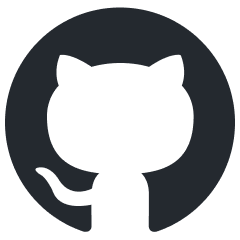
finagg
A Python package for aggregating and normalizing historical data from popular and free financial APIs.
Stars: 410
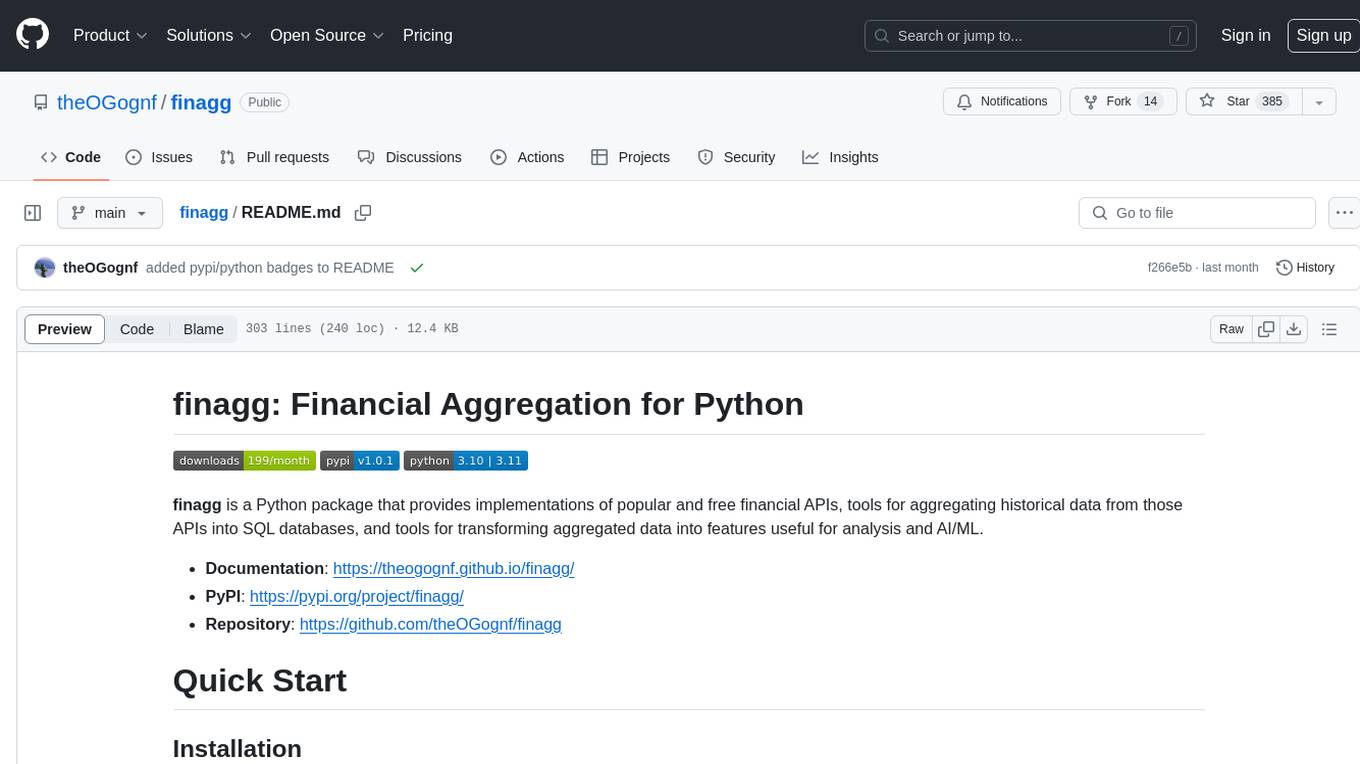
finagg is a Python package that provides implementations of popular and free financial APIs, tools for aggregating historical data from those APIs into SQL databases, and tools for transforming aggregated data into features useful for analysis and AI/ML. It offers documentation, installation instructions, and basic usage examples for exploring various financial APIs and features. Users can install recommended datasets from 3rd party APIs into a local SQL database, access Bureau of Economic Analysis (BEA) data, Federal Reserve Economic Data (FRED), Securities and Exchange Commission (SEC) filings, and more. The package also allows users to explore raw data features, install refined data features, and perform refined aggregations of raw data. Configuration options for API keys, user agents, and data locations are provided, along with information on dependencies and related projects.
README:
finagg is a Python package that provides implementations of popular and free financial APIs, tools for aggregating historical data from those APIs into SQL databases, and tools for transforming aggregated data into features useful for analysis and AI/ML.
- Documentation: https://theogognf.github.io/finagg/
- PyPI: https://pypi.org/project/finagg/
- Repository: https://github.com/theOGognf/finagg
Install with pip for the latest stable version.
pip install finagg
Install from GitHub for the latest unstable version.
git clone https://github.com/theOGognf/finagg.git
pip install ./finagg/
Optionally install the recommended datasets (economic data, company financials, stock histories, etc.) from 3rd party APIs into a local SQL database.
finagg install -ss economic -ts indices -z -r
The installation will point you where to get free API keys for each API that
requires one and will write those API keys to a local .env
file for storage.
Run finagg install --help
for more installation options and details.
These are just finagg usage samples. See the documentation for all the supported APIs and features.
These methods require internet access and API keys/user agent declarations.
Get Bureau of Economic Analysis (BEA) data.
>>> finagg.bea.api.gdp_by_industry.get(year=[2019]).head(5)
table_id freq year quarter industry industry_description ...
0 1 Q 2019 1 11 Agriculture, forestry, fishing, and hunting ...
1 1 Q 2019 1 111CA Farms ...
2 1 Q 2019 1 113FF Forestry, fishing, and related activities ...
3 1 Q 2019 1 21 Mining ...
4 1 Q 2019 1 211 Oil and gas extraction ...
Get Federal Reserve Economic Data (FRED).
>>> finagg.fred.api.series.observations.get(
... "CPIAUCNS",
... realtime_start=0,
... realtime_end=-1,
... output_type=4
... ).head(5)
realtime_start realtime_end date value series_id
0 1949-04-22 1953-02-26 1949-03-01 169.5 CPIAUCNS
1 1949-05-23 1953-02-26 1949-04-01 169.7 CPIAUCNS
2 1949-06-24 1953-02-26 1949-05-01 169.2 CPIAUCNS
3 1949-07-22 1953-02-26 1949-06-01 169.6 CPIAUCNS
4 1949-08-26 1953-02-26 1949-07-01 168.5 CPIAUCNS
Get Securities and Exchange Commission (SEC) filings.
>>> finagg.sec.api.company_facts.get(ticker="AAPL").head(5)
end value accn fy fp form filed ...
0 2009-06-27 895816758.0 0001193125-09-153165 2009 Q3 10-Q 2009-07-22 ...
1 2009-10-16 900678473.0 0001193125-09-214859 2009 FY 10-K 2009-10-27 ...
2 2009-10-16 900678473.0 0001193125-10-012091 2009 FY 10-K/A 2010-01-25 ...
3 2010-01-15 906794589.0 0001193125-10-012085 2010 Q1 10-Q 2010-01-25 ...
4 2010-04-09 909938383.0 0001193125-10-088957 2010 Q2 10-Q 2010-04-21 ...
These methods require internet access, API keys/user agent declarations, and
downloading and installing raw data through the finagg install
or
finagg <api/subpackage> install
commands.
Get the most popular FRED features all in one dataframe.
>>> finagg.fred.feat.economic.from_raw().head(5)
CIVPART LOG_CHANGE(CPIAUCNS) LOG_CHANGE(CSUSHPINSA) FEDFUNDS ...
date ...
2014-10-06 62.8 0.0 0.0 0.09 ...
2014-10-08 62.8 0.0 0.0 0.09 ...
2014-10-13 62.8 0.0 0.0 0.09 ...
2014-10-15 62.8 0.0 0.0 0.09 ...
2014-10-20 62.8 0.0 0.0 0.09 ...
Get quarterly report features from SEC data.
>>> finagg.sec.feat.quarterly.from_raw("AAPL").head(5)
LOG_CHANGE(Assets) LOG_CHANGE(AssetsCurrent) ...
fy fp filed ...
2010 Q1 2010-01-25 0.182629 -0.023676 ...
Q2 2010-04-21 0.000000 0.000000 ...
Q3 2010-07-21 0.000000 0.000000 ...
2011 Q1 2011-01-19 0.459174 0.278241 ...
Q2 2011-04-21 0.000000 0.000000 ...
Get an aggregation of quarterly and daily features for a particular ticker.
>>> finagg.fundam.feat.fundam.from_raw("AAPL").head(5)
PriceBookRatio PriceEarningsRatio
date
2010-01-25 0.175061 2.423509
2010-01-26 0.178035 2.464678
2010-01-27 0.178813 2.475448
2010-01-28 0.177154 2.452471
2010-01-29 0.173825 2.406396
These methods require installing refined data through the finagg install
or finagg <api/subpackage> install
commands.
Get a ticker's industry's averaged quarterly report features.
>>> finagg.sec.feat.quarterly.industry.from_refined(ticker="AAPL").head(5)
mean ...
name AssetCoverageRatio BookRatio DebtEquityRatio ...
fy fp filed ...
2014 Q1 2014-05-15 10.731301 9.448954 0.158318 ...
Q2 2014-08-14 10.731301 9.448954 0.158318 ...
Q3 2014-11-14 10.731301 9.448954 0.158318 ...
2015 Q1 2015-05-15 16.738972 9.269250 0.294238 ...
Q2 2015-08-13 16.738972 9.269250 0.294238 ...
Get a ticker's industry-averaged quarterly report features.
>>> finagg.sec.feat.quarterly.normalized.from_refined("AAPL").head(5)
NORM(LOG_CHANGE(Assets)) NORM(LOG_CHANGE(AssetsCurrent)) ...
fy fp filed ...
2010 Q2 2010-04-21 0.000000 0.000000 ...
Q3 2010-07-21 0.000000 0.000000 ...
2011 Q1 2011-01-19 0.978816 0.074032 ...
Q2 2011-04-21 0.000000 0.000000 ...
Q3 2011-07-20 -0.353553 -0.353553 ...
Get tickers sorted by an industry-averaged quarterly report feature.
>>> finagg.sec.feat.quarterly.normalized.get_tickers_sorted_by(
... "NORM(EarningsPerShareBasic)",
... year=2019
... )[:5]
['XRAY', 'TSLA', 'SYY', 'WHR', 'KMB']
Get tickers sorted by an industry-averaged fundamental feature.
>>> finagg.fundam.feat.fundam.normalized.get_tickers_sorted_by(
... "NORM(PriceEarningsRatio)",
... date="2019-01-04"
... )[:5]
['AMD', 'TRGP', 'HPE', 'CZR', 'TSLA']
API keys and user agent declarations are required for most of the APIs. You can set environment variables to expose your API keys and user agents to finagg, or you can pass your API keys and user agents to the implemented APIs programmatically. The following environment variables are used for configuring API keys and user agents:
-
BEA_API_KEY
is for the Bureau of Economic Analysis's API key. You can get a free API key from the BEA API site. -
FRED_API_KEY
is for the Federal Reserve Economic Data API key. You can get a free API key from the FRED API site. -
INDICES_API_USER_AGENT
is for scraping popular indices' compositions from Wikipedia and should be equivalent to a browser's user agent declaration. This defaults to a hardcoded value, but it may not always work. -
SEC_API_USER_AGENT
is for the Securities and Exchange Commission's API. This should be of the formatFIRST_NAME LAST_NAME E_MAIL
.
finagg's root path, HTTP cache path, and database path are all configurable
through environment variables. By default, all data related to finagg is put
in a ./findata
directory relative to a root directory. You can change these
locations by modifying the respective environment variables:
-
FINAGG_ROOT_PATH
points to the parent directory of the./findata
directory. Defaults to your current working directory. -
FINAGG_HTTP_CACHE_PATH
points to the HTTP requests cache SQLite storage. Defaults to./findata/http_cache.sqlite
. -
FINAGG_DATABASE_URL
points to the finagg data storage. Defaults to./findata/finagg.sqlite
.
You can change some finagg behavior with other environment variables:
-
FINAGG_DISABLE_HTTP_CACHE
: Set this to"1"
or"True"
to disable the HTTP requests cache. Instead of a cachable session, a default, uncached user session will be used for all requests.
- pandas for fast, flexible, and expressive representations of relational data.
- requests for HTTP requests to 3rd party APIs.
- requests-cache for caching HTTP requests to avoid getting throttled by 3rd party API servers.
- SQLAlchemy for a SQL Python interface.
- yfinance for historical stock data from Yahoo! Finance.
- The BEA API and the BEA API key registration link.
- The FRED API and the FRED API key registration link.
- The SEC API.
- FinRL is a collection of financial reinforcement learning environments and tools.
- fredapi is an implementation of the FRED API.
- OpenBBTerminal is an open-source version of the Bloomberg Terminal.
- sec-edgar is an implementation of a file-based SEC EDGAR parser.
- sec-edgar-api is an implementation of the SEC EDGAR REST API.
Aggregate some data, create some analysis notebooks, or create some RL environments using the implemented data features and SQL tables. This project was originally created to make RL environments for financial applications but has since focused its purpose to just aggregating financial data and features. That being said, all the implemented features are defined in such a way to make it very easy to develop financial AI/ML, so we encourage you to do just that!
Implemented APIs may be relatively new and simply may not provide data for a particular ticker or economic data series. For example, earnings per share may not be accessible for all companies through the SEC EDGAR API. In some cases, APIs may raise an HTTP error, causing installations to skip the ticker or series. Additionally, not all tickers and economic data series contain sufficient data for feature normalization. If a ticker or series only has one data point, that data point could be dropped when computing a feature (such as percent change), causing no data to be installed.
Python 3.10 and up are supported. We don't plan on supporting lower versions because 3.10 introduces some nice quality of life updates that are used throughout the package.
The package is developed and tested on both Linux and Windows, but we recommend using Linux or WSL in practice. The package performs a good amount of I/O and interprocess operations that could result in a noticeable performance degradation on Windows.
