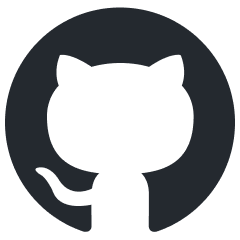
LarAgent
Power of AI Agents in your Laravel project
Stars: 78
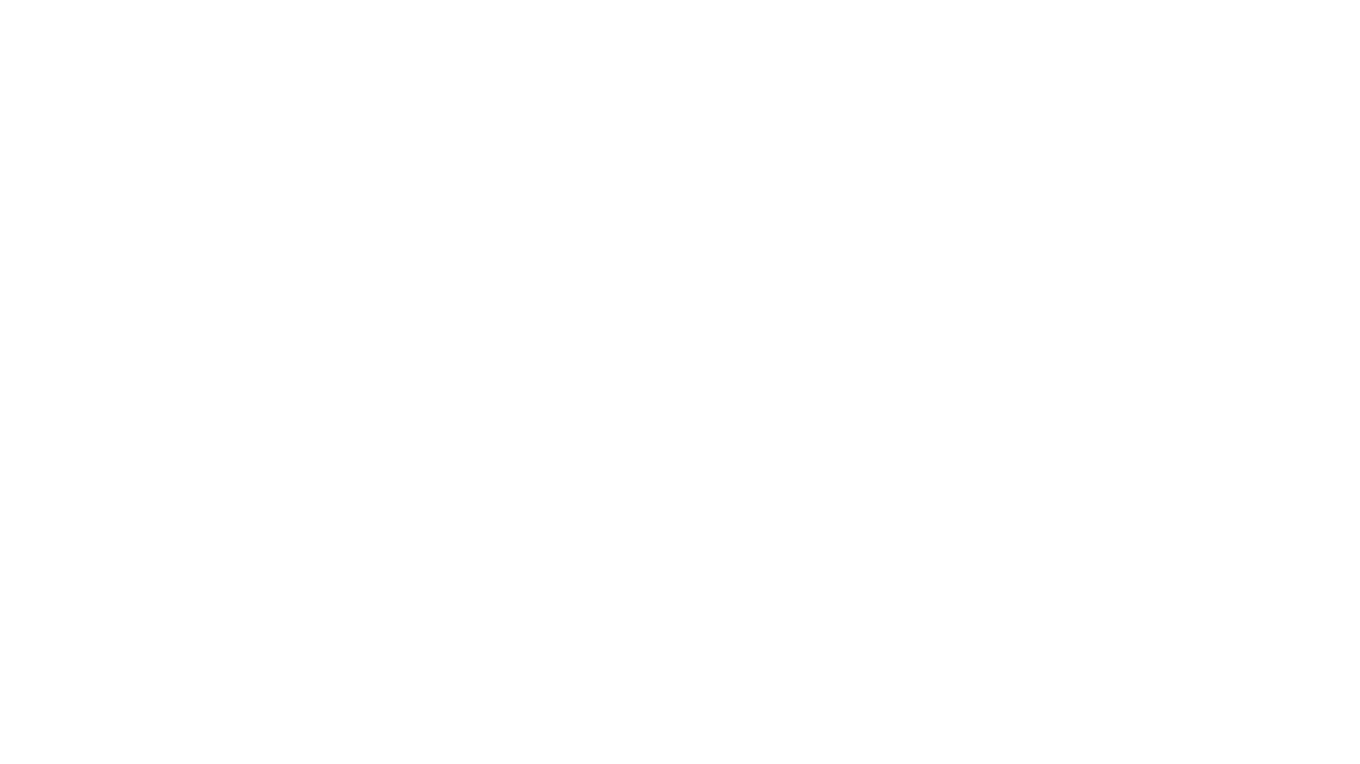
LarAgent is a framework designed to simplify the creation and management of AI agents within Laravel projects. It offers an Eloquent-like syntax for creating and managing AI agents, Laravel-style artisan commands, flexible agent configuration, structured output handling, image input support, and extensibility. LarAgent supports multiple chat history storage options, custom tool creation, event system for agent interactions, multiple provider support, and can be used both in Laravel and standalone environments. The framework is constantly evolving to enhance developer experience, improve AI capabilities, enhance security and storage features, and enable advanced integrations like provider fallback system, Laravel Actions integration, and voice chat support.
README:
The easiest way to create and maintain AI agents in your Laravel projects.
Jump to Table of Contents
Need to use LarAgent outside of Laravel? Check out this Docs.
If you prefer article to get started, check it out Laravel AI Agent Development Made Easy
LarAgent brings the power of AI agents to your Laravel projects with an elegant syntax. Create, extend, and manage AI agents with ease while maintaining Laravel's fluent API design patterns.
What if you can create AI agents just like you create any other Eloquent model?
Why not?! ๐
php artisan make:agent YourAgentName
And it looks familiar, isn't it?
namespace App\AiAgents;
use LarAgent\Agent;
class YourAgentName extends Agent
{
protected $model = 'gpt-4';
protected $history = 'in_memory';
protected $provider = 'default';
protected $tools = [];
public function instructions()
{
return "Define your agent's instructions here.";
}
public function prompt($message)
{
return $message;
}
}
And you can tweak the configs, like history
// ...
protected $history = \LarAgent\History\CacheChatHistory::class;
// ...
Or add temperature
:
// ...
protected $temperature = 0.5;
// ...
Even disable parallel tool calls:
// ...
protected $parallelToolCalls = false;
// ...
Oh, and add a new tool as well:
// ...
#[Tool('Get the current weather in a given location')]
public function exampleWeatherTool($location, $unit = 'celsius')
{
return 'The weather in '.$location.' is '.'20'.' degrees '.$unit;
}
// ...
And run it, per user:
Use App\AiAgents\YourAgentName;
// ...
YourAgentName::forUser(auth()->user())->respond($message);
Or use a custom name for the chat history:
Use App\AiAgents\YourAgentName;
// ...
YourAgentName::for("custom_history_name")->respond($message);
Let's find out more with documentation below ๐
- Eloquent-like syntax for creating and managing AI agents
- Laravel-style artisan commands
- Flexible agent configuration (model, temperature, context window, etc.)
- Structured output handling
- Image input support
- Easily extendable, including chat histories and LLM drivers
- Multiple built-in chat history storage options (in-memory, cache, json, etc.)
- Per-user chat history management
- Custom chat history naming support
- Custom tool creation with attribute-based configuration
- Tools via classes
- Tools via methods of AI agent class (Auto)
-
Tool
facade for shortened tool creation - Parallel tool execution capability (can be disabled)
- Extensive Event system for agent interactions (Nearly everything is hookable)
- Multiple provider support (Can be set per model)
- Support for both Laravel and standalone usage
Here's what's coming next to make LarAgent even more powerful:
-
Artisan Commands for Rapid Development
-
chat-history:clear AgentName
- Clear all chat histories for a specific agent -
make:agent:tool
- Generate tool classes with ready-to-use stubs -
make:agent:chat-history
- Scaffold custom chat history implementations -
make:llm-driver
- Create custom LLM driver integrations
-
- Prism Package Integration - Additional LLM providers support
- Streaming Support - Out-of-the-box support for streaming responses
-
RAG & Knowledge Base
- Built-in vector storage providers
- Seamless document embeddings integration
- Smart context management
- Ready-to-use Tools - Built-in tools as traits
- Structured Output at runtime - Allow defining the response JSON Schema at runtime.
- Enhanced Chat History Security - Optional encryption for sensitive conversations
- Provider Fallback System - Automatic fallback to alternative providers
- Laravel Actions Integration - Use your existing Actions as agent tools
- Voice Chat Support - Out of the box support for voice interactions with your agents
Stay tuned! We're constantly working on making LarAgent the most versatile AI agent framework for Laravel.
- ๐ Introduction
- ๐ Getting Started
- โ๏ธ Core Concepts
- ๐ฅ Events
- ๐ฌ Commands
- ๐ Advanced Usage
- ๐ค Contributing
- ๐งช Testing
- ๐ Security
- ๐ Credits
- ๐ License
- ๐ฃ๏ธ Roadmap
- Laravel 10.x or higher
- PHP 8.3 or higher
You can install the package via composer:
composer require maestroerror/laragent
You can publish the config file with:
php artisan vendor:publish --tag="laragent-config"
This is the contents of the published config file:
return [
'default_driver' => \LarAgent\Drivers\OpenAi\OpenAiDriver::class,
'default_chat_history' => \LarAgent\History\InMemoryChatHistory::class,
'providers' => [
'default' => [
'name' => 'openai',
'api_key' => env('OPENAI_API_KEY'),
'default_context_window' => 50000,
'default_max_completion_tokens' => 100,
'default_temperature' => 1,
],
],
];
You can configure the package by editing the config/laragent.php
file. Here is an example of custom provider with all possible configurations you can apply:
// Example custom provider with all possible configurations
'custom_provider' => [
// Just name for reference, changes nothing
'name' => 'mini',
'model' => 'gpt-3.5-turbo',
'api_key' => env('CUSTOM_API_KEY'),
'api_url' => env('CUSTOM_API_URL'),
// Default driver and chat history
'driver' => \LarAgent\Drivers\OpenAi\OpenAiDriver::class,
'chat_history' => \LarAgent\History\InMemoryChatHistory::class,
'default_context_window' => 15000,
'default_max_completion_tokens' => 100,
'default_temperature' => 1,
// Enable/disable parallel tool calls
'parallel_tool_calls' => true,
// Store metadata with messages
'store_meta' => true,
// Save chat keys to memory via chatHistory
'save_chat_keys' => true,
],
Provider just gives you the defaults. Every config can be overridden per agent in agent class.
@todo Table of contents for Agents section
Agents are the core of LarAgent. They represent a conversational AI model that can be used to interact with users, systems, or any other source of input.
You can create a new agent by extending the LarAgent\Agent
class. This is the foundation for building your custom AI agent with specific capabilities and behaviors.
namespace App\AiAgents;
use LarAgent\Agent;
class MyAgent extends Agent
{
// Your agent implementation
}
For rapid development, you can use the artisan command to generate a new agent with a basic structure:
php artisan make:agent MyAgent
This will create a new agent class in the App\AiAgents
directory with all the necessary boilerplate code.
Agents can be configured through various properties and methods to customize their behavior. Here are the core configuration options:
/** @var string - Define the agent's behavior and role */
protected $instructions;
/** @var string - Create your or Choose from built-in chat history: "in_memory", "session", "cache", "file", or "json" */
protected $history;
/** @var string - Specify which LLM driver to use */
protected $driver;
/** @var string - Select the AI provider configuration from your config file */
protected $provider = 'default';
/** @var string - Choose which language model to use */
protected $model = 'gpt-4o-mini';
/** @var int - Set the maximum number of tokens in the completion */
protected $maxCompletionTokens;
/** @var float - Control response creativity (0.0 for focused, 2.0 for creative) */
protected $temperature;
/** @var string|null - Current message being processed */
protected $message;
The agent also provides three core methods that you can override:
/**
* Define the agent's system instructions
* This sets the behavior, role, and capabilities of your agent
* For simple textual instructions, use the `instructions` property
* For more complex instructions or dynamic behavior, use the `instructions` method
*/
public function instructions()
{
return "Define your agent's instructions here.";
}
/**
* Customize how messages are processed before sending to the AI
* Useful for formatting, adding context (RAG), or standardizing input
*/
public function prompt(string $message)
{
return $message;
}
/**
* Decide which model to use dynamically with custom logic
* Or use property $model to statically set the model
*/
public function model()
{
return $this->model;
}
Example:
class WeatherAgent extends Agent
{
protected $model = 'gpt-4';
protected $history = 'cache';
protected $temperature = 0.7;
public function instructions()
{
return "You are a weather expert assistant. Provide accurate weather information.";
}
public function prompt(string $message)
{
return "Weather query: " . $message;
}
}
There are two ways to interact with your agent: direct response or chainable methods.
The simplest way is to use the for()
method to specify a chat history name and get an immediate response:
// Using a specific chat history name
echo WeatherAgent::for('test_chat')->respond('What is the weather like?');
For more control over the interaction, you can use the chainable syntax:
$response = WeatherAgent::for('test_chat')
->message('What is the weather like?') // Set the message
->temperature(0.7) // Optional: Override temperature
->respond(); // Get the response
The for()
and forUser()
method allows you to maintain separate conversation histories for different contexts or users:
// Different histories for different users
echo WeatherAgent::for('user_1_chat')->respond('What is the weather like?');
echo WeatherAgent::for('user_2_chat')->respond('How about tomorrow?');
echo WeatherAgent::forUser(auth()->user())->respond('How about tomorrow?');
Here are some chainable methods to modify the agents behavior on the fly:
/**
* Set the message for the agent to process
*/
public function message(string $message);
/**
* Add images to the agent's input (mesasge)
* @param array $imageUrls Array of image URLs
*/
public function withImages(array $imageUrls);
/**
* Decide model dynamically in your controller
* @param string $model Model identifier (e.g., 'gpt-4o', 'gpt-3.5-turbo')
*/
public function withModel(string $model);
/**
* Clear the chat history
* This removes all messages from the chat history
*/
public function clear();
/**
* Set other chat history instance
*/
public function setChatHistory(ChatHistoryInterface $chatHistory);
/**
* Add tool to the agent's registered tools
*/
public function withTool(ToolInterface $tool);
/**
* Remove tool for this specific call
*/
public function removeTool(string $name);
/**
* Override the temperature for this specific call
*/
public function temperature(float $temp);
You can access the agent's properties using these methods on an instance of the agent:
/**
* Get the current chat session ID
* String like "[AGENT_NAME]_[MODEL_NAME]_[CHAT_NAME]"
* CHAT_NAME is defined by "for" method
* Example: WeatherAgent_gtp-4o-mini_test-chat
*/
public function getChatSessionId(): string;
/**
* Returns the provider name
*/
public function getProviderName(): string;
/**
* Returns an array of registered tools
*/
public function getTools(): array;
/**
* Returns current chat history instance
*/
public function chatHistory(): ChatHistoryInterface;
/**
* Returns the current message
*/
public function currentMessage(): ?string;
/**
* Returns the last message
*/
public function lastMessage(): ?MessageInterface;
/**
* Get all chat keys associated with this agent class
*/
public function getChatKeys(): array;
Tools are used to extend the functionality of agents. They can be used to perform tasks such as sending messages, running jobs, making API calls, or executing shell commands.
Here's a quick example of creating a tool using the #[Tool]
attribute:
use LarAgent\Attributes\Tool;
// ...
#[Tool('Get the current weather')]
public function getWeather(string $city)
{
return WeatherService::getWeather($city);
}
Tools in LarAgent can be configured using these properties:
/** @var bool - Controls whether tools can be executed in parallel */
protected $parallelToolCalls;
/** @var array - List of tool classes to be registered with the agent */
protected $tools = [];
There are three ways to create and register tools in your agent:
-
Using the registerTools Method
This method allows you to programmatically create and register tools using the
LarAgent\Tool
class:
use LarAgent\Tool;
// ...
public function registerTools()
{
return [
$user = auth()->user();
Tool::create("user_location", "Returns user's current location")
->setCallback(function () use ($user) {
return $user->location()->city;
}),
Tool::create("get_current_weather", "Returns the current weather in a given location")
->addProperty("location", "string", "The city and state, e.g. San Francisco, CA")
->setCallback("getWeather"),
];
}
-
Using the #[Tool] Attribute
The
#[Tool]
attribute provides a simple way to create tools from class methods:
use LarAgent\Attributes\Tool;
// Basic tool with parameters
#[Tool('Get the current weather in a given location')]
public function weatherTool($location, $unit = 'celsius')
{
return 'The weather in '.$location.' is '.'20'.' degrees '.$unit;
}
Agent will automatically register tool with given description as Tool
attribute's first argument and other method info,
such as method name, required and optional parameters.
Tool
attribute also accepts a second argument, which is an array mapping parameter names to their descriptions for more precise control. Also, it can be used with Static methods and parameters with Enum as type, where you can specify the values for the Agent to choose from.
Enum
namespace App\Enums;
enum Unit: string
{
case CELSIUS = 'celsius';
case FAHRENHEIT = 'fahrenheit';
}
Agent class
use LarAgent\Attributes\Tool;
use App\Enums\Unit;
// ...
#[Tool(
'Get the current weather in a given location',
['unit' => 'Unit of temperature', 'location' => 'The city and state, e.g. San Francisco, CA']
)]
public static function weatherToolForNewYork(Unit $unit, $location = 'New York')
{
return WeatherService::getWeather($location, $unit->value);
}
So the tool registered for your LLM will define $unit
as enum of "celsius" and "fahrenheit" and required parameter, but $location
will be optional, of course with coresponding descriptions from Tool
attribute's second argument.
Recommended to use #[Tool]
attribute with static methods if there is no need for agent instance ($this)
-
Using Tool Classes
You can create separate tool classes and add them to the
$tools
property:
protected $tools = [
WeatherTool::class,
LocationTool::class
];
It's recommended to use tool classes with any complex workflows as they provide: more control over the tool's behavior, maintainability and reusability (can be easily used in different agents).
Tool creation command coming soon
Tool class example:
class WeatherTool extends LarAgent\Tool
{
protected string $name = 'get_current_weather';
protected string $description = 'Get the current weather in a given location';
protected array $properties = [
'location' => [
'type' => 'string',
'description' => 'The city and state, e.g. San Francisco, CA',
],
'unit' => [
'type' => 'string',
'description' => 'The unit of temperature',
'enum' => ['celsius', 'fahrenheit'],
],
];
protected array $required = ['location'];
protected array $metaData = ['sent_at' => '2024-01-01'];
public function execute(array $input): mixed
{
// Call the weather API
return 'The weather in '.$input['location'].' is '.rand(10, 60).' degrees '.$input['unit'];
}
}
Chat history is used to store the conversation history between the user and the agent. LarAgent provides several built-in chat history implementations and allows for custom implementations.
In Laravel:
protected $history = 'in_memory'; // Stores chat history temporarily in memory (lost after request)
protected $history = 'session'; // Uses Laravel's session storage
protected $history = 'cache'; // Uses Laravel's cache system
protected $history = 'file'; // Stores in files (storage/app/chat-histories)
protected $history = 'json'; // Stores in JSON files (storage/app/chat-histories)
Outside Laravel:
LarAgent\History\InMemoryChatHistory::class // Stores chat history in memory
LarAgent\History\JsonChatHistory::class // Stores in JSON files
Chat histories can be configured using these properties in your Agent class.
reinjectInstructionsPer
/** @var int - Number of messages after which to reinject the agent's instructions */
protected $reinjectInstructionsPer;
Instructions are always injected at the beginning of the chat history, $reinjectInstructionsPer
defined when to reinject the instructions. By default it is set to 0
(disabled).
contextWindowSize
/** @var int - Maximum number of tokens to keep in context window */
protected $contextWindowSize;
After the context window is exceeded, the oldest messages are removed until the context window is satisfied or the limit is reached. You can implement custom logic for the context window management using events and chat history instance inside your agent.
storeMeta
/** @var bool - Whether to store additional metadata with messages */
protected $storeMeta;
Some LLM drivers such as OpenAI provide additional data with the response, such as token usage, completion time, etc. By default it is set to false
(disabled).
saveChatKeys
/** @var bool - Store chat keys in memory */
protected $saveChatKeys;
By default it is true, since it is required for chat history bunch clearing command to work.
You can create your own chat history by implementing the ChatHistoryInterface
and extending the LarAgent\Core\Abstractions\ChatHistory
abstract class.
