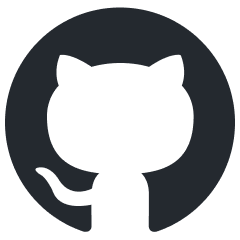
Chinese-LLaMA-Alpaca-3
中文羊驼大模型三期项目 (Chinese Llama-3 LLMs) developed from Meta Llama 3
Stars: 825
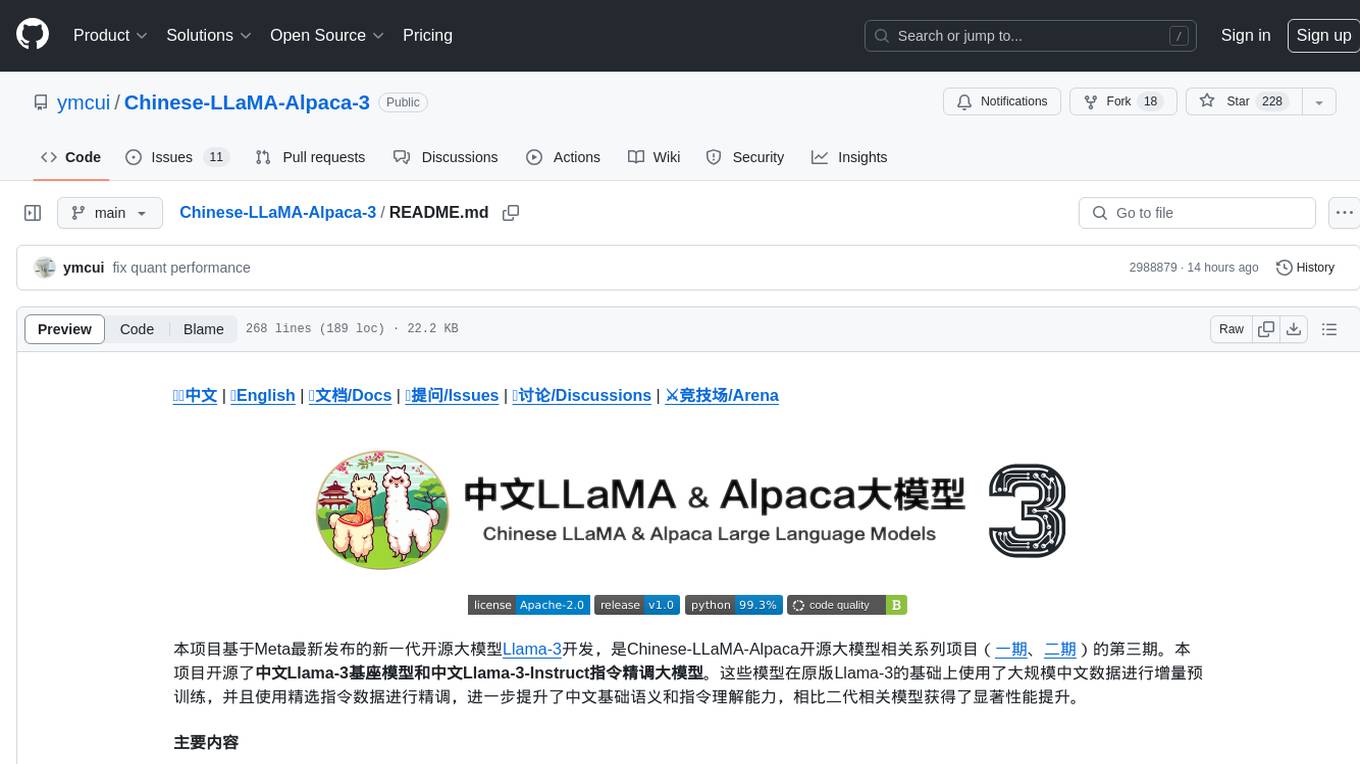
Chinese-LLaMA-Alpaca-3 is a project based on Meta's latest release of the new generation open-source large model Llama-3. It is the third phase of the Chinese-LLaMA-Alpaca open-source large model series projects (Phase 1, Phase 2). This project open-sources the Chinese Llama-3 base model and the Chinese Llama-3-Instruct instruction fine-tuned large model. These models incrementally pre-train with a large amount of Chinese data on the basis of the original Llama-3 and further fine-tune using selected instruction data, enhancing Chinese basic semantics and instruction understanding capabilities. Compared to the second-generation related models, significant performance improvements have been achieved.
README:
🇨🇳中文 | 🌐English | 📖文档/Docs | ❓提问/Issues | 💬讨论/Discussions | ⚔️竞技场/Arena
本项目基于Meta最新发布的新一代开源大模型Llama-3开发,是Chinese-LLaMA-Alpaca开源大模型相关系列项目(一期、二期)的第三期。本项目开源了中文Llama-3基座模型和中文Llama-3-Instruct指令精调大模型。这些模型在原版Llama-3的基础上使用了大规模中文数据进行增量预训练,并且使用精选指令数据进行精调,进一步提升了中文基础语义和指令理解能力,相比二代相关模型获得了显著性能提升。
- 🚀 开源Llama-3-Chinese基座模型和Llama-3-Chinese-Instruct指令模型
- 🚀 开源了预训练脚本、指令精调脚本,用户可根据需要进一步训练或微调模型
- 🚀 开源了alpaca_zh_51k, stem_zh_instruction, ruozhiba_gpt4 (4o/4T) 指令精调数据
- 🚀 提供了利用个人电脑CPU/GPU快速在本地进行大模型量化和部署的教程
- 🚀 支持🤗transformers, llama.cpp, text-generation-webui, vLLM, Ollama等Llama-3生态
中文Mixtral大模型 | 中文LLaMA-2&Alpaca-2大模型 | 中文LLaMA&Alpaca大模型 | 多模态中文LLaMA&Alpaca大模型 | 多模态VLE | 中文MiniRBT | 中文LERT | 中英文PERT | 中文MacBERT | 中文ELECTRA | 中文XLNet | 中文BERT | 知识蒸馏工具TextBrewer | 模型裁剪工具TextPruner | 蒸馏裁剪一体化GRAIN
[2024/05/08] 发布Llama-3-Chinese-8B-Instruct-v2版指令模型,直接采用500万条指令数据在 Meta-Llama-3-8B-Instruct 上进行精调。详情查看:📚v2.0版本发布日志
[2024/05/07] 添加预训练脚本、指令精调脚本。详情查看:📚v1.1版本发布日志
[2024/04/30] 发布Llama-3-Chinese-8B基座模型和Llama-3-Chinese-8B-Instruct指令模型。详情查看:📚v1.0版本发布日志
[2024/04/19] 🚀 正式启动Chinese-LLaMA-Alpaca-3项目
章节 | 描述 |
---|---|
💁🏻♂️模型简介 | 简要介绍本项目相关模型的技术特点 |
⏬模型下载 | 中文Llama-3大模型下载地址 |
💻推理与部署 | 介绍了如何对模型进行量化并使用个人电脑部署并体验大模型 |
💯模型效果 | 介绍了模型在部分任务上的效果 |
📝训练与精调 | 介绍了如何训练和精调中文Llama-3大模型 |
❓常见问题 | 一些常见问题的回复 |
本项目推出了基于Meta Llama-3的中文开源大模型Llama-3-Chinese以及Llama-3-Chinese-Instruct。主要特点如下:
- Llama-3相比其前两代显著扩充了词表大小,由32K扩充至128K,并且改为BPE词表
- 初步实验发现Llama-3词表的编码效率与我们扩充词表的中文LLaMA-2相当,效率约为中文LLaMA-2词表的95%(基于维基百科数据上的编码效率测试)
- 结合我们在中文Mixtral上的相关经验及实验结论1,我们并未对词表进行额外扩充
- Llama-3将原生上下文窗口长度从4K提升至8K,能够进一步处理更长的上下文信息
- 用户也可通过PI、NTK、YaRN等方法对模型进行长上下文的扩展,以支持更长文本的处理
- Llama-3采用了Llama-2中大参数量版本应用的分组查询注意力(GQA)机制,能够进一步提升模型的效率
- Llama-3-Instruct采用了全新的指令模板,与Llama-2-chat不兼容,使用时应遵循官方指令模板(见指令模板)
以下是本项目的模型对比以及建议使用场景。如需聊天交互,请选择Instruct版。
对比项 | Llama-3-Chinese-8B | Llama-3-Chinese-8B-Instruct |
---|---|---|
模型类型 | 基座模型 | 指令/Chat模型(类ChatGPT) |
模型大小 | 8B | 8B |
训练类型 | Causal-LM (CLM) | 指令精调 |
训练方式 | LoRA + 全量emb/lm-head | LoRA + 全量emb/lm-head |
初始化模型 | 原版Meta-Llama-3-8B | v1: Llama-3-Chinese-8B v2: 原版Meta-Llama-3-8B-Instruct |
训练语料 | 无标注通用语料(约120GB) | 有标注指令数据(约500万条) |
词表大小 | 原版词表(128,256) | 原版词表(128,256) |
支持上下文长度 | 8K | 8K |
输入模板 | 不需要 | 需要套用Llama-3-Instruct模板 |
适用场景 | 文本续写:给定上文,让模型生成下文 | 指令理解:问答、写作、聊天、交互等 |
模型名称 | 完整版 | LoRA版 | GGUF版 |
---|---|---|---|
Llama-3-Chinese-8B-Instruct-v2 (指令模型) |
[🤗Hugging Face] [🤖ModelScope] [wisemodel] |
[🤗Hugging Face] [🤖ModelScope] [wisemodel] |
[🤗Hugging Face] [🤖ModelScope] |
Llama-3-Chinese-8B-Instruct (指令模型) |
[🤗Hugging Face] [🤖ModelScope] [wisemodel] |
[🤗Hugging Face] [🤖ModelScope] [wisemodel] |
[🤗Hugging Face] [🤖ModelScope] |
Llama-3-Chinese-8B (基座模型) |
[🤗Hugging Face] [🤖ModelScope] [wisemodel] |
[🤗Hugging Face] [🤖ModelScope] [wisemodel] |
[🤗Hugging Face] [🤖ModelScope] |
模型类型说明:
- 完整模型:可直接用于训练和推理,无需其他合并步骤
-
LoRA模型:需要与基模型合并并才能转为完整版模型,合并方法:💻 模型合并步骤
- v1基模型:原版Meta-Llama-3-8B
- v2基模型:原版Meta-Llama-3-8B-Instruct
-
GGUF模型:llama.cpp推出的量化格式,适配ollama等常见推理工具,推荐只需要做推理部署的用户下载;模型名后缀为
-im
表示使用了importance matrix进行量化,通常具有更低的PPL,建议使用(用法与常规版相同)
[!NOTE] 若无法访问HF,可考虑一些镜像站点(如hf-mirror.com),具体方法请自行查找解决。
本项目中的相关模型主要支持以下量化、推理和部署方式,具体内容请参考对应教程。
工具 | 特点 | CPU | GPU | 量化 | GUI | API | vLLM | 教程 |
---|---|---|---|---|---|---|---|---|
llama.cpp | 丰富的GGUF量化选项和高效本地推理 | ✅ | ✅ | ✅ | ✅ | ✅ | ❌ | [link] |
🤗transformers | 原生transformers推理接口 | ✅ | ✅ | ✅ | ✅ | ❌ | ✅ | [link] |
仿OpenAI API调用 | 仿OpenAI API接口的服务器Demo | ✅ | ✅ | ✅ | ❌ | ✅ | ✅ | [link] |
text-generation-webui | 前端Web UI界面的部署方式 | ✅ | ✅ | ✅ | ✅ | ✅ | ❌ | [link] |
LM Studio | 多平台聊天软件(带界面) | ✅ | ✅ | ✅ | ✅ | ✅ | ❌ | [link] |
Ollama | 本地运行大模型推理 | ✅ | ✅ | ✅ | ❌ | ✅ | ❌ | [link] |
为了评测相关模型的效果,本项目分别进行了生成效果评测和客观效果评测(NLU类),从不同角度对大模型进行评估。推荐用户在自己关注的任务上进行测试,选择适配相关任务的模型。
- 本项目仿照Fastchat Chatbot Arena推出了模型在线对战平台,可浏览和评测模型回复质量。对战平台提供了胜率、Elo评分等评测指标,并且可以查看两两模型的对战胜率等结果。⚔️ 模型竞技场:http://llm-arena.ymcui.com
- examples目录中提供了Llama-3-Chinese-8B-Instruct和Chinese-Mixtral-Instruct的输出样例,并通过GPT-4-turbo进行了打分对比,Llama-3-Chinese-8B-Instruct平均得分为8.1、Chinese-Mixtral-Instruct平均得分为7.8。📄 输出样例对比:examples
- 本项目已入驻机器之心SOTA!模型平台,后期将实现在线体验:https://sota.jiqizhixin.com/project/chinese-llama-alpaca-3
C-Eval是一个全面的中文基础模型评估套件,其中验证集和测试集分别包含1.3K和12.3K个选择题,涵盖52个学科。C-Eval推理代码请参考本项目:📖GitHub Wiki
Models | Valid (0-shot) | Valid (5-shot) | Test (0-shot) | Test (5-shot) |
---|---|---|---|---|
Llama-3-Chinese-8B-Instruct-v2 | 51.6 | 51.6 | 49.7 | 49.8 |
Llama-3-Chinese-8B-Instruct | 49.3 | 51.5 | 48.3 | 49.4 |
Llama-3-Chinese-8B | 47.0 | 50.5 | 46.1 | 49.0 |
Meta-Llama-3-8B-Instruct | 51.3 | 51.3 | 49.5 | 51.0 |
Meta-Llama-3-8B | 49.3 | 51.2 | 46.1 | 49.4 |
Chinese-Mixtral-Instruct (8x7B) | 51.7 | 55.0 | 50.0 | 51.5 |
Chinese-Mixtral (8x7B) | 45.8 | 54.2 | 43.1 | 49.1 |
Chinese-Alpaca-2-13B | 44.3 | 45.9 | 42.6 | 44.0 |
Chinese-LLaMA-2-13B | 40.6 | 42.7 | 38.0 | 41.6 |
CMMLU是另一个综合性中文评测数据集,专门用于评估语言模型在中文语境下的知识和推理能力,涵盖了从基础学科到高级专业水平的67个主题,共计11.5K个选择题。CMMLU推理代码请参考本项目:📖GitHub Wiki
Models | Test (0-shot) | Test (5-shot) |
---|---|---|
Llama-3-Chinese-8B-Instruct-v2 | 51.8 | 52.4 |
Llama-3-Chinese-8B-Instruct | 49.7 | 51.5 |
Llama-3-Chinese-8B | 48.0 | 50.9 |
Meta-Llama-3-8B-Instruct | 53.0 | 53.5 |
Meta-Llama-3-8B | 47.8 | 50.8 |
Chinese-Mixtral-Instruct (8x7B) | 50.0 | 53.0 |
Chinese-Mixtral (8x7B) | 42.5 | 51.0 |
Chinese-Alpaca-2-13B | 43.2 | 45.5 |
Chinese-LLaMA-2-13B | 38.9 | 42.5 |
MMLU是一个用于评测自然语言理解能力的英文评测数据集,是当今用于评测大模型能力的主要数据集之一,其中验证集和测试集分别包含1.5K和14.1K个选择题,涵盖57个学科。MMLU推理代码请参考本项目:📖GitHub Wiki
Models | Valid (0-shot) | Valid (5-shot) | Test (0-shot) | Test (5-shot) |
---|---|---|---|---|
Llama-3-Chinese-8B-Instruct-v2 | 62.1 | 63.9 | 62.6 | 63.7 |
Llama-3-Chinese-8B-Instruct | 60.1 | 61.3 | 59.8 | 61.8 |
Llama-3-Chinese-8B | 55.5 | 58.5 | 57.3 | 61.1 |
Meta-Llama-3-8B-Instruct | 63.4 | 64.8 | 65.1 | 66.4 |
Meta-Llama-3-8B | 58.6 | 62.5 | 60.5 | 65.0 |
Chinese-Mixtral-Instruct (8x7B) | 65.1 | 69.6 | 67.5 | 69.8 |
Chinese-Mixtral (8x7B) | 63.2 | 67.1 | 65.5 | 68.3 |
Chinese-Alpaca-2-13B | 49.6 | 53.2 | 50.9 | 53.5 |
Chinese-LLaMA-2-13B | 46.8 | 50.0 | 46.6 | 51.8 |
LongBench是一个大模型长文本理解能力的评测基准,由6大类、20个不同的任务组成,多数任务的平均长度在5K-15K之间,共包含约4.75K条测试数据。以下是本项目模型在该中文任务(含代码任务)上的评测效果。LongBench推理代码请参考本项目:📖GitHub Wiki
Models | 单文档QA | 多文档QA | 摘要 | FS学习 | 代码 | 合成 | 平均 |
---|---|---|---|---|---|---|---|
Llama-3-Chinese-8B-Instruct-v2 | 57.3 | 27.1 | 13.9 | 30.3 | 60.6 | 89.5 | 46.4 |
Llama-3-Chinese-8B-Instruct | 44.1 | 24.0 | 12.4 | 33.5 | 51.8 | 11.5 | 29.6 |
Llama-3-Chinese-8B | 16.4 | 19.3 | 4.3 | 28.7 | 14.3 | 4.6 | 14.6 |
Meta-Llama-3-8B-Instruct | 55.1 | 15.1 | 0.1 | 24.0 | 51.3 | 94.5 | 40.0 |
Meta-Llama-3-8B | 21.2 | 22.9 | 2.7 | 35.8 | 65.9 | 40.8 | 31.6 |
Chinese-Mixtral-Instruct (8x7B) | 50.3 | 34.2 | 16.4 | 42.0 | 56.1 | 89.5 | 48.1 |
Chinese-Mixtral (8x7B) | 32.0 | 23.7 | 0.4 | 42.5 | 27.4 | 14.0 | 23.3 |
Chinese-Alpaca-2-13B-16K | 47.9 | 26.7 | 13.0 | 22.3 | 46.6 | 21.5 | 29.7 |
Chinese-LLaMA-2-13B-16K | 36.7 | 17.7 | 3.1 | 29.8 | 13.8 | 3.0 | 17.3 |
Chinese-Alpaca-2-7B-64K | 44.7 | 28.1 | 14.4 | 39.0 | 44.6 | 5.0 | 29.3 |
Chinese-LLaMA-2-7B-64K | 27.2 | 16.4 | 6.5 | 33.0 | 7.8 | 5.0 | 16.0 |
Open LLM Leaderboard是由HuggingFaceH4团队发起的大模型综合能力评测基准(英文),包含ARC、HellaSwag、MMLU、TruthfulQA、Winograde、GSM8K等6个单项测试。以下是本项目模型在该榜单上的评测效果。
Models | ARC | HellaS | MMLU | TQA | WinoG | GSM8K | 平均 |
---|---|---|---|---|---|---|---|
Llama-3-Chinese-8B-Instruct-v2 | 62.63 | 79.72 | 66.48 | 53.93 | 76.72 | 60.58 | 66.68 |
Llama-3-Chinese-8B-Instruct | 61.26 | 80.24 | 63.10 | 55.15 | 75.06 | 44.43 | 63.21 |
Llama-3-Chinese-8B | 55.88 | 79.53 | 63.70 | 41.14 | 77.03 | 37.98 | 59.21 |
Meta-Llama-3-8B-Instruct | 60.75 | 78.55 | 67.07 | 51.65 | 74.51 | 68.69 | 66.87 |
Meta-Llama-3-8B | 59.47 | 82.09 | 66.69 | 43.90 | 77.35 | 45.79 | 62.55 |
Chinese-Mixtral-Instruct (8x7B) | 67.75 | 85.67 | 71.53 | 57.46 | 83.11 | 55.65 | 70.19 |
Chinese-Mixtral (8x7B) | 67.58 | 85.34 | 70.38 | 46.86 | 82.00 | 0.00 | 58.69 |
注:MMLU结果与不同的主要原因是评测脚本不同导致。
在llama.cpp下,测试了Llama-3-Chinese-8B(基座模型)的量化性能,如下表所示。实测速度相比二代Llama-2-7B略慢。
F16 | Q8_0 | Q6_K | Q5_K | Q5_0 | Q4_K | Q4_0 | Q3_K | Q2_K | |
---|---|---|---|---|---|---|---|---|---|
Size (GB) | 14.97 | 7.95 | 6.14 | 5.34 | 5.21 | 4.58 | 4.34 | 3.74 | 2.96 |
BPW | 16.00 | 8.50 | 6.56 | 5.70 | 5.57 | 4.89 | 4.64 | 4.00 | 3.16 |
PPL | 5.130 | 5.135 | 5.148 | 5.181 | 5.222 | 5.312 | 5.549 | 5.755 | 11.859 |
PP Speed | 5.99 | 6.10 | 7.17 | 7.34 | 6.65 | 6.38 | 6.00 | 6.85 | 6.43 |
TG Speed | 44.03 | 26.08 | 21.61 | 22.33 | 20.93 | 18.93 | 17.09 | 22.50 | 19.21 |
[!NOTE]
- 模型大小:单位GB
- BPW(Bits-Per-Weight):单位参数比特,例如Q8_0实际平均精度为8.50
- PPL(困惑度):以8K上下文测量(原生支持长度),数值越低越好
- PP/TG速度:提供了Apple M3 Max(Metal)的指令处理(PP)和文本生成(TG)速度,单位ms/token,数值越低越快
- 使用无标注数据进行预训练:📖预训练脚本Wiki
- 使用有标注数据进行指令精调:📖指令精调脚本Wiki
本项目Llama-3-Chinese-Instruct沿用原版Llama-3-Instruct的指令模板。以下是一组对话示例:
<|begin_of_text|><|start_header_id|>system<|end_header_id|>
You are a helpful assistant. 你是一个乐于助人的助手。<|eot_id|><|start_header_id|>user<|end_header_id|>
你好<|eot_id|><|start_header_id|>assistant<|end_header_id|>
你好!有什么可以帮助你的吗?<|eot_id|>
以下是本项目开源的部分指令数据。详情请查看:📚 指令数据
数据名称 | 说明 | 数量 |
---|---|---|
alpaca_zh_51k | 使用gpt-3.5翻译的Alpaca数据 | 51K |
stem_zh_instruction | 使用gpt-3.5爬取的STEM数据,包含物理、化学、医学、生物学、地球科学 | 256K |
ruozhiba_gpt4 | 使用GPT-4o和GPT-4T获取的ruozhiba问答数据 | 2449 |
请在提交Issue前务必先查看FAQ中是否已存在解决方案。具体问题和解答请参考本项目 📖GitHub Wiki
问题1:为什么没有像一期、二期项目一样做词表扩充?
