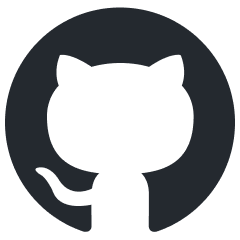
ParrotServe
[OSDI'24] Serving LLM-based Applications Efficiently with Semantic Variable
Stars: 89
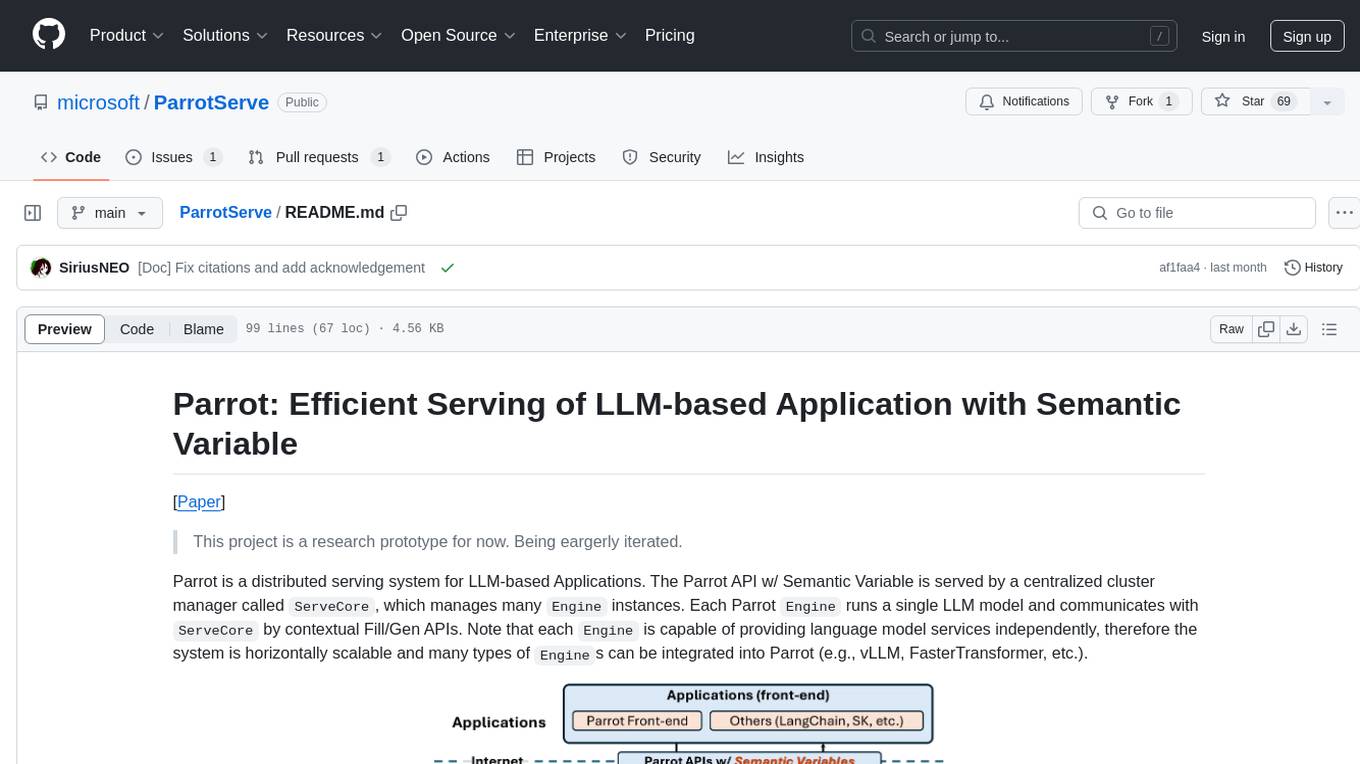
Parrot is a distributed serving system for LLM-based Applications, designed to efficiently serve LLM-based applications by adding Semantic Variable in the OpenAI-style API. It allows for horizontal scalability with multiple Engine instances running LLM models communicating with ServeCore. The system enables AI agents to interact with LLMs via natural language prompts for collaborative tasks.
README:
Paper | Documentation | Slides | Poster
This repo is current a research prototype and is not actively maintained. Please open issue or contact the authors when you need help.
Parrot is a distributed, multi-tenant serving system for LLM-based Applications. With the Semantic Variable abstraction, Parrot can easily grasp the app-level information like LLM computation graph (DAG) or the prompt structure, which enables many interesting features like:
- Automatically parallelize and batch LLM requests in complex LLM applications. Asynchronous communication between dependent requests.
- Performance objective deduction and DAG-aware scheduling.
- Sharing common prompt prefix between requests with optimized attention kernel, Context-aware scheduling.
The powerful language understanding capability of large language models (LLMs) has enabled a new application paradigm, where one or multiple application entities, known as AI agents or co-pilots, communicate with LLMs via natural language, known as “prompts”, to accomplish a task collaboratively.
Parrot is designed to serve these LLM-based applications efficiently by adding Semantic Variable
in current OpenAI-style API, exposing richer application-level knowledge to backend systems and engines for better optimization.
For more background knowledge and our motivation, please refer our OSDI'24 paper Parrot: Efficient Serving of LLM-based Applications with Semantic Variable.
- [2024/08] A Post about Parrot is published in the WeChat account of MSRA (Microsoft Research Asia)!
- [2024/07] Parrot is published and presented in OSDI'24. Please find the paper and presentation Here if you are interested!
- [2024/06] Blogs about the technical details behind Parrot: Blog (Chinese) or Blog Zhihu (Chinese).
- [2024/04] Release Parrot as a Microsoft open source project.
- [2024/03] Parrot has been accepted to OSDI'24!
See INSTALL for installation instructions.
- Get Started: In this chapter, you will learn how to install Parrot and run your first application using Parrot.
-
Documentation for Users: User documentation of Parrot. It contains the API specification of Parrot's OpenAI-like API, and the grammar of Parrot's frontend
pfunc
. - Parrot System Design: If you want to hack/modify Parrot, it's what you need. This chapter offers an overview of Parrot's system architecture and provides detailed explanation of Parrot's internal code organization and implementation.
- Version Drafts: Learn about the refactor history and some of our brainstorm ideas when developing Parrot from these drafts.
We learned a lot from the following projects when developing Parrot.
If you find Parrot useful or relevant to your research, please cite our paper as below:
@inproceedings{lin2024parrot,
author = {Chaofan Lin and Zhenhua Han and Chengruidong Zhang and Yuqing Yang and Fan Yang and Chen Chen and Lili Qiu},
title = {Parrot: Efficient Serving of LLM-based Applications with Semantic Variable},
booktitle = {18th USENIX Symposium on Operating Systems Design and Implementation (OSDI 24)},
year = {2024},
address = {Santa Clara, CA},
publisher = {USENIX Association},
url = {https://www.usenix.org/conference/osdi24/presentation/lin-chaofan},
month = jul
}
This project welcomes contributions and suggestions. Most contributions require you to agree to a Contributor License Agreement (CLA) declaring that you have the right to, and actually do, grant us the rights to use your contribution. For details, visit https://cla.opensource.microsoft.com.
When you submit a pull request, a CLA bot will automatically determine whether you need to provide a CLA and decorate the PR appropriately (e.g., status check, comment). Simply follow the instructions provided by the bot. You will only need to do this once across all repos using our CLA.
This project has adopted the Microsoft Open Source Code of Conduct. For more information see the Code of Conduct FAQ or contact [email protected] with any additional questions or comments.
This project may contain trademarks or logos for projects, products, or services. Authorized use of Microsoft trademarks or logos is subject to and must follow Microsoft's Trademark & Brand Guidelines. Use of Microsoft trademarks or logos in modified versions of this project must not cause confusion or imply Microsoft sponsorship. Any use of third-party trademarks or logos are subject to those third-party's policies.
For Tasks:
Click tags to check more tools for each tasksFor Jobs:
Alternative AI tools for ParrotServe
Similar Open Source Tools
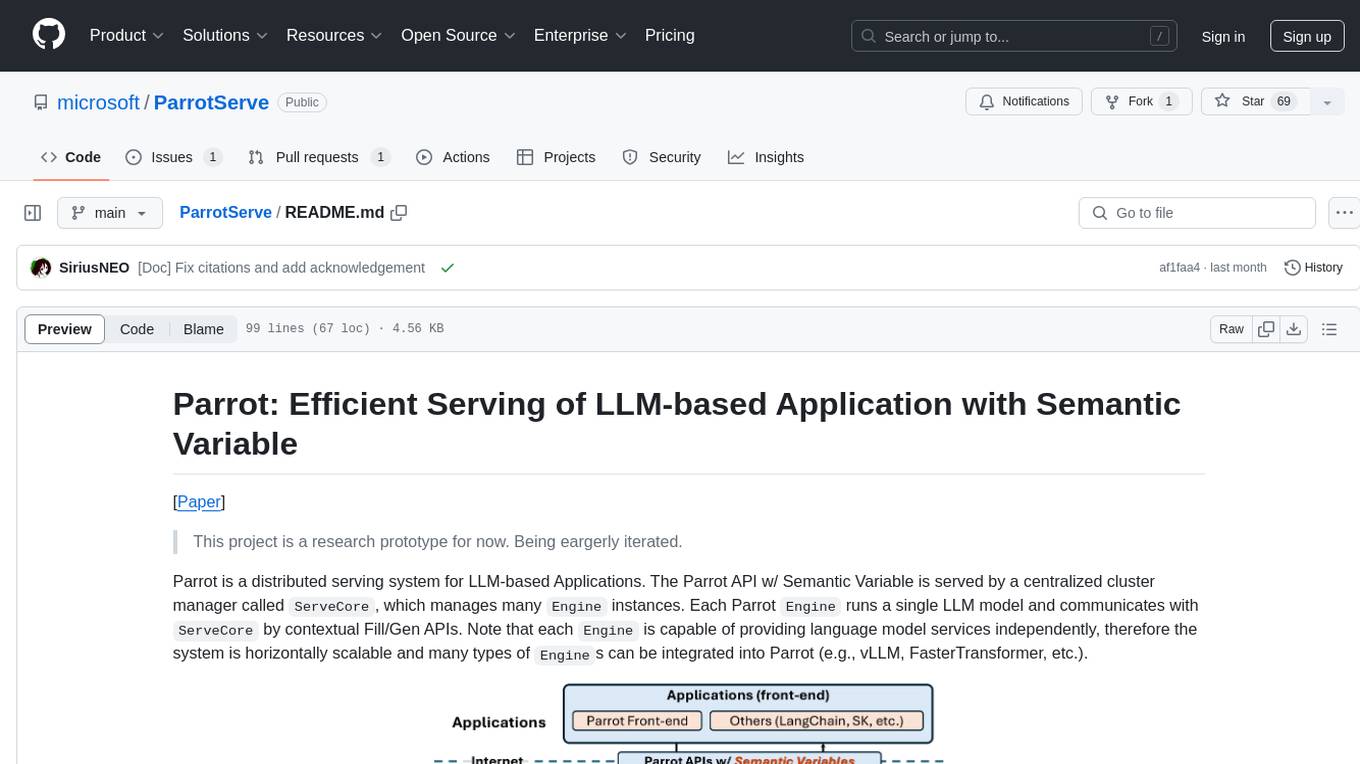
ParrotServe
Parrot is a distributed serving system for LLM-based Applications, designed to efficiently serve LLM-based applications by adding Semantic Variable in the OpenAI-style API. It allows for horizontal scalability with multiple Engine instances running LLM models communicating with ServeCore. The system enables AI agents to interact with LLMs via natural language prompts for collaborative tasks.
