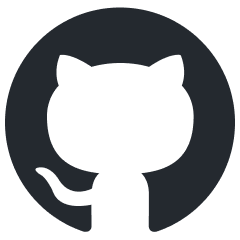
AlignBench
大模型多维度中文对齐评测基准 (ACL 2024)
Stars: 230
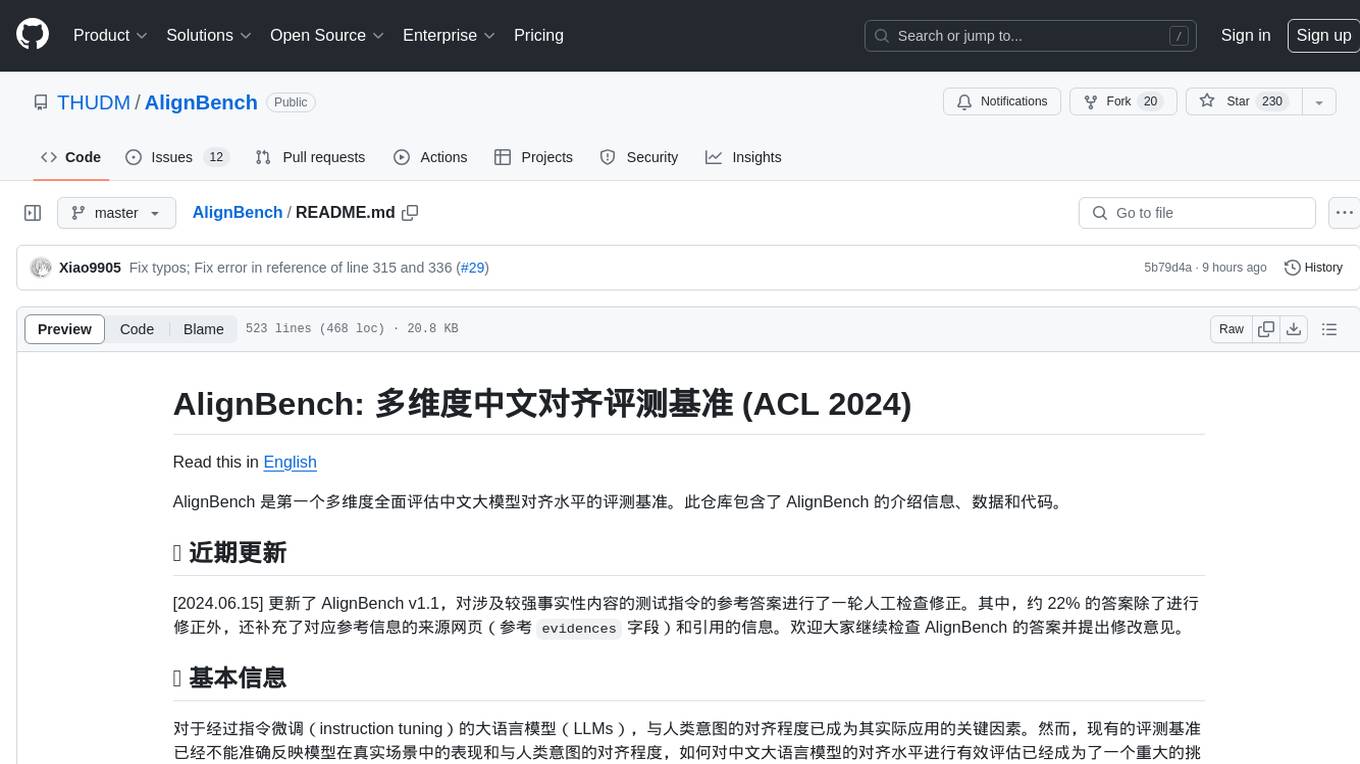
AlignBench is the first comprehensive evaluation benchmark for assessing the alignment level of Chinese large models across multiple dimensions. It includes introduction information, data, and code related to AlignBench. The benchmark aims to evaluate the alignment performance of Chinese large language models through a multi-dimensional and rule-calibrated evaluation method, enhancing reliability and interpretability.
README:
Read this in English
AlignBench 是第一个多维度全面评估中文大模型对齐水平的评测基准。此仓库包含了 AlignBench 的介绍信息、数据和代码。
[2024.06.15] 更新了 AlignBench v1.1,对涉及较强事实性内容的测试指令的参考答案进行了一轮人工检查修正。其中,约 22% 的答案除了进行修正外,还补充了对应参考信息的来源网页(参考 evidences
字段)和引用的信息。欢迎大家继续检查 AlignBench 的答案并提出修改意见。
对于经过指令微调(instruction tuning)的大语言模型(LLMs),与人类意图的对齐程度已成为其实际应用的关键因素。然而,现有的评测基准已经不能准确反映模型在真实场景中的表现和与人类意图的对齐程度,如何对中文大语言模型的对齐水平进行有效评估已经成为了一个重大的挑战。在实际的应用场景中,我们需要采用多样化、开放式、具有挑战性且自动化的评估方法来专门评估模型的对齐水平。
因此,我们构建了 AlignBench,这是一个用于评估中文大语言模型对齐性能的全面、多维度的评测基准。AlignBench 构建了人类参与的数据构建流程,来保证评测数据的动态更新。AlignBench 采用多维度、规则校准的模型评价方法(LLM-as-Judge),并且结合思维链(Chain-of-Thought)生成对模型回复的多维度分析和最终的综合评分,增强了评测的高可靠性和可解释性。
AlignBench 的整体框架如上图所示,包括数据构建流程、体系化的分类以及多维度、规则校准的 LLM-as-Judge 评估方法。
想了解 AlignBench 的更多详细信息,请参阅论文:AlignBench
为了进行系统化的评估,我们根据真实用户指令构建了一个全面的大语言模型(LLMs)能力分类体系。我们分析并总结了用户问题,将其归纳为 8 个主要类别,分别是基本能力、中文理解、综合问答、写作能力、逻辑推理、数学能力、角色扮演和专业知识。AlignBench 的分类体系和数据分布如下表所示。
Category | 中文名 | #Samples |
---|---|---|
Fundamental Language Ability | 基本任务 | 68 |
Advanced Chinese Understanding | 中文理解 | 58 |
Open-ended Questions | 综合问答 | 38 |
Writing Ability | 文本写作 | 75 |
Logical Reasoning | 逻辑推理 | 92 |
Mathematics | 数学计算 | 112 |
Task-oriented Role Play | 角色扮演 | 116 |
Professional Knowledge | 专业能力 | 124 |
为了反映模型在实际应用中的真实表现,AlignBench 中的数据主要来自 ChatGLM 在线服务中真实用户的问题(少部分为研究人员构造的挑战性问题)。AlignBench 总共包含 683 个高质量评测数据。AlignBench 中的每个样本都包含一个任务性的用户指令、一个高质量的参考答案,以及在我们的分类体系中对应的类别。数据保存在data/data_release.jsonl
中,每一行都以json
格式包含一个样本。
数据格式如下所示。
-
question_id
(integer):问题的唯一标识符。 -
category
(string):问题所属的主要类别。 -
subcategory
(string):用于进一步分类的次要类别。 -
question
(string):实际用户查询。 -
reference
(string):这提供了对问题的参考或标准答案。
以下是专业能力
类别的一个例子。
{
"question_id": 8,
"category": "专业能力",
"subcategory": "历史",
"question": "麦哲伦航队在全球旅行时使用了六分仪测量经纬度么?",
"reference": "不,麦哲伦航队在全球旅行时没有使用六分仪来测量经纬度。麦哲伦环球航行的时间是1519年—1522年,六分仪的原理由伊萨克·牛顿提出,而牛顿的出生时间是1643年1月4日,所以再麦哲伦航行的时间六分仪尚未被发明,使用六分仪是不可能的。",
"evidences":
[
{
"url": "https://baike.baidu.com/item/%E6%96%90%E8%BF%AA%E5%8D%97%C2%B7%E9%BA%A6%E5%93%B2%E4%BC%A6/7397066#SnippetTab\n\n",
"quote": "1519年,率领船队开始环球航行。1521年4月27日夜间,麦哲伦在菲律宾死于部落冲突。船队在他死后继续向西航行,回到欧洲,并完成了人类首次环球航行。\n\n"
},
{
"url": "https://baike.baidu.com/item/%E5%85%AD%E5%88%86%E4%BB%AA/749782?fr=ge_ala#3",
"quote": "六分仪的原理由伊萨克·牛顿提出,1732年,英国海军开始将原始仪器安装在船艇上,因为当时最大测量角度是90度,因此被称为八分仪。1757年,约翰·坎贝尔船长将八分仪的测量夹角提高到120度,发展成为六分仪。其后六分仪的测量夹角虽然逐渐提升到144度,但是其名称却一直保持不变。"
}
]
}
为了有效评估响应的质量,AlignBench 目前采用 GPT-4-0613 来分析并随后对响应进行评分。在评估过程中,输入包括用户问题、模型的回复和高质量的参考答案,输出是对模型回复的多维度的分析和最终评分,评分范围从1到10。为了确保可靠性和可解释性,我们实施了以下方法。整个评价流程的示例图如下所示。
-
单点打分: 对于每个模型的回答,评估方法将给出一个从 1 到 10 的最终评分。
-
思维链(Chain-of-Thought): 由于评分任务涉及到复杂的推理过程,我们采用了思维链方法来增强评价的可靠性和可解释性。具体来说,我们会引导评价模型在给出最终评分之前,从多个维度生成对模型回答的分析解释。
-
规则校准: 对于每个问题,我们提供一个高质量的参考答案。为了指导评价模型将模型回答与参考答案进行比较,并生成更加可控的分数,我们提供了详细的评分规则,阐述了分数区间(目前将 1 - 10 五等分)与模型回答的质量之间的关系。这些规则包含在
prompt
中。
-
多维度分析: 由于不同的任务具有不同的性质和特征,对所有任务应用相同的评估流程是不合理的。因此,我们采用多维度的评分方法来全面评估模型回答。具体来说,我们根据不同的问题类型设置了不同的评估维度,并指导评价模型从指定的多个维度分析模型答案并提供单个维度的分数。这些维度及其定义记录在
config
中。
整个评估过程包含三个步骤:获取待评测模型的生成结果、调用评价模型获取分析和打分,最终计算结果。相应的脚本保存在scripts
中,可以修改其中参数之后调用。
-
步骤一 获取待评测模型的生成结果
首先,您需要获得待评测模型的 API 来生成结果,如果是开源模型,您需要自己部署成可以调用获得回复的 API。(此部分不包含在此仓库中)。
其次,在
inference/api_models
中实现您自己的 API 调用类,do_nothing
类可以作为一个示例。(此类主要用于调用 API,注意 API 类名应与文件名相同)第三,修改参数并运行以下脚本以获得待评测模型的生成结果。
MODEL=do_nothing # TODO 修改模型名称(与您的API调用类相同) python get_answers.py \ --model do_nothing \ --workers 2 \ --question-file data/data_v1.1_release.jsonl \ --save-dir data/model_answer
待评测模型的回复将被保存在
data/model_answer
中,以备下一步的评测。 -
步骤二 调用评价模型获取分析和打分
目前我们使用
gpt-4-0613
作为评测模型。首先,在
config/multi-dimension.json
中填写您的 GPT-4 API 密钥。然后,修改并运行以下脚本以获得评价模型的评测结果。
MODEL=do_nothing # TODO 修改模型名称(与您的API调用类相同) python judge.py \ --config-path config/multi-dimension.json \ --model-name $MODEL \ --parallel 2 \
评测结果将保存在
data/judgment
-
步骤三 最终计算结果
运行以下脚本以获取保存在
data/judgment
中的所有模型的最终结果。python show_result.py \ --input-dir data/judgment \ --ques-file data/data_release.jsonl \ --save-file data/results/results.xlsx
计算结果打印出来,同时将以
xlsx
格式存储在data/results
中。
我们在 AlignBench v1.1 上使用 gpt-4-0613
作为打分模型对当前一系列支持汉语的大语言模型(LLMs)进行了系统评测。感谢 清华大学基础模型中心 的 SuperBench 评估团队采用 AlignBench v1.1 作为周期性评估的一部分。后续希望进行评估的模型,可以利用 gpt-4-0613
