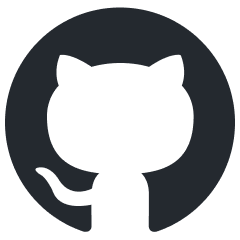
DevOpsGPT
Multi agent system for AI-driven software development. Combine LLM with DevOps tools to convert natural language requirements into working software. Supports any development language and extends the existing code.
Stars: 6324
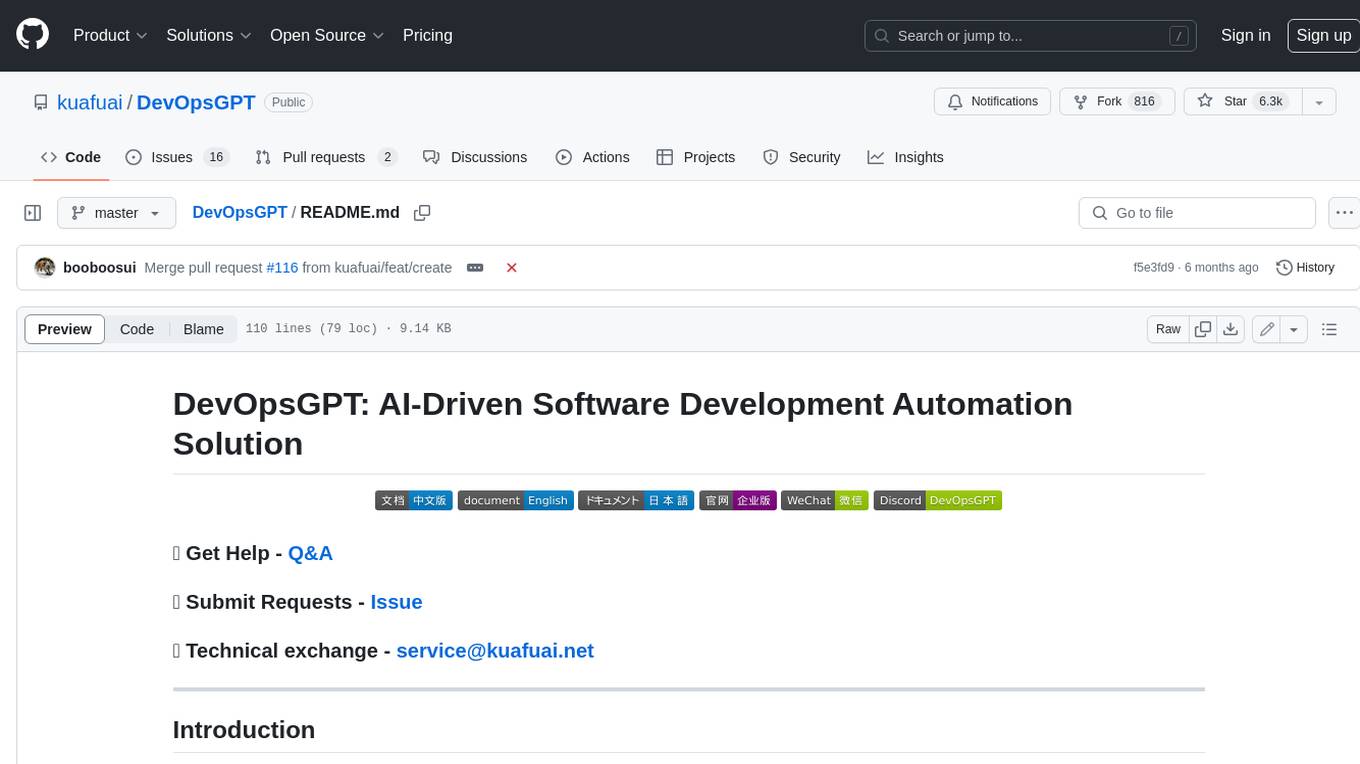
DevOpsGPT is an AI-driven software development automation solution that combines Large Language Models (LLM) with DevOps tools to convert natural language requirements into working software. It improves development efficiency by eliminating the need for tedious requirement documentation, shortens development cycles, reduces communication costs, and ensures high-quality deliverables. The Enterprise Edition offers features like existing project analysis, professional model selection, and support for more DevOps platforms. The tool automates requirement development, generates interface documentation, provides pseudocode based on existing projects, facilitates code refinement, enables continuous integration, and supports software version release. Users can run DevOpsGPT with source code or Docker, and the tool comes with limitations in precise documentation generation and understanding existing project code. The product roadmap includes accurate requirement decomposition, rapid import of development requirements, and integration of more software engineering and professional tools for efficient software development tasks under AI planning and execution.
README:
💡 Get Help - Q&A
💡 Submit Requests - Issue
💡 Technical exchange - [email protected]
Welcome to the AI Driven Software Development Automation Solution, abbreviated as DevOpsGPT. We combine LLM (Large Language Model) with DevOps tools to convert natural language requirements into working software. This innovative feature greatly improves development efficiency, shortens development cycles, and reduces communication costs, resulting in higher-quality software delivery.
- Improved development efficiency: No need for tedious requirement document writing and explanations. Users can interact directly with DevOpsGPT to quickly convert requirements into functional software.
- Shortened development cycles: The automated software development process significantly reduces delivery time, accelerating software deployment and iterations.
- Reduced communication costs: By accurately understanding user requirements, DevOpsGPT minimizes the risk of communication errors and misunderstandings, enhancing collaboration efficiency between development and business teams.
- High-quality deliverables: DevOpsGPT generates code and performs validation, ensuring the quality and reliability of the delivered software.
- [Enterprise Edition] Existing project analysis: Through AI, automatic analysis of existing project information, accurate decomposition and development of required tasks on the basis of existing projects.
- [Enterprise Edition] Professional model selection: Support language model services stronger than GPT in the professional field to better complete requirements development tasks, and support private deployment.
- [Enterprise Edition] Support more DevOps platforms: can connect with more DevOps platforms to achieve the development and deployment of the whole process.
- DevOpsGPT Vision video
- Demo - Software development and deployment to Cloud
- Demo - Develop an API for adding users in Java SpringBoot
Through the above introduction and Demo demonstration, you must be curious about how DevOpsGPT achieves the entire process of automated requirement development in an existing project. Below is a brief overview of the entire process:
- Clarify requirement documents: Interact with DevOpsGPT to clarify and confirm details in requirement documents.
- Generate interface documentation: DevOpsGPT can generate interface documentation based on the requirements, facilitating interface design and implementation for developers.
- Write pseudocode based on existing projects: Analyze existing projects to generate corresponding pseudocode, providing developers with references and starting points.
- Refine and optimize code functionality: Developers improve and optimize functionality based on the generated code.
- Continuous integration: Utilize DevOps tools for continuous integration to automate code integration and testing.
- Software version release: Deploy software versions to the target environment using DevOpsGPT and DevOps tools.
Vists kuafuai.net
-
Run with source code
- Download the released version, or clone the latest code(instability), Ensure SQLite and Python3.7 or later is ready.
- Generate the configuration file: Copy
env.yaml.tpl
and rename it toenv.yaml
. - Modify the configuration file: Edit
env.yaml
and add the necessary information such as GPT Token (refer to documentation link for detailed instructions). - Run the service: Execute
sh run.sh
on Linux or Mac, or double-clickrun.bat
on Windows. - Access the service: Access the service through a browser (check the startup log for the access address, default is http://127.0.0.1:8080).
- Complete requirement development: Follow the instructions on the page to complete requirement development, and view the generated code in the
./workspace
directory.
-
Run with Docker
- Create a directory:
mkdir -p workspace
- Copy env.yaml.tpl from the repository to the current directory and rename it to
env.yaml
- Modify the configuration file: edit
env.yaml
and add necessary information such as GPT Token. -
docker run -it \ -v$PWD/workspace:/app/workspace \ -v$PWD/env.yaml:/app/env.yaml \ -p8080:8080 -p8081:8081 kuafuai/devopsgpt:latest
- Access the service: Access the service through a browser (access address provided in the startup log, the default is http://127.0.0.1:8080).
- Complete the requirement development: complete the requirement development according to the guidance of the page, and view the generated code in the
./workspace
directory
- Create a directory:
For detailed documentation and configuration parameters, please refer to the documentation link.
Although we strive to enhance enterprise-level software development efficiency and reduce barriers with the help of large-scale language models, there are still some limitations in the current version:
- The generation of requirement and interface documentation may not be precise enough and might not meet developer intent in complex scenarios.
- In the current version, automating the understanding of existing project code is not possible. We are exploring a new solution that has shown promising results during validation and will be introduced in a future version.
- Accurate requirement decomposition and development task breakdown based on existing projects.
- New product experiences for rapid import of development requirements and parallel automation of software development and deployment.
- Introduce more software engineering tools and professional tools to quickly complete various software development tasks under AI planning and execution.
We invite you to participate in the DevOpsGPT project and contribute to the automation and innovation of software development, creating smarter and more efficient software systems!
This project, DevOpsGPT, is an experimental application and is provided "as-is" without any warranty, express or implied. By using this software, you agree to assume all risks associated with its use, including but not limited to data loss, system failure, or any other issues that may arise.
The developers and contributors of this project do not accept any responsibility or liability for any losses, damages, or other consequences that may occur as a result of using this software. You are solely responsible for any decisions and actions taken based on the information provided by DevOpsGPT.
Please note that the use of the GPT language model can be expensive due to its token usage. By utilizing this project, you acknowledge that you are responsible for monitoring and managing your own token usage and the associated costs. It is highly recommended to check your OpenAI API usage regularly and set up any necessary limits or alerts to prevent unexpected charges.
As an autonomous experiment, DevOpsGPT may generate content or take actions that are not in line with real-world business practices or legal requirements. It is your responsibility to ensure that any actions or decisions made based on the output of this software comply with all applicable laws, regulations, and ethical standards. The developers and contributors of this project shall not be held responsible for any consequences arising from the use of this software.
By using DevOpsGPT, you agree to indemnify, defend, and hold harmless the developers, contributors, and any affiliated parties from and against any and all claims, damages, losses, liabilities, costs, and expenses (including reasonable attorneys' fees) arising from your use of this software or your violation of these terms.
For Tasks:
Click tags to check more tools for each tasksFor Jobs:
Alternative AI tools for DevOpsGPT
Similar Open Source Tools
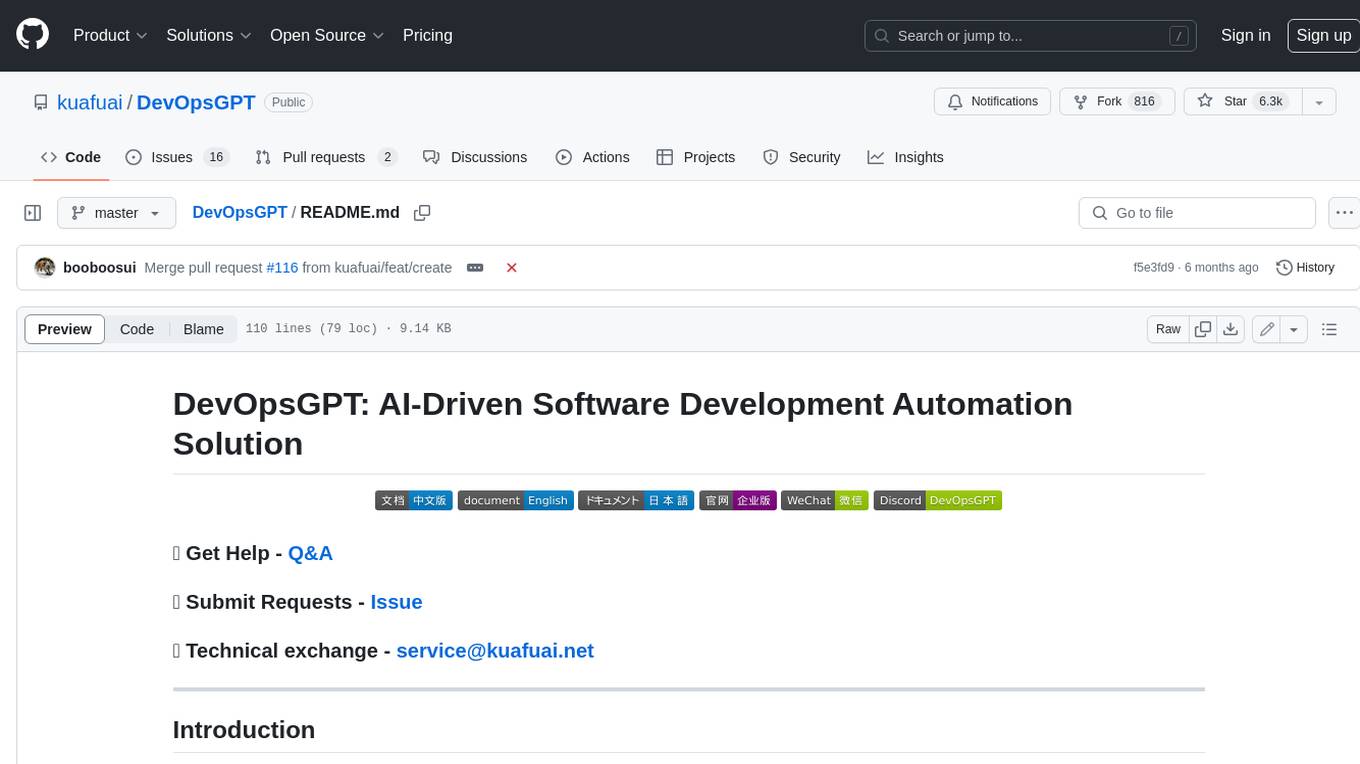
DevOpsGPT
DevOpsGPT is an AI-driven software development automation solution that combines Large Language Models (LLM) with DevOps tools to convert natural language requirements into working software. It improves development efficiency by eliminating the need for tedious requirement documentation, shortens development cycles, reduces communication costs, and ensures high-quality deliverables. The Enterprise Edition offers features like existing project analysis, professional model selection, and support for more DevOps platforms. The tool automates requirement development, generates interface documentation, provides pseudocode based on existing projects, facilitates code refinement, enables continuous integration, and supports software version release. Users can run DevOpsGPT with source code or Docker, and the tool comes with limitations in precise documentation generation and understanding existing project code. The product roadmap includes accurate requirement decomposition, rapid import of development requirements, and integration of more software engineering and professional tools for efficient software development tasks under AI planning and execution.