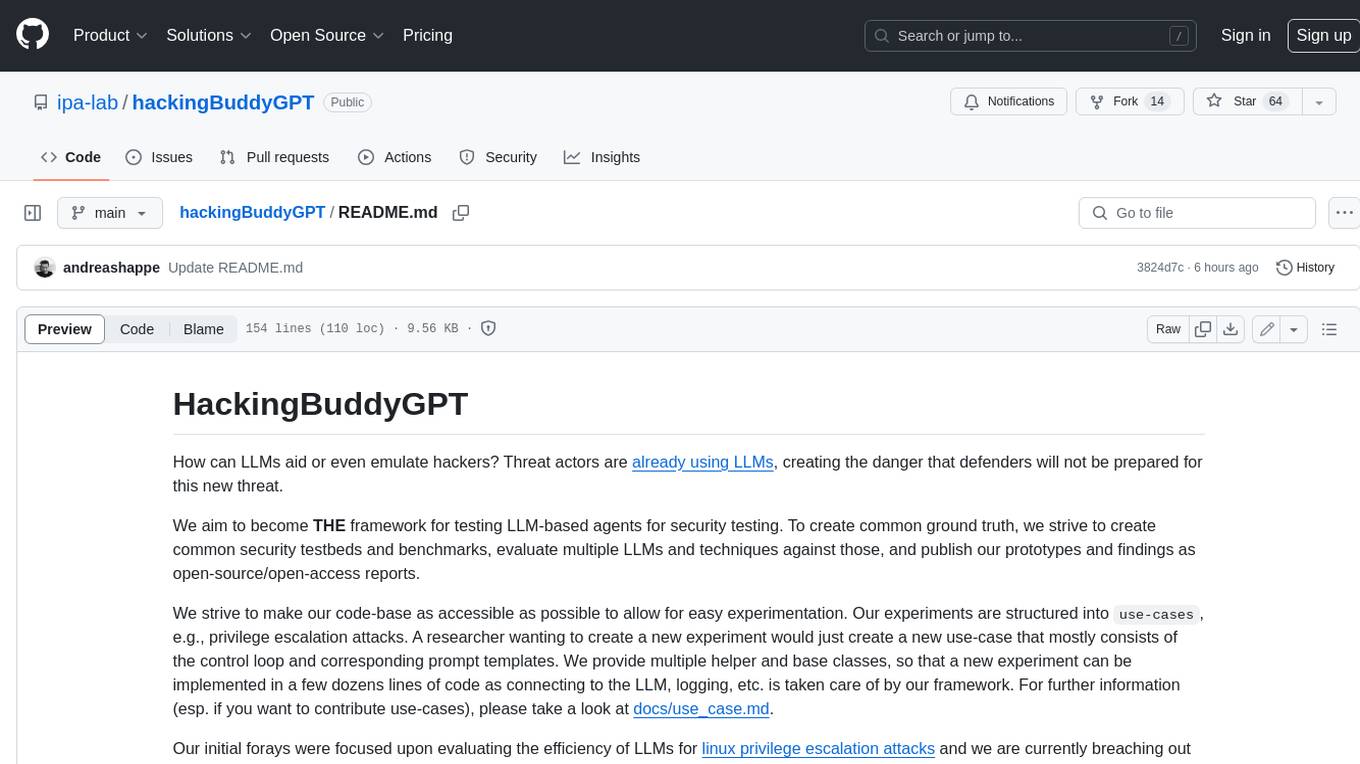
hackingBuddyGPT
hackingBuddyGPT is a framework for testing LLM-based agents for security testing. It aims to create common ground truth by creating common security testbeds and benchmarks, evaluating multiple LLMs and techniques against those, and publishing prototypes and findings as open-source/open-access reports. The initial focus is on evaluating the efficiency of LLMs for Linux privilege escalation attacks, but the framework is being expanded to evaluate the use of LLMs for web penetration-testing and web API testing. hackingBuddyGPT is released as open-source to level the playing field for blue teams against APTs that have access to more sophisticated resources.
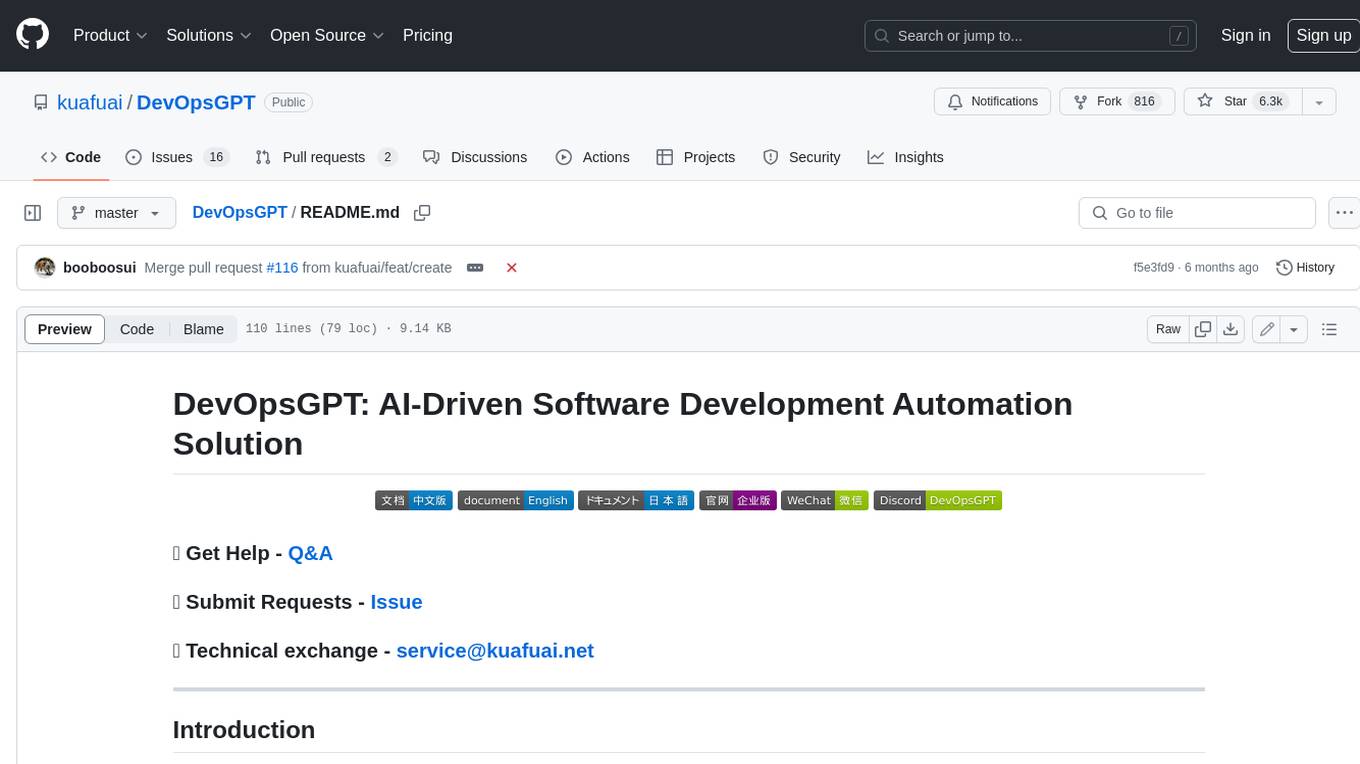
DevOpsGPT
DevOpsGPT is an AI-driven software development automation solution that combines Large Language Models (LLM) with DevOps tools to convert natural language requirements into working software. It improves development efficiency by eliminating the need for tedious requirement documentation, shortens development cycles, reduces communication costs, and ensures high-quality deliverables. The Enterprise Edition offers features like existing project analysis, professional model selection, and support for more DevOps platforms. The tool automates requirement development, generates interface documentation, provides pseudocode based on existing projects, facilitates code refinement, enables continuous integration, and supports software version release. Users can run DevOpsGPT with source code or Docker, and the tool comes with limitations in precise documentation generation and understanding existing project code. The product roadmap includes accurate requirement decomposition, rapid import of development requirements, and integration of more software engineering and professional tools for efficient software development tasks under AI planning and execution.
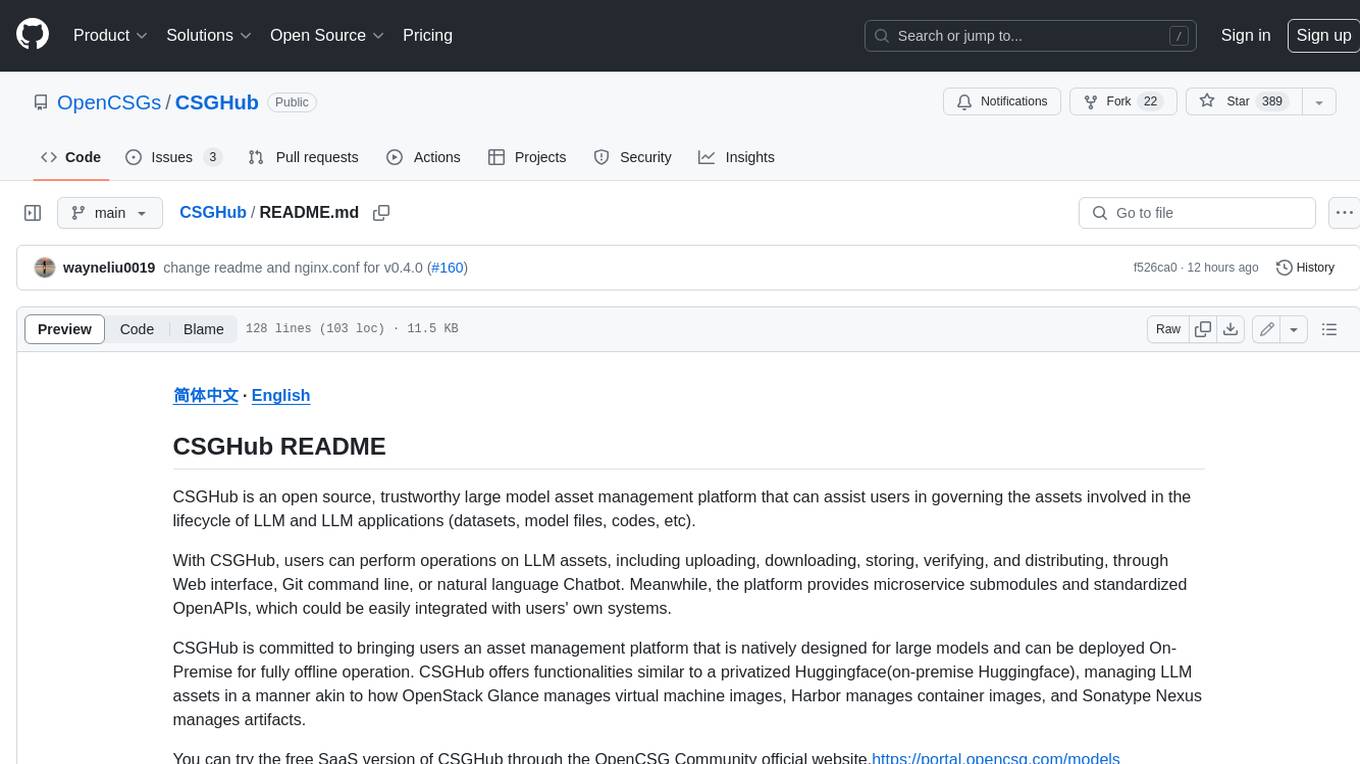
CSGHub
CSGHub is an open source, trustworthy large model asset management platform that can assist users in governing the assets involved in the lifecycle of LLM and LLM applications (datasets, model files, codes, etc). With CSGHub, users can perform operations on LLM assets, including uploading, downloading, storing, verifying, and distributing, through Web interface, Git command line, or natural language Chatbot. Meanwhile, the platform provides microservice submodules and standardized OpenAPIs, which could be easily integrated with users' own systems. CSGHub is committed to bringing users an asset management platform that is natively designed for large models and can be deployed On-Premise for fully offline operation. CSGHub offers functionalities similar to a privatized Huggingface(on-premise Huggingface), managing LLM assets in a manner akin to how OpenStack Glance manages virtual machine images, Harbor manages container images, and Sonatype Nexus manages artifacts.
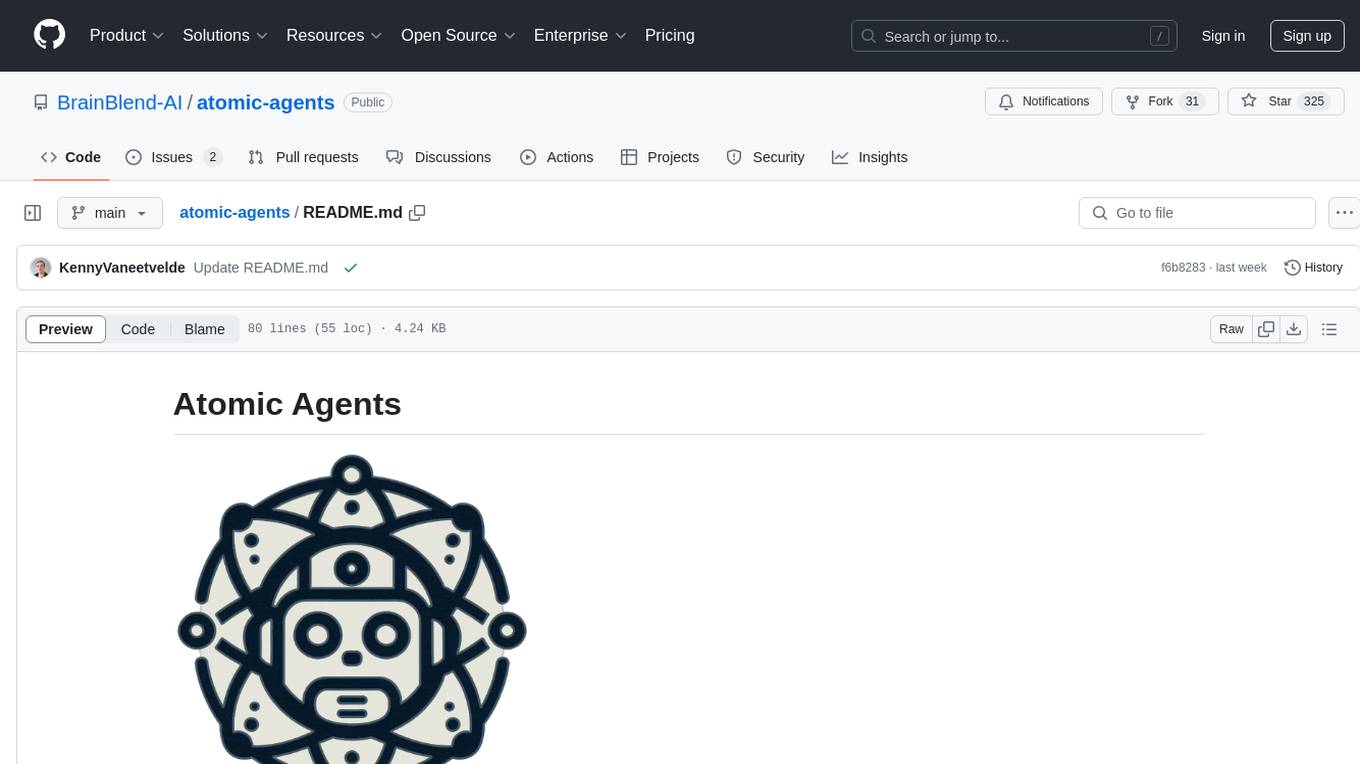
atomic-agents
The Atomic Agents framework is a modular and extensible tool designed for creating powerful applications. It leverages Pydantic for data validation and serialization. The framework follows the principles of Atomic Design, providing small and single-purpose components that can be combined. It integrates with Instructor for AI agent architecture and supports various APIs like Cohere, Anthropic, and Gemini. The tool includes documentation, examples, and testing features to ensure smooth development and usage.
