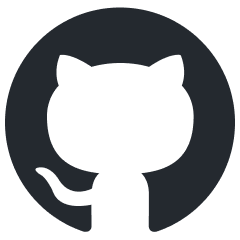
financial-datasets
Financial datasets for LLMs 🧪
Stars: 166
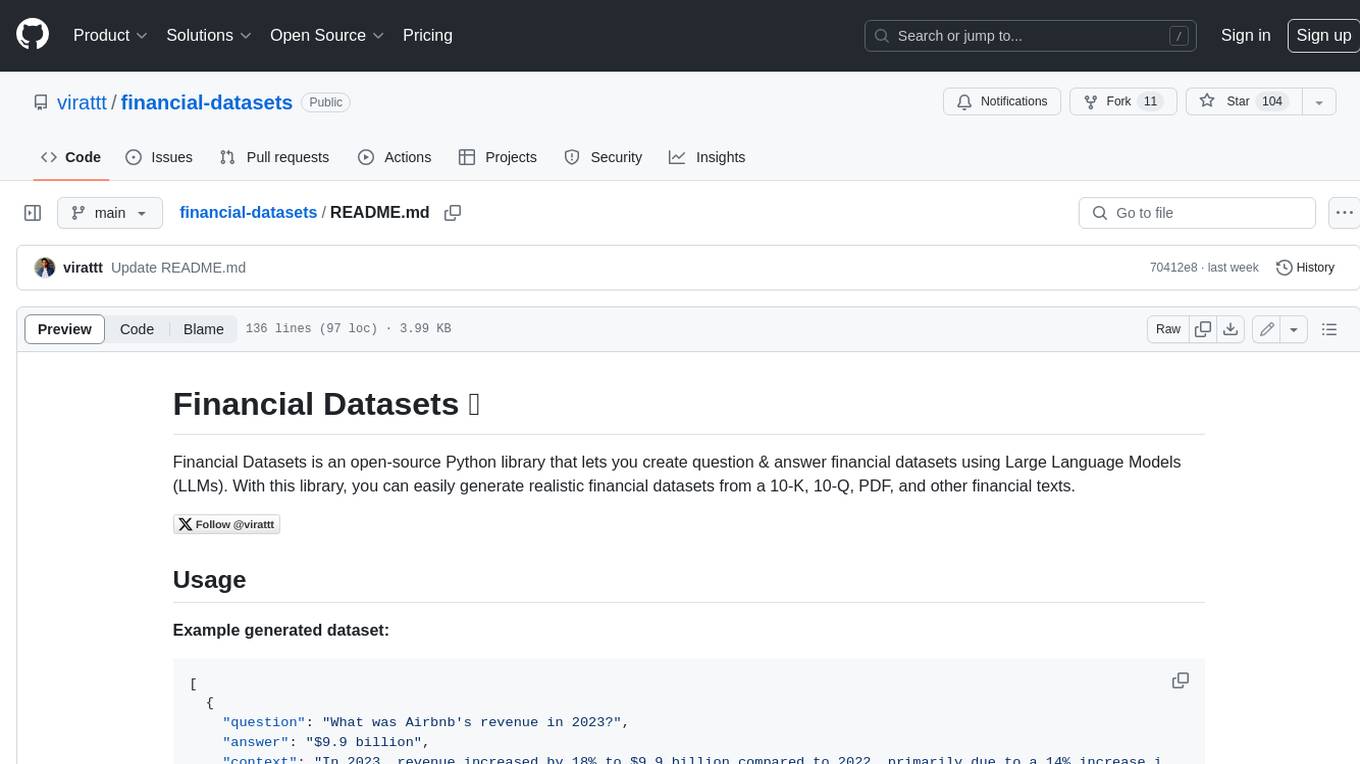
Financial Datasets is an open-source Python library that allows users to create question and answer financial datasets using Large Language Models (LLMs). With this library, users can easily generate realistic financial datasets from 10-K, 10-Q, PDF, and other financial texts. The library provides three main methods for generating datasets: from any text, from a 10-K filing, or from a PDF URL. Financial Datasets can be used for a variety of tasks, including financial analysis, research, and education.
README:
Financial Datasets is an open-source Python library that lets you create question & answer financial datasets using Large Language Models (LLMs). With this library, you can easily generate realistic financial datasets from a 10-K, 10-Q, PDF, and other financial texts.
Example generated dataset:
[
{
"question": "What was Airbnb's revenue in 2023?",
"answer": "$9.9 billion",
"context": "In 2023, revenue increased by 18% to $9.9 billion compared to 2022, primarily due to a 14% increase in Nights and Experiences Booked of 54.5 million combined with higher average daily rates driving a 16% increase in Gross Booking Value of $10.0 billion."
},
{
"question": "By what percentage did Airbnb's net income increase in 2023 compared to the prior year?",
"answer": "153%",
"context": "Net income in 2023 increased by 153% to $4.8 billion, compared to the prior year, driven by our revenue growth, increased interest income, discipline in managing our cost structure, and the release of a portion of our valuation allowance on deferred tax assets of $2.9 billion."
}
]
Example #1 - generate from any text
Most flexible option. Generates dataset using a list of string texts
. Colab code
example here.
from financial_datasets.generator import DatasetGenerator
# Your list of texts
texts = ...
# Create dataset generator
generator = DatasetGenerator(model="gpt-4-turbo", api_key="your-openai-key")
# Generate dataset from texts
dataset = generator.generate_from_texts(
texts=texts,
max_questions=100,
)
Example #2 - generate from PDF
Generate a dataset using a PDF url
only. Colab code
example here.
from financial_datasets.generator import DatasetGenerator
# Create dataset generator
generator = DatasetGenerator(model="gpt-4-turbo", api_key="your-openai-key")
# Generate dataset from PDF url
dataset = generator.generate_from_pdf(
url="https://www.berkshirehathaway.com/letters/2023ltr.pdf",
max_questions=100,
)
Example #3 - generate from 10-K
Generate a dataset using a ticker
and year
. Colab code
example here.
from financial_datasets.generator import DatasetGenerator
# Create dataset generator
generator = DatasetGenerator(model="gpt-4-turbo", api_key="your-openai-key")
# Generate dataset from 10-K
dataset = generator.generate_from_10K(
ticker="AAPL",
year=2023,
max_questions=100,
item_names=["Item 1", "Item 7"], # optional - specify Item names to use
)
You can install the Financial Datasets library using pip:
pip install financial-datasets
If you prefer to use Poetry for dependency management, you can add Financial Datasets to your project:
poetry add financial-datasets
If you want to install the library directly from the repository, follow these steps:
-
Clone the repository:
git clone https://github.com/virattt/financial-datasets.git
-
Navigate to the project directory:
cd financial-datasets
-
Install the dependencies using Poetry:
poetry install
-
You can now use the library in your Python projects.
Contributions are welcome! If you find any issues or have suggestions for improvements, please open an issue or submit a pull request.
This project is licensed under the MIT License.
For Tasks:
Click tags to check more tools for each tasksFor Jobs:
Alternative AI tools for financial-datasets
Similar Open Source Tools
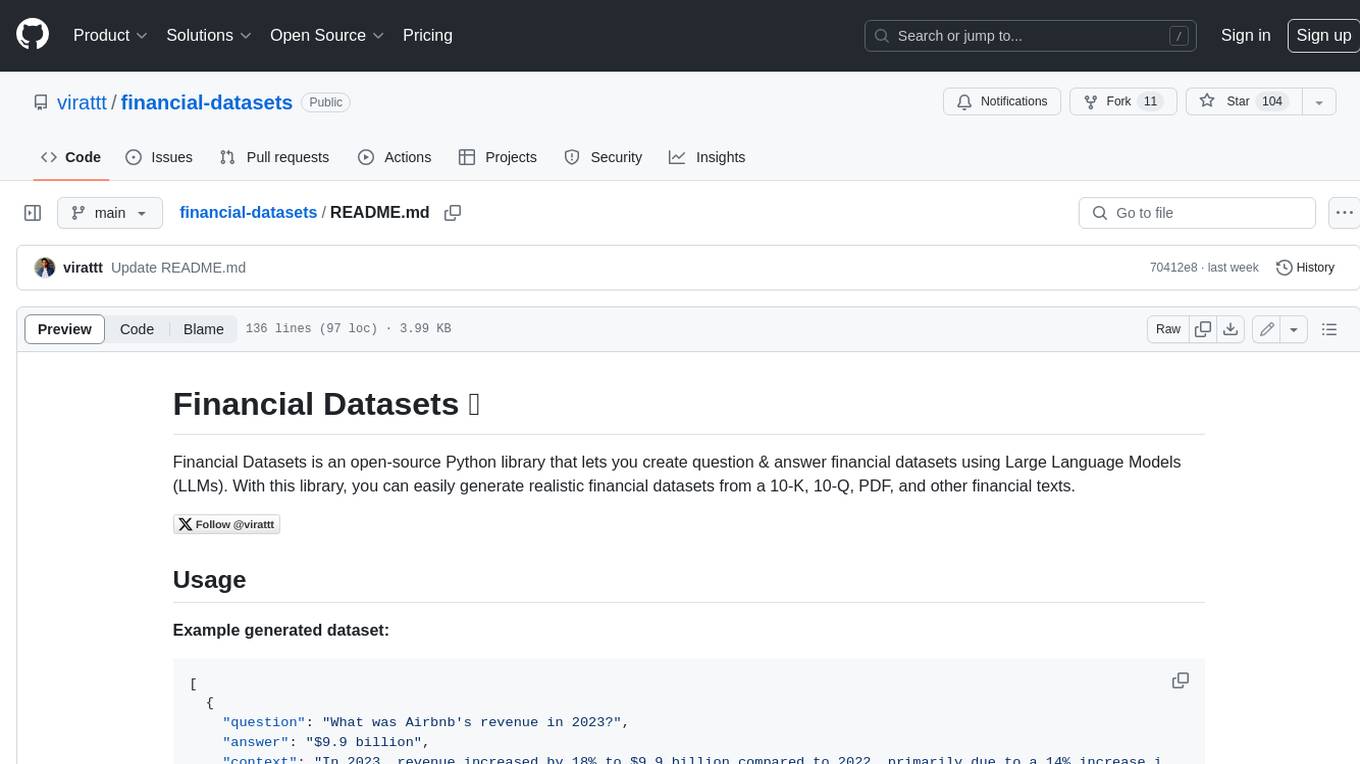
financial-datasets
Financial Datasets is an open-source Python library that allows users to create question and answer financial datasets using Large Language Models (LLMs). With this library, users can easily generate realistic financial datasets from 10-K, 10-Q, PDF, and other financial texts. The library provides three main methods for generating datasets: from any text, from a 10-K filing, or from a PDF URL. Financial Datasets can be used for a variety of tasks, including financial analysis, research, and education.
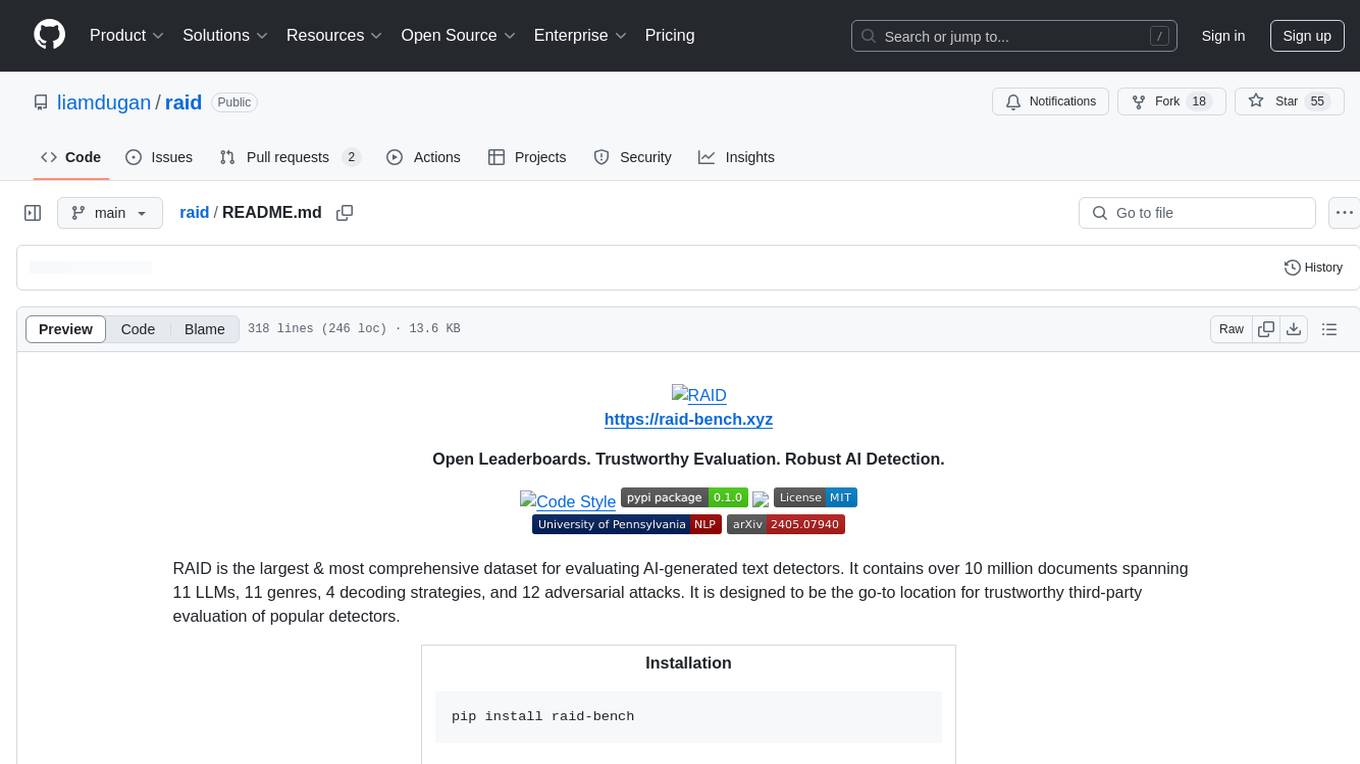
raid
RAID is the largest and most comprehensive dataset for evaluating AI-generated text detectors. It contains over 10 million documents spanning 11 LLMs, 11 genres, 4 decoding strategies, and 12 adversarial attacks. RAID is designed to be the go-to location for trustworthy third-party evaluation of popular detectors. The dataset covers diverse models, domains, sampling strategies, and attacks, making it a valuable resource for training detectors, evaluating generalization, protecting against adversaries, and comparing to state-of-the-art models from academia and industry.
