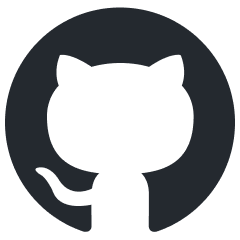
DataFrame
C++ DataFrame for statistical, Financial, and ML analysis -- in modern C++ using native types and contiguous memory storage
Stars: 2648
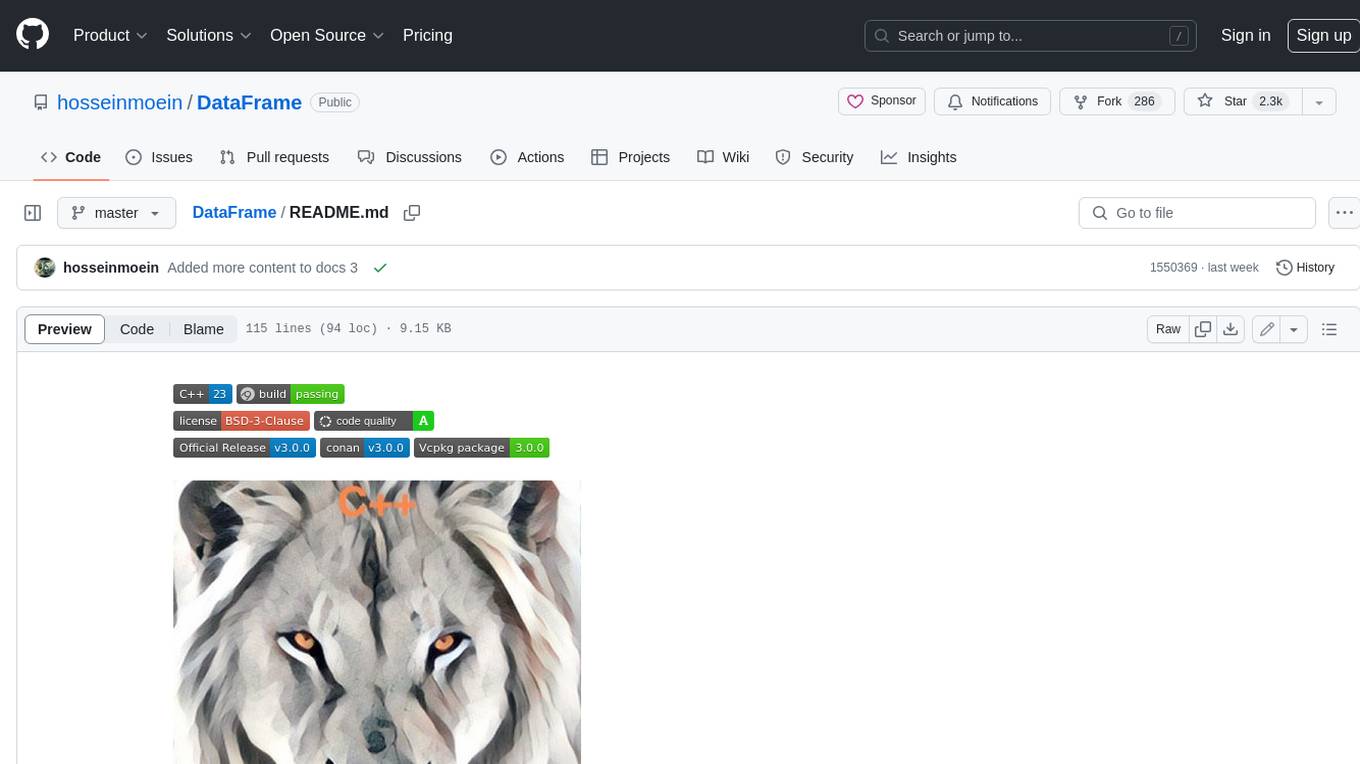
DataFrame is a C++ analytical library designed for data analysis similar to libraries in Python and R. It allows you to slice, join, merge, group-by, and perform various statistical, summarization, financial, and ML algorithms on your data. DataFrame also includes a large collection of analytical algorithms in form of visitors, ranging from basic stats to more involved analysis. You can easily add your own algorithms as well. DataFrame employs extensive multithreading in almost all its APIs, making it suitable for analyzing large datasets. Key principles followed in the library include supporting any type without needing new code, avoiding pointer chasing, having all column data in contiguous memory space, minimizing space usage, avoiding data copying, using multi-threading judiciously, and not protecting the user against garbage in, garbage out.
README:
This is a C++ analytical library designed for data analysis similar to libraries in Python and R. For example, you would compare this to Pandas or R data.frame. The depth and breadth of functionalities offered by C++ DataFrame alone are many times greater than functionalities offered by packages such as Pandas, data.frame, and Polars combined.
You can slice the data in many different ways. You can join, merge, group-by the data. You can run various statistical, summarization, financial, and ML algorithms on the data. You can add your custom algorithms easily. You can multi-column sort, custom pick and delete the data. And more …
DataFrame also includes a large collection of analytical algorithms in form of visitors. These are from basic stats such as Mean, Std Deviation, Return, … to more involved analysis such as PCA, Polynomial Fit, Fast Fourier transform of arbitrary length … including a good collection of trading indicators. You can also easily add your own algorithms.
DataFrame also employs extensive multithreading in almost all its API’s, for large datasets. That makes DataFrame especially suitable for analyzing large datasets.
For basic operations to start you off, see Hello World. For a complete list of features with code samples, see documentation.
I have followed a few principles in this library:
- Support any type either built-in or user defined without needing new code
- Never chase pointers ala linked lists, std::any, pointer to base, ...
- Have all column data in contiguous memory space
- Never use more space than you need ala unions, std::variant, ...
- Avoid copying data as much as possible
- Use multi-threading but only when it makes sense
- Do not attempt to protect the user against garbage in, garbage out
- Keep DataFrame library self-contained, meaning DataFrame must only depend on C++ language and its standard library
You have probably heard of Polars DataFrame. It is implemented in Rust and ported with zero-overhead to Python (as long as you don’t have a loop). I have been asked by many people to write a comparison for DataFrame vs. Polars. So, I finally found some time to learn a bit about Polars and write a very simple benchmark.
I wrote the following identical programs for both Polars and C++ DataFrame (and Pandas). I used Polars version: 0.19.14 (Pandas version: 1.5.3, Numpy version: 1.24.2). And I used C++23 GCC-14 compiler with -O3 option. I ran both on my, somewhat outdated, MacBook Pro (Intel chip, 96GB RAM).
In both cases, I created a dataframe with 3 random columns. The C++ DataFrame also required an additional index column of the same size. Polars doesn’t believe in index columns (that has its own pros and cons. I am not going through it here).
Each program has three identical parts. First it generates and populates 3 columns with 300m random numbers each (in case of C++ DataFrame, it must also generate a sequential index column of the same size). That is the part I am not interested in. In the second part, it calculates the mean of the first column, the variance of the second column, and the Pearson correlation of the second and third columns. In the third part, it does a select (or filter as Polars calls it) on one of the columns.
Results:
The maximum dataset I could load into Polars was 300m rows per column. Any bigger dataset blew up the memory and caused OS to kill it. I ran C++ DataFrame with 10b rows per column and I am sure it would have run with bigger datasets too. So, I was forced to run both with 300m rows to compare.
I ran each test 4 times and took the best time. Polars numbers varied a lot from one run to another, especially calculation and selection times. C++ DataFrame numbers were significantly more consistent.
C++ DataFrame | Polars | Pandas | |
---|---|---|---|
Data generation/load time | 26.9459 secs | 28.4686 secs | 36.6799 secs |
Calculation time | 1.2602 secs | 4.8766 secs | 40.3264 secs |
Selection time | 0.4215 secs | 3.8766 secs | 8.3264 secs |
Overall time: | 28.9486 secs | 36.8763 secs | 85.8451 secs |
For Tasks:
Click tags to check more tools for each tasksFor Jobs:
Alternative AI tools for DataFrame
Similar Open Source Tools
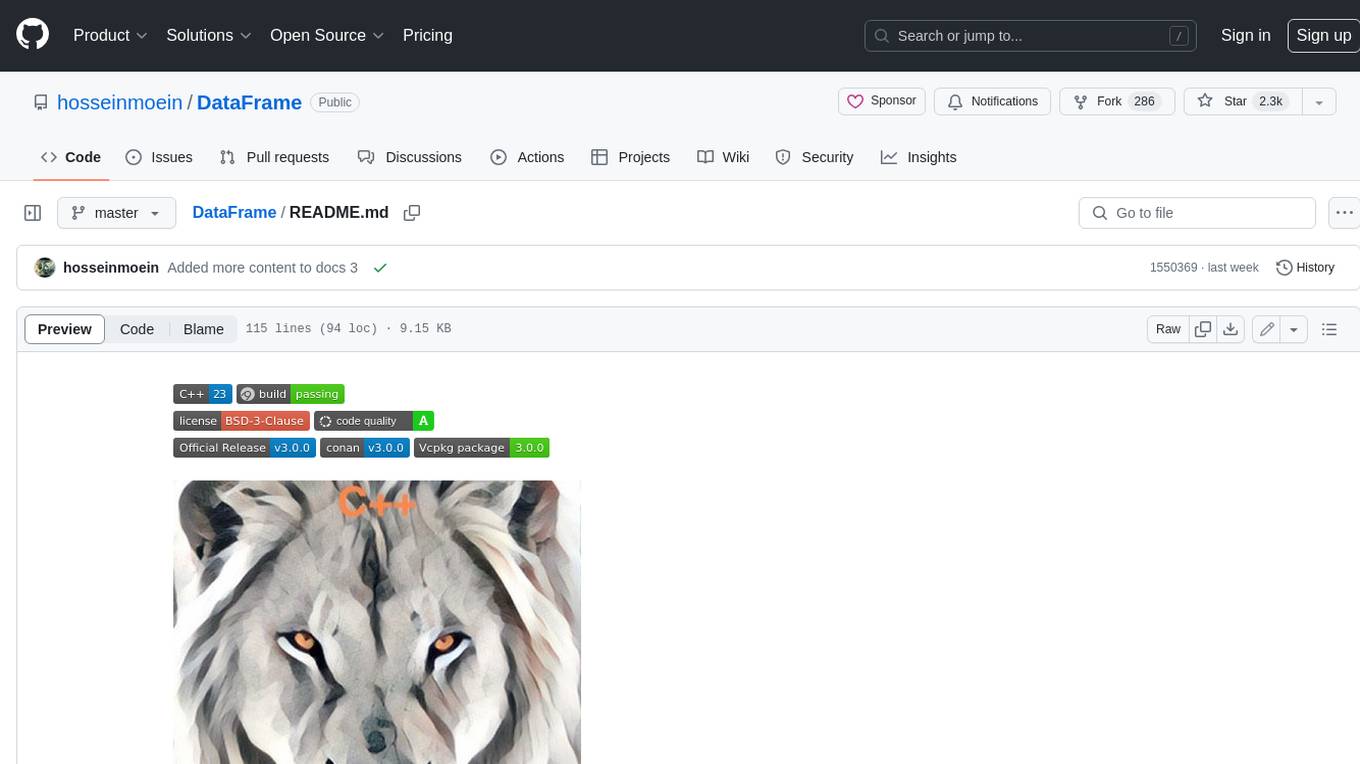
DataFrame
DataFrame is a C++ analytical library designed for data analysis similar to libraries in Python and R. It allows you to slice, join, merge, group-by, and perform various statistical, summarization, financial, and ML algorithms on your data. DataFrame also includes a large collection of analytical algorithms in form of visitors, ranging from basic stats to more involved analysis. You can easily add your own algorithms as well. DataFrame employs extensive multithreading in almost all its APIs, making it suitable for analyzing large datasets. Key principles followed in the library include supporting any type without needing new code, avoiding pointer chasing, having all column data in contiguous memory space, minimizing space usage, avoiding data copying, using multi-threading judiciously, and not protecting the user against garbage in, garbage out.
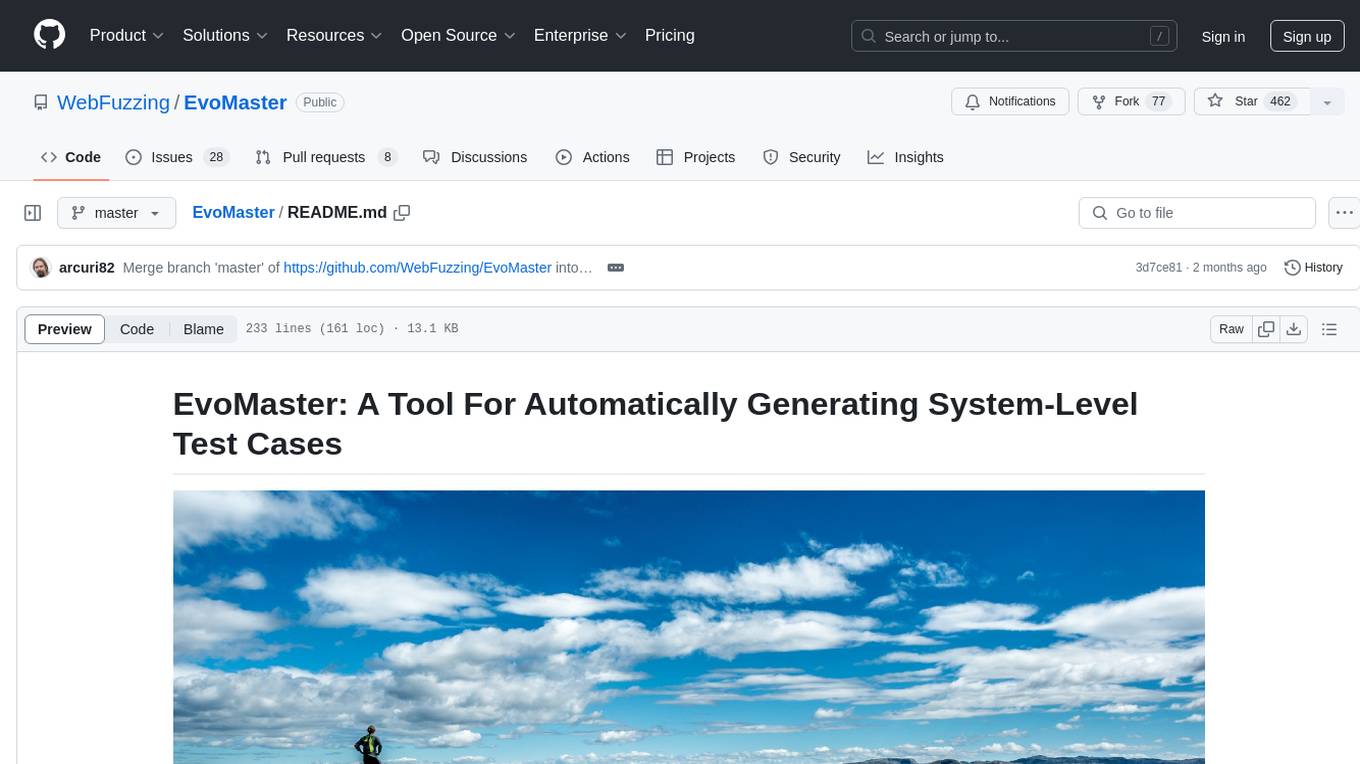
EvoMaster
EvoMaster is an open-source AI-driven tool that automatically generates system-level test cases for web/enterprise applications. It uses an Evolutionary Algorithm and Dynamic Program Analysis to evolve test cases, maximizing code coverage and fault detection. The tool supports REST, GraphQL, and RPC APIs, with whitebox testing for JVM-compiled languages. It generates JUnit tests, detects faults, handles SQL databases, and supports authentication. EvoMaster has been funded by the European Research Council and the Research Council of Norway.
