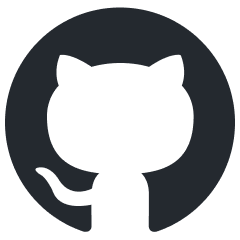
ai-accelerators
None
Stars: 82
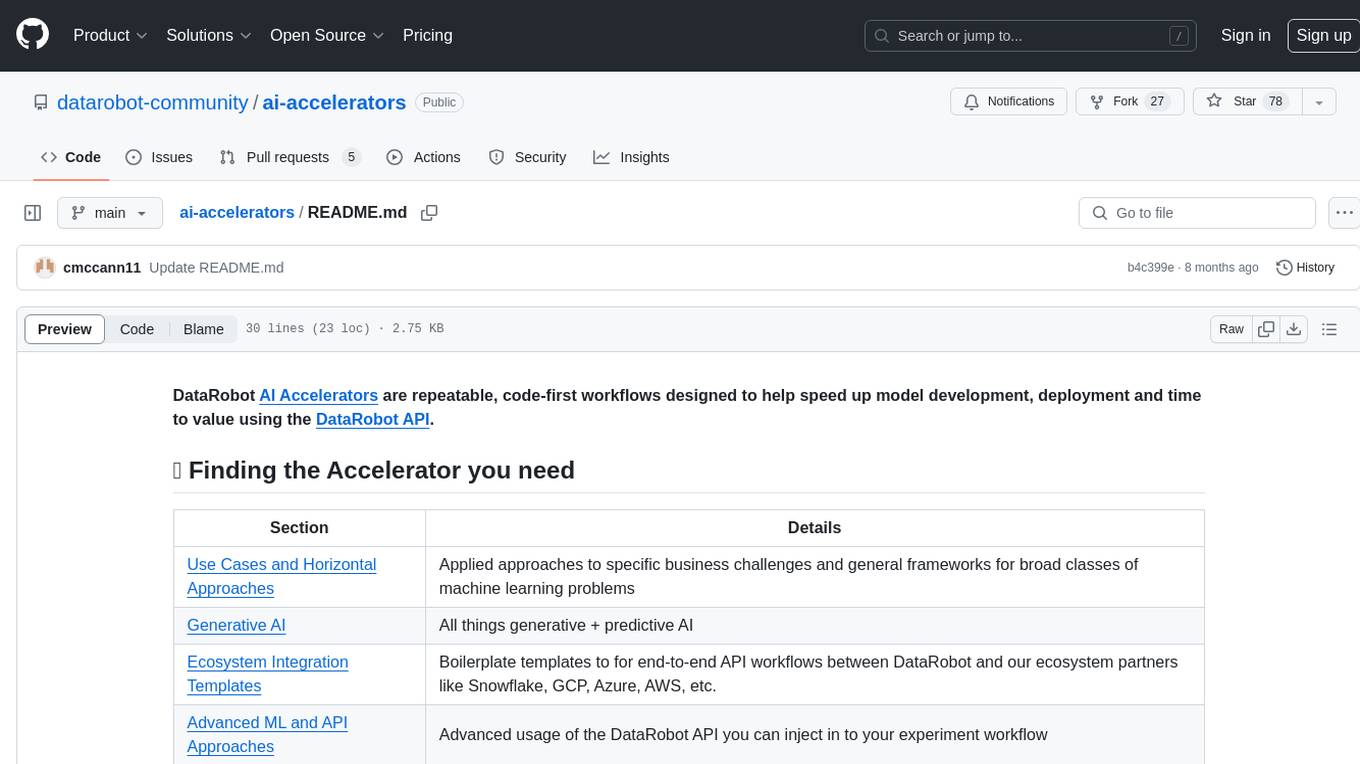
DataRobot AI Accelerators are code-first workflows to speed up model development, deployment, and time to value using the DataRobot API. The accelerators include approaches for specific business challenges, generative AI, ecosystem integration templates, and advanced ML and API usage. Users can clone the repo, import desired accelerators into notebooks, execute them, learn and modify content to solve their own problems.
README:
DataRobot AI Accelerators are repeatable, code-first workflows designed to help speed up model development, deployment and time to value using the DataRobot API.
Section | Details |
---|---|
Use Cases and Horizontal Approaches | Applied approaches to specific business challenges and general frameworks for broad classes of machine learning problems |
Generative AI | All things generative + predictive AI |
Ecosystem Integration Templates | Boilerplate templates to for end-to-end API workflows between DataRobot and our ecosystem partners like Snowflake, GCP, Azure, AWS, etc. |
Advanced ML and API Approaches | Advanced usage of the DataRobot API you can inject in to your experiment workflow |
Follow our channel @DataRobot. For more tutorials and demonstrations, checkout the Generative AI + DataRobot and DataRobot AI Accelerators playlists. More videos coming soon!
Install the DataRobot Python Client Package.
- Clone this repo
- Import the desired accelerator into your preferred notebook (e.g., jupyter, Kaggle, Databricks Notebooks, Google Colab). We recommend using DR-Notebooks.
- Execute the notebook.
- Learn and understand the accelerator content.
- You should now be able to modify the accelerator to solve your own problem. The easiest place to start is to replace the input data with your own.
Please report feedback and problems by opening a Github Issue in this repo. Please note: The code in these repos is sourced from the DataRobot user community and is not owned or maintained by DataRobot, Inc. You may need to make edits or updates for this code to function properly in your environment.
For Tasks:
Click tags to check more tools for each tasksFor Jobs:
Alternative AI tools for ai-accelerators
Similar Open Source Tools
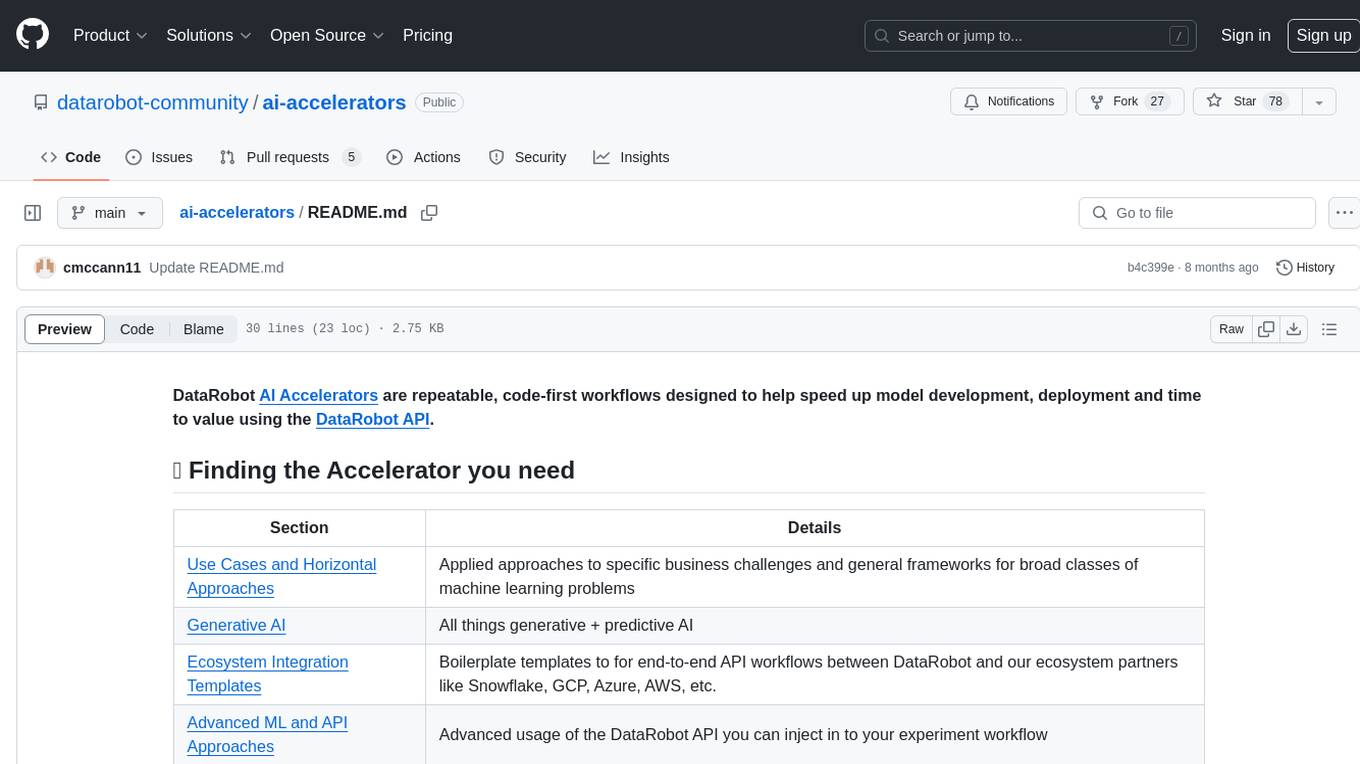
ai-accelerators
DataRobot AI Accelerators are code-first workflows to speed up model development, deployment, and time to value using the DataRobot API. The accelerators include approaches for specific business challenges, generative AI, ecosystem integration templates, and advanced ML and API usage. Users can clone the repo, import desired accelerators into notebooks, execute them, learn and modify content to solve their own problems.
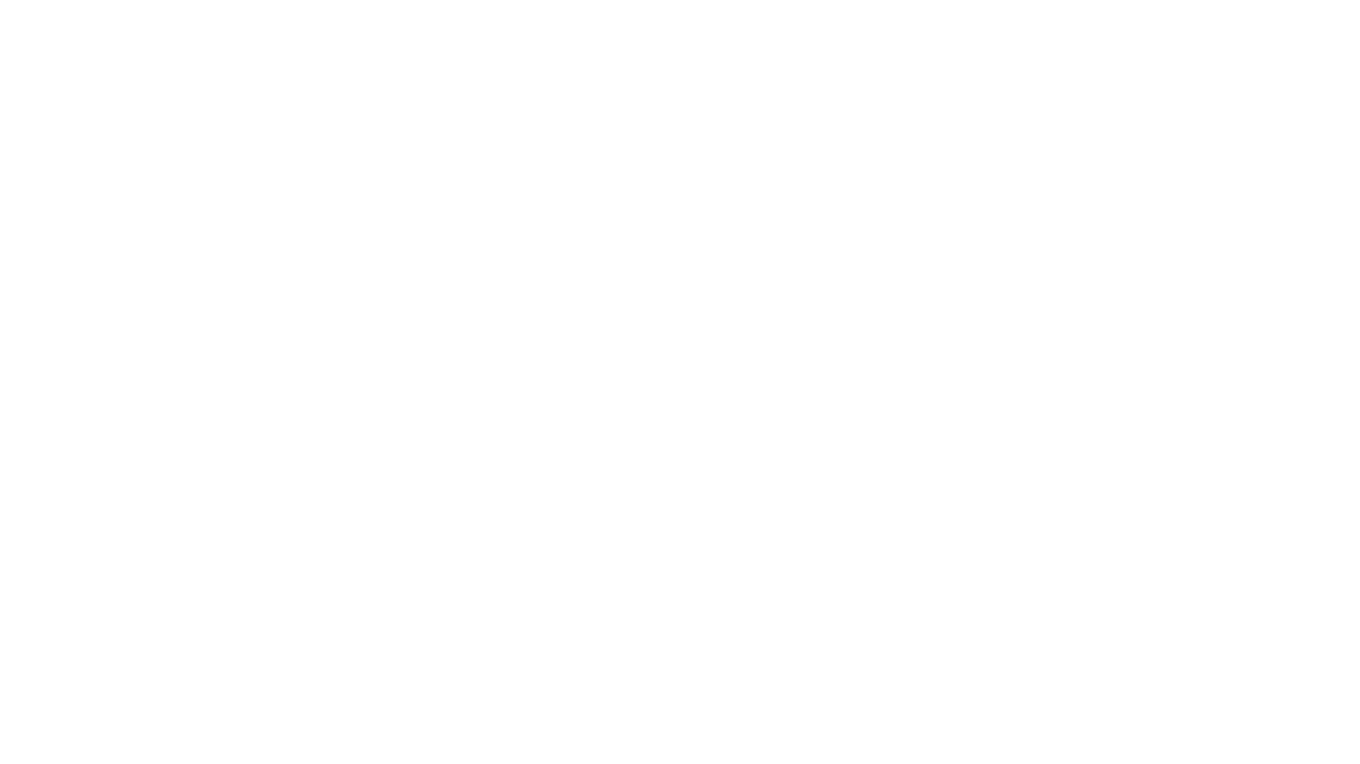
aitour-interact-with-llms
This repository is for the AI Tour workshop: Interacting with Multimodal models in Azure AI Foundry. The workshop provides a hands-on introduction to core concepts and best practices for interacting with OpenAI models in Azure AI Foundry portal. Participants can innovate with Azure OpenAI's GPT-4o multimodal model to generate text, sound, and images using GPT-4o-mini, DALL-E, and GPT-4o-realtime. The workshop also covers creating AI Agents to enhance user experiences and drive innovation. It includes instructions, resources for continued learning, and information on responsible AI practices.
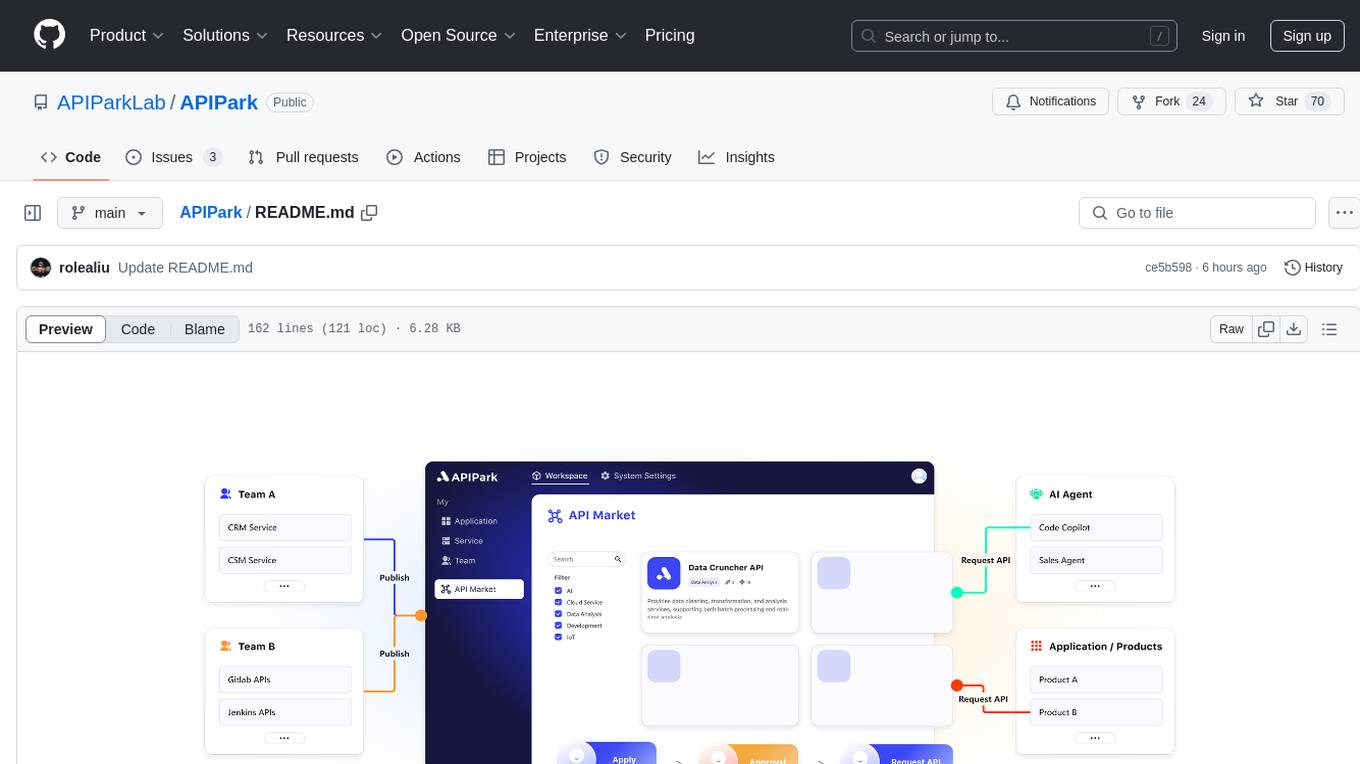
APIPark
APIPark is an open-source AI Gateway and Developer Portal that enables users to easily manage, integrate, and deploy AI and API services. It provides robust API management features, including creation, monitoring, and access control, to help developers efficiently and securely develop and manage their APIs. The platform aims to solve challenges such as connecting to powerful AI models, managing complex AI & API call relationships, overseeing API creation and security, simplifying fault detection and troubleshooting, and enhancing the visibility and valuation of data assets.