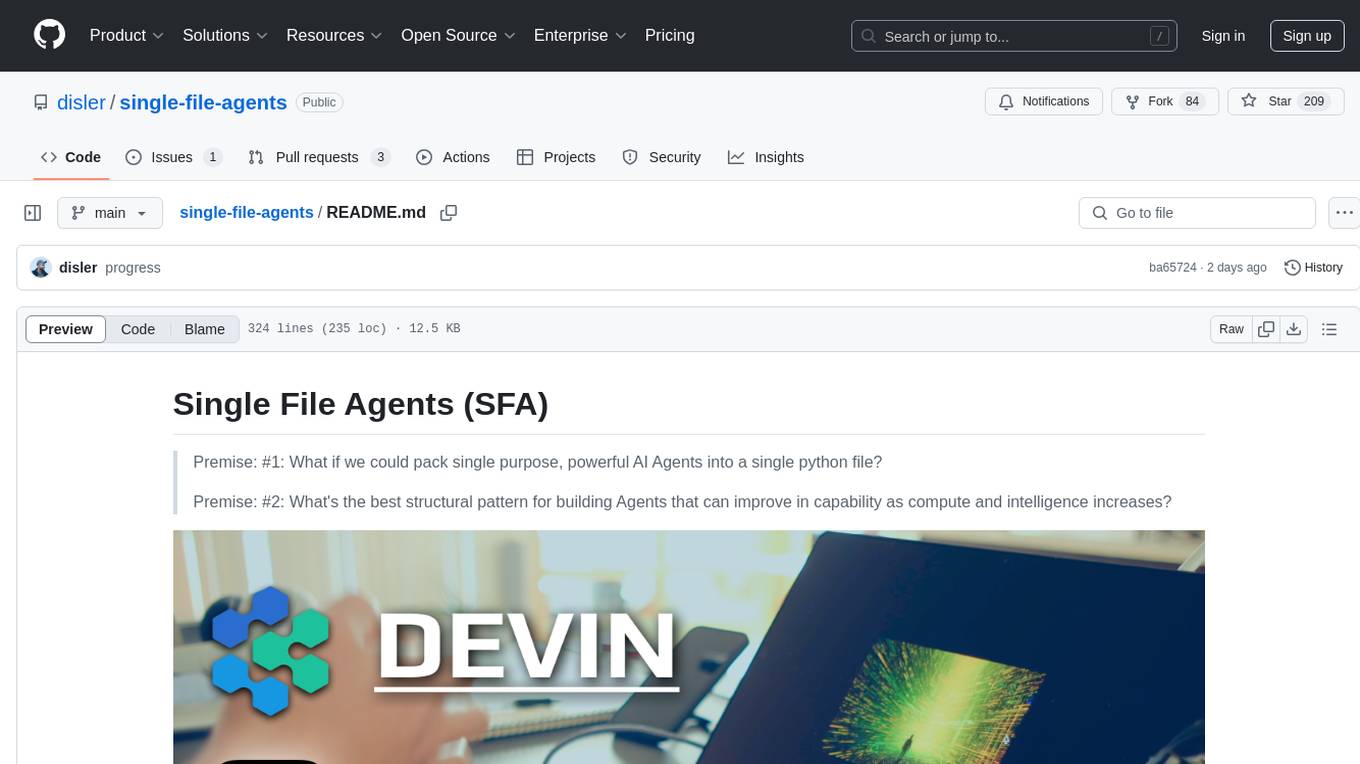
single-file-agents
Single File Agents (SFA) is a collection of powerful single-file agents built on top of uv, a modern Python package installer and resolver. These agents aim to perform specific tasks efficiently, demonstrating precise prompt engineering and GenAI patterns. The repository contains agents built across major GenAI providers like Gemini, OpenAI, and Anthropic. Each agent is self-contained, minimal, and built on modern Python for fast and reliable dependency management. Users can run these scripts from their server or directly from a gist. The agents are patternful, emphasizing the importance of setting up effective prompts, tools, and processes for reusability.
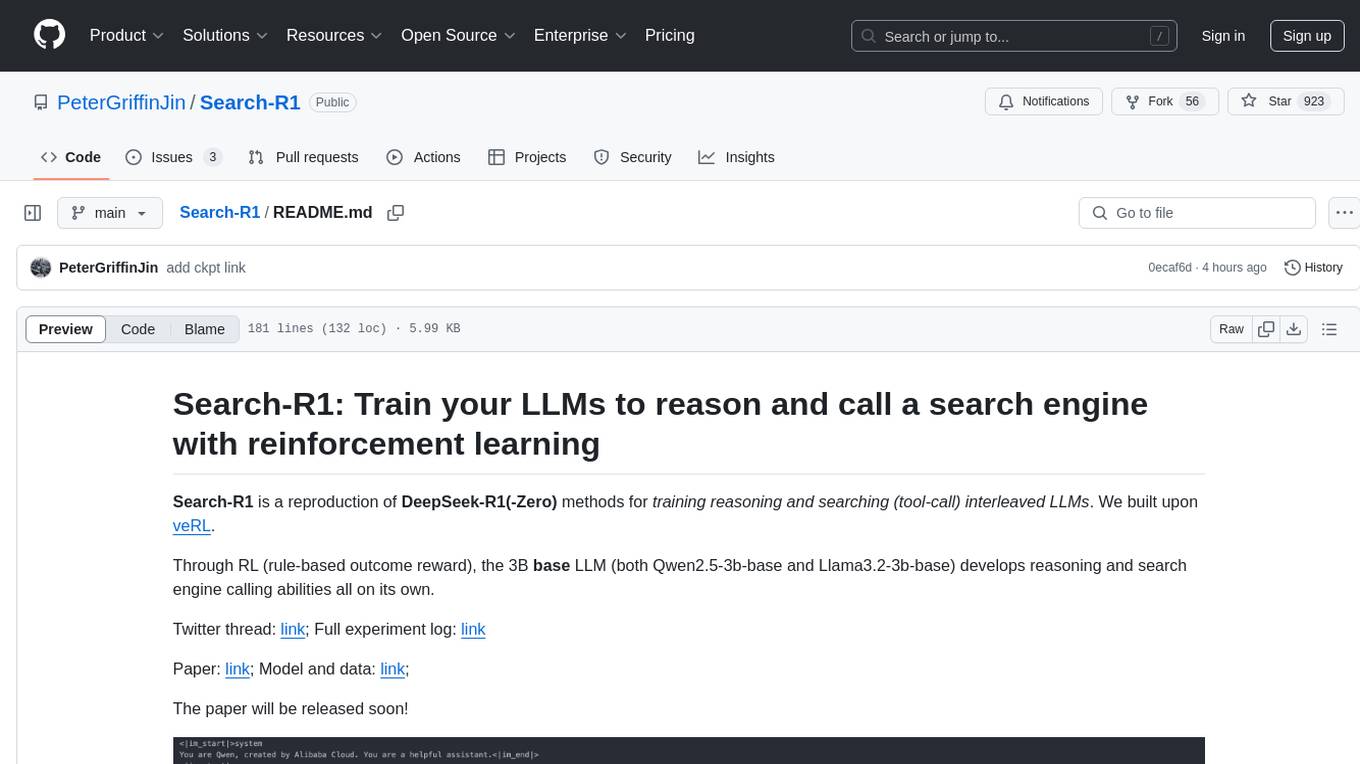
Search-R1
Search-R1 is a tool that trains large language models (LLMs) to reason and call a search engine using reinforcement learning. It is a reproduction of DeepSeek-R1 methods for training reasoning and searching interleaved LLMs, built upon veRL. Through rule-based outcome reward, the base LLM develops reasoning and search engine calling abilities independently. Users can train LLMs on their own datasets and search engines, with preliminary results showing improved performance in search engine calling and reasoning tasks.
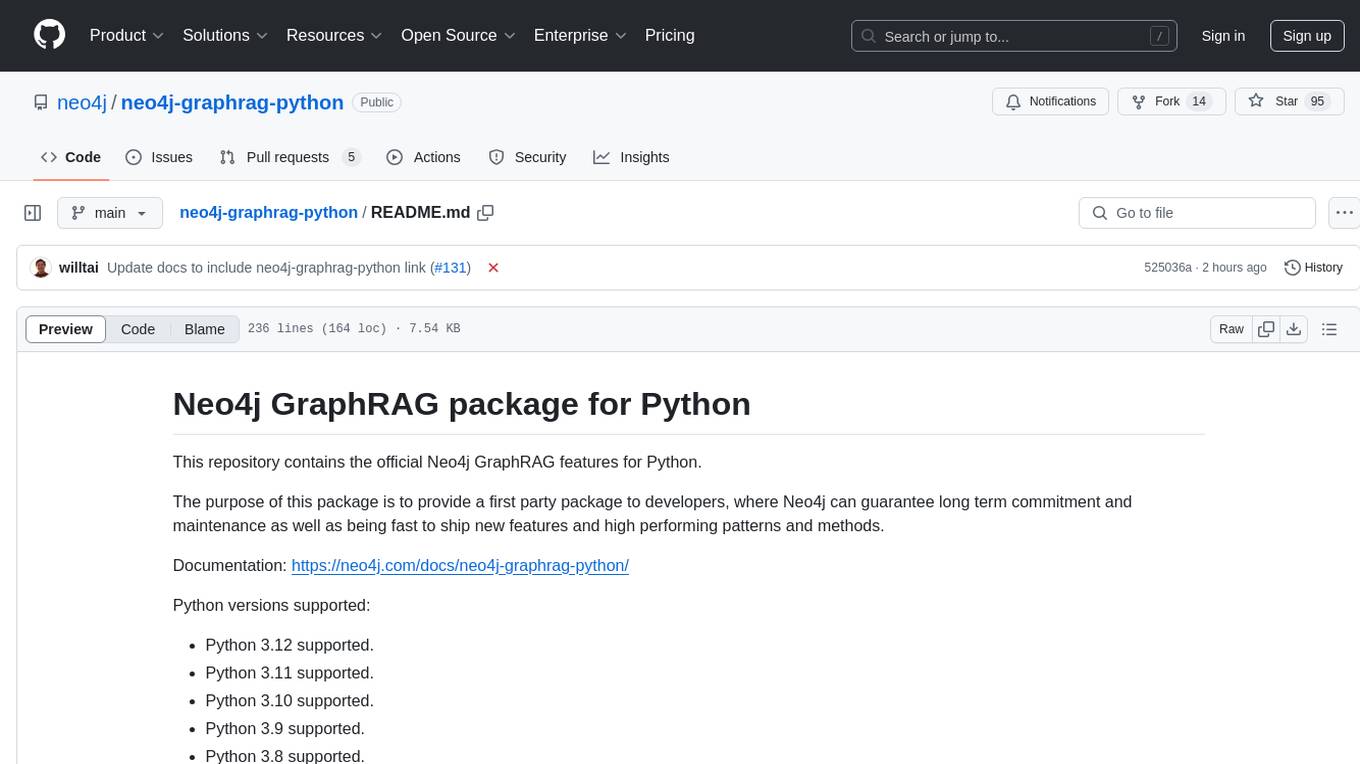
neo4j-graphrag-python
The Neo4j GraphRAG package for Python is an official repository that provides features for creating and managing vector indexes in Neo4j databases. It aims to offer developers a reliable package with long-term commitment, maintenance, and fast feature updates. The package supports various Python versions and includes functionalities for creating vector indexes, populating them, and performing similarity searches. It also provides guidelines for installation, examples, and development processes such as installing dependencies, making changes, and running tests.