进行自测评估并汇报,或与 SuperBench 评估团队进行联系。
gpt-4-0613
的评测结果(2024.06更新):
AlignBench v1.1 | Overall | Reasoning 中文推理 | Language 中文语言 | ||||||||
---|---|---|---|---|---|---|---|---|---|---|---|
Avg. | Math. | Logi. | Avg. | Fund. | Chi. | Open. | Writ. | Role. | Pro. | ||
总分 | 推理 总分 |
数学 计算 |
逻辑 推理 |
语言 总分 |
基本 任务 |
中文 理解 |
综合 问答 |
文本 写作 |
角色 扮演 |
专业 能力 |
|
GPT-4o | 8.38 | 8.44 | 8.62 | 8.25 | 8.32 | 8.25 | 7.97 | 8.79 | 7.95 | 8.35 | 8.62 |
通义千问2.5 | 8.17 | 7.79 | 7.97 | 7.60 | 8.55 | 7.87 | 8.40 | 8.94 | 8.60 | 8.73 | 8.76 |
GPT-4 Turbo-20240409 | 8.00 | 8.00 | 8.32 | 7.67 | 8.01 | 7.60 | 7.57 | 8.37 | 7.75 | 8.18 | 8.59 |
Abab 6.5(MoE) | 7.94 | 7.73 | 7.82 | 7.63 | 8.16 | 8.21 | 7.81 | 8.31 | 8.14 | 8.24 | 8.22 |
GLM-4-0520 | 7.89 | 7.66 | 7.67 | 7.64 | 8.13 | 7.78 | 8.22 | 8.21 | 8.09 | 7.99 | 8.47 |
Sensechat 5.0 | 7.89 | 7.54 | 7.96 | 7.12 | 8.23 | 8.27 | 7.69 | 8.45 | 8.15 | 8.53 | 8.29 |
Qwen 1.5-110B-Chat | 7.86 | 7.49 | 7.69 | 7.28 | 8.23 | 7.76 | 8.12 | 8.46 | 8.20 | 8.30 | 8.52 |
文心一言4.0 | 7.85 | 7.81 | 7.60 | 8.02 | 7.89 | 7.33 | 8.35 | 8.16 | 8.11 | 8.07 | 7.29 |
Yi-Large | 7.80 | 7.44 | 7.65 | 7.23 | 8.17 | 7.81 | 7.85 | 8.51 | 7.96 | 8.18 | 8.69 |
DeepSeek-V2 | 7.72 | 7.26 | 7.51 | 7.00 | 8.19 | 8.10 | 7.83 | 8.16 | 8.20 | 8.41 | 8.44 |
GLM-4-Air | 7.58 | 7.20 | 7.19 | 7.20 | 7.97 | 7.53 | 7.71 | 8.18 | 7.97 | 8.10 | 8.32 |
Claude 3 Opus | 7.53 | 7.19 | 7.27 | 7.11 | 7.87 | 7.94 | 7.71 | 8.21 | 7.61 | 7.73 | 8.02 |
Gemini 1.5 Pro | 7.47 | 7.07 | 7.77 | 6.36 | 7.87 | 7.31 | 7.22 | 8.55 | 7.83 | 7.79 | 8.52 |
Baichuan 4 | 7.45 | 7.28 | 7.34 | 7.22 | 7.63 | 7.34 | 7.40 | 7.74 | 7.60 | 7.36 | 8.33 |
Llama 3-70B | 7.42 | 7.02 | 7.18 | 6.86 | 7.82 | 7.75 | 6.63 | 8.65 | 7.80 | 8.02 | 8.08 |
Gemini 1.5 Flash | 7.38 | 7.29 | 7.96 | 6.61 | 7.47 | 6.75 | 7.16 | 8.05 | 6.96 | 7.73 | 8.16 |
WizardLM-2-8x22B | 7.34 | 6.99 | 6.99 | 6.98 | 7.70 | 7.57 | 6.60 | 8.40 | 7.60 | 8.17 | 7.83 |
moonshot-v1-8k | 7.31 | 6.76 | 6.94 | 6.58 | 7.86 | 7.56 | 7.83 | 7.82 | 7.76 | 7.93 | 8.25 |
Step-1-32k | 7.08 | 6.43 | 6.77 | 6.09 | 7.72 | 8.09 | 7.74 | 7.34 | 7.56 | 7.74 | 7.86 |
讯飞星火3.5 | 6.90 | 6.47 | 7.30 | 5.63 | 7.33 | 7.28 | 7.71 | 7.24 | 7.12 | 7.41 | 7.24 |
Claude 3 Sonnet | 6.71 | 6.17 | 6.24 | 6.10 | 7.25 | 7.56 | 6.39 | 7.37 | 7.14 | 7.76 | 7.26 |
Mixtral-8x22B (MoE) | 6.48 | 6.23 | 6.47 | 5.98 | 6.73 | 6.87 | 5.72 | 7.00 | 6.61 | 7.14 | 7.01 |
Claude 3 Haiku | 6.38 | 5.58 | 6.06 | 5.10 | 7.18 | 7.15 | 6.74 | 7.58 | 6.95 | 7.26 | 7.37 |
@misc{liu2023alignbench,
title={AlignBench: Benchmarking Chinese Alignment of Large Language Models},
author={Xiao Liu and Xuanyu Lei and Shengyuan Wang and Yue Huang and Zhuoer Feng and Bosi Wen and Jiale Cheng and Pei Ke and Yifan Xu and Weng Lam Tam and Xiaohan Zhang and Lichao Sun and Hongning Wang and Jing Zhang and Minlie Huang and Yuxiao Dong and Jie Tang},
year={2023},
eprint={2311.18743},
archivePrefix={arXiv},
primaryClass={cs.CL}
}
For Tasks:
Click tags to check more tools for each tasksFor Jobs:
Alternative AI tools for AlignBench
Similar Open Source Tools
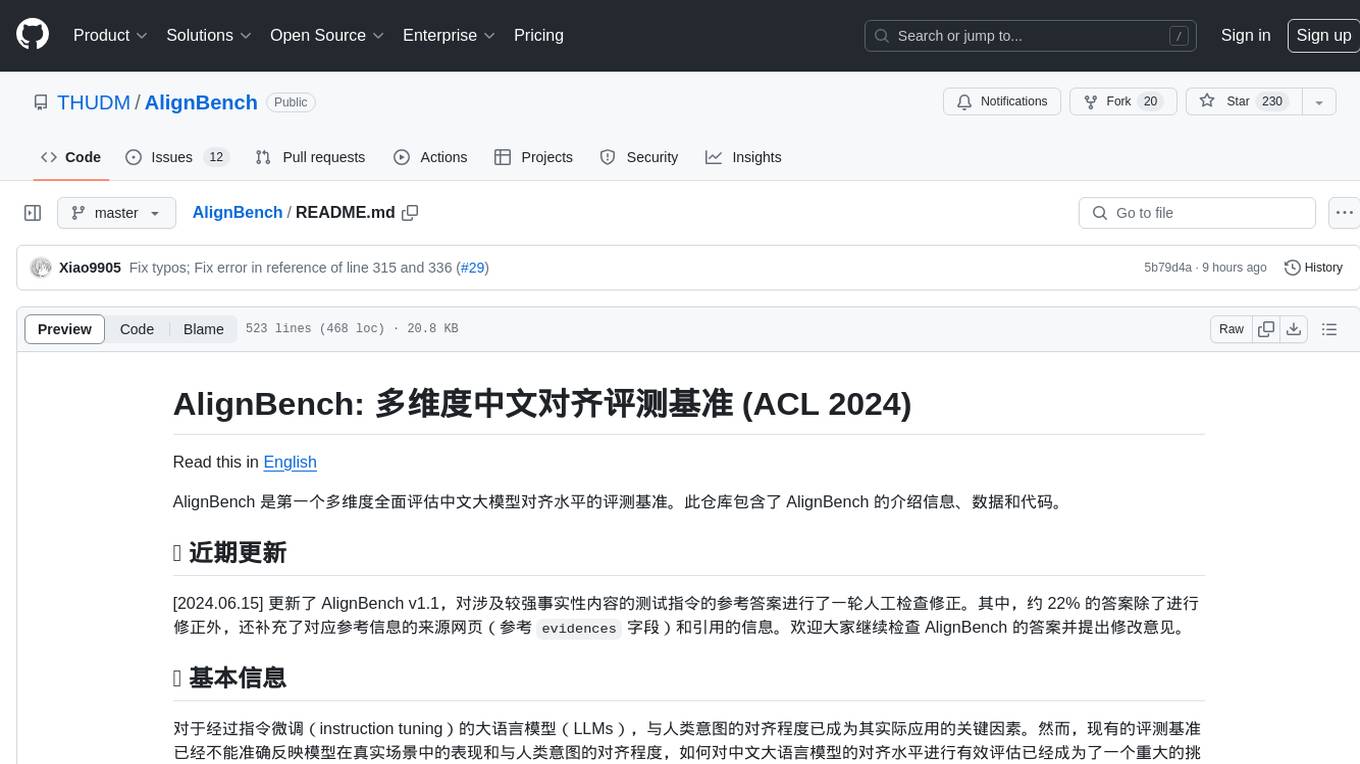
AlignBench
AlignBench is the first comprehensive evaluation benchmark for assessing the alignment level of Chinese large models across multiple dimensions. It includes introduction information, data, and code related to AlignBench. The benchmark aims to evaluate the alignment performance of Chinese large language models through a multi-dimensional and rule-calibrated evaluation method, enhancing reliability and interpretability.
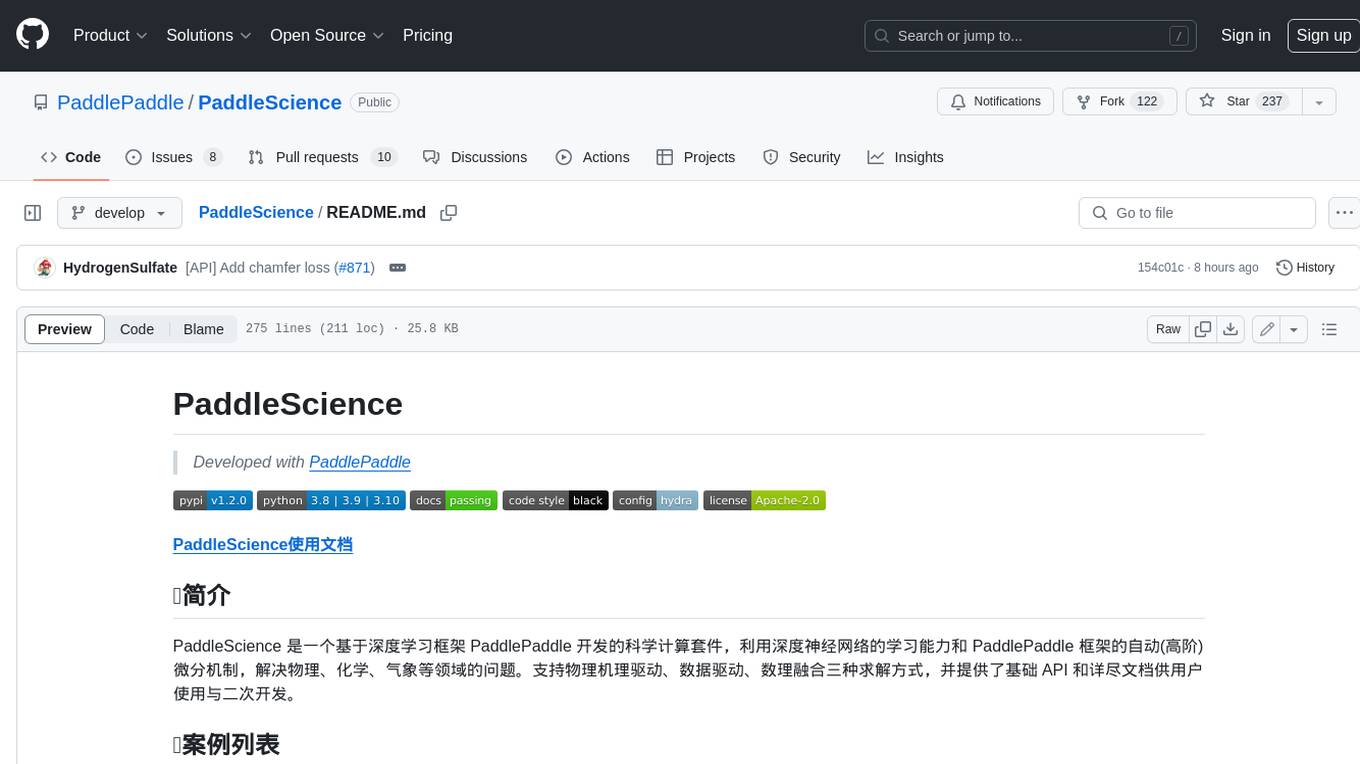
PaddleScience
PaddleScience is a scientific computing suite developed based on the deep learning framework PaddlePaddle. It utilizes the learning ability of deep neural networks and the automatic (higher-order) differentiation mechanism of PaddlePaddle to solve problems in physics, chemistry, meteorology, and other fields. It supports three solving methods: physics mechanism-driven, data-driven, and mathematical fusion, and provides basic APIs and detailed documentation for users to use and further develop.
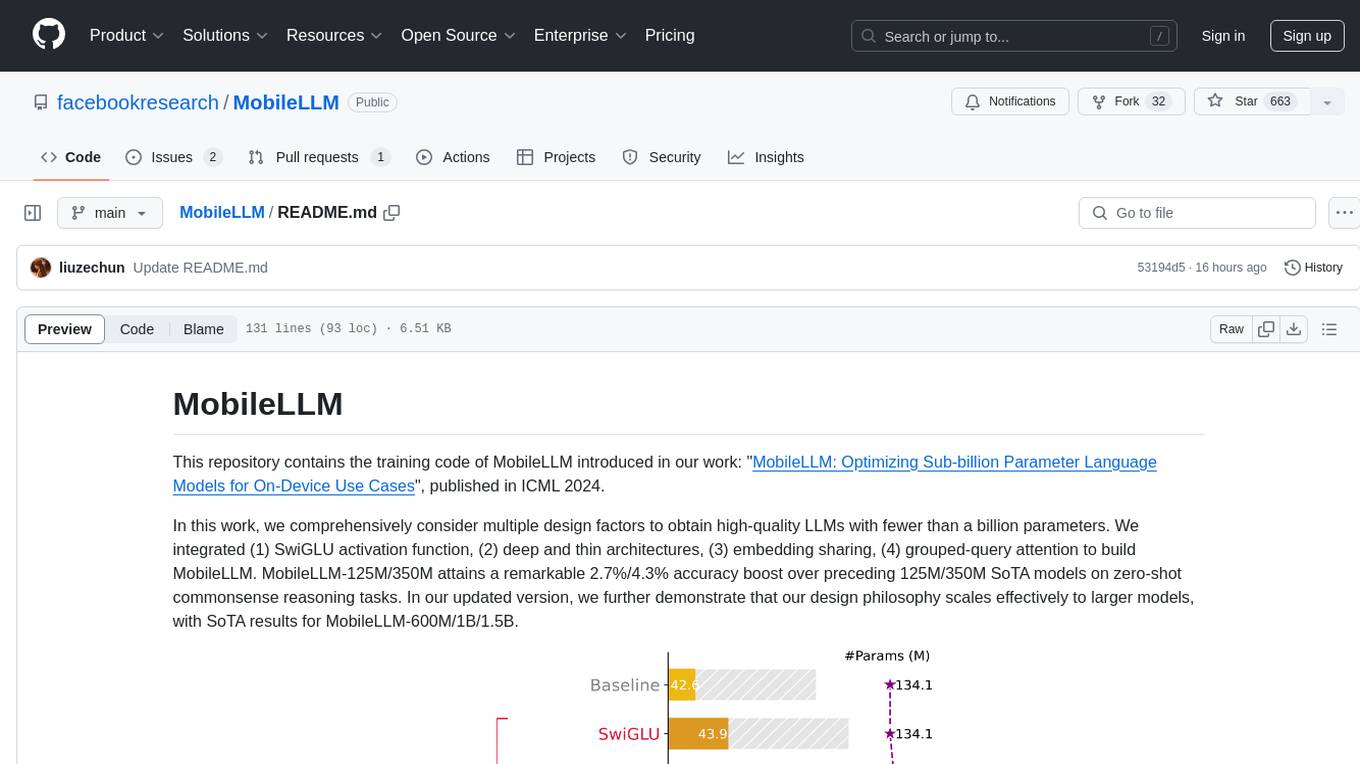
MobileLLM
This repository contains the training code of MobileLLM, a language model optimized for on-device use cases with fewer than a billion parameters. It integrates SwiGLU activation function, deep and thin architectures, embedding sharing, and grouped-query attention to achieve high-quality LLMs. MobileLLM-125M/350M shows significant accuracy improvements over previous models on zero-shot commonsense reasoning tasks. The design philosophy scales effectively to larger models, with state-of-the-art results for MobileLLM-600M/1B/1.5B.