Check example implementations in src/History
There are two ways to register your custom chat history into an agent. If you use standard constructor only with $name
parameter, you can define it by class in $history
property or provider configuration:
Agent Class
protected $history = \App\ChatHistories\CustomChatHistory::class;
Provider Configuration (config/laragent.php)
'chat_history' => \App\ChatHistories\CustomChatHistory::class,
If you need any other configuration other than $name
, you can override createChatHistory()
method:
public function createChatHistory($name)
{
return new \App\ChatHistories\CustomChatHistory($name, ['folder' => __DIR__.'/history']);
}
Chat histories are automatically managed based on the chat session ID. You can use the for()
or forUser()
methods to specify different chat sessions:
// Using specific chat history name
$agent = WeatherAgent::for('weather-chat');
// Using user-specific chat history
$agent = WeatherAgent::forUser(auth()->user());
// Clear chat history
$agent->clear();
// Get last message
$lastMessage = $agent->lastMessage();
You can access chat history instance with chatHistory()
method from the agent instance:
// Access chat history instance
$history = $agent->chatHistory();
Here are several methods you can use with Chat History:
public function addMessage(MessageInterface $message): void;
public function getMessages(): array;
public function getIdentifier(): string;
public function getLastMessage(): ?MessageInterface;
public function count(): int;
public function clear(): void;
public function toArray(): array;
public function toArrayWithMeta(): array;
public function setContextWindow(int $tokens): void;
public function exceedsContextWindow(int $tokens): bool;
The chat history is created with the following configuration:
$historyInstance = new $historyClass($sessionId, [
'context_window' => $this->contextWindowSize, // Control token limit
'store_meta' => $this->storeMeta, // Store additional message metadata
]);
Structured output allows you to define the exact format of the agent's response using JSON Schema. When structured output is enabled, the respond()
method will return an array instead of a string, formatted according to your schema.
You can define the response schema in your agent class using the $responseSchema
property:
protected $responseSchema = [
'name' => 'weather_info',
'schema' => [
'type' => 'object',
'properties' => [
'temperature' => [
'type' => 'number',
'description' => 'Temperature in degrees'
],
],
'required' => ['temperature']
'additionalProperties' => false,
],
'strict' => true,
];
For defining more complex schemas you can add the structuredOutput
method in you agent class:
public function structuredOutput()
{
return [
'name' => 'weather_info',
'schema' => [
'type' => 'object',
'properties' => [
'temperature' => [
'type' => 'number',
'description' => 'Temperature in degrees'
],
'conditions' => [
'type' => 'string',
'description' => 'Weather conditions (e.g., sunny, rainy)'
],
'forecast' => [
'type' => 'array',
'items' => [
'type' => 'object',
'properties' => [
'day' => ['type' => 'string'],
'temp' => ['type' => 'number']
],
'required' => ['day', 'temp'],
'additionalProperties' => false,
],
'description' => '5-day forecast'
]
],
'required' => ['temperature', 'conditions']
'additionalProperties' => false,
],
'strict' => true,
];
}
Pay attention to "required", "additionalProperties", and "strict" properties - it is recommended by OpenAI to set them when defining the schema to get the exact structure you need
The schema follows the JSON Schema specification and supports all its features including:
- Basic types (string, number, boolean, array, object)
- Required properties
- Nested objects and arrays
- Property descriptions
- Enums and patterns
When structured output is defined, the agent's response will be automatically formatted and returned as an array according to the schema:
// Returns:
[
'temperature' => 25.5,
'conditions' => 'sunny',
'forecast' => [
['day' => 'tomorrow', 'temp' => 28],
['day' => 'Wednesday', 'temp' => 24]
]
]
The schema can be accessed or modified using the structuredOutput()
method at runtime:
// Get current schema
$schema = $agent->structuredOutput();
// Check if structured output is enabled
if ($agent->structuredOutput()) {
// Handle structured response
}
Agent classes is powered by LarAgent's main class LarAgent\LarAgent
, which often refered as "LarAgent engine".
Laragent engine is standalone part which holds all abstractions and doesn't depend on Laravel. It is used to create and manage agents, tools, chat histories, structured output and etc.
So you can use LarAgent's engine outside of Laravel as well. Usage is a bit different than inside Laravel, but the principles are the same.
Check out the Docs for more information.
LarAgent provides a comprehensive event system that allows you to hook into various stages of the agent's lifecycle and conversation flow. The event system is divided into two main types of hooks:
-
Agent Hooks: These hooks are focused on the agent's lifecycle events such as initialization, conversation flow, and termination. They are perfect for setting up agent-specific configurations, handling conversation state, and managing cleanup operations.
-
Engine Hooks: These hooks dive deeper into the conversation processing pipeline, allowing you to intercept and modify the behavior at crucial points such as message handling, tool execution, and response processing. Each engine hook returns a boolean value to control the flow of execution.
Nearly every aspect of LarAgent is hookable, giving you fine-grained control over the agent's behavior. You can intercept and modify:
- Agent lifecycle events
- Message processing
- Tool execution
- Chat history management
- Response handling
- Structured output processing
-
Agent
- onInitialize - Agent initialization
- onConversationStart - New conversation step started
- onConversationEnd - Conversation step completed
- onToolChange - Tool added or removed
- onClear - Chat history cleared
- onTerminate - Agent termination
-
Engine
- beforeReinjectingInstructions - Before system instructions reinjection
- beforeSend & afterSend - Message handling
- beforeSaveHistory - Chat history persistence
- beforeResponse / afterResponse - LLM interaction
- beforeToolExecution / afterToolExecution - Tool execution
- beforeStructuredOutput - Structured output processing
- Using Laravel events with hooks
The Agent class provides several hooks that allow you to tap into various points of the agent's lifecycle. Each hook can be overridden in your agent implementation.
You can find an example implementation for each hook below.
The onInitialize
hook is called when the agent is fully initialized. This is the perfect place to set up any initial state or configurations your agent needs. For example, use logic to set temperature dynamically based on the user type:
protected function onInitialize()
{
if (auth()->check() && auth()->user()->prefersCreative()) {
$this->temperature(1.4);
}
}
This hook is triggered at the beginning of each respond
method call, signaling the start of a new step in conversation. Use this to prepare conversation-specific resources or logging.
// Log agent class and message
protected function onConversationStart()
{
Log::info(
'Starting new conversation',
[
'agent' => self::class,
'message' => $this->currentMessage()
]
);
}
Called at the end of each respond
method, this hook allows you to perform cleanup, logging or any other logic your application might need after a conversation ends.
/** @param MessageInterface|array|null $message */
protected function onConversationEnd($message)
{
// Clean the history
$this->clear();
// Save the last response
DB::table('chat_histories')->insert(
[
'chat_session_id' => $this->chatHistory()->getIdentifier(),
'message' => $message,
]
);
}
This hook is triggered whenever a tool is added to or removed from the agent. It receives the tool instance and a boolean indicating whether the tool was added (true
) or removed (false
).
/**
* @param ToolInterface $tool
* @param bool $added
*/
protected function onToolChange($tool, $added = true)
{
// If 'my_tool' tool is added
if($added && $tool->getName() == 'my_tool') {
// Update metadata
$newMetaData = ['using_in' => self::class, ...$tool->getMetaData()];
$tool->setMetaData($newMetaData);
}
}
Triggered before the agent's chat history is cleared. Use this hook to perform any necessary logic before the chat history is cleared.
protected function onClear()
{
// Backup chat history
file_put_contents('backup.json', json_encode($this->chatHistory()->toArrayWithMeta()));
}
This hook is called when the agent is being terminated. It's the ideal place to perform final cleanup, save state, or close connections.
protected function onTerminate()
{
Log::info('Agent terminated successfully');
}
The Engine provides several hooks that allow fine-grained control over the conversation flow, message handling, and tool execution. Each hook returns a boolean value where true
allows the operation to proceed and false
prevents it.
In most cases, it's wise to throw and handle exception instead of just returning false
, since returning false
silently stops execution
You can override any engine level hook in your agent class.
You can find an example implementations for each hook below.
The beforeReinjectingInstructions
hook is called before the engine reinjects system instructions into the chat history. Use this to modify or validate the chat history before instructions are reinjected or even change the instructions completely.
As mentioned above, instructions are always injected at the beginning of the chat history, $reinjectInstructionsPer
defined when to reinject the instructions again. By default it is set to 0
(disabled).
/**
* @param ChatHistoryInterface $chatHistory
* @return bool
*/
protected function beforeReinjectingInstructions($chatHistory)
{
// Prevent reinjecting instructions for specific chat types
if ($chatHistory->count() > 1000) {
$this->instuctions = view("agents/new_instructions", ['user' => auth()->user()])->render();
}
return true;
}
These hooks are called before and after a message is added to the chat history. Use them to modify, validate, or log messages.
/**
* @param ChatHistoryInterface $history
* @param MessageInterface|null $message
* @return bool
*/
protected function beforeSend($history, $message)
{
// Filter out sensitive information
if ($message && Checker::containsSensitiveData($message->getContent())) {
throw new \Exception("Message contains sensitive data");
}
return true;
}
protected function afterSend($history, $message)
{
// Log successful messages
Log::info('Message sent', [
'session' => $history->getIdentifier(),
'content_length' => Tokenizer::count($message->getContent())
]);
return true;
}
Triggered before the chat history is saved. Perfect for validation or modification of the history before persistence.
protected function beforeSaveHistory($history)
{
// Add metadata before saving
$updatedMeta = [
'saved_at' => now()->timestamp,
'message_count' => $history->count()
...$history->getMetadata()
]
$history->setMetadata($updatedMeta);
return true;
}
These hooks are called before sending a message (message is already added to the chat history) to the LLM and after receiving its response. Use them for request/response manipulation or monitoring.
/**
* @param ChatHistoryInterface $history
* @param MessageInterface|null $message
*/
protected function beforeResponse($history, $message)
{
// Add context to the message
if ($message) {
Log::info('User message: ' . $message->getContent());
}
return true;
}
/**
* @param MessageInterface $message
*/
protected function afterResponse($message)
{
// Process or validate the LLM response
if (is_array($message->getContent())) {
Log::info('Structured response received');
}
return true;
}
These hooks are triggered before and after a tool is executed. Perfect for tool-specific validation, logging, or result modification.
/**
* @param ToolInterface $tool
* @return bool
*/
protected function beforeToolExecution($tool)
{
// Check tool permissions
if (!$this->hasToolPermission($tool->getName())) {
Log::warning("Unauthorized tool execution attempt: {$tool->getName()}");
return false;
}
return true;
}
/**
* @param ToolInterface $tool
* @param mixed &$result
* @return bool
*/
protected function afterToolExecution($tool, &$result)
{
// Modify or format tool results
if (is_array($result)) {
// Since tool result is reference (&$result), we can safely modify it
$result = array_map(fn($item) => trim($item), $result);
}
return true;
}
This hook is called before processing structured output. Use it to modify or validate the response structure.
protected function beforeStructuredOutput(array &$response)
{
// Return false if response contains something unexpected
if (!$this->checkArrayContent($response)) {
return false; // After returning false, the method stops executing and 'respond' will return `null`
}
// Add additional data to output
$response['timestamp'] = now()->timestamp;
return true;
}
LarAgent hooks can be integrated with Laravel's event system to provide more flexibility and better separation of concerns. This allows you to:
- Decouple event handling logic from your agent class
- Use event listeners and subscribers
- Leverage Laravel's event broadcasting capabilities
- Handle events asynchronously using queues
Consider checking Laravel Events documentation before proceeding.
Here's how you can integrate Laravel events with LarAgent hooks.
First, define your event classes:
// app/Events/AgentMessageReceived.php
class AgentMessageReceived
{
use Dispatchable, InteractsWithSockets, SerializesModels;
public function __construct(
public ChatHistoryInterface $history,
public MessageInterface $message
) {}
}
Then, implement the hook in your agent class:
protected function afterSend($history, $message)
{
// Dispatch Laravel event
AgentMessageReceived::dispatch($history, $message);
return true;
}
In case you want to pass agent in event handler, please use toDTO
method: $this->toDTO()
Create dedicated listeners for your agent events:
// app/Listeners/LogAgentMessage.php
class LogAgentMessage
{
public function handle(AgentMessageReceived $event)
{
Log::info('Agent message received', [
'content' => $event->message->getContent(),
'tokens' => Tokenizer::count($event->message->getContent()),
'history_id' => $event->history->getIdentifier()
]);
}
}
Register the event-listener mapping in your EventServiceProvider
:
// app/Providers/EventServiceProvider.php
protected $listen = [
AgentMessageReceived::class => [
LogAgentMessage::class,
NotifyAdminAboutMessage::class,
// Add more listeners as needed
],
];
You can quickly create a new agent using the make:agent
command:
php artisan make:agent AgentName
This will create a new agent class in your app/AiAgents
directory with the basic structure and methods needed to get started.
You can start an interactive chat session with any of your agents using the agent:chat
command:
# Start a chat with default history name
php artisan agent:chat AgentName
# Start a chat with a specific history name
php artisan agent:chat AgentName --history=weather_chat_1
The chat session allows you to:
- Send messages to your agent
- Get responses in real-time
- Use any tools configured for the agent
- Type 'exit' to end the chat session
You can clear all chat history for a specific agent using the agent:chat:clear
command:
php artisan agent:chat:clear AgentName
This command will remove all stored conversations for the specified agent, regarding of the chat history storage method being used (json, cache, file, etc.).
You can create tools which calls another agent and bind the result to the agent to create a chain or complex workflow.
// @todo add example
// @todo add example
// @todo add example
// @todo add example
We welcome contributions to LarAgent! Whether it's improving documentation, fixing bugs, or adding new features, your help is appreciated. Here's how you can contribute:
- Fork the repository
- Clone your fork:
git clone https://github.com/YOUR_USERNAME/LarAgent.git
cd LarAgent
- Install dependencies:
composer install
- Create a new branch:
git checkout -b feature/your-feature-name
-
Code Style
- Use type hints and return types where possible
- Add PHPDoc blocks for classes and methods
- Keep methods focused and concise
-
Testing
- Add tests for new features
- Ensure all tests pass before submitting:
composer test
- Maintain or improve code coverage
-
Documentation
- Update README.md for significant changes
- Add PHPDoc blocks for new classes and methods
- Include examples for new features
-
Commits
- Use clear, descriptive commit messages
- Reference issues and pull requests
- Keep commits focused and atomic
- Update your fork with the latest changes from main:
git remote add upstream https://github.com/MaestroError/LarAgent.git
git fetch upstream
git rebase upstream/main
- Push your changes:
git push origin feature/your-feature-name
- Create a Pull Request with:
- Clear title and description
- List of changes and impact
- Any breaking changes highlighted
- Screenshots/examples if relevant
- Open an issue for bugs or feature requests
- Join discussions in existing issues (@todo add discord channel invite link)
- Reach out to maintainers for guidance
We aim to review all pull requests within a 2 weeks. Thank you for contributing to LarAgent!
composer test
Please review our security policy on how to report security vulnerabilities.
The MIT License (MIT). Please see License File for more information.
Please see Planned for more information on the future development of LarAgent.
For Tasks:
Click tags to check more tools for each tasksFor Jobs:
Alternative AI tools for LarAgent
Similar Open Source Tools
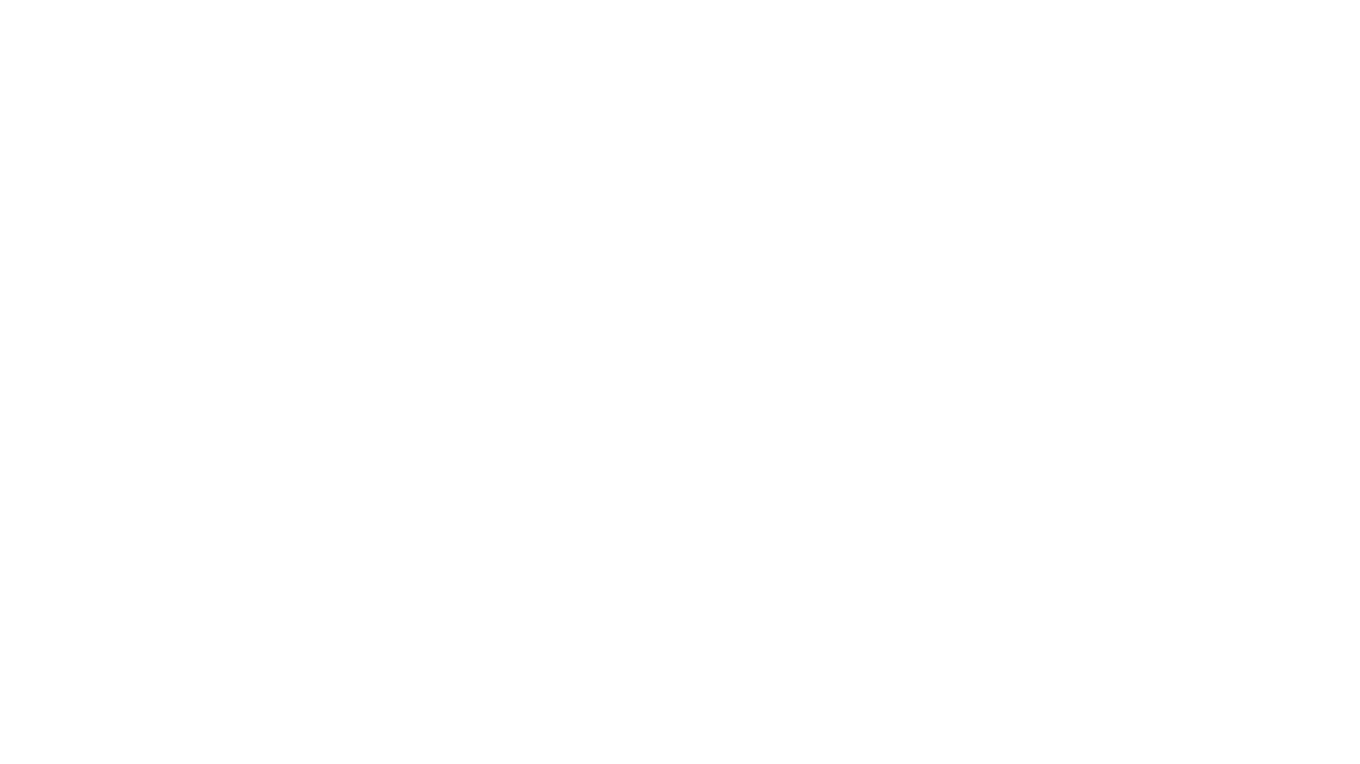
LarAgent
LarAgent is a framework designed to simplify the creation and management of AI agents within Laravel projects. It offers an Eloquent-like syntax for creating and managing AI agents, Laravel-style artisan commands, flexible agent configuration, structured output handling, image input support, and extensibility. LarAgent supports multiple chat history storage options, custom tool creation, event system for agent interactions, multiple provider support, and can be used both in Laravel and standalone environments. The framework is constantly evolving to enhance developer experience, improve AI capabilities, enhance security and storage features, and enable advanced integrations like provider fallback system, Laravel Actions integration, and voice chat support.