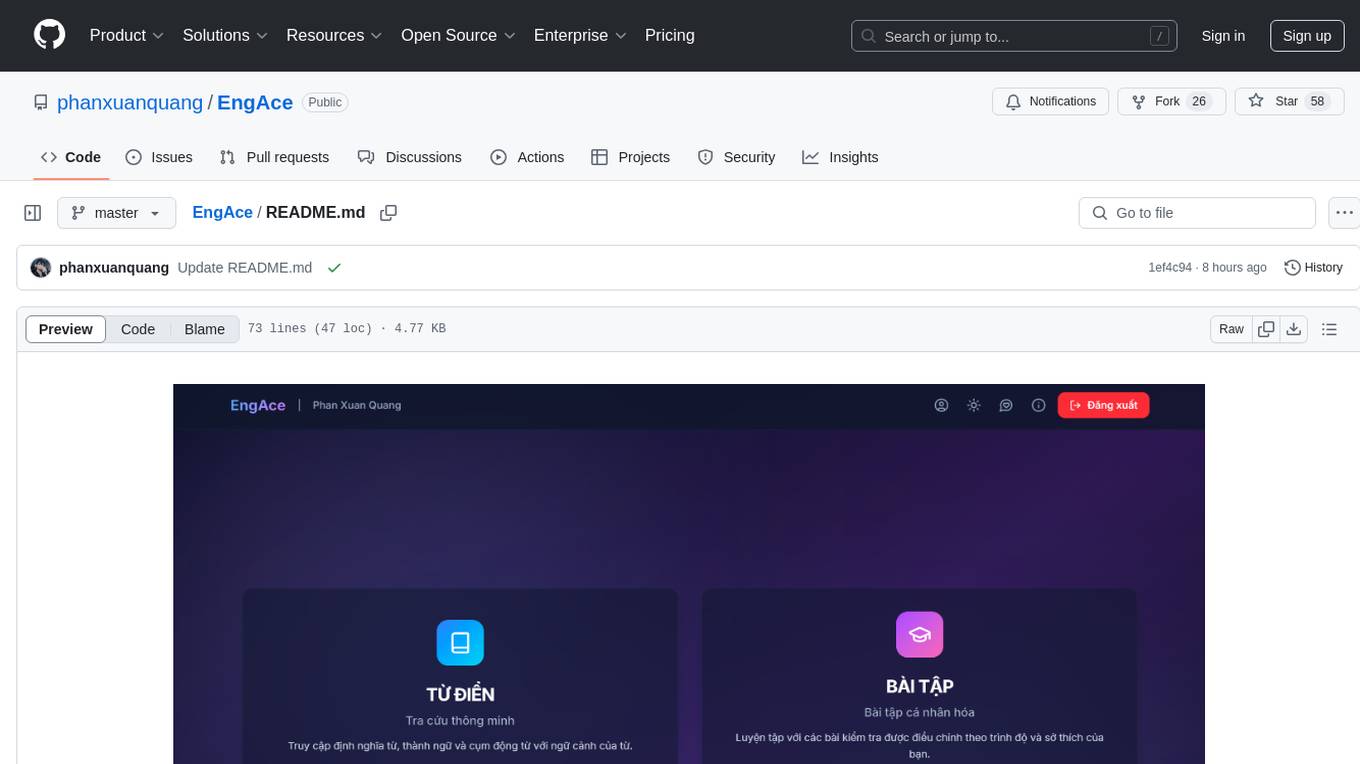
EngAce
EngAce is a cutting-edge, generative AI-powered application revolutionizing Vietnamese English learning. It offers personalized learning experiences combining AI with comprehensive features. The repository contains source code, documentation, and resources for the app.
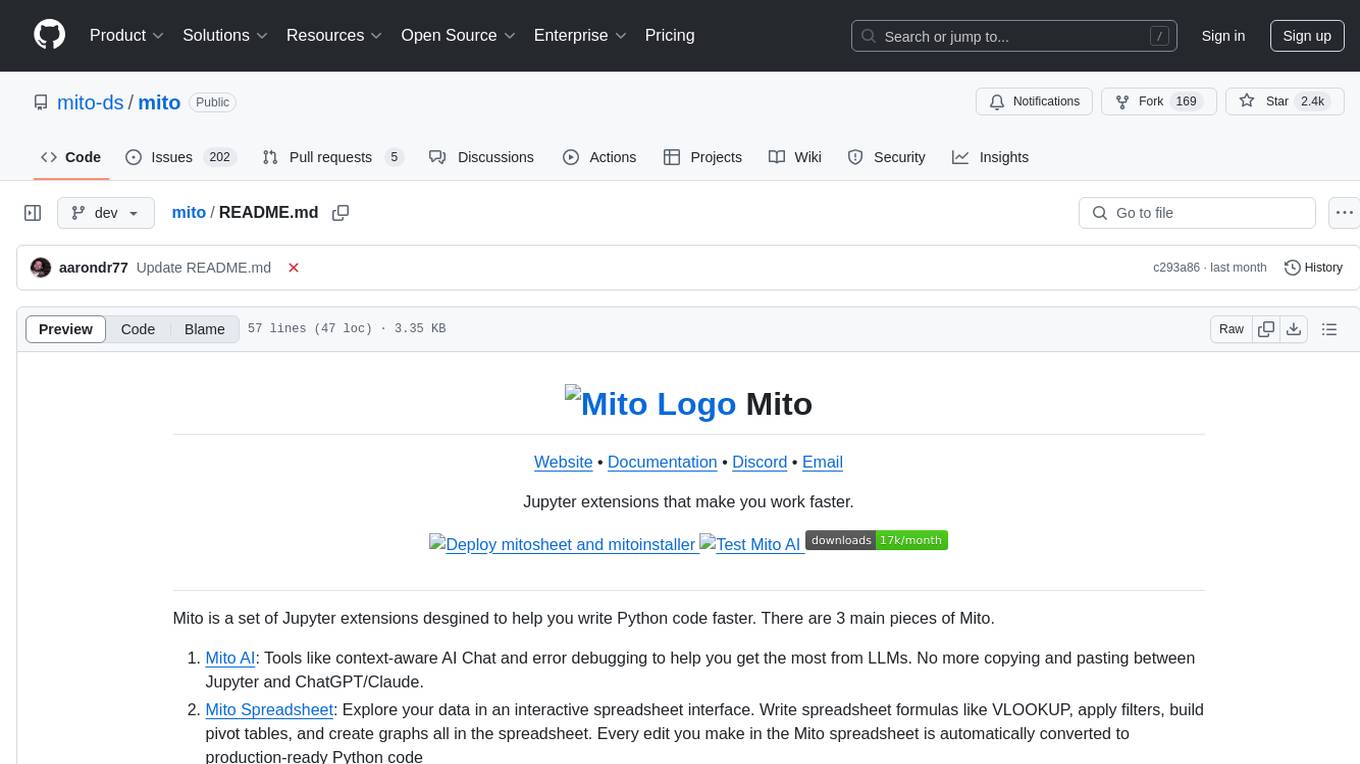
mito
Mito is a set of Jupyter extensions designed to help users write Python code faster. It consists of Mito AI, providing tools like context-aware AI Chat and error debugging; Mito Spreadsheet, enabling data exploration with interactive spreadsheet features; and Mito for Streamlit and Dash, allowing easy integration of spreadsheets into dashboards with minimal code. Mito is open source and community-driven, with options to purchase Mito Pro for further development support.
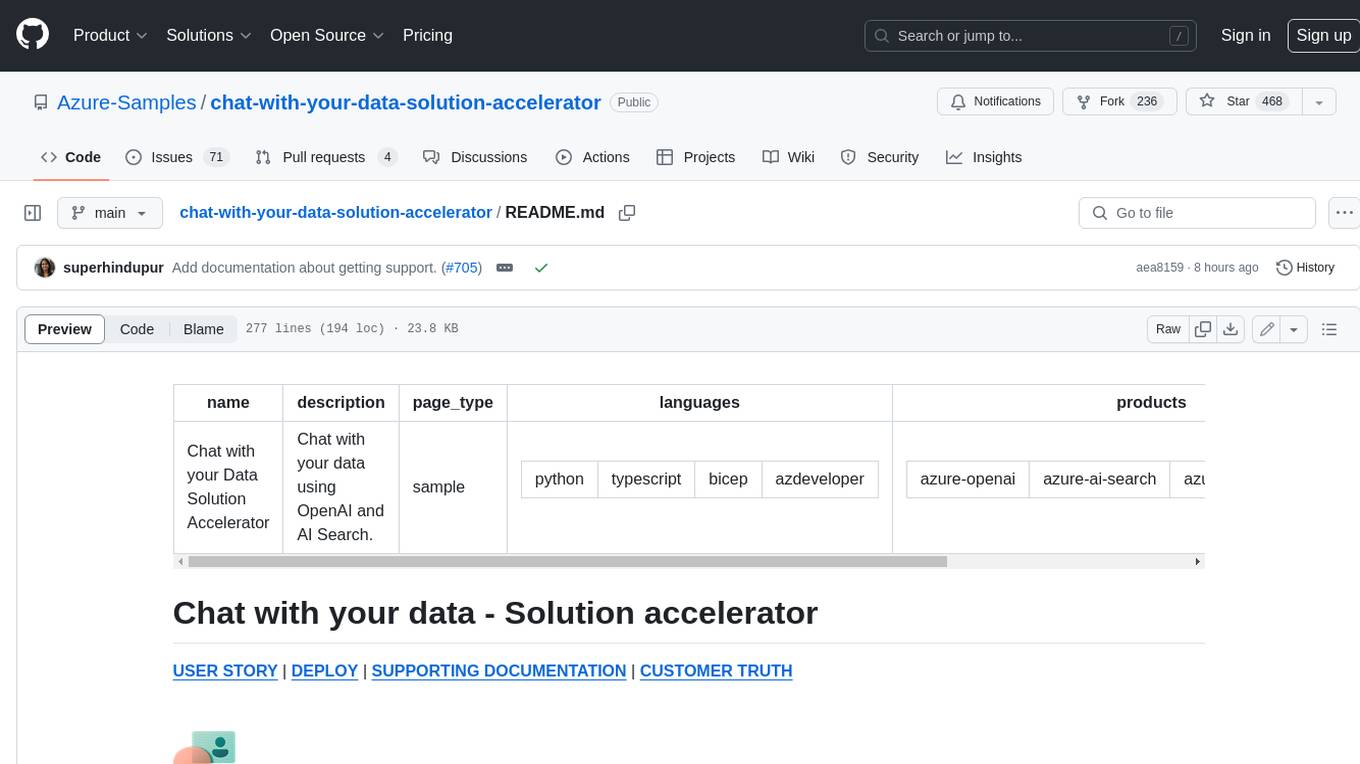
chat-with-your-data-solution-accelerator
Chat with your data using OpenAI and AI Search. This solution accelerator uses an Azure OpenAI GPT model and an Azure AI Search index generated from your data, which is integrated into a web application to provide a natural language interface, including speech-to-text functionality, for search queries. Users can drag and drop files, point to storage, and take care of technical setup to transform documents. There is a web app that users can create in their own subscription with security and authentication.
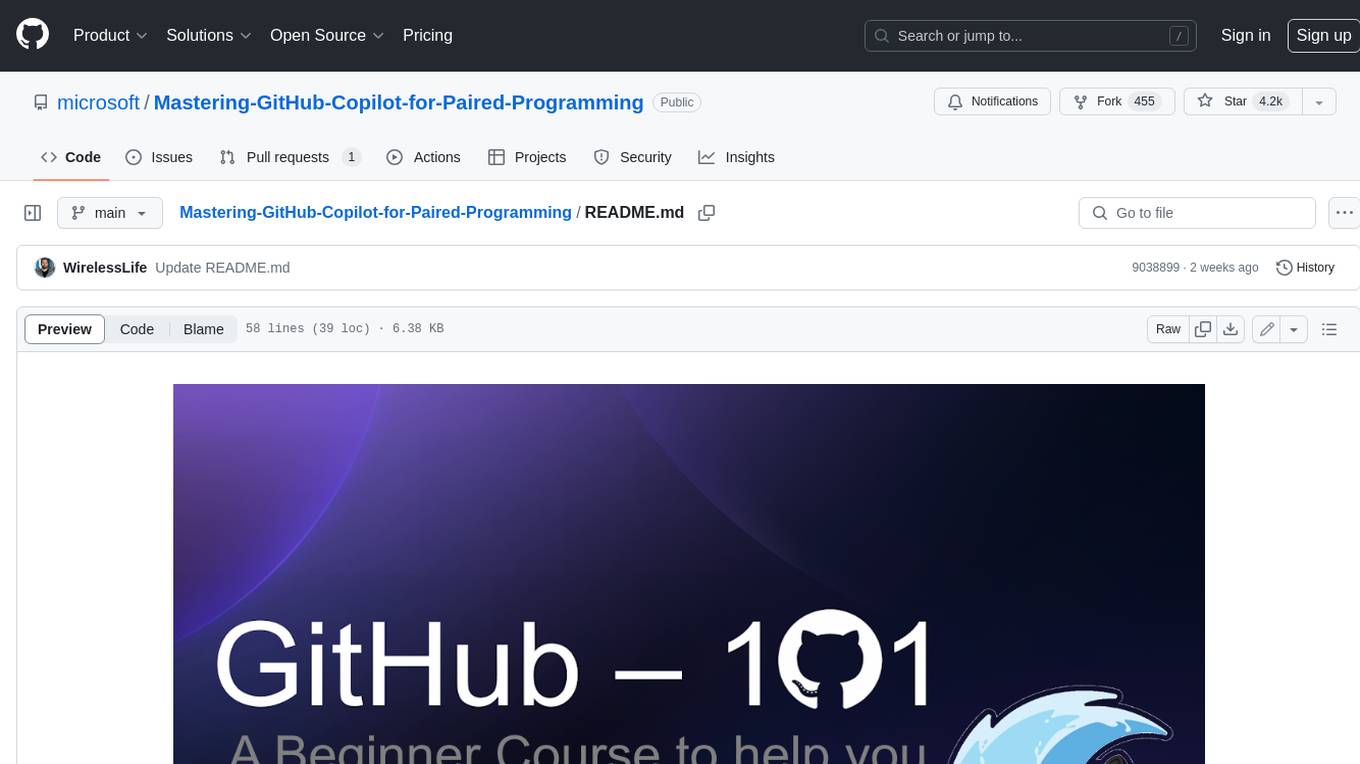
Mastering-GitHub-Copilot-for-Paired-Programming
Mastering GitHub Copilot for AI Paired Programming is a comprehensive course designed to equip you with the skills and knowledge necessary to harness the power of GitHub Copilot, an AI-driven coding assistant. Through a series of engaging lessons, you will learn how to seamlessly integrate GitHub Copilot into your workflow, leveraging its autocompletion, customizable features, and advanced programming techniques. This course is tailored to provide you with a deep understanding of AI-driven algorithms and best practices, enabling you to enhance code quality and accelerate your coding skills. By embracing the transformative power of AI paired programming, you will gain the tools and confidence needed to succeed in today's dynamic software development landscape.
