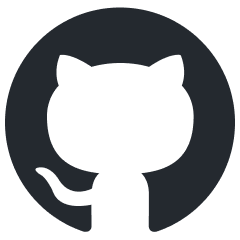
ai2apps
Setup AI2Apps at local system so you can use your own OpenAI key or make more back-end features.
Stars: 278
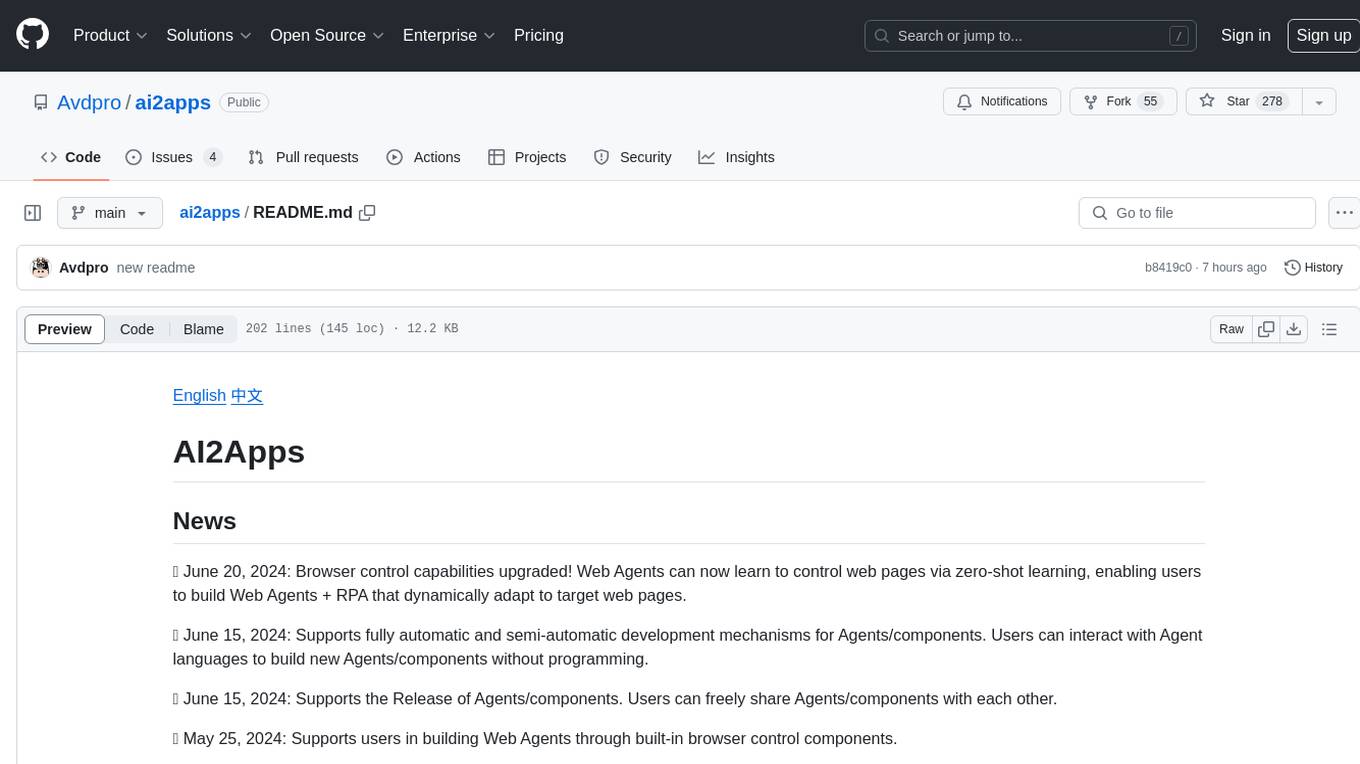
AI2Apps is a visual IDE for building LLM-based AI agent applications, enabling developers to efficiently create AI agents through drag-and-drop, with features like design-to-development for rapid prototyping, direct packaging of agents into apps, powerful debugging capabilities, enhanced user interaction, efficient team collaboration, flexible deployment, multilingual support, simplified product maintenance, and extensibility through plugins.
README:
🔥 June 20, 2024: Browser control capabilities upgraded! Web Agents can now learn to control web pages via zero-shot learning, enabling users to build Web Agents + RPA that dynamically adapt to target web pages.
🔥 June 15, 2024: Supports fully automatic and semi-automatic development mechanisms for Agents/components. Users can interact with Agent languages to build new Agents/components without programming.
🔥 June 15, 2024: Supports the Release of Agents/components. Users can freely share Agents/components with each other.
🔥 May 25, 2024: Supports users in building Web Agents through built-in browser control components.
🔥 May 25, 2024: Supports users in invoking large language models on local computers via Ollama.
🔥 May 25, 2024: Supports users in utilizing existing Agents as APIs or components.
🔥 May 9, 2024: Vitalbridge Capitalhas released an interview article featuring members of the AI2Apps team.
🔥 Apr 14, 2024: Our paper has been published the paper on arXiv:“AI2Apps: A Visual IDE for Building LLM-based AI Agent Applications”!
🎉 Oct 29, 2023: AI2Apps has been creatd!
Welcome to our community!
Feishu |
---|
![]() |
AI2Apps, as the inaugural visual IDE for LLM-based AI agent applications, encompasses the entire development cycle—from prototyping, coding, and agent debugging to final packaging and release—enabling developers to efficiently build AI Agent applications. It integrates engineering-level development tools and full-stack visualization components for both front-end and back-end, empowering developers to swiftly create AI Agents via drag-and-drop, generating publishable and installable apps directly.
https://github.com/pilgrim00/ai2apps/assets/66883561/4c5eaf0d-a426-4cca-88cc-63cd79fab4aa
AI2Apps can be used directly via Web Browser or deployed locally using this project.
Accessed with a desktop browser: https://www.ai2apps.com
When you first open the webpage, the development environment will install and set up. Depending on your browser and internet speed, this process usually takes from a few seconds to one minute. During testing, accessing AI models requires registering and logging into Tab-OS (signing up for a Tab-OS account is completely free). Once you successfully register or log in, you can use the project wizard to create AI Agent projects.
Download this project in your local environment:
git clone https://github.com/Avdpro/ai2apps.git
Within the ai2apps directory:
Edit the .env
file and configure the correct OpenAI Key and server port. The default port is 3015:
APIROOT=https://www.ai2apps.com/ws/
OPENAI_API_KEY=sk-XXXXXXXXXXXXXXXXXXXXXXXXXXXXXXXXXXXXXXXXXXXXXXXX
PORT=3015
Install dependecies:
nmp install
Start services:
node ./start.js
Assuming the specified port is 3015, open the following URL in your browser:
http://localhost:3015/
Just like accessing ai2apps.com directly, the first visit will initiate the installation and configuration process.
Easily design Agent logic with drag-and-drop to create topological diagrams, which automatically synchronize with Agent code, saving significant programming time.
Completed AI Agents can be packaged into standalone web/mobile apps (currently supporting iOS and Android systems). They can also integrate seamlessly as AI extensions into existing websites/apps with just a few lines of code.
AI2Apps offers robust Agent debugging features: use breakpoints, step-by-step execution, and GPT camouflage to quickly identify issues and improve Agent performance, saving both development time and AI invocation costs.
In many scenarios, chatting isn't the most effective form of user interaction. AI2Apps supports various UI controls during development such as menus, buttons, and charts, enabling Agents to interact with users like professional apps.
Taking cues from popular collaborative design tools like Figma, AI2Apps supports real-time sharing of creative progress via QR code links and facilitates collaborative development with built-in version control.
AI2Apps is equipped with Web-IDE features, which can be used after opening the browser after deployment, ensuring seamless connectivity across smartphones, computers, tablets, VR headsets, and other devices.Each Agent project operates within a secure sandbox environment, isolated in independent browser tab pages without the need for server-side Docker or container configurations.
Traditional approaches to multilingual support often prove cumbersome and tedious. AI2Apps streamlines multilingual development with AI assistance, enabling developers to efficiently localize entire Agent projects with just a few clicks.
Unlike traditional methods where code implementation can diverge from original designs, the "design-to-development" of AI2Apps approach ensures continuous synchronization between design and code. Topological diagrams embedded in AI Agent code provide clear insights into original design intentions, facilitating faster issue resolution compared to navigating dense code.
Similar to popular open-source IDEs like VSCode, AI2Apps supports full extensibility. Developers can encapsulate external code into microservices and easily extend functionality via Add-Ons, creating customized visual plugins as needed.
In AI2Apps, each Agent is an independent js file, and the topology map information is saved in the form of comments at the end of the file, ensuring real-time synchronization between design and implementation. The Agent file editing interface offers both "Code" and "Canvas" modes, with "Canvas" mode as the default upon opening an Agent.
In the Canvas mode of the development IDE:
On the left side, you have the organizational view of the Agent. Here, you can see the Agent objects and the list of "execution segments" they contain. Clicking on a project selects the object. In the middle, there's the Agent topology diagram canvas. Here, you can drag and drop to create "execution segments" and connect them together by dragging connections between segments. On the right side, there's the object property editor view. Here, editable properties of the currently selected object are listed. For example, parameters like model selection and temperature settings when calling ChatGPT.
In the Code mode of the development IDE:
On the left and right sides, the organizational structure of the Agent and the object property editing view remain unchanged. In the center, however, is the Agent code. When editing the Agent topology diagram and object properties, the code updates automatically. Developers can also manually write code to implement logic that cannot be achieved through visual editing alone.
The run button is located in both the organizational structure panel on the left side of the IDE and the comprehensive toolbar at the bottom. Clicking the run button starts either the debugging mode or terminal mode to run the current Agent project. Once the Agent is launched, you can test it through dialogue interactions. Launching an AI Agent in Debug mode activates the debugging environment. In Debug mode, click the "Debug" button at the top of the UI to access the debugging view. **Information Flow and Breakpoints** On the left side of the debugging view is the message flow, where you can review detailed conversation sequences, including the content of each step in and out. Clicking on a step's name within the flow opens detailed logs on the right side and allows you to set breakpoints.Single Step Execution and Breakpoint Operations At the bottom of the UI, you can turn on the single-step execution function. When executing the AI Agent in a single step or encountering a breakpoint, the debugger will pause the execution and report the execution information of the current step to the user, so that the user can modify the input/output information of the step to see different effects.
GPT Cheat GPT Cheat can be used during debugging by clicking on the step to call GPT and adding a GPT Cheat in the right panel. with GPT Cheat it is possible to simulate (bypass) a ChatGPT call with a preconfigured result, which saves time and costs.
Topology Trace and Debug
While debugging an AI Agent, the Agent's topology diagram updates dynamically, highlighting the paths and parameter transfers involved in each call. Executed paths are highlighted with bold blue lines, and input/output details are listed in the Trace Log within the object properties view.
If using a self-deployed AI2Apps environment configured with an OpenAI Key, invoking ChatGPT utilizes the developer's own OpenAI credits without any system-imposed restrictions. When running/debugging an Agent on www.ai2apps.com, the system covers the costs incurred from ChatGPT calls. To prevent unexpected bills, the system limits ChatGPT usage through an "energy" system.
After successfully registering and logging into Tab-OS, users receive a certain amount of free energy credits. Additionally, each day upon logging in, users receive a replenishment of energy based on their current user level. Successfully referring new members also earns users free system tokens, which can be exchanged for energy credits.
Once an Agent is edited and finalized, it can be packaged and released as a web or mobile application (iOS/Android).
- Support for Wechat bot
- More related documents and examples
If you find our work useful for your research or application, please cite our paper AI2Apps
@article{pang2024ai2apps,
title={AI2Apps: A Visual IDE for Building LLM-based AI Agent Applications},
author={Pang, Xin and Li, Zhucong and Chen, Jiaxiang and Cheng, Yuan and Xu, Yinghui and Qi, Yuan},
journal={arXiv preprint arXiv:2404.04902},
year={2024}
}
For Tasks:
Click tags to check more tools for each tasksFor Jobs:
Alternative AI tools for ai2apps
Similar Open Source Tools
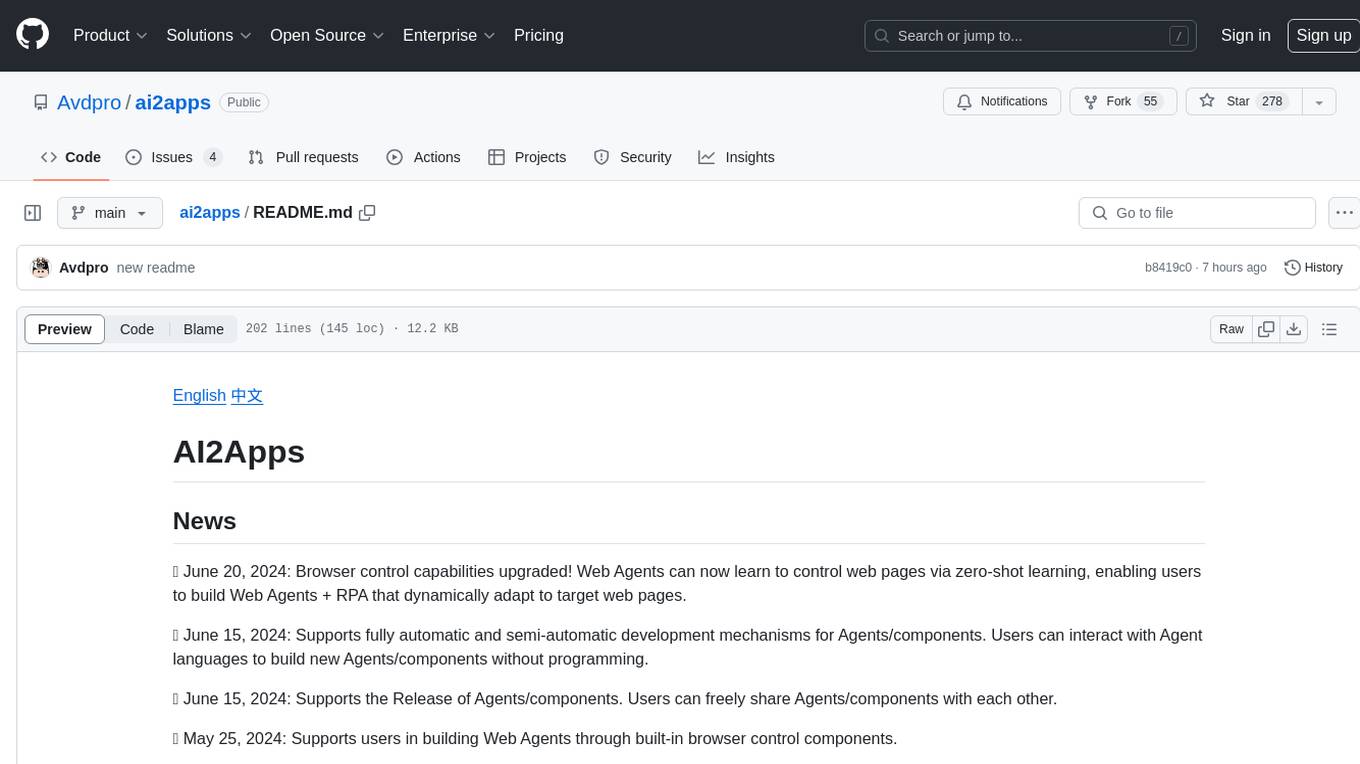
ai2apps
AI2Apps is a visual IDE for building LLM-based AI agent applications, enabling developers to efficiently create AI agents through drag-and-drop, with features like design-to-development for rapid prototyping, direct packaging of agents into apps, powerful debugging capabilities, enhanced user interaction, efficient team collaboration, flexible deployment, multilingual support, simplified product maintenance, and extensibility through plugins.
