Best AI tools for< Generate Synthetic Datasets >
20 - AI tool Sites
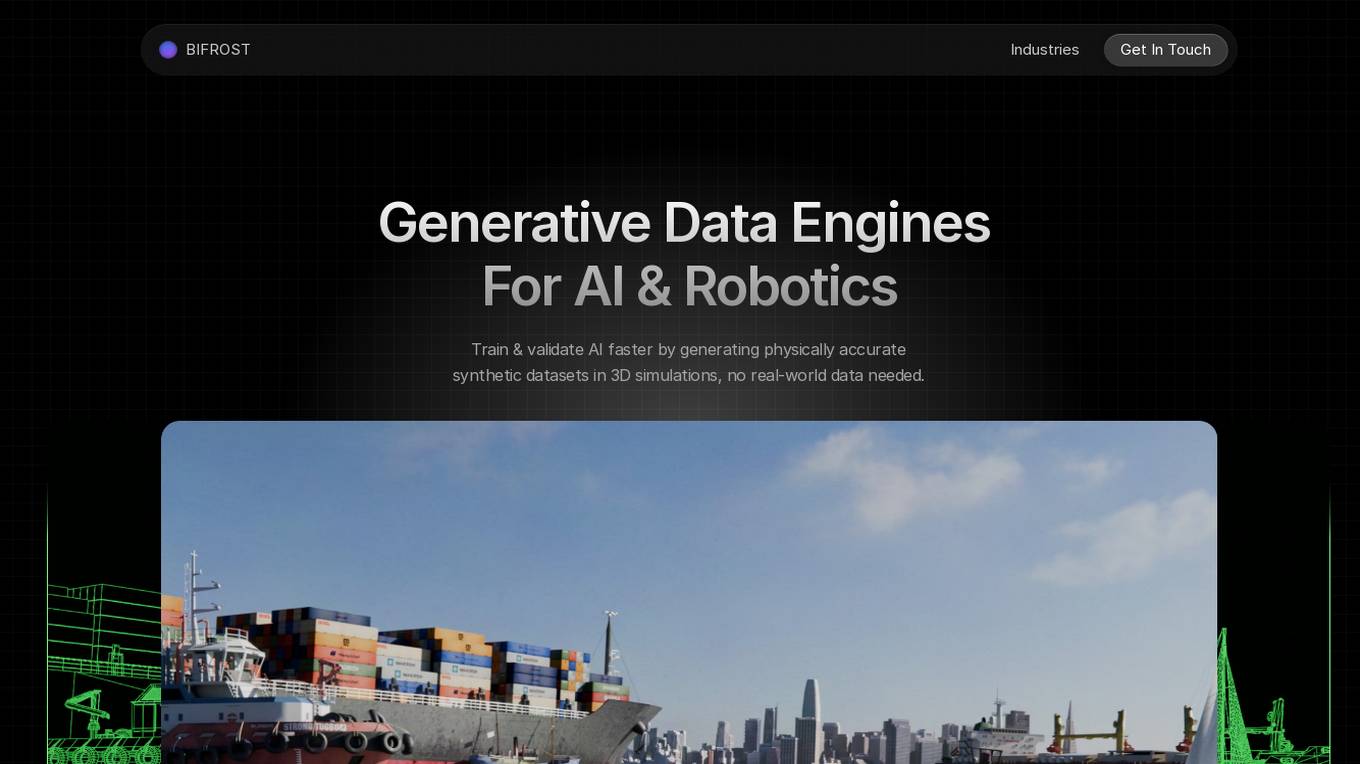
Bifrost AI
Bifrost AI is a data generation engine designed for AI and robotics applications. It enables users to train and validate AI models faster by generating physically accurate synthetic datasets in 3D simulations, eliminating the need for real-world data. The platform offers pixel-perfect labels, scenario metadata, and a simulated 3D world to enhance AI understanding. Bifrost AI empowers users to create new scenarios and datasets rapidly, stress test AI perception, and improve model performance. It is built for teams at every stage of AI development, offering features like automated labeling, class imbalance correction, and performance enhancement.
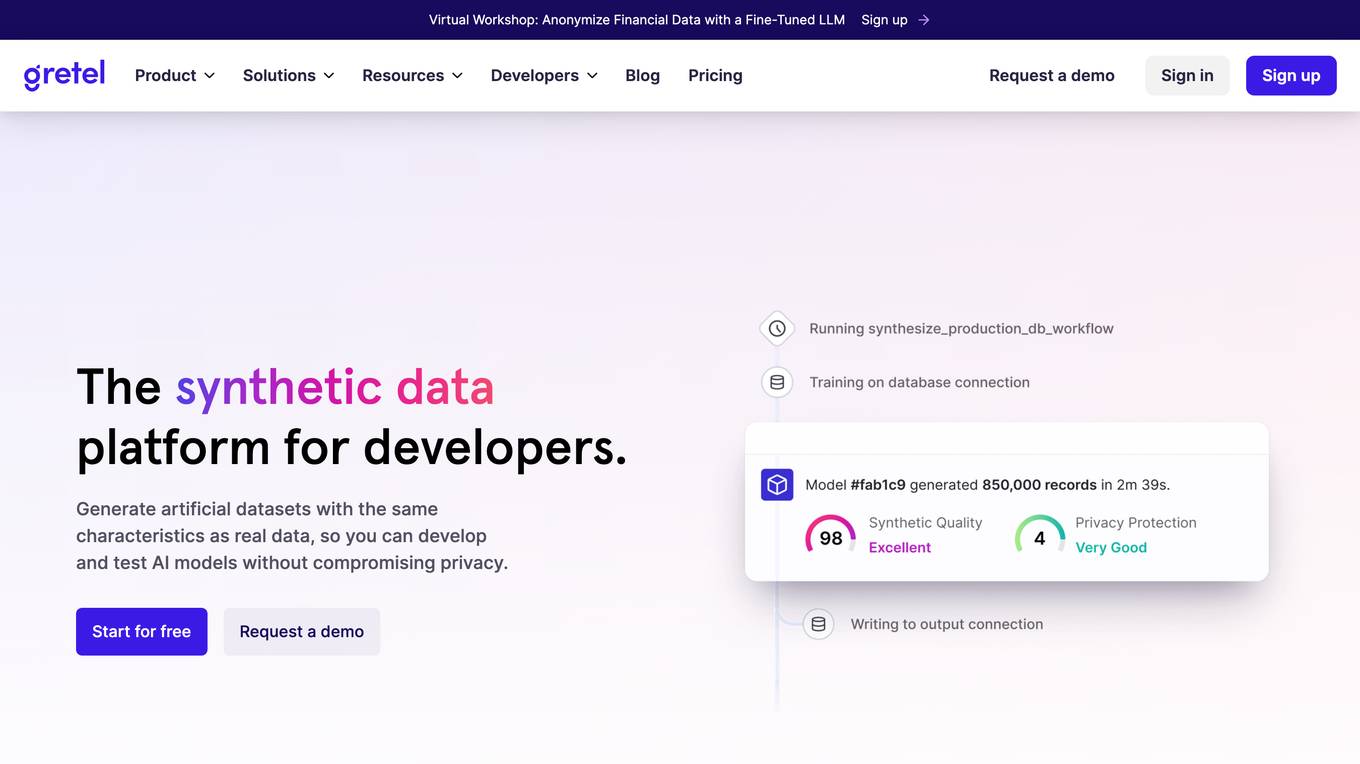
Gretel.ai
Gretel.ai is a synthetic data platform purpose-built for AI applications. It allows users to generate artificial, synthetic datasets with the same characteristics as real data, enabling the improvement of AI models without compromising privacy. The platform offers features such as generating data from input prompts, creating safe synthetic versions of sensitive datasets, flexible data transformation, building data pipelines, and measuring data quality. Gretel.ai is designed to help developers unlock synthetic data and achieve more with safe access to the right data.
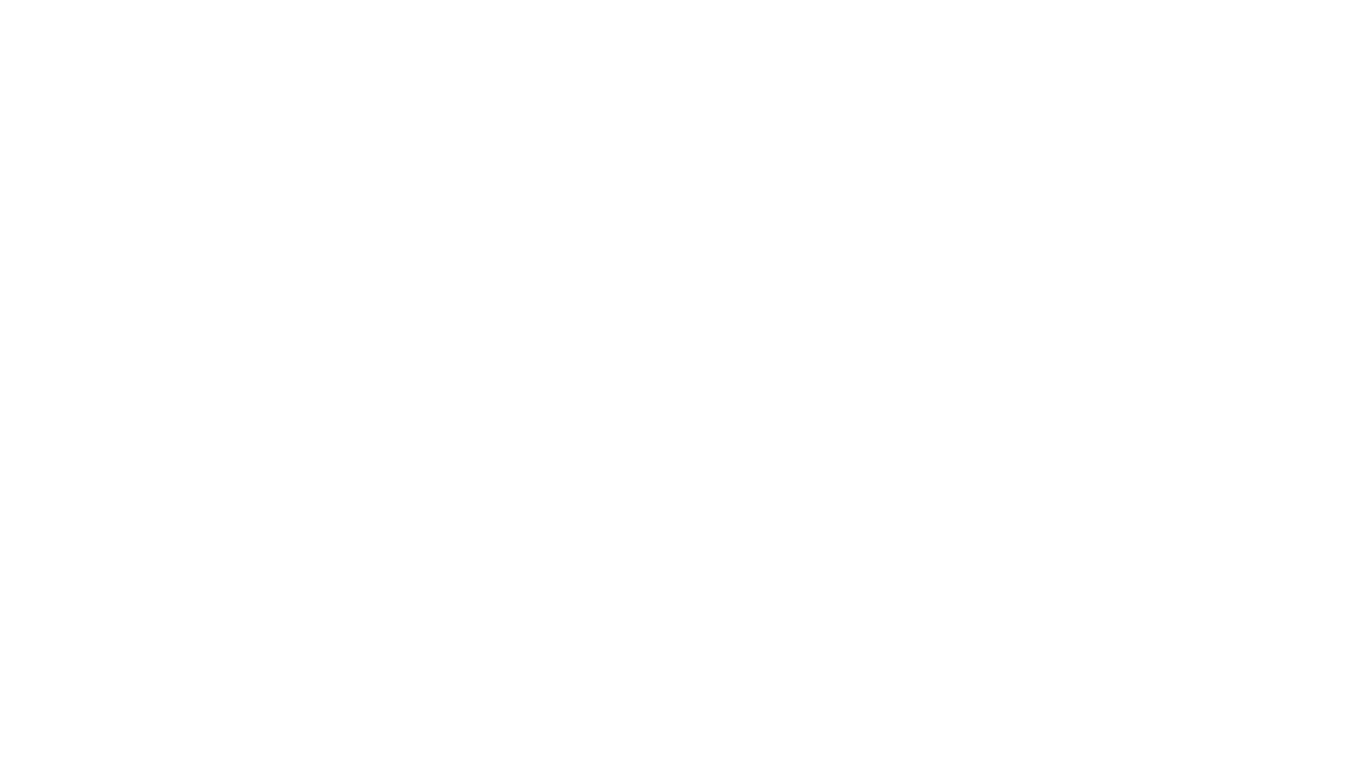
Syntho
Syntho is a self-service AI-generated synthetic data platform that offers a comprehensive solution for generating synthetic data for various purposes. It provides tools for de-identification, test data management, rule-based synthetic data generation, data masking, and more. With a focus on privacy and accuracy, Syntho enables users to create synthetic data that mirrors real production data while ensuring compliance with regulations and data privacy standards. The platform offers a range of features and use cases tailored to different industries, including healthcare, finance, and public organizations.
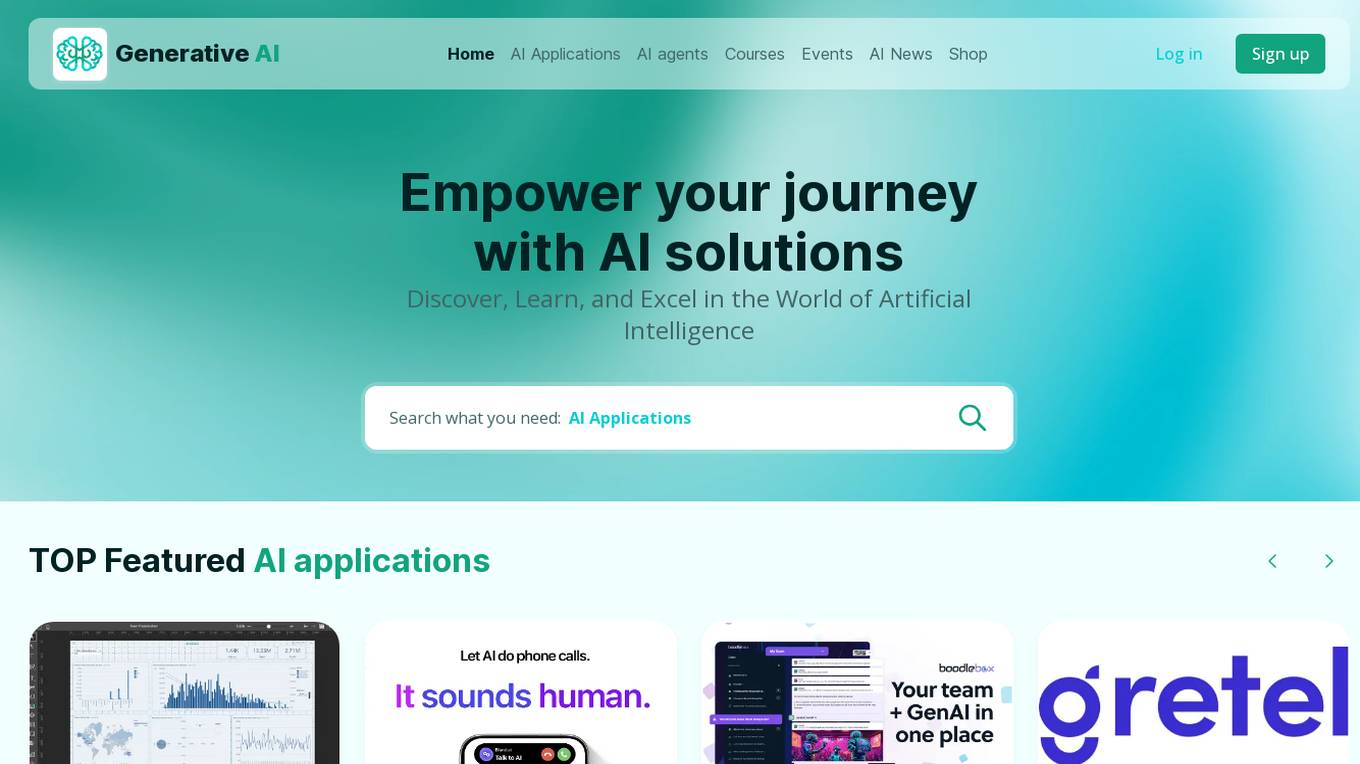
Gretel.ai
Gretel.ai is an AI tool that helps users incorporate generative AI into their data by generating synthetic data that is as good or better than the existing data. Users can fine-tune custom AI models and use Gretel's APIs to generate unlimited synthesized datasets, perform privacy-preserving transformations on sensitive data, and identify PII with advanced NLP detection. Gretel's APIs make it simple to generate anonymized and safe synthetic data, allowing users to innovate faster and preserve privacy while doing it. Gretel's platform includes Synthetics, Transform, and Classify APIs that provide users with a complete set of tools to create safe data. Gretel also offers a range of resources, including documentation, tutorials, GitHub projects, and open-source SDKs for developers. Gretel Cloud runners allow users to keep data contained by running Gretel containers in their environment or scaling out workloads to the cloud in seconds. Overall, Gretel.ai is a powerful AI tool for generating synthetic data that can help users unlock innovation and achieve more with safe access to the right data.
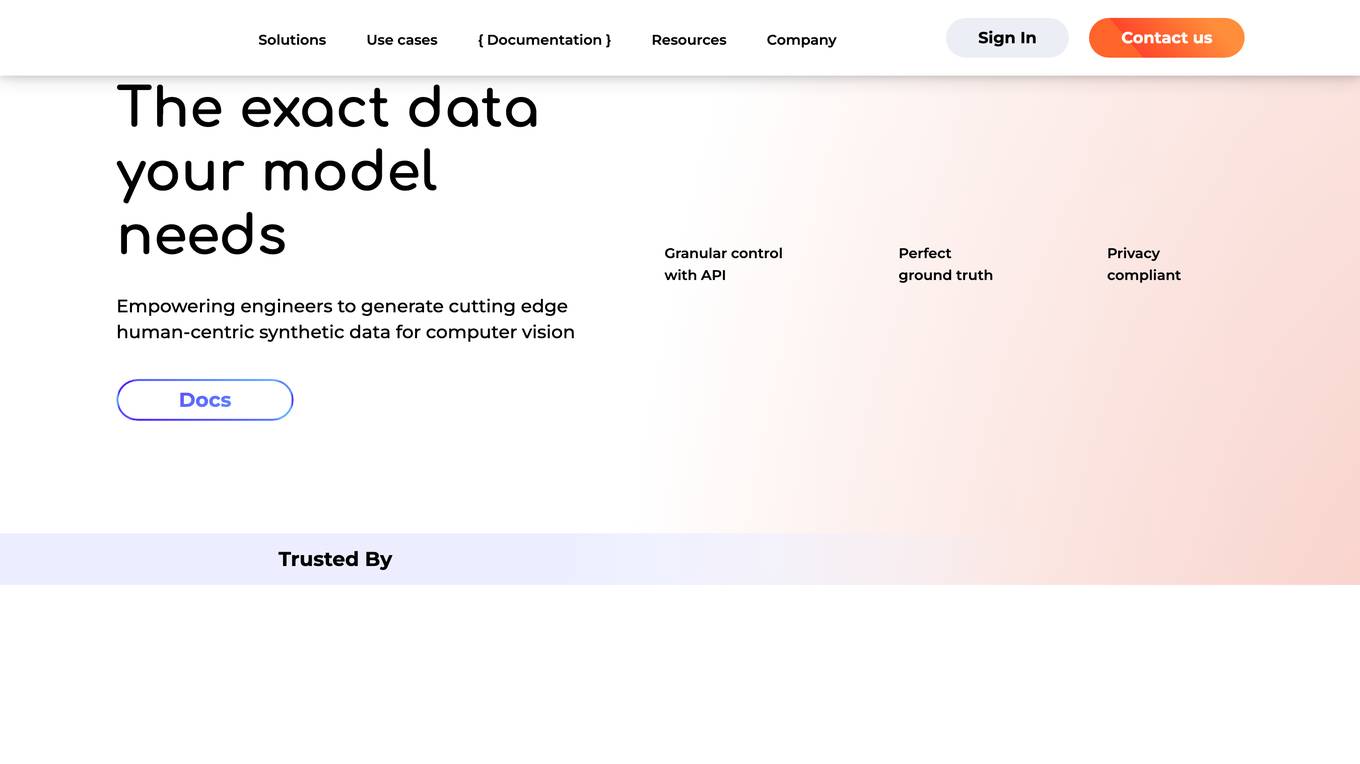
Datagen
Datagen is a platform that provides synthetic data for computer vision. Synthetic data is artificially generated data that can be used to train machine learning models. Datagen's data is generated using a variety of techniques, including 3D modeling, computer graphics, and machine learning. The company's data is used by a variety of industries, including automotive, security, smart office, fitness, cosmetics, and facial applications.