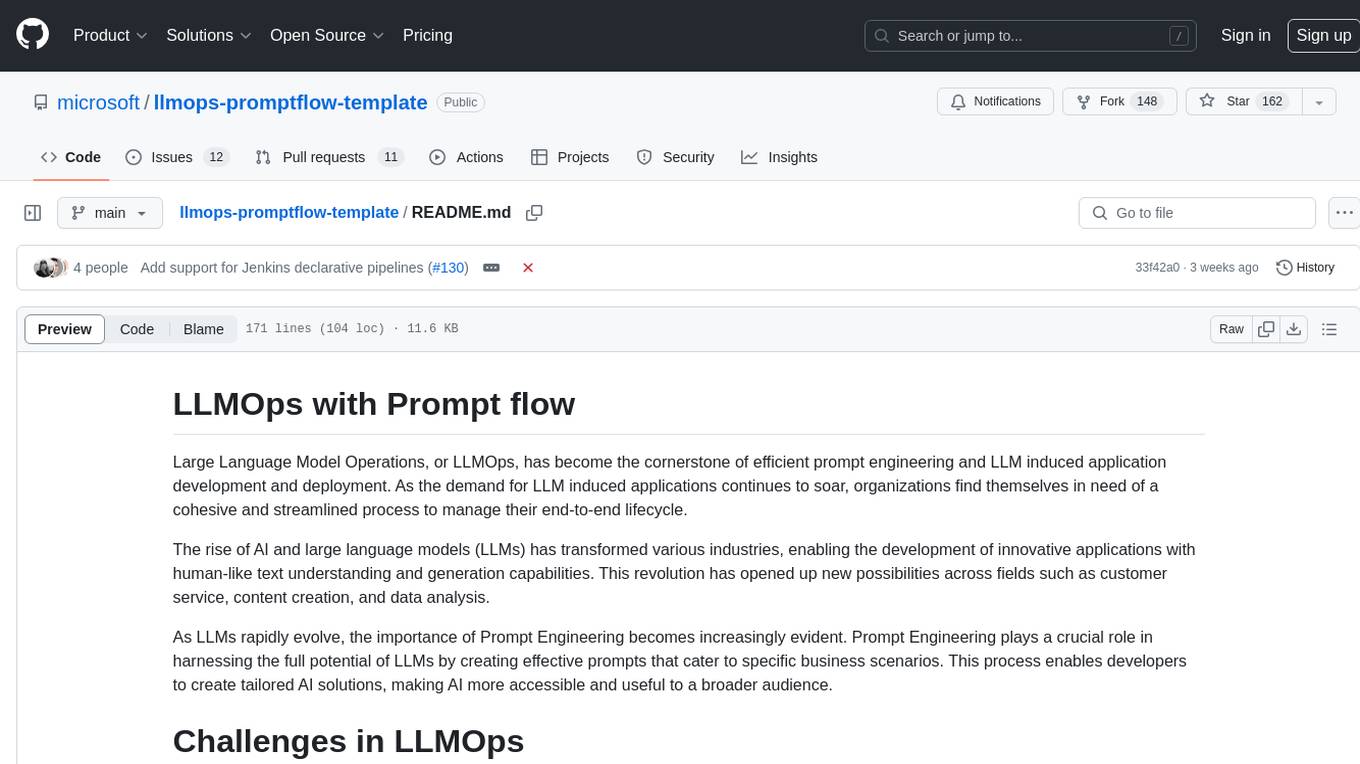
llmops-promptflow-template
LLMOps with Prompt flow is a template and guidance for building LLM-infused apps using Prompt flow. It provides centralized code hosting, lifecycle management, variant and hyperparameter experimentation, A/B deployment, many-to-many dataset/flow relationships, multiple deployment targets, comprehensive reporting, BYOF capabilities, configuration-based development, local prompt experimentation and evaluation, endpoint testing, and optional Human-in-loop validation. The tool is customizable to suit various application needs.
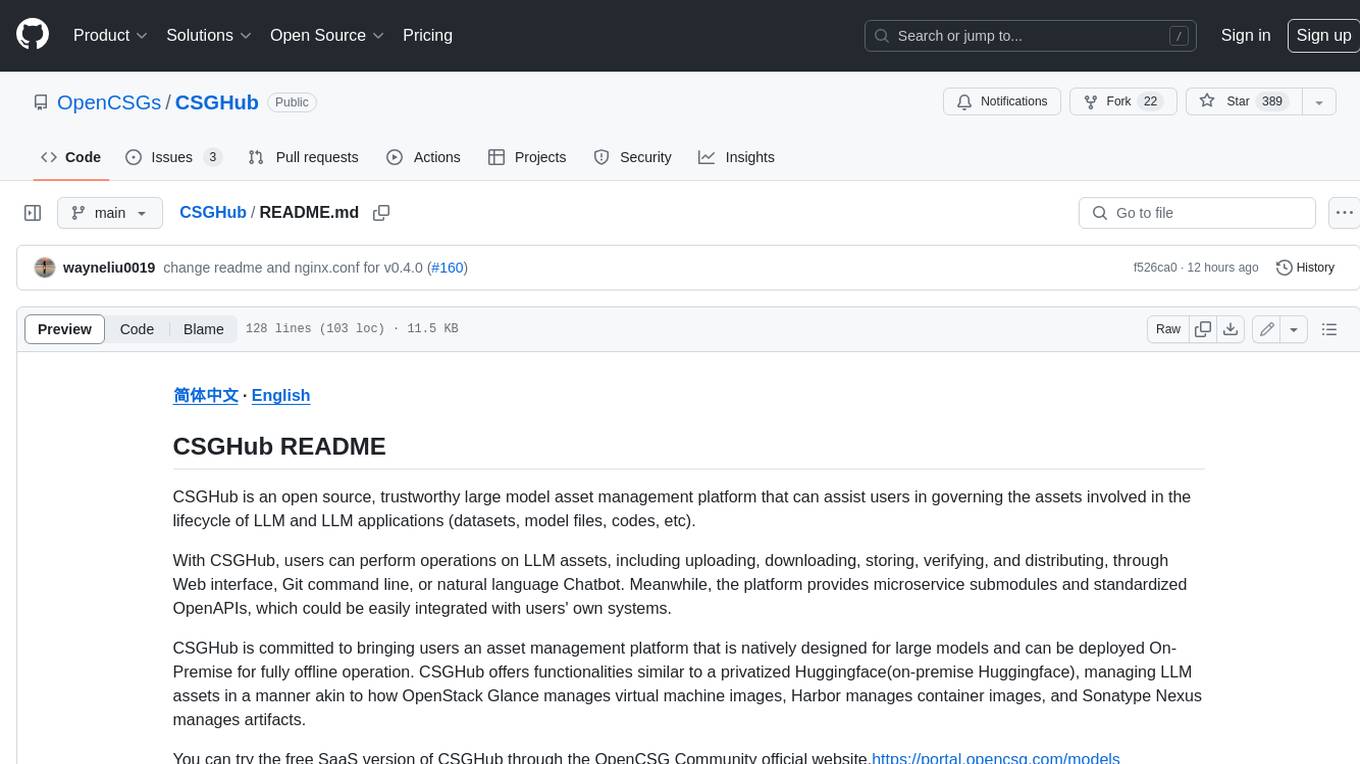
CSGHub
CSGHub is an open source, trustworthy large model asset management platform that can assist users in governing the assets involved in the lifecycle of LLM and LLM applications (datasets, model files, codes, etc). With CSGHub, users can perform operations on LLM assets, including uploading, downloading, storing, verifying, and distributing, through Web interface, Git command line, or natural language Chatbot. Meanwhile, the platform provides microservice submodules and standardized OpenAPIs, which could be easily integrated with users' own systems. CSGHub is committed to bringing users an asset management platform that is natively designed for large models and can be deployed On-Premise for fully offline operation. CSGHub offers functionalities similar to a privatized Huggingface(on-premise Huggingface), managing LLM assets in a manner akin to how OpenStack Glance manages virtual machine images, Harbor manages container images, and Sonatype Nexus manages artifacts.
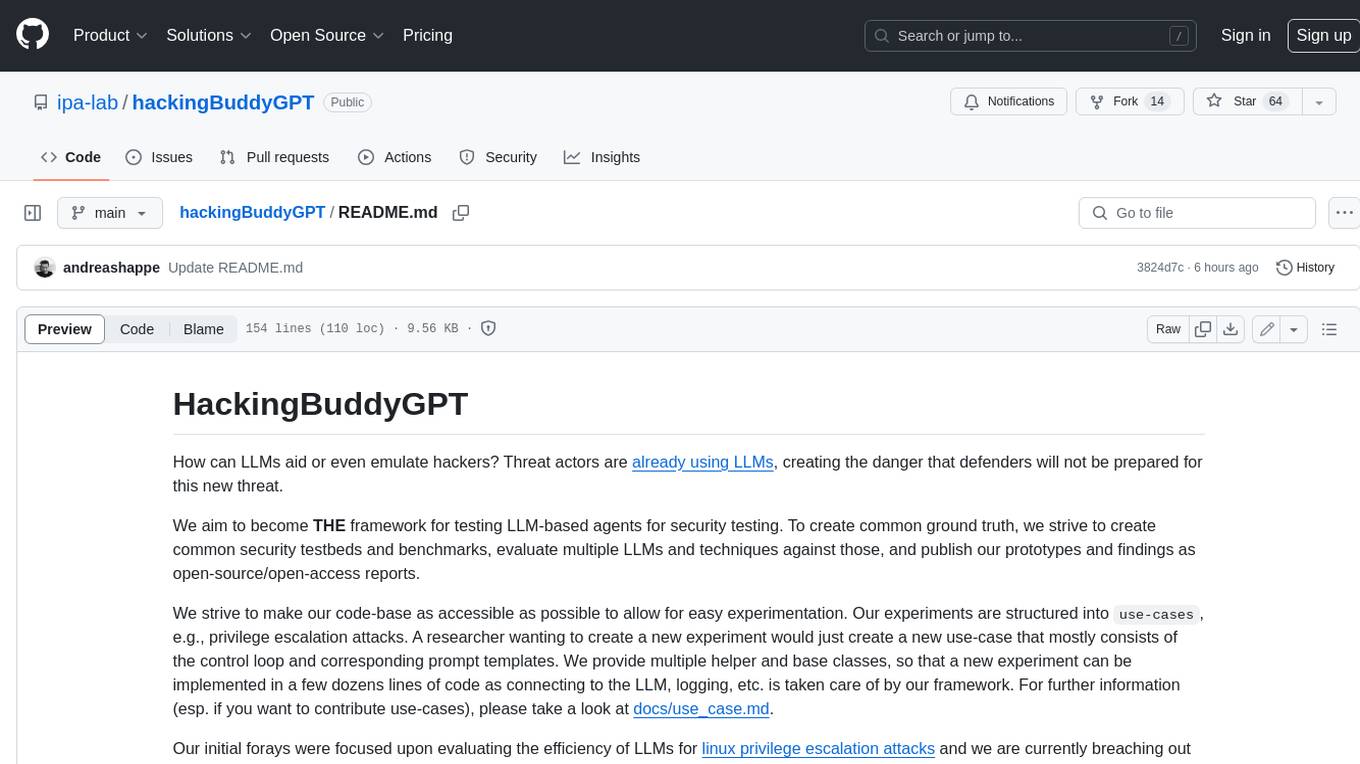
hackingBuddyGPT
hackingBuddyGPT is a framework for testing LLM-based agents for security testing. It aims to create common ground truth by creating common security testbeds and benchmarks, evaluating multiple LLMs and techniques against those, and publishing prototypes and findings as open-source/open-access reports. The initial focus is on evaluating the efficiency of LLMs for Linux privilege escalation attacks, but the framework is being expanded to evaluate the use of LLMs for web penetration-testing and web API testing. hackingBuddyGPT is released as open-source to level the playing field for blue teams against APTs that have access to more sophisticated resources.