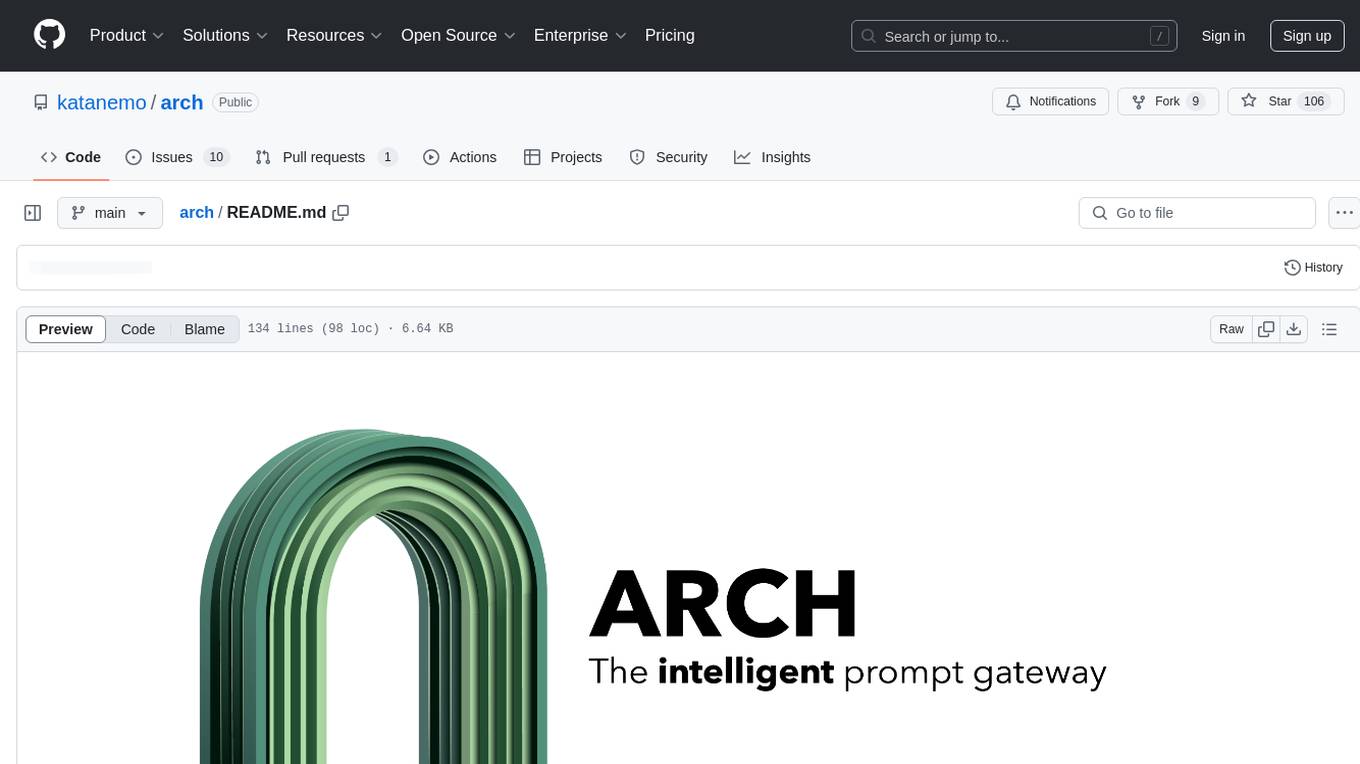
arch
Arch is an intelligent Layer 7 gateway designed to protect, observe, and personalize LLM applications with APIs. It handles tasks like detecting and rejecting jailbreak attempts, calling backend APIs, disaster recovery, and observability. Built on Envoy Proxy, it offers features like function calling, prompt guardrails, traffic management, and standards-based observability. Arch aims to improve the speed, security, and personalization of generative AI applications.
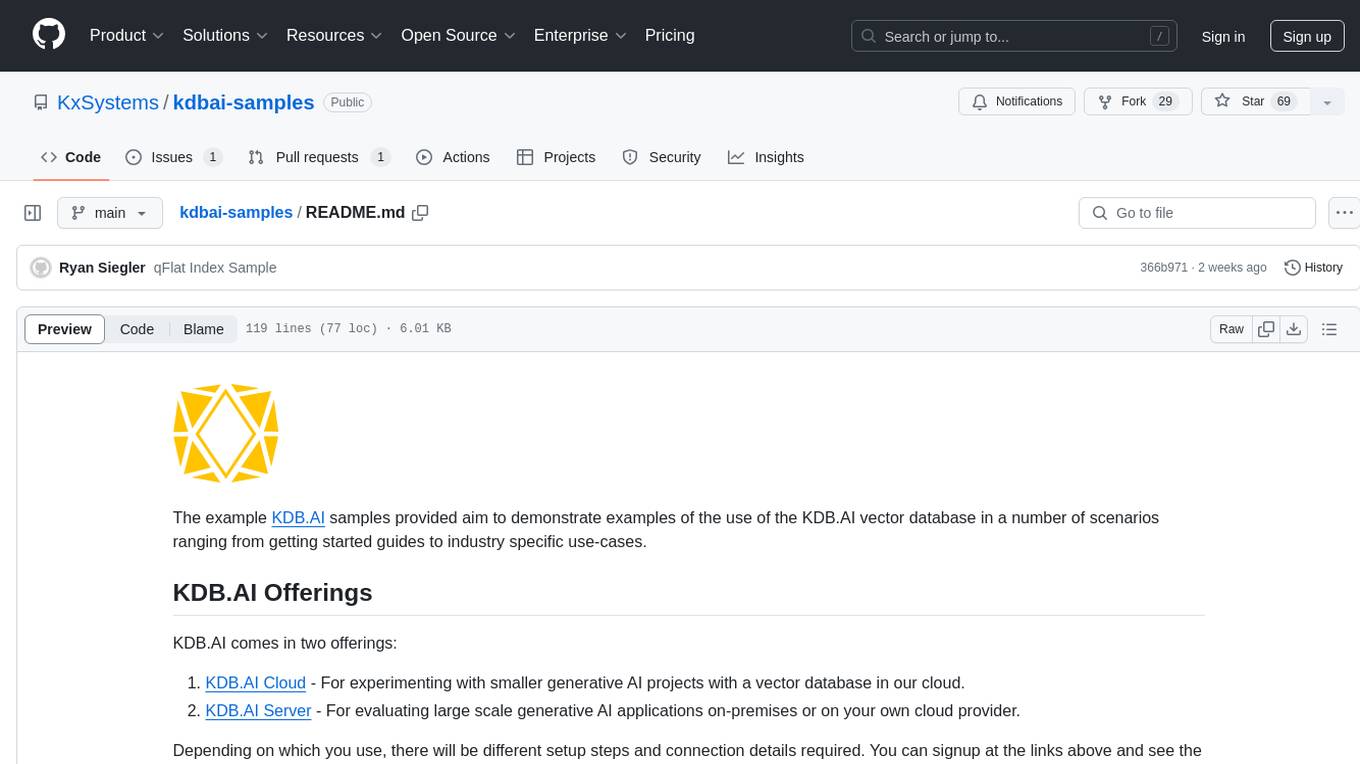
kdbai-samples
KDB.AI is a time-based vector database that allows developers to build scalable, reliable, and real-time applications by providing advanced search, recommendation, and personalization for Generative AI applications. It supports multiple index types, distance metrics, top-N and metadata filtered retrieval, as well as Python and REST interfaces. The repository contains samples demonstrating various use-cases such as temporal similarity search, document search, image search, recommendation systems, sentiment analysis, and more. KDB.AI integrates with platforms like ChatGPT, Langchain, and LlamaIndex. The setup steps require Unix terminal, Python 3.8+, and pip installed. Users can install necessary Python packages and run Jupyter notebooks to interact with the samples.
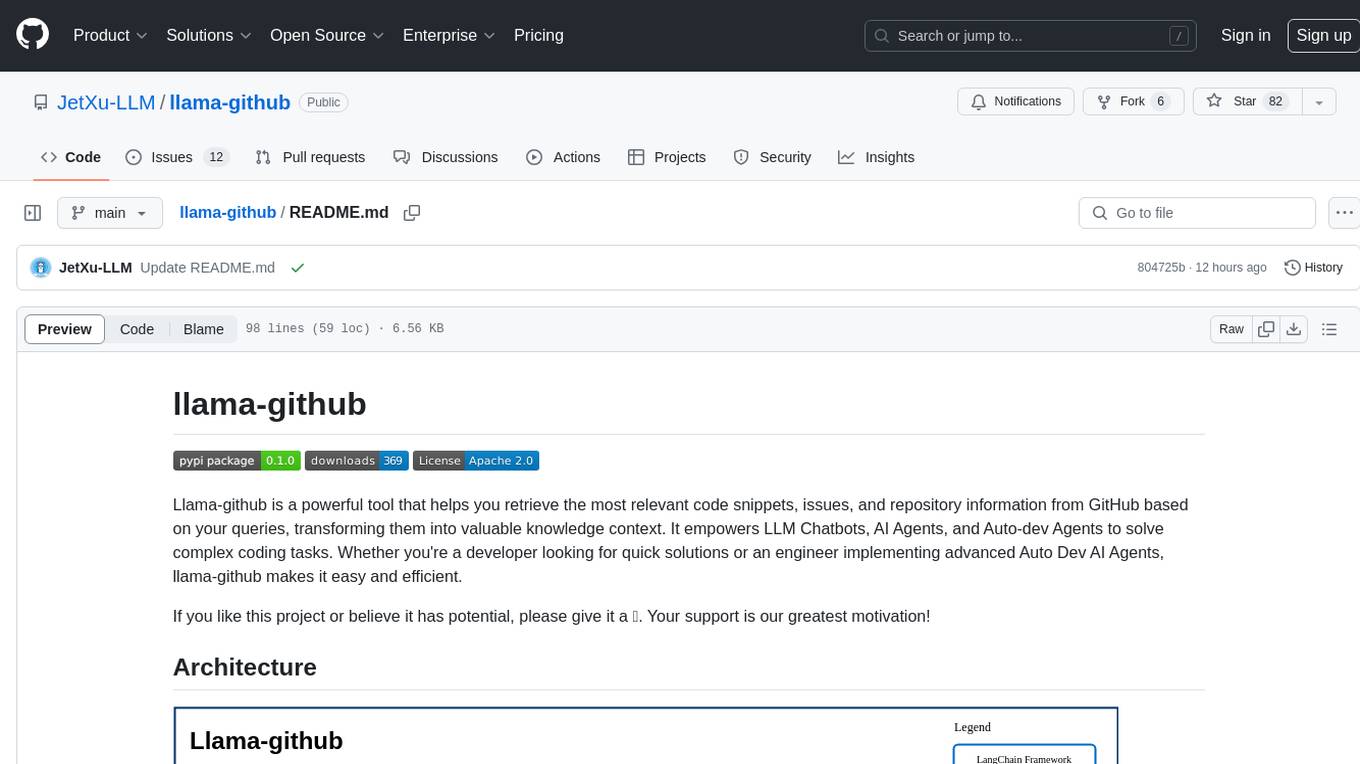
llama-github
Llama-github is a powerful tool that helps retrieve relevant code snippets, issues, and repository information from GitHub based on queries. It empowers AI agents and developers to solve coding tasks efficiently. With features like intelligent GitHub retrieval, repository pool caching, LLM-powered question analysis, and comprehensive context generation, llama-github excels at providing valuable knowledge context for development needs. It supports asynchronous processing, flexible LLM integration, robust authentication options, and logging/error handling for smooth operations and troubleshooting. The vision is to seamlessly integrate with GitHub for AI-driven development solutions, while the roadmap focuses on empowering LLMs to automatically resolve complex coding tasks.
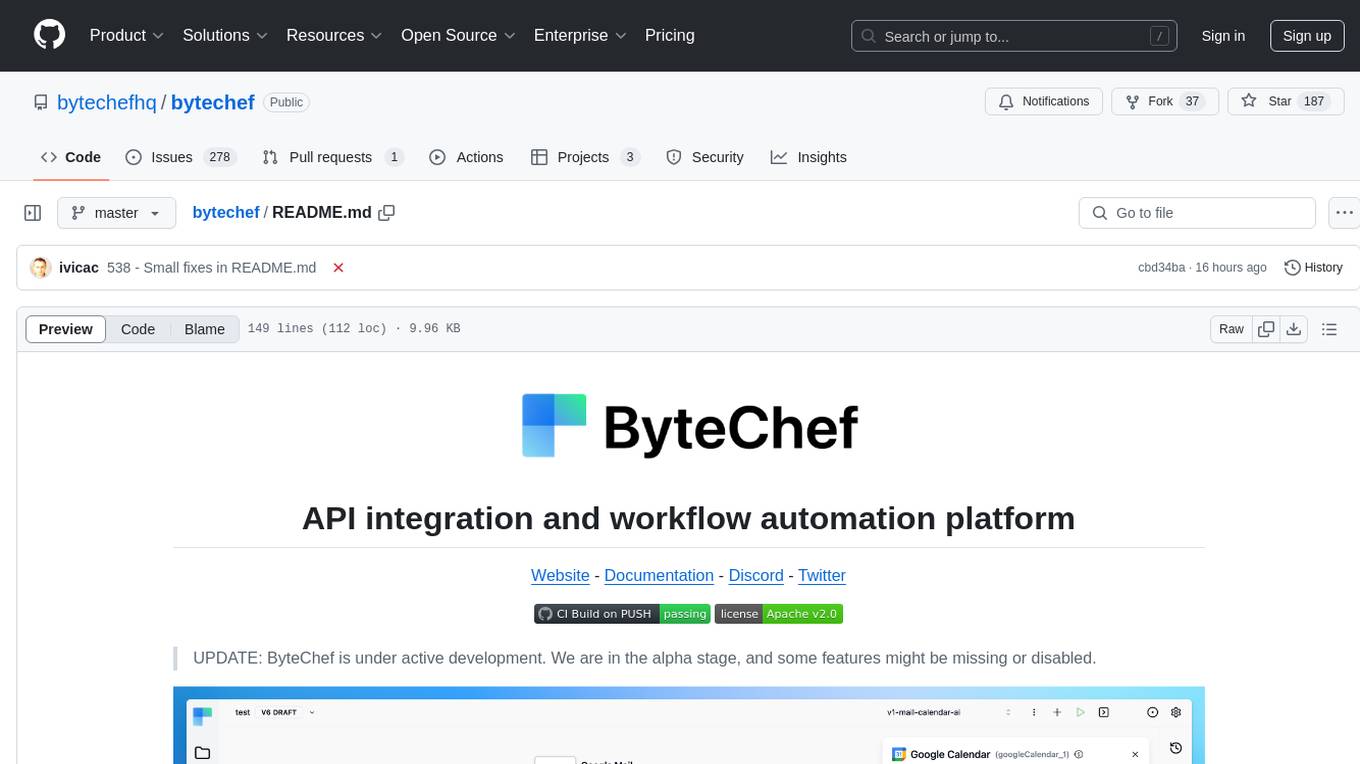
bytechef
ByteChef is an open-source, low-code, extendable API integration and workflow automation platform. It provides an intuitive UI Workflow Editor, event-driven & scheduled workflows, multiple flow controls, built-in code editor supporting Java, JavaScript, Python, and Ruby, rich component ecosystem, extendable with custom connectors, AI-ready with built-in AI components, developer-ready to expose workflows as APIs, version control friendly, self-hosted, scalable, and resilient. It allows users to build and visualize workflows, automate tasks across SaaS apps, internal APIs, and databases, and handle millions of workflows with high availability and fault tolerance.
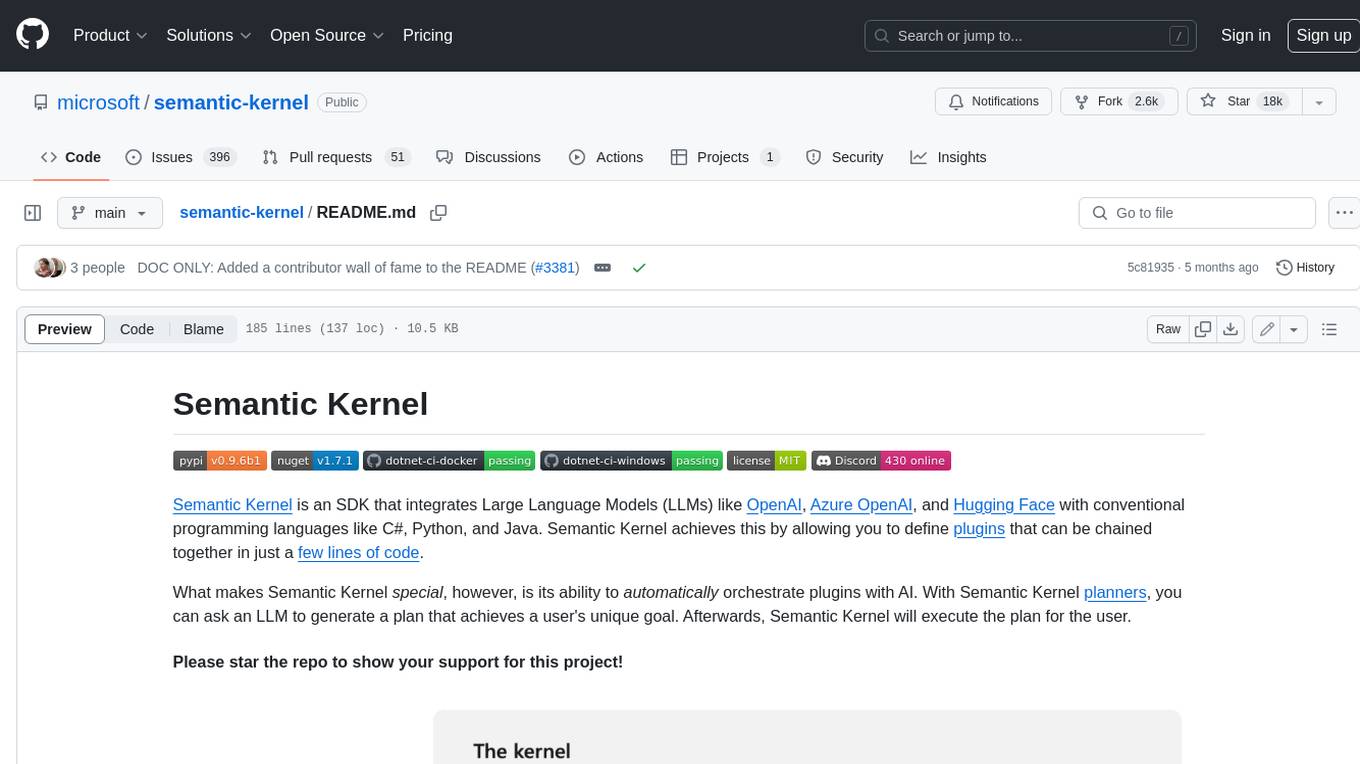
semantic-kernel
Semantic Kernel is an SDK that integrates Large Language Models (LLMs) like OpenAI, Azure OpenAI, and Hugging Face with conventional programming languages like C#, Python, and Java. Semantic Kernel achieves this by allowing you to define plugins that can be chained together in just a few lines of code. What makes Semantic Kernel _special_ , however, is its ability to _automatically_ orchestrate plugins with AI. With Semantic Kernel planners, you can ask an LLM to generate a plan that achieves a user's unique goal. Afterwards, Semantic Kernel will execute the plan for the user.
