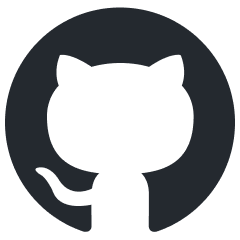
EvoMaster
The first open-source AI-driven tool for automatically generating system-level test cases (also known as fuzzing) for web/enterprise applications. Currently targeting whitebox and blackbox testing of Web APIs, like REST, GraphQL and RPC (e.g., gRPC and Thrift).
Stars: 554
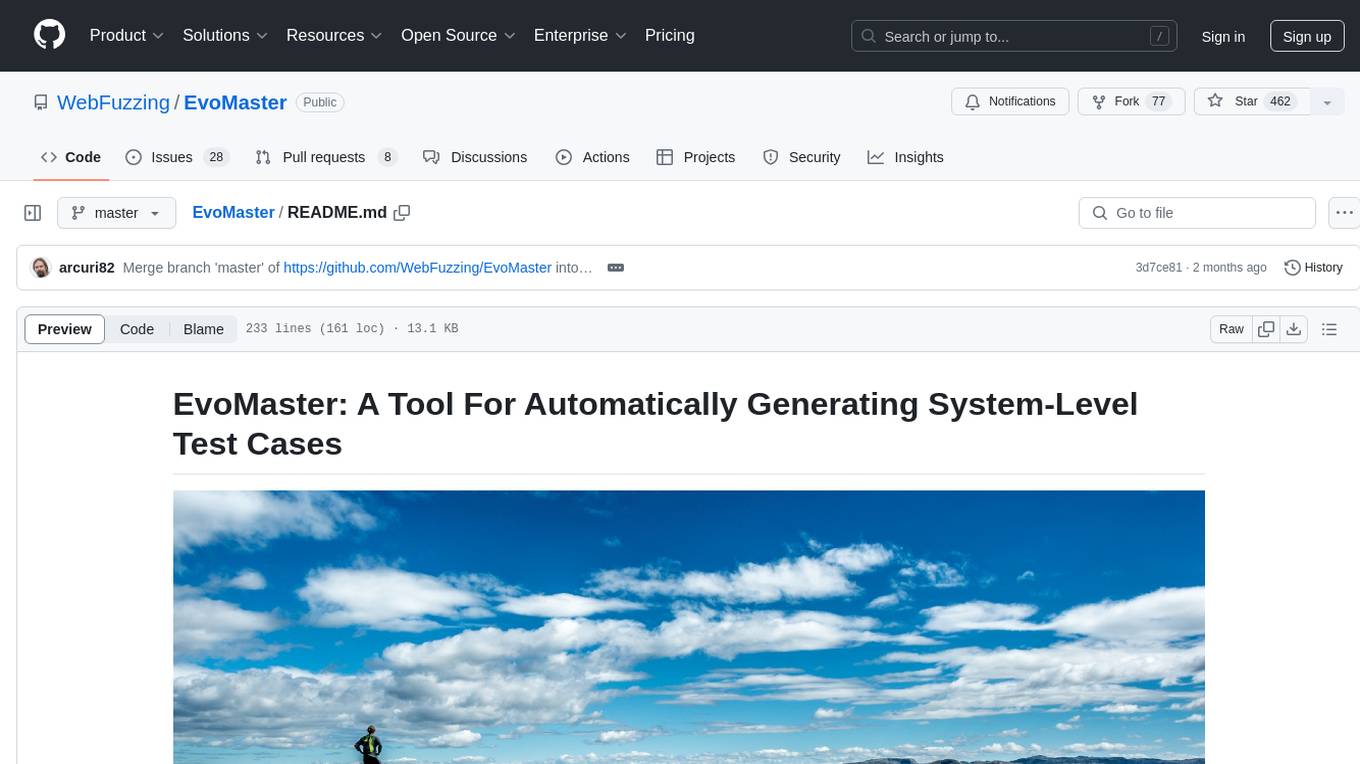
EvoMaster is an open-source AI-driven tool that automatically generates system-level test cases for web/enterprise applications. It uses an Evolutionary Algorithm and Dynamic Program Analysis to evolve test cases, maximizing code coverage and fault detection. The tool supports REST, GraphQL, and RPC APIs, with whitebox testing for JVM-compiled languages. It generates JUnit tests, detects faults, handles SQL databases, and supports authentication. EvoMaster has been funded by the European Research Council and the Research Council of Norway.
README:
EvoMaster (www.evomaster.org) is the first (2016) open-source AI-driven tool that automatically generates system-level test cases for web/enterprise applications. This is related to Fuzzing. In particular, EvoMaster can fuzz APIs such as REST, GraphQL and RPC. Not only EvoMaster can generate inputs that find program crashes, but also it generates small effective test suites (e.g., in Python, JS and Java/Kotlin JUnit format) that can be used for regression testing.
EvoMaster is an AI driven tool. In particular, internally it uses an Evolutionary Algorithm and Dynamic Program Analysis to be able to generate effective test cases. The approach is to evolve test cases from an initial population of random ones, trying to maximize measures like code coverage and fault detection. EvoMaster uses several kinds of AI heuristics to improve performance even further, building on decades of research in the field of Search-Based Software Testing.
On a console, copy&paste the following (requires Docker installed). It will fuzz the PetClinic example API from Swagger, for 30 seconds.
docker run -v "$(pwd)/generated_tests":/generated_tests webfuzzing/evomaster --blackBox true --maxTime 30s --ratePerMinute 60 --bbSwaggerUrl https://petstore.swagger.io/v2/swagger.json
Note, if run in a MSYS shell on Windows like Git Bash, there is the need of an extra / before the $ (as in the following video).
Once the command is executed, you can inspect the generated files under generated_tests
folder.
-
Web APIs: At the moment, EvoMaster can generate test cases for REST, GraphQL and RPC (e.g., gRPC and Thrift) APIs.
-
Black-Box testing mode: can run on any API (regardless of its programming language, e.g., Python and Go). However, results for black-box testing will be worse than white-box testing (e.g., due to lack of code analysis). Default test case output is in Python, but other formats are available as well.
-
White-Box testing mode: can be used for APIs compiled to JVM (e.g., Java and Kotlin). EvoMaster analyses the bytecode of the tested applications, and uses several heuristics such as testability transformations and taint analysis to be able to generate more effective test cases. We support JDK 8 and the major LTS versions after that (currently JDK 21). Might work on other JVM versions, but we provide NO support for it. Note: there was initial support for other languages as well, like for example JavaScript/TypeScript and C#, but they were not in a stable, feature-complete state. The support for those languages for white-box testing has been dropped, at least for the time being.
-
Installation: we provide installers for the main operating systems: Windows (
.msi
), OSX (.dmg
) and Linux (.deb
). We also provide an uber-fat JAR file. To download them, see the Release page. Release notes are present in the file release_notes.md. If you are using the uber-fat JAR, it should work with any major LTS version (from JDK 8 on). Whereas for the client library, needed for white-box testing, we will support JDK 8 likely for a long, long while, be warned that future versions of the executable JAR might start to require higher versions of the JDK in a non-so-distant future. If that is going to be higher than your current version of the JVM, if you cannot upgrade or have 2 different JDKs on your machine, then you should not use the uber-jar but rather one of the installers. When you use one of the installers, keep in mind that currently they do not update thePATH
variable. This needs to be done manually, see documentation. -
Docker: EvoMaster is now released via Docker as well, under webfuzzing/evomaster on Docker Hub. For more information on how to use EvoMaster via Docker, see documentation.
-
GitHub Action: it is possible to run EvoMaster in GitHub Actions, as part of Continuous Integration, by using the following custom action (which is in a different GitHub repository).
-
State-of-the-art: an independent study (2022), comparing 10 fuzzers on 20 RESTful APIs, shows that EvoMaster gives the best results. Another independent study (2024) done by a different research group confirms these results.
-
Schema: REST APIs must provide a schema in OpenAPI/Swagger format (either v2 or v3).
-
Output: the tool generates JUnit (version 4 or 5) tests, written in either Java or Kotlin, as well as test suites in Python and JavaScript. For a complete list, see the documentation for the CLI parameter --outputFormat. Some examples are: PYTHON_UNITTEST, KOTLIN_JUNIT_5, JAVA_JUNIT_4 and JS_JEST. Note that the generated tests rely on third-party libraries (e.g., to make HTTP calls). These will need to be setup in your projects, see documentation.
-
Fault detection: EvoMaster can generate tests cases that reveal faults/bugs in the tested applications. Different heuristics are employed, like checking for 500 status codes and mismatches from the API schemas.
-
Self-contained tests: for white-box testing, the generated tests do start/stop the application, binding to an ephemeral port. This means that the generated tests can be used for regression testing (e.g., added to the Git repository of the application, and run with any build tool such as Maven and Gradle). For black-box testing, you will need to make sure the application is up and running before executing the tests.
-
SQL handling: for white-box testing, EvoMaster can intercept and analyse all communications done with SQL databases, and use such information to generate higher code coverage test cases. Furthermore, it can generate data directly into the databases, and have such initialization automatically added in the generated tests. At the moment, EvoMaster supports Postgres, MySQL and H2 databases.
-
Authentication: we support auth based on authentication headers and cookies. Besides using fixed HTTP headers, it is also possible to declaratively specify which login endpoint should be used to dynamically obtain authentication info (e.g., auth tokens or cookies) for each test execution. See documentation.
-
Driver: to be used for white-box testing, users need to write a driver manually. We recommend to try black-box mode first (should just need a few minutes to get it up and running) to get an idea of what EvoMaster can do for you.
-
JDK 9+: white-box testing requires bytecode manipulation. Each new release of the JDK makes doing this harder and harder. Dealing with JDKs above 8 is doable, but it requires some settings. See documentation.
-
Execution time: to get good results, you might need to run the search for several hours. We recommend to first try the search for 10 minutes, just to get an idea of what type of tests can be generated. But, then, you should run EvoMaster for something like between 1 and 24 hours (the longer the better, but it is unlikely to get better results after 24 hours).
-
RPC APIs: for the moment, we do not directly support RPC schema definitions. Fuzzing RPC APIs requires to write a driver, using the client library of the API to make the calls.
-
External services: (e.g., other RESTful APIs) currently there is no support for them (e.g., to automatically mock them). It is work in progress.
-
NoSQL databases: (e.g., MongoDB) currently no support. It is work in progress.
-
Failing tests: the tests generated by EvoMaster should all pass, and not fail, even when they detect a fault. In those cases, comments/test-names would point out that a test is revealing a possible fault, while still passing. However, in some cases the generated tests might fail. This is due to the so called flaky tests, e.g., when a test has assertions based on the time clock (e.g., dates and timestamps). There is ongoing effort to address this problem, but it is still not fully solved.
Several enterprises use EvoMaster to fuzz their Web APIs. We do few academia-industry collaborations (see more info here), where we help test engineers to apply EvoMaster on their systems, as long as we can then report on such experience. Example of Fortune 500 companies using EvoMaster are:
-
A short video (5 minutes) shows the use of EvoMaster on one of the case studies in EMB.
-
This 13-minute video shows how to write a white-box driver for EvoMaster, for the rest-api-example.
-
How to Download and Install EvoMaster on Windows 10, using its .msi installer.
-
Short presentation (5 minutes) about version 2.0.0.
In the last few years, several few tools have been proposed in the academic literature and in the open-source community. You can read more details in this 2023 survey on REST API testing.
Existing open-source tools for REST API fuzzing are for example (in alphabetic order): CATS, Dredd, Fuzz-lightyear, ResTest, RestCT, Restler, RestTestGen, and Schemathesis.
All these tools are black-box, i.e., they do not analyze the source-code of the tested APIs to generate more effective test data. As we are the authors of EvoMaster, we are too biased to compare it properly with those other black-box tools. However, different independent studies (e.g., in 2022 and 2024) shows that EvoMaster is among the best performant. Furthermore, if your APIs are running on the JVM (e.g., written in Java or Kotlin), then EvoMaster has clearly an advantage, as it supports white-box testing.
If you are trying to use EvoMaster, but the instructions in this documentation are not enough to get you started, or they are too unclear, then it means it is a bug in the documentation, which then would need to be clarified and updated. In such cases, please create a new issue.
Also, feel free to start new discussion topics in the Discussions forum. If you have time, please consider answering the polls there.
If you are working on an open-source API, you can drop us a message if you have problems using EvoMaster on it. Otherwise, if you are working in industry on closed-source APIs, we have options for academia-industry collaborations (see more info here).
- Example of generated tests
- Download and Install EvoMaster
- Build EvoMaster from source
- Command-Line Interface (CLI) options
- OpenApi/Swagger Schema
- Using EvoMaster for Black-Box Testing (easier to setup, but worse results)
- Using EvoMaster for White-Box Testing (harder to setup, but better results)
- Configuring authentication
- Console output
- Library dependencies for the generated tests
- How to contribute
- Troubleshooting
- More Info
EvoMaster has been funded by:
- 2020-2026: a 2 million Euro grant by the European Research Council (ERC), as part of the ERC Consolidator project Using Evolutionary Algorithms to Understand and Secure Web/Enterprise Systems.
- 2018-2021: a 7.8 million Norwegian Kroner grant by the Research Council of Norway (RCN), as part of the Frinatek project Evolutionary Enterprise Testing.
This project has received funding from the European Research Council (ERC) under the European Union’s Horizon 2020 research and innovation programme (grant agreement No 864972).
EvoMaster's source code is released under the LGPL (v3) license. For a list of the used third-party libraries, you can directly see the root pom.xml file. For a list of code directly imported (and then possibly modified/updated) from other open-source projects, see here.
For Tasks:
Click tags to check more tools for each tasksFor Jobs:
Alternative AI tools for EvoMaster
Similar Open Source Tools
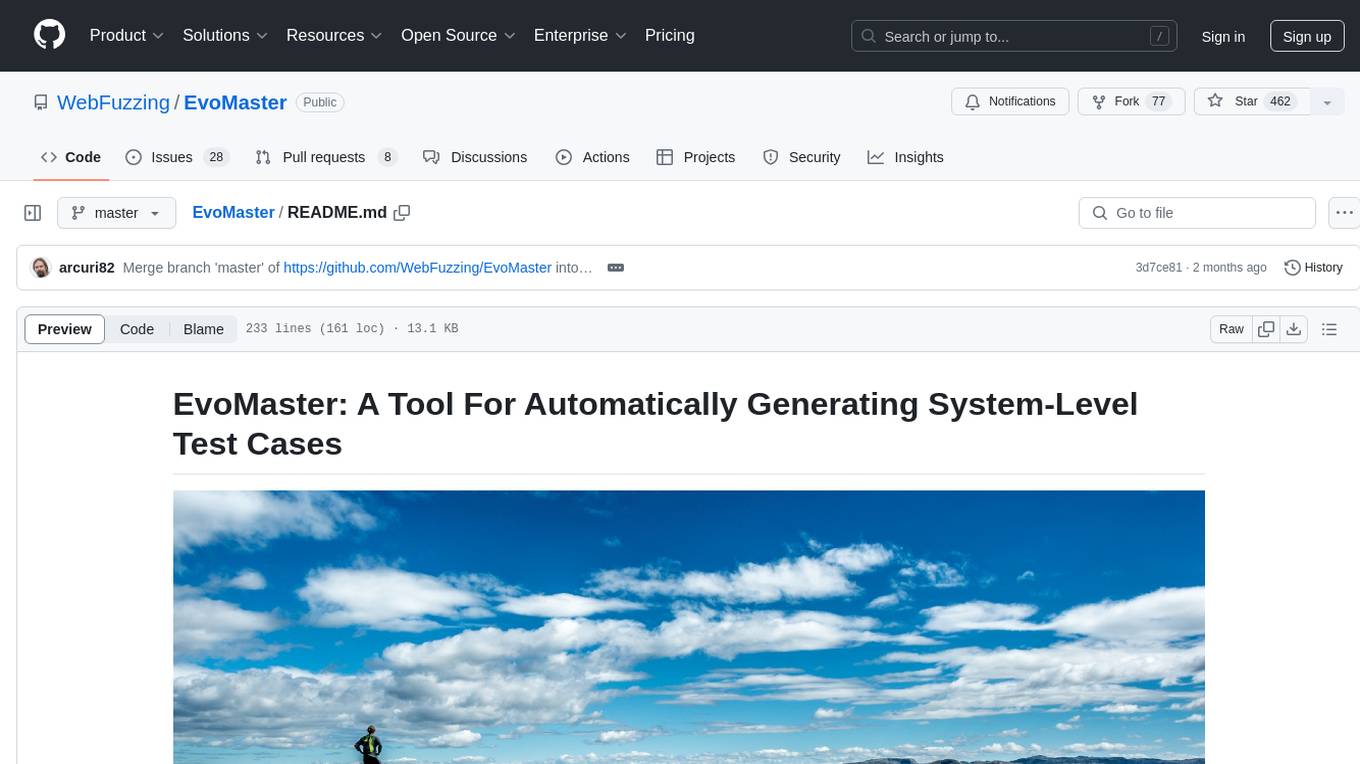
EvoMaster
EvoMaster is an open-source AI-driven tool that automatically generates system-level test cases for web/enterprise applications. It uses an Evolutionary Algorithm and Dynamic Program Analysis to evolve test cases, maximizing code coverage and fault detection. The tool supports REST, GraphQL, and RPC APIs, with whitebox testing for JVM-compiled languages. It generates JUnit tests, detects faults, handles SQL databases, and supports authentication. EvoMaster has been funded by the European Research Council and the Research Council of Norway.