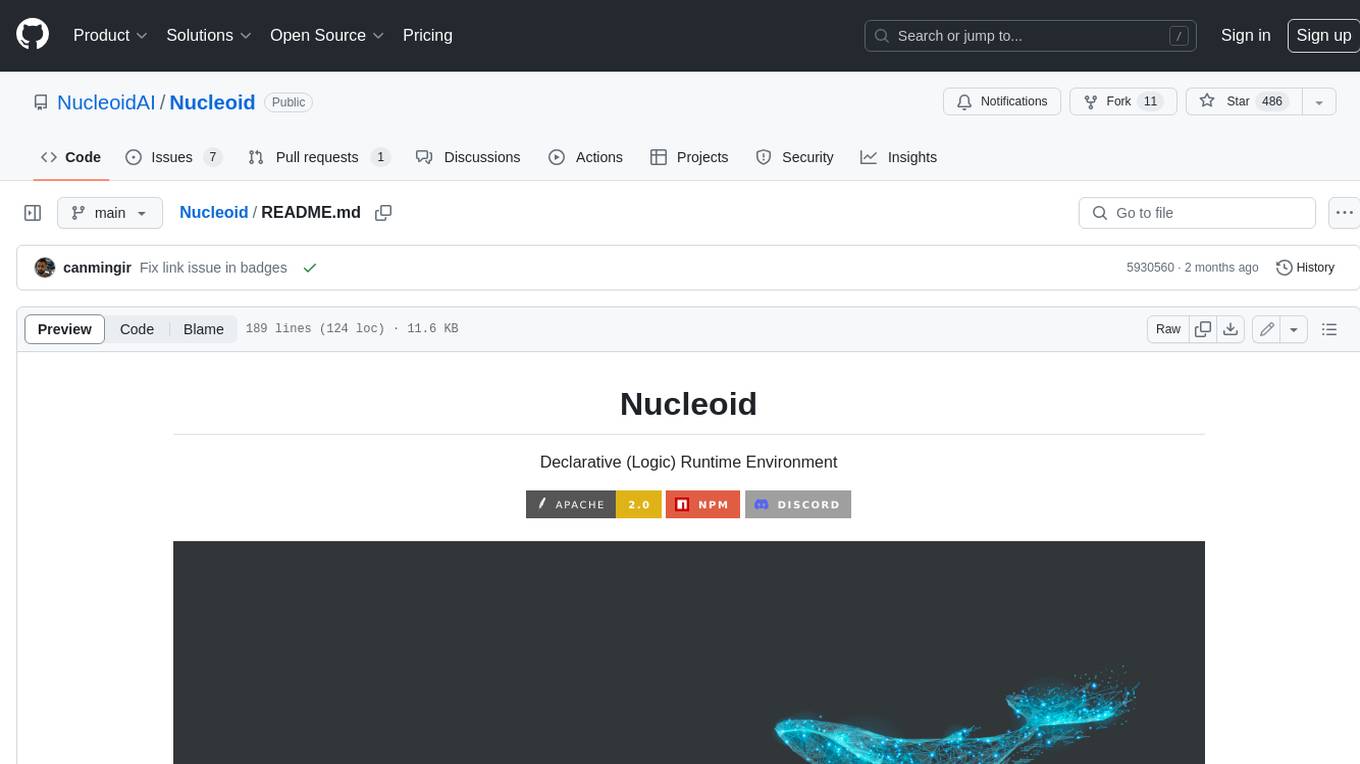
Nucleoid
Nucleoid is a declarative (logic) runtime environment that manages both data and logic under the same runtime. It uses a declarative programming paradigm, which allows developers to focus on the business logic of the application, while the runtime manages the technical details. This allows for faster development and reduces the amount of code that needs to be written. Additionally, the sharding feature can help to distribute the load across multiple instances, which can further improve the performance of the system.
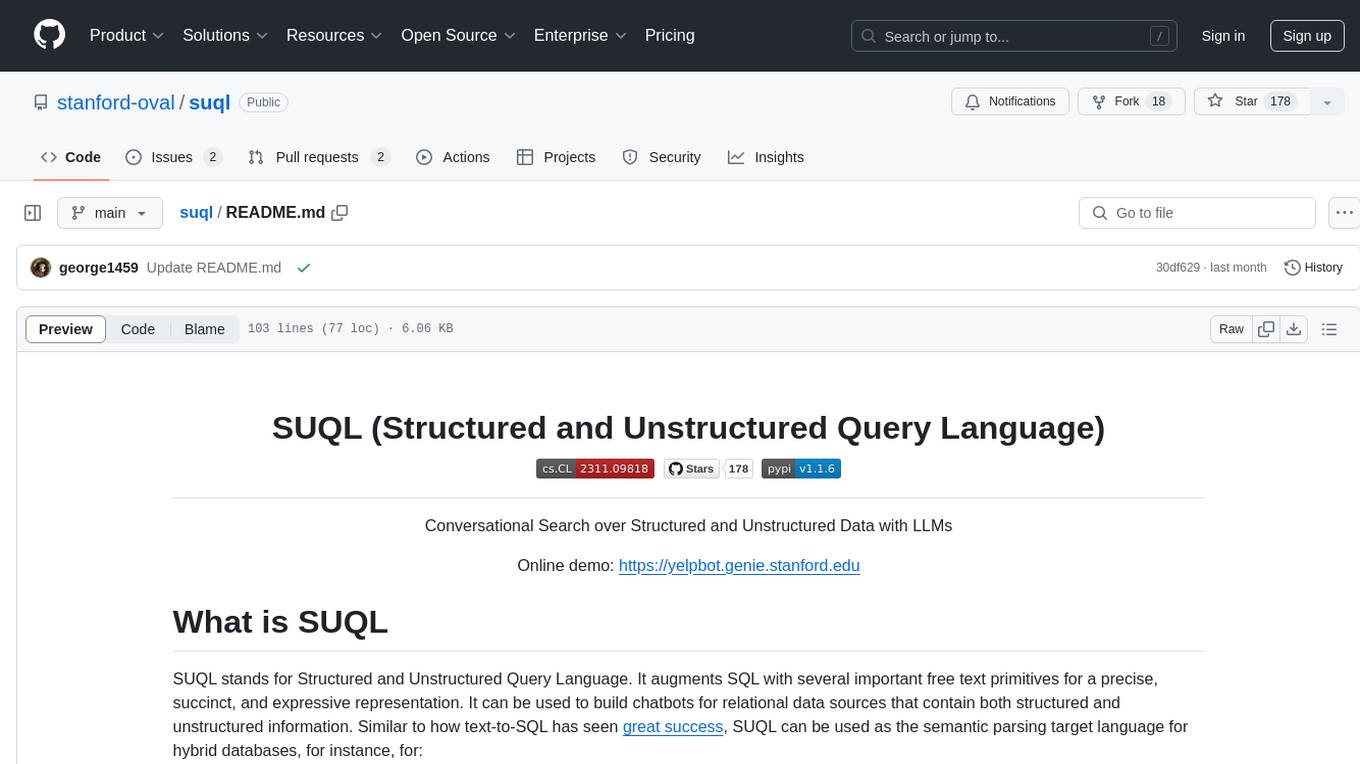
suql
SUQL (Structured and Unstructured Query Language) is a tool that augments SQL with free text primitives for building chatbots that can interact with relational data sources containing both structured and unstructured information. It seamlessly integrates retrieval models, large language models (LLMs), and traditional SQL to provide a clean interface for hybrid data access. SUQL supports optimizations to minimize expensive LLM calls, scalability to large databases with PostgreSQL, and general SQL operations like JOINs and GROUP BYs.
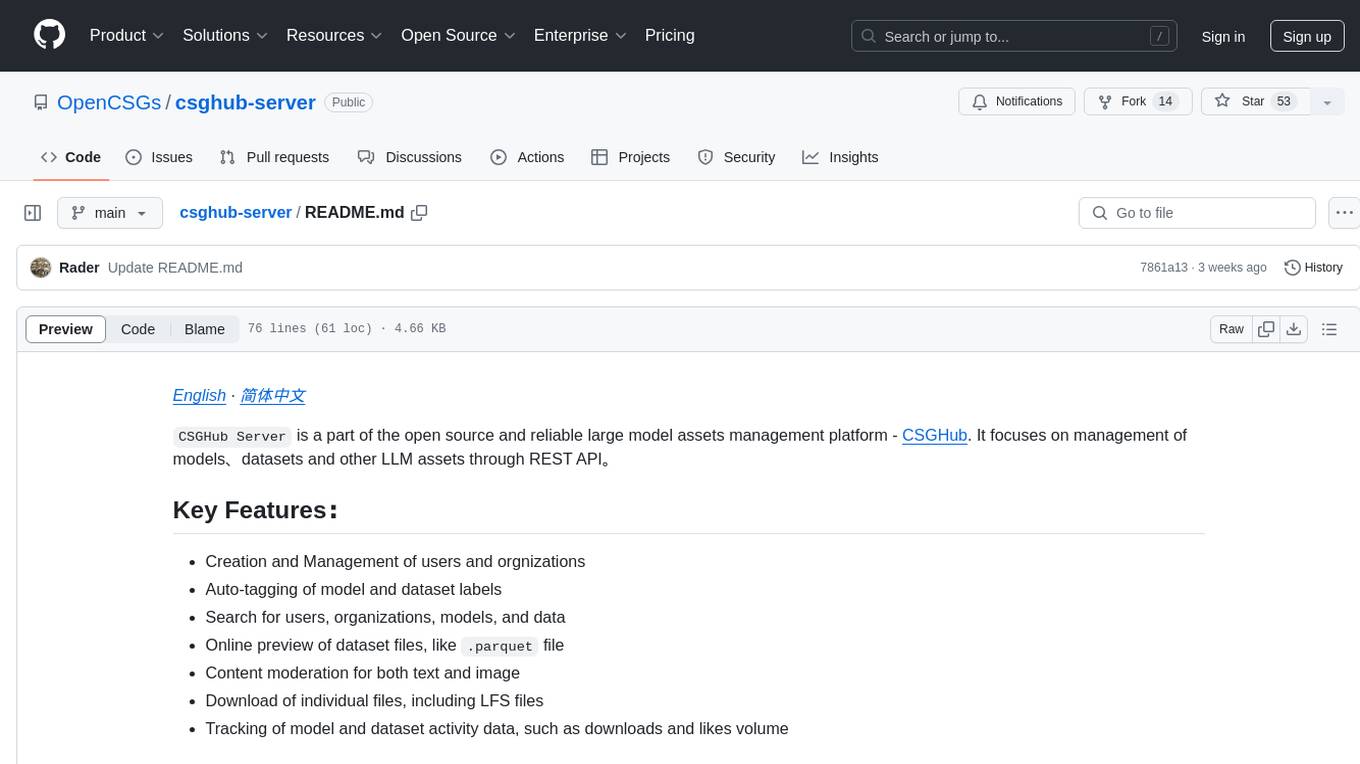
csghub-server
CSGHub Server is a part of the open source and reliable large model assets management platform - CSGHub. It focuses on management of models, datasets, and other LLM assets through REST API. Key features include creation and management of users and organizations, auto-tagging of model and dataset labels, search functionality, online preview of dataset files, content moderation for text and image, download of individual files, tracking of model and dataset activity data. The tool is extensible and customizable, supporting different git servers, flexible LFS storage system configuration, and content moderation options. The roadmap includes support for more Git servers, Git LFS, dataset online viewer, model/dataset auto-tag, S3 protocol support, model format conversion, and model one-click deploy. The project is licensed under Apache 2.0 and welcomes contributions.