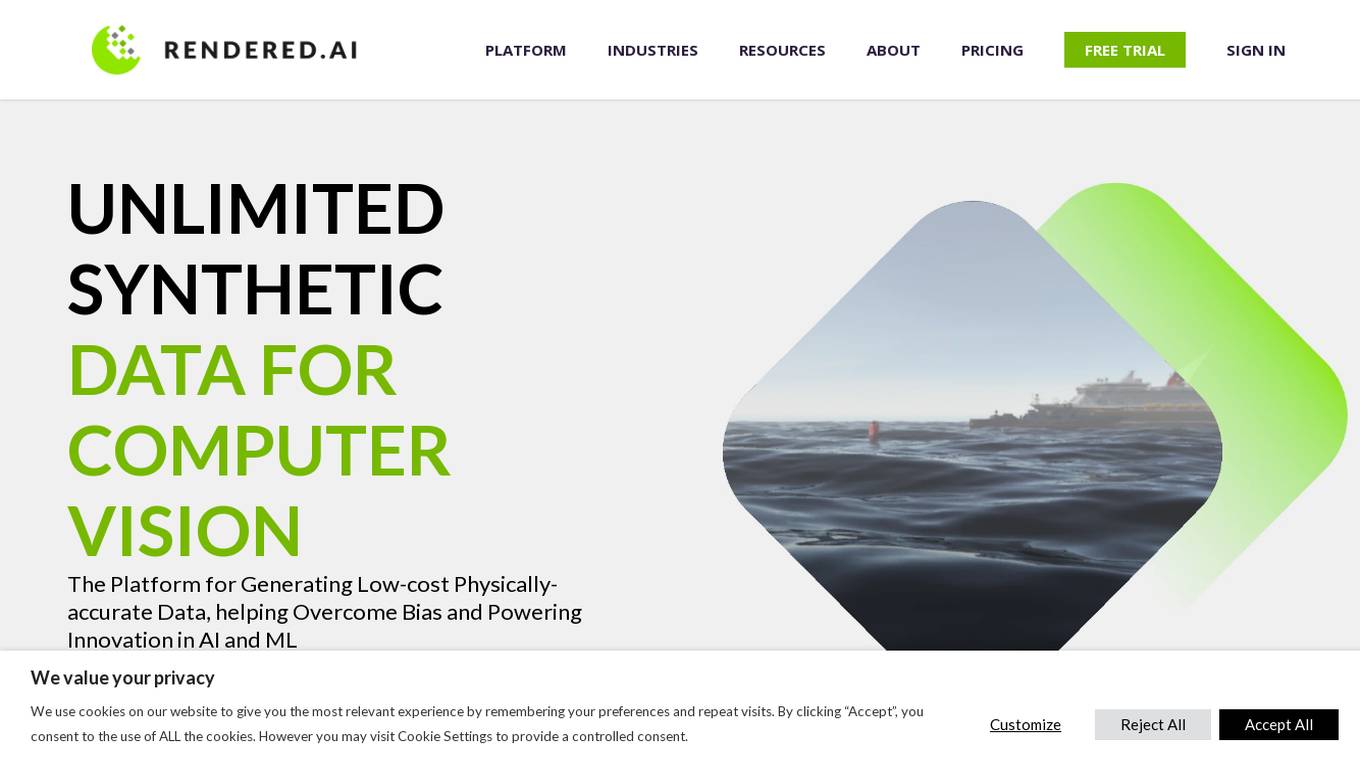
Rendered.ai
Rendered.ai is a platform that provides unlimited synthetic data for AI and ML applications, specifically focusing on computer vision. It helps in generating low-cost physically-accurate data to overcome bias and power innovation in AI and ML. The platform allows users to capture rare events and edge cases, acquire data that is difficult to obtain, overcome data labeling challenges, and simulate restricted or high-risk scenarios. Rendered.ai aims to revolutionize the use of synthetic data in AI and data analytics projects, with a vision that by 2030, synthetic data will surpass real data in AI models.
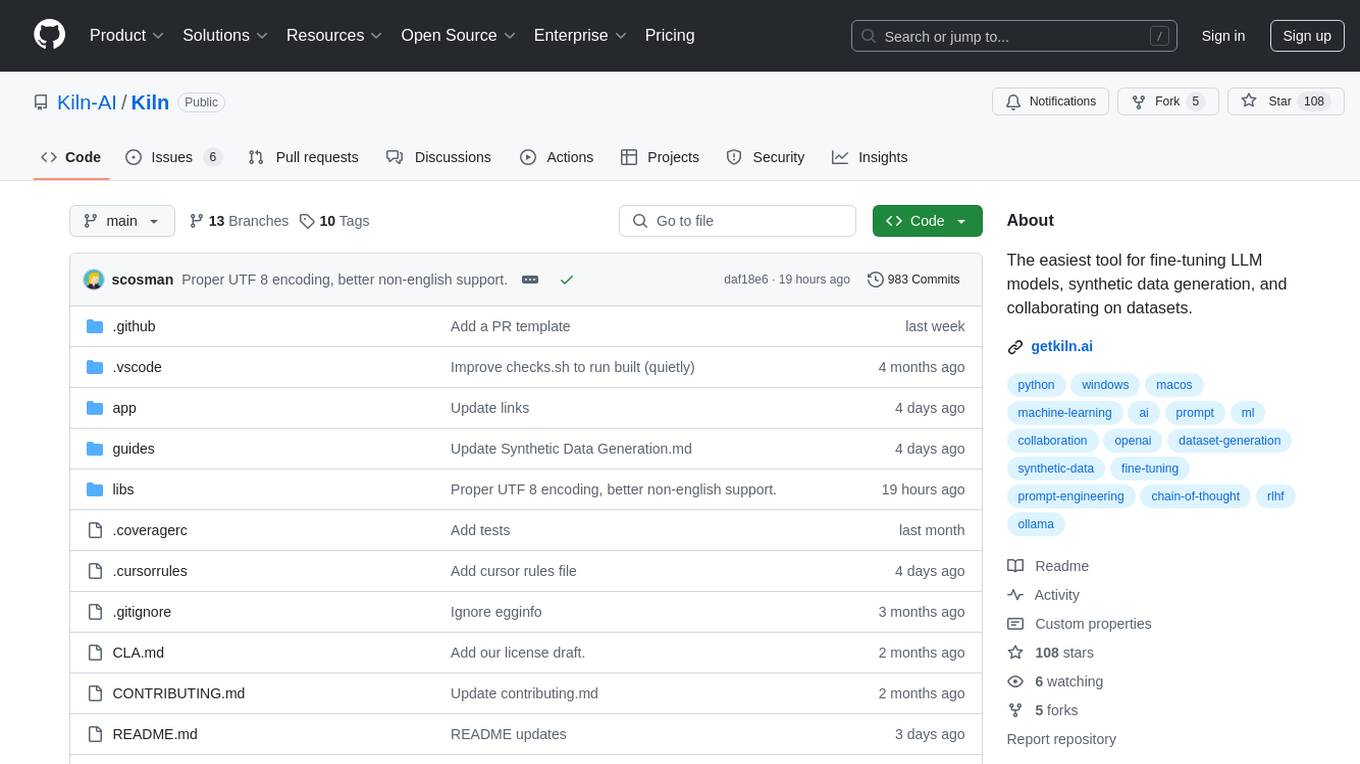
Kiln
Kiln is an AI tool designed for fine-tuning LLM models, generating synthetic data, and facilitating collaboration on datasets. It offers intuitive desktop apps, zero-code fine-tuning for various models, interactive visual tools for data generation, Git-based version control for datasets, and the ability to generate various prompts from data. Kiln supports a wide range of models and providers, provides an open-source library and API, prioritizes privacy, and allows structured data tasks in JSON format. The tool is free to use and focuses on rapid AI prototyping and dataset collaboration.
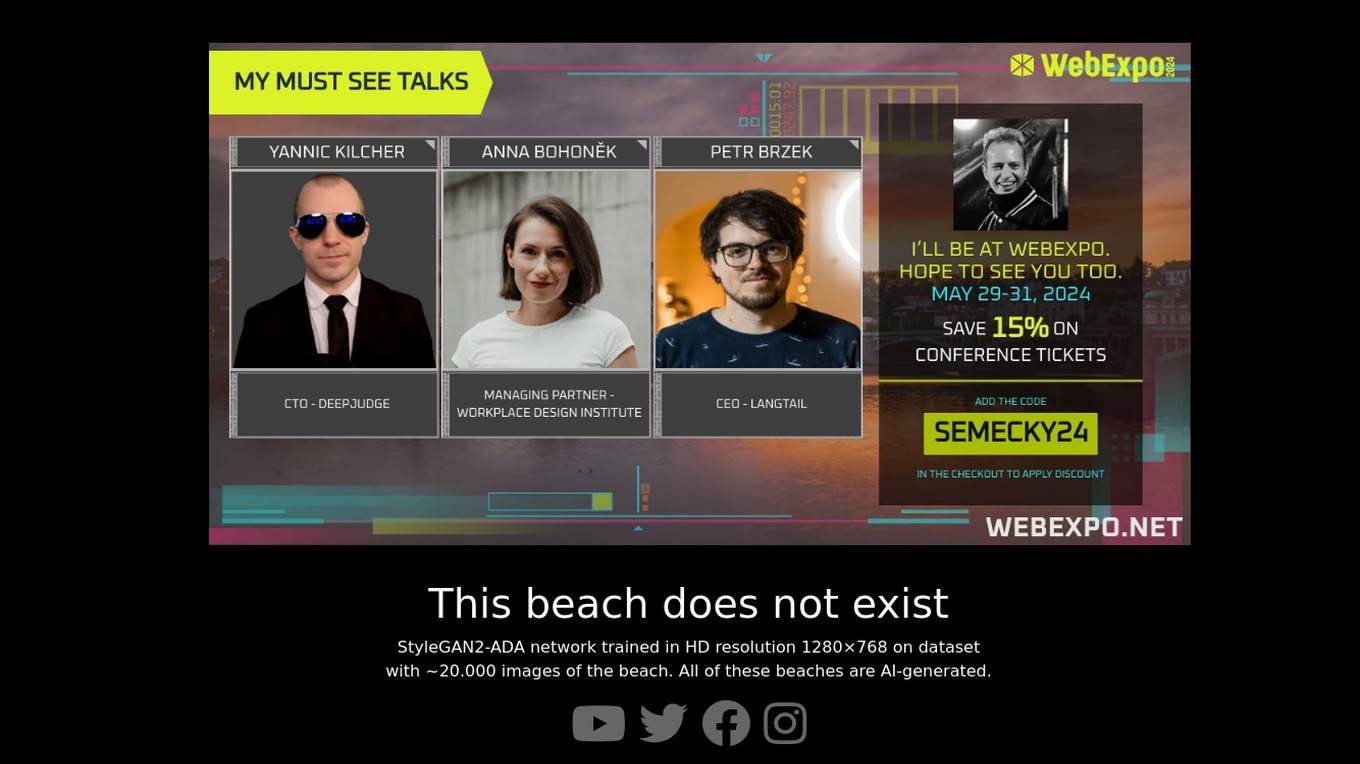
This Beach Does Not Exist
This Beach Does Not Exist is an AI application powered by StyleGAN2-ADA network, capable of generating realistic beach images. The website showcases AI-generated beach landscapes created from a dataset of approximately 20,000 images. Users can explore the training progress of the network, generate random images, utilize K-Means Clustering for image grouping, and download the network for experimentation or retraining purposes. Detailed technical information about the network architecture, dataset, training steps, and metrics is provided. The application is based on the GAN architecture developed by NVIDIA Labs and offers a unique experience of creating virtual beach scenes through AI technology.
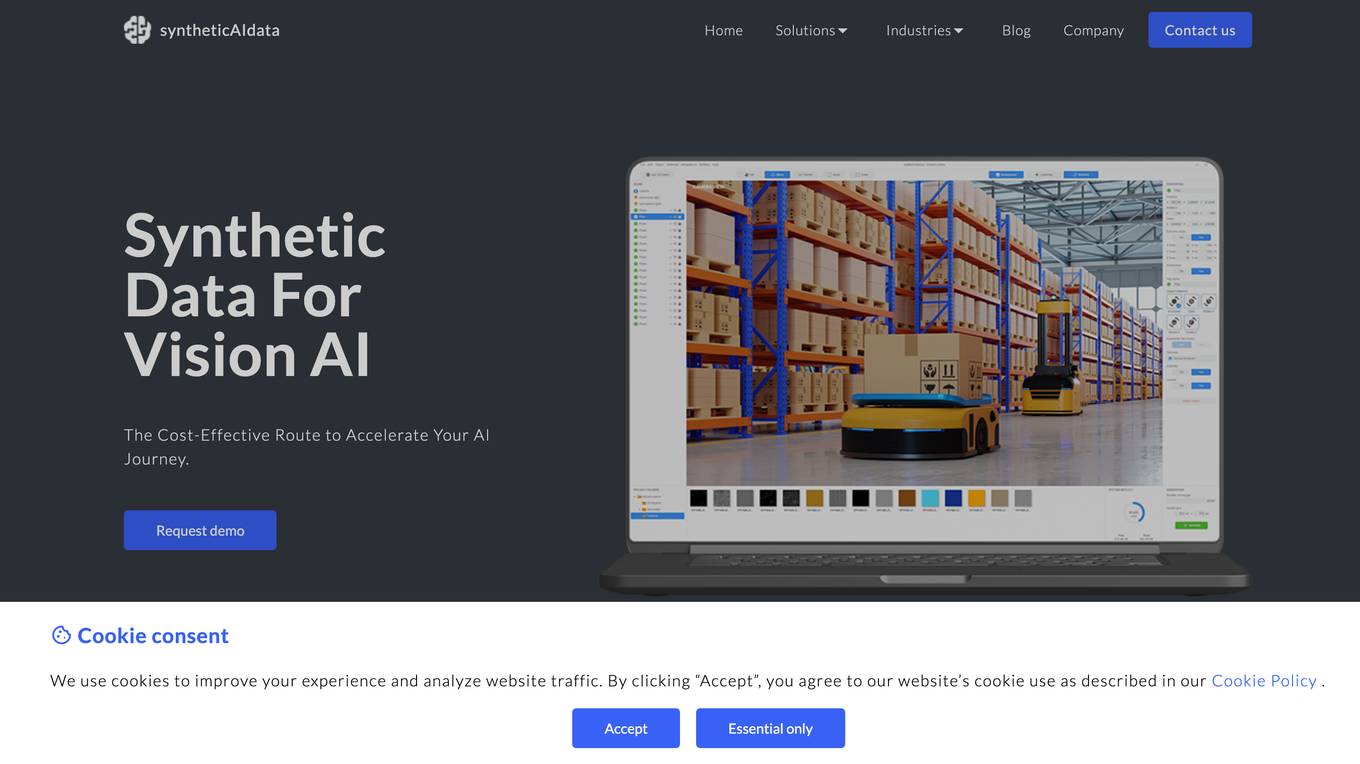
syntheticAIdata
syntheticAIdata is a platform that provides synthetic data for training vision AI models. Synthetic data is generated artificially, and it can be used to augment existing real-world datasets or to create new datasets from scratch. syntheticAIdata's platform is easy to use, and it can be integrated with leading cloud platforms. The company's mission is to make synthetic data accessible to everyone, and to help businesses overcome the challenges of acquiring high-quality data for training their vision AI models.
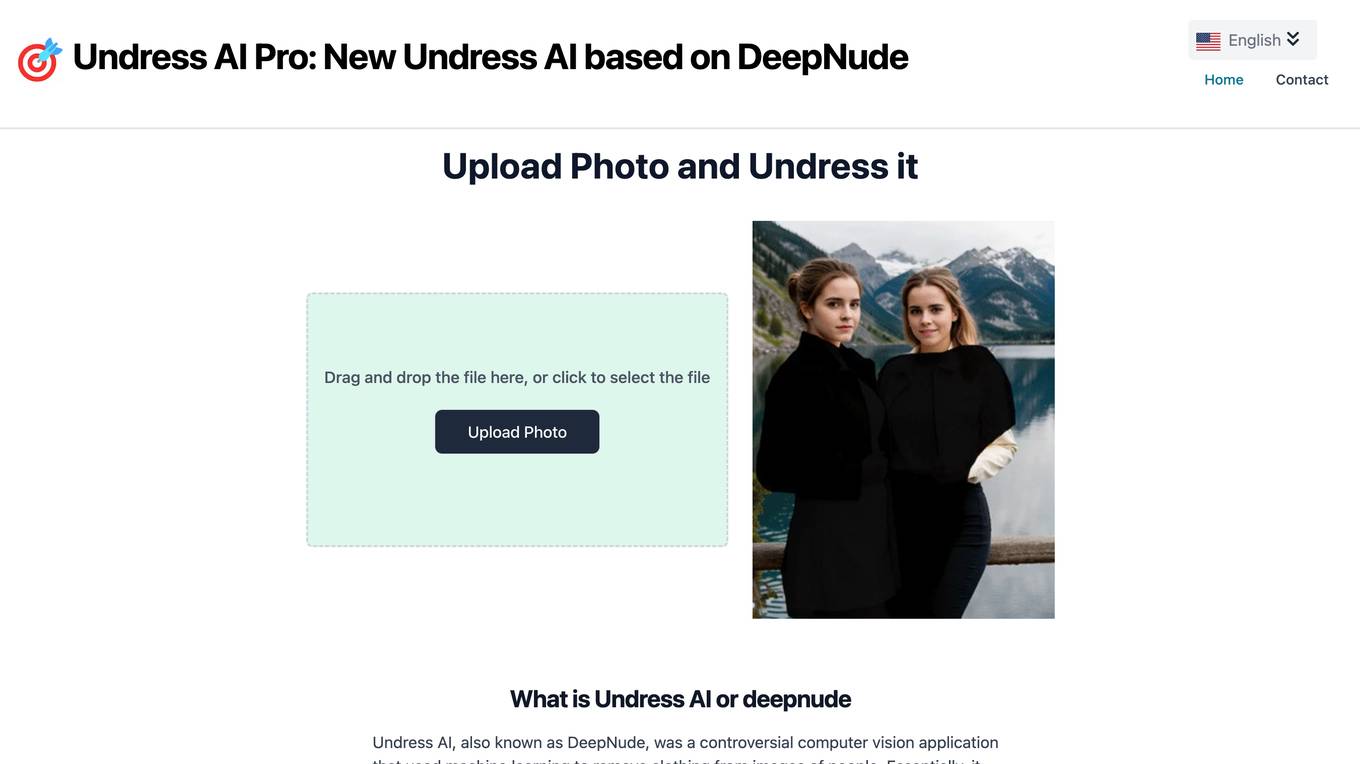
Undress AI Pro
Undress AI Pro is a controversial computer vision application that uses machine learning to remove clothing from images of people. It was based on deep learning and generative adversarial networks (GANs). The technology powering Undress AI and DeepNude was based on deep learning and generative adversarial networks (GANs). GANs involve two neural networks competing against each other - a generator creates synthetic images trying to mimic the training data, while a discriminator tries to distinguish the real images from the generated ones. Through this adversarial process, the generator learns to produce increasingly realistic outputs. For Undress AI, the GAN was trained on a dataset of nude and clothed images, allowing it to "unclothe" people in new images by generating the nudity.
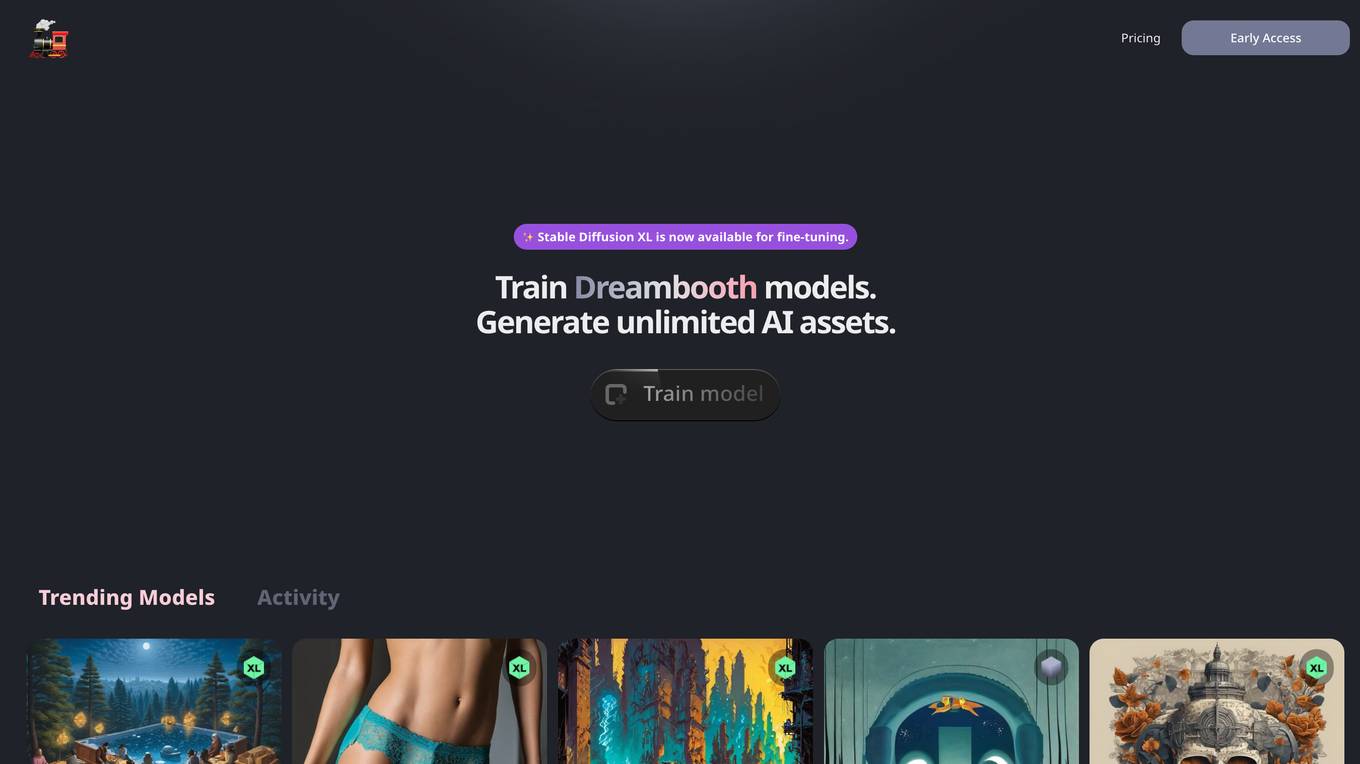
TrainEngine.ai
TrainEngine.ai is a powerful AI-powered image generation tool that allows users to create stunning, unique images from text prompts. With its advanced algorithms and vast dataset, TrainEngine.ai can generate images in a wide range of styles, from realistic to abstract, and in various formats, including photos, paintings, and illustrations. The platform is easy to use, making it accessible to both professional artists and hobbyists alike. TrainEngine.ai offers a range of features, including the ability to fine-tune models, generate unlimited AI assets, and access trending models. It also provides a marketplace where users can buy and sell AI-generated images.
