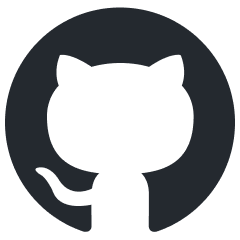
ServerlessLLM
Serverless LLM Serving for Everyone.
Stars: 402
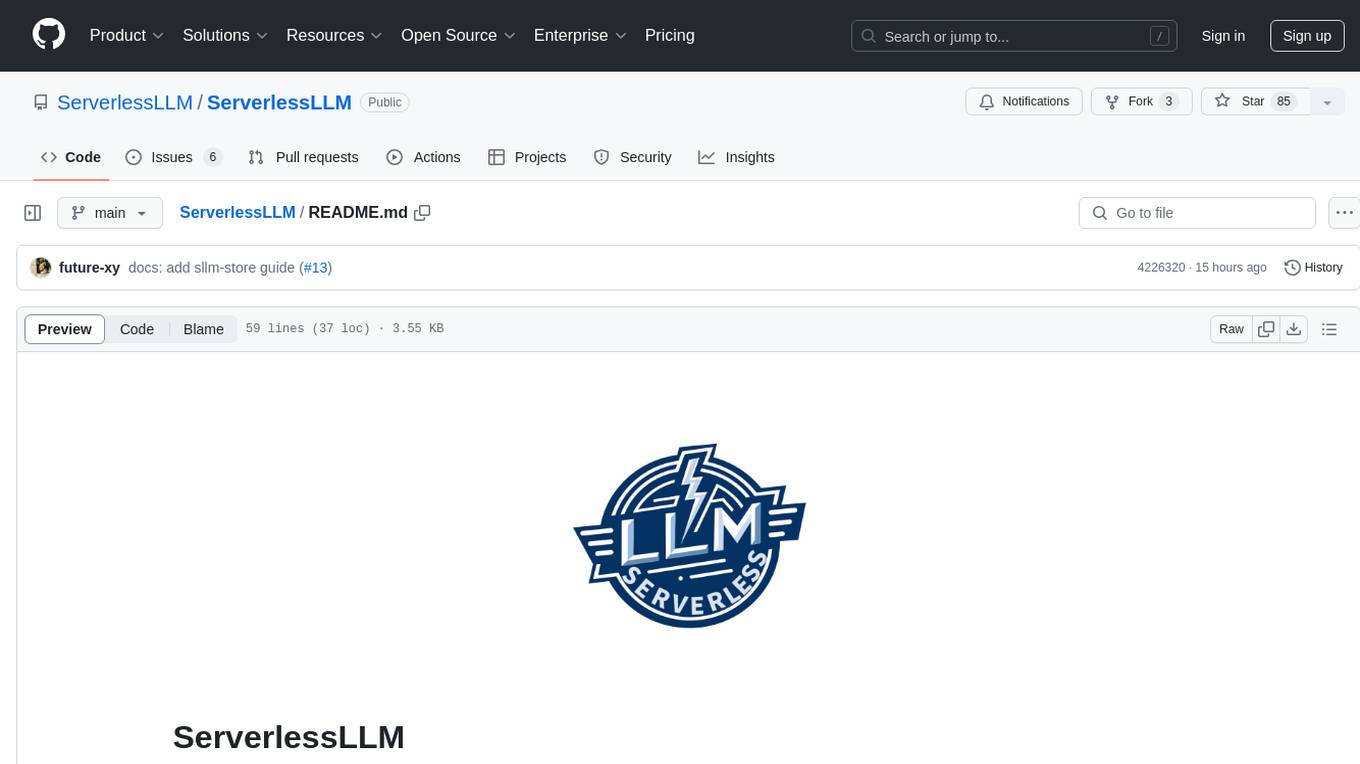
ServerlessLLM is a fast, affordable, and easy-to-use library designed for multi-LLM serving, optimized for environments with limited GPU resources. It supports loading various leading LLM inference libraries, achieving fast load times, and reducing model switching overhead. The library facilitates easy deployment via Ray Cluster and Kubernetes, integrates with the OpenAI Query API, and is actively maintained by contributors.
README:
| Documentation | Paper | Discord | WeChat |
ServerlessLLM (sllm
, pronounced "slim") is an open-source serverless framework designed to make custom and elastic LLM deployment easy, fast, and affordable. As LLMs grow in size and complexity, deploying them on AI hardware has become increasingly costly and technically challenging, limiting custom LLM deployment to only a select few. ServerlessLLM solves these challenges with a full-stack, LLM-centric serverless system design, optimizing everything from checkpoint formats and inference runtimes to the storage layer and cluster scheduler.
- [11/24] We have added experimental support of fast checkpoint loading for AMD GPUs (ROCm) when using with vLLM, PyTorch and HuggingFace Accelerate. Please refer to the documentation for more details.
- [10/24] ServerlessLLM was invited to present at a global AI tech vision forum in Singapore.
- [10/24] We hosted the first ServerlessLLM developer meetup in Edinburgh, attracting over 50 attendees both offline and online. Together, we brainstormed many exciting new features to develop. If you have great ideas, we’d love for you to join us!
- [10/24] We made the first public release of ServerlessLLM. Check out the details of the release here.
- [09/24] ServerlessLLM now supports embedding-based RAG + LLM deployment. We’re preparing a blog and demo—stay tuned!
- [08/24] ServerlessLLM added support for vLLM.
- [07/24] We presented ServerlessLLM at Nvidia's headquarters.
- [06/24] ServerlessLLM officially went public.
ServerlessLLM is designed to support multiple LLMs in efficiently sharing limited AI hardware and dynamically switching between them on demand, which can increase hardware utilization and reduce the cost of LLM services. This multi-LLM scenario, commonly referred to as Serverless, is highly sought after by AI practitioners, as seen in solutions like Serverless Inference, Inference Endpoints, and Model Endpoints. However, these existing offerings often face performance overhead and scalability challenges, which ServerlessLLM effectively addresses through three key capabilities:
ServerlessLLM is Fast:
- Supports leading LLM inference libraries like vLLM and HuggingFace Transformers. Through vLLM, ServerlessLLM can support various types of AI hardware (summarized by vLLM at here)
- Achieves 5-10X faster loading speeds compared to Safetensors and the PyTorch Checkpoint Loader.
- Features an optimized model loading scheduler, offering 5-100X lower start-up latency than Ray Serve and KServe.
ServerlessLLM is Cost-Efficient:
- Allows multiple LLM models to share GPUs with minimal model switching overhead and supports seamless inference live migration.
- Maximizes the use of local storage on multi-GPU servers, reducing the need for expensive storage servers and excessive network bandwidth.
ServerlessLLM is Easy-to-Use:
- Simplifies deployment through Ray Cluster and Kubernetes via KubeRay.
- Supports seamless deployment of HuggingFace Transformers and custom LLM models.
- Supports NVIDIA and AMD GPUs
- Easily integrates with the OpenAI Query API.
- Install ServerlessLLM with pip or from source.
# On the head node
conda create -n sllm python=3.10 -y
conda activate sllm
pip install serverless-llm
# On a worker node
conda create -n sllm-worker python=3.10 -y
conda activate sllm-worker
pip install serverless-llm[worker]
-
Start a local ServerlessLLM cluster using the Quick Start Guide.
-
Want to try fast checkpoint loading in your own code? Check out the ServerlessLLM Store Guide.
To install ServerlessLLM, please follow the steps outlined in our documentation. ServerlessLLM also offers Python APIs for loading and unloading checkpoints, as well as CLI tools to launch an LLM cluster. Both the CLI tools and APIs are demonstrated in the documentation.
Benchmark results for ServerlessLLM can be found here.
ServerlessLLM is maintained by a global team of over 10 developers, and this number is growing. If you're interested in learning more or getting involved, we invite you to join our community on Discord and WeChat. Share your ideas, ask questions, and contribute to the development of ServerlessLLM. For becoming a contributor, please refer to our Contributor Guide.
If you use ServerlessLLM for your research, please cite our paper:
@inproceedings{fu2024serverlessllm,
title={ServerlessLLM: Low-Latency Serverless Inference for Large Language Models},
author={Fu, Yao and Xue, Leyang and Huang, Yeqi and Brabete, Andrei-Octavian and Ustiugov, Dmitrii and Patel, Yuvraj and Mai, Luo},
booktitle={18th USENIX Symposium on Operating Systems Design and Implementation (OSDI 24)},
pages={135--153},
year={2024}
}
For Tasks:
Click tags to check more tools for each tasksFor Jobs:
Alternative AI tools for ServerlessLLM
Similar Open Source Tools
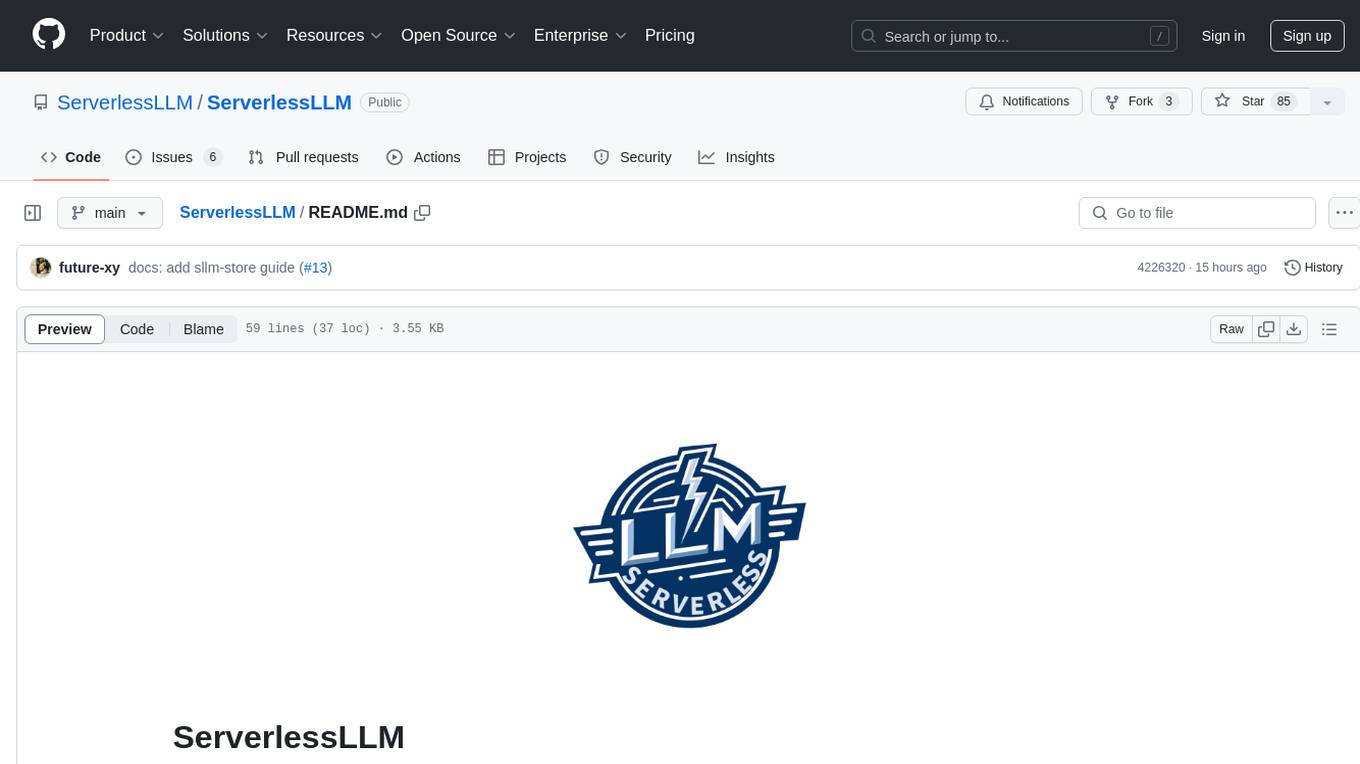
ServerlessLLM
ServerlessLLM is a fast, affordable, and easy-to-use library designed for multi-LLM serving, optimized for environments with limited GPU resources. It supports loading various leading LLM inference libraries, achieving fast load times, and reducing model switching overhead. The library facilitates easy deployment via Ray Cluster and Kubernetes, integrates with the OpenAI Query API, and is actively maintained by contributors.
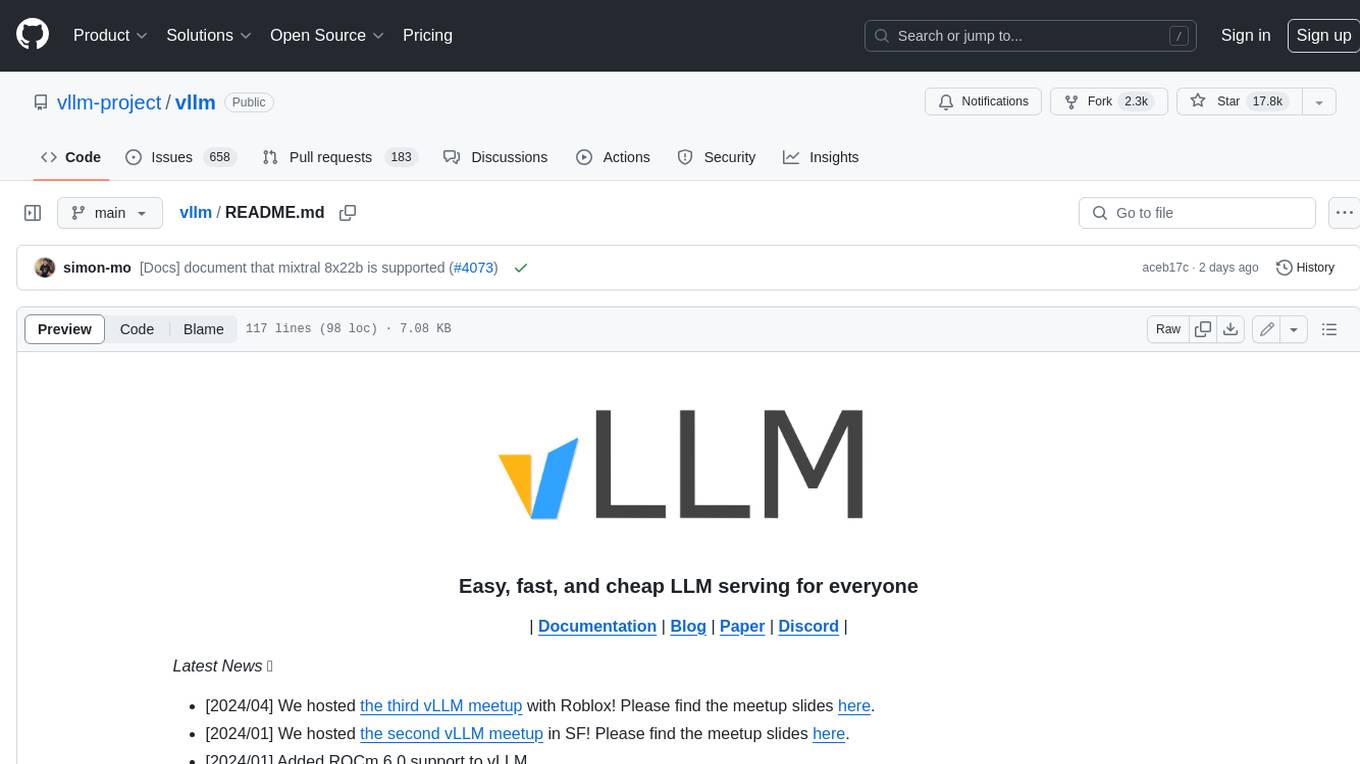
vllm
vLLM is a fast and easy-to-use library for LLM inference and serving. It is designed to be efficient, flexible, and easy to use. vLLM can be used to serve a variety of LLM models, including Hugging Face models. It supports a variety of decoding algorithms, including parallel sampling, beam search, and more. vLLM also supports tensor parallelism for distributed inference and streaming outputs. It is open-source and available on GitHub.
