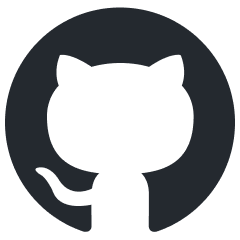
sample-apps
Repository of sample applications for https://vespa.ai, the open big data serving engine
Stars: 351
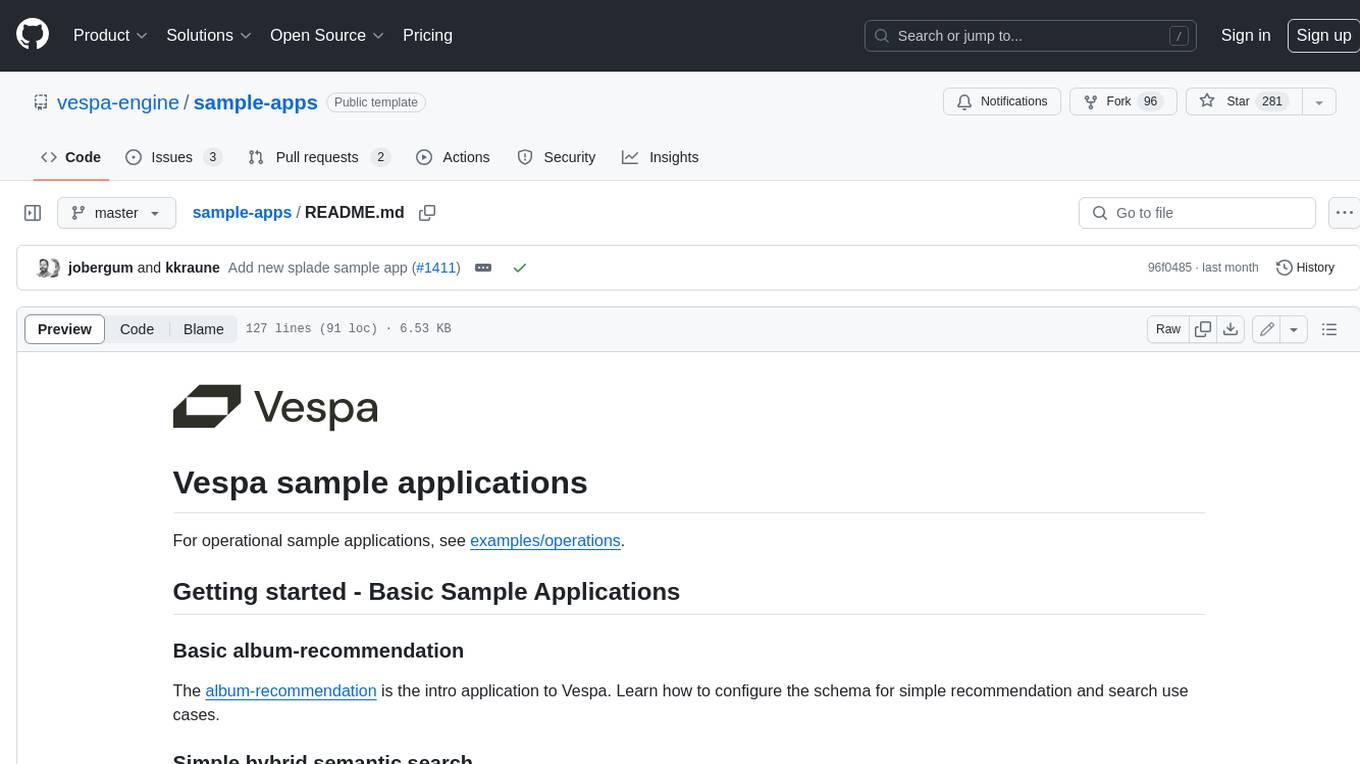
Vespa is an open-source search and AI engine that provides a unified platform for building and deploying search and AI applications. Vespa sample applications showcase various use cases and features of Vespa, including basic search, recommendation, semantic search, image search, text ranking, e-commerce search, question answering, search-as-you-type, and ML inference serving.
README:
The Vespa sample applications are created to run both self-hosted and on Vespa Cloud.
You can easily deploy the sample applications to Vespa Cloud without changing the files -
just follow the same steps as for
Managed Vector Search using Vespa Cloud,
adding security credentials.
First-time users should go through the getting-started guides first.
See examples/operations for operational sample applications.
Album Recommendations is the intro application to Vespa.
Learn how to configure the schema for simple recommendation and search use cases.
Pyvespa: Hybrid Search - Quickstart and
Pyvespa: Hybrid Search - Quickstart on Vespa Cloud
create a hybrid text search application combining traditional keyword matching with semantic vector search (dense retrieval).
They also demonstrate the Vespa native embedder functionality.
These are intro level applications for Python users using more advanced Vespa features.
Use
Pyvespa: Authenticating to Vespa Cloud for Vespa Cloud credentials.
Pyvespa: Querying Vespa
is a good start for Python users, exploring how to query Vespa using the Vespa Query Language (YQL).
Pyvespa: Read and write operations
documents ways to feed, get, update and delete data;
Using context manager with for efficiently managing resources
and feeding streams of data using
feed_iter
which can feed from streams, Iterables, Lists
and files by the use of generators.
Pyvespa: Application packages
is a good intro to the concept of application packages in Vespa.
Try
Pyvespa: Advanced Configuration for Vespa Services configuration.
Pyvespa: Examples
is a repository of small snippets and examples, e.g. really simple vector distance search applications.
The News and Recommendation Tutorial
demonstrates basic search functionality, and is a great place to start exploring Vespa features.
It creates a recommendation system where the approximate nearest neighbor search in a shared user/item embedding space
is used to retrieve recommended content for a user.
This app also demonstrates using parent-child relationships.
The Text Search Tutorial
demonstrates traditional text search using
BM25/Vespa nativeRank,
and is a good start into using the MS Marco dataset.
There is a growing interest in AI-powered vector representations of unstructured multimodal data
and searching efficiently over these representations.
Managed Vector Search using Vespa Cloud
describes how to unlock the full potential of multimodal AI-powered vector representations using Vespa Cloud.
Simple Semantic Search
demonstrates indexed vector search using HNSW,
creating embedding vectors from a transformer language model inside Vespa, and hybrid text and semantic ranking.