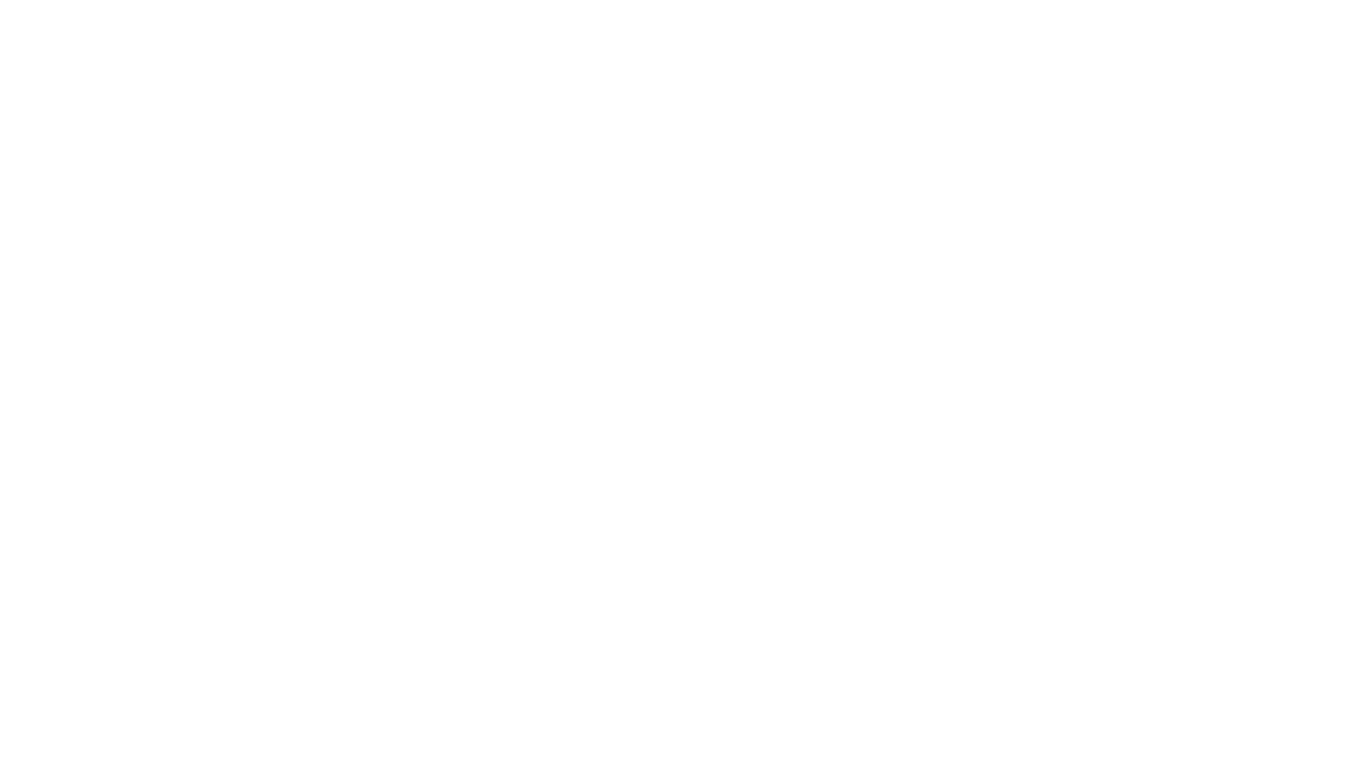
EvoMaster
EvoMaster is an open-source AI-driven tool that automatically generates system-level test cases for web/enterprise applications. It uses Evolutionary Algorithm and Dynamic Program Analysis to evolve test cases, maximizing code coverage and fault detection. It supports REST, GraphQL, and RPC APIs, with whitebox testing for JVM-compiled APIs. The tool generates JUnit tests in Java or Kotlin, focusing on fault detection, self-contained tests, SQL handling, and authentication. Known limitations include manual driver creation for whitebox testing and longer execution times for better results. EvoMaster has been funded by ERC and RCN grants.
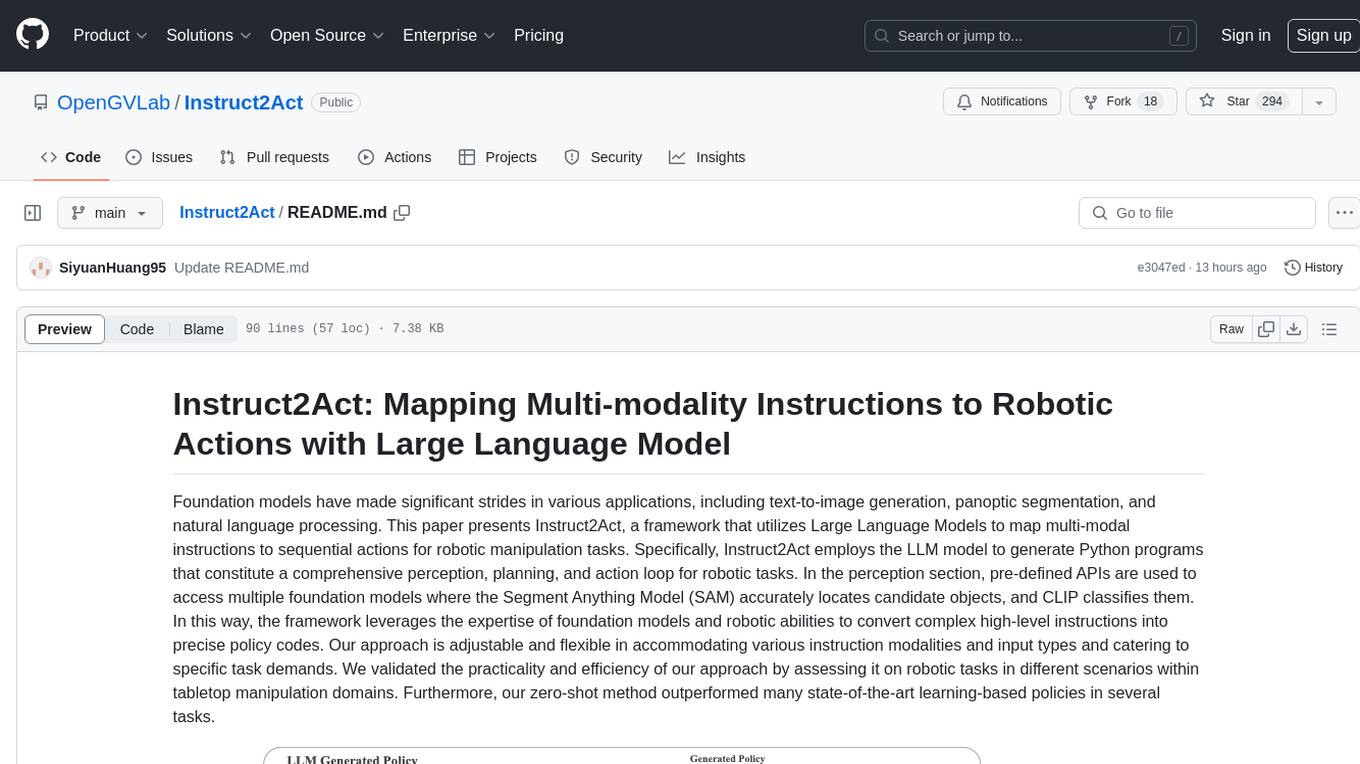
Instruct2Act
Instruct2Act is a framework that utilizes Large Language Models to map multi-modal instructions to sequential actions for robotic manipulation tasks. It generates Python programs using the LLM model for perception, planning, and action. The framework leverages foundation models like SAM and CLIP to convert high-level instructions into policy codes, accommodating various instruction modalities and task demands. Instruct2Act has been validated on robotic tasks in tabletop manipulation domains, outperforming learning-based policies in several tasks.
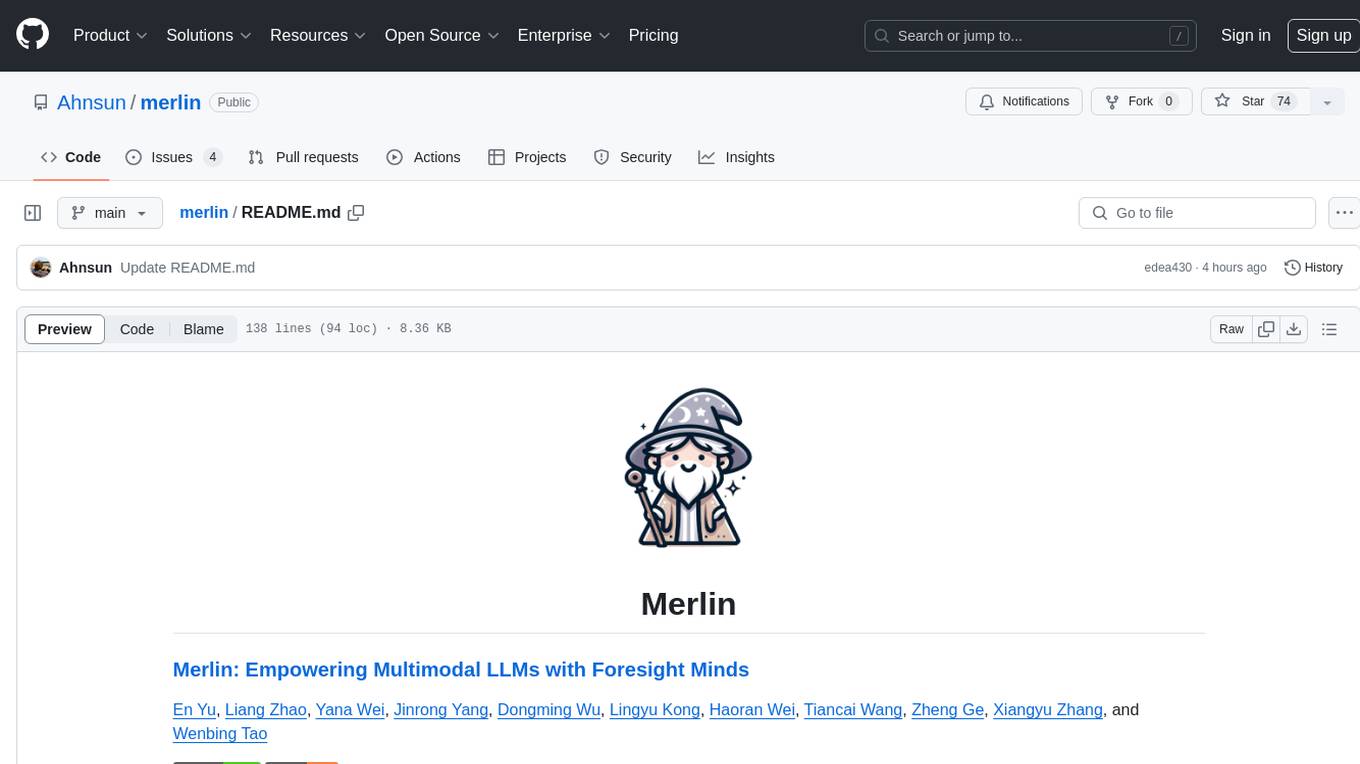
merlin
Merlin is a groundbreaking model capable of generating natural language responses intricately linked with object trajectories of multiple images. It excels in predicting and reasoning about future events based on initial observations, showcasing unprecedented capability in future prediction and reasoning. Merlin achieves state-of-the-art performance on the Future Reasoning Benchmark and multiple existing multimodal language models benchmarks, demonstrating powerful multi-modal general ability and foresight minds.
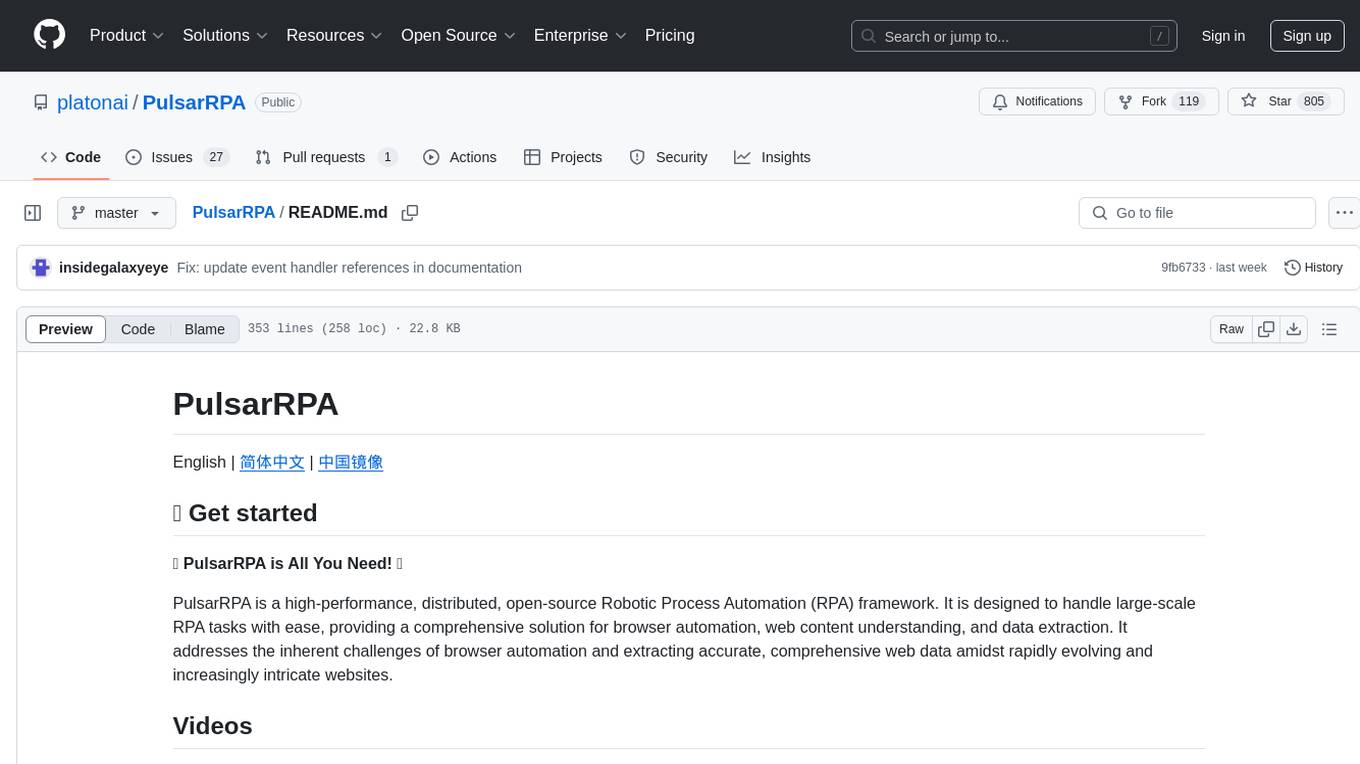
PulsarRPA
PulsarRPA is a high-performance, distributed, open-source Robotic Process Automation (RPA) framework designed to handle large-scale RPA tasks with ease. It provides a comprehensive solution for browser automation, web content understanding, and data extraction. PulsarRPA addresses challenges of browser automation and accurate web data extraction from complex and evolving websites. It incorporates innovative technologies like browser rendering, RPA, intelligent scraping, advanced DOM parsing, and distributed architecture to ensure efficient, accurate, and scalable web data extraction. The tool is open-source, customizable, and supports cutting-edge information extraction technology, making it a preferred solution for large-scale web data extraction.