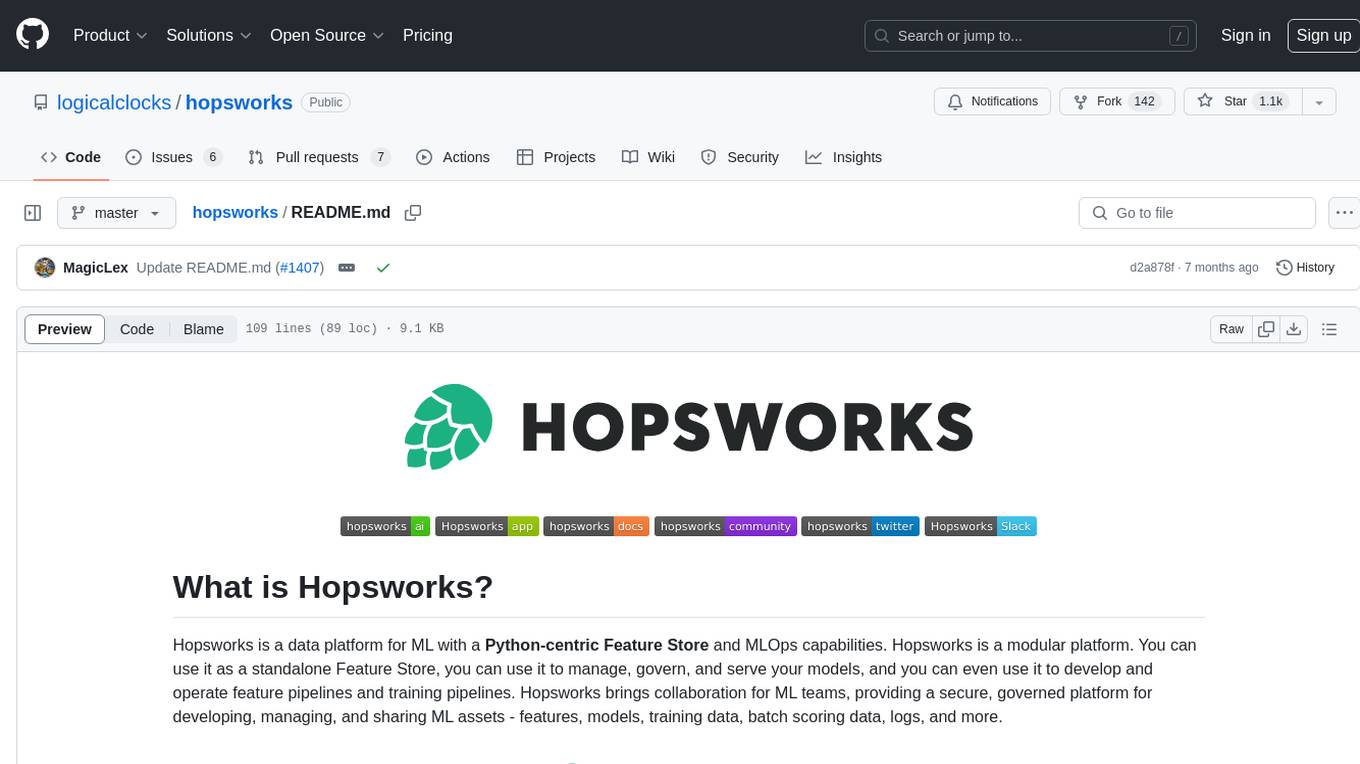
hopsworks
Hopsworks is a data platform for ML with a Python-centric Feature Store and MLOps capabilities. It provides collaboration for ML teams, offering a secure, governed platform for developing, managing, and sharing ML assets. Hopsworks supports project-based multi-tenancy, team collaboration, development tools for Data Science, and is available on any platform including managed cloud services and on-premise installations. The platform enables end-to-end responsibility from raw data to managed features and models, supports versioning, lineage, and provenance, and facilitates the complete MLOps life cycle.
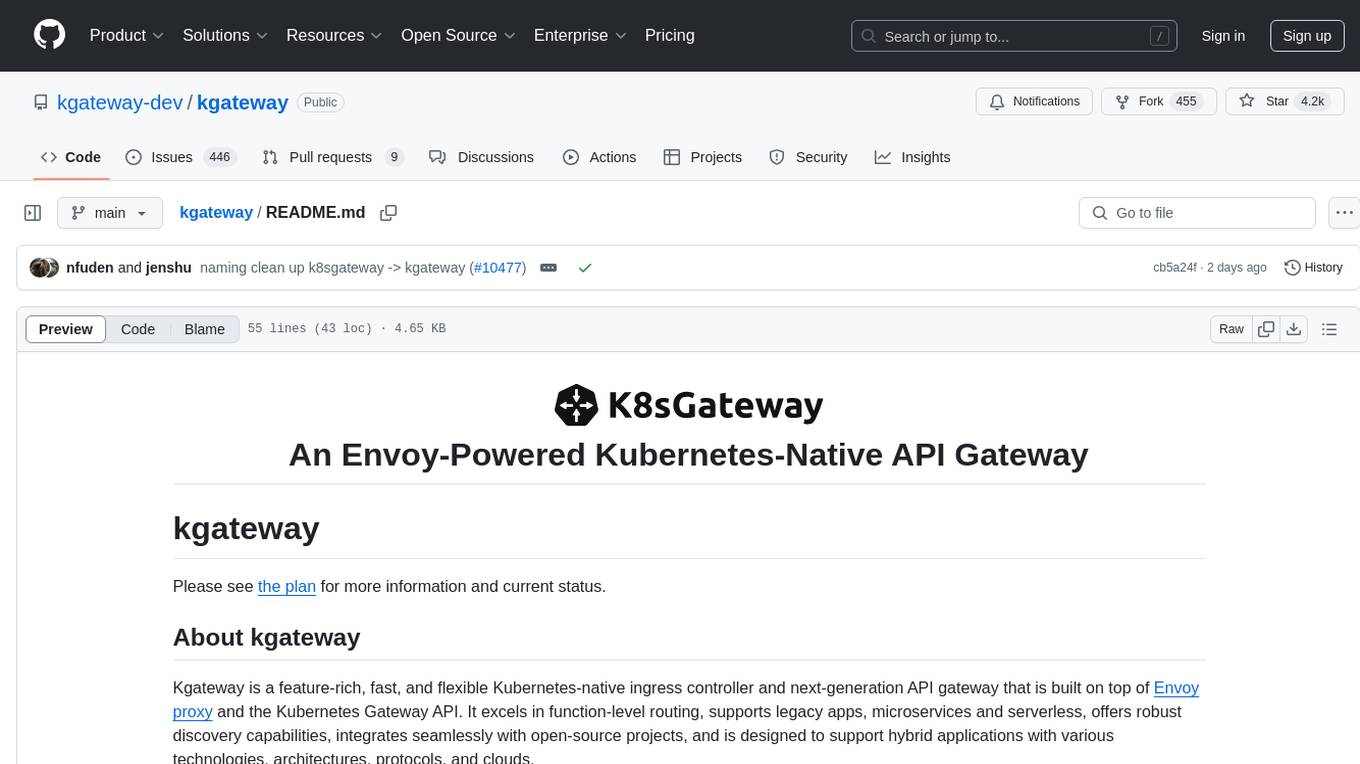
kgateway
Kgateway is a feature-rich, fast, and flexible Kubernetes-native API gateway built on top of Envoy proxy and the Kubernetes Gateway API. It excels in function-level routing, supports legacy apps, microservices, and serverless, offers robust discovery capabilities, integrates seamlessly with open-source projects, and is designed to support hybrid applications with various technologies, architectures, protocols, and clouds.
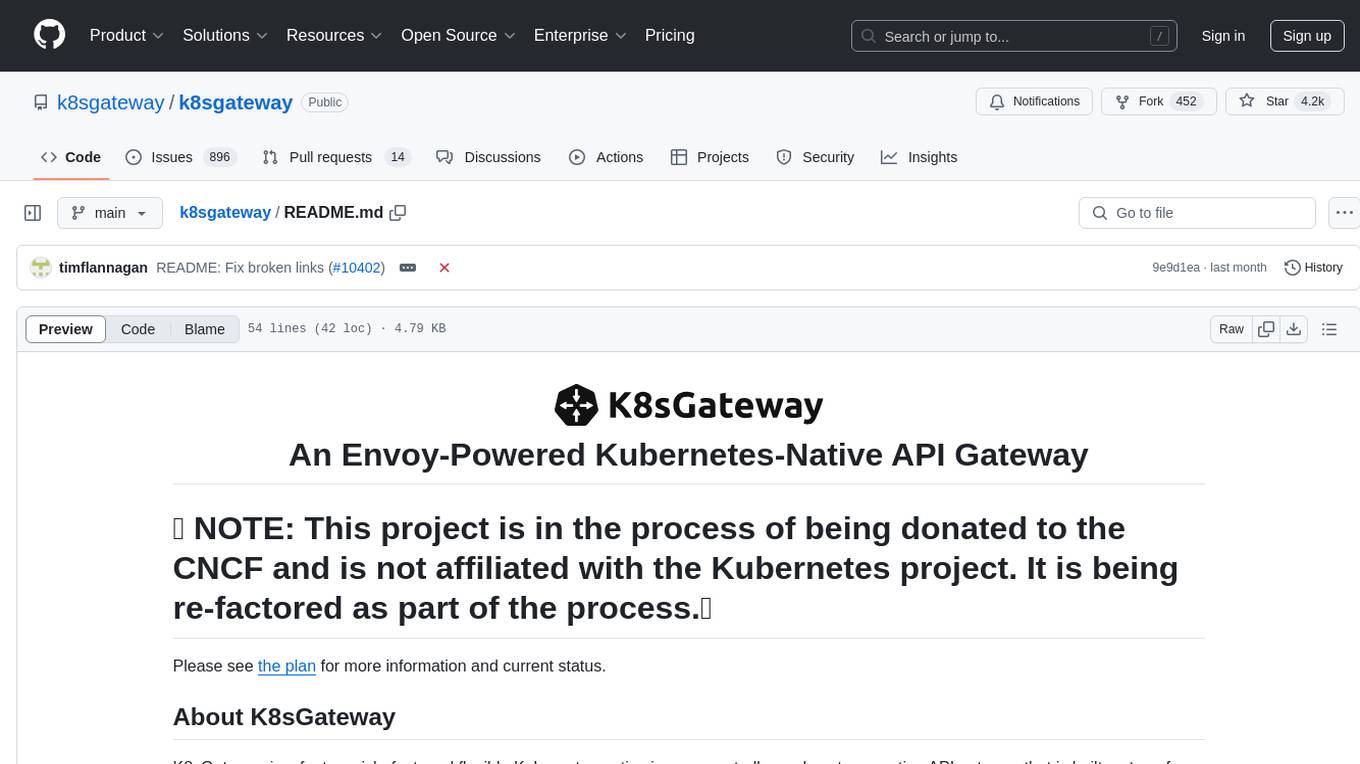
k8sgateway
K8sGateway is a feature-rich, fast, and flexible Kubernetes-native API gateway built on Envoy proxy and Kubernetes Gateway API. It excels in function-level routing, supports legacy apps, microservices, and serverless. It offers robust discovery capabilities, seamless integration with open-source projects, and supports hybrid applications with various technologies, architectures, protocols, and clouds.
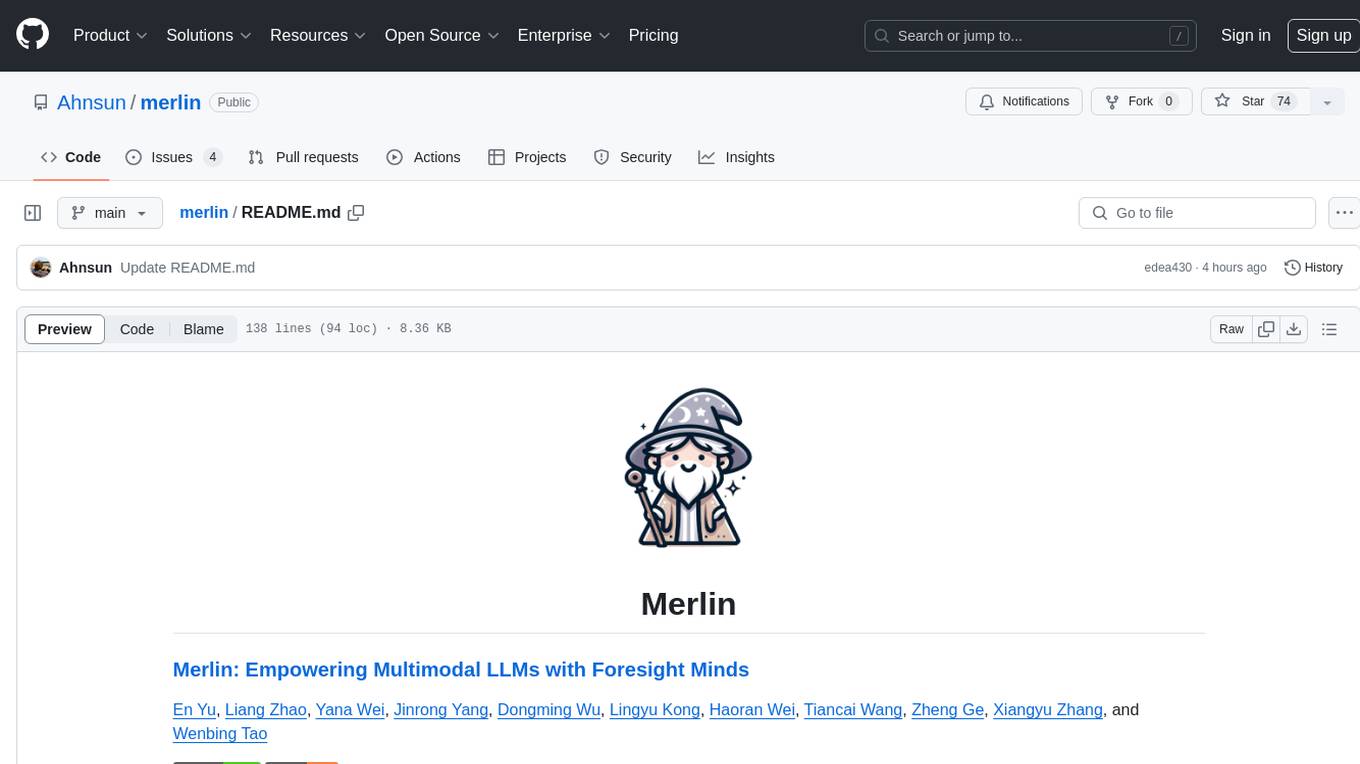
merlin
Merlin is a groundbreaking model capable of generating natural language responses intricately linked with object trajectories of multiple images. It excels in predicting and reasoning about future events based on initial observations, showcasing unprecedented capability in future prediction and reasoning. Merlin achieves state-of-the-art performance on the Future Reasoning Benchmark and multiple existing multimodal language models benchmarks, demonstrating powerful multi-modal general ability and foresight minds.