This app also demonstrates using native Vespa embedders.
Vespa Multi-Vector Indexing with HNSW and
Pyvespa: Multi-vector indexing with HNSW
demonstrate how to index multiple vectors per document field for semantic search for longer documents.
Vector Streaming Search
uses vector streaming search for naturally partitioned data, she the
blog post for details.
Multilingual Search with multilingual embeddings
demonstrates multilingual semantic search with multilingual text embedding models.
Simple hybrid search with SPLADE
uses the Vespa splade-embedder for
semantic search using sparse vector representations,
and is a good intro into SPLADE and sparse learned weights for ranking.
Customizing Frozen Data Embeddings in Vespa
demonstrates how to adapt frozen embeddings from foundational embedding models -
see the blog post.
Frozen data embeddings from foundational models is an emerging industry practice
for reducing the complexity of maintaining and versioning embeddings.
The frozen data embeddings are re-used for various tasks, such as classification, search, or recommendations.
Pyvespa: Using Cohere Binary Embeddings in Vespa
demonstrates how to use the Cohere binary vectors with Vespa,
including a re-ranking phase that uses the float query vector version for improved accuracy.
Pyvespa: Billion-scale vector search with Cohere binary embeddings in Vespa
uses the Cohere int8 & binary Embeddings
with a coarse-to-fine search and re-ranking pipeline that reduces costs, but offers the same retrieval (nDCG) accuracy.
The packed binary vector representation is stored in memory,
with an optional HNSW index using
hamming distance.
The
int8
vector representation is stored on disk
using Vespa’s paged option.
Pyvespa: Multilingual Hybrid Search with Cohere binary embeddings and Vespa
demonstrates:
- Building a multilingual search application over a sample of the German split of Wikipedia using binarized Cohere embeddings.
- Indexing multiple binary embeddings per document; without having to split the chunks across multiple retrievable units.
- Hybrid search, combining the lexical matching capabilities of Vespa with Cohere binary embeddings.
- Re-scoring the binarized vectors for improved accuracy.
Pyvespa: BGE-M3 - The Mother of all embedding models
demonstrates how to use the BGE-M3 embeddings
and represent all three embedding representations in Vespa.
This code is inspired by the BAAI/bge-m3 README.
Pyvespa: Evaluating retrieval with Snowflake arctic embed
shows how different rank profiles in Vespa can be set up and evaluated.
For the rank profiles that use semantic search,
we will use the small version of Snowflake’s arctic embed model series for generating embeddings.
Pyvespa: Exploring the potential of OpenAI Matryoshka 🪆 embeddings with Vespa
demonstrates the effectiveness of using the recently released (as of January 2024) OpenAI
text-embedding-3
embeddings with Vespa.
Specifically, we are interested in the Matryoshka Representation Learning technique used in training,
which lets us "shorten embeddings (i.e. remove some numbers from the end of the sequence) without the embedding losing its concept-representing properties".
This allow us to trade off a small amount of accuracy in exchange for much smaller embedding sizes,
so we can store more documents and search them faster.
Pyvespa: Using Mixedbread.ai embedding model with support for binary vectors
shows how to use the mixedbread-ai/mxbai-embed-large-v1 model
with support for binary vectors with Vespa.
The notebook example also includes a re-ranking phase that uses the float query vector version for improved accuracy.
The re-ranking step makes the model perform at 96.45% of the full float version,
with a 32x decrease in storage footprint.
Retrieval Augmented Generation (RAG) in Vespa
is an end-to-end RAG application where all the steps are run within Vespa.
This application focuses on the generation part of RAG,
with a simple text search using BM25.
This application has three versions of an end-to-end RAG application:
- Using an external LLM service to generate the final response.
- Using local LLM inference to generate the final response.
- Deploying to Vespa Cloud and using GPU accelerated LLM inference to generate the final response. This includes using Vespa Cloud's Secret Store to save the OpenAI API key.
Pyvespa: Visual PDF RAG with Vespa - ColPali demo application
is an end-to-end demo application for visual retrieval of PDF pages, including a frontend web application -
try vespa-engine-colpali-vespa-visual-retrieval.hf.space for a live demo.
The main goal of the demo is to make it easy to create your own PDF Enterprise Search application using Vespa!
Pyvespa: Building cost-efficient retrieval-augmented personal AI assistants
uses streaming mode
for cost-efficient retrieval for applications that store and retrieve personal data.
This notebook connects a custom LlamaIndex Retriever
with a Vespa app using streaming mode to retrieve personal data.
Pyvespa: Turbocharge RAG with LangChain and Vespa Streaming Mode for Partitioned Data
uses streaming mode
to build cost-efficient RAG applications over naturally sharded data - also available as a blog post:
Turbocharge RAG with LangChain and Vespa Streaming Mode for Sharded Data.
Also try
Pyvespa: Chat with your pdfs with ColBERT, LangChain, and Vespa -
this demonstrates how you can now use ColBERT ranking natively in Vespa,
which handles the ColBERT embedding process with no custom code.
Pyvespa: Vespa 🤝 ColPali: Efficient Document Retrieval with Vision Language Models
demonstrates how to retrieve PDF pages using the embeddings generated by the ColPali model.
ColPali is a powerful Vision Language Model (VLM) that can generate embeddings for images and text.
This notebook uses ColPali to generate embeddings for images of PDF pages and store them in Vespa.
We also store the base64-encoded image of the PDF page and some metadata like title and url.
Pyvespa: Scaling ColPALI (VLM) Retrieval
demonstrates how to represent ColPali in Vespa and to scale to large collections.
Also see the Scaling ColPali to billions of PDFs with Vespa blog post.
Pyvespa: ColPali Ranking Experiments on DocVQA
shows how to reproduce the ColPali results on DocVQA with Vespa.
The dataset consists of PDF documents with questions and answers.
We demonstrate how we can binarize the patch embeddings
and replace the float MaxSim scoring
with a hamming-based MaxSim
without much loss in ranking accuracy but with a significant speedup (close to 4x) and reducing the memory (and storage) requirements by 32x.
Pyvespa: PDF-Retrieval using ColQWen2 (ColPali) with Vespa
is a continuation of the notebooks related to the ColPali models (above) for complex document retrieval,
and demonstrates use of the ColQWen2 model checkpoint.