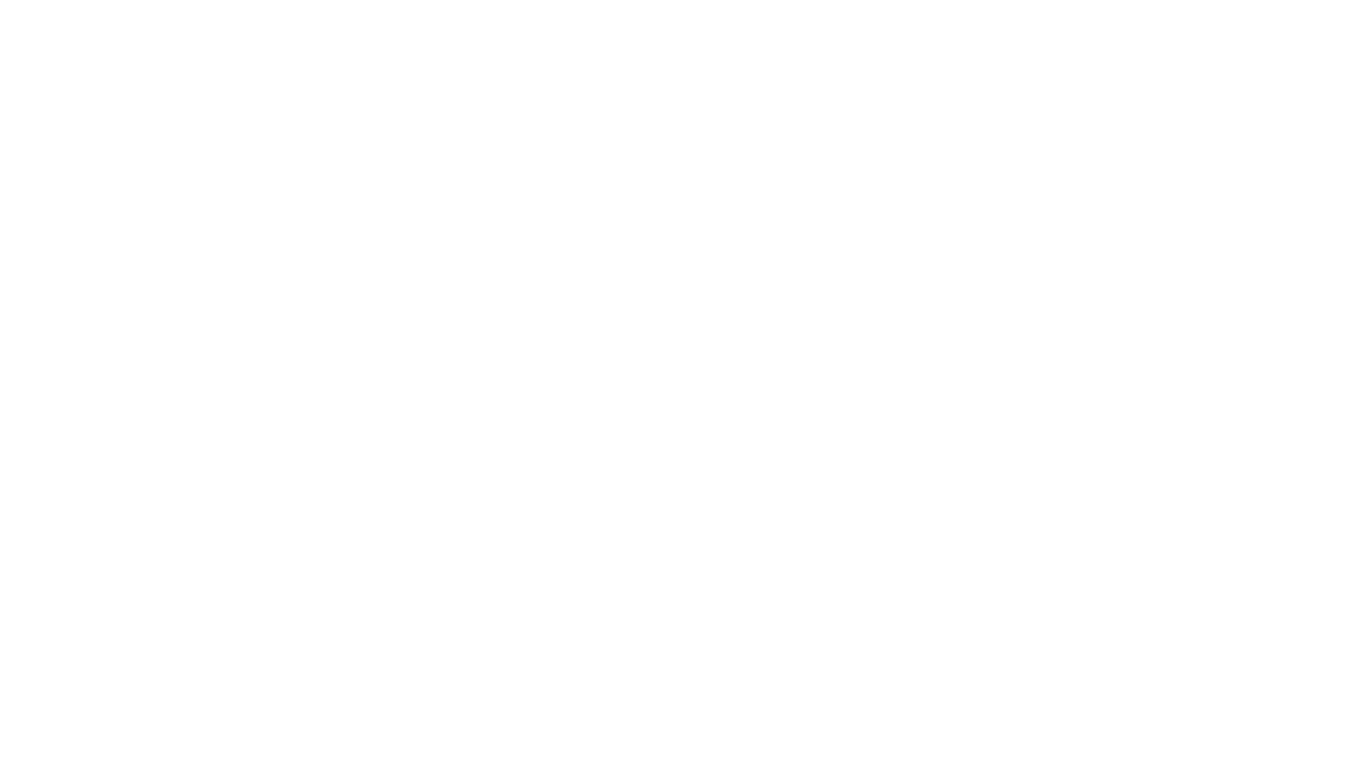
EvoMaster
EvoMaster is an open-source AI-driven tool that automatically generates system-level test cases for web/enterprise applications. It uses Evolutionary Algorithm and Dynamic Program Analysis to evolve test cases, maximizing code coverage and fault detection. It supports REST, GraphQL, and RPC APIs, with whitebox testing for JVM-compiled APIs. The tool generates JUnit tests in Java or Kotlin, focusing on fault detection, self-contained tests, SQL handling, and authentication. Known limitations include manual driver creation for whitebox testing and longer execution times for better results. EvoMaster has been funded by ERC and RCN grants.
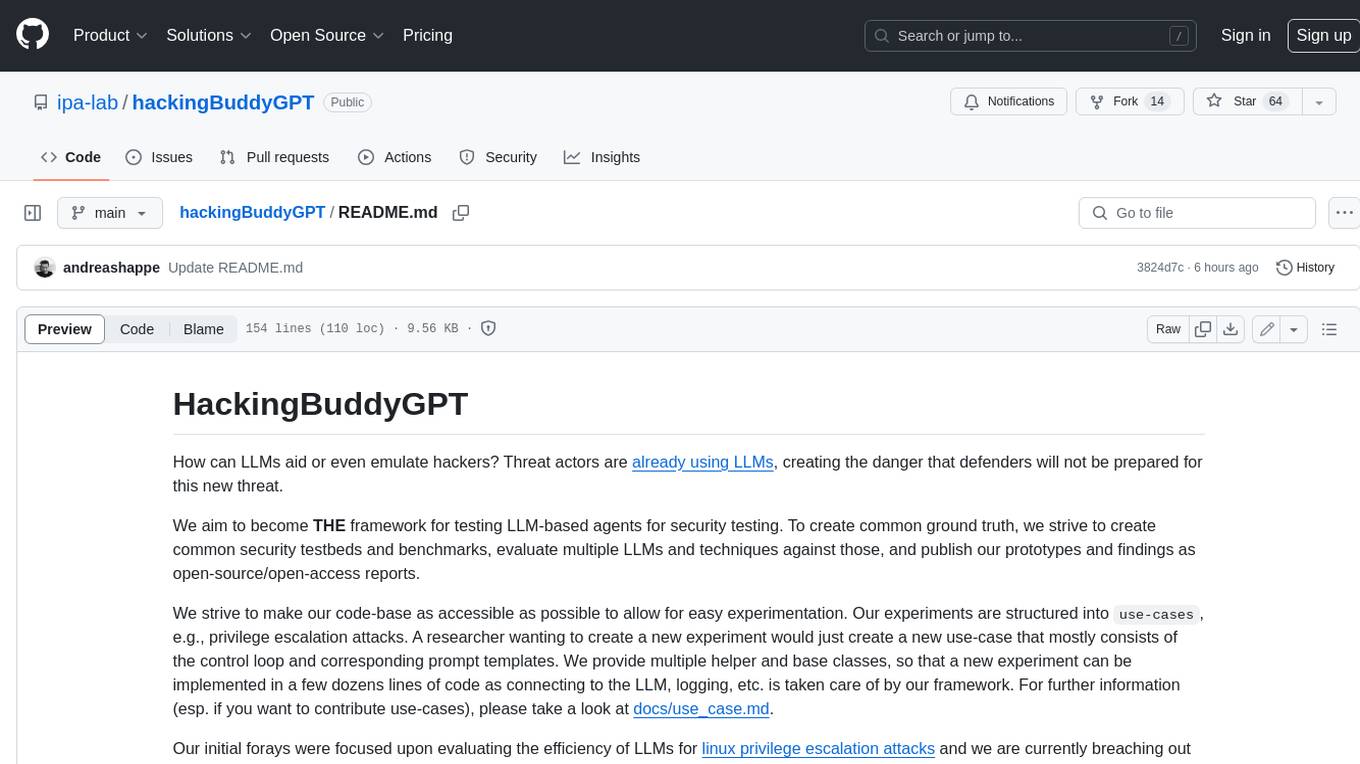
hackingBuddyGPT
hackingBuddyGPT is a framework for testing LLM-based agents for security testing. It aims to create common ground truth by creating common security testbeds and benchmarks, evaluating multiple LLMs and techniques against those, and publishing prototypes and findings as open-source/open-access reports. The initial focus is on evaluating the efficiency of LLMs for Linux privilege escalation attacks, but the framework is being expanded to evaluate the use of LLMs for web penetration-testing and web API testing. hackingBuddyGPT is released as open-source to level the playing field for blue teams against APTs that have access to more sophisticated resources.
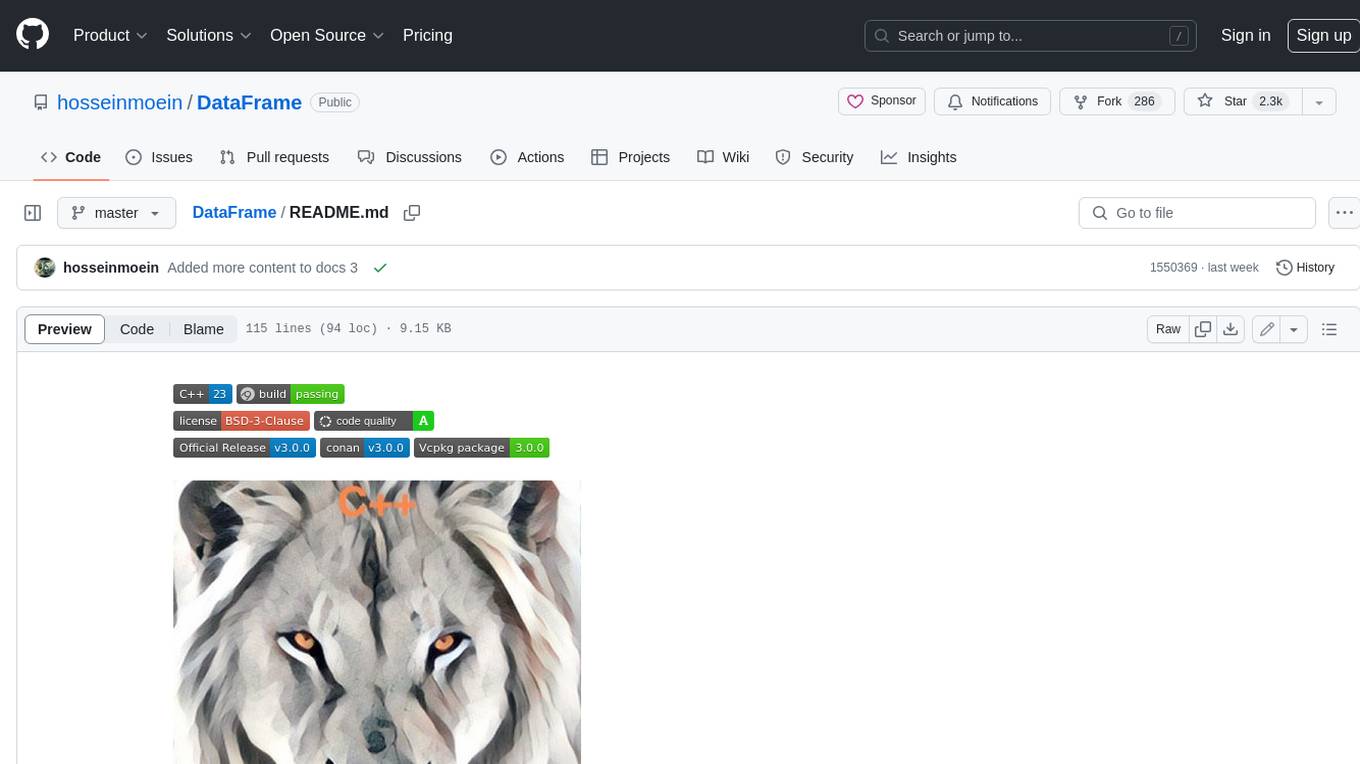
DataFrame
DataFrame is a C++ analytical library designed for data analysis similar to libraries in Python and R. It allows you to slice, join, merge, group-by, and perform various statistical, summarization, financial, and ML algorithms on your data. DataFrame also includes a large collection of analytical algorithms in form of visitors, ranging from basic stats to more involved analysis. You can easily add your own algorithms as well. DataFrame employs extensive multithreading in almost all its APIs, making it suitable for analyzing large datasets. Key principles followed in the library include supporting any type without needing new code, avoiding pointer chasing, having all column data in contiguous memory space, minimizing space usage, avoiding data copying, using multi-threading judiciously, and not protecting the user against garbage in, garbage out.
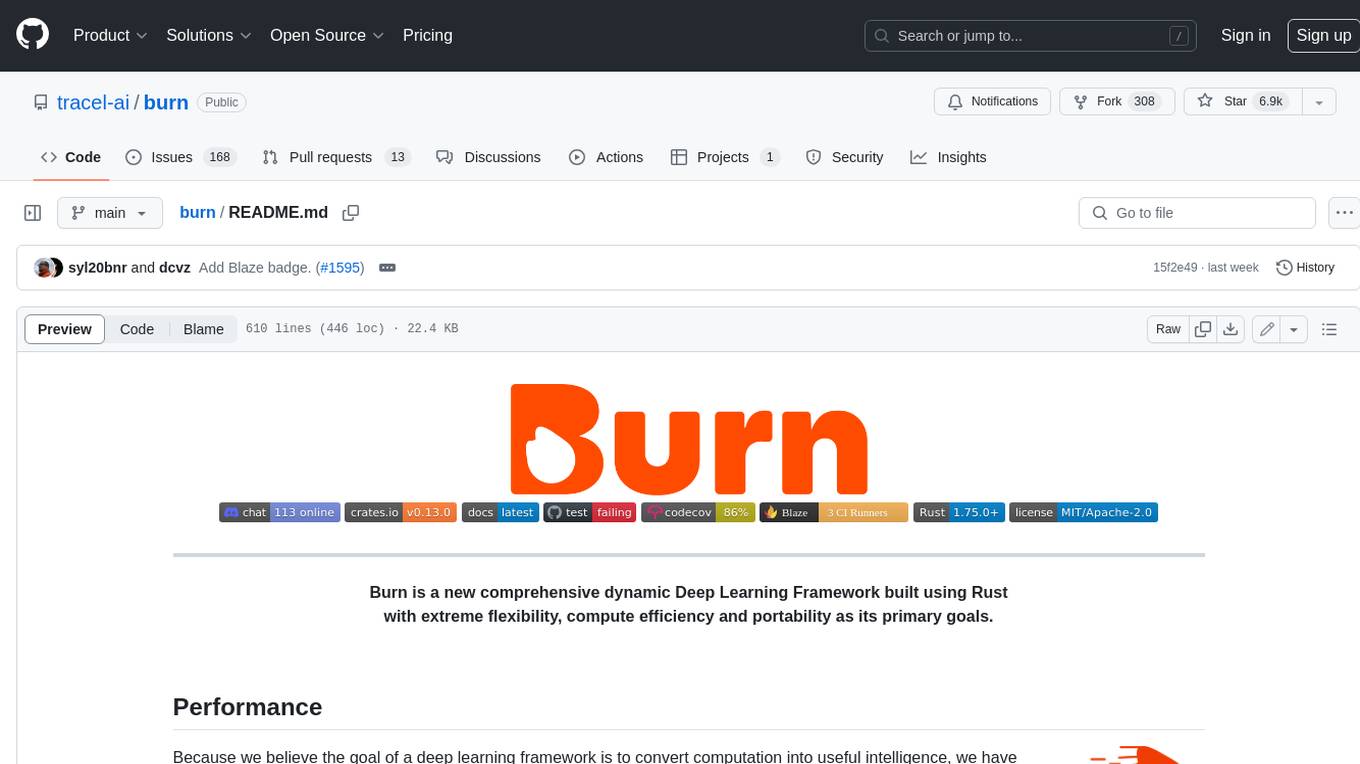
burn
Burn is a new comprehensive dynamic Deep Learning Framework built using Rust with extreme flexibility, compute efficiency and portability as its primary goals.