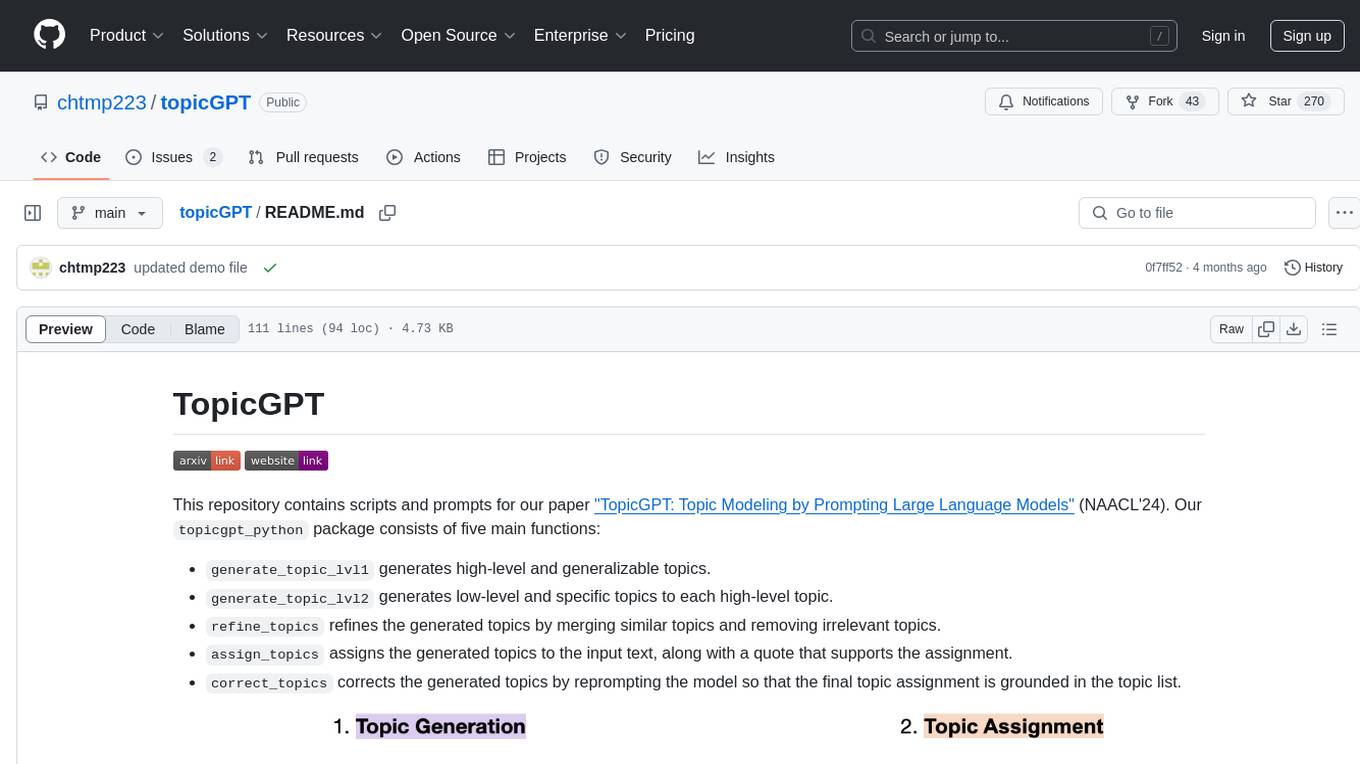
topicGPT
TopicGPT is a repository containing scripts and prompts for the paper 'TopicGPT: Topic Modeling by Prompting Large Language Models' (NAACL'24). The 'topicgpt_python' package offers functions to generate high-level and specific topics, refine topics, assign topics to input text, and correct generated topics. It supports various APIs like OpenAI, VertexAI, Azure, Gemini, and vLLM for inference. Users can prepare data in JSONL format, run the pipeline using provided scripts, and evaluate topic alignment with ground-truth labels.
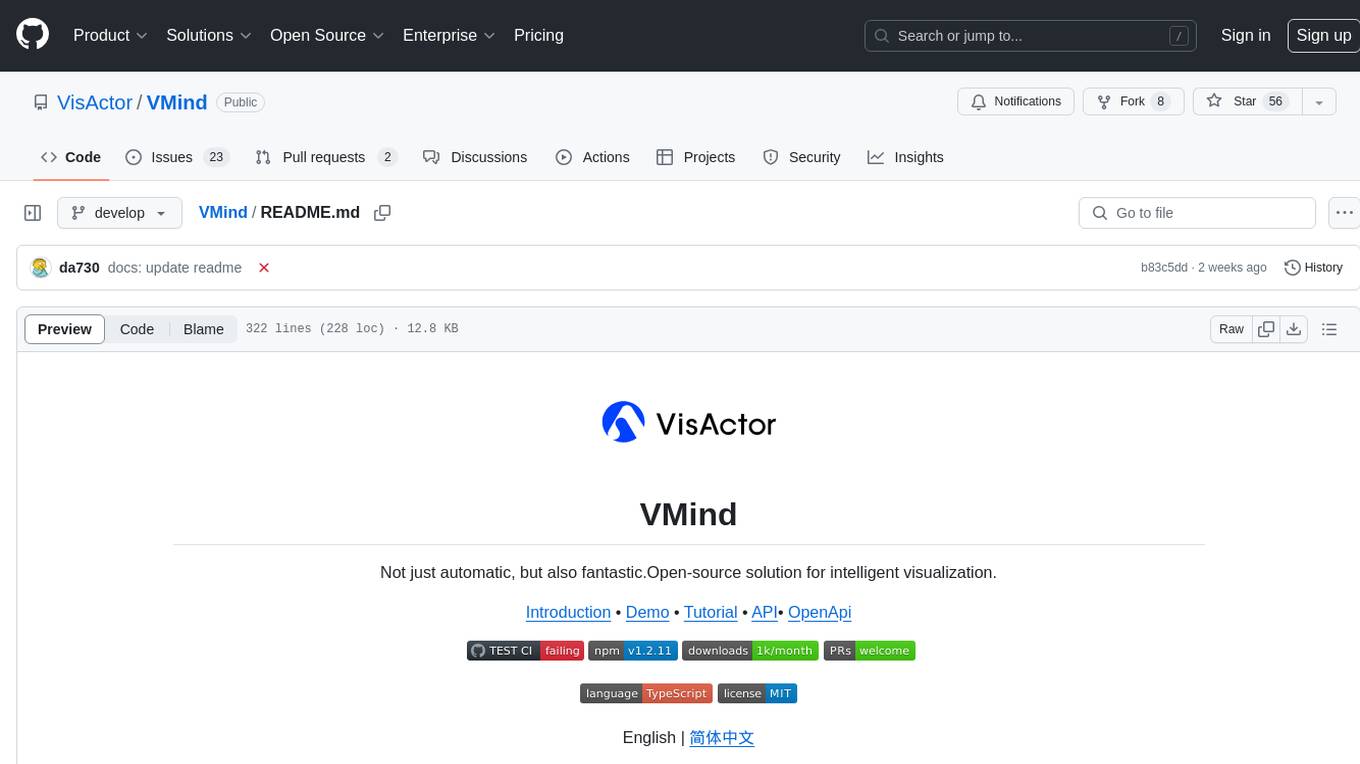
VMind
VMind is an open-source solution for intelligent visualization, providing an intelligent chart component based on LLM by VisActor. It allows users to create chart narrative works with natural language interaction, edit charts through dialogue, and export narratives as videos or GIFs. The tool is easy to use, scalable, supports various chart types, and offers one-click export functionality. Users can customize chart styles, specify themes, and aggregate data using LLM models. VMind aims to enhance efficiency in creating data visualization works through dialogue-based editing and natural language interaction.
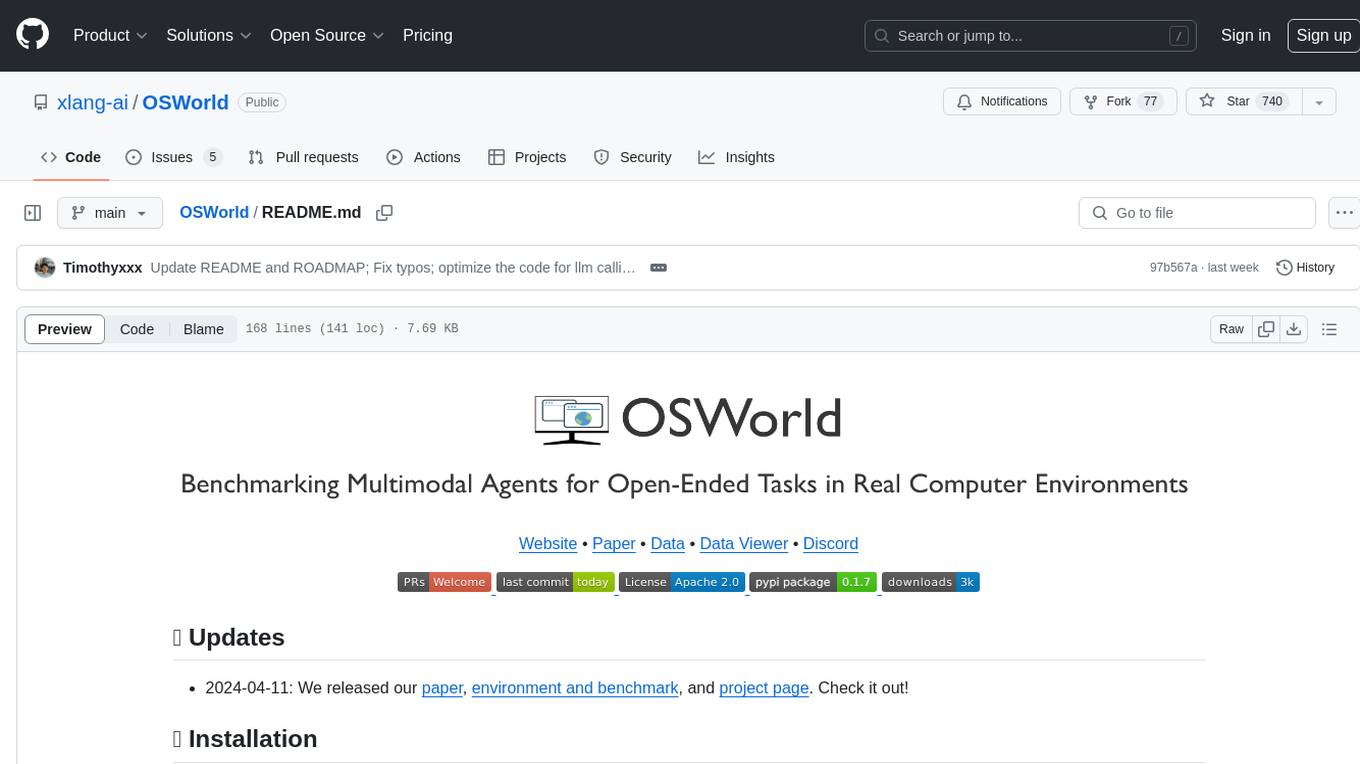
OSWorld
OSWorld is a benchmarking tool designed to evaluate multimodal agents for open-ended tasks in real computer environments. It provides a platform for running experiments, setting up virtual machines, and interacting with the environment using Python scripts. Users can install the tool on their desktop or server, manage dependencies with Conda, and run benchmark tasks. The tool supports actions like executing commands, checking for specific results, and evaluating agent performance. OSWorld aims to facilitate research in AI by providing a standardized environment for testing and comparing different agent baselines.
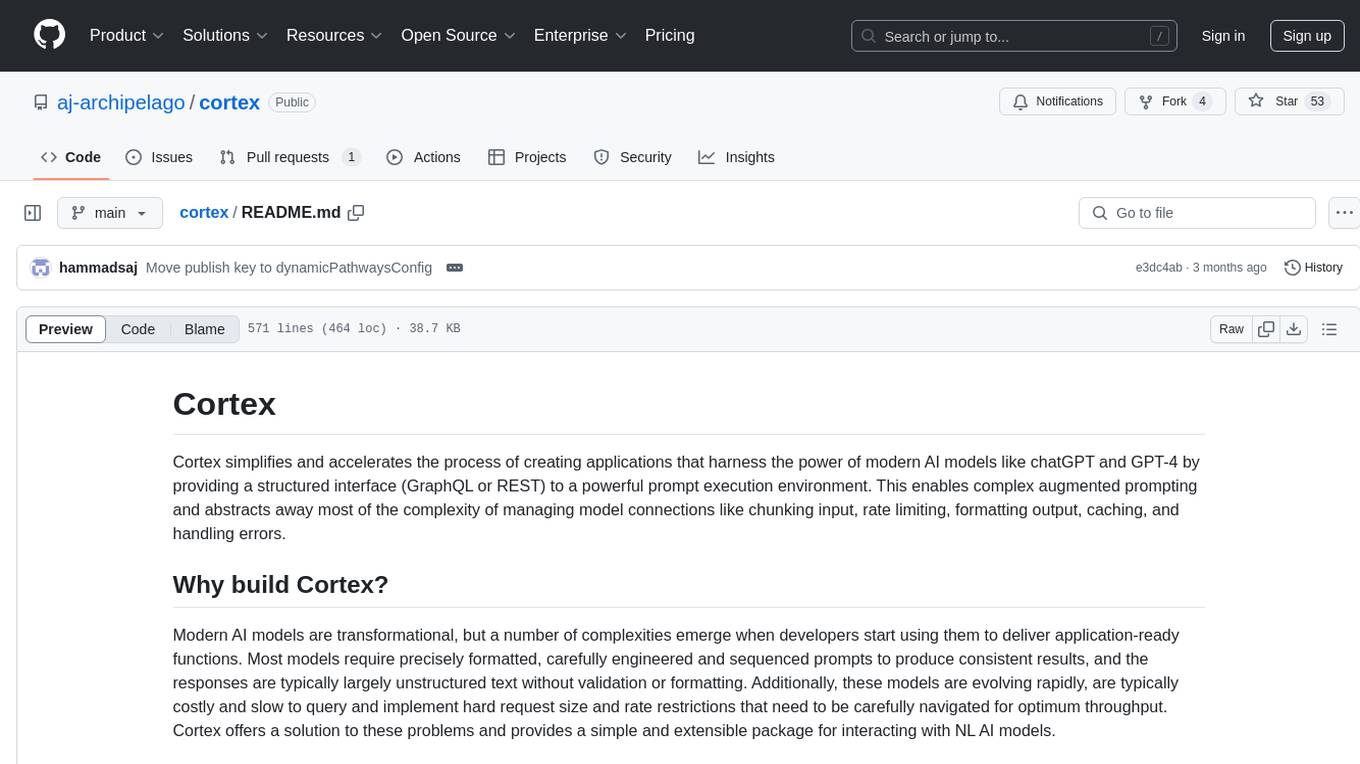
cortex
Cortex is a tool that simplifies and accelerates the process of creating applications utilizing modern AI models like chatGPT and GPT-4. It provides a structured interface (GraphQL or REST) to a prompt execution environment, enabling complex augmented prompting and abstracting away model connection complexities like input chunking, rate limiting, output formatting, caching, and error handling. Cortex offers a solution to challenges faced when using AI models, providing a simple package for interacting with NL AI models.