With Vespa’s phased ranking capabilities,
doing cross-encoder inference for a subset of documents at a later stage in the ranking pipeline
can be a good trade-off between ranking performance and latency.
Pyvespa: Using Mixedbread.ai cross-encoder for reranking in Vespa.ai
shows how to use the Mixedbread.ai
cross-encoder for global-phase reranking in Vespa.
Pyvespa: Standalone ColBERT with Vespa for end-to-end retrieval and ranking
illustrates using the colbert-ai package to produce token vectors,
instead of using the native Vespa ColBERT embedder.
The guide illustrates how to feed and query using a single passage representation:
- Compress token vectors using binarization compatible with Vespa's
unpack_bits
used in ranking. This implements the binarization of token-level vectors usingnumpy
. - Use Vespa hex feed format for binary vectors.
- Query examples.
As a bonus, this also demonstrates how to use ColBERT end-to-end with Vespa for both retrieval and ranking. The retrieval step searches the binary token-level representations using hamming distance. This uses 32 nearestNeighbor operators in the same query, each finding 100 nearest hits in hamming space. Then the results are re-ranked using the full-blown MaxSim calculation.
ColBERT token-level embeddings:
-
Simple hybrid search with ColBERT uses a single vector embedding model for retrieval and ColBERT (multi-token vector representation) for re-ranking. This semantic search application demonstrates the colbert-embedder and the tensor expressions for ColBERT MaxSim. It also features reciprocal rank fusion to fuse different rankings.
-
Long-Context ColBERT demonstrates Long-Context ColBERT (multi-token vector representation) with extended context windows for long-document retrieval, as announced in Vespa Long-Context ColBERT. The app demonstrates the colbert-embedder and the tensor expressions for performing two types of extended ColBERT late-interaction for long-context retrieval. This app uses trec-eval for evaluation using nDCG.
-
Pyvespa: Standalone ColBERT + Vespa for long-context ranking is a guide on how to use the ColBERT package to produce token-level vectors, as an alternative to using the native Vespa ColBERT embedder. It illustrates how to feed multiple passages per Vespa document (long-context):
- Compress token vectors using binarization compatible with Vespa's
unpack_bits
. - Use Vespa hex feed format for binary vectors with mixed vespa tensors.
- How to query Vespa with the ColBERT query tensor representation.
- Compress token vectors using binarization compatible with Vespa's
Pyvespa: LightGBM: Training the model with Vespa features
deploys and uses a LightGBM model in a Vespa application.
The tutorial runs through how to:
- Train a LightGBM classification model with variable names supported by Vespa.
- Create Vespa application package files and export then to an application folder.
- Export the trained LightGBM model to the Vespa application folder.
- Deploy the Vespa application using the application folder.
- Feed data to the Vespa application.
- Assert that the LightGBM predictions from the deployed model are correct.
Pyvespa: LightGBM: Mapping model features to Vespa features
shows how to deploy a LightGBM model with feature names that do not match Vespa feature names.
In addition to the steps in the app above, this tutorial:
- Trains a LightGBM classification model with generic feature names that will not be available in the Vespa application.
- Creates an application package and include a mapping from Vespa feature names to LightGBM model feature names.
Pyvespa: Feeding performance
intends to shine some light on the different modes of feeding documents to Vespa, looking at 4 different methods:
- Using
VespaSync
- Using
VespaAsync
- Using
feed_iterable()
- Using Vespa CLI
Use Feeding to Vespa Cloud
to test feeding using Vespa Cloud.
Billion-Scale Image Search
demonstrates billion-scale image search using a CLIP model
exported in ONNX-format for retrieval.
It features separation of compute from storage and query-time vector similarity de-duping.
It uses PCA to reduce from 768 to 128 dimensions.
MS Marco Passage Ranking
shows how to represent state-of-the-art text ranking using Transformer (BERT) models.
It uses the MS Marco passage ranking datasets and features
bi-encoders, cross-encoders, and late-interaction models (ColBERT).
The e-commerce application is an end-to-end shopping engine,
using the Amazon product data set.
This use case bundles a frontend application.
It demonstrates building next generation E-commerce Search using Vespa,
and is a good intro into using the Vespa Cloud CI/CD tests.
Also try Vespa Product Ranking for using
learning-to-rank (LTR) techniques (using XGBoost and LightGBM)
for improving product search ranking.
Incremental Search shows search-as-you-type functionality,
where for each keystroke of the user, it retrieves matching documents.
It also demonstrates search suggestions (query auto-completion).
Stateless model evaluation demonstrates
using Vespa as a stateless ML model inference server
where Vespa takes care of distributing ML models to multiple serving containers,
offering horizontal scaling and safe deployment.
It features model versioning and a feature processing pipeline,
as well as using custom code in Searchers,
Document Processors and
Request Handlers.
Vespa Documentation Search
is the search application that powers search.vespa.ai -
refer to this for GitHub Actions automation.
This sample app is a good start for automated deployments,
as it has system, staging and production test examples.
It uses the Document API
both for regular PUT operations but also for UPDATE with create-if-nonexistent.
It also has Vespa Components
for custom code.
cord19.vespa.ai is a full-featured application,
based on the Covid-19 Open Research Dataset:
- cord-19: frontend
- cord-19-search: search backend
Note: Applications with pom.xml are Java/Maven projects and must be built before deployment. Refer to the Developer Guide for more information.
Contribute to the Vespa sample applications.