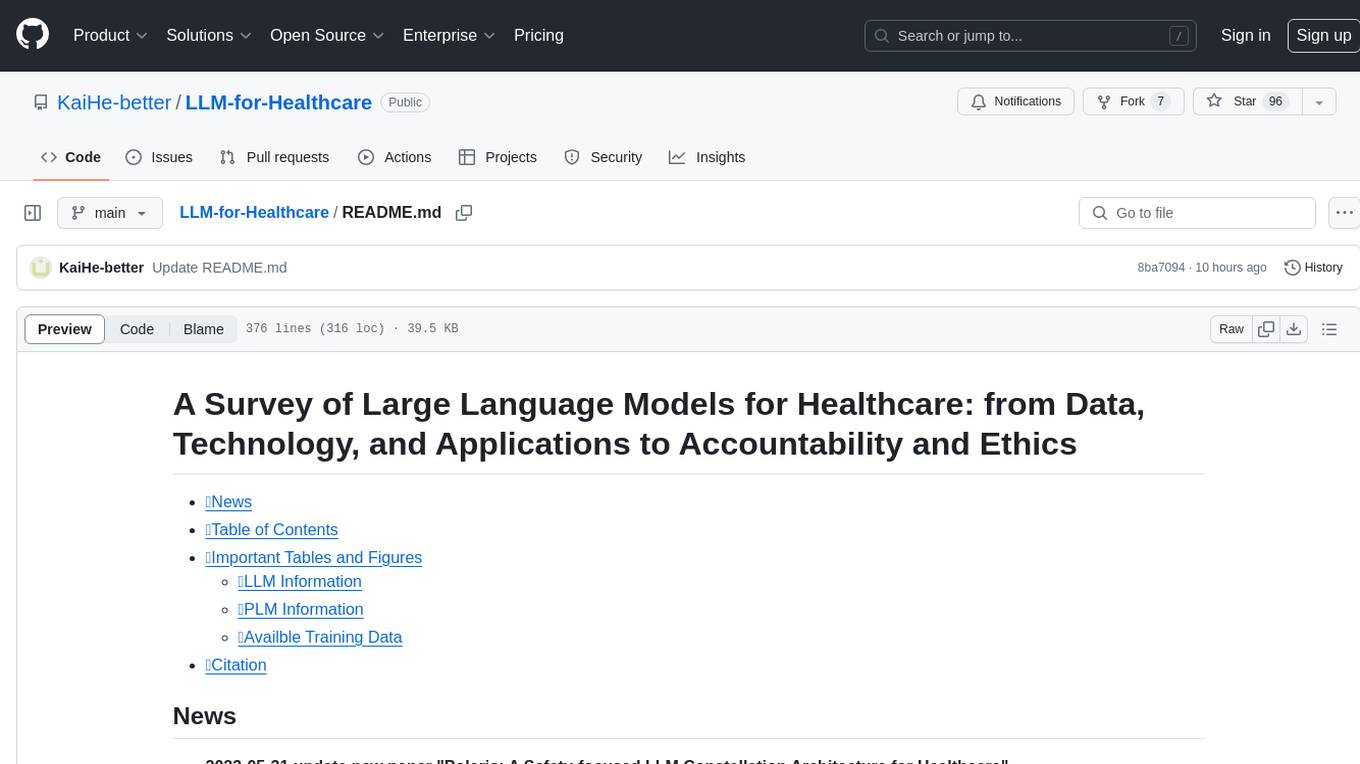
LLM-for-Healthcare
The repository 'LLM-for-Healthcare' provides a comprehensive survey of large language models (LLMs) for healthcare, covering data, technology, applications, and accountability and ethics. It includes information on various LLM models, training data, evaluation methods, and computation costs. The repository also discusses tasks such as NER, text classification, question answering, dialogue systems, and generation of medical reports from images in the healthcare domain.
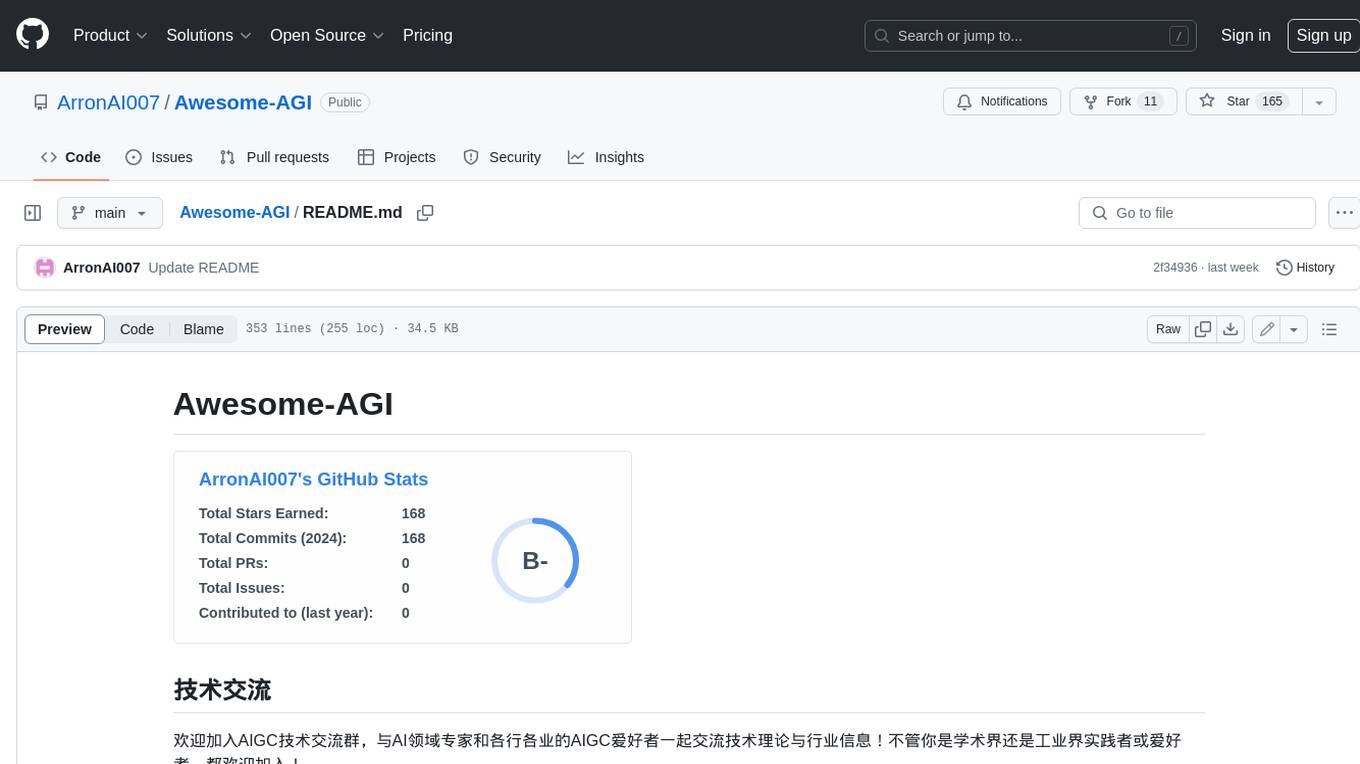
Awesome-AGI
Awesome-AGI is a curated list of resources related to Artificial General Intelligence (AGI), including models, pipelines, applications, and concepts. It provides a comprehensive overview of the current state of AGI research and development, covering various aspects such as model training, fine-tuning, deployment, and applications in different domains. The repository also includes resources on prompt engineering, RLHF, LLM vocabulary expansion, long text generation, hallucination mitigation, controllability and safety, and text detection. It serves as a valuable resource for researchers, practitioners, and anyone interested in the field of AGI.
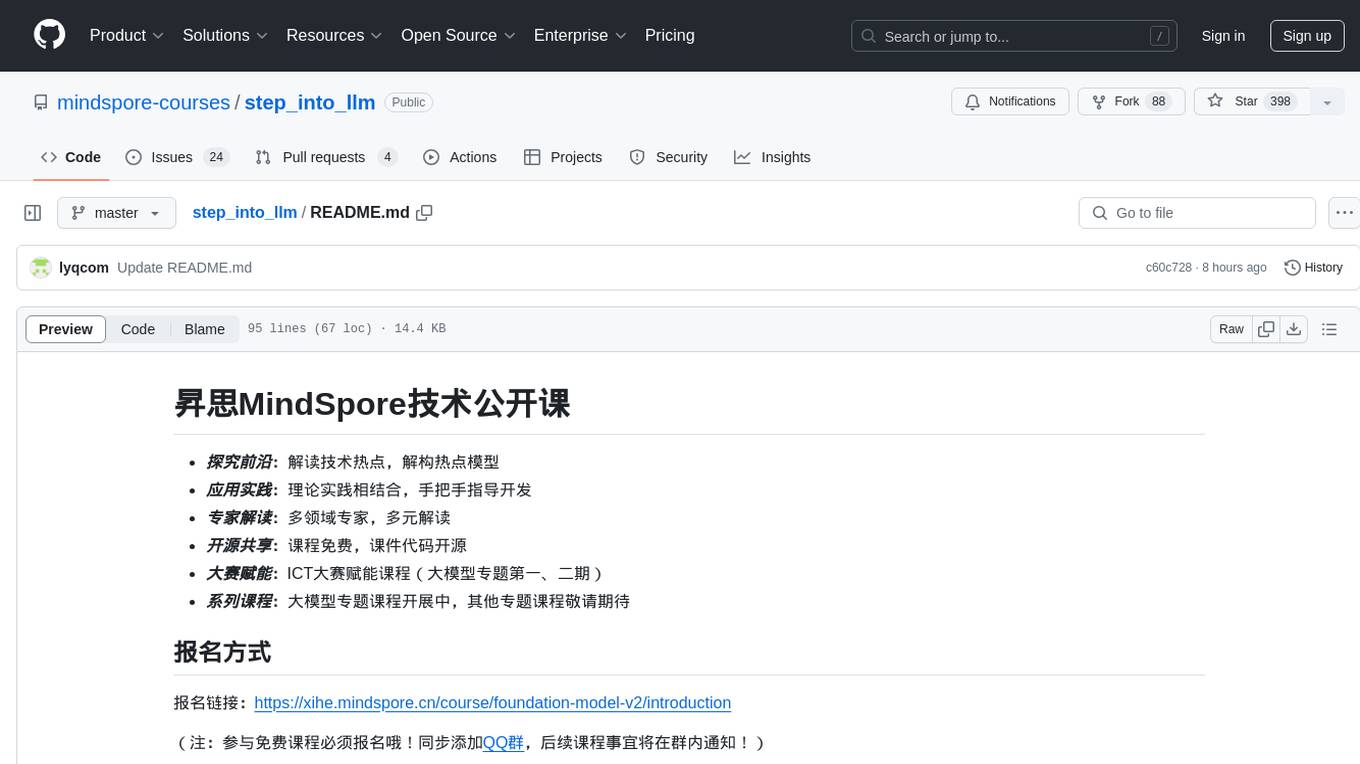
step_into_llm
The 'step_into_llm' repository is dedicated to the 昇思MindSpore technology open class, which focuses on exploring cutting-edge technologies, combining theory with practical applications, expert interpretations, open sharing, and empowering competitions. The repository contains course materials, including slides and code, for the ongoing second phase of the course. It covers various topics related to large language models (LLMs) such as Transformer, BERT, GPT, GPT2, and more. The course aims to guide developers interested in LLMs from theory to practical implementation, with a special emphasis on the development and application of large models.
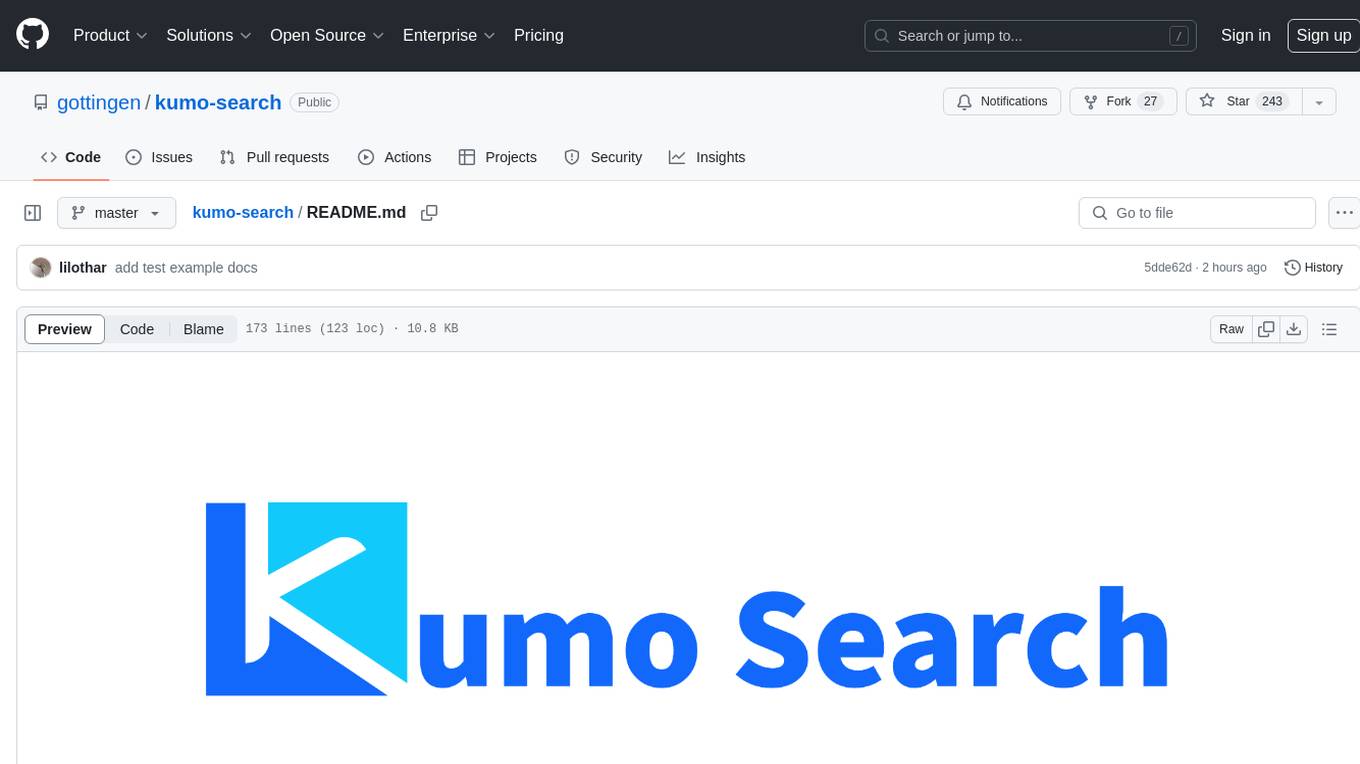
kumo-search
Kumo search is an end-to-end search engine framework that supports full-text search, inverted index, forward index, sorting, caching, hierarchical indexing, intervention system, feature collection, offline computation, storage system, and more. It runs on the EA (Elastic automic infrastructure architecture) platform, enabling engineering automation, service governance, real-time data, service degradation, and disaster recovery across multiple data centers and clusters. The framework aims to provide a ready-to-use search engine framework to help users quickly build their own search engines. Users can write business logic in Python using the AOT compiler in the project, which generates C++ code and binary dynamic libraries for rapid iteration of the search engine.