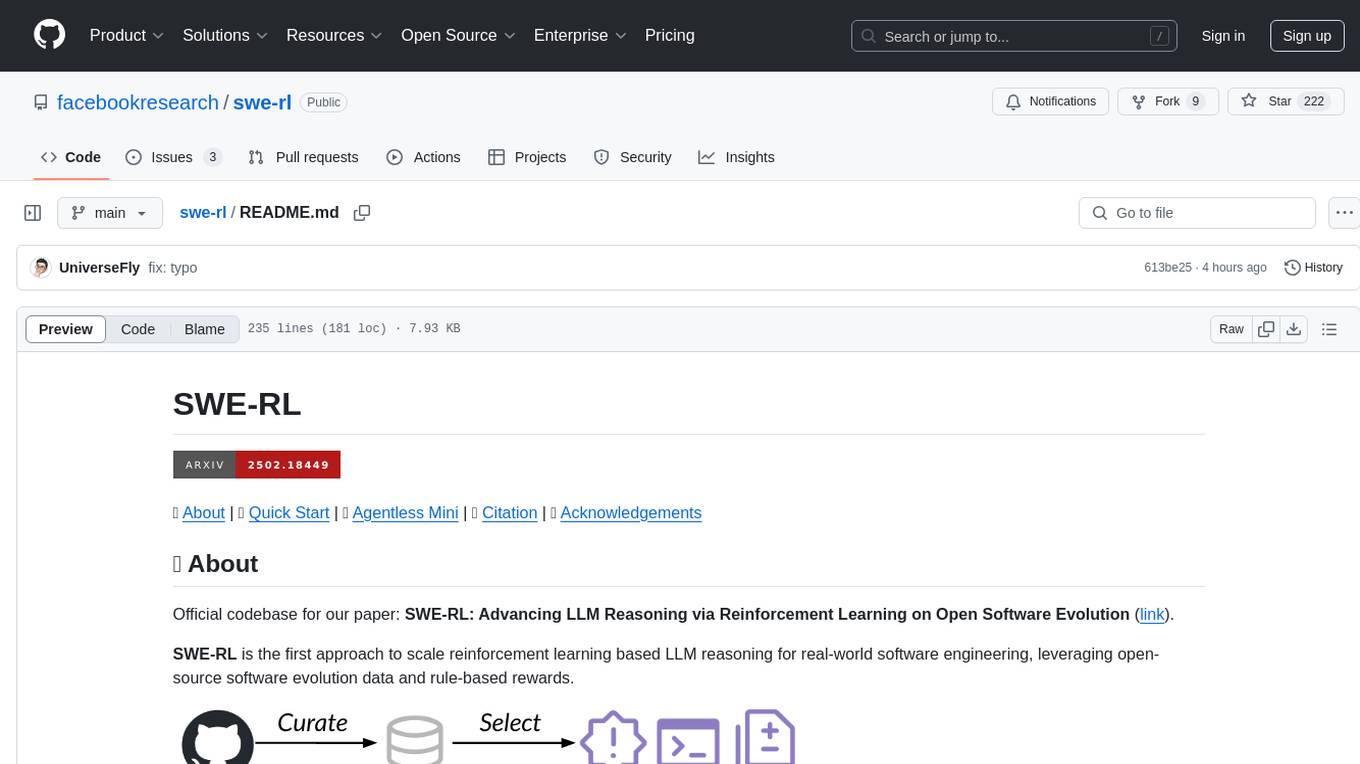
swe-rl
SWE-RL is the official codebase for the paper 'SWE-RL: Advancing LLM Reasoning via Reinforcement Learning on Open Software Evolution'. It is the first approach to scale reinforcement learning based LLM reasoning for real-world software engineering, leveraging open-source software evolution data and rule-based rewards. The code provides prompt templates and the implementation of the reward function based on sequence similarity. Agentless Mini, a part of SWE-RL, builds on top of Agentless with improvements like fast async inference, code refactoring for scalability, and support for using multiple reproduction tests for reranking. The tool can be used for localization, repair, and reproduction test generation in software engineering tasks.
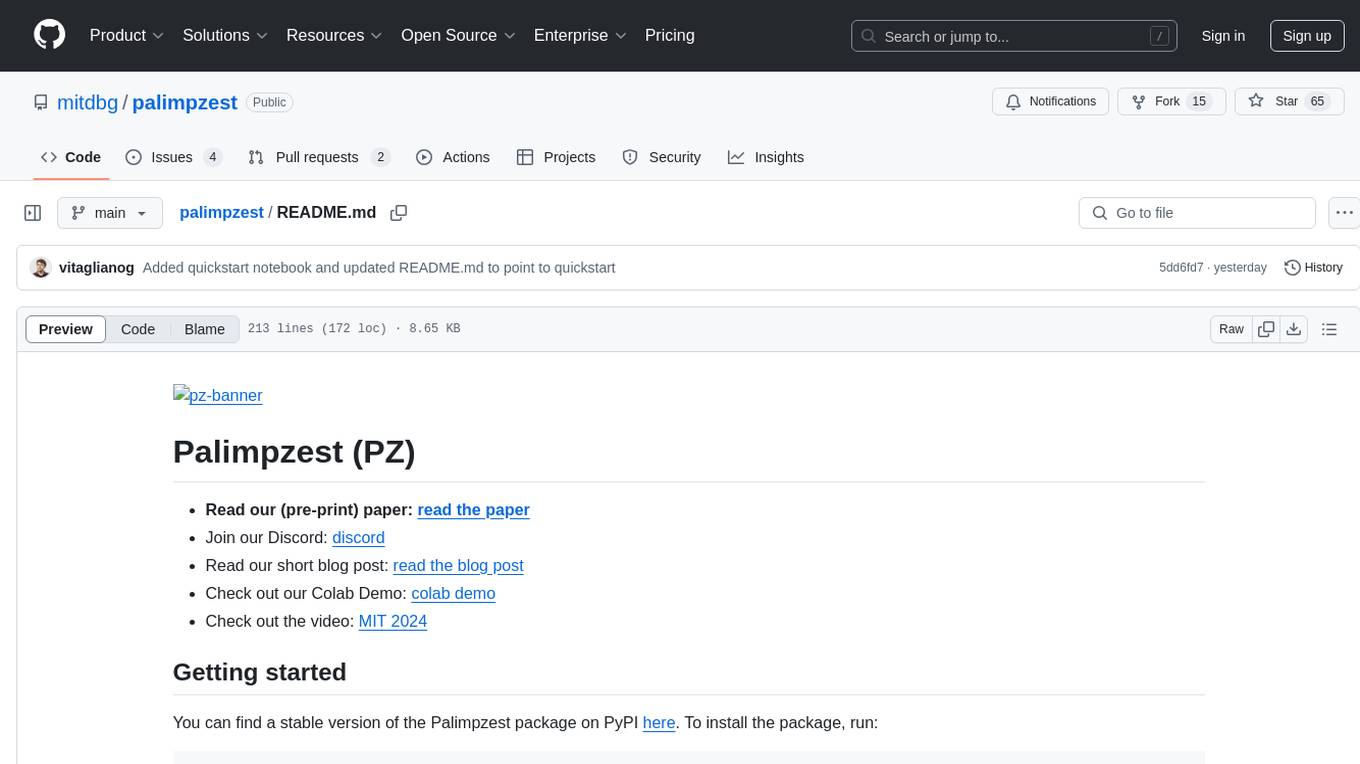
palimpzest
Palimpzest (PZ) is a tool for managing and optimizing workloads, particularly for data processing tasks. It provides a CLI tool and Python demos for users to register datasets, run workloads, and access results. Users can easily initialize their system, register datasets, and manage configurations using the CLI commands provided. Palimpzest also supports caching intermediate results and configuring for parallel execution with remote services like OpenAI and together.ai. The tool aims to streamline the workflow of working with datasets and optimizing performance for data extraction tasks.
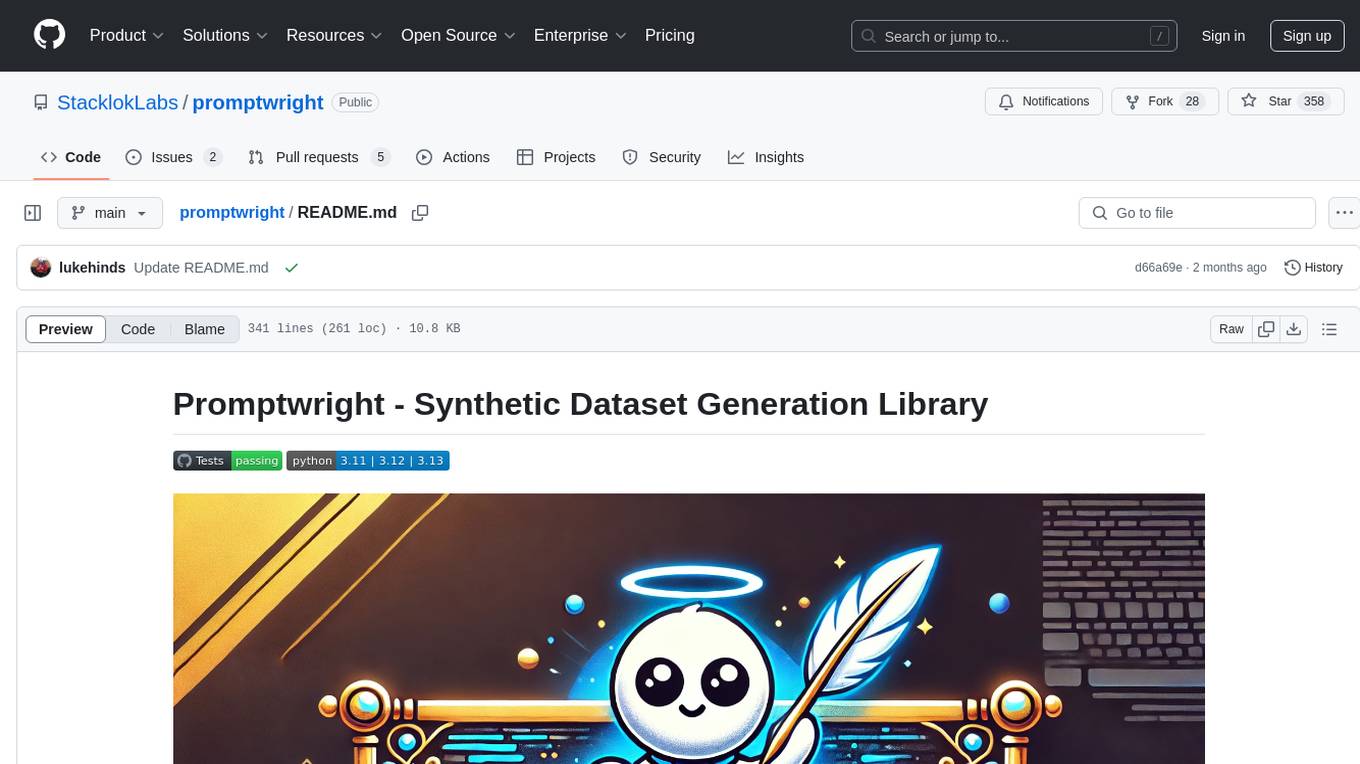
promptwright
Promptwright is a Python library designed for generating large synthetic datasets using a local LLM and various LLM service providers. It offers flexible interfaces for generating prompt-led synthetic datasets. The library supports multiple providers, configurable instructions and prompts, YAML configuration for tasks, command line interface for running tasks, push to Hugging Face Hub for dataset upload, and system message control. Users can define generation tasks using YAML configuration or Python code. Promptwright integrates with LiteLLM to interface with LLM providers and supports automatic dataset upload to Hugging Face Hub.
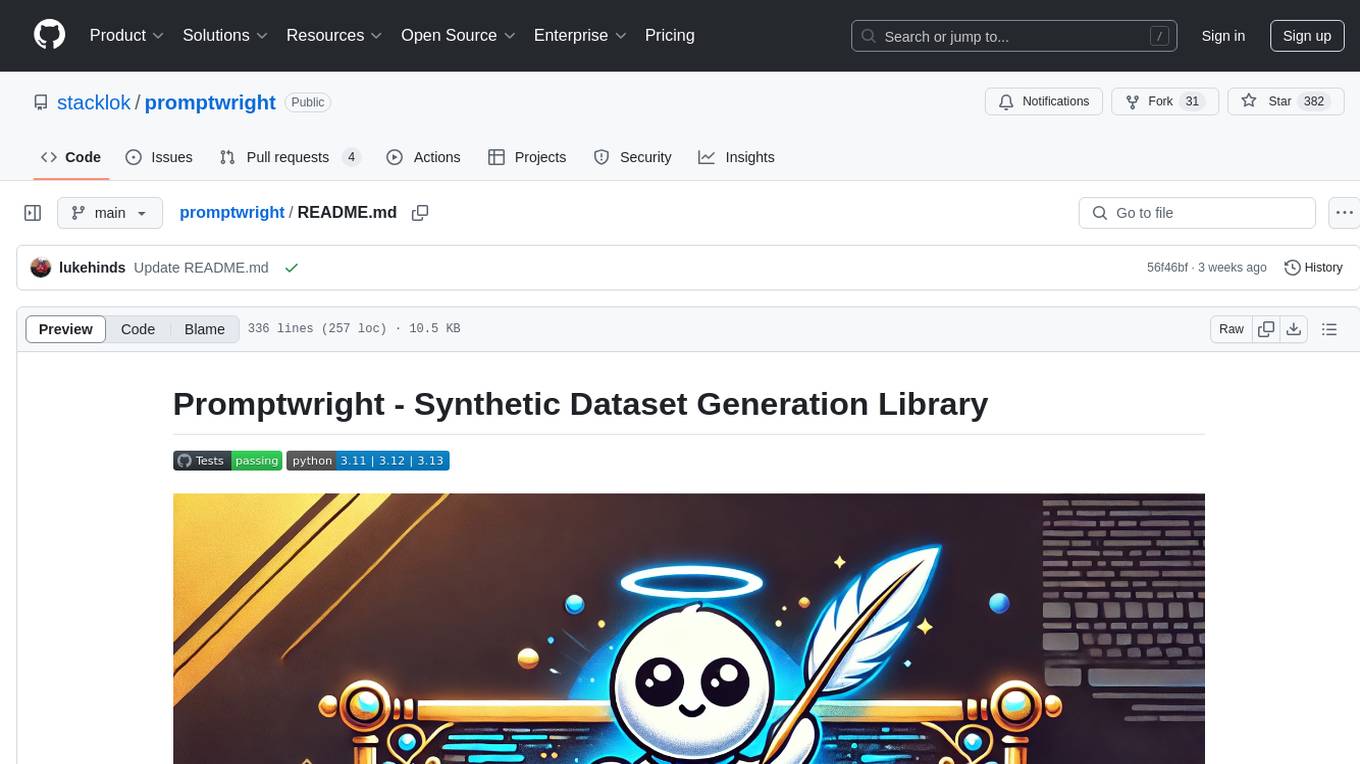
promptwright
Promptwright is a Python library designed for generating large synthetic datasets using local LLM and various LLM service providers. It offers flexible interfaces for generating prompt-led synthetic datasets. The library supports multiple providers, configurable instructions and prompts, YAML configuration, command line interface, push to Hugging Face Hub, and system message control. Users can define generation tasks using YAML configuration files or programmatically using Python code. Promptwright integrates with LiteLLM for LLM providers and supports automatic dataset upload to Hugging Face Hub. The library is not responsible for the content generated by models and advises users to review the data before using it in production environments.