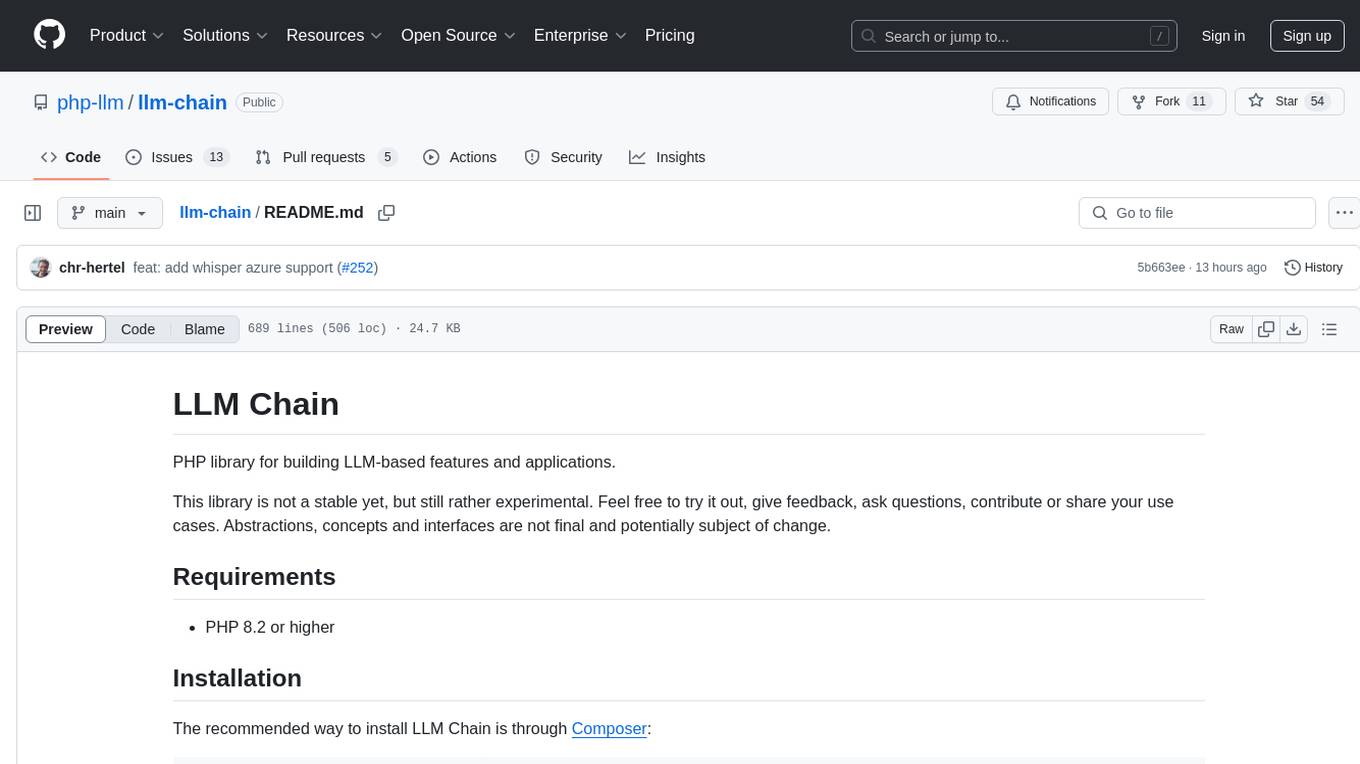
llm-chain
LLM Chain is a PHP library for building LLM-based features and applications. It provides abstractions for Language Models and Embeddings Models from platforms like OpenAI, Azure, Google, Replicate, and others. The core feature is to interact with language models via messages, supporting different message types and content. LLM Chain also supports tool calling, document embedding, vector stores, similarity search, structured output, response streaming, image processing, audio processing, embeddings, parallel platform calls, and input/output processing. Contributions are welcome, and the repository contains fixture licenses for testing multi-modal features.
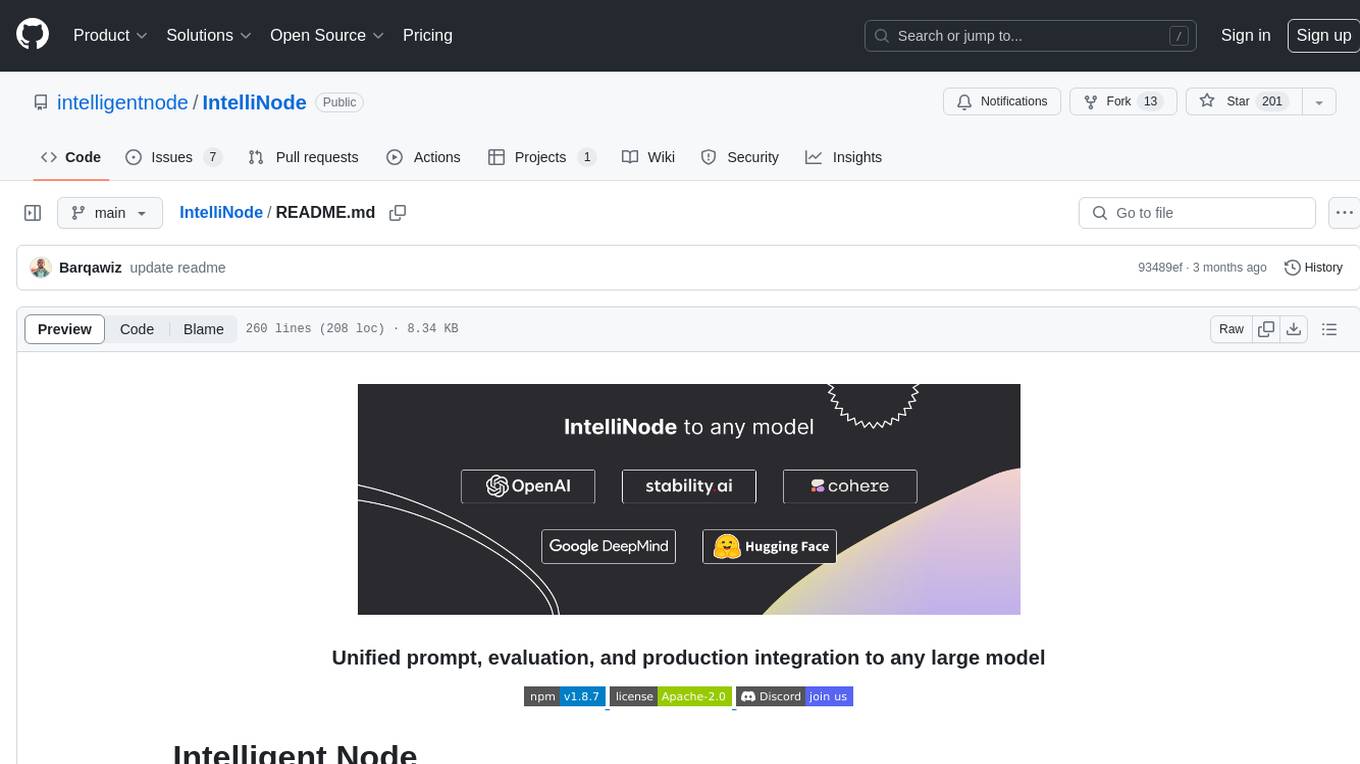
IntelliNode
IntelliNode is a javascript module that integrates cutting-edge AI models like ChatGPT, LLaMA, WaveNet, Gemini, and Stable diffusion into projects. It offers functions for generating text, speech, and images, as well as semantic search, multi-model evaluation, and chatbot capabilities. The module provides a wrapper layer for low-level model access, a controller layer for unified input handling, and a function layer for abstract functionality tailored to various use cases.
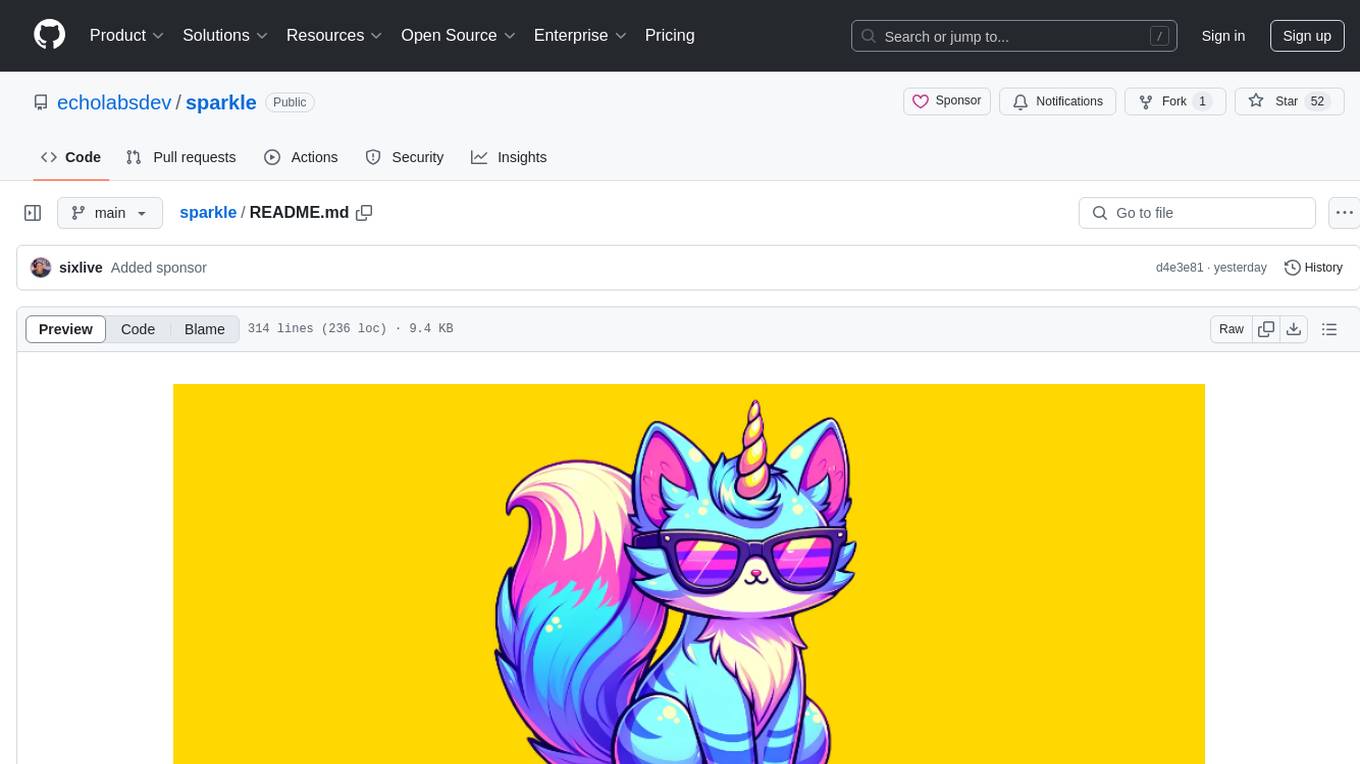
sparkle
Sparkle is a tool that streamlines the process of building AI-driven features in applications using Large Language Models (LLMs). It guides users through creating and managing agents, defining tools, and interacting with LLM providers like OpenAI. Sparkle allows customization of LLM provider settings, model configurations, and provides a seamless integration with Sparkle Server for exposing agents via an OpenAI-compatible chat API endpoint.
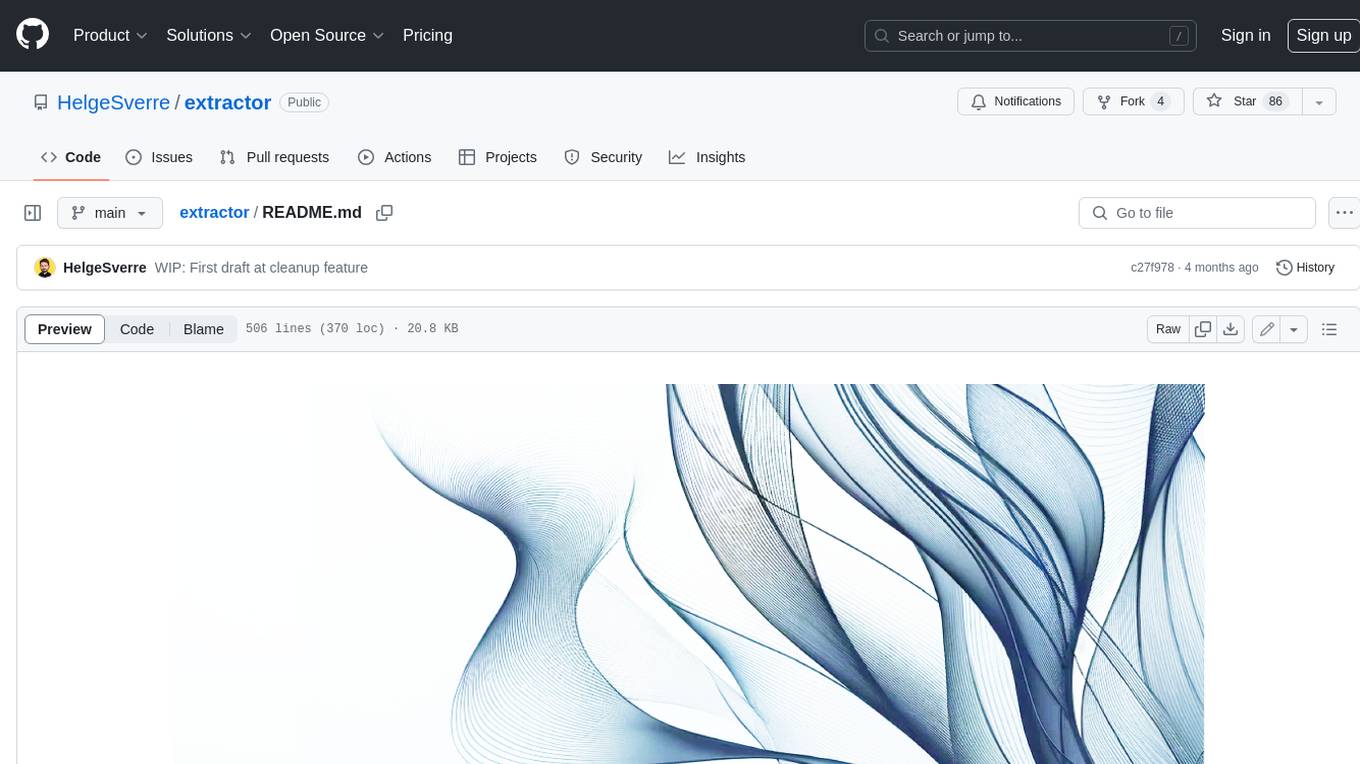
extractor
Extractor is an AI-powered data extraction library for Laravel that leverages OpenAI's capabilities to effortlessly extract structured data from various sources, including images, PDFs, and emails. It features a convenient wrapper around OpenAI Chat and Completion endpoints, supports multiple input formats, includes a flexible Field Extractor for arbitrary data extraction, and integrates with Textract for OCR functionality. Extractor utilizes JSON Mode from the latest GPT-3.5 and GPT-4 models, providing accurate and efficient data extraction.
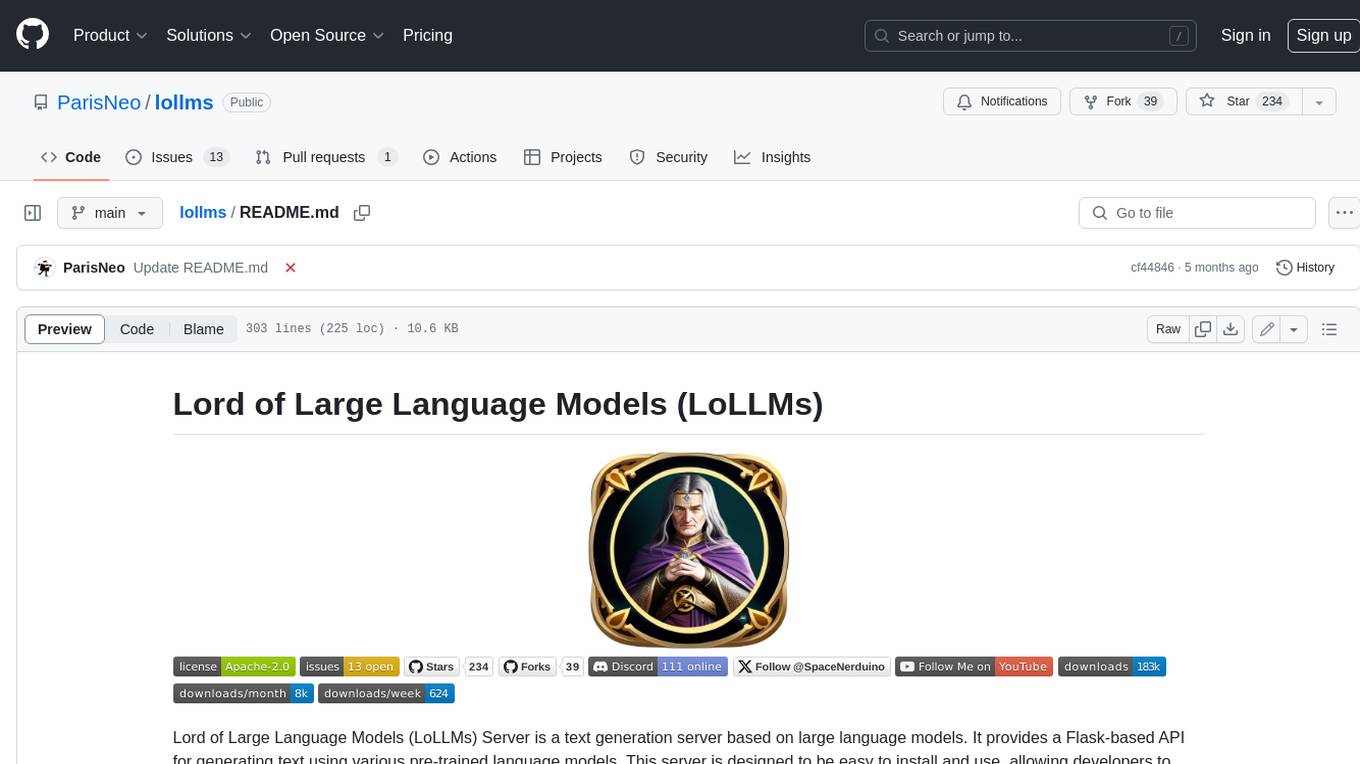
lollms
LoLLMs Server is a text generation server based on large language models. It provides a Flask-based API for generating text using various pre-trained language models. This server is designed to be easy to install and use, allowing developers to integrate powerful text generation capabilities into their applications.
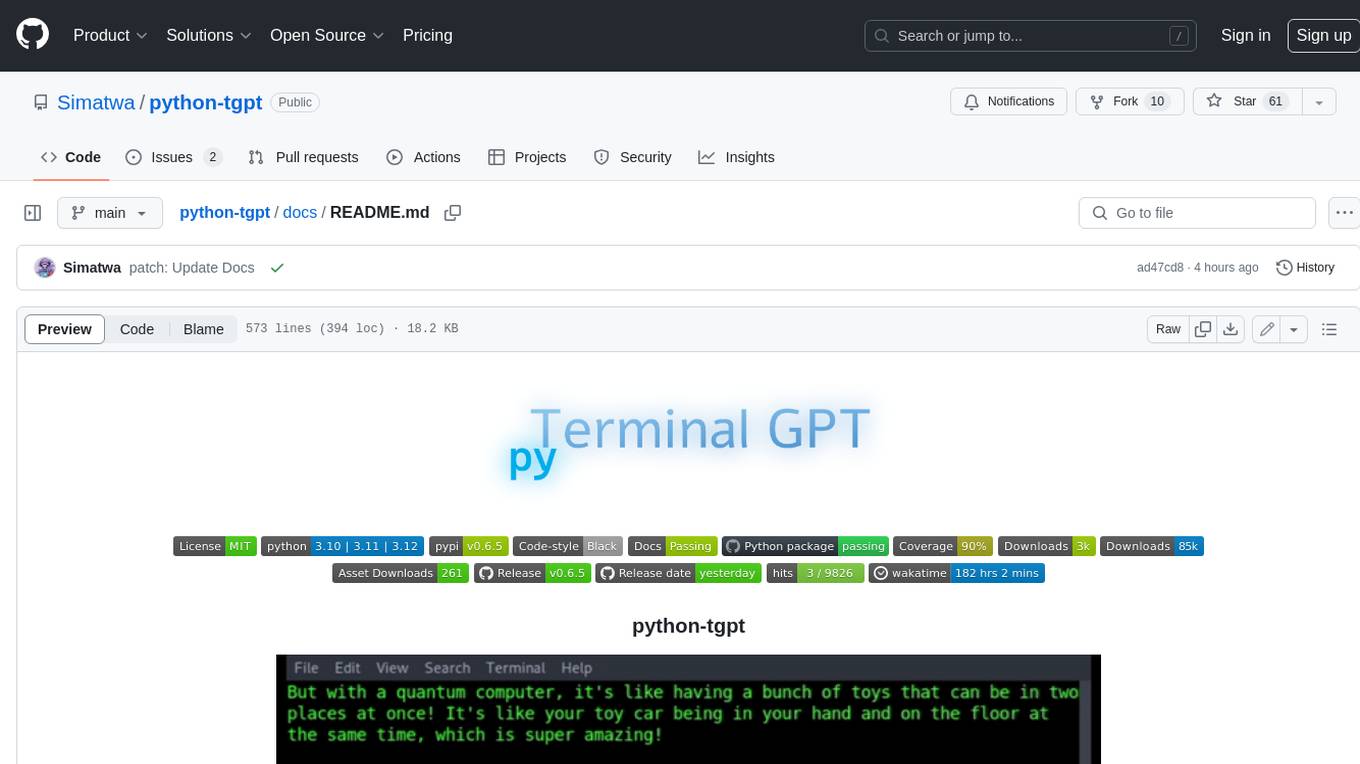
python-tgpt
Python-tgpt is a Python package that enables seamless interaction with over 45 free LLM providers without requiring an API key. It also provides image generation capabilities. The name _python-tgpt_ draws inspiration from its parent project tgpt, which operates on Golang. Through this Python adaptation, users can effortlessly engage with a number of free LLMs available, fostering a smoother AI interaction experience.