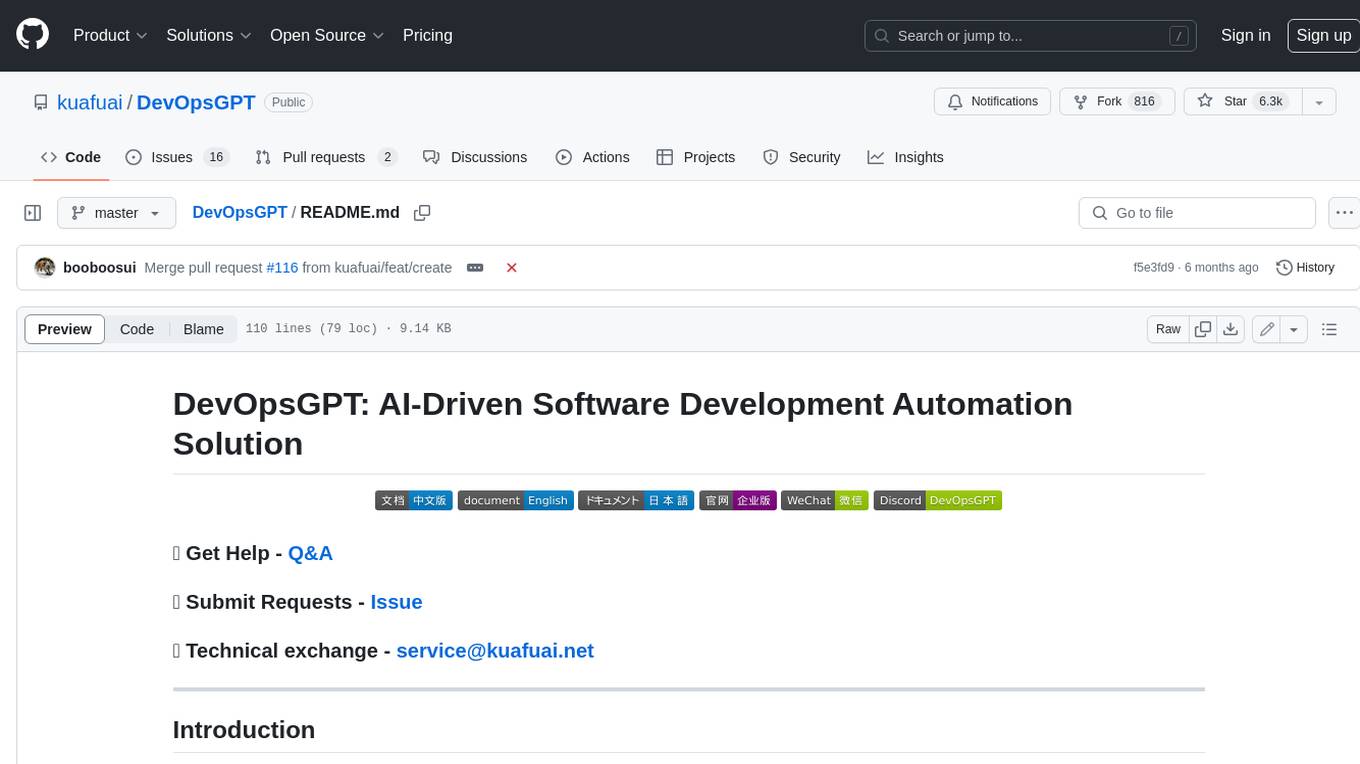
DevOpsGPT
DevOpsGPT is an AI-driven software development automation solution that combines Large Language Models (LLM) with DevOps tools to convert natural language requirements into working software. It improves development efficiency by eliminating the need for tedious requirement documentation, shortens development cycles, reduces communication costs, and ensures high-quality deliverables. The Enterprise Edition offers features like existing project analysis, professional model selection, and support for more DevOps platforms. The tool automates requirement development, generates interface documentation, provides pseudocode based on existing projects, facilitates code refinement, enables continuous integration, and supports software version release. Users can run DevOpsGPT with source code or Docker, and the tool comes with limitations in precise documentation generation and understanding existing project code. The product roadmap includes accurate requirement decomposition, rapid import of development requirements, and integration of more software engineering and professional tools for efficient software development tasks under AI planning and execution.
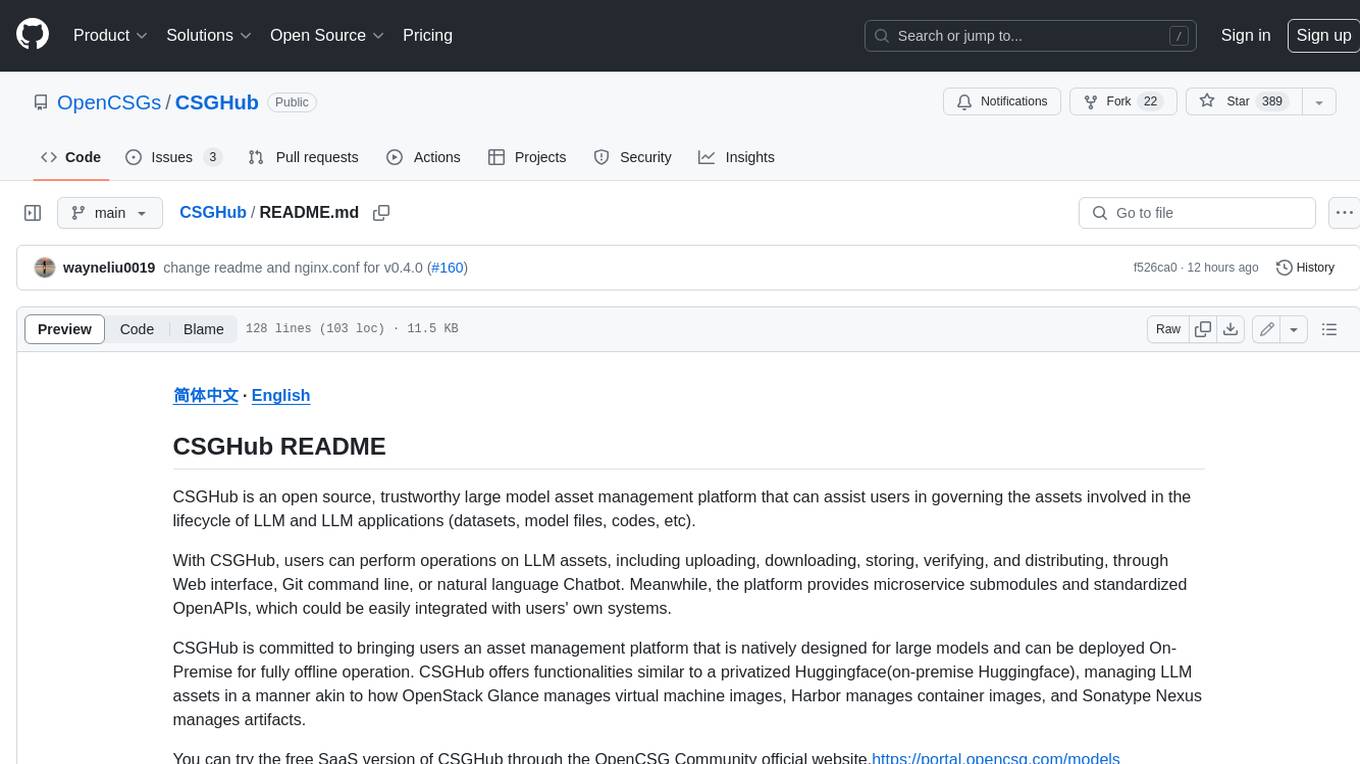
CSGHub
CSGHub is an open source, trustworthy large model asset management platform that can assist users in governing the assets involved in the lifecycle of LLM and LLM applications (datasets, model files, codes, etc). With CSGHub, users can perform operations on LLM assets, including uploading, downloading, storing, verifying, and distributing, through Web interface, Git command line, or natural language Chatbot. Meanwhile, the platform provides microservice submodules and standardized OpenAPIs, which could be easily integrated with users' own systems. CSGHub is committed to bringing users an asset management platform that is natively designed for large models and can be deployed On-Premise for fully offline operation. CSGHub offers functionalities similar to a privatized Huggingface(on-premise Huggingface), managing LLM assets in a manner akin to how OpenStack Glance manages virtual machine images, Harbor manages container images, and Sonatype Nexus manages artifacts.
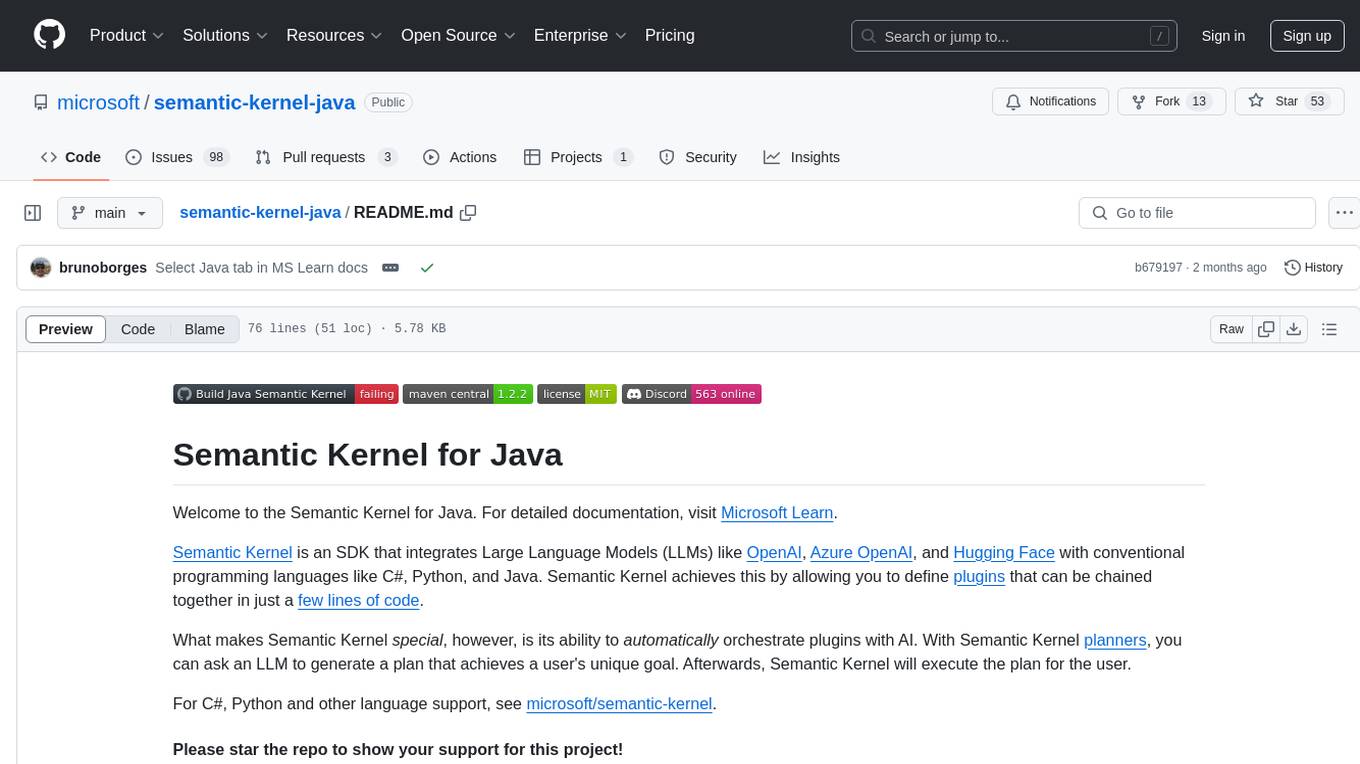
semantic-kernel-java
Semantic Kernel for Java is an SDK that integrates Large Language Models (LLMs) like OpenAI, Azure OpenAI, and Hugging Face with conventional programming languages like C#, Python, and Java. It allows defining plugins that can be chained together in just a few lines of code. The tool automatically orchestrates plugins with AI, enabling users to generate plans to achieve unique goals and execute them. The project welcomes contributions, bug reports, and suggestions from the community.
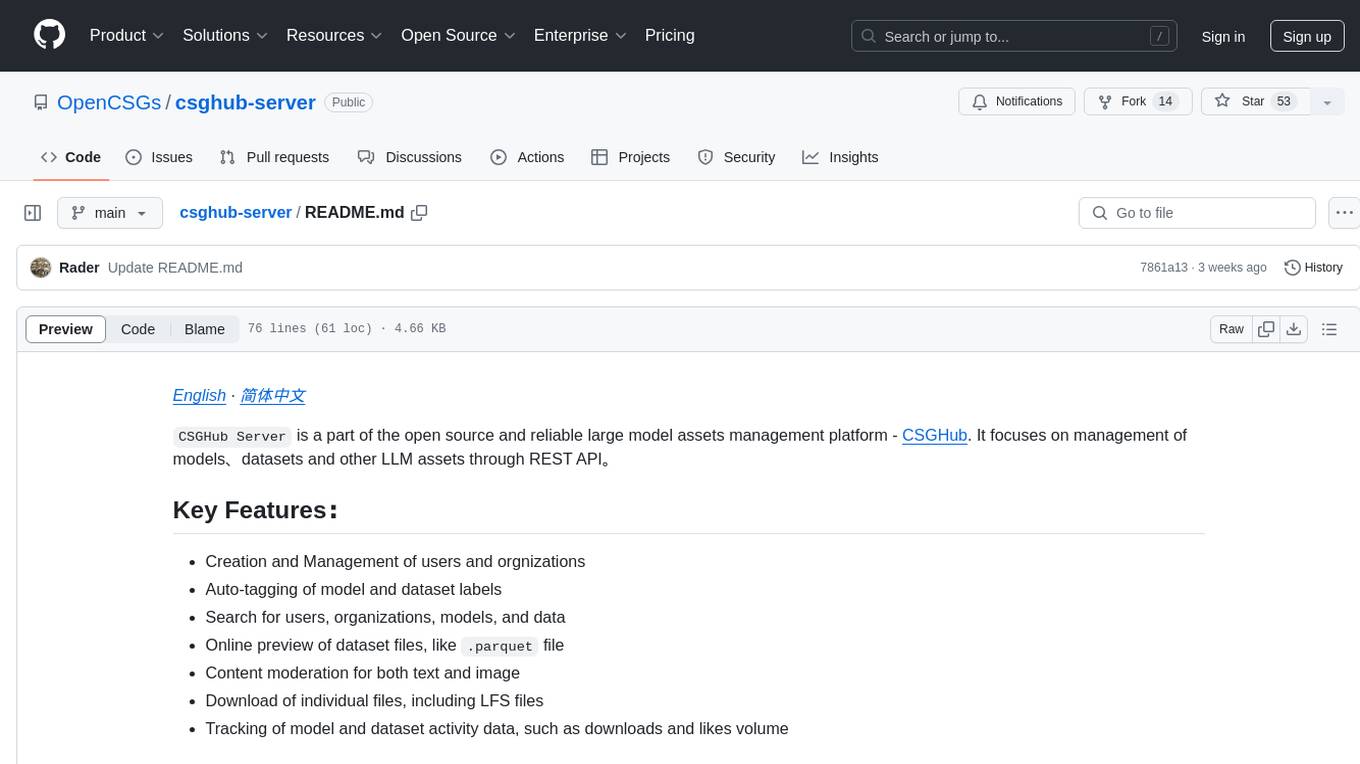
csghub-server
CSGHub Server is a part of the open source and reliable large model assets management platform - CSGHub. It focuses on management of models, datasets, and other LLM assets through REST API. Key features include creation and management of users and organizations, auto-tagging of model and dataset labels, search functionality, online preview of dataset files, content moderation for text and image, download of individual files, tracking of model and dataset activity data. The tool is extensible and customizable, supporting different git servers, flexible LFS storage system configuration, and content moderation options. The roadmap includes support for more Git servers, Git LFS, dataset online viewer, model/dataset auto-tag, S3 protocol support, model format conversion, and model one-click deploy. The project is licensed under Apache 2.0 and welcomes contributions.