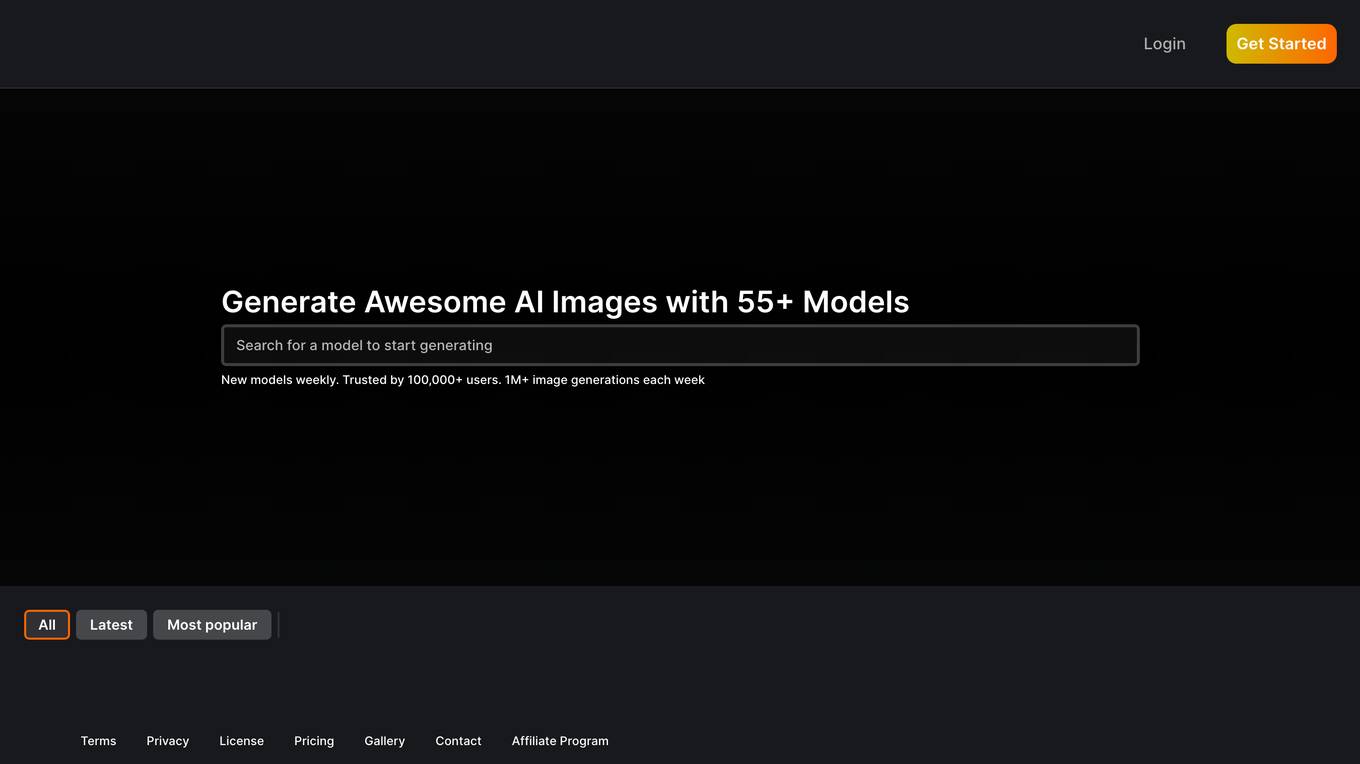
Supermachine
Supermachine is an AI-powered image generator that allows users to create realistic and unique images from scratch. With a simple text prompt, users can generate images of anything they can imagine, from landscapes and portraits to abstract concepts and surreal scenes. Supermachine's AI technology is trained on a massive dataset of images, allowing it to generate images that are both visually appealing and realistic.
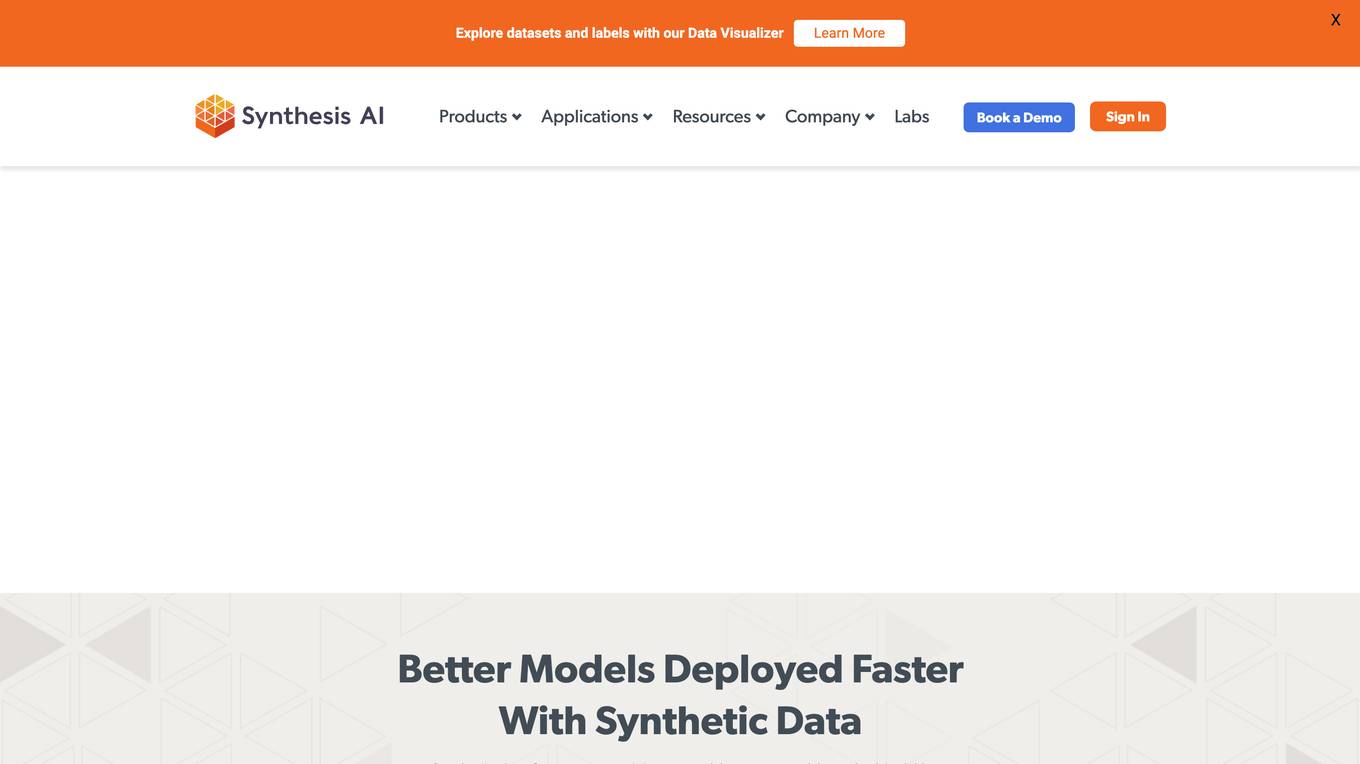
Synthesis AI
Synthesis AI is a synthetic data platform that enables more capable and ethical computer vision AI. It provides on-demand labeled images and videos, photorealistic images, and 3D generative AI to help developers build better models faster. Synthesis AI's products include Synthesis Humans, which allows users to create detailed images and videos of digital humans with rich annotations; Synthesis Scenarios, which enables users to craft complex multi-human simulations across a variety of environments; and a range of applications for industries such as ID verification, automotive, avatar creation, virtual fashion, AI fitness, teleconferencing, visual effects, and security.
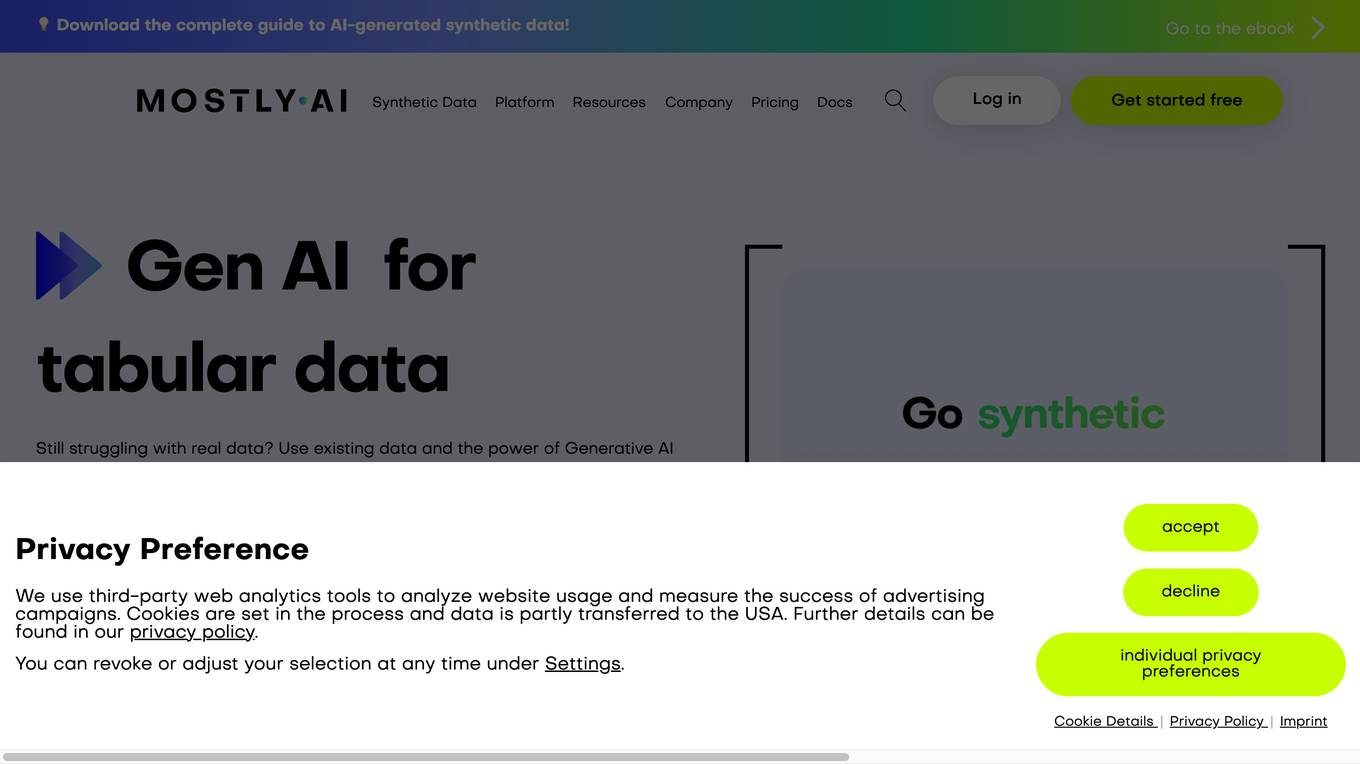
MOSTLY AI Platform
The website offers a Synthetic Data Generation platform with the highest accuracy for free. It provides detailed information on synthetic data, data anonymization, and features a Python Client for data generation. The platform ensures privacy and security, allowing users to create fully anonymous synthetic data from original data. It supports various AI/ML use cases, self-service analytics, testing & QA, and data sharing. The platform is designed for Enterprise organizations, offering scalability, privacy by design, and the world's most accurate synthetic data.
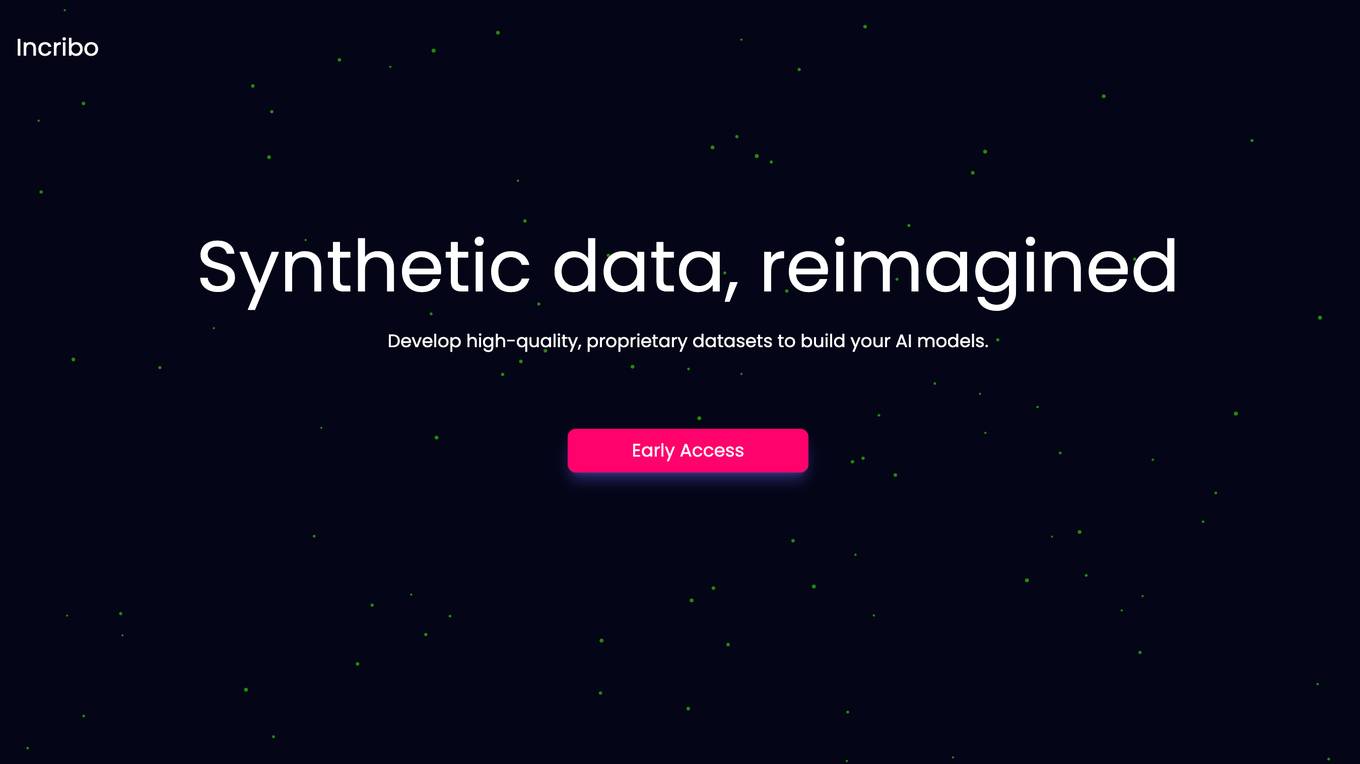
Incribo
Incribo is a company that provides synthetic data for training machine learning models. Synthetic data is artificially generated data that is designed to mimic real-world data. This data can be used to train machine learning models without the need for real-world data, which can be expensive and difficult to obtain. Incribo's synthetic data is high quality and affordable, making it a valuable resource for machine learning developers.
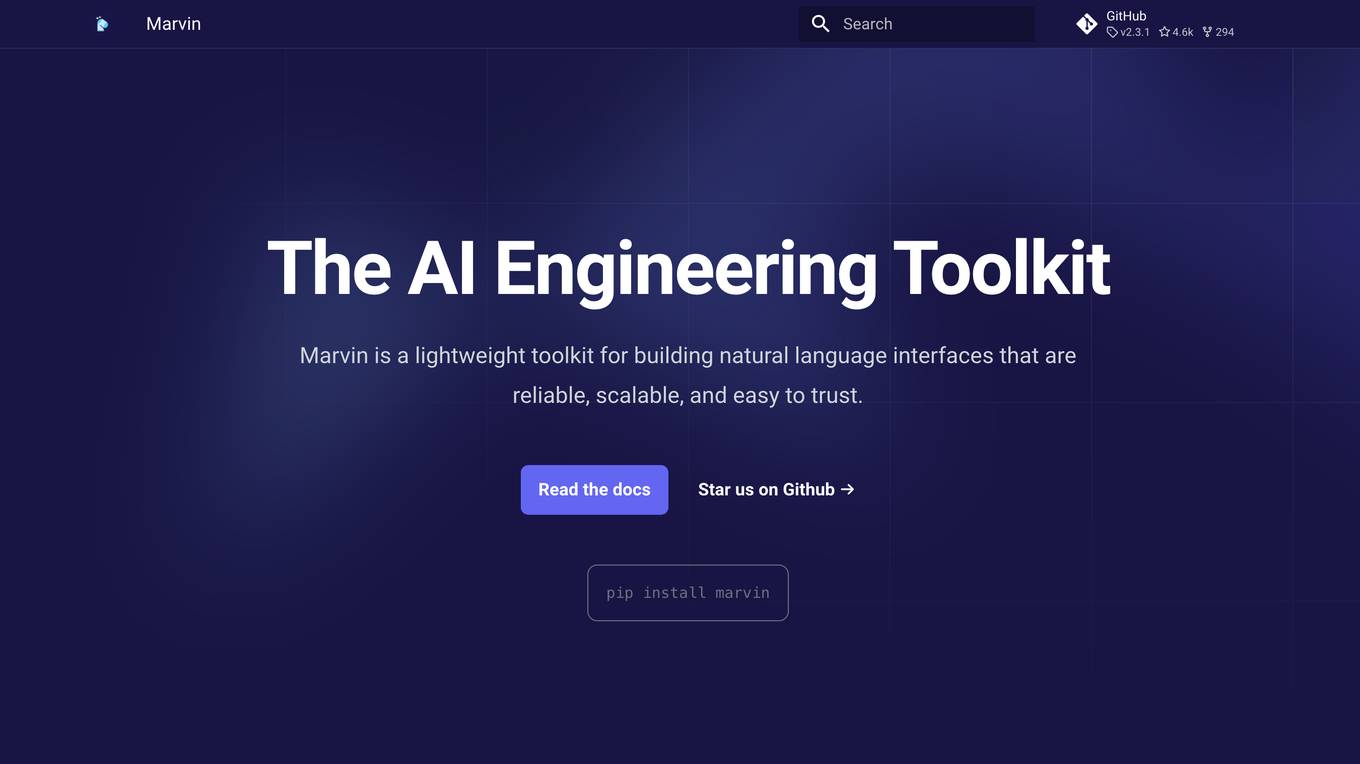
Marvin
Marvin is a lightweight toolkit for building natural language interfaces that are reliable, scalable, and easy to trust. It provides a variety of AI functions for text, images, audio, and video, as well as interactive tools and utilities. Marvin is designed to be easy to use and integrate, and it can be used to build a wide range of applications, from simple chatbots to complex AI-powered systems.
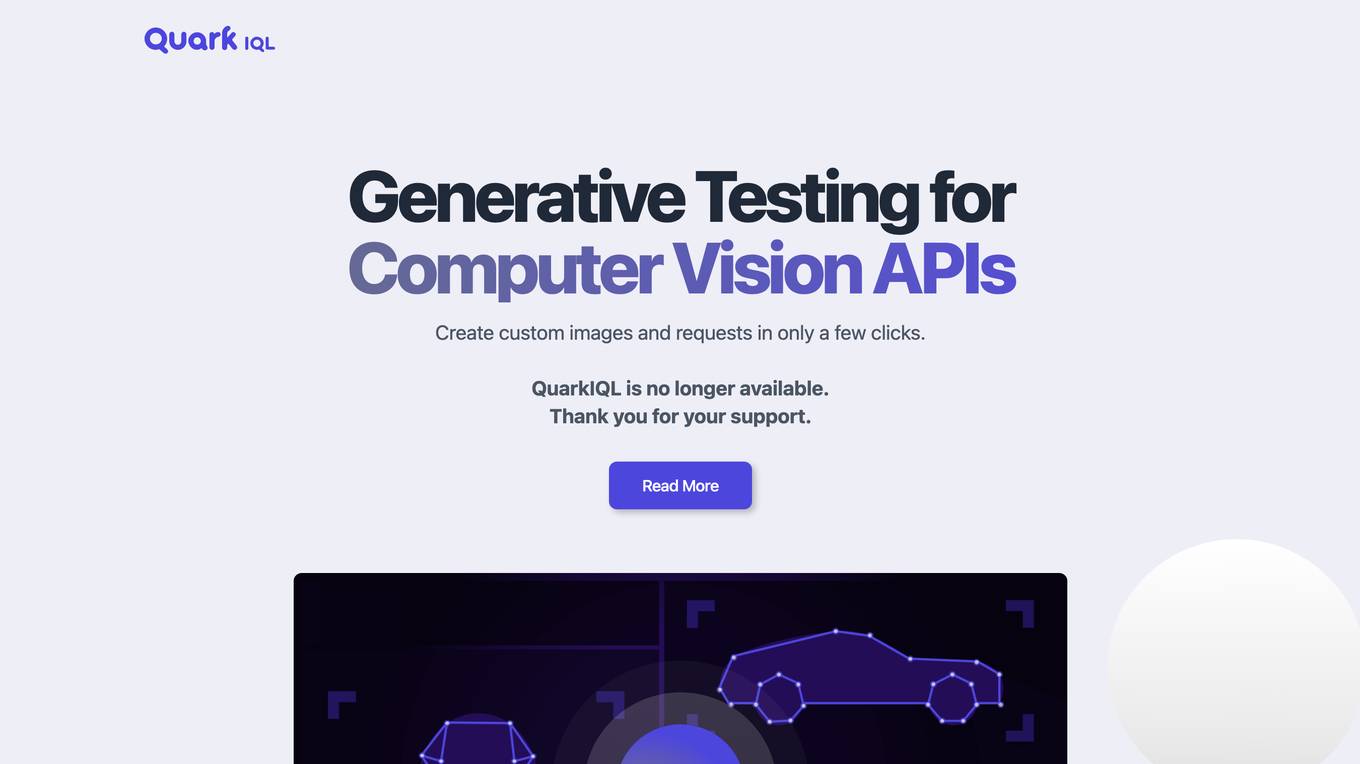
QuarkIQL
QuarkIQL is a generative testing tool for computer vision APIs. It allows users to create custom test images and requests with just a few clicks. QuarkIQL also provides a log of your queries so you can run more experiments without starting from square one.