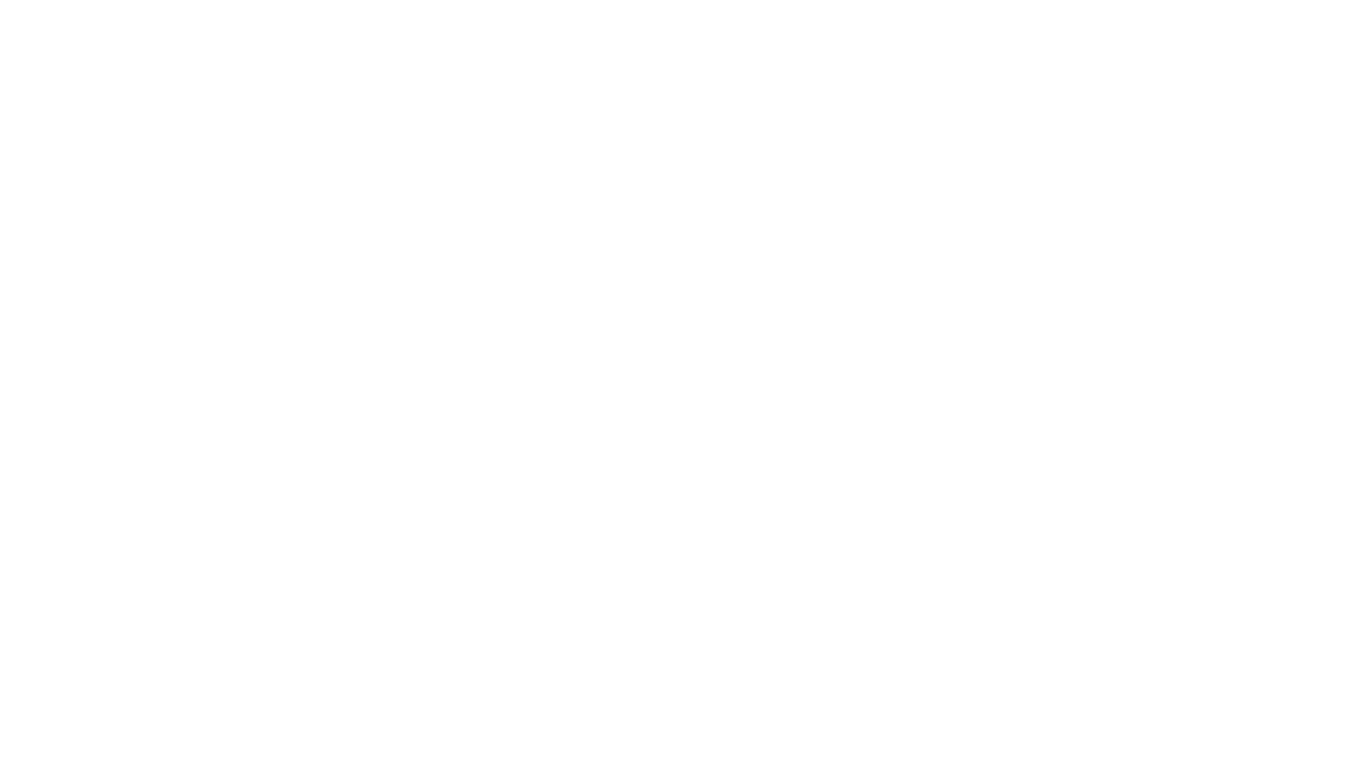
instructor-php
Instructor for PHP is a library designed for structured data extraction in PHP, powered by Large Language Models (LLMs). It simplifies the process of extracting structured, validated data from unstructured text or chat sequences. Instructor enhances workflow by providing a response model, validation capabilities, and max retries for requests. It supports classes as response models and provides features like partial results, string input, extracting scalar and enum values, and specifying data models using PHP type hints or DocBlock comments. The library allows customization of validation and provides detailed event notifications during request processing. Instructor is compatible with PHP 8.2+ and leverages PHP reflection, Symfony components, and SaloonPHP for communication with LLM API providers.
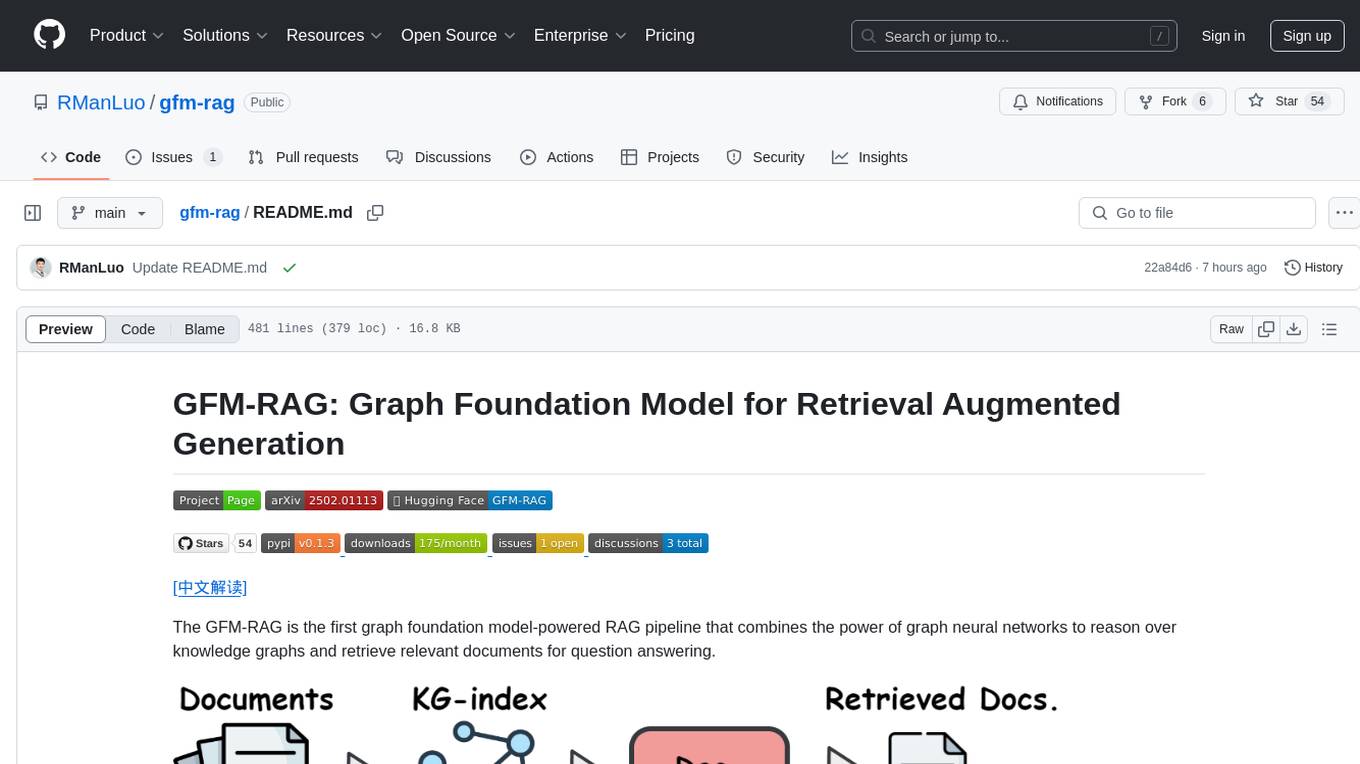
gfm-rag
The GFM-RAG is a graph foundation model-powered pipeline that combines graph neural networks to reason over knowledge graphs and retrieve relevant documents for question answering. It features a knowledge graph index, efficiency in multi-hop reasoning, generalizability to unseen datasets, transferability for fine-tuning, compatibility with agent-based frameworks, and interpretability of reasoning paths. The tool can be used for conducting retrieval and question answering tasks using pre-trained models or fine-tuning on custom datasets.
For similar tasks
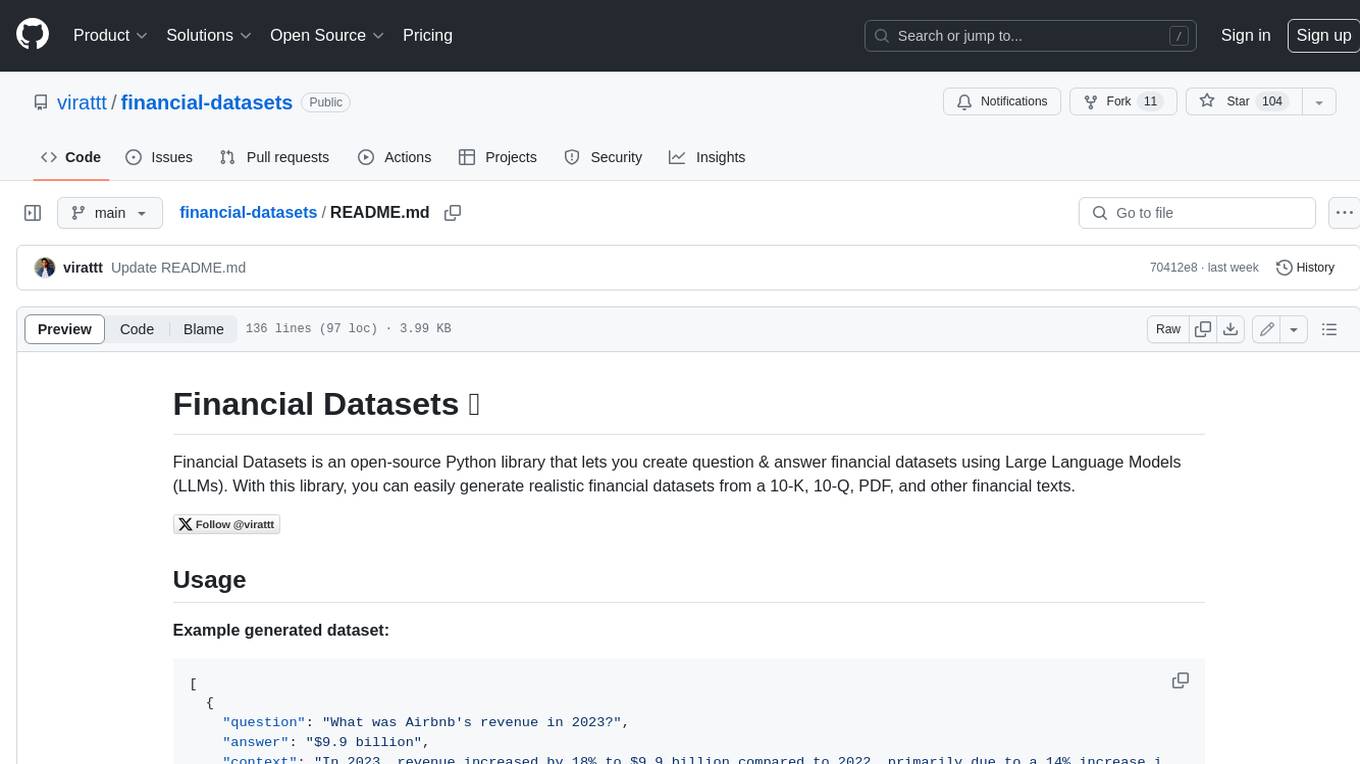
financial-datasets
Financial Datasets is an open-source Python library that allows users to create question and answer financial datasets using Large Language Models (LLMs). With this library, users can easily generate realistic financial datasets from 10-K, 10-Q, PDF, and other financial texts. The library provides three main methods for generating datasets: from any text, from a 10-K filing, or from a PDF URL. Financial Datasets can be used for a variety of tasks, including financial analysis, research, and education.