问题2:会有70B版本发布吗?
问题3:为什么指令模型不叫Alpaca了?
问题4:本仓库模型能否商用?
问题5:为什么不对模型做全量预训练而是用LoRA?
问题6:为什么Llama-3-Chinese对话效果不好?
问题7:为什么指令模型会回复说自己是ChatGPT?
问题8:Instrcut模型的v1(原版)和v2有什么区别?
本项目基于由Meta发布的Llama-3模型进行开发,使用过程中请严格遵守Llama-3的开源许可协议。如果涉及使用第三方代码,请务必遵从相关的开源许可协议。模型生成的内容可能会因为计算方法、随机因素以及量化精度损失等影响其准确性,因此,本项目不对模型输出的准确性提供任何保证,也不会对任何因使用相关资源和输出结果产生的损失承担责任。如果将本项目的相关模型用于商业用途,开发者应遵守当地的法律法规,确保模型输出内容的合规性,本项目不对任何由此衍生的产品或服务承担责任。
如有疑问,请在GitHub Issue中提交。礼貌地提出问题,构建和谐的讨论社区。
- 在提交问题之前,请先查看FAQ能否解决问题,同时建议查阅以往的issue是否能解决你的问题。
- 提交问题请使用本项目设置的Issue模板,以帮助快速定位具体问题。
- 重复以及与本项目无关的issue会被stable-bot处理,敬请谅解。
For Tasks:
Click tags to check more tools for each tasksFor Jobs:
Alternative AI tools for Chinese-LLaMA-Alpaca-3
Similar Open Source Tools
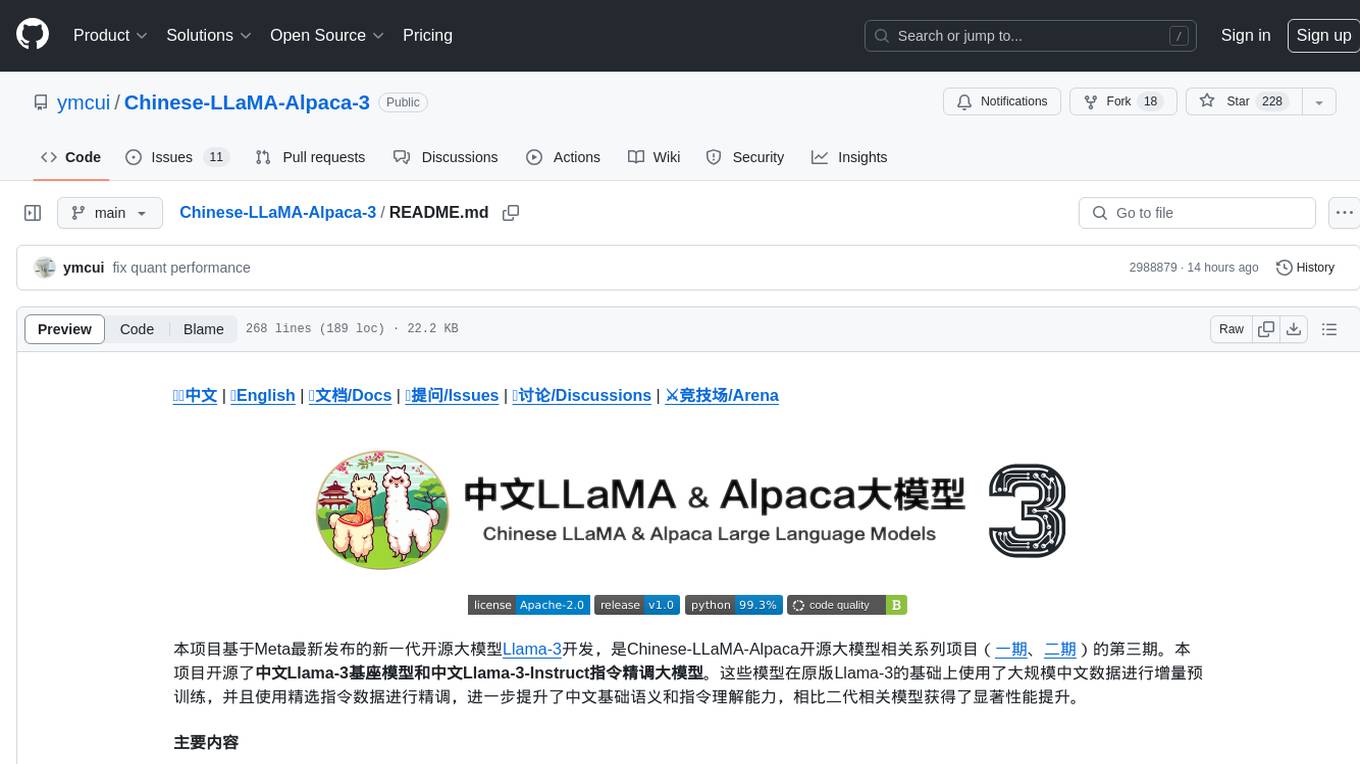
Chinese-LLaMA-Alpaca-3
Chinese-LLaMA-Alpaca-3 is a project based on Meta's latest release of the new generation open-source large model Llama-3. It is the third phase of the Chinese-LLaMA-Alpaca open-source large model series projects (Phase 1, Phase 2). This project open-sources the Chinese Llama-3 base model and the Chinese Llama-3-Instruct instruction fine-tuned large model. These models incrementally pre-train with a large amount of Chinese data on the basis of the original Llama-3 and further fine-tune using selected instruction data, enhancing Chinese basic semantics and instruction understanding capabilities. Compared to the second-generation related models, significant performance improvements have been achieved.
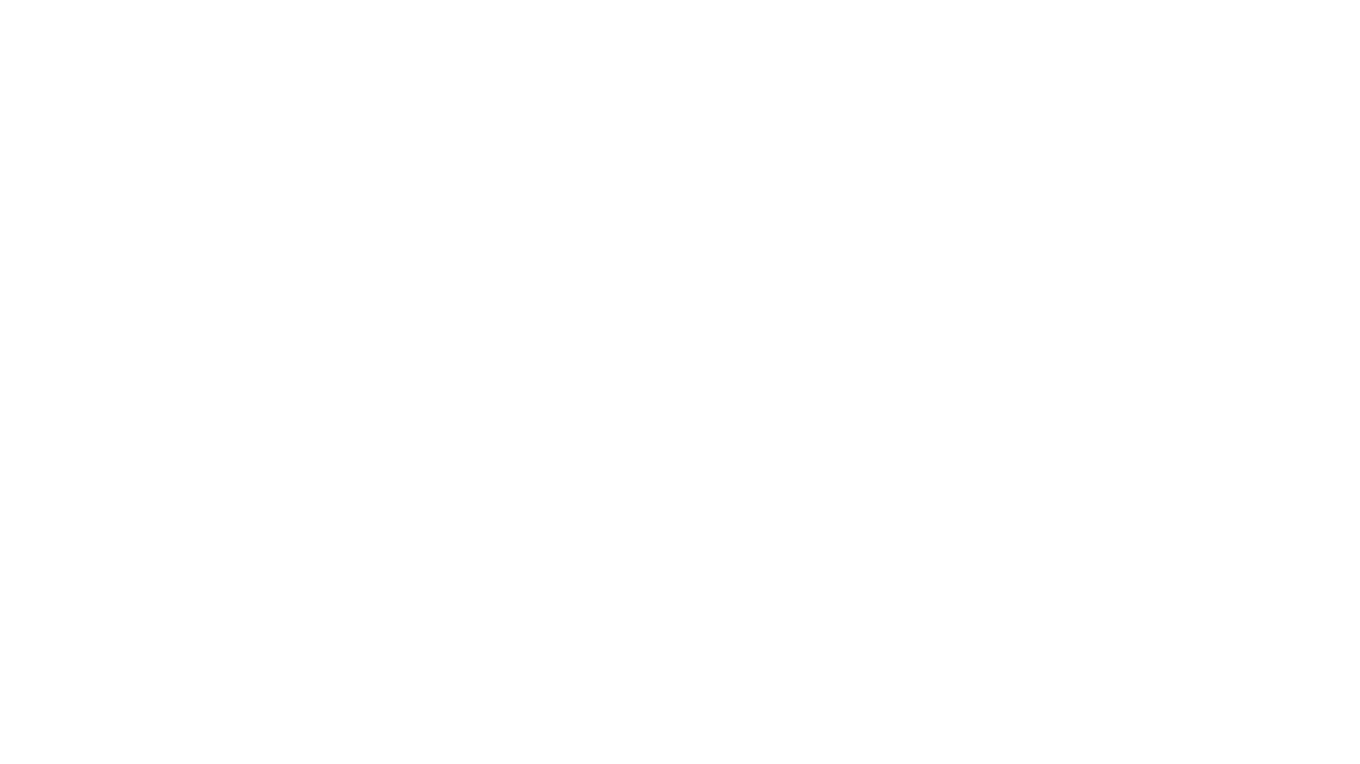
Chinese-LLaMA-Alpaca-2
Chinese-LLaMA-Alpaca-2 is a large Chinese language model developed by Meta AI. It is based on the Llama-2 model and has been further trained on a large dataset of Chinese text. Chinese-LLaMA-Alpaca-2 can be used for a variety of natural language processing tasks, including text generation, question answering, and machine translation. Here are some of the key features of Chinese-LLaMA-Alpaca-2: * It is the largest Chinese language model ever trained, with 13 billion parameters. * It is trained on a massive dataset of Chinese text, including books, news articles, and social media posts. * It can be used for a variety of natural language processing tasks, including text generation, question answering, and machine translation. * It is open-source and available for anyone to use. Chinese-LLaMA-Alpaca-2 is a powerful tool that can be used to improve the performance of a wide range of natural language processing tasks. It is a valuable resource for researchers and developers working in the field of artificial intelligence.
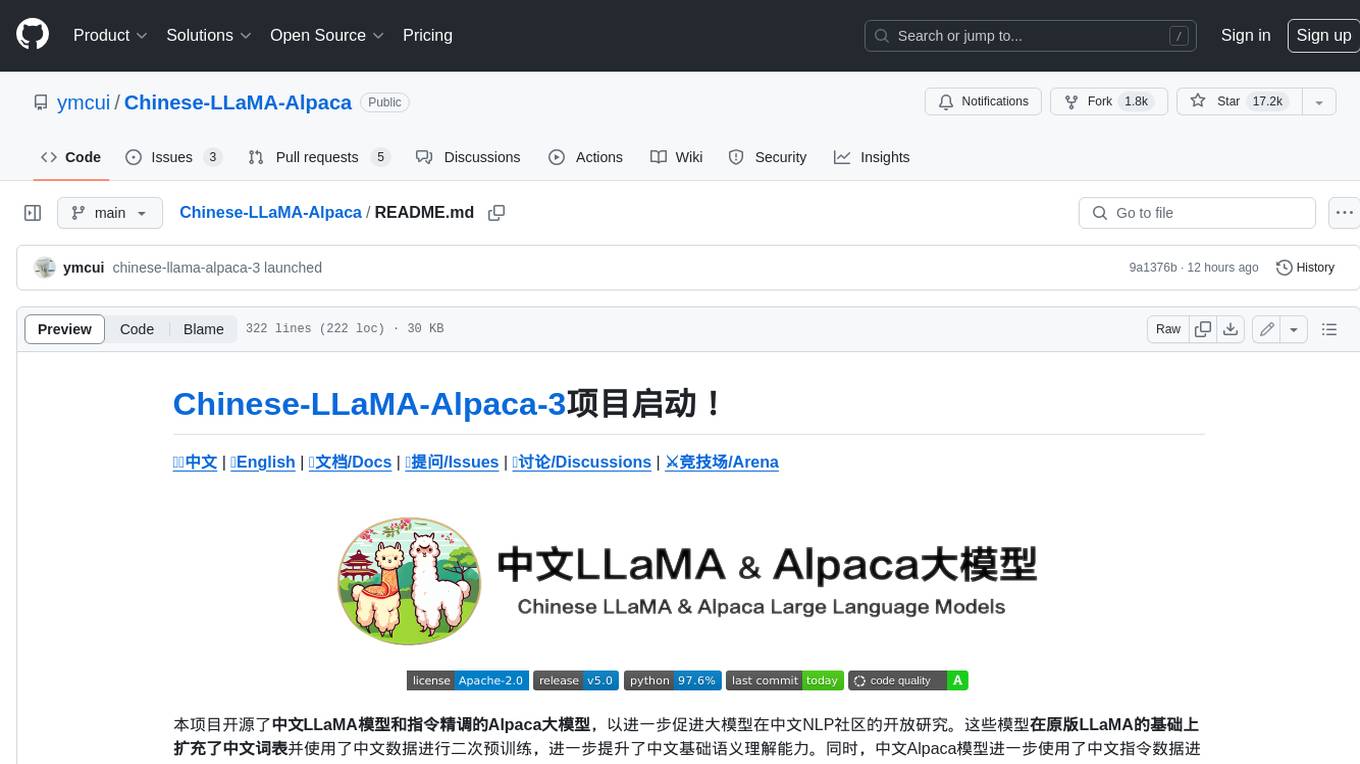
Chinese-LLaMA-Alpaca
This project open sources the **Chinese LLaMA model and the Alpaca large model fine-tuned with instructions**, to further promote the open research of large models in the Chinese NLP community. These models **extend the Chinese vocabulary based on the original LLaMA** and use Chinese data for secondary pre-training, further enhancing the basic Chinese semantic understanding ability. At the same time, the Chinese Alpaca model further uses Chinese instruction data for fine-tuning, significantly improving the model's understanding and execution of instructions.