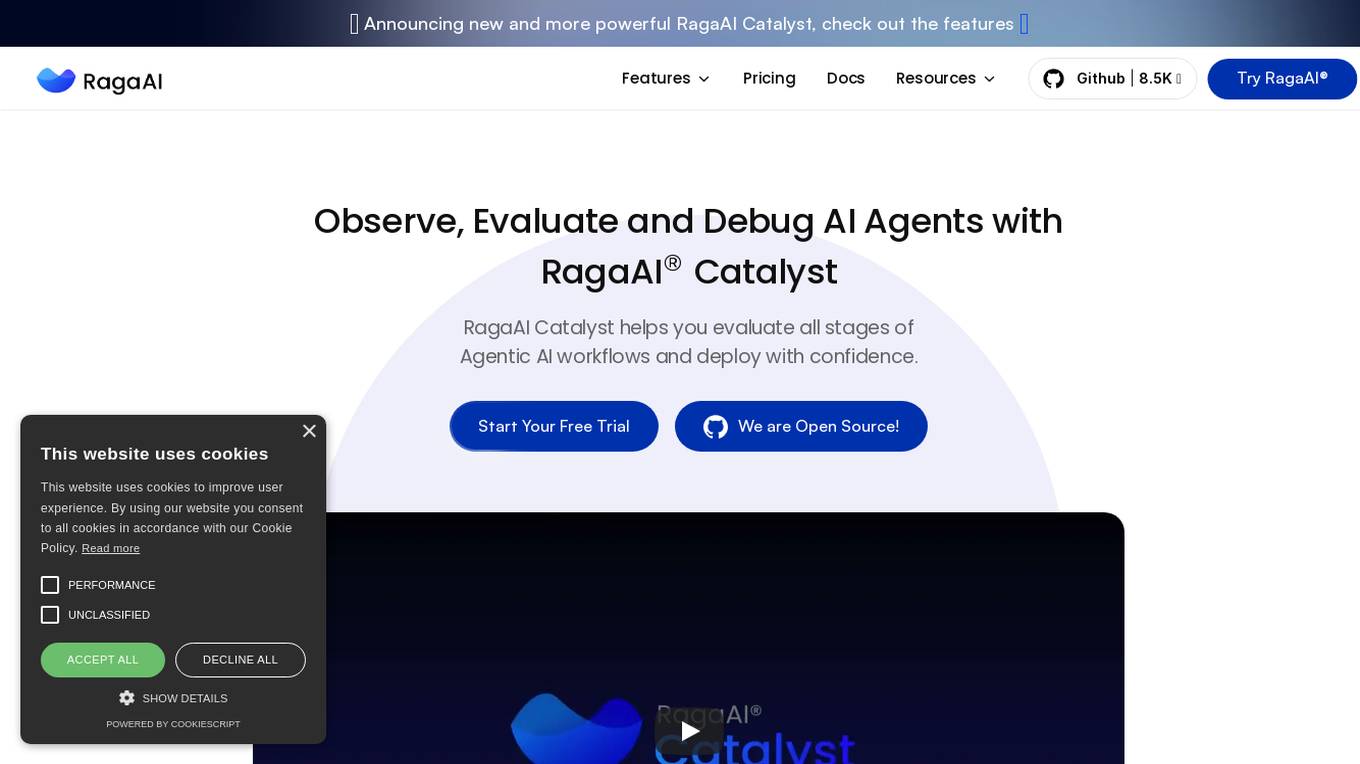
RagaAI Catalyst
RagaAI Catalyst is a sophisticated AI observability, monitoring, and evaluation platform designed to help users observe, evaluate, and debug AI agents at all stages of Agentic AI workflows. It offers features like visualizing trace data, instrumenting and monitoring tools and agents, enhancing AI performance, agentic testing, comprehensive trace logging, evaluation for each step of the agent, enterprise-grade experiment management, secure and reliable LLM outputs, finetuning with human feedback integration, defining custom evaluation logic, generating synthetic data, and optimizing LLM testing with speed and precision. The platform is trusted by AI leaders globally and provides a comprehensive suite of tools for AI developers and enterprises.
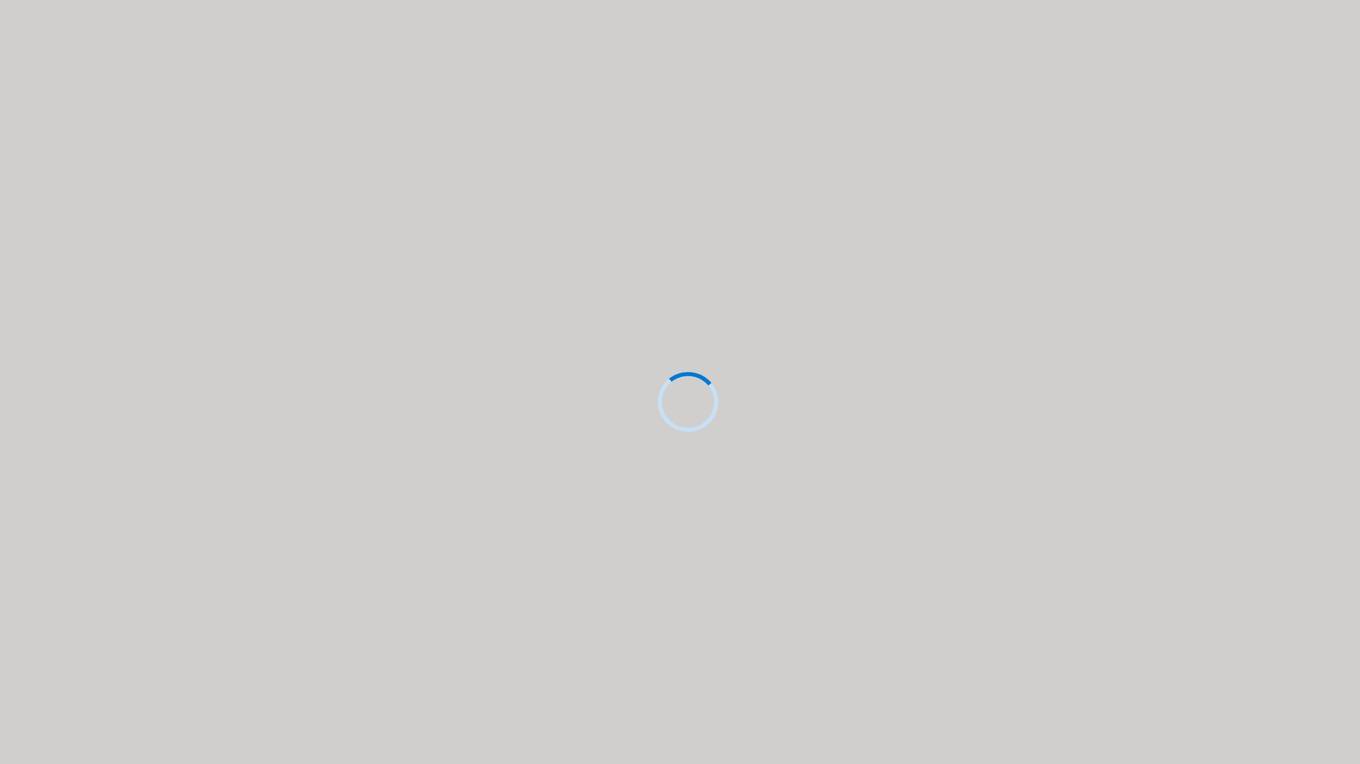
Speech Studio
Speech Studio is a cloud-based speech-to-text and text-to-speech platform that enables developers to add speech capabilities to their applications. With Speech Studio, developers can easily transcribe audio and video files, generate synthetic speech, and build custom speech models. Speech Studio is a powerful tool that can be used to improve the accessibility, efficiency, and user experience of any application.
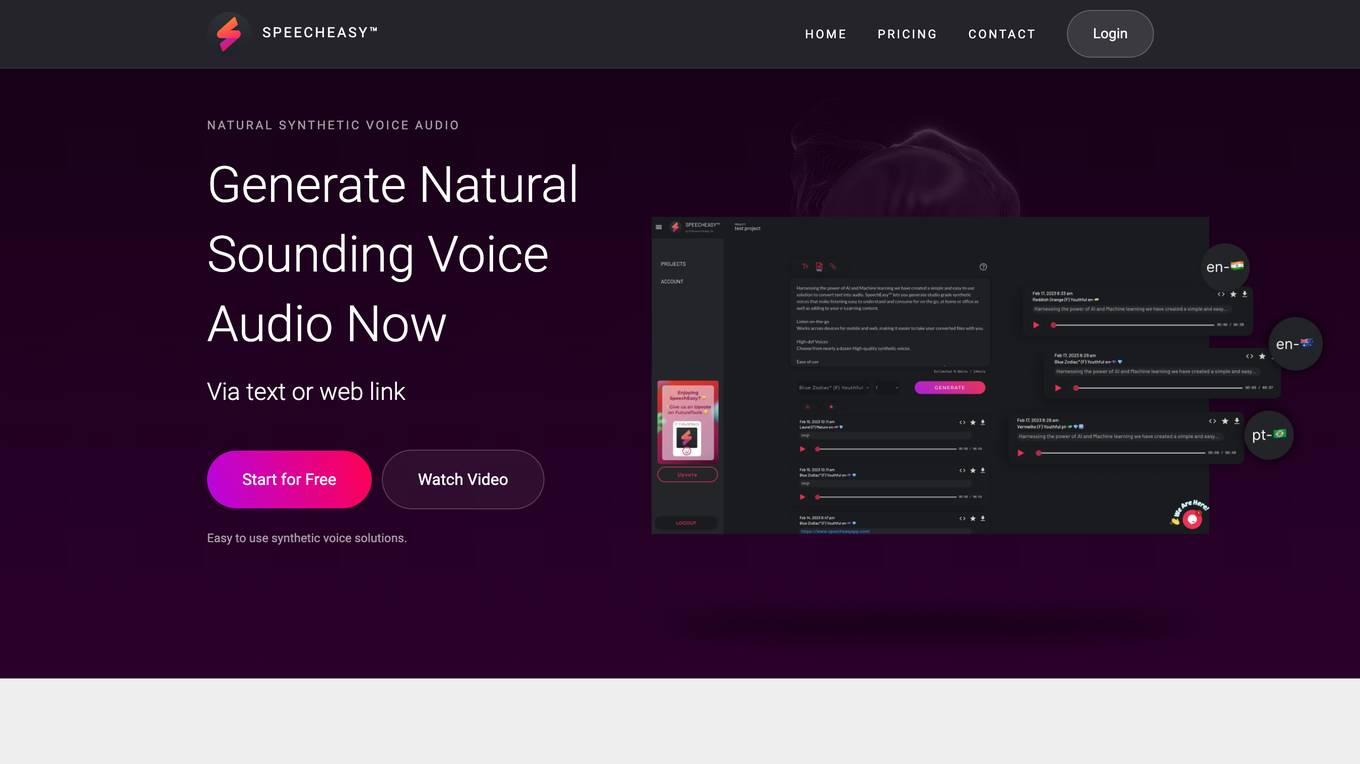
SpeechEasy
SpeechEasy is a high-quality text-to-speech tool that harnesses the power of AI and machine learning to convert text into natural-sounding audio. With SpeechEasy, you can generate studio-grade synthetic voices that are easy to understand and consume, making it perfect for on-the-go listening, home or office use, and e-learning content.
20 - Open Source AI Tools
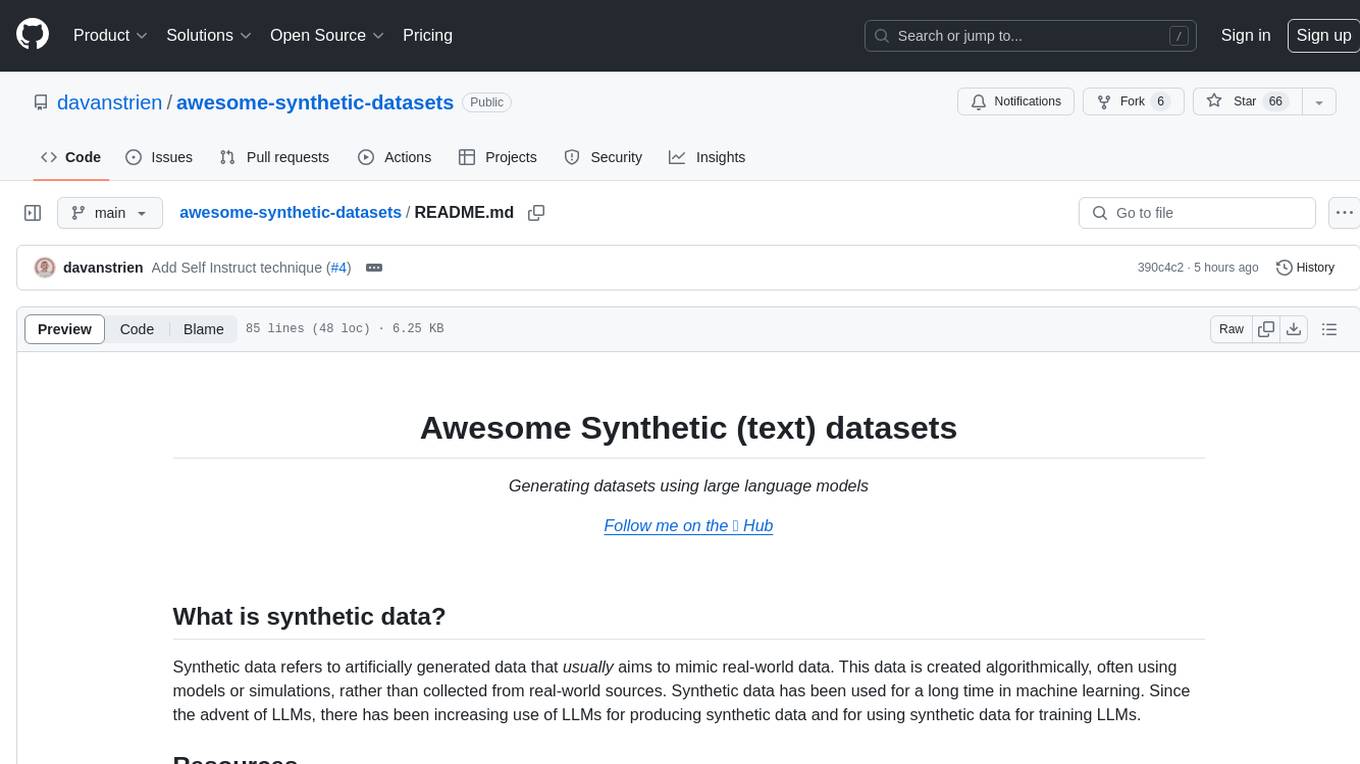
awesome-synthetic-datasets
This repository focuses on organizing resources for building synthetic datasets using large language models. It covers important datasets, libraries, tools, tutorials, and papers related to synthetic data generation. The goal is to provide pragmatic and practical resources for individuals interested in creating synthetic datasets for machine learning applications.
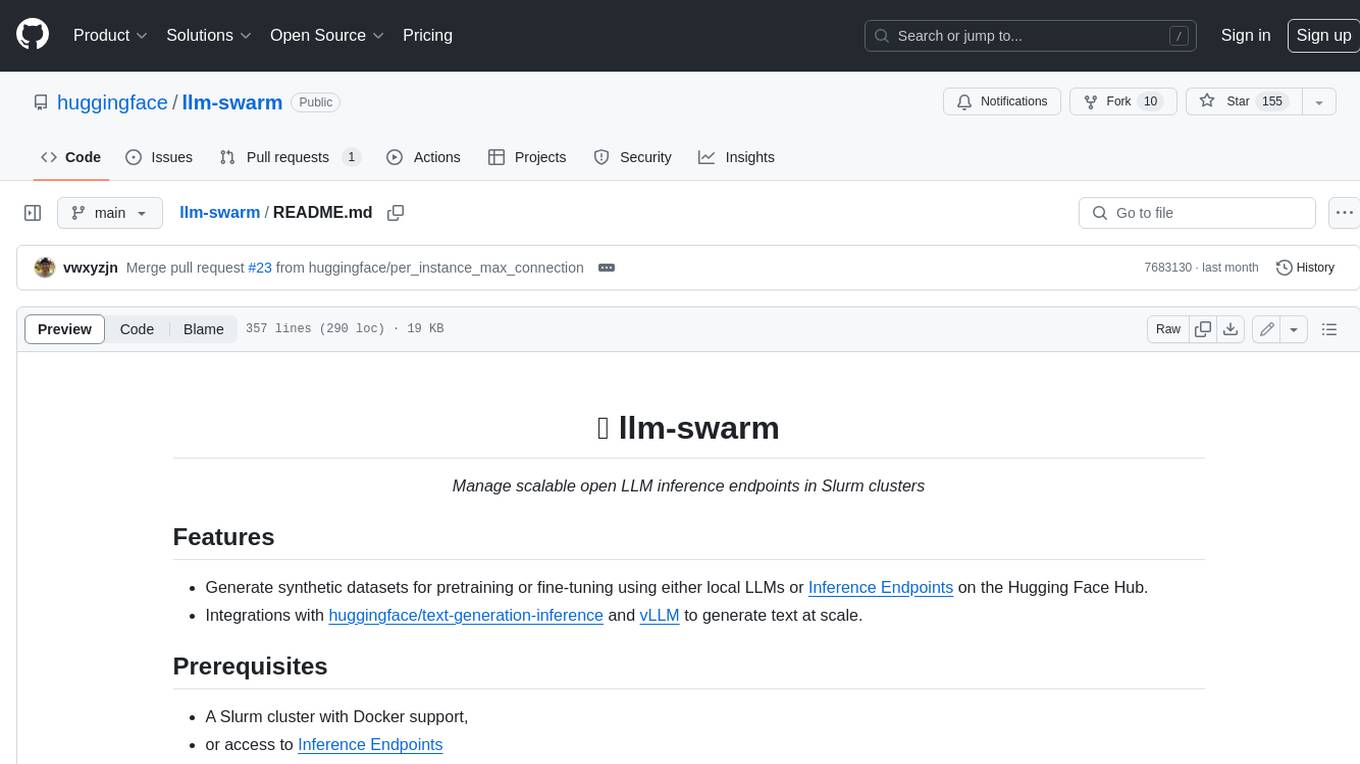
llm-swarm
llm-swarm is a tool designed to manage scalable open LLM inference endpoints in Slurm clusters. It allows users to generate synthetic datasets for pretraining or fine-tuning using local LLMs or Inference Endpoints on the Hugging Face Hub. The tool integrates with huggingface/text-generation-inference and vLLM to generate text at scale. It manages inference endpoint lifetime by automatically spinning up instances via `sbatch`, checking if they are created or connected, performing the generation job, and auto-terminating the inference endpoints to prevent idling. Additionally, it provides load balancing between multiple endpoints using a simple nginx docker for scalability. Users can create slurm files based on default configurations and inspect logs for further analysis. For users without a Slurm cluster, hosted inference endpoints are available for testing with usage limits based on registration status.