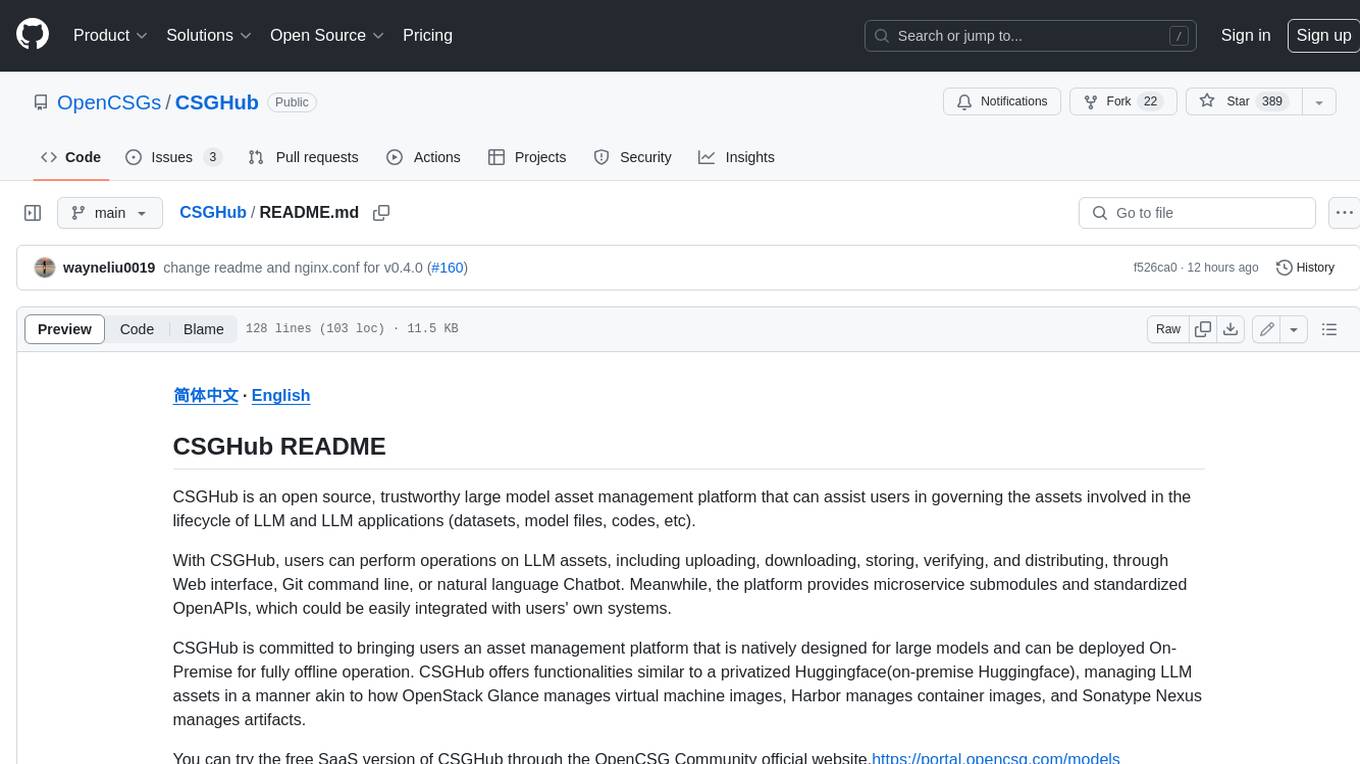
CSGHub
CSGHub is an open source, trustworthy large model asset management platform that can assist users in governing the assets involved in the lifecycle of LLM and LLM applications (datasets, model files, codes, etc). With CSGHub, users can perform operations on LLM assets, including uploading, downloading, storing, verifying, and distributing, through Web interface, Git command line, or natural language Chatbot. Meanwhile, the platform provides microservice submodules and standardized OpenAPIs, which could be easily integrated with users' own systems. CSGHub is committed to bringing users an asset management platform that is natively designed for large models and can be deployed On-Premise for fully offline operation. CSGHub offers functionalities similar to a privatized Huggingface(on-premise Huggingface), managing LLM assets in a manner akin to how OpenStack Glance manages virtual machine images, Harbor manages container images, and Sonatype Nexus manages artifacts.
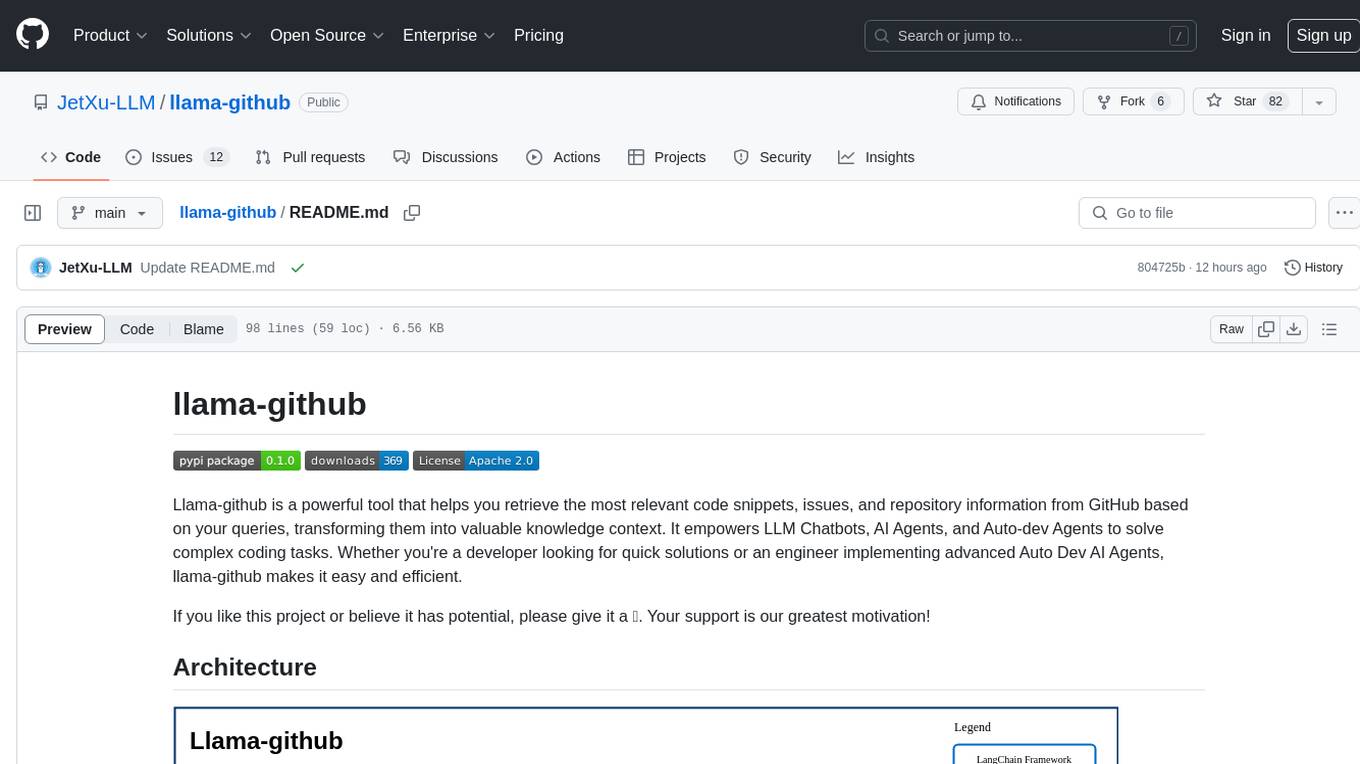
llama-github
Llama-github is a powerful tool that helps retrieve relevant code snippets, issues, and repository information from GitHub based on queries. It empowers AI agents and developers to solve coding tasks efficiently. With features like intelligent GitHub retrieval, repository pool caching, LLM-powered question analysis, and comprehensive context generation, llama-github excels at providing valuable knowledge context for development needs. It supports asynchronous processing, flexible LLM integration, robust authentication options, and logging/error handling for smooth operations and troubleshooting. The vision is to seamlessly integrate with GitHub for AI-driven development solutions, while the roadmap focuses on empowering LLMs to automatically resolve complex coding tasks.
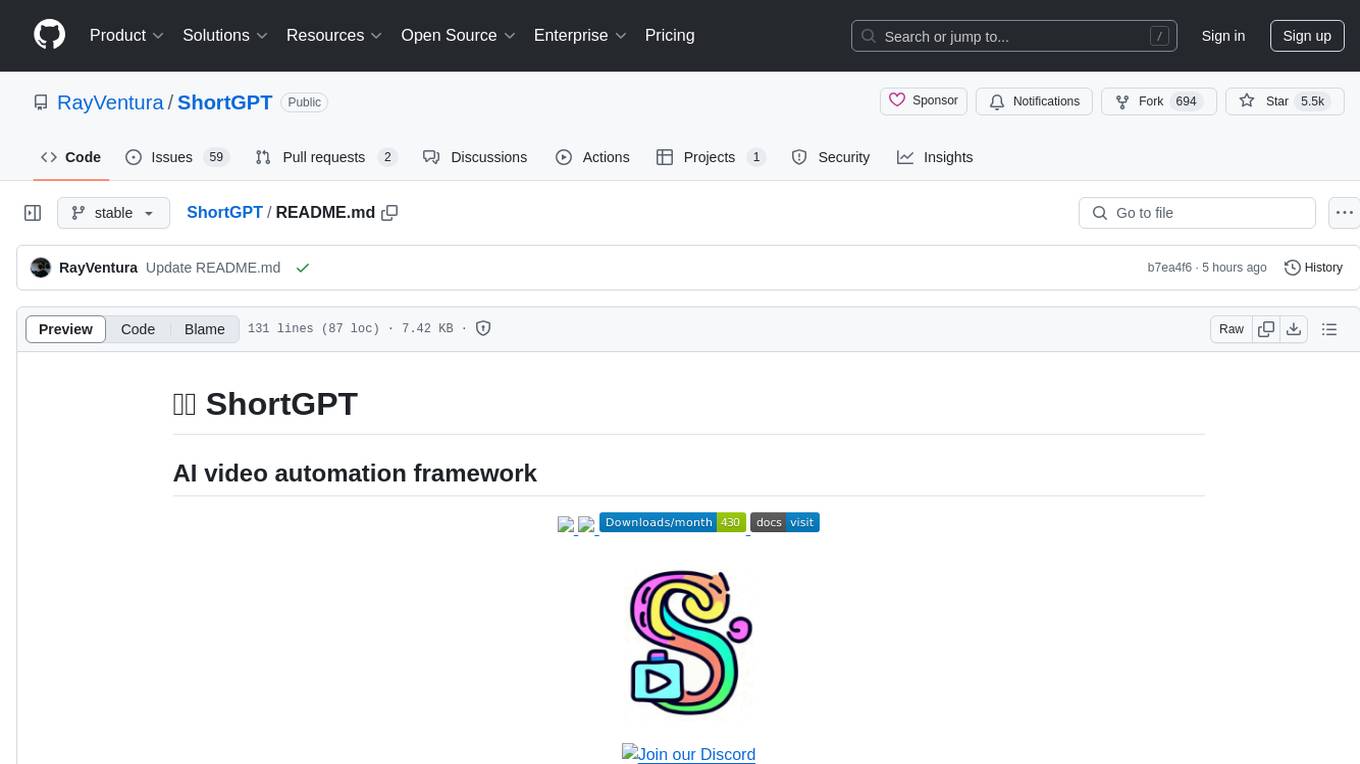
ShortGPT
ShortGPT is a powerful framework for automating content creation, simplifying video creation, footage sourcing, voiceover synthesis, and editing tasks. It offers features like automated editing framework, scripts and prompts, voiceover support in multiple languages, caption generation, asset sourcing, and persistency of editing variables. The tool is designed for youtube automation, Tiktok creativity program automation, and offers customization options for efficient and creative content creation.
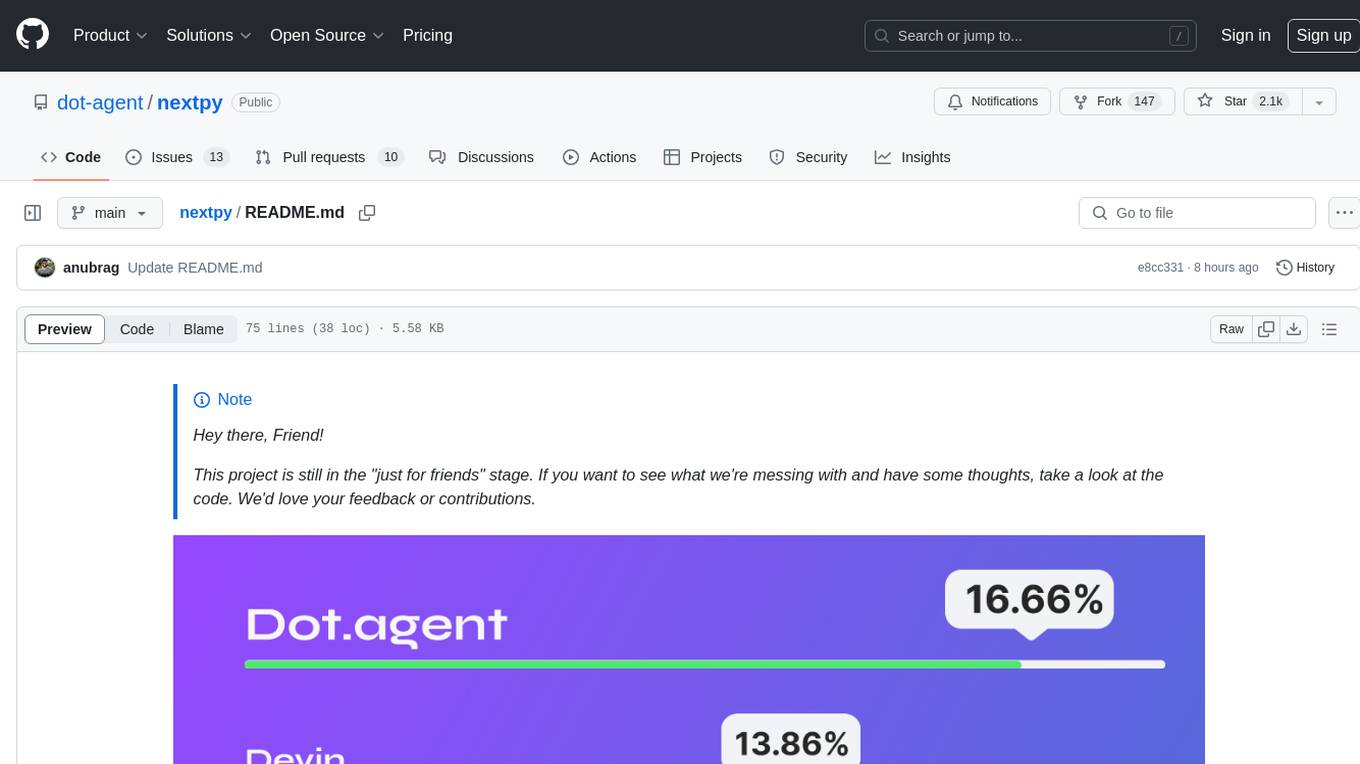
nextpy
Nextpy is a cutting-edge software development framework optimized for AI-based code generation. It provides guardrails for defining AI system boundaries, structured outputs for prompt engineering, a powerful prompt engine for efficient processing, better AI generations with precise output control, modularity for multiplatform and extensible usage, developer-first approach for transferable knowledge, and containerized & scalable deployment options. It offers 4-10x faster performance compared to Streamlit apps, with a focus on cooperation within the open-source community and integration of key components from various projects.
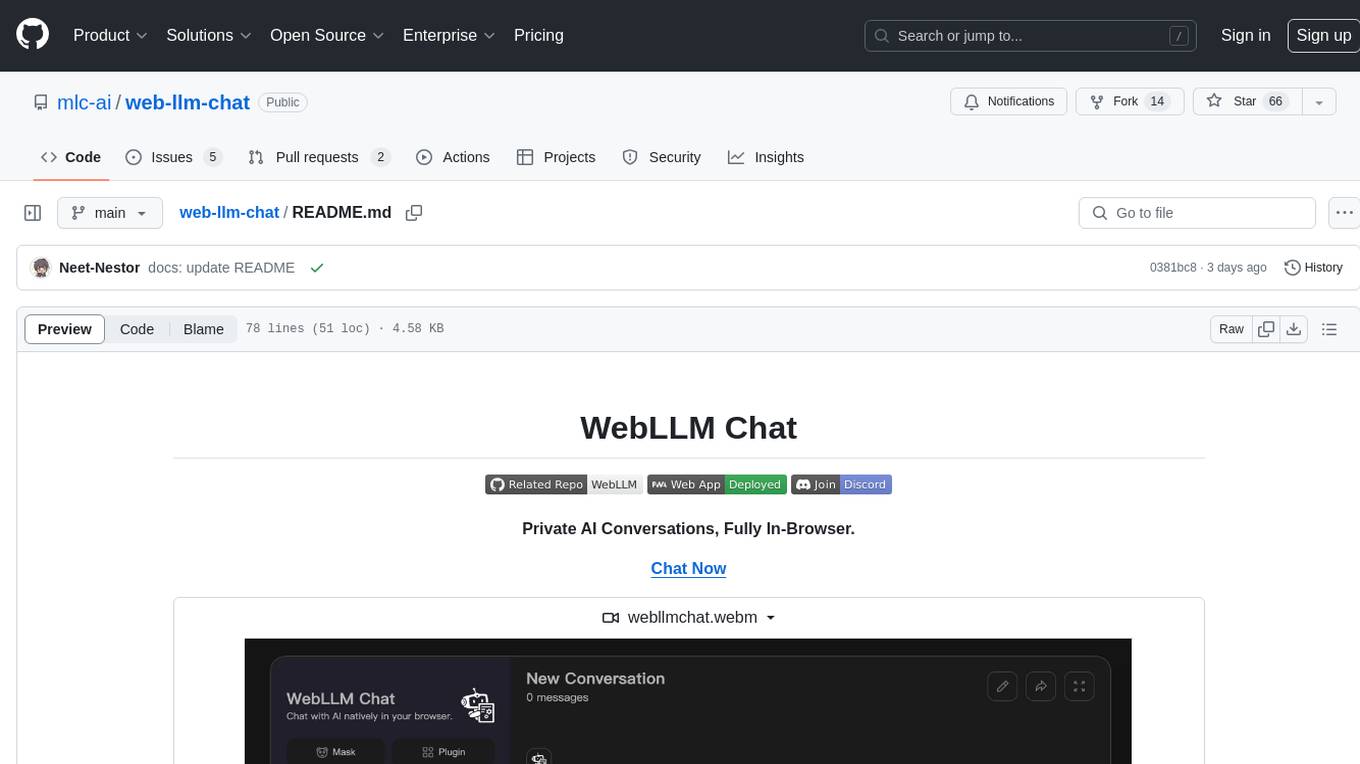
web-llm-chat
WebLLM Chat is a private AI chat interface that combines WebLLM with a user-friendly design, leveraging WebGPU to run large language models natively in your browser. It offers browser-native AI experience with WebGPU acceleration, guaranteed privacy as all data processing happens locally, offline accessibility, user-friendly interface with markdown support, and open-source customization. The project aims to democratize AI technology by making powerful tools accessible directly to end-users, enhancing the chatting experience and broadening the scope for deployment of self-hosted and customizable language models.