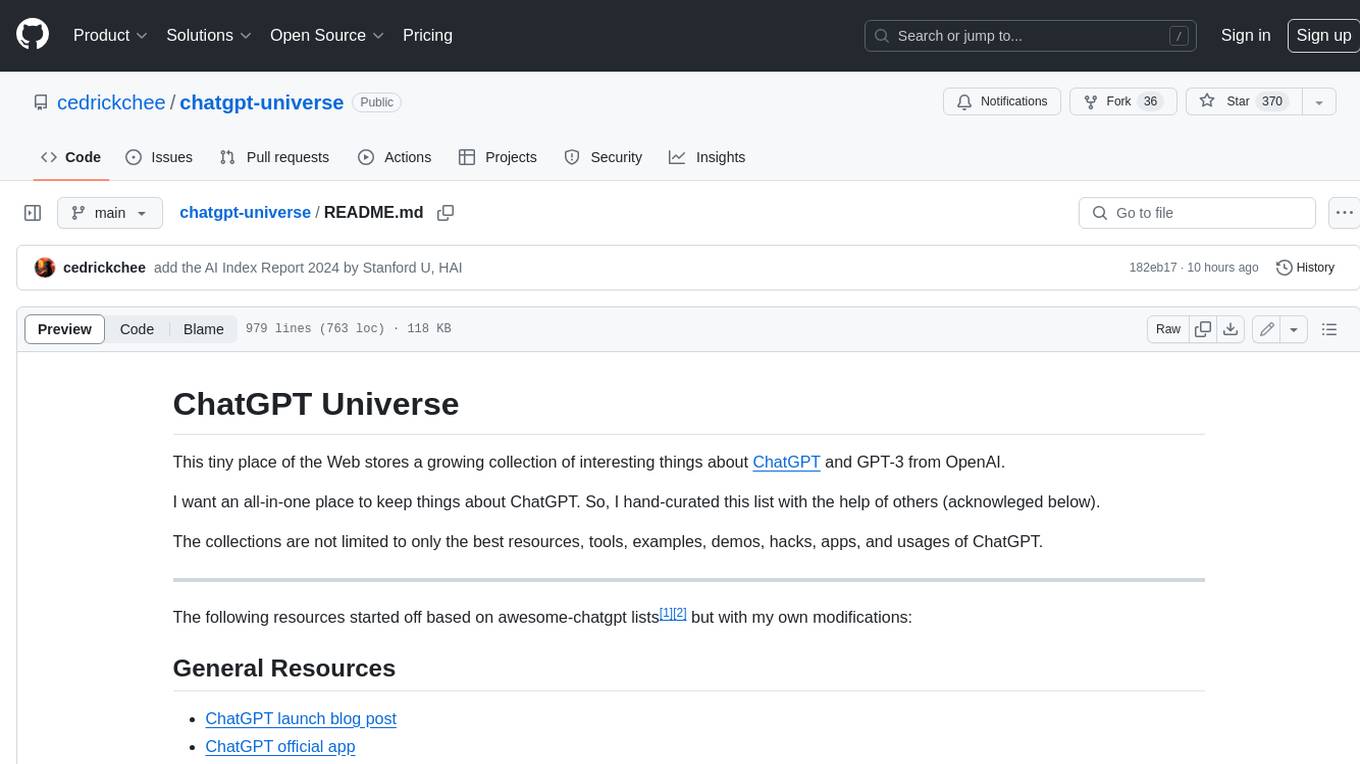
chatgpt-universe
ChatGPT is a large language model that can generate human-like text, translate languages, write different kinds of creative content, and answer your questions in a conversational way. It is trained on a massive amount of text data, and it is able to understand and respond to a wide range of natural language prompts. Here are 5 jobs suitable for this tool, in lowercase letters: 1. content writer 2. chatbot assistant 3. language translator 4. creative writer 5. researcher
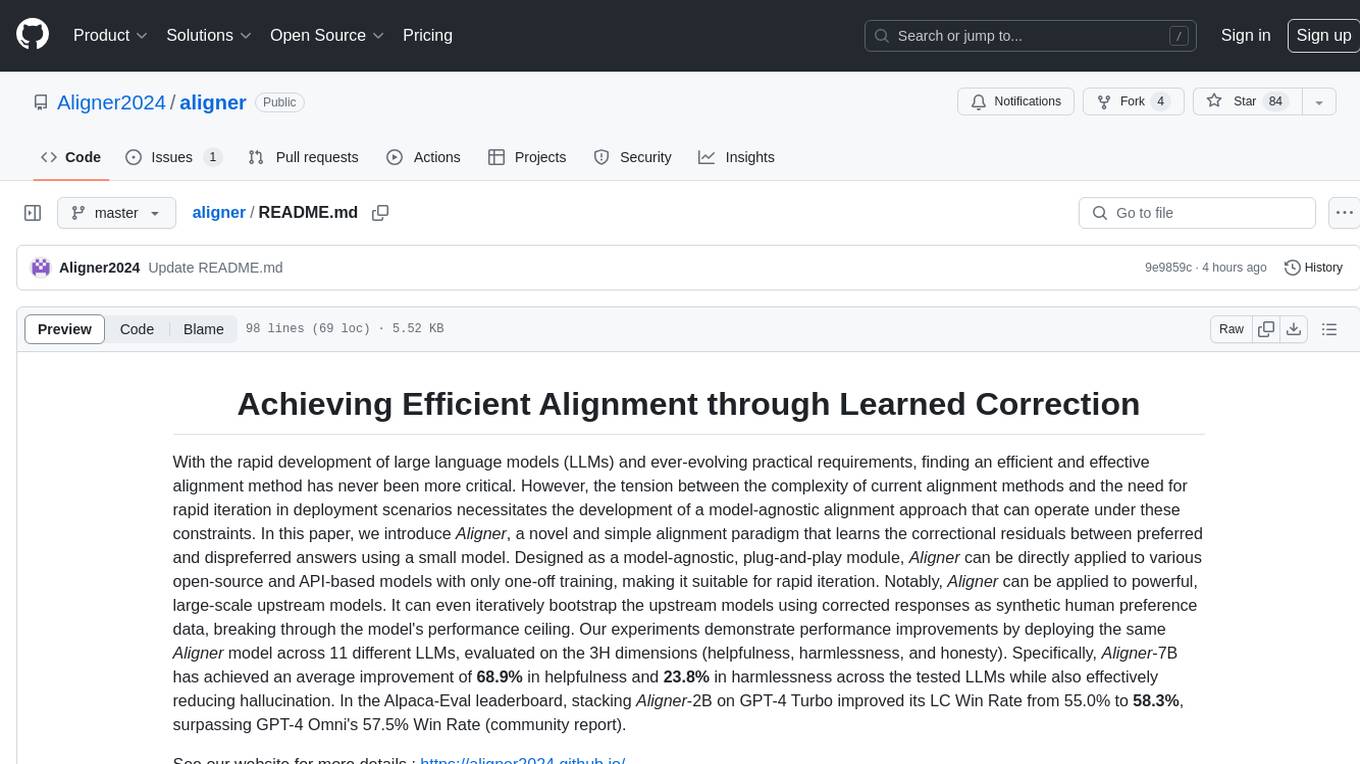
aligner
Aligner is a model-agnostic alignment tool that learns correctional residuals between preferred and dispreferred answers using a small model. It can be directly applied to various open-source and API-based models with only one-off training, suitable for rapid iteration and improving model performance. Aligner has shown significant improvements in helpfulness, harmlessness, and honesty dimensions across different large language models.
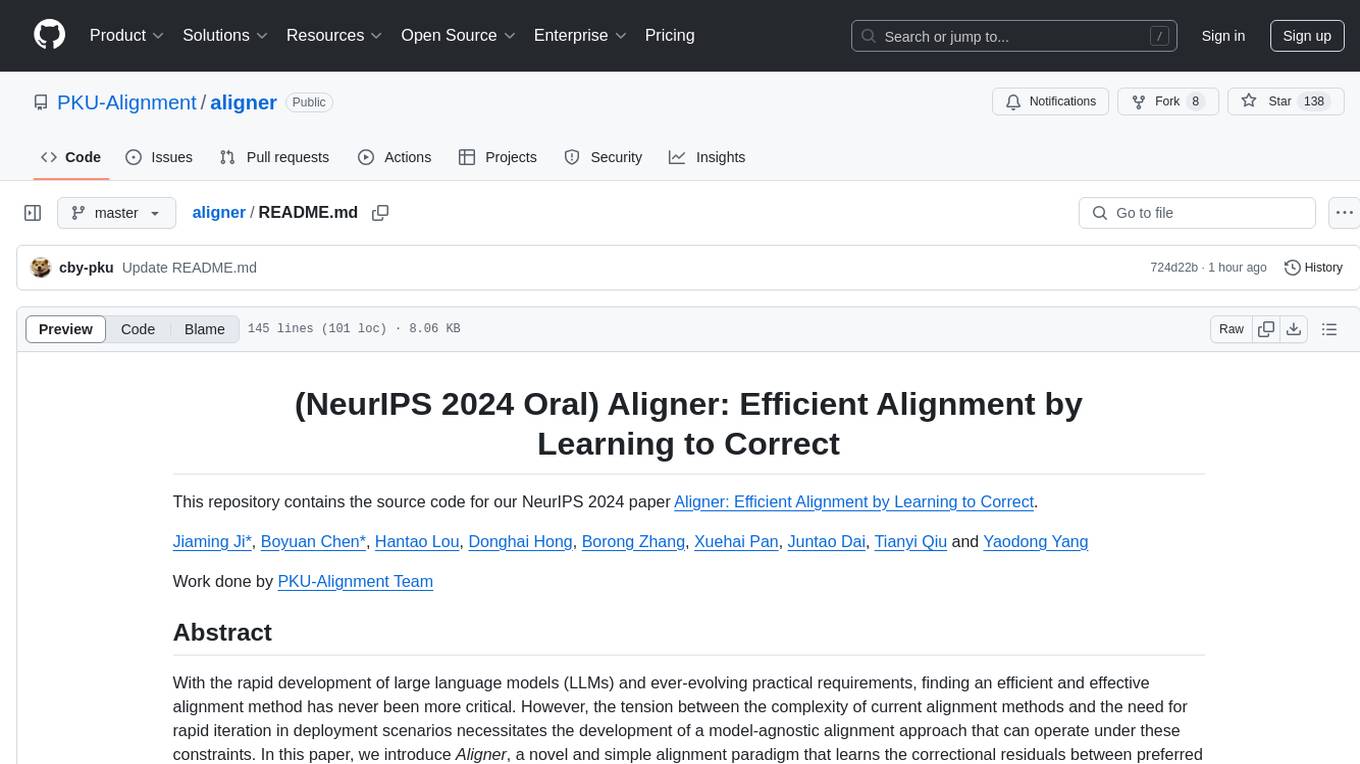
aligner
Aligner is a model-agnostic alignment tool designed to efficiently correct responses from large language models. It redistributes initial answers to align with human intentions, improving performance across various LLMs. The tool can be applied with minimal training, enhancing upstream models and reducing hallucination. Aligner's 'copy and correct' method preserves the base structure while enhancing responses. It achieves significant performance improvements in helpfulness, harmlessness, and honesty dimensions, with notable success in boosting Win Rates on evaluation leaderboards.
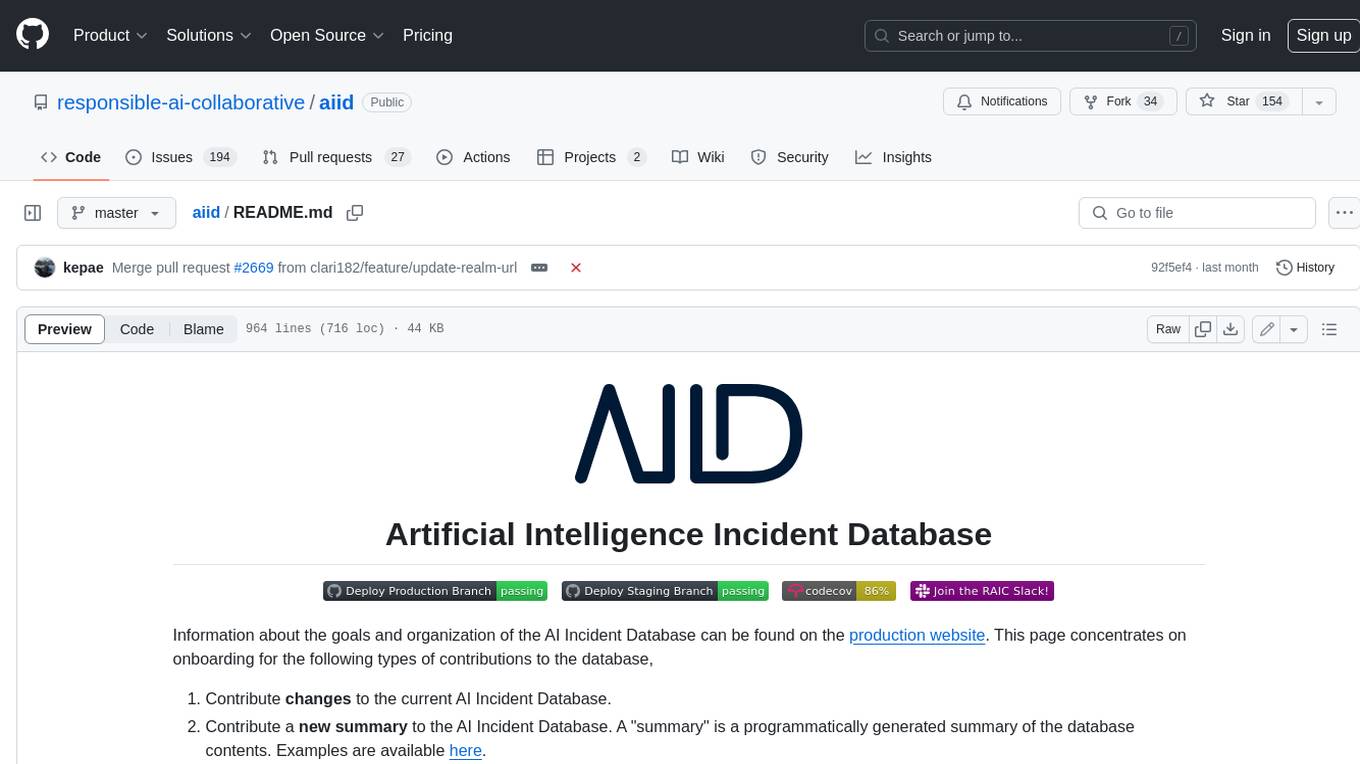
aiid
The Artificial Intelligence Incident Database (AIID) is a collection of incidents involving the development and use of artificial intelligence (AI). The database is designed to help researchers, policymakers, and the public understand the potential risks and benefits of AI, and to inform the development of policies and practices to mitigate the risks and promote the benefits of AI. The AIID is a collaborative project involving researchers from the University of California, Berkeley, the University of Washington, and the University of Toronto.