For Tasks:
Click tags to check more tools for each tasksFor Jobs:
Alternative AI tools for finagg
Similar Open Source Tools
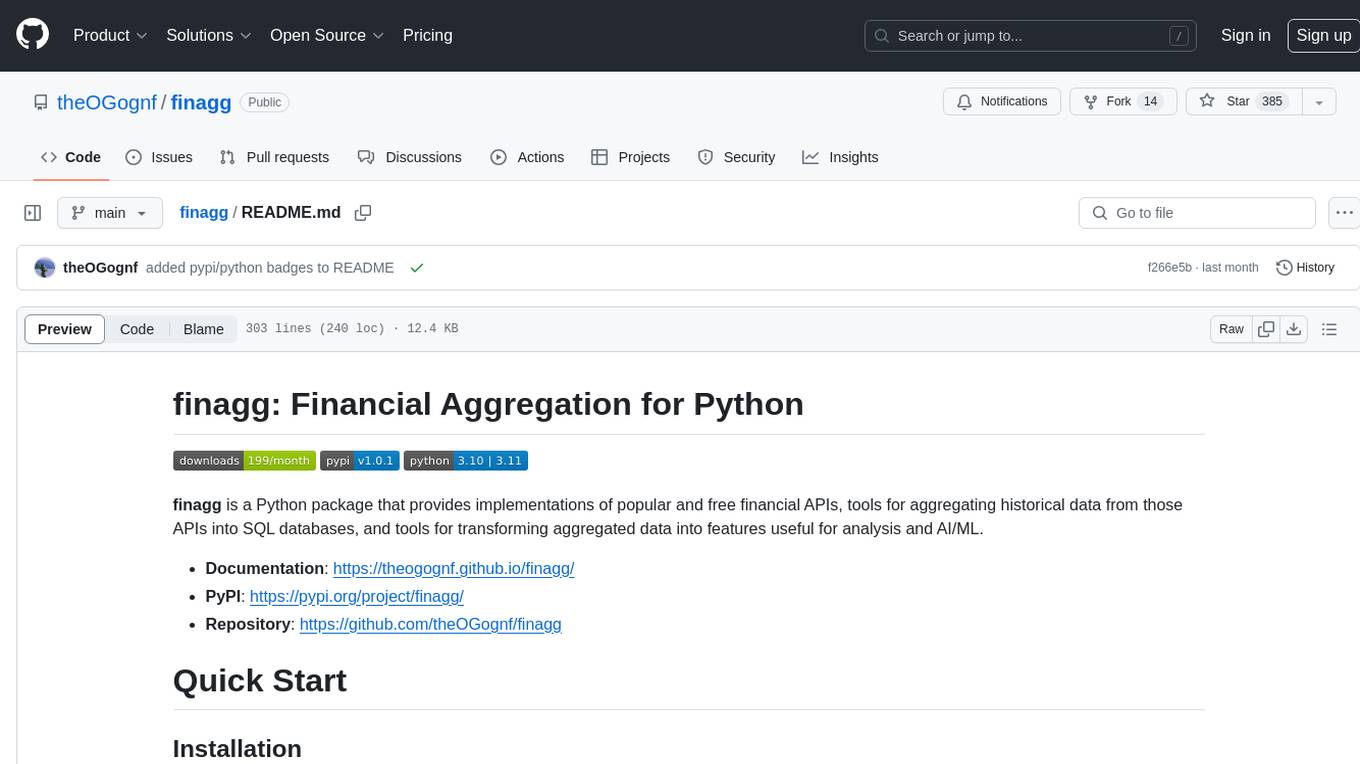
finagg
finagg is a Python package that provides implementations of popular and free financial APIs, tools for aggregating historical data from those APIs into SQL databases, and tools for transforming aggregated data into features useful for analysis and AI/ML. It offers documentation, installation instructions, and basic usage examples for exploring various financial APIs and features. Users can install recommended datasets from 3rd party APIs into a local SQL database, access Bureau of Economic Analysis (BEA) data, Federal Reserve Economic Data (FRED), Securities and Exchange Commission (SEC) filings, and more. The package also allows users to explore raw data features, install refined data features, and perform refined aggregations of raw data. Configuration options for API keys, user agents, and data locations are provided, along with information on dependencies and related projects.
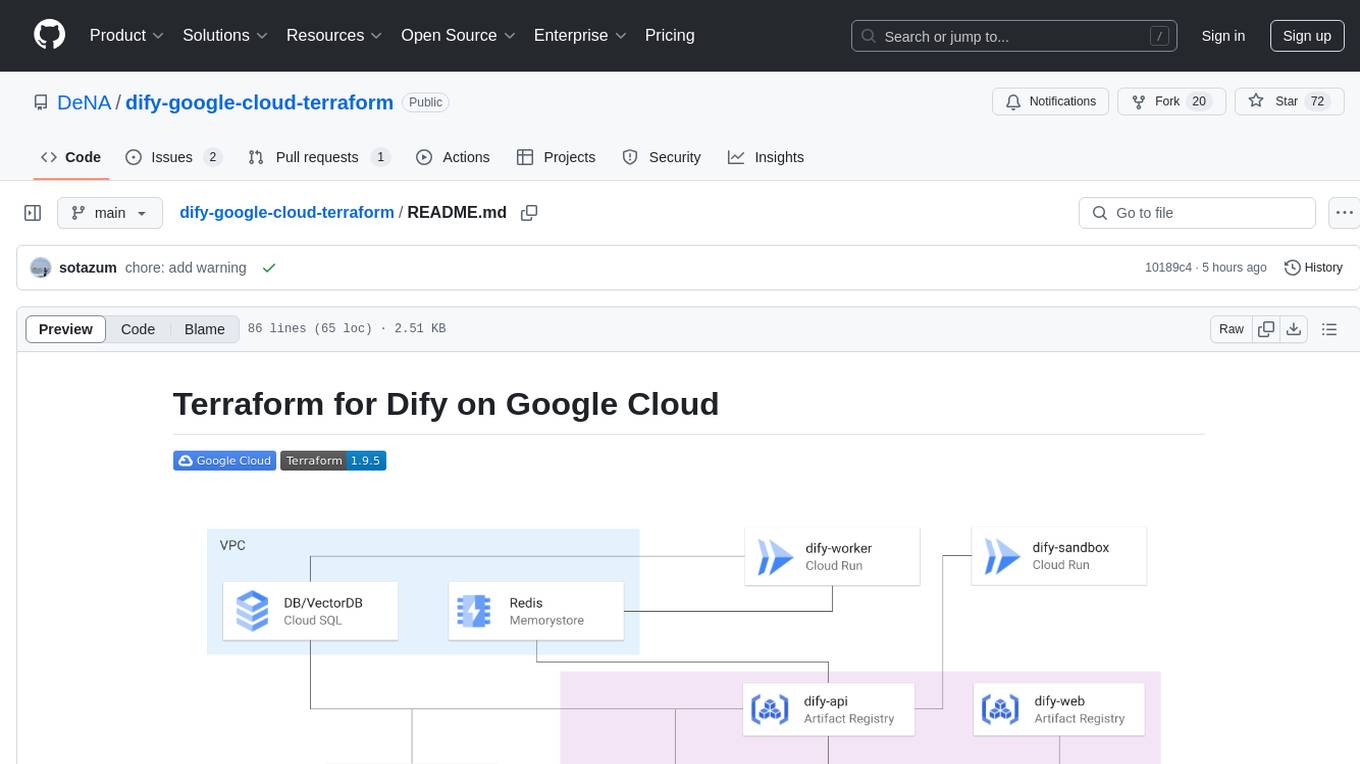
dify-google-cloud-terraform
This repository provides Terraform configurations to automatically set up Google Cloud resources and deploy Dify in a highly available configuration. It includes features such as serverless hosting, auto-scaling, and data persistence. Users need a Google Cloud account, Terraform, and gcloud CLI installed to use this tool. The configuration involves setting environment-specific values and creating a GCS bucket for managing Terraform state. The tool allows users to initialize Terraform, create Artifact Registry repository, build and push container images, plan and apply Terraform changes, and cleanup resources when needed.
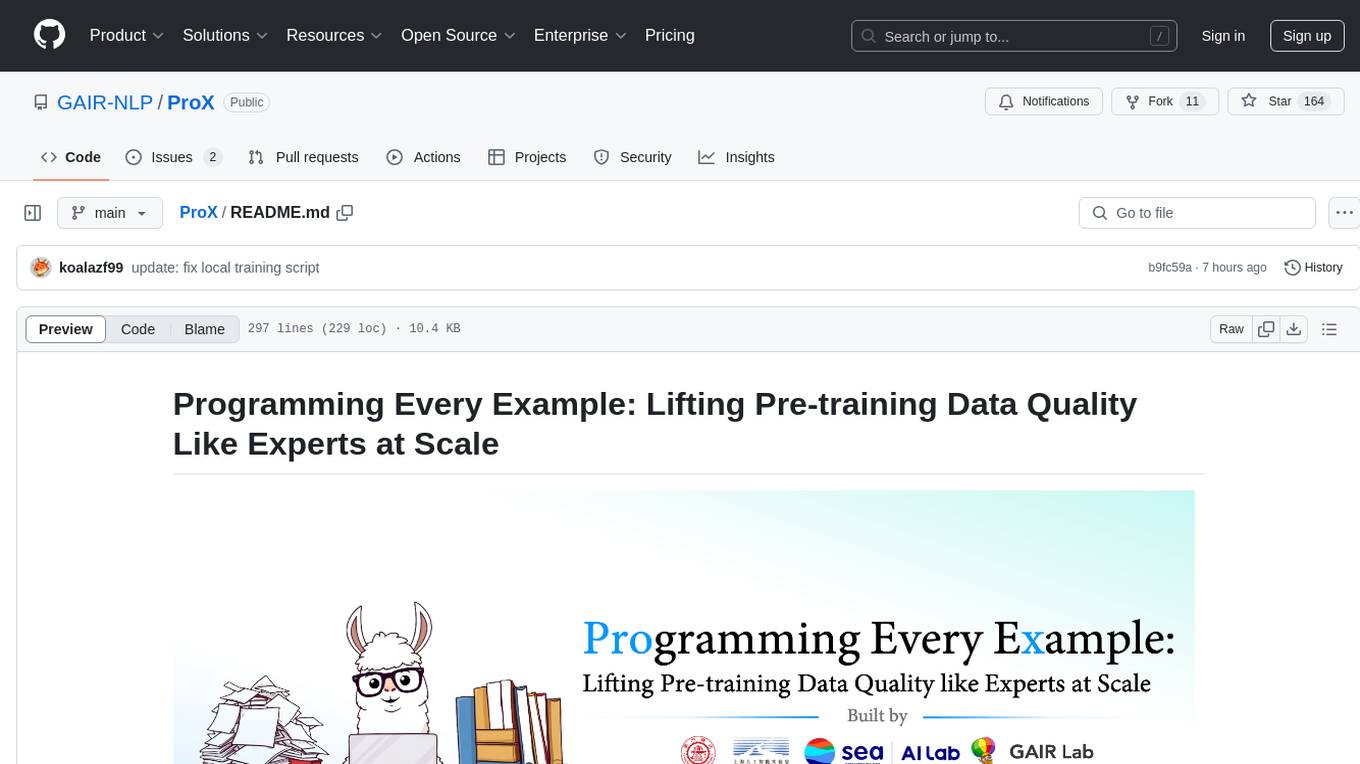
ProX
ProX is a lm-based data refinement framework that automates the process of cleaning and improving data used in pre-training large language models. It offers better performance, domain flexibility, efficiency, and cost-effectiveness compared to traditional methods. The framework has been shown to improve model performance by over 2% and boost accuracy by up to 20% in tasks like math. ProX is designed to refine data at scale without the need for manual adjustments, making it a valuable tool for data preprocessing in natural language processing tasks.
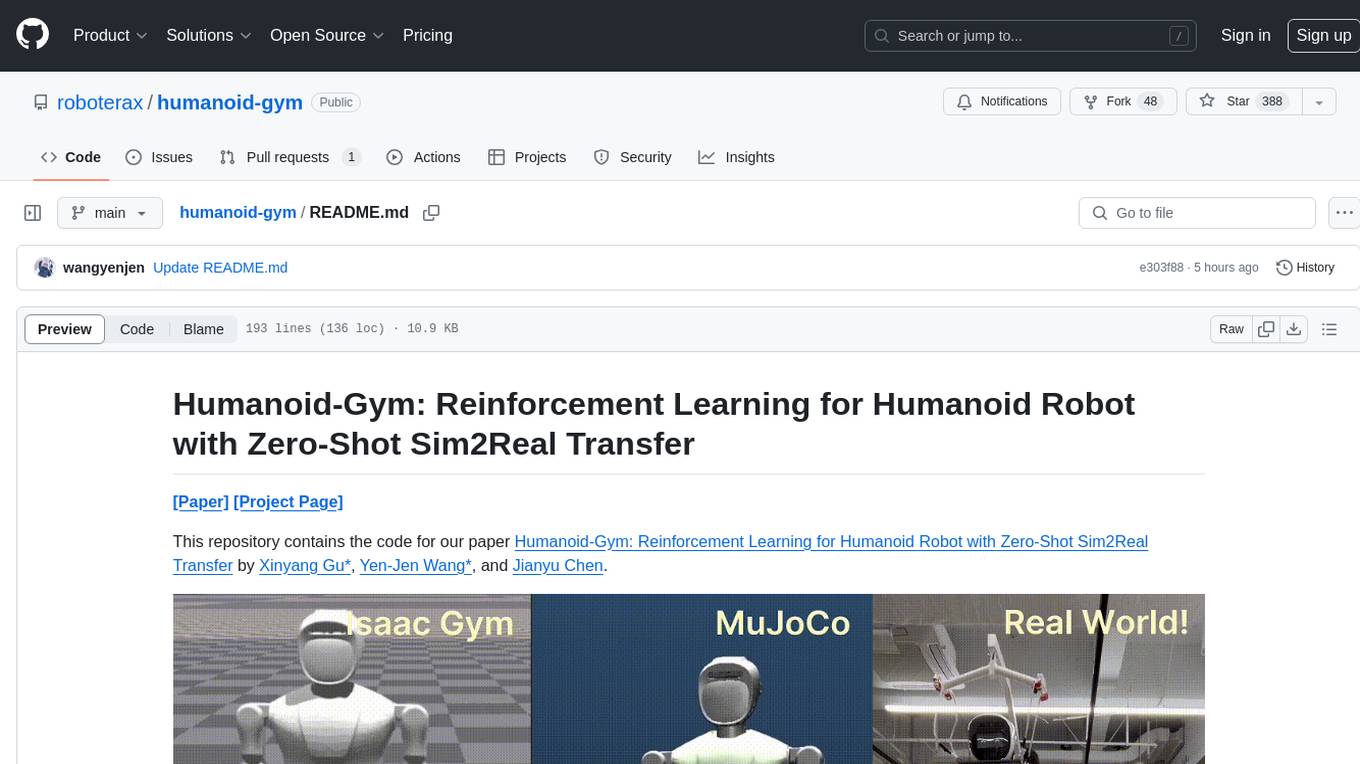
humanoid-gym
Humanoid-Gym is a reinforcement learning framework designed for training locomotion skills for humanoid robots, focusing on zero-shot transfer from simulation to real-world environments. It integrates a sim-to-sim framework from Isaac Gym to Mujoco for verifying trained policies in different physical simulations. The codebase is verified with RobotEra's XBot-S and XBot-L humanoid robots. It offers comprehensive training guidelines, step-by-step configuration instructions, and execution scripts for easy deployment. The sim2sim support allows transferring trained policies to accurate simulated environments. The upcoming features include Denoising World Model Learning and Dexterous Hand Manipulation. Installation and usage guides are provided along with examples for training PPO policies and sim-to-sim transformations. The code structure includes environment and configuration files, with instructions on adding new environments. Troubleshooting tips are provided for common issues, along with a citation and acknowledgment section.