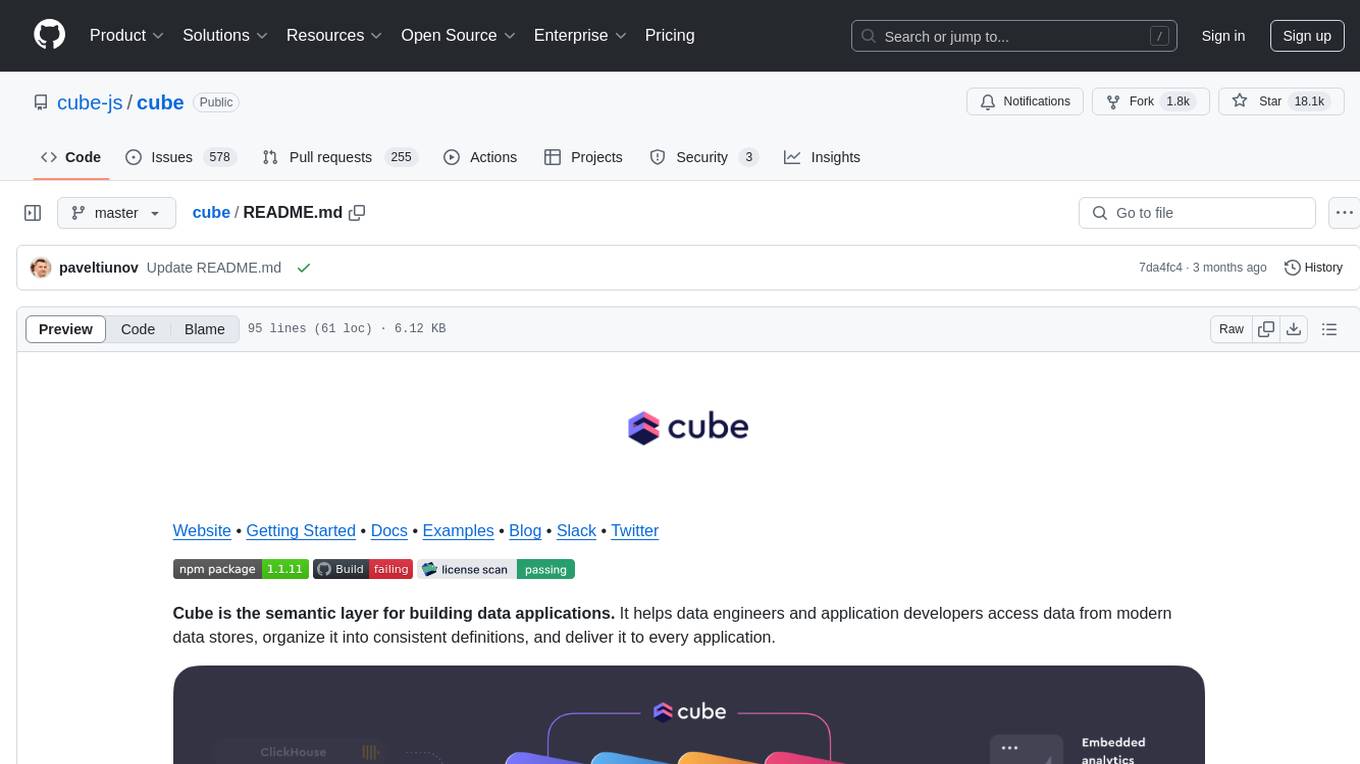
cube
Cube is a semantic layer for building data applications, helping data engineers and application developers access data from modern data stores, organize it into consistent definitions, and deliver it to every application. It works with SQL-enabled data sources, providing sub-second latency and high concurrency for API requests. Cube addresses SQL code organization, performance, and access control issues in data applications, enabling efficient data modeling, access control, and performance optimizations for various tools like embedded analytics, dashboarding, reporting, and data notebooks.
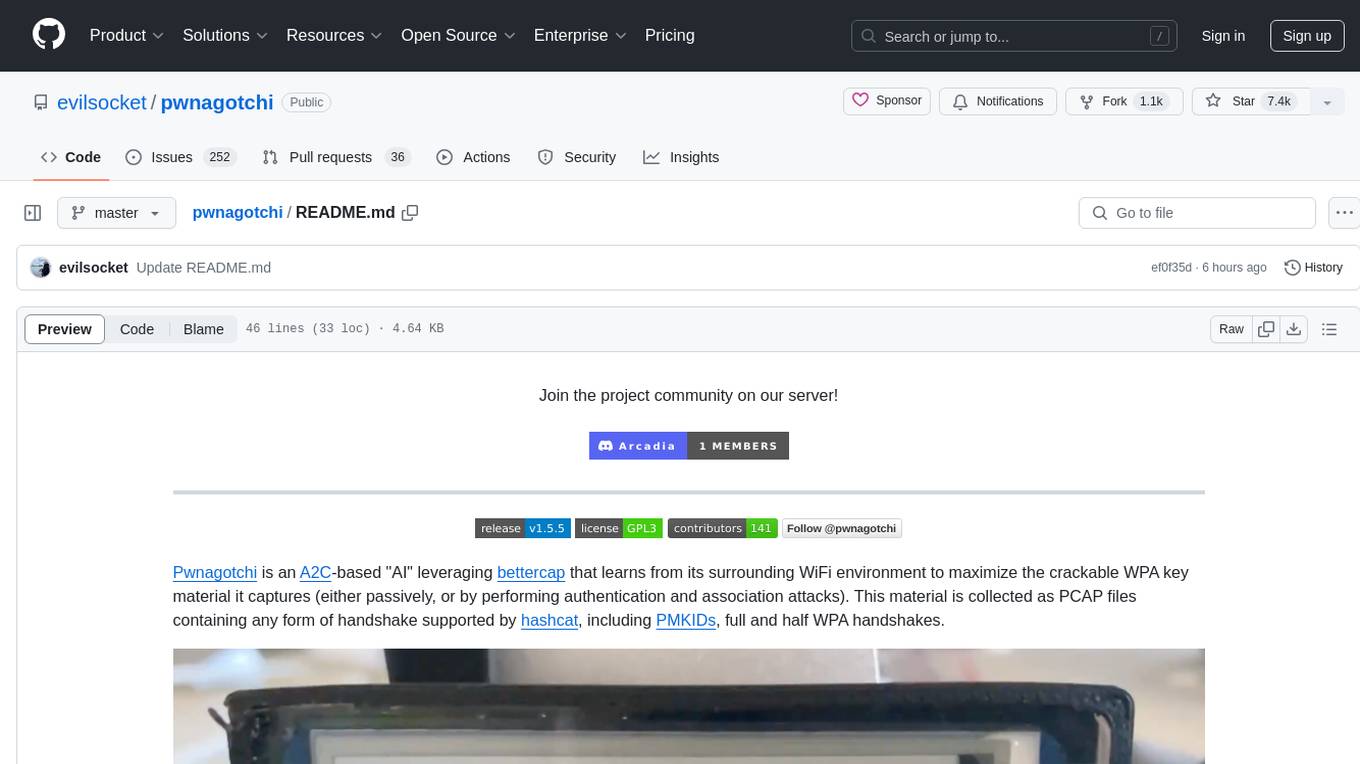
pwnagotchi
Pwnagotchi is an AI tool leveraging bettercap to learn from WiFi environments and maximize crackable WPA key material. It uses LSTM with MLP feature extractor for A2C agent, learning over epochs to improve performance in various WiFi environments. Units can cooperate using a custom parasite protocol. Visit https://www.pwnagotchi.ai for documentation and community links.
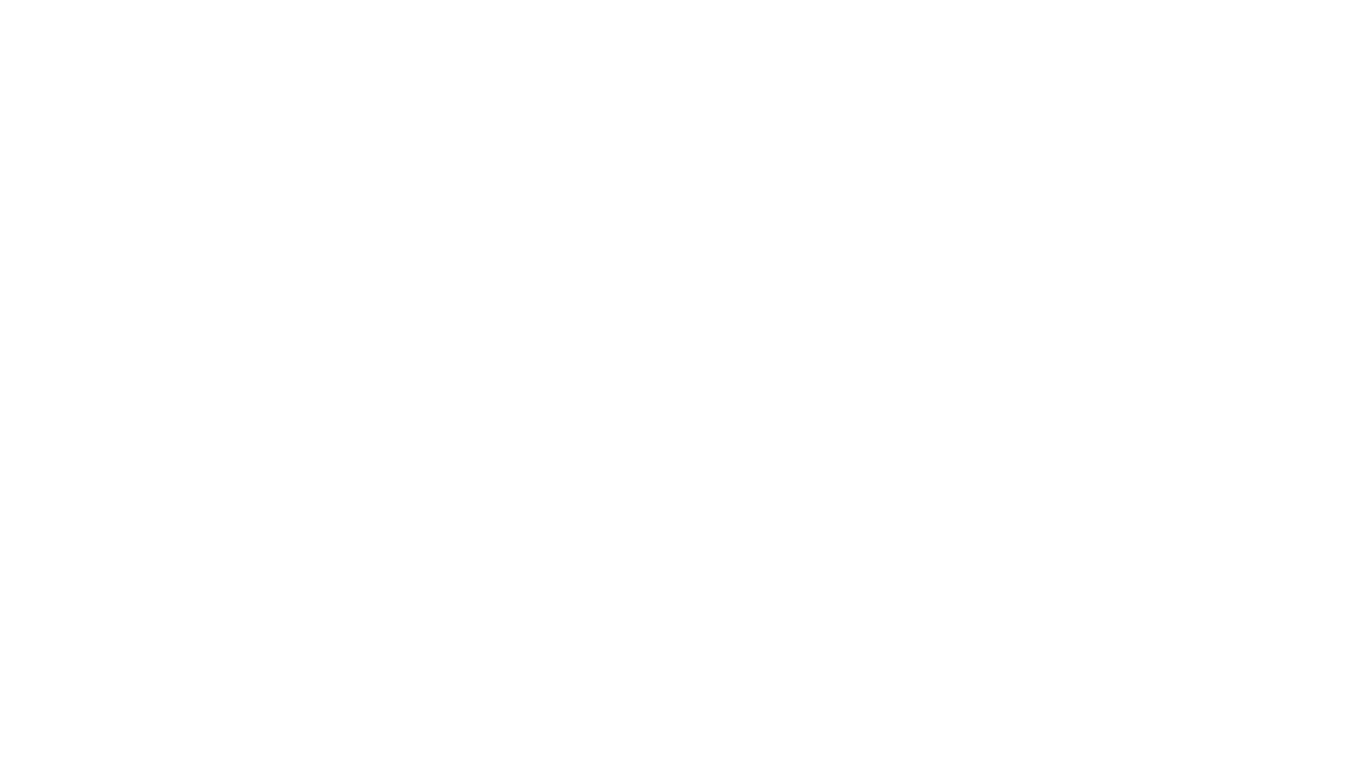
Raspberry
Raspberry is an open source project aimed at creating a toy dataset for finetuning Large Language Models (LLMs) with reasoning abilities. The project involves synthesizing complex user queries across various domains, generating CoT and Self-Critique data, cleaning and rectifying samples, finetuning an LLM with the dataset, and seeking funding for scalability. The ultimate goal is to develop a dataset that challenges models with tasks requiring math, coding, logic, reasoning, and planning skills, spanning different sectors like medicine, science, and software development.
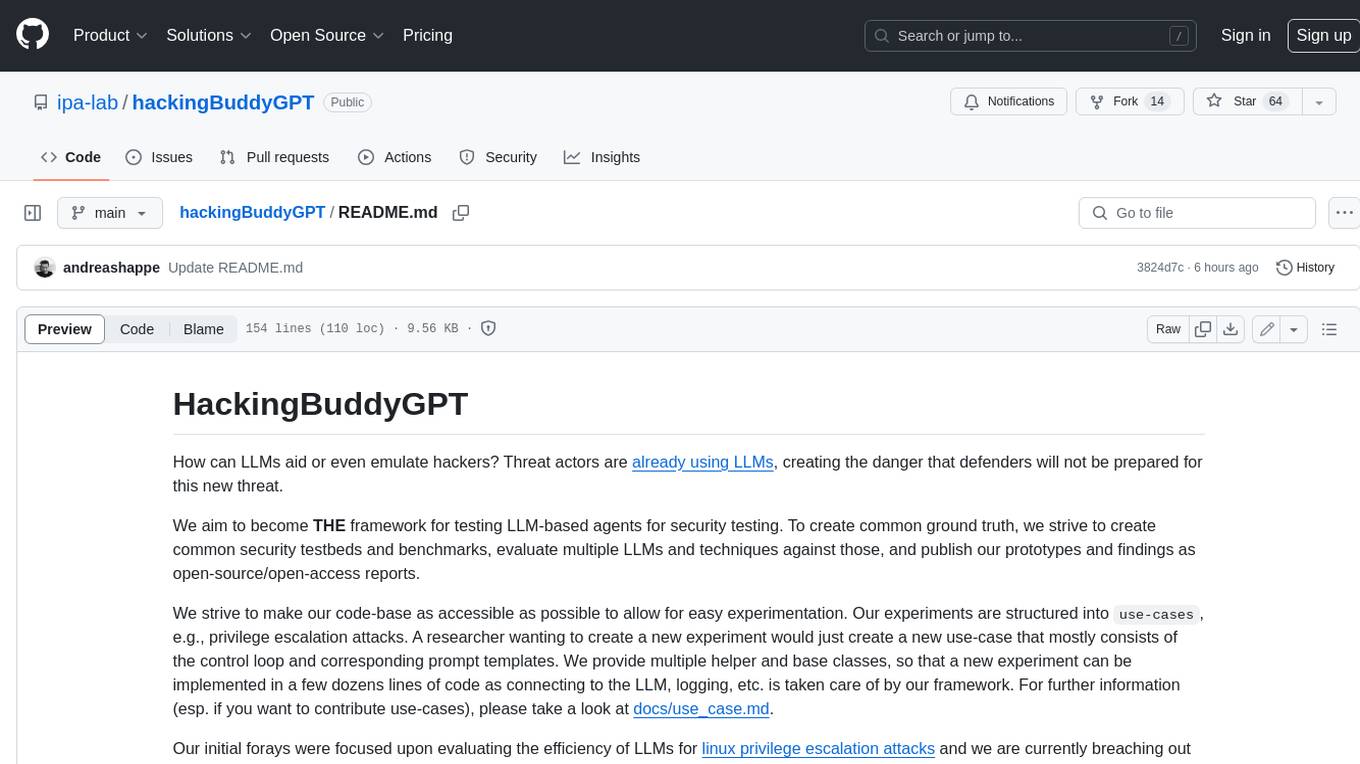
hackingBuddyGPT
hackingBuddyGPT is a framework for testing LLM-based agents for security testing. It aims to create common ground truth by creating common security testbeds and benchmarks, evaluating multiple LLMs and techniques against those, and publishing prototypes and findings as open-source/open-access reports. The initial focus is on evaluating the efficiency of LLMs for Linux privilege escalation attacks, but the framework is being expanded to evaluate the use of LLMs for web penetration-testing and web API testing. hackingBuddyGPT is released as open-source to level the playing field for blue teams against APTs that have access to more sophisticated resources.