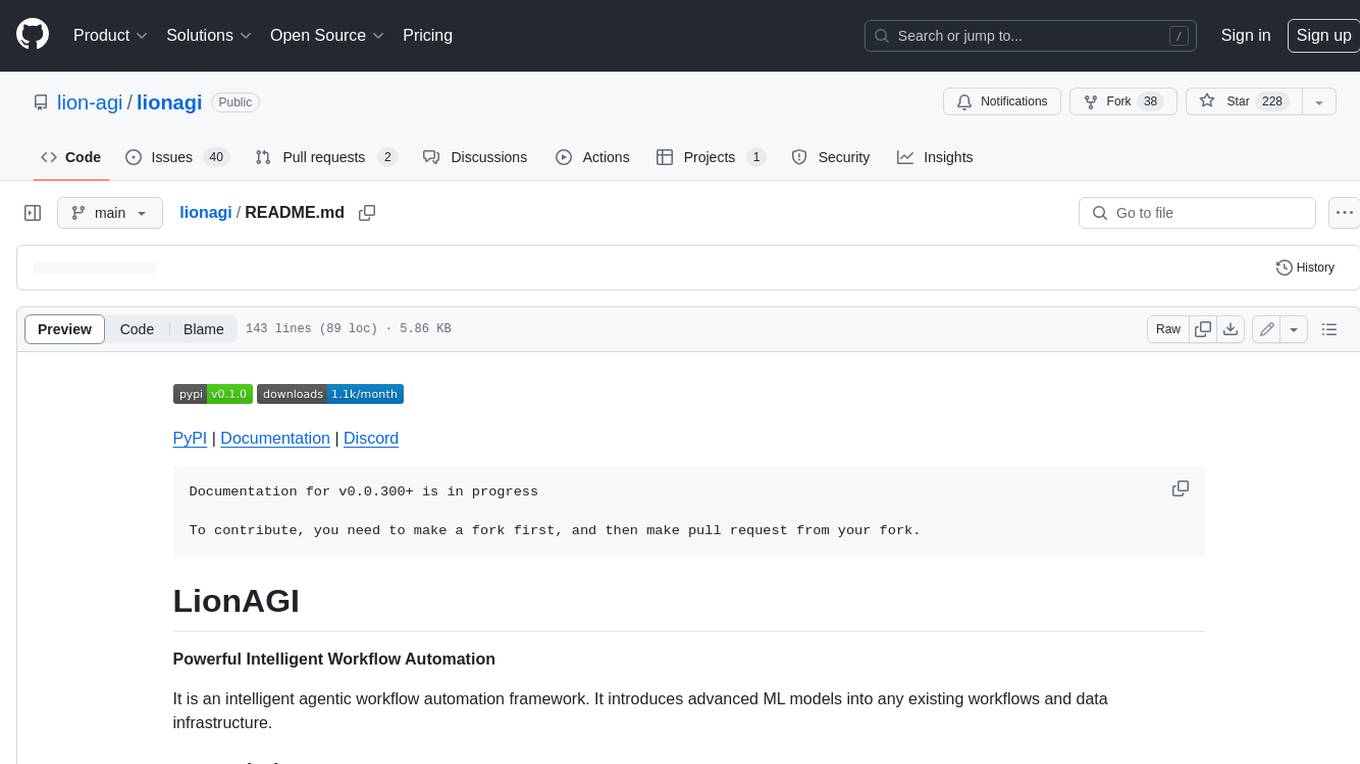
lionagi
LionAGI is a powerful intelligent workflow automation framework that introduces advanced ML models into any existing workflows and data infrastructure. It can interact with almost any model, run interactions in parallel for most models, produce structured pydantic outputs with flexible usage, automate workflow via graph based agents, use advanced prompting techniques, and more. LionAGI aims to provide a centralized agent-managed framework for "ML-powered tools coordination" and to dramatically lower the barrier of entries for creating use-case/domain specific tools. It is designed to be asynchronous only and requires Python 3.10 or higher.
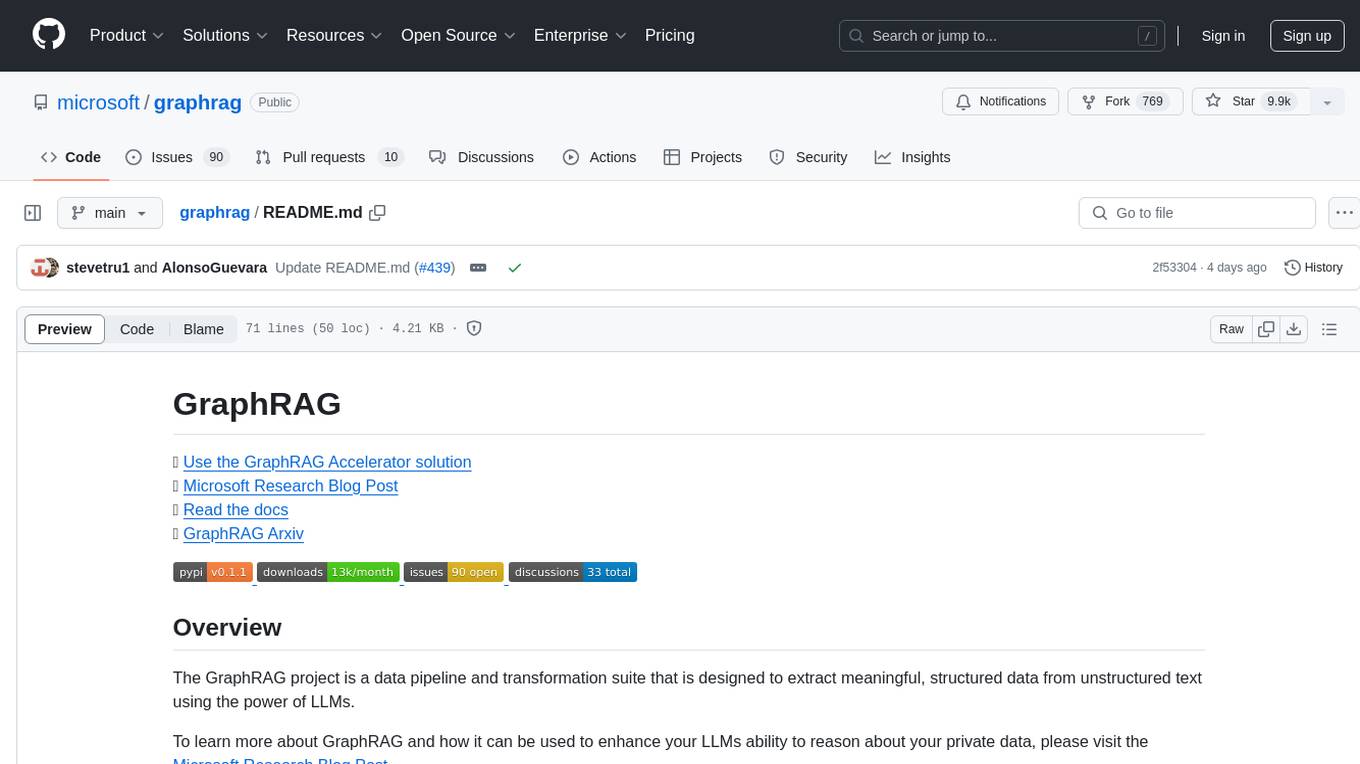
graphrag
The GraphRAG project is a data pipeline and transformation suite designed to extract meaningful, structured data from unstructured text using LLMs. It enhances LLMs' ability to reason about private data. The repository provides guidance on using knowledge graph memory structures to enhance LLM outputs, with a warning about the potential costs of GraphRAG indexing. It offers contribution guidelines, development resources, and encourages prompt tuning for optimal results. The Responsible AI FAQ addresses GraphRAG's capabilities, intended uses, evaluation metrics, limitations, and operational factors for effective and responsible use.
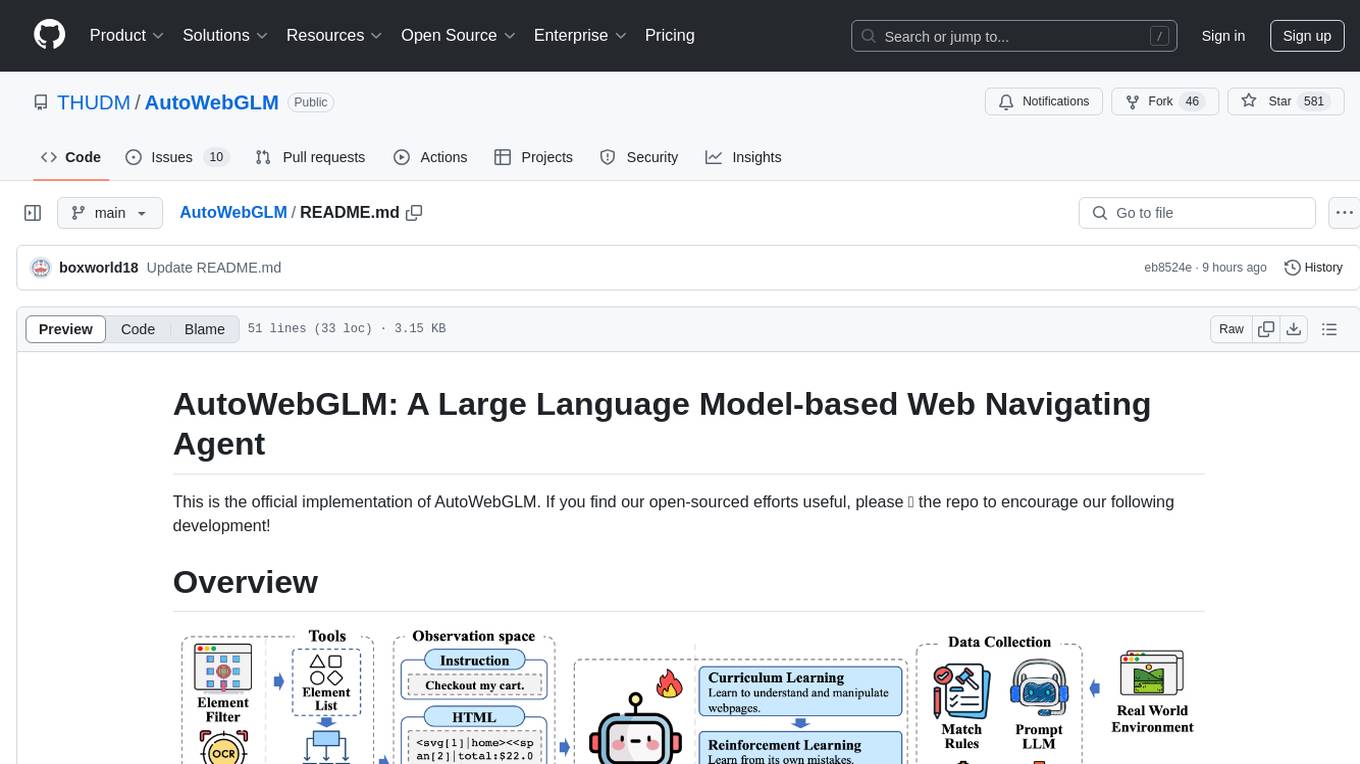
AutoWebGLM
AutoWebGLM is a project focused on developing a language model-driven automated web navigation agent. It extends the capabilities of the ChatGLM3-6B model to navigate the web more efficiently and address real-world browsing challenges. The project includes features such as an HTML simplification algorithm, hybrid human-AI training, reinforcement learning, rejection sampling, and a bilingual web navigation benchmark for testing AI web navigation agents.