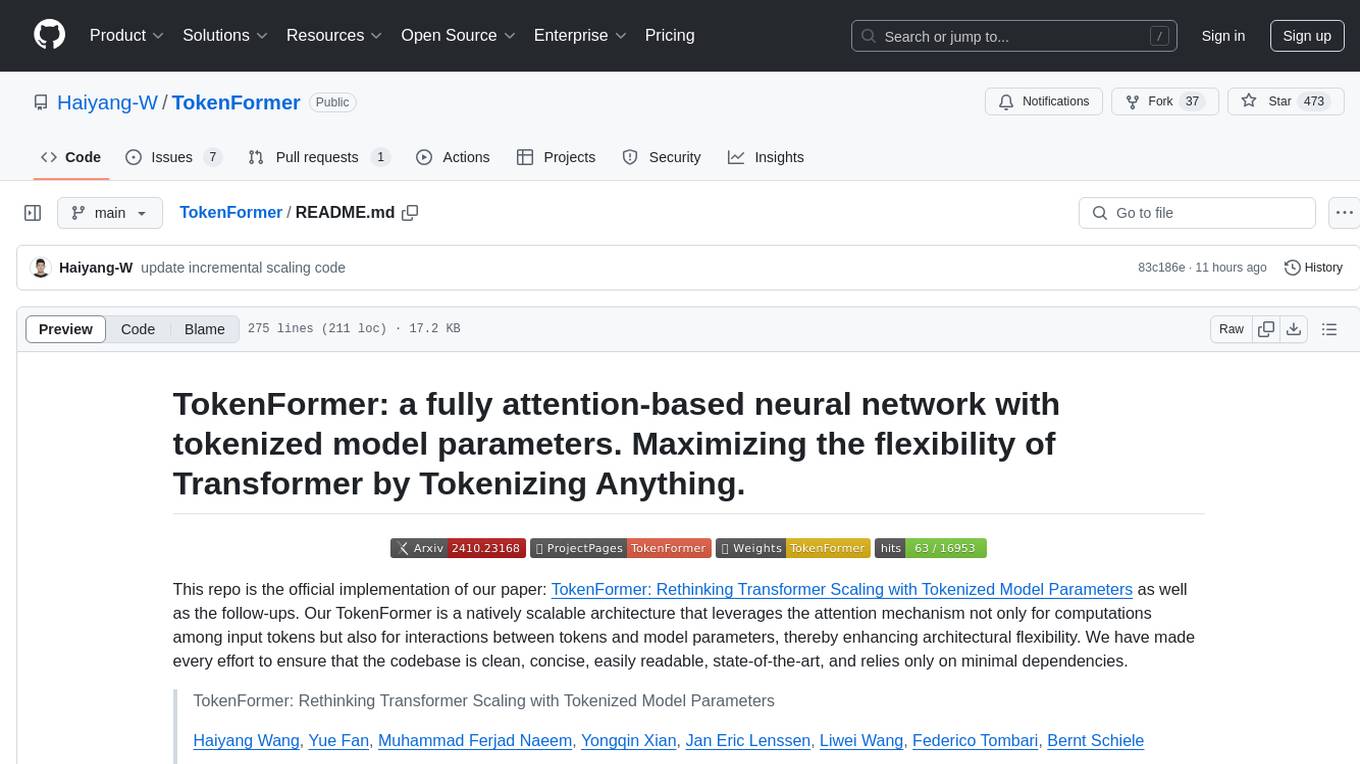
TokenFormer
TokenFormer is a fully attention-based neural network architecture that leverages tokenized model parameters to enhance architectural flexibility. It aims to maximize the flexibility of neural networks by unifying token-token and token-parameter interactions through the attention mechanism. The architecture allows for incremental model scaling and has shown promising results in language modeling and visual modeling tasks. The codebase is clean, concise, easily readable, state-of-the-art, and relies on minimal dependencies.
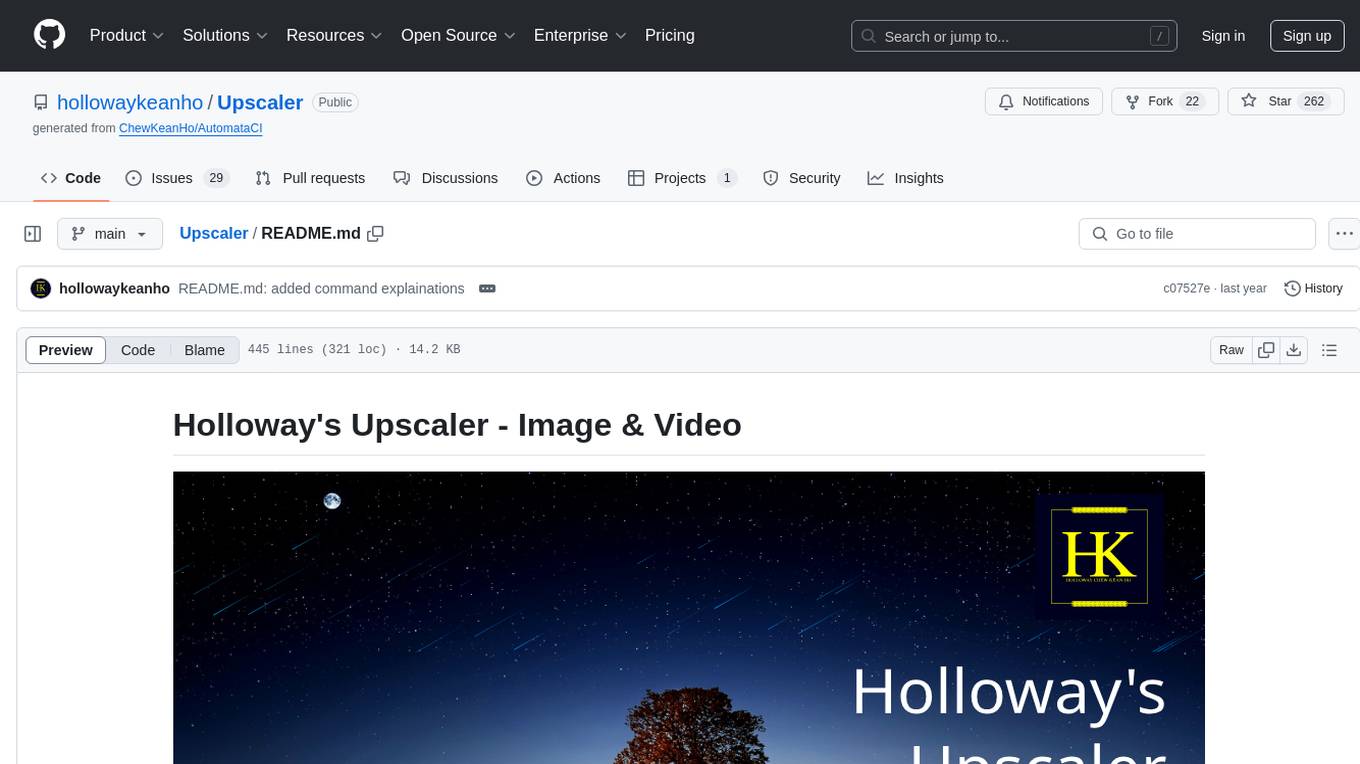
Upscaler
Holloway's Upscaler is a consolidation of various compiled open-source AI image/video upscaling products for a CLI-friendly image and video upscaling program. It provides low-cost AI upscaling software that can run locally on a laptop, programmable for albums and videos, reliable for large video files, and works without GUI overheads. The repository supports hardware testing on various systems and provides important notes on GPU compatibility, video types, and image decoding bugs. Dependencies include ffmpeg and ffprobe for video processing. The user manual covers installation, setup pathing, calling for help, upscaling images and videos, and contributing back to the project. Benchmarks are provided for performance evaluation on different hardware setups.
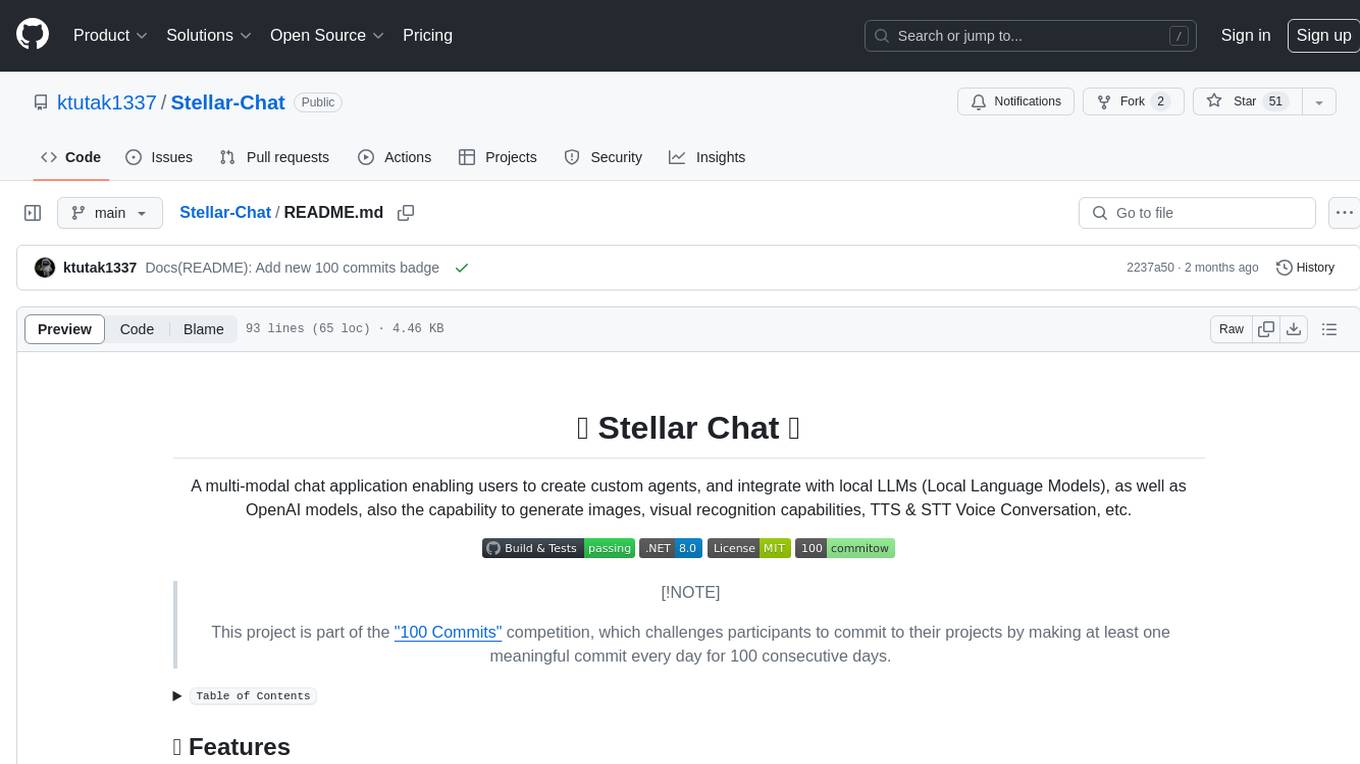
Stellar-Chat
Stellar Chat is a multi-modal chat application that enables users to create custom agents and integrate with local language models and OpenAI models. It provides capabilities for generating images, visual recognition, text-to-speech, and speech-to-text functionalities. Users can engage in multimodal conversations, create custom agents, search messages and conversations, and integrate with various applications for enhanced productivity. The project is part of the '100 Commits' competition, challenging participants to make meaningful commits daily for 100 consecutive days.
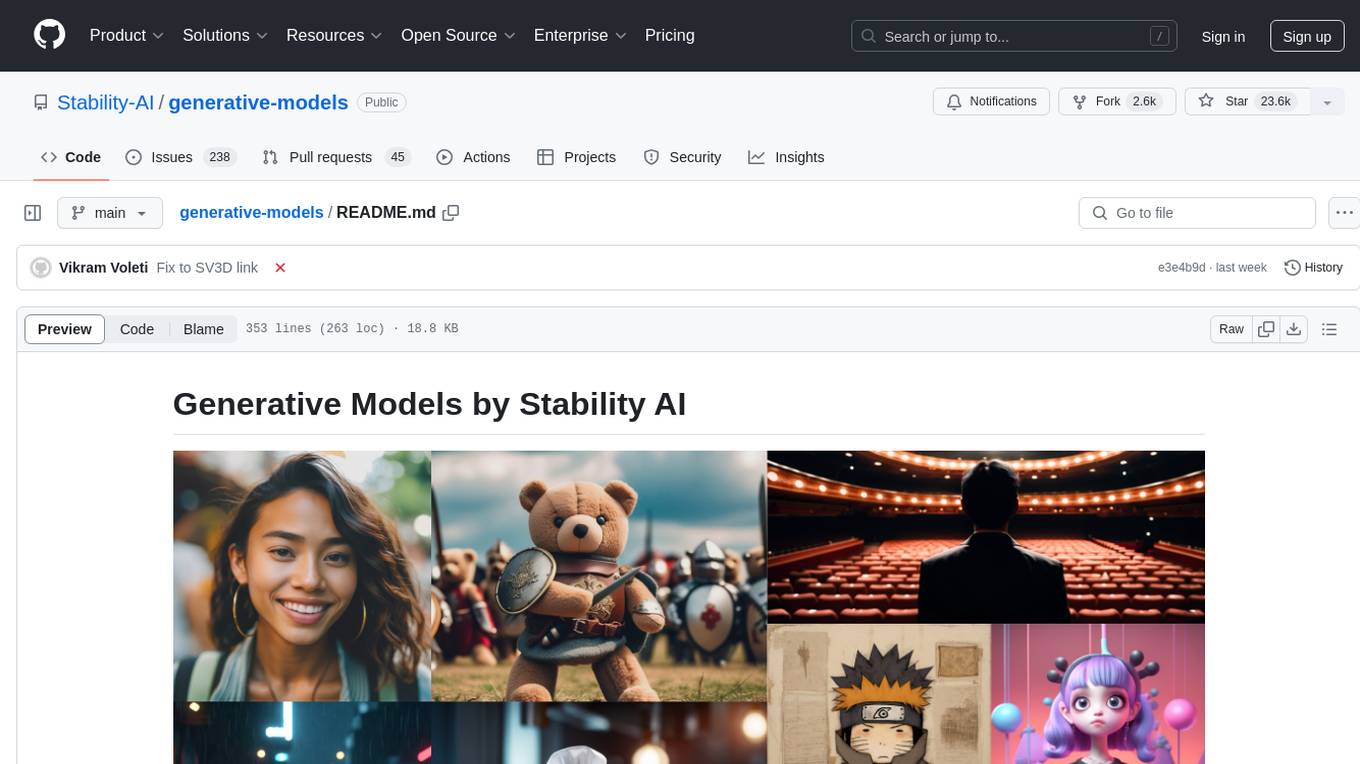
generative-models
Generative Models by Stability AI is a repository that provides various generative models for research purposes. It includes models like Stable Video 4D (SV4D) for video synthesis, Stable Video 3D (SV3D) for multi-view synthesis, SDXL-Turbo for text-to-image generation, and more. The repository focuses on modularity and implements a config-driven approach for building and combining submodules. It supports training with PyTorch Lightning and offers inference demos for different models. Users can access pre-trained models like SDXL-base-1.0 and SDXL-refiner-1.0 under a CreativeML Open RAIL++-M license. The codebase also includes tools for invisible watermark detection in generated images.