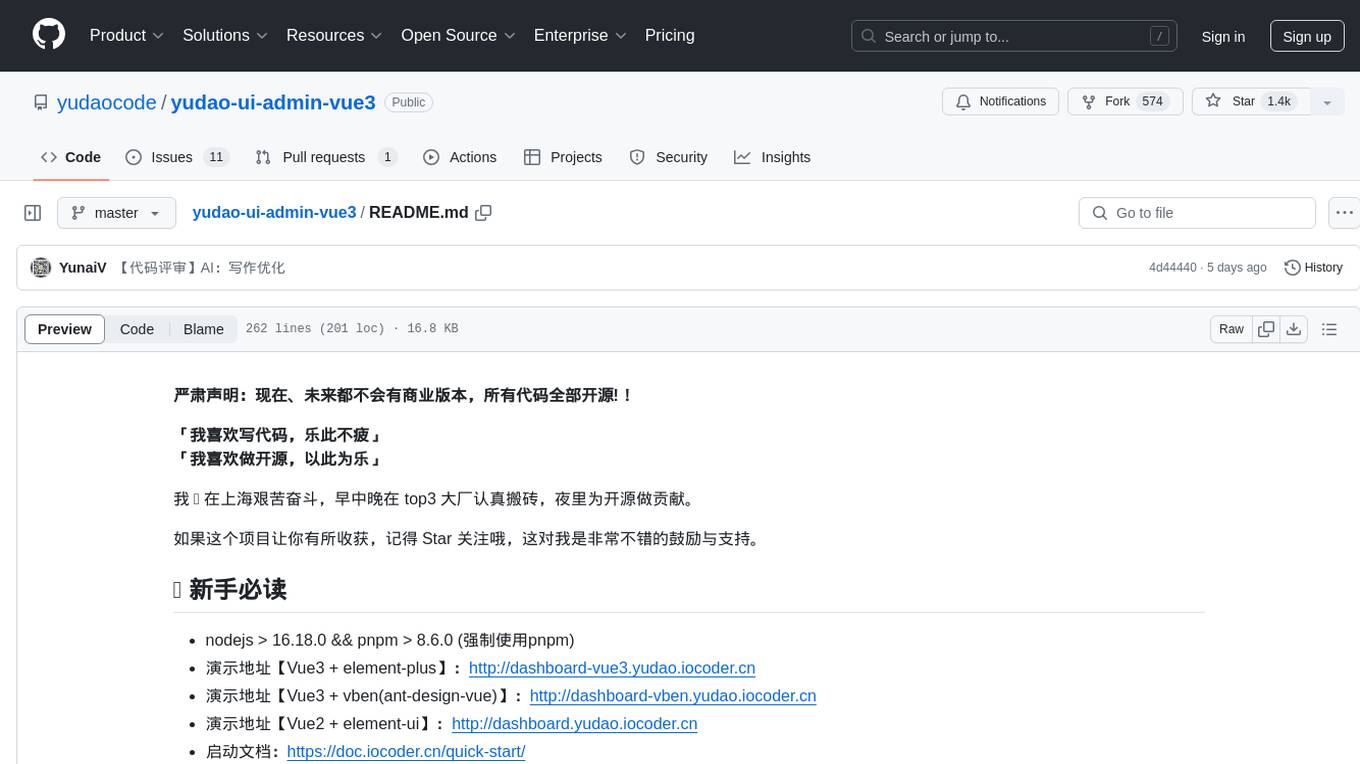
yudao-ui-admin-vue3
The yudao-ui-admin-vue3 repository is an open-source project focused on building a fast development platform for developers in China. It utilizes Vue3 and Element Plus to provide features such as configurable themes, internationalization, dynamic route permission generation, common component encapsulation, and rich examples. The project supports the latest front-end technologies like Vue3 and Vite4, and also includes tools like TypeScript, pinia, vueuse, vue-i18n, vue-router, unocss, iconify, and wangeditor. It offers a range of development tools and features for system functions, infrastructure, workflow management, payment systems, member centers, data reporting, e-commerce systems, WeChat public accounts, ERP systems, and CRM systems.
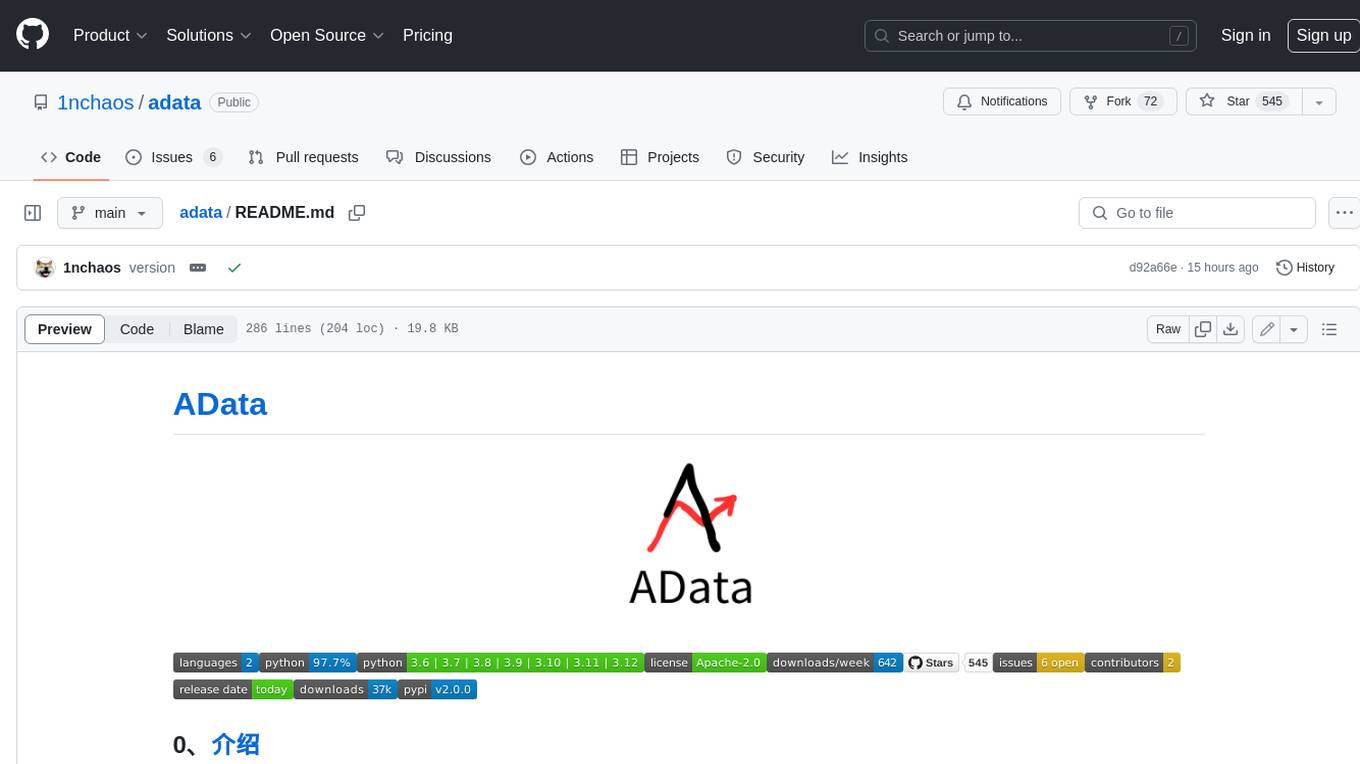
adata
AData is a free and open-source A-share database that focuses on transaction-related data. It provides comprehensive data on stocks, including basic information, market data, and sentiment analysis. AData is designed to be easy to use and integrate with other applications, making it a valuable tool for quantitative trading and AI training.
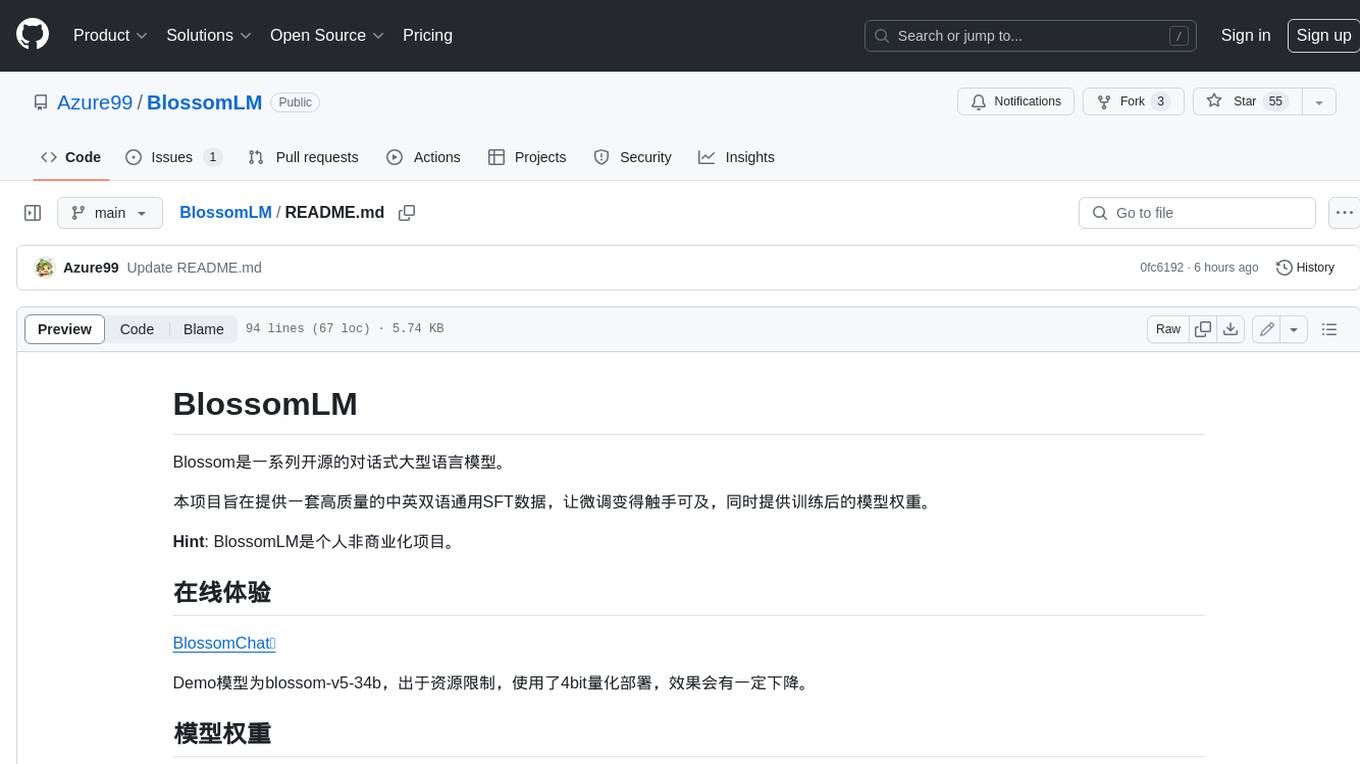
BlossomLM
BlossomLM is a series of open-source conversational large language models. This project aims to provide a high-quality general-purpose SFT dataset in both Chinese and English, making fine-tuning accessible while also providing pre-trained model weights. **Hint**: BlossomLM is a personal non-commercial project.
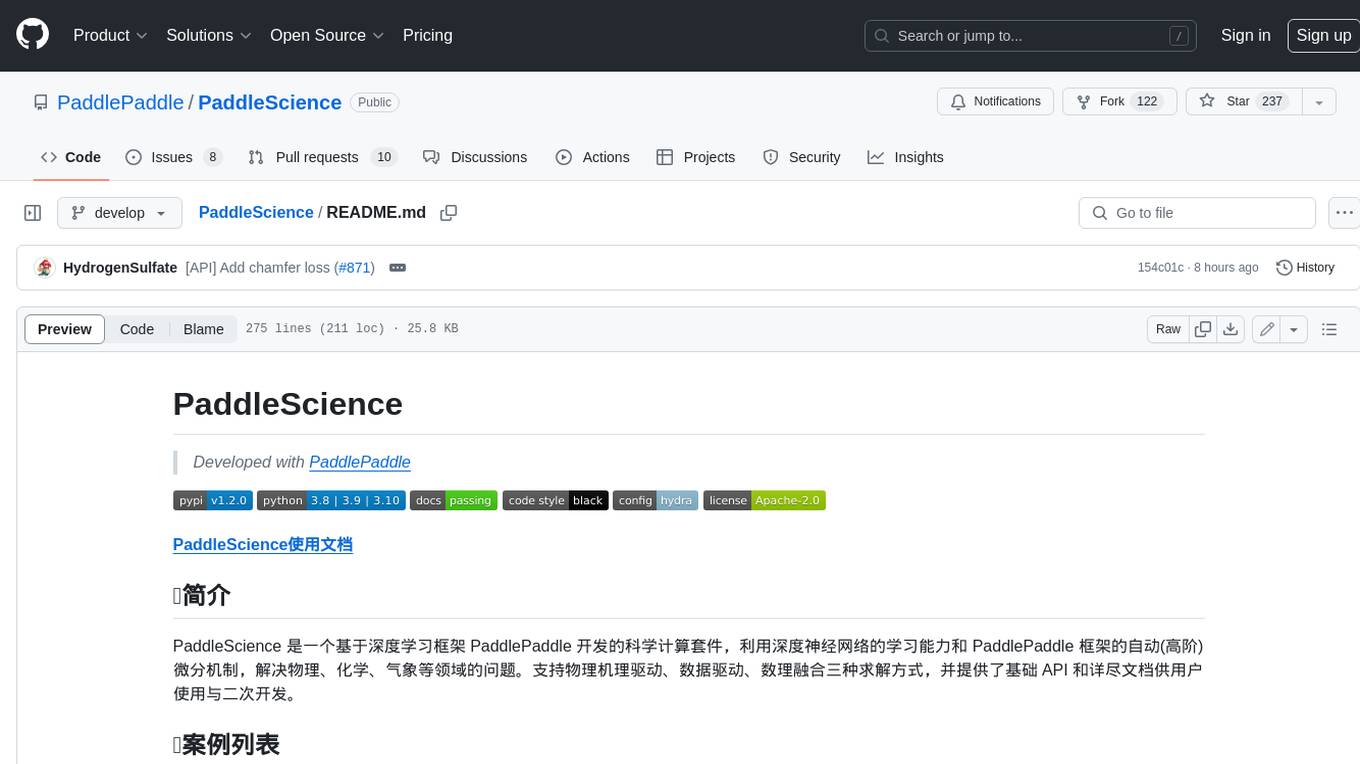
PaddleScience
PaddleScience is a scientific computing suite developed based on the deep learning framework PaddlePaddle. It utilizes the learning ability of deep neural networks and the automatic (higher-order) differentiation mechanism of PaddlePaddle to solve problems in physics, chemistry, meteorology, and other fields. It supports three solving methods: physics mechanism-driven, data-driven, and mathematical fusion, and provides basic APIs and detailed documentation for users to use and further develop.
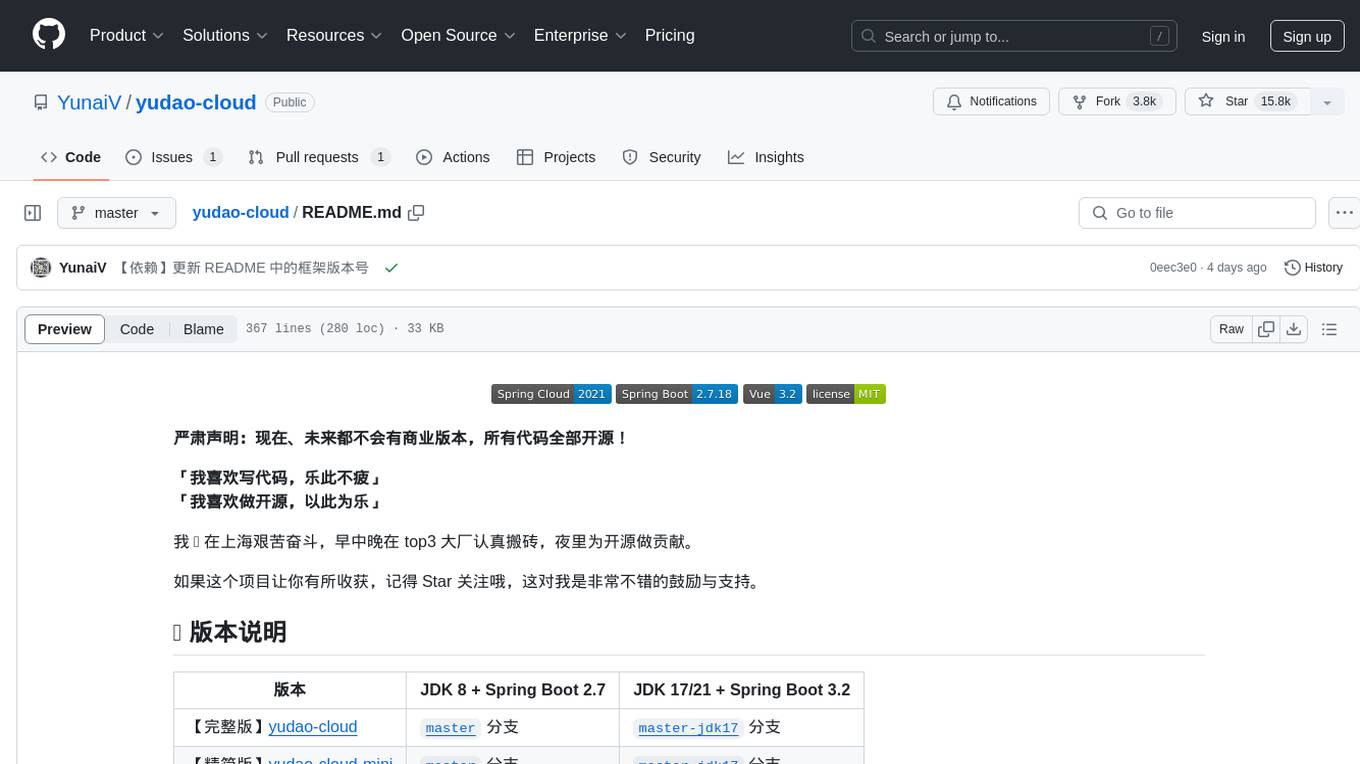
yudao-cloud
Yudao-cloud is an open-source project designed to provide a fast development platform for developers in China. It includes various system functions, infrastructure, member center, data reports, workflow, mall system, WeChat public account, CRM, ERP, etc. The project is based on Java backend with Spring Boot and Spring Cloud Alibaba microservices architecture. It supports multiple databases, message queues, authentication systems, dynamic menu loading, SaaS multi-tenant system, code generator, real-time communication, integration with third-party services like WeChat, Alipay, and more. The project is well-documented and follows the Alibaba Java development guidelines, ensuring clean code and architecture.