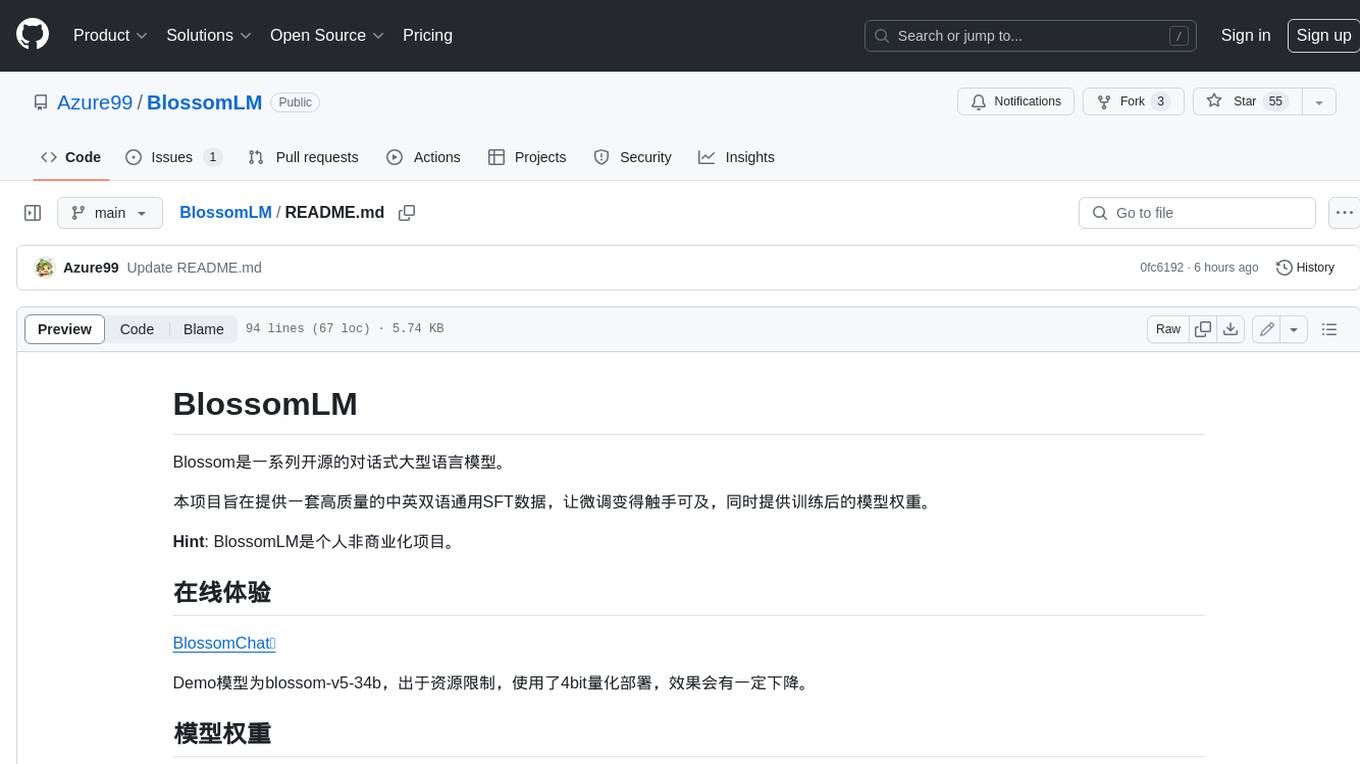
BlossomLM
BlossomLM is a series of open-source conversational large language models. This project aims to provide a high-quality general-purpose SFT dataset in both Chinese and English, making fine-tuning accessible while also providing pre-trained model weights. **Hint**: BlossomLM is a personal non-commercial project.
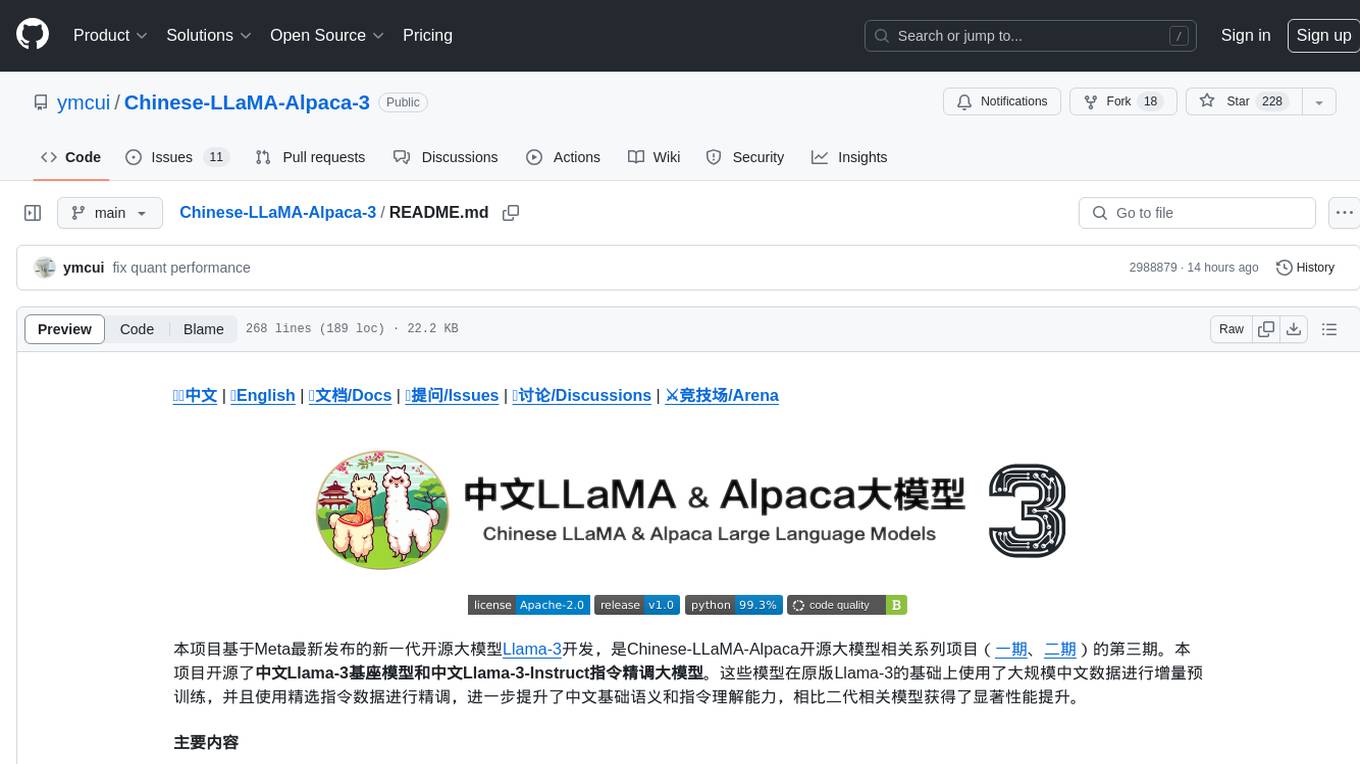
Chinese-LLaMA-Alpaca-3
Chinese-LLaMA-Alpaca-3 is a project based on Meta's latest release of the new generation open-source large model Llama-3. It is the third phase of the Chinese-LLaMA-Alpaca open-source large model series projects (Phase 1, Phase 2). This project open-sources the Chinese Llama-3 base model and the Chinese Llama-3-Instruct instruction fine-tuned large model. These models incrementally pre-train with a large amount of Chinese data on the basis of the original Llama-3 and further fine-tune using selected instruction data, enhancing Chinese basic semantics and instruction understanding capabilities. Compared to the second-generation related models, significant performance improvements have been achieved.
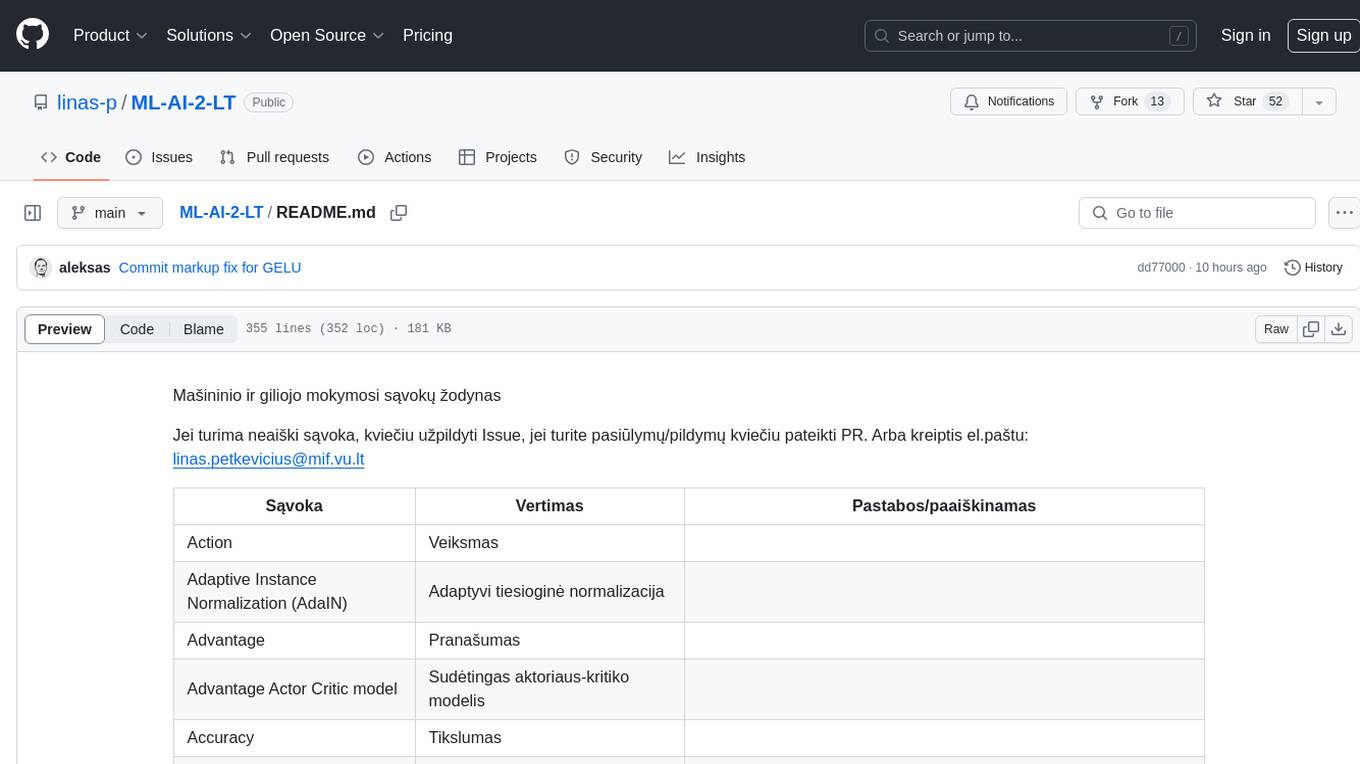
ML-AI-2-LT
ML-AI-2-LT is a repository that serves as a glossary for machine learning and deep learning concepts. It contains translations and explanations of various terms related to artificial intelligence, including definitions and notes. Users can contribute by filling issues for unclear concepts or by submitting pull requests with suggestions or additions. The repository aims to provide a comprehensive resource for understanding key terminology in the field of AI and machine learning.
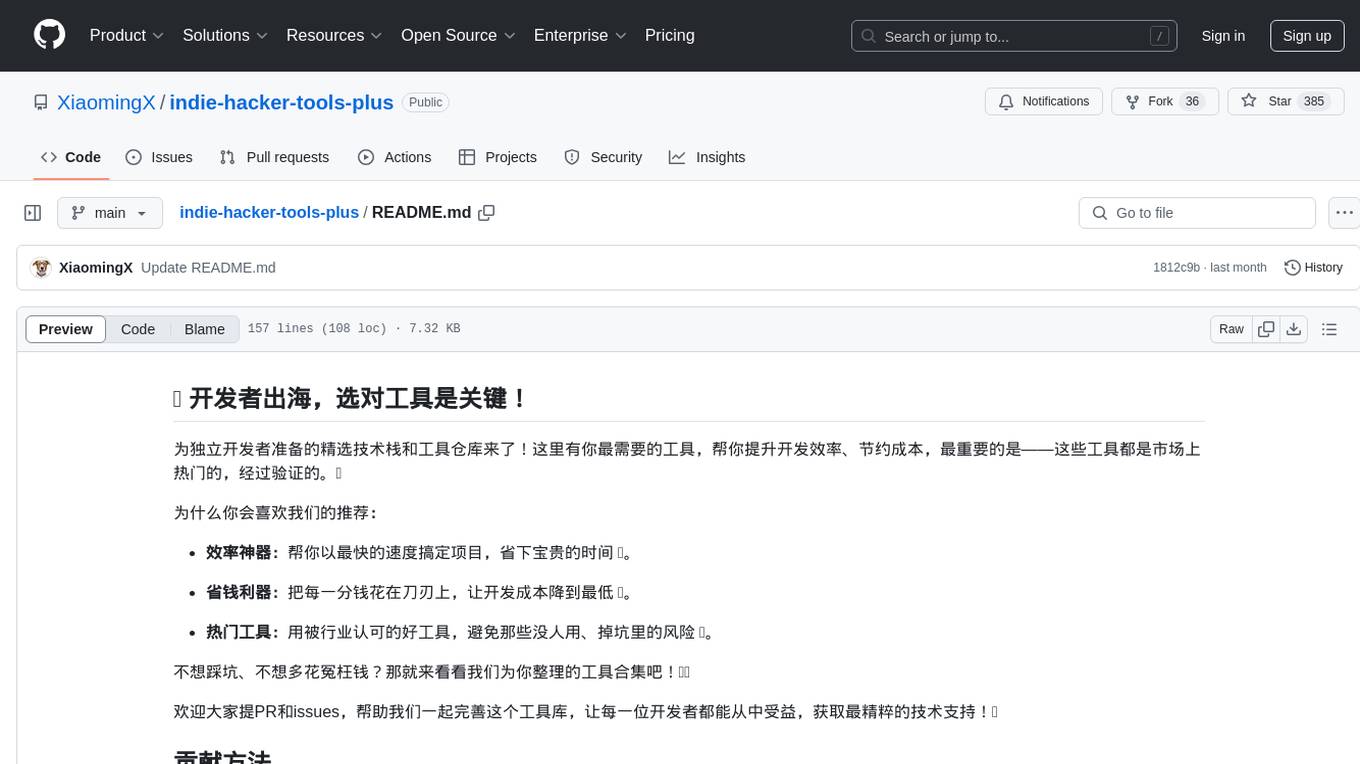
indie-hacker-tools-plus
Indie Hacker Tools Plus is a curated repository of essential tools and technology stacks for independent developers. The repository aims to help developers enhance efficiency, save costs, and mitigate risks by using popular and validated tools. It provides a collection of tools recognized by the industry to empower developers with the most refined technical support. Developers can contribute by submitting articles, software, or resources through issues or pull requests.