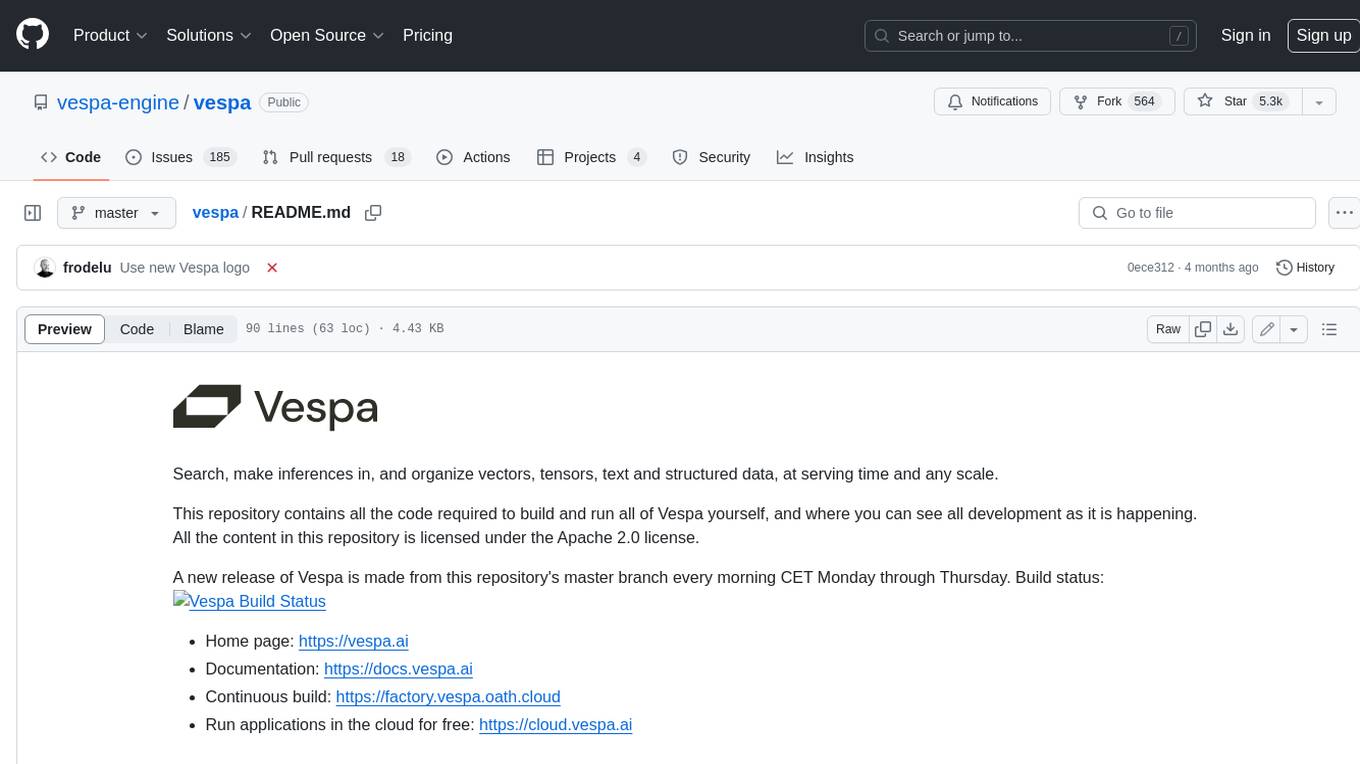
vespa
Vespa is a platform that performs operations such as selecting a subset of data in a large corpus, evaluating machine-learned models over the selected data, organizing and aggregating it, and returning it, typically in less than 100 milliseconds, all while the data corpus is continuously changing. It has been in development for many years and is used on a number of large internet services and apps which serve hundreds of thousands of queries from Vespa per second.
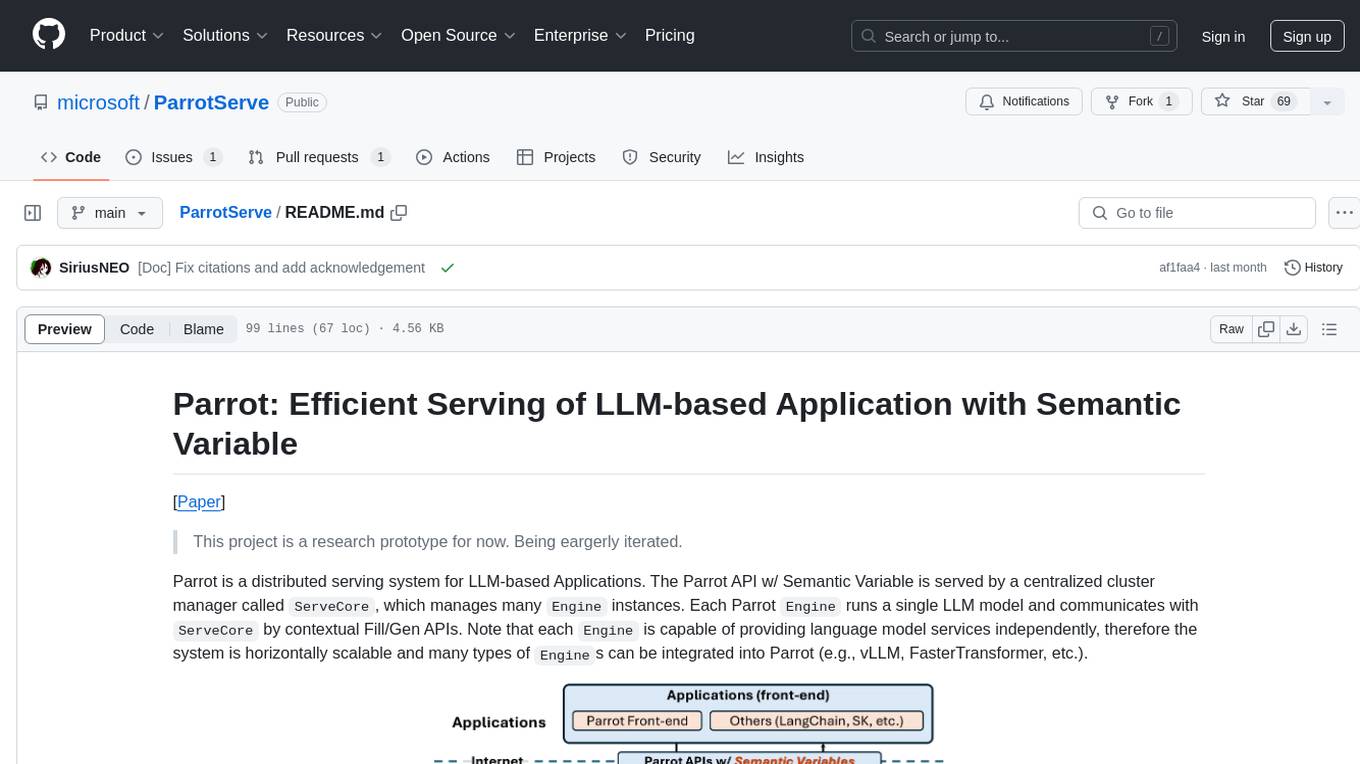
ParrotServe
Parrot is a distributed serving system for LLM-based Applications, designed to efficiently serve LLM-based applications by adding Semantic Variable in the OpenAI-style API. It allows for horizontal scalability with multiple Engine instances running LLM models communicating with ServeCore. The system enables AI agents to interact with LLMs via natural language prompts for collaborative tasks.
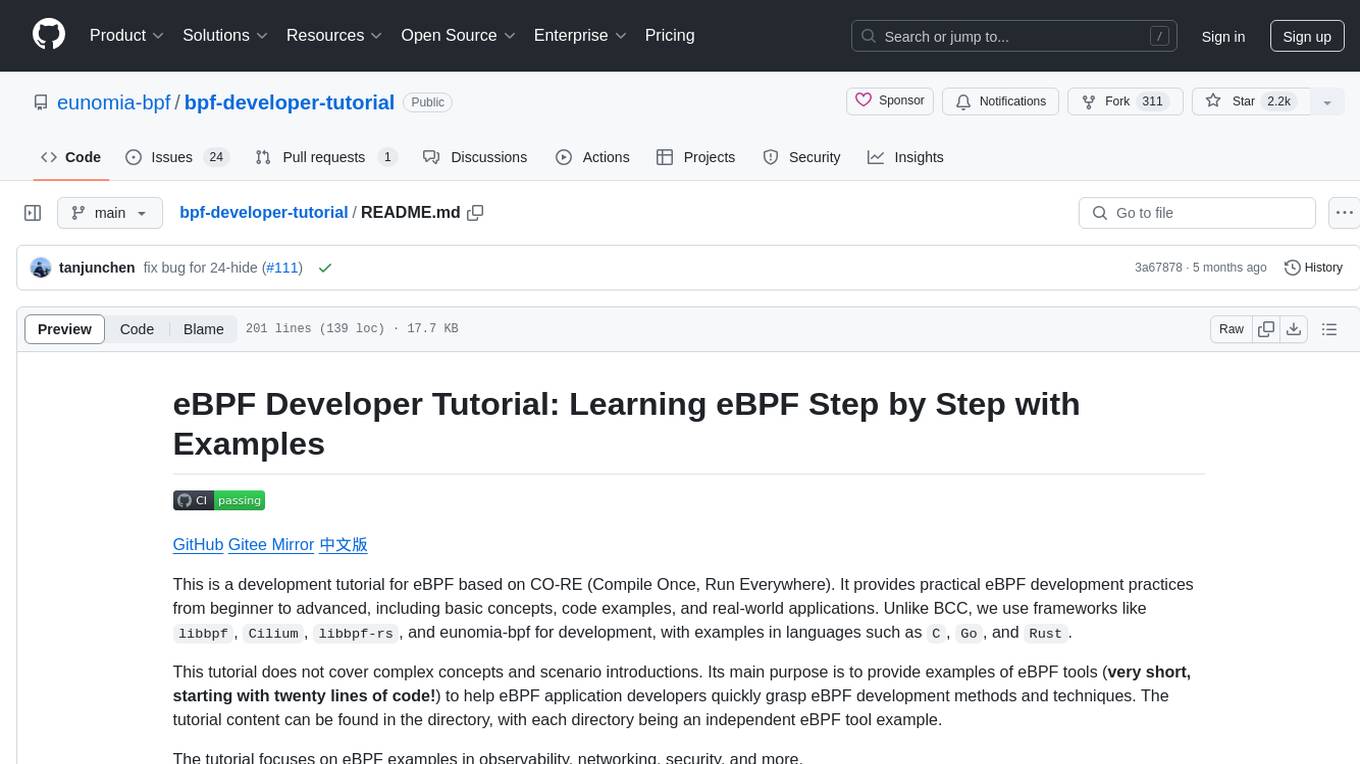
bpf-developer-tutorial
This is a development tutorial for eBPF based on CO-RE (Compile Once, Run Everywhere). It provides practical eBPF development practices from beginner to advanced, including basic concepts, code examples, and real-world applications. The tutorial focuses on eBPF examples in observability, networking, security, and more. It aims to help eBPF application developers quickly grasp eBPF development methods and techniques through examples in languages such as C, Go, and Rust. The tutorial is structured with independent eBPF tool examples in each directory, covering topics like kprobes, fentry, opensnoop, uprobe, sigsnoop, execsnoop, exitsnoop, runqlat, hardirqs, and more. The project is based on libbpf and frameworks like libbpf, Cilium, libbpf-rs, and eunomia-bpf for development.
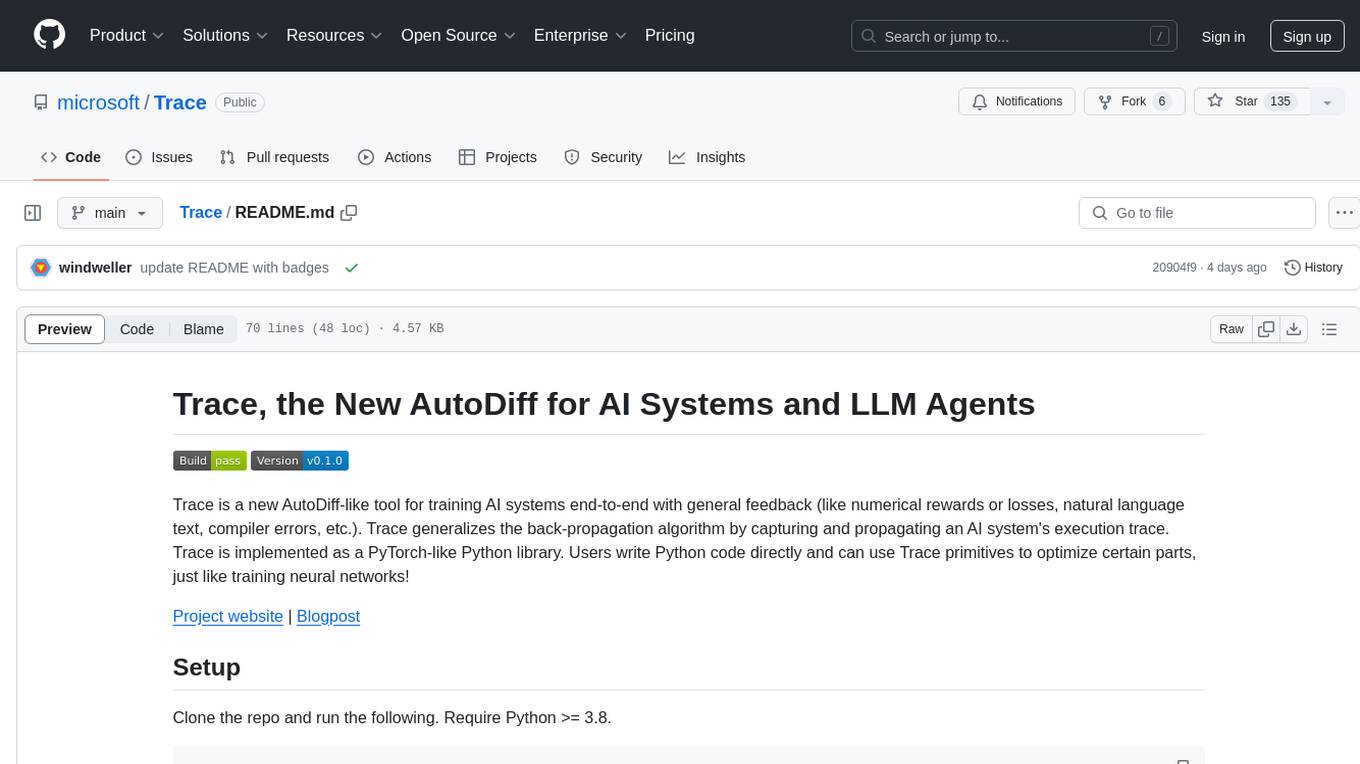
Trace
Trace is a new AutoDiff-like tool for training AI systems end-to-end with general feedback. It generalizes the back-propagation algorithm by capturing and propagating an AI system's execution trace. Implemented as a PyTorch-like Python library, users can write Python code directly and use Trace primitives to optimize certain parts, similar to training neural networks.