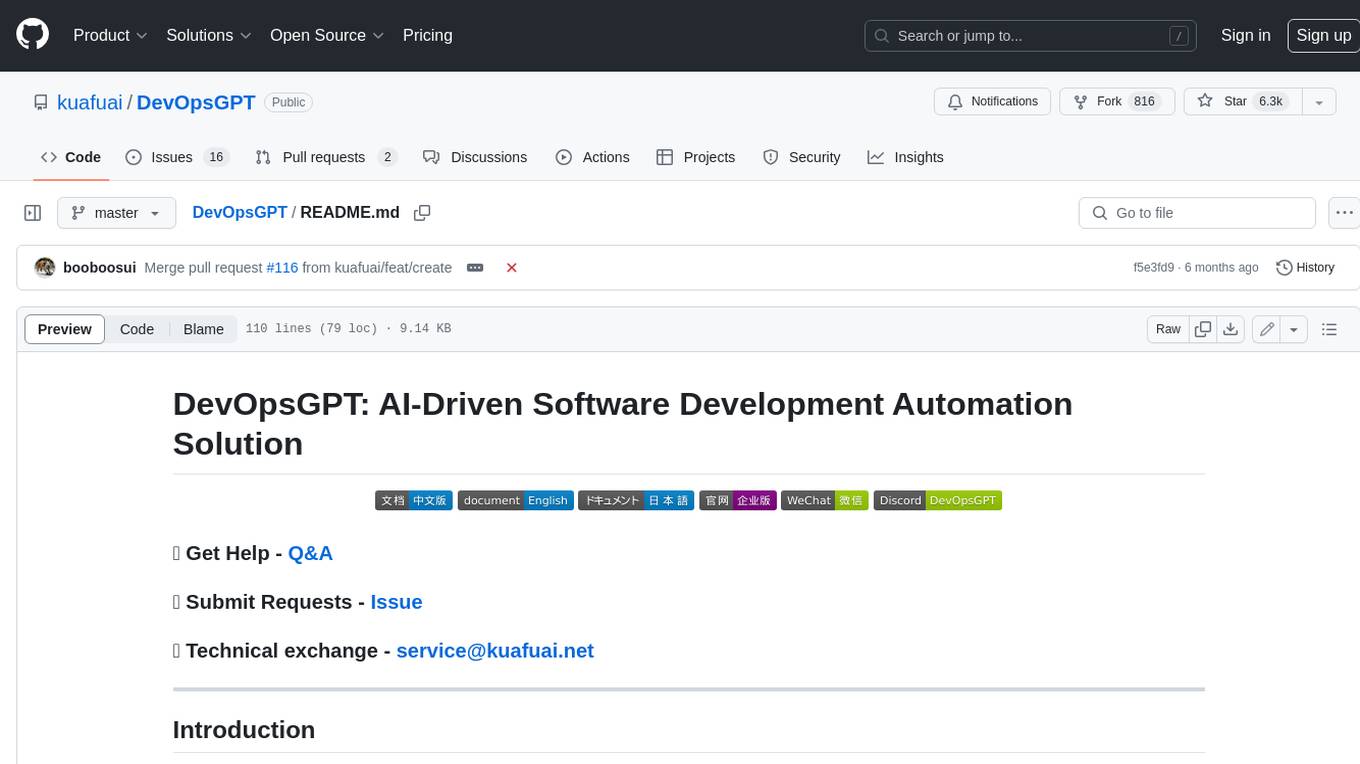
DevOpsGPT
DevOpsGPT is an AI-driven software development automation solution that combines Large Language Models (LLM) with DevOps tools to convert natural language requirements into working software. It improves development efficiency by eliminating the need for tedious requirement documentation, shortens development cycles, reduces communication costs, and ensures high-quality deliverables. The Enterprise Edition offers features like existing project analysis, professional model selection, and support for more DevOps platforms. The tool automates requirement development, generates interface documentation, provides pseudocode based on existing projects, facilitates code refinement, enables continuous integration, and supports software version release. Users can run DevOpsGPT with source code or Docker, and the tool comes with limitations in precise documentation generation and understanding existing project code. The product roadmap includes accurate requirement decomposition, rapid import of development requirements, and integration of more software engineering and professional tools for efficient software development tasks under AI planning and execution.
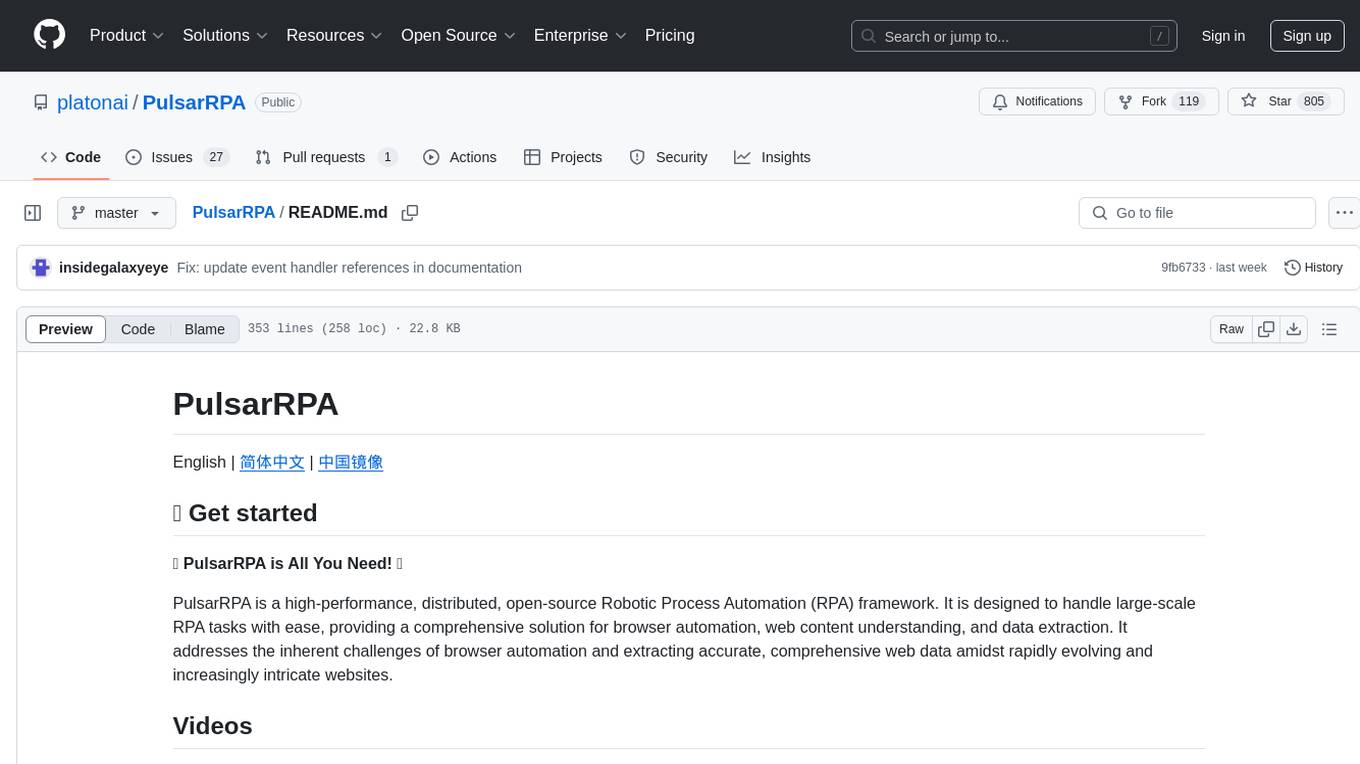
PulsarRPA
PulsarRPA is a high-performance, distributed, open-source Robotic Process Automation (RPA) framework designed to handle large-scale RPA tasks with ease. It provides a comprehensive solution for browser automation, web content understanding, and data extraction. PulsarRPA addresses challenges of browser automation and accurate web data extraction from complex and evolving websites. It incorporates innovative technologies like browser rendering, RPA, intelligent scraping, advanced DOM parsing, and distributed architecture to ensure efficient, accurate, and scalable web data extraction. The tool is open-source, customizable, and supports cutting-edge information extraction technology, making it a preferred solution for large-scale web data extraction.
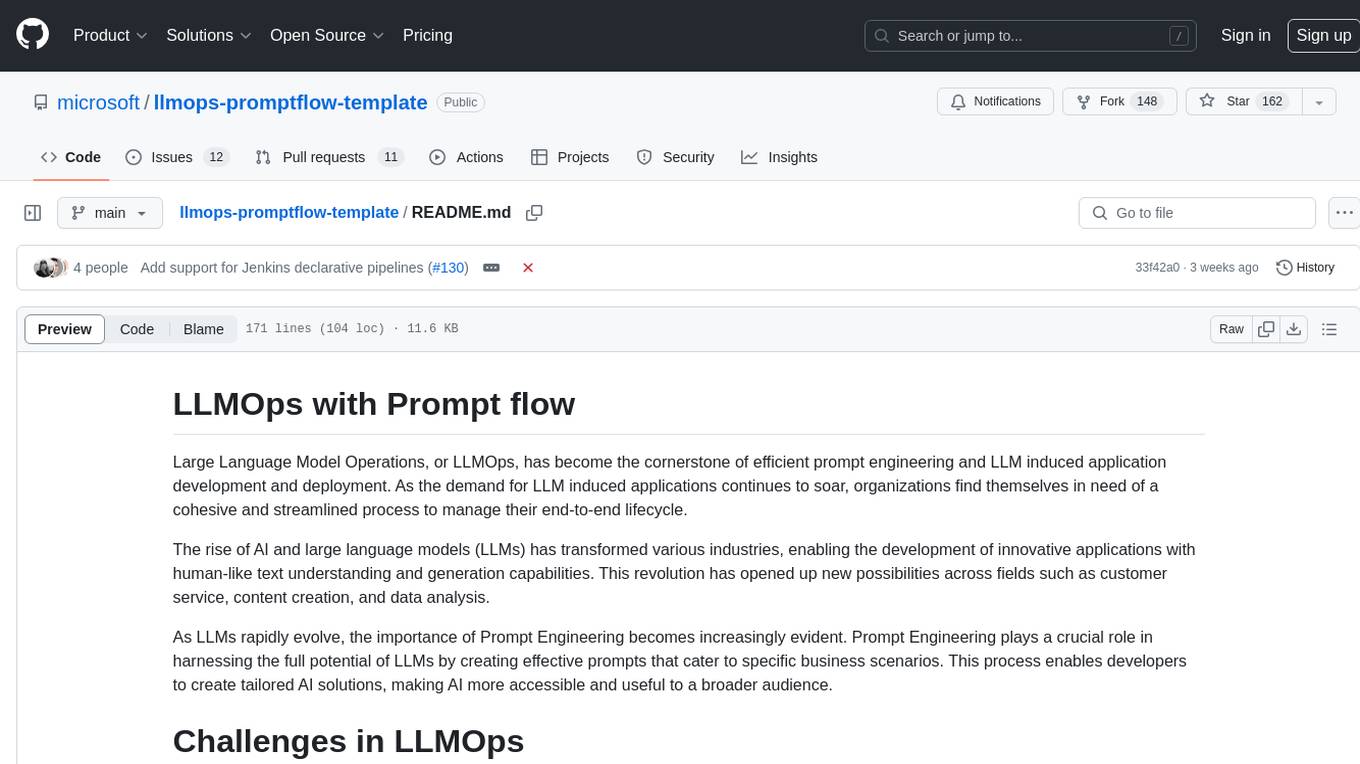
llmops-promptflow-template
LLMOps with Prompt flow is a template and guidance for building LLM-infused apps using Prompt flow. It provides centralized code hosting, lifecycle management, variant and hyperparameter experimentation, A/B deployment, many-to-many dataset/flow relationships, multiple deployment targets, comprehensive reporting, BYOF capabilities, configuration-based development, local prompt experimentation and evaluation, endpoint testing, and optional Human-in-loop validation. The tool is customizable to suit various application needs.
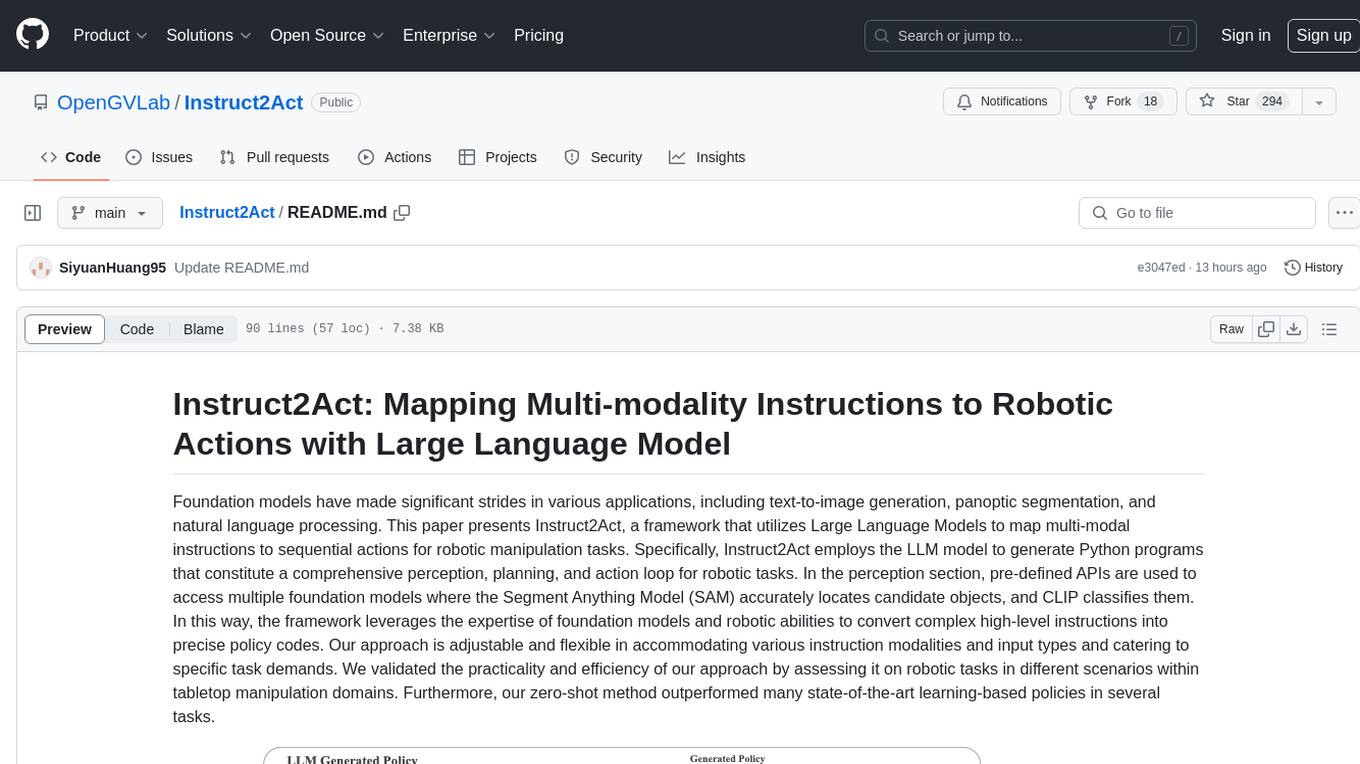
Instruct2Act
Instruct2Act is a framework that utilizes Large Language Models to map multi-modal instructions to sequential actions for robotic manipulation tasks. It generates Python programs using the LLM model for perception, planning, and action. The framework leverages foundation models like SAM and CLIP to convert high-level instructions into policy codes, accommodating various instruction modalities and task demands. Instruct2Act has been validated on robotic tasks in tabletop manipulation domains, outperforming learning-based policies in several tasks.