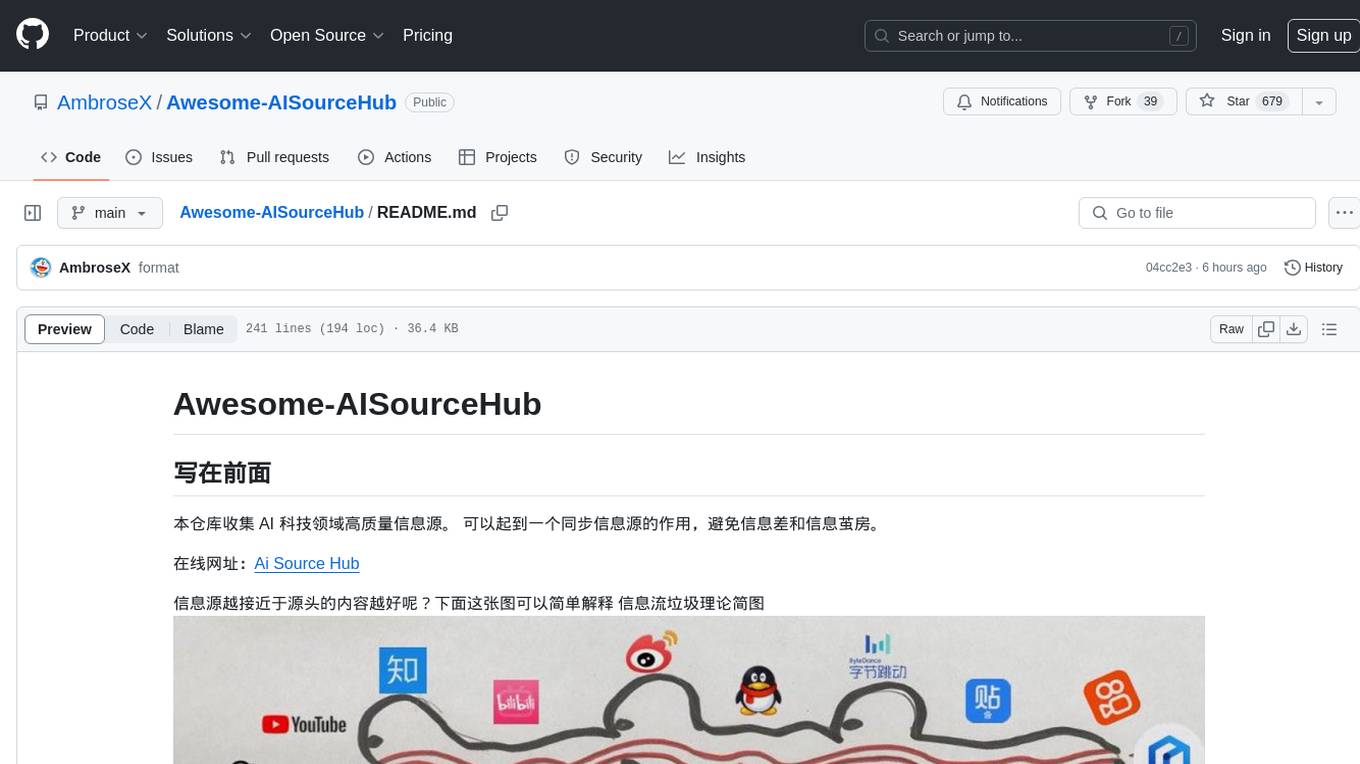
Awesome-AISourceHub
Awesome-AISourceHub is a repository that collects high-quality information sources in the field of AI technology. It serves as a synchronized source of information to avoid information gaps and information silos. The repository aims to provide valuable resources for individuals such as AI book authors, enterprise decision-makers, and tool developers who frequently use Twitter to share insights and updates related to AI advancements. The platform emphasizes the importance of accessing information closer to the source for better quality content. Users can contribute their own high-quality information sources to the repository by following specific steps outlined in the contribution guidelines. The repository covers various platforms such as Twitter, public accounts, knowledge planets, podcasts, blogs, websites, YouTube channels, and more, offering a comprehensive collection of AI-related resources for individuals interested in staying updated with the latest trends and developments in the AI field.
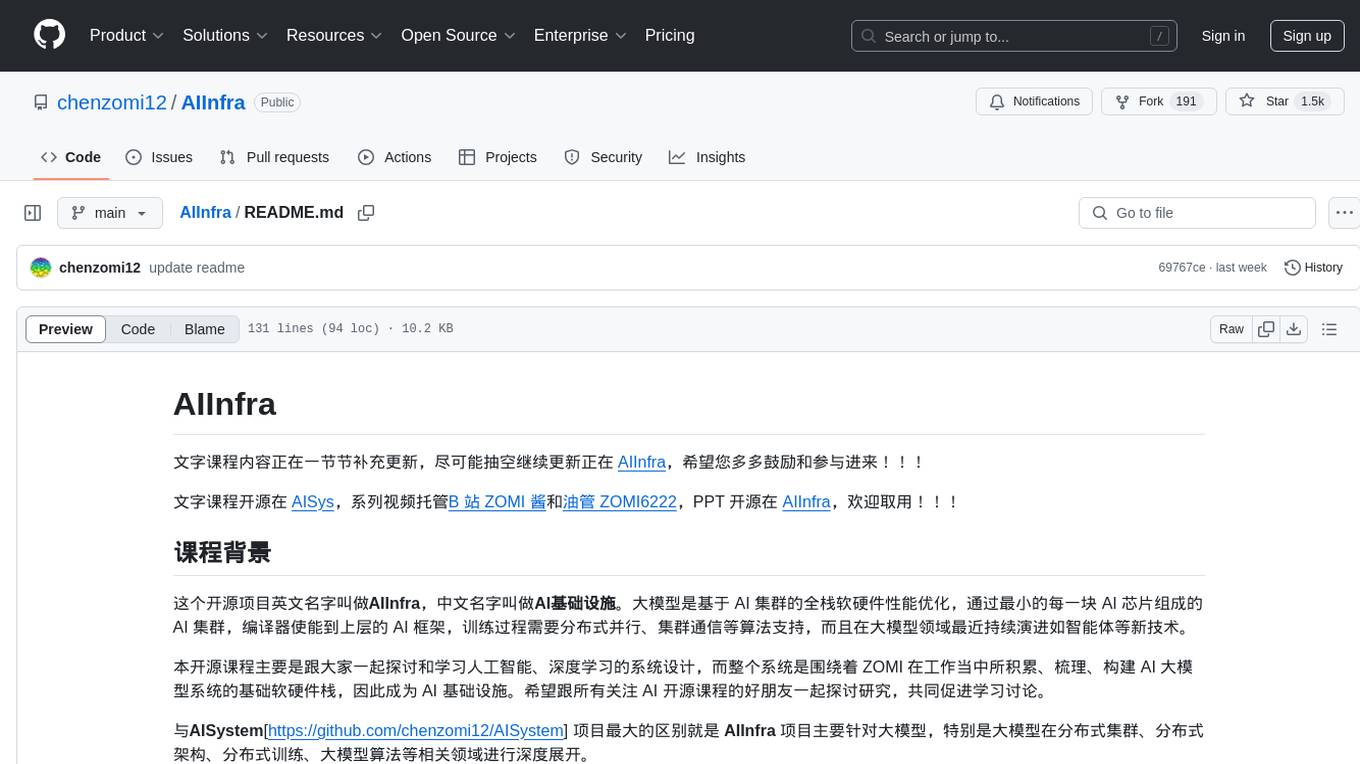
AIInfra
AIInfra is an open-source project focused on AI infrastructure, specifically targeting large models in distributed clusters, distributed architecture, distributed training, and algorithms related to large models. The project aims to explore and study system design in artificial intelligence and deep learning, with a focus on the hardware and software stack for building AI large model systems. It provides a comprehensive curriculum covering topics such as AI chip principles, communication and storage, AI clusters, large model training, and inference, as well as algorithms for large models. The course is designed for undergraduate and graduate students, as well as professionals working with AI large model systems, to gain a deep understanding of AI computer system architecture and design.
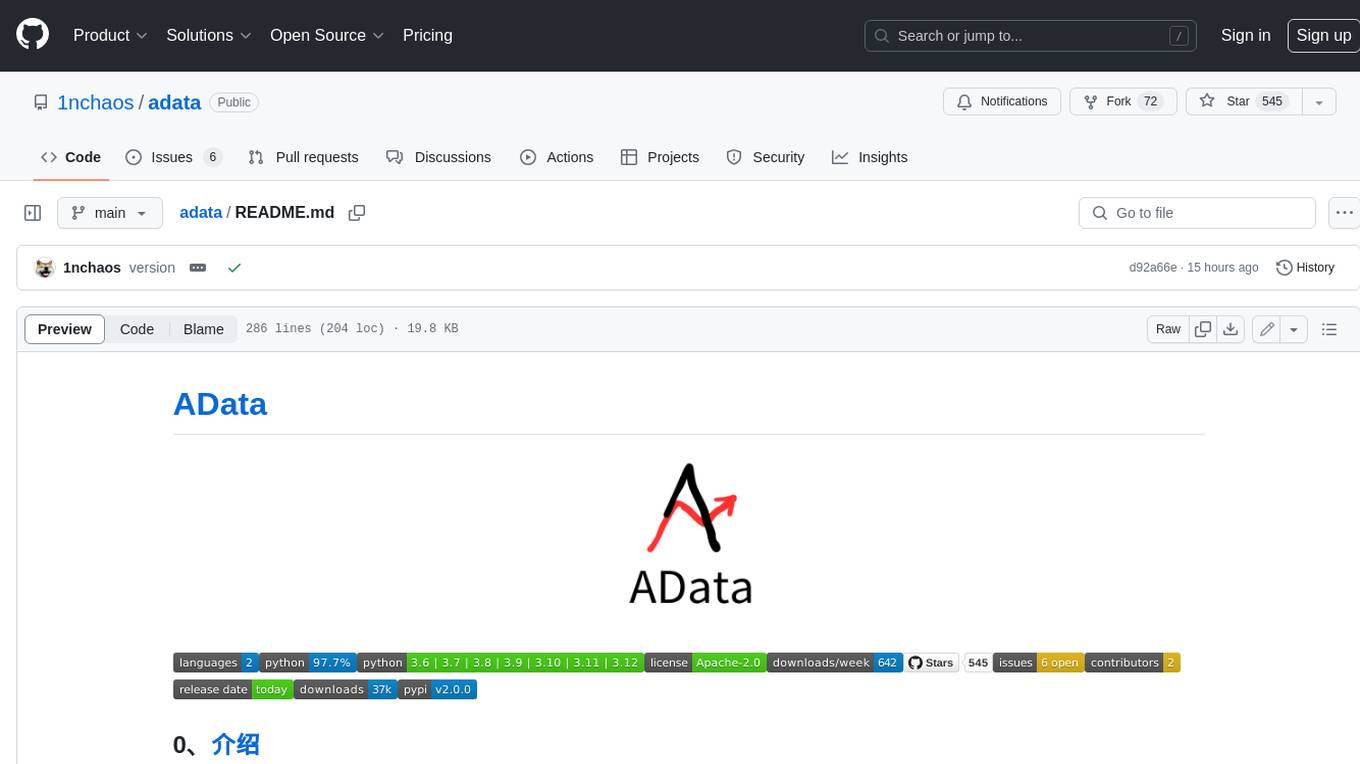
adata
AData is a free and open-source A-share database that focuses on transaction-related data. It provides comprehensive data on stocks, including basic information, market data, and sentiment analysis. AData is designed to be easy to use and integrate with other applications, making it a valuable tool for quantitative trading and AI training.