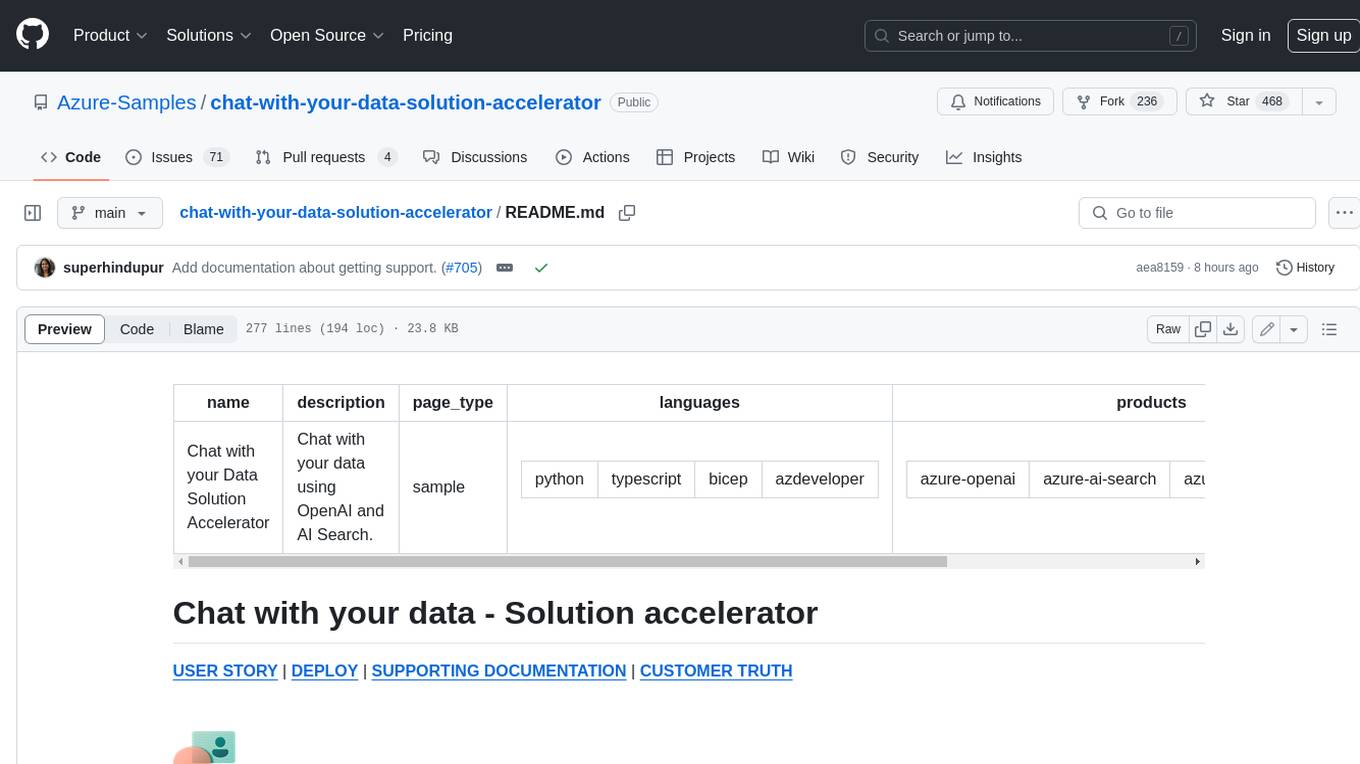
chat-with-your-data-solution-accelerator
Chat with your data using OpenAI and AI Search. This solution accelerator uses an Azure OpenAI GPT model and an Azure AI Search index generated from your data, which is integrated into a web application to provide a natural language interface, including speech-to-text functionality, for search queries. Users can drag and drop files, point to storage, and take care of technical setup to transform documents. There is a web app that users can create in their own subscription with security and authentication.
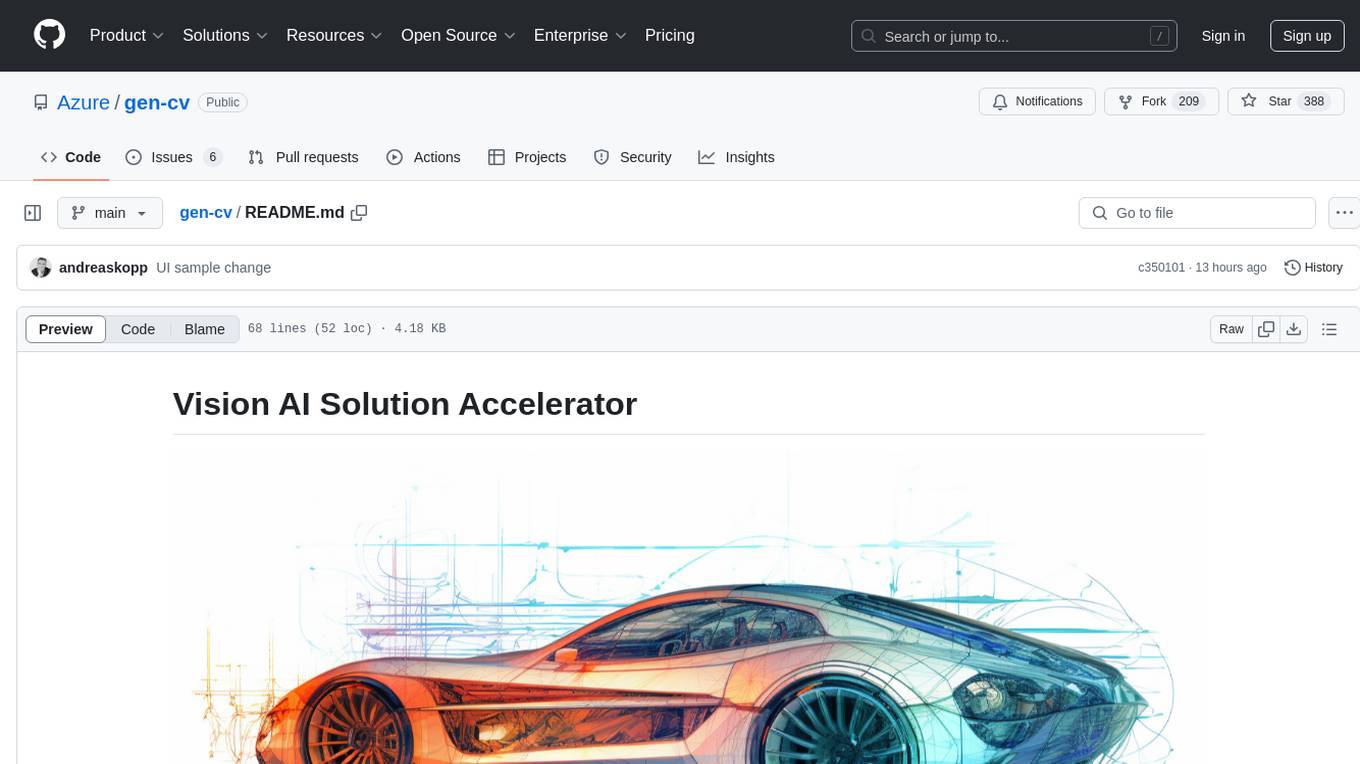
gen-cv
This repository is a rich resource offering examples of synthetic image generation, manipulation, and reasoning using Azure Machine Learning, Computer Vision, OpenAI, and open-source frameworks like Stable Diffusion. It provides practical insights into image processing applications, including content generation, video analysis, avatar creation, and image manipulation with various tools and APIs.
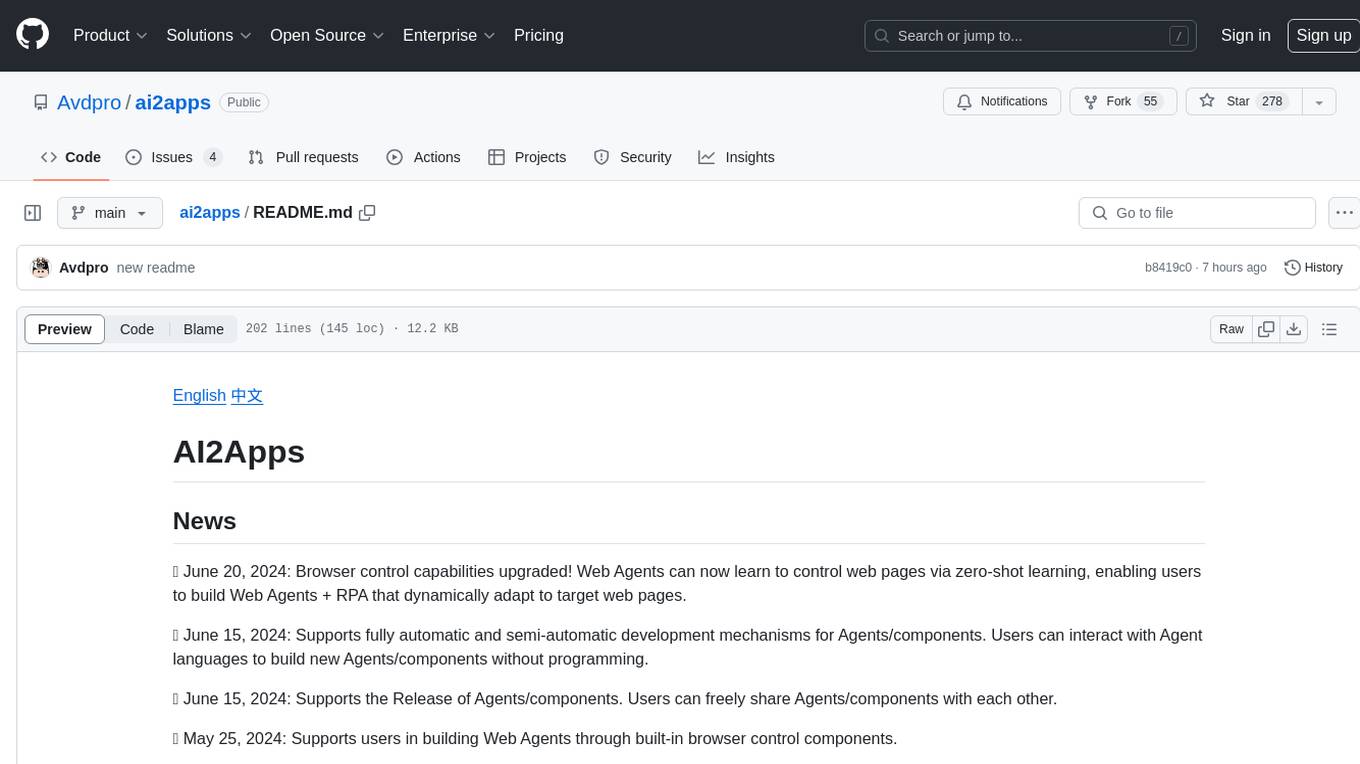
ai2apps
AI2Apps is a visual IDE for building LLM-based AI agent applications, enabling developers to efficiently create AI agents through drag-and-drop, with features like design-to-development for rapid prototyping, direct packaging of agents into apps, powerful debugging capabilities, enhanced user interaction, efficient team collaboration, flexible deployment, multilingual support, simplified product maintenance, and extensibility through plugins.