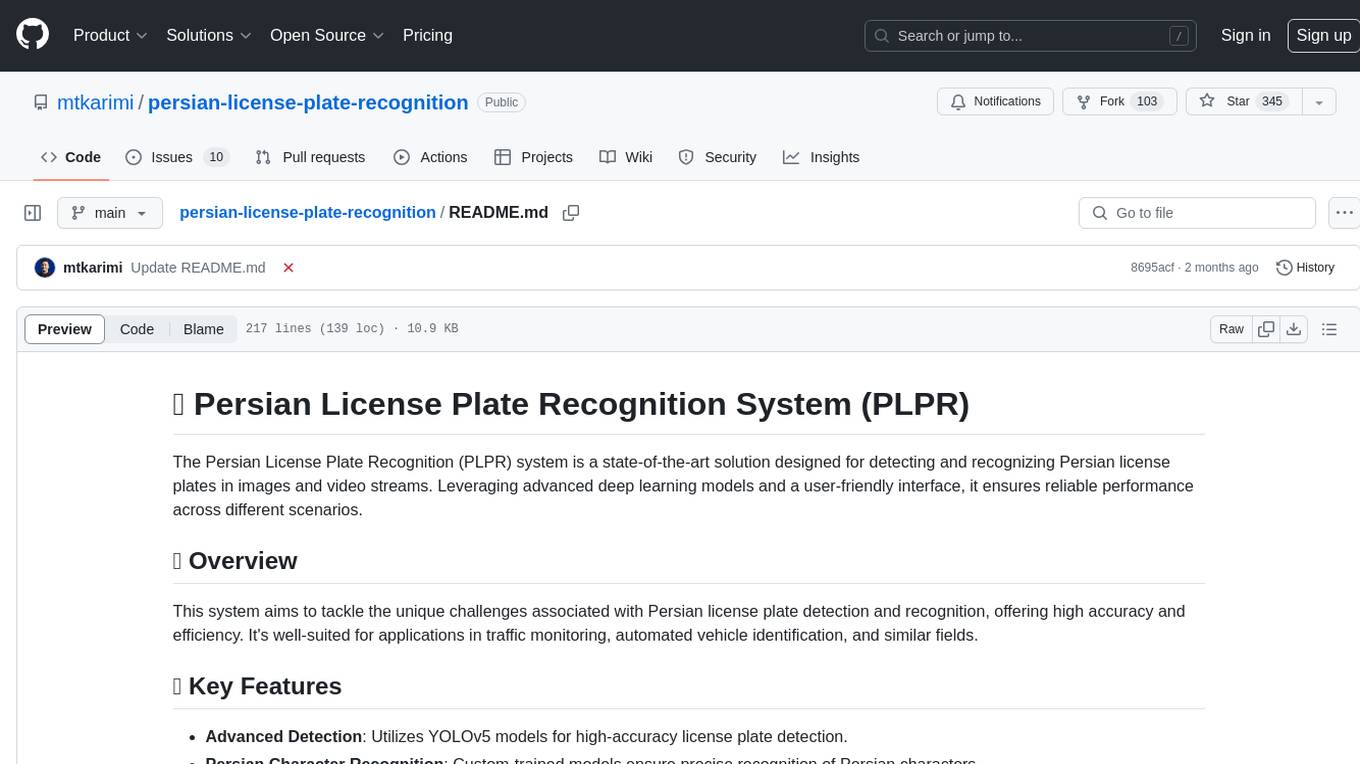
persian-license-plate-recognition
The Persian License Plate Recognition (PLPR) system is a state-of-the-art solution designed for detecting and recognizing Persian license plates in images and video streams. Leveraging advanced deep learning models and a user-friendly interface, it ensures reliable performance across different scenarios. The system offers advanced detection using YOLOv5 models, precise recognition of Persian characters, real-time processing capabilities, and a user-friendly GUI. It is well-suited for applications in traffic monitoring, automated vehicle identification, and similar fields. The system's architecture includes modules for resident management, entrance management, and a detailed flowchart explaining the process from system initialization to displaying results in the GUI. Hardware requirements include an Intel Core i5 processor, 8 GB RAM, a dedicated GPU with at least 4 GB VRAM, and an SSD with 20 GB of free space. The system can be installed by cloning the repository and installing required Python packages. Users can customize the video source for processing and run the application to upload and process images or video streams. The system's GUI allows for parameter adjustments to optimize performance, and the Wiki provides in-depth information on the system's architecture and model training.
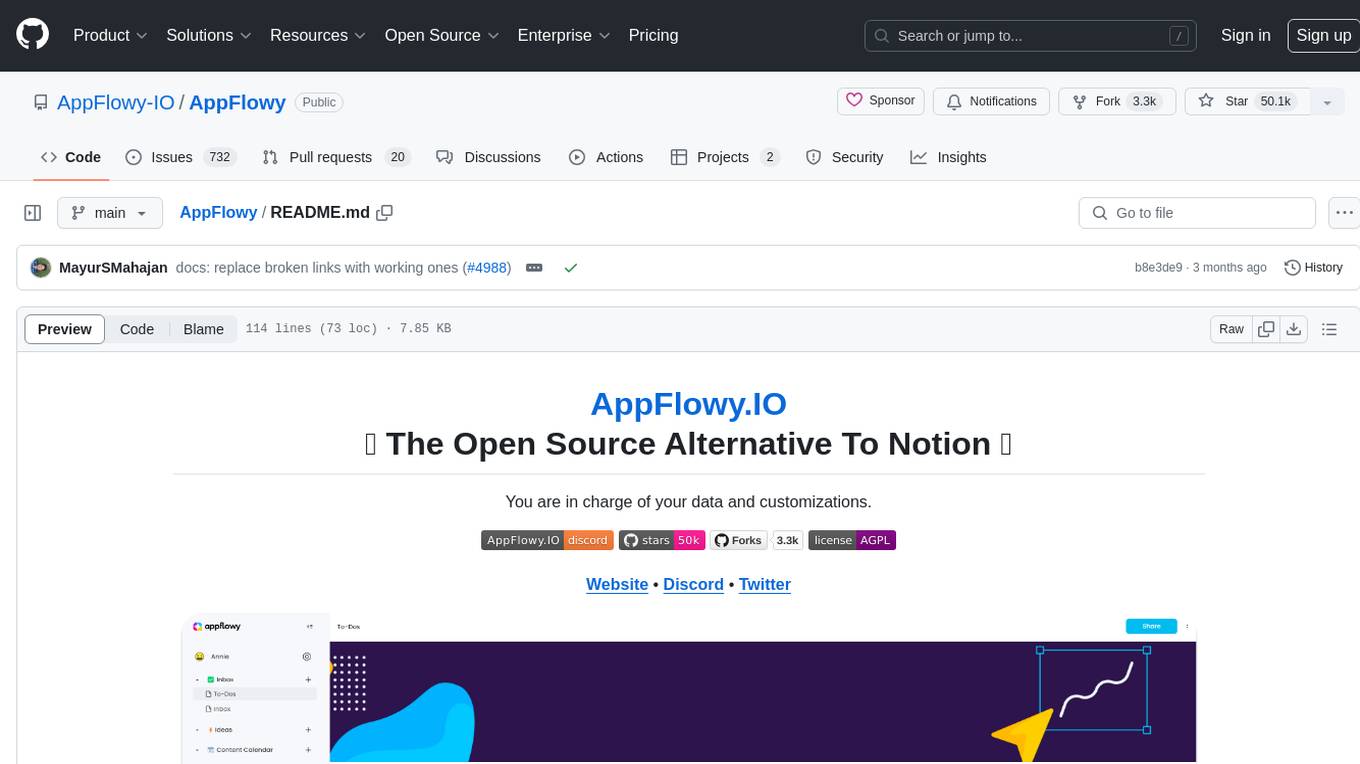
AppFlowy
AppFlowy.IO is an open-source alternative to Notion, providing users with control over their data and customizations. It aims to offer functionality, data security, and cross-platform native experience to individuals, as well as building blocks and collaboration infra services to enterprises and hackers. The tool is built with Flutter and Rust, supporting multiple platforms and emphasizing long-term maintainability. AppFlowy prioritizes data privacy, reliable native experience, and community-driven extensibility, aiming to democratize the creation of complex workplace management tools.
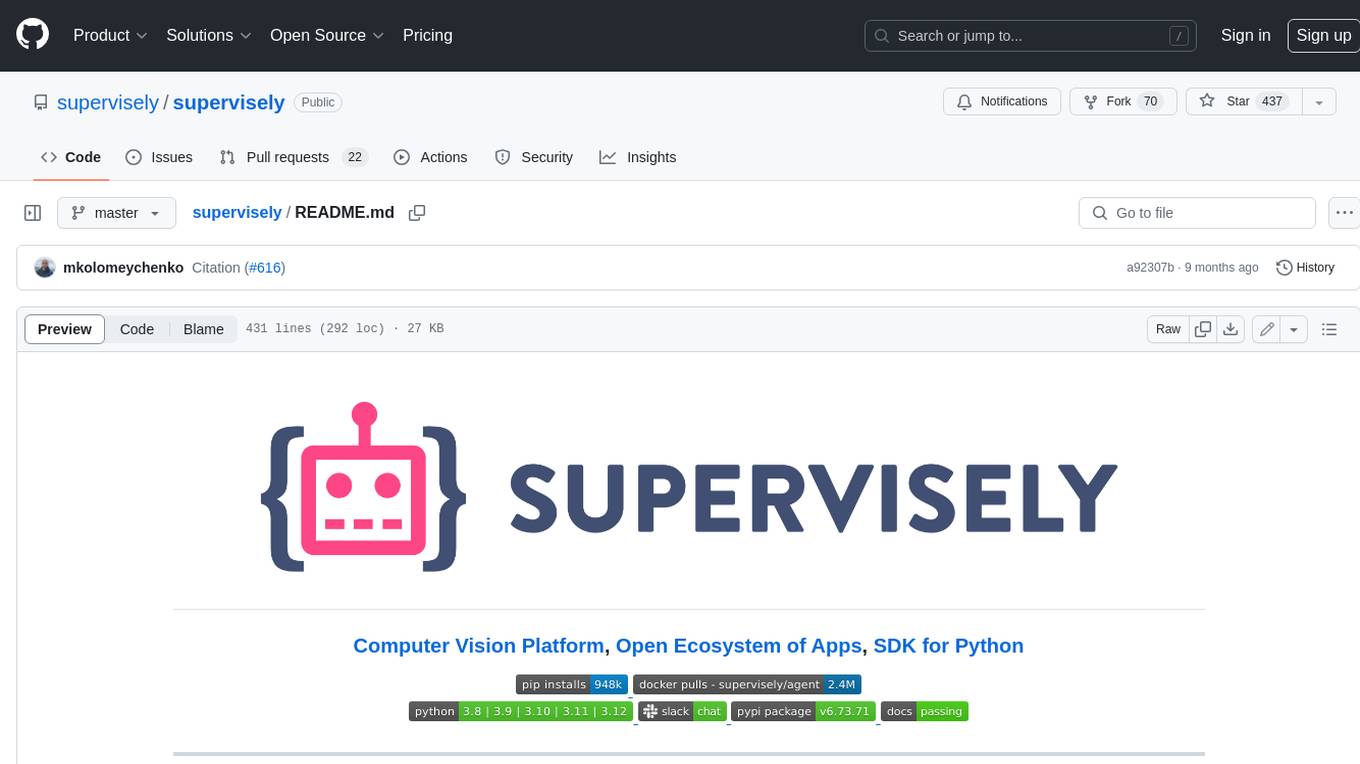
supervisely
Supervisely is a computer vision platform that provides a range of tools and services for developing and deploying computer vision solutions. It includes a data labeling platform, a model training platform, and a marketplace for computer vision apps. Supervisely is used by a variety of organizations, including Fortune 500 companies, research institutions, and government agencies.
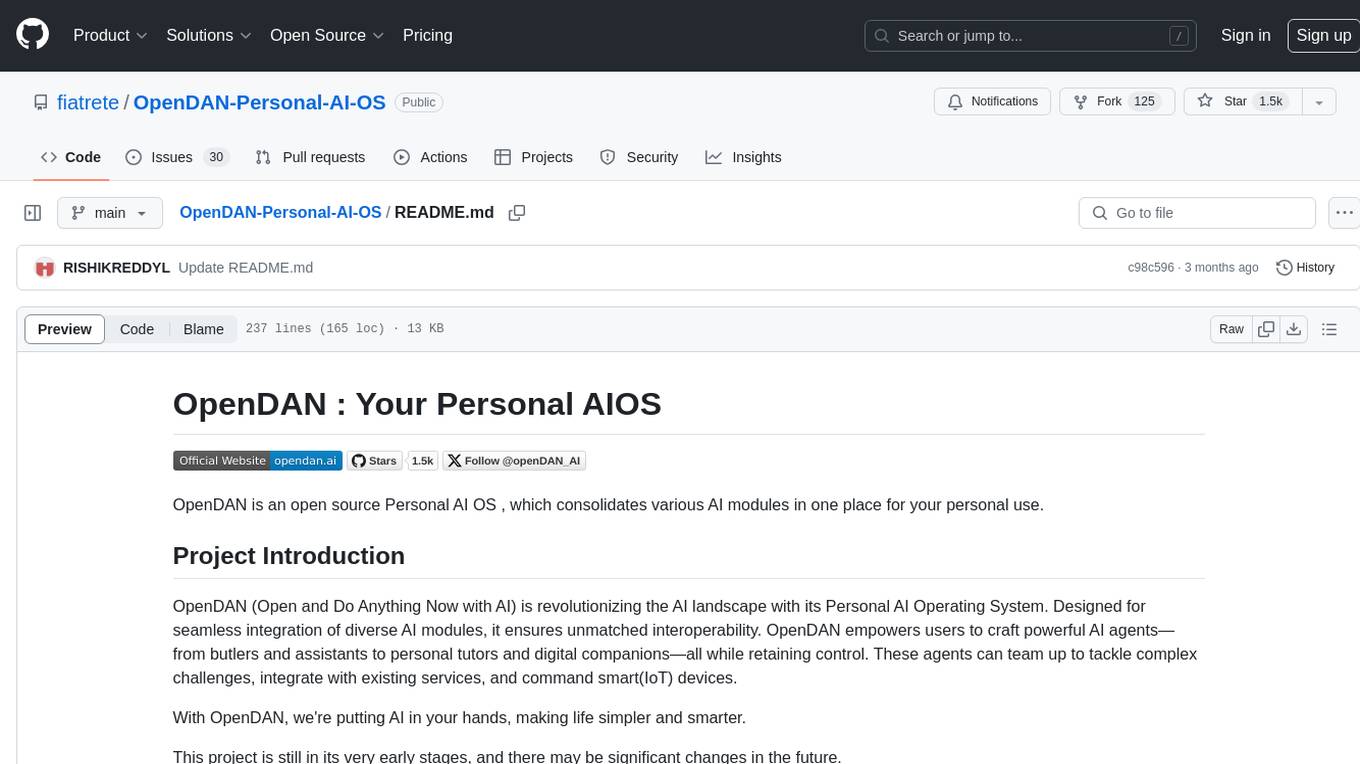
OpenDAN-Personal-AI-OS
OpenDAN is an open source Personal AI OS that consolidates various AI modules for personal use. It empowers users to create powerful AI agents like assistants, tutors, and companions. The OS allows agents to collaborate, integrate with services, and control smart devices. OpenDAN offers features like rapid installation, AI agent customization, connectivity via Telegram/Email, building a local knowledge base, distributed AI computing, and more. It aims to simplify life by putting AI in users' hands. The project is in early stages with ongoing development and future plans for user and kernel mode separation, home IoT device control, and an official OpenDAN SDK release.