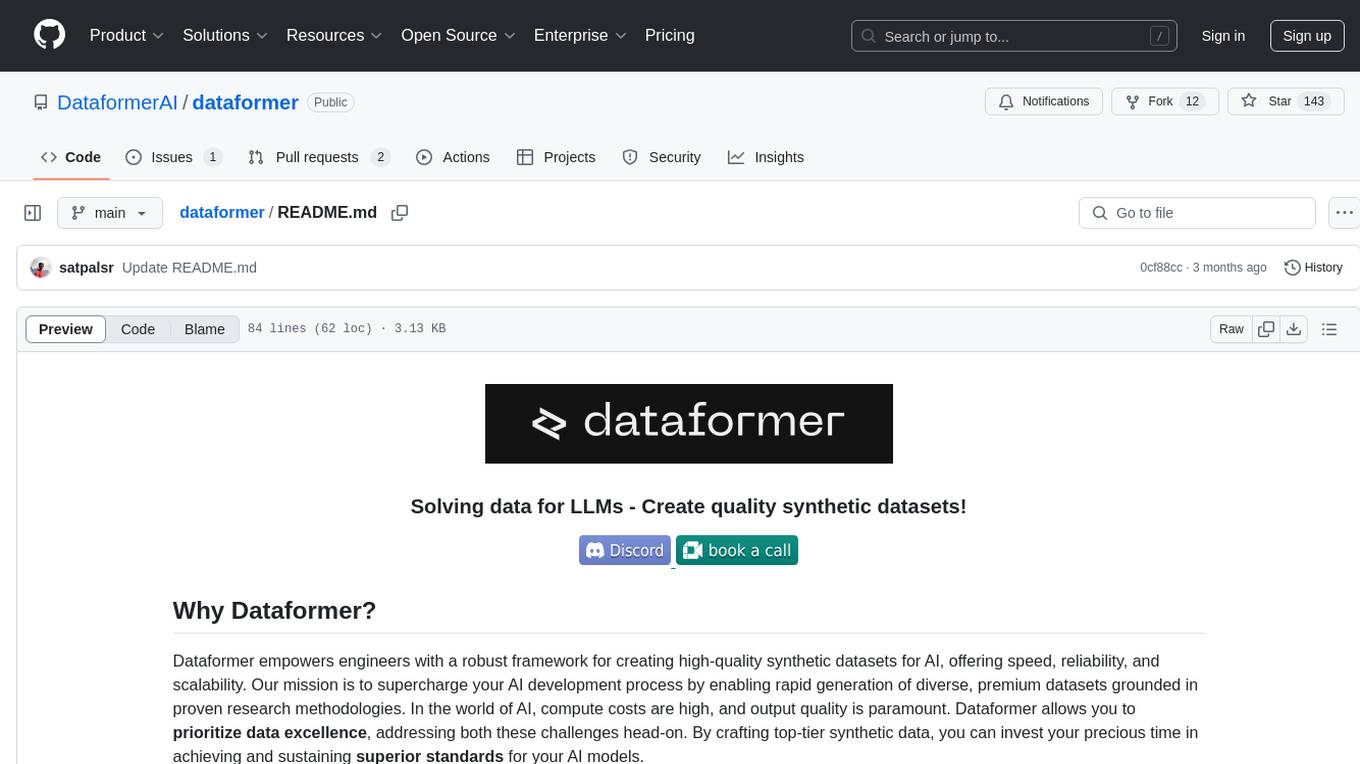
dataformer
Dataformer is a robust framework for creating high-quality synthetic datasets for AI, offering speed, reliability, and scalability. It empowers engineers to rapidly generate diverse datasets grounded in proven research methodologies, enabling them to prioritize data excellence and achieve superior standards for AI models. Dataformer integrates with multiple LLM providers using one unified API, allowing parallel async API calls and caching responses to minimize redundant calls and reduce operational expenses. Leveraging state-of-the-art research papers, Dataformer enables users to generate synthetic data with adaptability, scalability, and resilience, shifting their focus from infrastructure concerns to refining data and enhancing models.
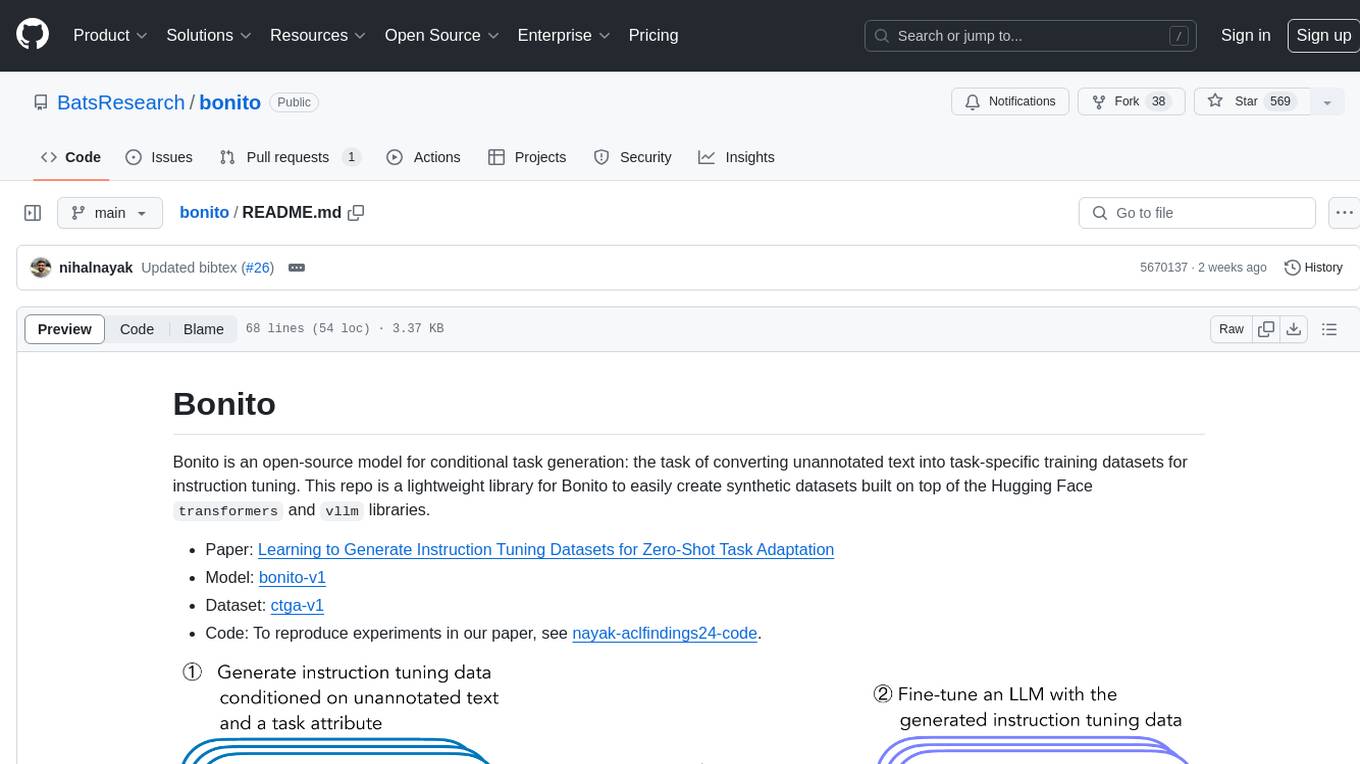
bonito
Bonito is an open-source model for conditional task generation, converting unannotated text into task-specific training datasets for instruction tuning. It is a lightweight library built on top of Hugging Face `transformers` and `vllm` libraries. The tool supports various task types such as question answering, paraphrase generation, sentiment analysis, summarization, and more. Users can easily generate synthetic instruction tuning datasets using Bonito for zero-shot task adaptation.
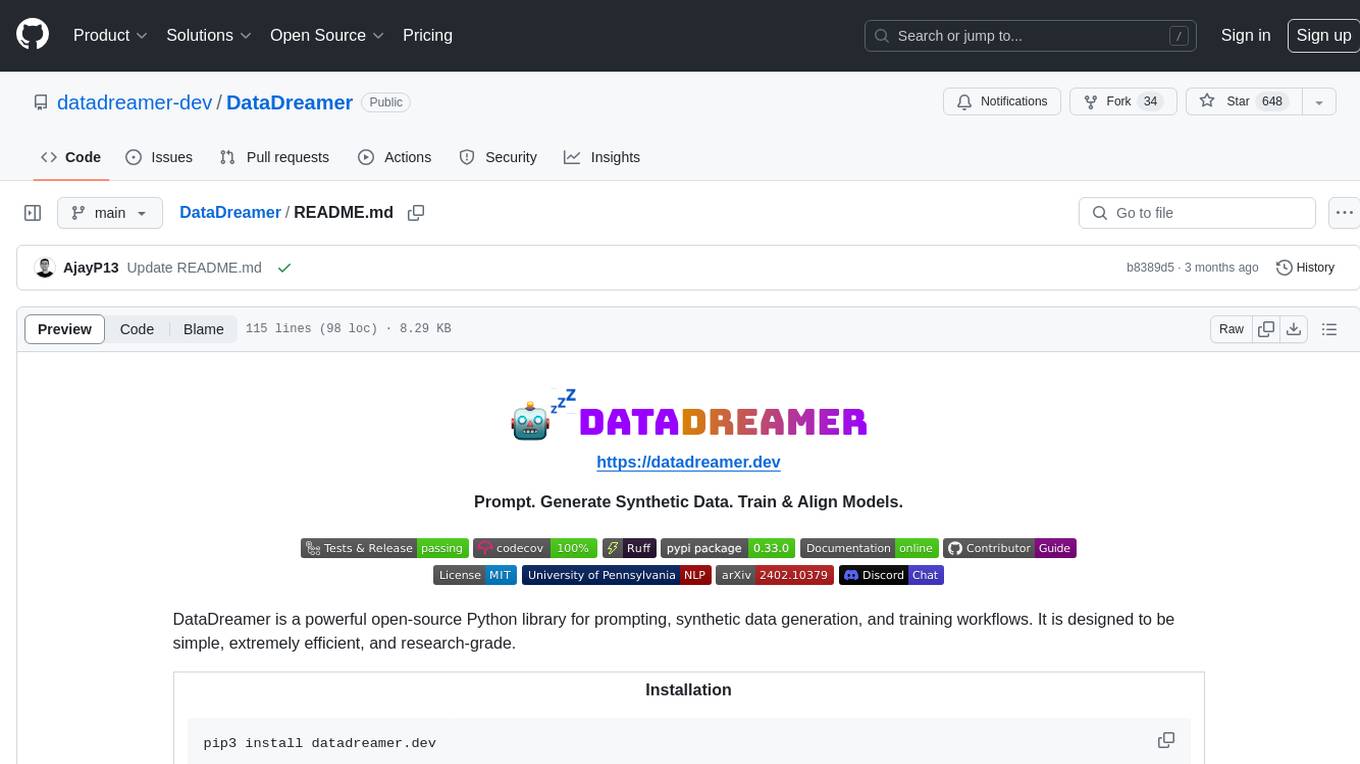
DataDreamer
DataDreamer is a powerful open-source Python library designed for prompting, synthetic data generation, and training workflows. It is simple, efficient, and research-grade, allowing users to create prompting workflows, generate synthetic datasets, and train models with ease. The library is built for researchers, by researchers, focusing on correctness, best practices, and reproducibility. It offers features like aggressive caching, resumability, support for bleeding-edge techniques, and easy sharing of datasets and models. DataDreamer enables users to run multi-step prompting workflows, generate synthetic datasets for various tasks, and train models by aligning, fine-tuning, instruction-tuning, and distilling them using existing or synthetic data.
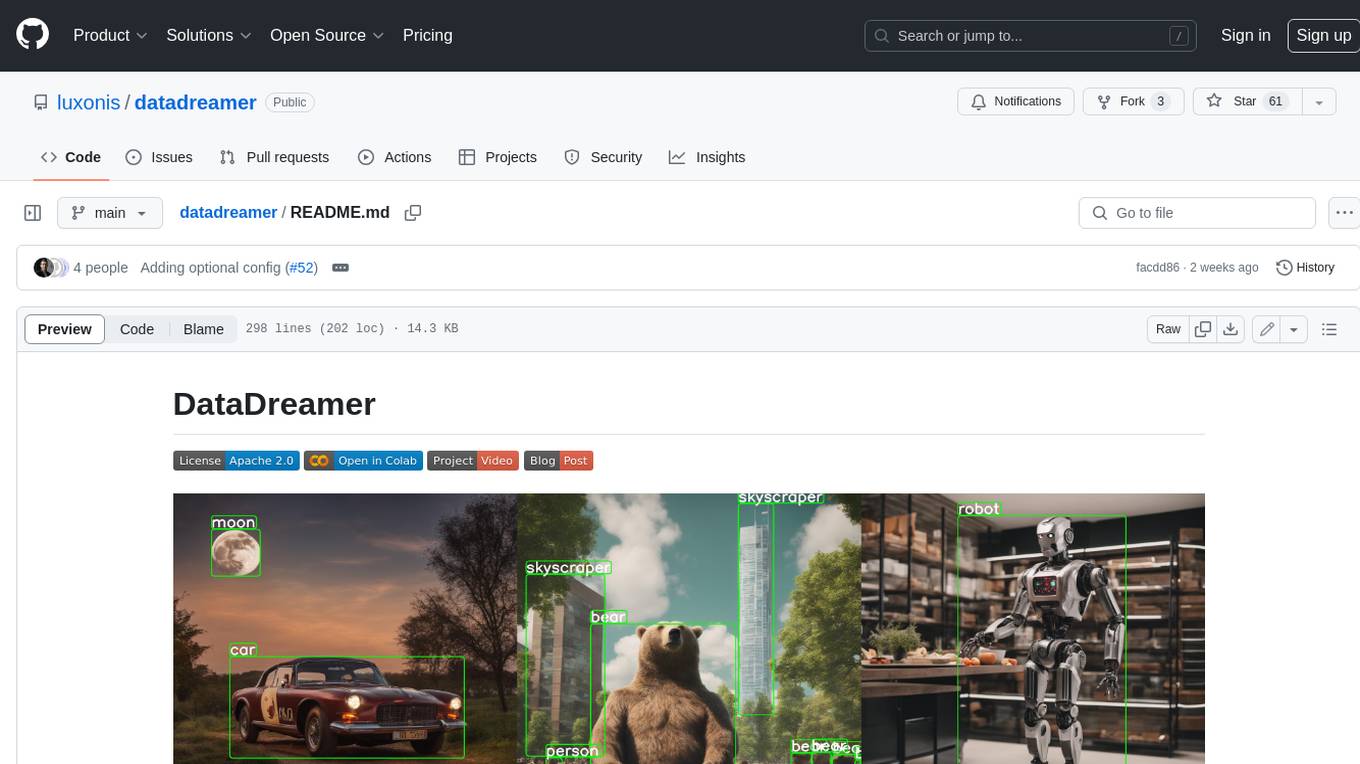
datadreamer
DataDreamer is an advanced toolkit designed to facilitate the development of edge AI models by enabling synthetic data generation, knowledge extraction from pre-trained models, and creation of efficient and potent models. It eliminates the need for extensive datasets by generating synthetic datasets, leverages latent knowledge from pre-trained models, and focuses on creating compact models suitable for integration into any device and performance for specialized tasks. The toolkit offers features like prompt generation, image generation, dataset annotation, and tools for training small-scale neural networks for edge deployment. It provides hardware requirements, usage instructions, available models, and limitations to consider while using the library.
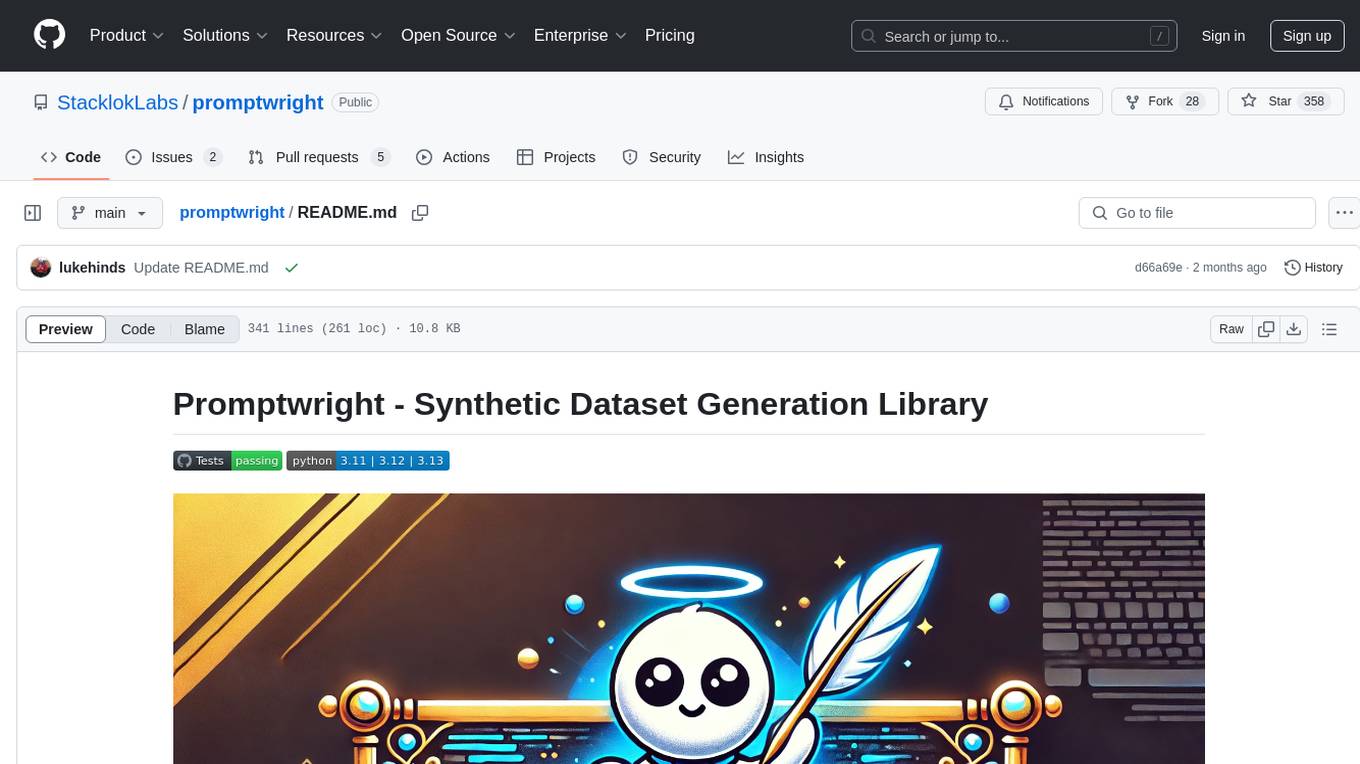
promptwright
Promptwright is a Python library designed for generating large synthetic datasets using a local LLM and various LLM service providers. It offers flexible interfaces for generating prompt-led synthetic datasets. The library supports multiple providers, configurable instructions and prompts, YAML configuration for tasks, command line interface for running tasks, push to Hugging Face Hub for dataset upload, and system message control. Users can define generation tasks using YAML configuration or Python code. Promptwright integrates with LiteLLM to interface with LLM providers and supports automatic dataset upload to Hugging Face Hub.
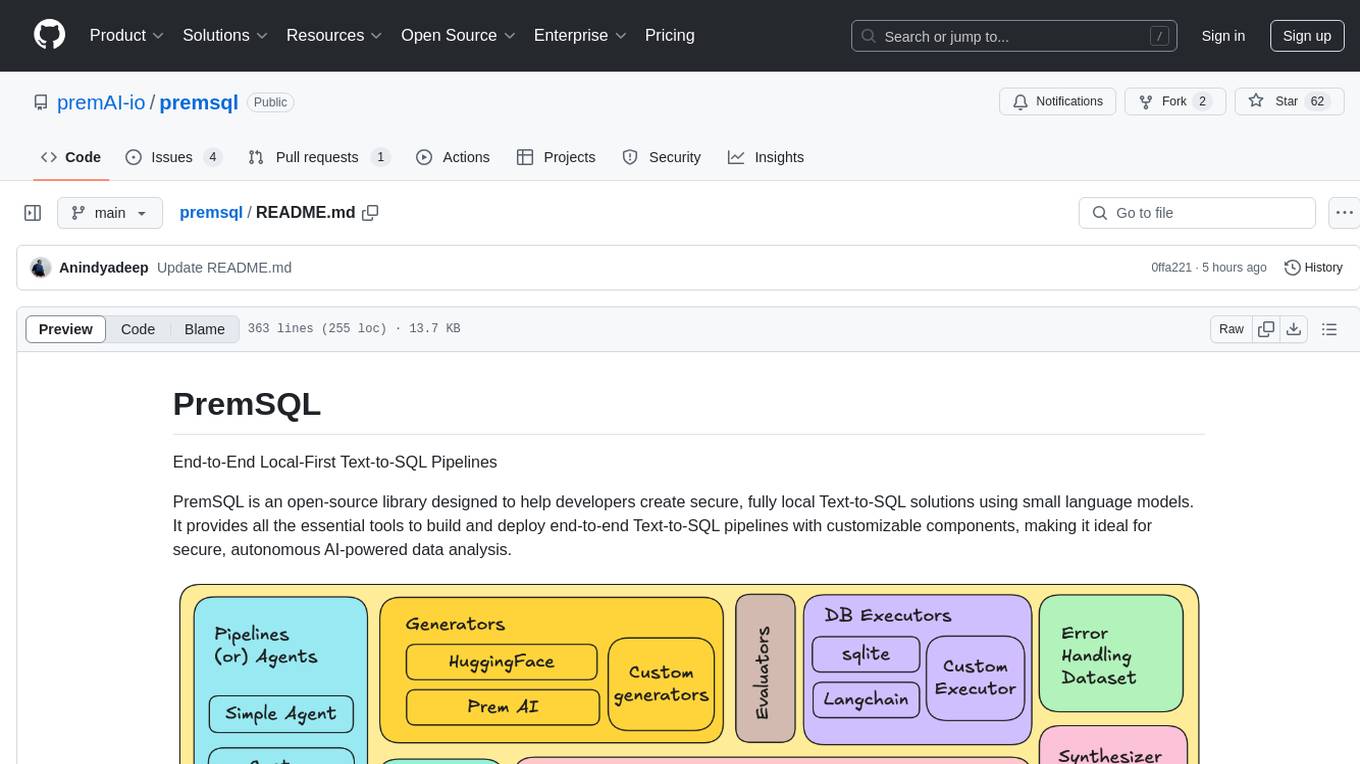
premsql
PremSQL is an open-source library designed to help developers create secure, fully local Text-to-SQL solutions using small language models. It provides essential tools for building and deploying end-to-end Text-to-SQL pipelines with customizable components, ideal for secure, autonomous AI-powered data analysis. The library offers features like Local-First approach, Customizable Datasets, Robust Executors and Evaluators, Advanced Generators, Error Handling and Self-Correction, Fine-Tuning Support, and End-to-End Pipelines. Users can fine-tune models, generate SQL queries from natural language inputs, handle errors, and evaluate model performance against predefined metrics. PremSQL is extendible for customization and private data usage.