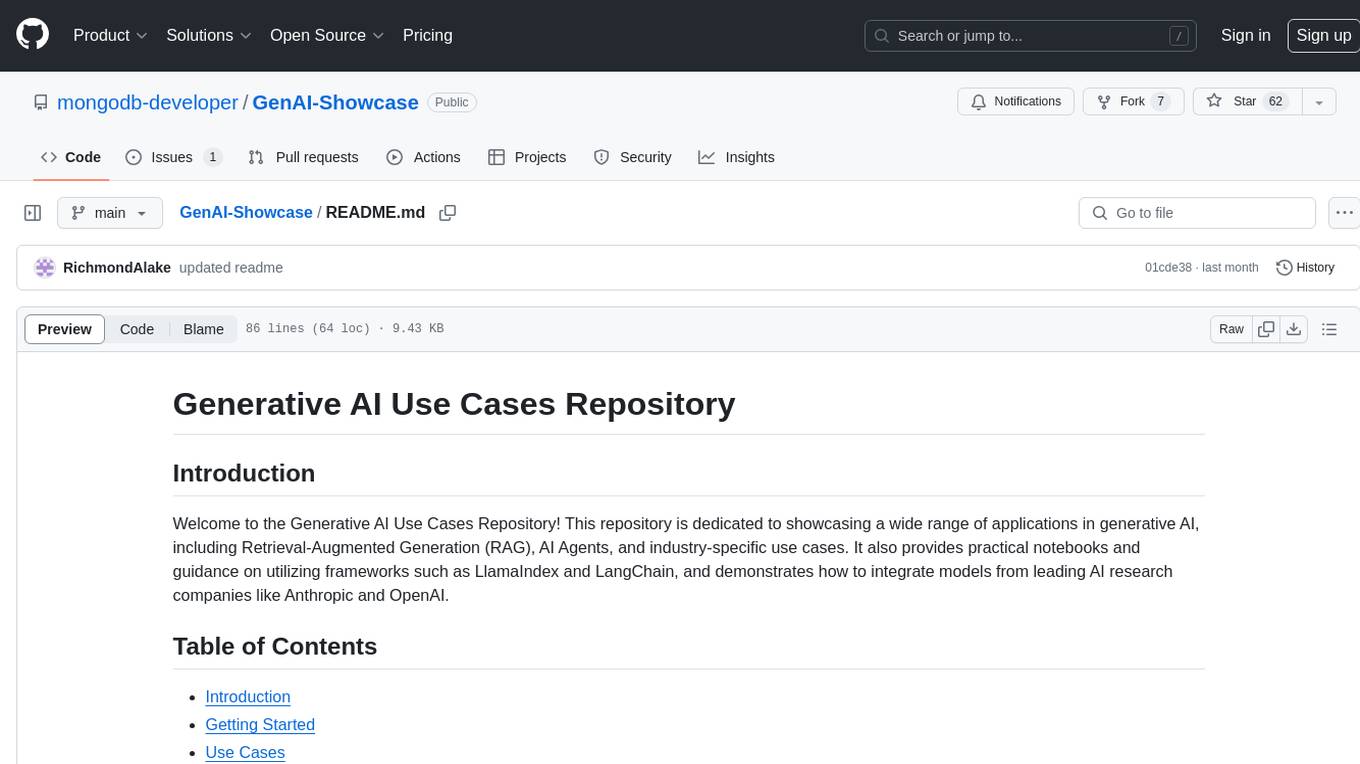
GenAI-Showcase
The Generative AI Use Cases Repository showcases a wide range of applications in generative AI, including Retrieval-Augmented Generation (RAG), AI Agents, and industry-specific use cases. It provides practical notebooks and guidance on utilizing frameworks such as LlamaIndex and LangChain, and demonstrates how to integrate models from leading AI research companies like Anthropic and OpenAI.
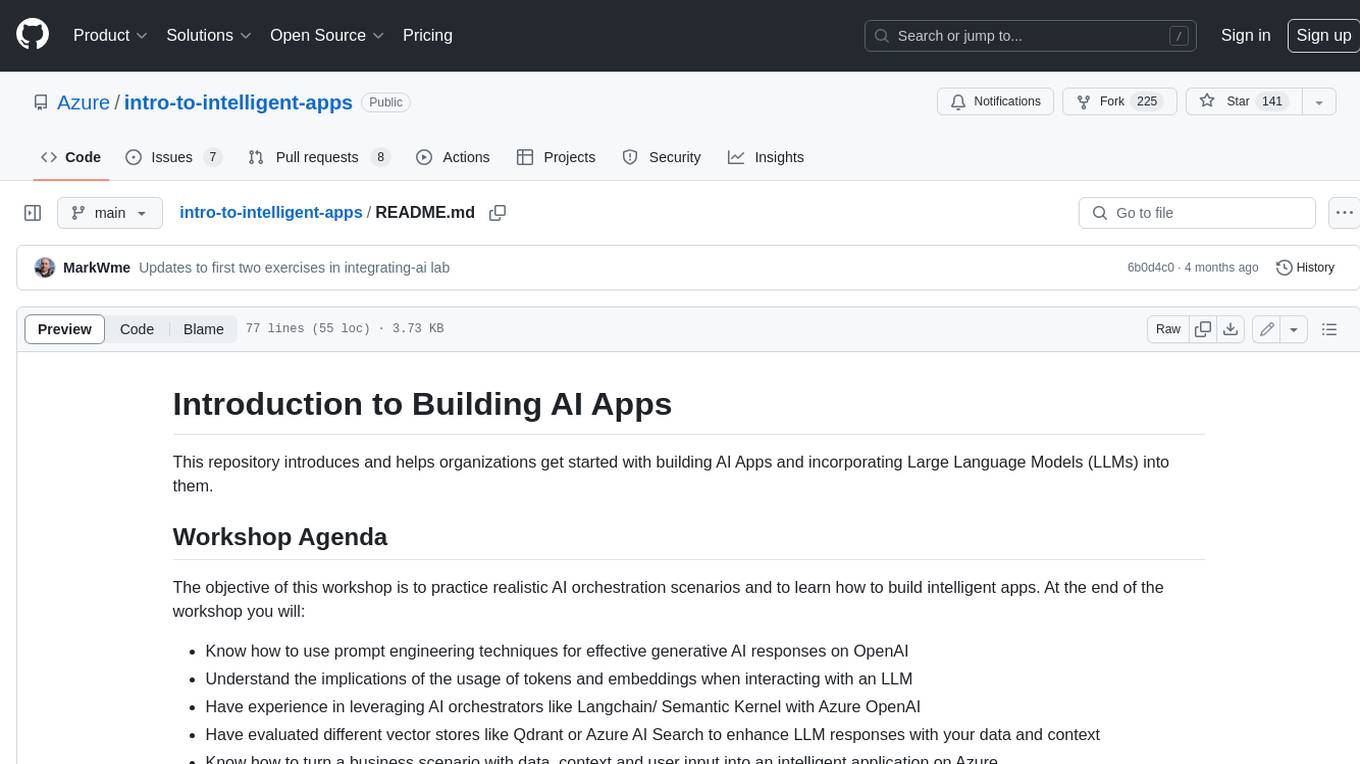
intro-to-intelligent-apps
This repository introduces and helps organizations get started with building AI Apps and incorporating Large Language Models (LLMs) into them. The workshop covers topics such as prompt engineering, AI orchestration, and deploying AI apps. Participants will learn how to use Azure OpenAI, Langchain/ Semantic Kernel, Qdrant, and Azure AI Search to build intelligent applications.
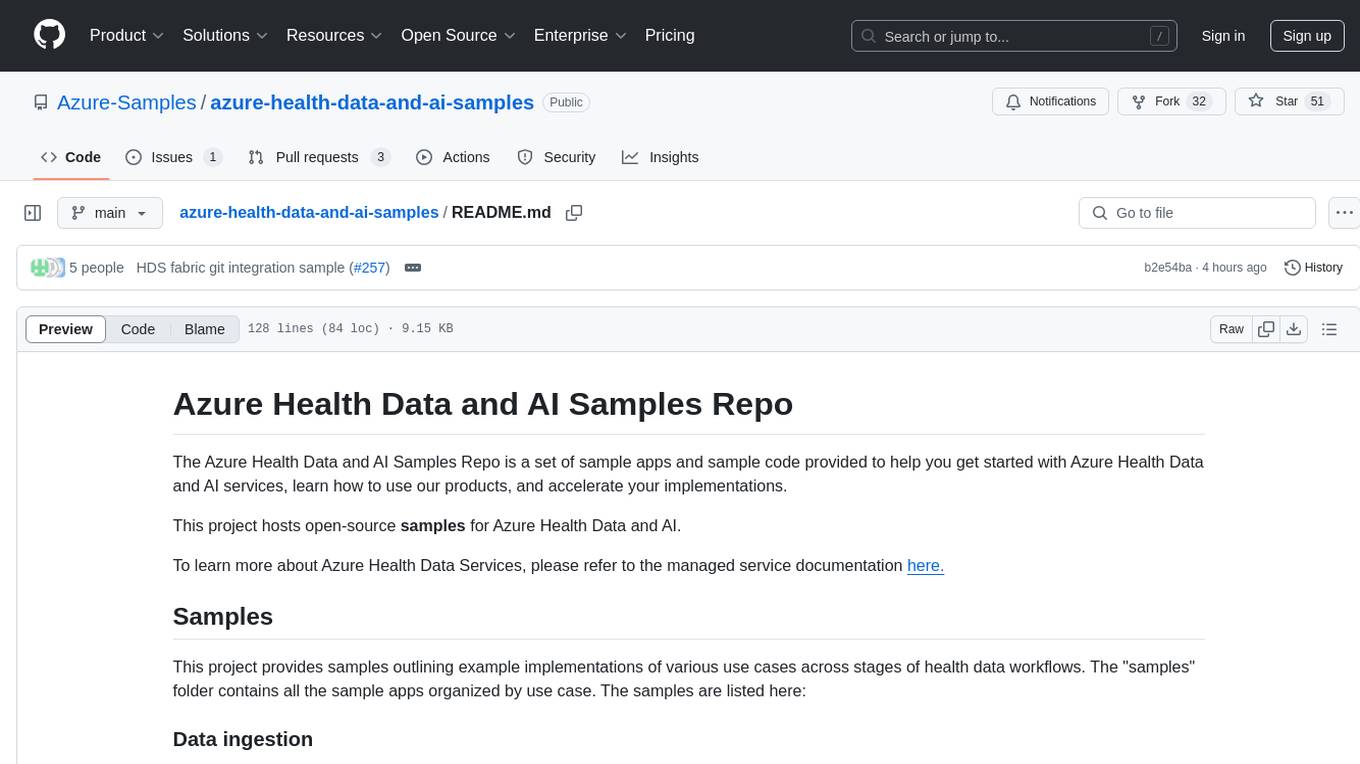
azure-health-data-and-ai-samples
The Azure Health Data and AI Samples Repo is a collection of sample apps and code to help users start with Azure Health Data and AI services, learn product usage, and speed up implementations. It includes samples for various health data workflows, such as data ingestion, analytics, machine learning, SMART on FHIR, patient services, FHIR service integration, Azure AD B2C access, DICOM service, MedTech service, and healthcare data solutions in Microsoft Fabric. These samples are simplified scenarios for testing purposes only.
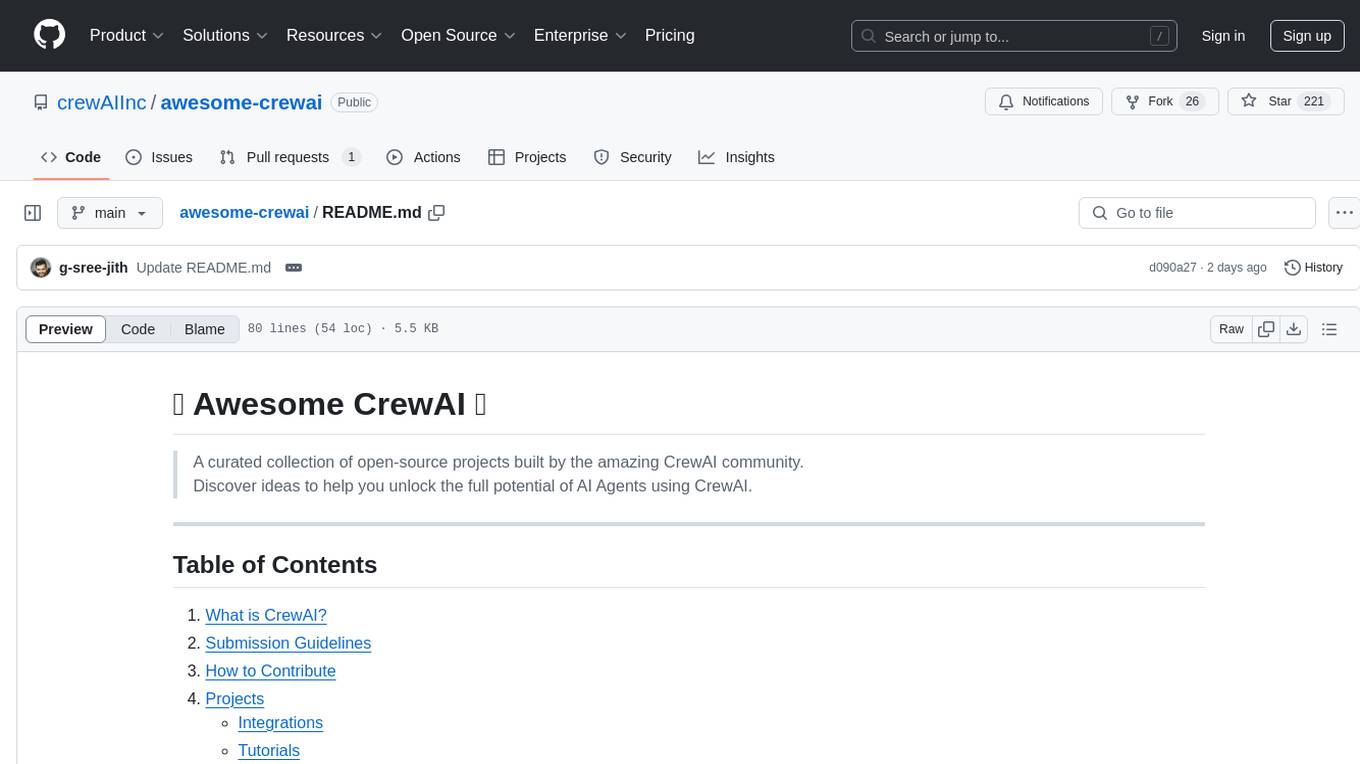
awesome-crewai
Awesome CrewAI is a curated collection of open-source projects built by the CrewAI community, aimed at unlocking the full potential of AI agents for supercharging business processes and decision-making. It includes integrations, tutorials, and tools that showcase the capabilities of CrewAI in various domains.