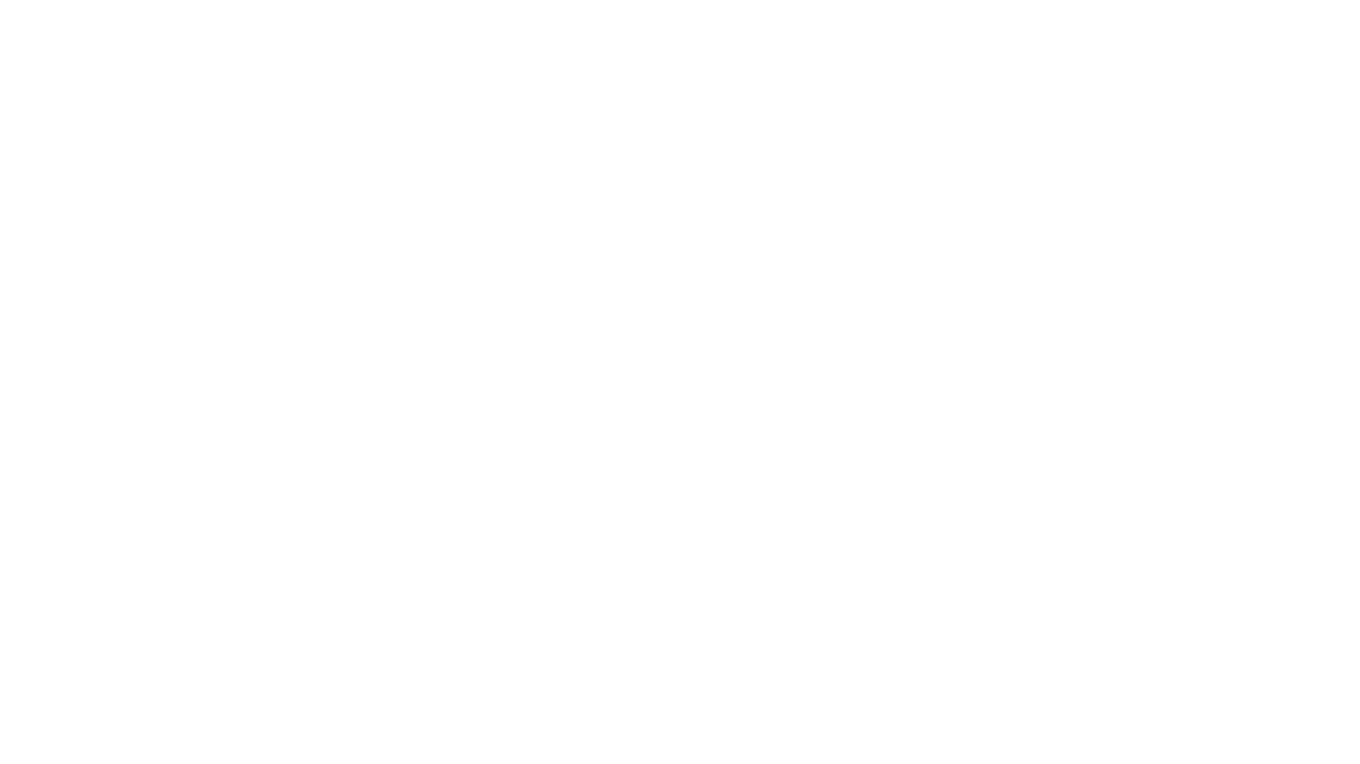
Me-LLaMA
Me LLaMA introduces a suite of open-source medical Large Language Models (LLMs), including Me LLaMA 13B/70B and their chat-enhanced versions. Developed through innovative continual pre-training and instruction tuning, these models leverage a vast medical corpus comprising PubMed papers, medical guidelines, and general domain data. Me LLaMA sets new benchmarks on medical reasoning tasks, making it a significant asset for medical NLP applications and research. The models are intended for computational linguistics and medical research, not for clinical decision-making without validation and regulatory approval.
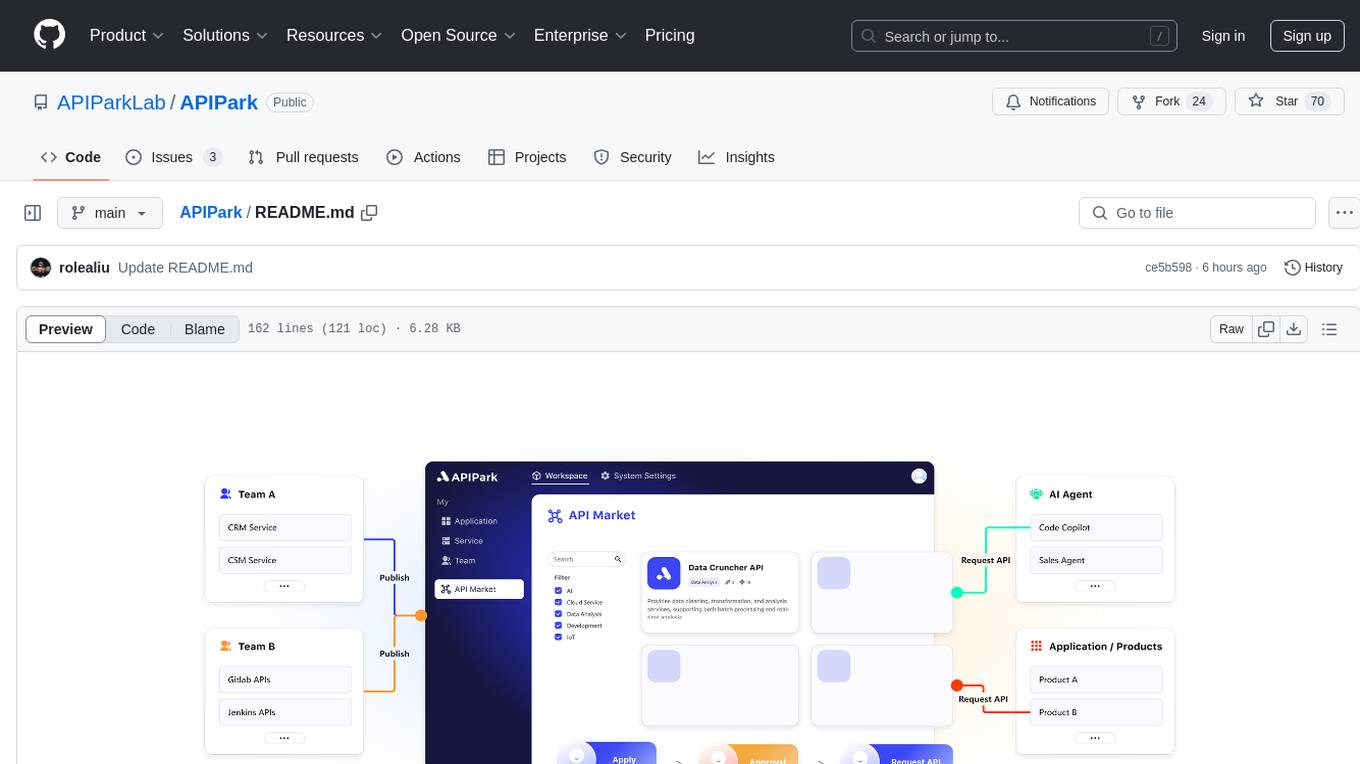
APIPark
APIPark is an open-source AI Gateway and Developer Portal that enables users to easily manage, integrate, and deploy AI and API services. It provides robust API management features, including creation, monitoring, and access control, to help developers efficiently and securely develop and manage their APIs. The platform aims to solve challenges such as connecting to powerful AI models, managing complex AI & API call relationships, overseeing API creation and security, simplifying fault detection and troubleshooting, and enhancing the visibility and valuation of data assets.
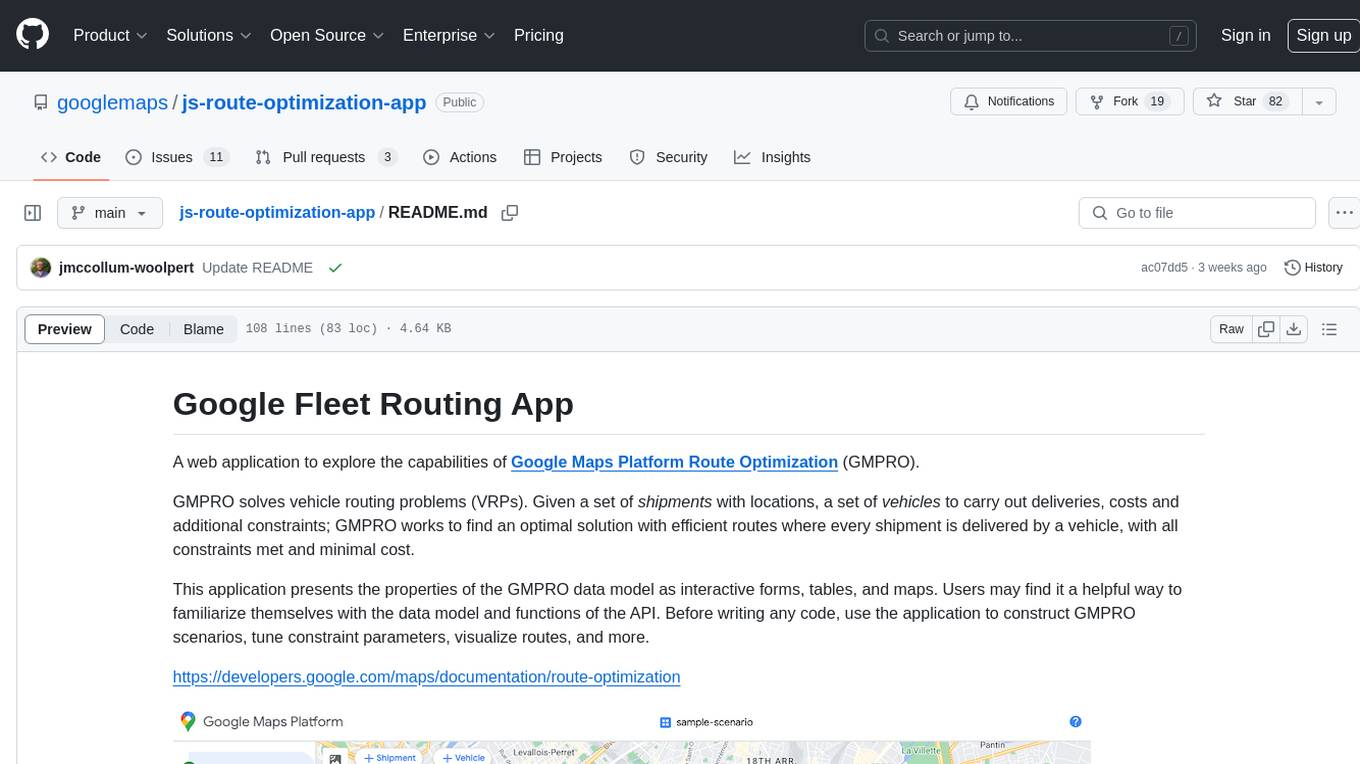
js-route-optimization-app
A web application to explore the capabilities of Google Maps Platform Route Optimization (GMPRO). It helps users understand the data model and functions of the API by presenting interactive forms, tables, and maps. The tool is intended for exploratory use only and should not be deployed in production. Users can construct scenarios, tune constraint parameters, and visualize routes before implementing their own solutions for integrating Route Optimization into their business processes. The application incurs charges related to cloud resources and API usage, and users should be cautious about generating high usage volumes, especially for large scenarios.
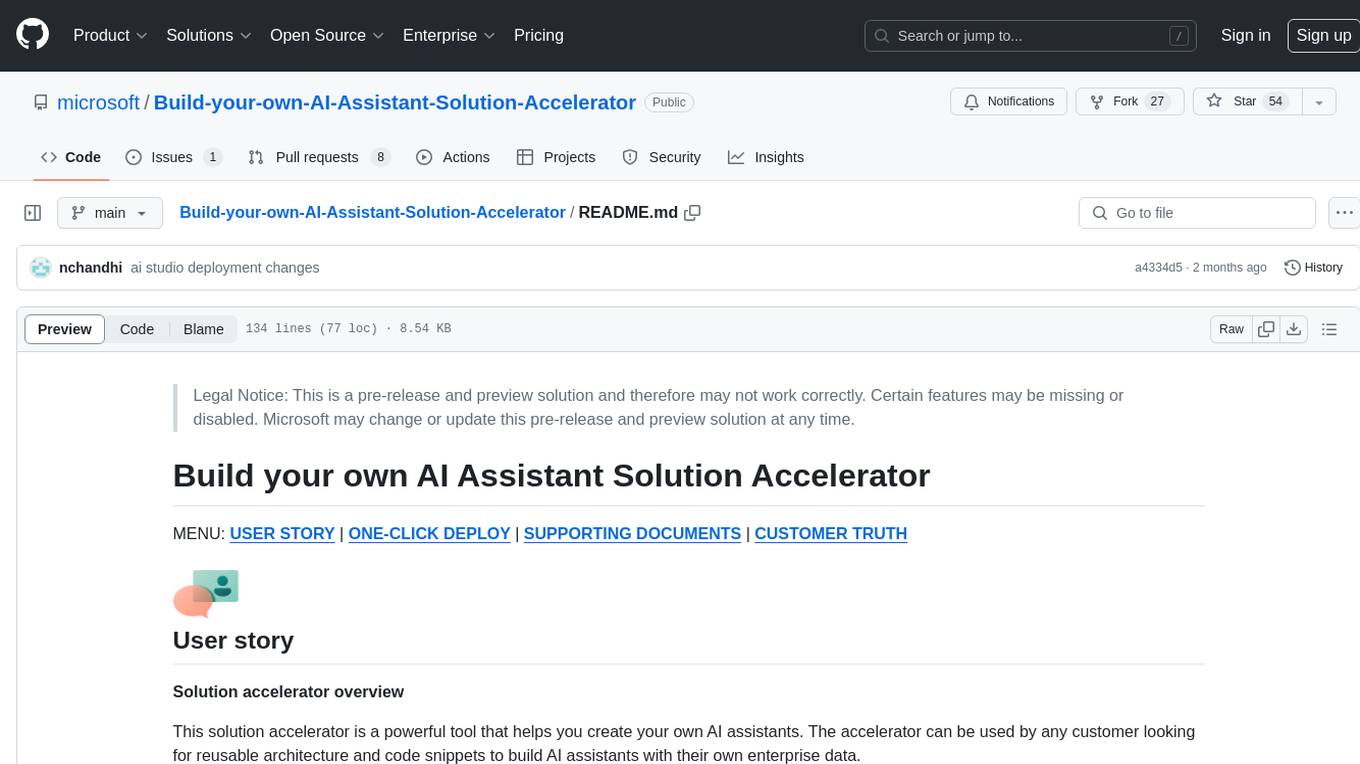
Build-your-own-AI-Assistant-Solution-Accelerator
Build-your-own-AI-Assistant-Solution-Accelerator is a pre-release and preview solution that helps users create their own AI assistants. It leverages Azure Open AI Service, Azure AI Search, and Microsoft Fabric to identify, summarize, and categorize unstructured information. Users can easily find relevant articles and grants, generate grant applications, and export them as PDF or Word documents. The solution accelerator provides reusable architecture and code snippets for building AI assistants with enterprise data. It is designed for researchers looking to explore flu vaccine studies and grants to accelerate grant proposal submissions.