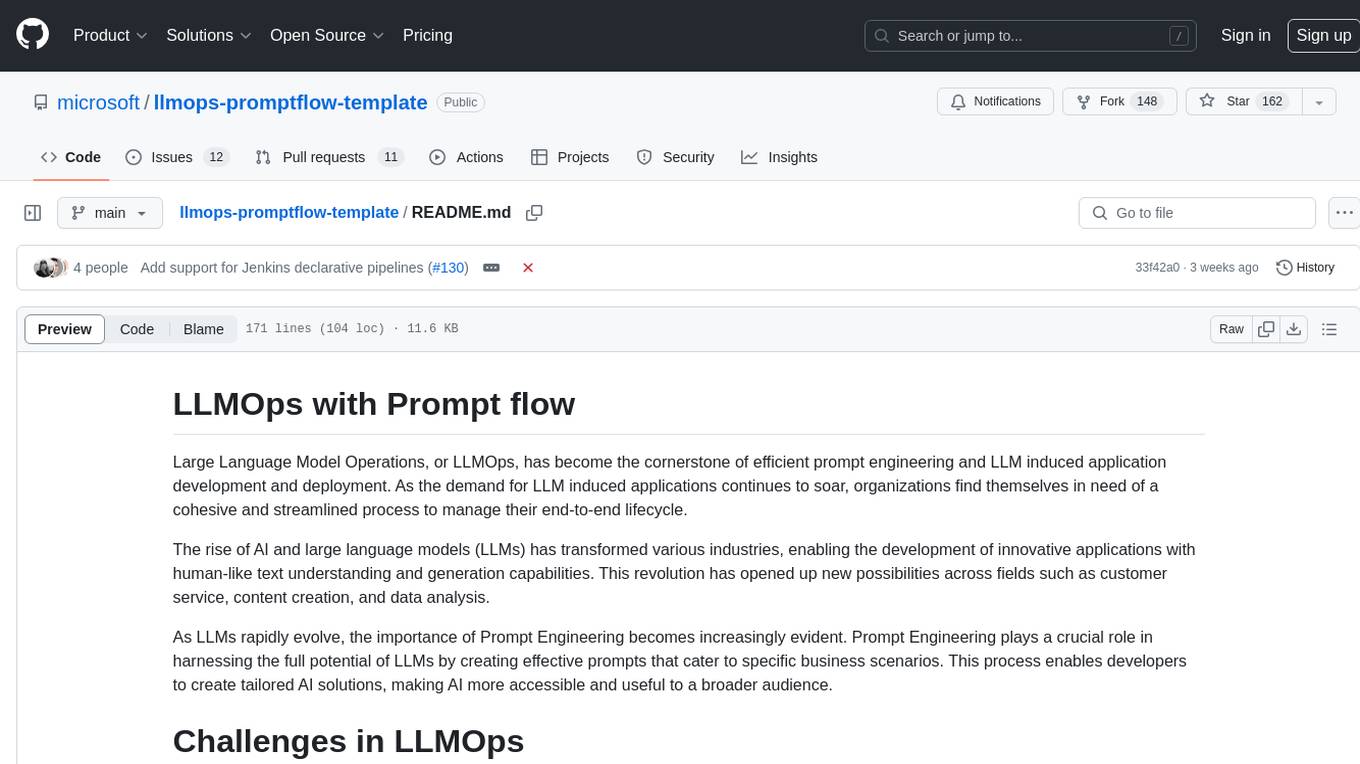
llmops-promptflow-template
LLMOps with Prompt flow is a template and guidance for building LLM-infused apps using Prompt flow. It provides centralized code hosting, lifecycle management, variant and hyperparameter experimentation, A/B deployment, many-to-many dataset/flow relationships, multiple deployment targets, comprehensive reporting, BYOF capabilities, configuration-based development, local prompt experimentation and evaluation, endpoint testing, and optional Human-in-loop validation. The tool is customizable to suit various application needs.
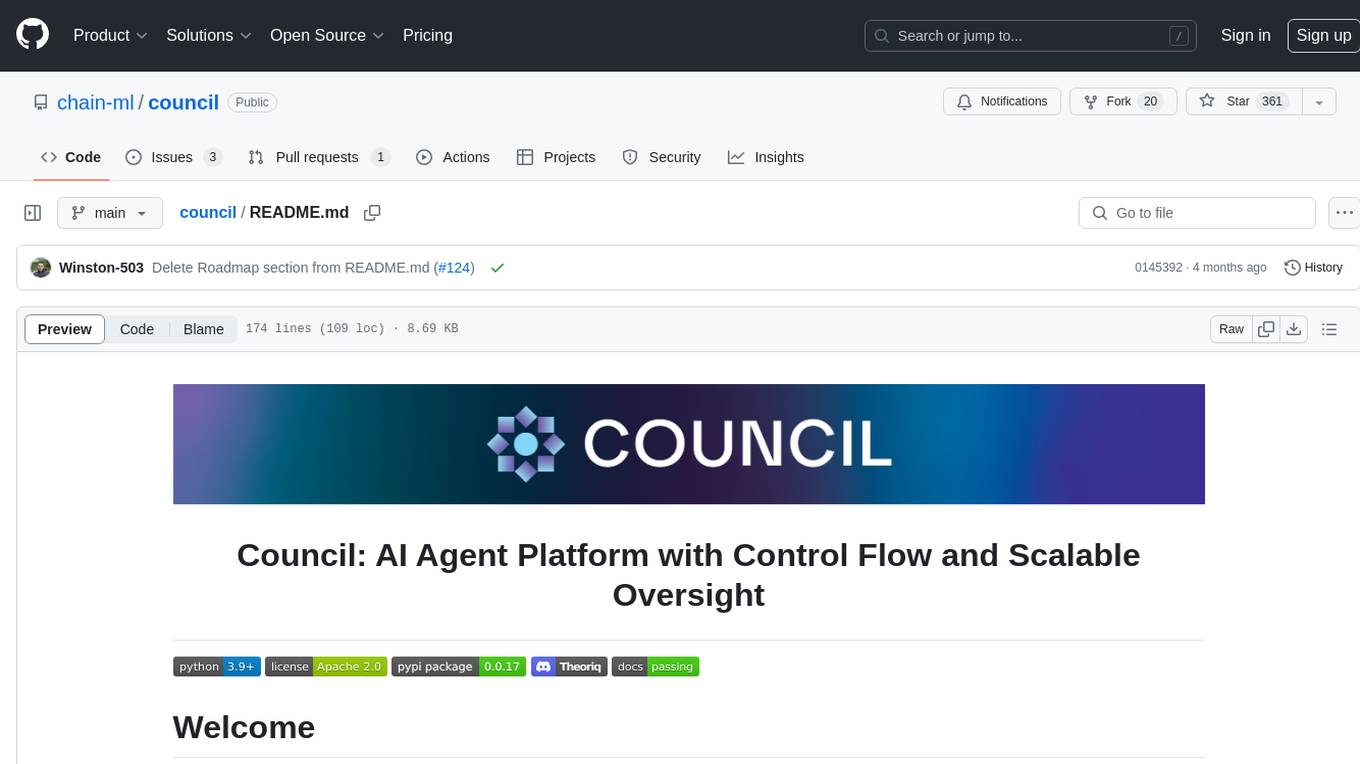
council
Council is an open-source platform designed for the rapid development and deployment of customized generative AI applications using teams of agents. It extends the LLM tool ecosystem by providing advanced control flow and scalable oversight for AI agents. Users can create sophisticated agents with predictable behavior by leveraging Council's powerful approach to control flow using Controllers, Filters, Evaluators, and Budgets. The framework allows for automated routing between agents, comparing, evaluating, and selecting the best results for a task. Council aims to facilitate packaging and deploying agents at scale on multiple platforms while enabling enterprise-grade monitoring and quality control.
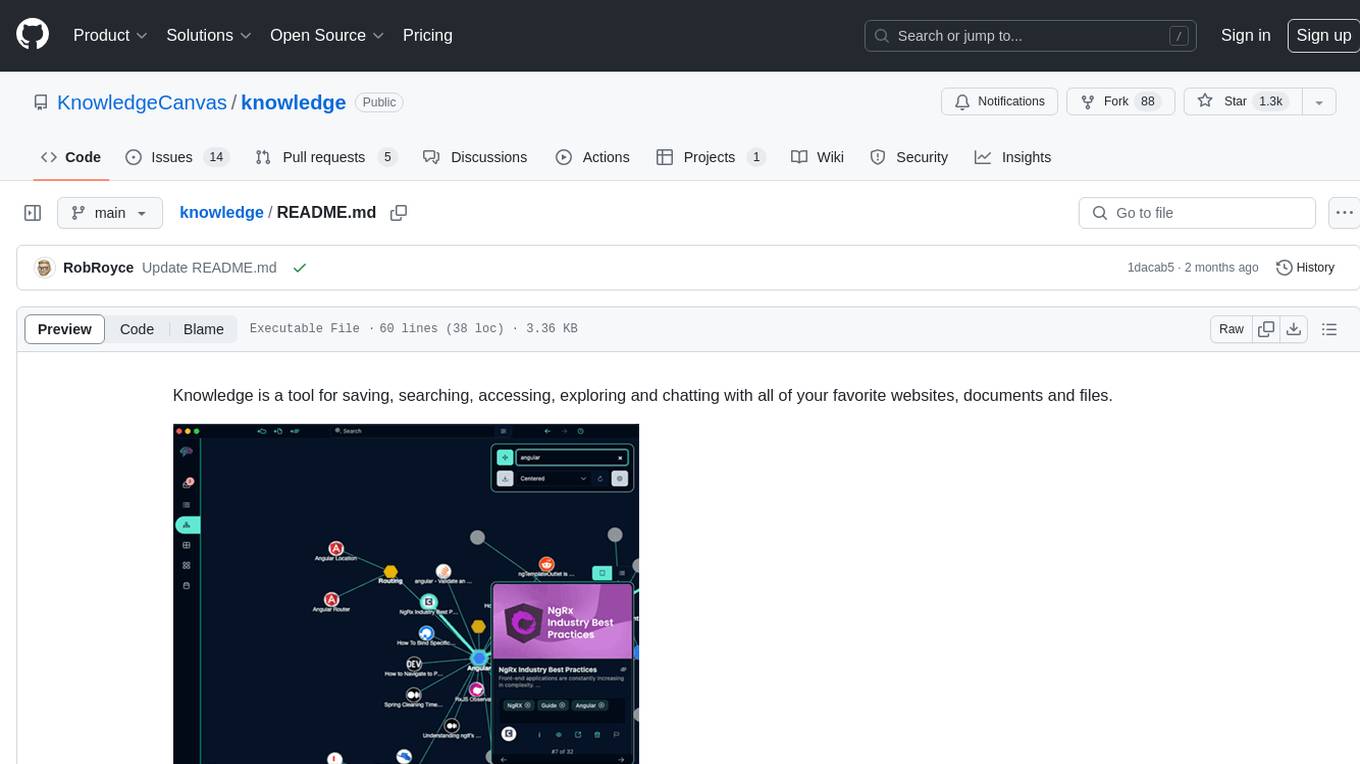
knowledge
Knowledge is a tool for saving, searching, accessing, exploring and chatting with all of your favorite websites, documents and files. Dive into a more interactive learning experience with Knowledge's new Chat feature! Engage in dynamic conversations with your Projects and Sources, leveraging the power of Large Language Models. The Chat feature is designed to transform the way you interact with your data, offering a more engaging and exploratory approach to learning. Unleash the power of context with the built-in Chromium browser. Transform your browsing into knowledge gathering effortlessly.
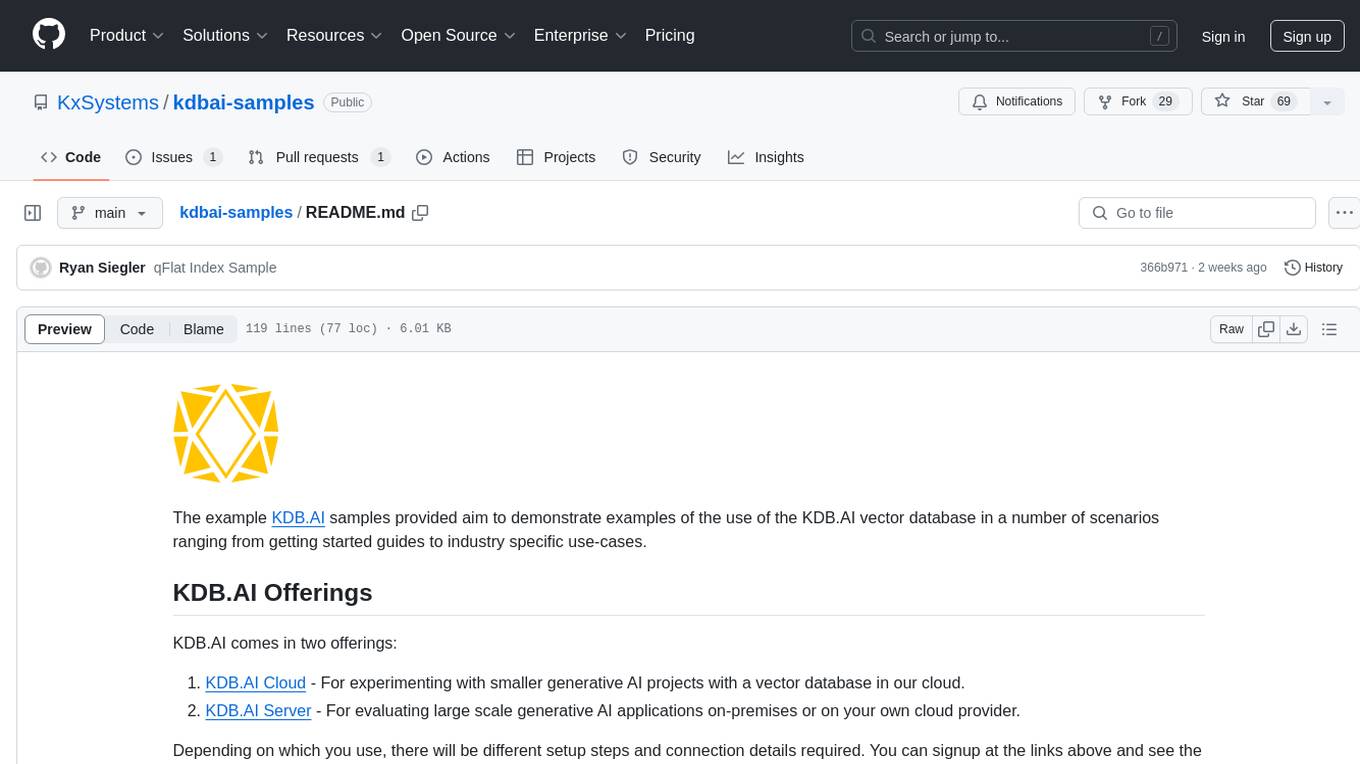
kdbai-samples
KDB.AI is a time-based vector database that allows developers to build scalable, reliable, and real-time applications by providing advanced search, recommendation, and personalization for Generative AI applications. It supports multiple index types, distance metrics, top-N and metadata filtered retrieval, as well as Python and REST interfaces. The repository contains samples demonstrating various use-cases such as temporal similarity search, document search, image search, recommendation systems, sentiment analysis, and more. KDB.AI integrates with platforms like ChatGPT, Langchain, and LlamaIndex. The setup steps require Unix terminal, Python 3.8+, and pip installed. Users can install necessary Python packages and run Jupyter notebooks to interact with the samples.