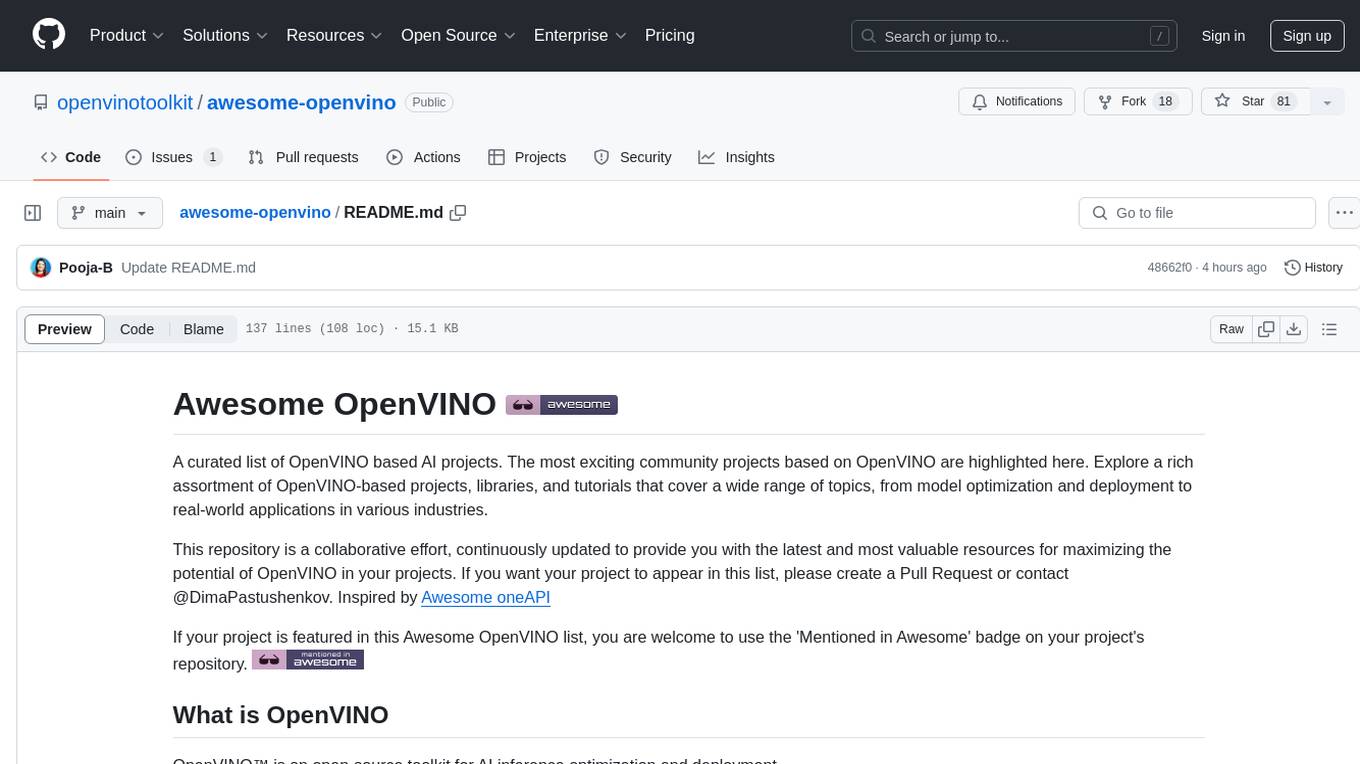
awesome-openvino
Awesome OpenVINO is a curated list of AI projects based on the OpenVINO toolkit, offering a rich assortment of projects, libraries, and tutorials covering various topics like model optimization, deployment, and real-world applications across industries. It serves as a valuable resource continuously updated to maximize the potential of OpenVINO in projects, featuring projects like Stable Diffusion web UI, Visioncom, FastSD CPU, OpenVINO AI Plugins for GIMP, and more.
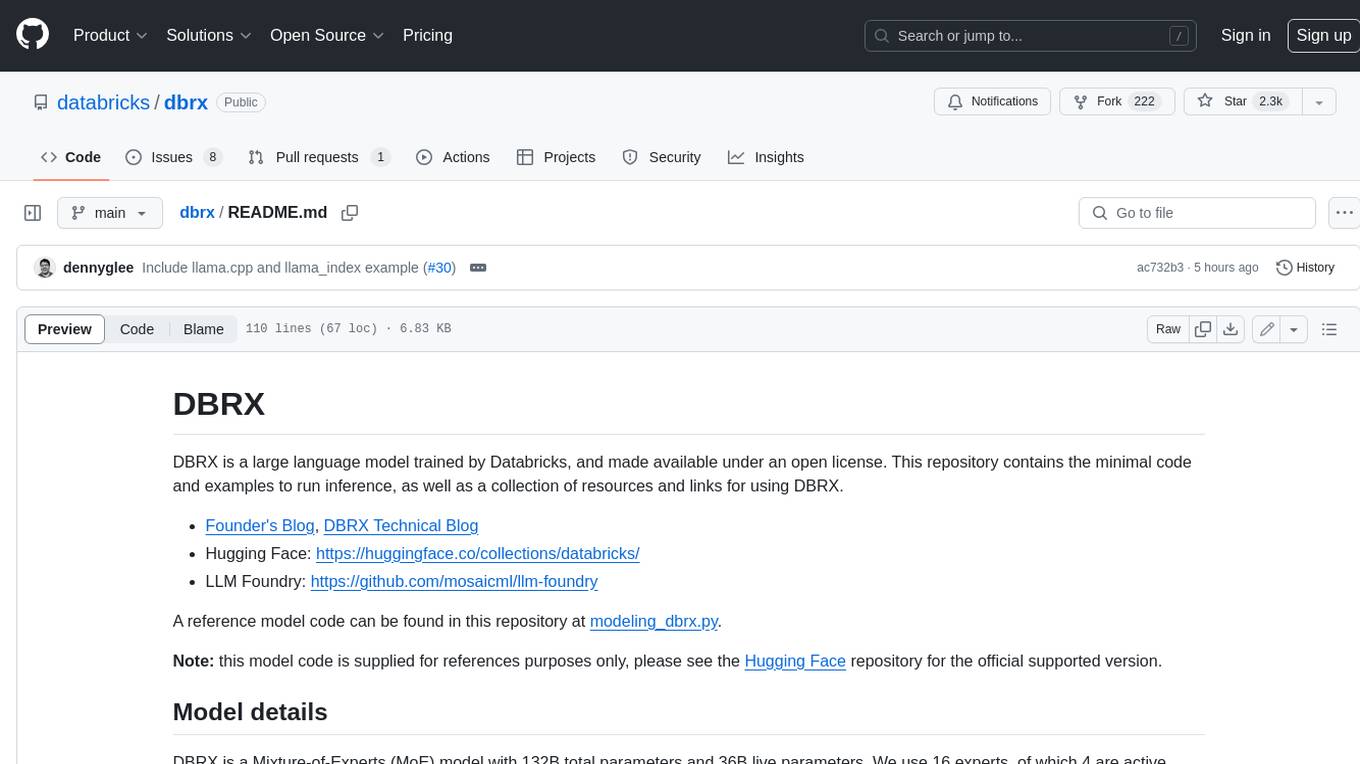
dbrx
DBRX is a large language model trained by Databricks and made available under an open license. It is a Mixture-of-Experts (MoE) model with 132B total parameters and 36B live parameters, using 16 experts, of which 4 are active during training or inference. DBRX was pre-trained for 12T tokens of text and has a context length of 32K tokens. The model is available in two versions: a base model and an Instruct model, which is finetuned for instruction following. DBRX can be used for a variety of tasks, including text generation, question answering, summarization, and translation.
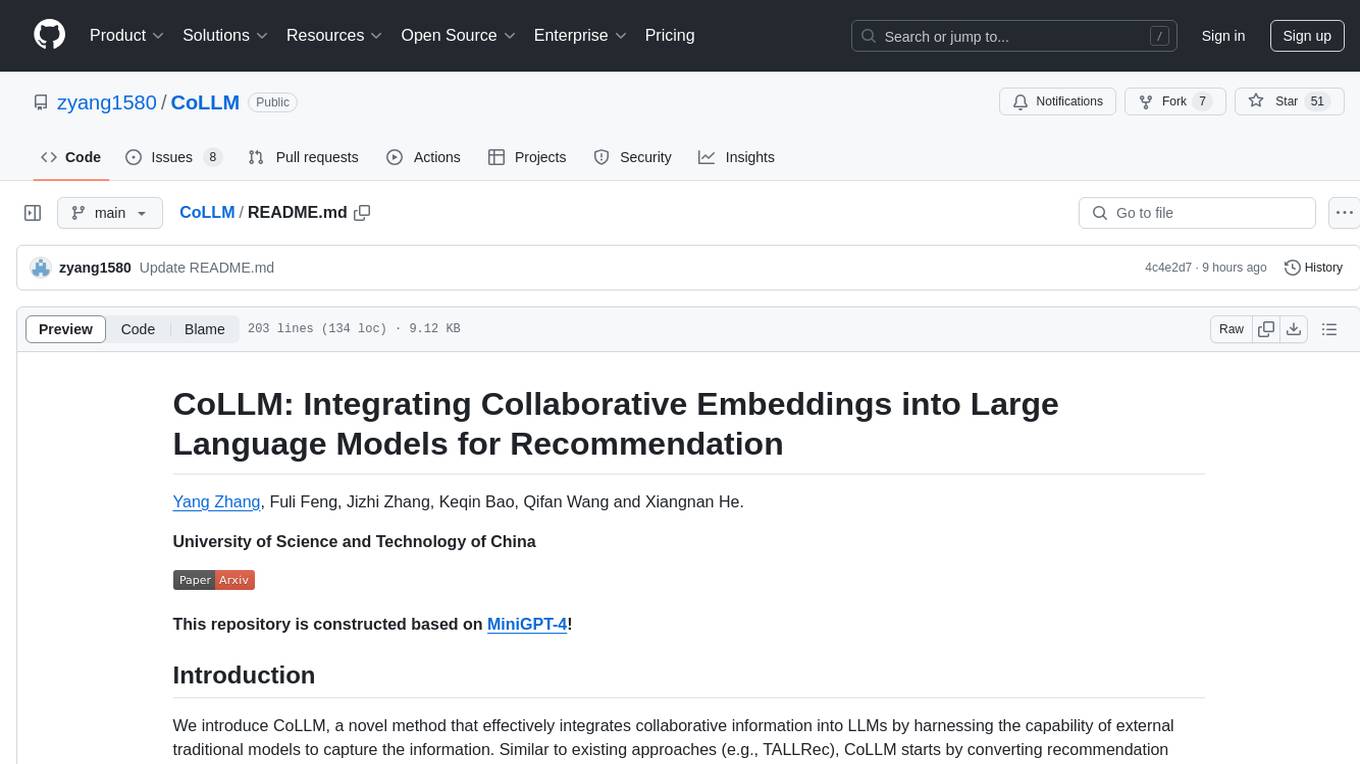
CoLLM
CoLLM is a novel method that integrates collaborative information into Large Language Models (LLMs) for recommendation. It converts recommendation data into language prompts, encodes them with both textual and collaborative information, and uses a two-step tuning method to train the model. The method incorporates user/item ID fields in prompts and employs a conventional collaborative model to generate user/item representations. CoLLM is built upon MiniGPT-4 and utilizes pretrained Vicuna weights for training.
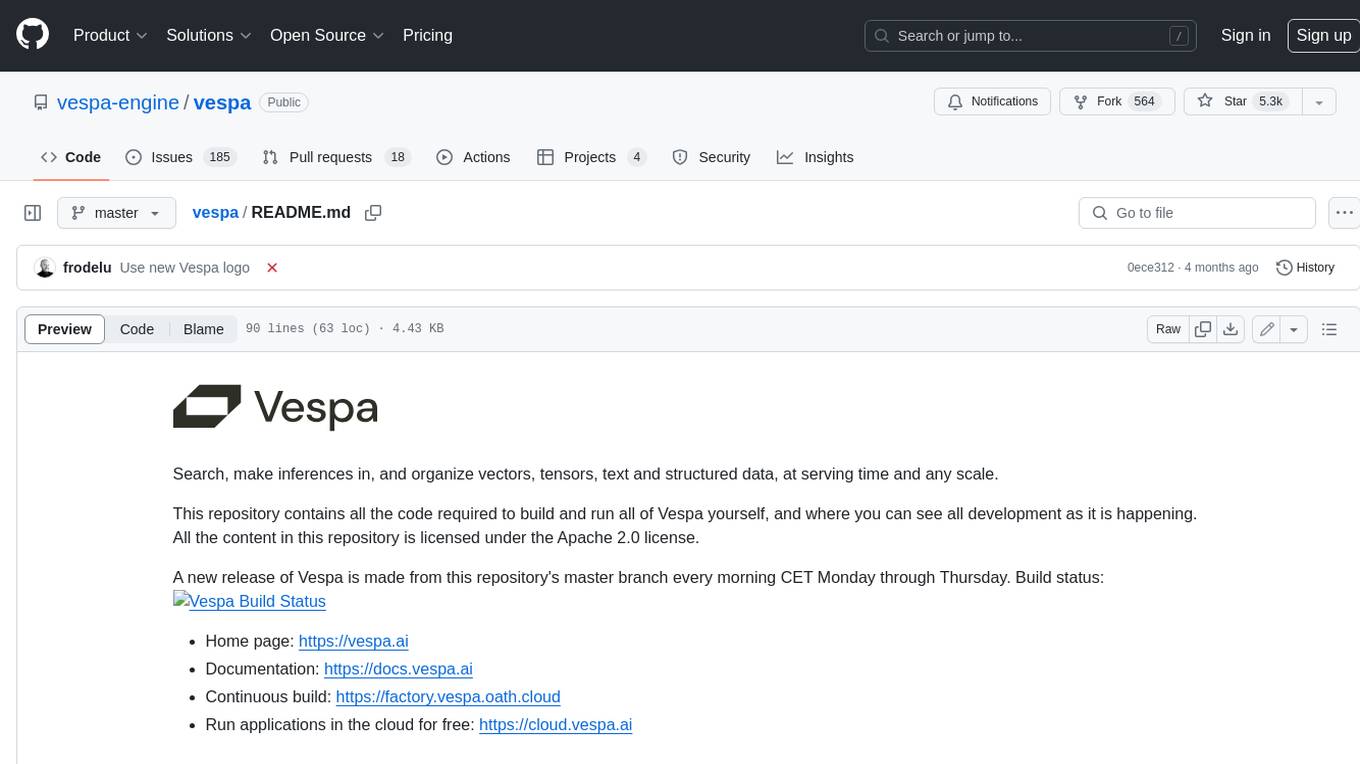
vespa
Vespa is a platform that performs operations such as selecting a subset of data in a large corpus, evaluating machine-learned models over the selected data, organizing and aggregating it, and returning it, typically in less than 100 milliseconds, all while the data corpus is continuously changing. It has been in development for many years and is used on a number of large internet services and apps which serve hundreds of thousands of queries from Vespa per second.