For Tasks:
Click tags to check more tools for each tasksFor Jobs:
Alternative AI tools for sample-apps
Similar Open Source Tools
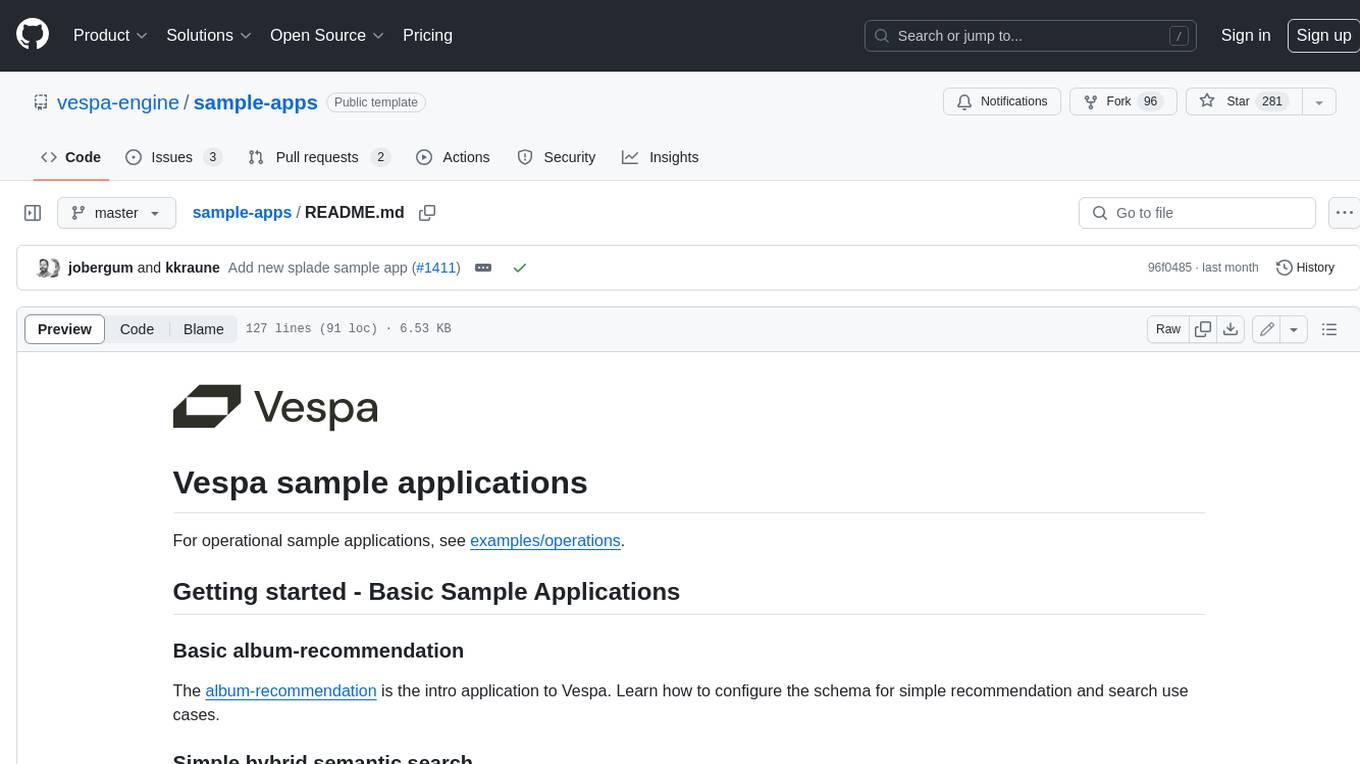
sample-apps
Vespa is an open-source search and AI engine that provides a unified platform for building and deploying search and AI applications. Vespa sample applications showcase various use cases and features of Vespa, including basic search, recommendation, semantic search, image search, text ranking, e-commerce search, question answering, search-as-you-type, and ML inference serving.
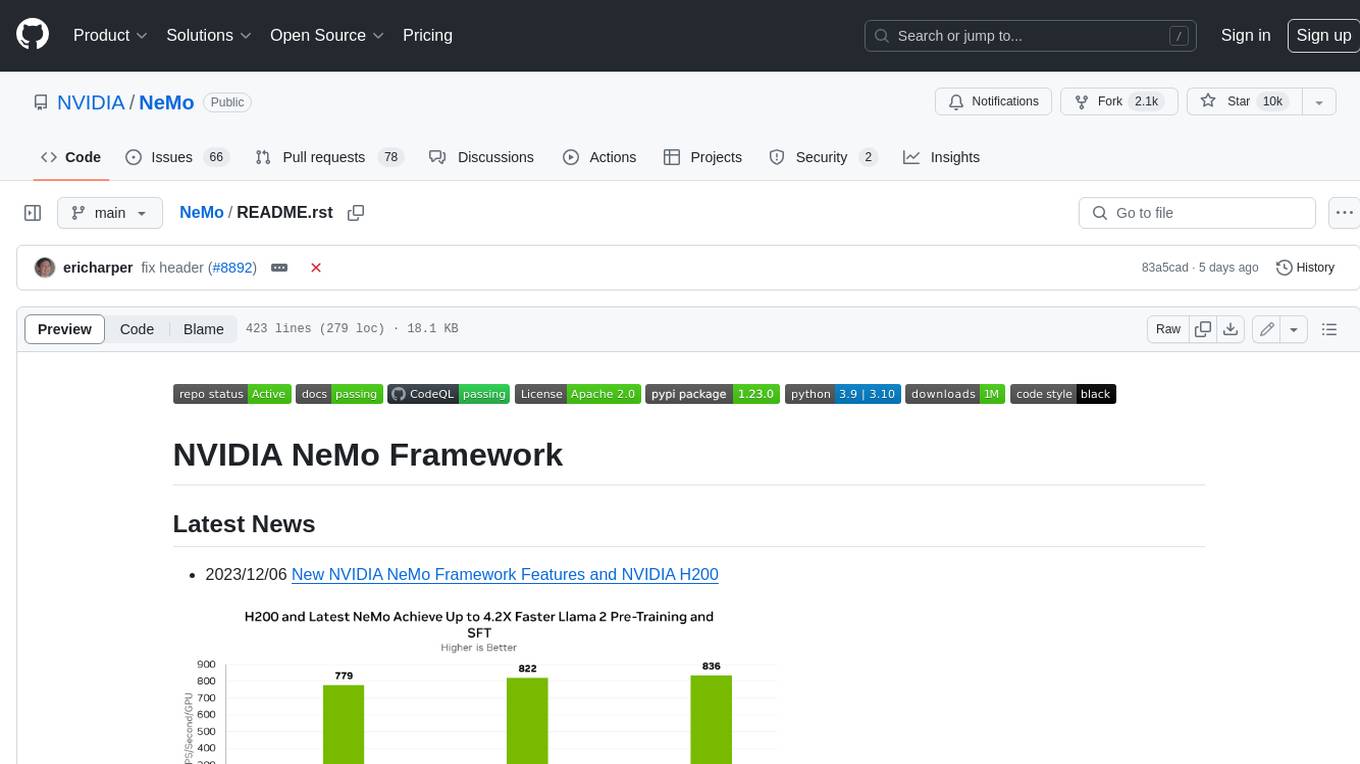
NeMo
NeMo Framework is a generative AI framework built for researchers and pytorch developers working on large language models (LLMs), multimodal models (MM), automatic speech recognition (ASR), and text-to-speech synthesis (TTS). The primary objective of NeMo is to provide a scalable framework for researchers and developers from industry and academia to more easily implement and design new generative AI models by being able to leverage existing code and pretrained models.