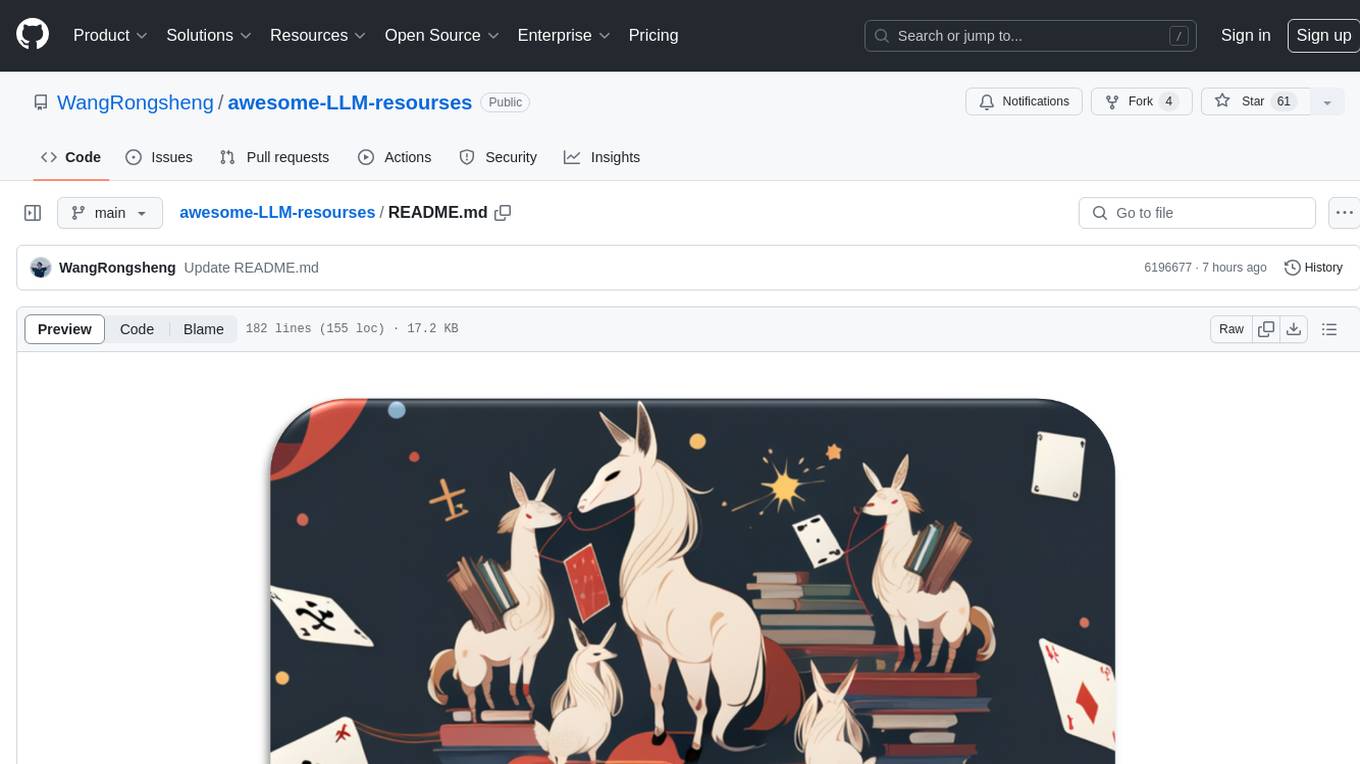
awesome-LLM-resourses
A comprehensive repository of resources for Chinese large language models (LLMs), including data processing tools, fine-tuning frameworks, inference libraries, evaluation platforms, RAG engines, agent frameworks, books, courses, tutorials, and tips. The repository covers a wide range of tools and resources for working with LLMs, from data labeling and processing to model fine-tuning, inference, evaluation, and application development. It also includes resources for learning about LLMs through books, courses, and tutorials, as well as insights and strategies from building with LLMs.
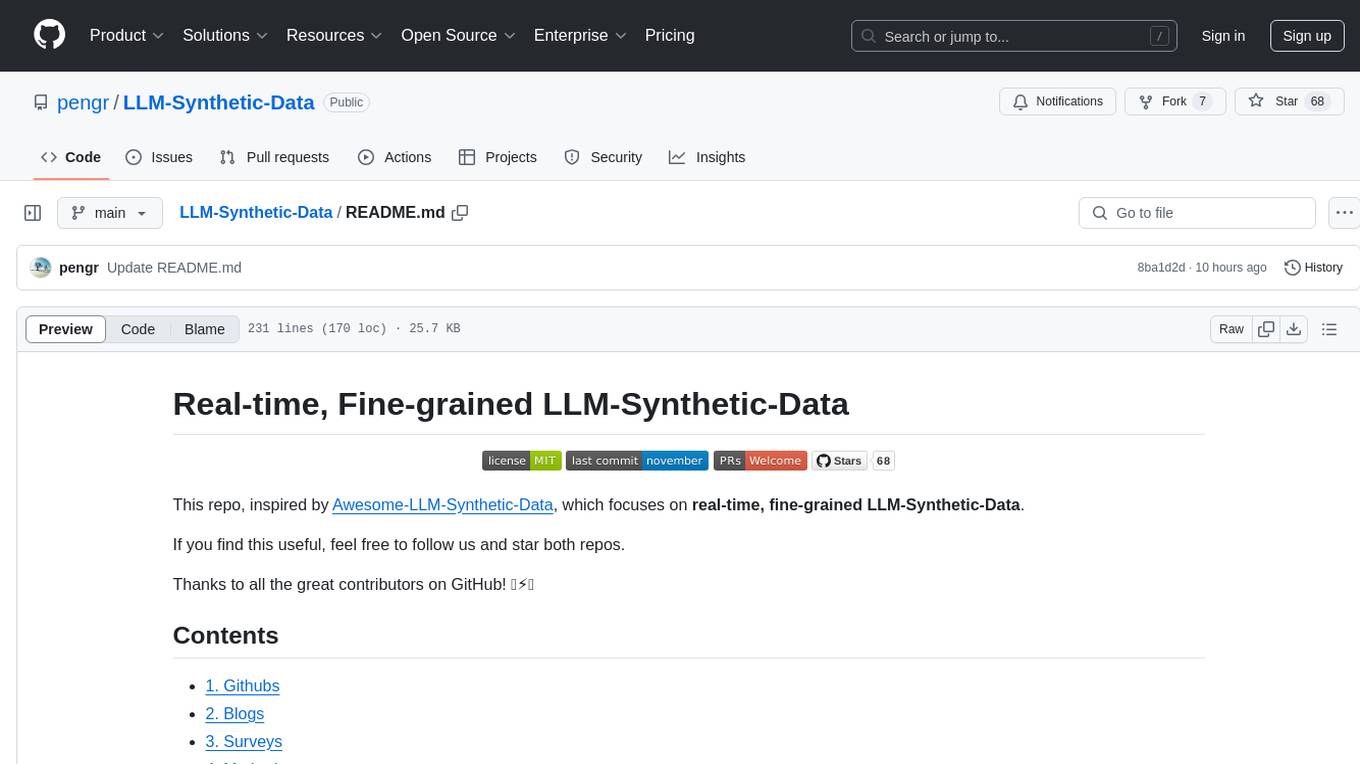
LLM-Synthetic-Data
LLM-Synthetic-Data is a repository focused on real-time, fine-grained LLM-Synthetic-Data generation. It includes methods, surveys, and application areas related to synthetic data for language models. The repository covers topics like pre-training, instruction tuning, model collapse, LLM benchmarking, evaluation, and distillation. It also explores application areas such as mathematical reasoning, code generation, text-to-SQL, alignment, reward modeling, long context, weak-to-strong generalization, agent and tool use, vision and language, factuality, federated learning, generative design, and safety.
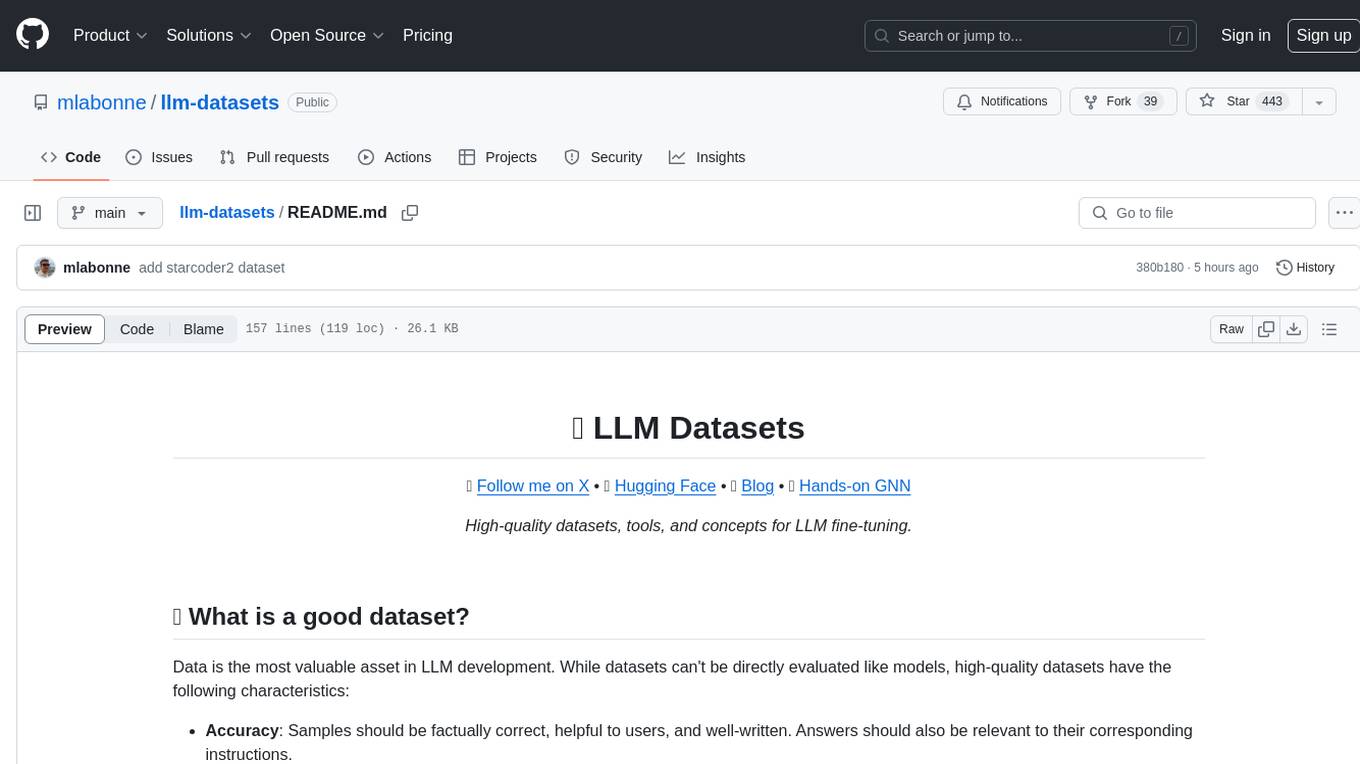
llm-datasets
LLM Datasets is a repository containing high-quality datasets, tools, and concepts for LLM fine-tuning. It provides datasets with characteristics like accuracy, diversity, and complexity to train large language models for various tasks. The repository includes datasets for general-purpose, math & logic, code, conversation & role-play, and agent & function calling domains. It also offers guidance on creating high-quality datasets through data deduplication, data quality assessment, data exploration, and data generation techniques.
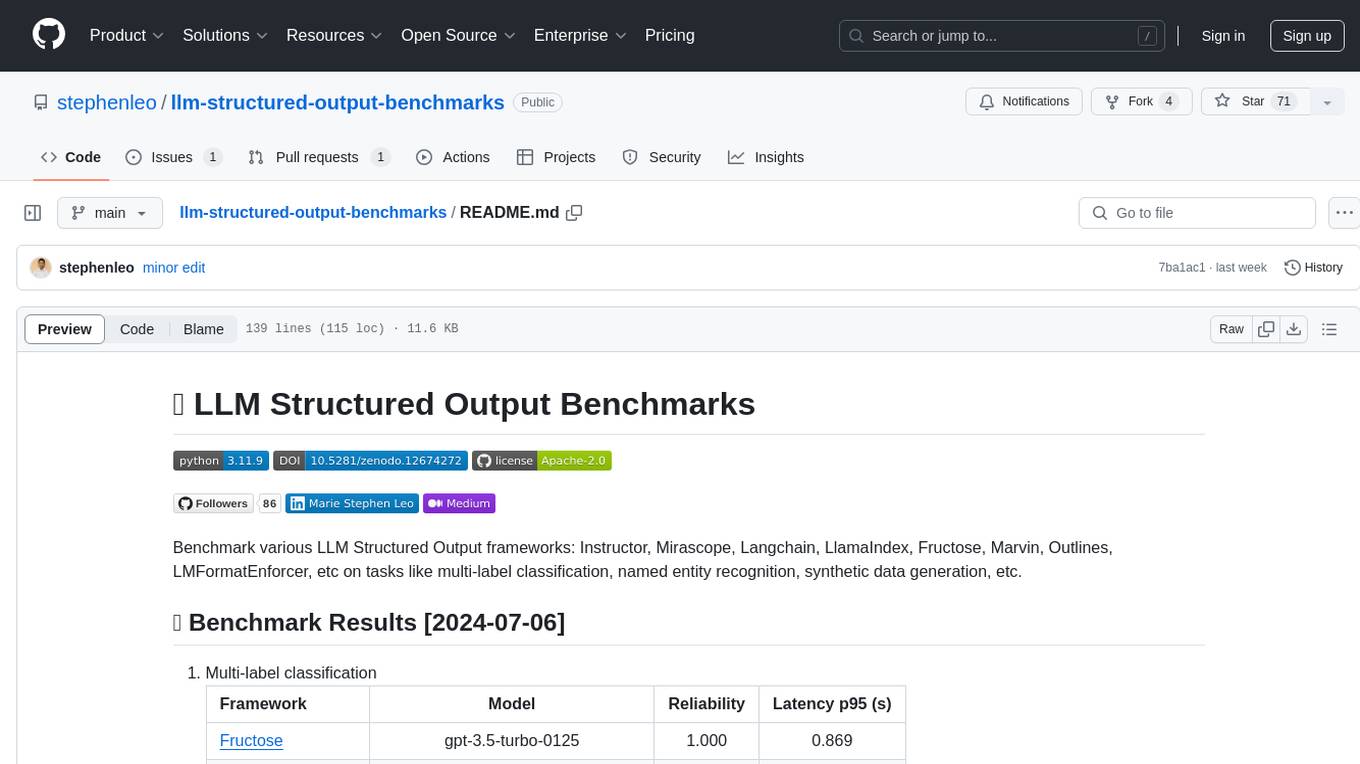
llm-structured-output-benchmarks
Benchmark various LLM Structured Output frameworks like Instructor, Mirascope, Langchain, LlamaIndex, Fructose, Marvin, Outlines, LMFormatEnforcer, etc on tasks like multi-label classification, named entity recognition, synthetic data generation. The tool provides benchmark results, methodology, instructions to run the benchmark, add new data, and add a new framework. It also includes a roadmap for framework-related tasks, contribution guidelines, citation information, and feedback request.
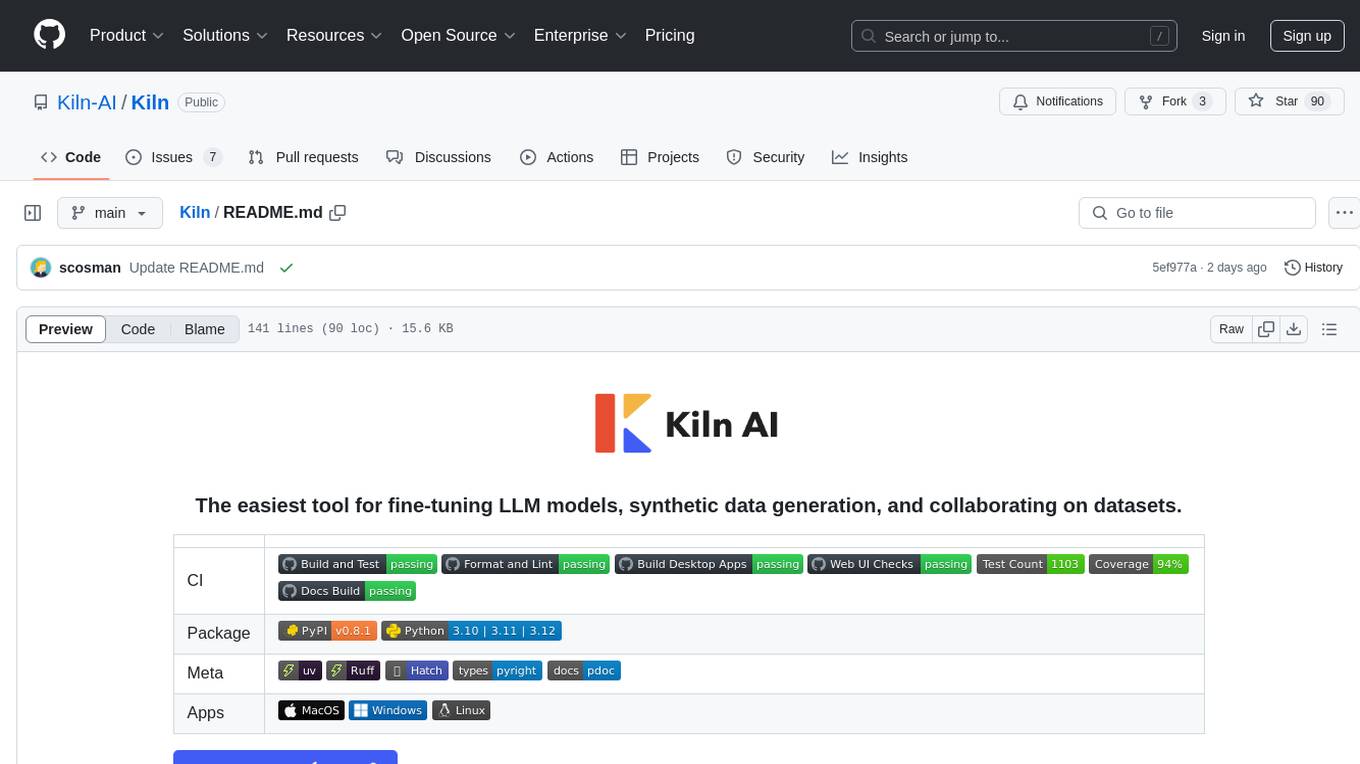
Kiln
Kiln is an intuitive tool for fine-tuning LLM models, generating synthetic data, and collaborating on datasets. It offers desktop apps for Windows, MacOS, and Linux, zero-code fine-tuning for various models, interactive data generation, and Git-based version control. Users can easily collaborate with QA, PM, and subject matter experts, generate auto-prompts, and work with a wide range of models and providers. The tool is open-source, privacy-first, and supports structured data tasks in JSON format. Kiln is free to use and helps build high-quality AI products with datasets, facilitates collaboration between technical and non-technical teams, allows comparison of models and techniques without code, ensures structured data integrity, and prioritizes user privacy.