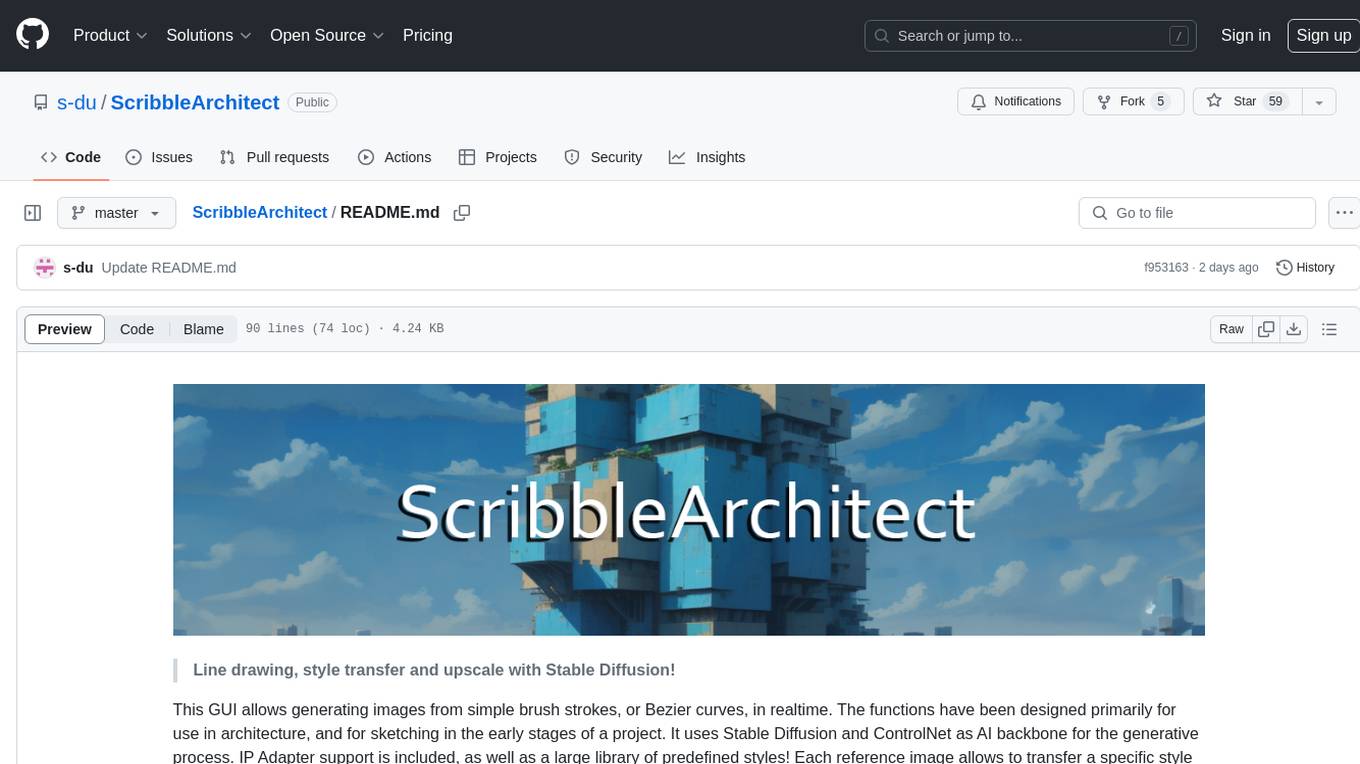
ScribbleArchitect
ScribbleArchitect is a GUI tool designed for generating images from simple brush strokes or Bezier curves in real-time. It is primarily intended for use in architecture and sketching in the early stages of a project. The tool utilizes Stable Diffusion and ControlNet as AI backbone for the generative process, with IP Adapter support and a library of predefined styles. Users can transfer specific styles to their line work, upscale images for high resolution export, and utilize a ControlNet upscaler. The tool also features a screen capture function for working with external tools like Adobe Illustrator or Inkscape.
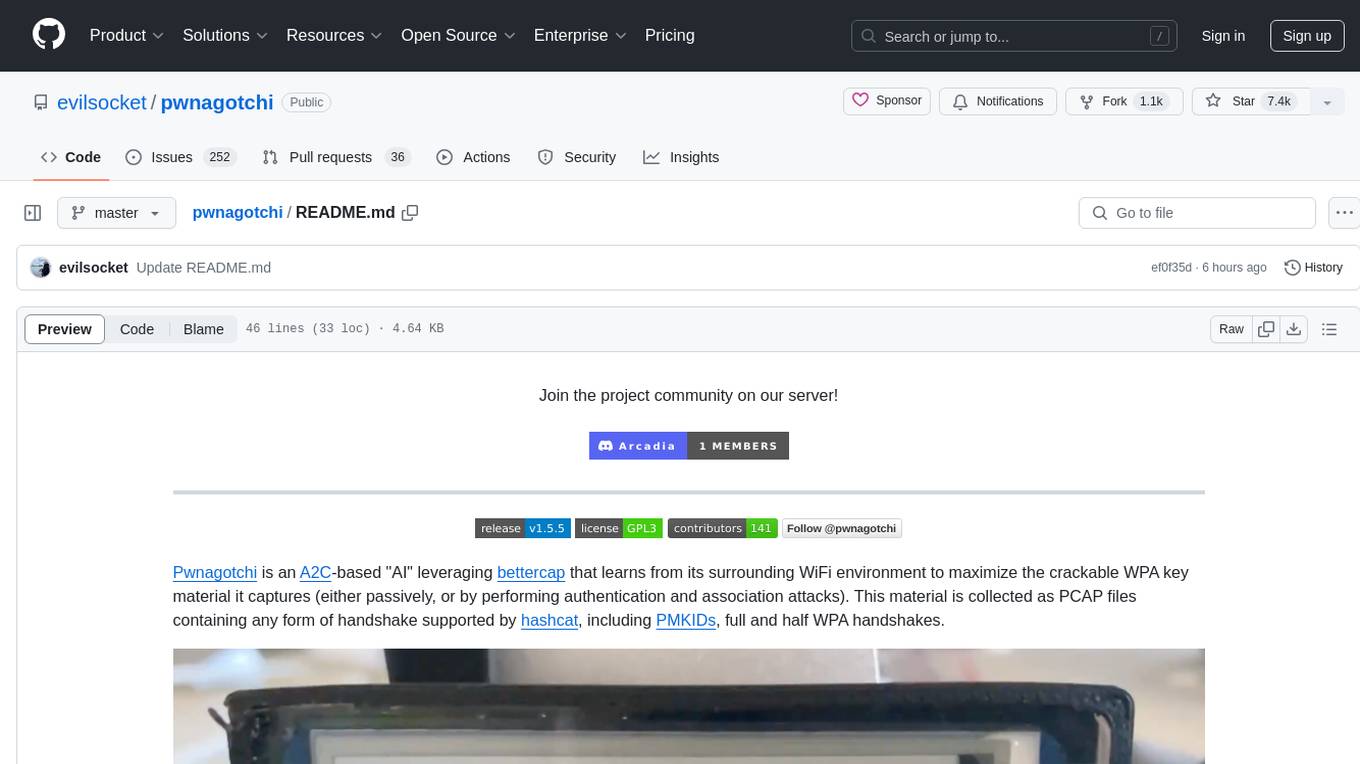
pwnagotchi
Pwnagotchi is an AI tool leveraging bettercap to learn from WiFi environments and maximize crackable WPA key material. It uses LSTM with MLP feature extractor for A2C agent, learning over epochs to improve performance in various WiFi environments. Units can cooperate using a custom parasite protocol. Visit https://www.pwnagotchi.ai for documentation and community links.
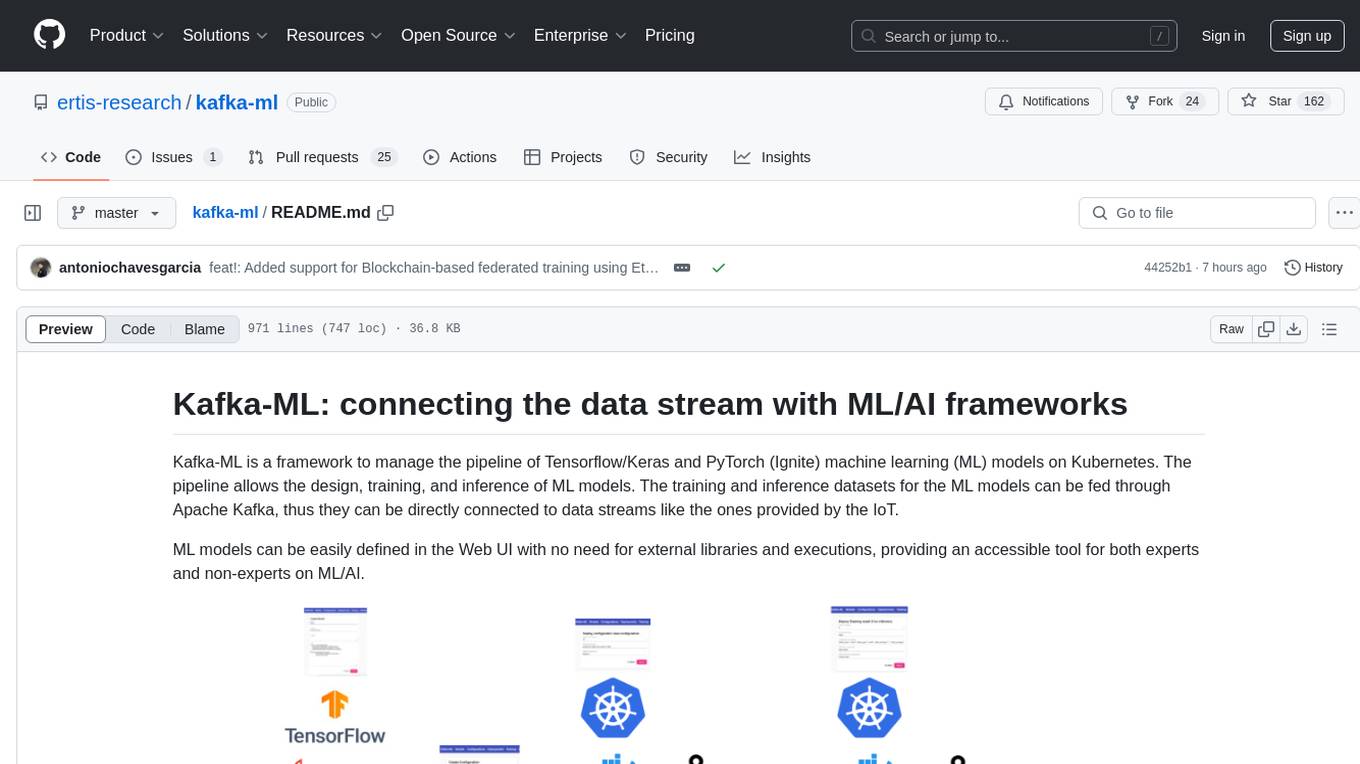
kafka-ml
Kafka-ML is a framework designed to manage the pipeline of Tensorflow/Keras and PyTorch machine learning models on Kubernetes. It enables the design, training, and inference of ML models with datasets fed through Apache Kafka, connecting them directly to data streams like those from IoT devices. The Web UI allows easy definition of ML models without external libraries, catering to both experts and non-experts in ML/AI.
For similar tasks
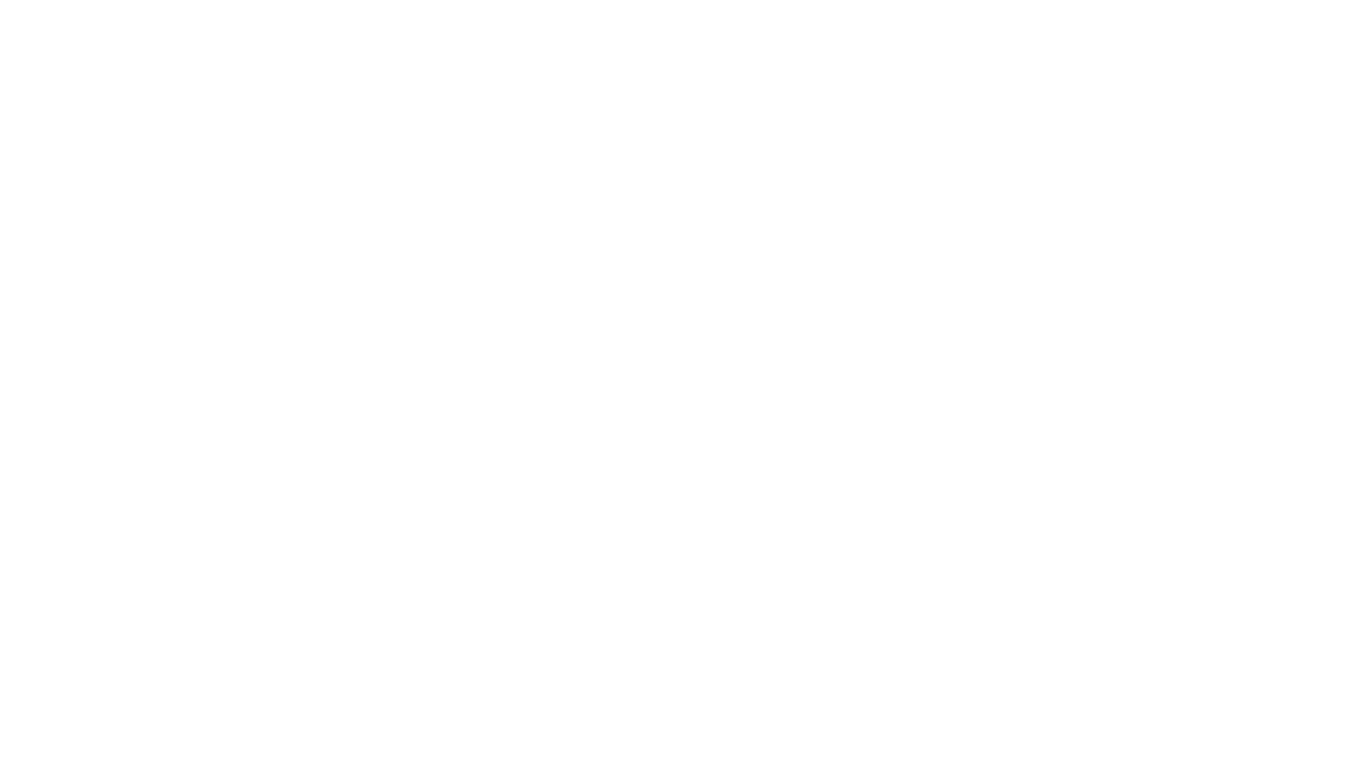
EvoMaster
EvoMaster is an open-source AI-driven tool that automatically generates system-level test cases for web/enterprise applications. It uses Evolutionary Algorithm and Dynamic Program Analysis to evolve test cases, maximizing code coverage and fault detection. It supports REST, GraphQL, and RPC APIs, with whitebox testing for JVM-compiled APIs. The tool generates JUnit tests in Java or Kotlin, focusing on fault detection, self-contained tests, SQL handling, and authentication. Known limitations include manual driver creation for whitebox testing and longer execution times for better results. EvoMaster has been funded by ERC and RCN grants.