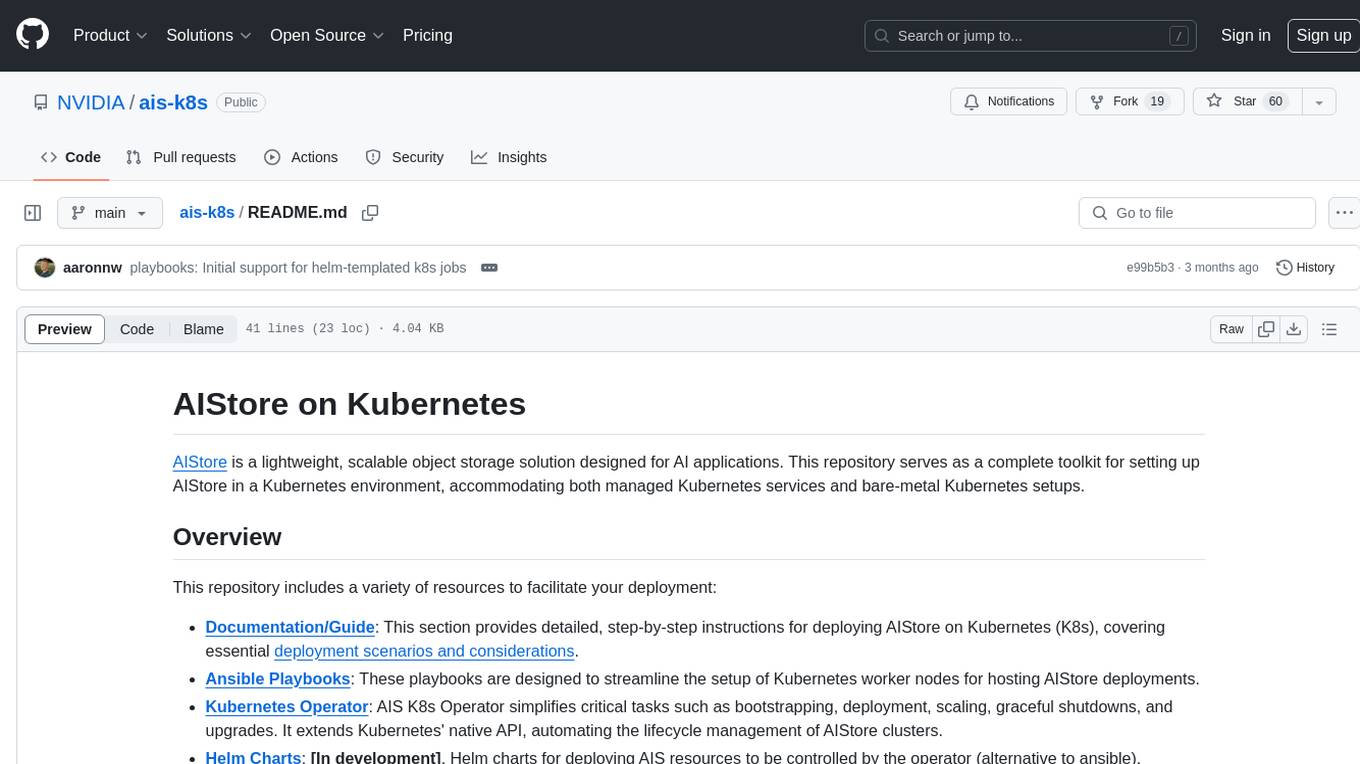
ais-k8s
AIStore on Kubernetes is a toolkit for deploying a lightweight, scalable object storage solution designed for AI applications in a Kubernetes environment. It includes documentation, Ansible playbooks, Kubernetes operator, Helm charts, and Terraform definitions for deployment on public cloud platforms. The system overview shows deployment across nodes with proxy and target pods utilizing Persistent Volumes. The AIStore Operator automates cluster management tasks. The repository focuses on production deployments but offers different deployment options. Thorough planning and configuration decisions are essential for successful multi-node deployment. The AIStore Operator simplifies tasks like starting, deploying, adjusting size, and updating AIStore resources within Kubernetes.
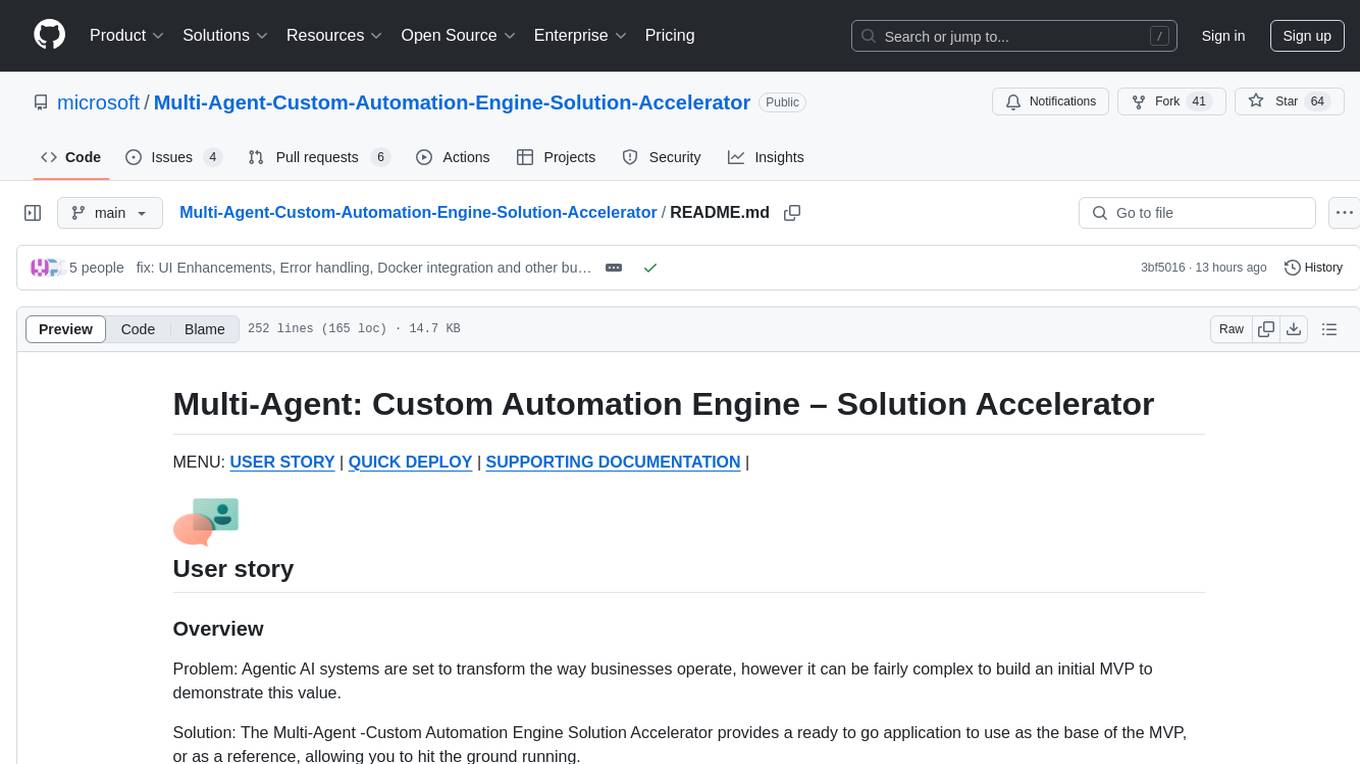
Multi-Agent-Custom-Automation-Engine-Solution-Accelerator
The Multi-Agent -Custom Automation Engine Solution Accelerator is an AI-driven orchestration system that manages a group of AI agents to accomplish tasks based on user input. It uses a FastAPI backend to handle HTTP requests, processes them through various specialized agents, and stores stateful information using Azure Cosmos DB. The system allows users to focus on what matters by coordinating activities across an organization, enabling GenAI to scale, and is applicable to most industries. It is intended for developing and deploying custom AI solutions for specific customers, providing a foundation to accelerate building out multi-agent systems.
For similar tasks
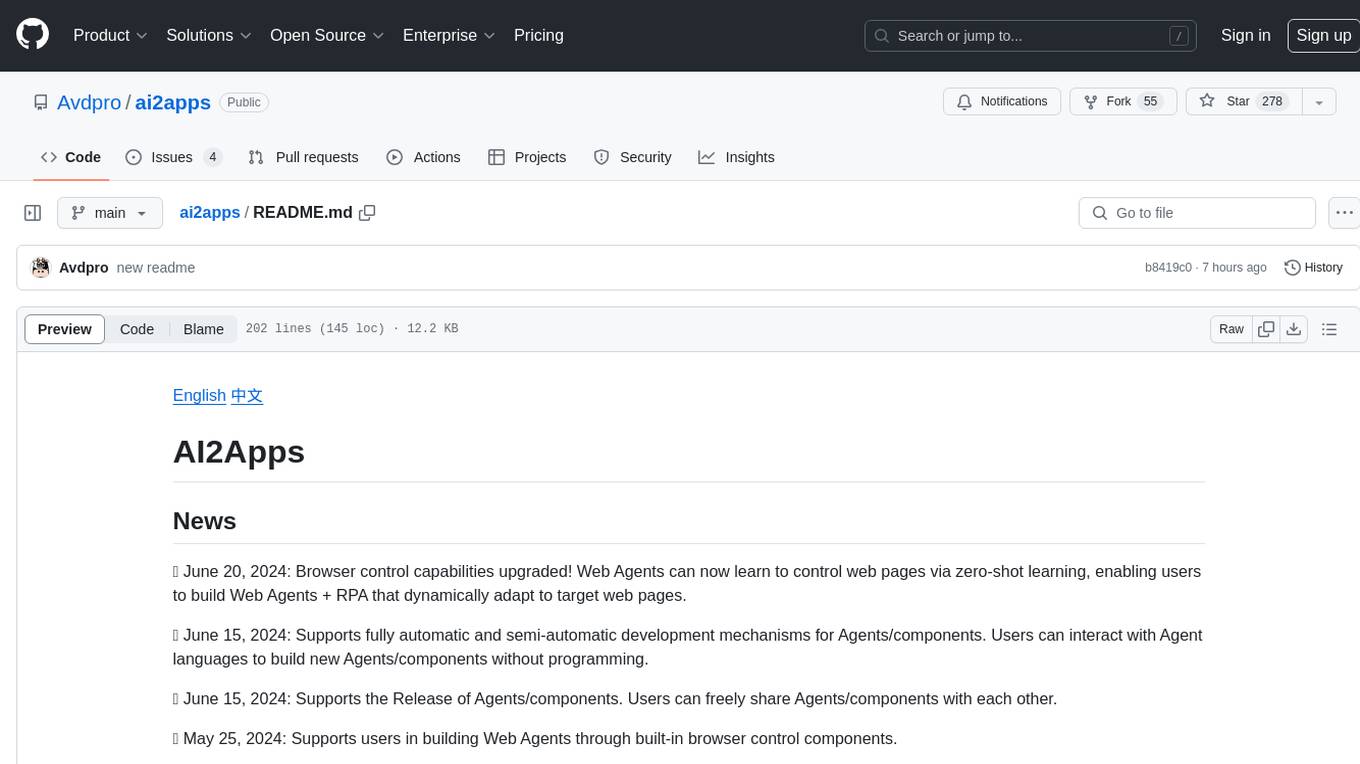
ai2apps
AI2Apps is a visual IDE for building LLM-based AI agent applications, enabling developers to efficiently create AI agents through drag-and-drop, with features like design-to-development for rapid prototyping, direct packaging of agents into apps, powerful debugging capabilities, enhanced user interaction, efficient team collaboration, flexible deployment, multilingual support, simplified product maintenance, and extensibility through plugins.
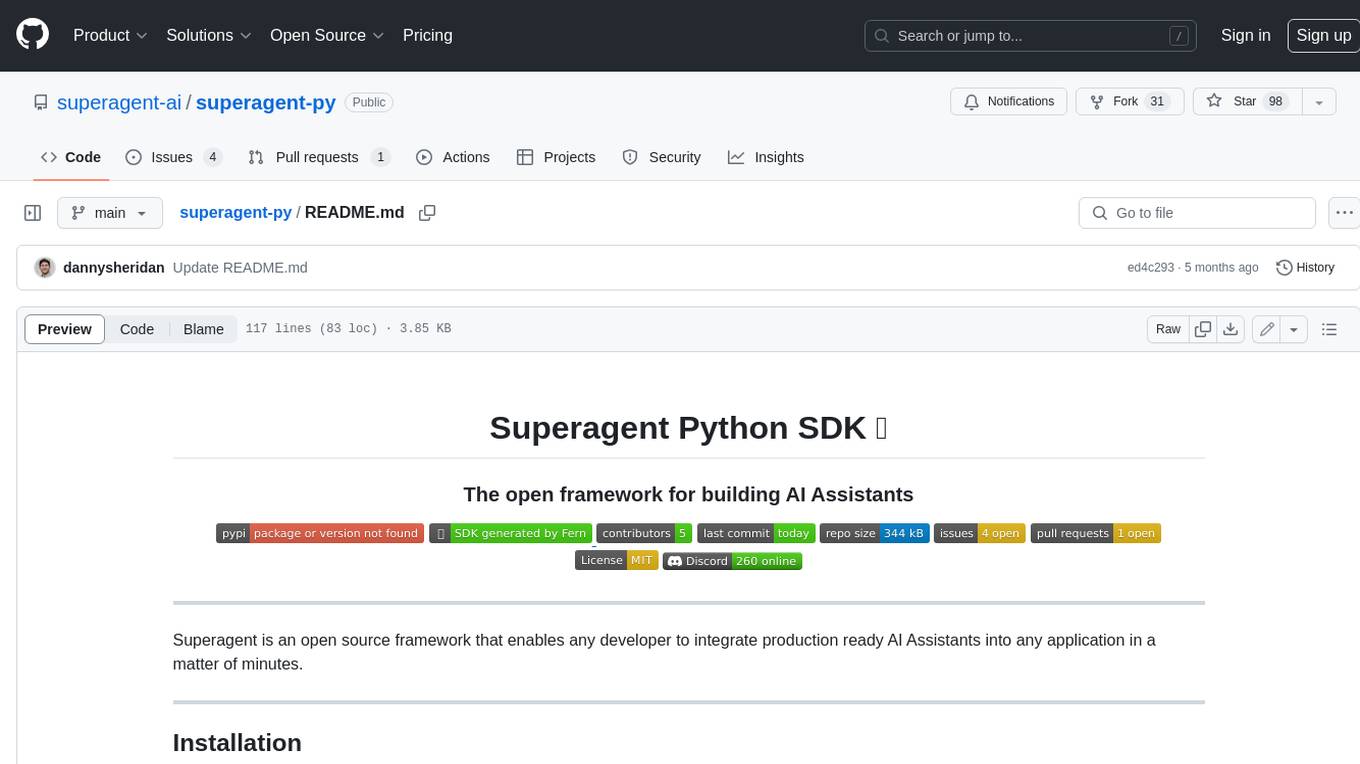
superagent-py
Superagent is an open-source framework that enables developers to integrate production-ready AI assistants into any application quickly and easily. It provides a Python SDK for interacting with the Superagent API, allowing developers to create, manage, and invoke AI agents. The SDK simplifies the process of building AI-powered applications, making it accessible to developers of all skill levels.
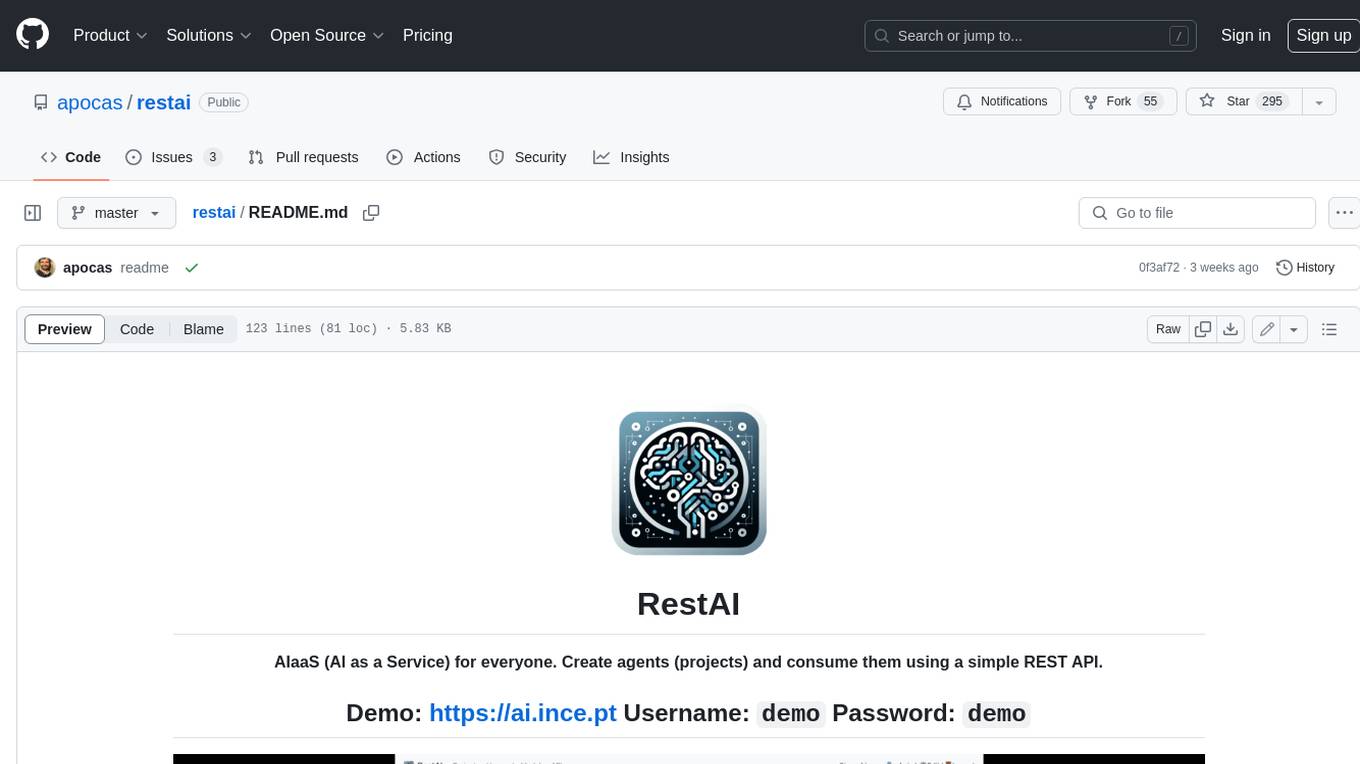
restai
RestAI is an AIaaS (AI as a Service) platform that allows users to create and consume AI agents (projects) using a simple REST API. It supports various types of agents, including RAG (Retrieval-Augmented Generation), RAGSQL (RAG for SQL), inference, vision, and router. RestAI features automatic VRAM management, support for any public LLM supported by LlamaIndex or any local LLM supported by Ollama, a user-friendly API with Swagger documentation, and a frontend for easy access. It also provides evaluation capabilities for RAG agents using deepeval.