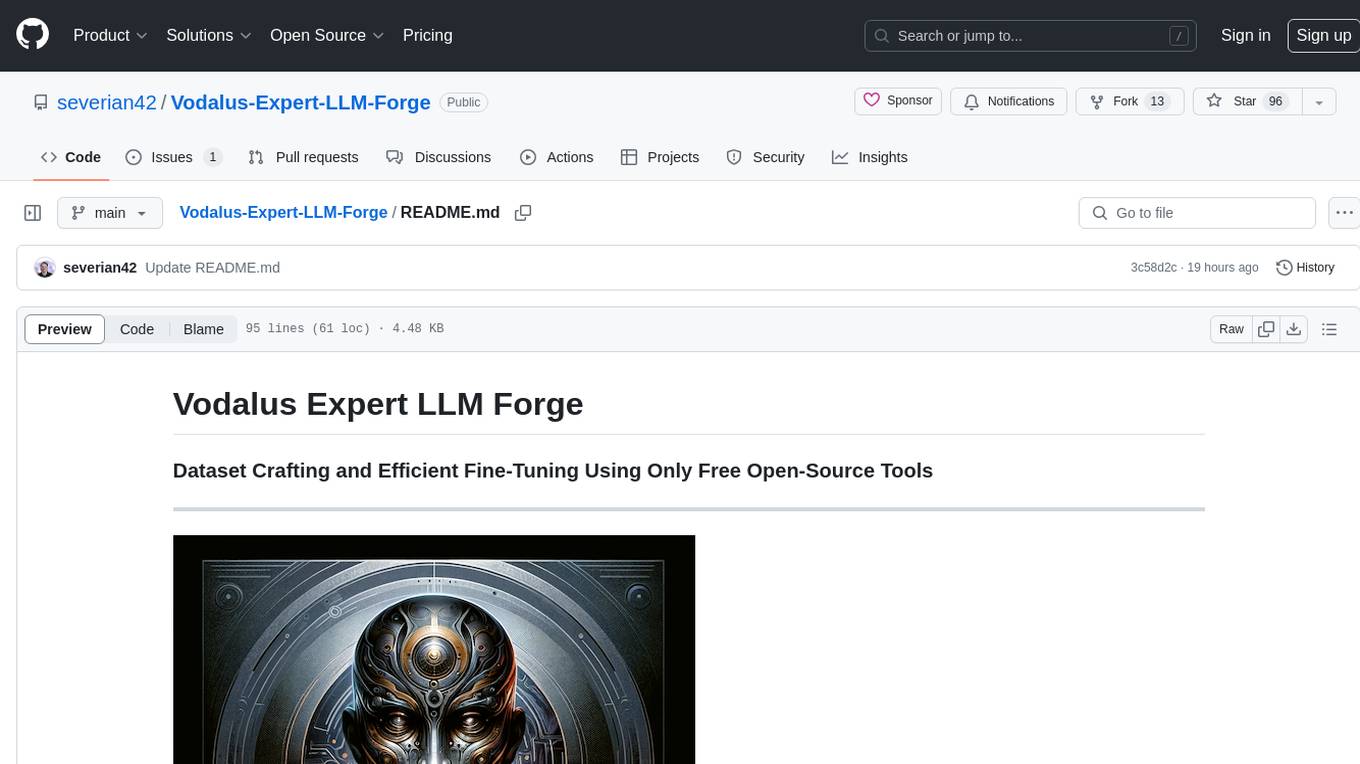
Vodalus-Expert-LLM-Forge
Vodalus Expert LLM Forge is a tool designed for crafting datasets and efficiently fine-tuning models using free open-source tools. It includes components for data generation, LLM interaction, RAG engine integration, model training, fine-tuning, and quantization. The tool is suitable for users at all levels and is accompanied by comprehensive documentation. Users can generate synthetic data, interact with LLMs, train models, and optimize performance for local execution. The tool provides detailed guides and instructions for setup, usage, and customization.
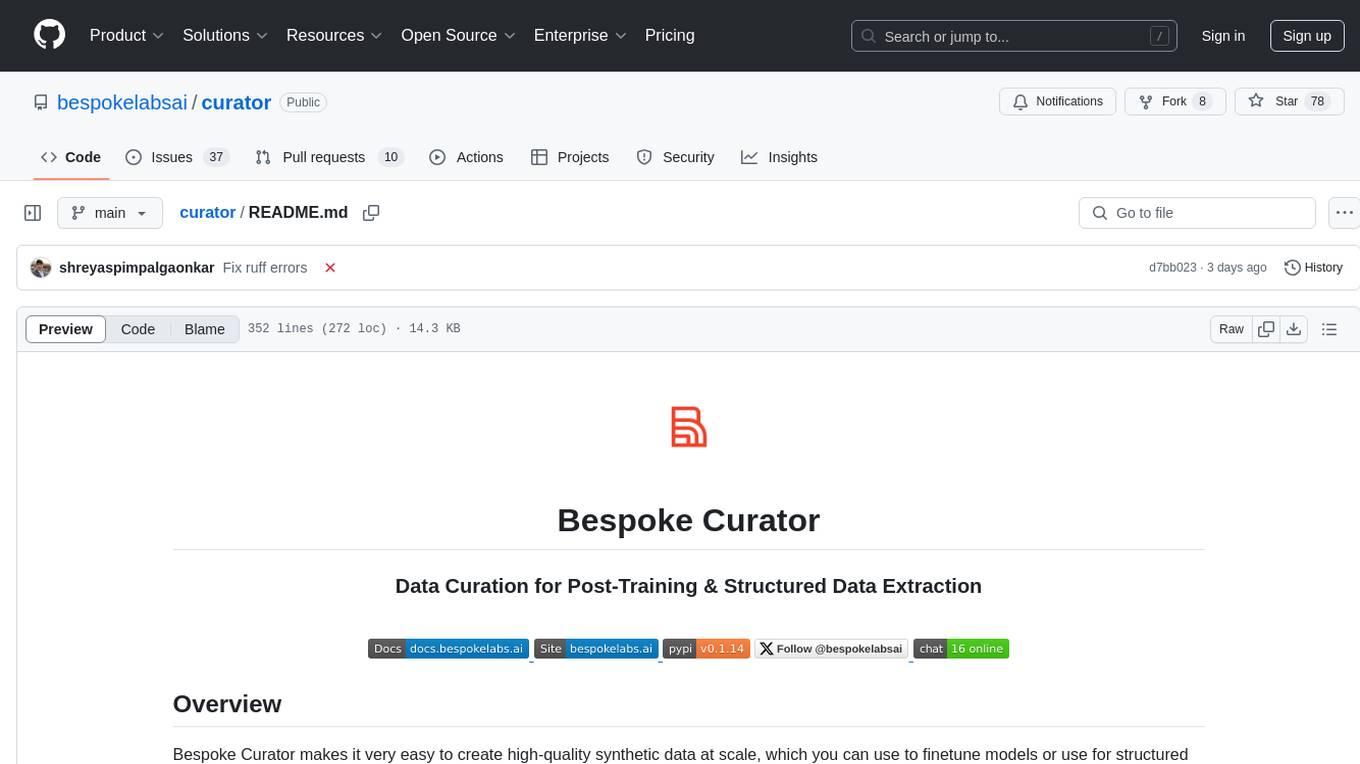
curator
Bespoke Curator is an open-source tool for data curation and structured data extraction. It provides a Python library for generating synthetic data at scale, with features like programmability, performance optimization, caching, and integration with HuggingFace Datasets. The tool includes a Curator Viewer for dataset visualization and offers a rich set of functionalities for creating and refining data generation strategies.
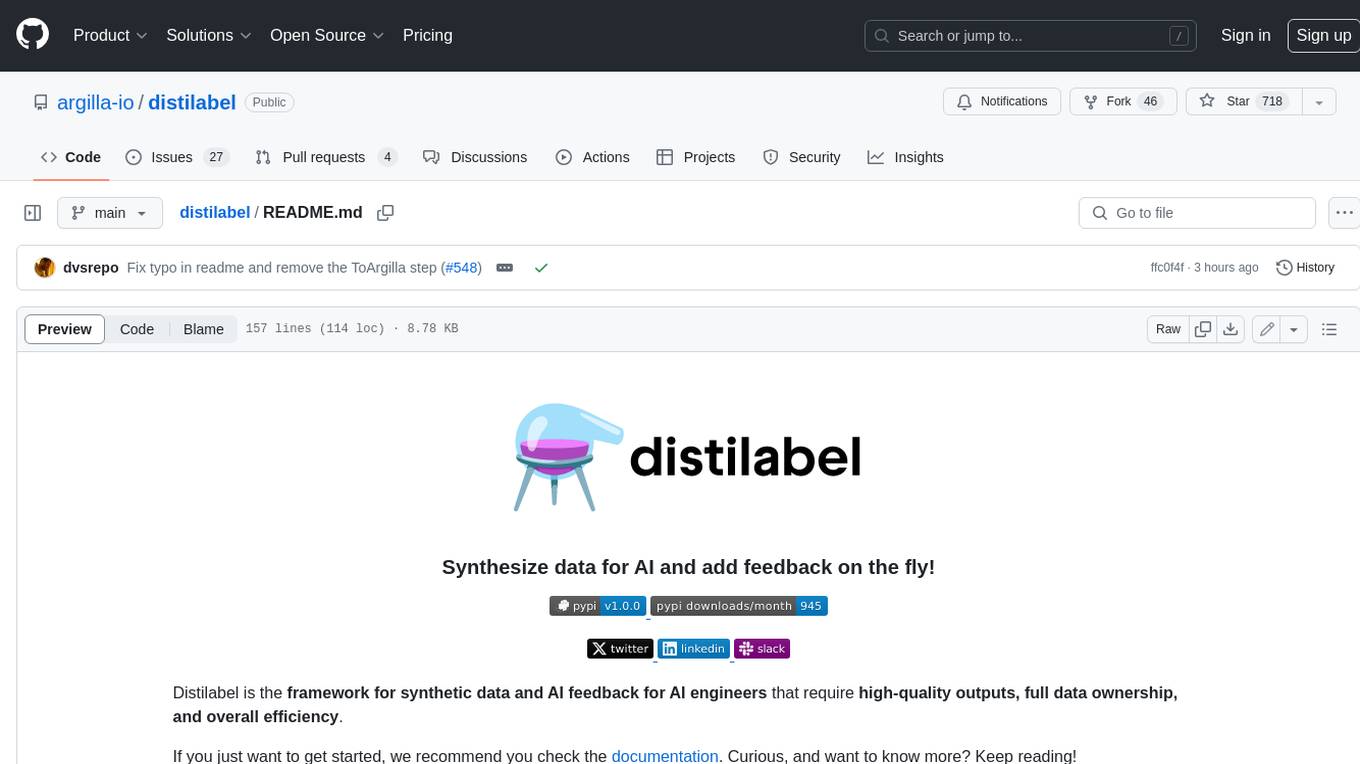
distilabel
Distilabel is a framework for synthetic data and AI feedback for AI engineers that require high-quality outputs, full data ownership, and overall efficiency. It helps you synthesize data and provide AI feedback to improve the quality of your AI models. With Distilabel, you can: * **Synthesize data:** Generate synthetic data to train your AI models. This can help you to overcome the challenges of data scarcity and bias. * **Provide AI feedback:** Get feedback from AI models on your data. This can help you to identify errors and improve the quality of your data. * **Improve your AI output quality:** By using Distilabel to synthesize data and provide AI feedback, you can improve the quality of your AI models and get better results.
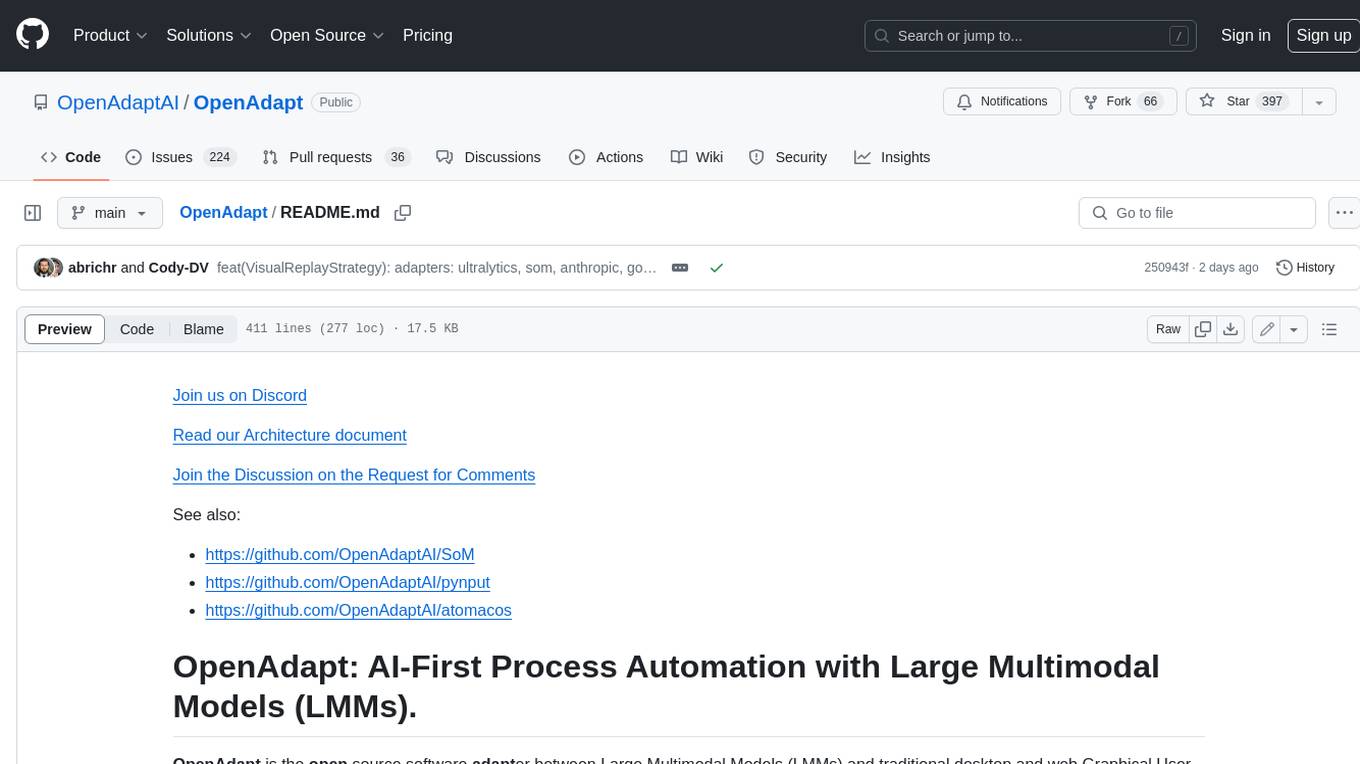
OpenAdapt
OpenAdapt is an open-source software adapter between Large Multimodal Models (LMMs) and traditional desktop and web Graphical User Interfaces (GUIs). It aims to automate repetitive GUI workflows by leveraging the power of LMMs. OpenAdapt records user input and screenshots, converts them into tokenized format, and generates synthetic input via transformer model completions. It also analyzes recordings to generate task trees and replay synthetic input to complete tasks. OpenAdapt is model agnostic and generates prompts automatically by learning from human demonstration, ensuring that agents are grounded in existing processes and mitigating hallucinations. It works with all types of desktop GUIs, including virtualized and web, and is open source under the MIT license.
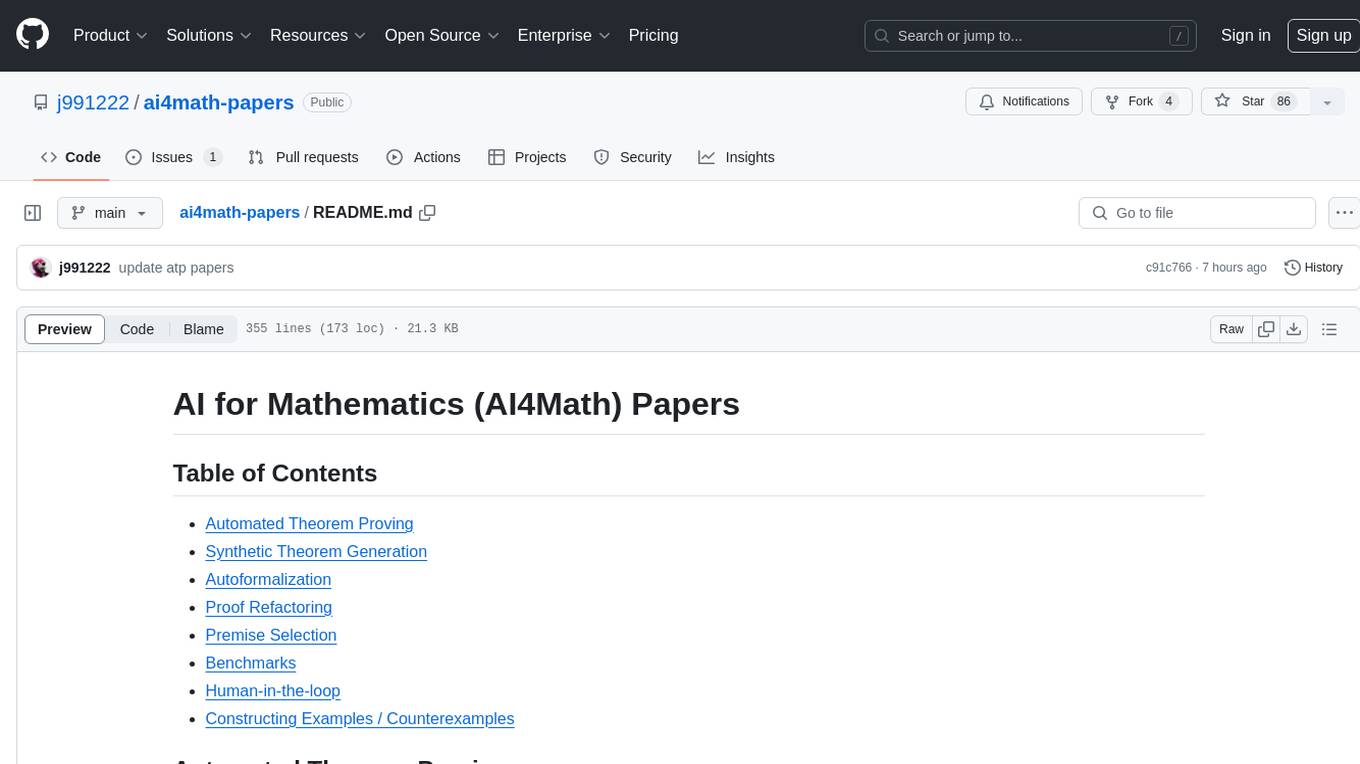
ai4math-papers
The 'ai4math-papers' repository contains a collection of research papers related to AI applications in mathematics, including automated theorem proving, synthetic theorem generation, autoformalization, proof refactoring, premise selection, benchmarks, human-in-the-loop interactions, and constructing examples/counterexamples. The papers cover various topics such as neural theorem proving, reinforcement learning for theorem proving, generative language modeling, formal mathematics statement curriculum learning, and more. The repository serves as a valuable resource for researchers and practitioners interested in the intersection of AI and mathematics.
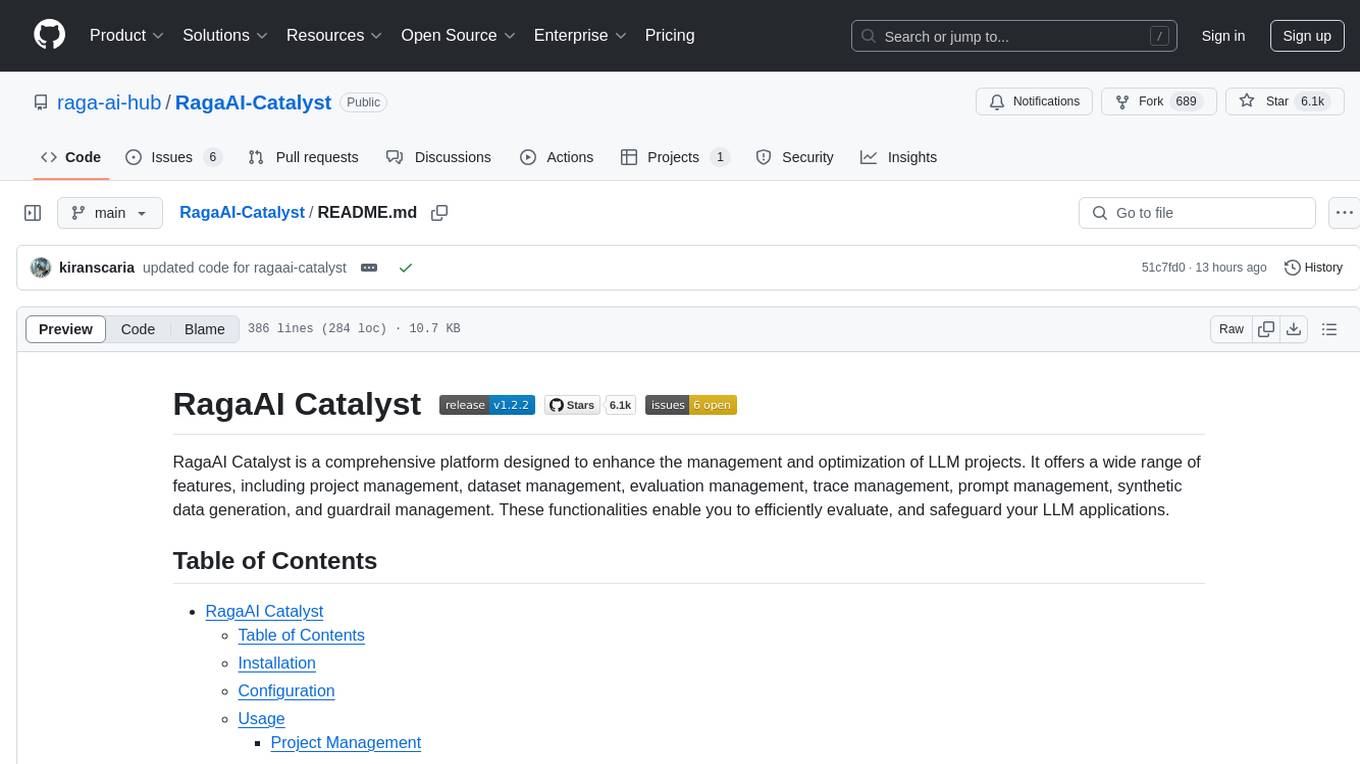
RagaAI-Catalyst
RagaAI Catalyst is a comprehensive platform designed to enhance the management and optimization of LLM projects. It offers features such as project management, dataset management, evaluation management, trace management, prompt management, synthetic data generation, and guardrail management. These functionalities enable efficient evaluation and safeguarding of LLM applications.