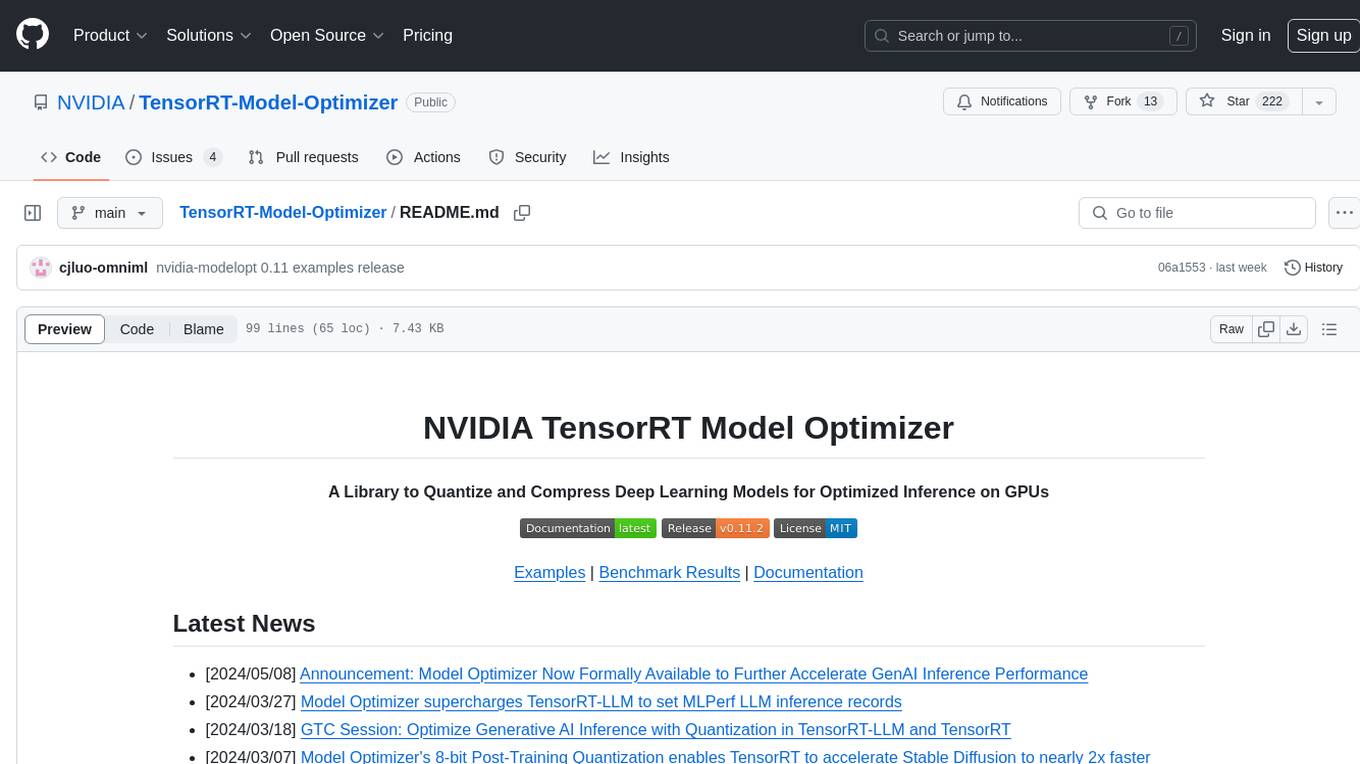
TensorRT-Model-Optimizer
The NVIDIA TensorRT Model Optimizer is a library designed to quantize and compress deep learning models for optimized inference on GPUs. It offers state-of-the-art model optimization techniques including quantization and sparsity to reduce inference costs for generative AI models. Users can easily stack different optimization techniques to produce quantized checkpoints from torch or ONNX models. The quantized checkpoints are ready for deployment in inference frameworks like TensorRT-LLM or TensorRT, with planned integrations for NVIDIA NeMo and Megatron-LM. The tool also supports 8-bit quantization with Stable Diffusion for enterprise users on NVIDIA NIM. Model Optimizer is available for free on NVIDIA PyPI, and this repository serves as a platform for sharing examples, GPU-optimized recipes, and collecting community feedback.