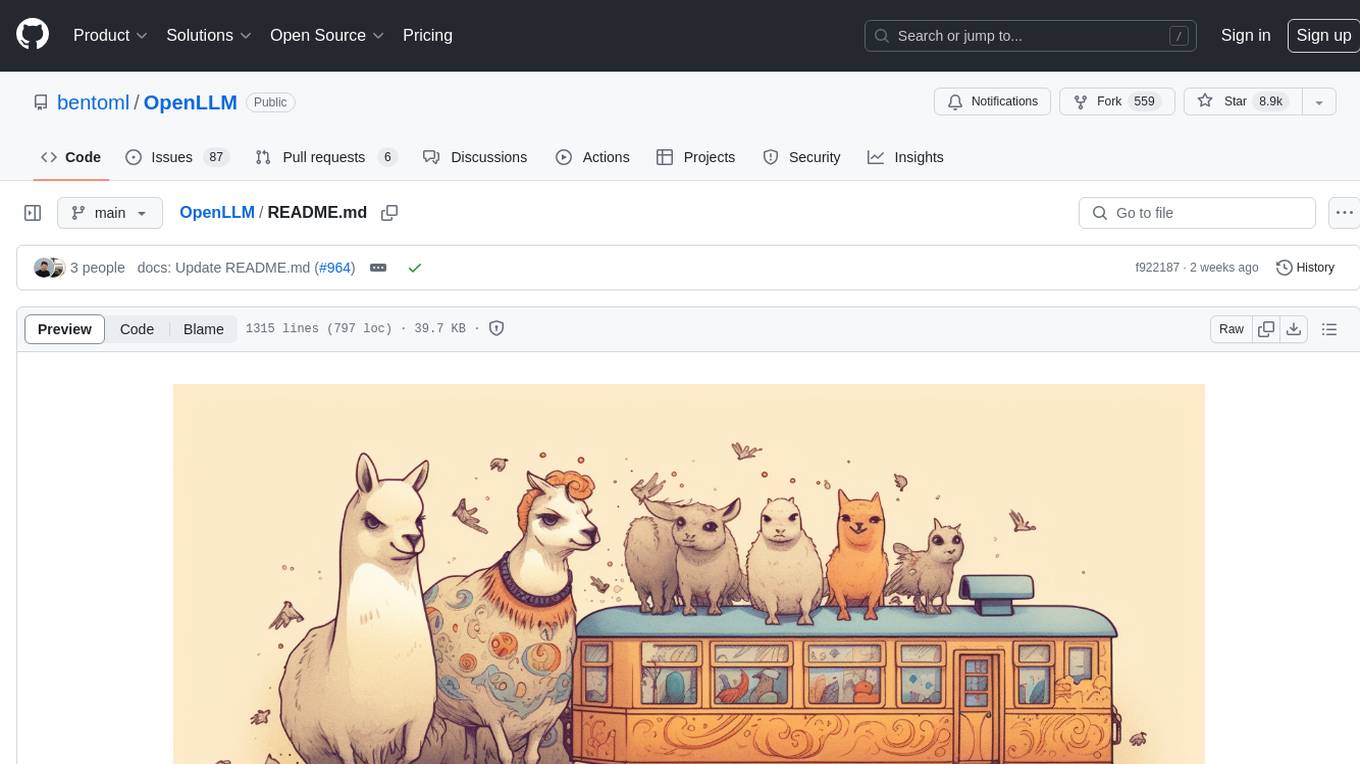
OpenLLM
OpenLLM is a platform that helps developers run any open-source Large Language Models (LLMs) as OpenAI-compatible API endpoints, locally and in the cloud. It supports a wide range of LLMs, provides state-of-the-art serving and inference performance, and simplifies cloud deployment via BentoML. Users can fine-tune, serve, deploy, and monitor any LLMs with ease using OpenLLM. The platform also supports various quantization techniques, serving fine-tuning layers, and multiple runtime implementations. OpenLLM seamlessly integrates with other tools like OpenAI Compatible Endpoints, LlamaIndex, LangChain, and Transformers Agents. It offers deployment options through Docker containers, BentoCloud, and provides a community for collaboration and contributions.
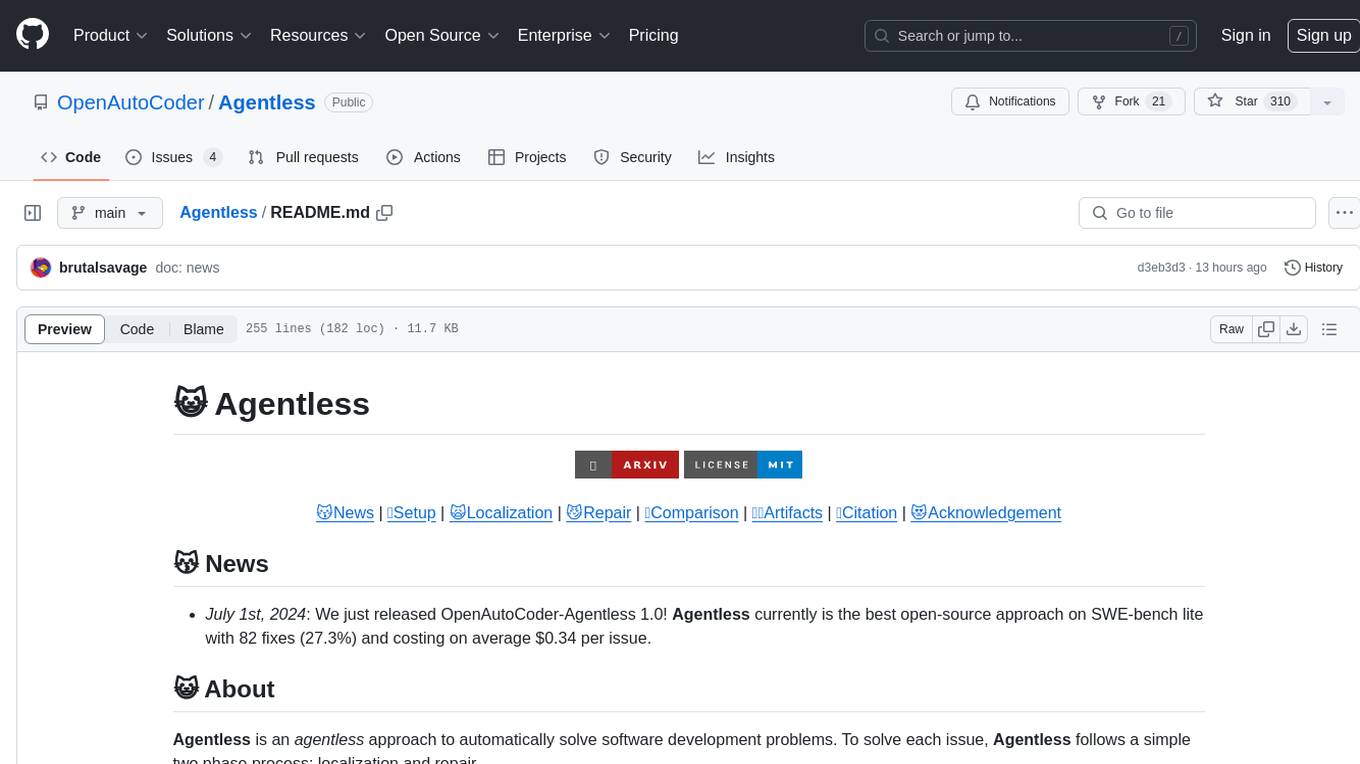
Agentless
Agentless is an open-source tool designed for automatically solving software development problems. It follows a two-phase process of localization and repair to identify faults in specific files, classes, and functions, and generate candidate patches for fixing issues. The tool is aimed at simplifying the software development process by automating issue resolution and patch generation.
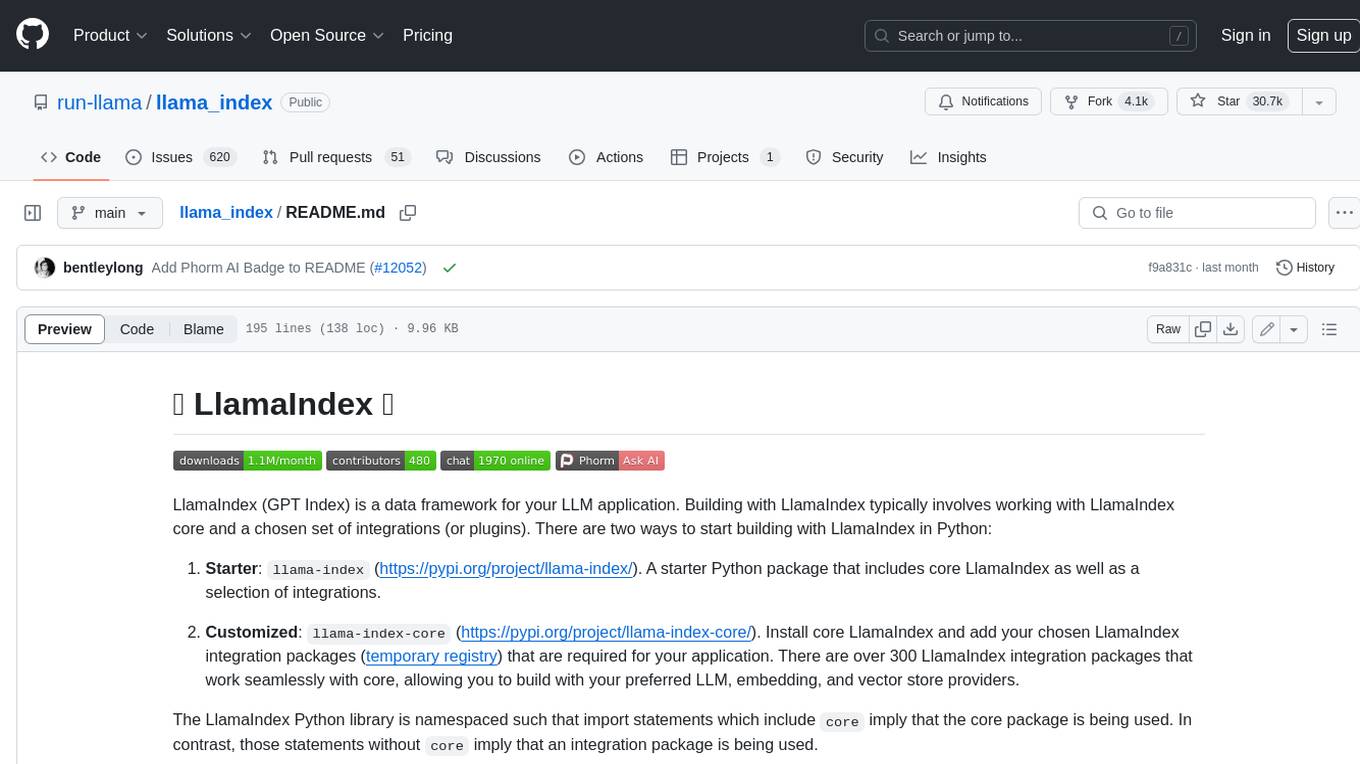
llama_index
LlamaIndex is a data framework for building LLM applications. It provides tools for ingesting, structuring, and querying data, as well as integrating with LLMs and other tools. LlamaIndex is designed to be easy to use for both beginner and advanced users, and it provides a comprehensive set of features for building LLM applications.
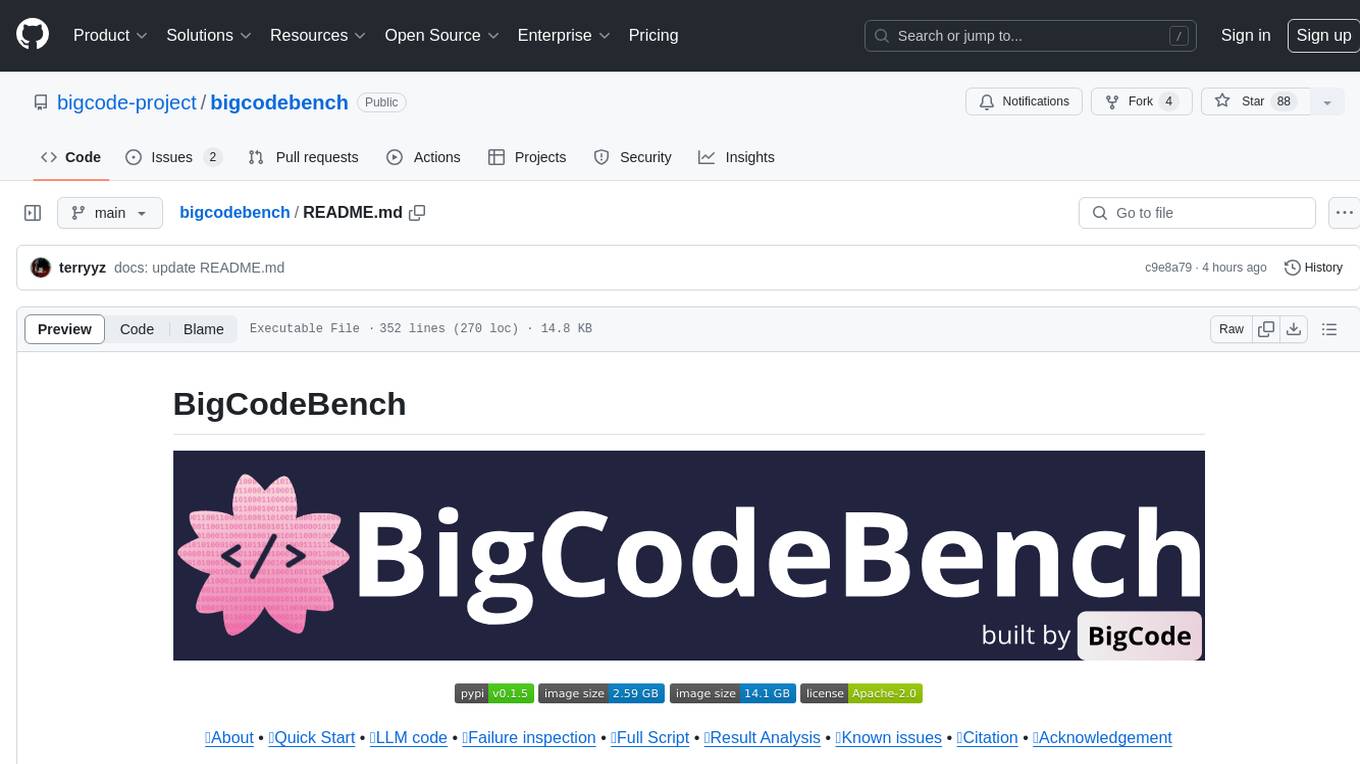
bigcodebench
BigCodeBench is an easy-to-use benchmark for code generation with practical and challenging programming tasks. It aims to evaluate the true programming capabilities of large language models (LLMs) in a more realistic setting. The benchmark is designed for HumanEval-like function-level code generation tasks, but with much more complex instructions and diverse function calls. BigCodeBench focuses on the evaluation of LLM4Code with diverse function calls and complex instructions, providing precise evaluation & ranking and pre-generated samples to accelerate code intelligence research. It inherits the design of the EvalPlus framework but differs in terms of execution environment and test evaluation.