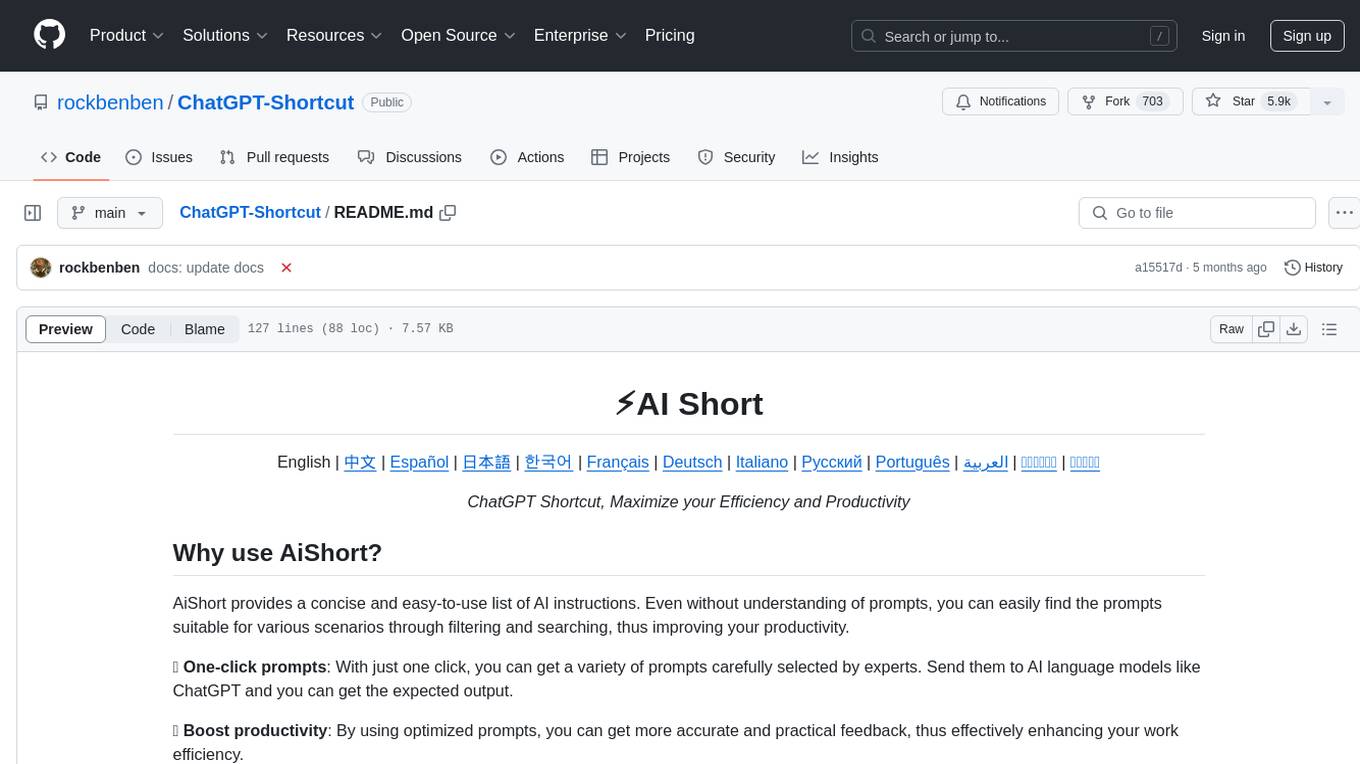
ChatGPT-Shortcut
ChatGPT Shortcut is an AI tool designed to maximize efficiency and productivity by providing a concise list of AI instructions. Users can easily find prompts suitable for various scenarios, boosting productivity and work efficiency. The tool offers one-click prompts, optimization for non-English languages, prompt saving and sharing, and a community voting system. It includes a browser extension compatible with Chrome, Edge, Firefox, and other Chromium-based browsers, as well as a Tampermonkey script for custom domain use. The tool is open-source, allowing users to modify the website's nomenclature, usage directives, and prompts for different languages.
For similar tasks
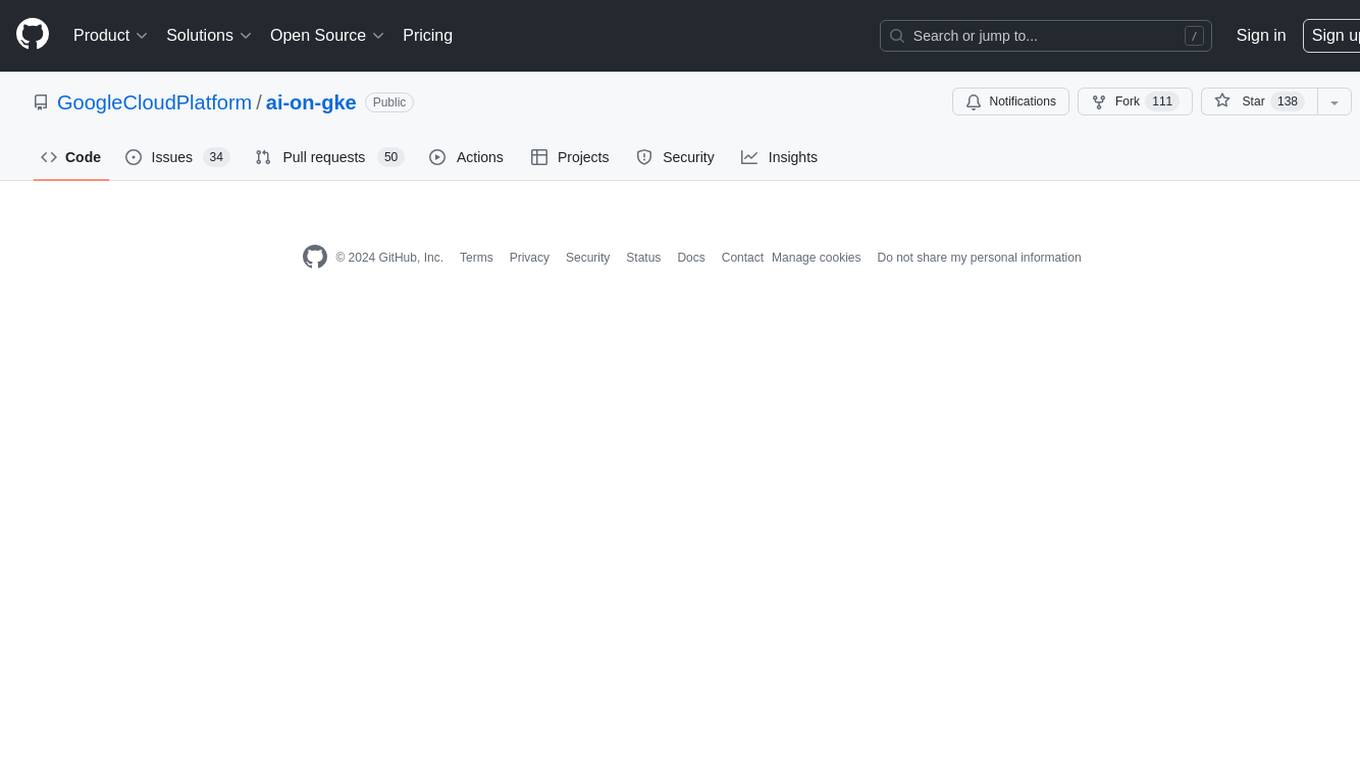
ai-on-gke
This repository contains assets related to AI/ML workloads on Google Kubernetes Engine (GKE). Run optimized AI/ML workloads with Google Kubernetes Engine (GKE) platform orchestration capabilities. A robust AI/ML platform considers the following layers: Infrastructure orchestration that support GPUs and TPUs for training and serving workloads at scale Flexible integration with distributed computing and data processing frameworks Support for multiple teams on the same infrastructure to maximize utilization of resources
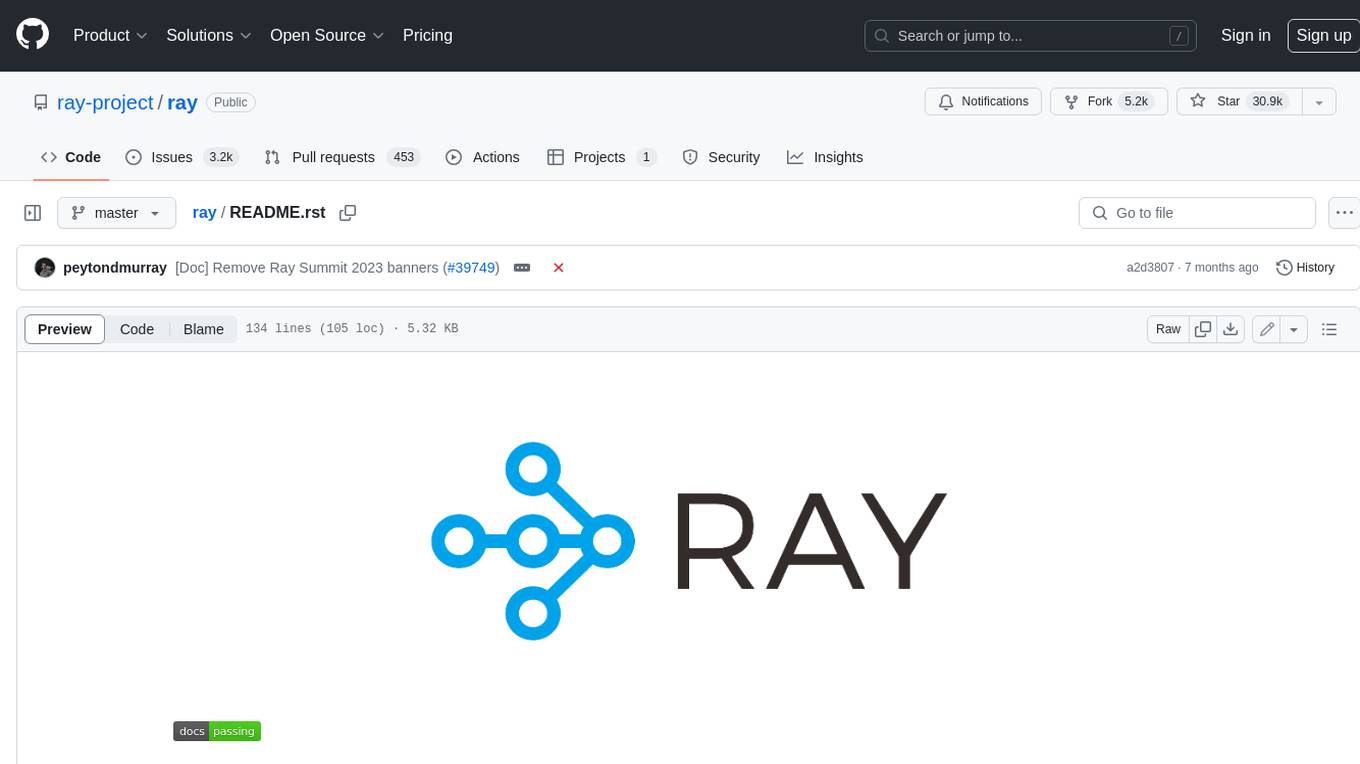
ray
Ray is a unified framework for scaling AI and Python applications. It consists of a core distributed runtime and a set of AI libraries for simplifying ML compute, including Data, Train, Tune, RLlib, and Serve. Ray runs on any machine, cluster, cloud provider, and Kubernetes, and features a growing ecosystem of community integrations. With Ray, you can seamlessly scale the same code from a laptop to a cluster, making it easy to meet the compute-intensive demands of modern ML workloads.
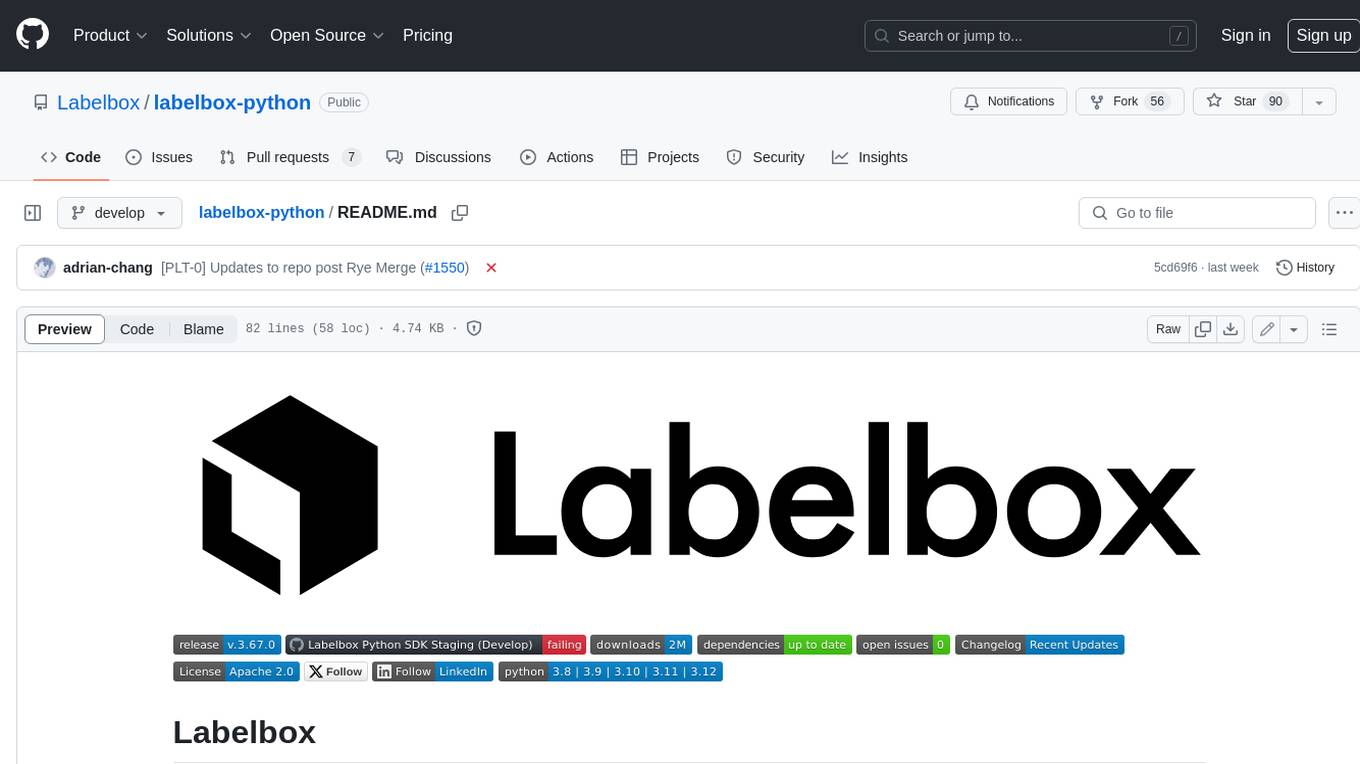
labelbox-python
Labelbox is a data-centric AI platform for enterprises to develop, optimize, and use AI to solve problems and power new products and services. Enterprises use Labelbox to curate data, generate high-quality human feedback data for computer vision and LLMs, evaluate model performance, and automate tasks by combining AI and human-centric workflows. The academic & research community uses Labelbox for cutting-edge AI research.