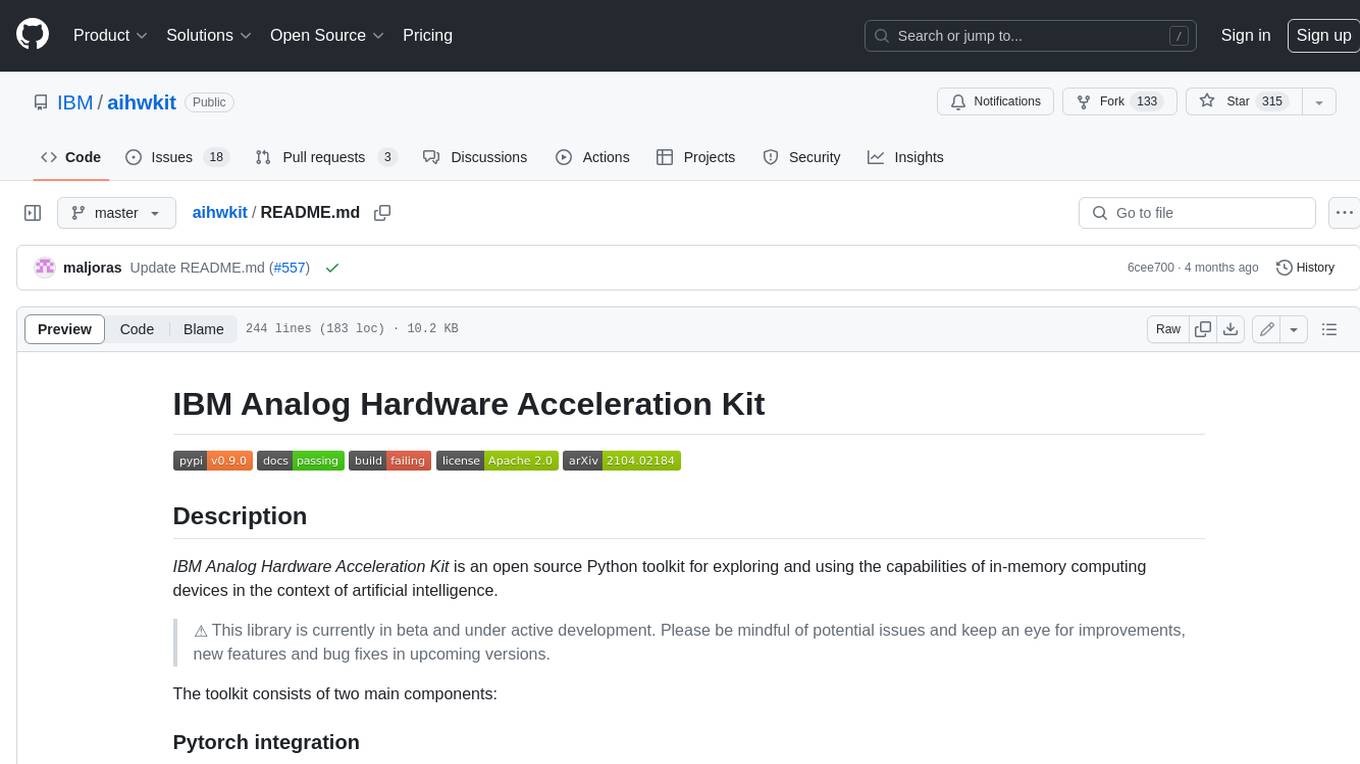
aihwkit
The IBM Analog Hardware Acceleration Kit is an open-source Python toolkit for exploring and using the capabilities of in-memory computing devices in the context of artificial intelligence. It consists of two main components: Pytorch integration and Analog devices simulator. The Pytorch integration provides a series of primitives and features that allow using the toolkit within PyTorch, including analog neural network modules, analog training using torch training workflow, and analog inference using torch inference workflow. The Analog devices simulator is a high-performant (CUDA-capable) C++ simulator that allows for simulating a wide range of analog devices and crossbar configurations by using abstract functional models of material characteristics with adjustable parameters. Along with the two main components, the toolkit includes other functionalities such as a library of device presets, a module for executing high-level use cases, a utility to automatically convert a downloaded model to its equivalent Analog model, and integration with the AIHW Composer platform. The toolkit is currently in beta and under active development, and users are advised to be mindful of potential issues and keep an eye for improvements, new features, and bug fixes in upcoming versions.
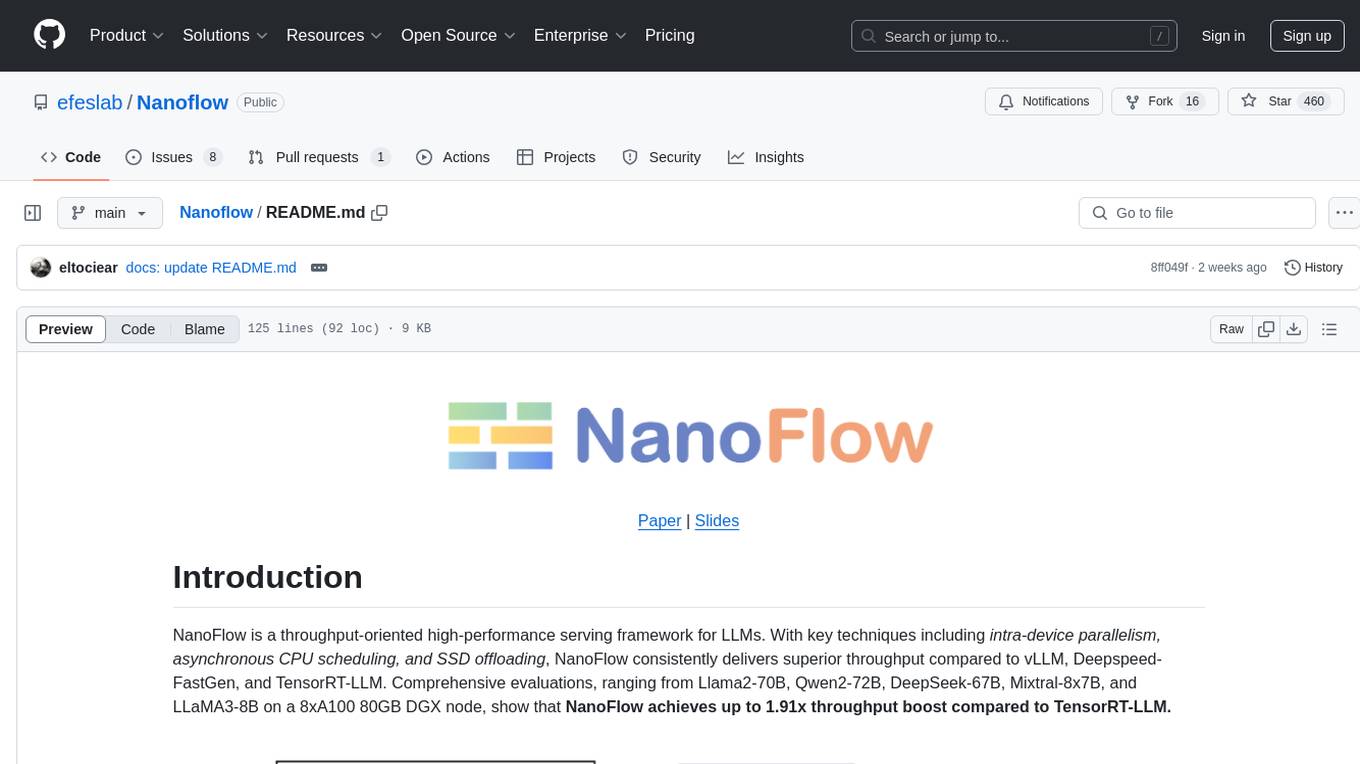
Nanoflow
NanoFlow is a throughput-oriented high-performance serving framework for Large Language Models (LLMs) that consistently delivers superior throughput compared to other frameworks by utilizing key techniques such as intra-device parallelism, asynchronous CPU scheduling, and SSD offloading. The framework proposes nano-batching to schedule compute-, memory-, and network-bound operations for simultaneous execution, leading to increased resource utilization. NanoFlow also adopts an asynchronous control flow to optimize CPU overhead and eagerly offloads KV-Cache to SSDs for multi-round conversations. The open-source codebase integrates state-of-the-art kernel libraries and provides necessary scripts for environment setup and experiment reproduction.