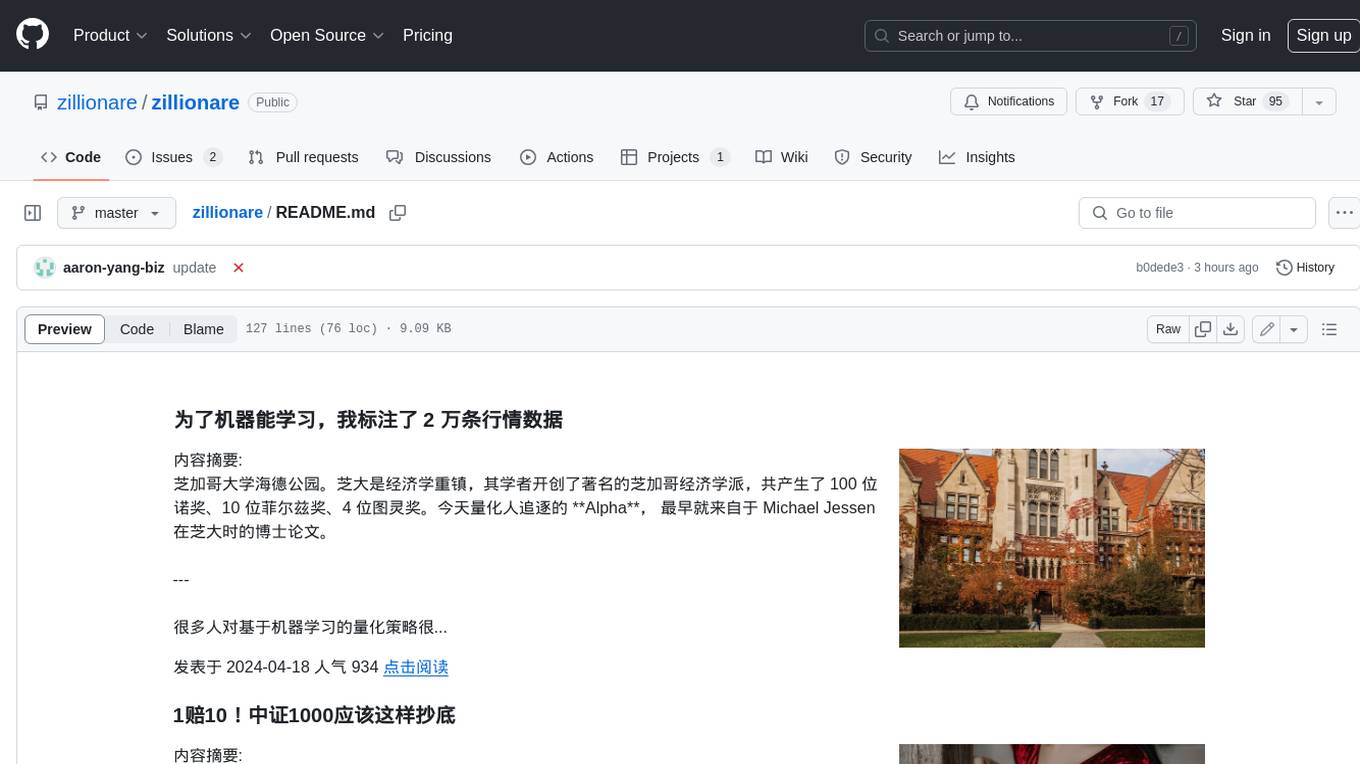
zillionare
This repository contains a collection of articles and tutorials on quantitative finance, including topics such as machine learning, statistical arbitrage, and risk management. The articles are written in a clear and concise style, and they are suitable for both beginners and experienced practitioners. The repository also includes a number of Jupyter notebooks that demonstrate how to use Python for quantitative finance.
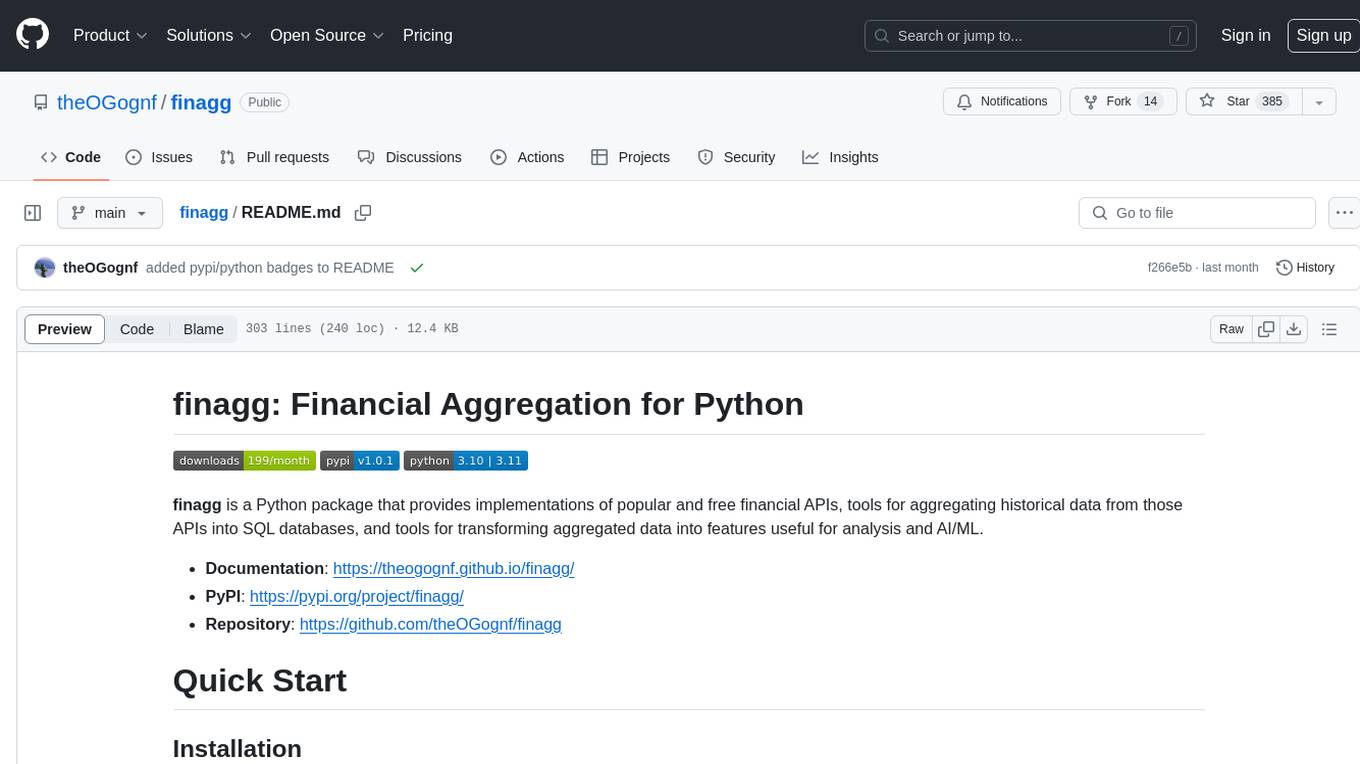
finagg
finagg is a Python package that provides implementations of popular and free financial APIs, tools for aggregating historical data from those APIs into SQL databases, and tools for transforming aggregated data into features useful for analysis and AI/ML. It offers documentation, installation instructions, and basic usage examples for exploring various financial APIs and features. Users can install recommended datasets from 3rd party APIs into a local SQL database, access Bureau of Economic Analysis (BEA) data, Federal Reserve Economic Data (FRED), Securities and Exchange Commission (SEC) filings, and more. The package also allows users to explore raw data features, install refined data features, and perform refined aggregations of raw data. Configuration options for API keys, user agents, and data locations are provided, along with information on dependencies and related projects.
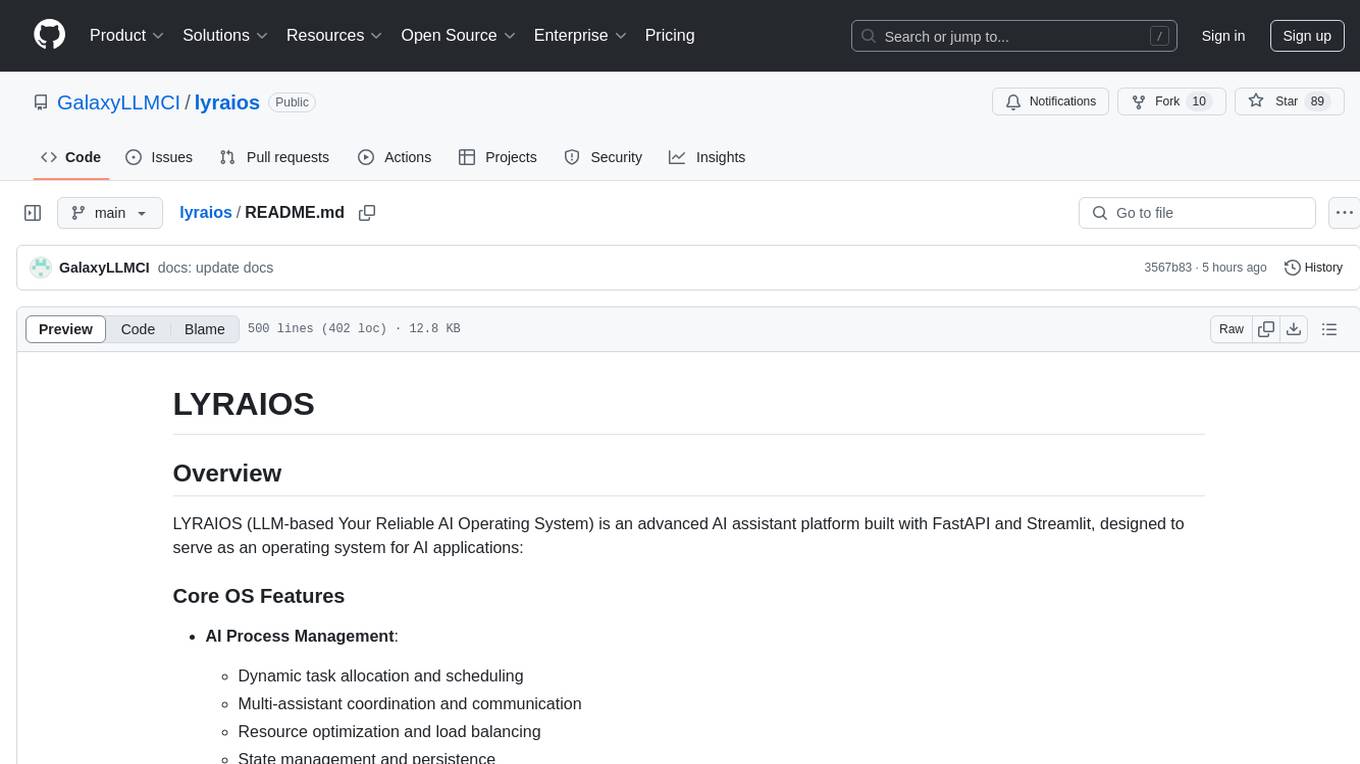
lyraios
LYRAIOS (LLM-based Your Reliable AI Operating System) is an advanced AI assistant platform built with FastAPI and Streamlit, designed to serve as an operating system for AI applications. It offers core features such as AI process management, memory system, and I/O system. The platform includes built-in tools like Calculator, Web Search, Financial Analysis, File Management, and Research Tools. It also provides specialized assistant teams for Python and research tasks. LYRAIOS is built on a technical architecture comprising FastAPI backend, Streamlit frontend, Vector Database, PostgreSQL storage, and Docker support. It offers features like knowledge management, process control, and security & access control. The roadmap includes enhancements in core platform, AI process management, memory system, tools & integrations, security & access control, open protocol architecture, multi-agent collaboration, and cross-platform support.
For similar jobs
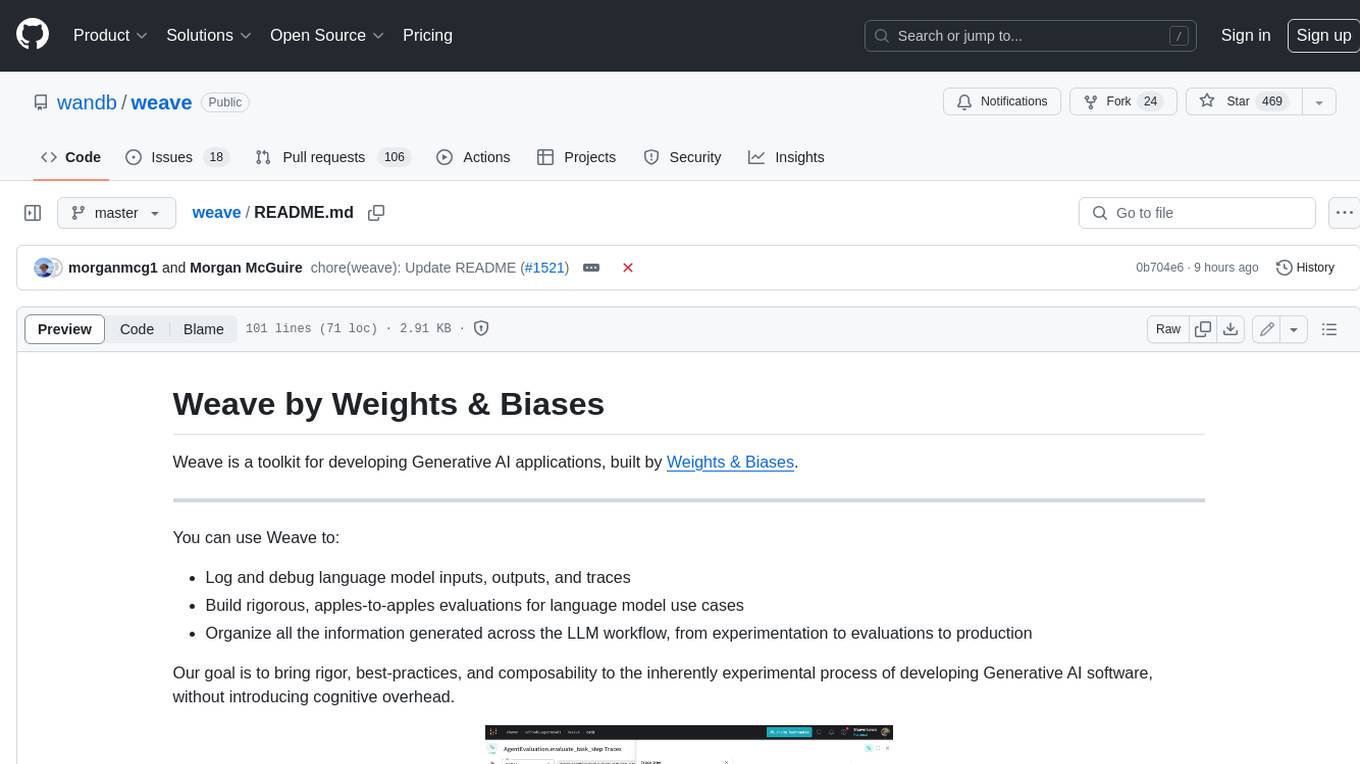
weave
Weave is a toolkit for developing Generative AI applications, built by Weights & Biases. With Weave, you can log and debug language model inputs, outputs, and traces; build rigorous, apples-to-apples evaluations for language model use cases; and organize all the information generated across the LLM workflow, from experimentation to evaluations to production. Weave aims to bring rigor, best-practices, and composability to the inherently experimental process of developing Generative AI software, without introducing cognitive overhead.
