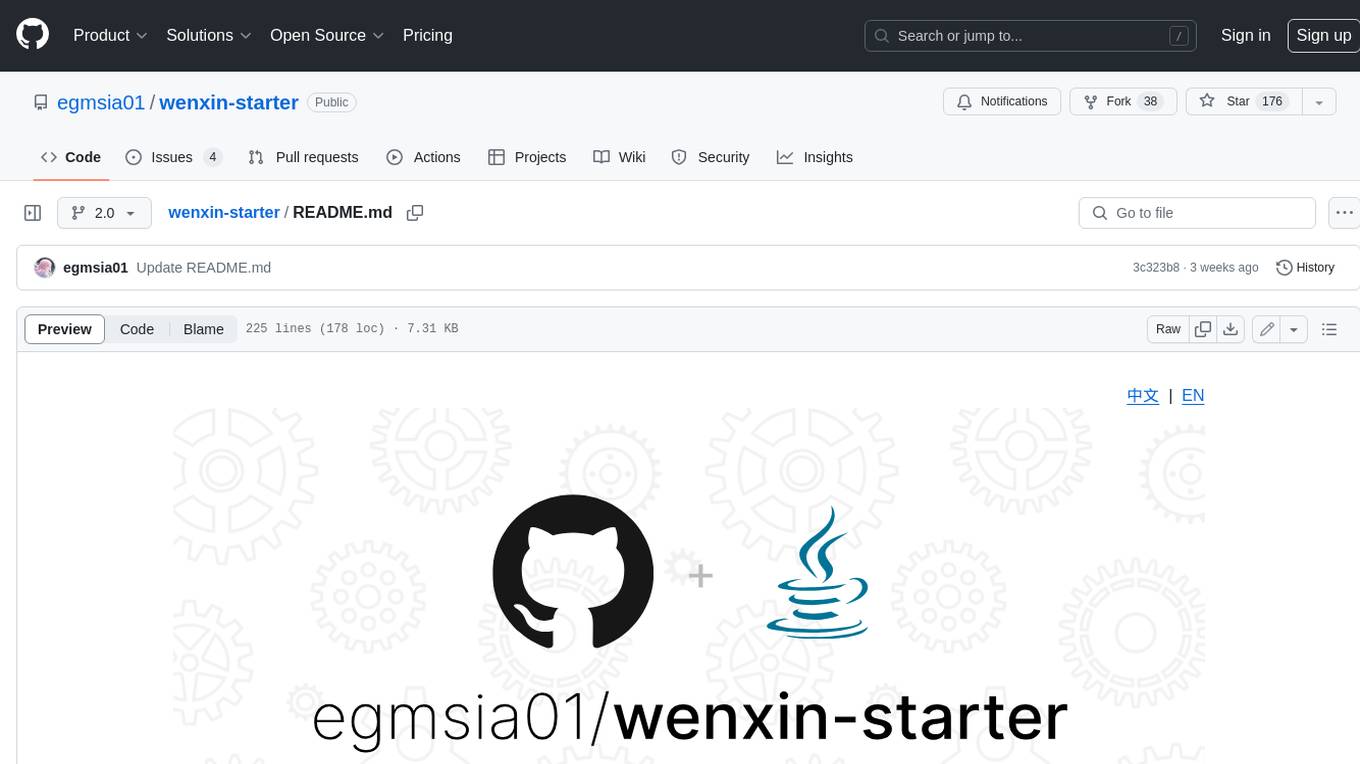
wenxin-starter
WenXin-Starter is a spring-boot-starter for Baidu's "Wenxin Qianfan WENXINWORKSHOP" large model, which can help you quickly access Baidu's AI capabilities. It fully integrates the official API documentation of Wenxin Qianfan. Supports text-to-image generation, built-in dialogue memory, and supports streaming return of dialogue. Supports QPS control of a single model and supports queuing mechanism. Plugins will be added soon.
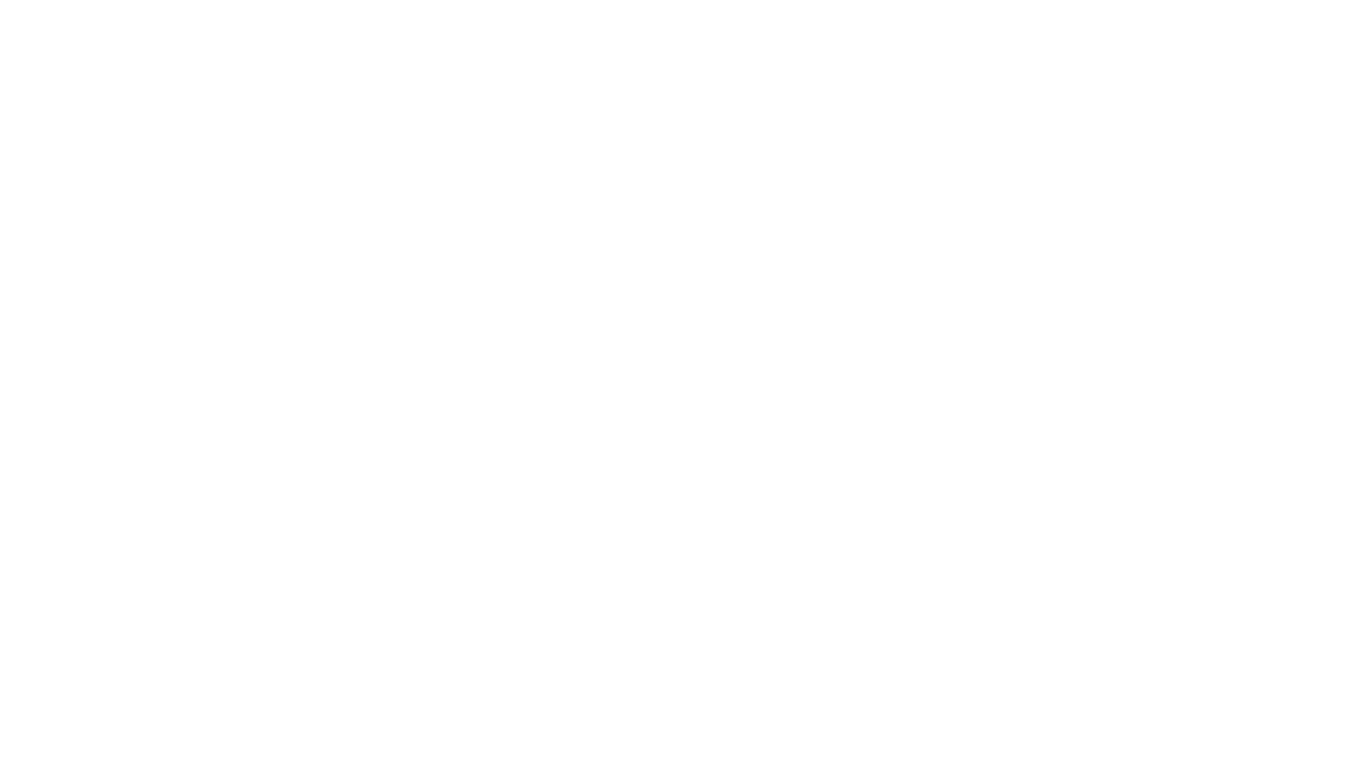
intel-extension-for-transformers
Intel® Extension for Transformers is an innovative toolkit designed to accelerate GenAI/LLM everywhere with the optimal performance of Transformer-based models on various Intel platforms, including Intel Gaudi2, Intel CPU, and Intel GPU. The toolkit provides the below key features and examples: * Seamless user experience of model compressions on Transformer-based models by extending [Hugging Face transformers](https://github.com/huggingface/transformers) APIs and leveraging [Intel® Neural Compressor](https://github.com/intel/neural-compressor) * Advanced software optimizations and unique compression-aware runtime (released with NeurIPS 2022's paper [Fast Distilbert on CPUs](https://arxiv.org/abs/2211.07715) and [QuaLA-MiniLM: a Quantized Length Adaptive MiniLM](https://arxiv.org/abs/2210.17114), and NeurIPS 2021's paper [Prune Once for All: Sparse Pre-Trained Language Models](https://arxiv.org/abs/2111.05754)) * Optimized Transformer-based model packages such as [Stable Diffusion](examples/huggingface/pytorch/text-to-image/deployment/stable_diffusion), [GPT-J-6B](examples/huggingface/pytorch/text-generation/deployment), [GPT-NEOX](examples/huggingface/pytorch/language-modeling/quantization#2-validated-model-list), [BLOOM-176B](examples/huggingface/pytorch/language-modeling/inference#BLOOM-176B), [T5](examples/huggingface/pytorch/summarization/quantization#2-validated-model-list), [Flan-T5](examples/huggingface/pytorch/summarization/quantization#2-validated-model-list), and end-to-end workflows such as [SetFit-based text classification](docs/tutorials/pytorch/text-classification/SetFit_model_compression_AGNews.ipynb) and [document level sentiment analysis (DLSA)](workflows/dlsa) * [NeuralChat](intel_extension_for_transformers/neural_chat), a customizable chatbot framework to create your own chatbot within minutes by leveraging a rich set of [plugins](https://github.com/intel/intel-extension-for-transformers/blob/main/intel_extension_for_transformers/neural_chat/docs/advanced_features.md) such as [Knowledge Retrieval](./intel_extension_for_transformers/neural_chat/pipeline/plugins/retrieval/README.md), [Speech Interaction](./intel_extension_for_transformers/neural_chat/pipeline/plugins/audio/README.md), [Query Caching](./intel_extension_for_transformers/neural_chat/pipeline/plugins/caching/README.md), and [Security Guardrail](./intel_extension_for_transformers/neural_chat/pipeline/plugins/security/README.md). This framework supports Intel Gaudi2/CPU/GPU. * [Inference](https://github.com/intel/neural-speed/tree/main) of Large Language Model (LLM) in pure C/C++ with weight-only quantization kernels for Intel CPU and Intel GPU (TBD), supporting [GPT-NEOX](https://github.com/intel/neural-speed/tree/main/neural_speed/models/gptneox), [LLAMA](https://github.com/intel/neural-speed/tree/main/neural_speed/models/llama), [MPT](https://github.com/intel/neural-speed/tree/main/neural_speed/models/mpt), [FALCON](https://github.com/intel/neural-speed/tree/main/neural_speed/models/falcon), [BLOOM-7B](https://github.com/intel/neural-speed/tree/main/neural_speed/models/bloom), [OPT](https://github.com/intel/neural-speed/tree/main/neural_speed/models/opt), [ChatGLM2-6B](https://github.com/intel/neural-speed/tree/main/neural_speed/models/chatglm), [GPT-J-6B](https://github.com/intel/neural-speed/tree/main/neural_speed/models/gptj), and [Dolly-v2-3B](https://github.com/intel/neural-speed/tree/main/neural_speed/models/gptneox). Support AMX, VNNI, AVX512F and AVX2 instruction set. We've boosted the performance of Intel CPUs, with a particular focus on the 4th generation Intel Xeon Scalable processor, codenamed [Sapphire Rapids](https://www.intel.com/content/www/us/en/products/docs/processors/xeon-accelerated/4th-gen-xeon-scalable-processors.html).