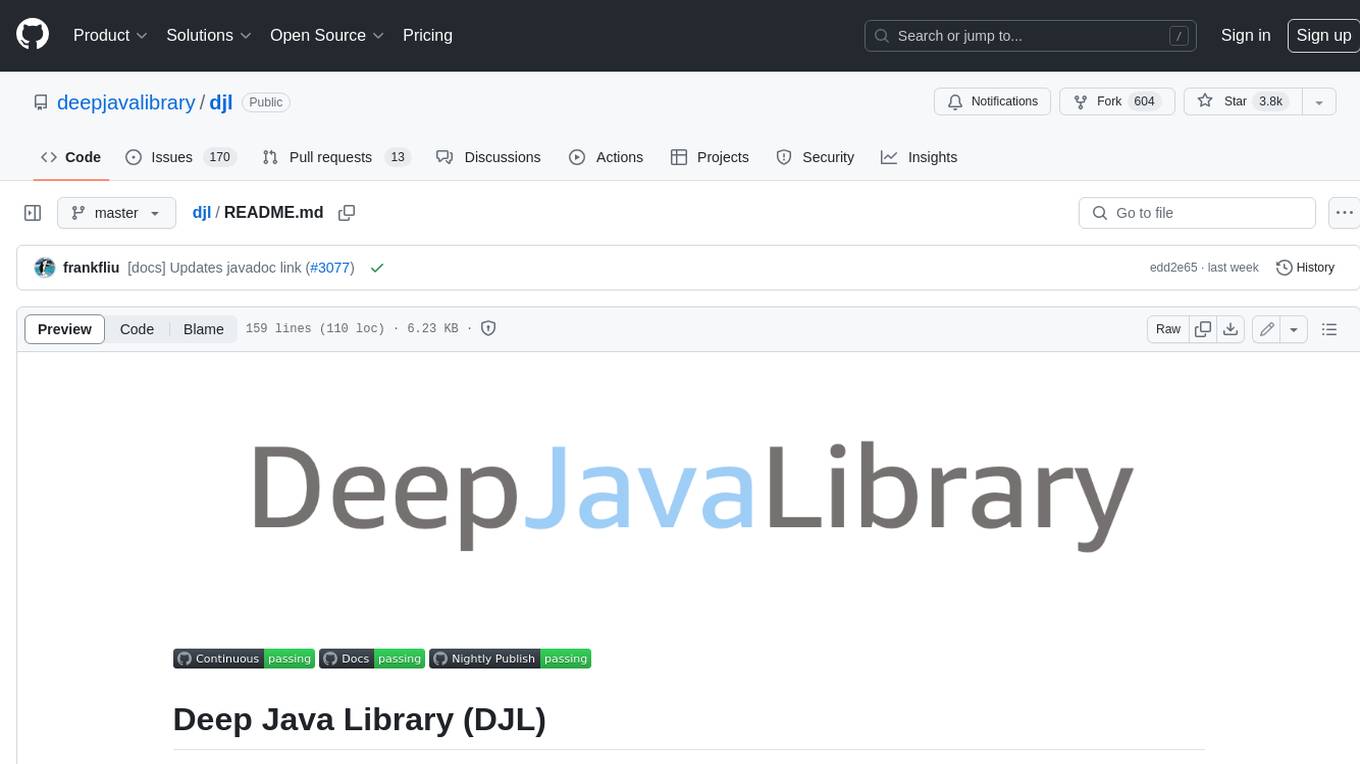
djl
Deep Java Library (DJL) is an open-source, high-level, engine-agnostic Java framework for deep learning. It is designed to be easy to get started with and simple to use for Java developers. DJL provides a native Java development experience and allows users to integrate machine learning and deep learning models with their Java applications. The framework is deep learning engine agnostic, enabling users to switch engines at any point for optimal performance. DJL's ergonomic API interface guides users with best practices to accomplish deep learning tasks, such as running inference and training neural networks.
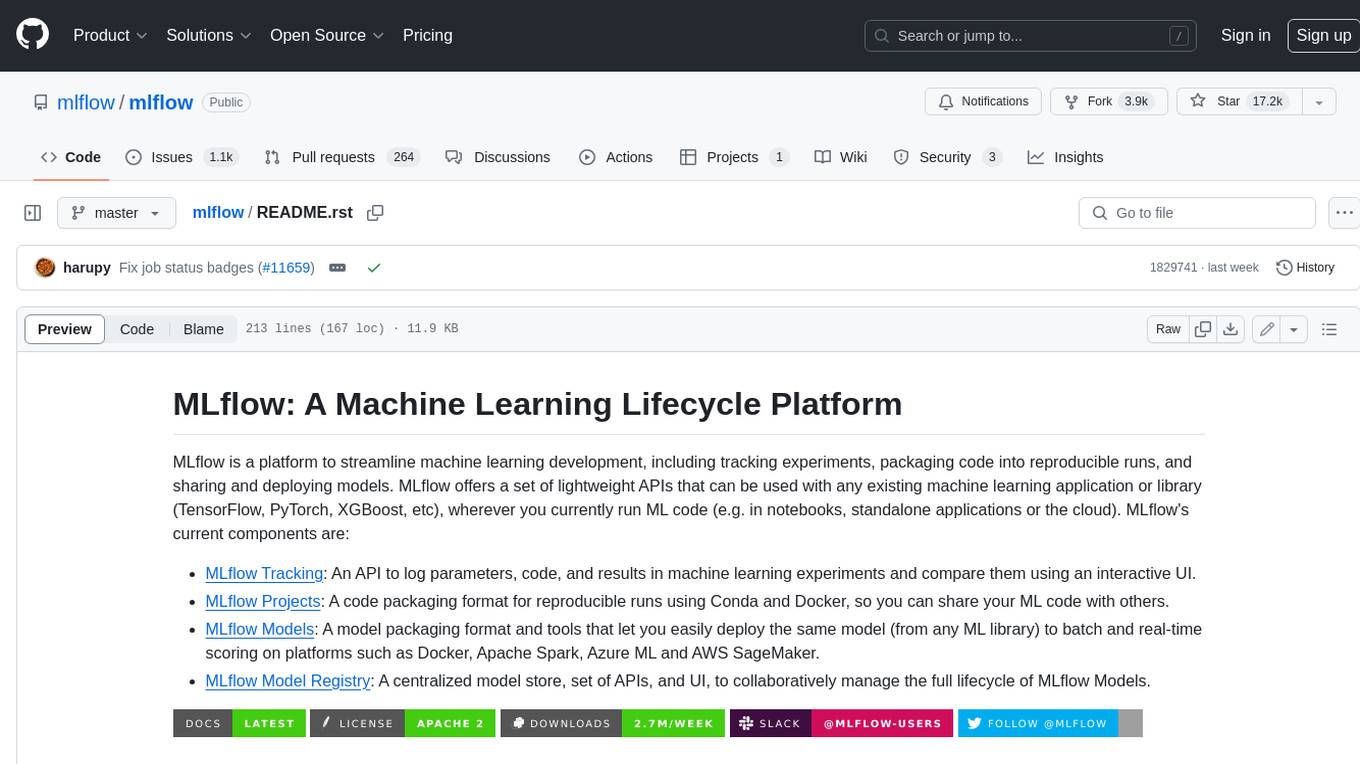
mlflow
MLflow is a platform to streamline machine learning development, including tracking experiments, packaging code into reproducible runs, and sharing and deploying models. MLflow offers a set of lightweight APIs that can be used with any existing machine learning application or library (TensorFlow, PyTorch, XGBoost, etc), wherever you currently run ML code (e.g. in notebooks, standalone applications or the cloud). MLflow's current components are:
* `MLflow Tracking
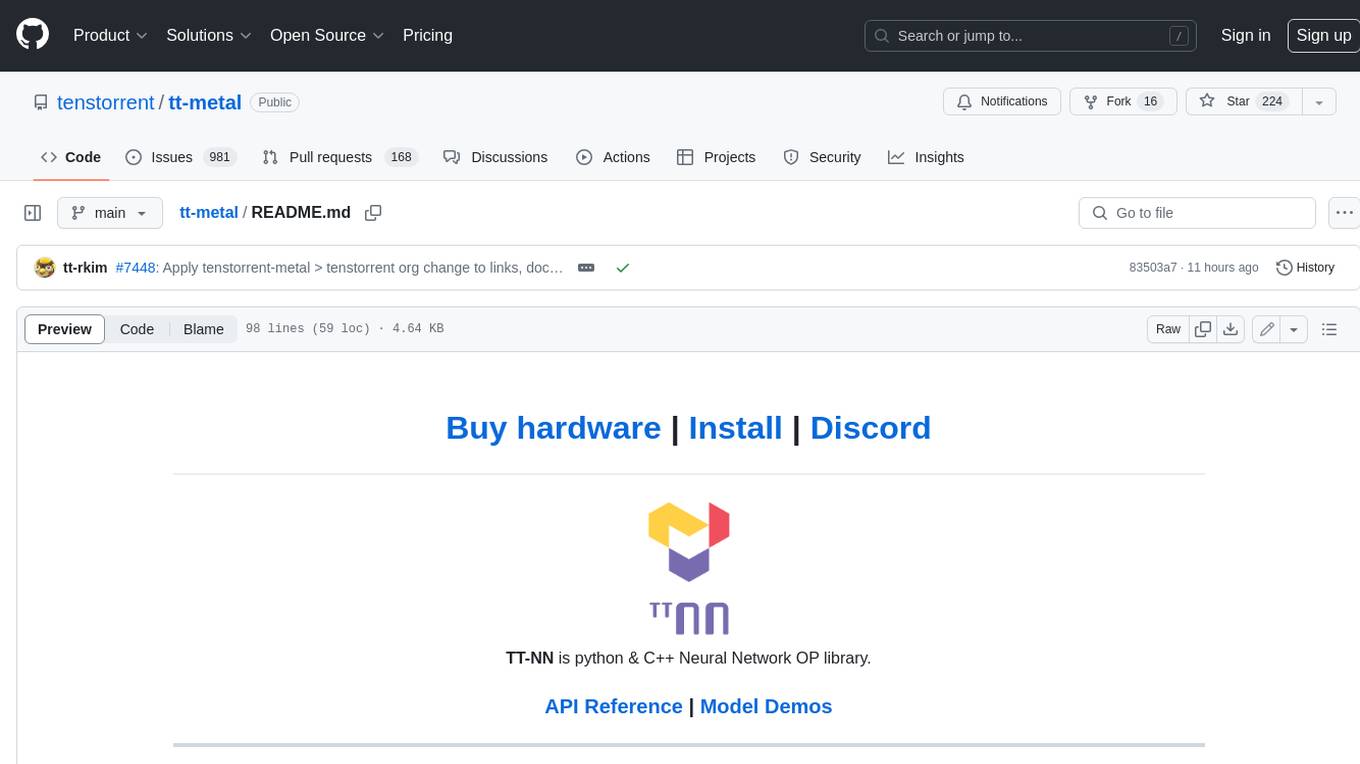
tt-metal
TT-NN is a python & C++ Neural Network OP library. It provides a low-level programming model, TT-Metalium, enabling kernel development for Tenstorrent hardware.
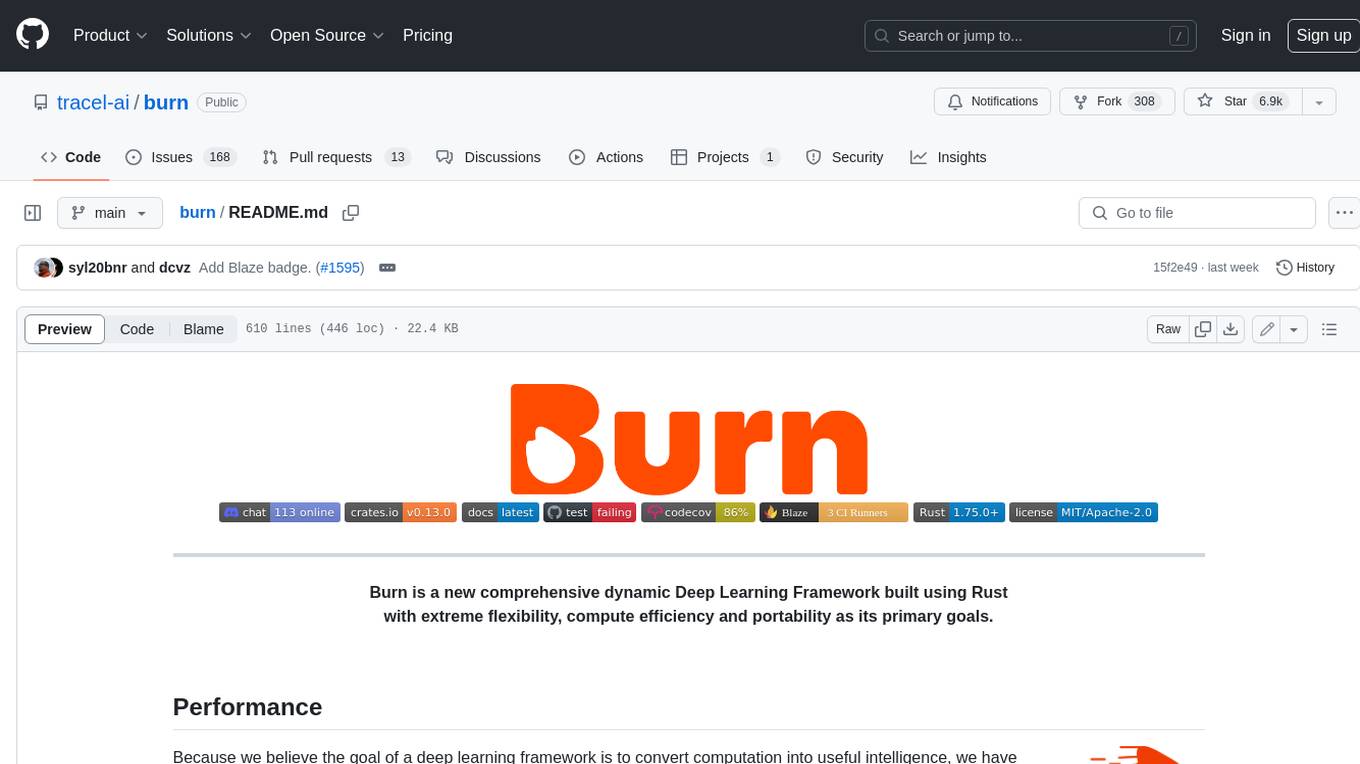
burn
Burn is a new comprehensive dynamic Deep Learning Framework built using Rust with extreme flexibility, compute efficiency and portability as its primary goals.