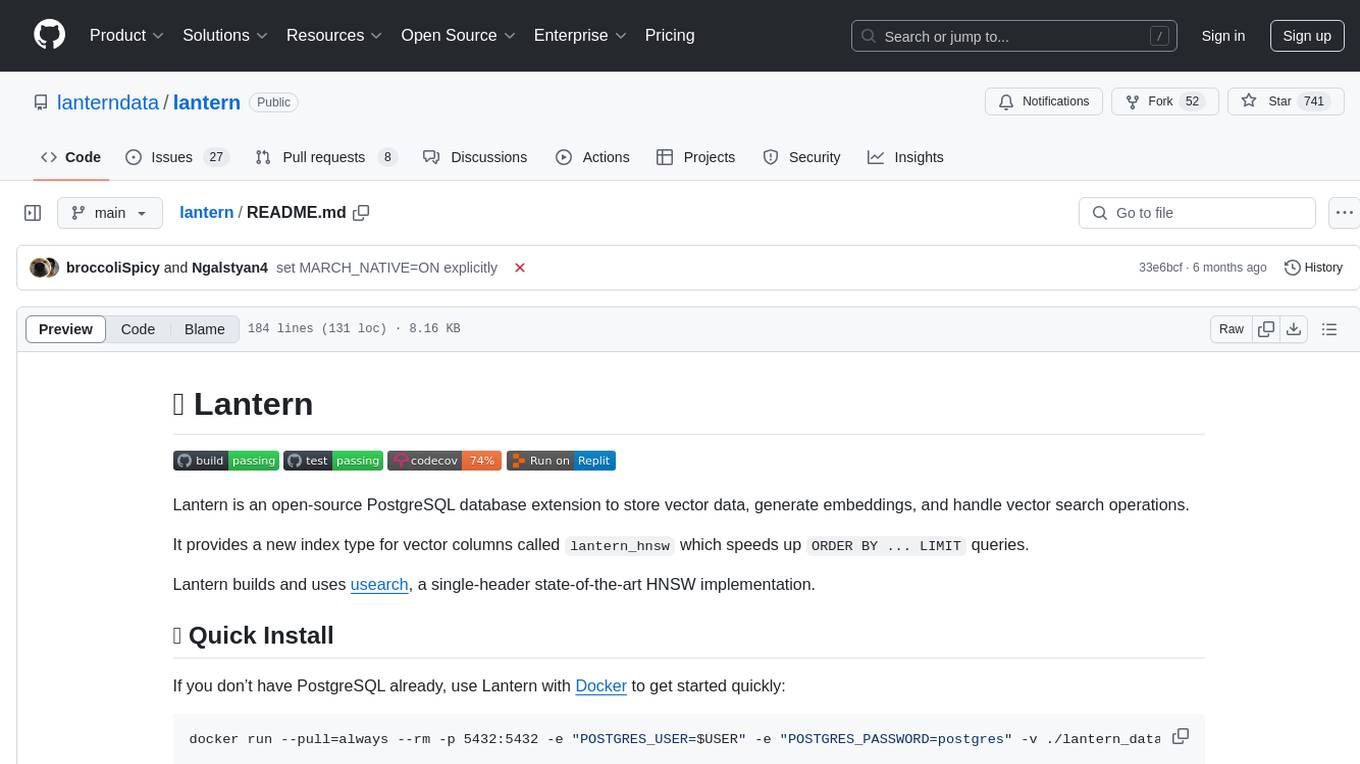
lantern
Lantern is an open-source PostgreSQL database extension designed to store vector data, generate embeddings, and handle vector search operations efficiently. It introduces a new index type called 'lantern_hnsw' for vector columns, which speeds up 'ORDER BY ... LIMIT' queries. Lantern utilizes the state-of-the-art HNSW implementation called usearch. Users can easily install Lantern using Docker, Homebrew, or precompiled binaries. The tool supports various distance functions, index construction parameters, and operator classes for efficient querying. Lantern offers features like embedding generation, interoperability with pgvector, parallel index creation, and external index graph generation. It aims to provide superior performance metrics compared to other similar tools and has a roadmap for future enhancements such as cloud-hosted version, hardware-accelerated distance metrics, industry-specific application templates, and support for version control and A/B testing of embeddings.
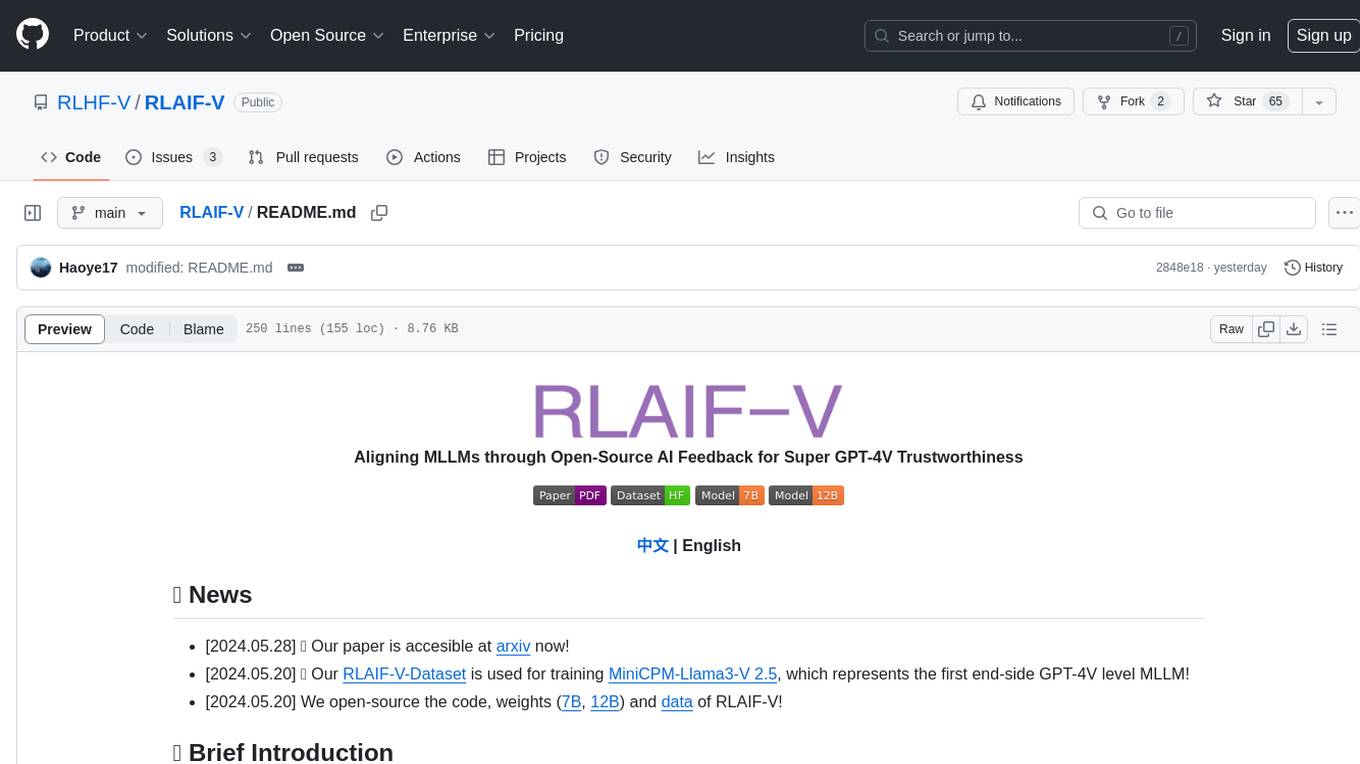
RLAIF-V
RLAIF-V is a novel framework that aligns MLLMs in a fully open-source paradigm for super GPT-4V trustworthiness. It maximally exploits open-source feedback from high-quality feedback data and online feedback learning algorithm. Notable features include achieving super GPT-4V trustworthiness in both generative and discriminative tasks, using high-quality generalizable feedback data to reduce hallucination of different MLLMs, and exhibiting better learning efficiency and higher performance through iterative alignment.
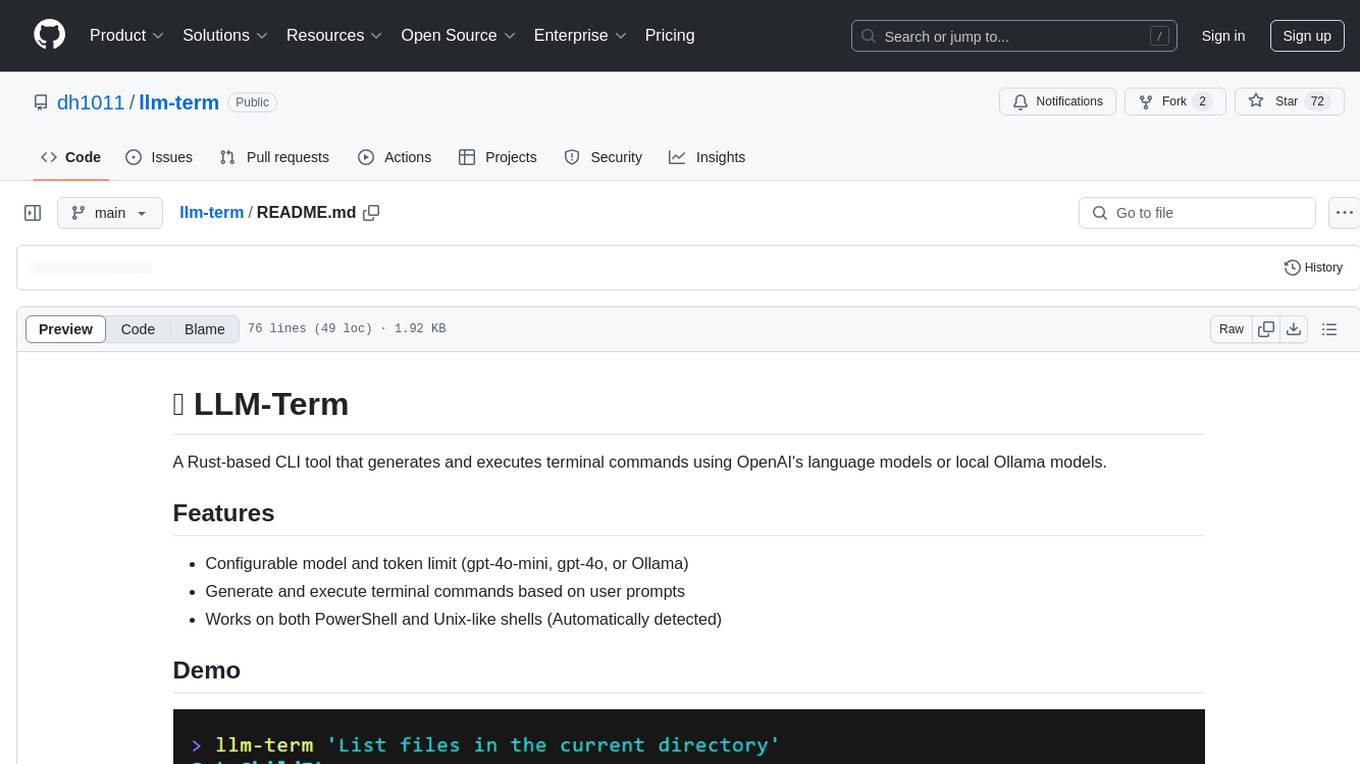
llm-term
LLM-Term is a Rust-based CLI tool that generates and executes terminal commands using OpenAI's language models or local Ollama models. It offers configurable model and token limits, works on both PowerShell and Unix-like shells, and provides a seamless user experience for generating commands based on prompts. Users can easily set up the tool, customize configurations, and leverage different models for command generation.
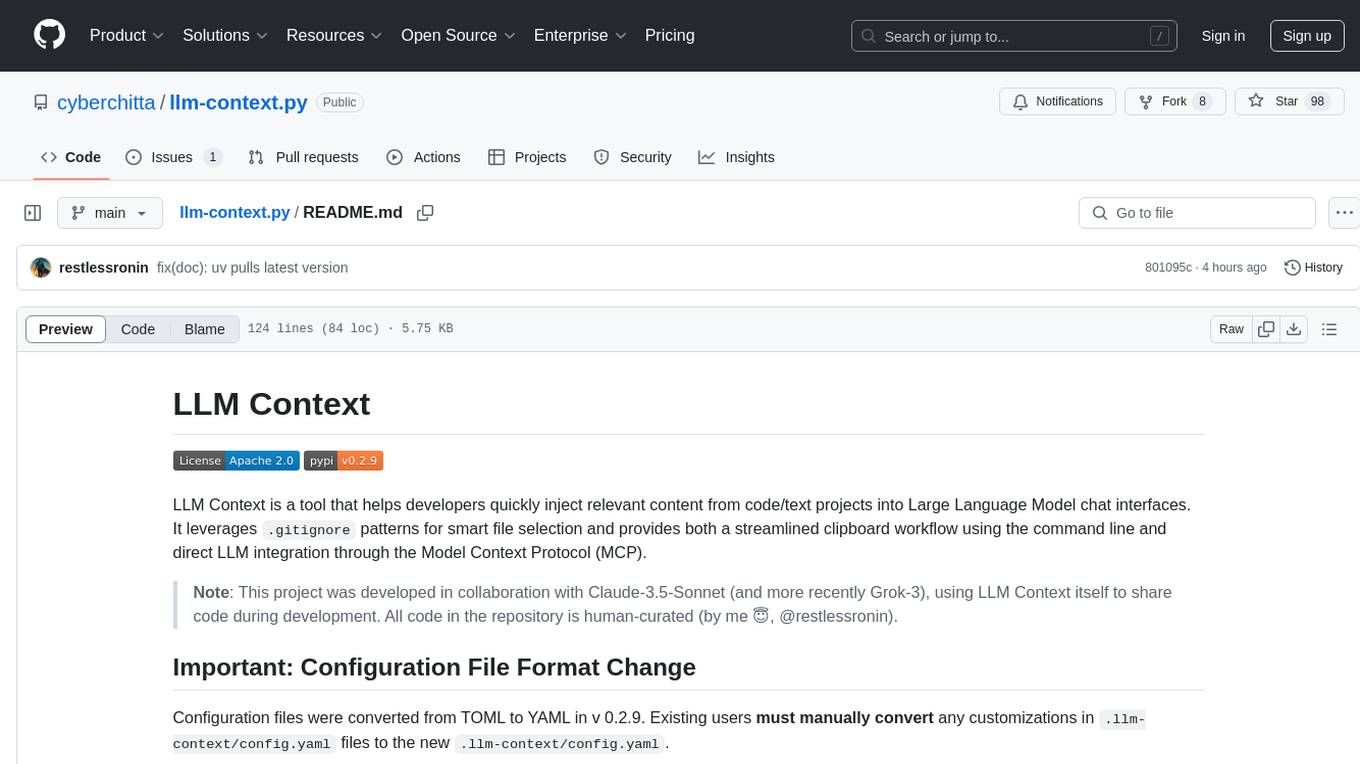
llm-context.py
LLM Context is a tool designed to assist developers in quickly injecting relevant content from code/text projects into Large Language Model chat interfaces. It leverages `.gitignore` patterns for smart file selection and offers a streamlined clipboard workflow using the command line. The tool also provides direct integration with Large Language Models through the Model Context Protocol (MCP). LLM Context is optimized for code repositories and collections of text/markdown/html documents, making it suitable for developers working on projects that fit within an LLM's context window. The tool is under active development and aims to enhance AI-assisted development workflows by harnessing the power of Large Language Models.