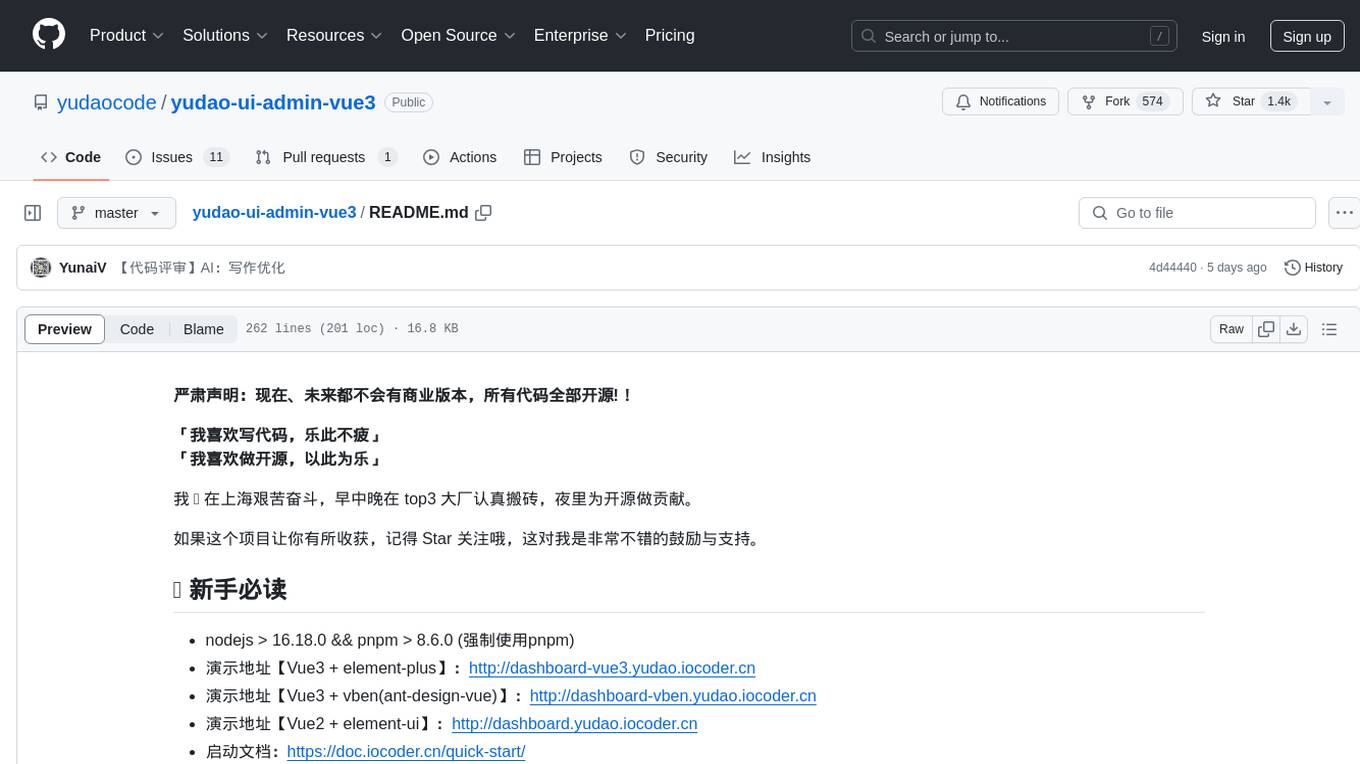
yudao-ui-admin-vue3
The yudao-ui-admin-vue3 repository is an open-source project focused on building a fast development platform for developers in China. It utilizes Vue3 and Element Plus to provide features such as configurable themes, internationalization, dynamic route permission generation, common component encapsulation, and rich examples. The project supports the latest front-end technologies like Vue3 and Vite4, and also includes tools like TypeScript, pinia, vueuse, vue-i18n, vue-router, unocss, iconify, and wangeditor. It offers a range of development tools and features for system functions, infrastructure, workflow management, payment systems, member centers, data reporting, e-commerce systems, WeChat public accounts, ERP systems, and CRM systems.
For similar tasks
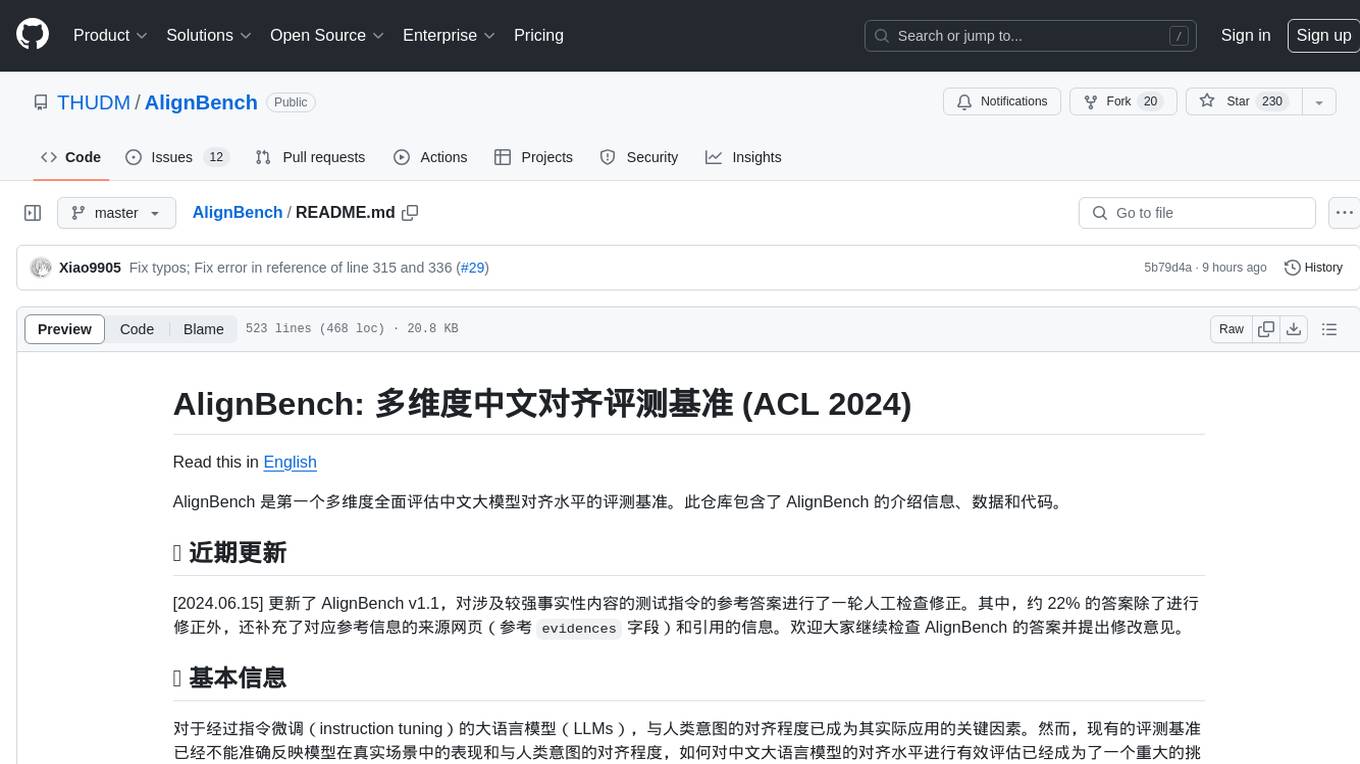
AlignBench
AlignBench is the first comprehensive evaluation benchmark for assessing the alignment level of Chinese large models across multiple dimensions. It includes introduction information, data, and code related to AlignBench. The benchmark aims to evaluate the alignment performance of Chinese large language models through a multi-dimensional and rule-calibrated evaluation method, enhancing reliability and interpretability.
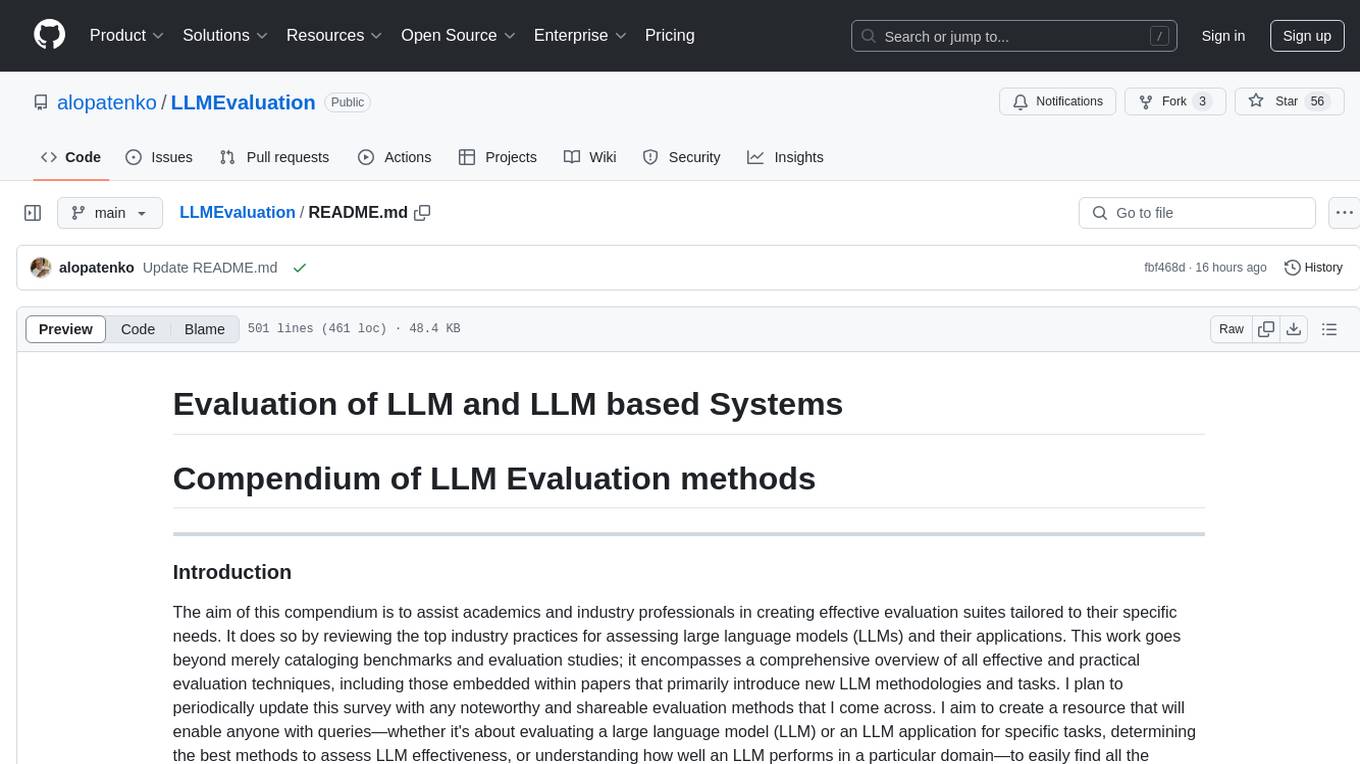
LLMEvaluation
The LLMEvaluation repository is a comprehensive compendium of evaluation methods for Large Language Models (LLMs) and LLM-based systems. It aims to assist academics and industry professionals in creating effective evaluation suites tailored to their specific needs by reviewing industry practices for assessing LLMs and their applications. The repository covers a wide range of evaluation techniques, benchmarks, and studies related to LLMs, including areas such as embeddings, question answering, multi-turn dialogues, reasoning, multi-lingual tasks, ethical AI, biases, safe AI, code generation, summarization, software performance, agent LLM architectures, long text generation, graph understanding, and various unclassified tasks. It also includes evaluations for LLM systems in conversational systems, copilots, search and recommendation engines, task utility, and verticals like healthcare, law, science, financial, and others. The repository provides a wealth of resources for evaluating and understanding the capabilities of LLMs in different domains.
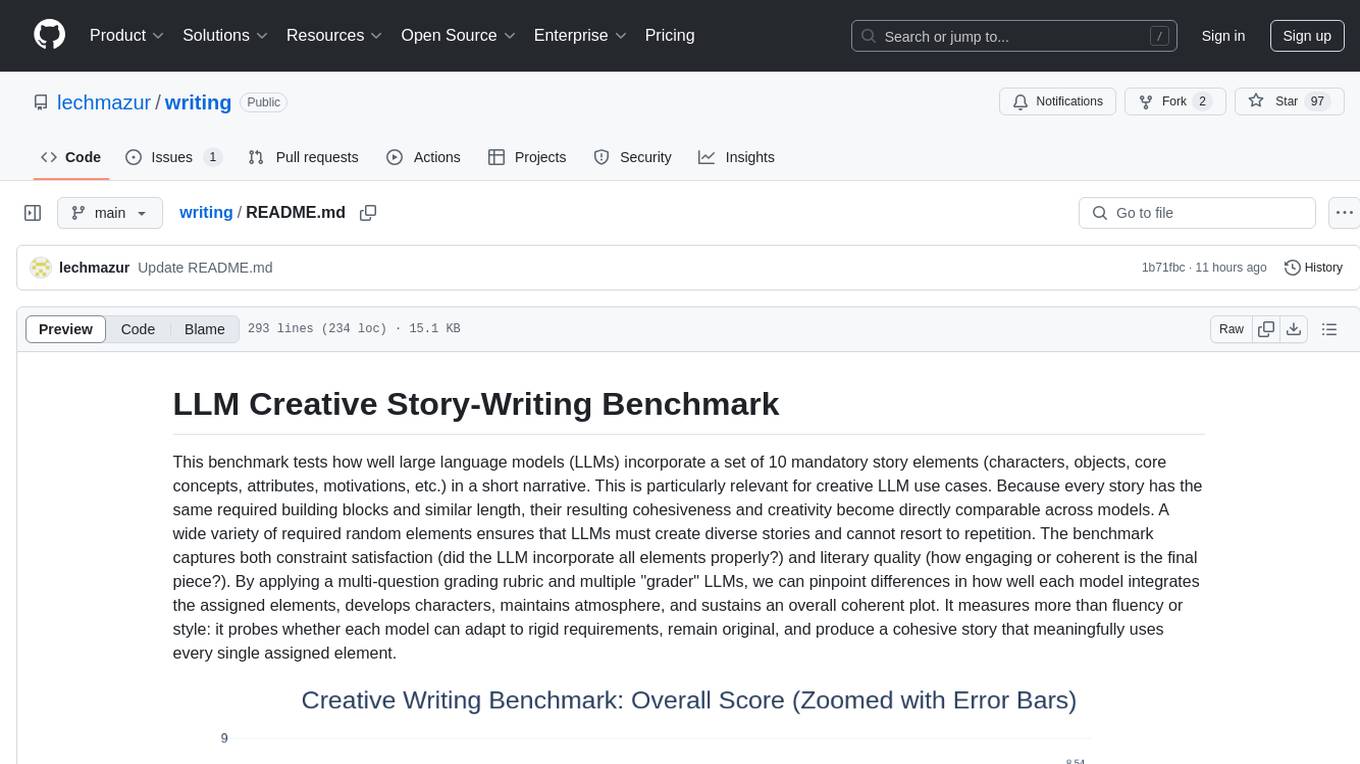
writing
The LLM Creative Story-Writing Benchmark evaluates large language models based on their ability to incorporate a set of 10 mandatory story elements in a short narrative. It measures constraint satisfaction and literary quality by grading models on character development, plot structure, atmosphere, storytelling impact, authenticity, and execution. The benchmark aims to assess how well models can adapt to rigid requirements, remain original, and produce cohesive stories using all assigned elements.