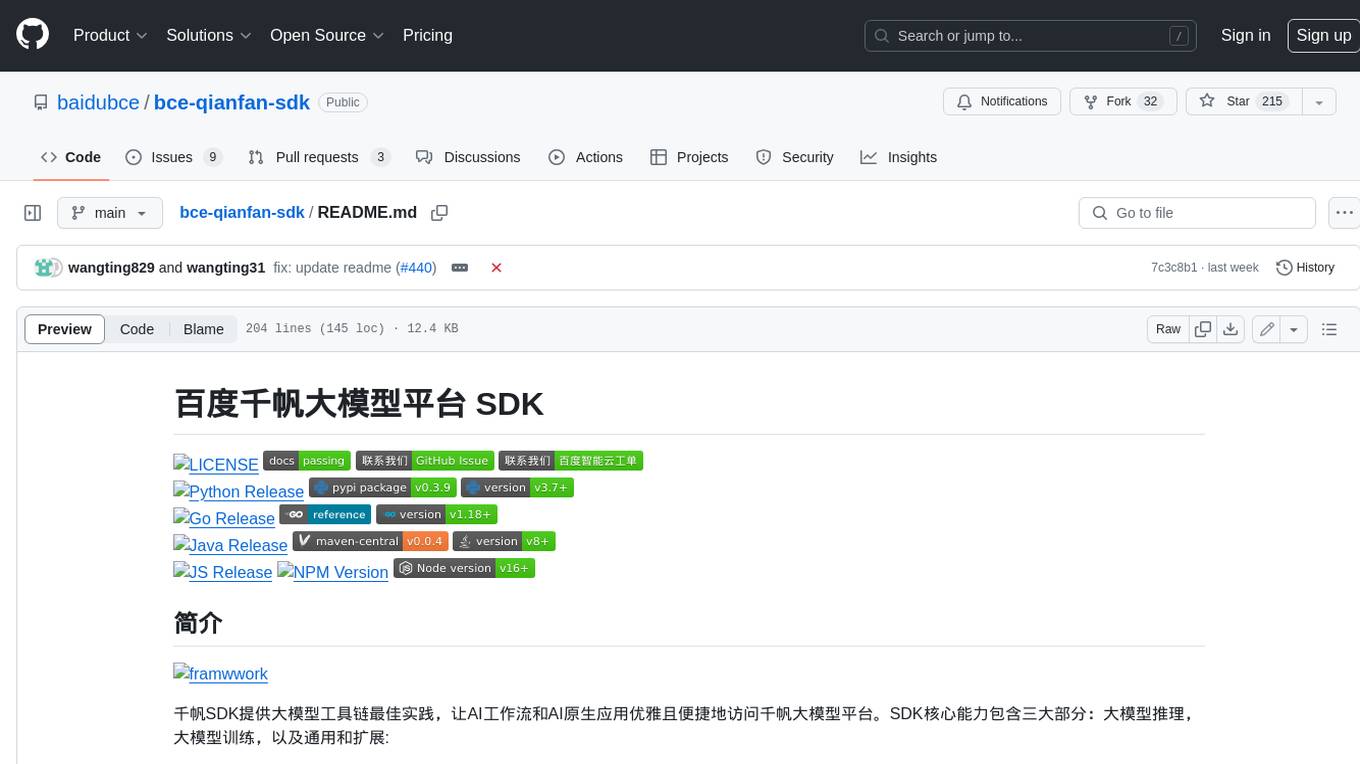
bce-qianfan-sdk
The Qianfan SDK provides best practices for large model toolchains, allowing AI workflows and AI-native applications to access the Qianfan large model platform elegantly and conveniently. The core capabilities of the SDK include three parts: large model reasoning, large model training, and general and extension: * `Large model reasoning`: Implements interface encapsulation for reasoning of Yuyan (ERNIE-Bot) series, open source large models, etc., supporting dialogue, completion, Embedding, etc. * `Large model training`: Based on platform capabilities, it supports end-to-end large model training process, including training data, fine-tuning/pre-training, and model services. * `General and extension`: General capabilities include common AI development tools such as Prompt/Debug/Client. The extension capability is based on the characteristics of Qianfan to adapt to common middleware frameworks.
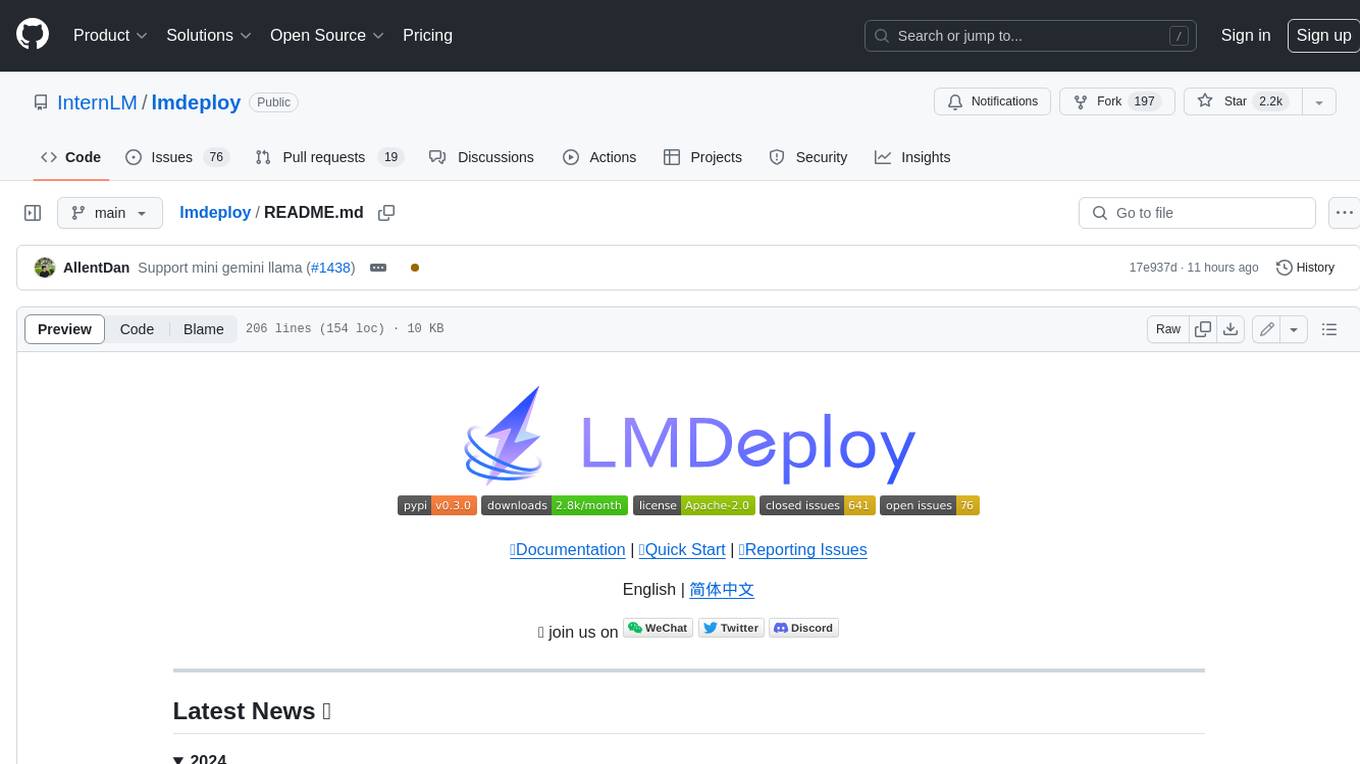
lmdeploy
LMDeploy is a toolkit for compressing, deploying, and serving LLM, developed by the MMRazor and MMDeploy teams. It has the following core features: * **Efficient Inference** : LMDeploy delivers up to 1.8x higher request throughput than vLLM, by introducing key features like persistent batch(a.k.a. continuous batching), blocked KV cache, dynamic split&fuse, tensor parallelism, high-performance CUDA kernels and so on. * **Effective Quantization** : LMDeploy supports weight-only and k/v quantization, and the 4-bit inference performance is 2.4x higher than FP16. The quantization quality has been confirmed via OpenCompass evaluation. * **Effortless Distribution Server** : Leveraging the request distribution service, LMDeploy facilitates an easy and efficient deployment of multi-model services across multiple machines and cards. * **Interactive Inference Mode** : By caching the k/v of attention during multi-round dialogue processes, the engine remembers dialogue history, thus avoiding repetitive processing of historical sessions.