For similar tasks
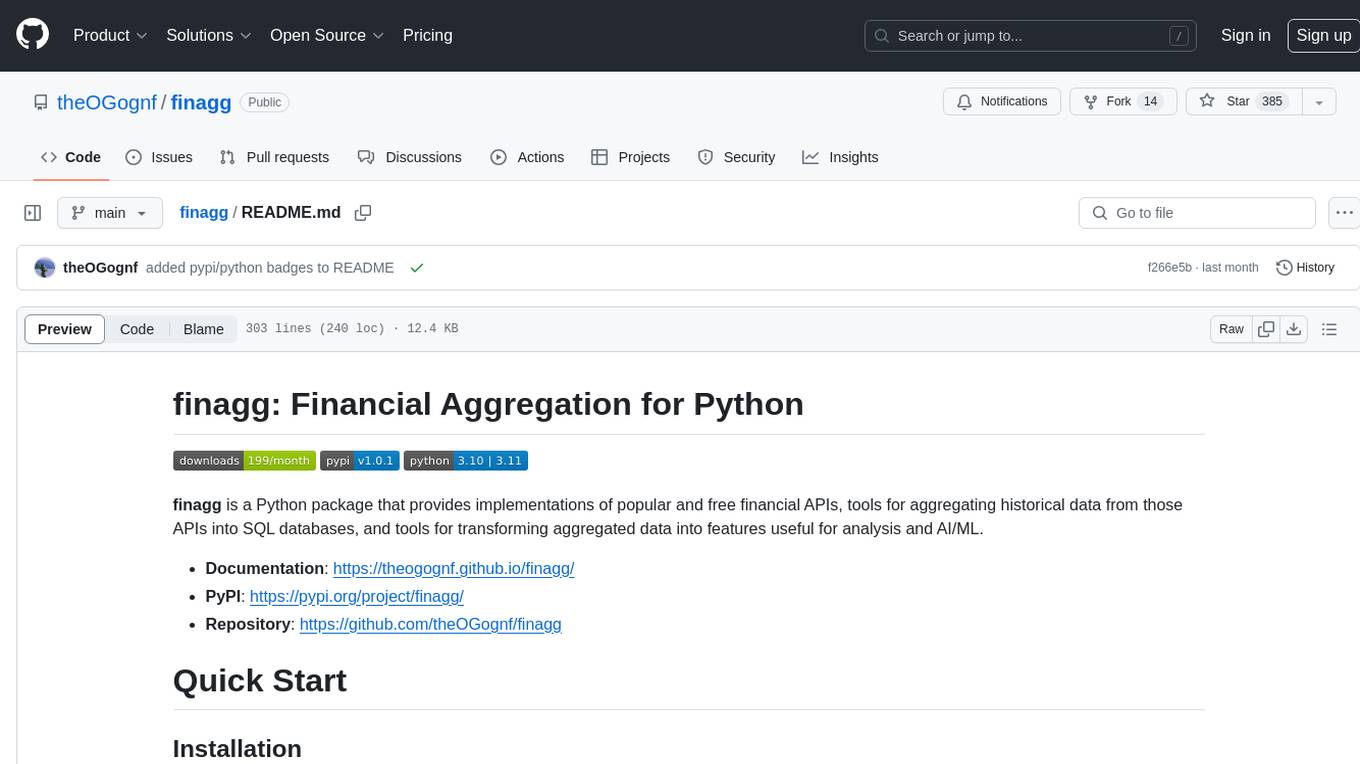
finagg
finagg is a Python package that provides implementations of popular and free financial APIs, tools for aggregating historical data from those APIs into SQL databases, and tools for transforming aggregated data into features useful for analysis and AI/ML. It offers documentation, installation instructions, and basic usage examples for exploring various financial APIs and features. Users can install recommended datasets from 3rd party APIs into a local SQL database, access Bureau of Economic Analysis (BEA) data, Federal Reserve Economic Data (FRED), Securities and Exchange Commission (SEC) filings, and more. The package also allows users to explore raw data features, install refined data features, and perform refined aggregations of raw data. Configuration options for API keys, user agents, and data locations are provided, along with information on dependencies and related projects.
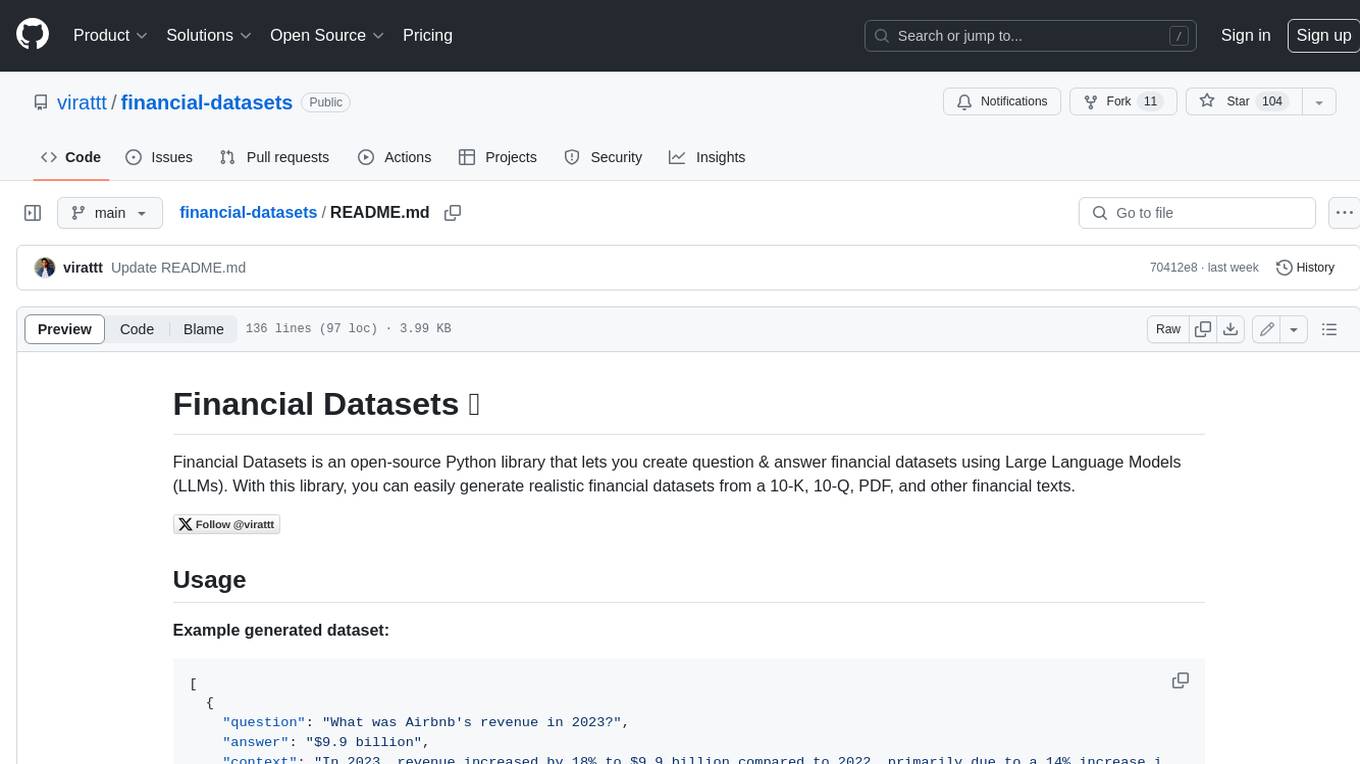
financial-datasets
Financial Datasets is an open-source Python library that allows users to create question and answer financial datasets using Large Language Models (LLMs). With this library, users can easily generate realistic financial datasets from 10-K, 10-Q, PDF, and other financial texts. The library provides three main methods for generating datasets: from any text, from a 10-K filing, or from a PDF URL. Financial Datasets can be used for a variety of tasks, including financial analysis, research, and education.
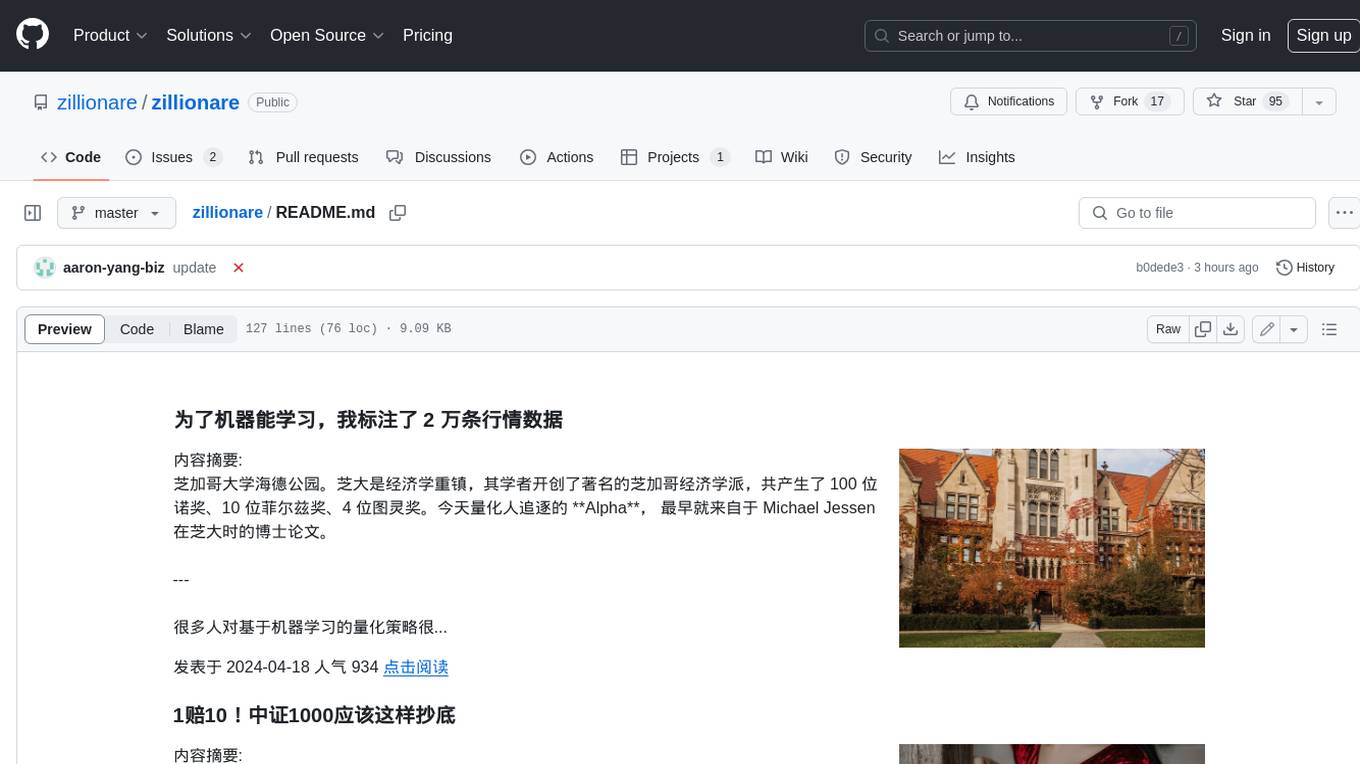
zillionare
This repository contains a collection of articles and tutorials on quantitative finance, including topics such as machine learning, statistical arbitrage, and risk management. The articles are written in a clear and concise style, and they are suitable for both beginners and experienced practitioners. The repository also includes a number of Jupyter notebooks that demonstrate how to use Python for quantitative finance.
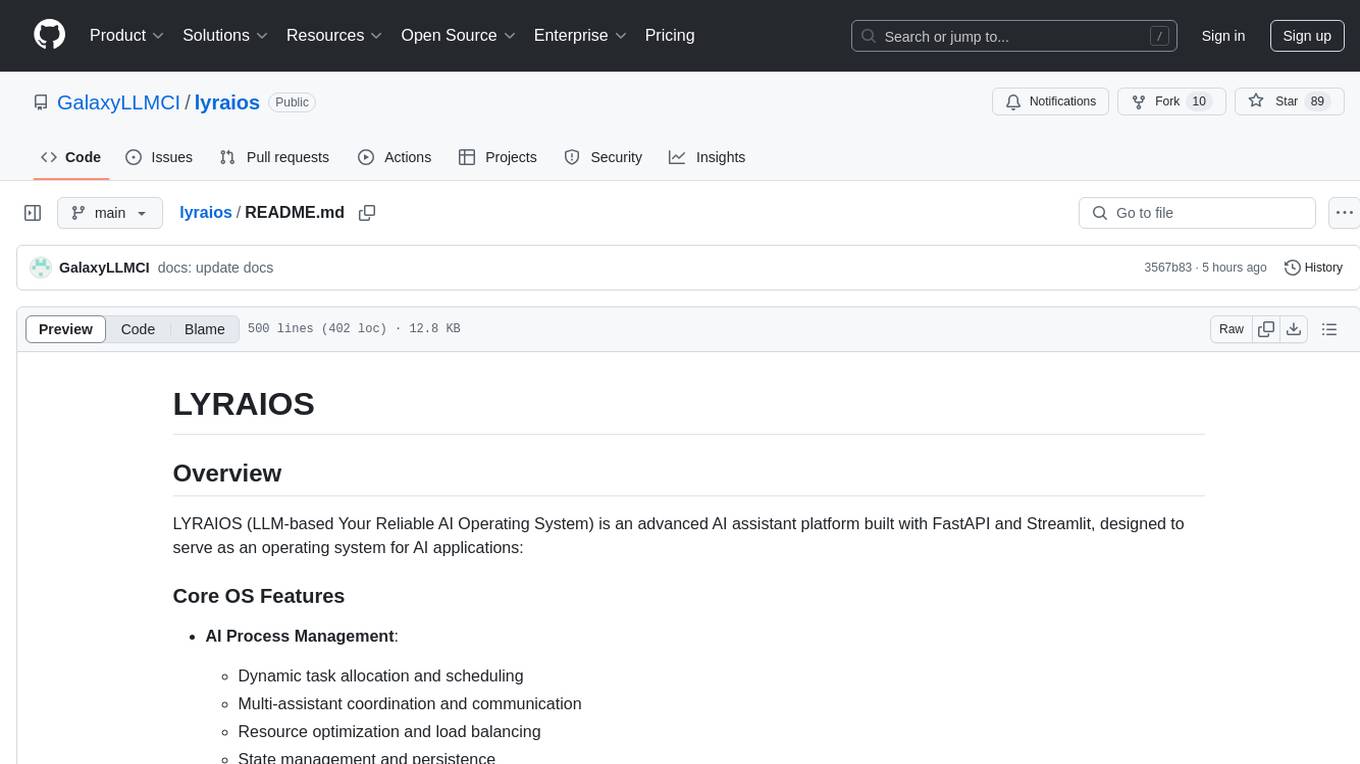
lyraios
LYRAIOS (LLM-based Your Reliable AI Operating System) is an advanced AI assistant platform built with FastAPI and Streamlit, designed to serve as an operating system for AI applications. It offers core features such as AI process management, memory system, and I/O system. The platform includes built-in tools like Calculator, Web Search, Financial Analysis, File Management, and Research Tools. It also provides specialized assistant teams for Python and research tasks. LYRAIOS is built on a technical architecture comprising FastAPI backend, Streamlit frontend, Vector Database, PostgreSQL storage, and Docker support. It offers features like knowledge management, process control, and security & access control. The roadmap includes enhancements in core platform, AI process management, memory system, tools & integrations, security & access control, open protocol architecture, multi-agent collaboration, and cross-platform support.