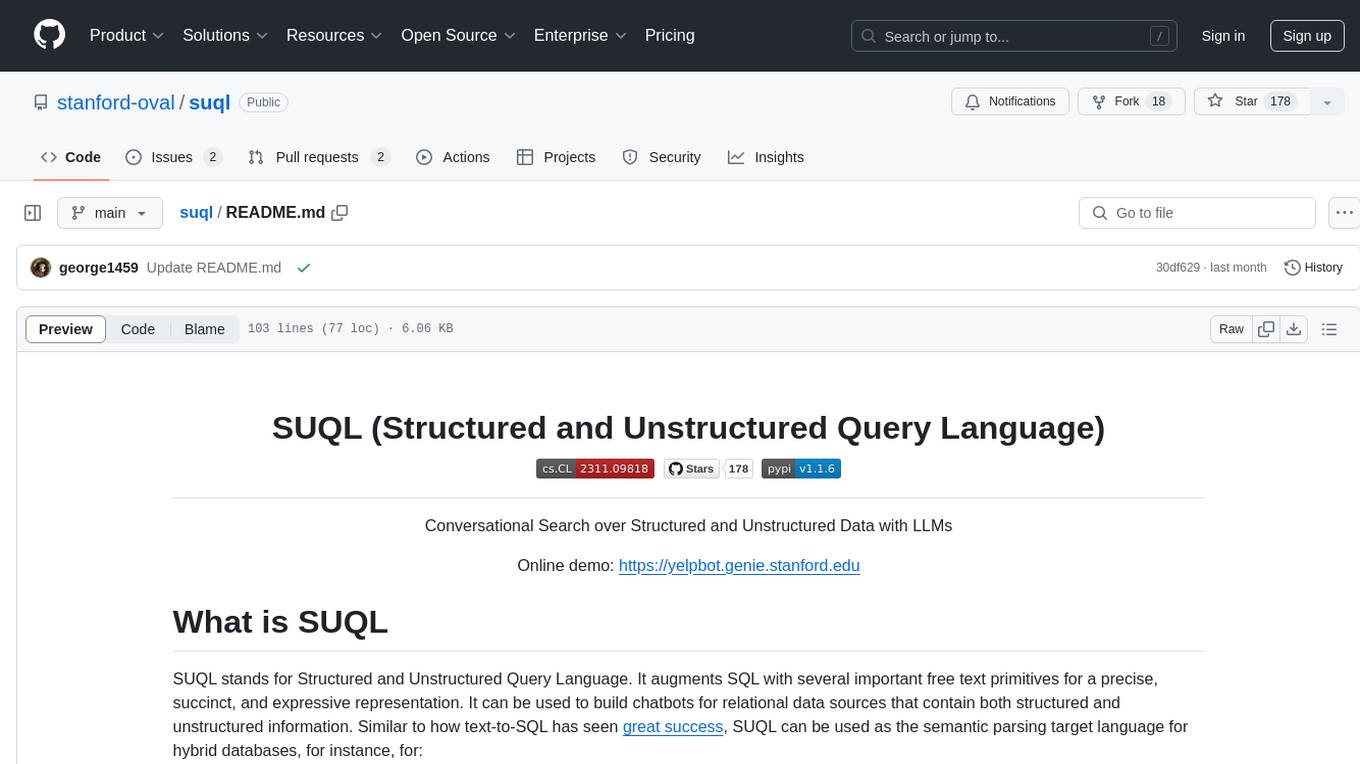
suql
SUQL (Structured and Unstructured Query Language) is a tool that augments SQL with free text primitives for building chatbots that can interact with relational data sources containing both structured and unstructured information. It seamlessly integrates retrieval models, large language models (LLMs), and traditional SQL to provide a clean interface for hybrid data access. SUQL supports optimizations to minimize expensive LLM calls, scalability to large databases with PostgreSQL, and general SQL operations like JOINs and GROUP BYs.
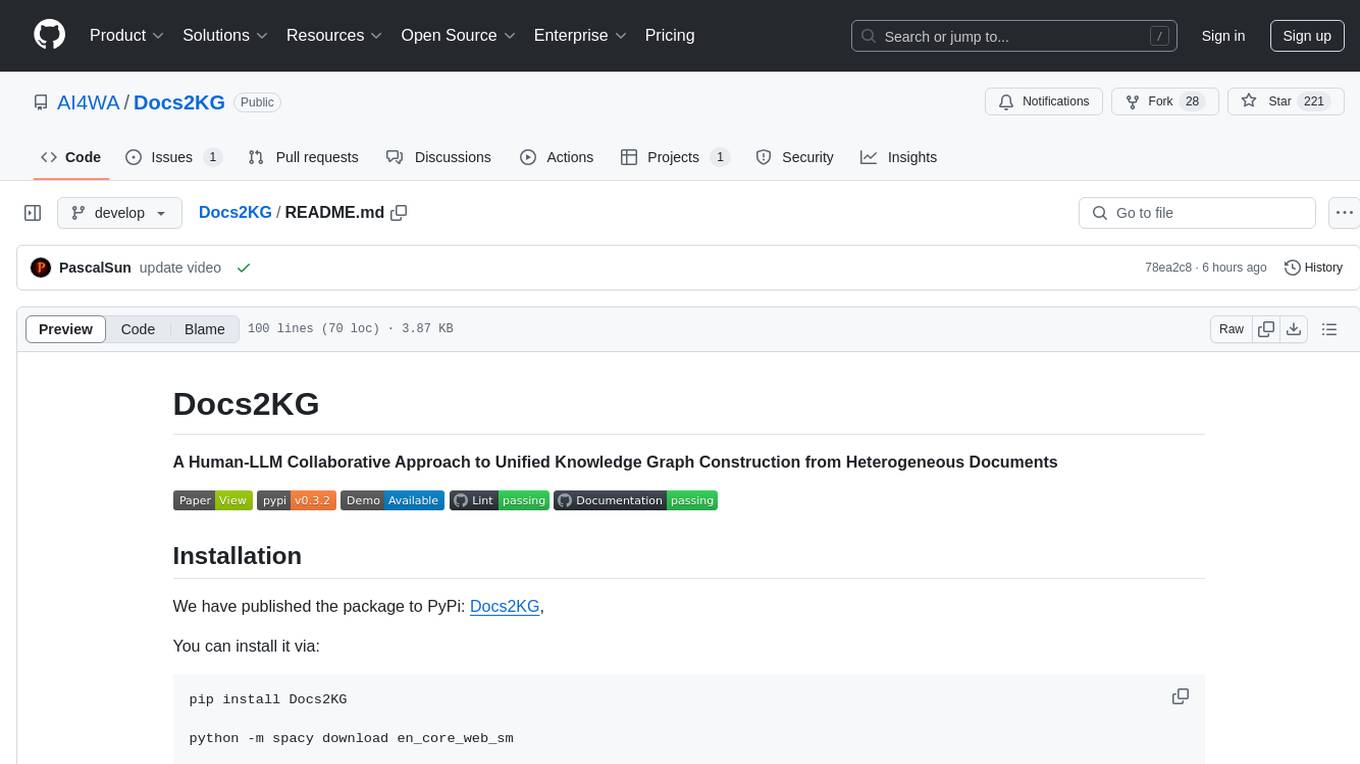
Docs2KG
Docs2KG is a tool designed for constructing a unified knowledge graph from heterogeneous documents. It addresses the challenges of digitizing diverse unstructured documents and constructing a high-quality knowledge graph with less effort. The tool combines bottom-up and top-down approaches, utilizing a human-LLM collaborative interface to enhance the generated knowledge graph. It organizes the knowledge graph into MetaKG, LayoutKG, and SemanticKG, providing a comprehensive view of document content. Docs2KG aims to streamline the process of knowledge graph construction and offers metrics for evaluating the quality of automatic construction.
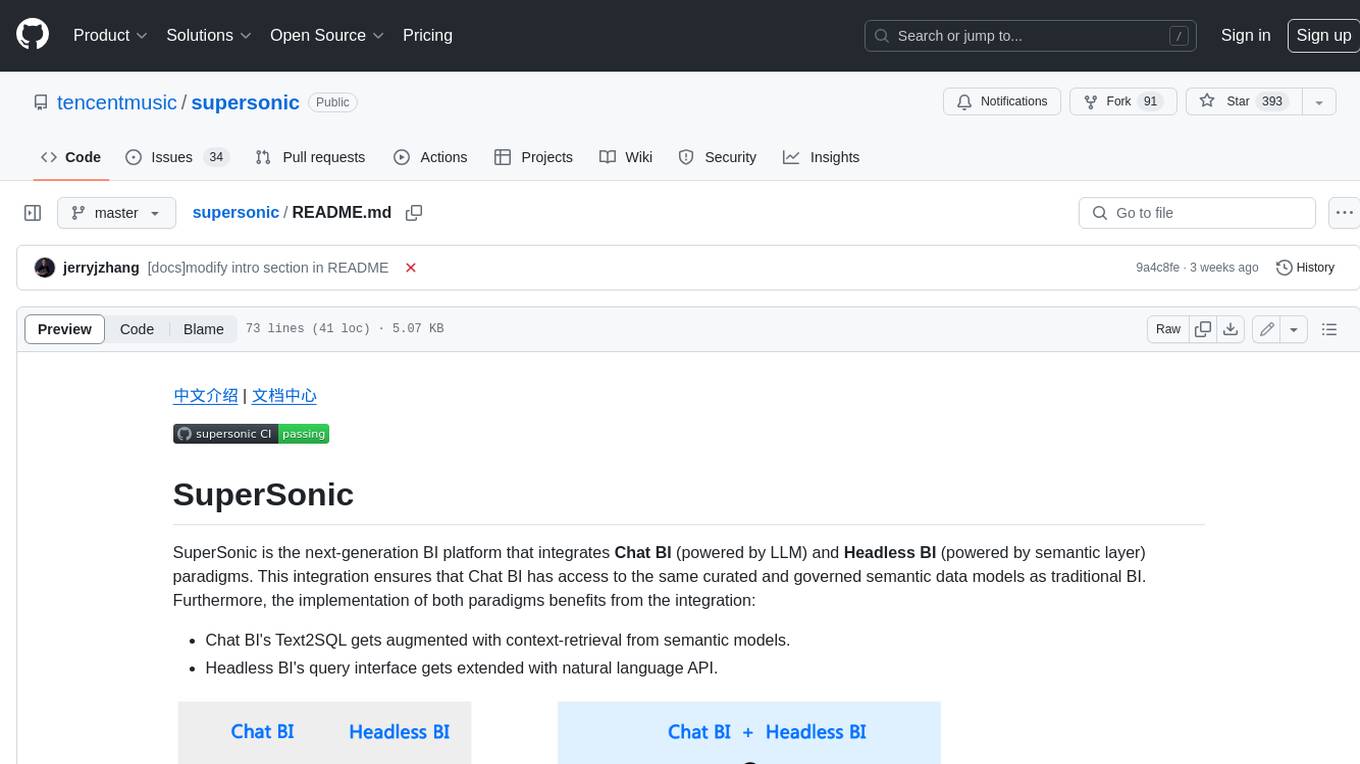
supersonic
SuperSonic is a next-generation BI platform that integrates Chat BI (powered by LLM) and Headless BI (powered by semantic layer) paradigms. This integration ensures that Chat BI has access to the same curated and governed semantic data models as traditional BI. Furthermore, the implementation of both paradigms benefits from the integration: * Chat BI's Text2SQL gets augmented with context-retrieval from semantic models. * Headless BI's query interface gets extended with natural language API. SuperSonic provides a Chat BI interface that empowers users to query data using natural language and visualize the results with suitable charts. To enable such experience, the only thing necessary is to build logical semantic models (definition of metric/dimension/tag, along with their meaning and relationships) through a Headless BI interface. Meanwhile, SuperSonic is designed to be extensible and composable, allowing custom implementations to be added and configured with Java SPI. The integration of Chat BI and Headless BI has the potential to enhance the Text2SQL generation in two dimensions: 1. Incorporate data semantics (such as business terms, column values, etc.) into the prompt, enabling LLM to better understand the semantics and reduce hallucination. 2. Offload the generation of advanced SQL syntax (such as join, formula, etc.) from LLM to the semantic layer to reduce complexity. With these ideas in mind, we develop SuperSonic as a practical reference implementation and use it to power our real-world products. Additionally, to facilitate further development we decide to open source SuperSonic as an extensible framework.
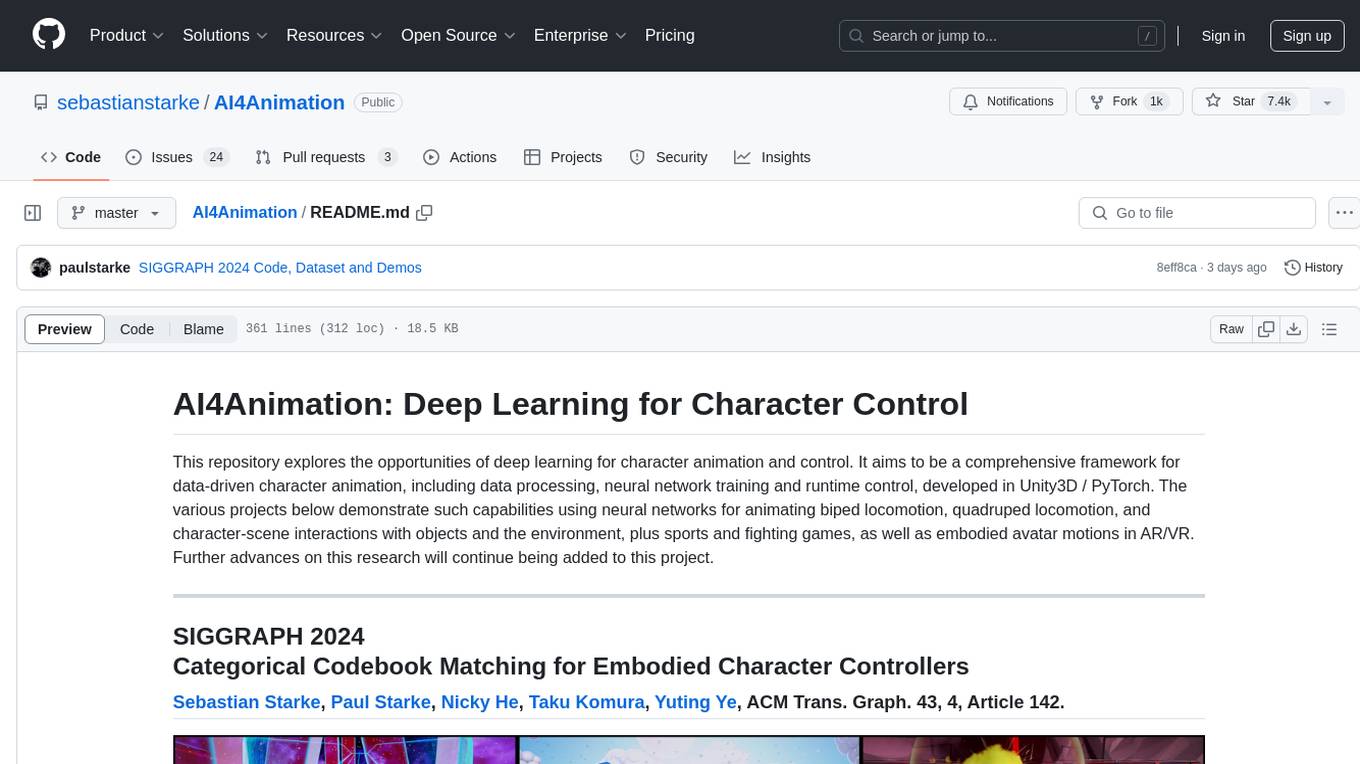
AI4Animation
AI4Animation is a comprehensive framework for data-driven character animation, including data processing, neural network training, and runtime control, developed in Unity3D/PyTorch. It explores deep learning opportunities for character animation, covering biped and quadruped locomotion, character-scene interactions, sports and fighting games, and embodied avatar motions in AR/VR. The research focuses on generative frameworks, codebook matching, periodic autoencoders, animation layering, local motion phases, and neural state machines for character control and animation.