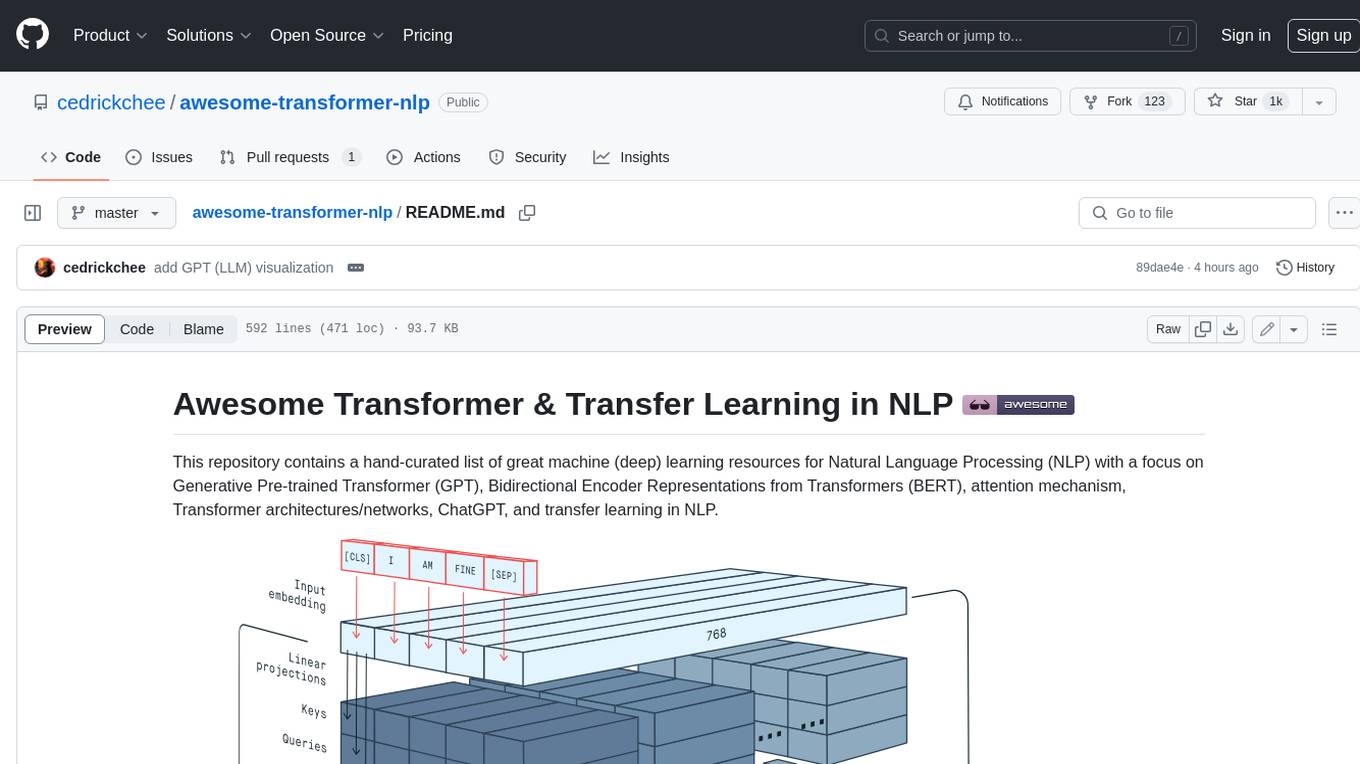
awesome-transformer-nlp
This repository contains a hand-curated list of great machine (deep) learning resources for Natural Language Processing (NLP) with a focus on Generative Pre-trained Transformer (GPT), Bidirectional Encoder Representations from Transformers (BERT), attention mechanism, Transformer architectures/networks, Chatbot, and transfer learning in NLP.