For similar jobs
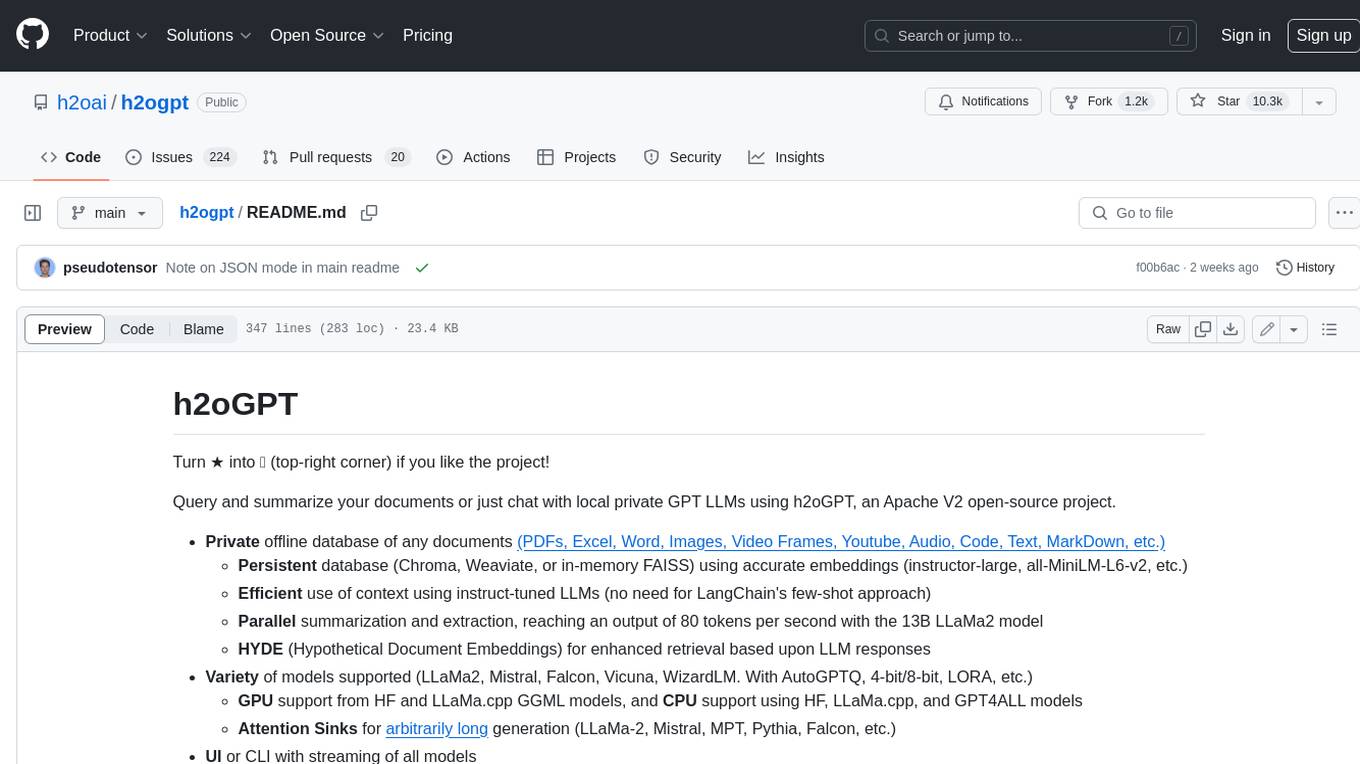
h2ogpt
h2oGPT is an Apache V2 open-source project that allows users to query and summarize documents or chat with local private GPT LLMs. It features a private offline database of any documents (PDFs, Excel, Word, Images, Video Frames, Youtube, Audio, Code, Text, MarkDown, etc.), a persistent database (Chroma, Weaviate, or in-memory FAISS) using accurate embeddings (instructor-large, all-MiniLM-L6-v2, etc.), and efficient use of context using instruct-tuned LLMs (no need for LangChain's few-shot approach). h2oGPT also offers parallel summarization and extraction, reaching an output of 80 tokens per second with the 13B LLaMa2 model, HYDE (Hypothetical Document Embeddings) for enhanced retrieval based upon LLM responses, a variety of models supported (LLaMa2, Mistral, Falcon, Vicuna, WizardLM. With AutoGPTQ, 4-bit/8-bit, LORA, etc.), GPU support from HF and LLaMa.cpp GGML models, and CPU support using HF, LLaMa.cpp, and GPT4ALL models. Additionally, h2oGPT provides Attention Sinks for arbitrarily long generation (LLaMa-2, Mistral, MPT, Pythia, Falcon, etc.), a UI or CLI with streaming of all models, the ability to upload and view documents through the UI (control multiple collaborative or personal collections), Vision Models LLaVa, Claude-3, Gemini-Pro-Vision, GPT-4-Vision, Image Generation Stable Diffusion (sdxl-turbo, sdxl) and PlaygroundAI (playv2), Voice STT using Whisper with streaming audio conversion, Voice TTS using MIT-Licensed Microsoft Speech T5 with multiple voices and Streaming audio conversion, Voice TTS using MPL2-Licensed TTS including Voice Cloning and Streaming audio conversion, AI Assistant Voice Control Mode for hands-free control of h2oGPT chat, Bake-off UI mode against many models at the same time, Easy Download of model artifacts and control over models like LLaMa.cpp through the UI, Authentication in the UI by user/password via Native or Google OAuth, State Preservation in the UI by user/password, Linux, Docker, macOS, and Windows support, Easy Windows Installer for Windows 10 64-bit (CPU/CUDA), Easy macOS Installer for macOS (CPU/M1/M2), Inference Servers support (oLLaMa, HF TGI server, vLLM, Gradio, ExLLaMa, Replicate, OpenAI, Azure OpenAI, Anthropic), OpenAI-compliant, Server Proxy API (h2oGPT acts as drop-in-replacement to OpenAI server), Python client API (to talk to Gradio server), JSON Mode with any model via code block extraction. Also supports MistralAI JSON mode, Claude-3 via function calling with strict Schema, OpenAI via JSON mode, and vLLM via guided_json with strict Schema, Web-Search integration with Chat and Document Q/A, Agents for Search, Document Q/A, Python Code, CSV frames (Experimental, best with OpenAI currently), Evaluate performance using reward models, and Quality maintained with over 1000 unit and integration tests taking over 4 GPU-hours.