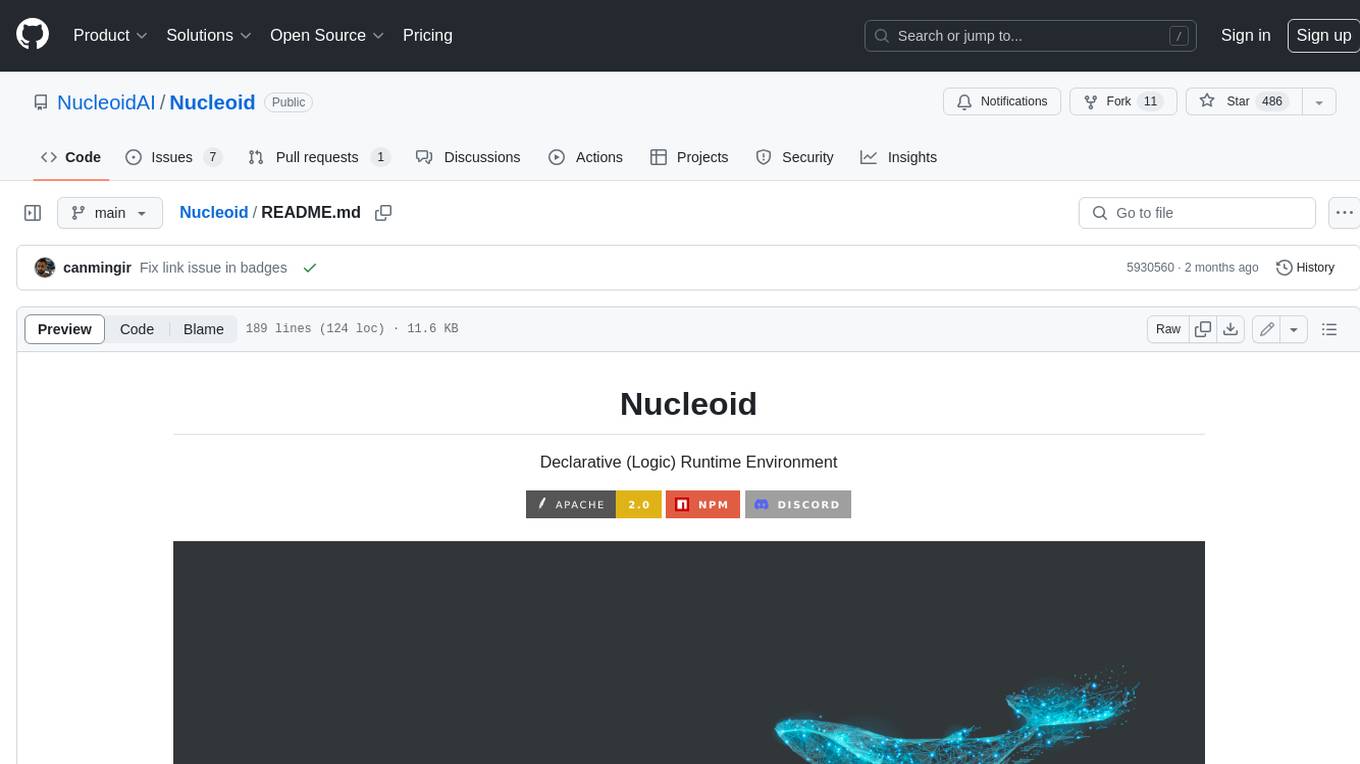
Nucleoid
Nucleoid is a declarative (logic) runtime environment that manages both data and logic under the same runtime. It uses a declarative programming paradigm, which allows developers to focus on the business logic of the application, while the runtime manages the technical details. This allows for faster development and reduces the amount of code that needs to be written. Additionally, the sharding feature can help to distribute the load across multiple instances, which can further improve the performance of the system.
For similar tasks
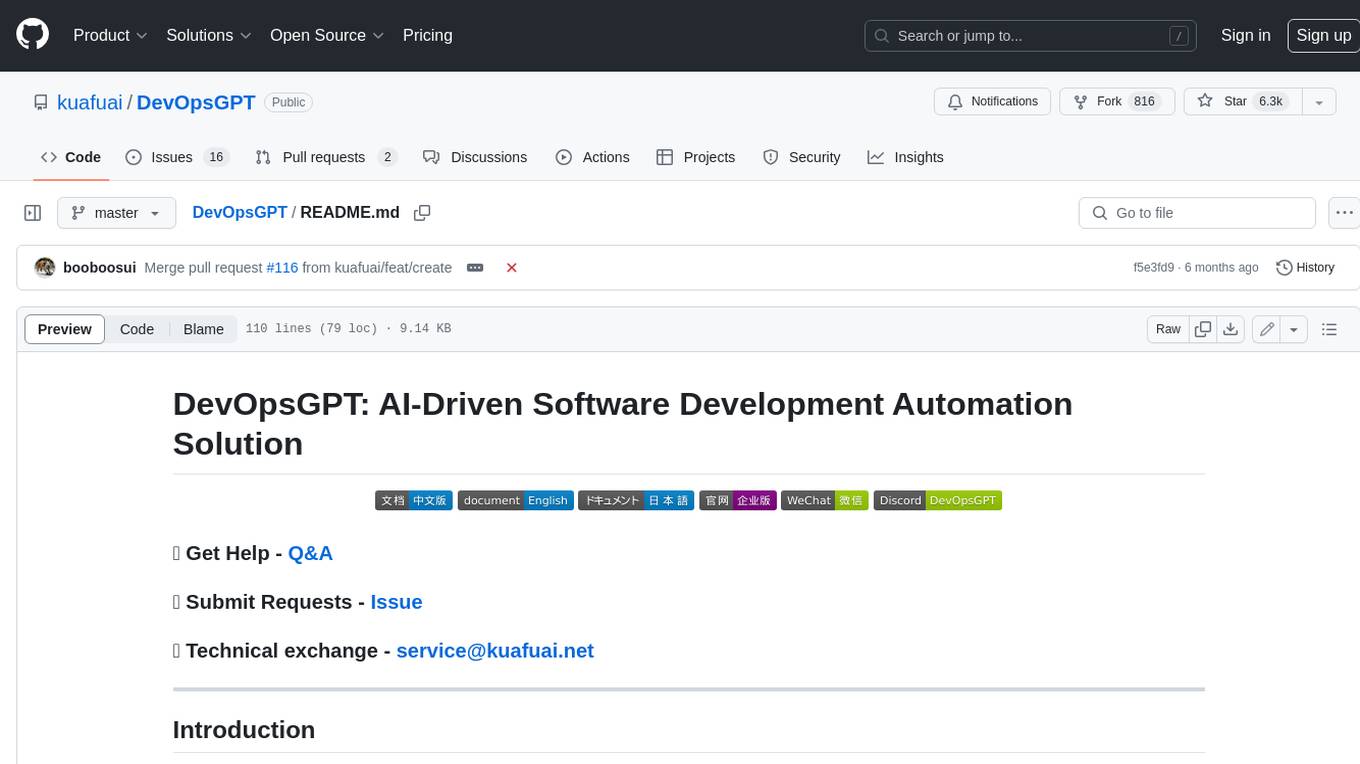
DevOpsGPT
DevOpsGPT is an AI-driven software development automation solution that combines Large Language Models (LLM) with DevOps tools to convert natural language requirements into working software. It improves development efficiency by eliminating the need for tedious requirement documentation, shortens development cycles, reduces communication costs, and ensures high-quality deliverables. The Enterprise Edition offers features like existing project analysis, professional model selection, and support for more DevOps platforms. The tool automates requirement development, generates interface documentation, provides pseudocode based on existing projects, facilitates code refinement, enables continuous integration, and supports software version release. Users can run DevOpsGPT with source code or Docker, and the tool comes with limitations in precise documentation generation and understanding existing project code. The product roadmap includes accurate requirement decomposition, rapid import of development requirements, and integration of more software engineering and professional tools for efficient software development tasks under AI planning and execution.
For similar jobs
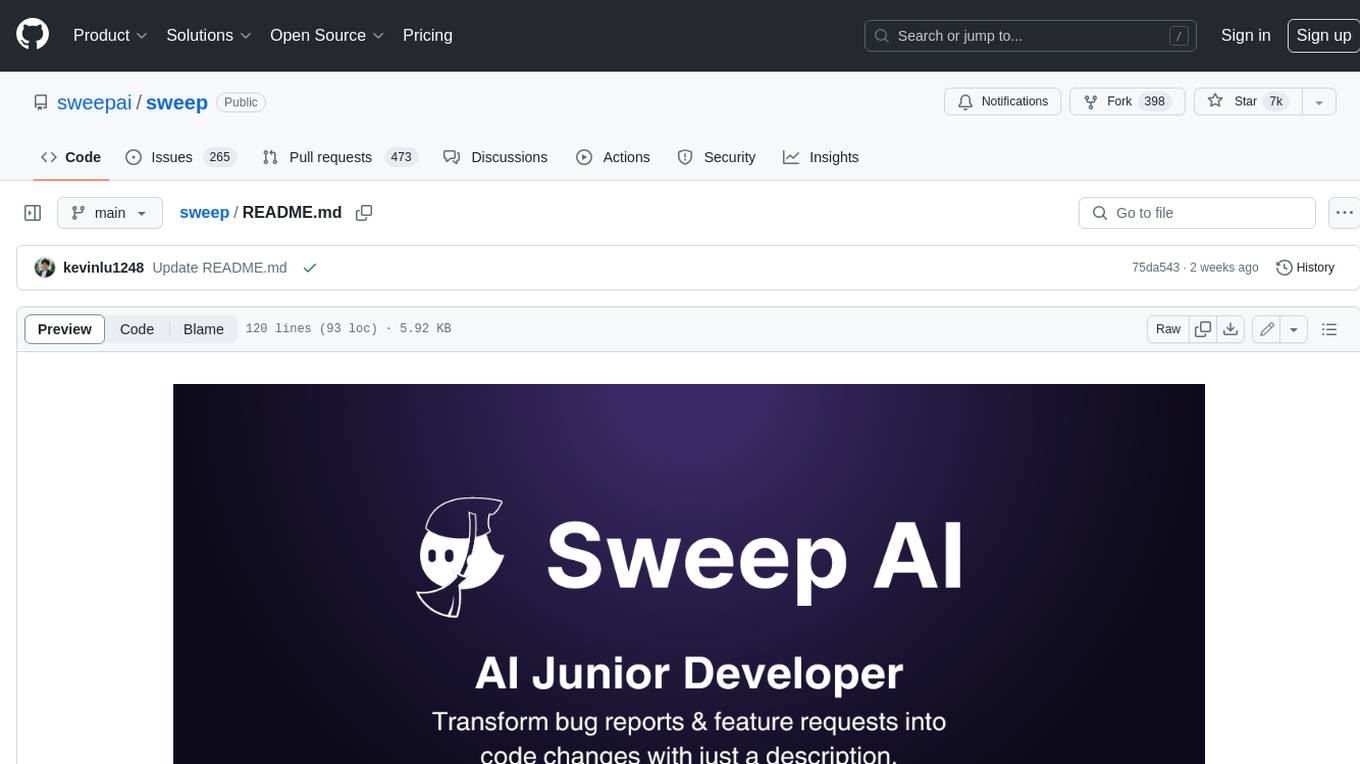
sweep
Sweep is an AI junior developer that turns bugs and feature requests into code changes. It automatically handles developer experience improvements like adding type hints and improving test coverage.