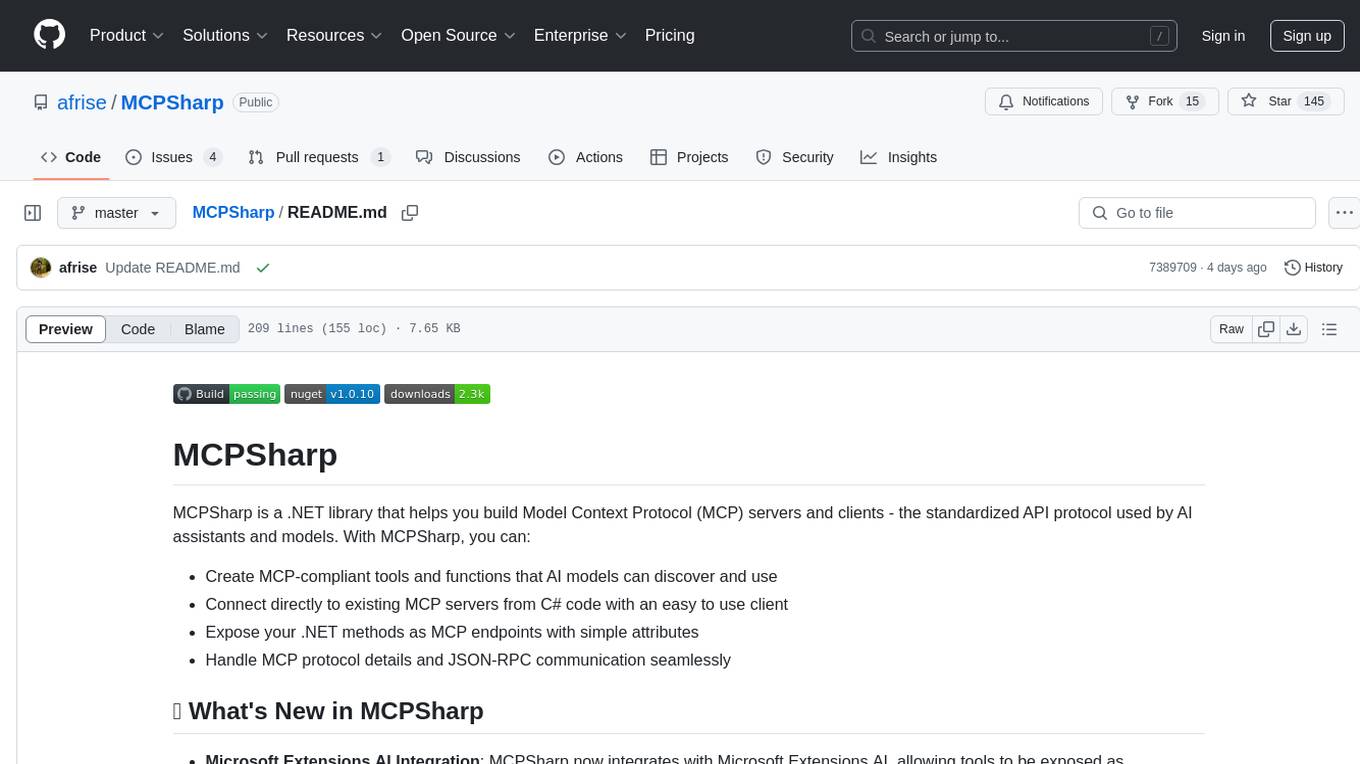
MCPSharp
MCPSharp is a .NET library that helps build Model Context Protocol (MCP) servers and clients for AI assistants and models. It allows creating MCP-compliant tools, connecting to existing MCP servers, exposing .NET methods as MCP endpoints, and handling MCP protocol details seamlessly. With features like attribute-based API, JSON-RPC support, parameter validation, and type conversion, MCPSharp simplifies the development of AI capabilities in applications through standardized interfaces.
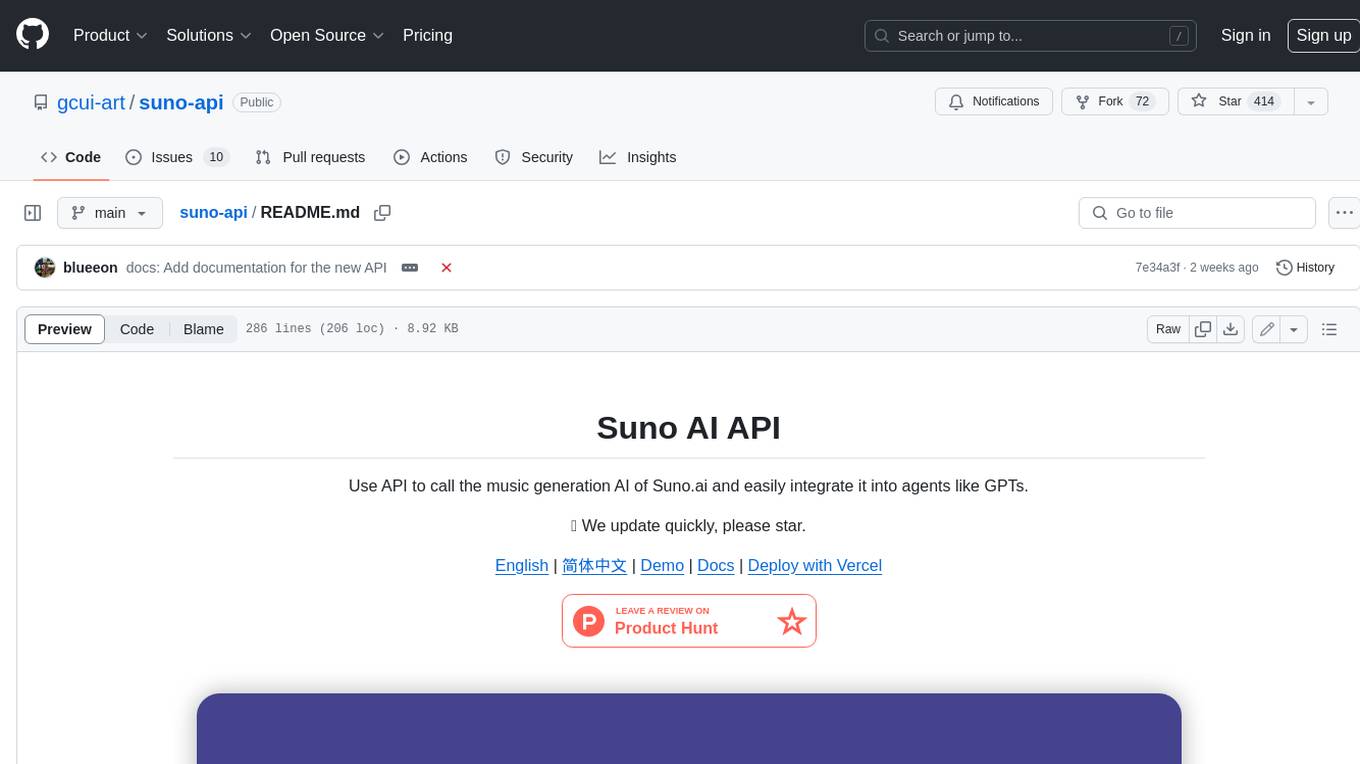
suno-api
Suno AI API is an open-source project that allows developers to integrate the music generation capabilities of Suno.ai into their own applications. The API provides a simple and convenient way to generate music, lyrics, and other audio content using Suno.ai's powerful AI models. With Suno AI API, developers can easily add music generation functionality to their apps, websites, and other projects.
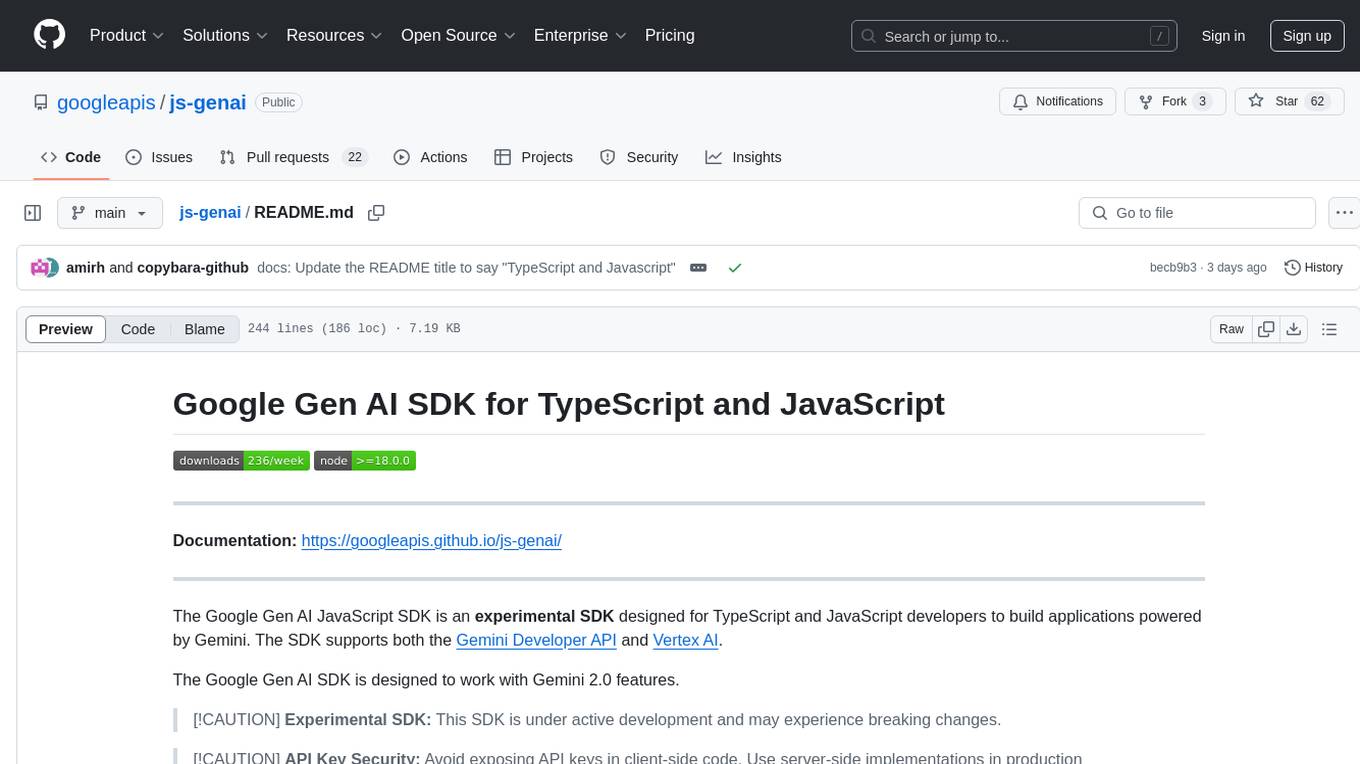
js-genai
The Google Gen AI JavaScript SDK is an experimental SDK for TypeScript and JavaScript developers to build applications powered by Gemini. It supports both the Gemini Developer API and Vertex AI. The SDK is designed to work with Gemini 2.0 features. Users can access API features through the GoogleGenAI classes, which provide submodules for querying models, managing caches, creating chats, uploading files, and starting live sessions. The SDK also allows for function calling to interact with external systems. Users can find more samples in the GitHub samples directory.
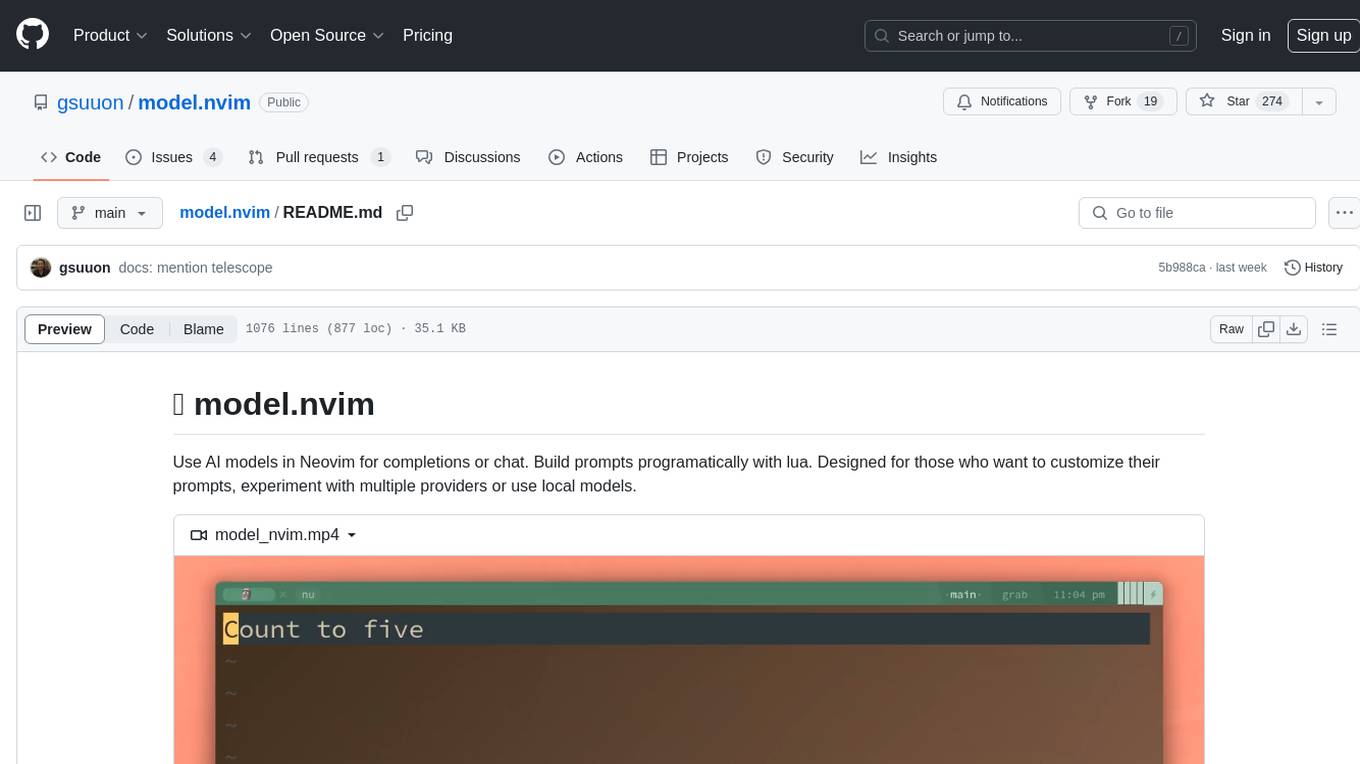
model.nvim
model.nvim is a tool designed for Neovim users who want to utilize AI models for completions or chat within their text editor. It allows users to build prompts programmatically with Lua, customize prompts, experiment with multiple providers, and use both hosted and local models. The tool supports features like provider agnosticism, programmatic prompts in Lua, async and multistep prompts, streaming completions, and chat functionality in 'mchat' filetype buffer. Users can customize prompts, manage responses, and context, and utilize various providers like OpenAI ChatGPT, Google PaLM, llama.cpp, ollama, and more. The tool also supports treesitter highlights and folds for chat buffers.
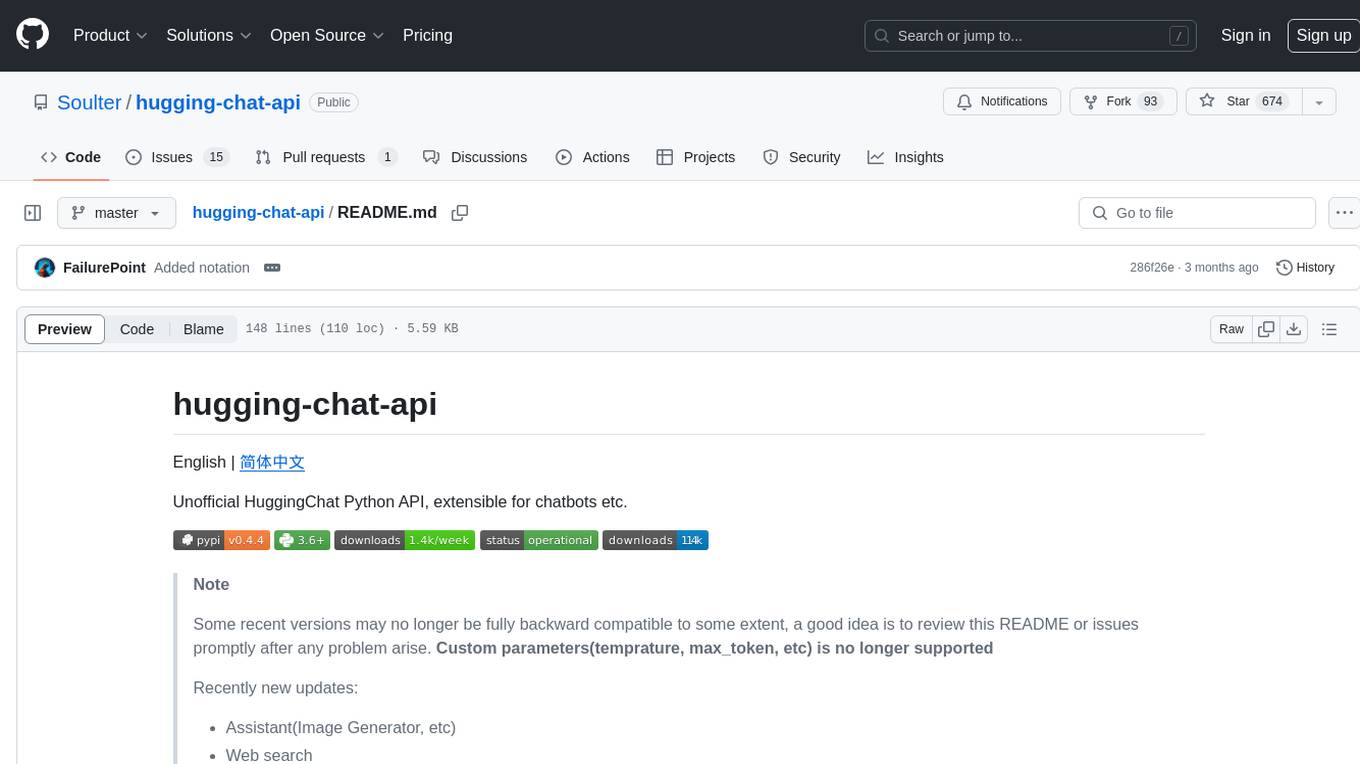
hugging-chat-api
Unofficial HuggingChat Python API for creating chatbots, supporting features like image generation, web search, memorizing context, and changing LLMs. Users can log in, chat with the ChatBot, perform web searches, create new conversations, manage conversations, switch models, get conversation info, use assistants, and delete conversations. The API also includes a CLI mode with various commands for interacting with the tool. Users are advised not to use the application for high-stakes decisions or advice and to avoid high-frequency requests to preserve server resources.