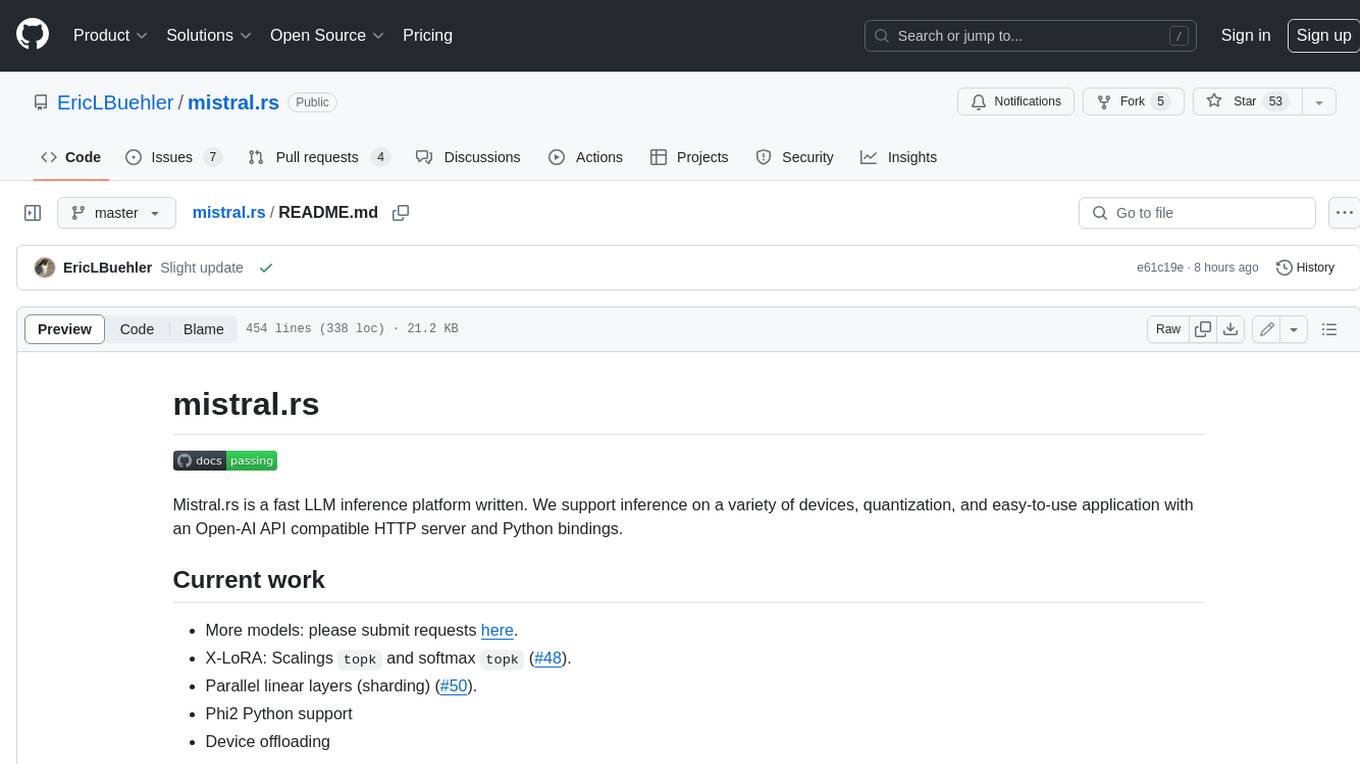
mistral.rs
Mistral.rs is a fast LLM inference platform written in Rust. We support inference on a variety of devices, quantization, and easy-to-use application with an Open-AI API compatible HTTP server and Python bindings.
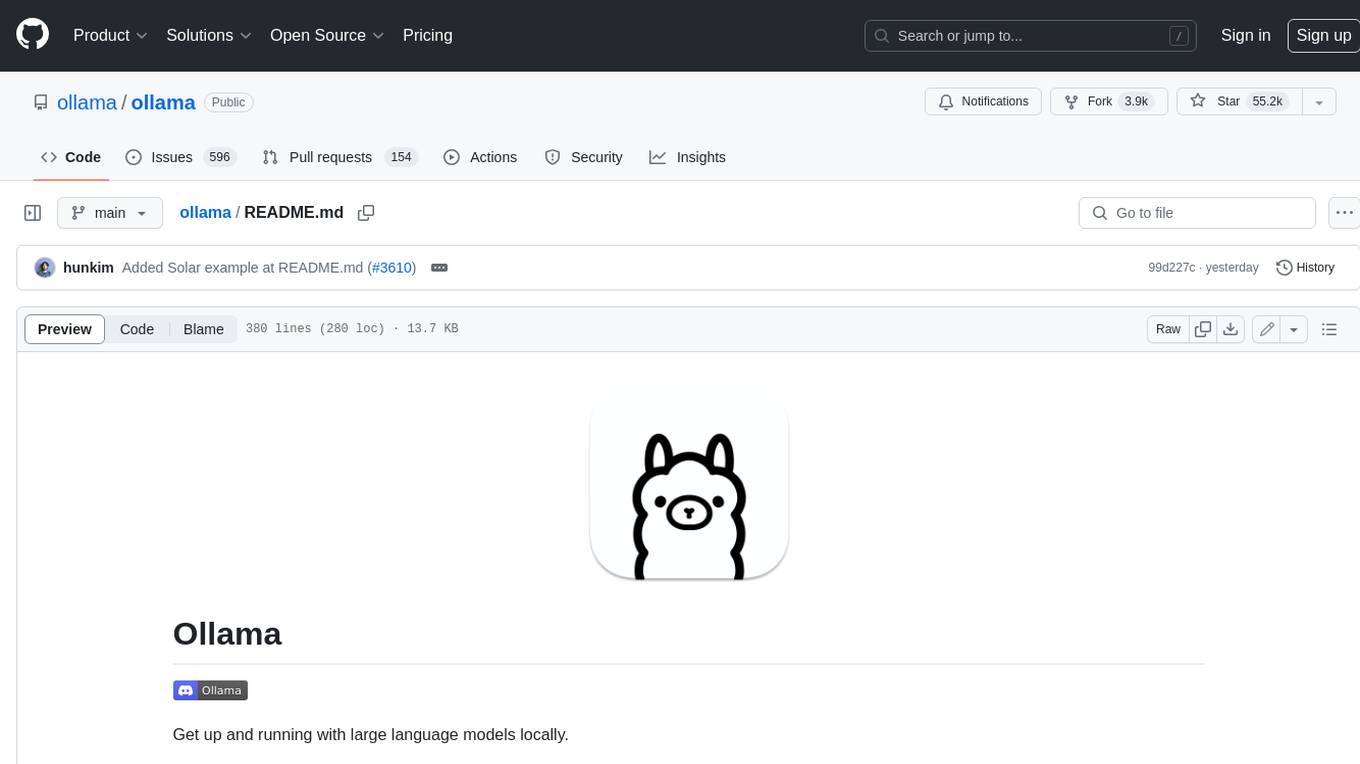
ollama
Ollama is a lightweight, extensible framework for building and running language models on the local machine. It provides a simple API for creating, running, and managing models, as well as a library of pre-built models that can be easily used in a variety of applications. Ollama is designed to be easy to use and accessible to developers of all levels. It is open source and available for free on GitHub.