For similar jobs
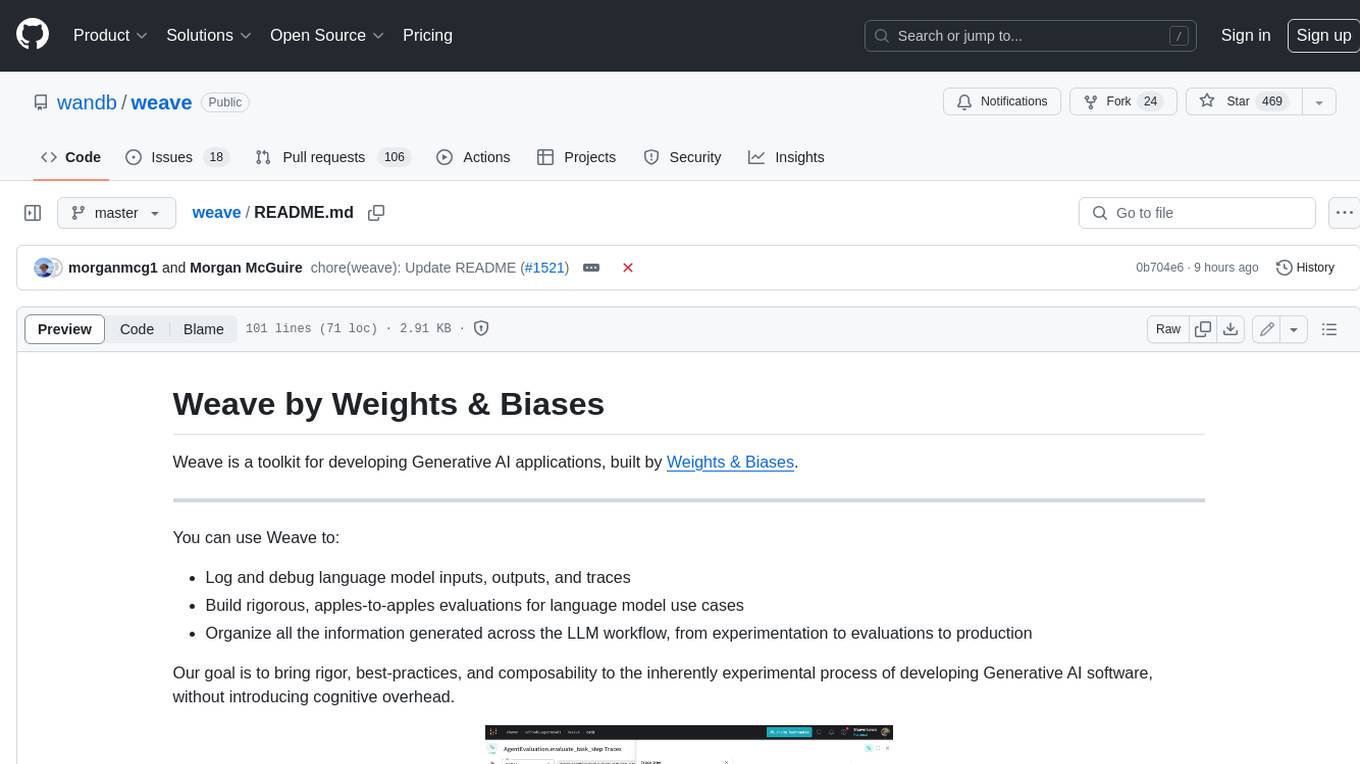
weave
Weave is a toolkit for developing Generative AI applications, built by Weights & Biases. With Weave, you can log and debug language model inputs, outputs, and traces; build rigorous, apples-to-apples evaluations for language model use cases; and organize all the information generated across the LLM workflow, from experimentation to evaluations to production. Weave aims to bring rigor, best-practices, and composability to the inherently experimental process of developing Generative AI software, without introducing cognitive overhead.
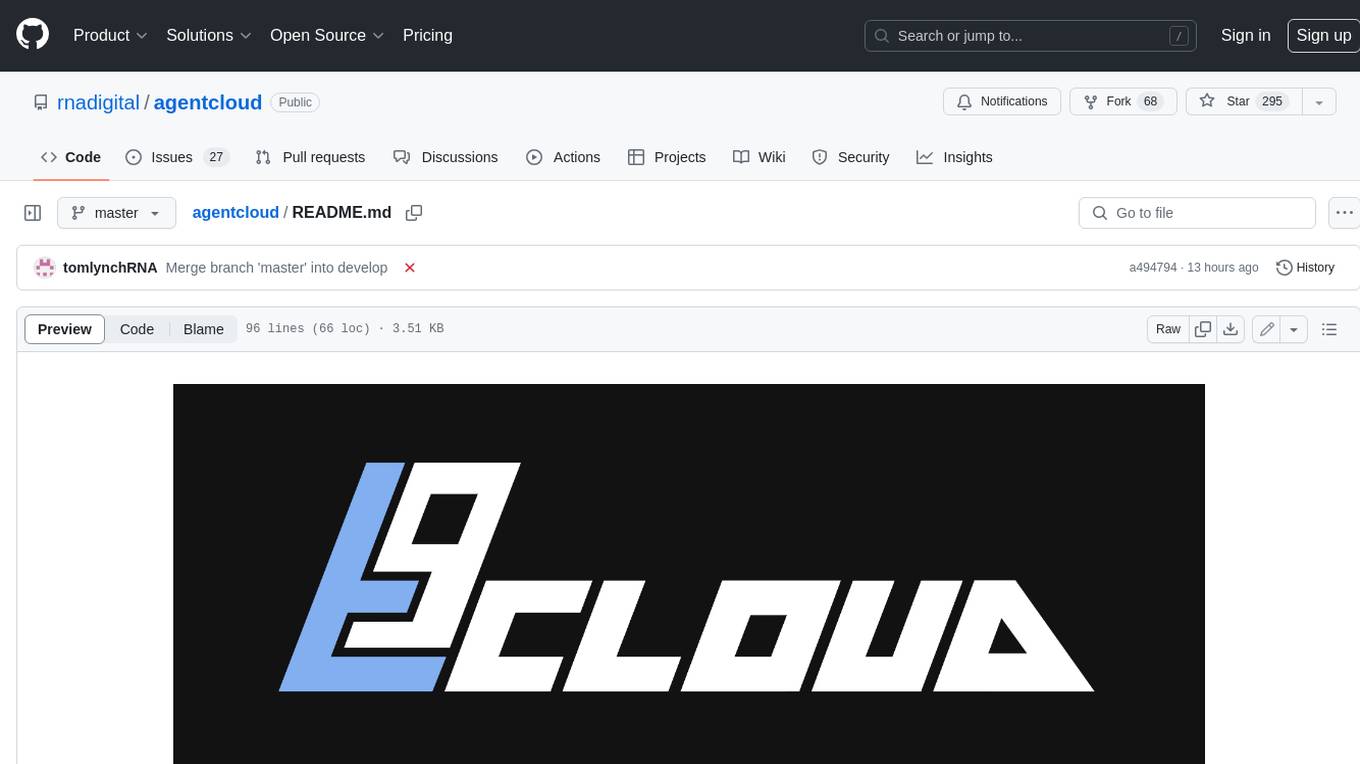
agentcloud
AgentCloud is an open-source platform that enables companies to build and deploy private LLM chat apps, empowering teams to securely interact with their data. It comprises three main components: Agent Backend, Webapp, and Vector Proxy. To run this project locally, clone the repository, install Docker, and start the services. The project is licensed under the GNU Affero General Public License, version 3 only. Contributions and feedback are welcome from the community.
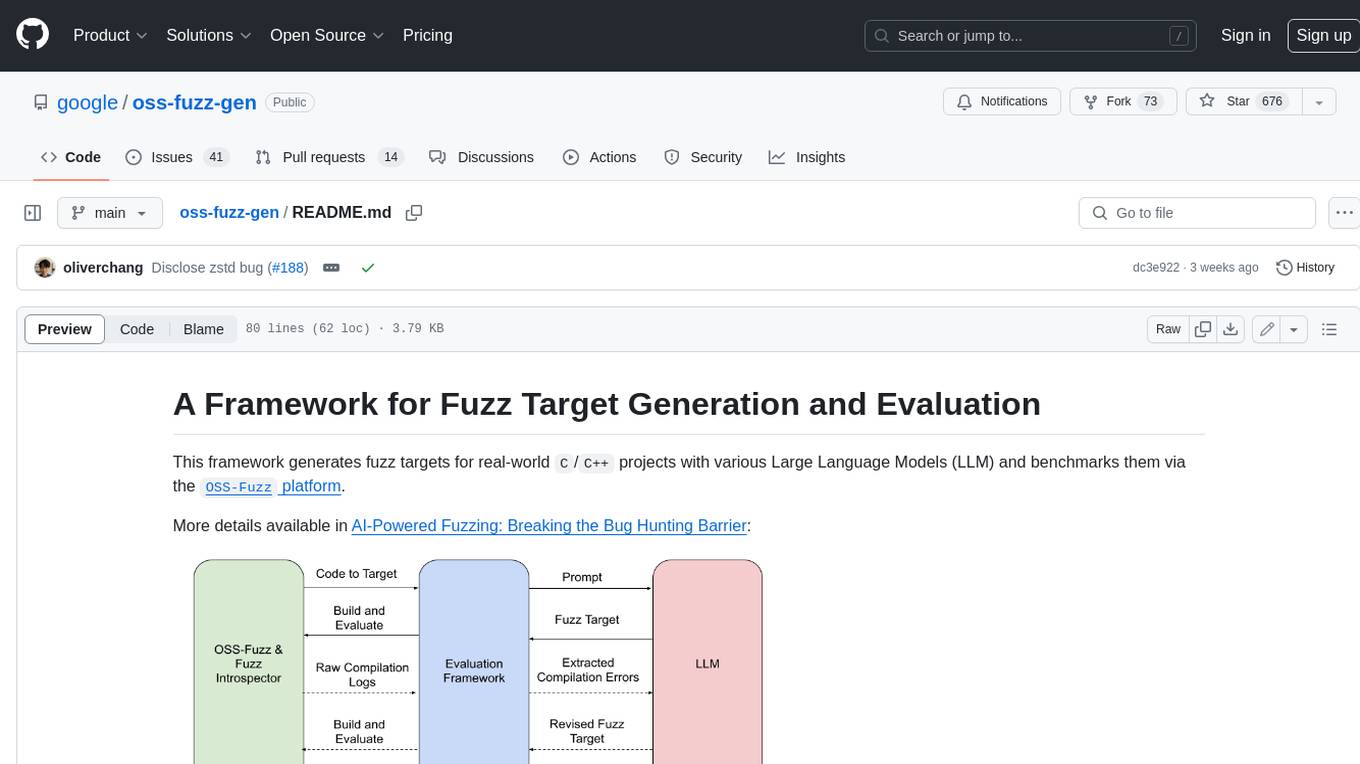
oss-fuzz-gen
This framework generates fuzz targets for real-world `C`/`C++` projects with various Large Language Models (LLM) and benchmarks them via the `OSS-Fuzz` platform. It manages to successfully leverage LLMs to generate valid fuzz targets (which generate non-zero coverage increase) for 160 C/C++ projects. The maximum line coverage increase is 29% from the existing human-written targets.
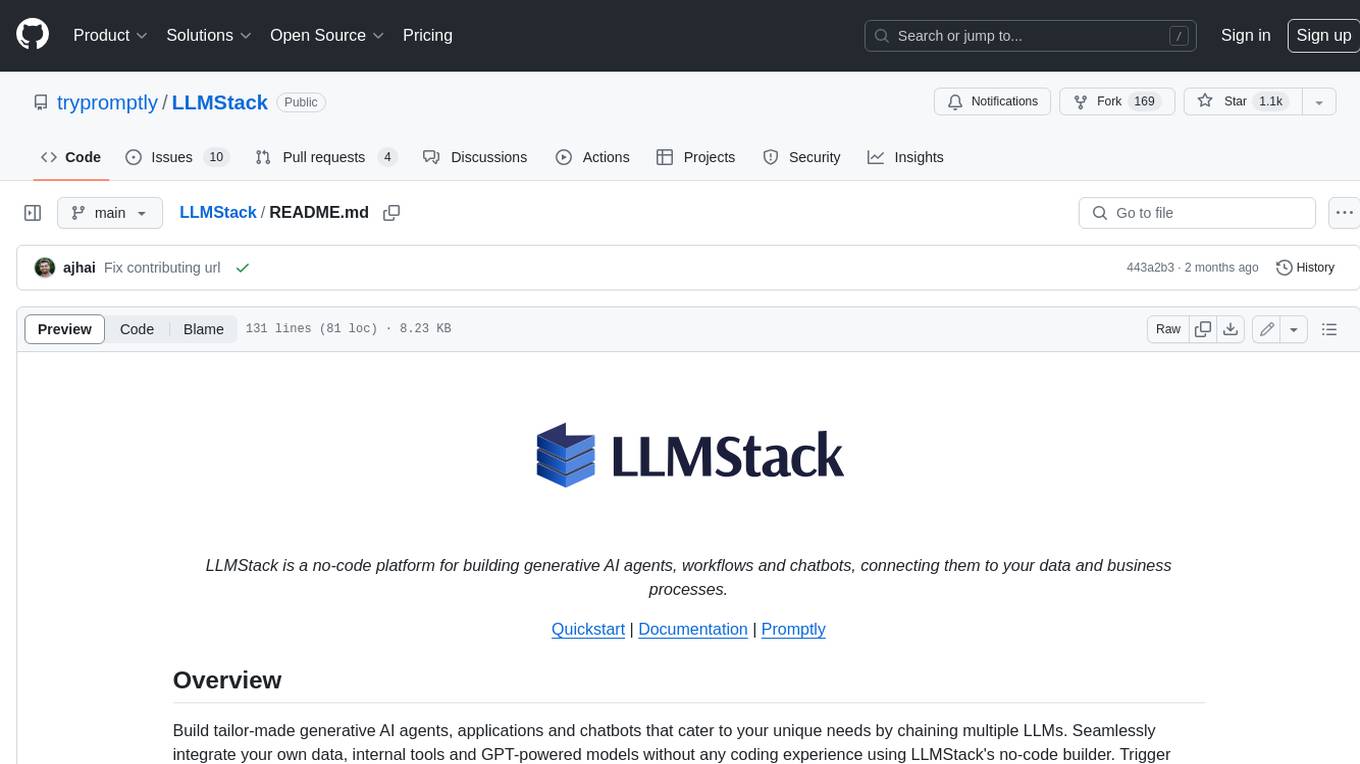
LLMStack
LLMStack is a no-code platform for building generative AI agents, workflows, and chatbots. It allows users to connect their own data, internal tools, and GPT-powered models without any coding experience. LLMStack can be deployed to the cloud or on-premise and can be accessed via HTTP API or triggered from Slack or Discord.