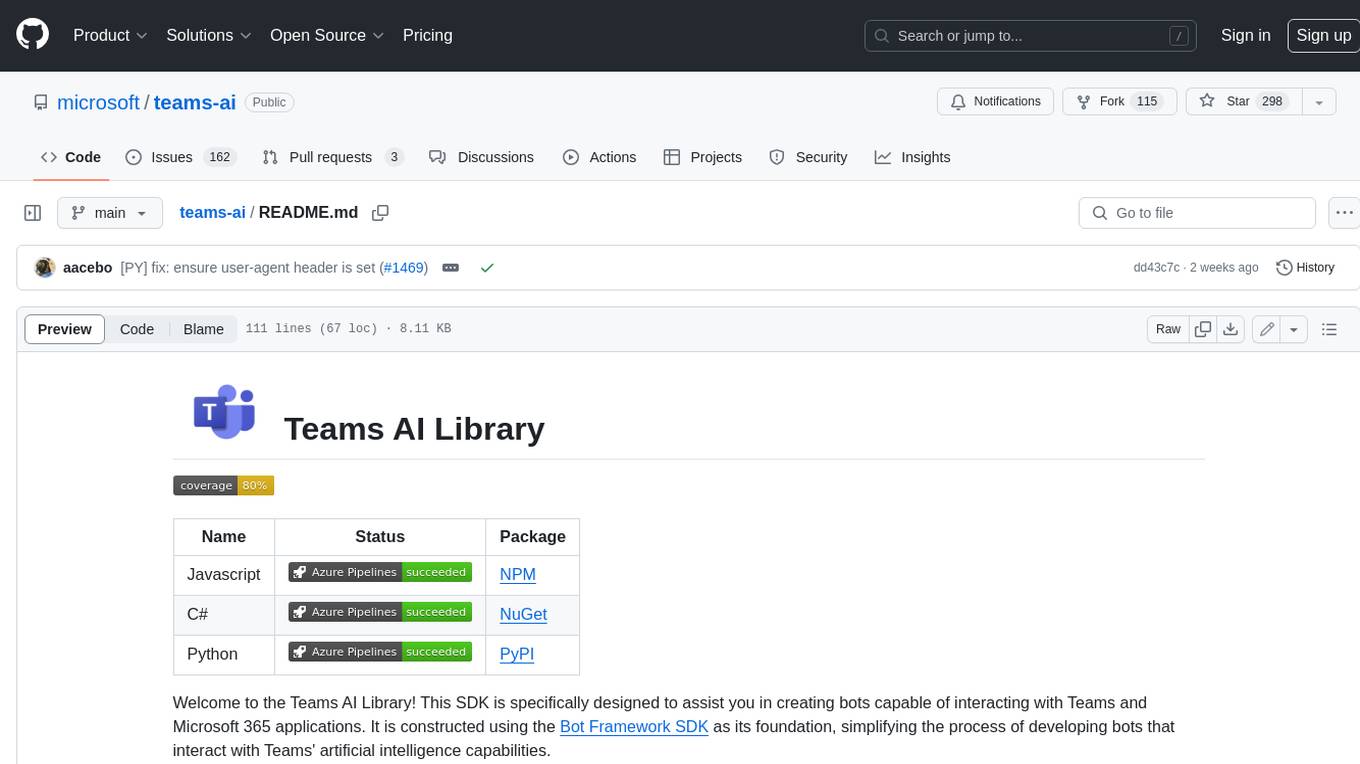
teams-ai
The Teams AI Library is a software development kit (SDK) that helps developers create bots that can interact with Teams and Microsoft 365 applications. It is built on top of the Bot Framework SDK and simplifies the process of developing bots that interact with Teams' artificial intelligence capabilities. The SDK is available for JavaScript/TypeScript, .NET, and Python.
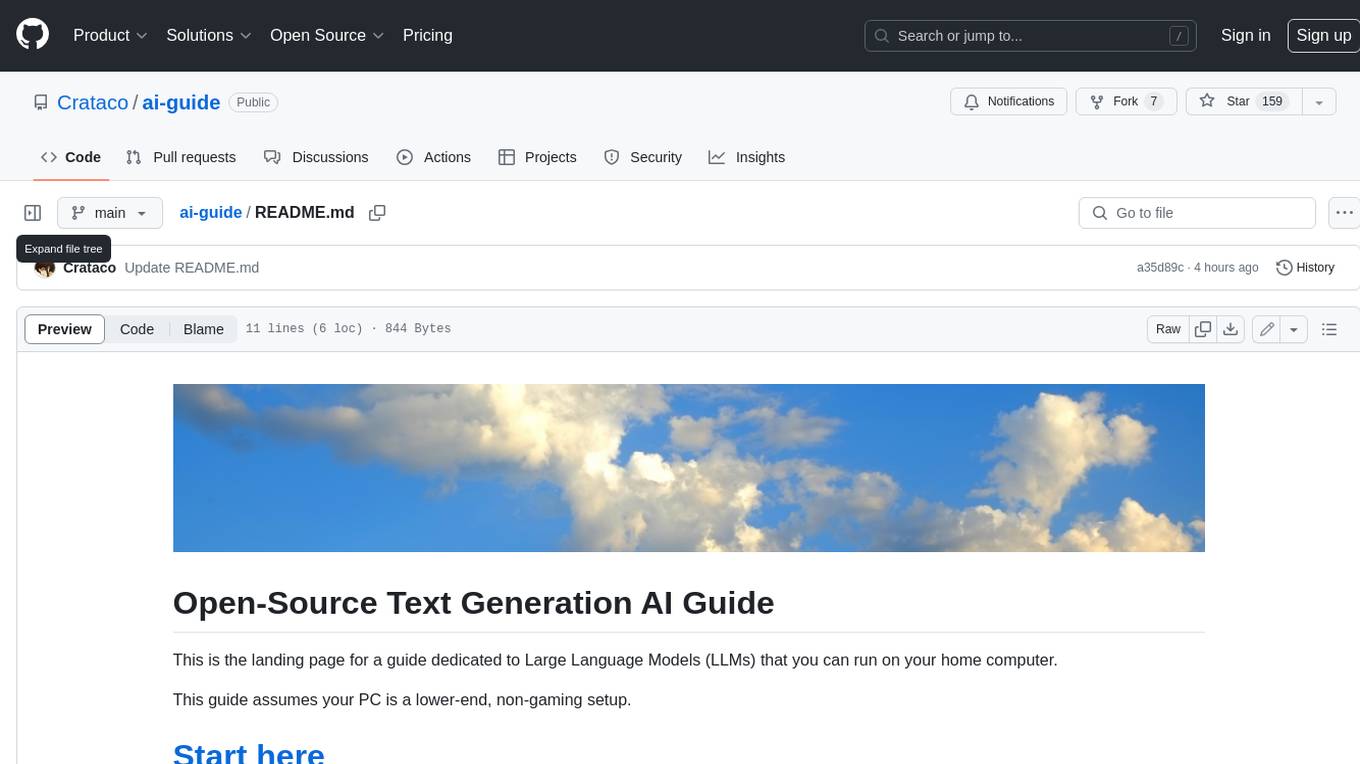
ai-guide
This guide is dedicated to Large Language Models (LLMs) that you can run on your home computer. It assumes your PC is a lower-end, non-gaming setup.
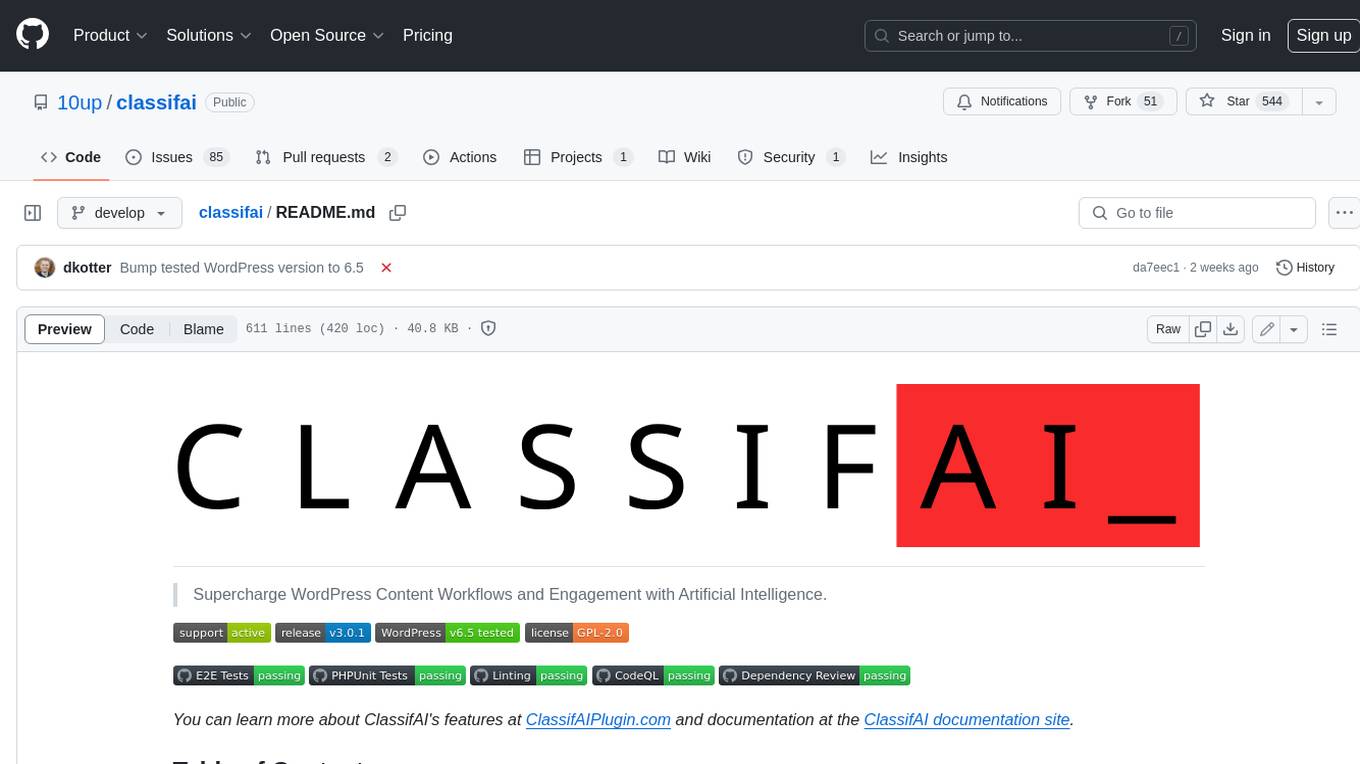
classifai
Supercharge WordPress Content Workflows and Engagement with Artificial Intelligence. Tap into leading cloud-based services like OpenAI, Microsoft Azure AI, Google Gemini and IBM Watson to augment your WordPress-powered websites. Publish content faster while improving SEO performance and increasing audience engagement. ClassifAI integrates Artificial Intelligence and Machine Learning technologies to lighten your workload and eliminate tedious tasks, giving you more time to create original content that matters.
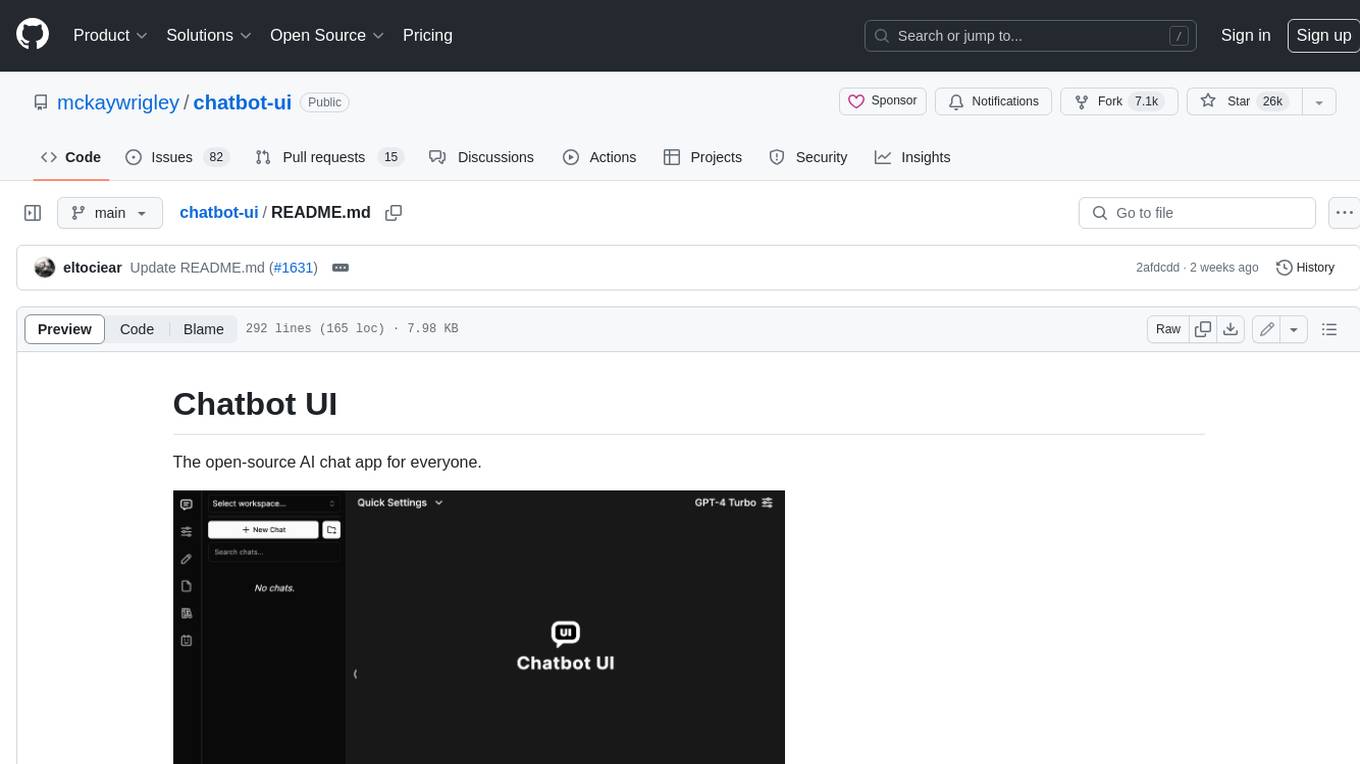
chatbot-ui
Chatbot UI is an open-source AI chat app that allows users to create and deploy their own AI chatbots. It is easy to use and can be customized to fit any need. Chatbot UI is perfect for businesses, developers, and anyone who wants to create a chatbot.