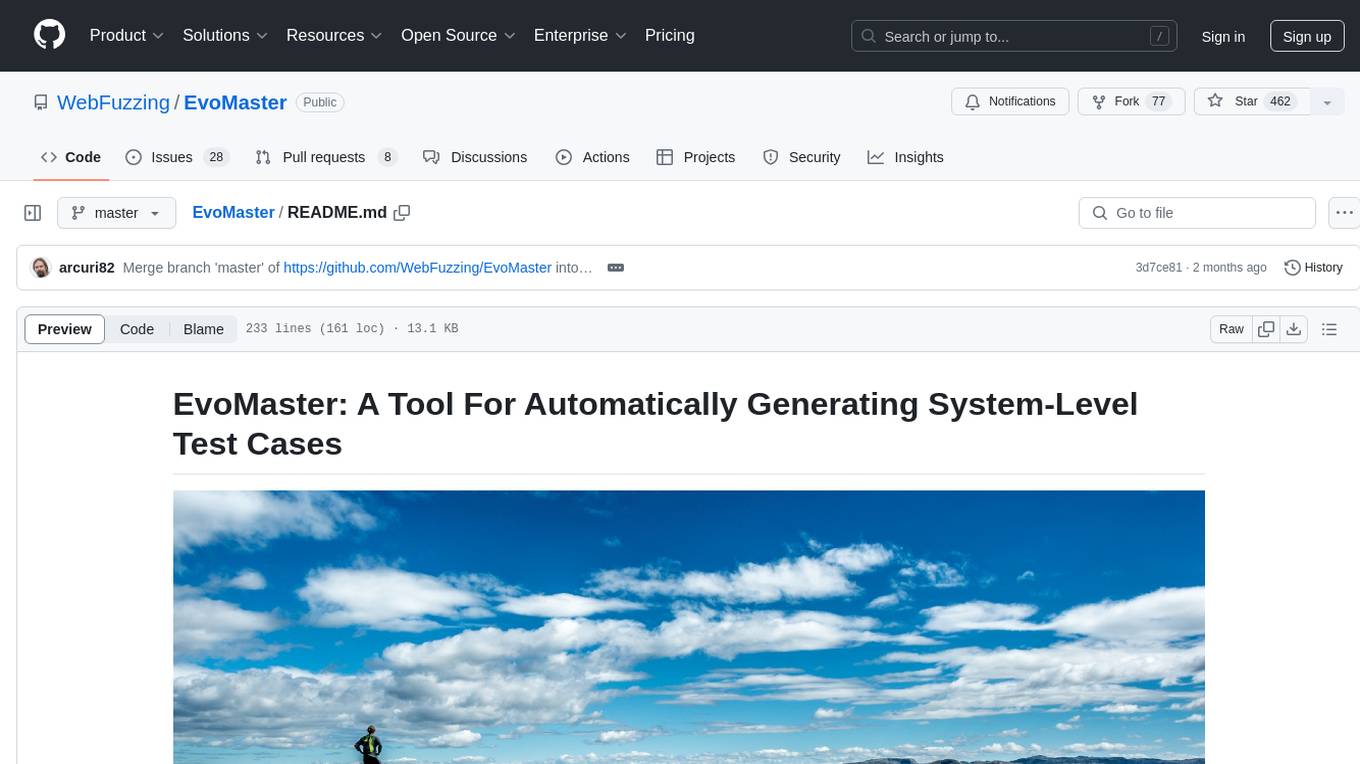
EvoMaster
EvoMaster is an open-source AI-driven tool that automatically generates system-level test cases for web/enterprise applications. It uses an Evolutionary Algorithm and Dynamic Program Analysis to evolve test cases, maximizing code coverage and fault detection. The tool supports REST, GraphQL, and RPC APIs, with whitebox testing for JVM-compiled languages. It generates JUnit tests, detects faults, handles SQL databases, and supports authentication. EvoMaster has been funded by the European Research Council and the Research Council of Norway.
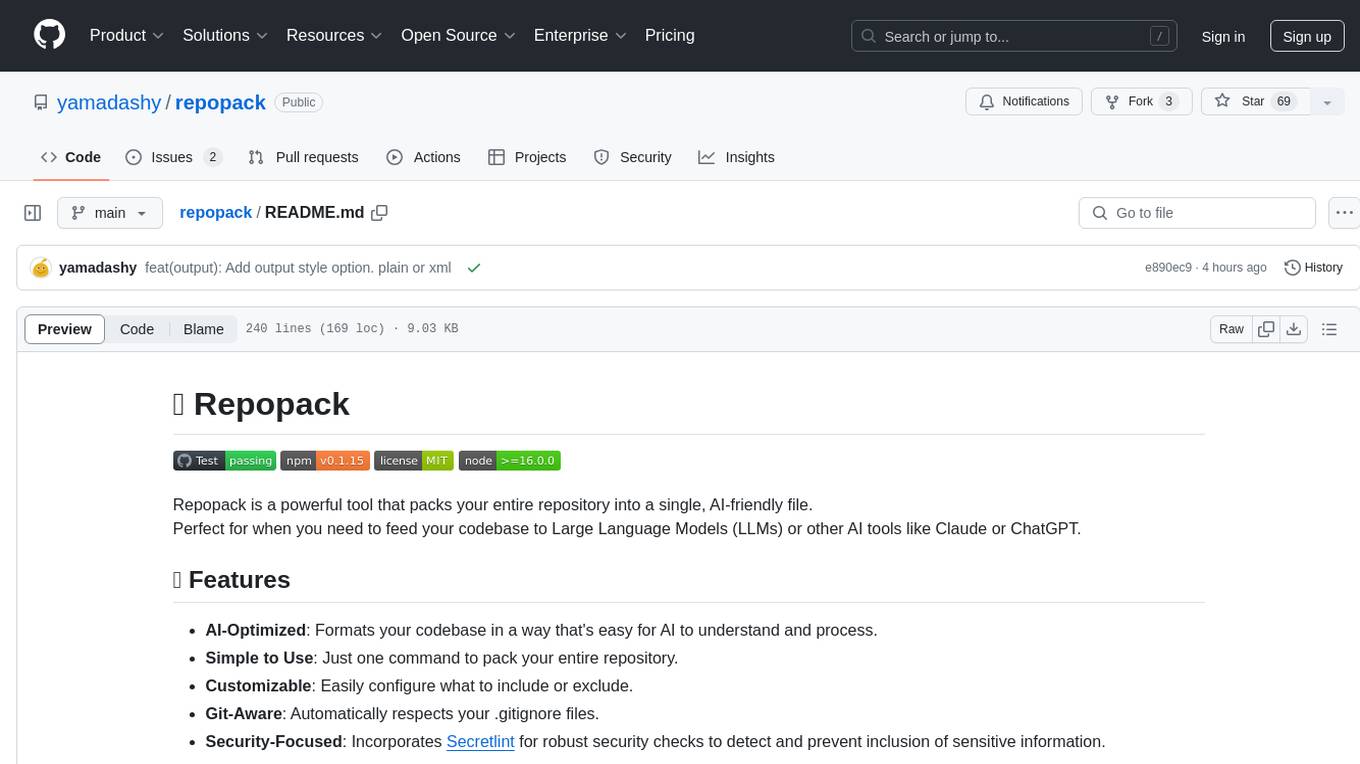
repopack
Repopack is a powerful tool that packs your entire repository into a single, AI-friendly file. It optimizes your codebase for AI comprehension, is simple to use with customizable options, and respects Gitignore files for security. The tool generates a packed file with clear separators and AI-oriented explanations, making it ideal for use with Generative AI tools like Claude or ChatGPT. Repopack offers command line options, configuration settings, and multiple methods for setting ignore patterns to exclude specific files or directories during the packing process. It includes features like comment removal for supported file types and a security check using Secretlint to detect sensitive information in files.
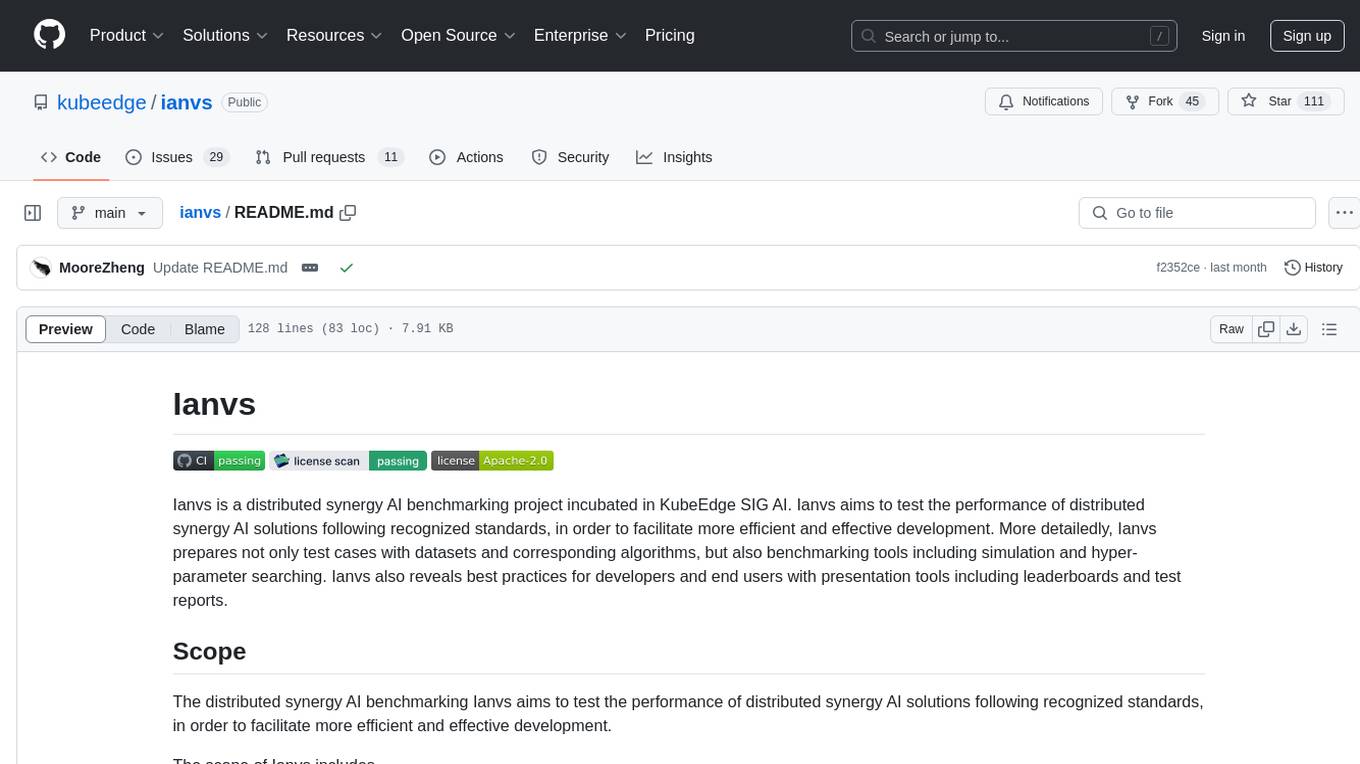
ianvs
Ianvs is a distributed synergy AI benchmarking project incubated in KubeEdge SIG AI. It aims to test the performance of distributed synergy AI solutions following recognized standards, providing end-to-end benchmark toolkits, test environment management tools, test case control tools, and benchmark presentation tools. It also collaborates with other organizations to establish comprehensive benchmarks and related applications. The architecture includes critical components like Test Environment Manager, Test Case Controller, Generation Assistant, Simulation Controller, and Story Manager. Ianvs documentation covers quick start, guides, dataset descriptions, algorithms, user interfaces, stories, and roadmap.
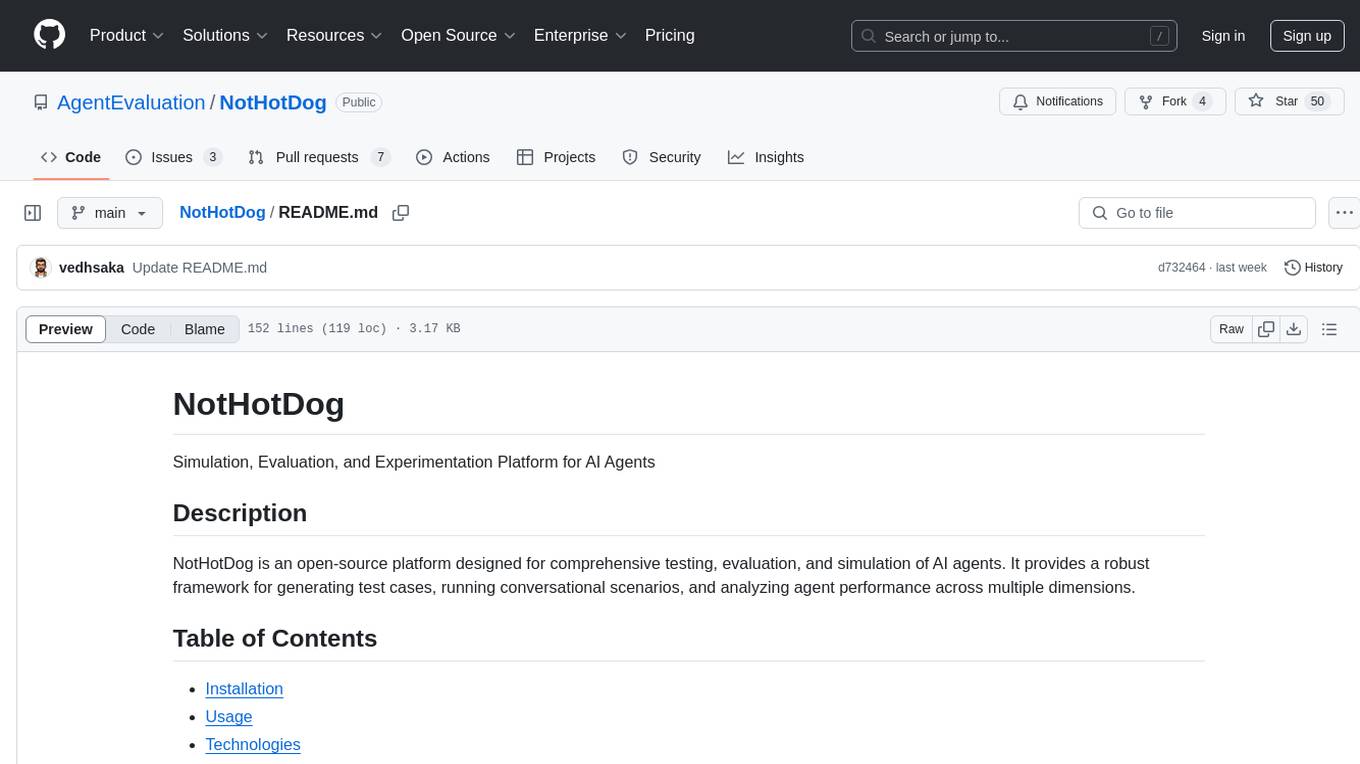
NotHotDog
NotHotDog is an open-source platform for testing, evaluating, and simulating AI agents. It offers a robust framework for generating test cases, running conversational scenarios, and analyzing agent performance.