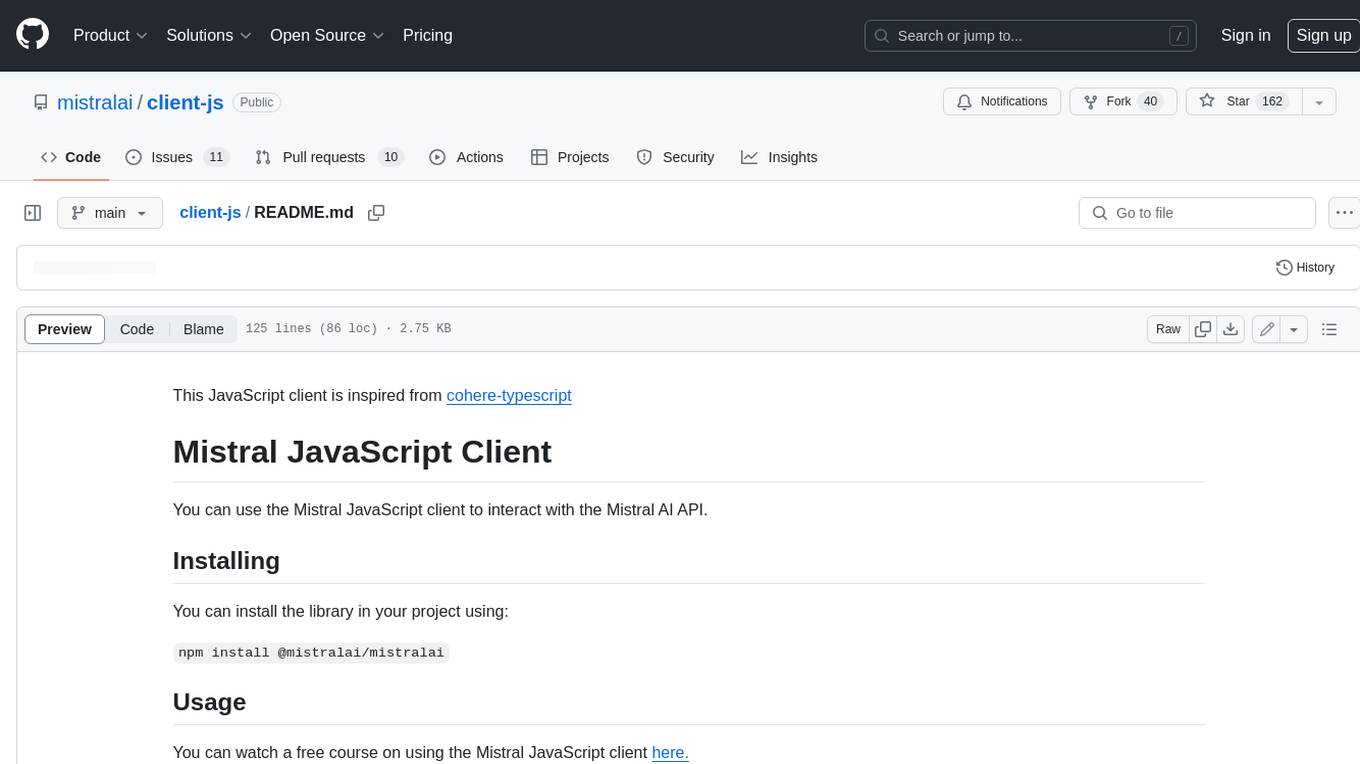
client-js
The Mistral JavaScript client is a library that allows you to interact with the Mistral AI API. With this client, you can perform various tasks such as listing models, chatting with streaming, chatting without streaming, and generating embeddings. To use the client, you can install it in your project using npm and then set up the client with your API key. Once the client is set up, you can use it to perform the desired tasks. For example, you can use the client to chat with a model by providing a list of messages. The client will then return the response from the model. You can also use the client to generate embeddings for a given input. The embeddings can then be used for various downstream tasks such as clustering or classification.
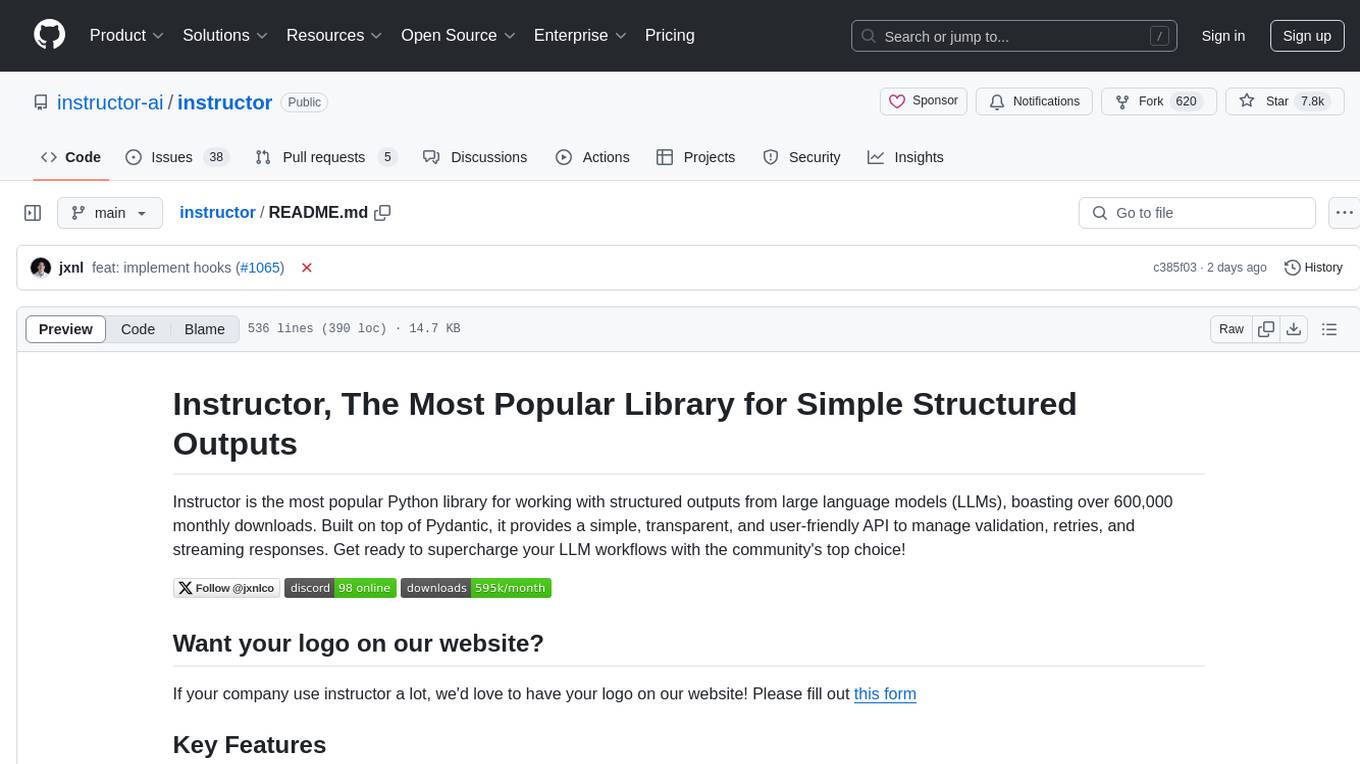
instructor
Instructor is a popular Python library for managing structured outputs from large language models (LLMs). It offers a user-friendly API for validation, retries, and streaming responses. With support for various LLM providers and multiple languages, Instructor simplifies working with LLM outputs. The library includes features like response models, retry management, validation, streaming support, and flexible backends. It also provides hooks for logging and monitoring LLM interactions, and supports integration with Anthropic, Cohere, Gemini, Litellm, and Google AI models. Instructor facilitates tasks such as extracting user data from natural language, creating fine-tuned models, managing uploaded files, and monitoring usage of OpenAI models.
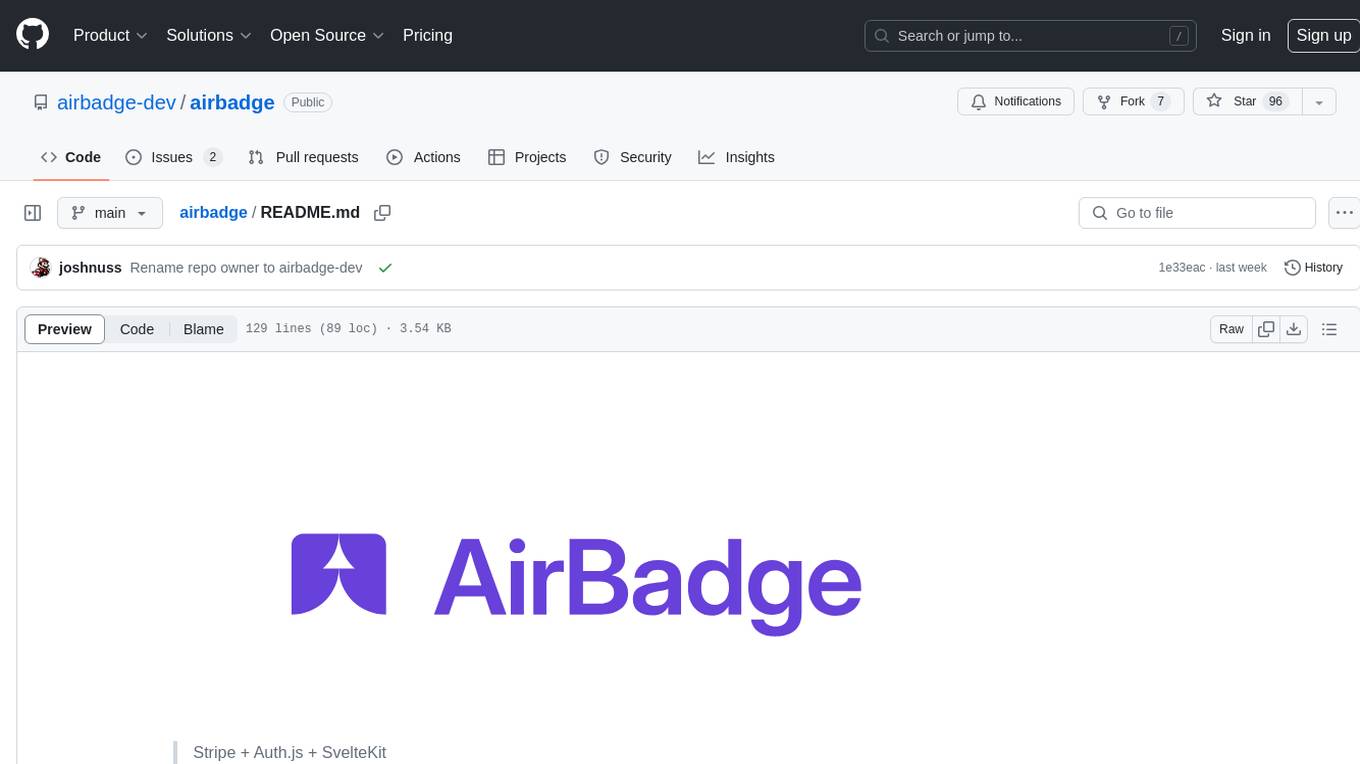
airbadge
Airbadge is a Stripe addon for Auth.js that simplifies the process of creating a SaaS site by integrating payment, authentication, gating, self-service account management, webhook handling, trials & free plans, session data, and more. It allows users to launch a SaaS app without writing any authentication or payment code. The project is open source and free to use with optional paid features under the BSL License.
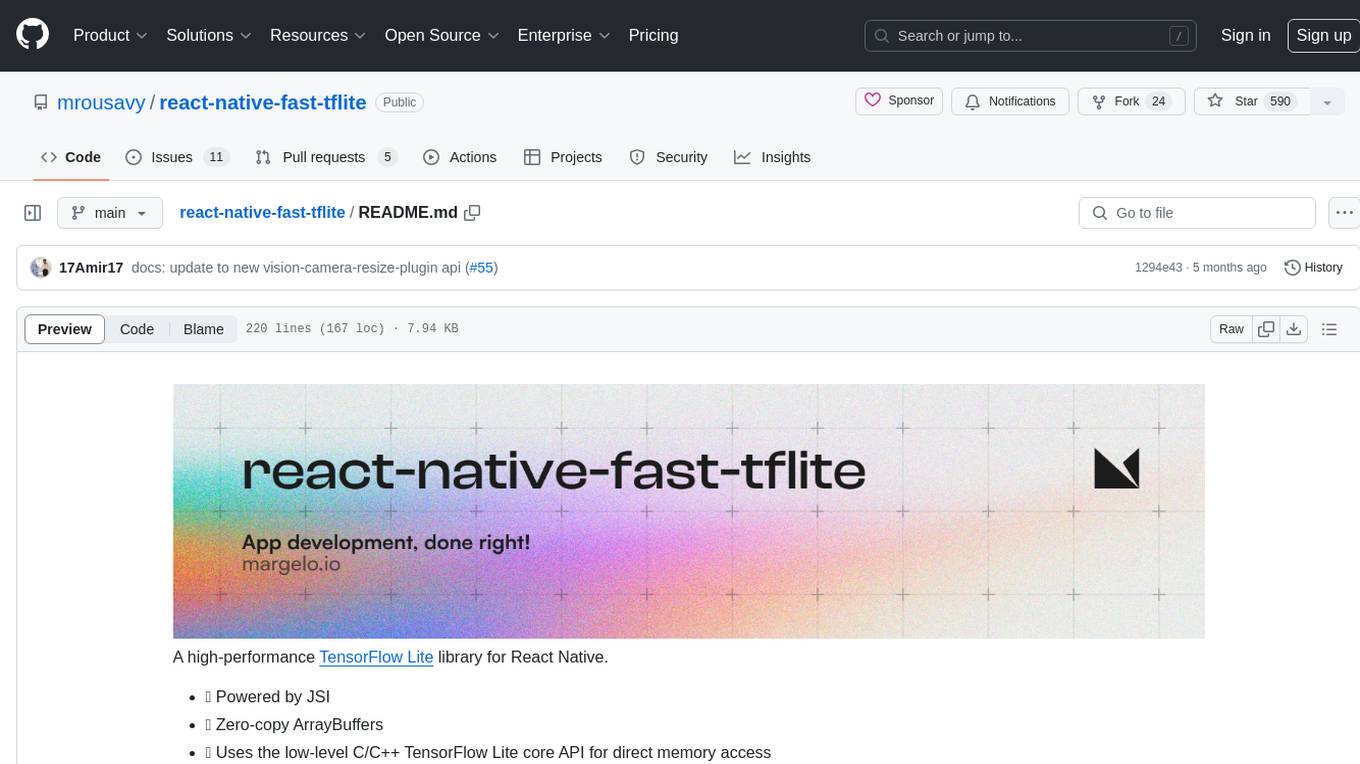
react-native-fast-tflite
A high-performance TensorFlow Lite library for React Native that utilizes JSI for power, zero-copy ArrayBuffers for efficiency, and low-level C/C++ TensorFlow Lite core API for direct memory access. It supports swapping out TensorFlow Models at runtime and GPU-accelerated delegates like CoreML/Metal/OpenGL. Easy VisionCamera integration allows for seamless usage. Users can load TensorFlow Lite models, interpret input and output data, and utilize GPU Delegates for faster computation. The library is suitable for real-time object detection, image classification, and other machine learning tasks in React Native applications.
For similar tasks
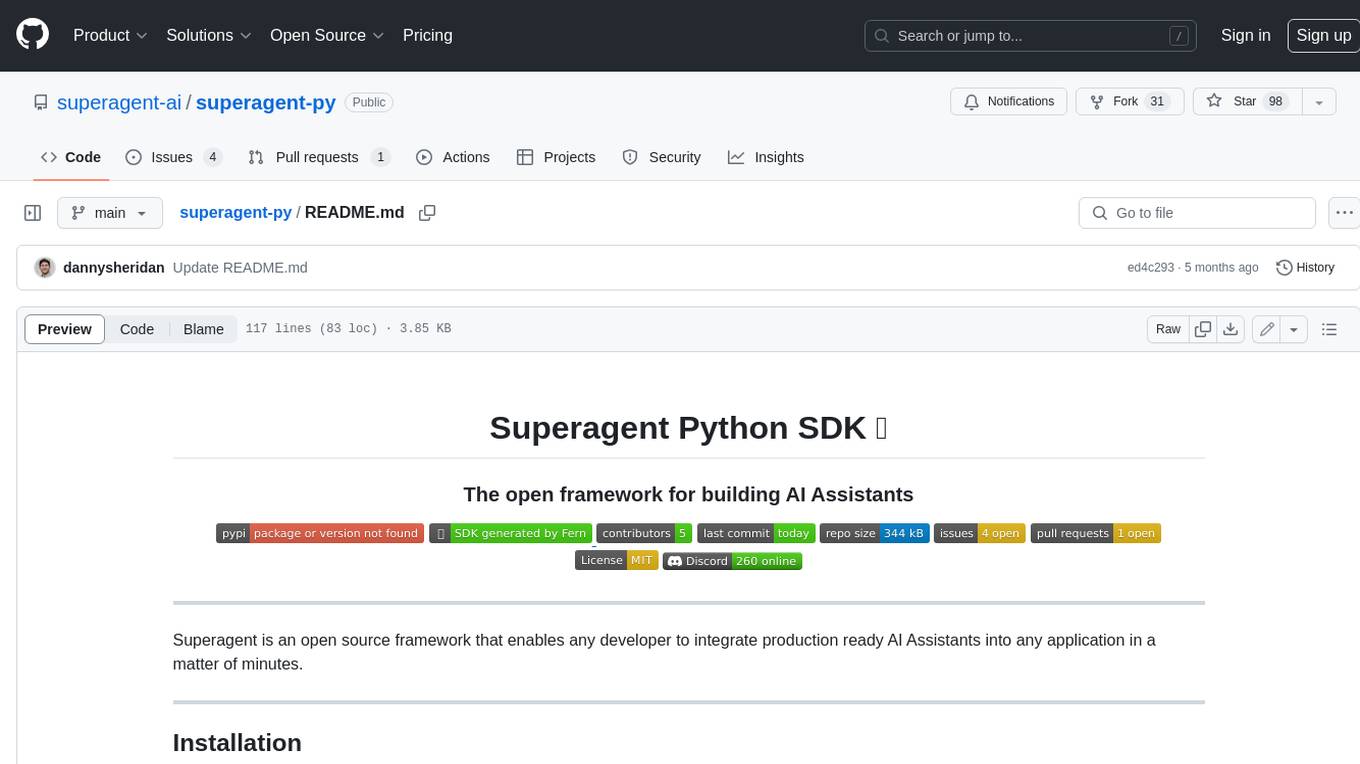
superagent-py
Superagent is an open-source framework that enables developers to integrate production-ready AI assistants into any application quickly and easily. It provides a Python SDK for interacting with the Superagent API, allowing developers to create, manage, and invoke AI agents. The SDK simplifies the process of building AI-powered applications, making it accessible to developers of all skill levels.