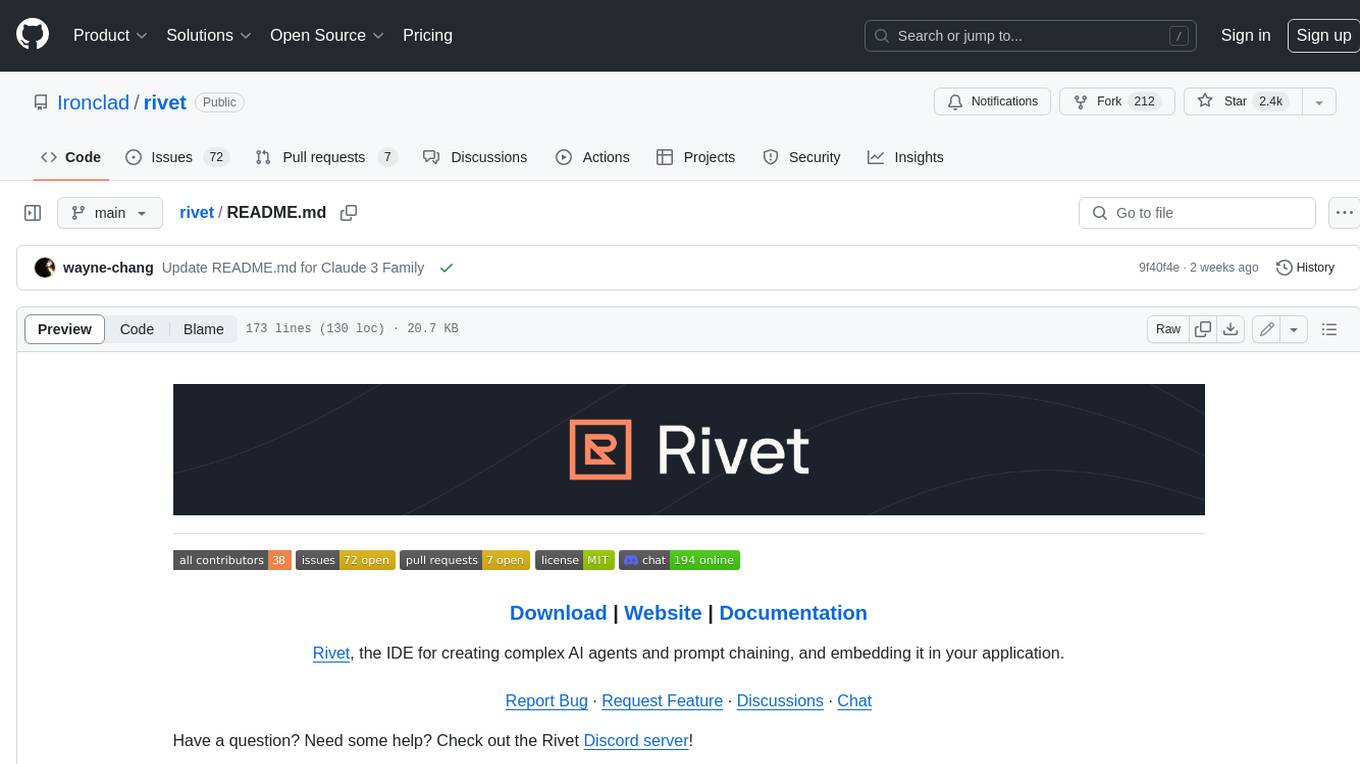
rivet
Rivet is a desktop application for creating complex AI agents and prompt chaining, and embedding it in your application. Rivet currently has LLM support for OpenAI GPT-3.5 and GPT-4, Anthropic Claude Instant and Claude 2, [Anthropic Claude 3 Haiku, Sonnet, and Opus](https://www.anthropic.com/news/claude-3-family), and AssemblyAI LeMUR framework for voice data. Rivet has embedding/vector database support for OpenAI Embeddings and Pinecone. Rivet also supports these additional integrations: Audio Transcription from AssemblyAI. Rivet core is a TypeScript library for running graphs created in Rivet. It is used by the Rivet application, but can also be used in your own applications, so that Rivet can call into your own application's code, and your application can call into Rivet graphs.
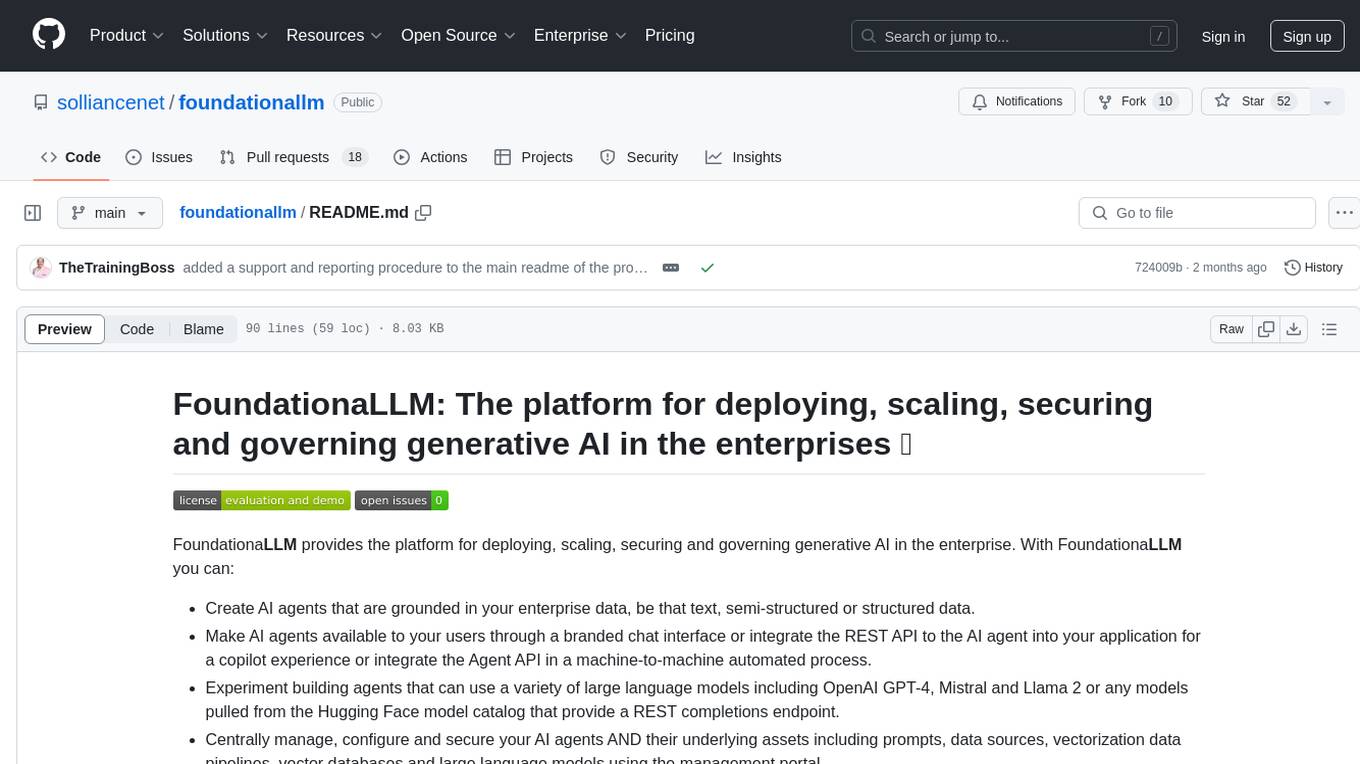
foundationallm
FoundationaLLM is a platform designed for deploying, scaling, securing, and governing generative AI in enterprises. It allows users to create AI agents grounded in enterprise data, integrate REST APIs, experiment with large language models, centrally manage AI agents and assets, deploy scalable vectorization data pipelines, enable non-developer users to create their own AI agents, control access with role-based access controls, and harness capabilities from Azure AI and Azure OpenAI. The platform simplifies integration with enterprise data sources, provides fine-grain security controls, load balances across multiple endpoints, and is extensible to new data sources and orchestrators. FoundationaLLM addresses the need for customized copilots or AI agents that are secure, licensed, flexible, and suitable for enterprise-scale production.
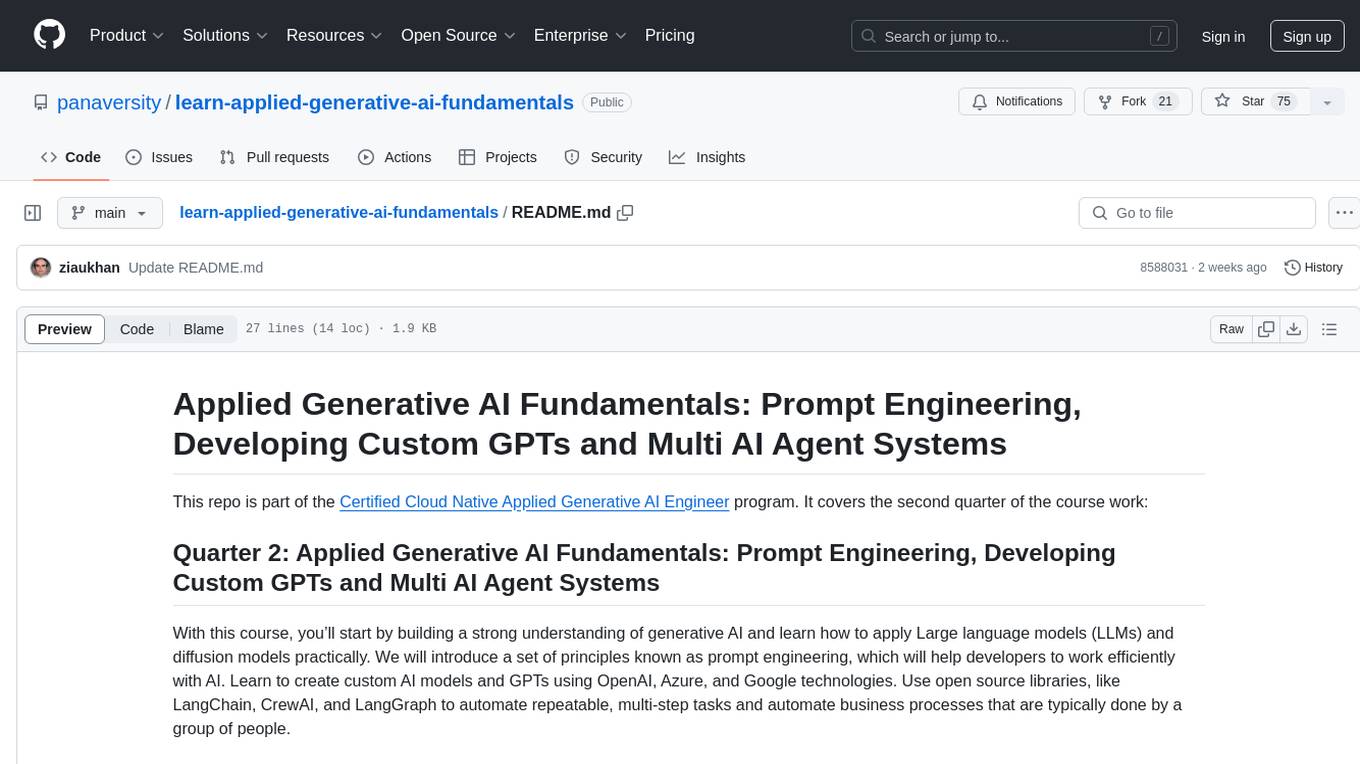
learn-applied-generative-ai-fundamentals
This repository is part of the Certified Cloud Native Applied Generative AI Engineer program, focusing on Applied Generative AI Fundamentals. It covers prompt engineering, developing custom GPTs, and Multi AI Agent Systems. The course helps in building a strong understanding of generative AI, applying Large Language Models (LLMs) and diffusion models practically. It introduces principles of prompt engineering to work efficiently with AI, creating custom AI models and GPTs using OpenAI, Azure, and Google technologies. It also utilizes open source libraries like LangChain, CrewAI, and LangGraph to automate tasks and business processes.
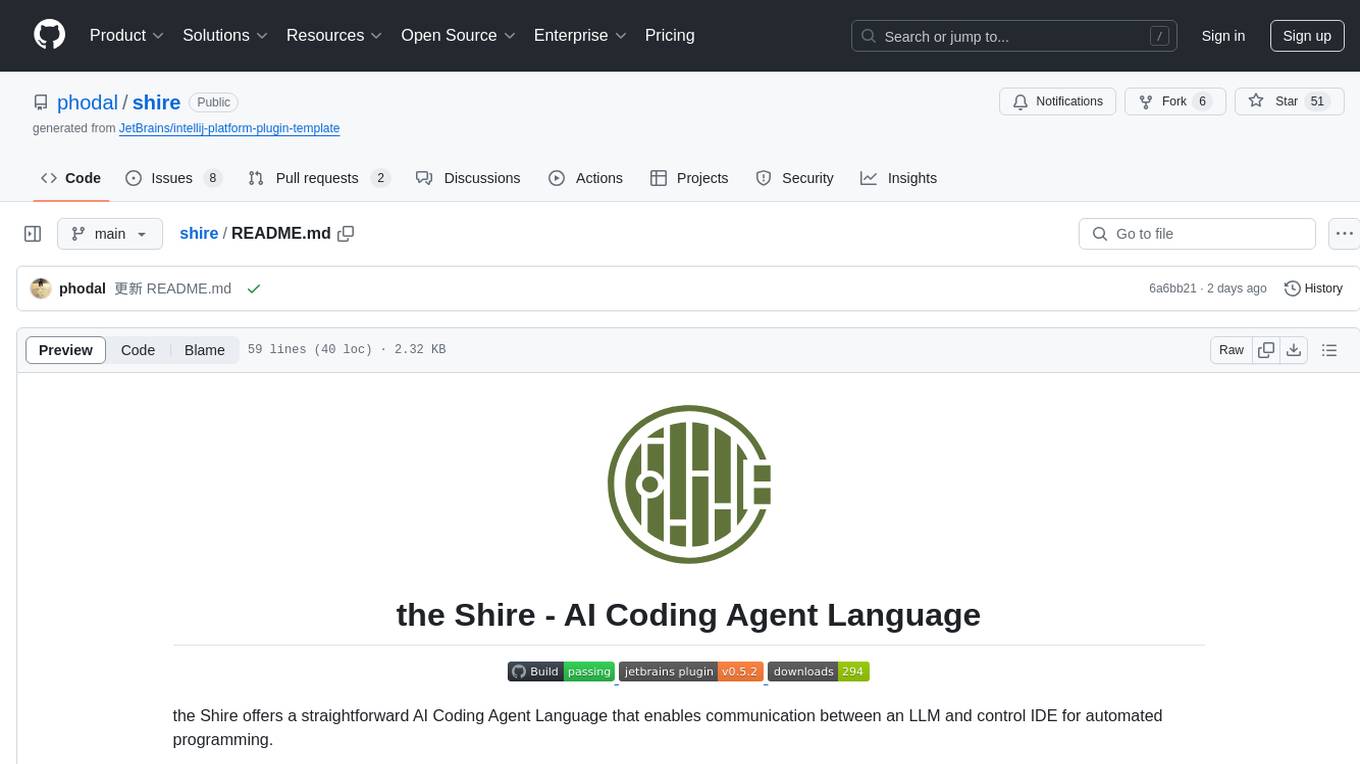
shire
The Shire is an AI Coding Agent Language that facilitates communication between an LLM and control IDE for automated programming. It offers a straightforward approach to creating AI agents tailored to individual IDEs, enabling users to build customized AI-driven development environments. The concept of Shire originated from AutoDev, a subproject of UnitMesh, with DevIns as its precursor. The tool provides documentation and resources for implementing AI in software engineering projects.