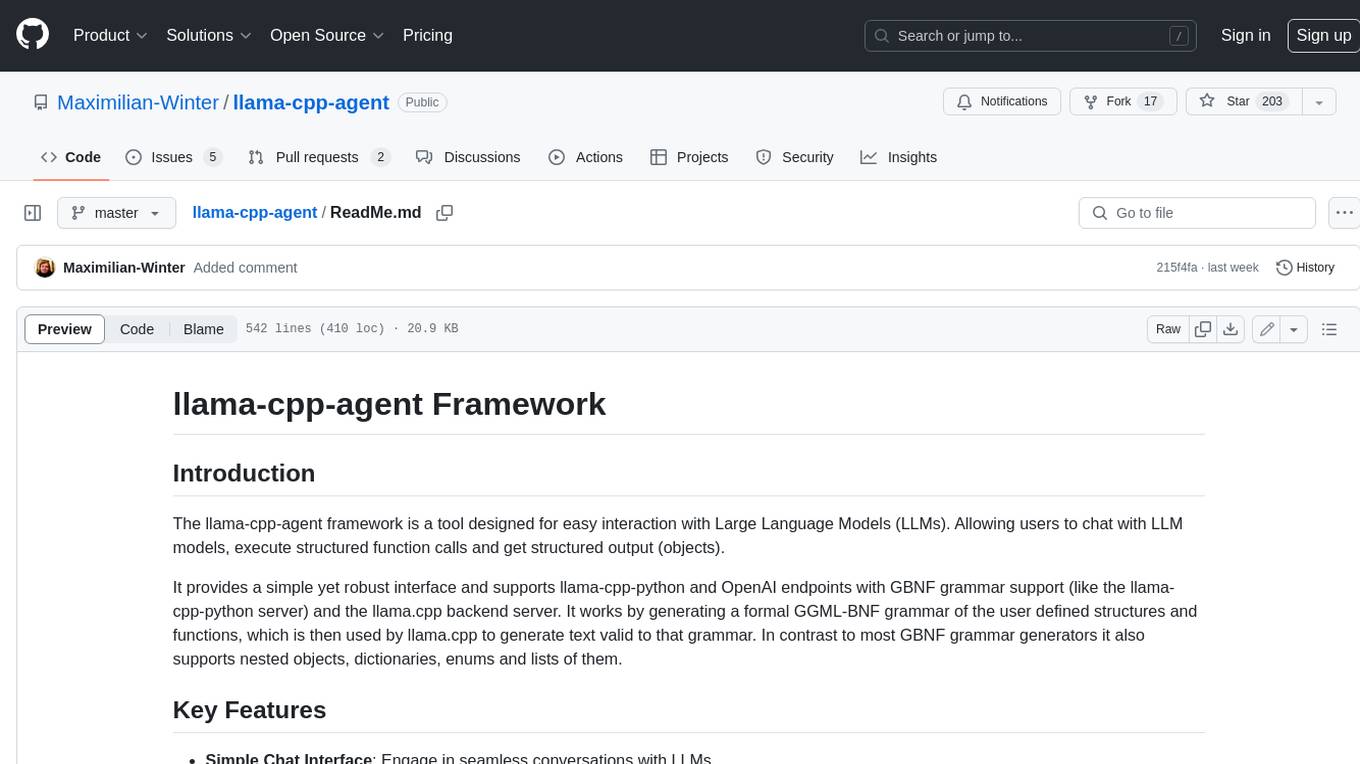
llama-cpp-agent
The llama-cpp-agent framework is a tool designed for easy interaction with Large Language Models (LLMs). Allowing users to chat with LLM models, execute structured function calls and get structured output (objects). It provides a simple yet robust interface and supports llama-cpp-python and OpenAI endpoints with GBNF grammar support (like the llama-cpp-python server) and the llama.cpp backend server. It works by generating a formal GGML-BNF grammar of the user defined structures and functions, which is then used by llama.cpp to generate text valid to that grammar. In contrast to most GBNF grammar generators it also supports nested objects, dictionaries, enums and lists of them.
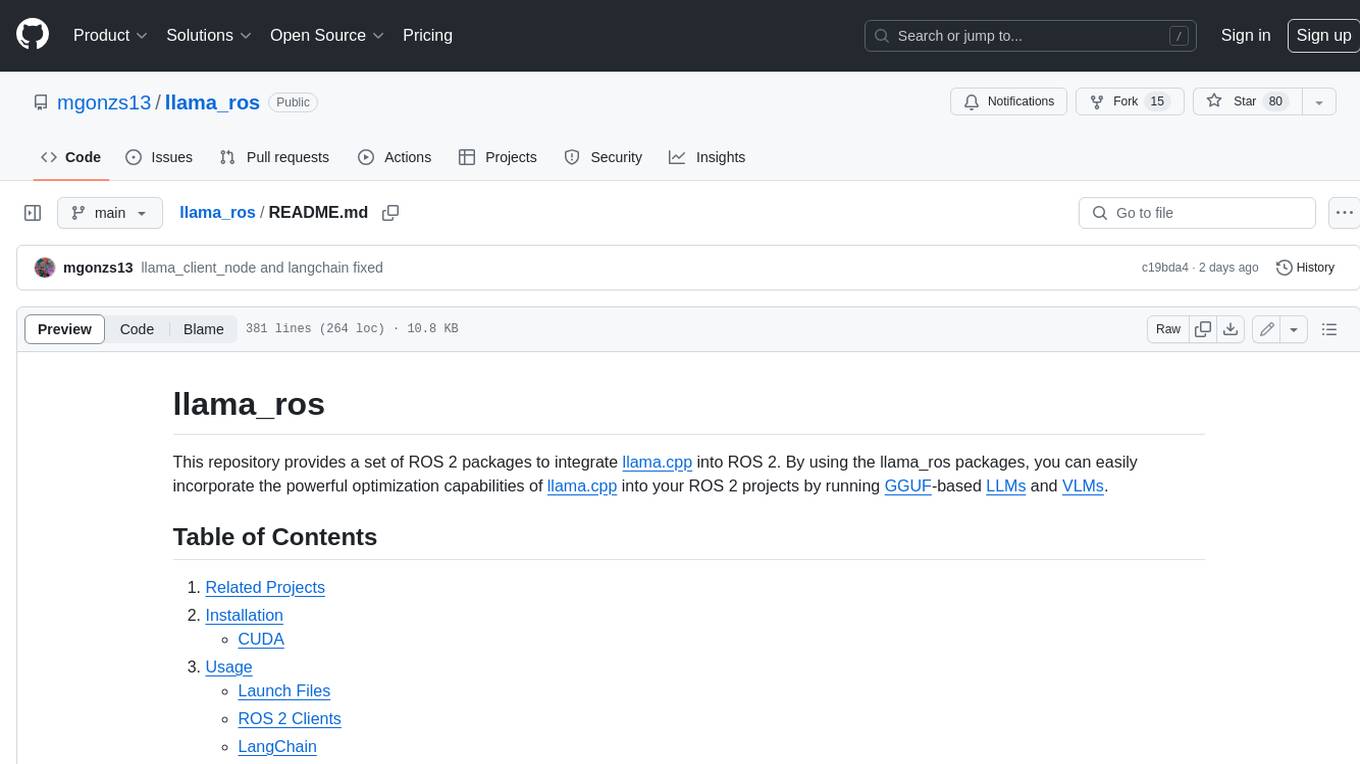
llama_ros
This repository provides a set of ROS 2 packages to integrate llama.cpp into ROS 2. By using the llama_ros packages, you can easily incorporate the powerful optimization capabilities of llama.cpp into your ROS 2 projects by running GGUF-based LLMs and VLMs.