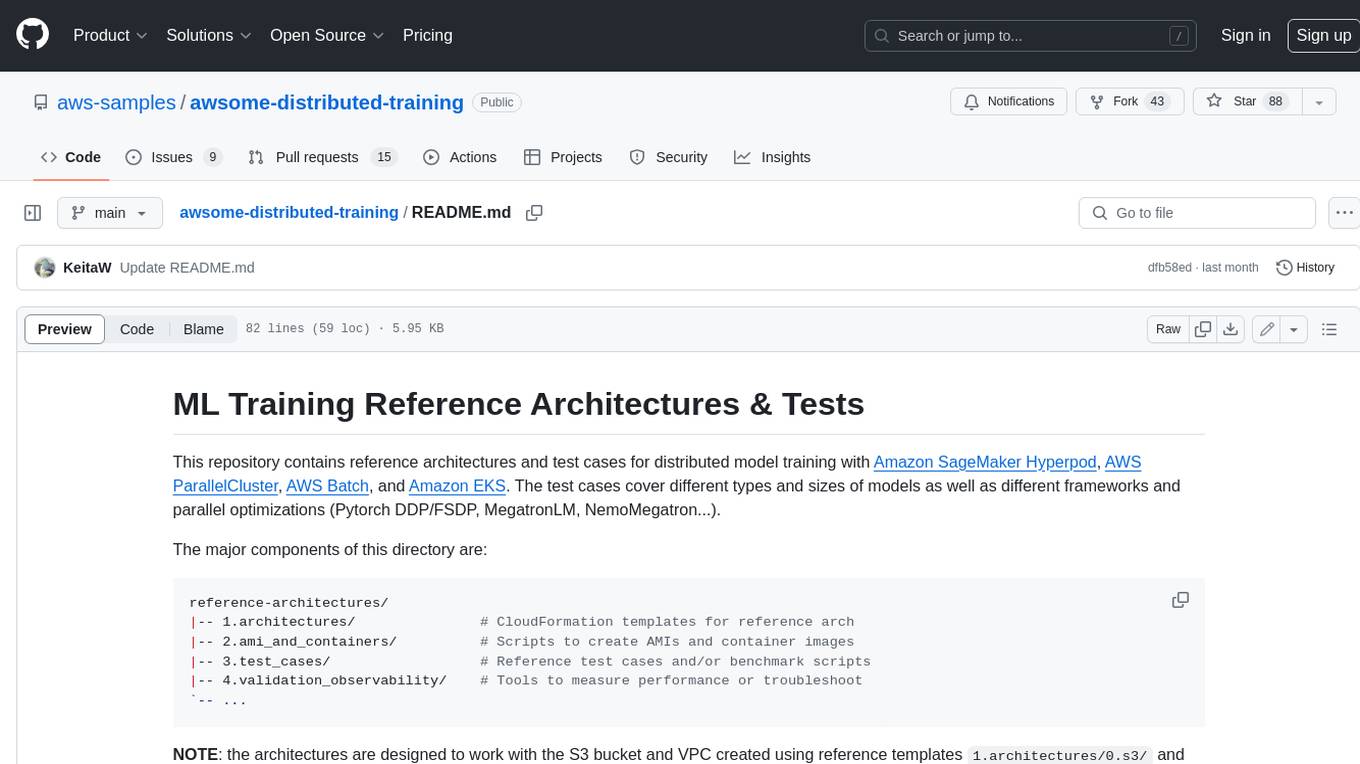
awsome-distributed-training
This repository contains reference architectures and test cases for distributed model training with Amazon SageMaker Hyperpod, AWS ParallelCluster, AWS Batch, and Amazon EKS. The test cases cover different types and sizes of models as well as different frameworks and parallel optimizations (Pytorch DDP/FSDP, MegatronLM, NemoMegatron...).
For similar jobs
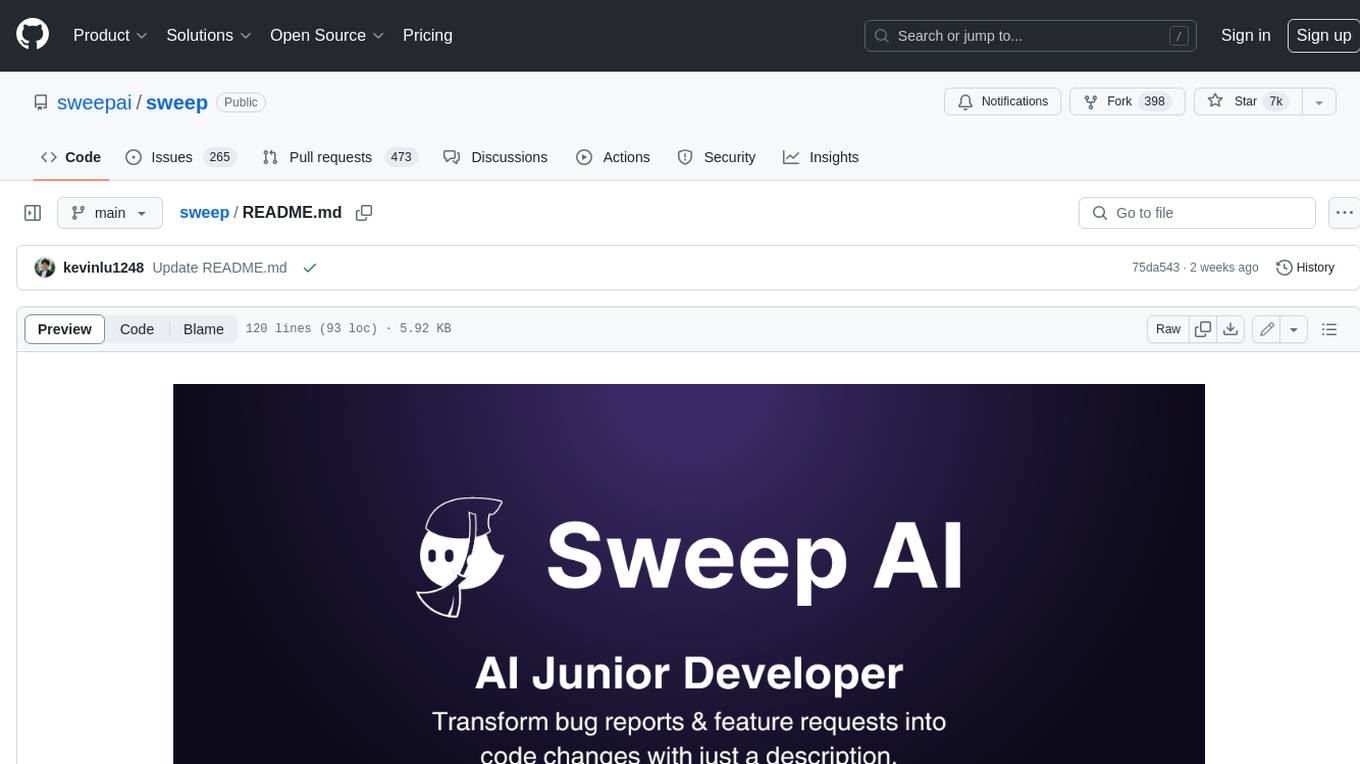
sweep
Sweep is an AI junior developer that turns bugs and feature requests into code changes. It automatically handles developer experience improvements like adding type hints and improving test coverage.
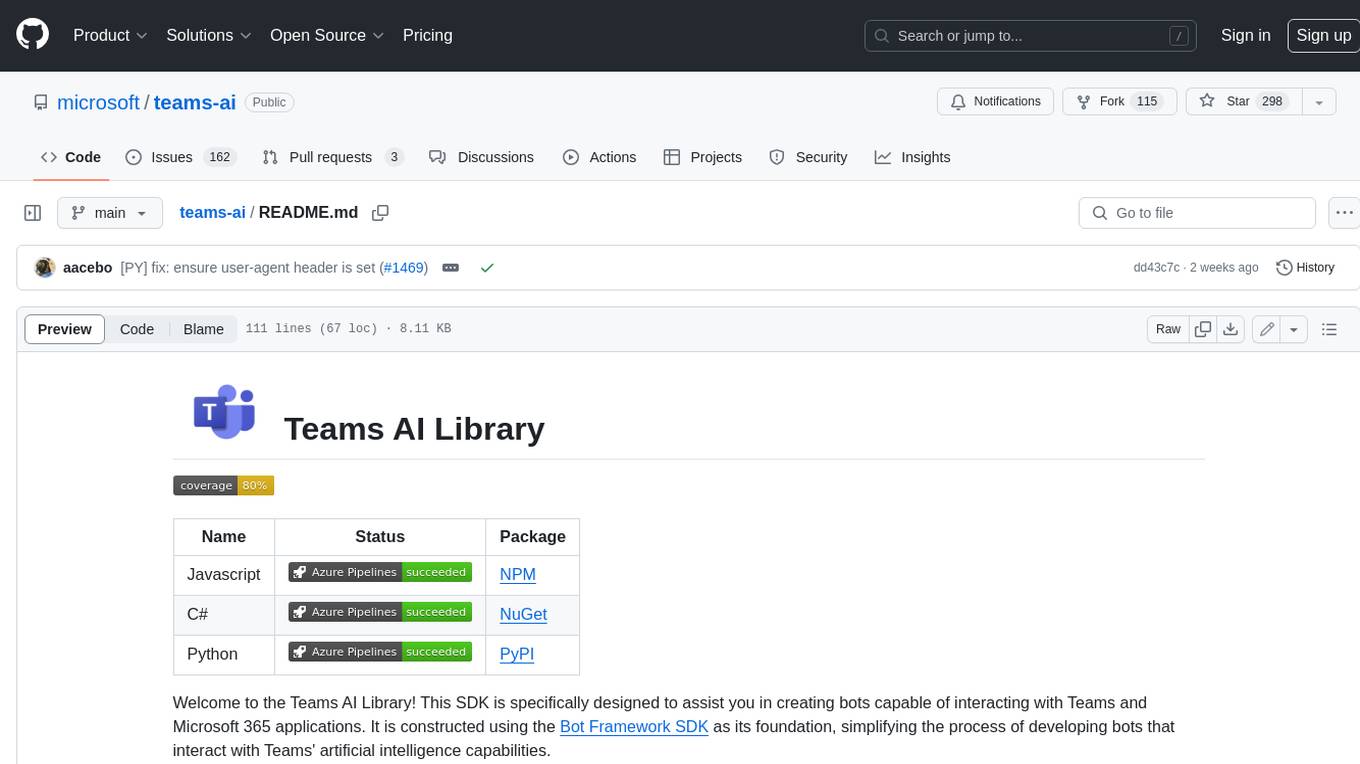
teams-ai
The Teams AI Library is a software development kit (SDK) that helps developers create bots that can interact with Teams and Microsoft 365 applications. It is built on top of the Bot Framework SDK and simplifies the process of developing bots that interact with Teams' artificial intelligence capabilities. The SDK is available for JavaScript/TypeScript, .NET, and Python.
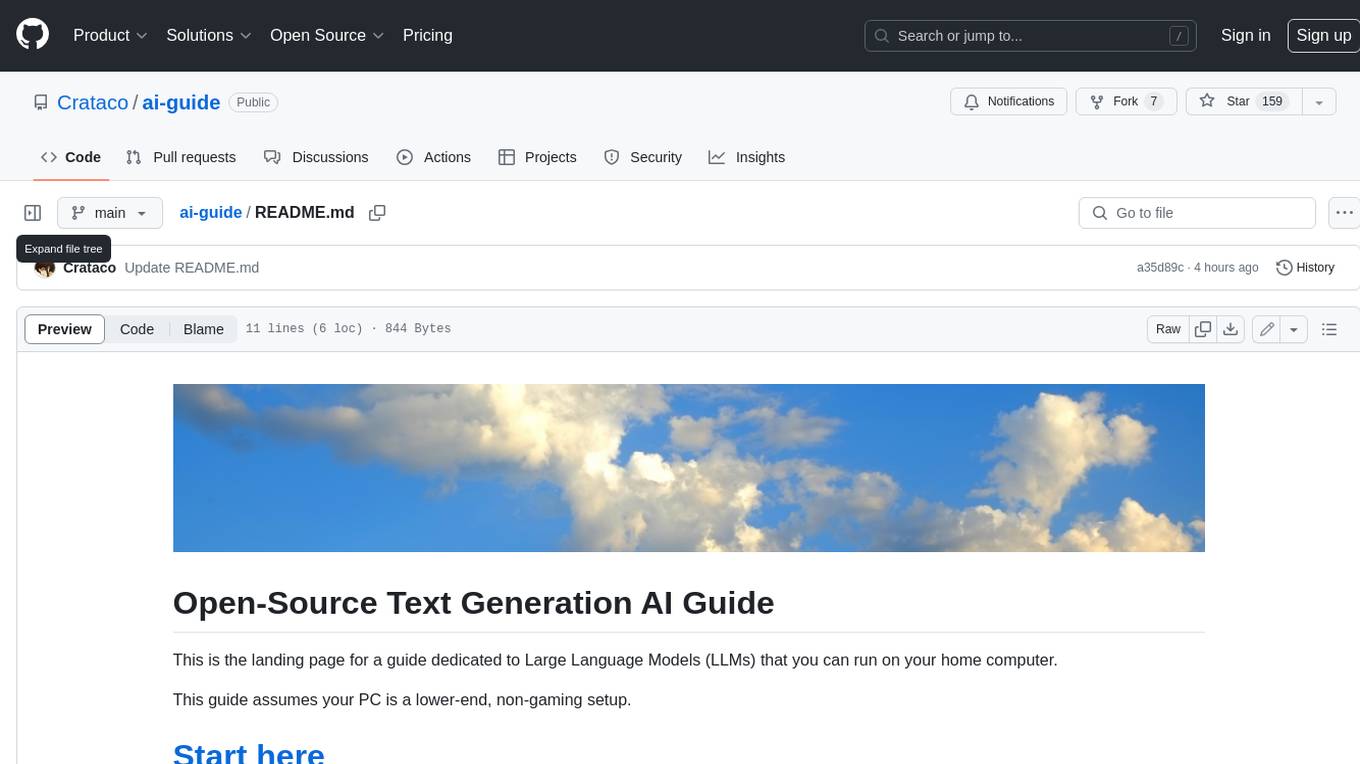
ai-guide
This guide is dedicated to Large Language Models (LLMs) that you can run on your home computer. It assumes your PC is a lower-end, non-gaming setup.
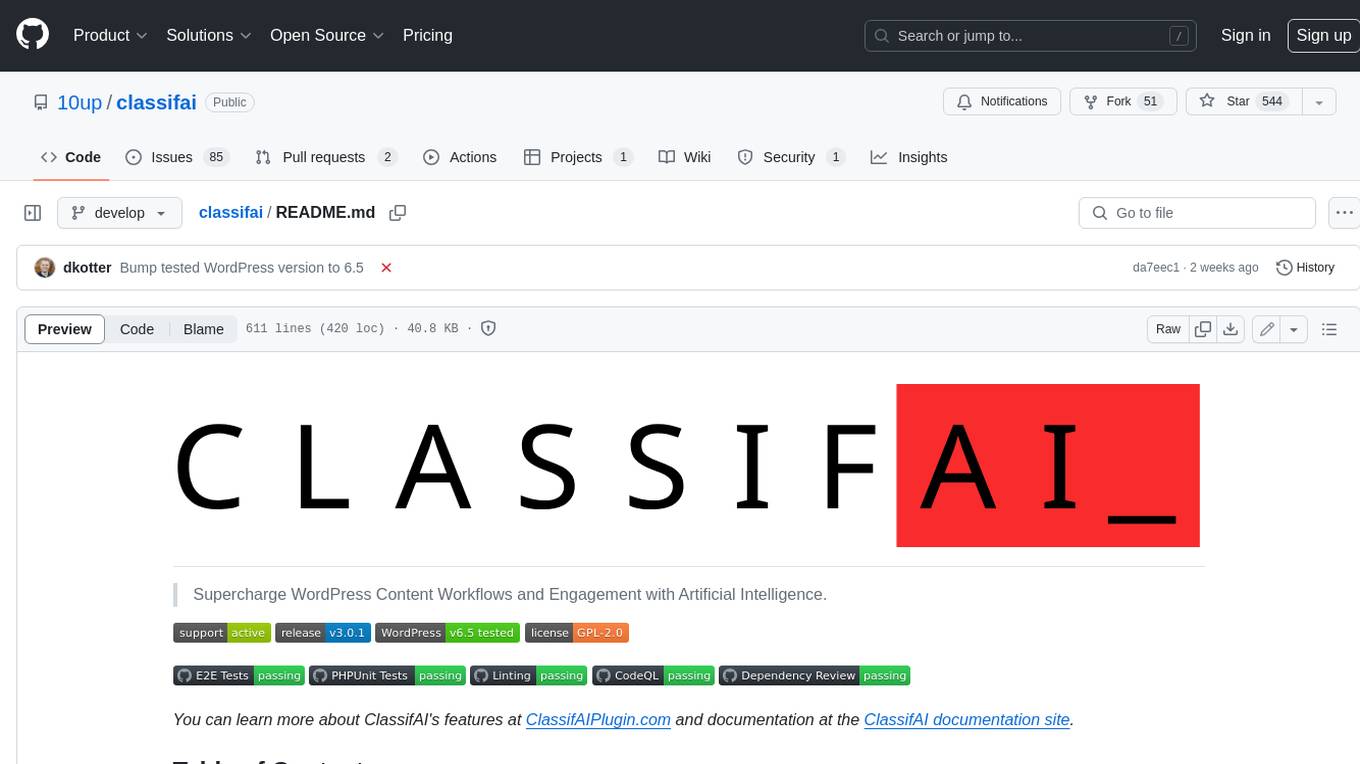
classifai
Supercharge WordPress Content Workflows and Engagement with Artificial Intelligence. Tap into leading cloud-based services like OpenAI, Microsoft Azure AI, Google Gemini and IBM Watson to augment your WordPress-powered websites. Publish content faster while improving SEO performance and increasing audience engagement. ClassifAI integrates Artificial Intelligence and Machine Learning technologies to lighten your workload and eliminate tedious tasks, giving you more time to create original content that matters.