For similar jobs
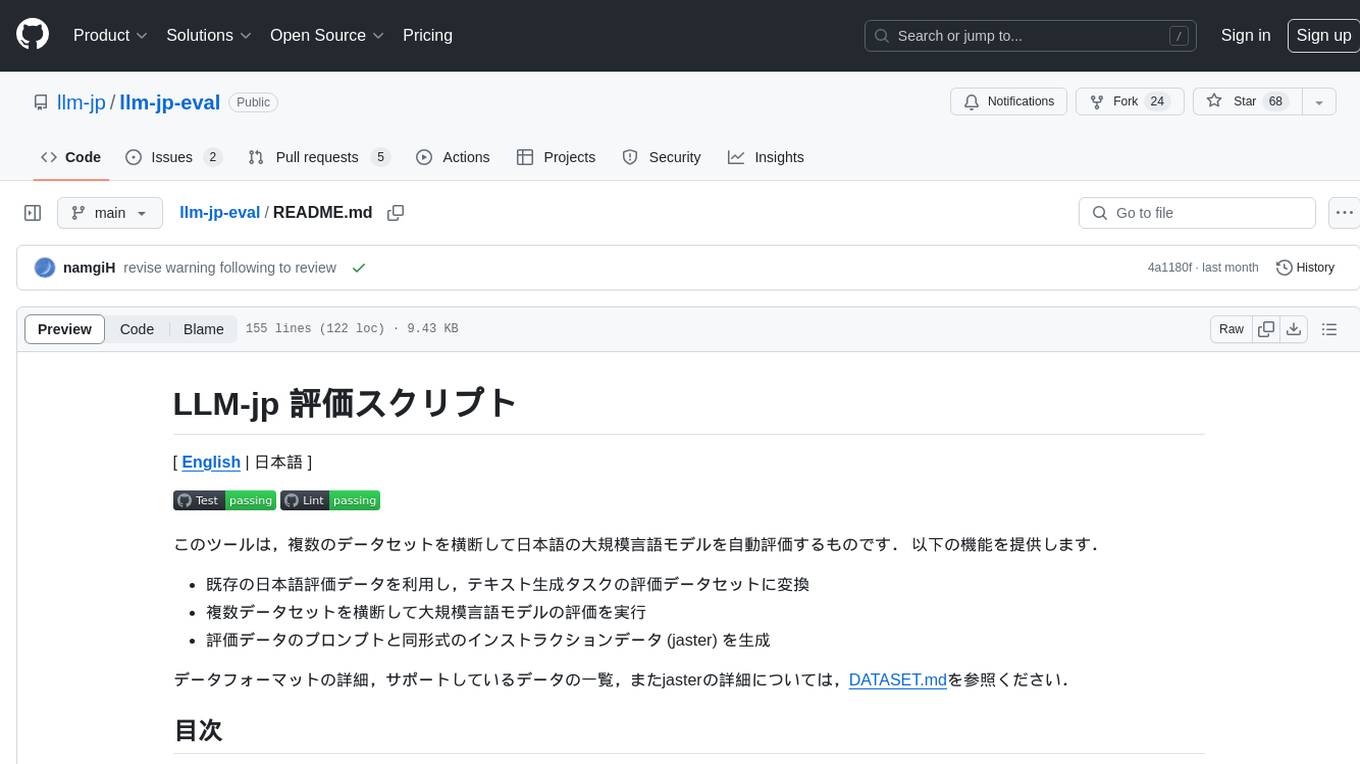
llm-jp-eval
LLM-jp-eval is a tool designed to automatically evaluate Japanese large language models across multiple datasets. It provides functionalities such as converting existing Japanese evaluation data to text generation task evaluation datasets, executing evaluations of large language models across multiple datasets, and generating instruction data (jaster) in the format of evaluation data prompts. Users can manage the evaluation settings through a config file and use Hydra to load them. The tool supports saving evaluation results and logs using wandb. Users can add new evaluation datasets by following specific steps and guidelines provided in the tool's documentation. It is important to note that using jaster for instruction tuning can lead to artificially high evaluation scores, so caution is advised when interpreting the results.
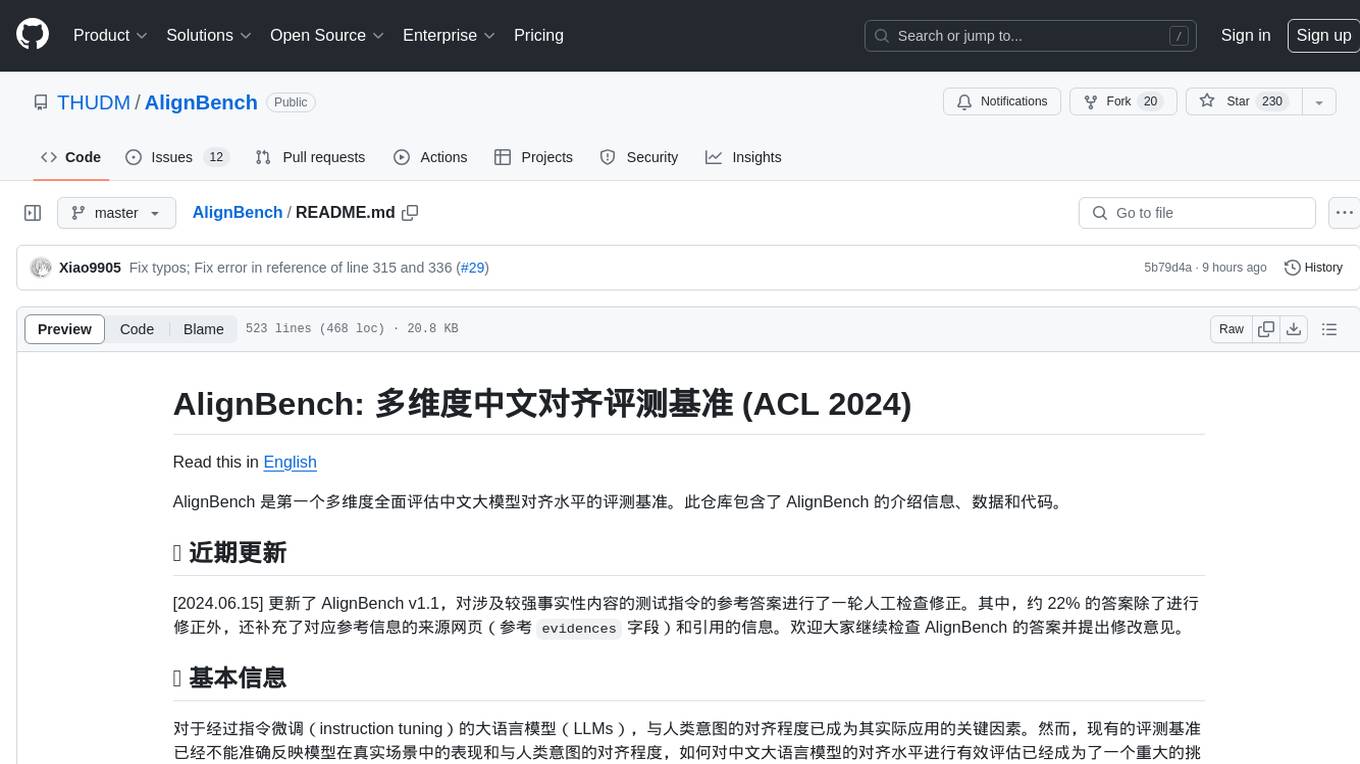
AlignBench
AlignBench is the first comprehensive evaluation benchmark for assessing the alignment level of Chinese large models across multiple dimensions. It includes introduction information, data, and code related to AlignBench. The benchmark aims to evaluate the alignment performance of Chinese large language models through a multi-dimensional and rule-calibrated evaluation method, enhancing reliability and interpretability.
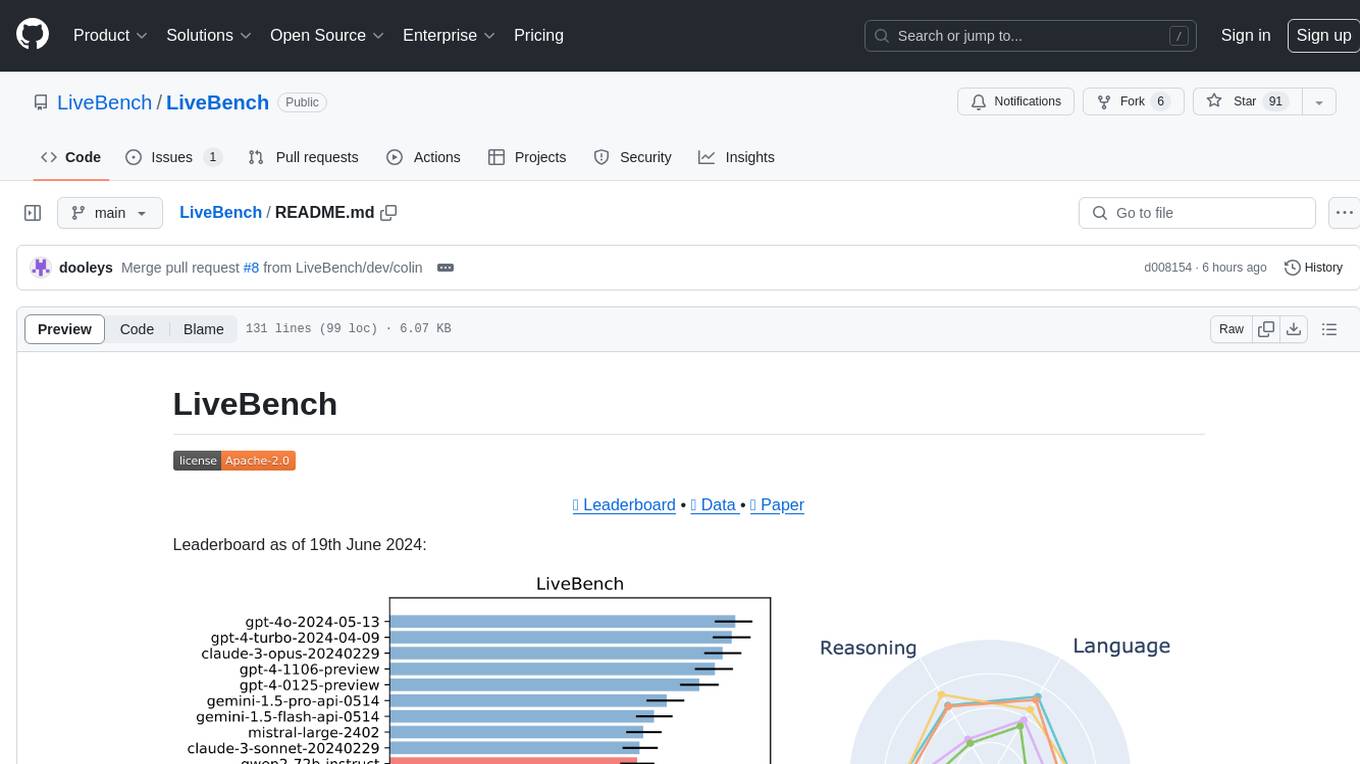
LiveBench
LiveBench is a benchmark tool designed for Language Model Models (LLMs) with a focus on limiting contamination through monthly new questions based on recent datasets, arXiv papers, news articles, and IMDb movie synopses. It provides verifiable, objective ground-truth answers for accurate scoring without an LLM judge. The tool offers 18 diverse tasks across 6 categories and promises to release more challenging tasks over time. LiveBench is built on FastChat's llm_judge module and incorporates code from LiveCodeBench and IFEval.
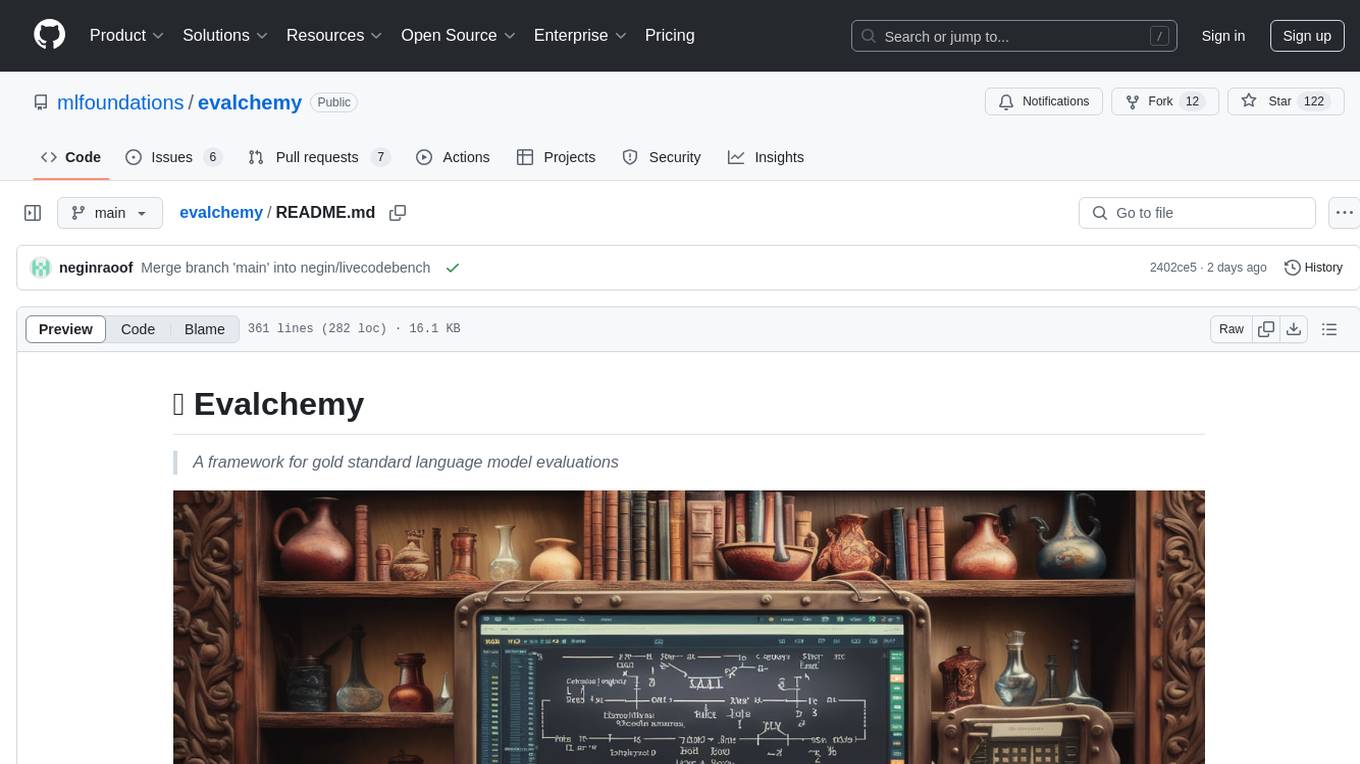
evalchemy
Evalchemy is a unified and easy-to-use toolkit for evaluating language models, focusing on post-trained models. It integrates multiple existing benchmarks such as RepoBench, AlpacaEval, and ZeroEval. Key features include unified installation, parallel evaluation, simplified usage, and results management. Users can run various benchmarks with a consistent command-line interface and track results locally or integrate with a database for systematic tracking and leaderboard submission.