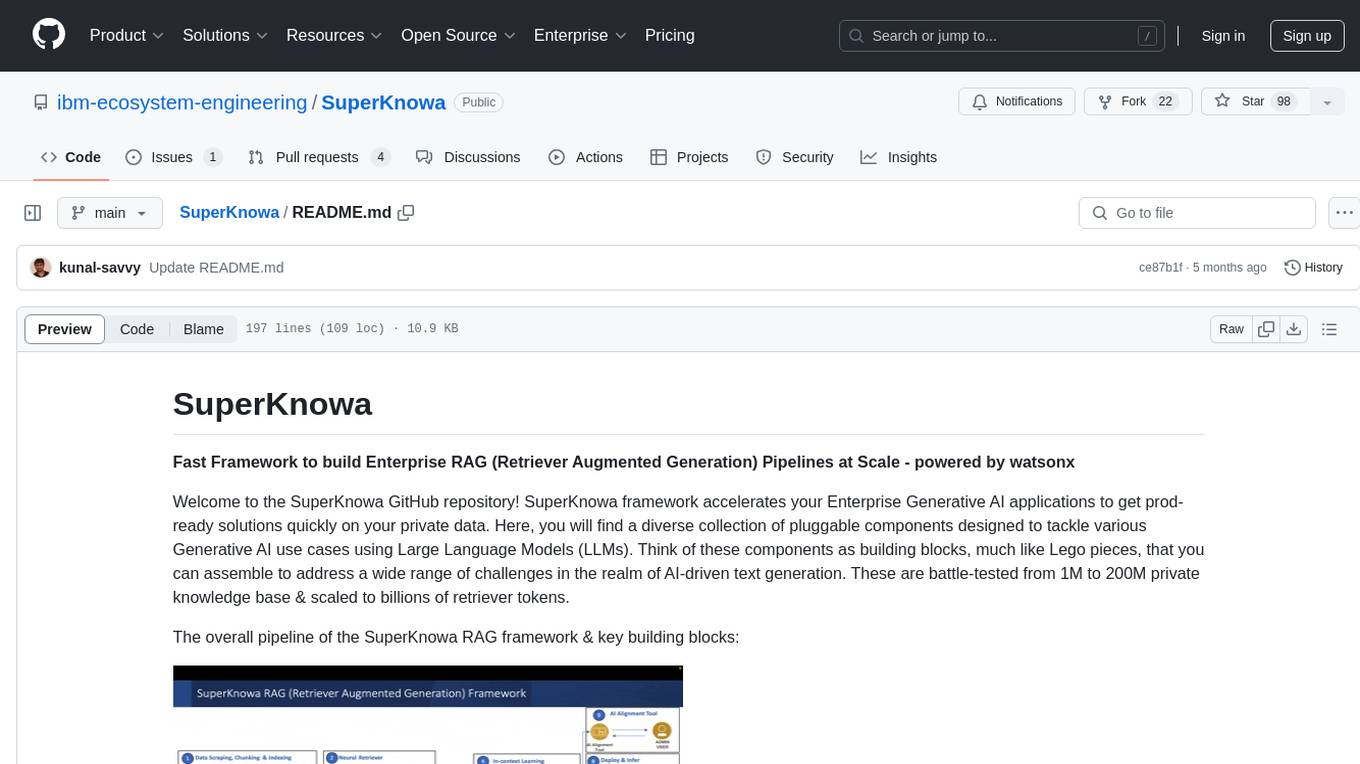
SuperKnowa
SuperKnowa is a fast framework to build Enterprise RAG (Retriever Augmented Generation) Pipelines at Scale, powered by watsonx. It accelerates Enterprise Generative AI applications to get prod-ready solutions quickly on private data. The framework provides pluggable components for tackling various Generative AI use cases using Large Language Models (LLMs), allowing users to assemble building blocks to address challenges in AI-driven text generation. SuperKnowa is battle-tested from 1M to 200M private knowledge base & scaled to billions of retriever tokens.
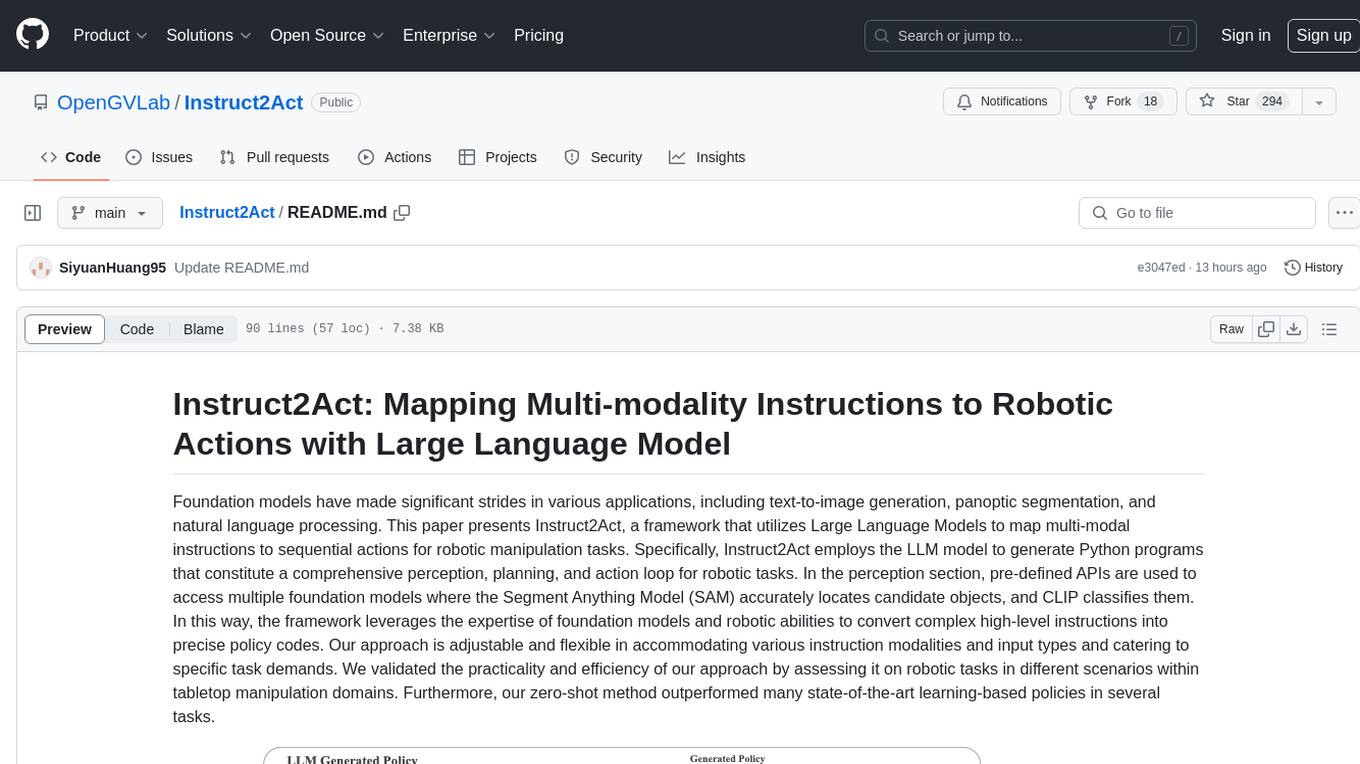
Instruct2Act
Instruct2Act is a framework that utilizes Large Language Models to map multi-modal instructions to sequential actions for robotic manipulation tasks. It generates Python programs using the LLM model for perception, planning, and action. The framework leverages foundation models like SAM and CLIP to convert high-level instructions into policy codes, accommodating various instruction modalities and task demands. Instruct2Act has been validated on robotic tasks in tabletop manipulation domains, outperforming learning-based policies in several tasks.
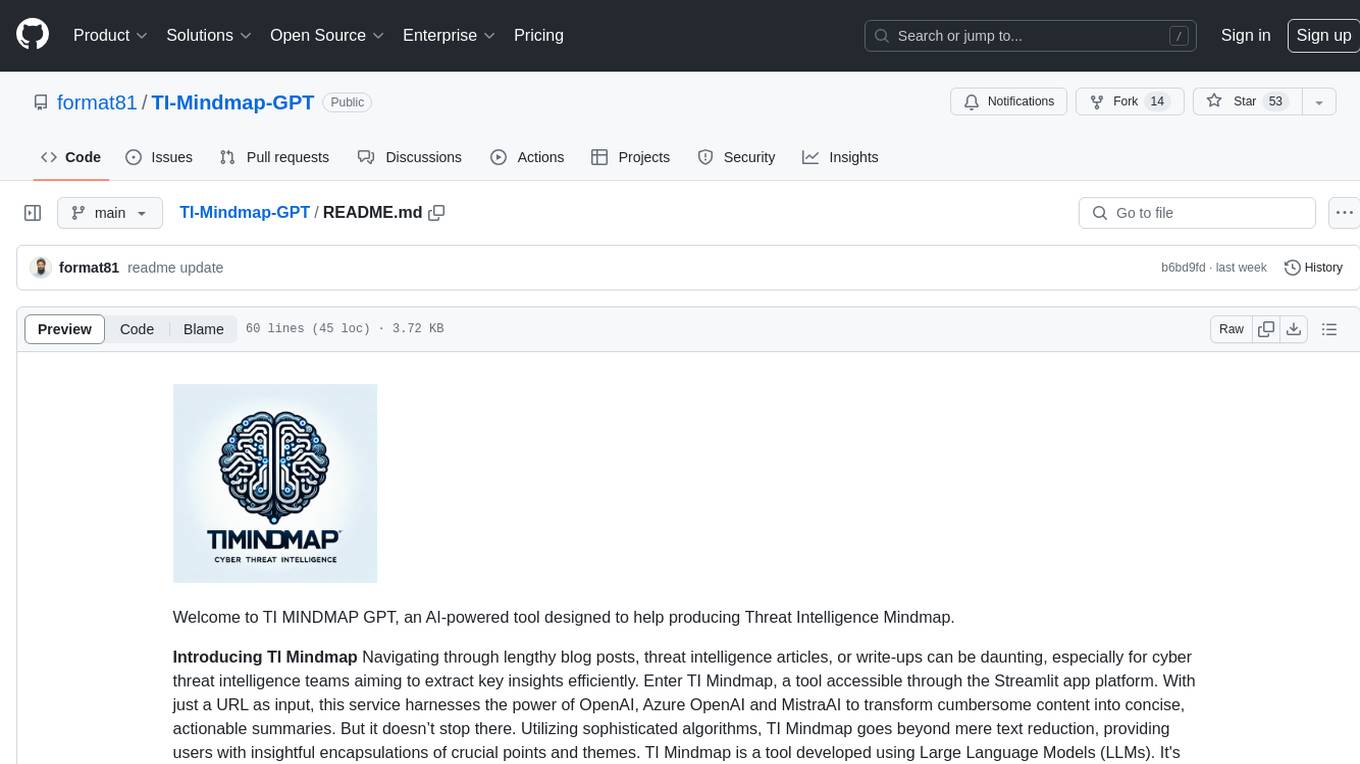
TI-Mindmap-GPT
TI MINDMAP GPT is an AI-powered tool designed to assist cyber threat intelligence teams in quickly synthesizing and visualizing key information from various Threat Intelligence sources. The tool utilizes Large Language Models (LLMs) to transform lengthy content into concise, actionable summaries, going beyond mere text reduction to provide insightful encapsulations of crucial points and themes. Users can leverage their own LLM keys for personalized and efficient information processing, streamlining data analysis and enabling teams to focus on strategic decision-making.
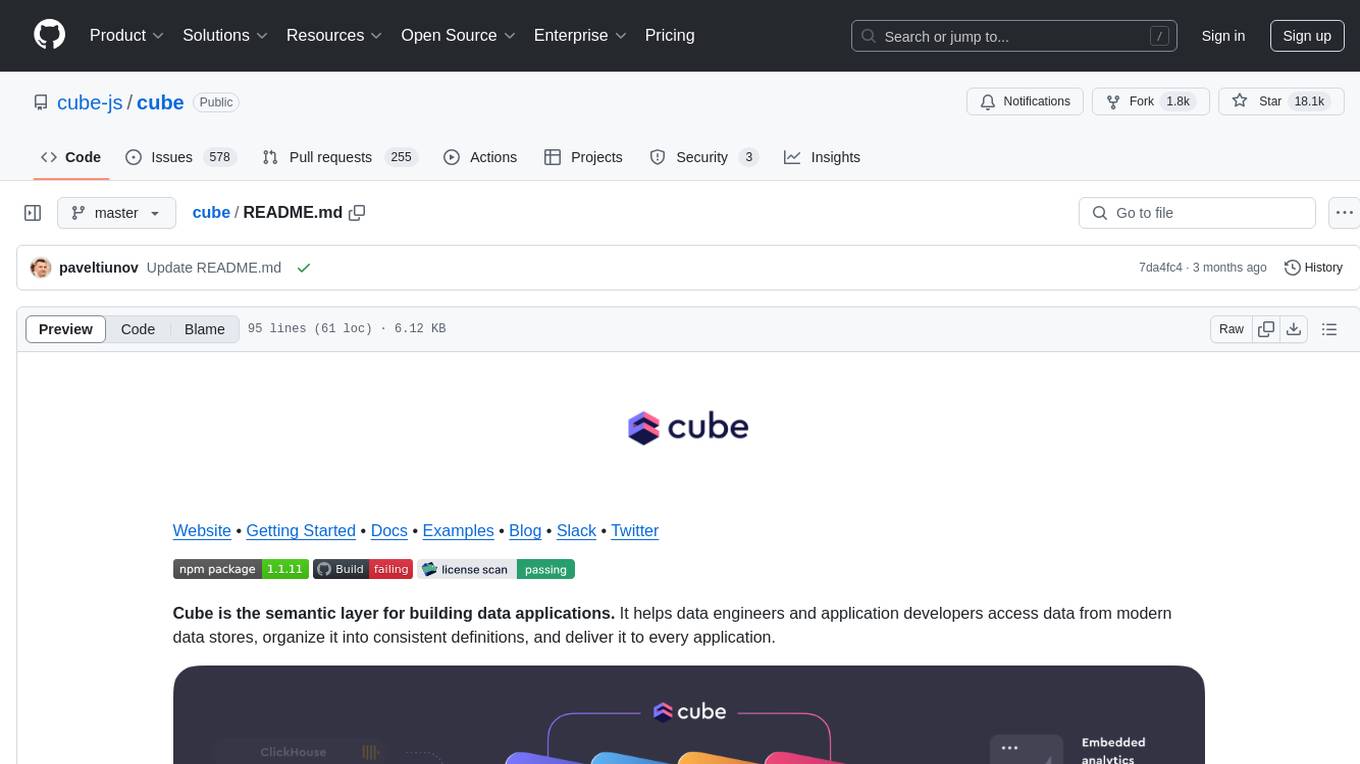
cube
Cube is a semantic layer for building data applications, helping data engineers and application developers access data from modern data stores, organize it into consistent definitions, and deliver it to every application. It works with SQL-enabled data sources, providing sub-second latency and high concurrency for API requests. Cube addresses SQL code organization, performance, and access control issues in data applications, enabling efficient data modeling, access control, and performance optimizations for various tools like embedded analytics, dashboarding, reporting, and data notebooks.