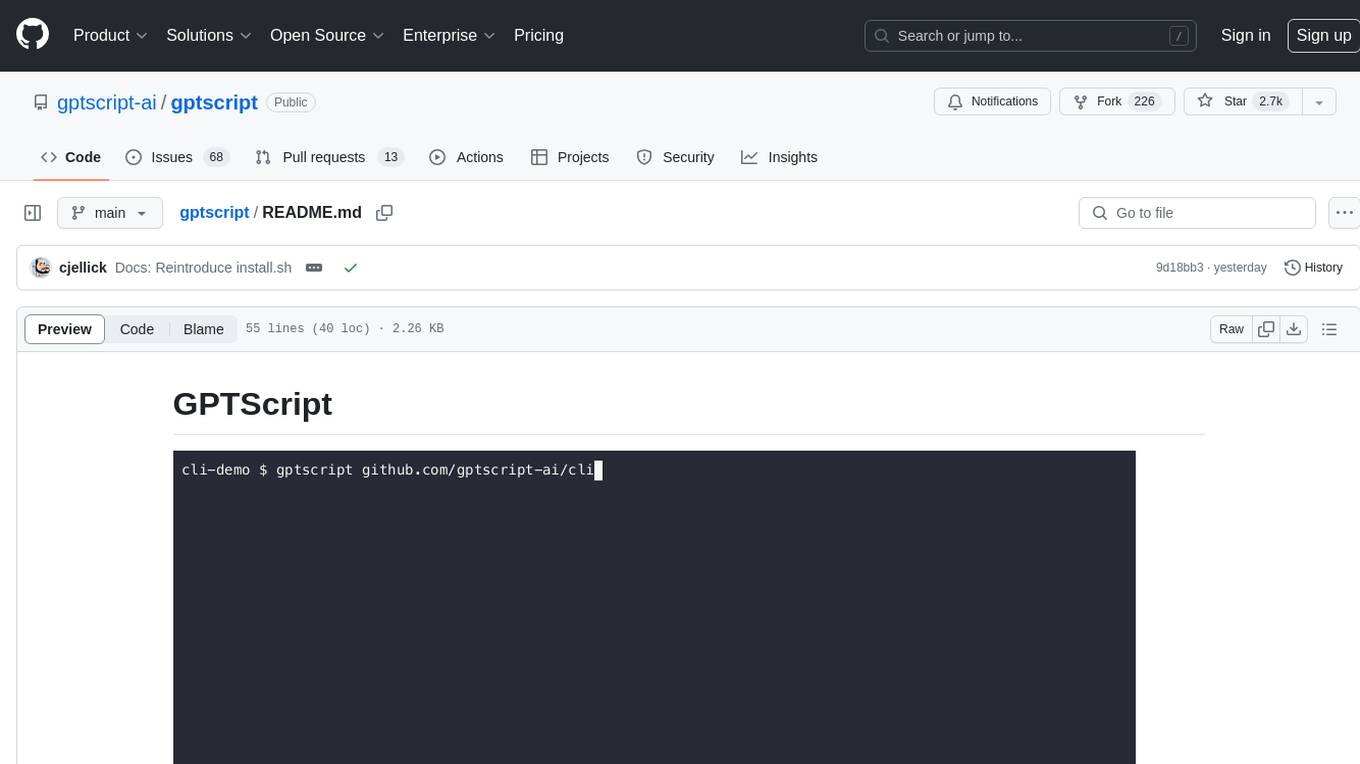
gptscript
GPTScript is a framework that enables Large Language Models (LLMs) to interact with various systems, including local executables, applications with OpenAPI schemas, SDK libraries, or RAG-based solutions. It simplifies the integration of systems with LLMs using minimal prompts. Sample use cases include chatting with a local CLI, OpenAPI compliant endpoint, local files/directories, and running automated workflows.
For similar tasks
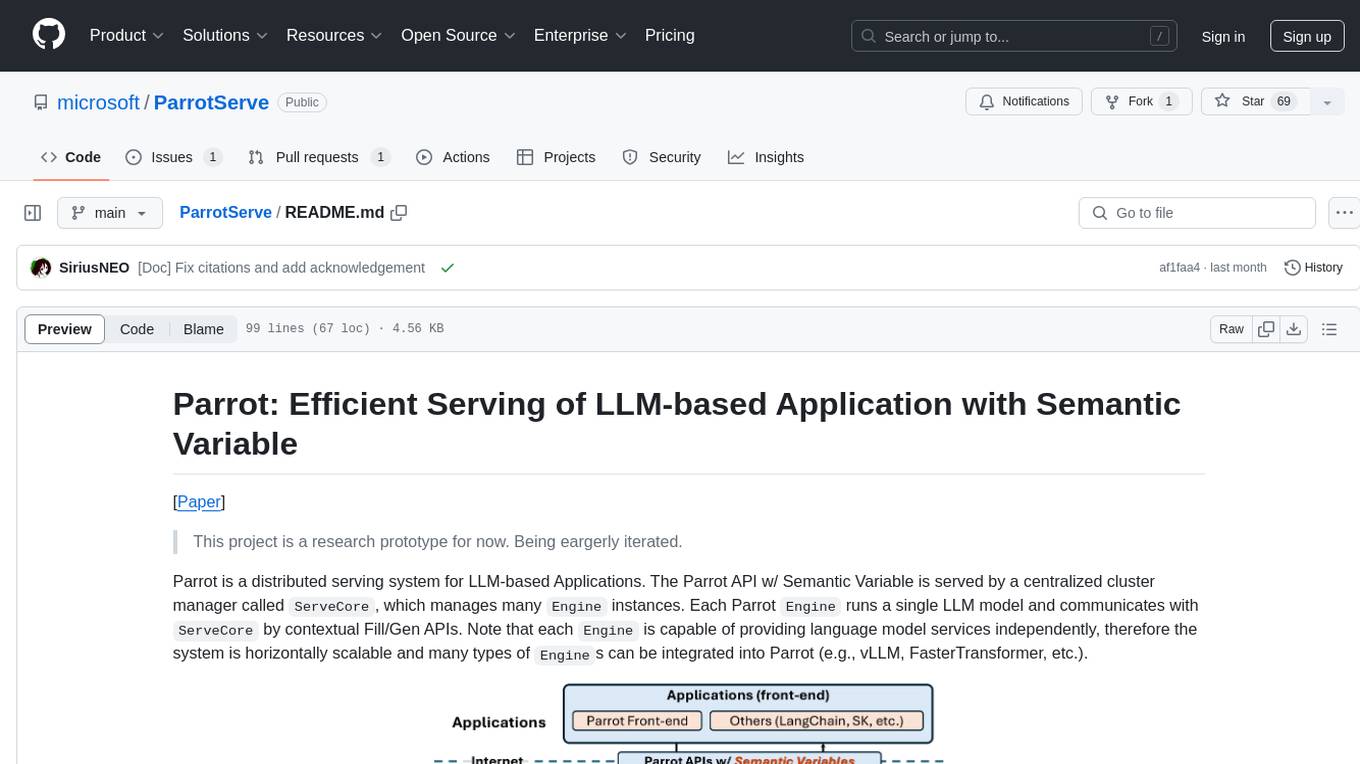
ParrotServe
Parrot is a distributed serving system for LLM-based Applications, designed to efficiently serve LLM-based applications by adding Semantic Variable in the OpenAI-style API. It allows for horizontal scalability with multiple Engine instances running LLM models communicating with ServeCore. The system enables AI agents to interact with LLMs via natural language prompts for collaborative tasks.
For similar jobs
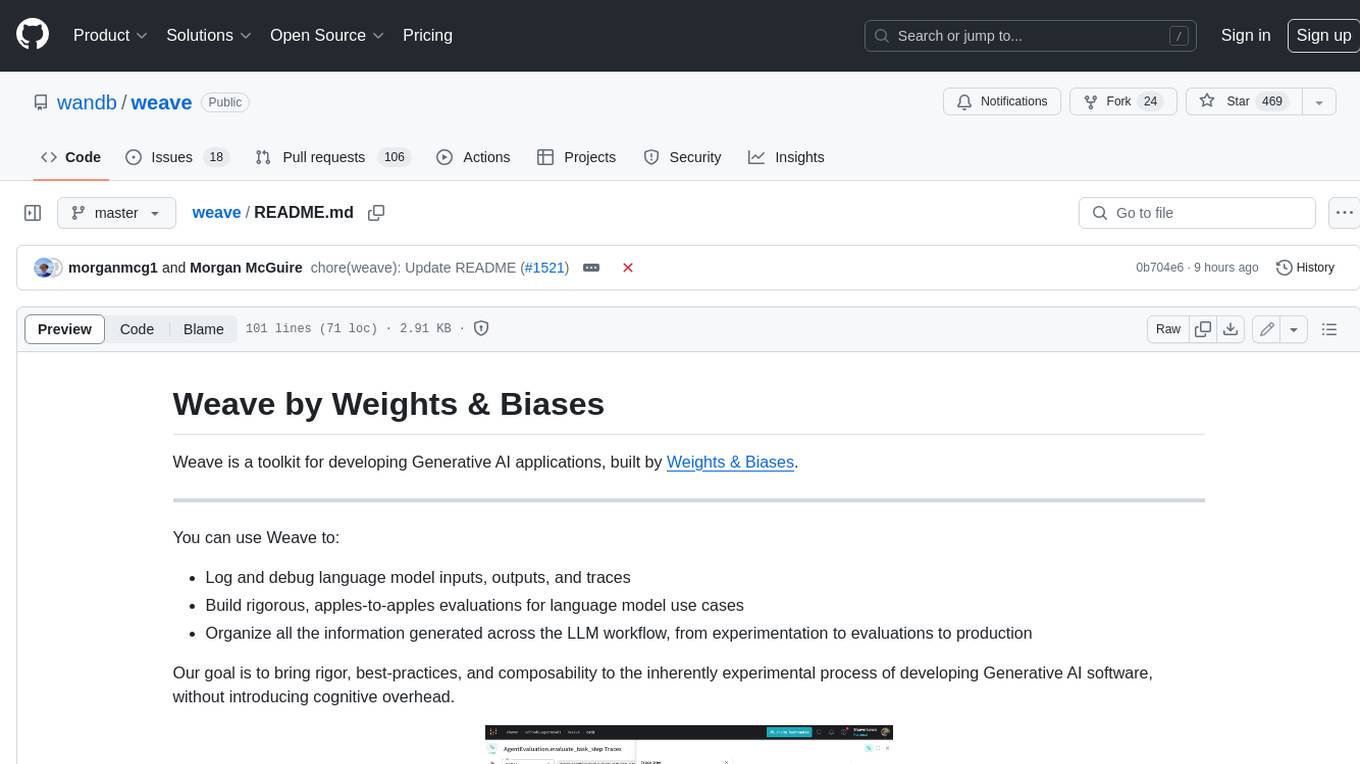
weave
Weave is a toolkit for developing Generative AI applications, built by Weights & Biases. With Weave, you can log and debug language model inputs, outputs, and traces; build rigorous, apples-to-apples evaluations for language model use cases; and organize all the information generated across the LLM workflow, from experimentation to evaluations to production. Weave aims to bring rigor, best-practices, and composability to the inherently experimental process of developing Generative AI software, without introducing cognitive overhead.