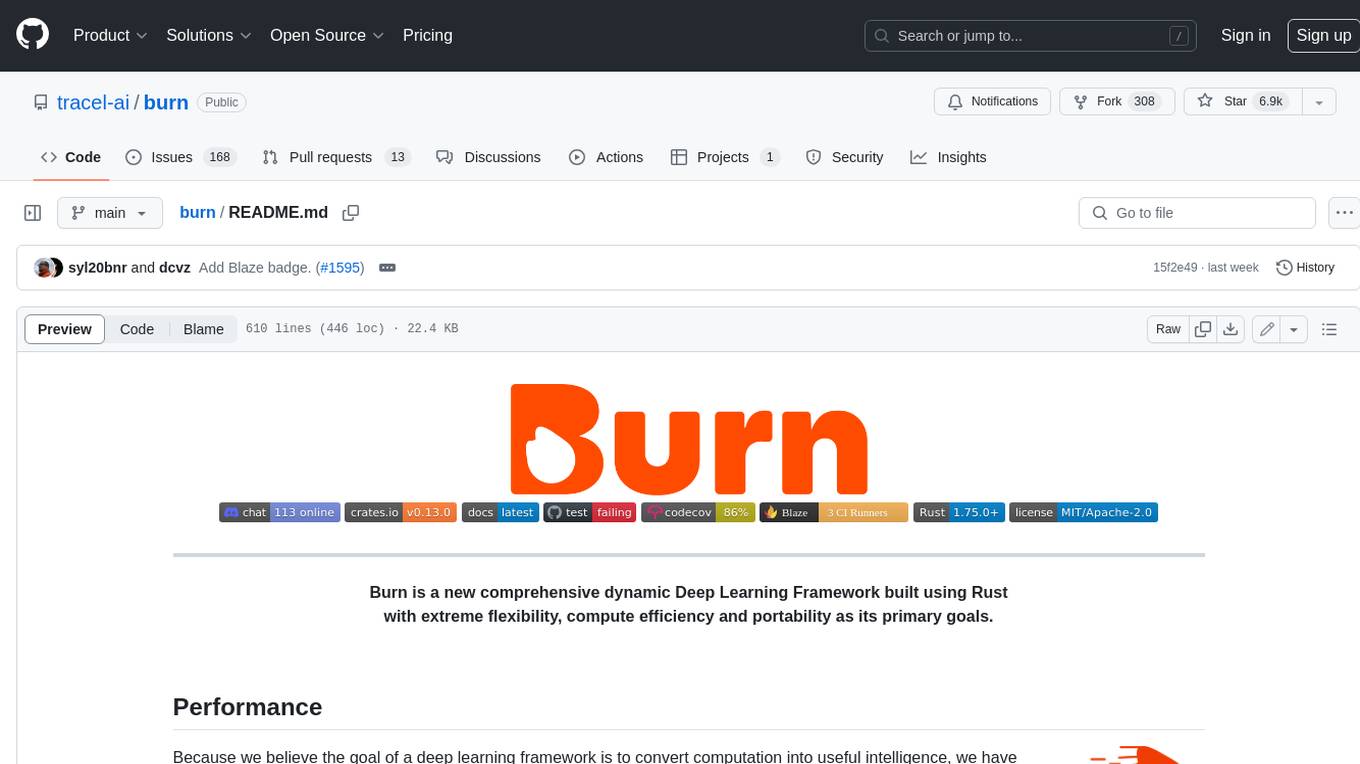
burn
Burn is a new comprehensive dynamic Deep Learning Framework built using Rust with extreme flexibility, compute efficiency and portability as its primary goals.
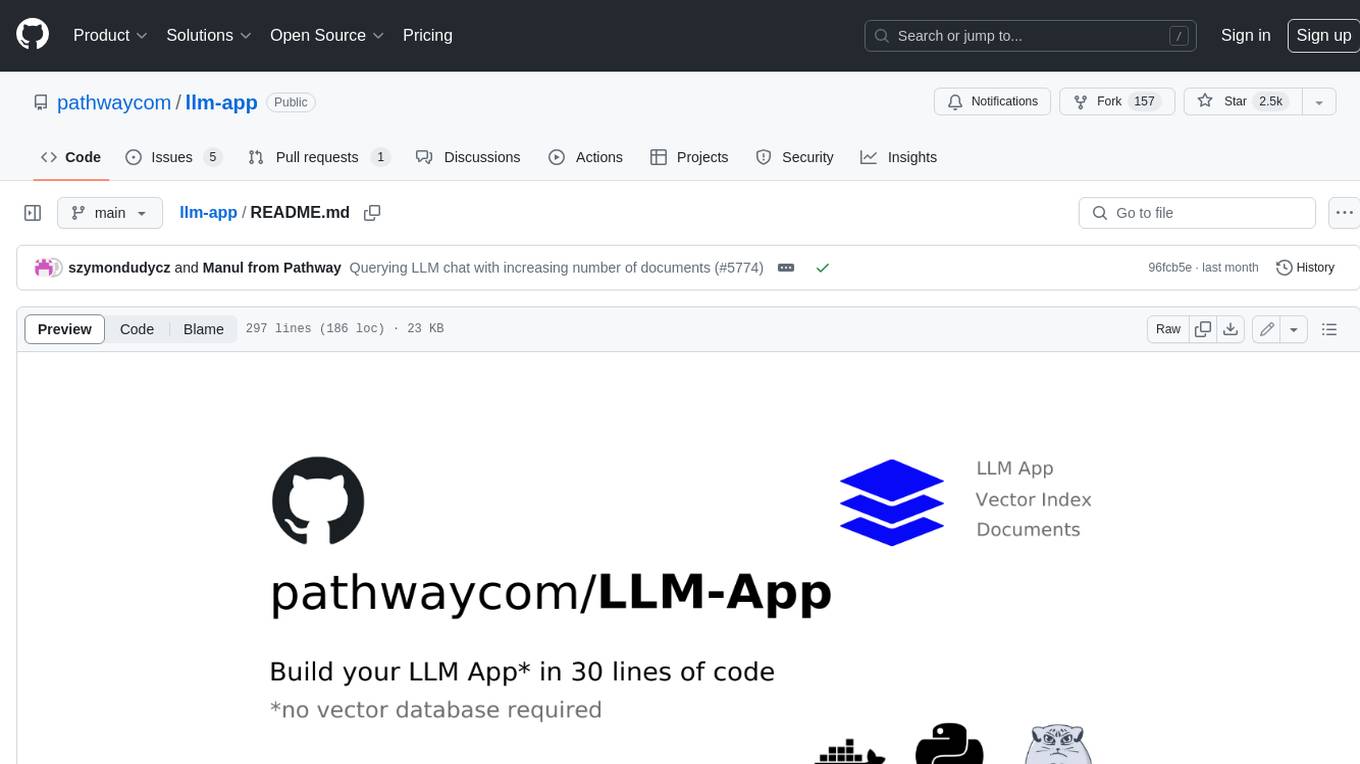
llm-app
Pathway's LLM (Large Language Model) Apps provide a platform to quickly deploy AI applications using the latest knowledge from data sources. The Python application examples in this repository are Docker-ready, exposing an HTTP API to the frontend. These apps utilize the Pathway framework for data synchronization, API serving, and low-latency data processing without the need for additional infrastructure dependencies. They connect to document data sources like S3, Google Drive, and Sharepoint, offering features like real-time data syncing, easy alert setup, scalability, monitoring, security, and unification of application logic.
For similar tasks
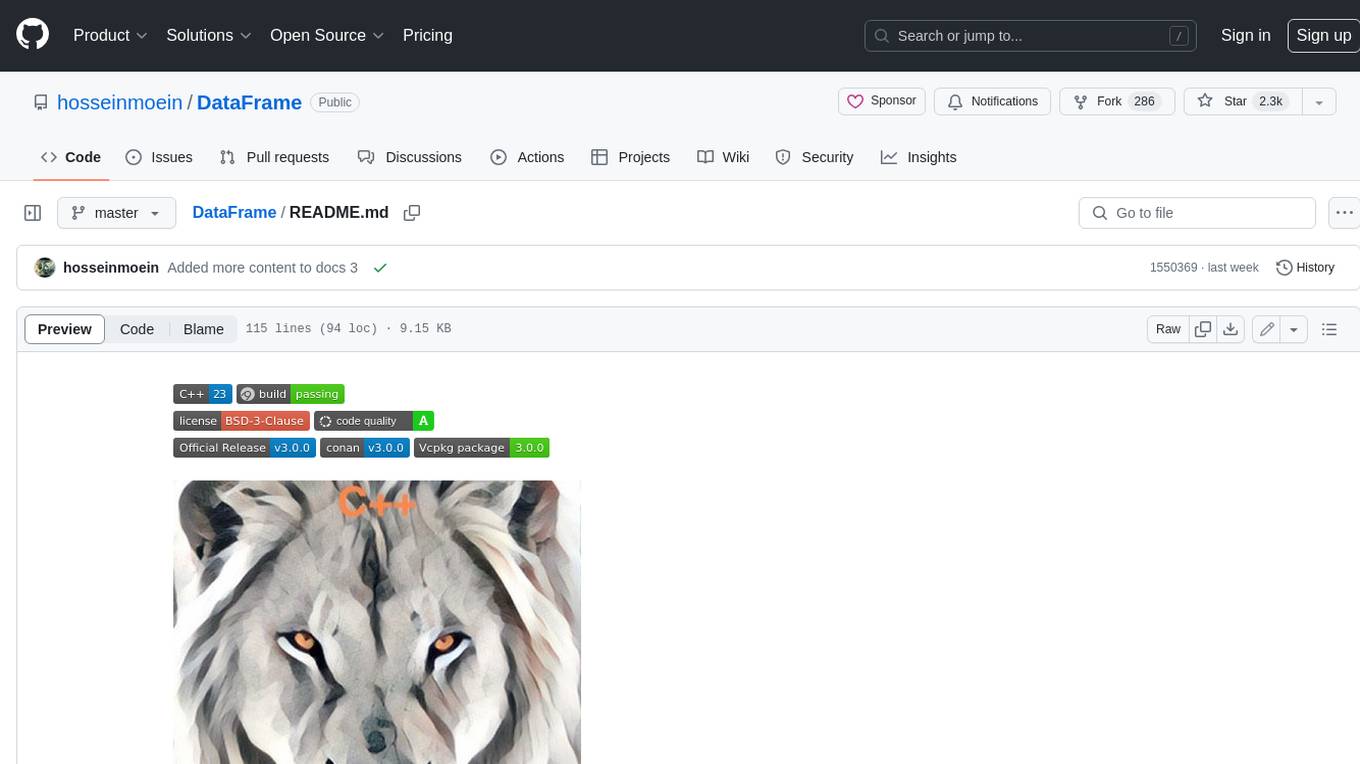
DataFrame
DataFrame is a C++ analytical library designed for data analysis similar to libraries in Python and R. It allows you to slice, join, merge, group-by, and perform various statistical, summarization, financial, and ML algorithms on your data. DataFrame also includes a large collection of analytical algorithms in form of visitors, ranging from basic stats to more involved analysis. You can easily add your own algorithms as well. DataFrame employs extensive multithreading in almost all its APIs, making it suitable for analyzing large datasets. Key principles followed in the library include supporting any type without needing new code, avoiding pointer chasing, having all column data in contiguous memory space, minimizing space usage, avoiding data copying, using multi-threading judiciously, and not protecting the user against garbage in, garbage out.
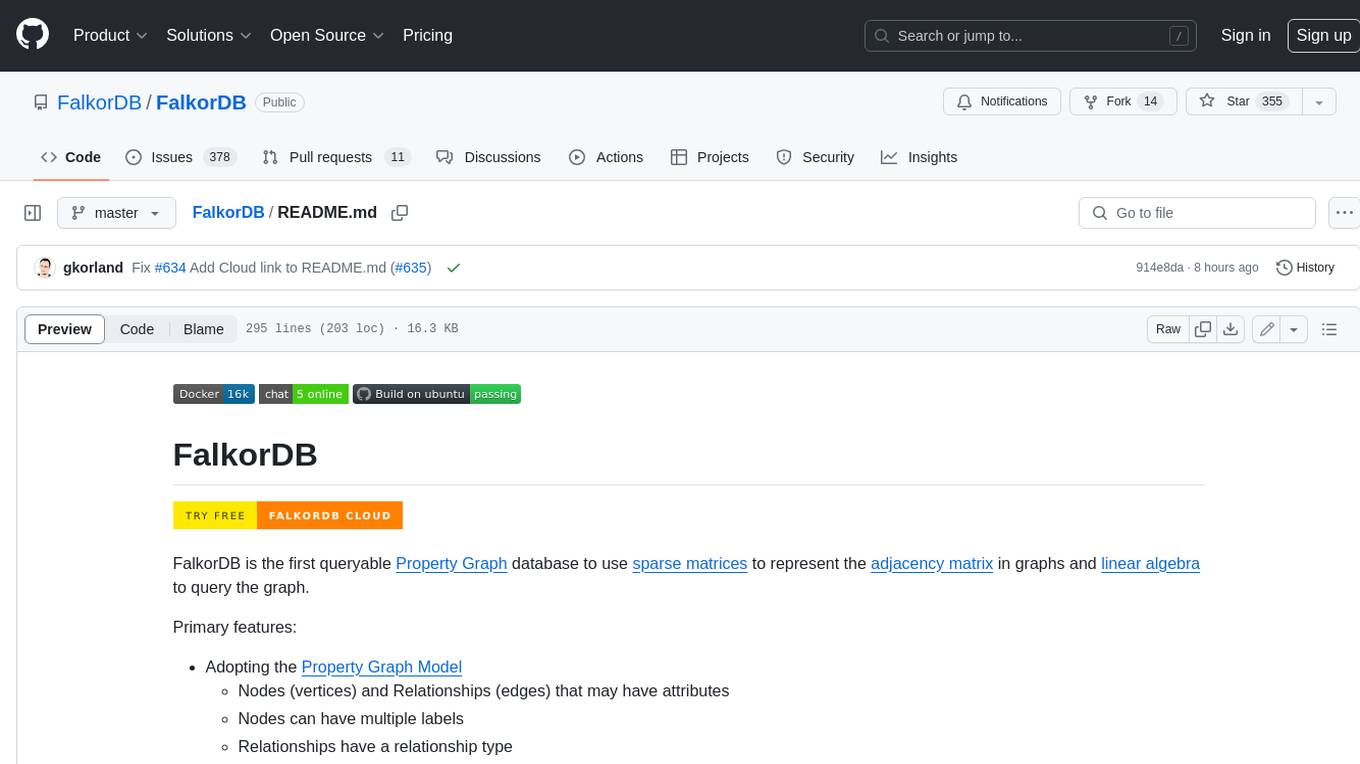
FalkorDB
FalkorDB is the first queryable Property Graph database to use sparse matrices to represent the adjacency matrix in graphs and linear algebra to query the graph. Primary features: * Adopting the Property Graph Model * Nodes (vertices) and Relationships (edges) that may have attributes * Nodes can have multiple labels * Relationships have a relationship type * Graphs represented as sparse adjacency matrices * OpenCypher with proprietary extensions as a query language * Queries are translated into linear algebra expressions
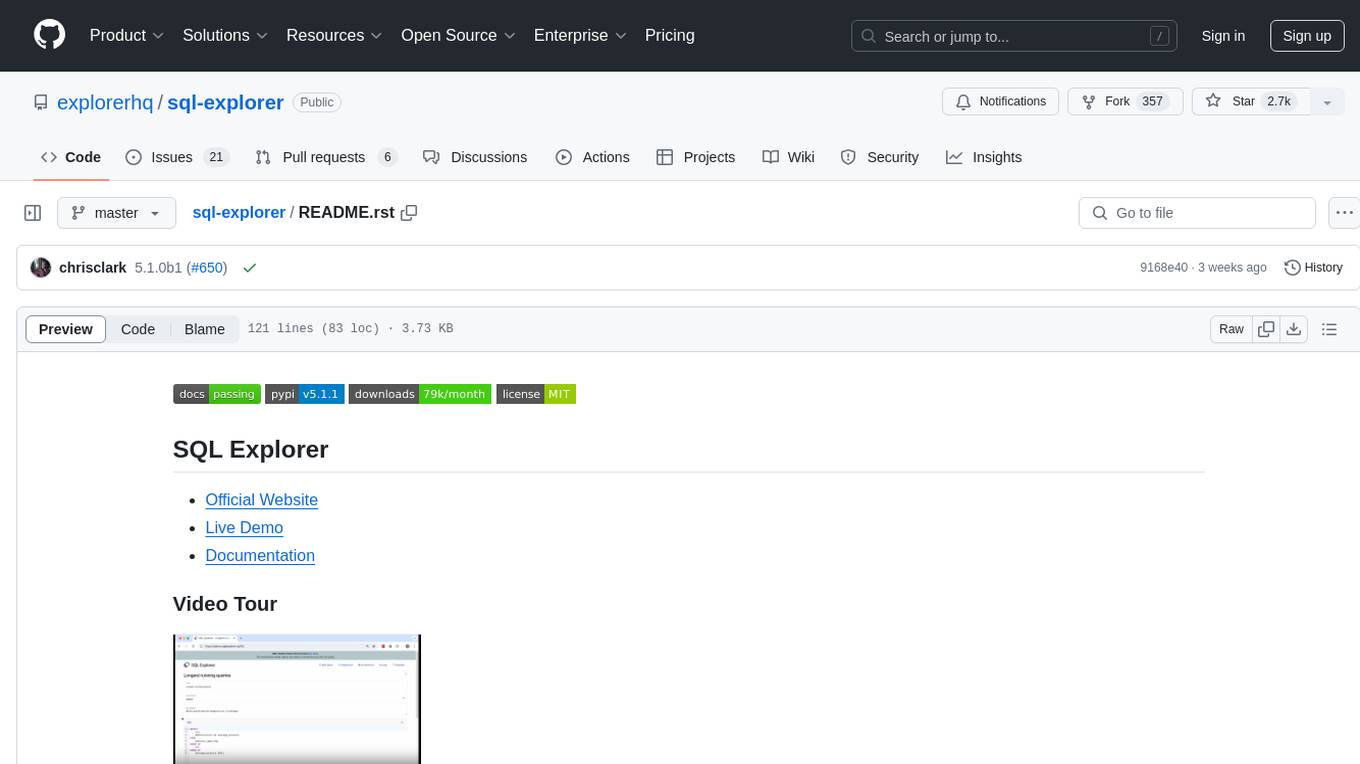
sql-explorer
SQL Explorer is a Django-based application that simplifies the flow of data between users by providing a user-friendly SQL editor to write and share queries. It supports multiple database connections, AI-powered SQL assistant, schema information access, query snapshots, in-browser statistics, parameterized queries, ad-hoc query running, email query results, and more. Users can upload and query JSON or CSV files, and the tool can connect to various SQL databases supported by Django. It aims for simplicity, stability, and ease of use, offering features like autocomplete, pivot tables, and query history logs.