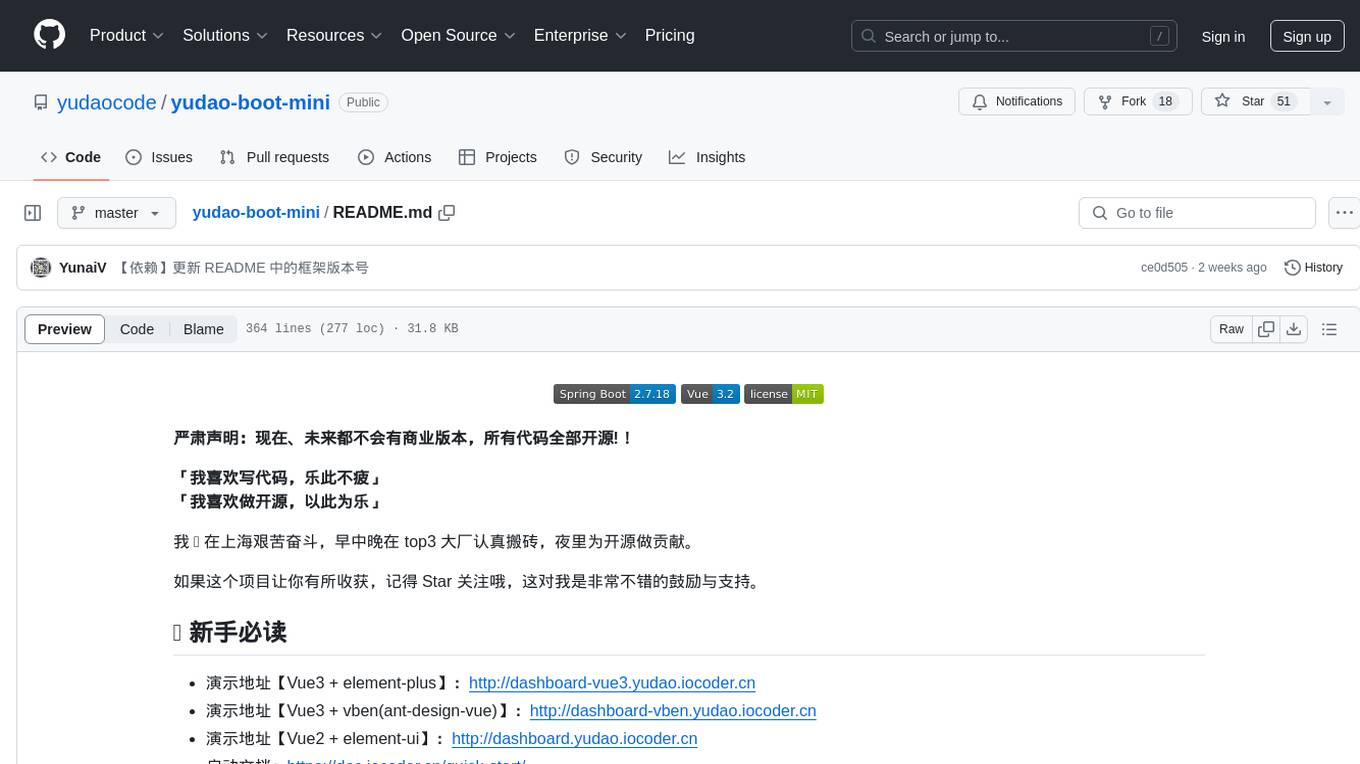
yudao-boot-mini
yudao-boot-mini is an open-source project focused on developing a rapid development platform for developers in China. It includes features like system functions, infrastructure, member center, data reports, workflow, mall system, WeChat official account, CRM, ERP, etc. The project is based on Spring Boot with Java backend and Vue for frontend. It offers various functionalities such as user management, role management, menu management, department management, workflow management, payment system, code generation, API documentation, database documentation, file service, WebSocket integration, message queue, Java monitoring, and more. The project is licensed under the MIT License, allowing both individuals and enterprises to use it freely without restrictions.
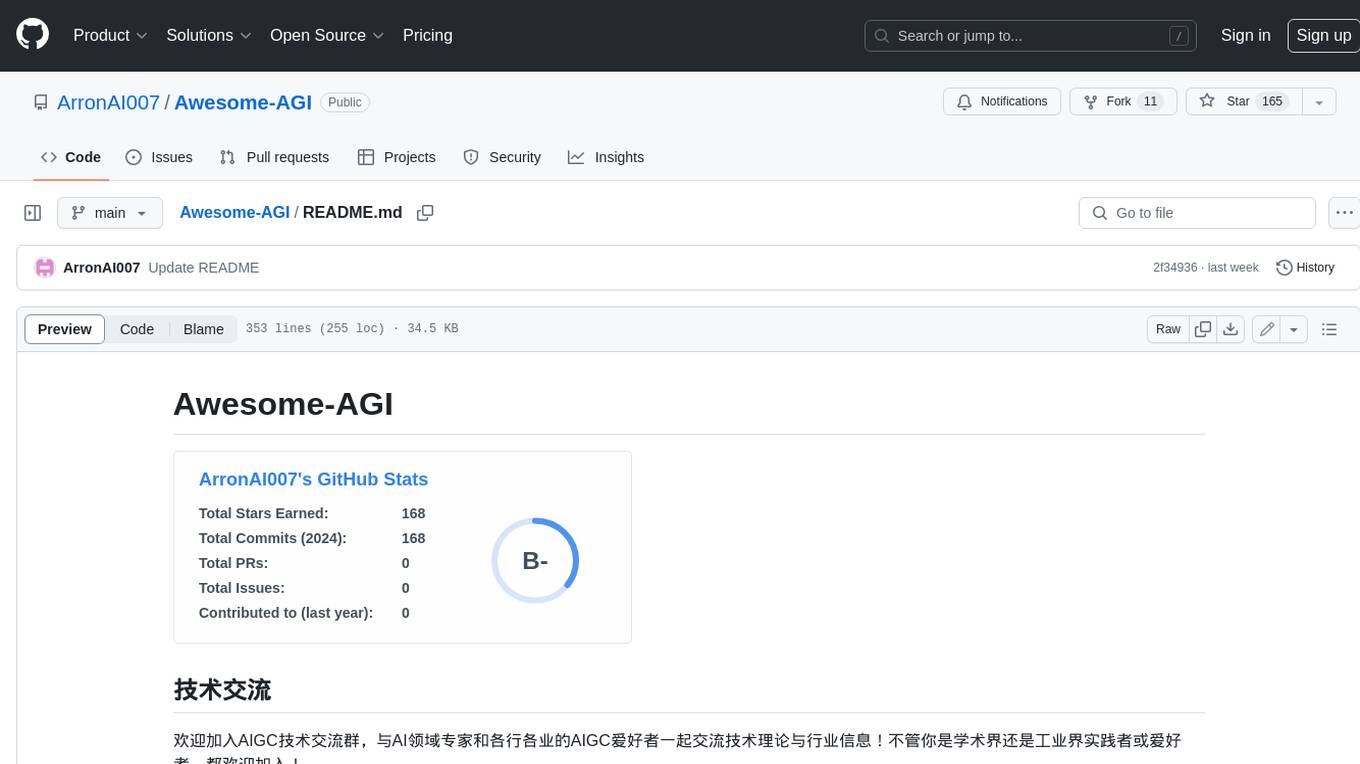
Awesome-AGI
Awesome-AGI is a curated list of resources related to Artificial General Intelligence (AGI), including models, pipelines, applications, and concepts. It provides a comprehensive overview of the current state of AGI research and development, covering various aspects such as model training, fine-tuning, deployment, and applications in different domains. The repository also includes resources on prompt engineering, RLHF, LLM vocabulary expansion, long text generation, hallucination mitigation, controllability and safety, and text detection. It serves as a valuable resource for researchers, practitioners, and anyone interested in the field of AGI.
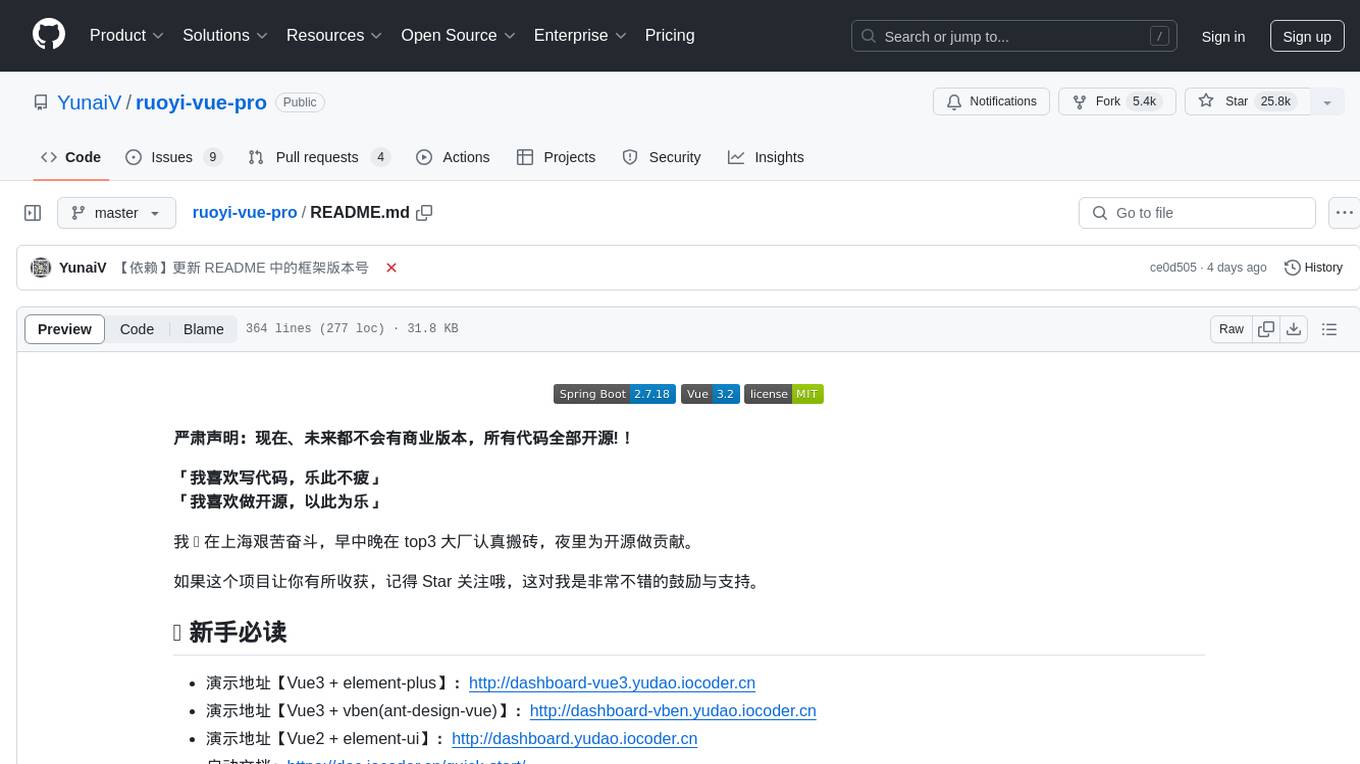
ruoyi-vue-pro
The ruoyi-vue-pro repository is an open-source project that provides a comprehensive development platform with various functionalities such as system features, infrastructure, member center, data reports, workflow, payment system, mall system, ERP system, CRM system, and AI big model. It is built using Java backend with Spring Boot framework and Vue frontend with different versions like Vue3 with element-plus, Vue3 with vben(ant-design-vue), and Vue2 with element-ui. The project aims to offer a fast development platform for developers and enterprises, supporting features like dynamic menu loading, button-level access control, SaaS multi-tenancy, code generator, real-time communication, integration with third-party services like WeChat, Alipay, and cloud services, and more.
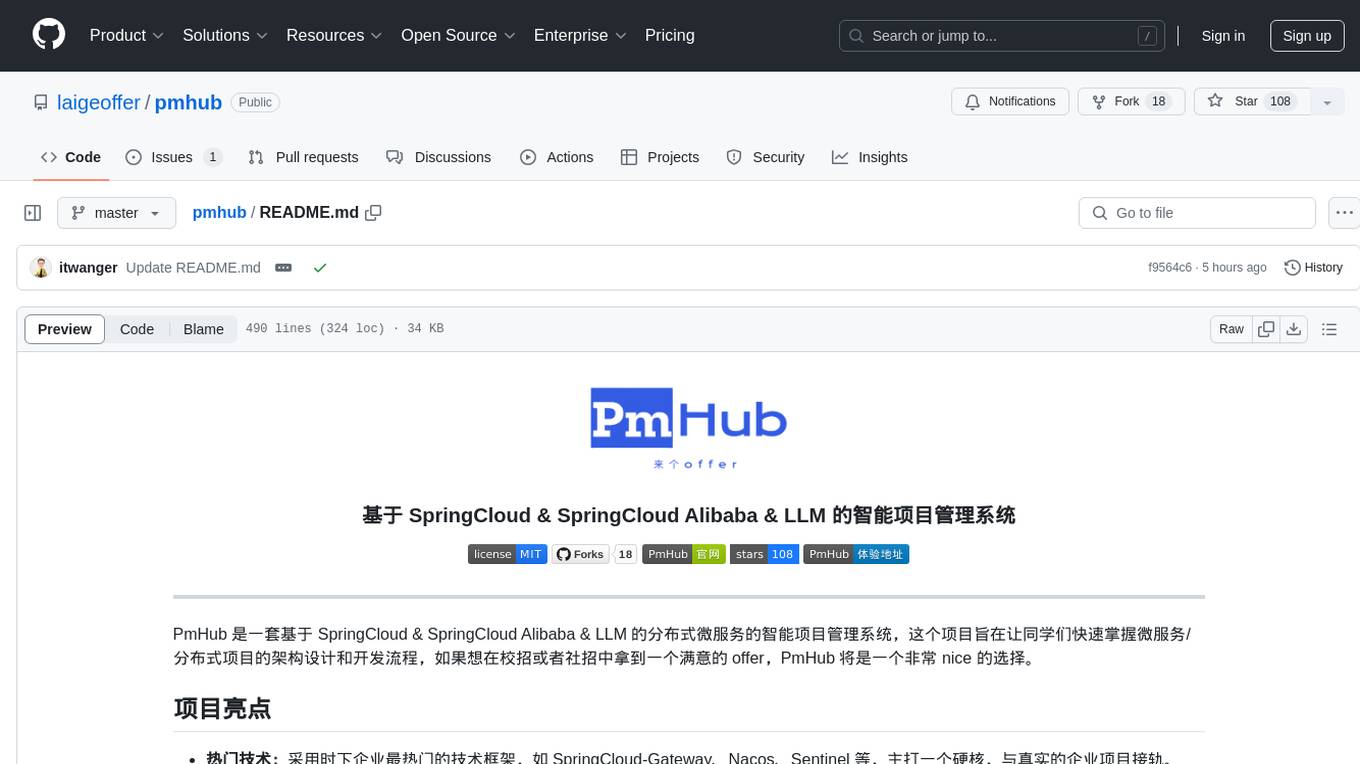
pmhub
PmHub is a smart project management system based on SpringCloud, SpringCloud Alibaba, and LLM. It aims to help students quickly grasp the architecture design and development process of microservices/distributed projects. PmHub provides a platform for students to experience the transformation from monolithic to microservices architecture, understand the pros and cons of both architectures, and prepare for job interviews. It offers popular technologies like SpringCloud-Gateway, Nacos, Sentinel, and provides high-quality code, continuous integration, product design documents, and an enterprise workflow system. PmHub is suitable for beginners and advanced learners who want to master core knowledge of microservices/distributed projects.