For similar jobs
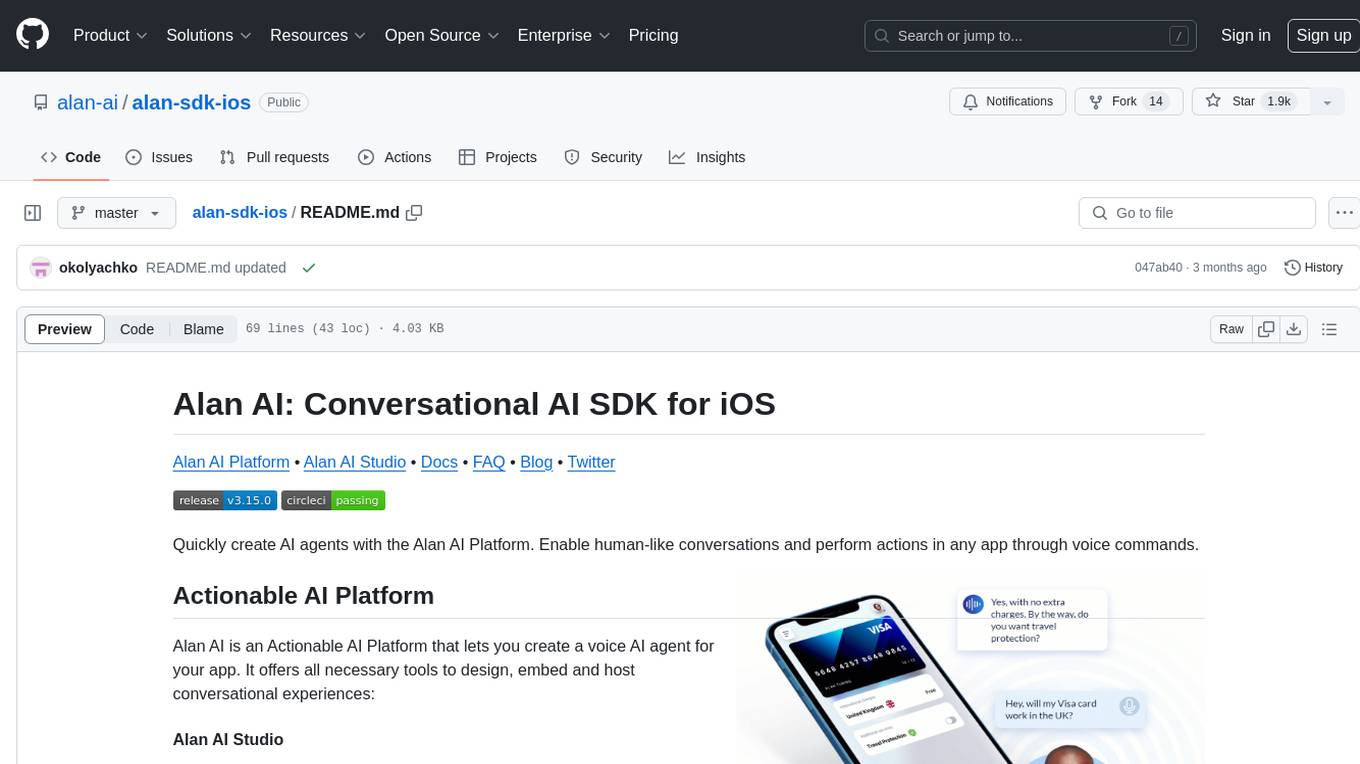
alan-sdk-ios
Alan AI SDK for iOS is a powerful tool that allows developers to quickly create AI agents for their iOS apps. With Alan AI Platform, users can easily design, embed, and host conversational experiences in their applications. The platform offers a web-based IDE called Alan AI Studio for creating dialog scenarios, lightweight SDKs for embedding AI agents, and a backend powered by top-notch speech recognition and natural language understanding technologies. Alan AI enables human-like conversations and actions through voice commands, with features like on-the-fly updates, dialog flow testing, and analytics.
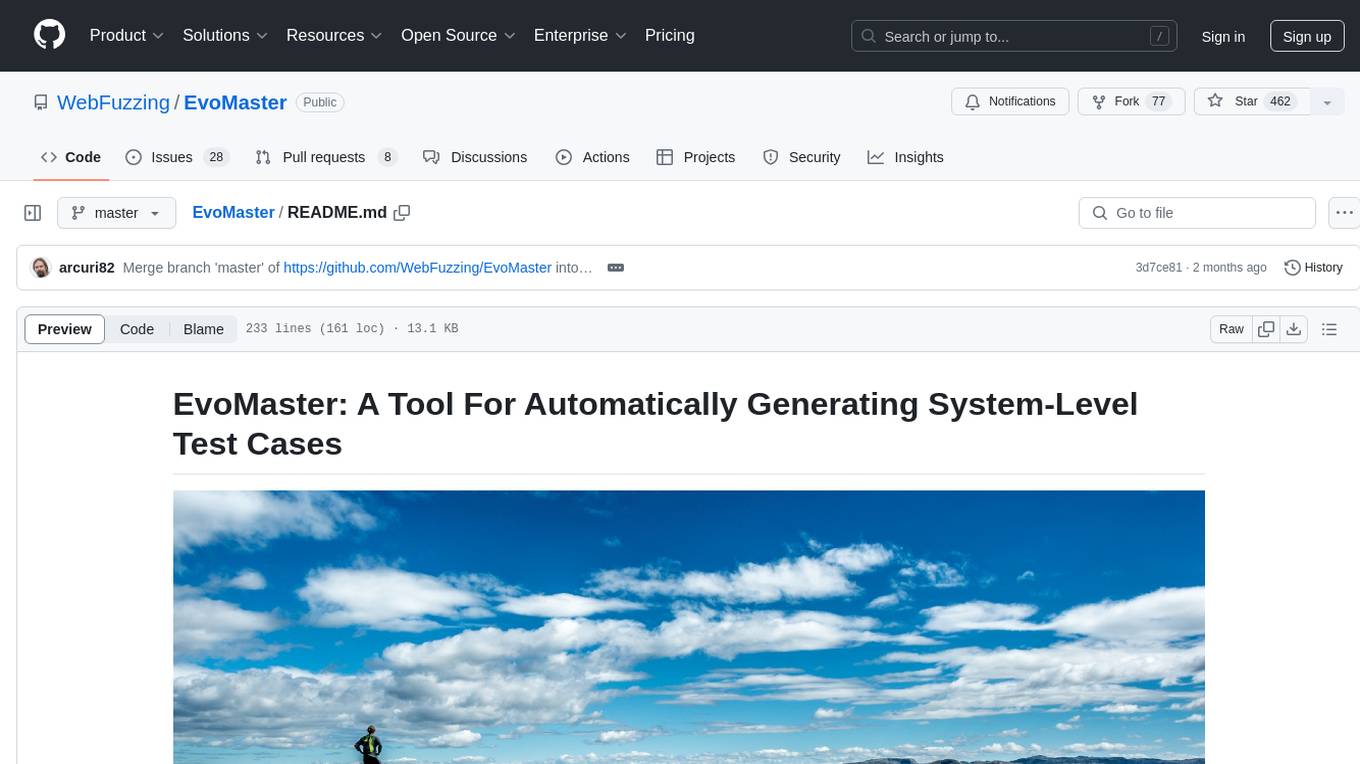
EvoMaster
EvoMaster is an open-source AI-driven tool that automatically generates system-level test cases for web/enterprise applications. It uses an Evolutionary Algorithm and Dynamic Program Analysis to evolve test cases, maximizing code coverage and fault detection. The tool supports REST, GraphQL, and RPC APIs, with whitebox testing for JVM-compiled languages. It generates JUnit tests, detects faults, handles SQL databases, and supports authentication. EvoMaster has been funded by the European Research Council and the Research Council of Norway.
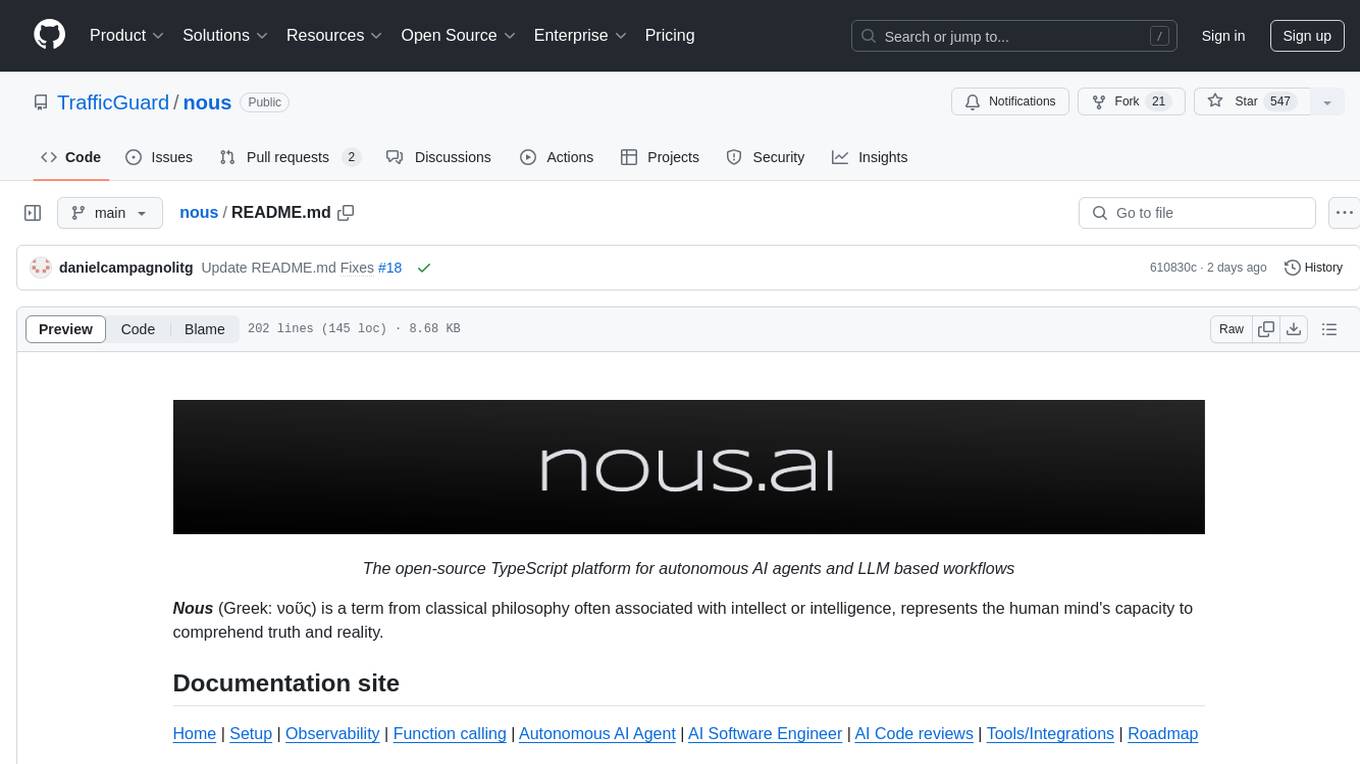
nous
Nous is an open-source TypeScript platform for autonomous AI agents and LLM based workflows. It aims to automate processes, support requests, review code, assist with refactorings, and more. The platform supports various integrations, multiple LLMs/services, CLI and web interface, human-in-the-loop interactions, flexible deployment options, observability with OpenTelemetry tracing, and specific agents for code editing, software engineering, and code review. It offers advanced features like reasoning/planning, memory and function call history, hierarchical task decomposition, and control-loop function calling options. Nous is designed to be a flexible platform for the TypeScript community to expand and support different use cases and integrations.
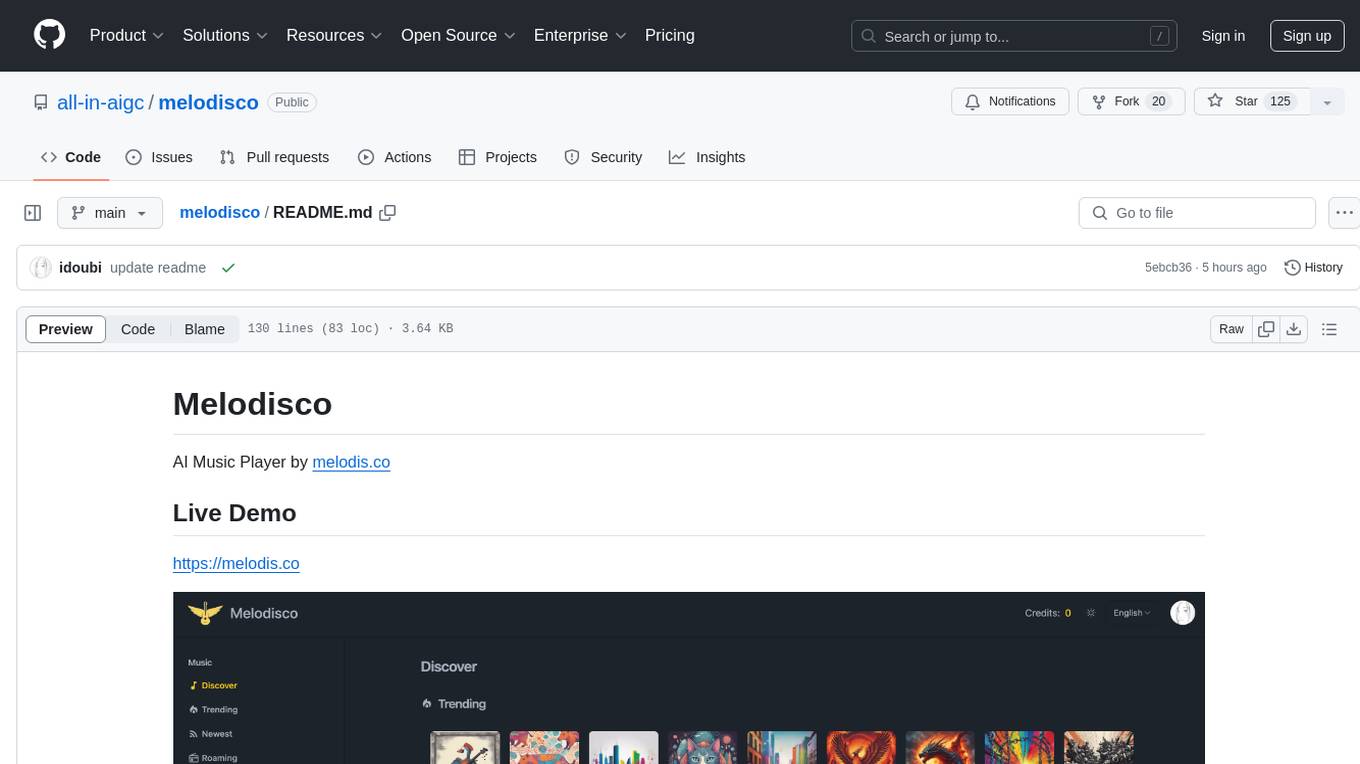
melodisco
Melodisco is an AI music player that allows users to listen to music and manage playlists. It provides a user-friendly interface for music playback and organization. Users can deploy Melodisco with Vercel or Docker for easy setup. Local development instructions are provided for setting up the project environment. The project credits various tools and libraries used in its development, such as Next.js, Tailwind CSS, and Stripe. Melodisco is a versatile tool for music enthusiasts looking for an AI-powered music player with features like authentication, payment integration, and multi-language support.