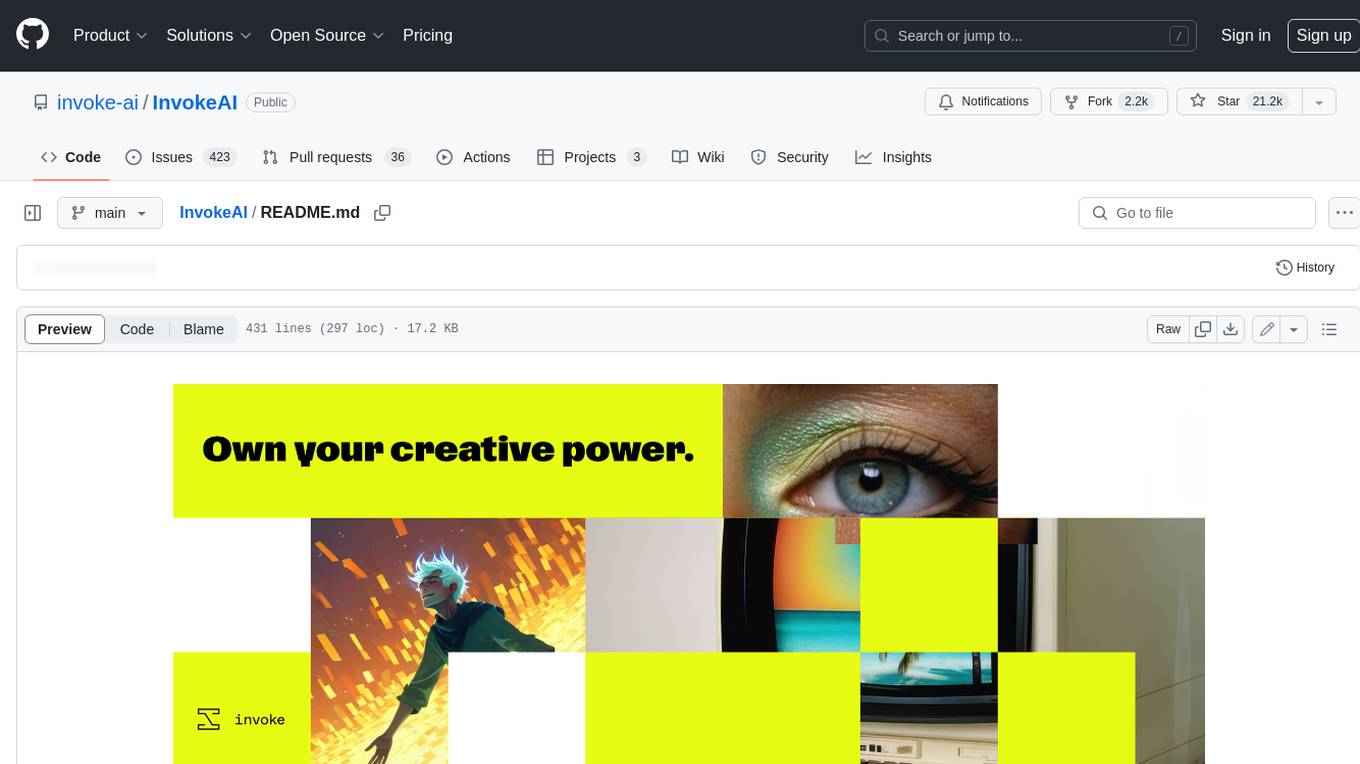
InvokeAI
InvokeAI is a leading creative engine built to empower professionals and enthusiasts alike. Generate and create stunning visual media using the latest AI-driven technologies. InvokeAI offers an industry leading Web Interface, interactive Command Line Interface, and also serves as the foundation for multiple commercial products.
For similar tasks
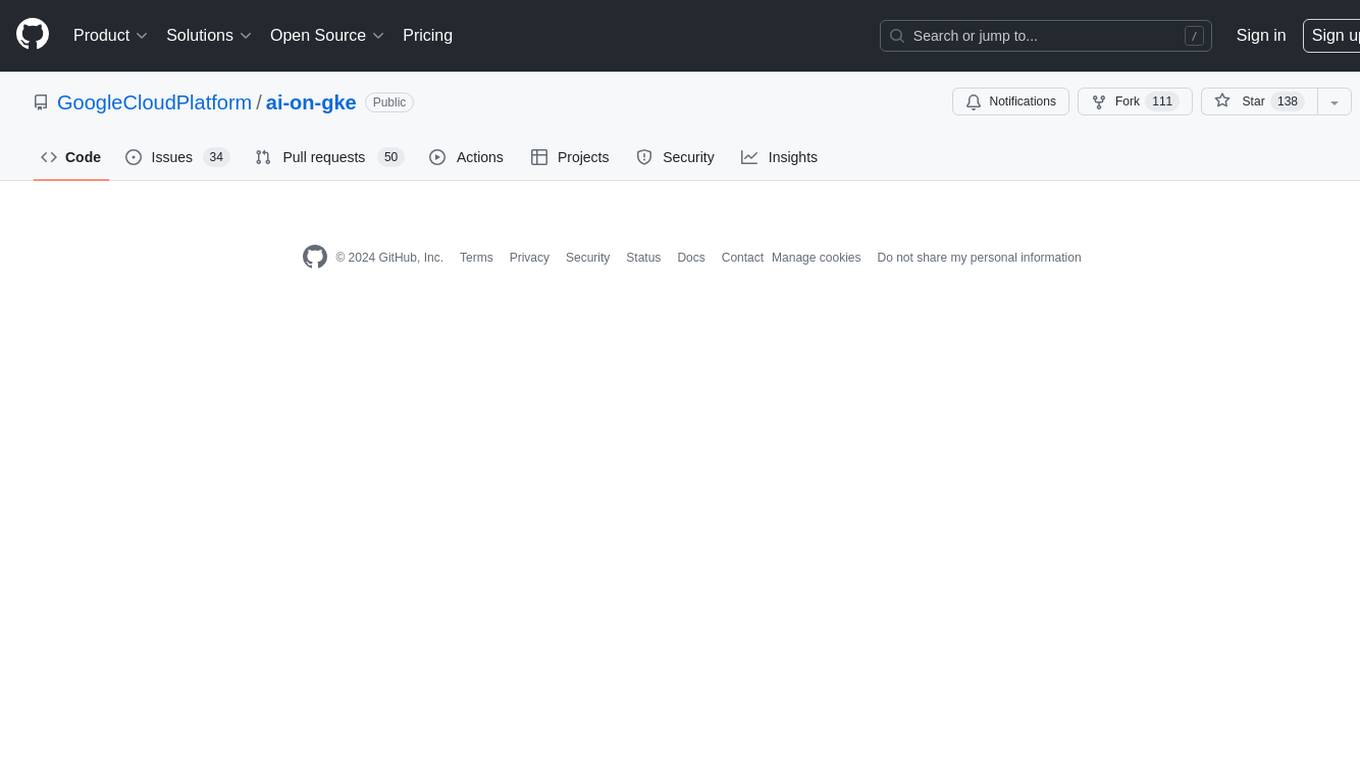
ai-on-gke
This repository contains assets related to AI/ML workloads on Google Kubernetes Engine (GKE). Run optimized AI/ML workloads with Google Kubernetes Engine (GKE) platform orchestration capabilities. A robust AI/ML platform considers the following layers: Infrastructure orchestration that support GPUs and TPUs for training and serving workloads at scale Flexible integration with distributed computing and data processing frameworks Support for multiple teams on the same infrastructure to maximize utilization of resources
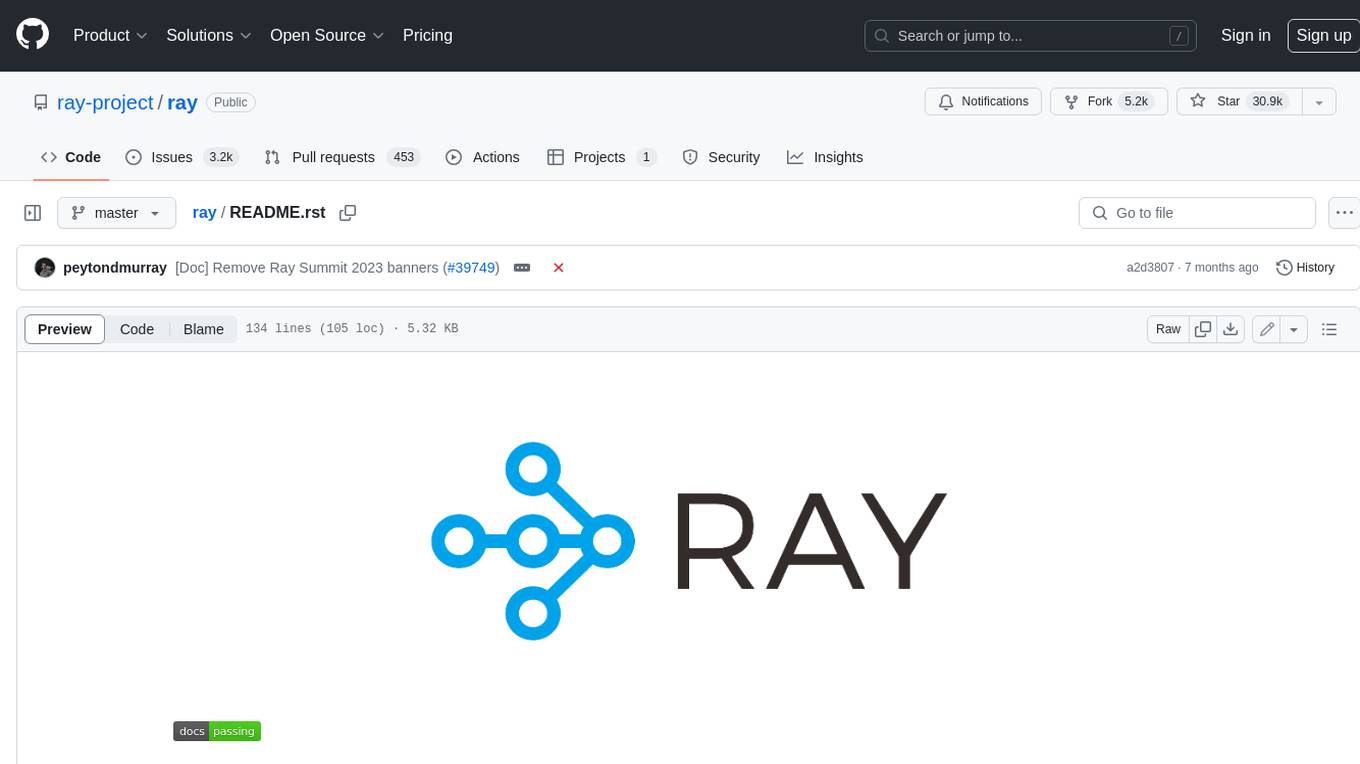
ray
Ray is a unified framework for scaling AI and Python applications. It consists of a core distributed runtime and a set of AI libraries for simplifying ML compute, including Data, Train, Tune, RLlib, and Serve. Ray runs on any machine, cluster, cloud provider, and Kubernetes, and features a growing ecosystem of community integrations. With Ray, you can seamlessly scale the same code from a laptop to a cluster, making it easy to meet the compute-intensive demands of modern ML workloads.
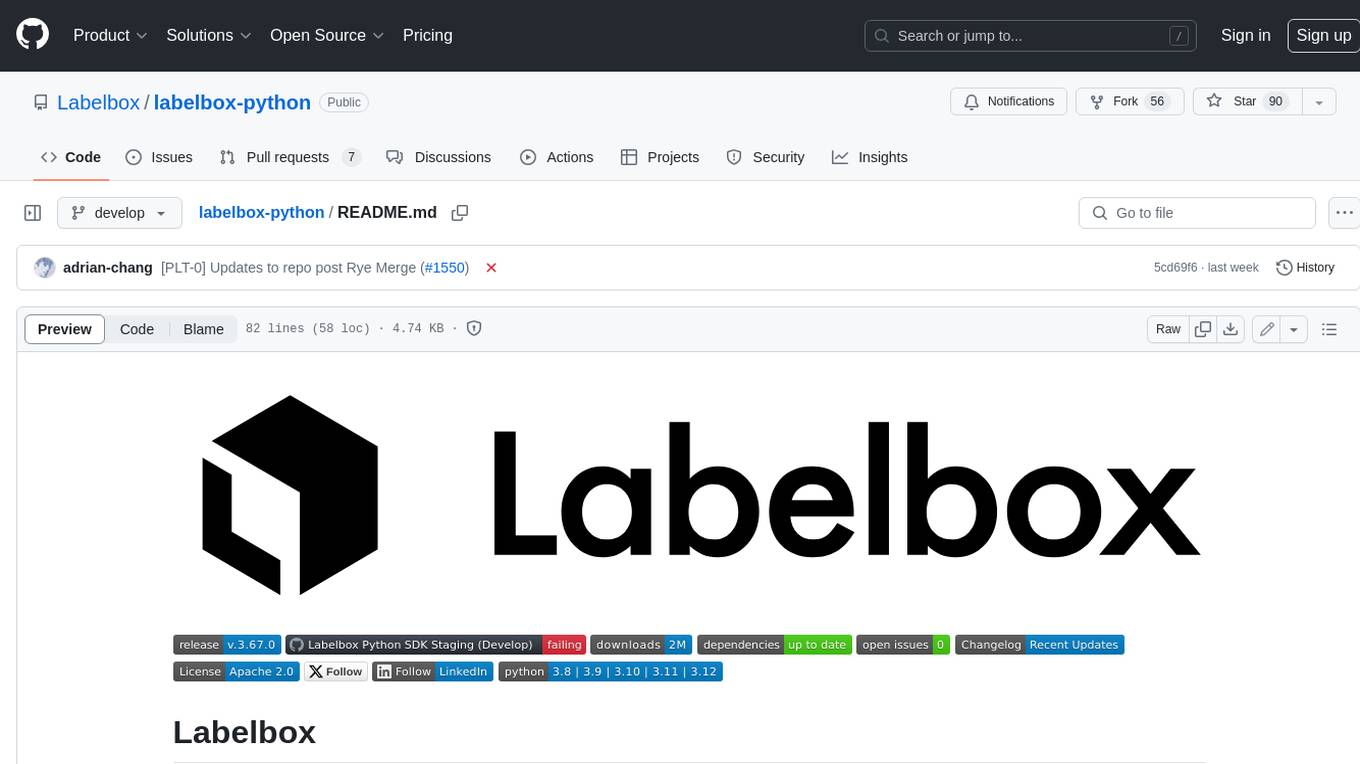
labelbox-python
Labelbox is a data-centric AI platform for enterprises to develop, optimize, and use AI to solve problems and power new products and services. Enterprises use Labelbox to curate data, generate high-quality human feedback data for computer vision and LLMs, evaluate model performance, and automate tasks by combining AI and human-centric workflows. The academic & research community uses Labelbox for cutting-edge AI research.