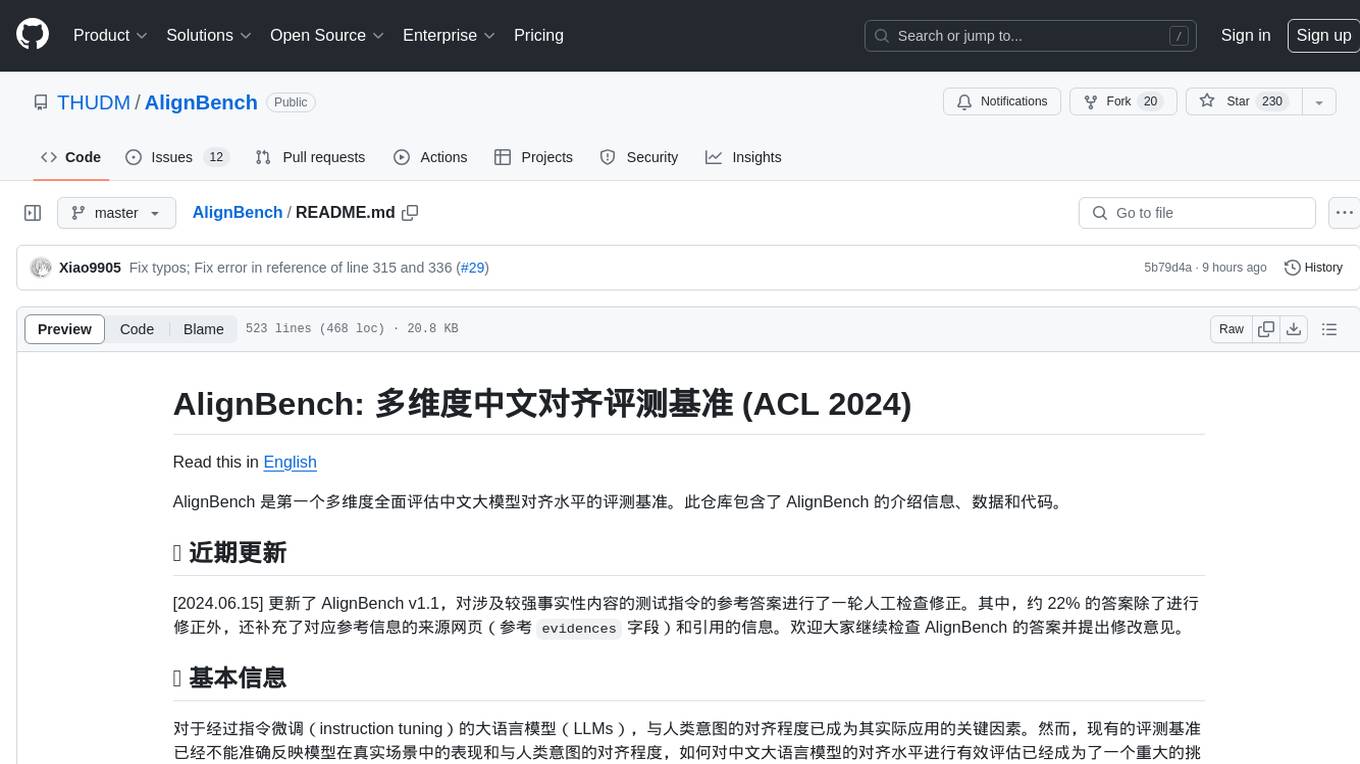
AlignBench
AlignBench is the first comprehensive evaluation benchmark for assessing the alignment level of Chinese large models across multiple dimensions. It includes introduction information, data, and code related to AlignBench. The benchmark aims to evaluate the alignment performance of Chinese large language models through a multi-dimensional and rule-calibrated evaluation method, enhancing reliability and interpretability.
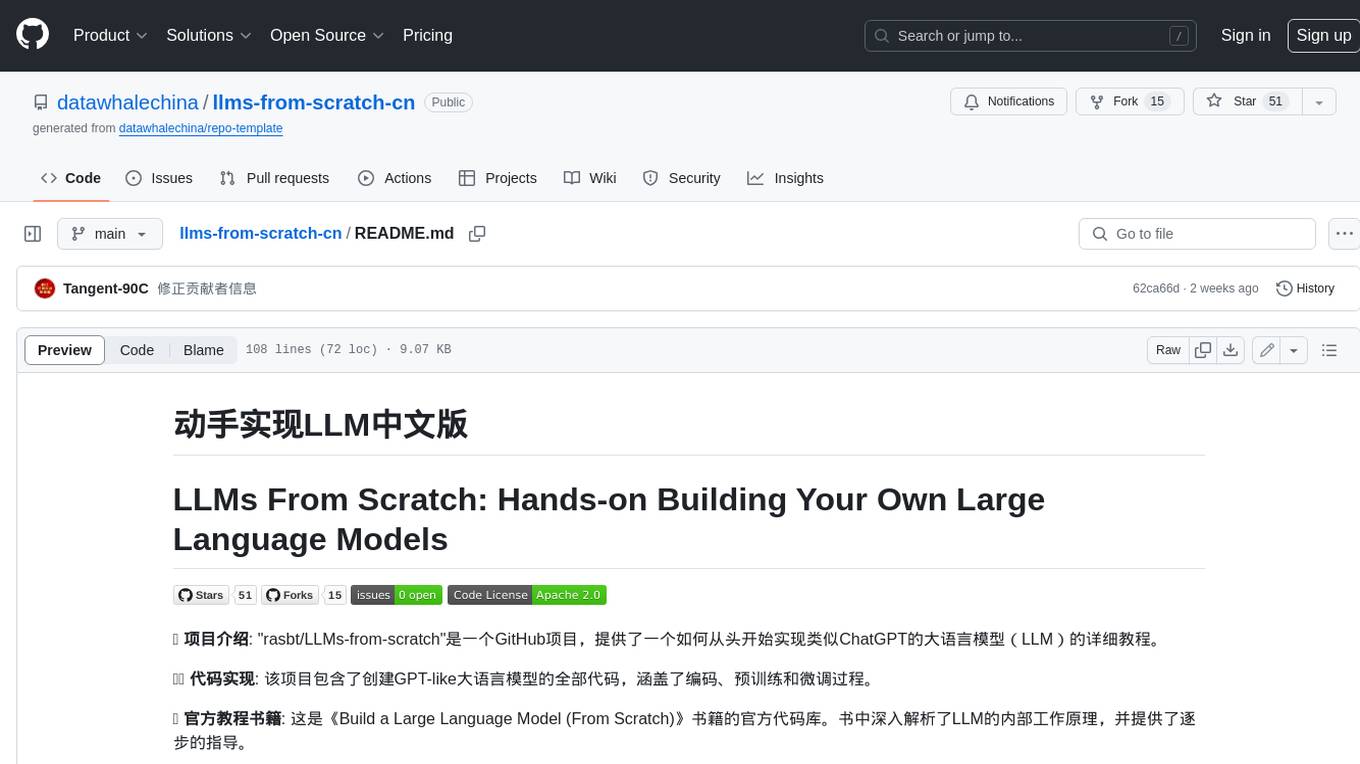
llms-from-scratch-cn
This repository provides a detailed tutorial on how to build your own large language model (LLM) from scratch. It includes all the code necessary to create a GPT-like LLM, covering the encoding, pre-training, and fine-tuning processes. The tutorial is written in a clear and concise style, with plenty of examples and illustrations to help you understand the concepts involved. It is suitable for developers and researchers with some programming experience who are interested in learning more about LLMs and how to build them.
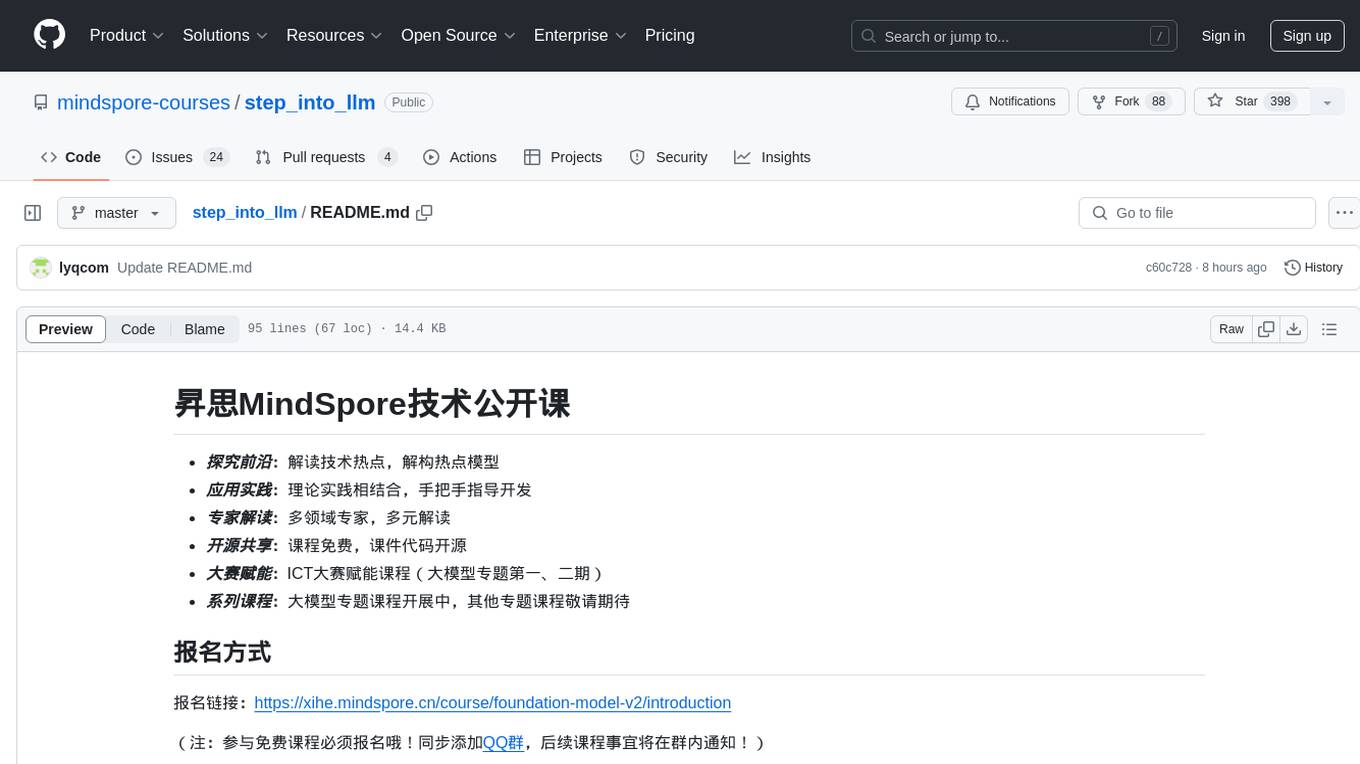
step_into_llm
The 'step_into_llm' repository is dedicated to the 昇思MindSpore technology open class, which focuses on exploring cutting-edge technologies, combining theory with practical applications, expert interpretations, open sharing, and empowering competitions. The repository contains course materials, including slides and code, for the ongoing second phase of the course. It covers various topics related to large language models (LLMs) such as Transformer, BERT, GPT, GPT2, and more. The course aims to guide developers interested in LLMs from theory to practical implementation, with a special emphasis on the development and application of large models.
For similar tasks
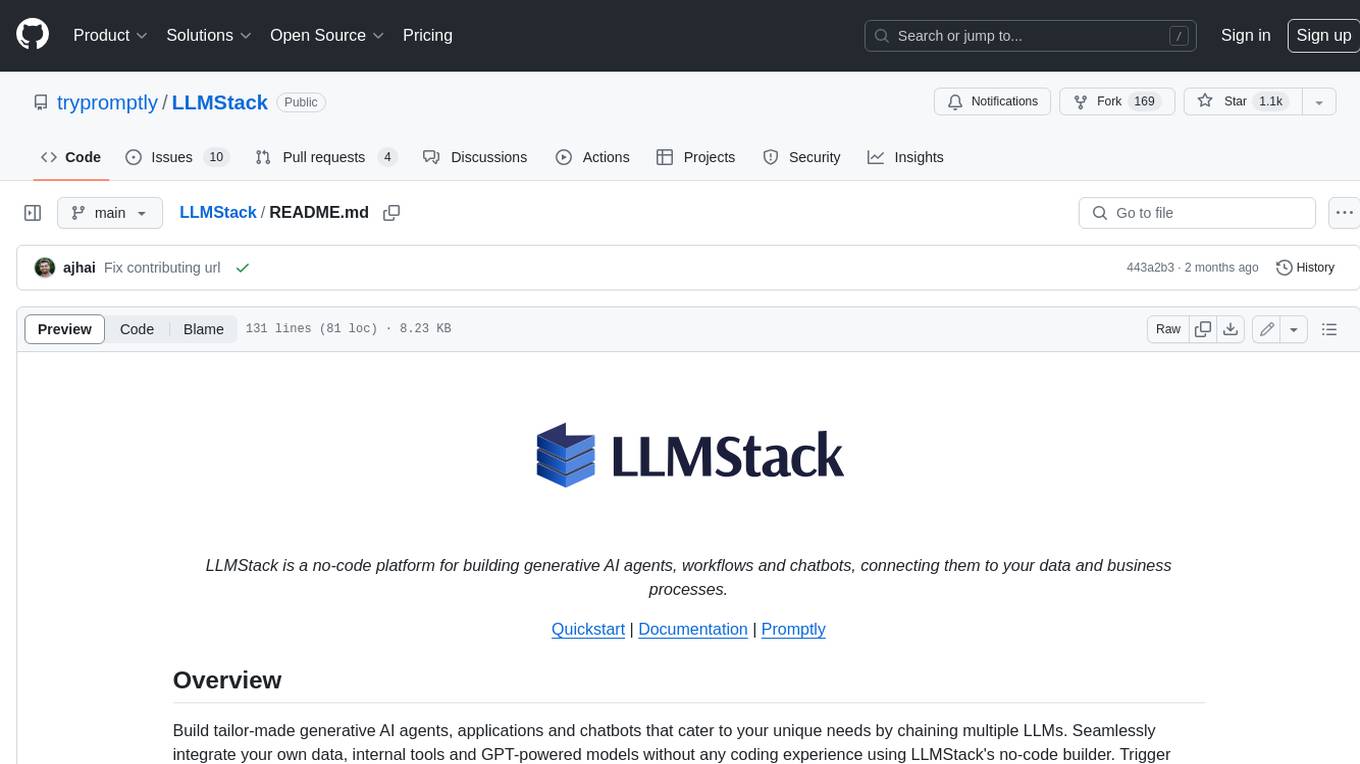
LLMStack
LLMStack is a no-code platform for building generative AI agents, workflows, and chatbots. It allows users to connect their own data, internal tools, and GPT-powered models without any coding experience. LLMStack can be deployed to the cloud or on-premise and can be accessed via HTTP API or triggered from Slack or Discord.
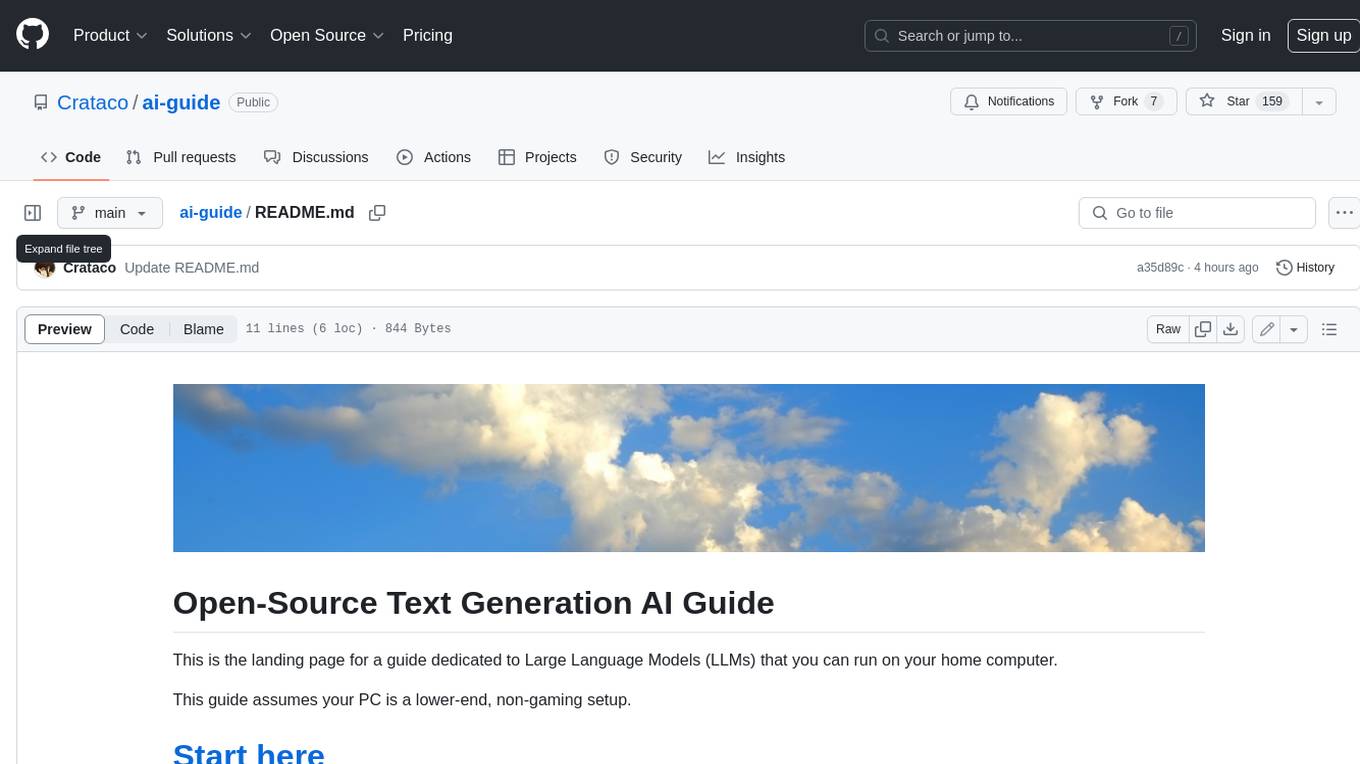
ai-guide
This guide is dedicated to Large Language Models (LLMs) that you can run on your home computer. It assumes your PC is a lower-end, non-gaming setup.