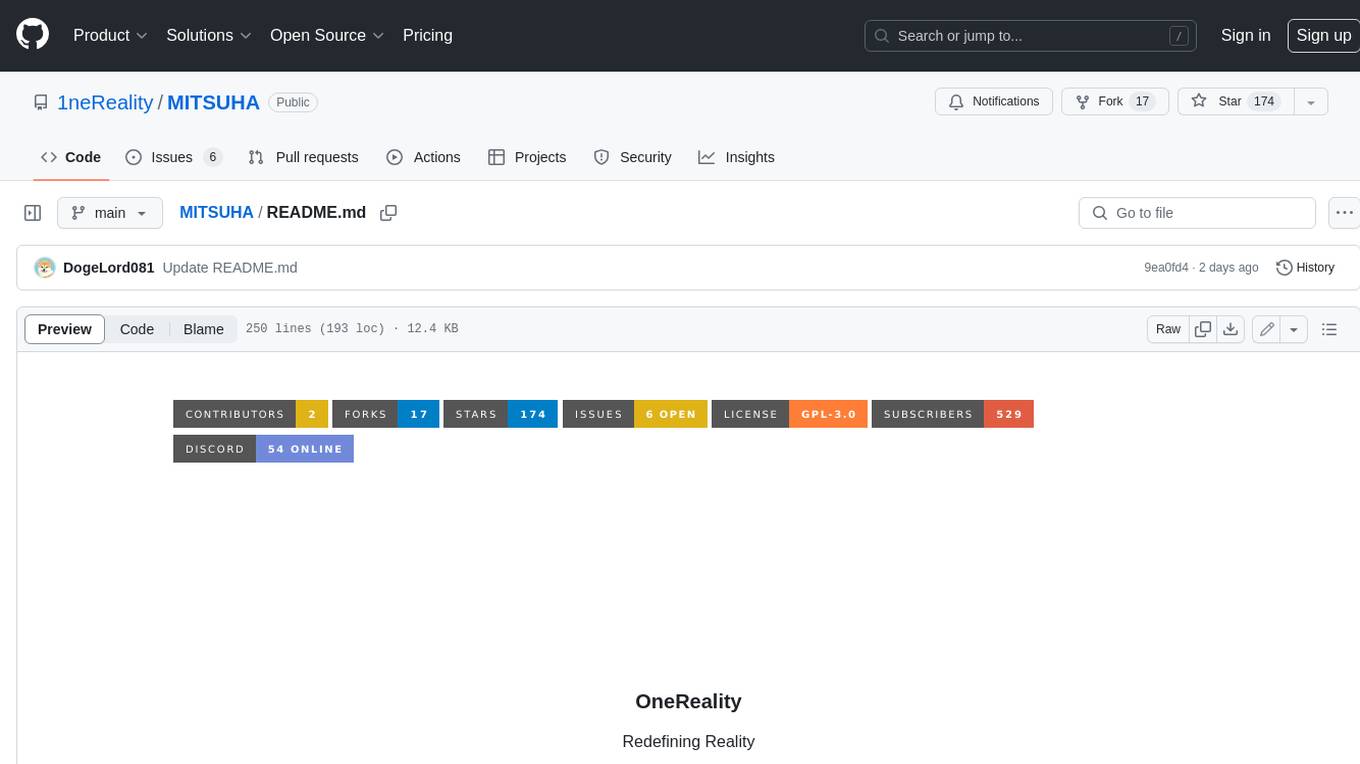
MITSUHA
OneReality is a virtual waifu/assistant that you can speak to through your mic and it'll speak back to you! It has many features such as: * You can speak to her with a mic * It can speak back to you * Has short-term memory and long-term memory * Can open apps * Smarter than you * Fluent in English, Japanese, Korean, and Chinese * Can control your smart home like Alexa if you set up Tuya (more info in Prerequisites) It is built with Python, Llama-cpp-python, Whisper, SpeechRecognition, PocketSphinx, VITS-fast-fine-tuning, VITS-simple-api, HyperDB, Sentence Transformers, and Tuya Cloud IoT.