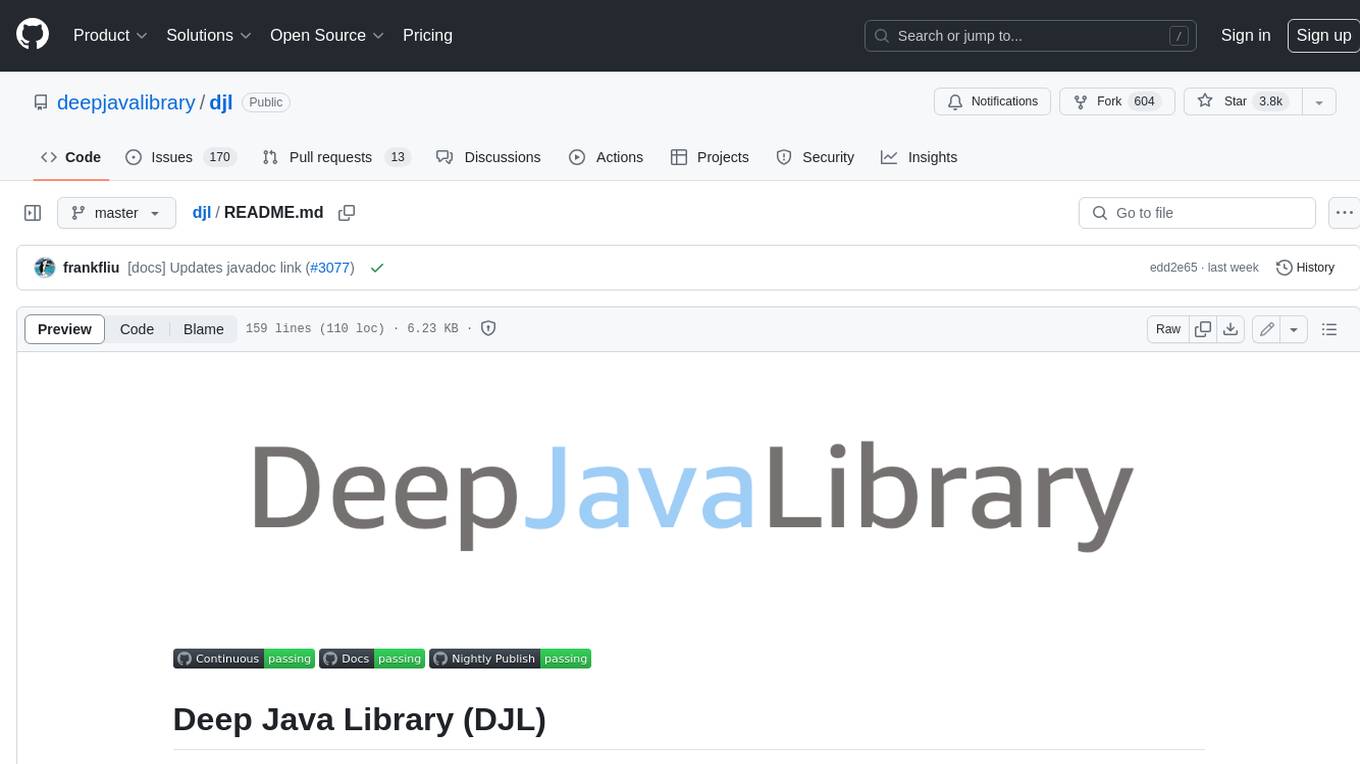
djl
Deep Java Library (DJL) is an open-source, high-level, engine-agnostic Java framework for deep learning. It is designed to be easy to get started with and simple to use for Java developers. DJL provides a native Java development experience and allows users to integrate machine learning and deep learning models with their Java applications. The framework is deep learning engine agnostic, enabling users to switch engines at any point for optimal performance. DJL's ergonomic API interface guides users with best practices to accomplish deep learning tasks, such as running inference and training neural networks.
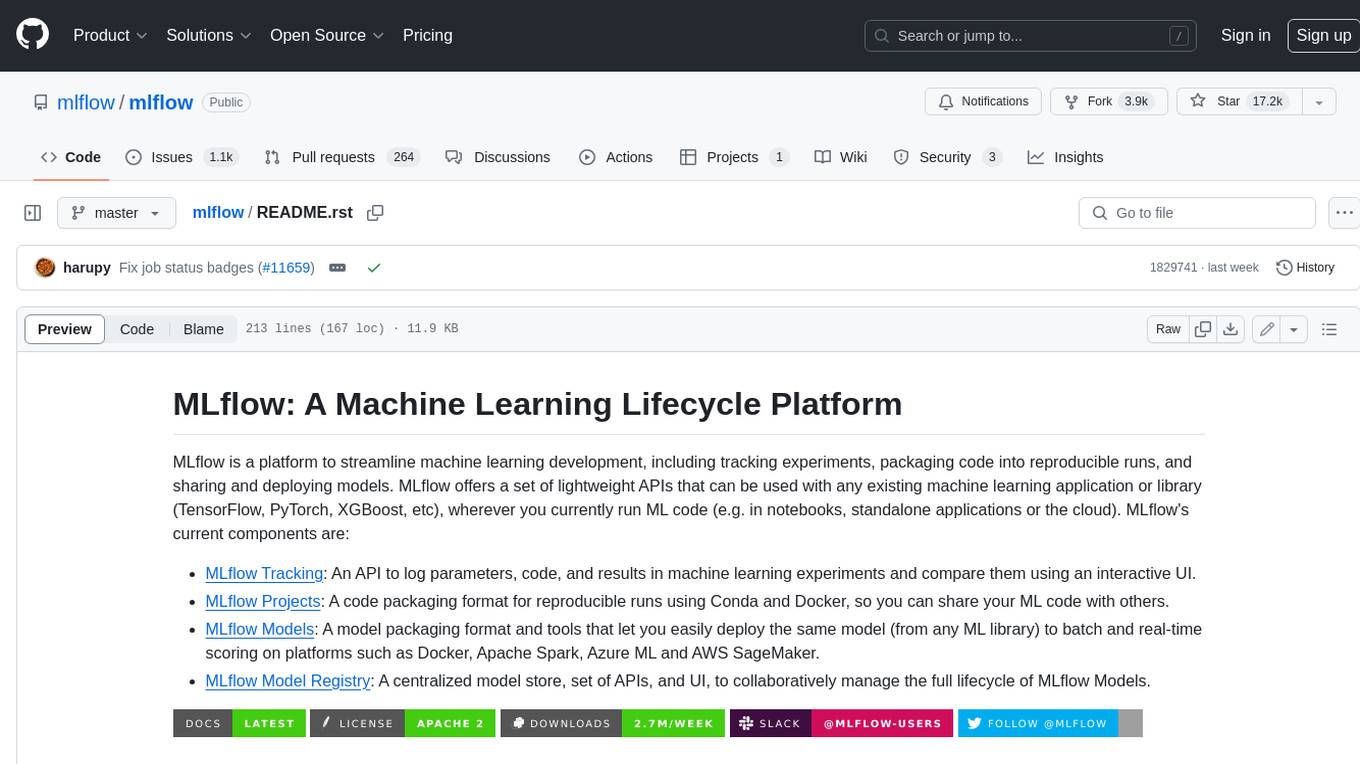
mlflow
MLflow is a platform to streamline machine learning development, including tracking experiments, packaging code into reproducible runs, and sharing and deploying models. MLflow offers a set of lightweight APIs that can be used with any existing machine learning application or library (TensorFlow, PyTorch, XGBoost, etc), wherever you currently run ML code (e.g. in notebooks, standalone applications or the cloud). MLflow's current components are:
* `MLflow Tracking
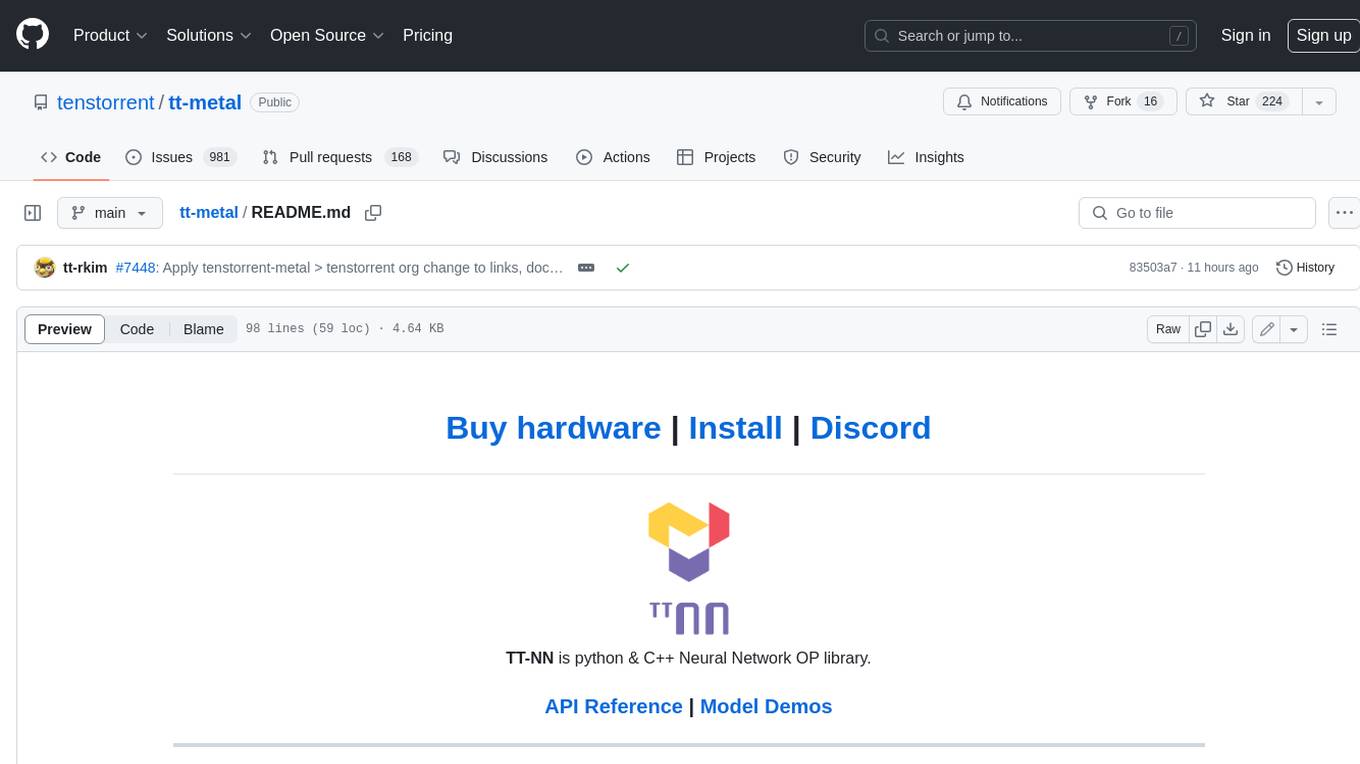
tt-metal
TT-NN is a python & C++ Neural Network OP library. It provides a low-level programming model, TT-Metalium, enabling kernel development for Tenstorrent hardware.
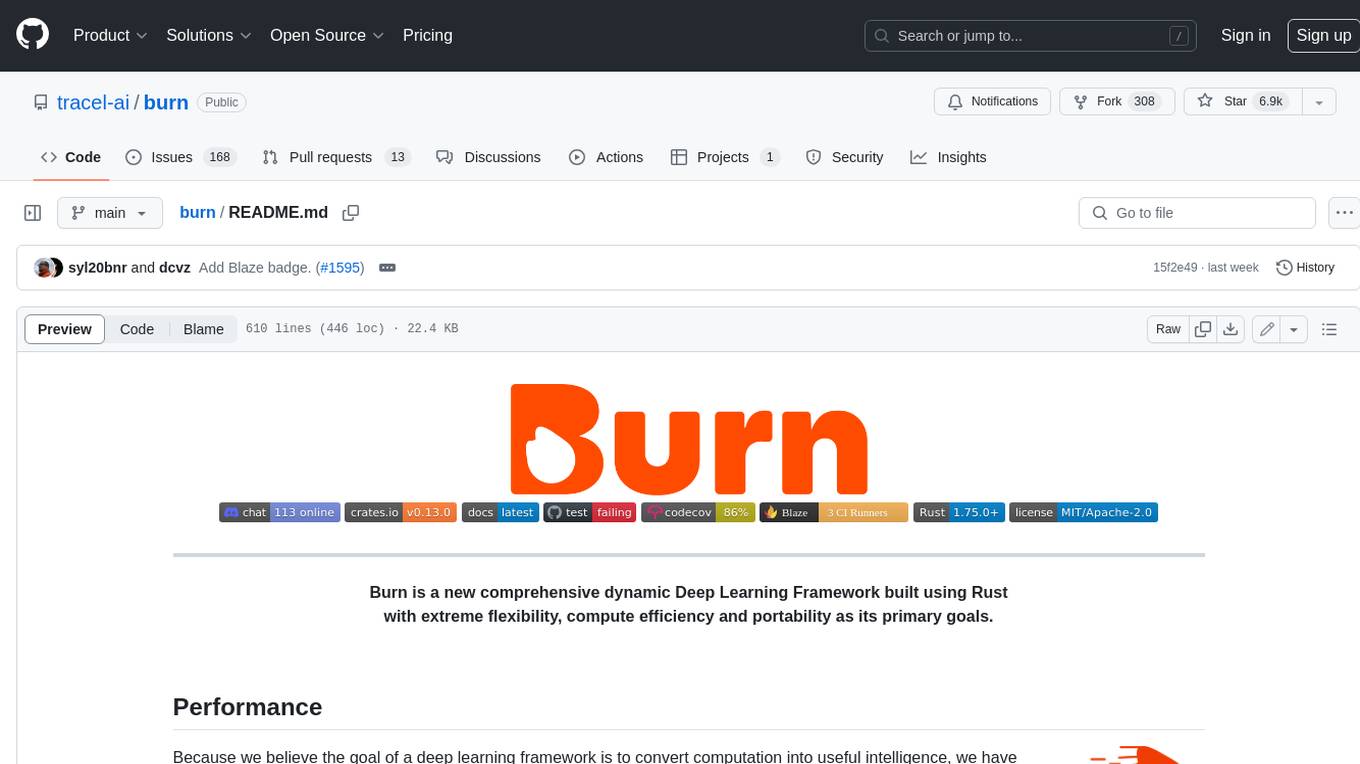
burn
Burn is a new comprehensive dynamic Deep Learning Framework built using Rust with extreme flexibility, compute efficiency and portability as its primary goals.
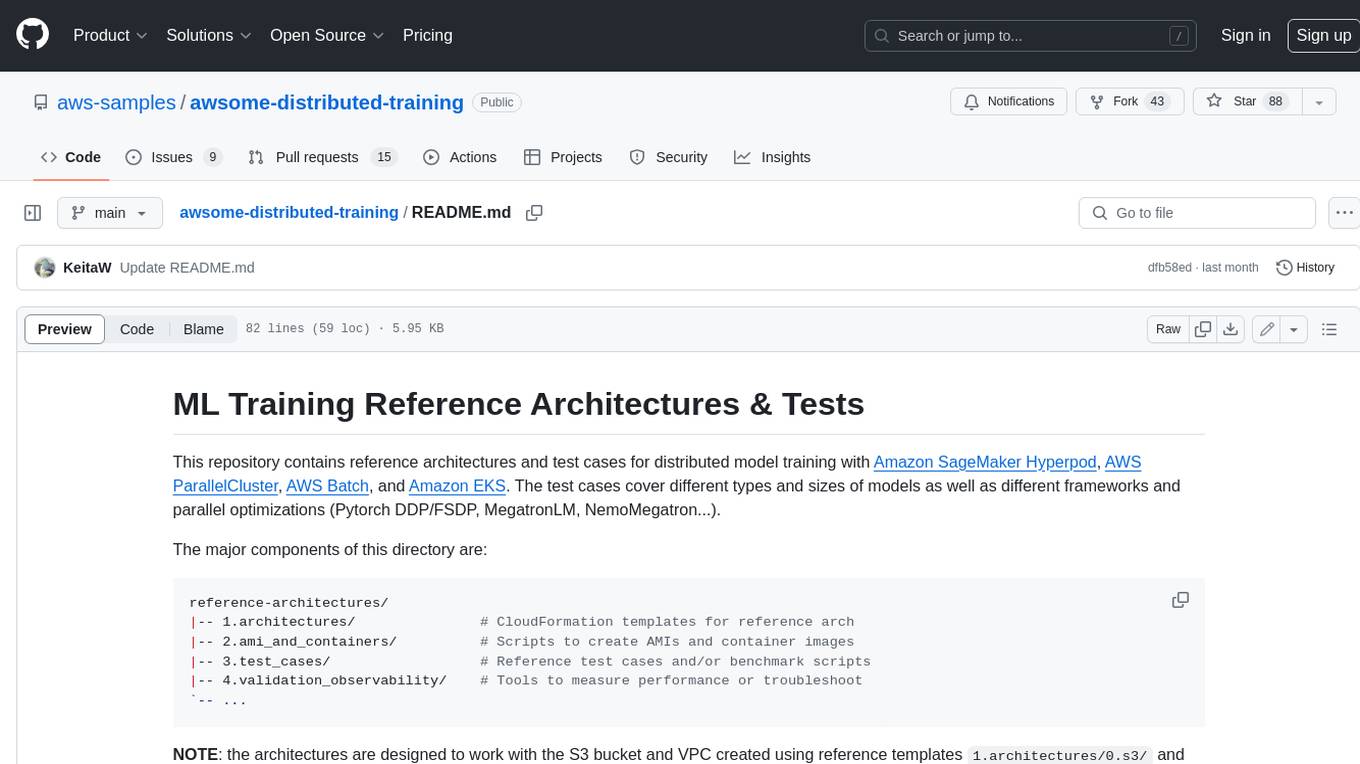
awsome-distributed-training
This repository contains reference architectures and test cases for distributed model training with Amazon SageMaker Hyperpod, AWS ParallelCluster, AWS Batch, and Amazon EKS. The test cases cover different types and sizes of models as well as different frameworks and parallel optimizations (Pytorch DDP/FSDP, MegatronLM, NemoMegatron...).