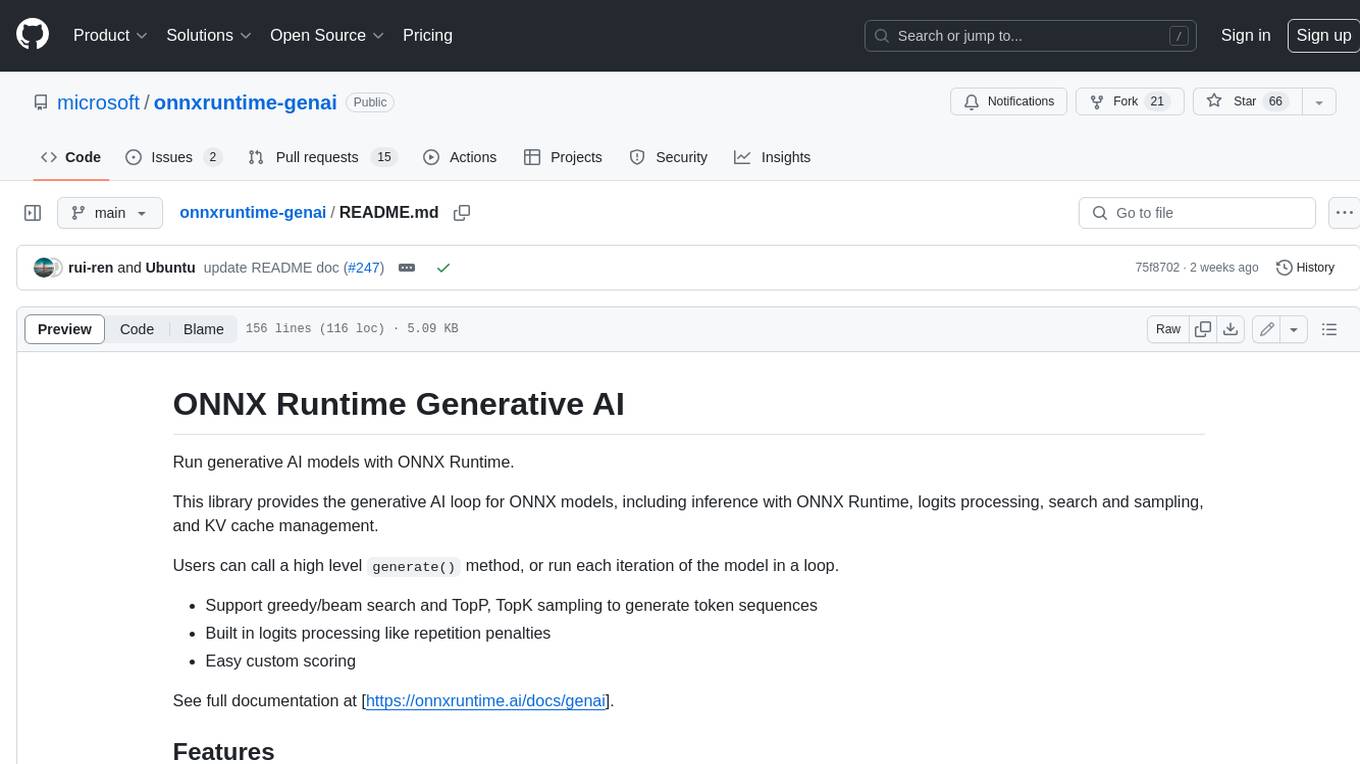
onnxruntime-genai
ONNX Runtime Generative AI is a library that provides the generative AI loop for ONNX models, including inference with ONNX Runtime, logits processing, search and sampling, and KV cache management. Users can call a high level `generate()` method, or run each iteration of the model in a loop. It supports greedy/beam search and TopP, TopK sampling to generate token sequences, has built in logits processing like repetition penalties, and allows for easy custom scoring.
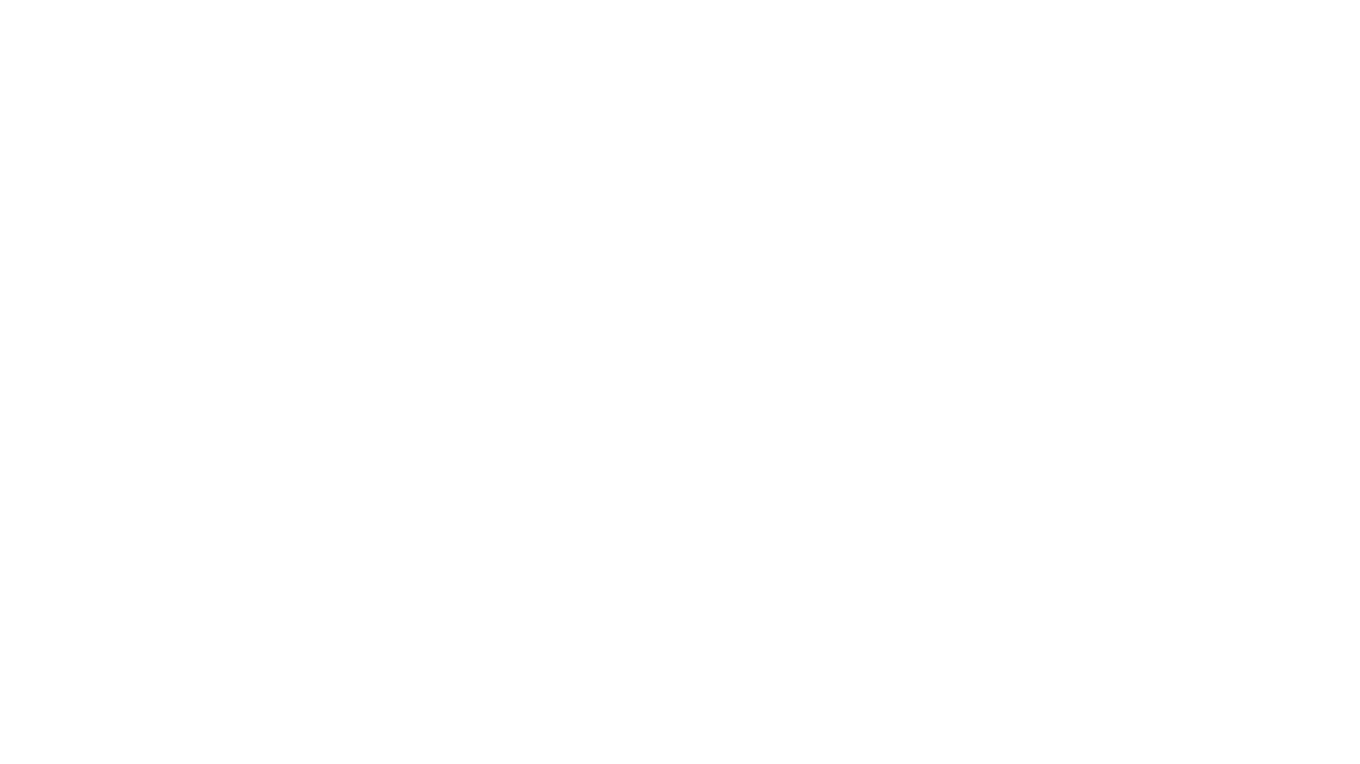
jupyter-ai
Jupyter AI connects generative AI with Jupyter notebooks. It provides a user-friendly and powerful way to explore generative AI models in notebooks and improve your productivity in JupyterLab and the Jupyter Notebook. Specifically, Jupyter AI offers: * An `%%ai` magic that turns the Jupyter notebook into a reproducible generative AI playground. This works anywhere the IPython kernel runs (JupyterLab, Jupyter Notebook, Google Colab, Kaggle, VSCode, etc.). * A native chat UI in JupyterLab that enables you to work with generative AI as a conversational assistant. * Support for a wide range of generative model providers, including AI21, Anthropic, AWS, Cohere, Gemini, Hugging Face, NVIDIA, and OpenAI. * Local model support through GPT4All, enabling use of generative AI models on consumer grade machines with ease and privacy.
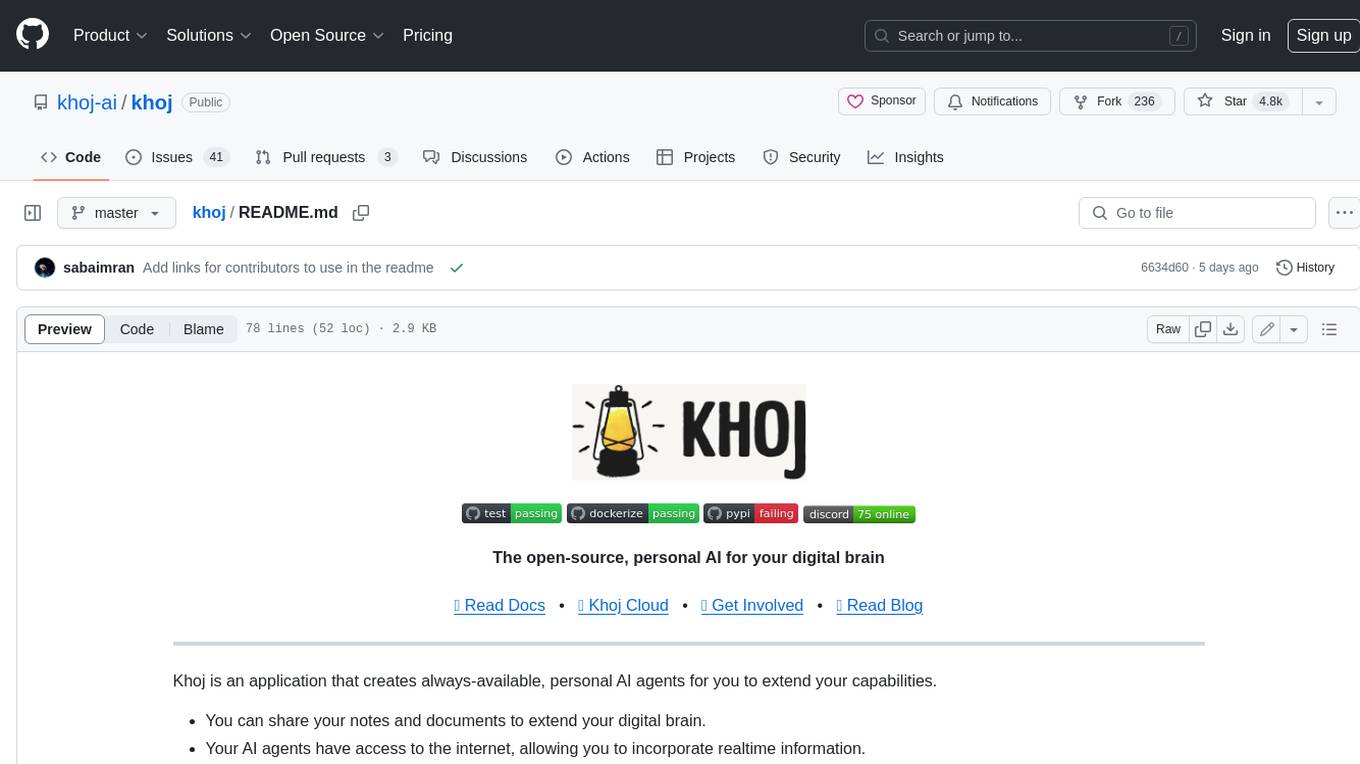
khoj
Khoj is an open-source, personal AI assistant that extends your capabilities by creating always-available AI agents. You can share your notes and documents to extend your digital brain, and your AI agents have access to the internet, allowing you to incorporate real-time information. Khoj is accessible on Desktop, Emacs, Obsidian, Web, and Whatsapp, and you can share PDF, markdown, org-mode, notion files, and GitHub repositories. You'll get fast, accurate semantic search on top of your docs, and your agents can create deeply personal images and understand your speech. Khoj is self-hostable and always will be.
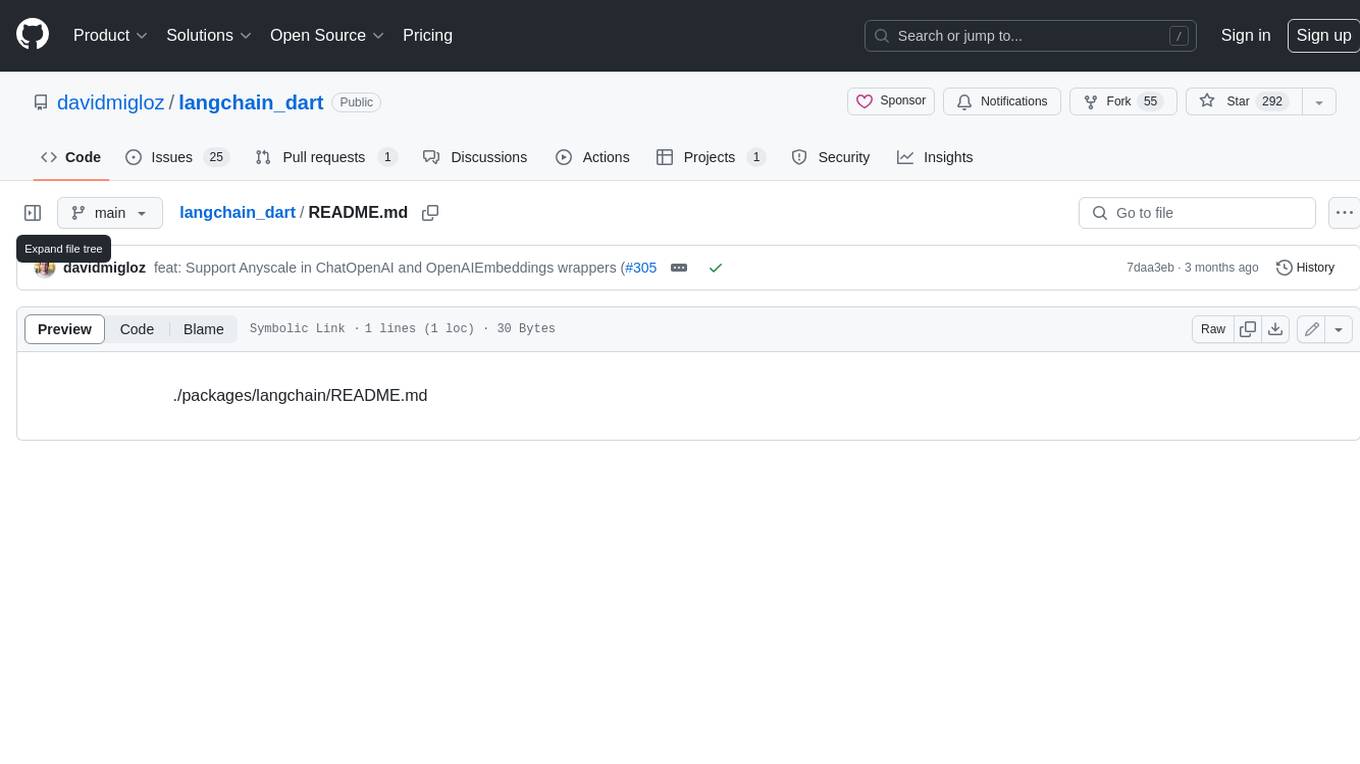
langchain_dart
LangChain.dart is a Dart port of the popular LangChain Python framework created by Harrison Chase. LangChain provides a set of ready-to-use components for working with language models and a standard interface for chaining them together to formulate more advanced use cases (e.g. chatbots, Q&A with RAG, agents, summarization, extraction, etc.). The components can be grouped into a few core modules: * **Model I/O:** LangChain offers a unified API for interacting with various LLM providers (e.g. OpenAI, Google, Mistral, Ollama, etc.), allowing developers to switch between them with ease. Additionally, it provides tools for managing model inputs (prompt templates and example selectors) and parsing the resulting model outputs (output parsers). * **Retrieval:** assists in loading user data (via document loaders), transforming it (with text splitters), extracting its meaning (using embedding models), storing (in vector stores) and retrieving it (through retrievers) so that it can be used to ground the model's responses (i.e. Retrieval-Augmented Generation or RAG). * **Agents:** "bots" that leverage LLMs to make informed decisions about which available tools (such as web search, calculators, database lookup, etc.) to use to accomplish the designated task. The different components can be composed together using the LangChain Expression Language (LCEL).