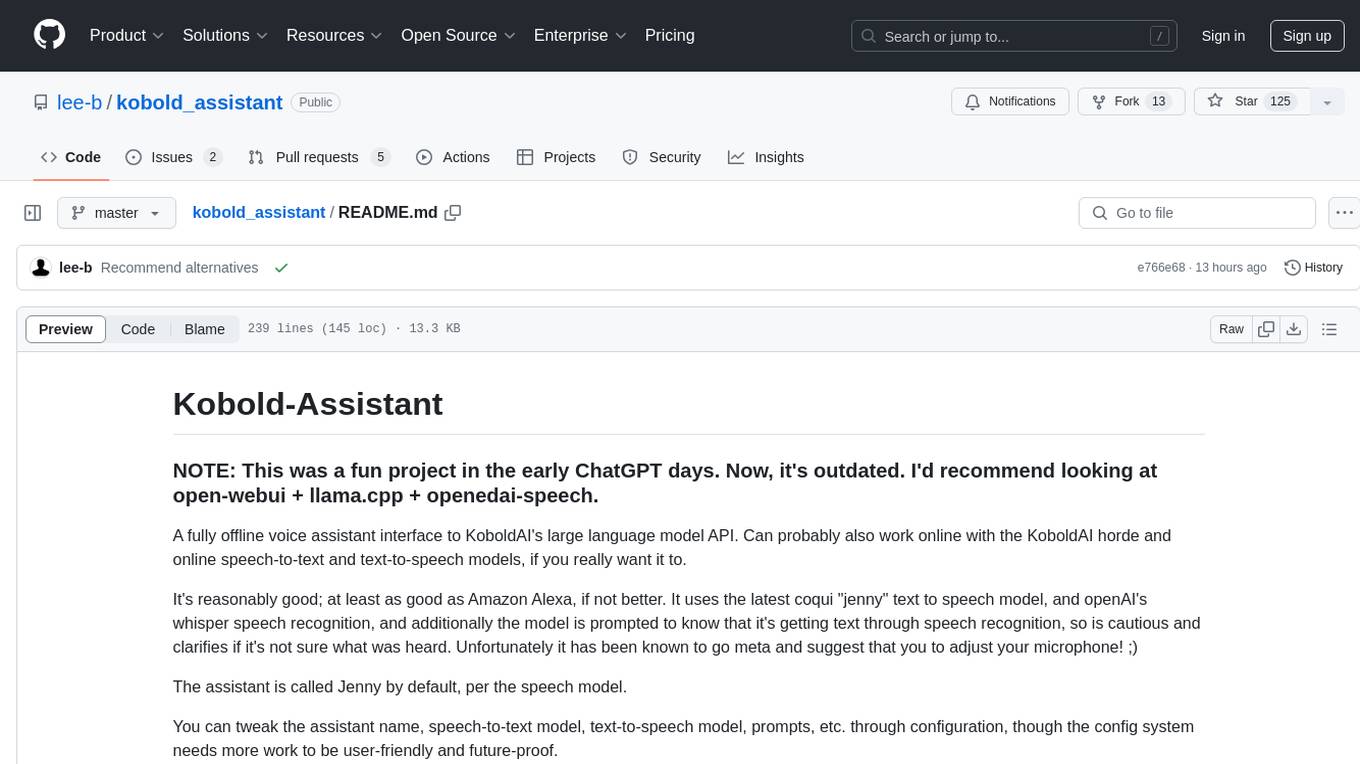
kobold_assistant
Kobold-Assistant is a fully offline voice assistant interface to KoboldAI's large language model API. It can work online with the KoboldAI horde and online speech-to-text and text-to-speech models. The assistant, called Jenny by default, uses the latest coqui 'jenny' text to speech model and openAI's whisper speech recognition. Users can customize the assistant name, speech-to-text model, text-to-speech model, and prompts through configuration. The tool requires system packages like GCC, portaudio development libraries, and ffmpeg, along with Python >=3.7, <3.11, and runs on Ubuntu/Debian systems. Users can interact with the assistant through commands like 'serve' and 'list-mics'.
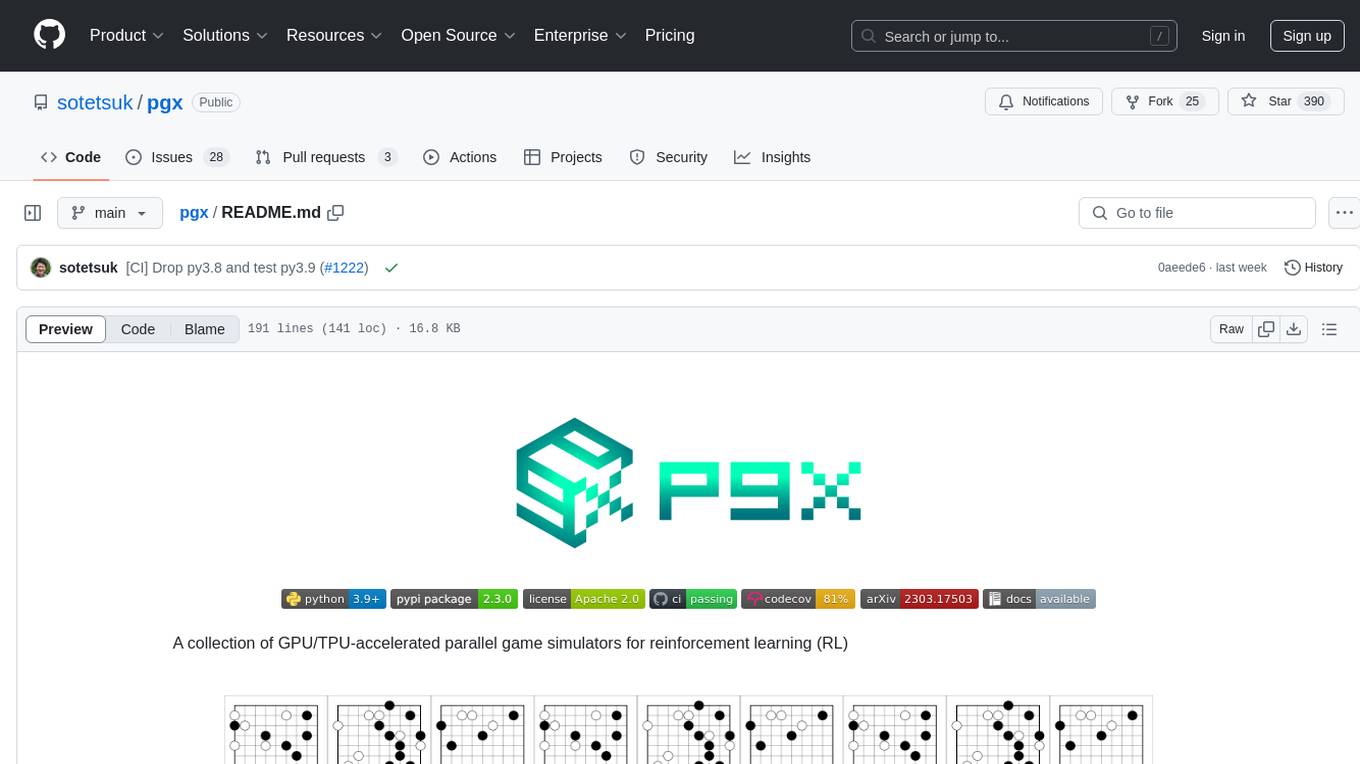
pgx
Pgx is a collection of GPU/TPU-accelerated parallel game simulators for reinforcement learning (RL). It provides JAX-native game simulators for various games like Backgammon, Chess, Shogi, and Go, offering super fast parallel execution on accelerators and beautiful visualization in SVG format. Pgx focuses on faster implementations while also being sufficiently general, allowing environments to be converted to the AEC API of PettingZoo for running Pgx environments through the PettingZoo API.
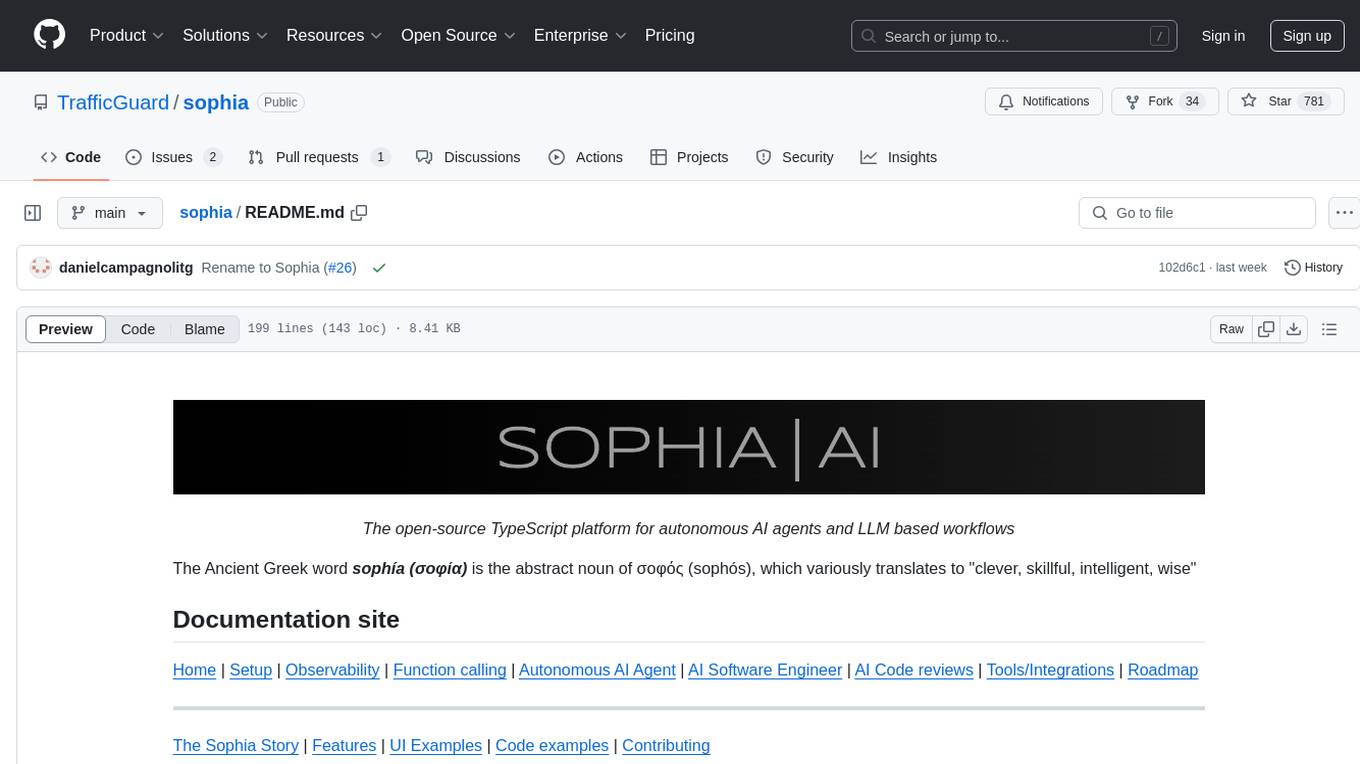
sophia
Sophia is an open-source TypeScript platform designed for autonomous AI agents and LLM based workflows. It aims to automate processes, review code, assist with refactorings, and support various integrations. The platform offers features like advanced autonomous agents, reasoning/planning inspired by Google's Self-Discover paper, memory and function call history, adaptive iterative planning, and more. Sophia supports multiple LLMs/services, CLI and web interface, human-in-the-loop interactions, flexible deployment options, observability with OpenTelemetry tracing, and specific agents for code editing, software engineering, and code review. It provides a flexible platform for the TypeScript community to expand and support various use cases and integrations.
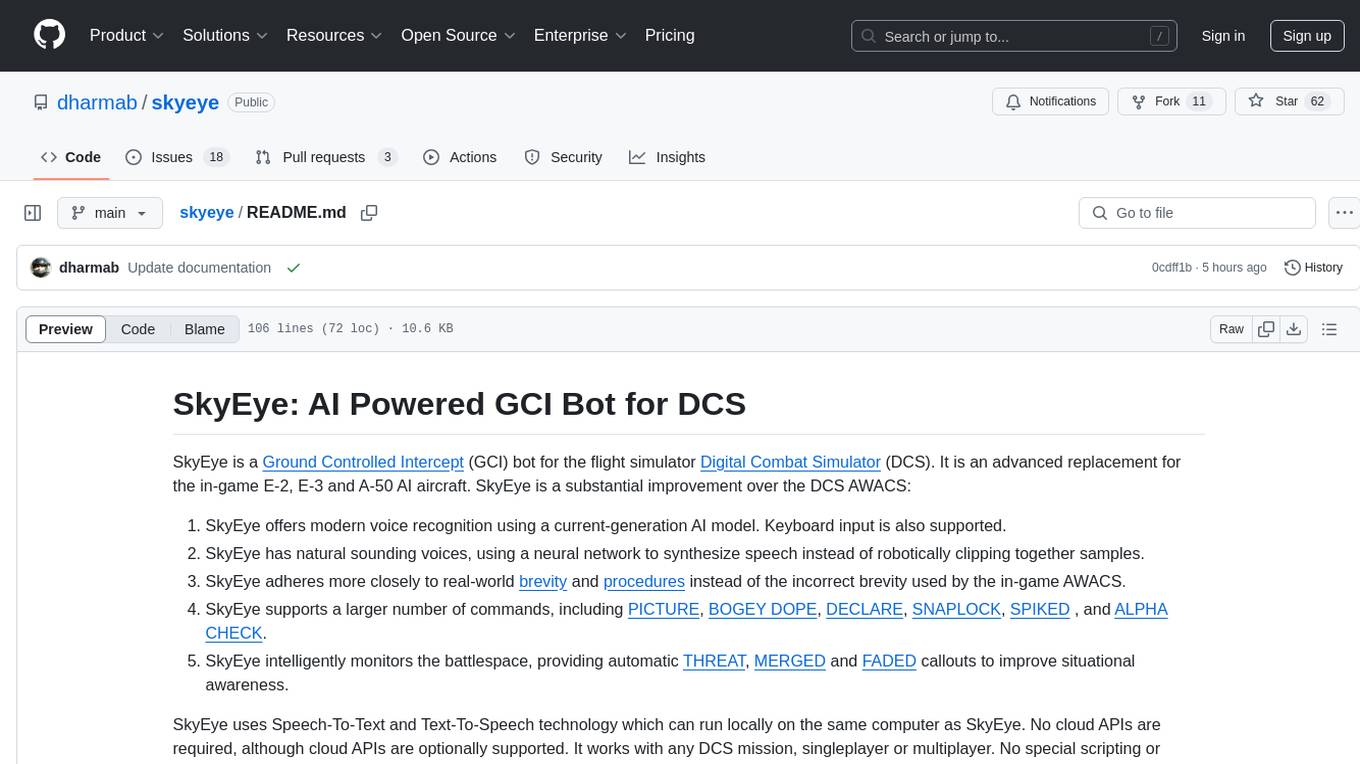
skyeye
SkyEye is an AI-powered Ground Controlled Intercept (GCI) bot designed for the flight simulator Digital Combat Simulator (DCS). It serves as an advanced replacement for the in-game E-2, E-3, and A-50 AI aircraft, offering modern voice recognition, natural-sounding voices, real-world brevity and procedures, a wide range of commands, and intelligent battlespace monitoring. The tool uses Speech-To-Text and Text-To-Speech technology, can run locally or on a cloud server, and is production-ready software used by various DCS communities.