For similar jobs
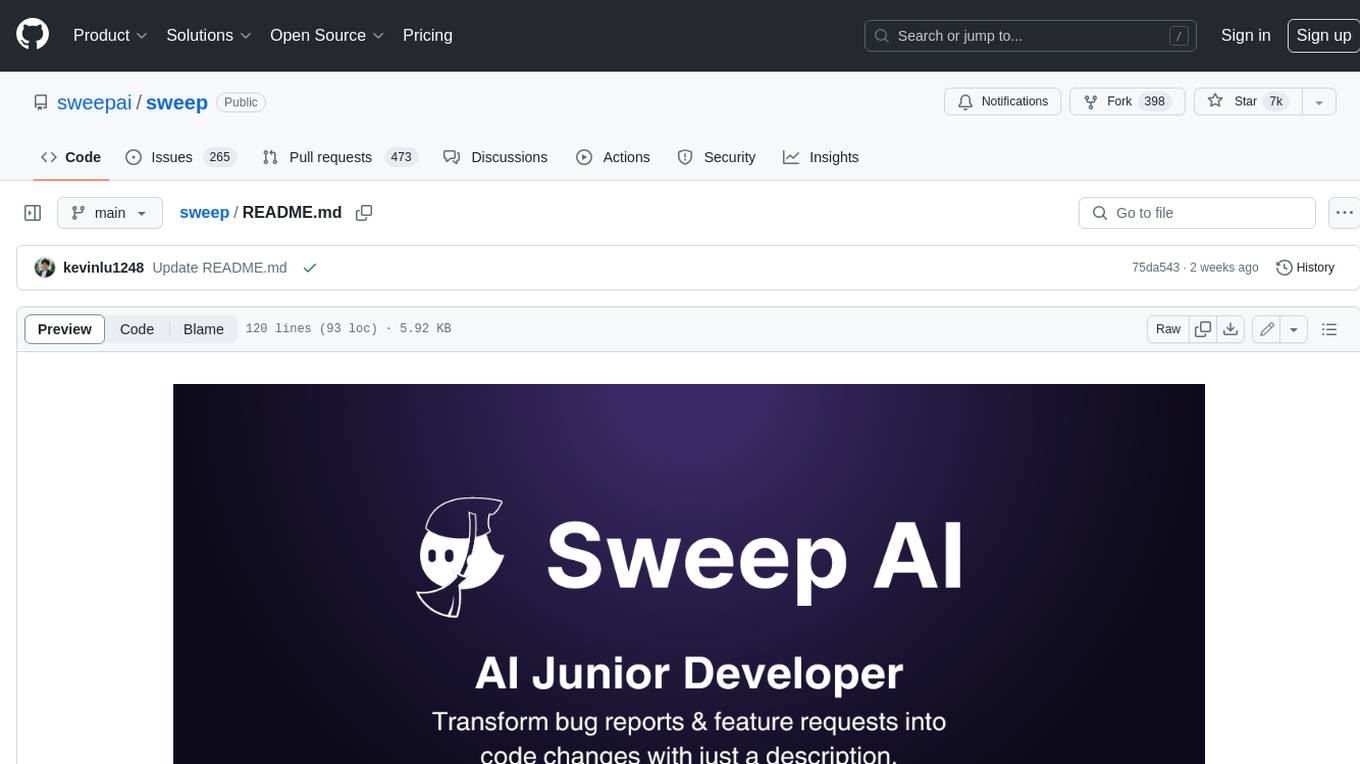
sweep
Sweep is an AI junior developer that turns bugs and feature requests into code changes. It automatically handles developer experience improvements like adding type hints and improving test coverage.
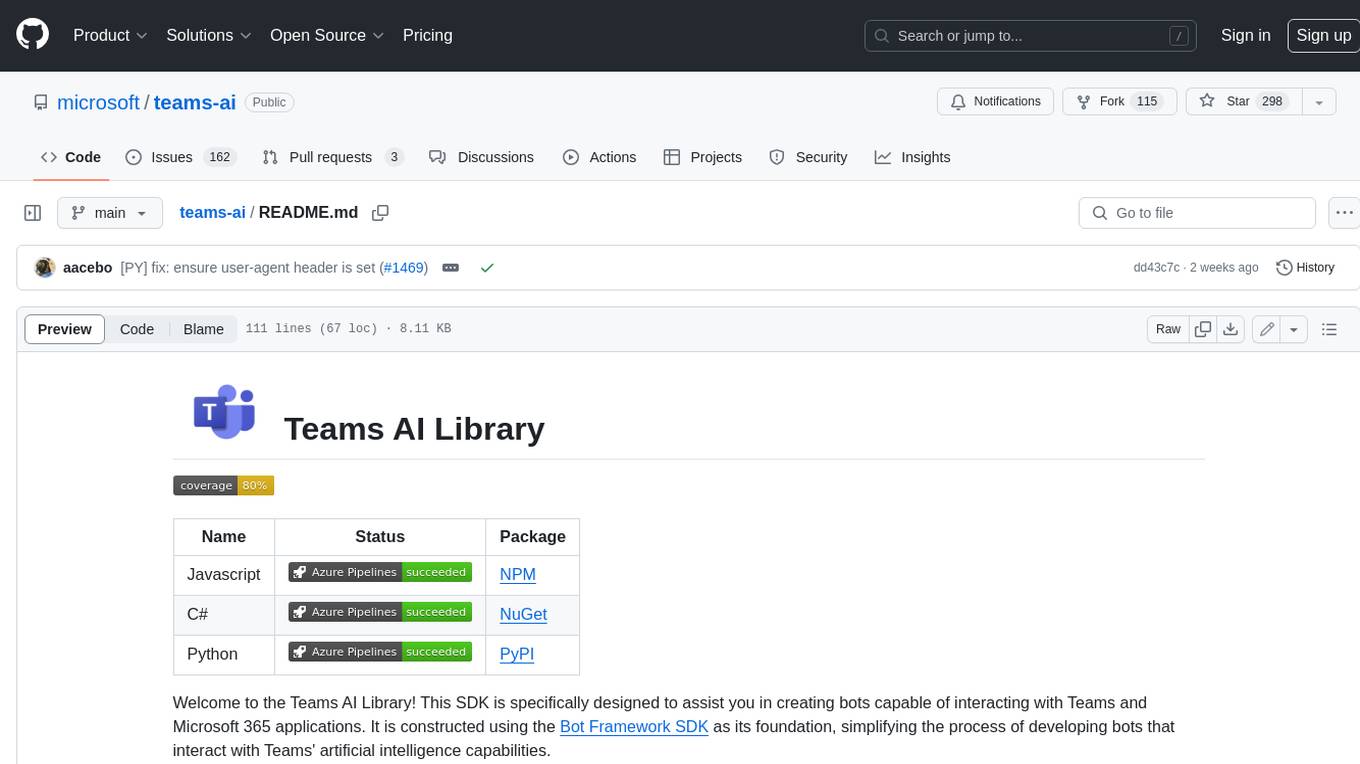
teams-ai
The Teams AI Library is a software development kit (SDK) that helps developers create bots that can interact with Teams and Microsoft 365 applications. It is built on top of the Bot Framework SDK and simplifies the process of developing bots that interact with Teams' artificial intelligence capabilities. The SDK is available for JavaScript/TypeScript, .NET, and Python.
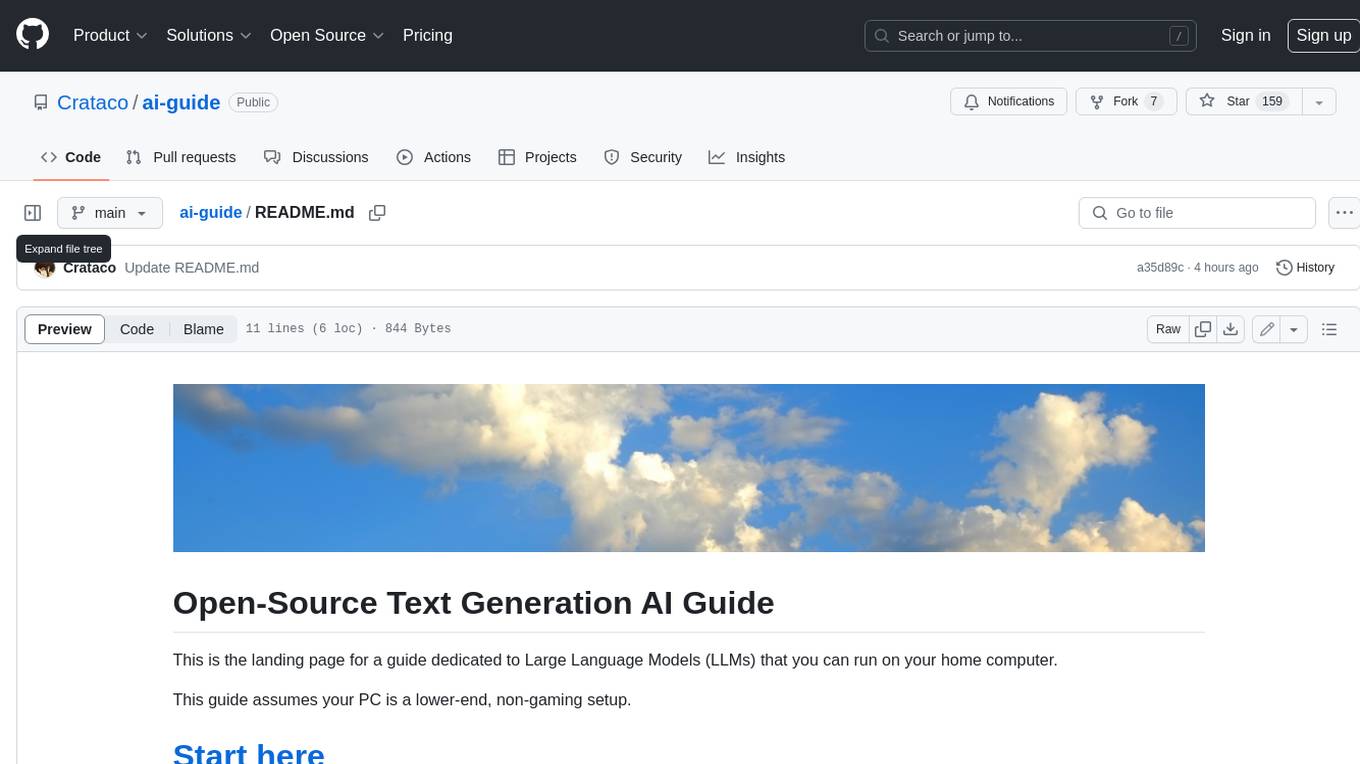
ai-guide
This guide is dedicated to Large Language Models (LLMs) that you can run on your home computer. It assumes your PC is a lower-end, non-gaming setup.
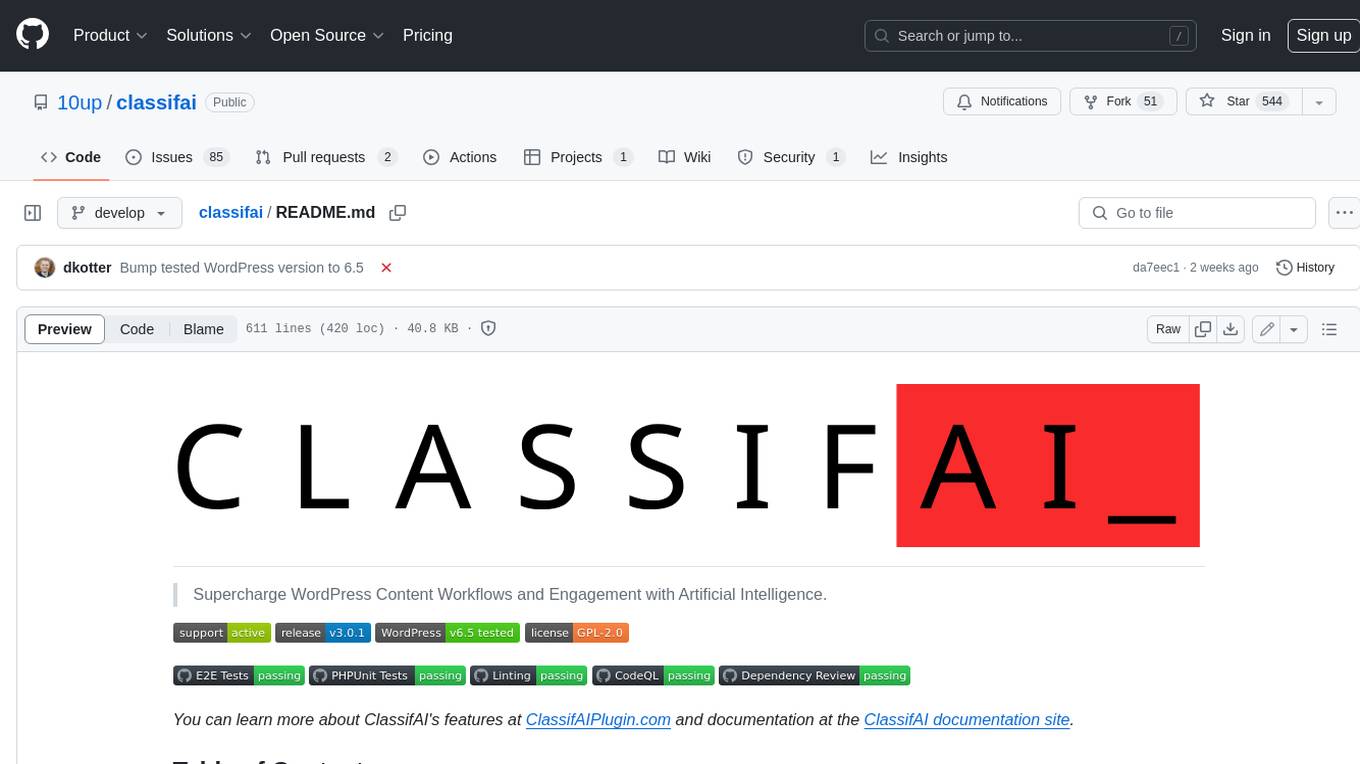
classifai
Supercharge WordPress Content Workflows and Engagement with Artificial Intelligence. Tap into leading cloud-based services like OpenAI, Microsoft Azure AI, Google Gemini and IBM Watson to augment your WordPress-powered websites. Publish content faster while improving SEO performance and increasing audience engagement. ClassifAI integrates Artificial Intelligence and Machine Learning technologies to lighten your workload and eliminate tedious tasks, giving you more time to create original content that matters.