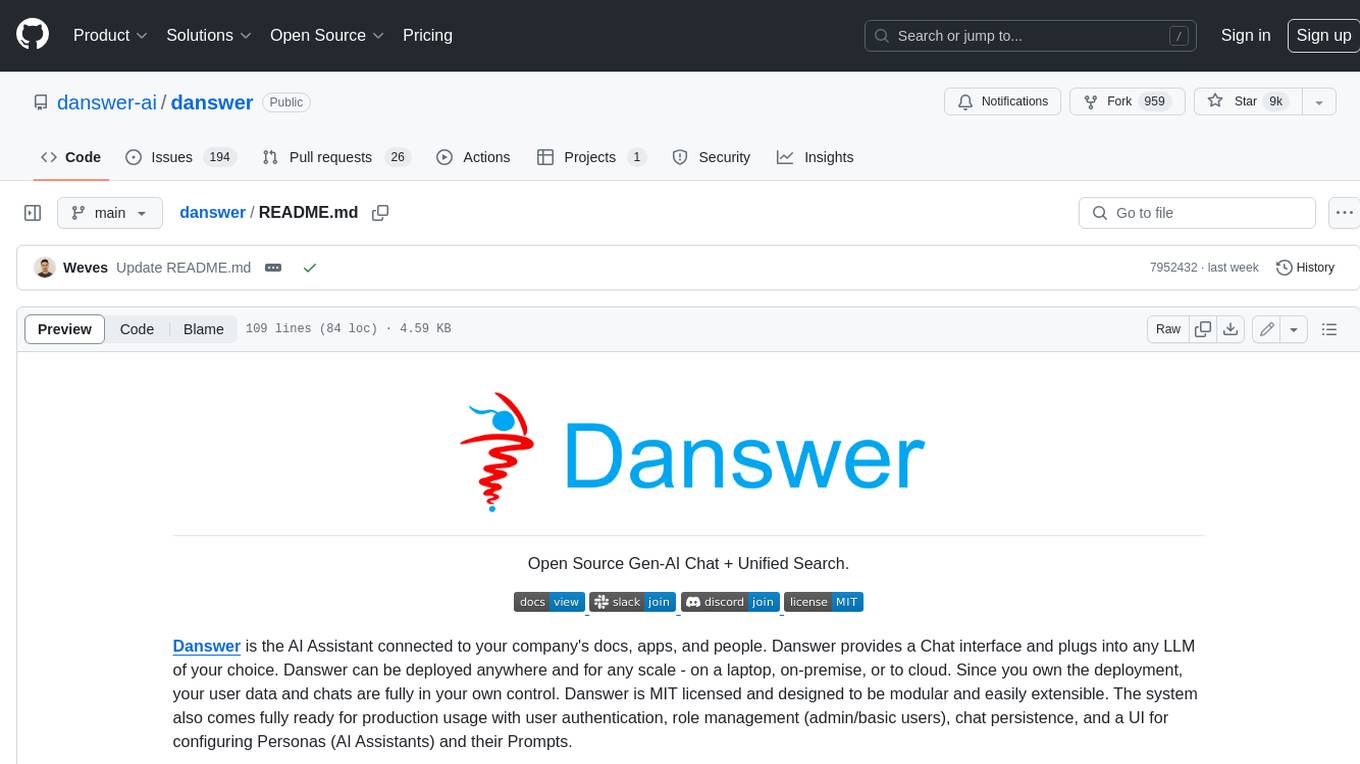
danswer
Danswer is an open-source Gen-AI Chat and Unified Search tool that connects to your company's docs, apps, and people. It provides a Chat interface and plugs into any LLM of your choice. Danswer can be deployed anywhere and for any scale - on a laptop, on-premise, or to cloud. Since you own the deployment, your user data and chats are fully in your own control. Danswer is MIT licensed and designed to be modular and easily extensible. The system also comes fully ready for production usage with user authentication, role management (admin/basic users), chat persistence, and a UI for configuring Personas (AI Assistants) and their Prompts. Danswer also serves as a Unified Search across all common workplace tools such as Slack, Google Drive, Confluence, etc. By combining LLMs and team specific knowledge, Danswer becomes a subject matter expert for the team. Imagine ChatGPT if it had access to your team's unique knowledge! It enables questions such as "A customer wants feature X, is this already supported?" or "Where's the pull request for feature Y?"
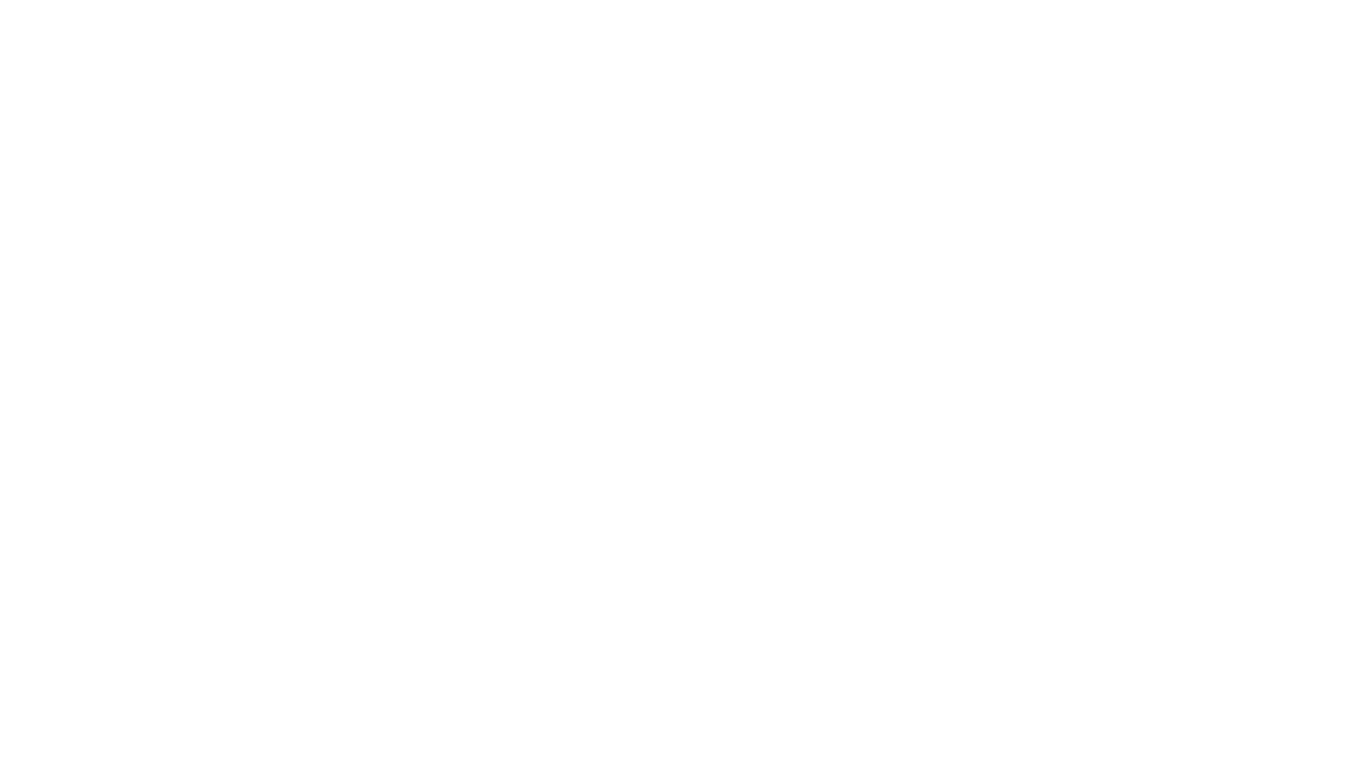
infinity
Infinity is an AI-native database designed for LLM applications, providing incredibly fast full-text and vector search capabilities. It supports a wide range of data types, including vectors, full-text, and structured data, and offers a fused search feature that combines multiple embeddings and full text. Infinity is easy to use, with an intuitive Python API and a single-binary architecture that simplifies deployment. It achieves high performance, with 0.1 milliseconds query latency on million-scale vector datasets and up to 15K QPS.
For similar jobs
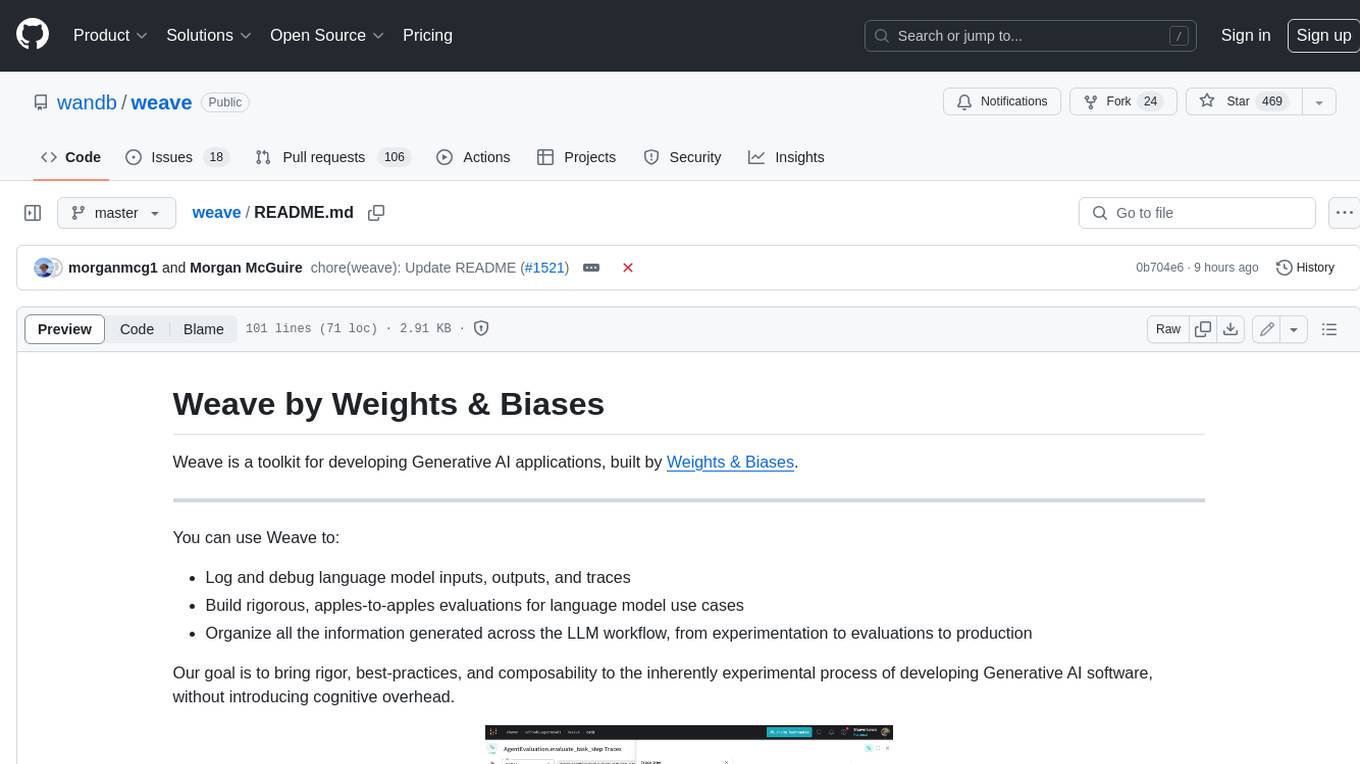
weave
Weave is a toolkit for developing Generative AI applications, built by Weights & Biases. With Weave, you can log and debug language model inputs, outputs, and traces; build rigorous, apples-to-apples evaluations for language model use cases; and organize all the information generated across the LLM workflow, from experimentation to evaluations to production. Weave aims to bring rigor, best-practices, and composability to the inherently experimental process of developing Generative AI software, without introducing cognitive overhead.
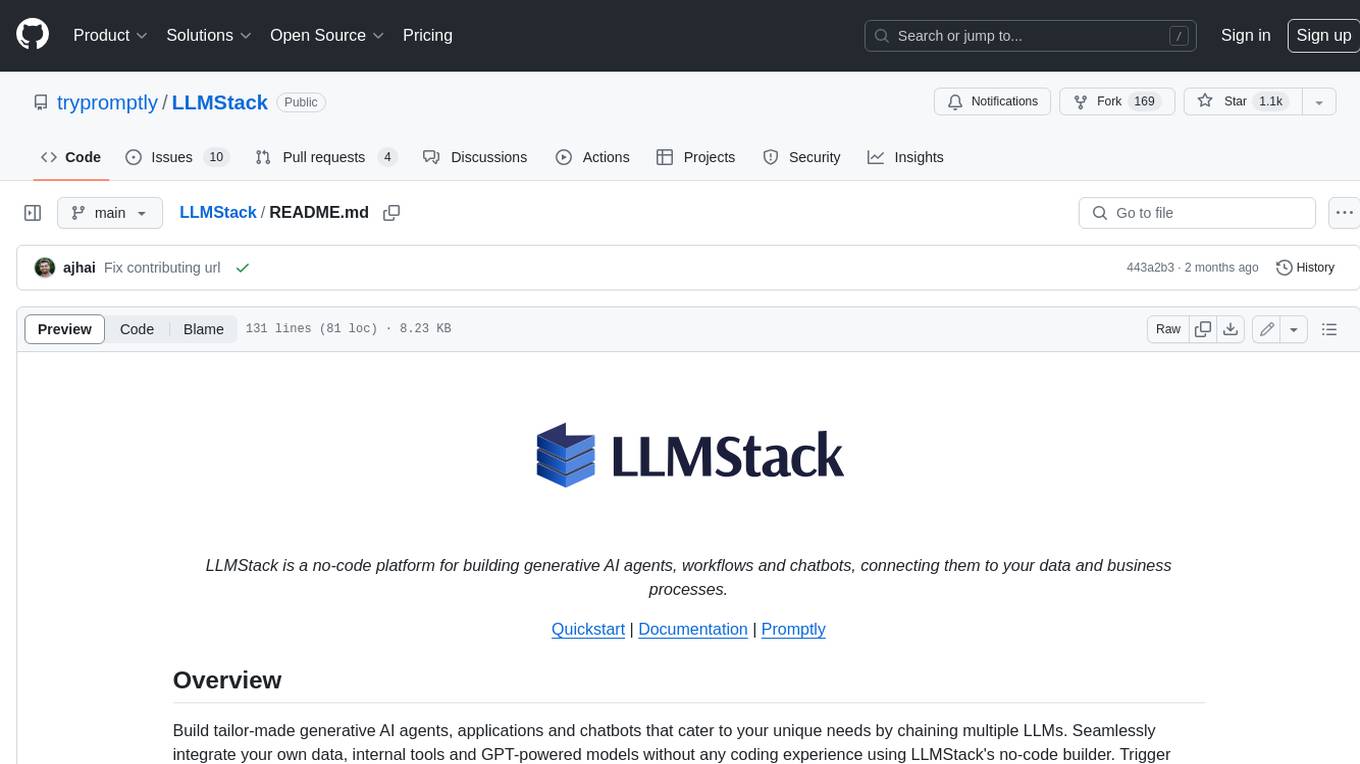
LLMStack
LLMStack is a no-code platform for building generative AI agents, workflows, and chatbots. It allows users to connect their own data, internal tools, and GPT-powered models without any coding experience. LLMStack can be deployed to the cloud or on-premise and can be accessed via HTTP API or triggered from Slack or Discord.
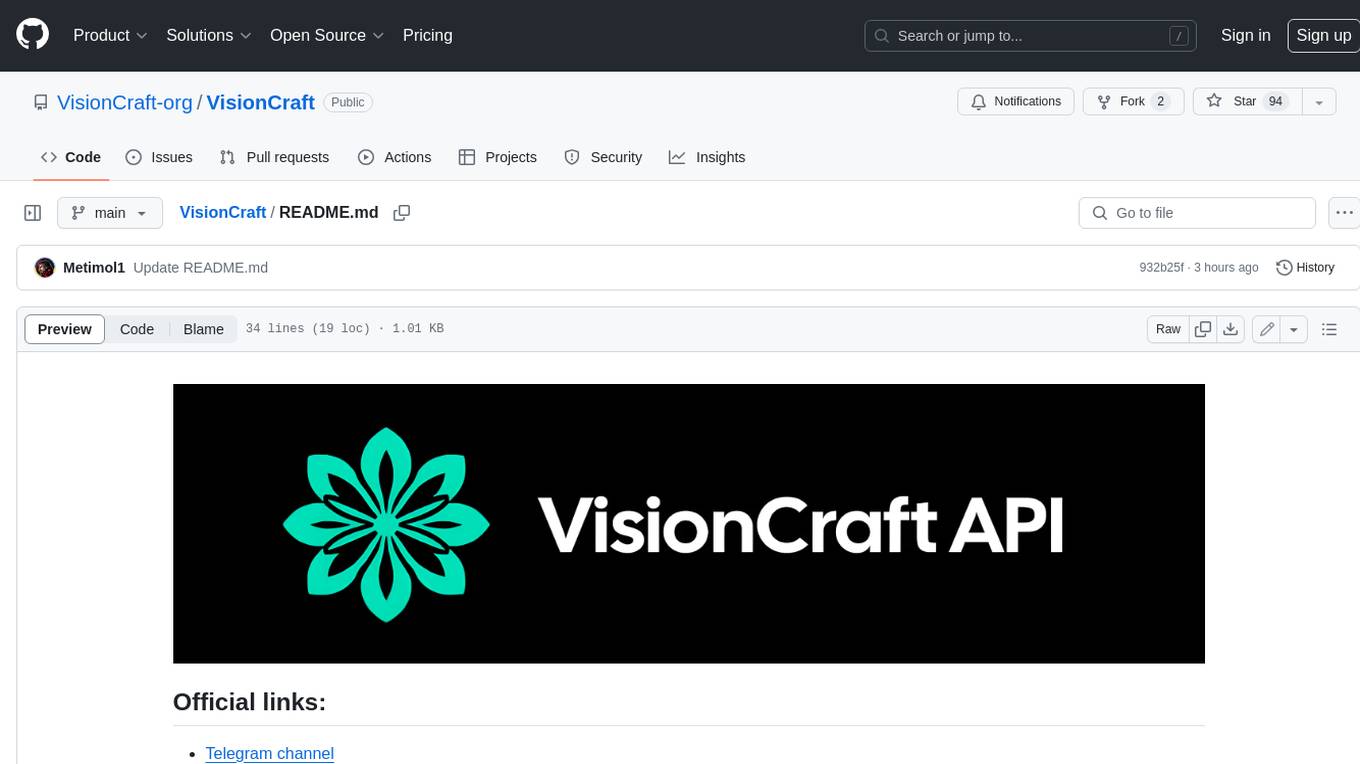
VisionCraft
The VisionCraft API is a free API for using over 100 different AI models. From images to sound.