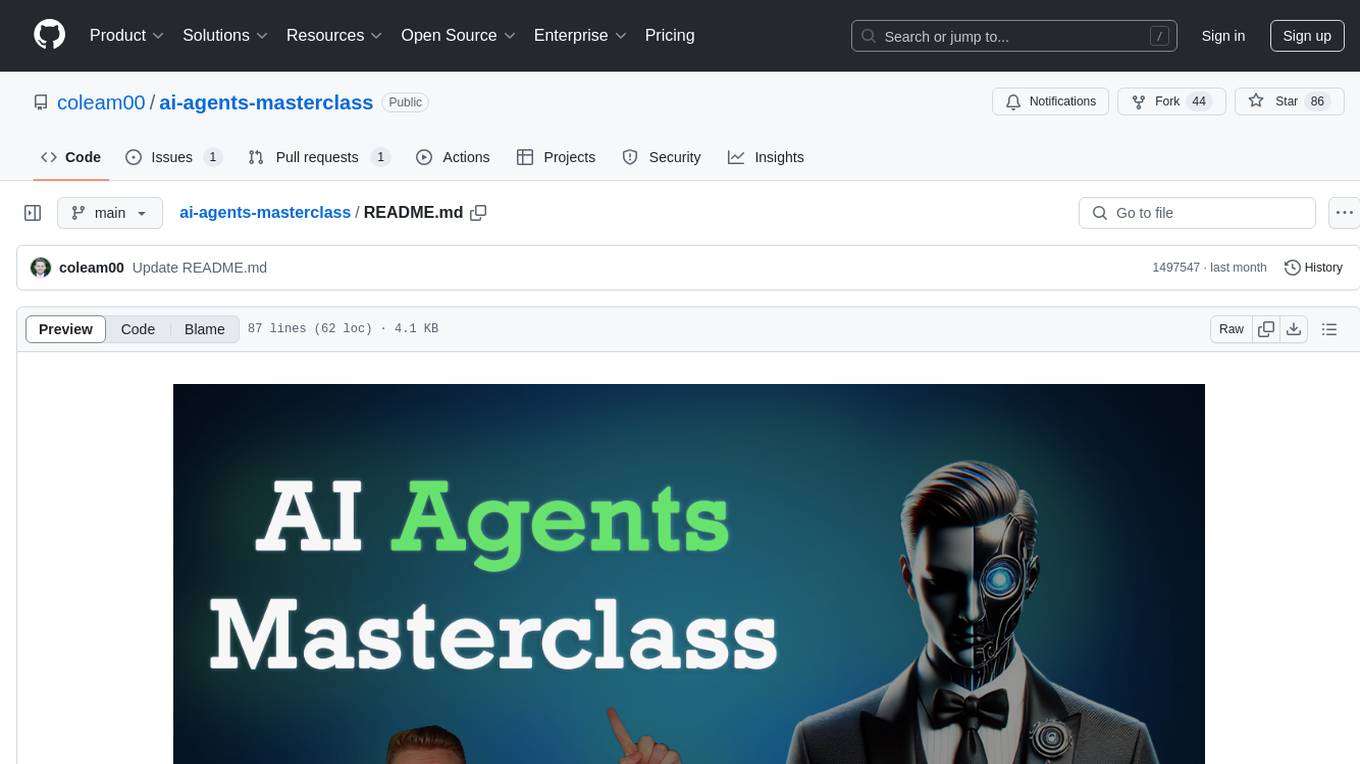
ai-agents-masterclass
AI Agents Masterclass is a repository dedicated to teaching developers how to use AI agents to transform businesses and create powerful software. It provides weekly videos with accompanying code folders, guiding users on setting up Python environments, using environment variables, and installing necessary packages to run the code. The focus is on Large Language Models that can interact with the outside world to perform tasks like drafting emails, booking appointments, and managing tasks, enabling users to create innovative applications with minimal coding effort.
For similar jobs
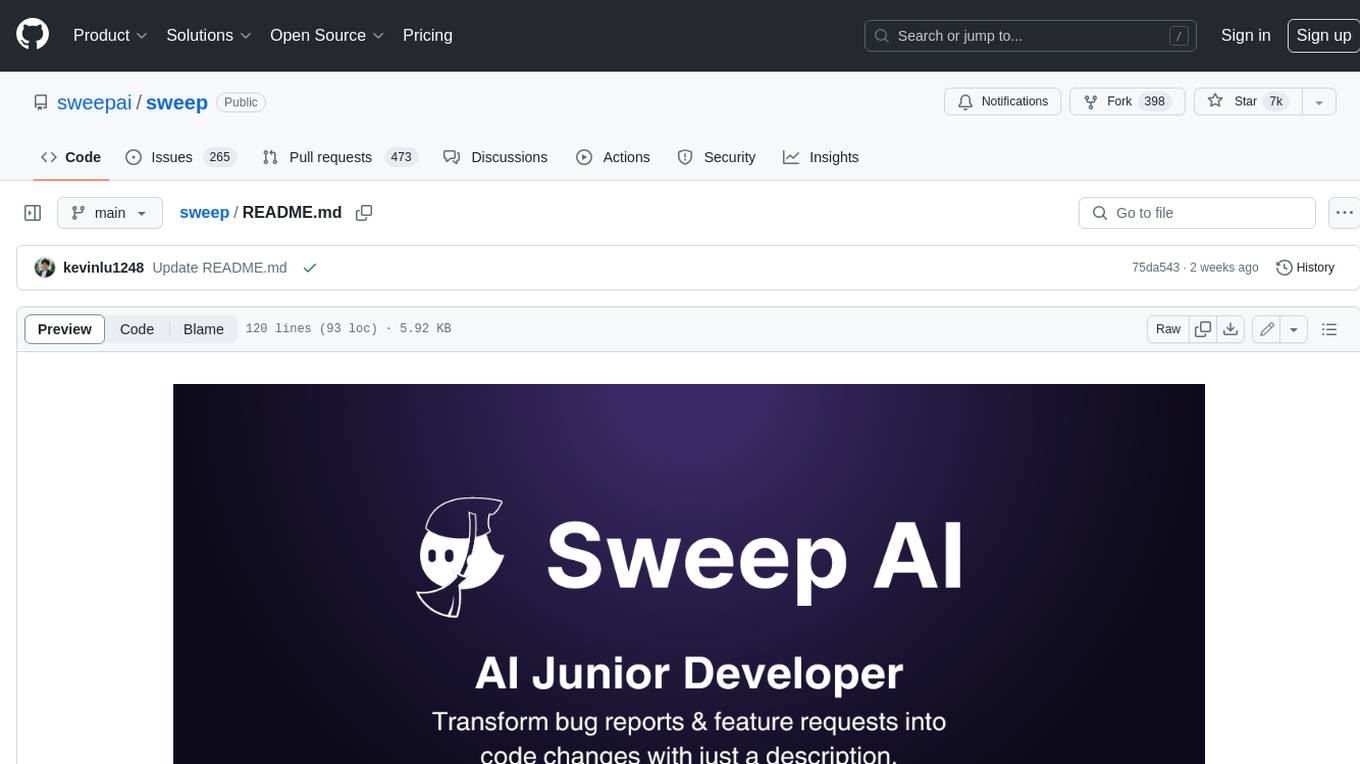
sweep
Sweep is an AI junior developer that turns bugs and feature requests into code changes. It automatically handles developer experience improvements like adding type hints and improving test coverage.
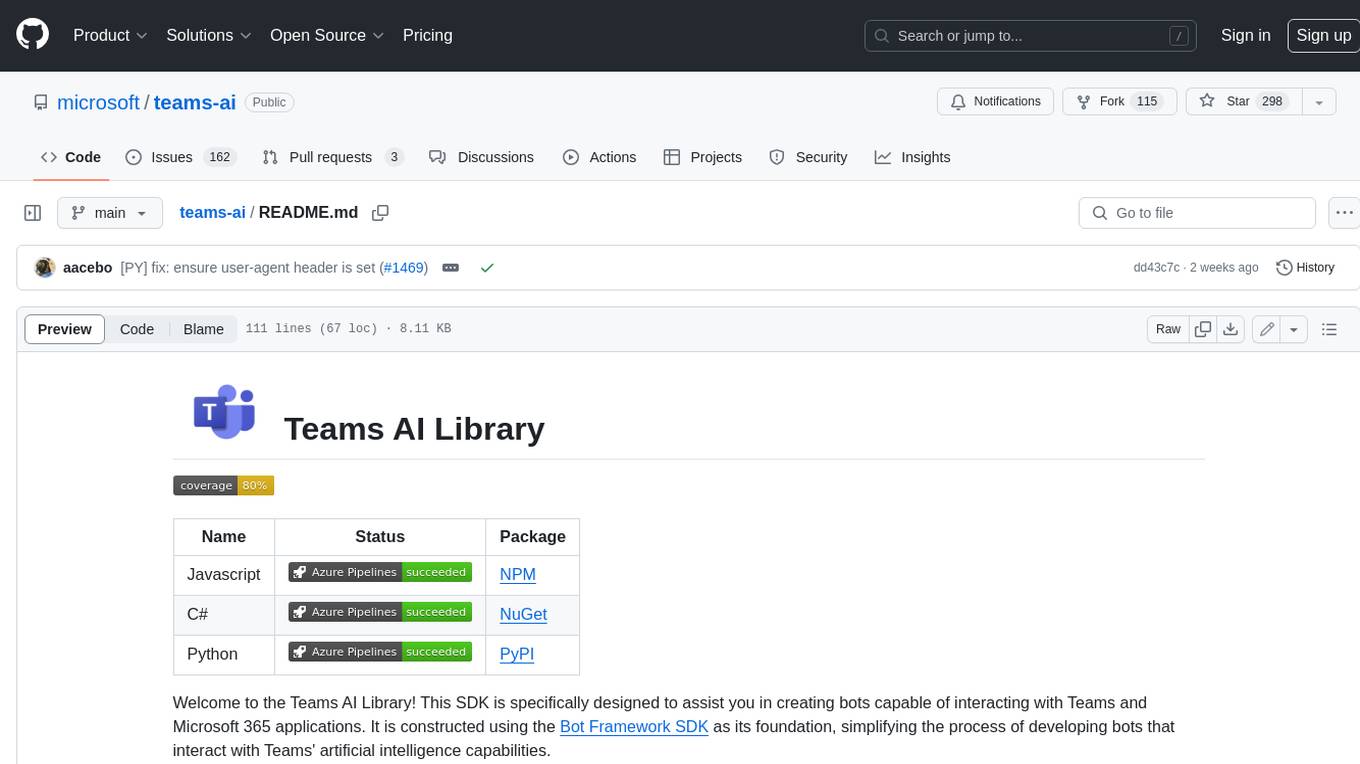
teams-ai
The Teams AI Library is a software development kit (SDK) that helps developers create bots that can interact with Teams and Microsoft 365 applications. It is built on top of the Bot Framework SDK and simplifies the process of developing bots that interact with Teams' artificial intelligence capabilities. The SDK is available for JavaScript/TypeScript, .NET, and Python.
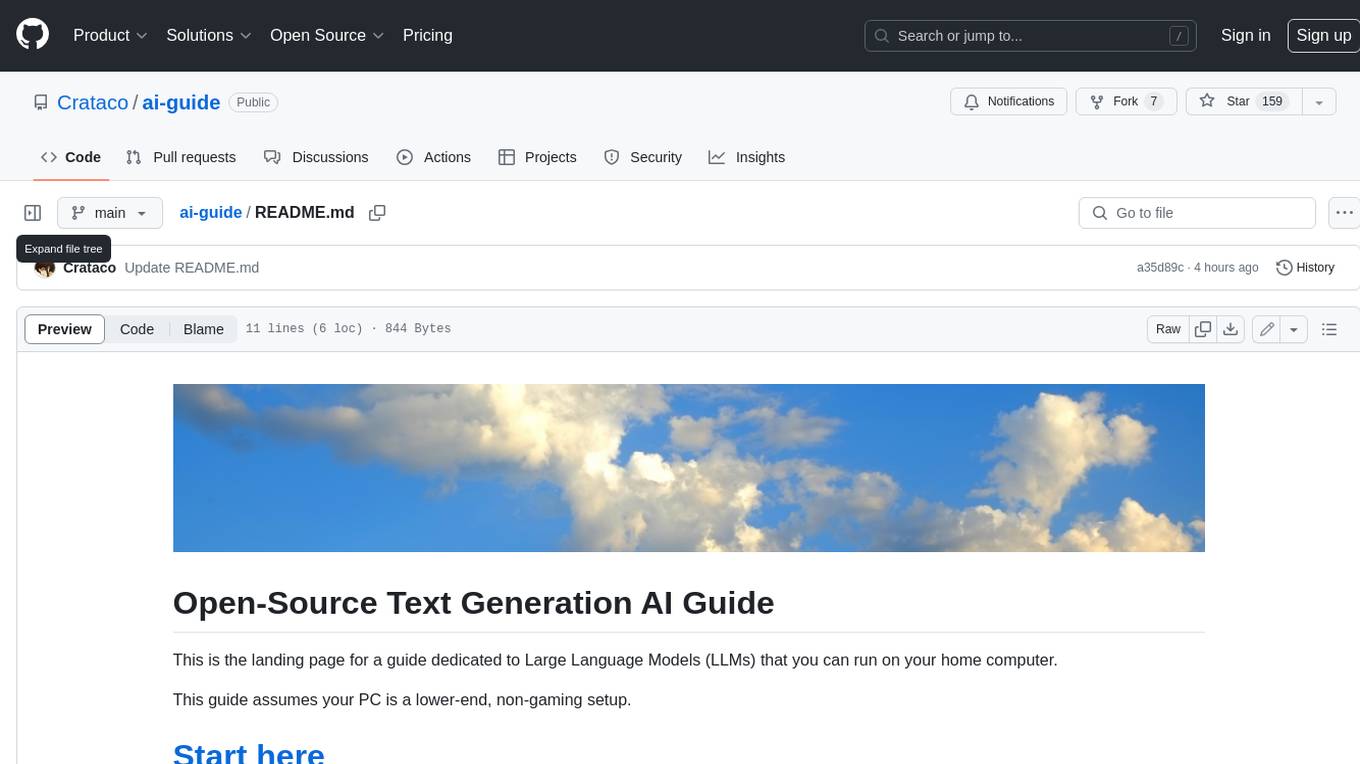
ai-guide
This guide is dedicated to Large Language Models (LLMs) that you can run on your home computer. It assumes your PC is a lower-end, non-gaming setup.
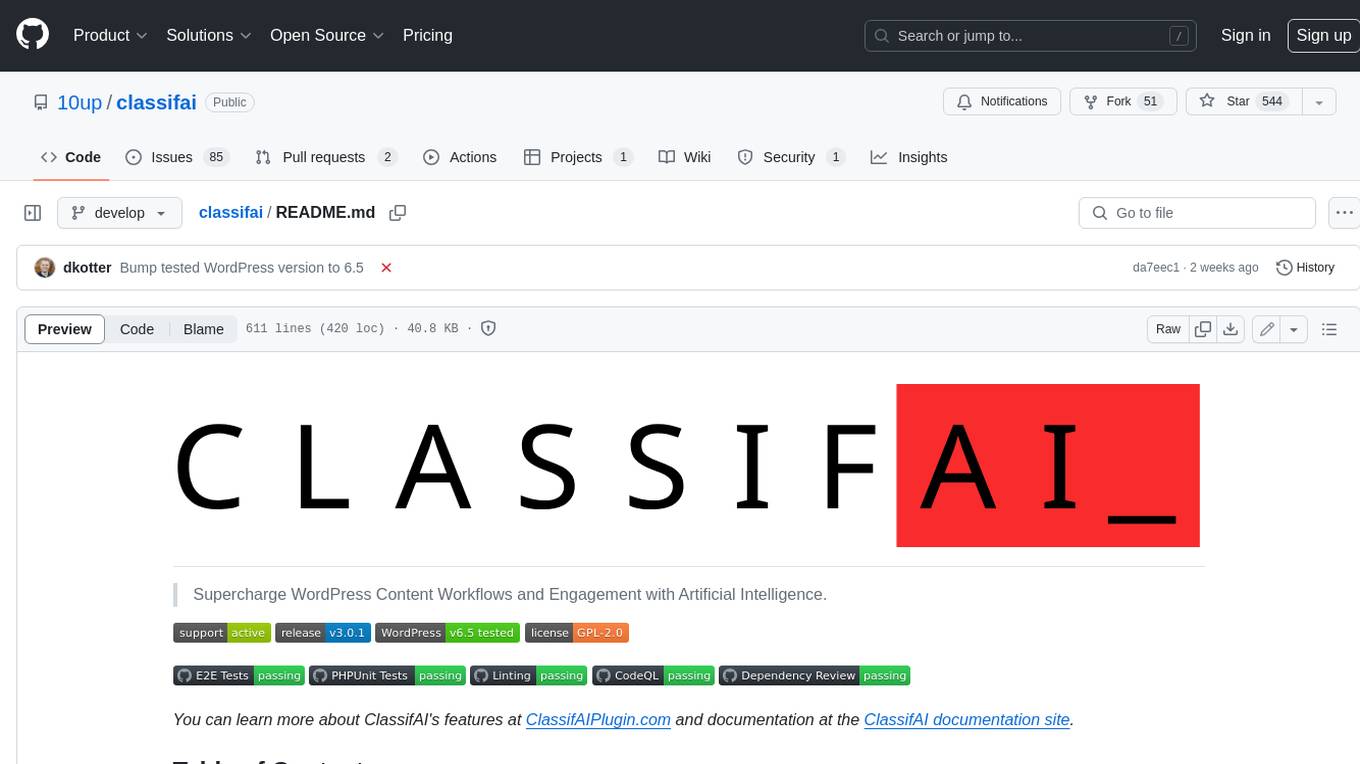
classifai
Supercharge WordPress Content Workflows and Engagement with Artificial Intelligence. Tap into leading cloud-based services like OpenAI, Microsoft Azure AI, Google Gemini and IBM Watson to augment your WordPress-powered websites. Publish content faster while improving SEO performance and increasing audience engagement. ClassifAI integrates Artificial Intelligence and Machine Learning technologies to lighten your workload and eliminate tedious tasks, giving you more time to create original content that matters.