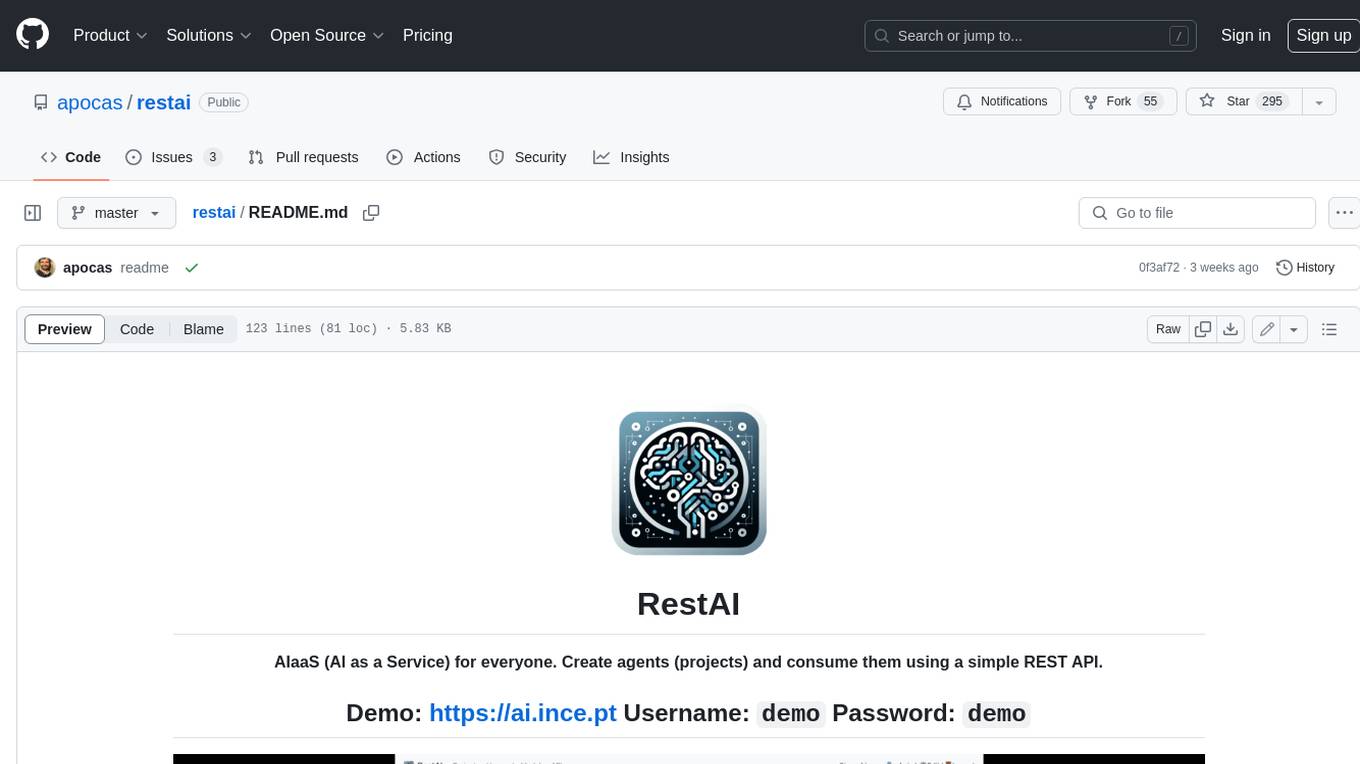
restai
RestAI is an AIaaS (AI as a Service) platform that allows users to create and consume AI agents (projects) using a simple REST API. It supports various types of agents, including RAG (Retrieval-Augmented Generation), RAGSQL (RAG for SQL), inference, vision, and router. RestAI features automatic VRAM management, support for any public LLM supported by LlamaIndex or any local LLM supported by Ollama, a user-friendly API with Swagger documentation, and a frontend for easy access. It also provides evaluation capabilities for RAG agents using deepeval.
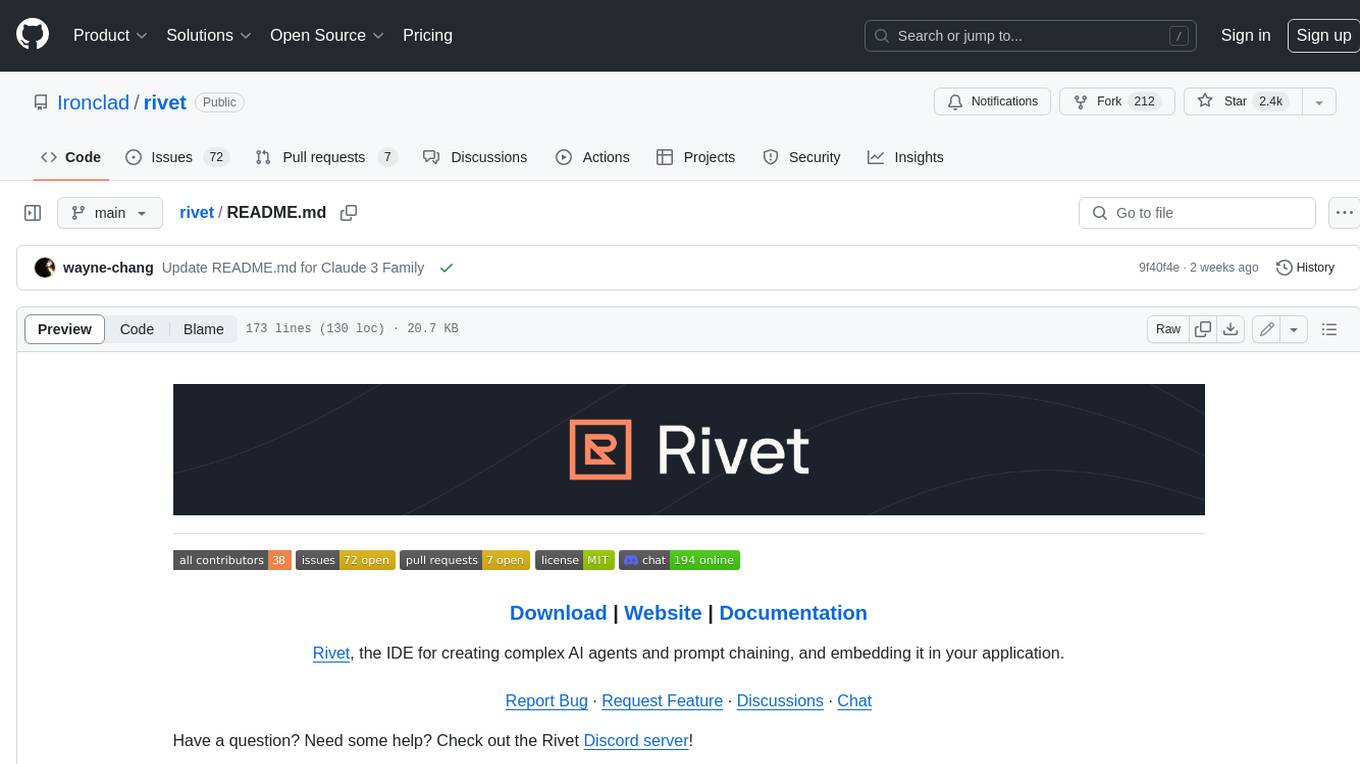
rivet
Rivet is a desktop application for creating complex AI agents and prompt chaining, and embedding it in your application. Rivet currently has LLM support for OpenAI GPT-3.5 and GPT-4, Anthropic Claude Instant and Claude 2, [Anthropic Claude 3 Haiku, Sonnet, and Opus](https://www.anthropic.com/news/claude-3-family), and AssemblyAI LeMUR framework for voice data. Rivet has embedding/vector database support for OpenAI Embeddings and Pinecone. Rivet also supports these additional integrations: Audio Transcription from AssemblyAI. Rivet core is a TypeScript library for running graphs created in Rivet. It is used by the Rivet application, but can also be used in your own applications, so that Rivet can call into your own application's code, and your application can call into Rivet graphs.
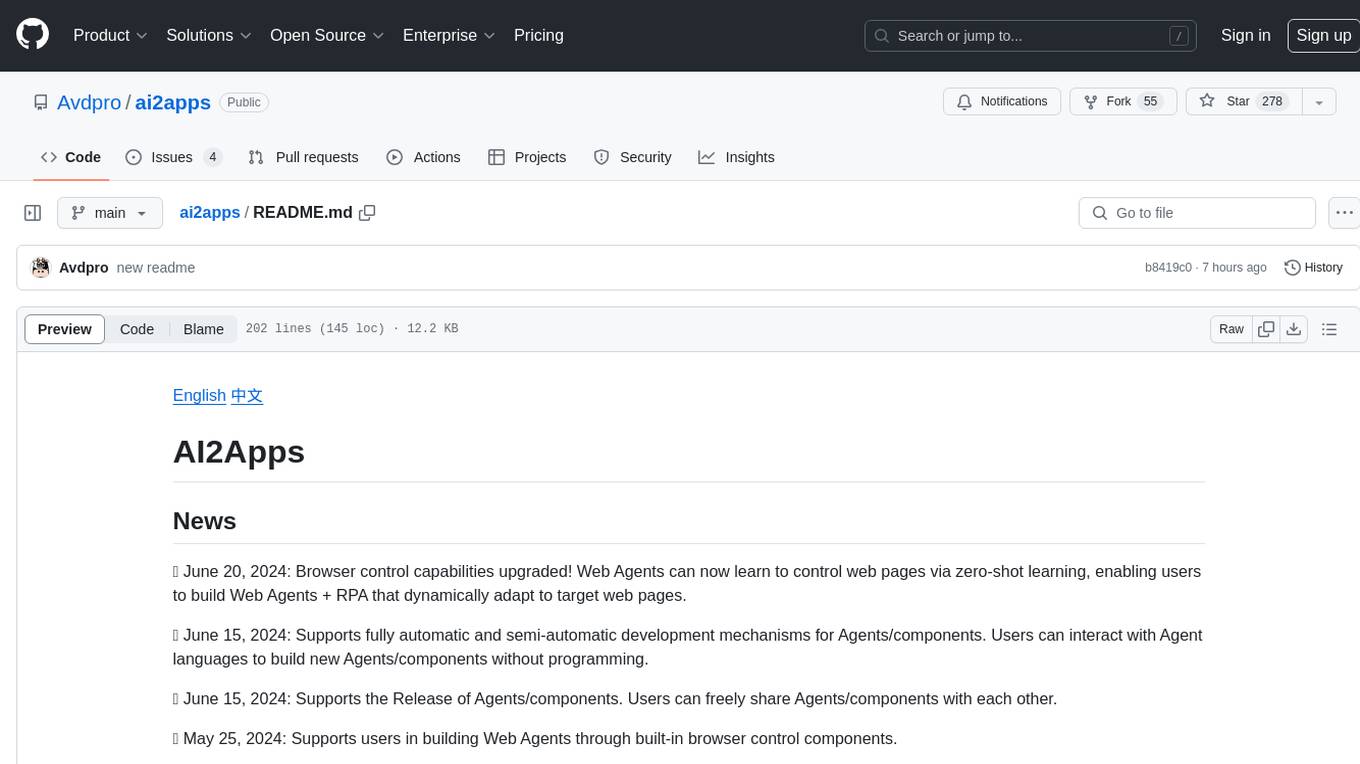
ai2apps
AI2Apps is a visual IDE for building LLM-based AI agent applications, enabling developers to efficiently create AI agents through drag-and-drop, with features like design-to-development for rapid prototyping, direct packaging of agents into apps, powerful debugging capabilities, enhanced user interaction, efficient team collaboration, flexible deployment, multilingual support, simplified product maintenance, and extensibility through plugins.
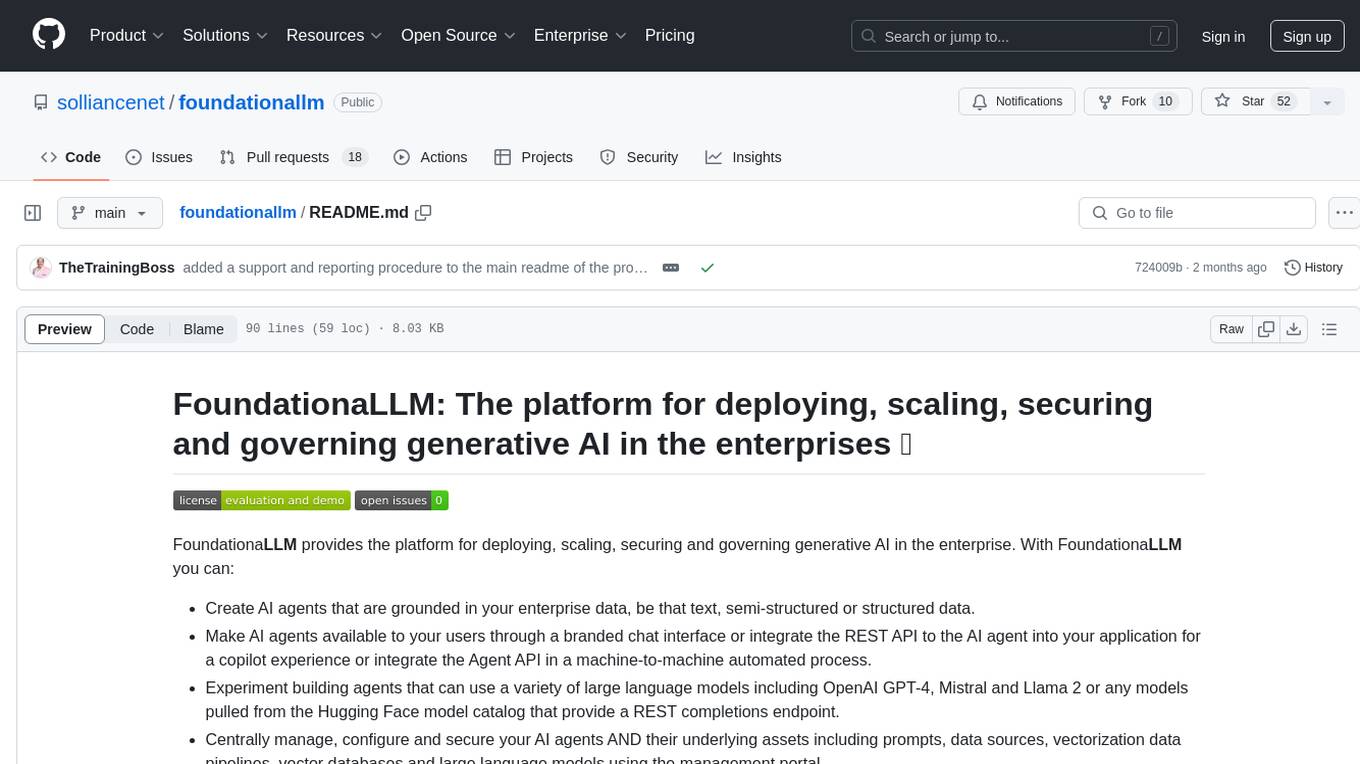
foundationallm
FoundationaLLM is a platform designed for deploying, scaling, securing, and governing generative AI in enterprises. It allows users to create AI agents grounded in enterprise data, integrate REST APIs, experiment with large language models, centrally manage AI agents and assets, deploy scalable vectorization data pipelines, enable non-developer users to create their own AI agents, control access with role-based access controls, and harness capabilities from Azure AI and Azure OpenAI. The platform simplifies integration with enterprise data sources, provides fine-grain security controls, load balances across multiple endpoints, and is extensible to new data sources and orchestrators. FoundationaLLM addresses the need for customized copilots or AI agents that are secure, licensed, flexible, and suitable for enterprise-scale production.
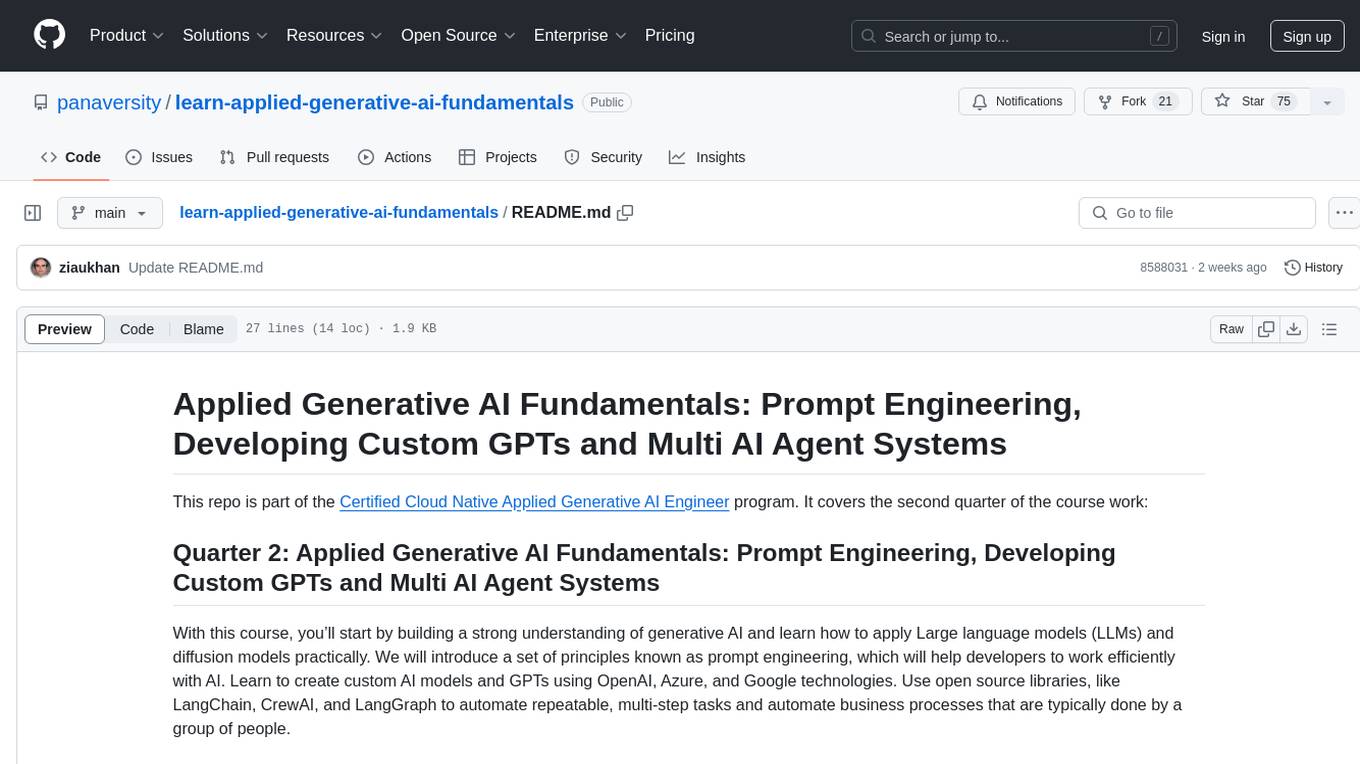
learn-applied-generative-ai-fundamentals
This repository is part of the Certified Cloud Native Applied Generative AI Engineer program, focusing on Applied Generative AI Fundamentals. It covers prompt engineering, developing custom GPTs, and Multi AI Agent Systems. The course helps in building a strong understanding of generative AI, applying Large Language Models (LLMs) and diffusion models practically. It introduces principles of prompt engineering to work efficiently with AI, creating custom AI models and GPTs using OpenAI, Azure, and Google technologies. It also utilizes open source libraries like LangChain, CrewAI, and LangGraph to automate tasks and business processes.