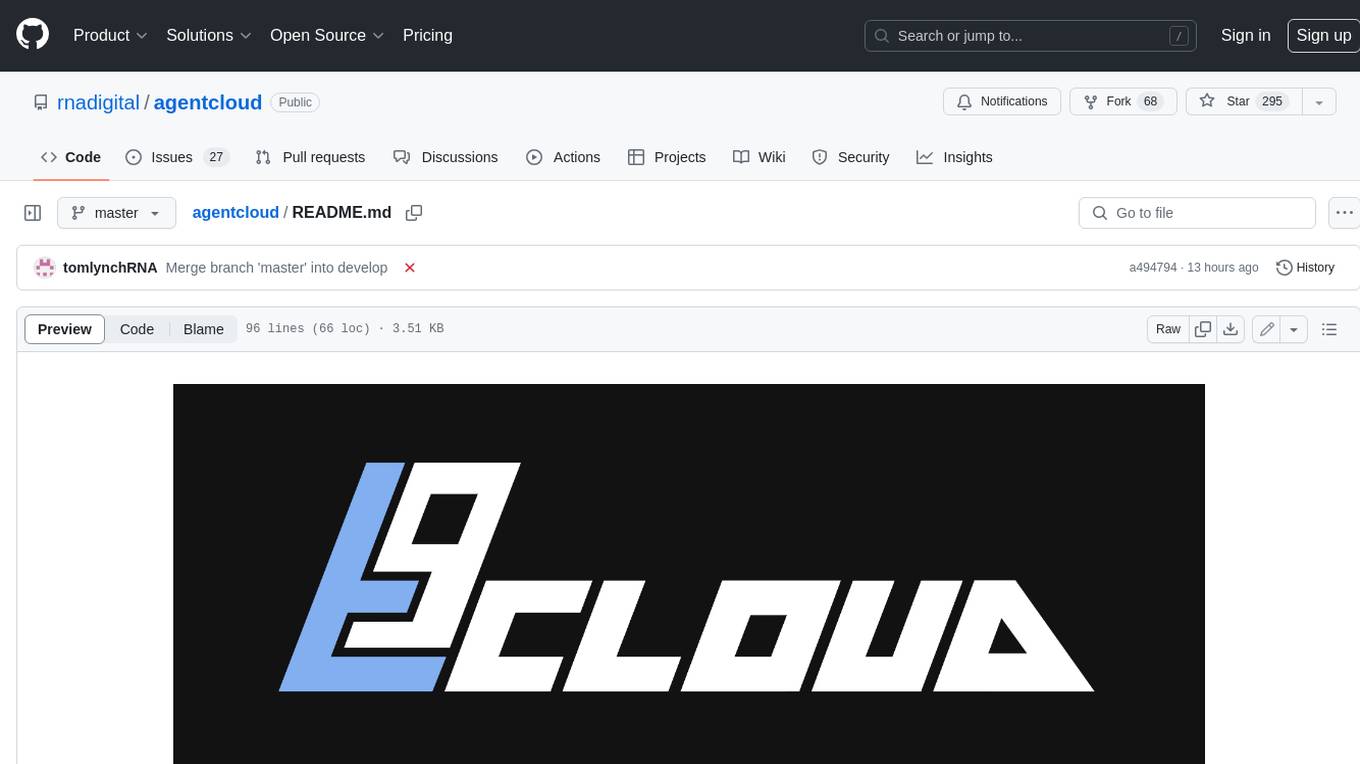
agentcloud
AgentCloud is an open-source platform that enables companies to build and deploy private LLM chat apps, empowering teams to securely interact with their data. It comprises three main components: Agent Backend, Webapp, and Vector Proxy. To run this project locally, clone the repository, install Docker, and start the services. The project is licensed under the GNU Affero General Public License, version 3 only. Contributions and feedback are welcome from the community.
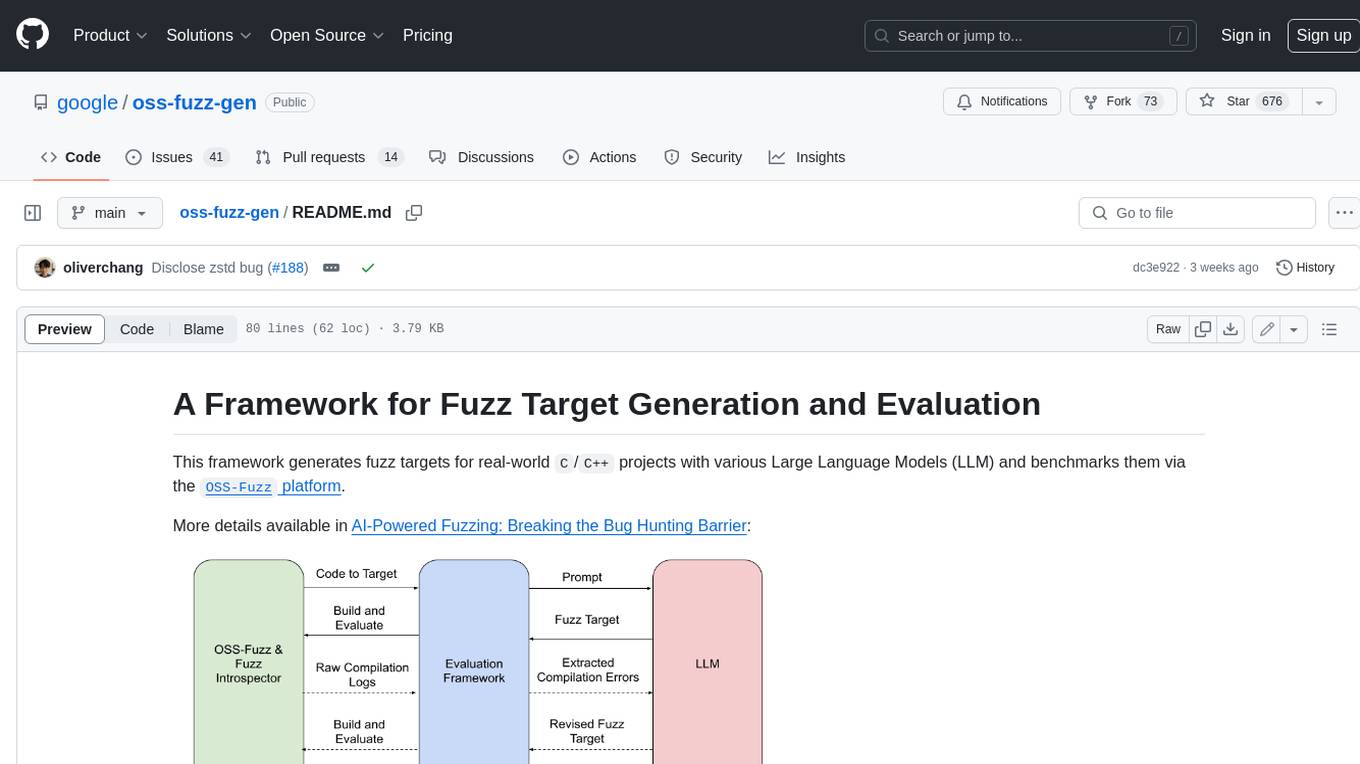
oss-fuzz-gen
This framework generates fuzz targets for real-world `C`/`C++` projects with various Large Language Models (LLM) and benchmarks them via the `OSS-Fuzz` platform. It manages to successfully leverage LLMs to generate valid fuzz targets (which generate non-zero coverage increase) for 160 C/C++ projects. The maximum line coverage increase is 29% from the existing human-written targets.
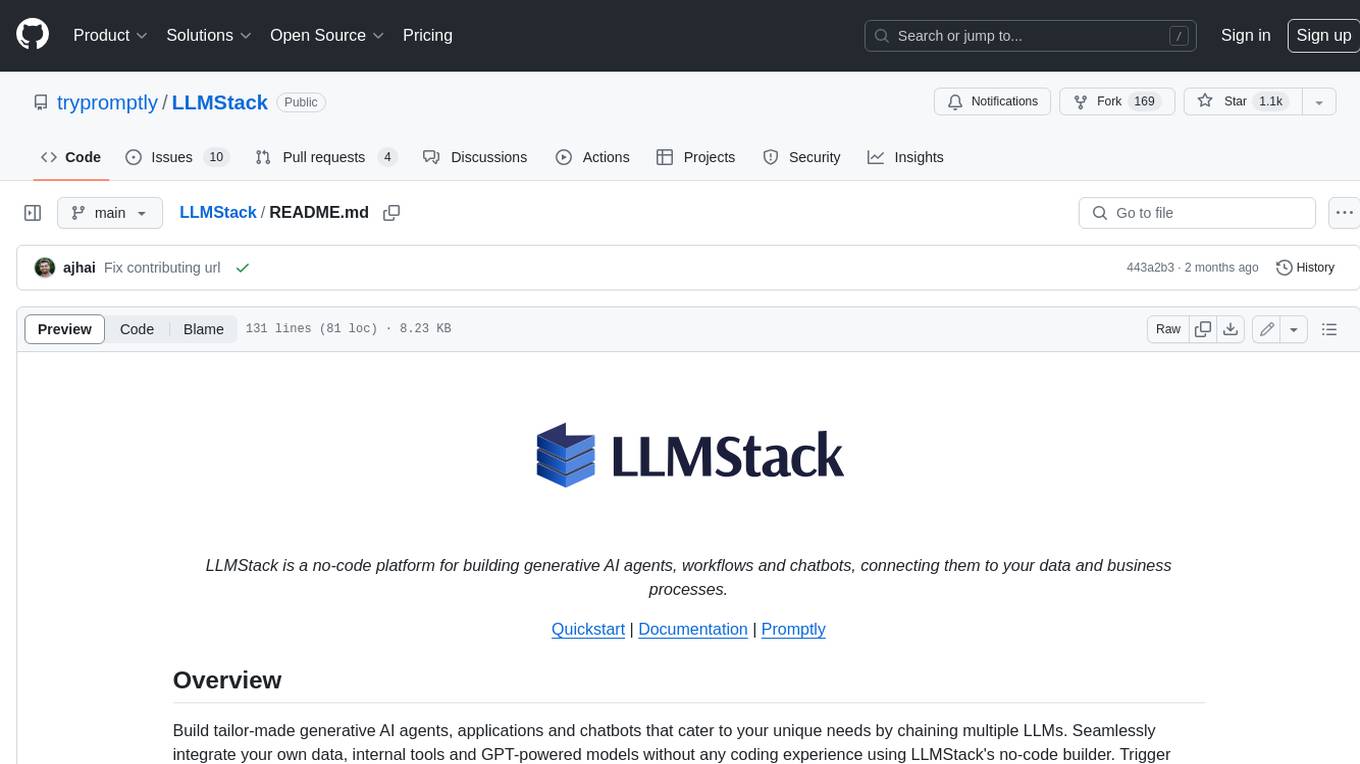
LLMStack
LLMStack is a no-code platform for building generative AI agents, workflows, and chatbots. It allows users to connect their own data, internal tools, and GPT-powered models without any coding experience. LLMStack can be deployed to the cloud or on-premise and can be accessed via HTTP API or triggered from Slack or Discord.