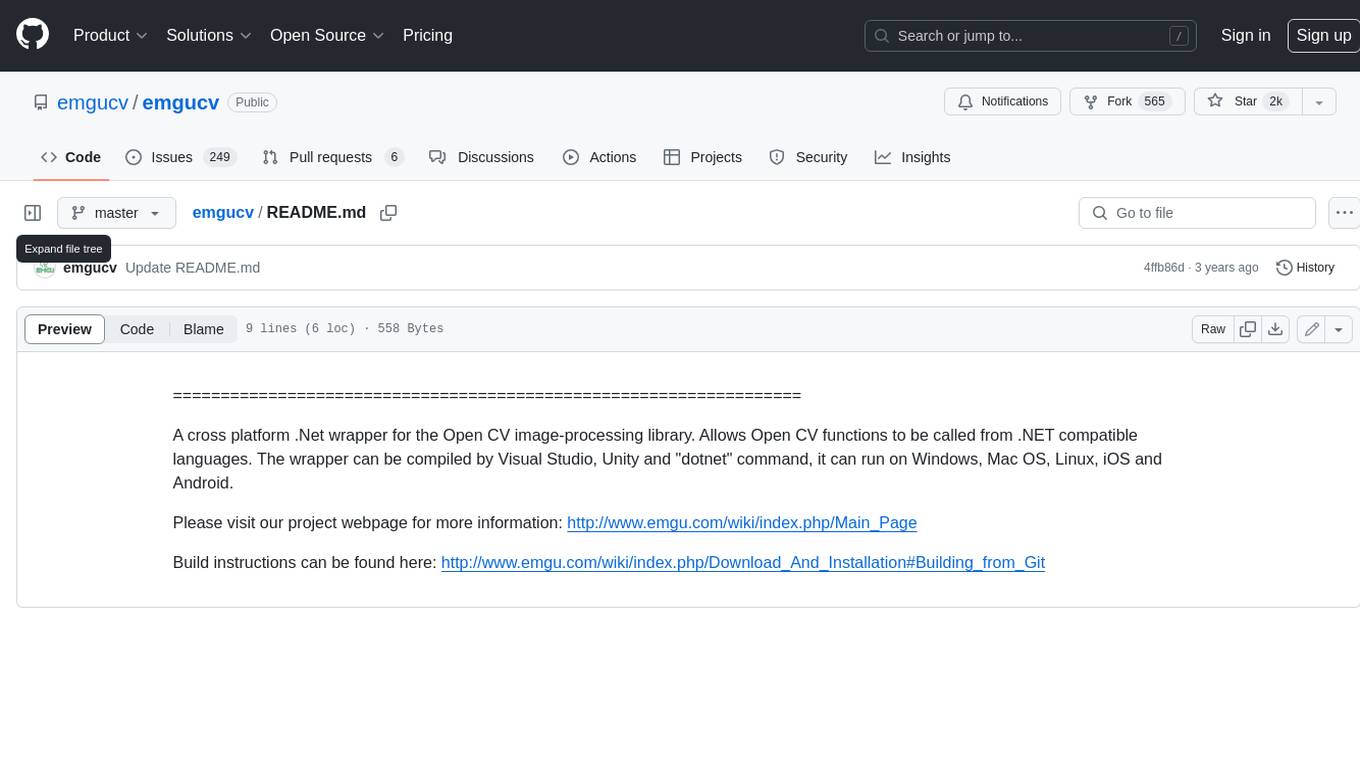
emgucv
Emgu CV is a cross-platform .Net wrapper for the OpenCV image-processing library. It allows OpenCV functions to be called from .NET compatible languages. The wrapper can be compiled by Visual Studio, Unity, and "dotnet" command, and it can run on Windows, Mac OS, Linux, iOS, and Android.
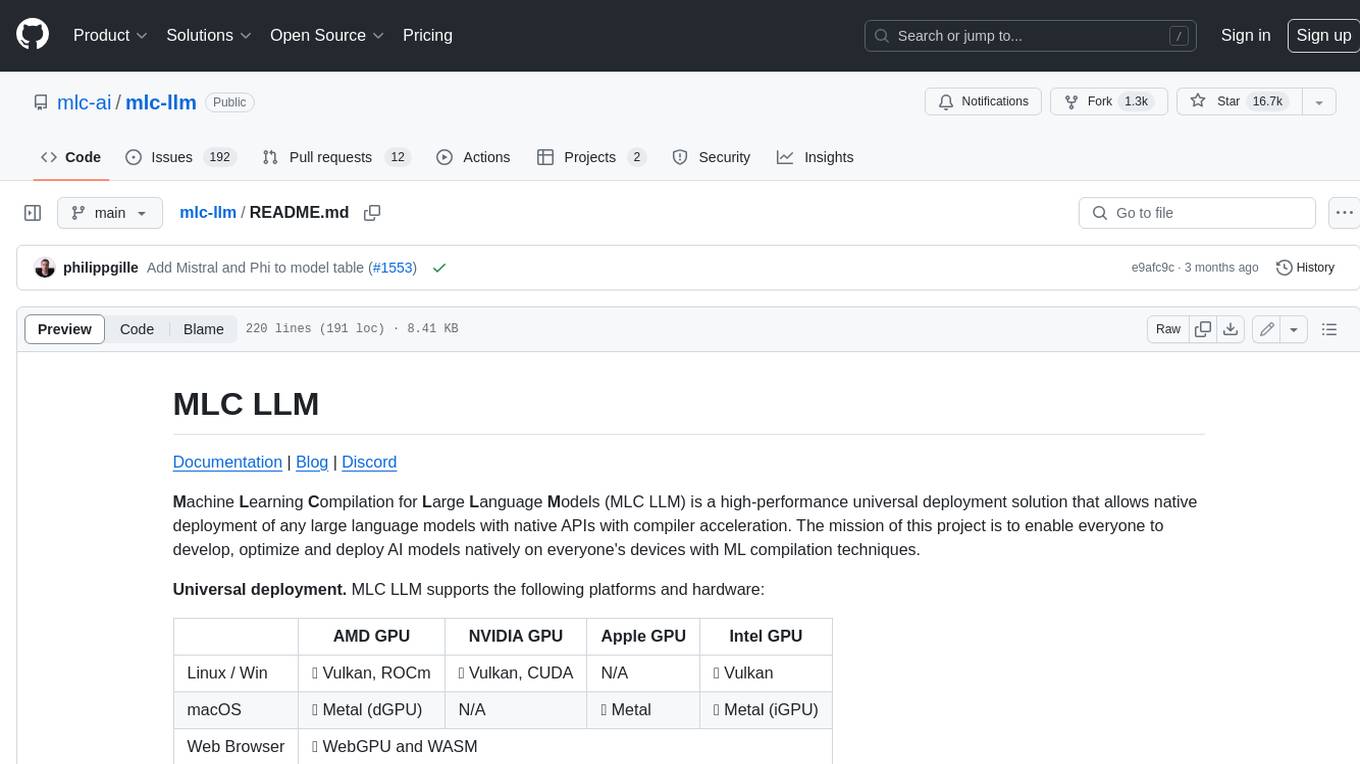
mlc-llm
MLC LLM is a high-performance universal deployment solution that allows native deployment of any large language models with native APIs with compiler acceleration. It supports a wide range of model architectures and variants, including Llama, GPT-NeoX, GPT-J, RWKV, MiniGPT, GPTBigCode, ChatGLM, StableLM, Mistral, and Phi. MLC LLM provides multiple sets of APIs across platforms and environments, including Python API, OpenAI-compatible Rest-API, C++ API, JavaScript API and Web LLM, Swift API for iOS App, and Java API and Android App.
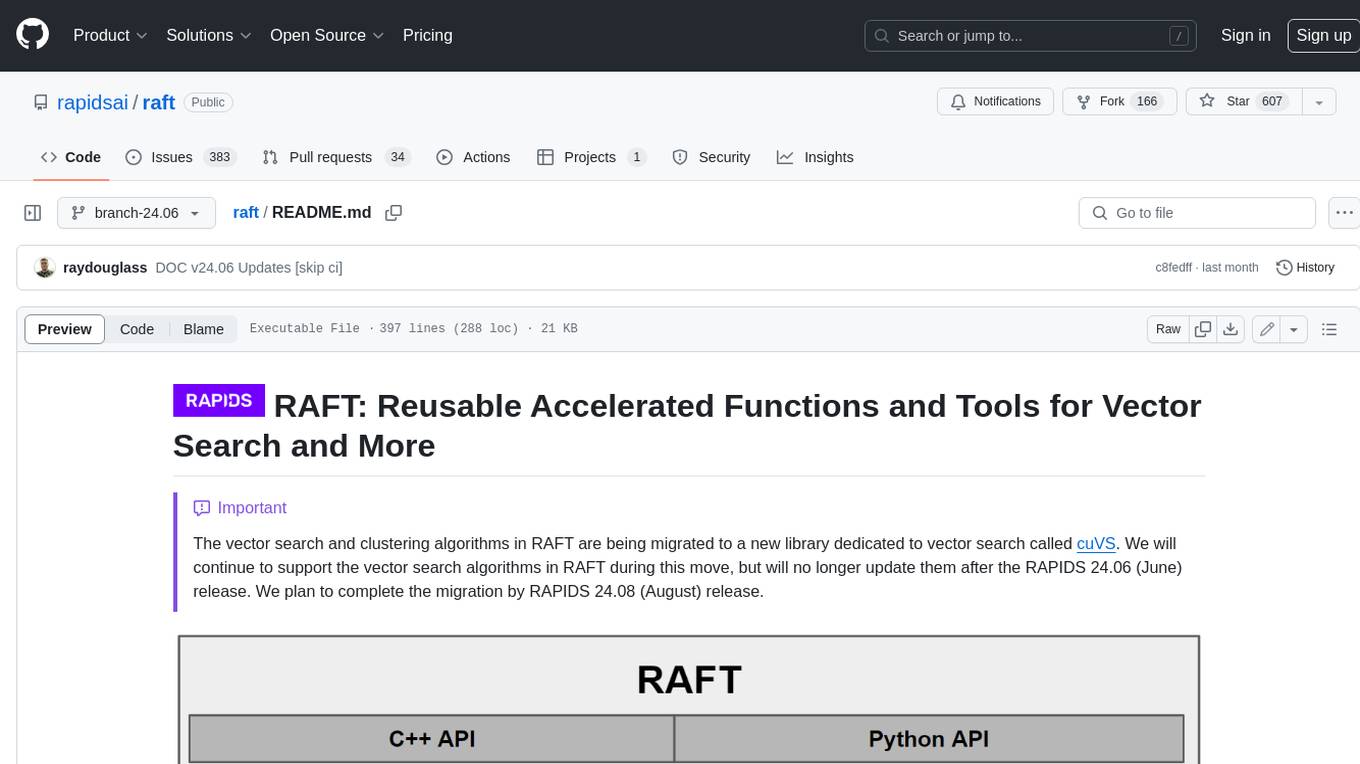
raft
RAFT (Reusable Accelerated Functions and Tools) is a C++ header-only template library with an optional shared library that contains fundamental widely-used algorithms and primitives for machine learning and information retrieval. The algorithms are CUDA-accelerated and form building blocks for more easily writing high performance applications.
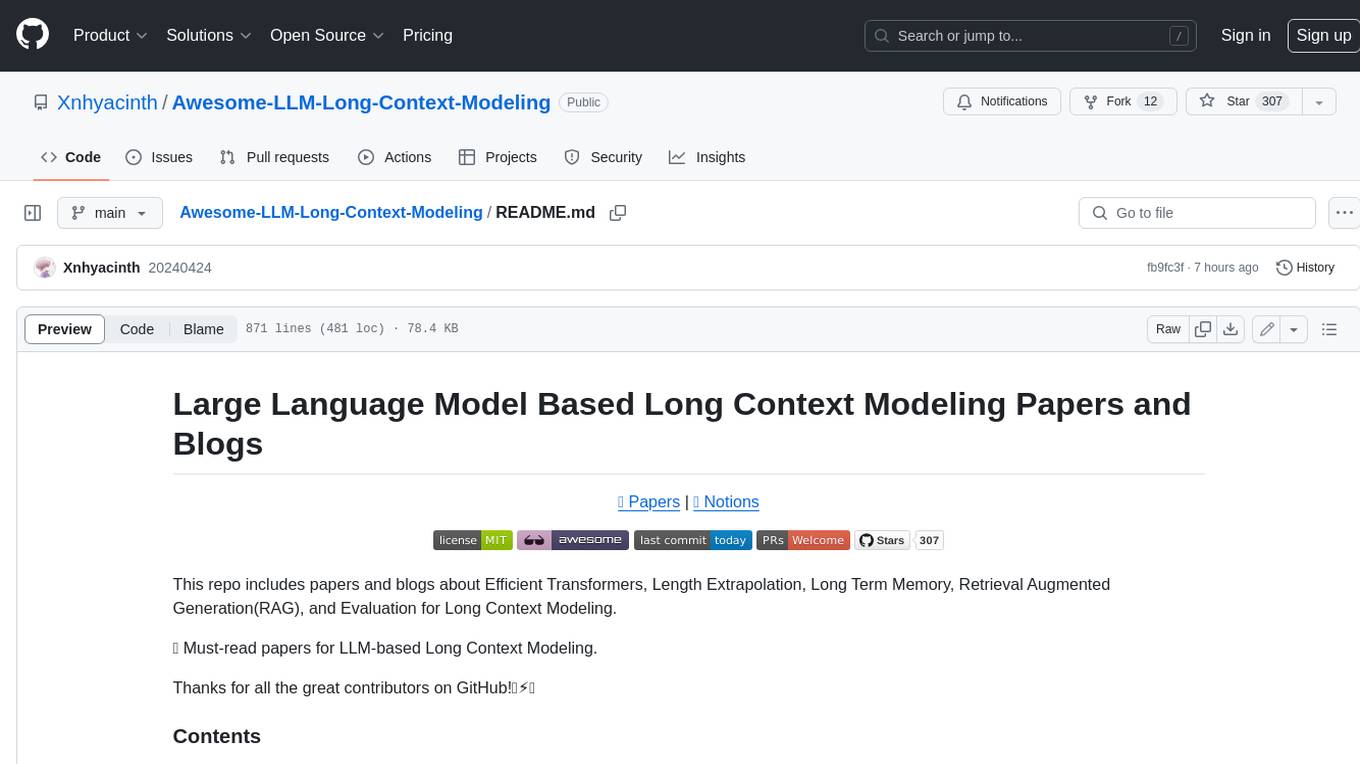
Awesome-LLM-Long-Context-Modeling
This repository includes papers and blogs about Efficient Transformers, Length Extrapolation, Long Term Memory, Retrieval Augmented Generation(RAG), and Evaluation for Long Context Modeling.