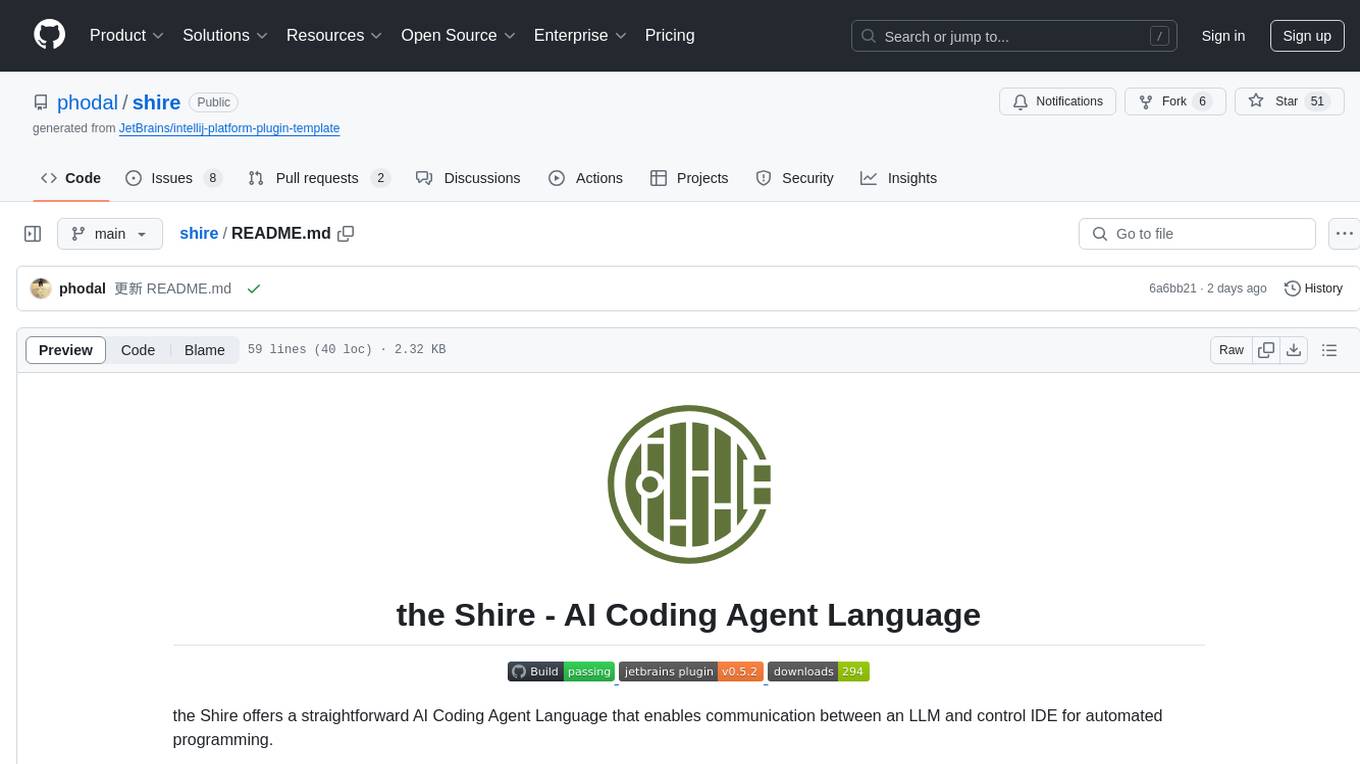
shire
The Shire is an AI Coding Agent Language that facilitates communication between an LLM and control IDE for automated programming. It offers a straightforward approach to creating AI agents tailored to individual IDEs, enabling users to build customized AI-driven development environments. The concept of Shire originated from AutoDev, a subproject of UnitMesh, with DevIns as its precursor. The tool provides documentation and resources for implementing AI in software engineering projects.
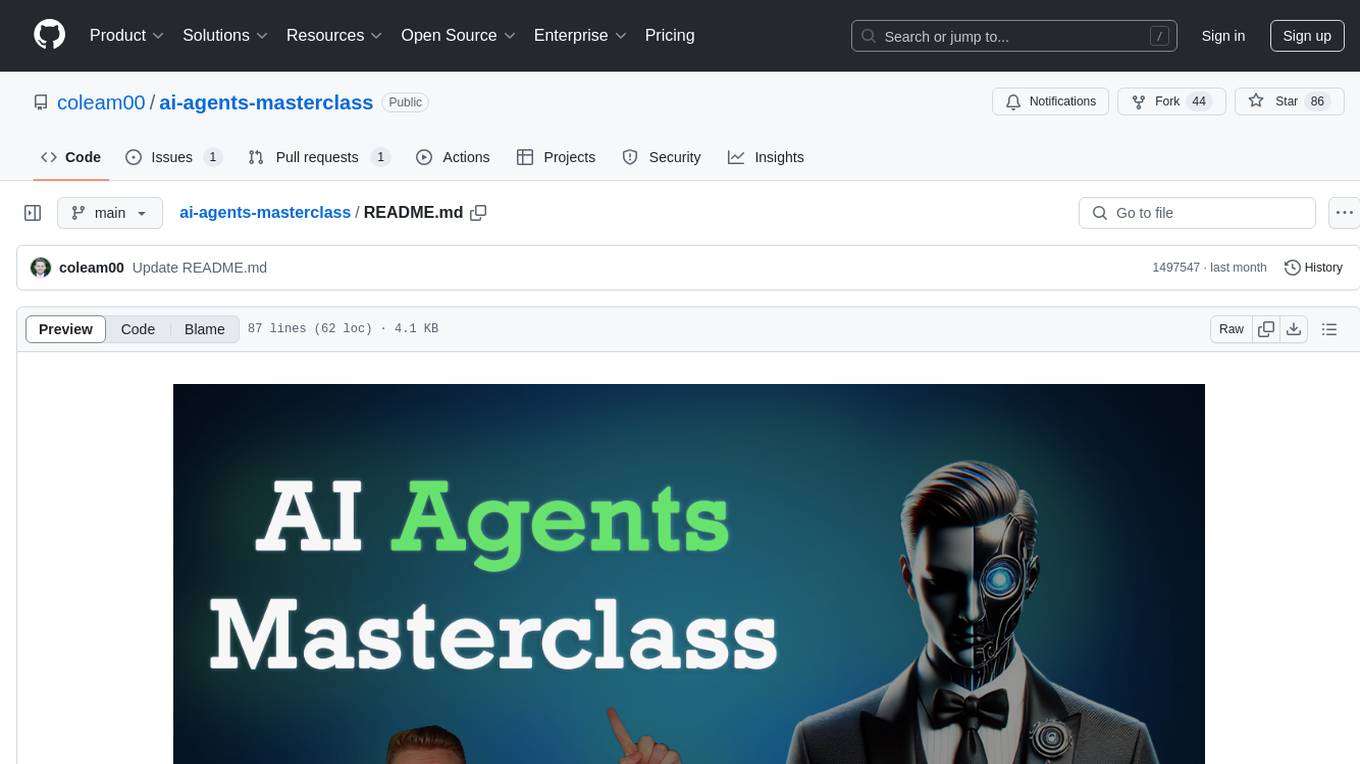
ai-agents-masterclass
AI Agents Masterclass is a repository dedicated to teaching developers how to use AI agents to transform businesses and create powerful software. It provides weekly videos with accompanying code folders, guiding users on setting up Python environments, using environment variables, and installing necessary packages to run the code. The focus is on Large Language Models that can interact with the outside world to perform tasks like drafting emails, booking appointments, and managing tasks, enabling users to create innovative applications with minimal coding effort.
For similar jobs
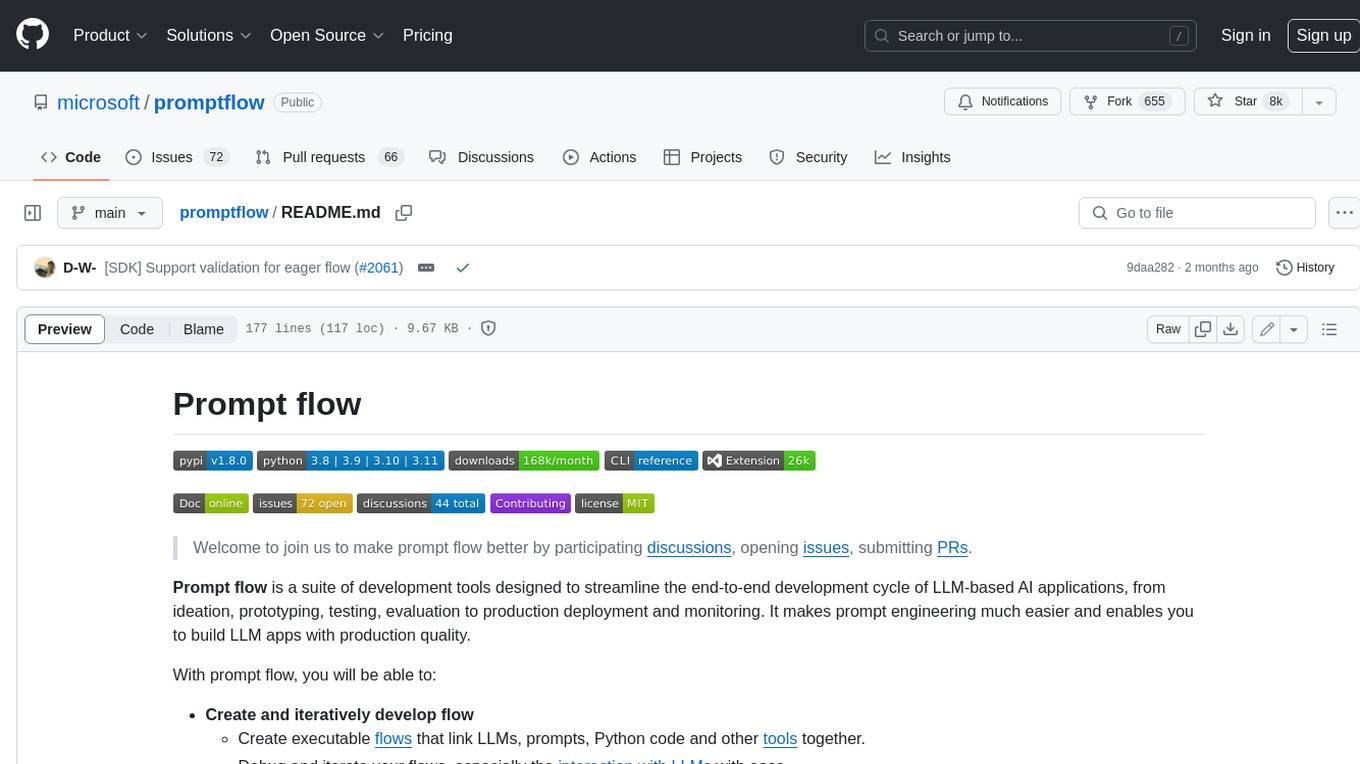
promptflow
**Prompt flow** is a suite of development tools designed to streamline the end-to-end development cycle of LLM-based AI applications, from ideation, prototyping, testing, evaluation to production deployment and monitoring. It makes prompt engineering much easier and enables you to build LLM apps with production quality.
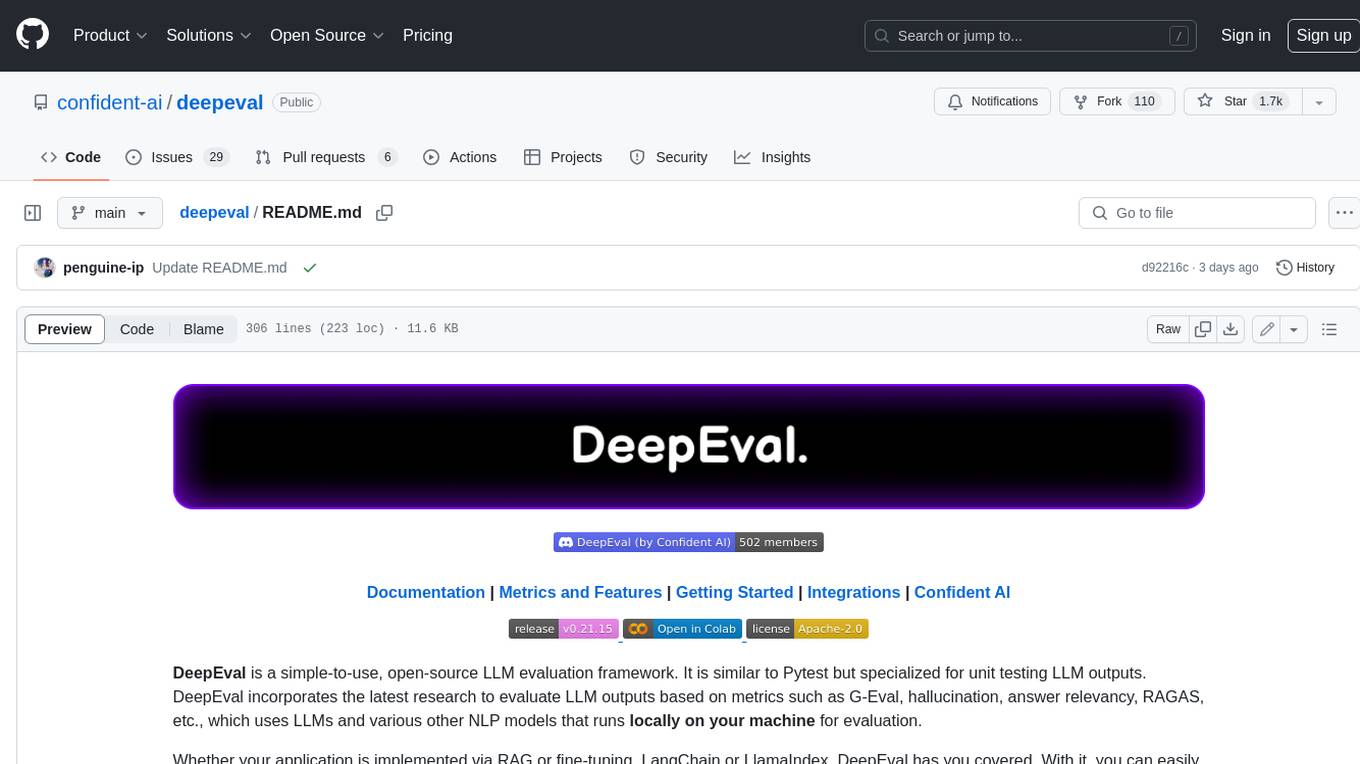
deepeval
DeepEval is a simple-to-use, open-source LLM evaluation framework specialized for unit testing LLM outputs. It incorporates various metrics such as G-Eval, hallucination, answer relevancy, RAGAS, etc., and runs locally on your machine for evaluation. It provides a wide range of ready-to-use evaluation metrics, allows for creating custom metrics, integrates with any CI/CD environment, and enables benchmarking LLMs on popular benchmarks. DeepEval is designed for evaluating RAG and fine-tuning applications, helping users optimize hyperparameters, prevent prompt drifting, and transition from OpenAI to hosting their own Llama2 with confidence.
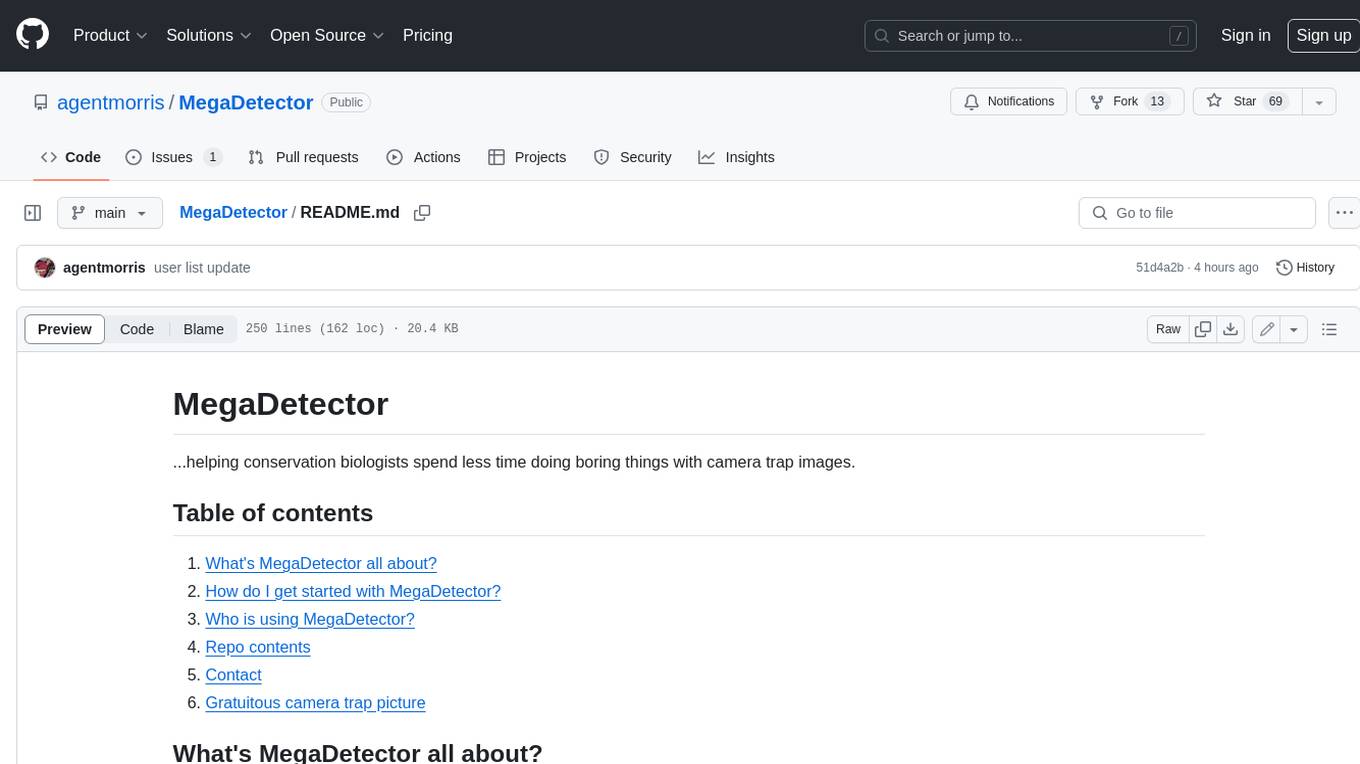
MegaDetector
MegaDetector is an AI model that identifies animals, people, and vehicles in camera trap images (which also makes it useful for eliminating blank images). This model is trained on several million images from a variety of ecosystems. MegaDetector is just one of many tools that aims to make conservation biologists more efficient with AI. If you want to learn about other ways to use AI to accelerate camera trap workflows, check out our of the field, affectionately titled "Everything I know about machine learning and camera traps".