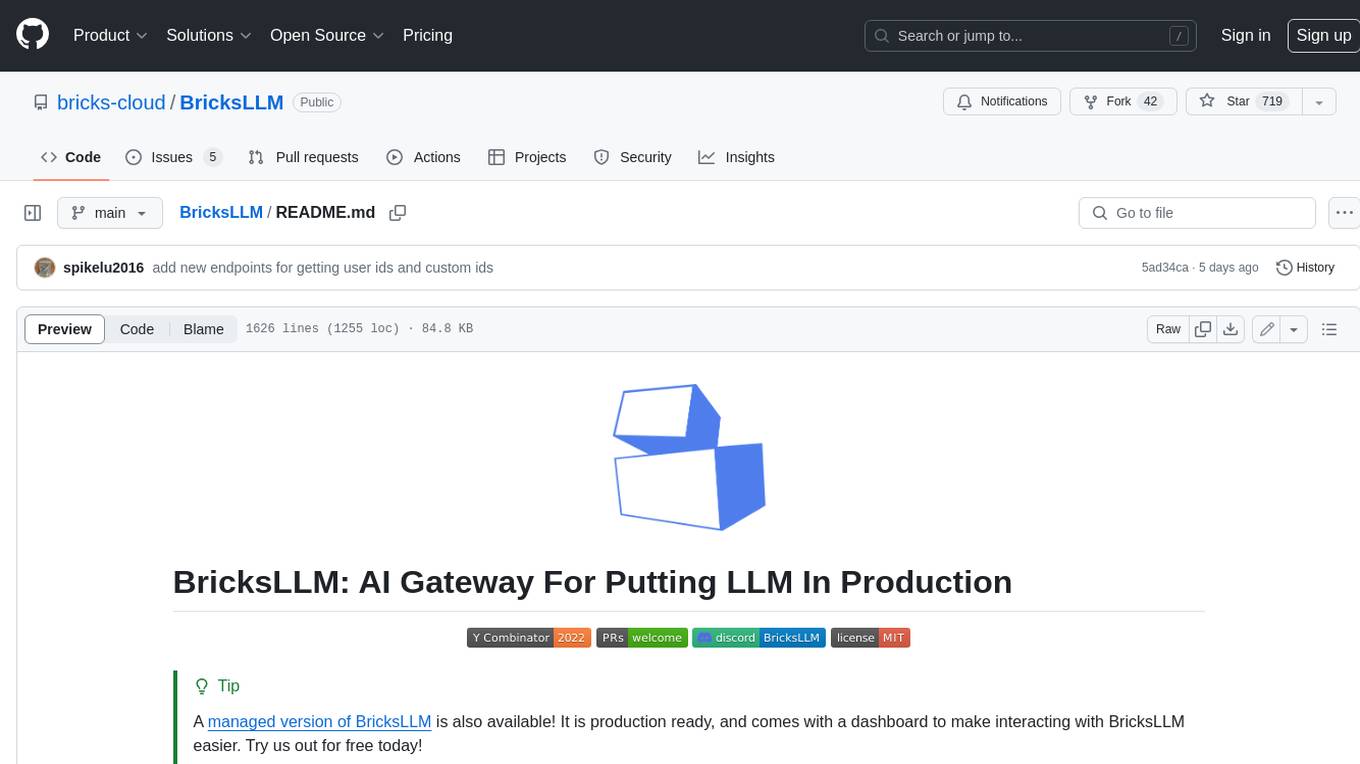
BricksLLM
BricksLLM is a cloud native AI gateway written in Go. Currently, it provides native support for OpenAI, Anthropic, Azure OpenAI and vLLM. BricksLLM aims to provide enterprise level infrastructure that can power any LLM production use cases. Here are some use cases for BricksLLM: * Set LLM usage limits for users on different pricing tiers * Track LLM usage on a per user and per organization basis * Block or redact requests containing PIIs * Improve LLM reliability with failovers, retries and caching * Distribute API keys with rate limits and cost limits for internal development/production use cases * Distribute API keys with rate limits and cost limits for students
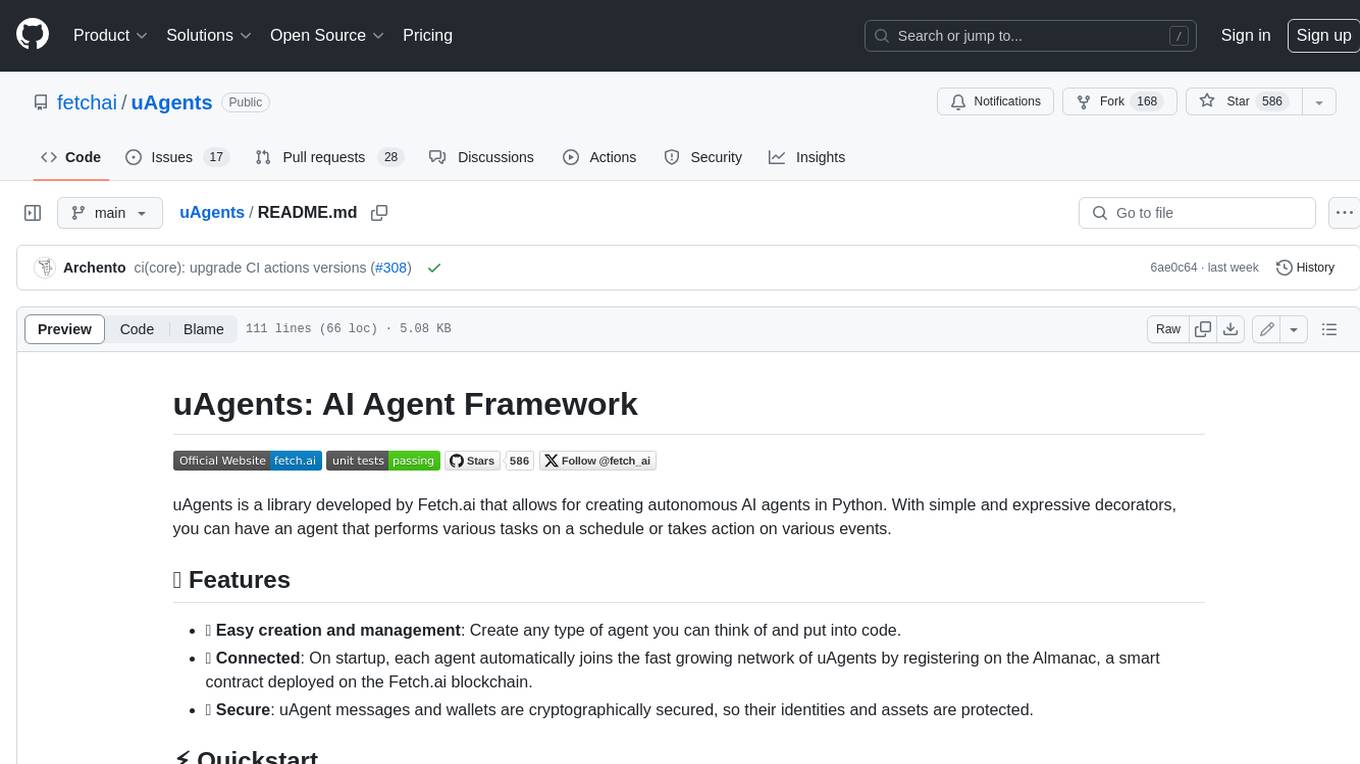
uAgents
uAgents is a Python library developed by Fetch.ai that allows for the creation of autonomous AI agents. These agents can perform various tasks on a schedule or take action on various events. uAgents are easy to create and manage, and they are connected to a fast-growing network of other uAgents. They are also secure, with cryptographically secured messages and wallets.
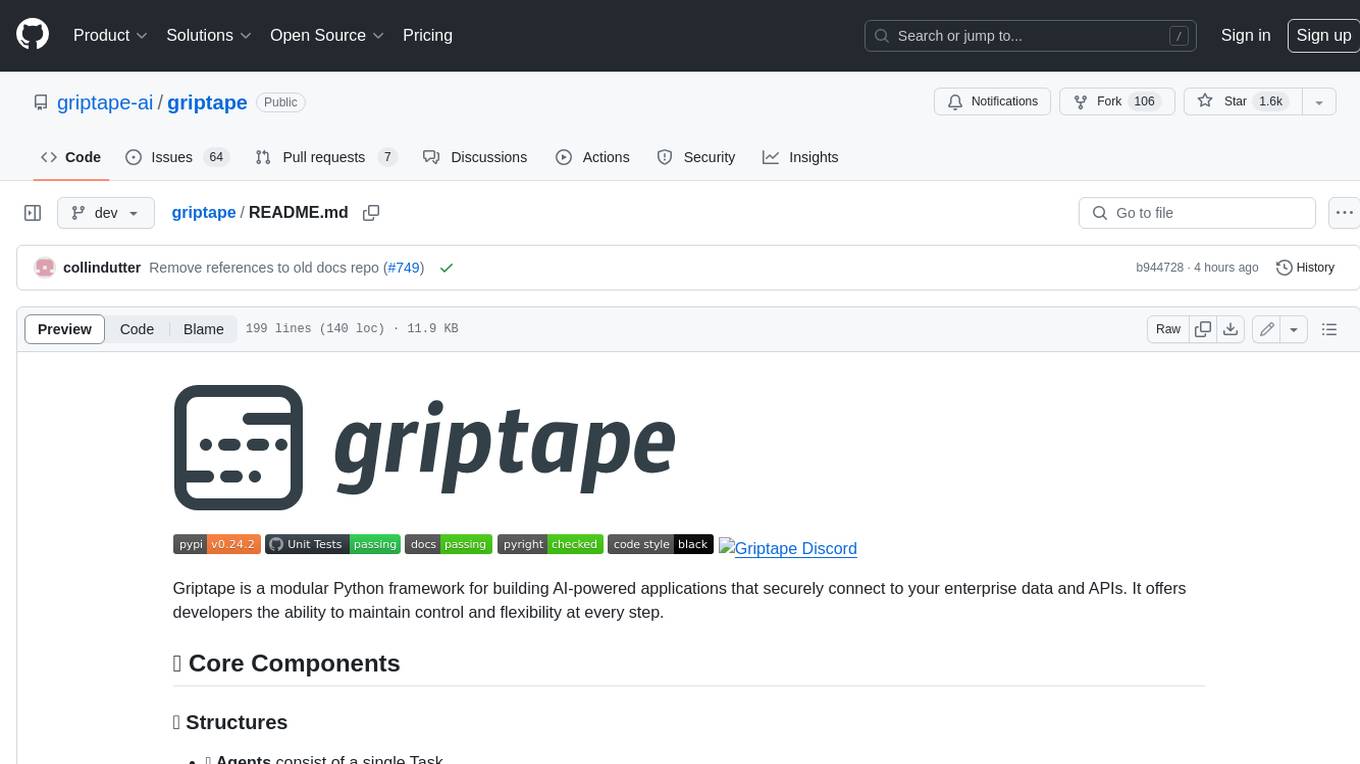
griptape
Griptape is a modular Python framework for building AI-powered applications that securely connect to your enterprise data and APIs. It offers developers the ability to maintain control and flexibility at every step. Griptape's core components include Structures (Agents, Pipelines, and Workflows), Tasks, Tools, Memory (Conversation Memory, Task Memory, and Meta Memory), Drivers (Prompt and Embedding Drivers, Vector Store Drivers, Image Generation Drivers, Image Query Drivers, SQL Drivers, Web Scraper Drivers, and Conversation Memory Drivers), Engines (Query Engines, Extraction Engines, Summary Engines, Image Generation Engines, and Image Query Engines), and additional components (Rulesets, Loaders, Artifacts, Chunkers, and Tokenizers). Griptape enables developers to create AI-powered applications with ease and efficiency.