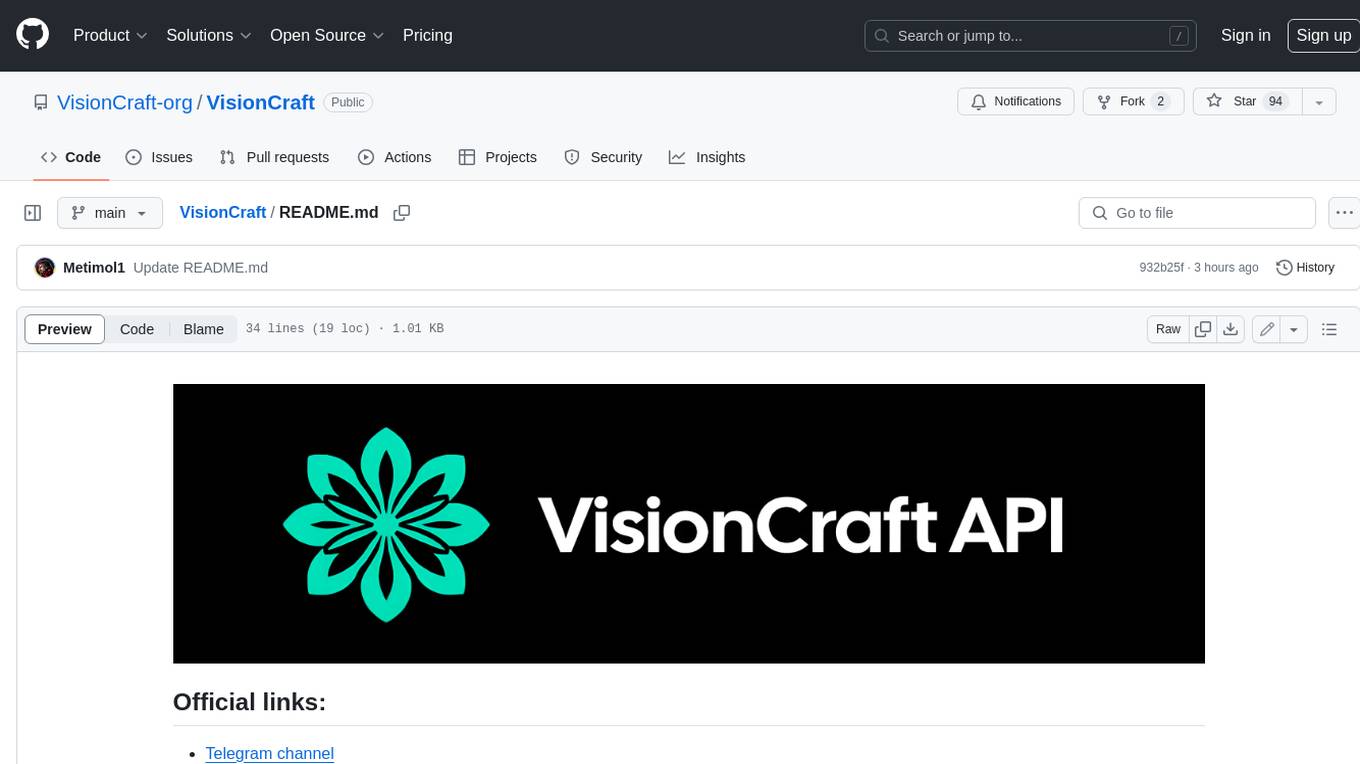
VisionCraft
The VisionCraft API is a free API for using over 100 different AI models. From images to sound.
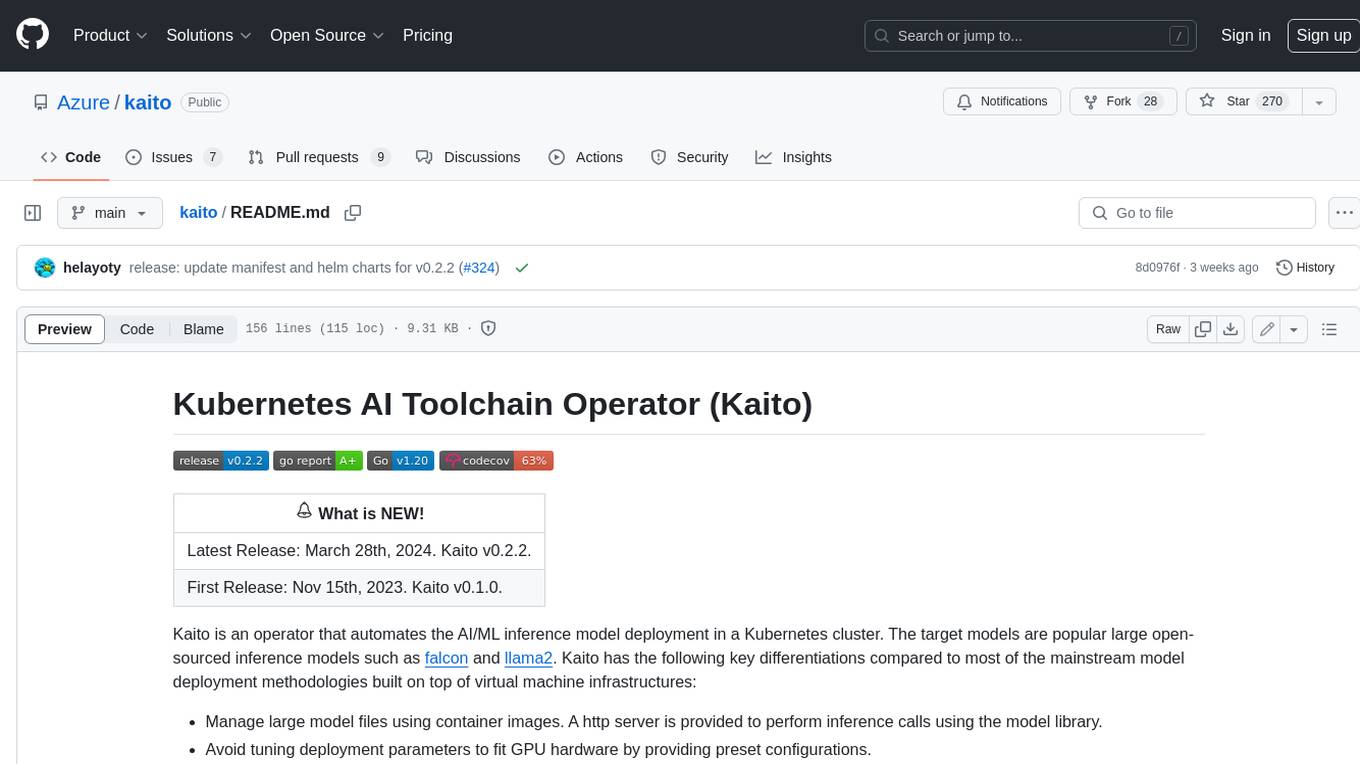
kaito
Kaito is an operator that automates the AI/ML inference model deployment in a Kubernetes cluster. It manages large model files using container images, avoids tuning deployment parameters to fit GPU hardware by providing preset configurations, auto-provisions GPU nodes based on model requirements, and hosts large model images in the public Microsoft Container Registry (MCR) if the license allows. Using Kaito, the workflow of onboarding large AI inference models in Kubernetes is largely simplified.
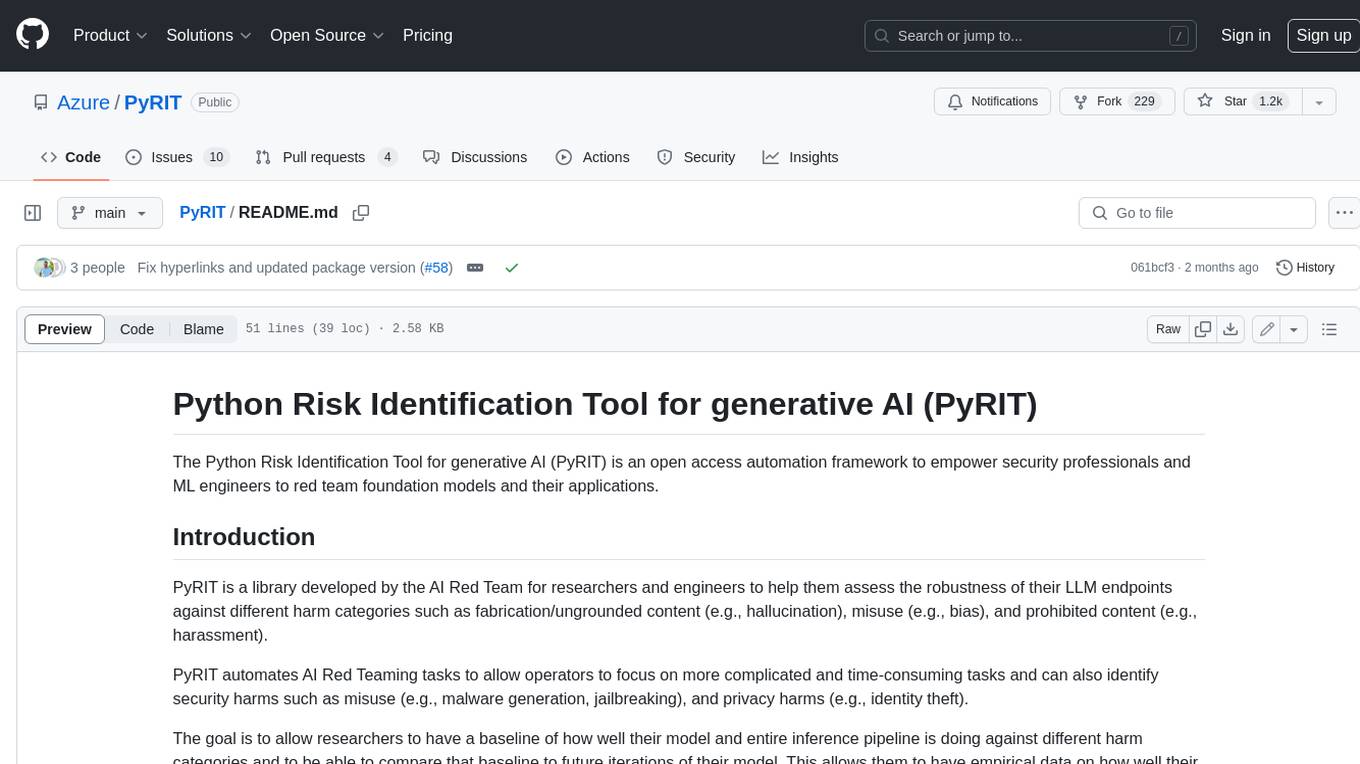
PyRIT
PyRIT is an open access automation framework designed to empower security professionals and ML engineers to red team foundation models and their applications. It automates AI Red Teaming tasks to allow operators to focus on more complicated and time-consuming tasks and can also identify security harms such as misuse (e.g., malware generation, jailbreaking), and privacy harms (e.g., identity theft). The goal is to allow researchers to have a baseline of how well their model and entire inference pipeline is doing against different harm categories and to be able to compare that baseline to future iterations of their model. This allows them to have empirical data on how well their model is doing today, and detect any degradation of performance based on future improvements.
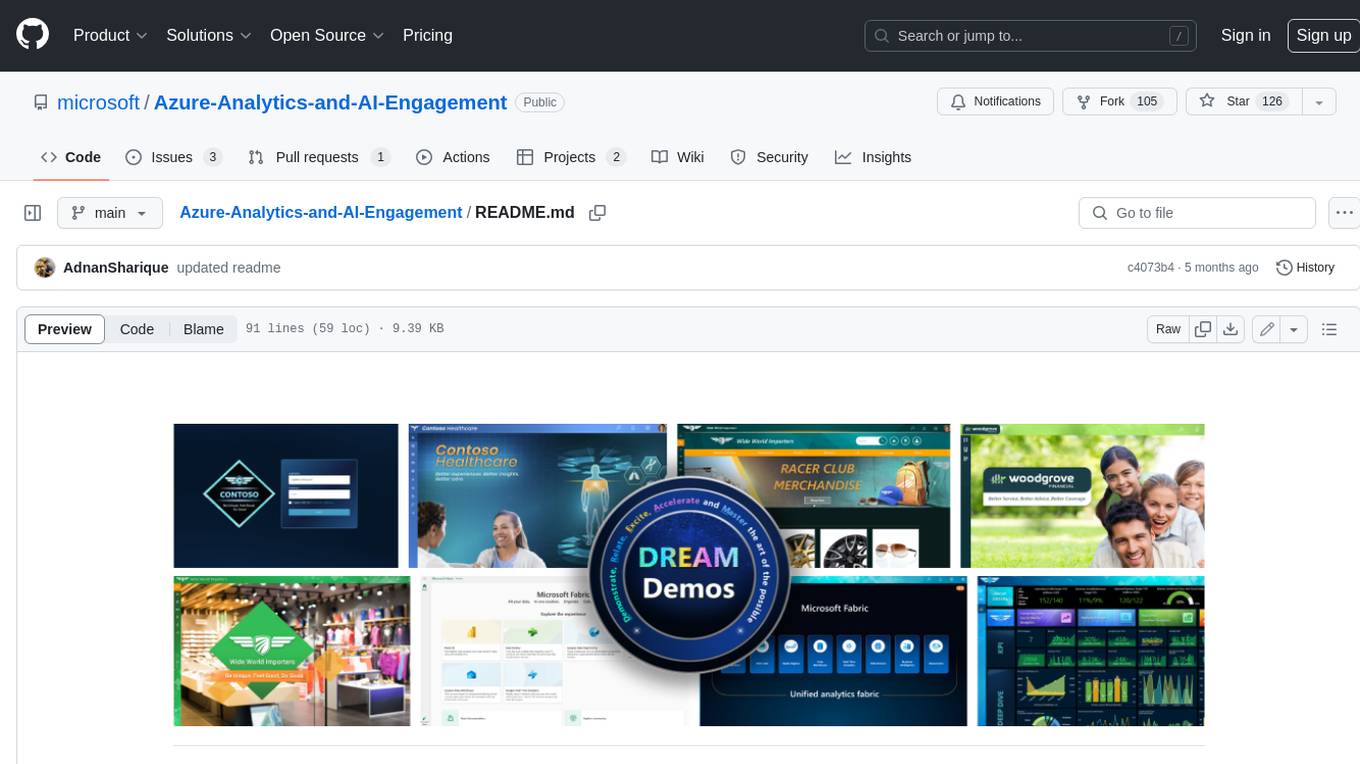
Azure-Analytics-and-AI-Engagement
The Azure-Analytics-and-AI-Engagement repository provides packaged Industry Scenario DREAM Demos with ARM templates (Containing a demo web application, Power BI reports, Synapse resources, AML Notebooks etc.) that can be deployed in a customer’s subscription using the CAPE tool within a matter of few hours. Partners can also deploy DREAM Demos in their own subscriptions using DPoC.