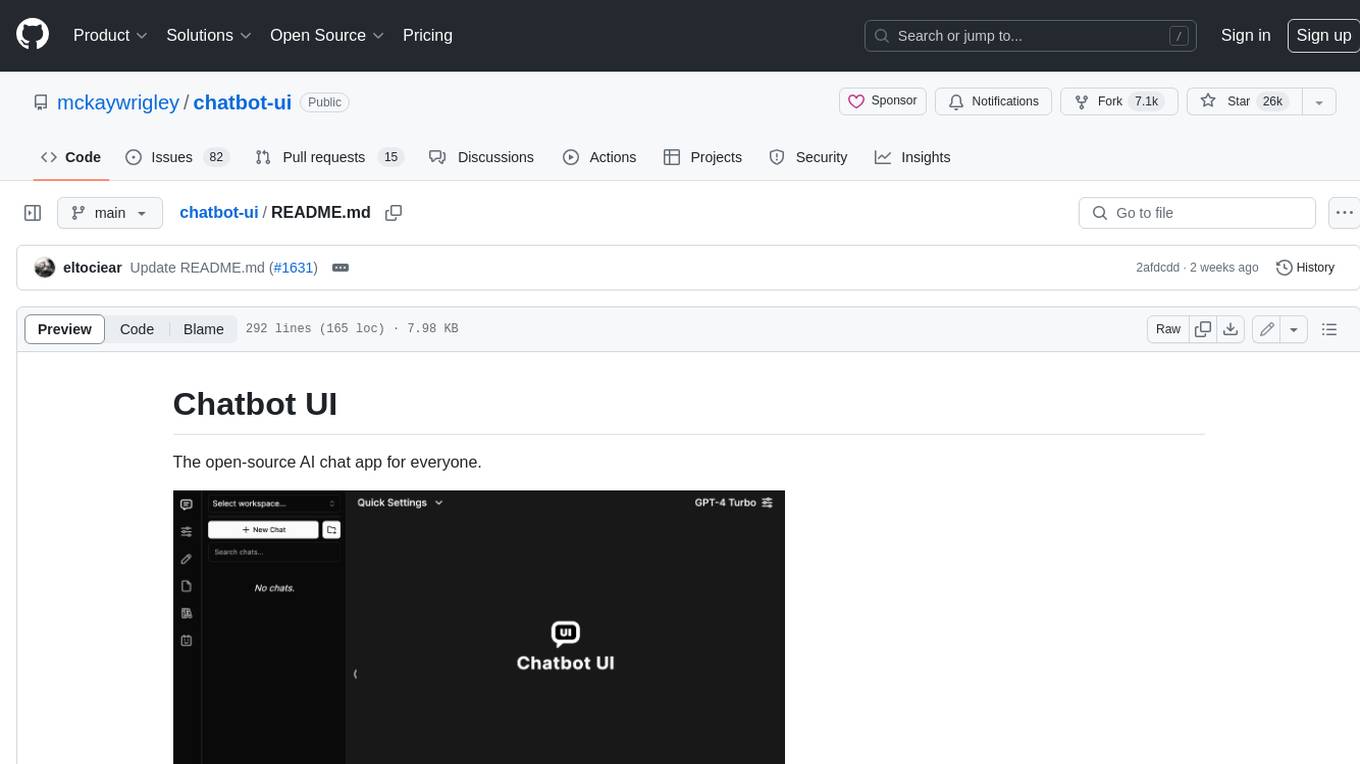
chatbot-ui
Chatbot UI is an open-source AI chat app that allows users to create and deploy their own AI chatbots. It is easy to use and can be customized to fit any need. Chatbot UI is perfect for businesses, developers, and anyone who wants to create a chatbot.
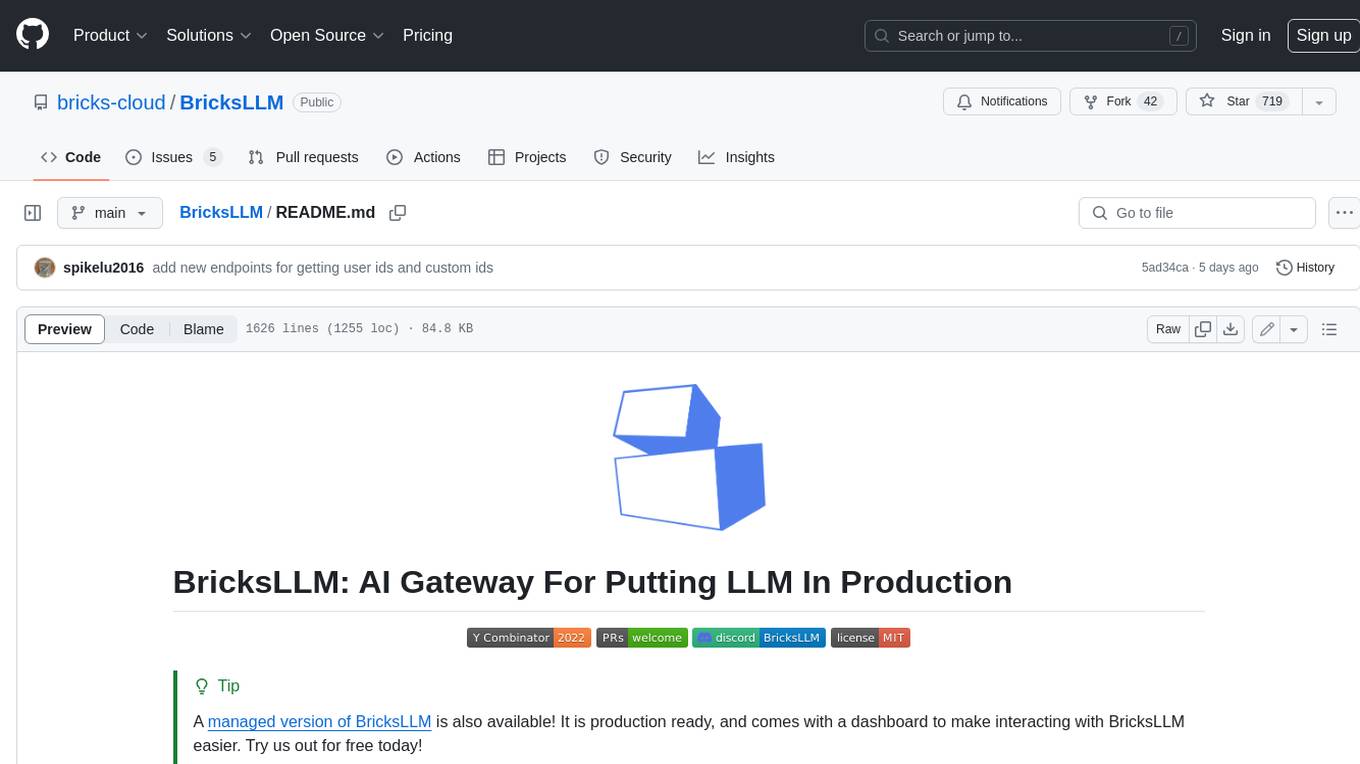
BricksLLM
BricksLLM is a cloud native AI gateway written in Go. Currently, it provides native support for OpenAI, Anthropic, Azure OpenAI and vLLM. BricksLLM aims to provide enterprise level infrastructure that can power any LLM production use cases. Here are some use cases for BricksLLM: * Set LLM usage limits for users on different pricing tiers * Track LLM usage on a per user and per organization basis * Block or redact requests containing PIIs * Improve LLM reliability with failovers, retries and caching * Distribute API keys with rate limits and cost limits for internal development/production use cases * Distribute API keys with rate limits and cost limits for students
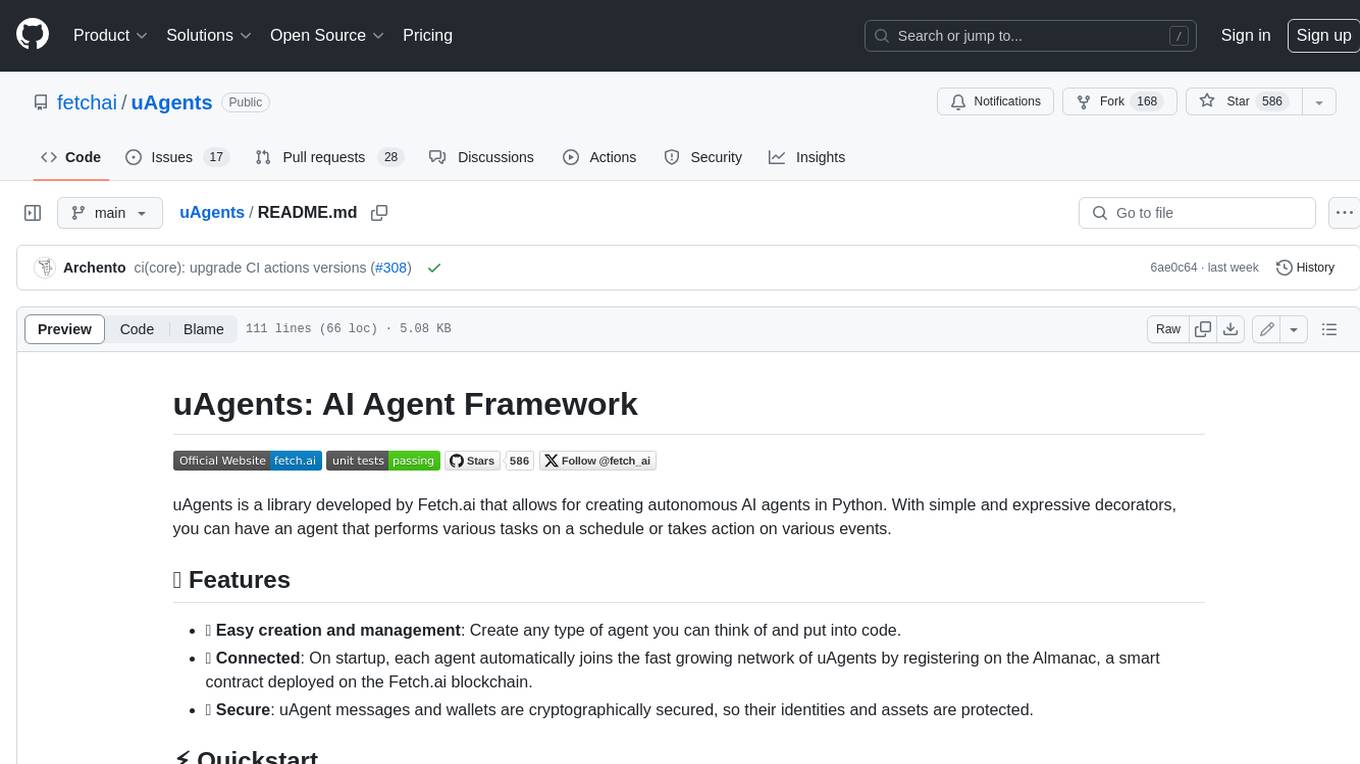
uAgents
uAgents is a Python library developed by Fetch.ai that allows for the creation of autonomous AI agents. These agents can perform various tasks on a schedule or take action on various events. uAgents are easy to create and manage, and they are connected to a fast-growing network of other uAgents. They are also secure, with cryptographically secured messages and wallets.
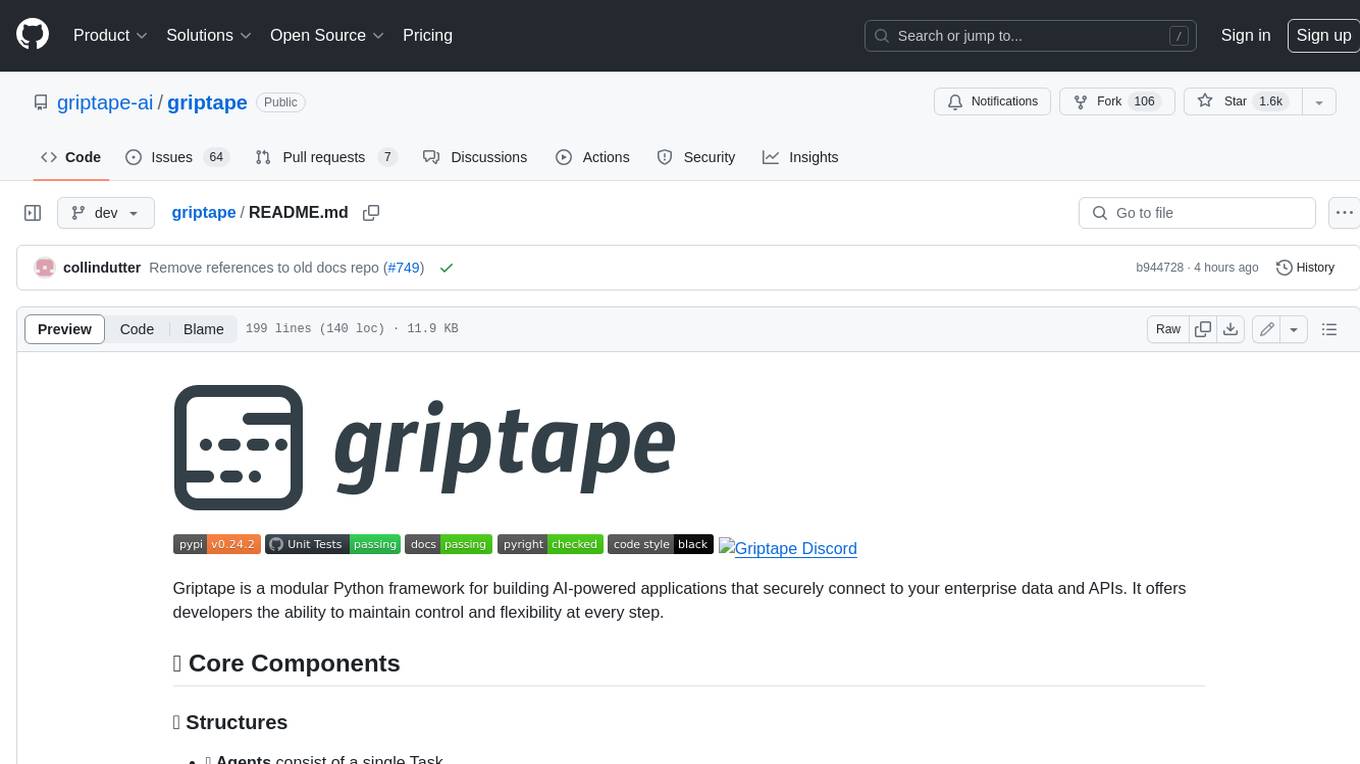
griptape
Griptape is a modular Python framework for building AI-powered applications that securely connect to your enterprise data and APIs. It offers developers the ability to maintain control and flexibility at every step. Griptape's core components include Structures (Agents, Pipelines, and Workflows), Tasks, Tools, Memory (Conversation Memory, Task Memory, and Meta Memory), Drivers (Prompt and Embedding Drivers, Vector Store Drivers, Image Generation Drivers, Image Query Drivers, SQL Drivers, Web Scraper Drivers, and Conversation Memory Drivers), Engines (Query Engines, Extraction Engines, Summary Engines, Image Generation Engines, and Image Query Engines), and additional components (Rulesets, Loaders, Artifacts, Chunkers, and Tokenizers). Griptape enables developers to create AI-powered applications with ease and efficiency.