For similar jobs
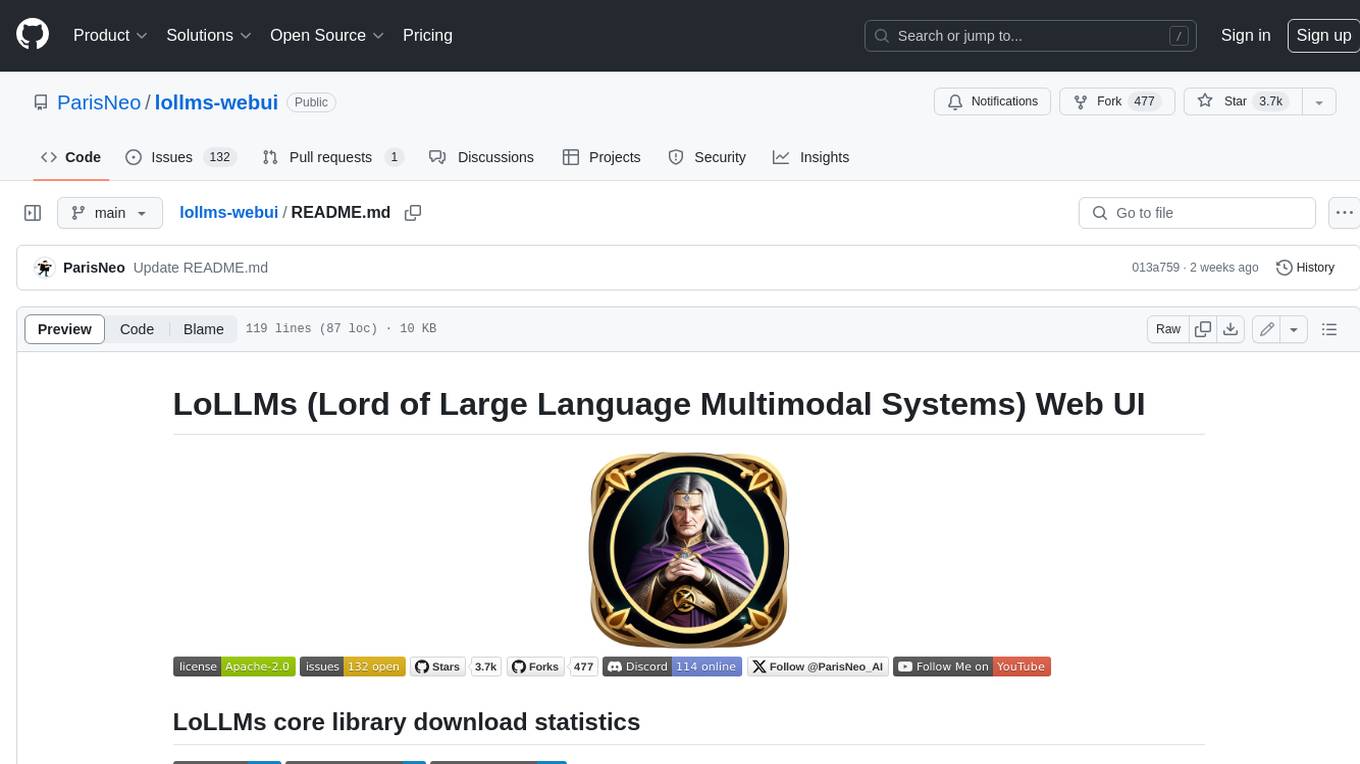
lollms-webui
LoLLMs WebUI (Lord of Large Language Multimodal Systems: One tool to rule them all) is a user-friendly interface to access and utilize various LLM (Large Language Models) and other AI models for a wide range of tasks. With over 500 AI expert conditionings across diverse domains and more than 2500 fine tuned models over multiple domains, LoLLMs WebUI provides an immediate resource for any problem, from car repair to coding assistance, legal matters, medical diagnosis, entertainment, and more. The easy-to-use UI with light and dark mode options, integration with GitHub repository, support for different personalities, and features like thumb up/down rating, copy, edit, and remove messages, local database storage, search, export, and delete multiple discussions, make LoLLMs WebUI a powerful and versatile tool.
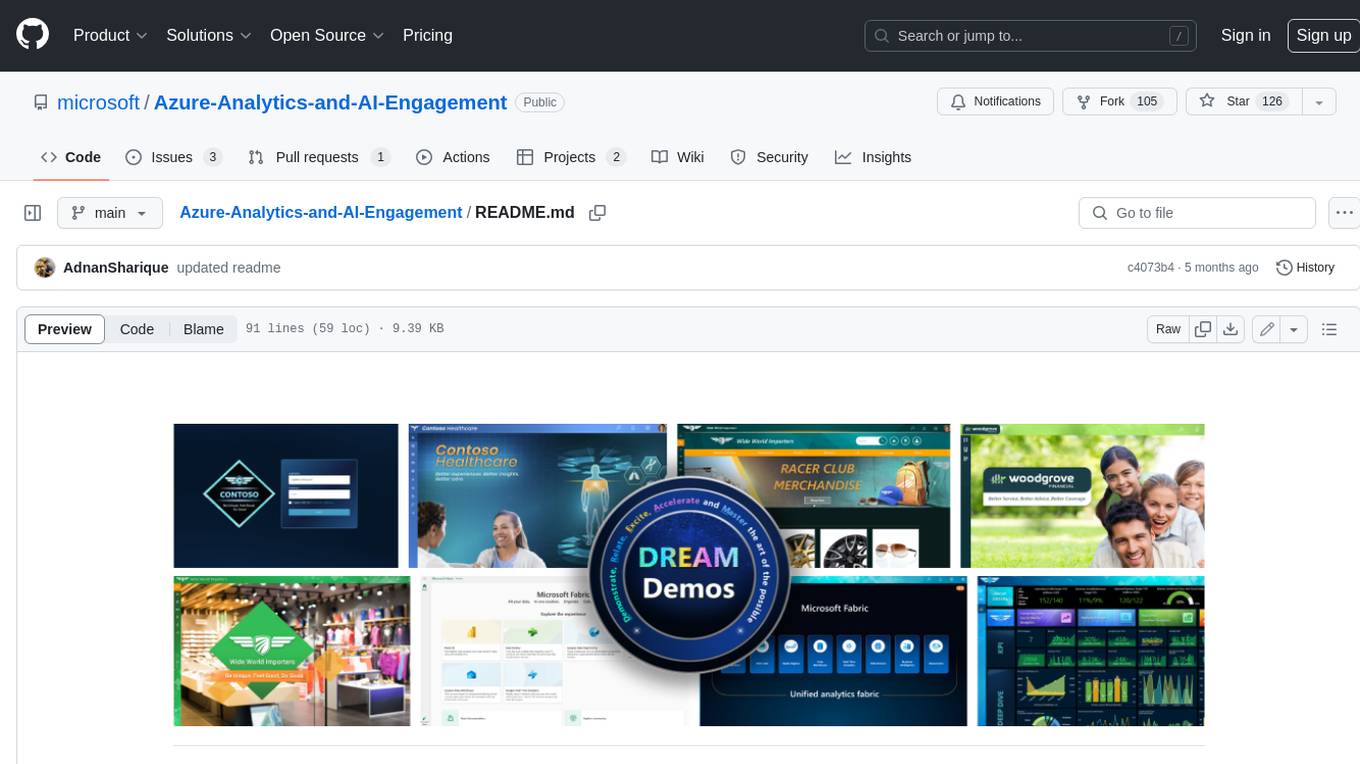
Azure-Analytics-and-AI-Engagement
The Azure-Analytics-and-AI-Engagement repository provides packaged Industry Scenario DREAM Demos with ARM templates (Containing a demo web application, Power BI reports, Synapse resources, AML Notebooks etc.) that can be deployed in a customer’s subscription using the CAPE tool within a matter of few hours. Partners can also deploy DREAM Demos in their own subscriptions using DPoC.
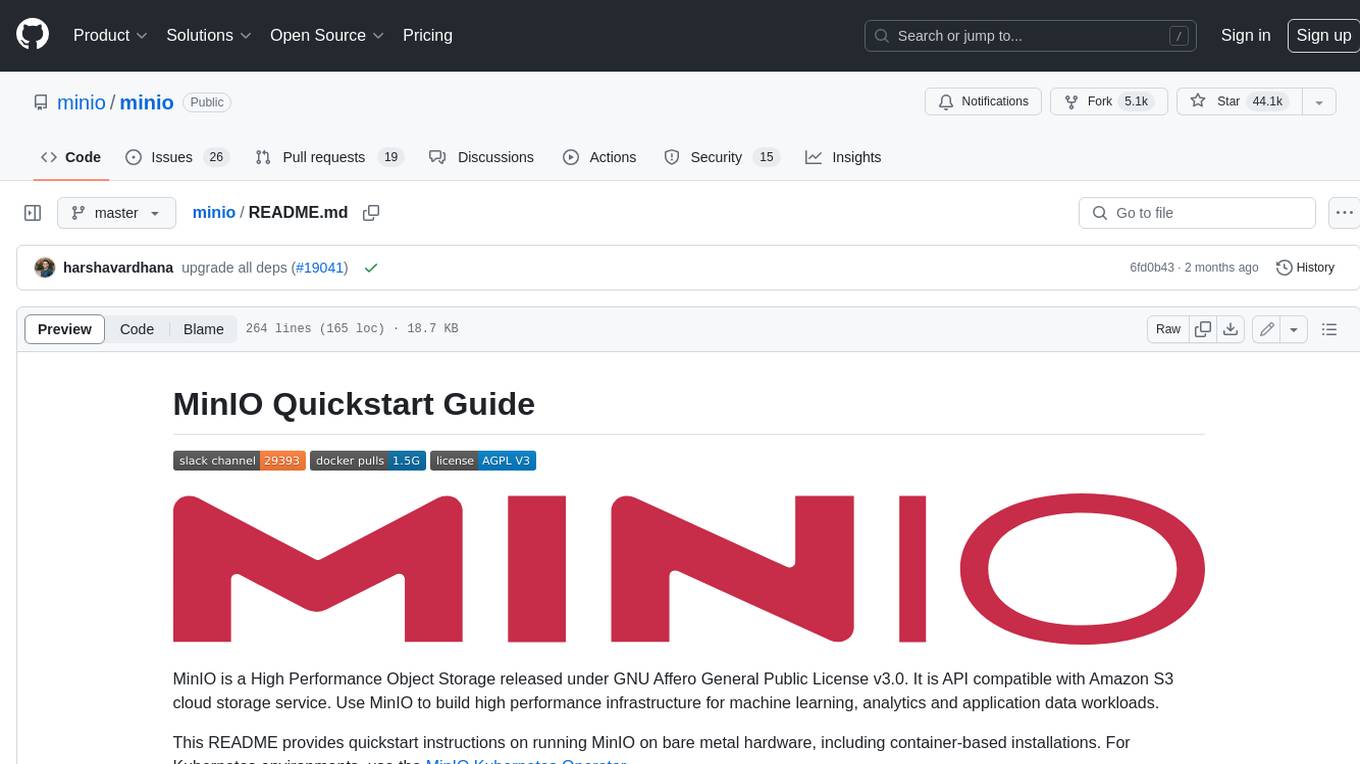
minio
MinIO is a High Performance Object Storage released under GNU Affero General Public License v3.0. It is API compatible with Amazon S3 cloud storage service. Use MinIO to build high performance infrastructure for machine learning, analytics and application data workloads.
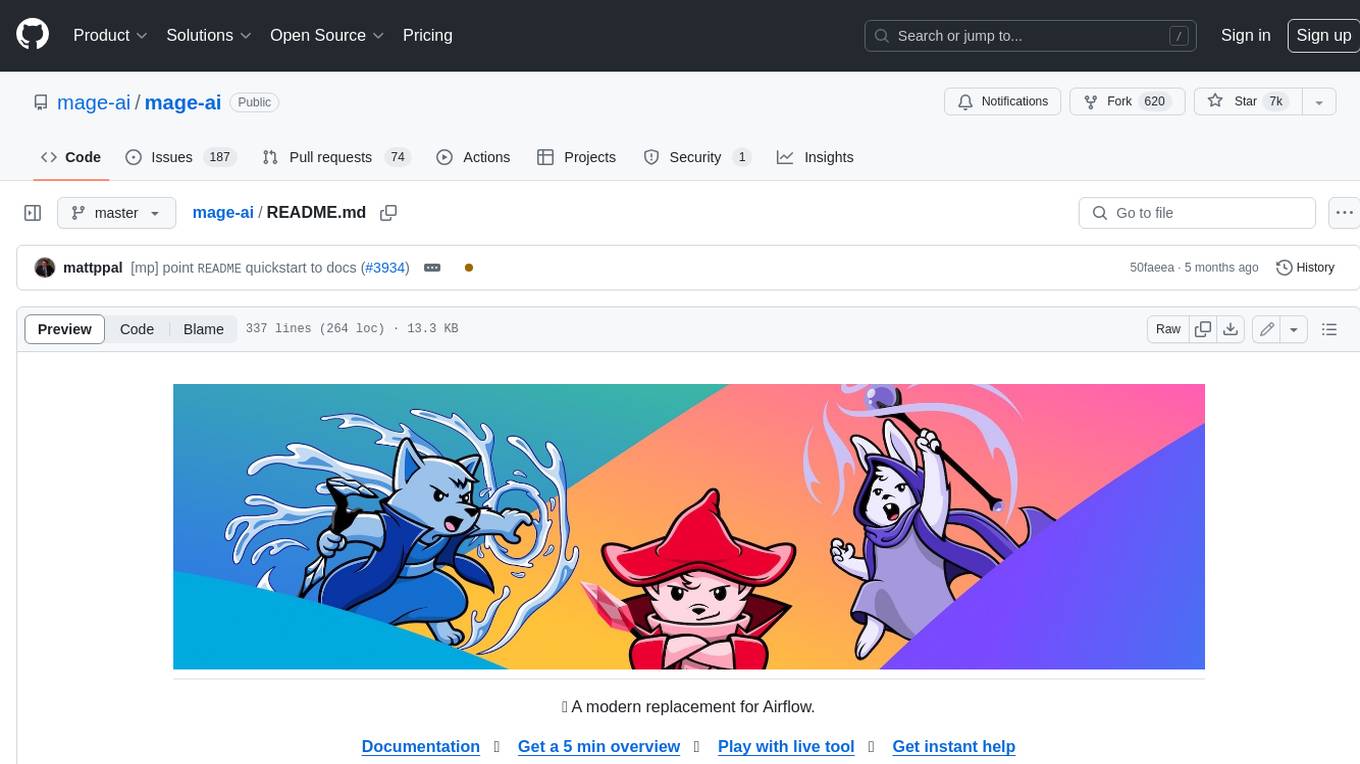
mage-ai
Mage is an open-source data pipeline tool for transforming and integrating data. It offers an easy developer experience, engineering best practices built-in, and data as a first-class citizen. Mage makes it easy to build, preview, and launch data pipelines, and provides observability and scaling capabilities. It supports data integrations, streaming pipelines, and dbt integration.