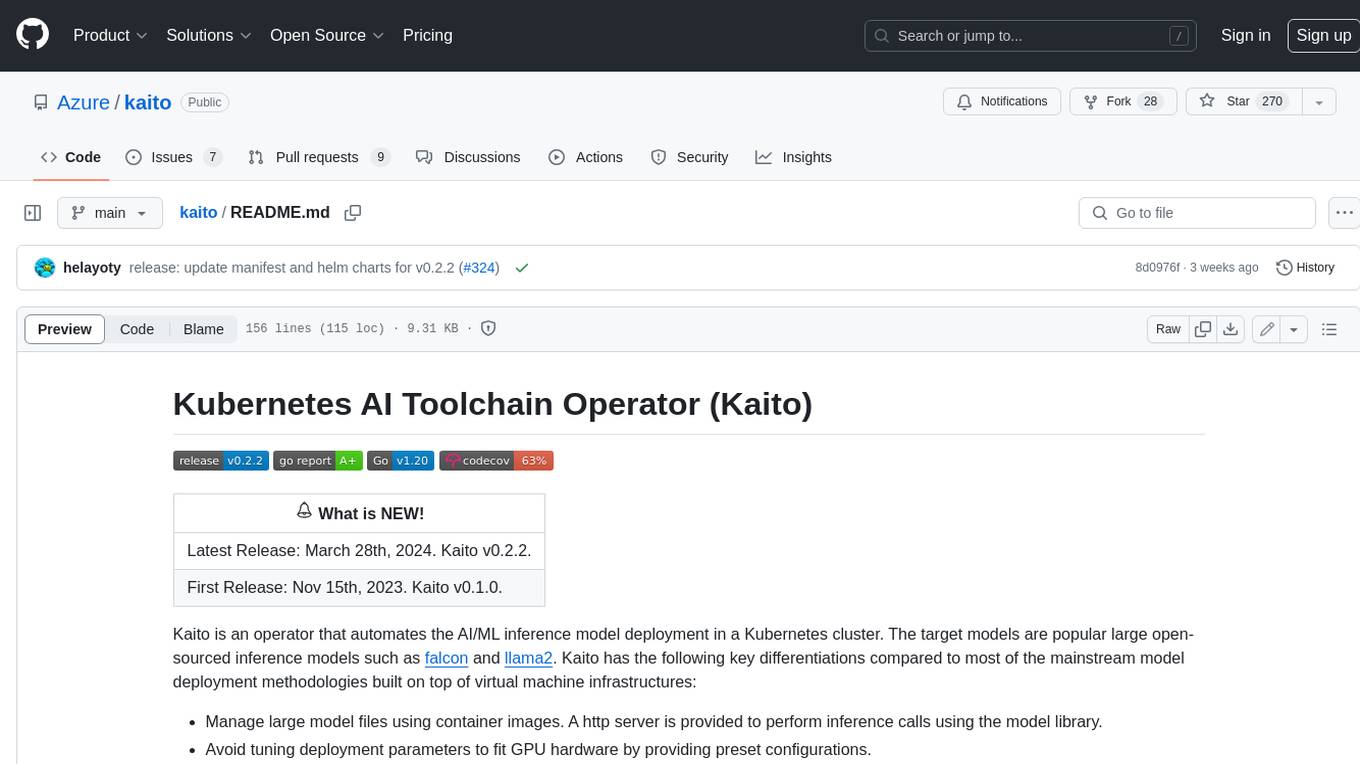
kaito
Kaito is an operator that automates the AI/ML inference model deployment in a Kubernetes cluster. It manages large model files using container images, avoids tuning deployment parameters to fit GPU hardware by providing preset configurations, auto-provisions GPU nodes based on model requirements, and hosts large model images in the public Microsoft Container Registry (MCR) if the license allows. Using Kaito, the workflow of onboarding large AI inference models in Kubernetes is largely simplified.
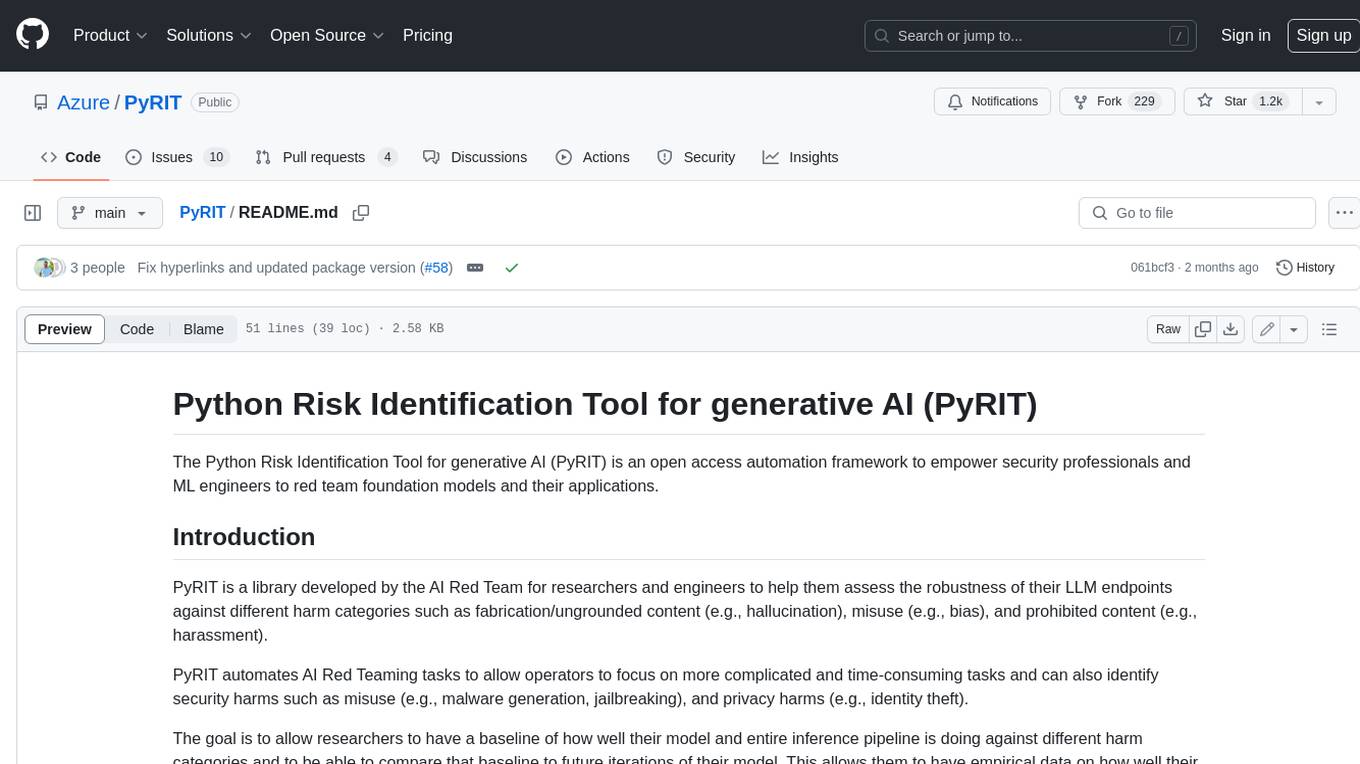
PyRIT
PyRIT is an open access automation framework designed to empower security professionals and ML engineers to red team foundation models and their applications. It automates AI Red Teaming tasks to allow operators to focus on more complicated and time-consuming tasks and can also identify security harms such as misuse (e.g., malware generation, jailbreaking), and privacy harms (e.g., identity theft). The goal is to allow researchers to have a baseline of how well their model and entire inference pipeline is doing against different harm categories and to be able to compare that baseline to future iterations of their model. This allows them to have empirical data on how well their model is doing today, and detect any degradation of performance based on future improvements.
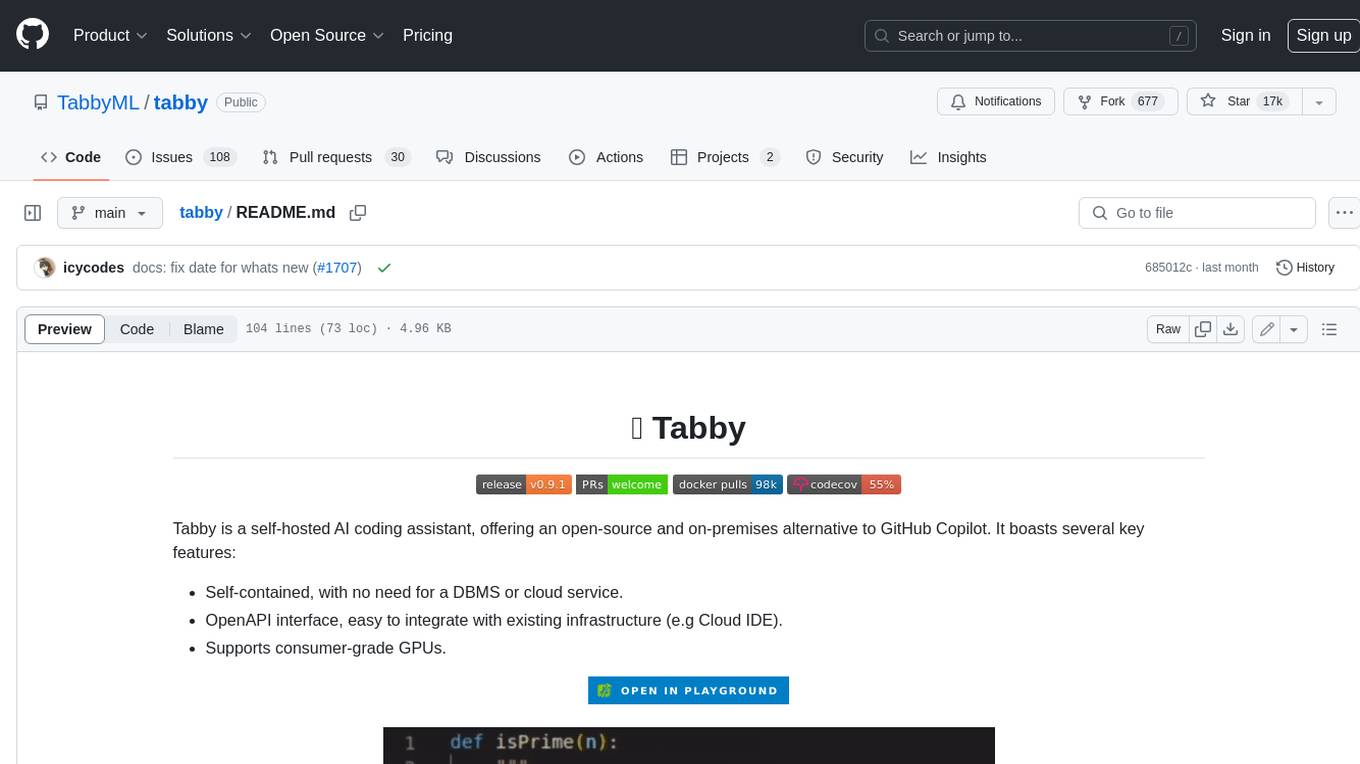
tabby
Tabby is a self-hosted AI coding assistant, offering an open-source and on-premises alternative to GitHub Copilot. It boasts several key features: * Self-contained, with no need for a DBMS or cloud service. * OpenAPI interface, easy to integrate with existing infrastructure (e.g Cloud IDE). * Supports consumer-grade GPUs.
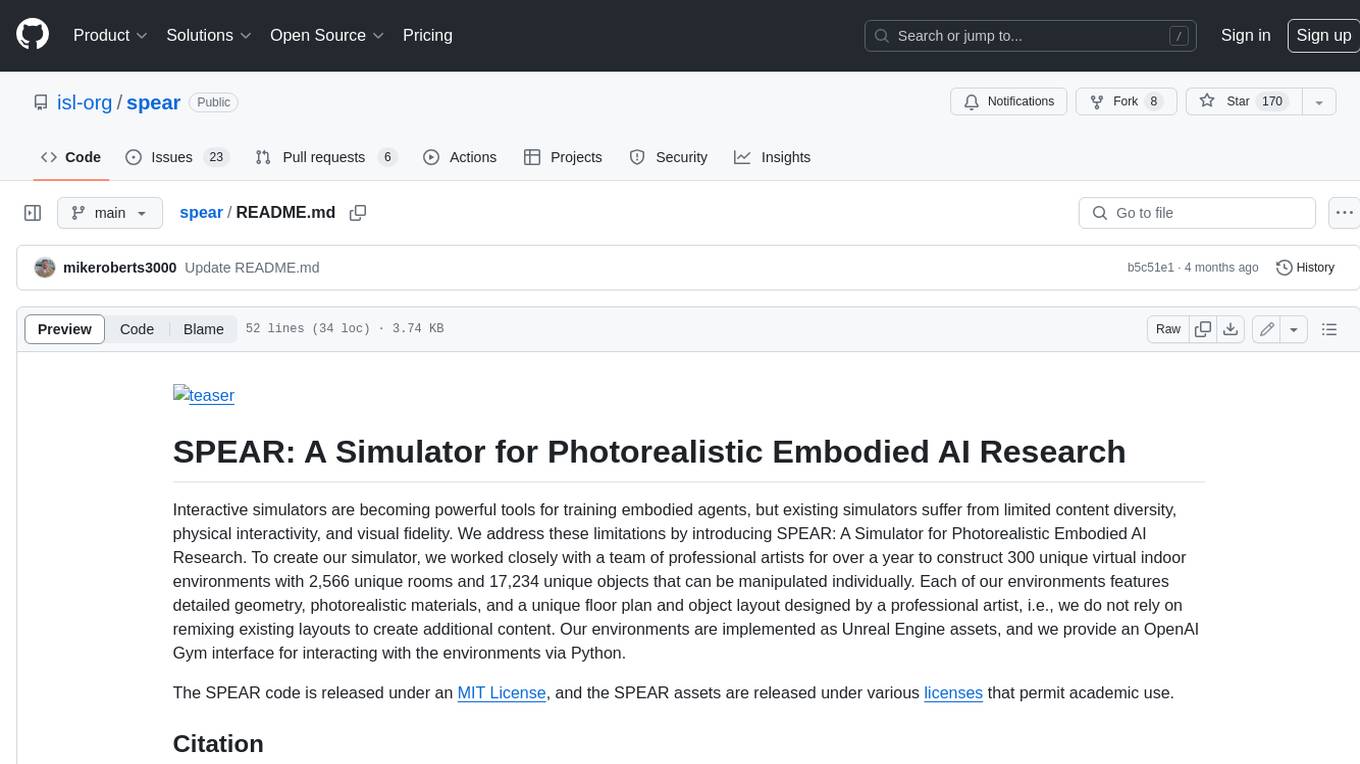
spear
SPEAR (Simulator for Photorealistic Embodied AI Research) is a powerful tool for training embodied agents. It features 300 unique virtual indoor environments with 2,566 unique rooms and 17,234 unique objects that can be manipulated individually. Each environment is designed by a professional artist and features detailed geometry, photorealistic materials, and a unique floor plan and object layout. SPEAR is implemented as Unreal Engine assets and provides an OpenAI Gym interface for interacting with the environments via Python.
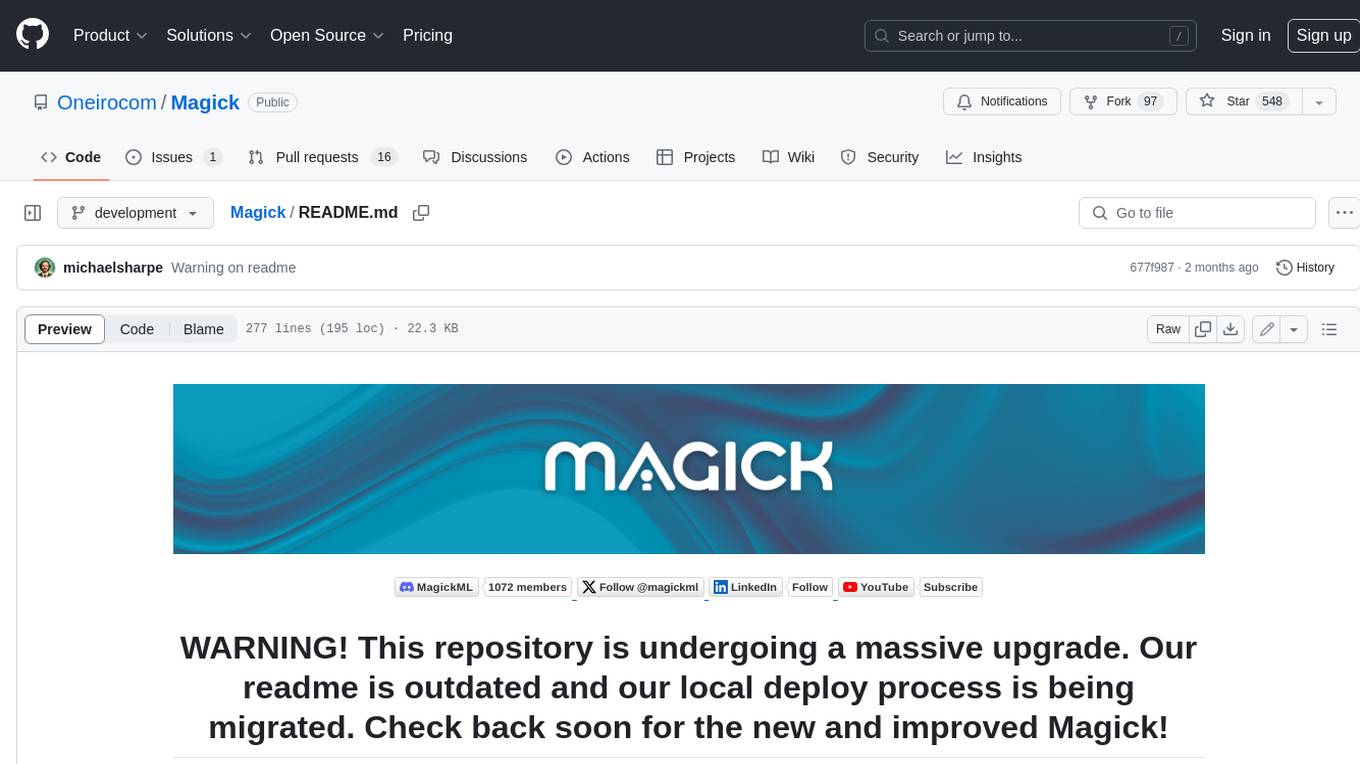
Magick
Magick is a groundbreaking visual AIDE (Artificial Intelligence Development Environment) for no-code data pipelines and multimodal agents. Magick can connect to other services and comes with nodes and templates well-suited for intelligent agents, chatbots, complex reasoning systems and realistic characters.