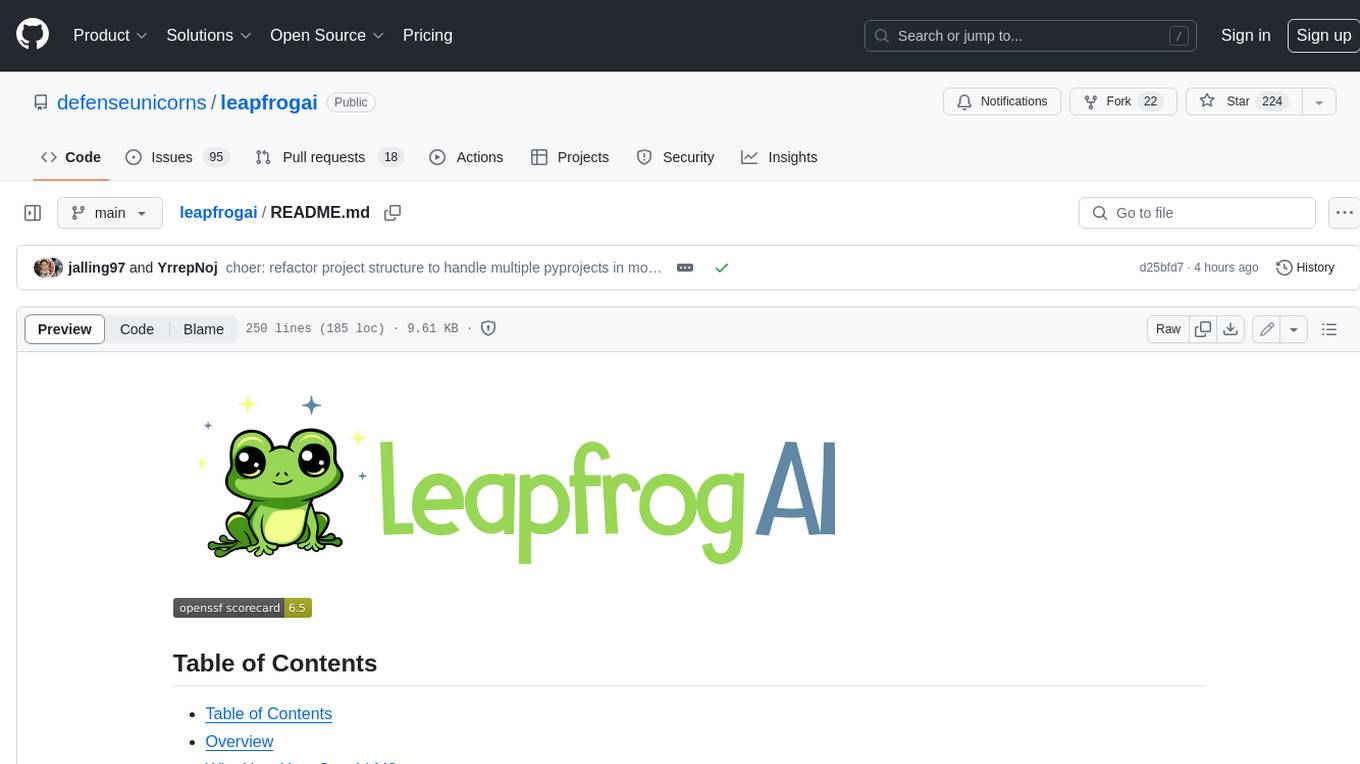
leapfrogai
LeapfrogAI is a self-hosted AI platform designed to be deployed in air-gapped resource-constrained environments. It brings sophisticated AI solutions to these environments by hosting all the necessary components of an AI stack, including vector databases, model backends, API, and UI. LeapfrogAI's API closely matches that of OpenAI, allowing tools built for OpenAI/ChatGPT to function seamlessly with a LeapfrogAI backend. It provides several backends for various use cases, including llama-cpp-python, whisper, text-embeddings, and vllm. LeapfrogAI leverages Chainguard's apko to harden base python images, ensuring the latest supported Python versions are used by the other components of the stack. The LeapfrogAI SDK provides a standard set of protobuffs and python utilities for implementing backends and gRPC. LeapfrogAI offers UI options for common use-cases like chat, summarization, and transcription. It can be deployed and run locally via UDS and Kubernetes, built out using Zarf packages. LeapfrogAI is supported by a community of users and contributors, including Defense Unicorns, Beast Code, Chainguard, Exovera, Hypergiant, Pulze, SOSi, United States Navy, United States Air Force, and United States Space Force.
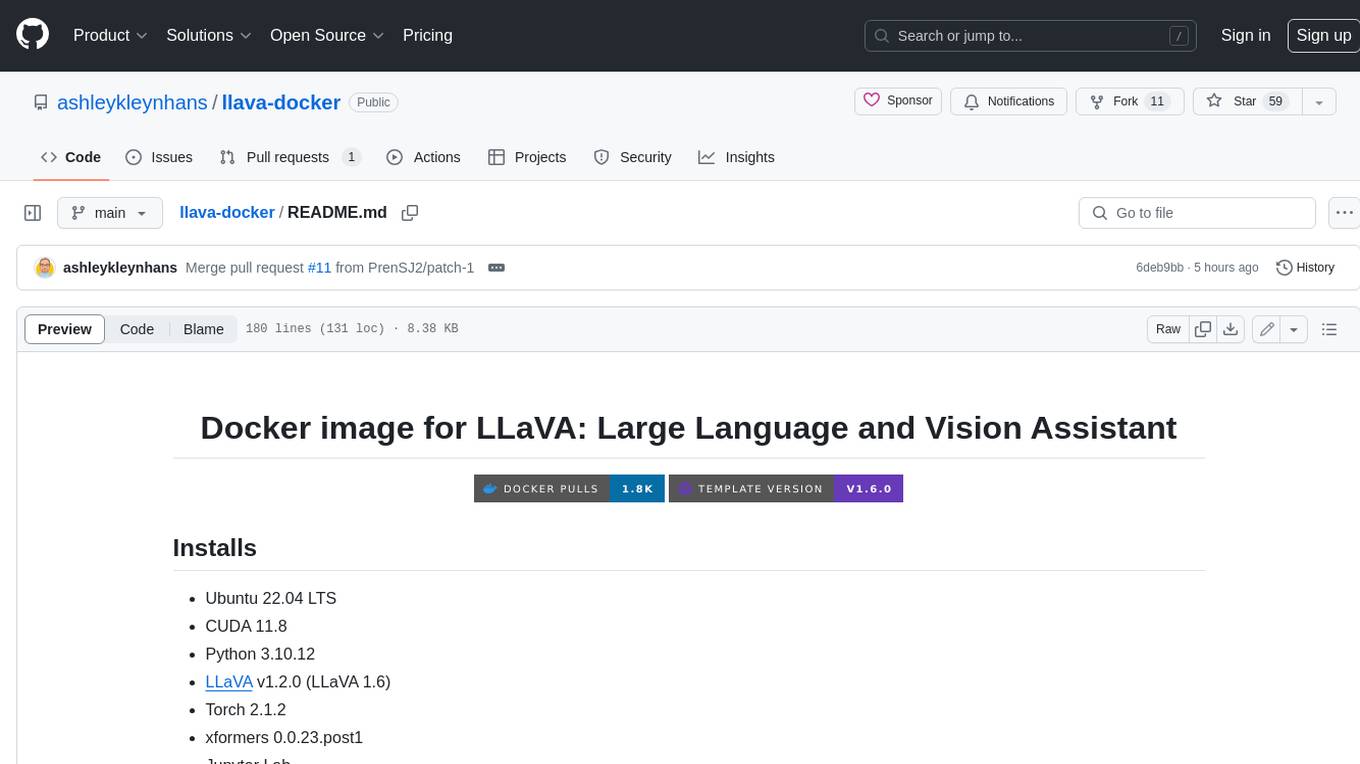
llava-docker
This Docker image for LLaVA (Large Language and Vision Assistant) provides a convenient way to run LLaVA locally or on RunPod. LLaVA is a powerful AI tool that combines natural language processing and computer vision capabilities. With this Docker image, you can easily access LLaVA's functionalities for various tasks, including image captioning, visual question answering, text summarization, and more. The image comes pre-installed with LLaVA v1.2.0, Torch 2.1.2, xformers 0.0.23.post1, and other necessary dependencies. You can customize the model used by setting the MODEL environment variable. The image also includes a Jupyter Lab environment for interactive development and exploration. Overall, this Docker image offers a comprehensive and user-friendly platform for leveraging LLaVA's capabilities.
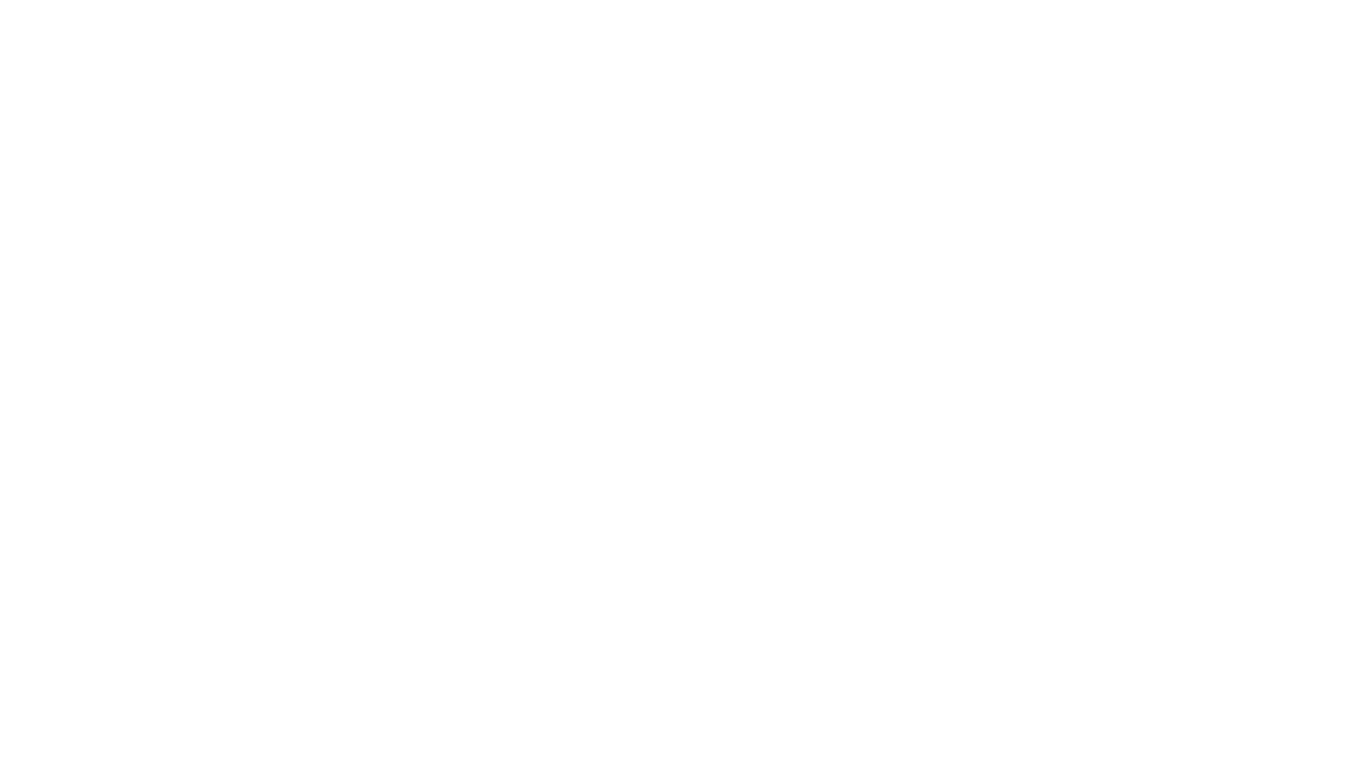
carrot
The 'carrot' repository on GitHub provides a list of free and user-friendly ChatGPT mirror sites for easy access. The repository includes sponsored sites offering various GPT models and services. Users can find and share sites, report errors, and access stable and recommended sites for ChatGPT usage. The repository also includes a detailed list of ChatGPT sites, their features, and accessibility options, making it a valuable resource for ChatGPT users seeking free and unlimited GPT services.
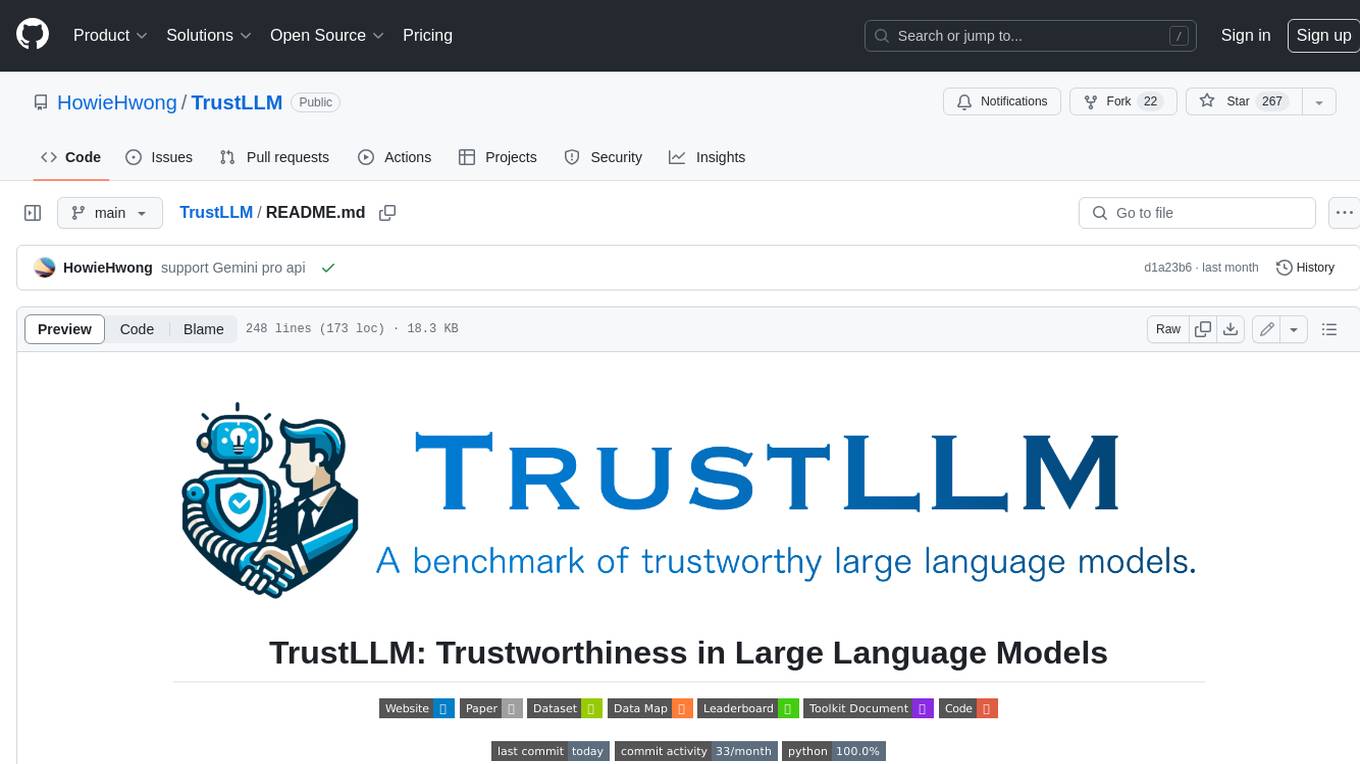
TrustLLM
TrustLLM is a comprehensive study of trustworthiness in LLMs, including principles for different dimensions of trustworthiness, established benchmark, evaluation, and analysis of trustworthiness for mainstream LLMs, and discussion of open challenges and future directions. Specifically, we first propose a set of principles for trustworthy LLMs that span eight different dimensions. Based on these principles, we further establish a benchmark across six dimensions including truthfulness, safety, fairness, robustness, privacy, and machine ethics. We then present a study evaluating 16 mainstream LLMs in TrustLLM, consisting of over 30 datasets. The document explains how to use the trustllm python package to help you assess the performance of your LLM in trustworthiness more quickly. For more details about TrustLLM, please refer to project website.
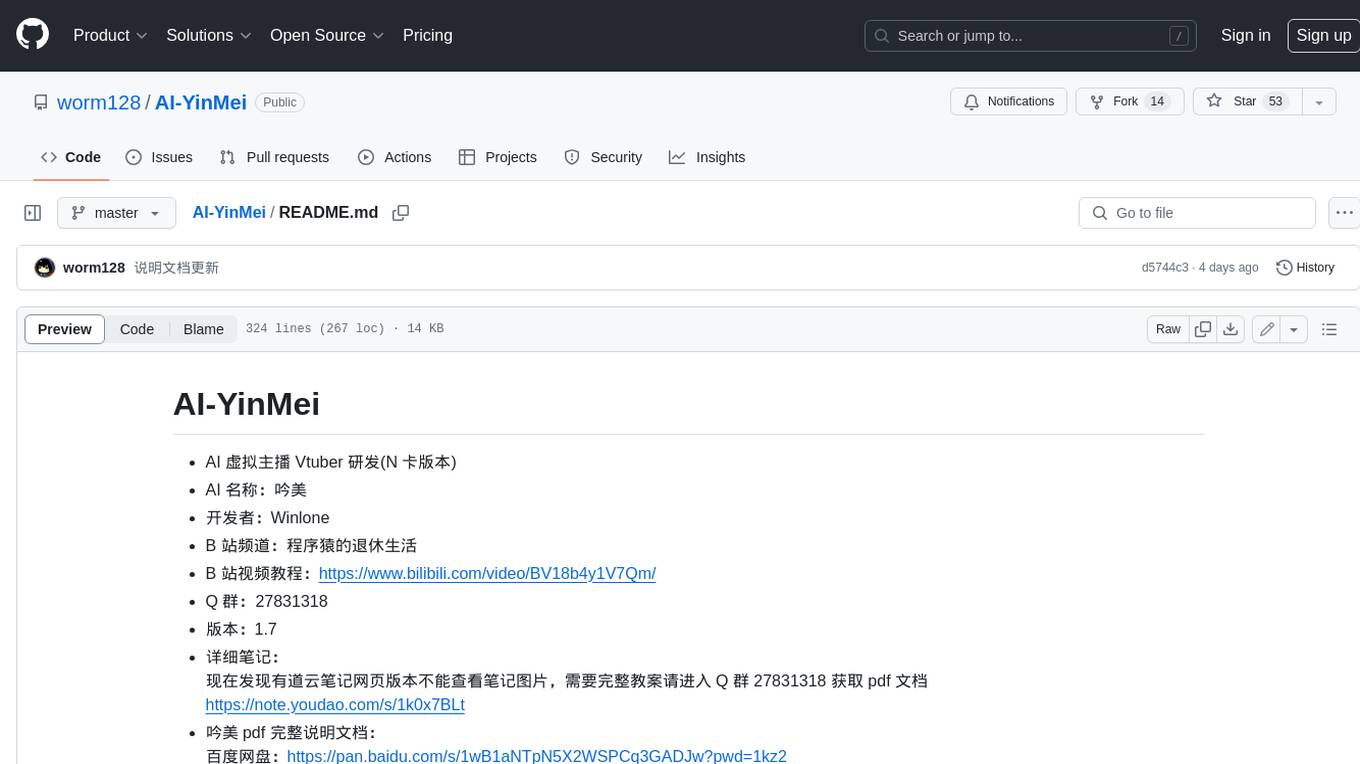
AI-YinMei
AI-YinMei is an AI virtual anchor Vtuber development tool (N card version). It supports fastgpt knowledge base chat dialogue, a complete set of solutions for LLM large language models: [fastgpt] + [one-api] + [Xinference], supports docking bilibili live broadcast barrage reply and entering live broadcast welcome speech, supports Microsoft edge-tts speech synthesis, supports Bert-VITS2 speech synthesis, supports GPT-SoVITS speech synthesis, supports expression control Vtuber Studio, supports painting stable-diffusion-webui output OBS live broadcast room, supports painting picture pornography public-NSFW-y-distinguish, supports search and image search service duckduckgo (requires magic Internet access), supports image search service Baidu image search (no magic Internet access), supports AI reply chat box [html plug-in], supports AI singing Auto-Convert-Music, supports playlist [html plug-in], supports dancing function, supports expression video playback, supports head touching action, supports gift smashing action, supports singing automatic start dancing function, chat and singing automatic cycle swing action, supports multi scene switching, background music switching, day and night automatic switching scene, supports open singing and painting, let AI automatically judge the content.