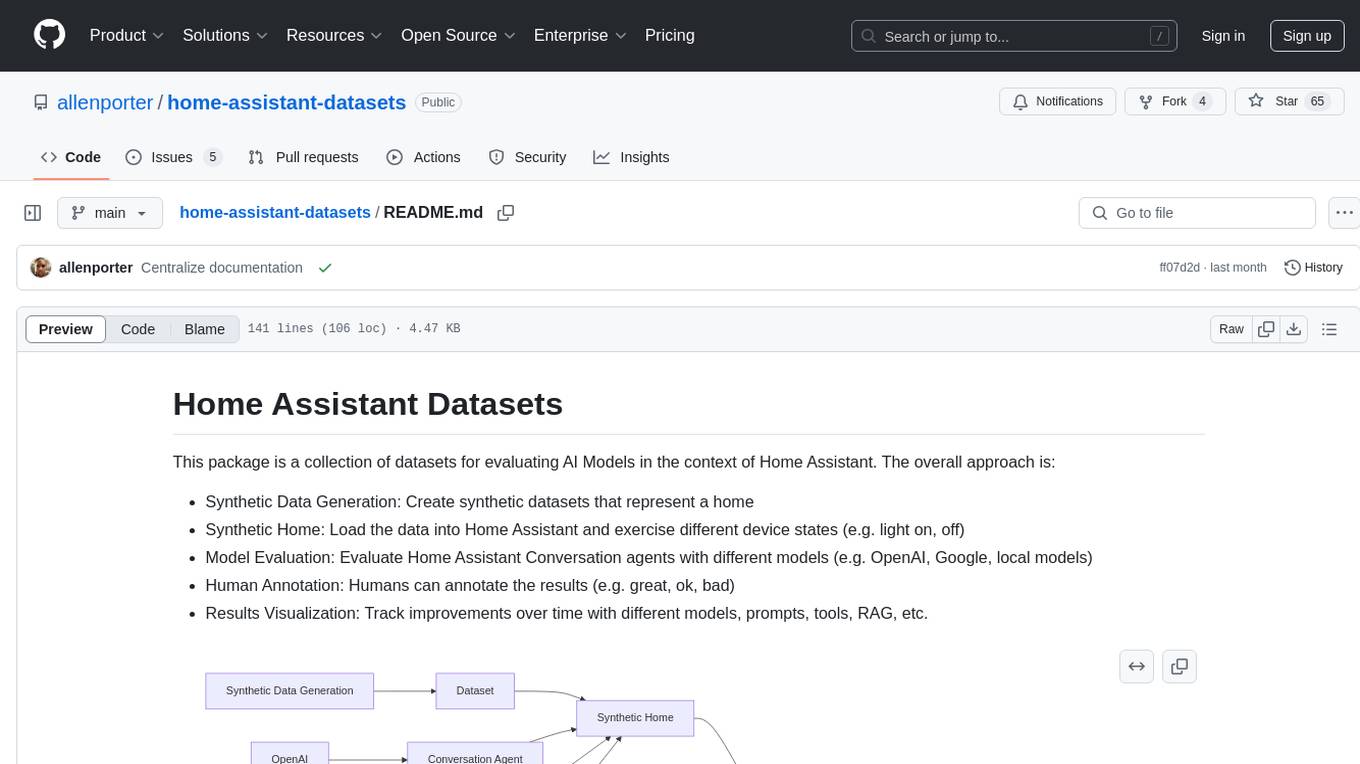
home-assistant-datasets
This package provides a collection of datasets for evaluating AI Models in the context of Home Assistant. It includes synthetic data generation, loading data into Home Assistant, model evaluation with different conversation agents, human annotation of results, and visualization of improvements over time. The datasets cover home descriptions, area descriptions, device descriptions, and summaries that can be performed on a home. The tool aims to build datasets for future training purposes.
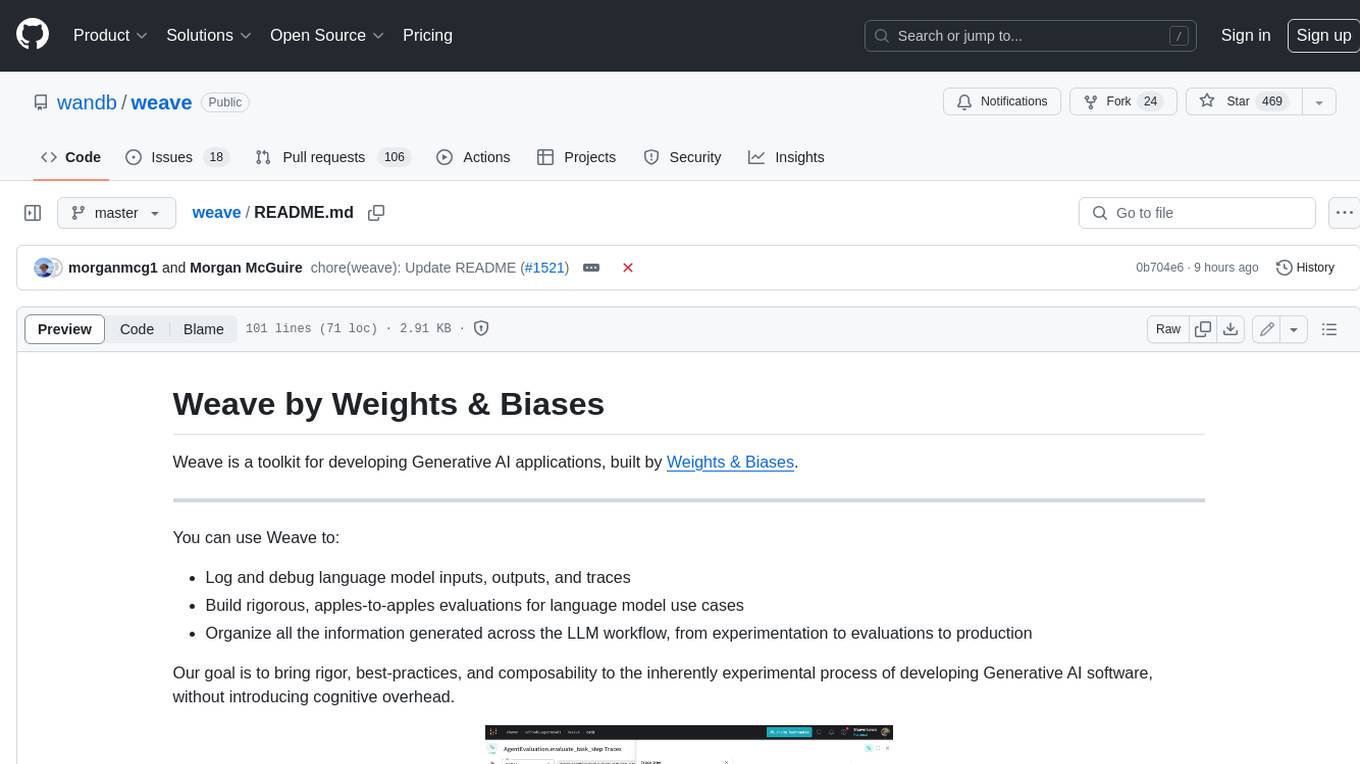
weave
Weave is a toolkit for developing Generative AI applications, built by Weights & Biases. With Weave, you can log and debug language model inputs, outputs, and traces; build rigorous, apples-to-apples evaluations for language model use cases; and organize all the information generated across the LLM workflow, from experimentation to evaluations to production. Weave aims to bring rigor, best-practices, and composability to the inherently experimental process of developing Generative AI software, without introducing cognitive overhead.
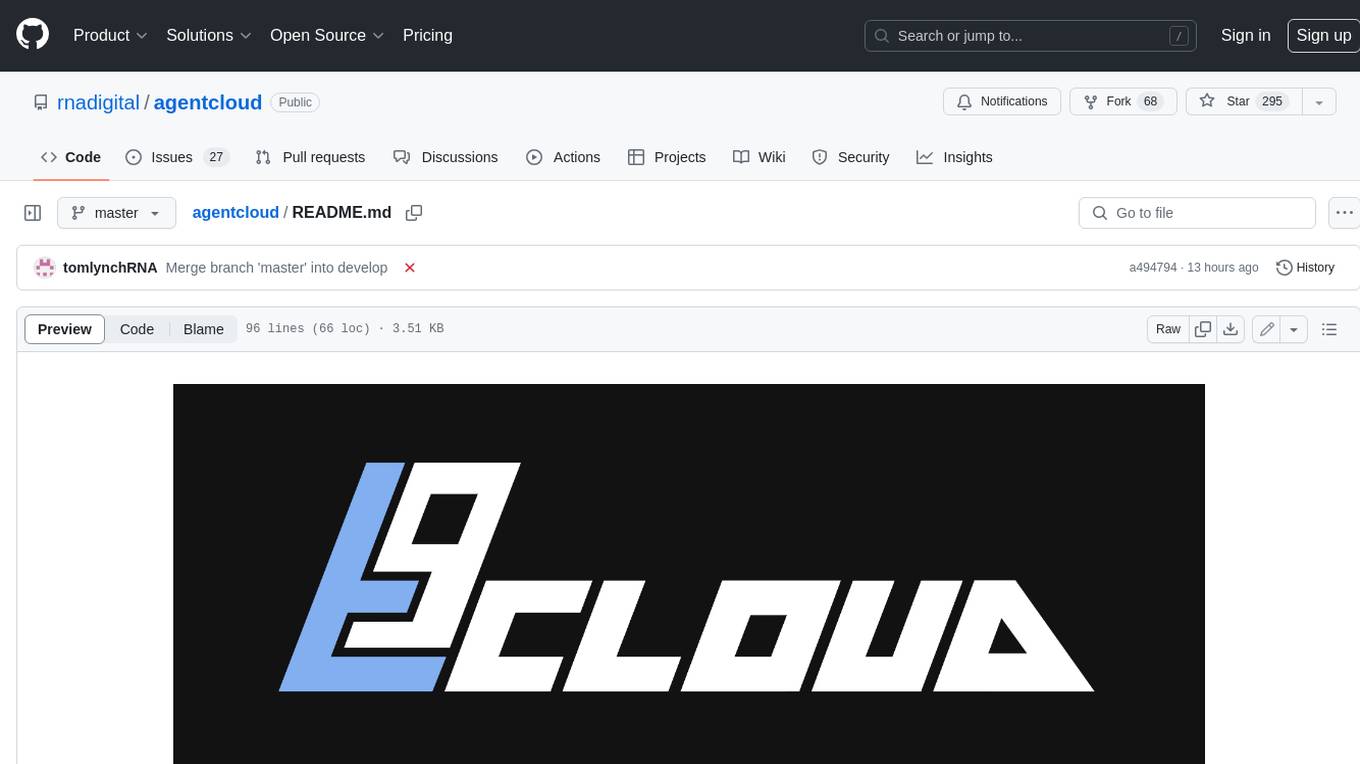
agentcloud
AgentCloud is an open-source platform that enables companies to build and deploy private LLM chat apps, empowering teams to securely interact with their data. It comprises three main components: Agent Backend, Webapp, and Vector Proxy. To run this project locally, clone the repository, install Docker, and start the services. The project is licensed under the GNU Affero General Public License, version 3 only. Contributions and feedback are welcome from the community.
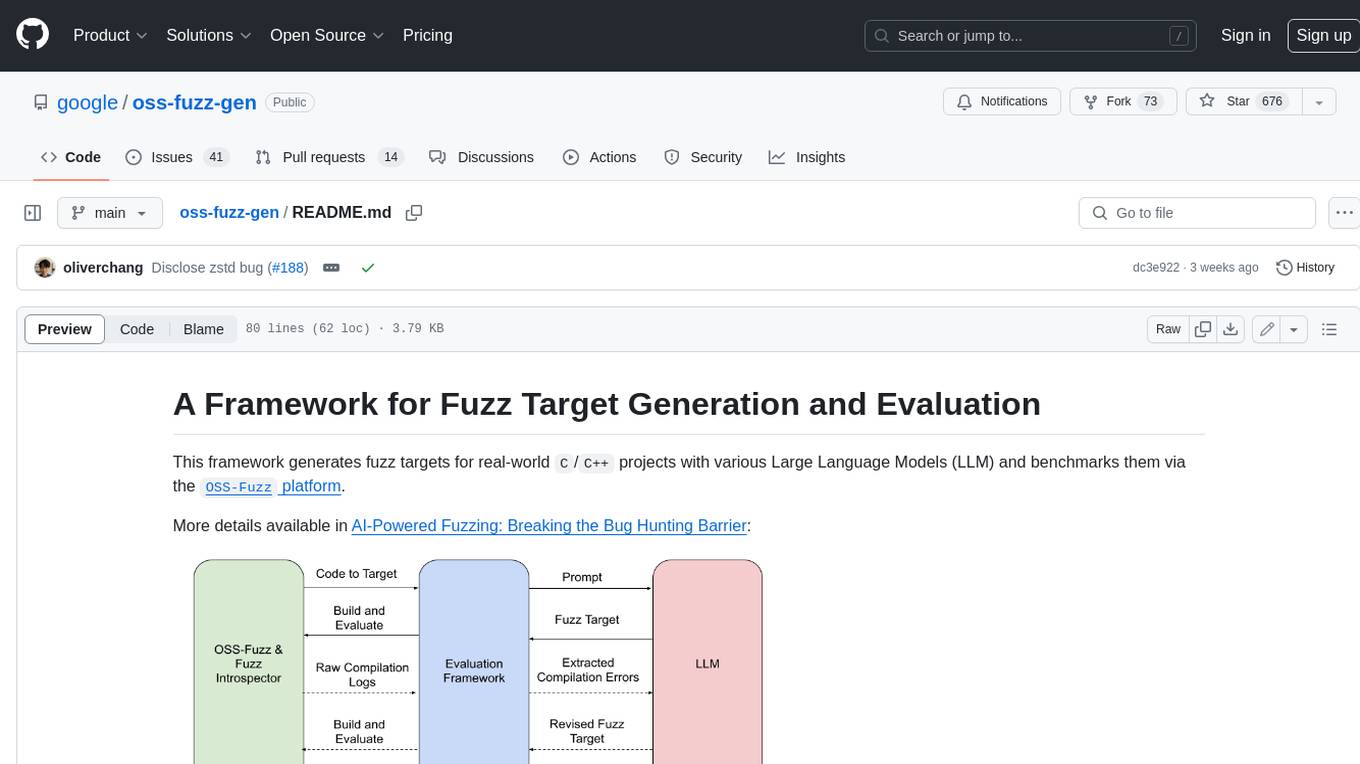
oss-fuzz-gen
This framework generates fuzz targets for real-world `C`/`C++` projects with various Large Language Models (LLM) and benchmarks them via the `OSS-Fuzz` platform. It manages to successfully leverage LLMs to generate valid fuzz targets (which generate non-zero coverage increase) for 160 C/C++ projects. The maximum line coverage increase is 29% from the existing human-written targets.