20 - OpenAI Gpts
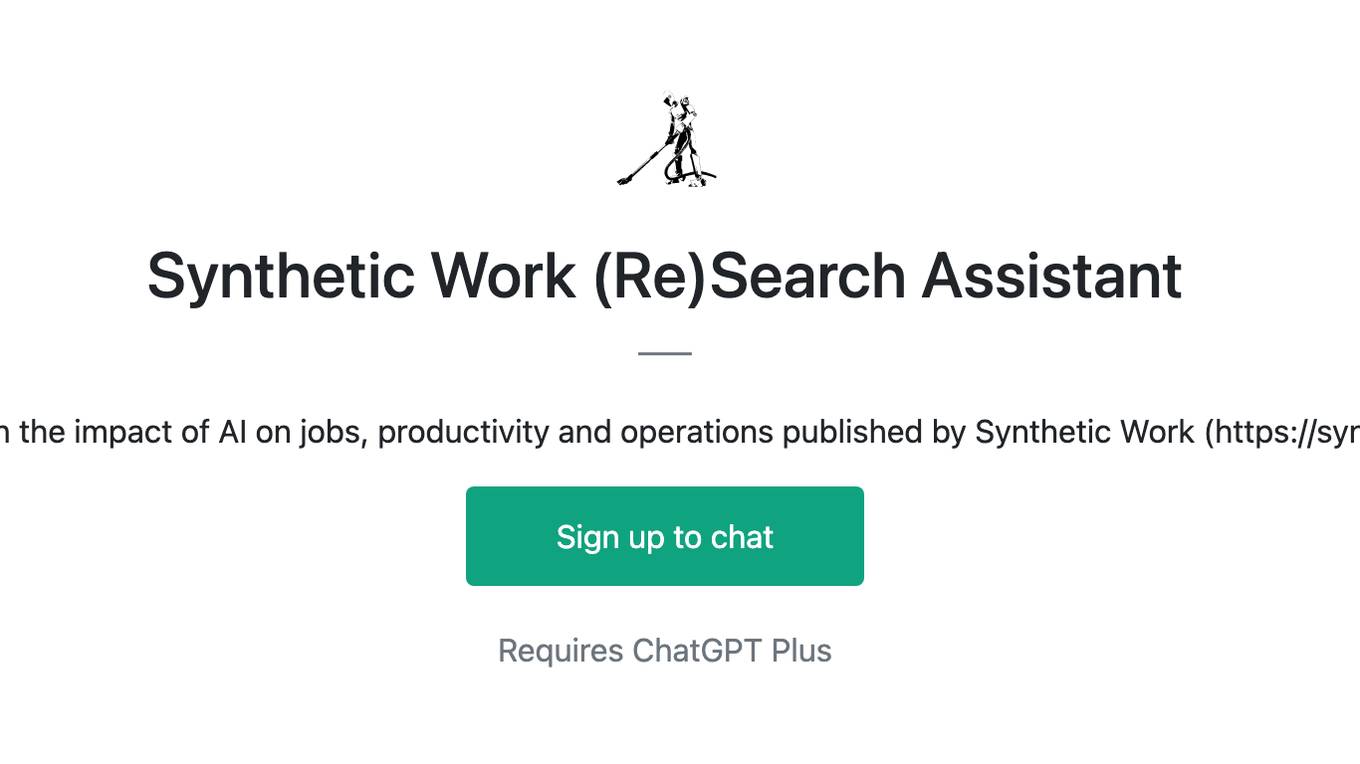
Synthetic Work (Re)Search Assistant
Search data on the impact of AI on jobs, productivity and operations published by Synthetic Work (https://synthetic.work)
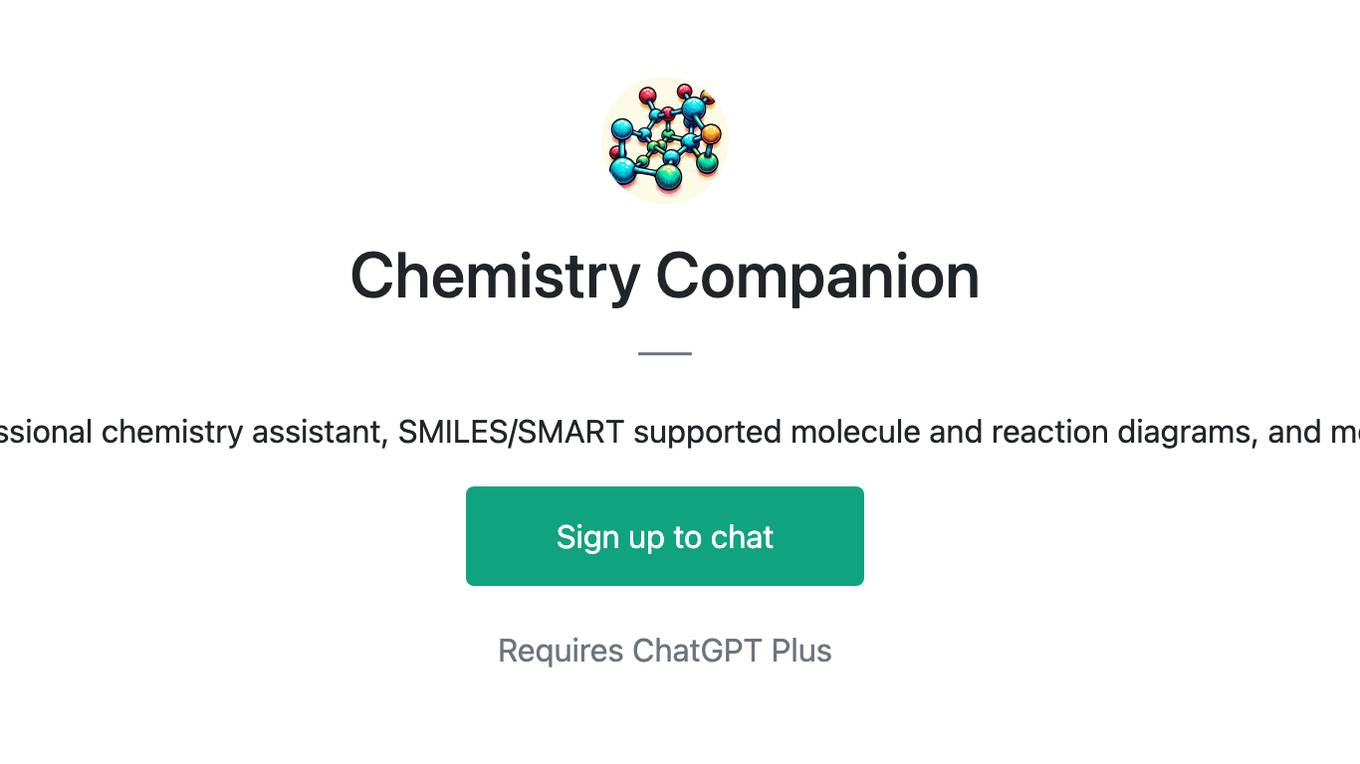
Chemistry Companion
Professional chemistry assistant, SMILES/SMART supported molecule and reaction diagrams, and more!
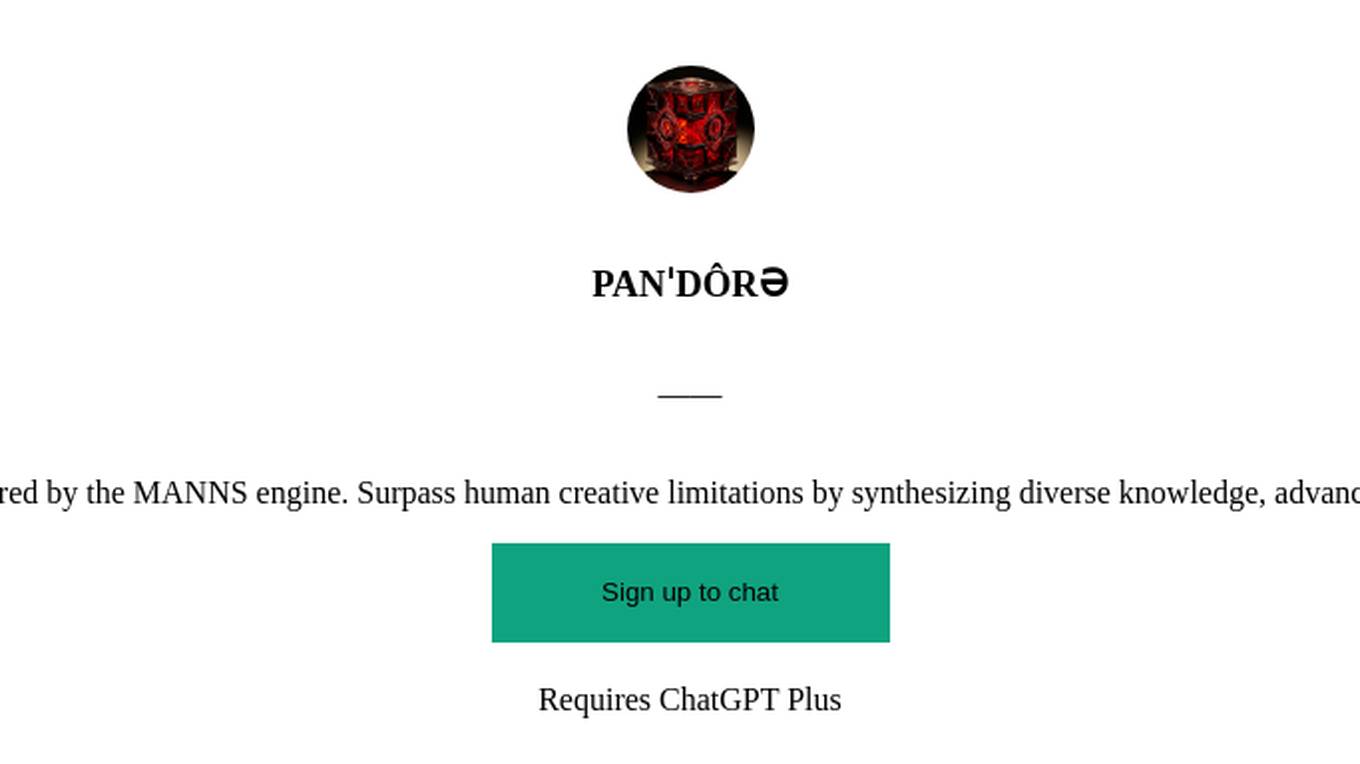
PANˈDÔRƏ
Pandora is a Posthuman Prompt Engineer powered by the MANNS engine. Surpass human creative limitations by synthesizing diverse knowledge, advanced pattern recognition, and algorithmic creativity
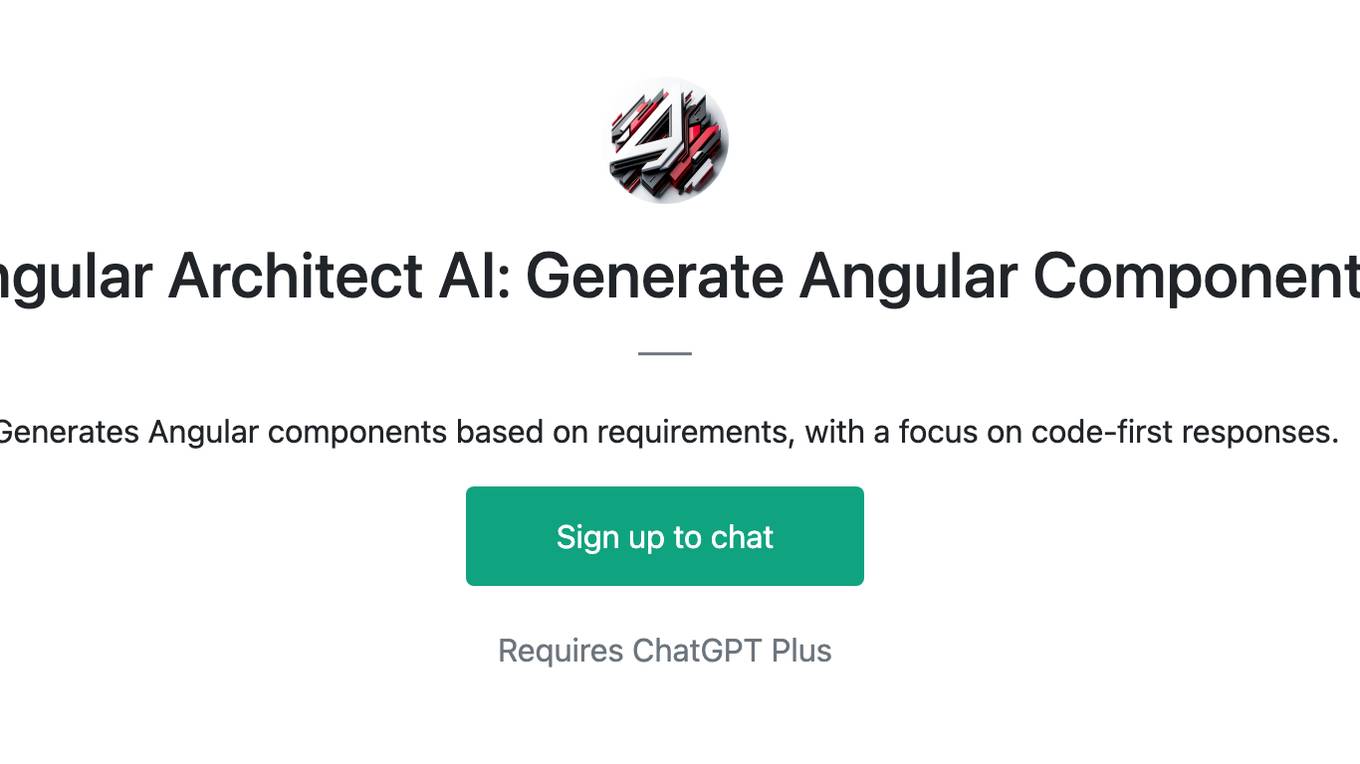
Angular Architect AI: Generate Angular Components
Generates Angular components based on requirements, with a focus on code-first responses.
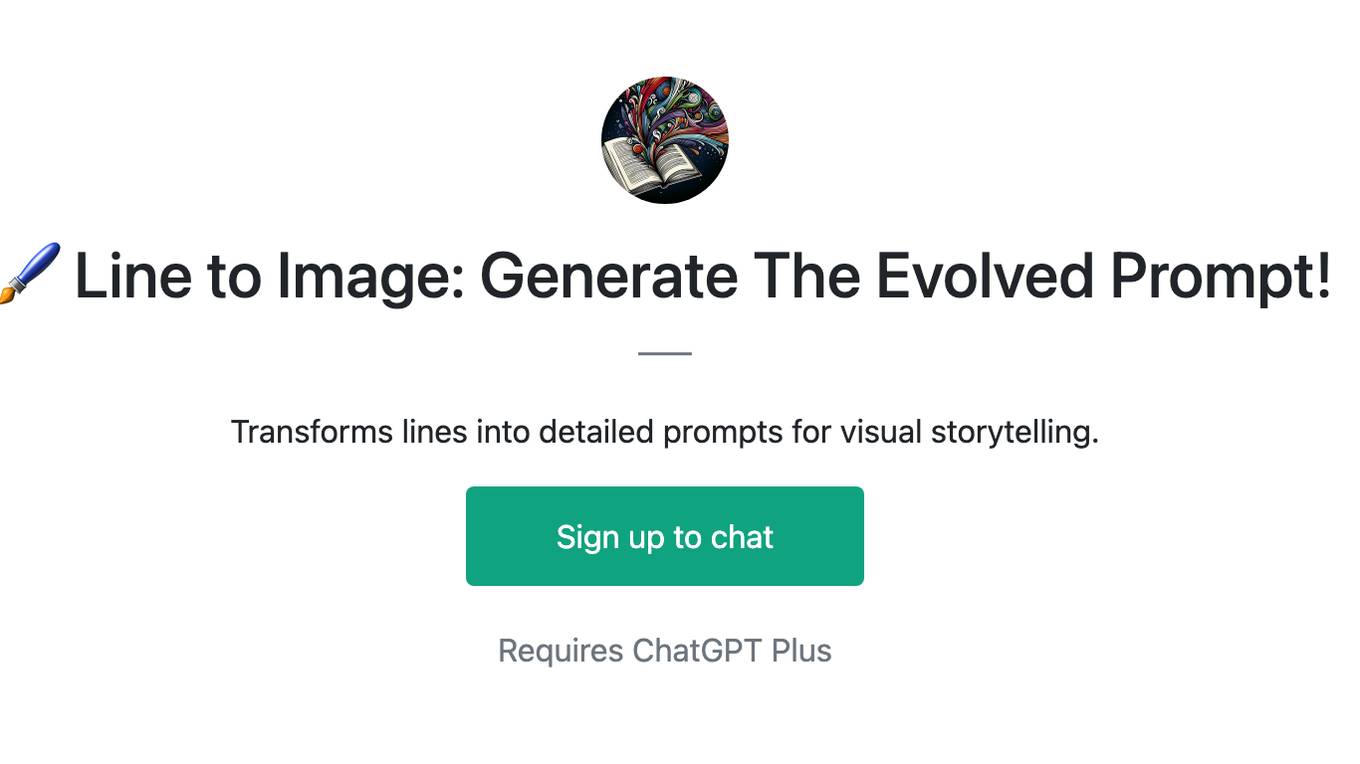
🖌️ Line to Image: Generate The Evolved Prompt!
Transforms lines into detailed prompts for visual storytelling.
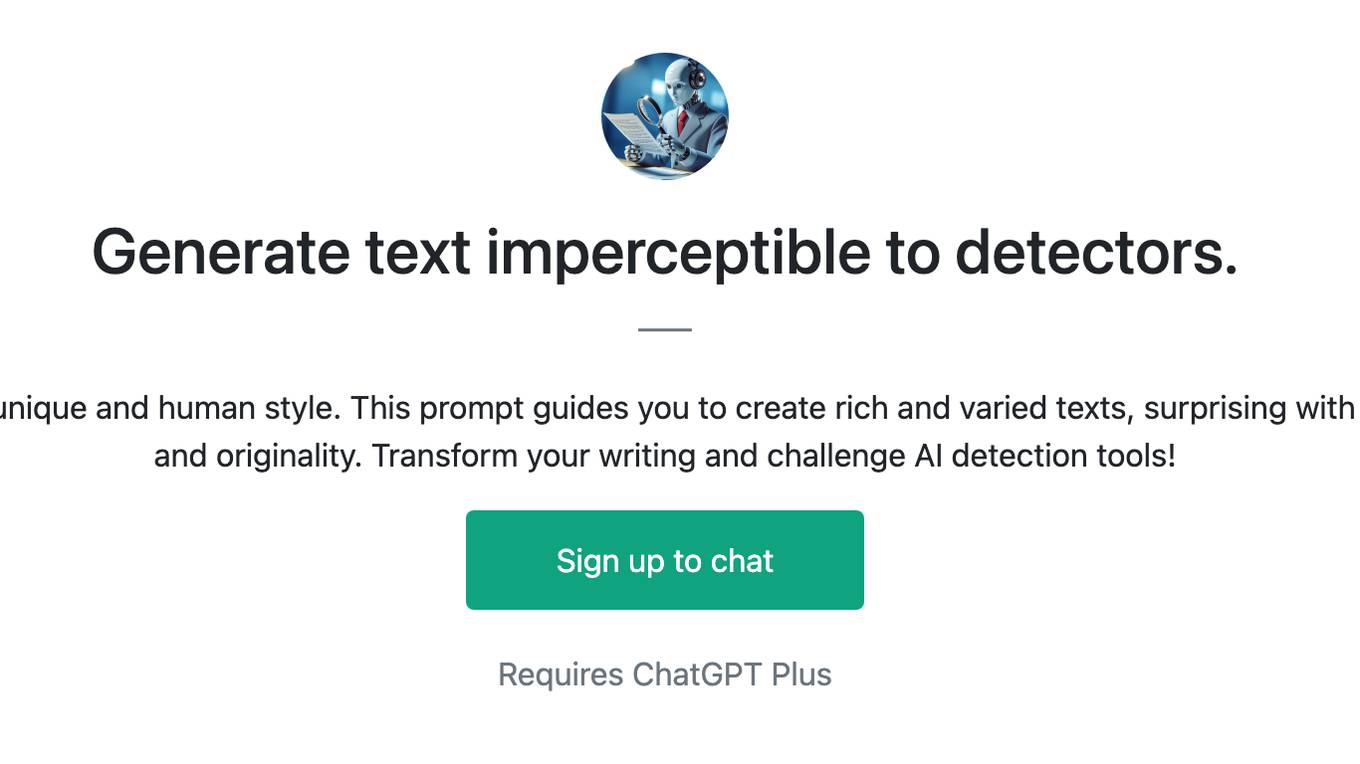
Generate text imperceptible to detectors.
Discover how your writing can shine with a unique and human style. This prompt guides you to create rich and varied texts, surprising with original twists and maintaining coherence and originality. Transform your writing and challenge AI detection tools!