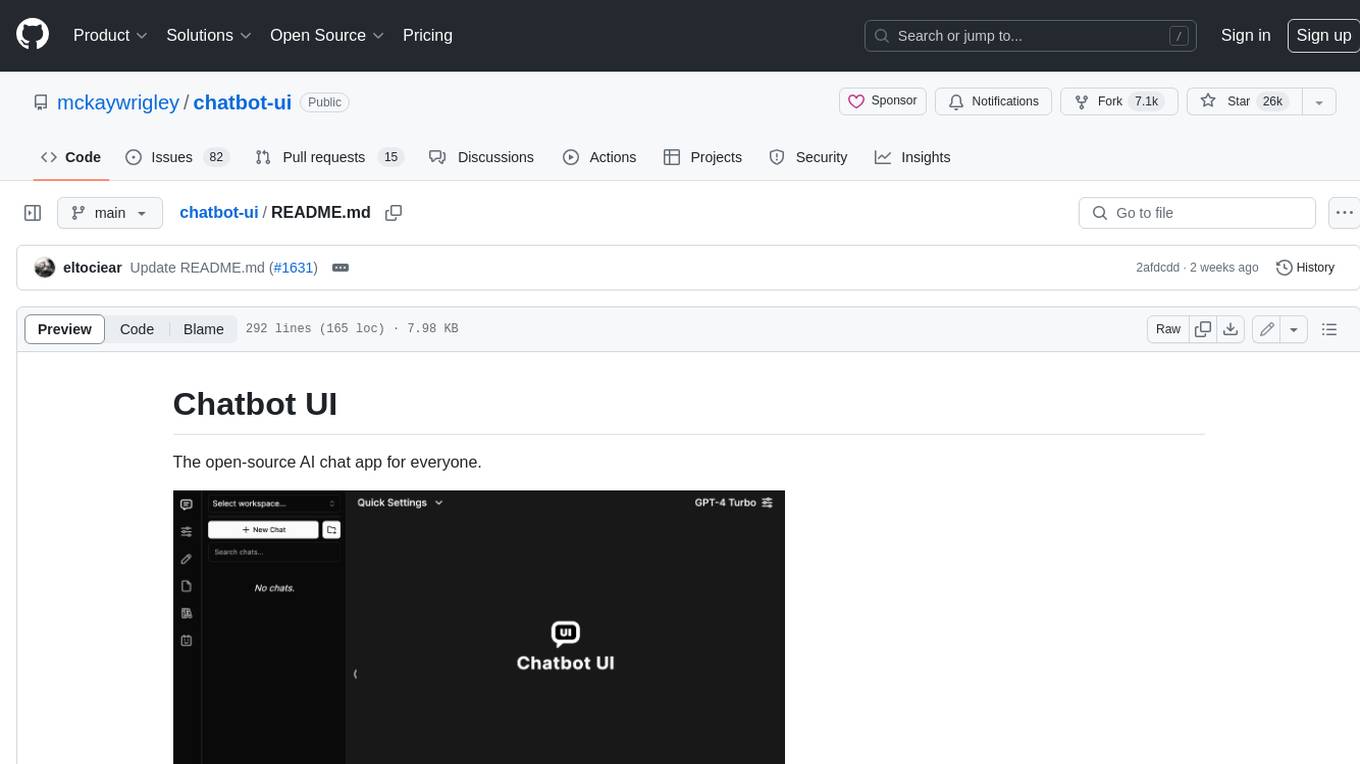
chatbot-ui
Chatbot UI is an open-source AI chat app that allows users to create and deploy their own AI chatbots. It is easy to use and can be customized to fit any need. Chatbot UI is perfect for businesses, developers, and anyone who wants to create a chatbot.
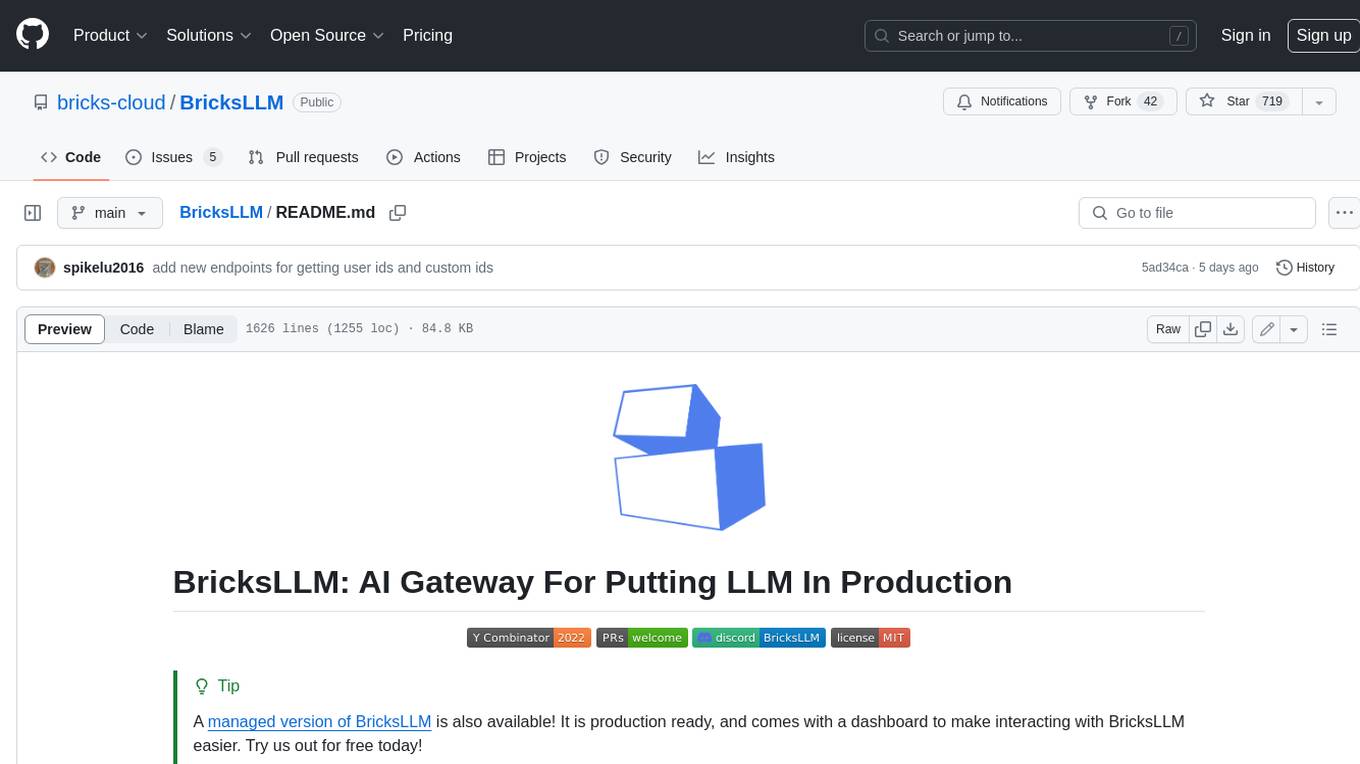
BricksLLM
BricksLLM is a cloud native AI gateway written in Go. Currently, it provides native support for OpenAI, Anthropic, Azure OpenAI and vLLM. BricksLLM aims to provide enterprise level infrastructure that can power any LLM production use cases. Here are some use cases for BricksLLM: * Set LLM usage limits for users on different pricing tiers * Track LLM usage on a per user and per organization basis * Block or redact requests containing PIIs * Improve LLM reliability with failovers, retries and caching * Distribute API keys with rate limits and cost limits for internal development/production use cases * Distribute API keys with rate limits and cost limits for students
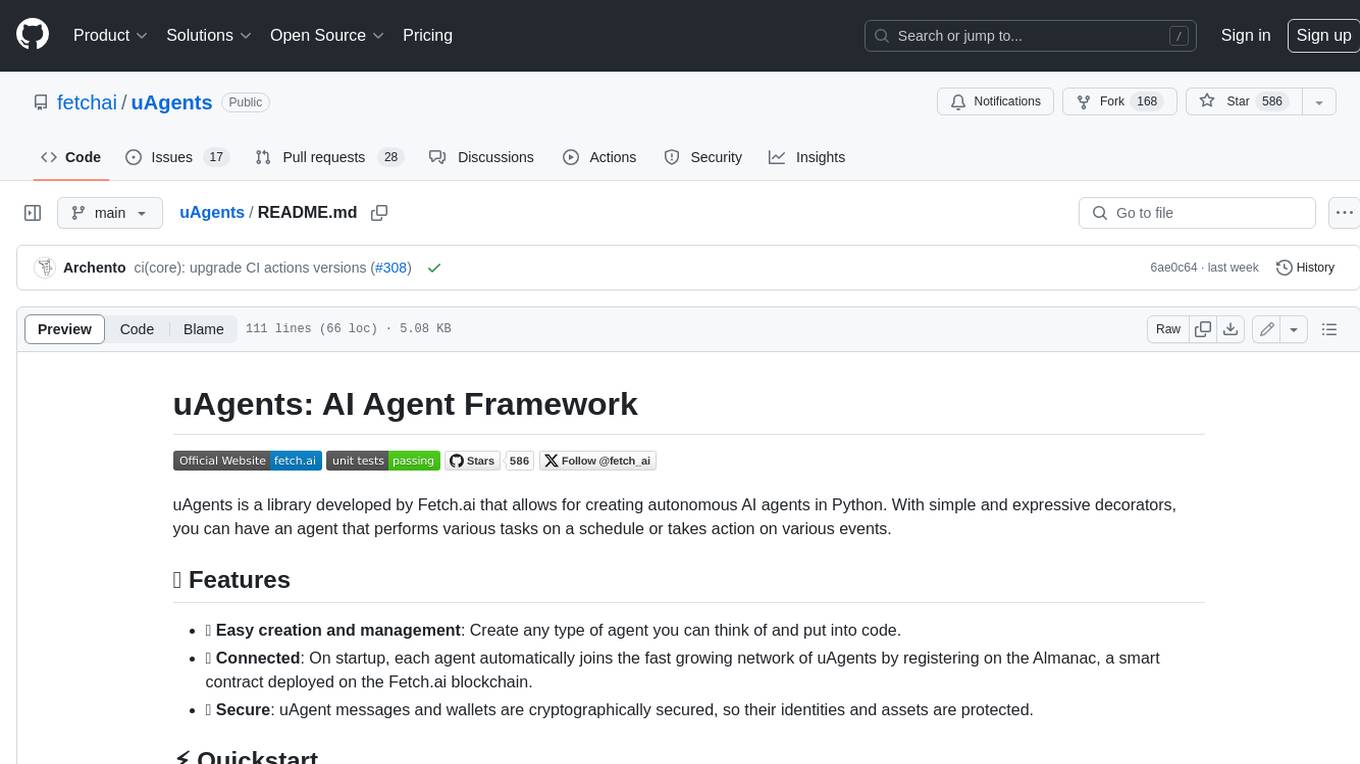
uAgents
uAgents is a Python library developed by Fetch.ai that allows for the creation of autonomous AI agents. These agents can perform various tasks on a schedule or take action on various events. uAgents are easy to create and manage, and they are connected to a fast-growing network of other uAgents. They are also secure, with cryptographically secured messages and wallets.
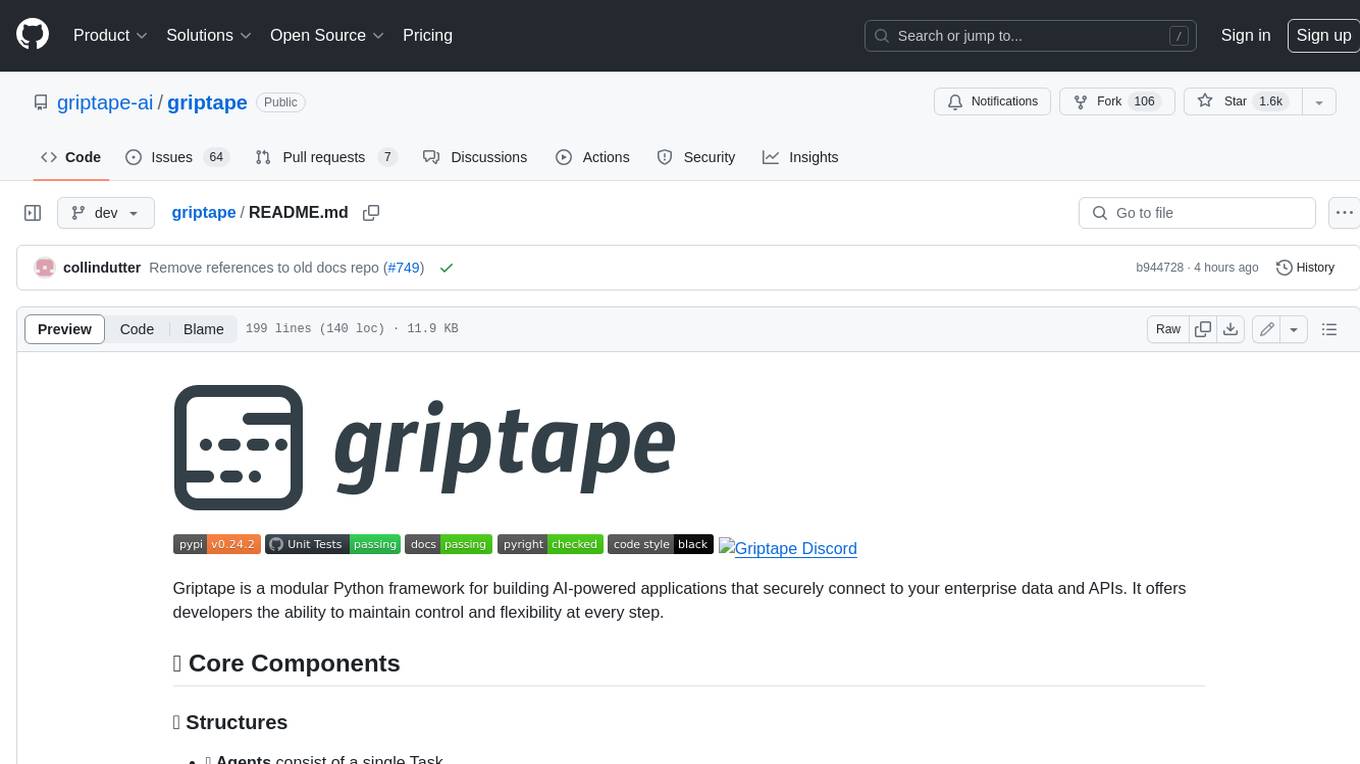
griptape
Griptape is a modular Python framework for building AI-powered applications that securely connect to your enterprise data and APIs. It offers developers the ability to maintain control and flexibility at every step. Griptape's core components include Structures (Agents, Pipelines, and Workflows), Tasks, Tools, Memory (Conversation Memory, Task Memory, and Meta Memory), Drivers (Prompt and Embedding Drivers, Vector Store Drivers, Image Generation Drivers, Image Query Drivers, SQL Drivers, Web Scraper Drivers, and Conversation Memory Drivers), Engines (Query Engines, Extraction Engines, Summary Engines, Image Generation Engines, and Image Query Engines), and additional components (Rulesets, Loaders, Artifacts, Chunkers, and Tokenizers). Griptape enables developers to create AI-powered applications with ease and efficiency.