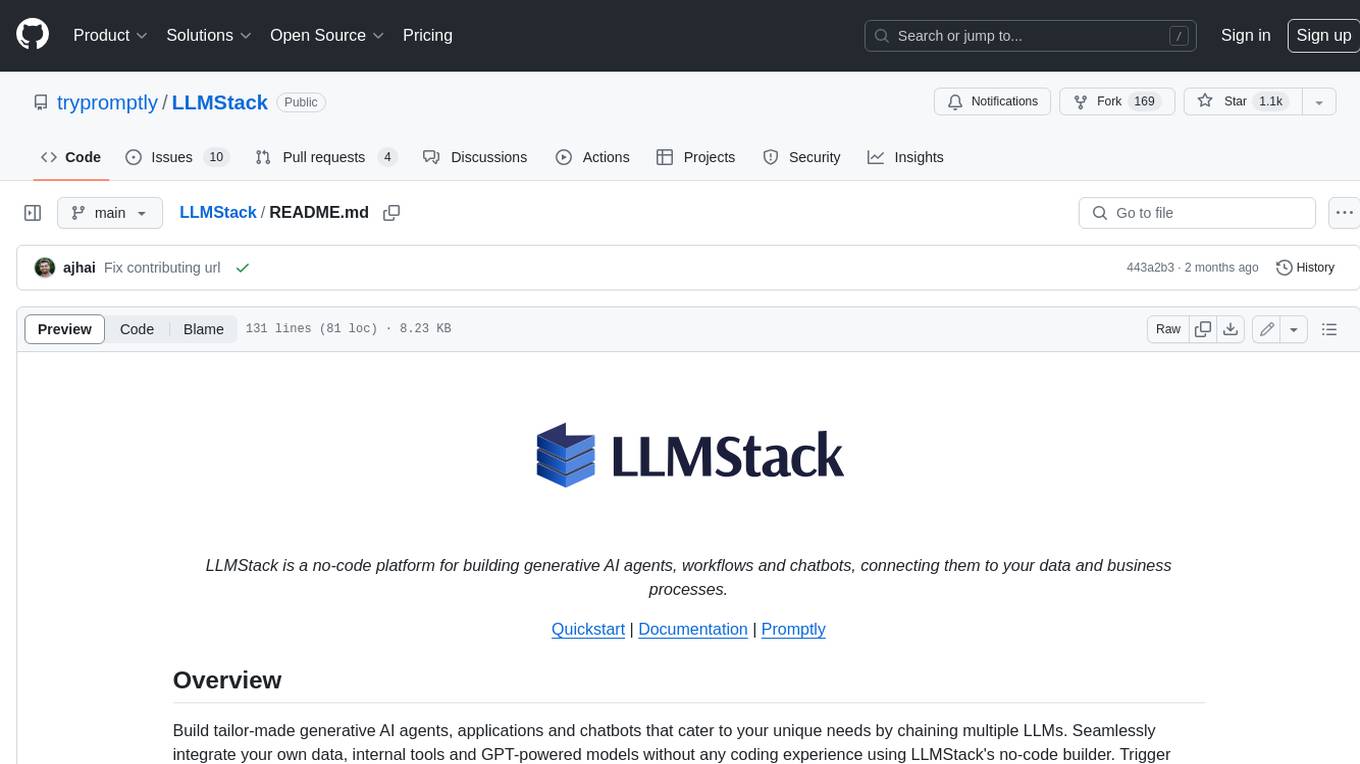
LLMStack
LLMStack is a no-code platform for building generative AI agents, workflows, and chatbots. It allows users to connect their own data, internal tools, and GPT-powered models without any coding experience. LLMStack can be deployed to the cloud or on-premise and can be accessed via HTTP API or triggered from Slack or Discord.
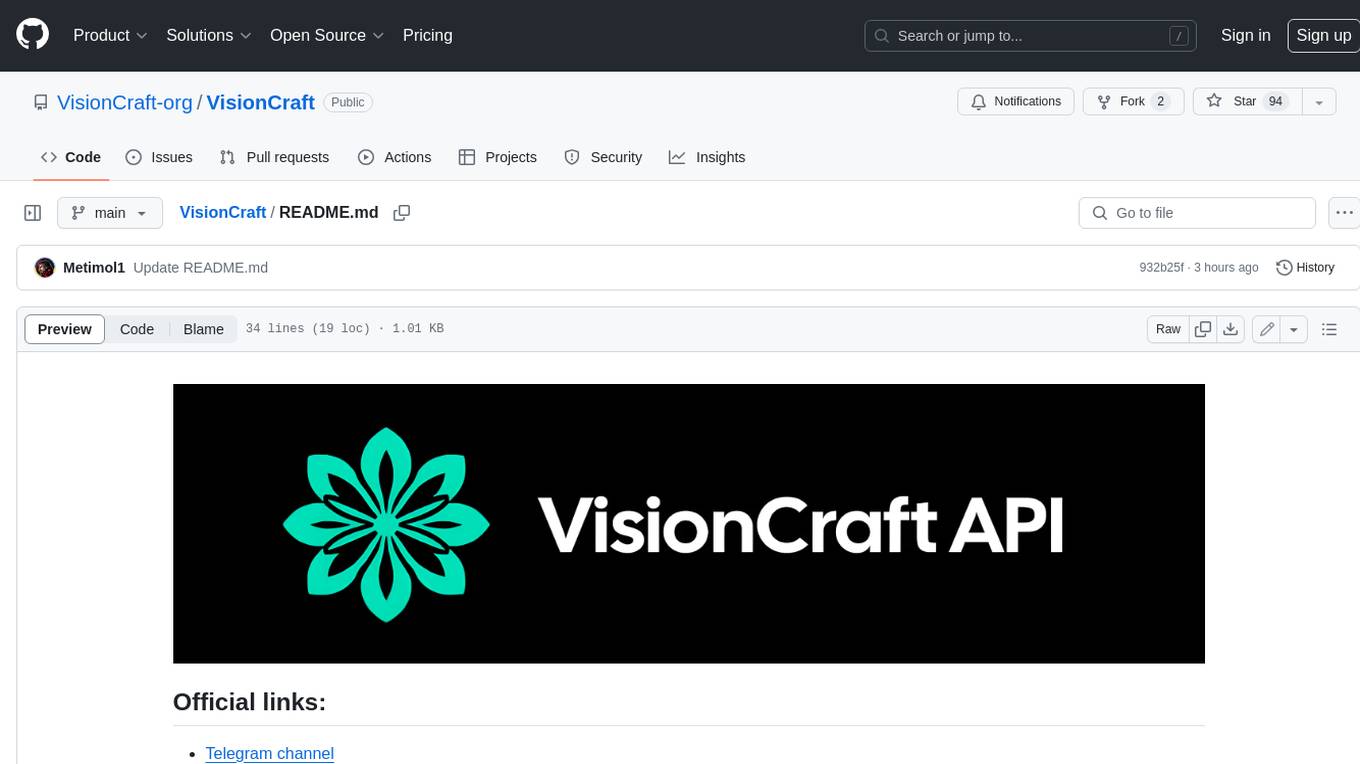
VisionCraft
The VisionCraft API is a free API for using over 100 different AI models. From images to sound.
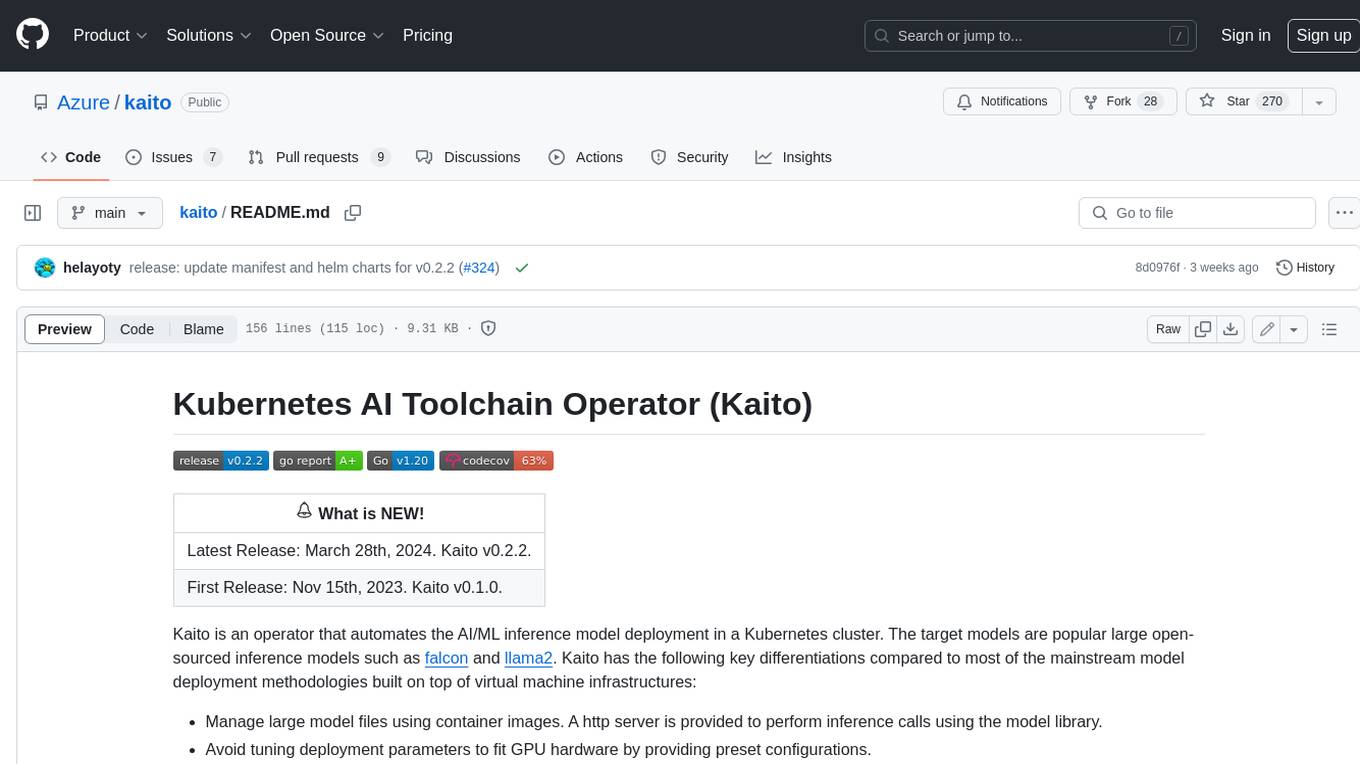
kaito
Kaito is an operator that automates the AI/ML inference model deployment in a Kubernetes cluster. It manages large model files using container images, avoids tuning deployment parameters to fit GPU hardware by providing preset configurations, auto-provisions GPU nodes based on model requirements, and hosts large model images in the public Microsoft Container Registry (MCR) if the license allows. Using Kaito, the workflow of onboarding large AI inference models in Kubernetes is largely simplified.
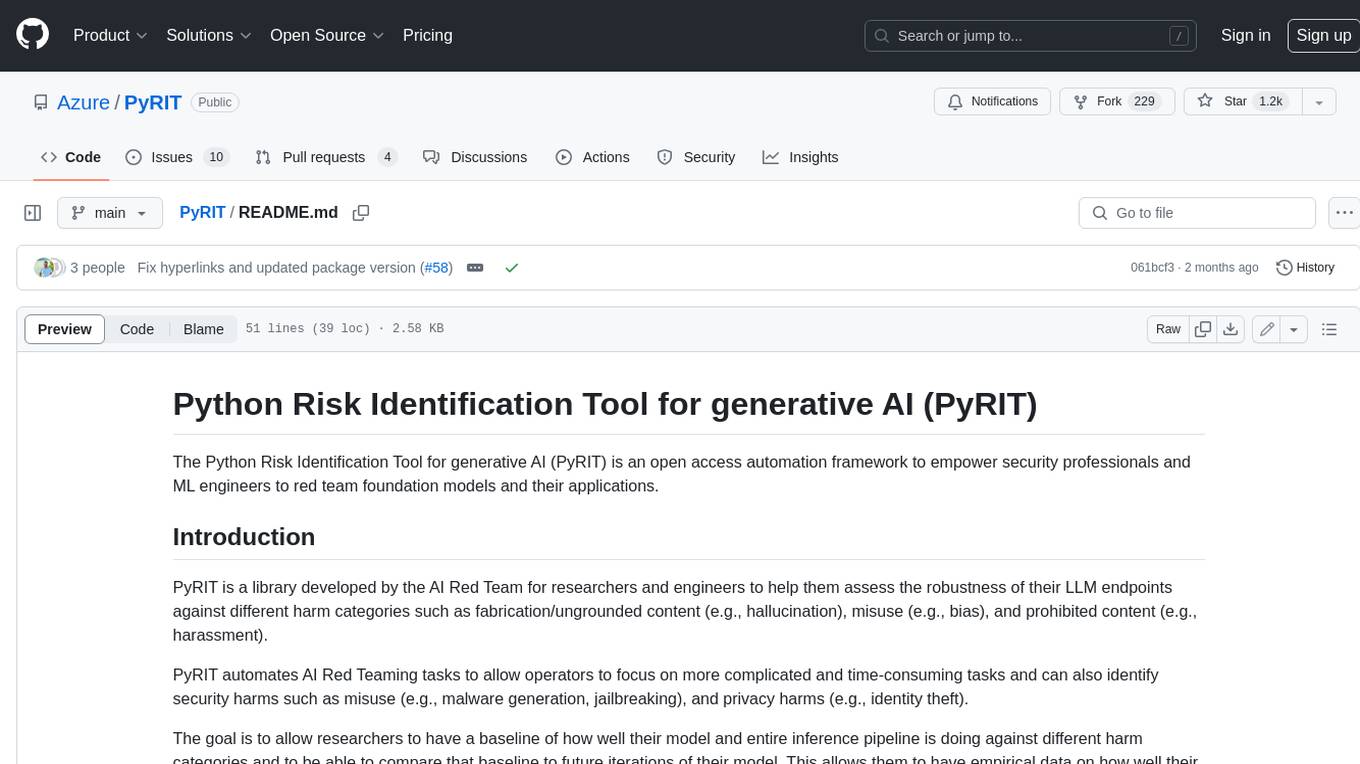
PyRIT
PyRIT is an open access automation framework designed to empower security professionals and ML engineers to red team foundation models and their applications. It automates AI Red Teaming tasks to allow operators to focus on more complicated and time-consuming tasks and can also identify security harms such as misuse (e.g., malware generation, jailbreaking), and privacy harms (e.g., identity theft). The goal is to allow researchers to have a baseline of how well their model and entire inference pipeline is doing against different harm categories and to be able to compare that baseline to future iterations of their model. This allows them to have empirical data on how well their model is doing today, and detect any degradation of performance based on future improvements.