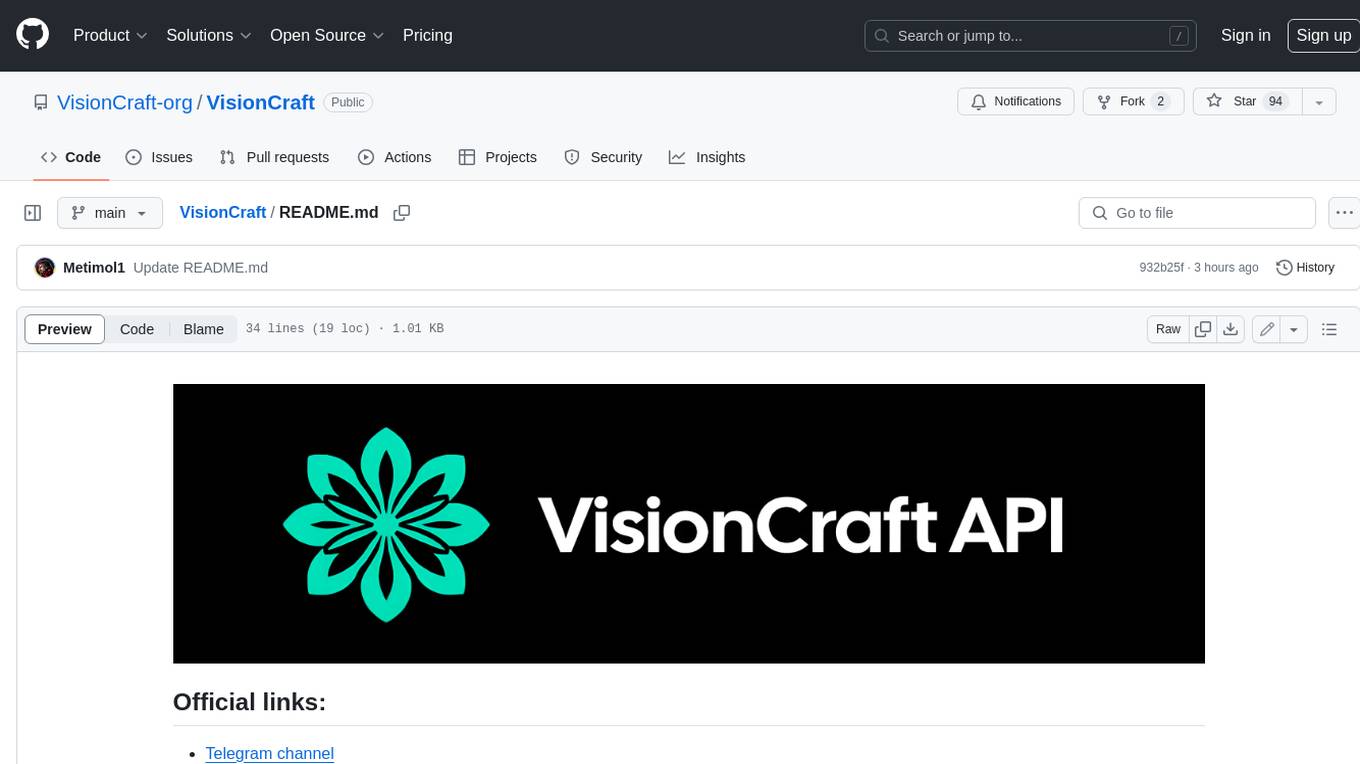
VisionCraft
The VisionCraft API is a free API for using over 100 different AI models. From images to sound.
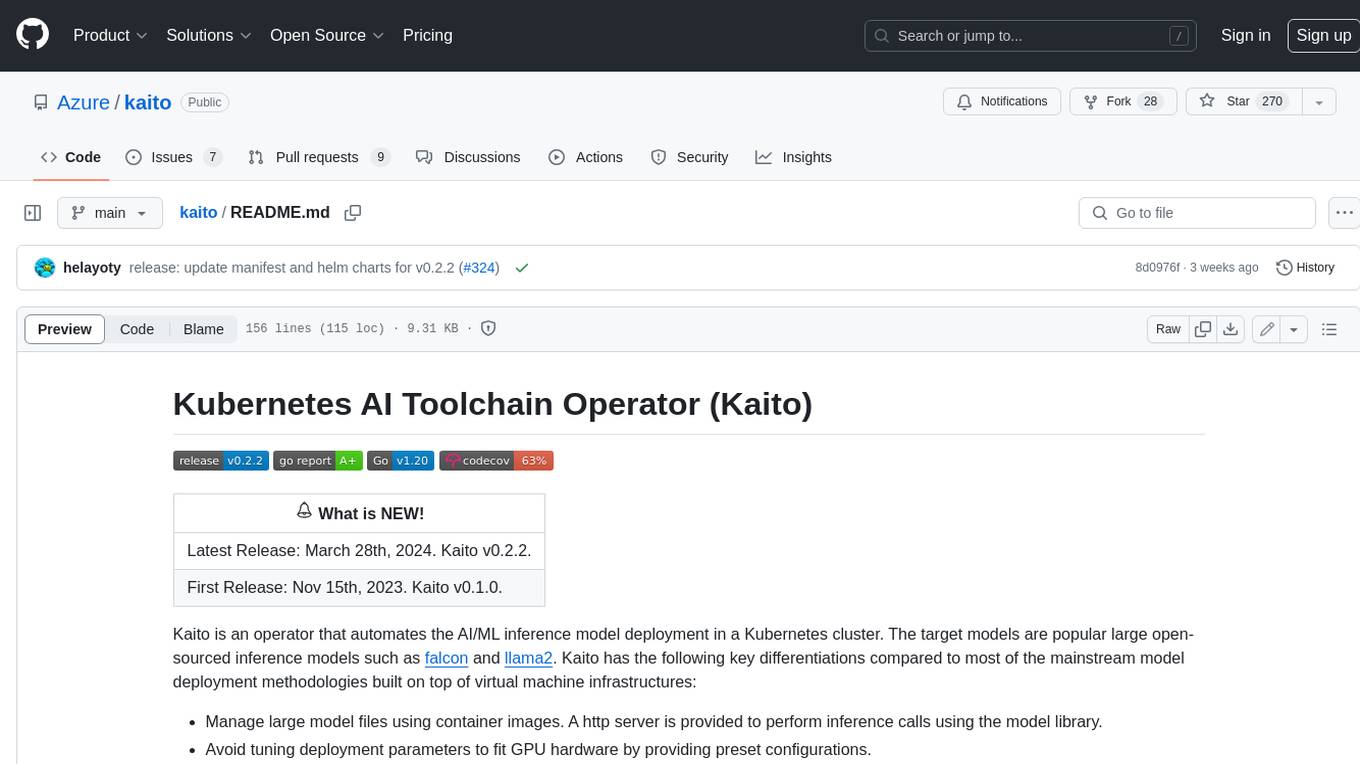
kaito
Kaito is an operator that automates the AI/ML inference model deployment in a Kubernetes cluster. It manages large model files using container images, avoids tuning deployment parameters to fit GPU hardware by providing preset configurations, auto-provisions GPU nodes based on model requirements, and hosts large model images in the public Microsoft Container Registry (MCR) if the license allows. Using Kaito, the workflow of onboarding large AI inference models in Kubernetes is largely simplified.
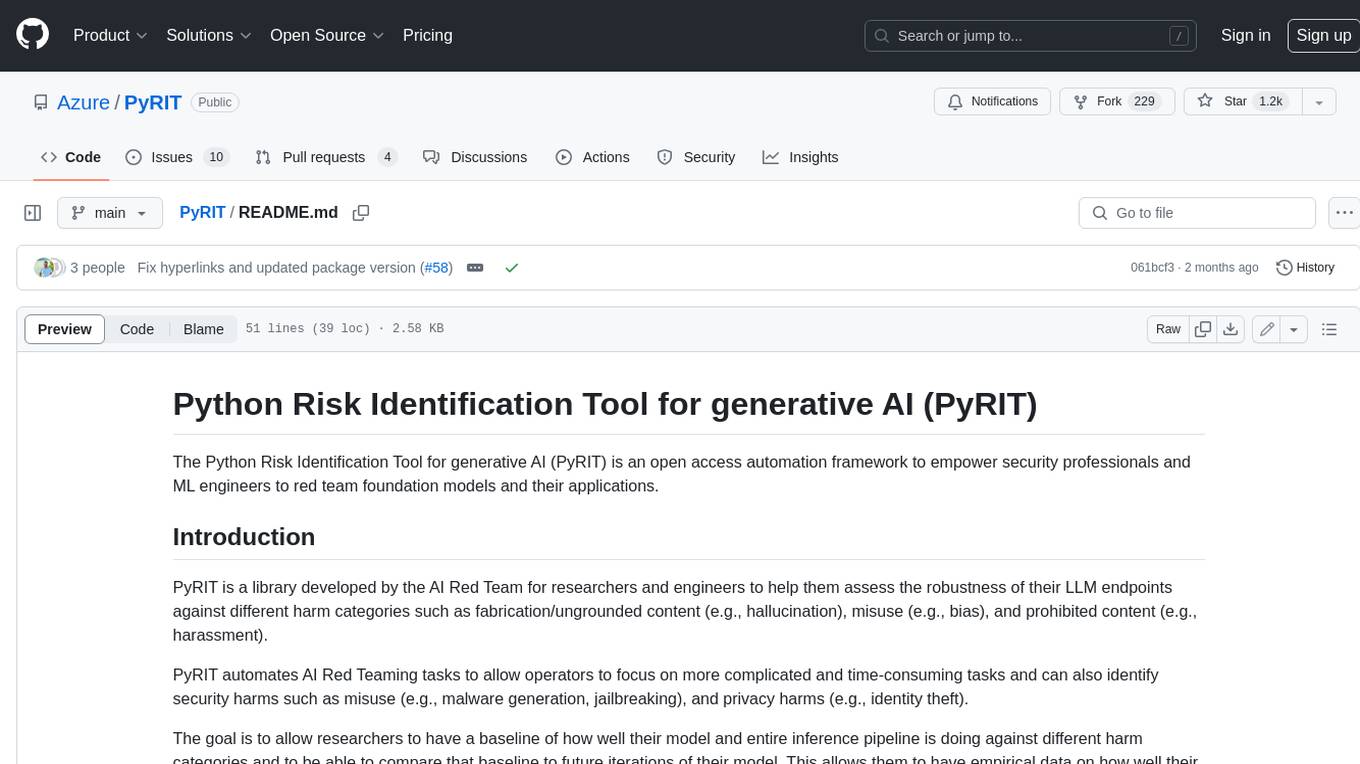
PyRIT
PyRIT is an open access automation framework designed to empower security professionals and ML engineers to red team foundation models and their applications. It automates AI Red Teaming tasks to allow operators to focus on more complicated and time-consuming tasks and can also identify security harms such as misuse (e.g., malware generation, jailbreaking), and privacy harms (e.g., identity theft). The goal is to allow researchers to have a baseline of how well their model and entire inference pipeline is doing against different harm categories and to be able to compare that baseline to future iterations of their model. This allows them to have empirical data on how well their model is doing today, and detect any degradation of performance based on future improvements.
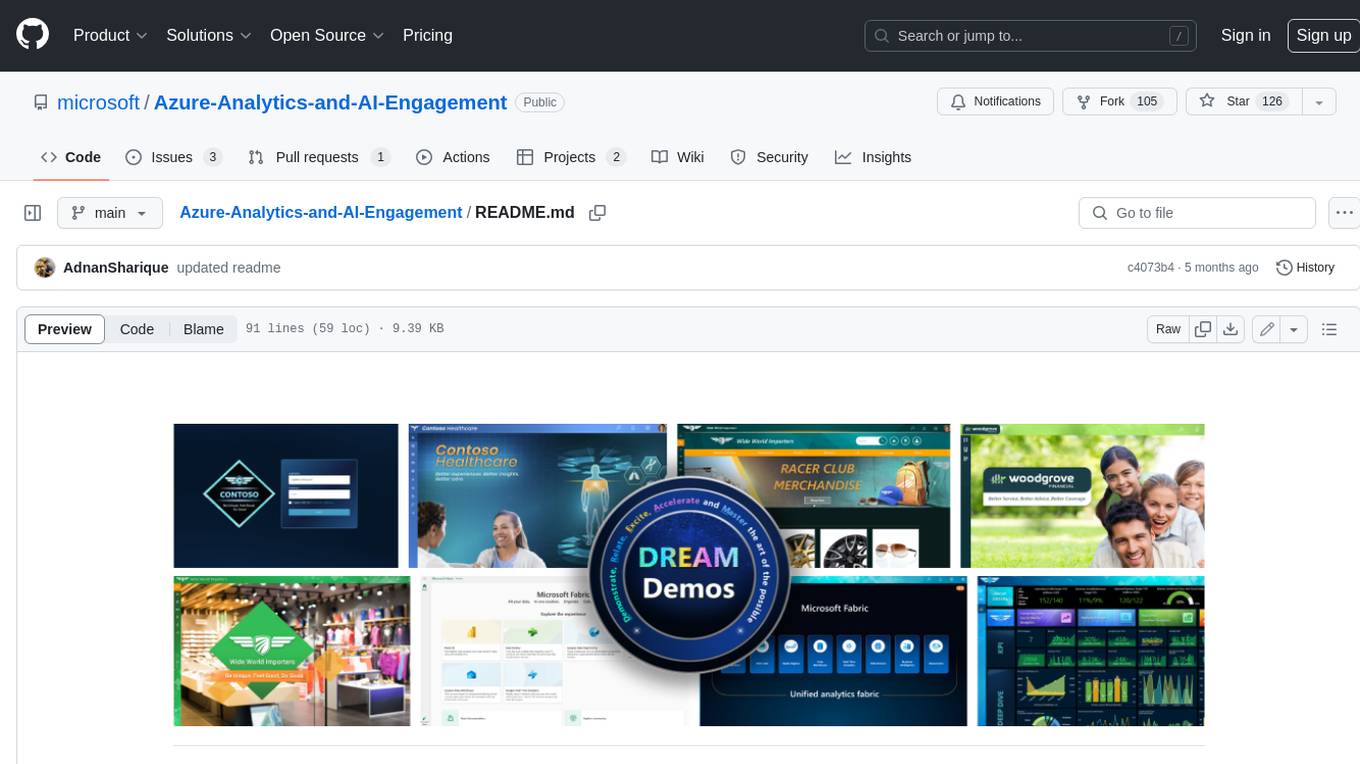
Azure-Analytics-and-AI-Engagement
The Azure-Analytics-and-AI-Engagement repository provides packaged Industry Scenario DREAM Demos with ARM templates (Containing a demo web application, Power BI reports, Synapse resources, AML Notebooks etc.) that can be deployed in a customer’s subscription using the CAPE tool within a matter of few hours. Partners can also deploy DREAM Demos in their own subscriptions using DPoC.