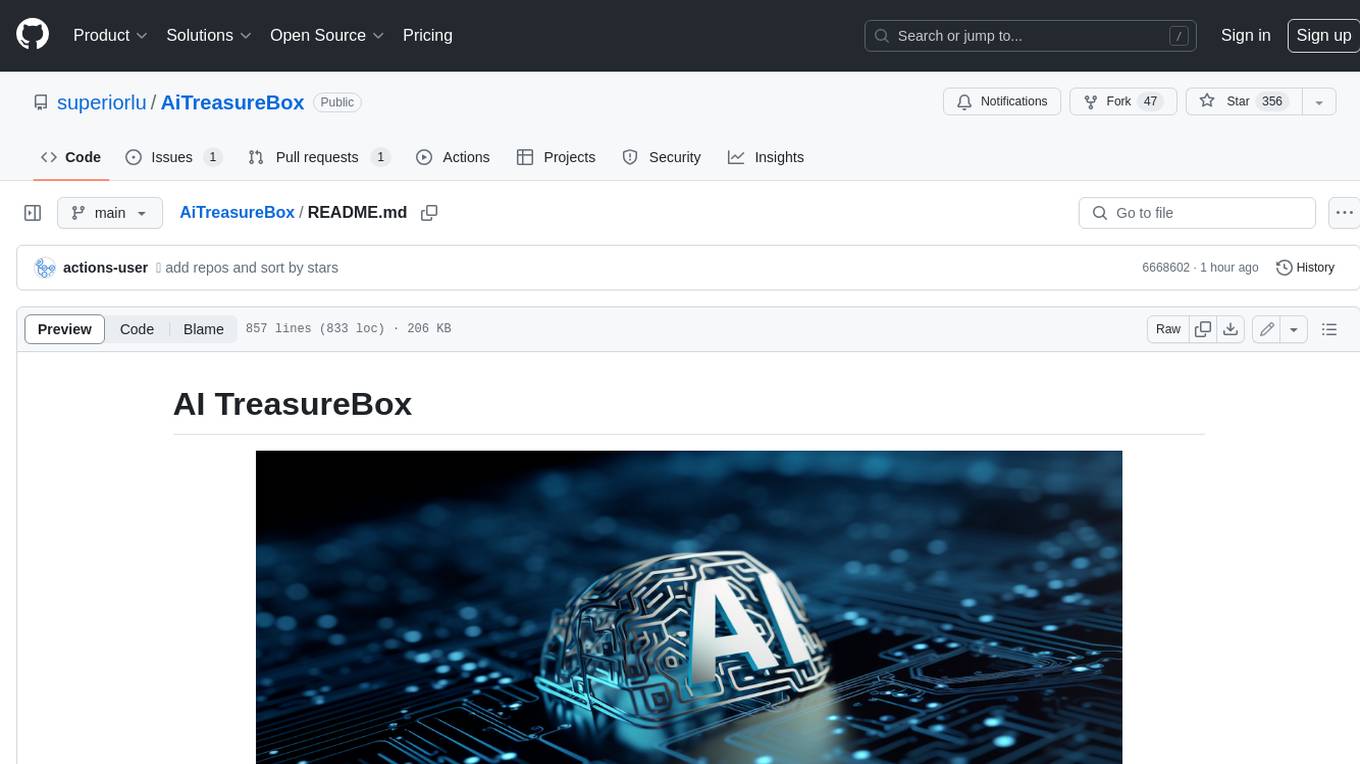
AiTreasureBox
AiTreasureBox is a versatile AI tool that provides a collection of pre-trained models and algorithms for various machine learning tasks. It simplifies the process of implementing AI solutions by offering ready-to-use components that can be easily integrated into projects. With AiTreasureBox, users can quickly prototype and deploy AI applications without the need for extensive knowledge in machine learning or deep learning. The tool covers a wide range of tasks such as image classification, text generation, sentiment analysis, object detection, and more. It is designed to be user-friendly and accessible to both beginners and experienced developers, making AI development more efficient and accessible to a wider audience.
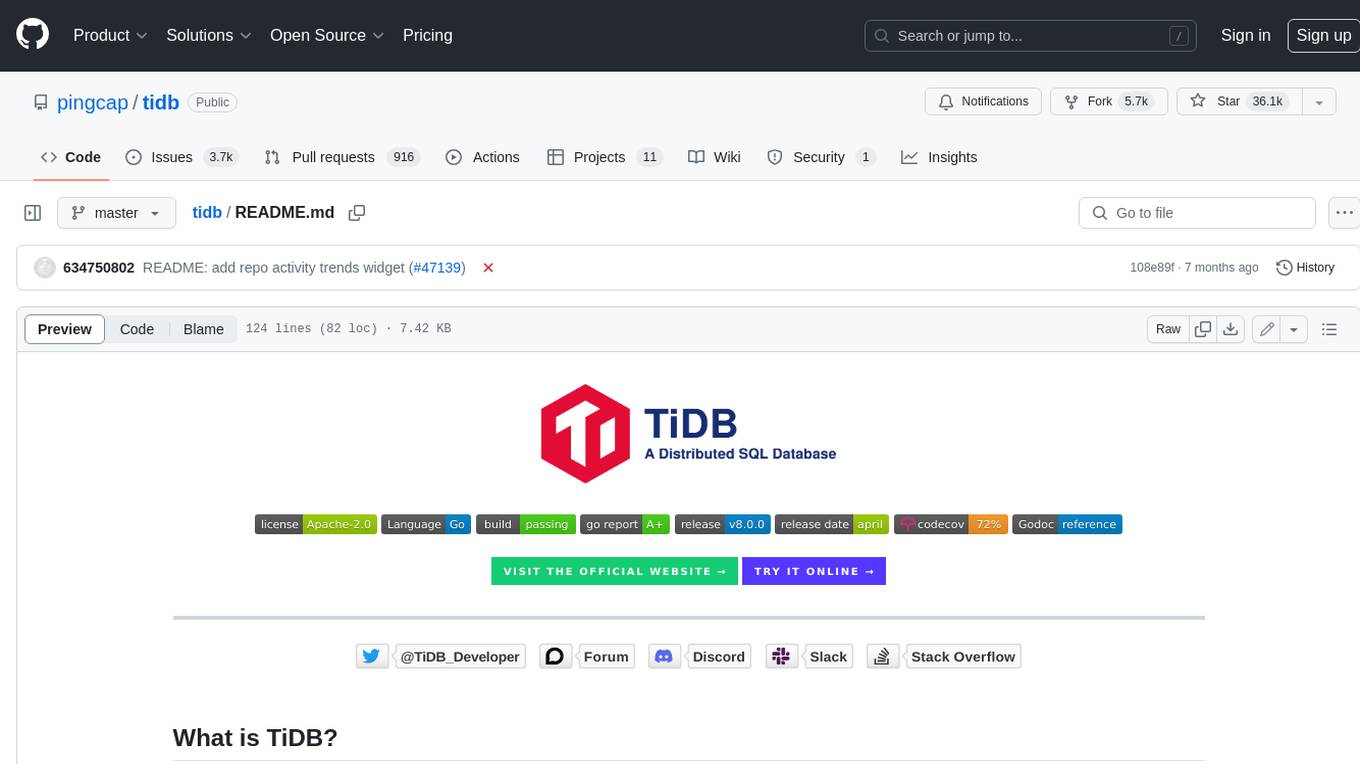
tidb
TiDB is an open-source distributed SQL database that supports Hybrid Transactional and Analytical Processing (HTAP) workloads. It is MySQL compatible and features horizontal scalability, strong consistency, and high availability.
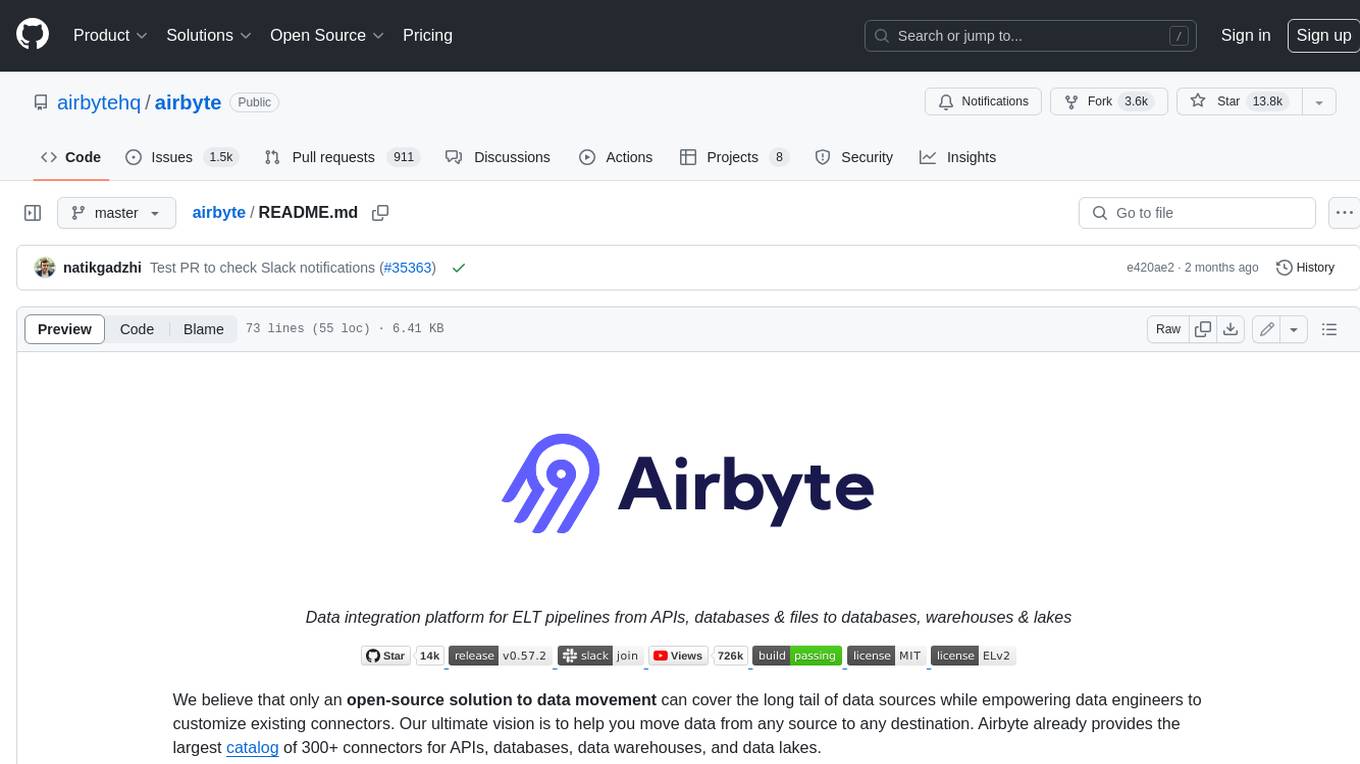
airbyte
Airbyte is an open-source data integration platform that makes it easy to move data from any source to any destination. With Airbyte, you can build and manage data pipelines without writing any code. Airbyte provides a library of pre-built connectors that make it easy to connect to popular data sources and destinations. You can also create your own connectors using Airbyte's no-code Connector Builder or low-code CDK. Airbyte is used by data engineers and analysts at companies of all sizes to build and manage their data pipelines.
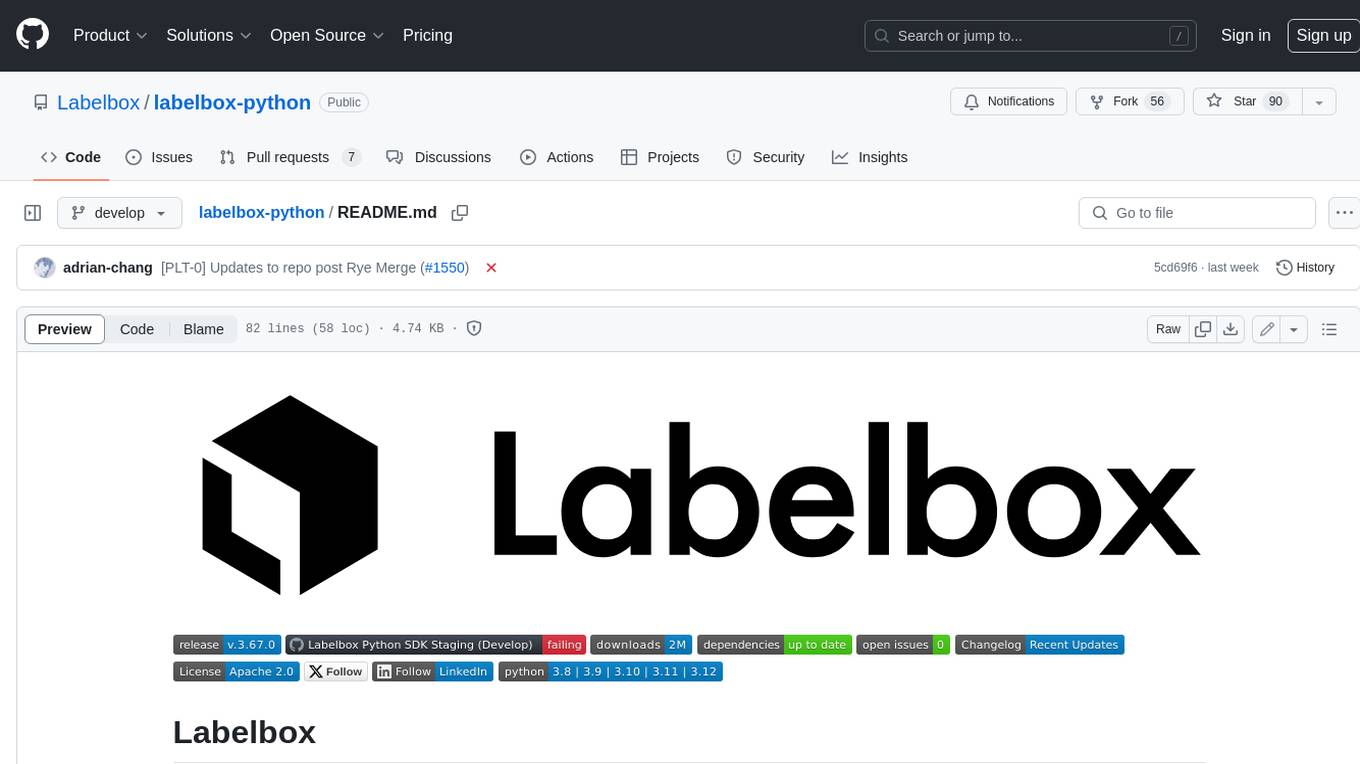
labelbox-python
Labelbox is a data-centric AI platform for enterprises to develop, optimize, and use AI to solve problems and power new products and services. Enterprises use Labelbox to curate data, generate high-quality human feedback data for computer vision and LLMs, evaluate model performance, and automate tasks by combining AI and human-centric workflows. The academic & research community uses Labelbox for cutting-edge AI research.