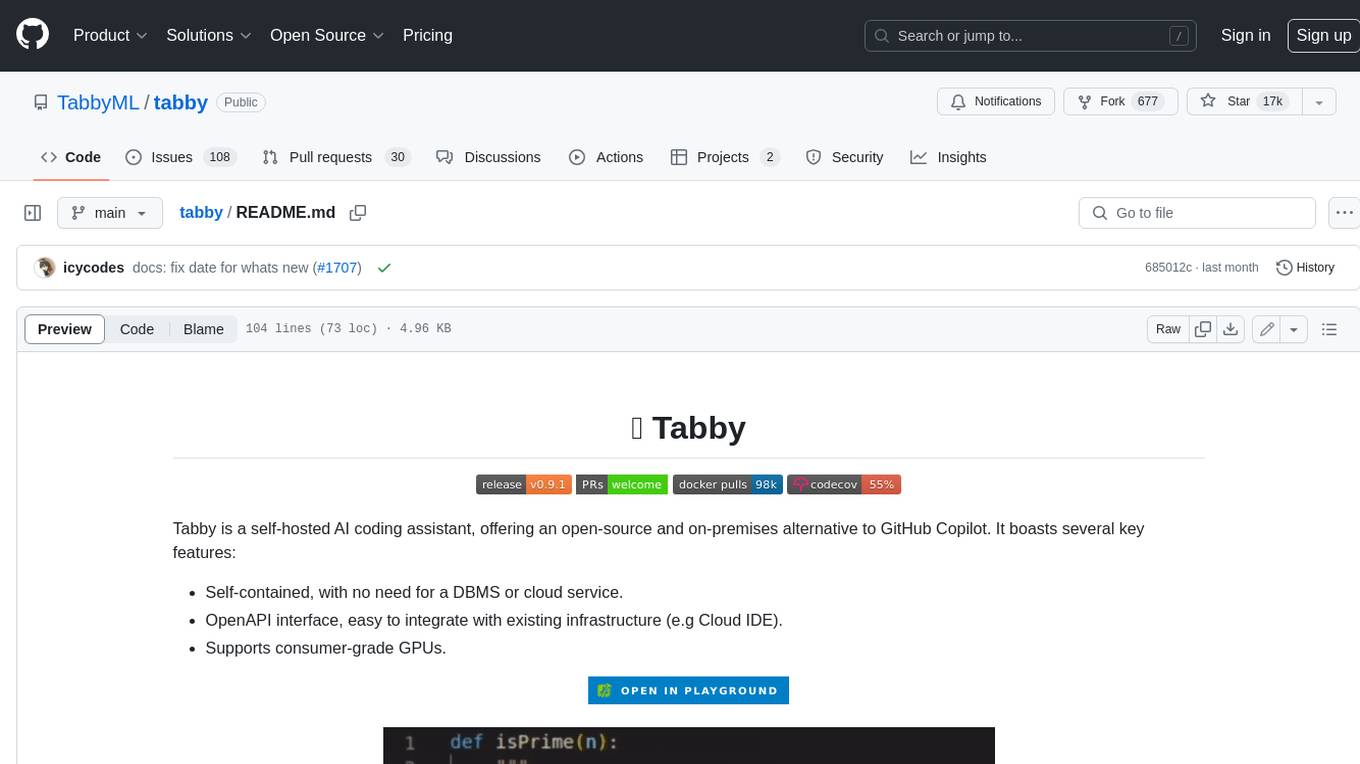
tabby
Tabby is a self-hosted AI coding assistant, offering an open-source and on-premises alternative to GitHub Copilot. It boasts several key features: * Self-contained, with no need for a DBMS or cloud service. * OpenAPI interface, easy to integrate with existing infrastructure (e.g Cloud IDE). * Supports consumer-grade GPUs.
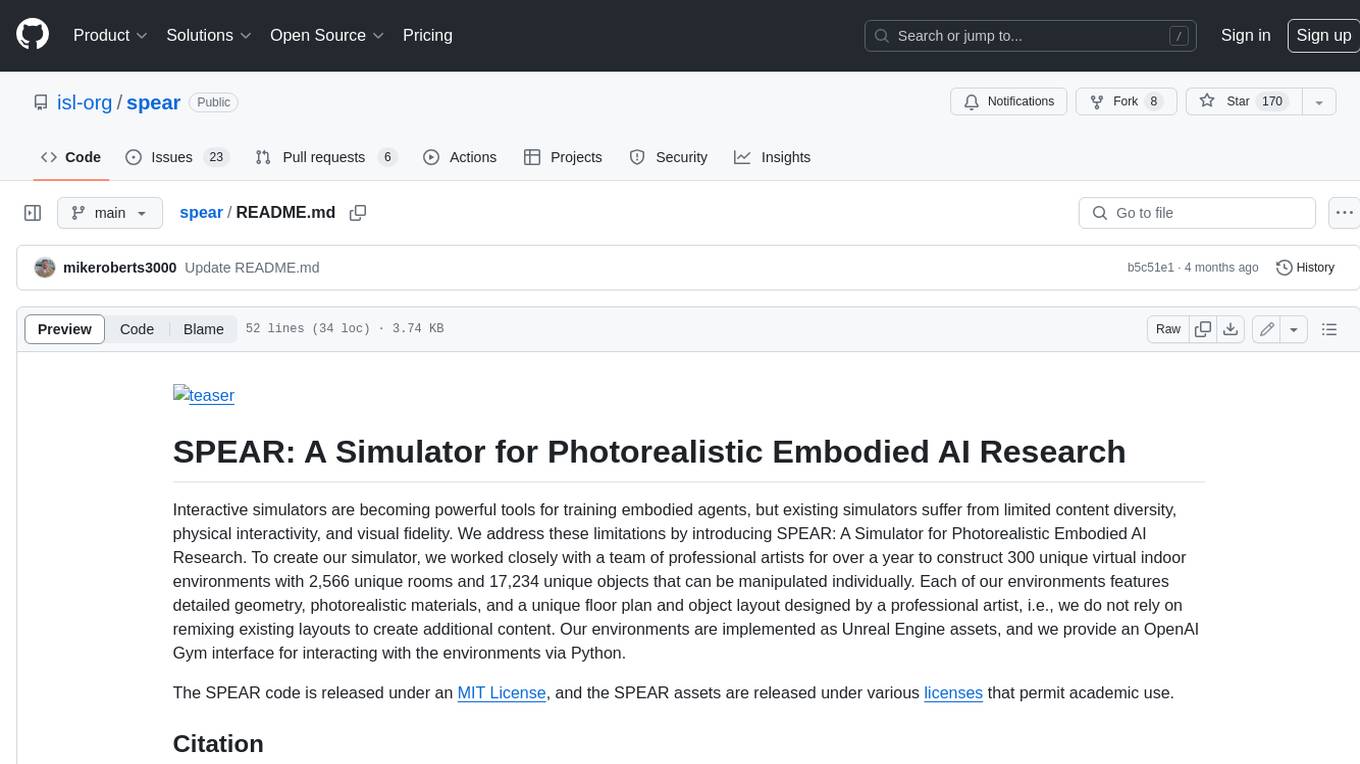
spear
SPEAR (Simulator for Photorealistic Embodied AI Research) is a powerful tool for training embodied agents. It features 300 unique virtual indoor environments with 2,566 unique rooms and 17,234 unique objects that can be manipulated individually. Each environment is designed by a professional artist and features detailed geometry, photorealistic materials, and a unique floor plan and object layout. SPEAR is implemented as Unreal Engine assets and provides an OpenAI Gym interface for interacting with the environments via Python.
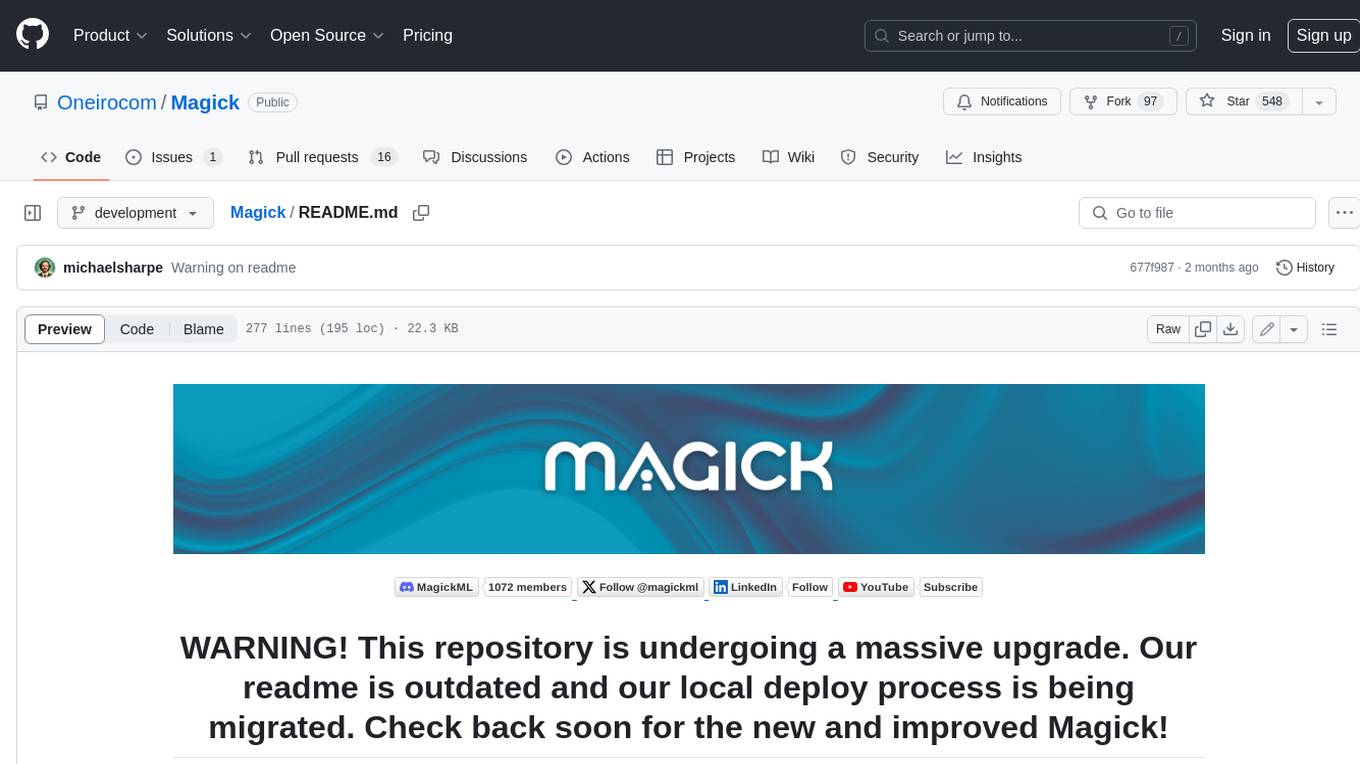
Magick
Magick is a groundbreaking visual AIDE (Artificial Intelligence Development Environment) for no-code data pipelines and multimodal agents. Magick can connect to other services and comes with nodes and templates well-suited for intelligent agents, chatbots, complex reasoning systems and realistic characters.