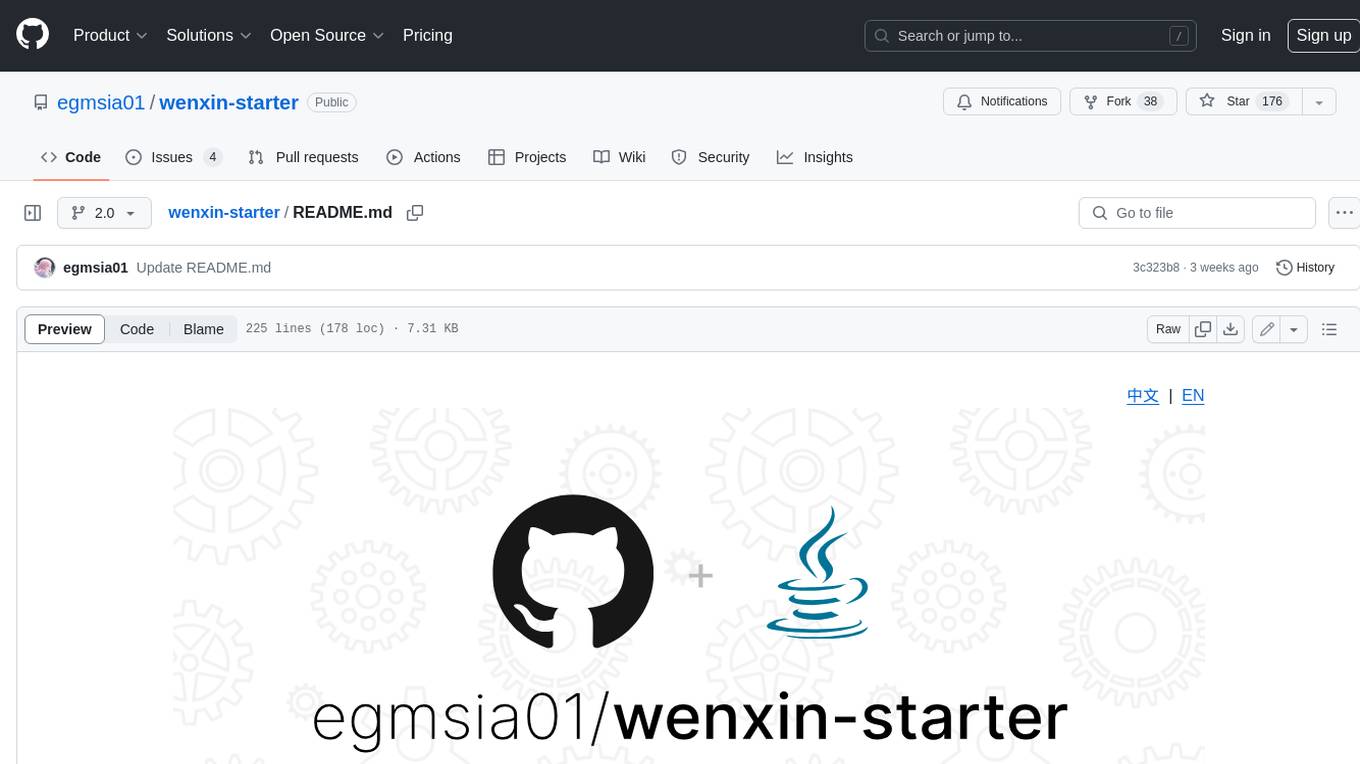
wenxin-starter
WenXin-Starter is a spring-boot-starter for Baidu's "Wenxin Qianfan WENXINWORKSHOP" large model, which can help you quickly access Baidu's AI capabilities. It fully integrates the official API documentation of Wenxin Qianfan. Supports text-to-image generation, built-in dialogue memory, and supports streaming return of dialogue. Supports QPS control of a single model and supports queuing mechanism. Plugins will be added soon.
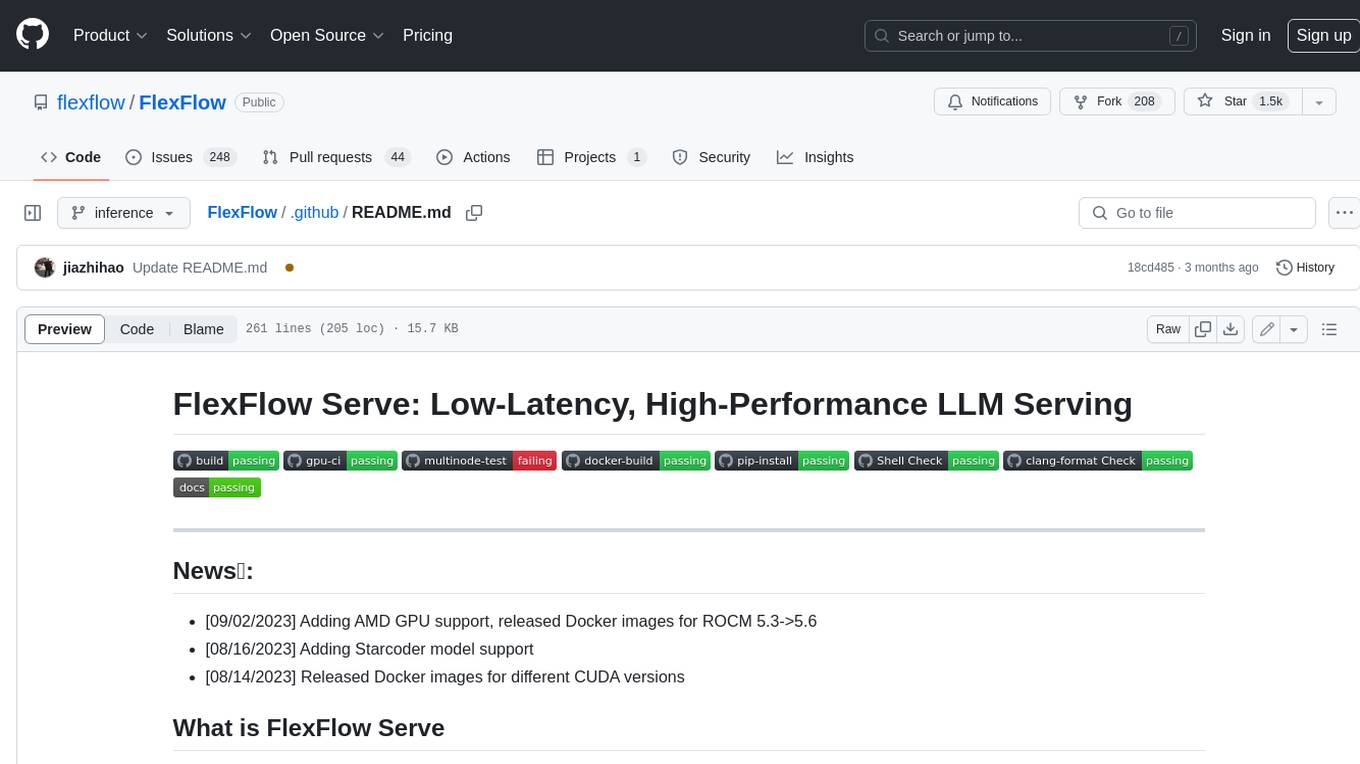
FlexFlow
FlexFlow Serve is an open-source compiler and distributed system for **low latency**, **high performance** LLM serving. FlexFlow Serve outperforms existing systems by 1.3-2.0x for single-node, multi-GPU inference and by 1.4-2.4x for multi-node, multi-GPU inference.