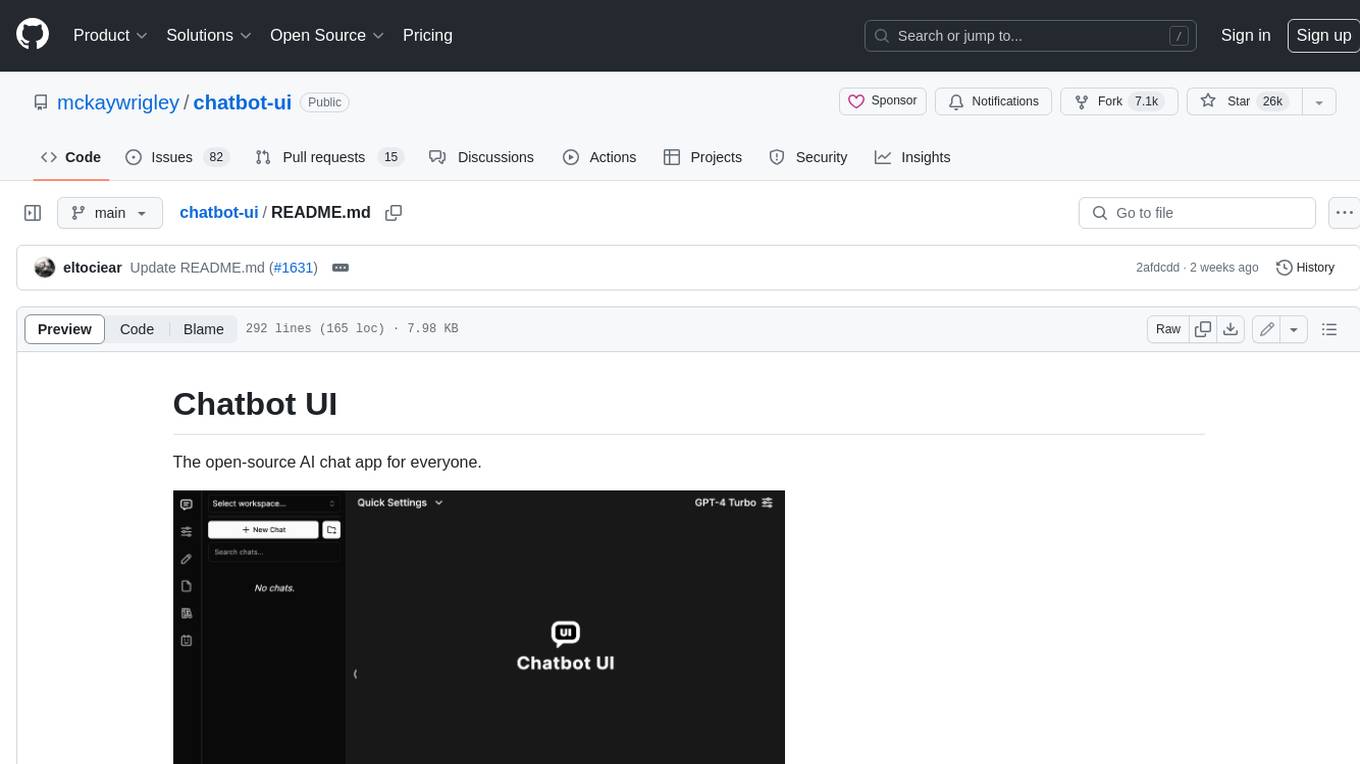
chatbot-ui
Chatbot UI is an open-source AI chat app that allows users to create and deploy their own AI chatbots. It is easy to use and can be customized to fit any need. Chatbot UI is perfect for businesses, developers, and anyone who wants to create a chatbot.
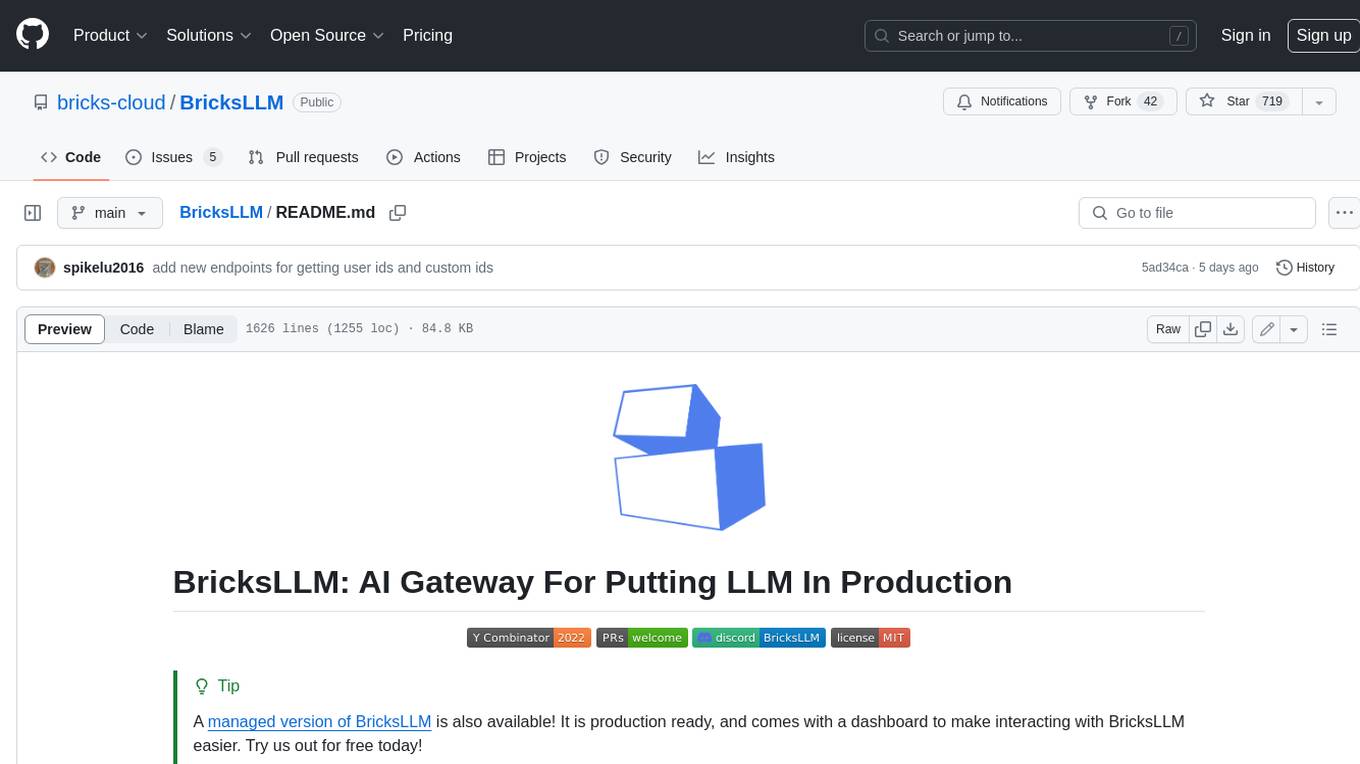
BricksLLM
BricksLLM is a cloud native AI gateway written in Go. Currently, it provides native support for OpenAI, Anthropic, Azure OpenAI and vLLM. BricksLLM aims to provide enterprise level infrastructure that can power any LLM production use cases. Here are some use cases for BricksLLM: * Set LLM usage limits for users on different pricing tiers * Track LLM usage on a per user and per organization basis * Block or redact requests containing PIIs * Improve LLM reliability with failovers, retries and caching * Distribute API keys with rate limits and cost limits for internal development/production use cases * Distribute API keys with rate limits and cost limits for students
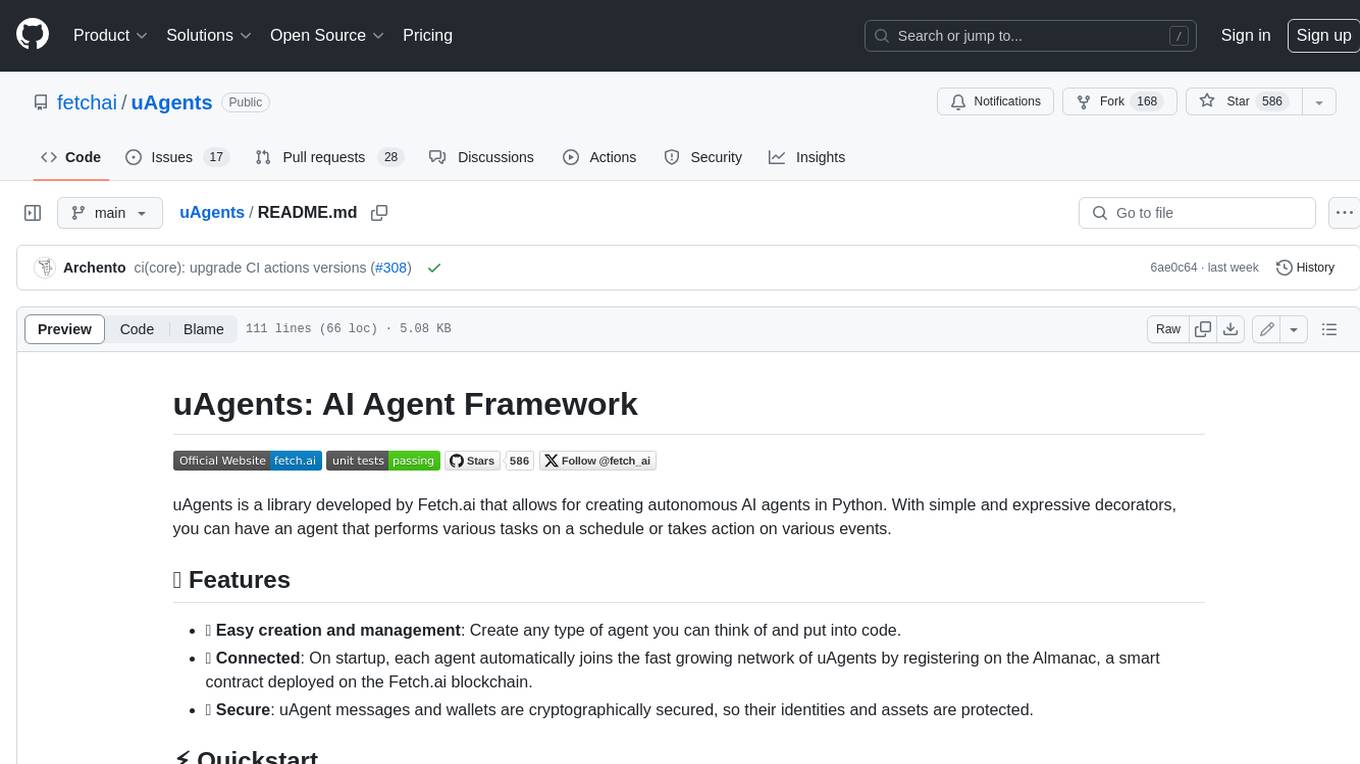
uAgents
uAgents is a Python library developed by Fetch.ai that allows for the creation of autonomous AI agents. These agents can perform various tasks on a schedule or take action on various events. uAgents are easy to create and manage, and they are connected to a fast-growing network of other uAgents. They are also secure, with cryptographically secured messages and wallets.
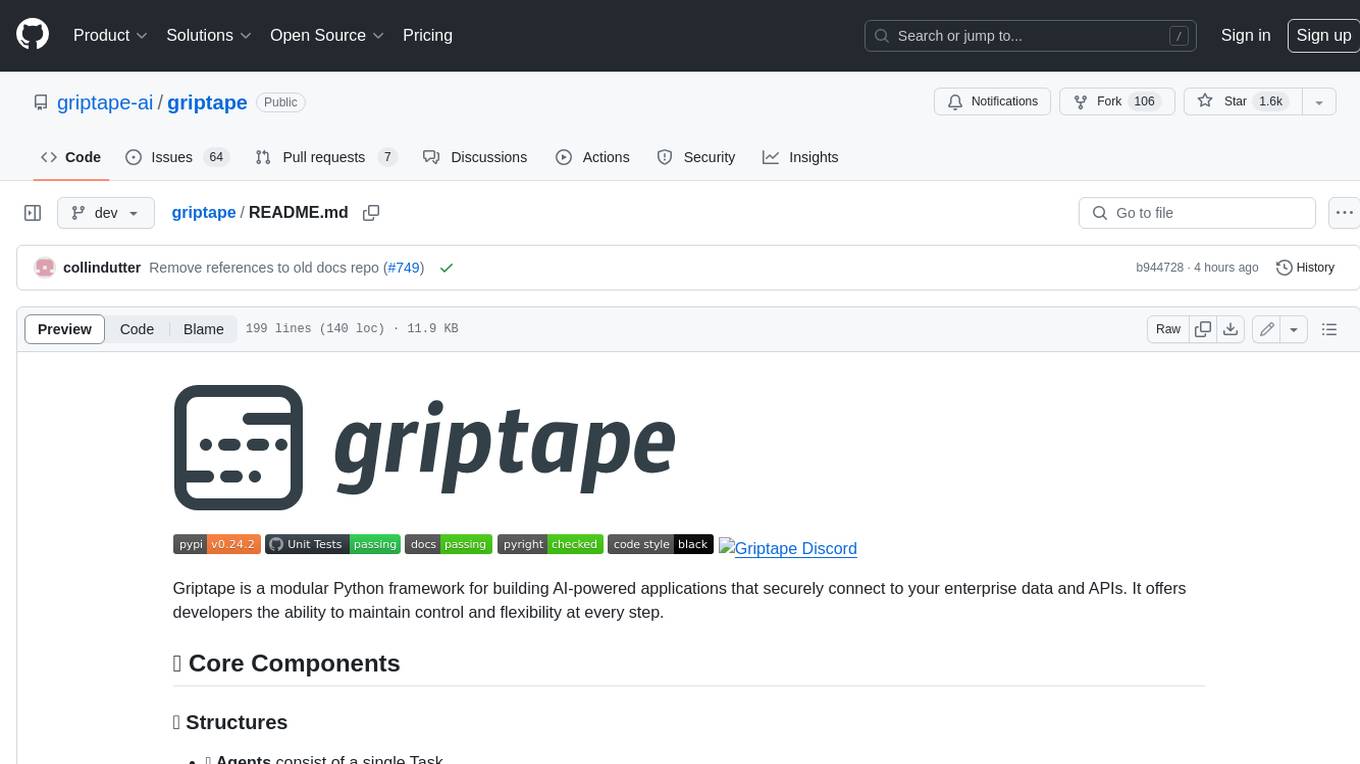
griptape
Griptape is a modular Python framework for building AI-powered applications that securely connect to your enterprise data and APIs. It offers developers the ability to maintain control and flexibility at every step. Griptape's core components include Structures (Agents, Pipelines, and Workflows), Tasks, Tools, Memory (Conversation Memory, Task Memory, and Meta Memory), Drivers (Prompt and Embedding Drivers, Vector Store Drivers, Image Generation Drivers, Image Query Drivers, SQL Drivers, Web Scraper Drivers, and Conversation Memory Drivers), Engines (Query Engines, Extraction Engines, Summary Engines, Image Generation Engines, and Image Query Engines), and additional components (Rulesets, Loaders, Artifacts, Chunkers, and Tokenizers). Griptape enables developers to create AI-powered applications with ease and efficiency.