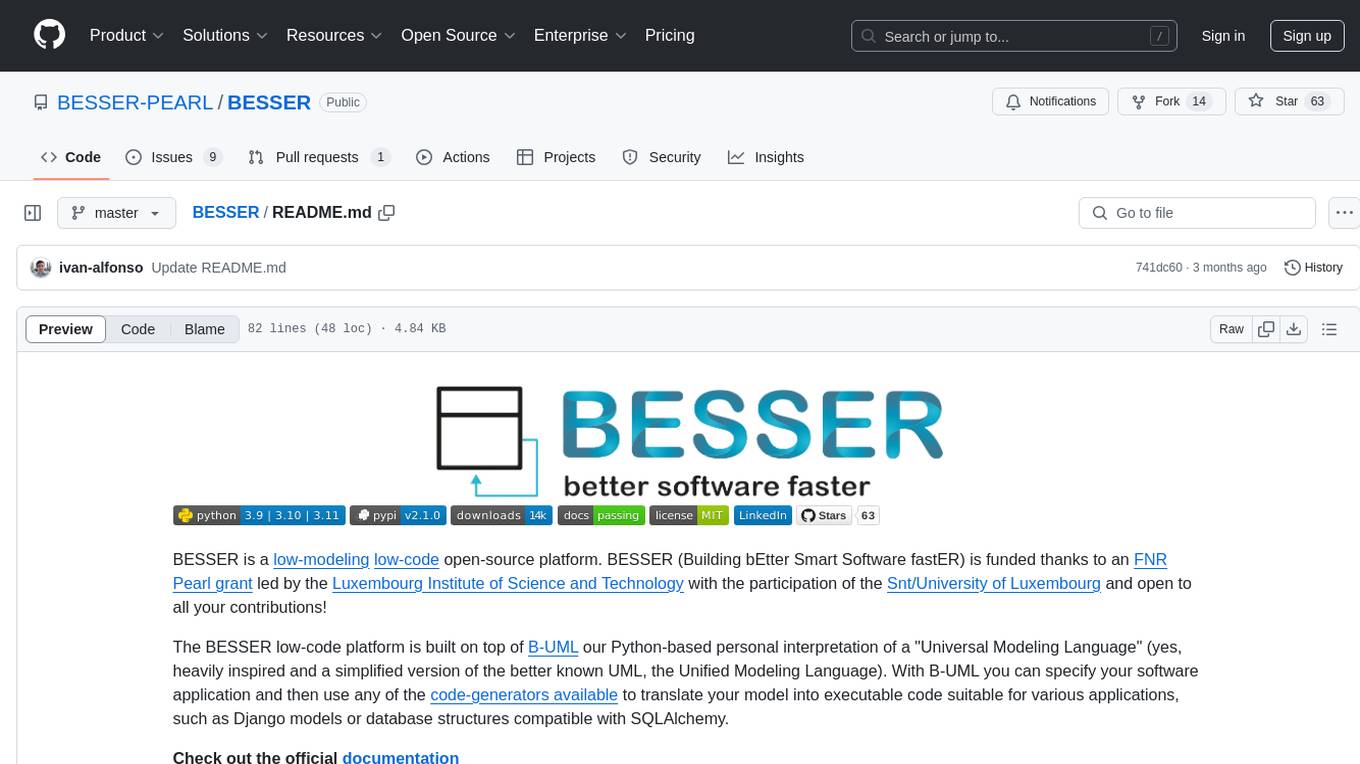
BESSER
BESSER is a low-modeling low-code open-source platform funded by an FNR Pearl grant. It is built on B-UML, a Python-based interpretation of a 'Universal Modeling Language'. Users can specify their software application using B-UML and generate executable code for various applications like Django models or SQLAlchemy-compatible database structures. BESSER is available on PyPi and can be installed with pip. It supports popular Python IDEs and encourages contributions from the community.
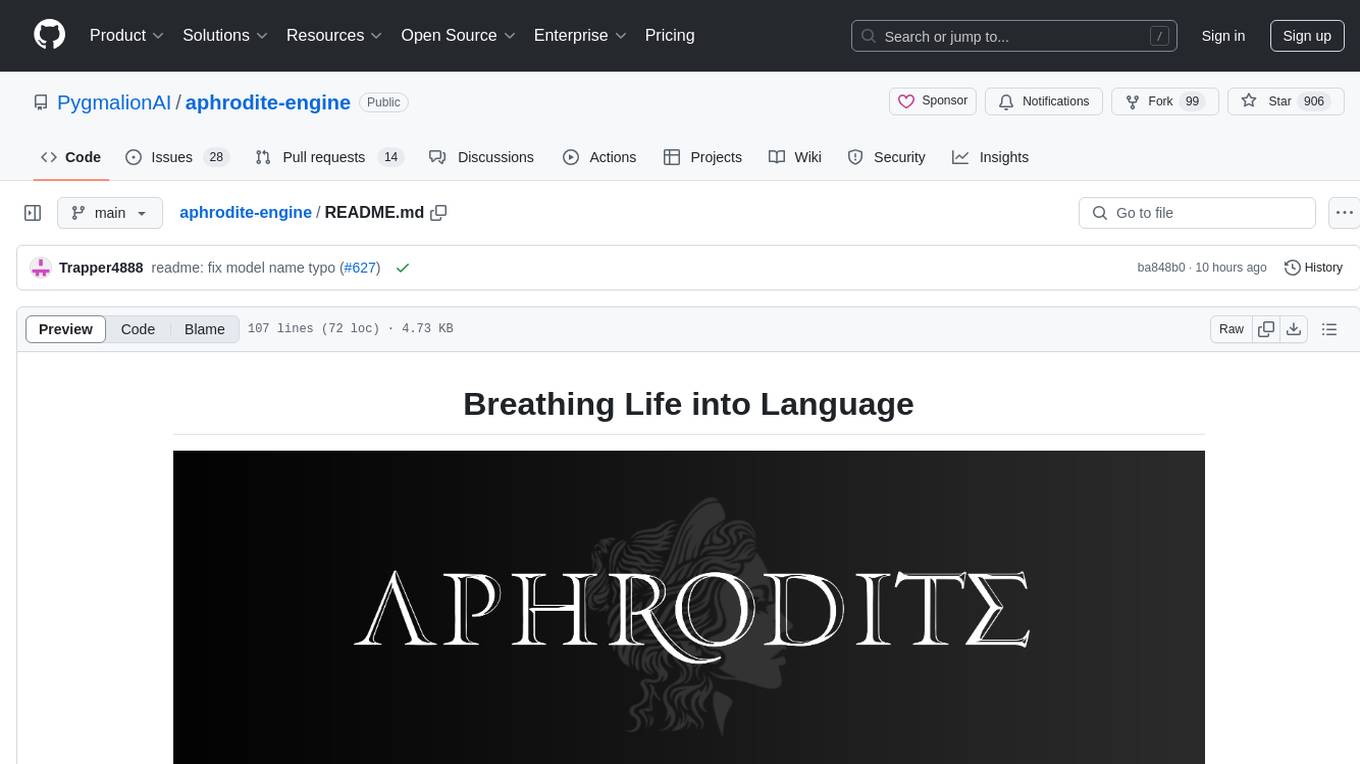
aphrodite-engine
Aphrodite is the official backend engine for PygmalionAI, serving as the inference endpoint for the website. It allows serving Hugging Face-compatible models with fast speeds. Features include continuous batching, efficient K/V management, optimized CUDA kernels, quantization support, distributed inference, and 8-bit KV Cache. The engine requires Linux OS and Python 3.8 to 3.12, with CUDA >= 11 for build requirements. It supports various GPUs, CPUs, TPUs, and Inferentia. Users can limit GPU memory utilization and access full commands via CLI.
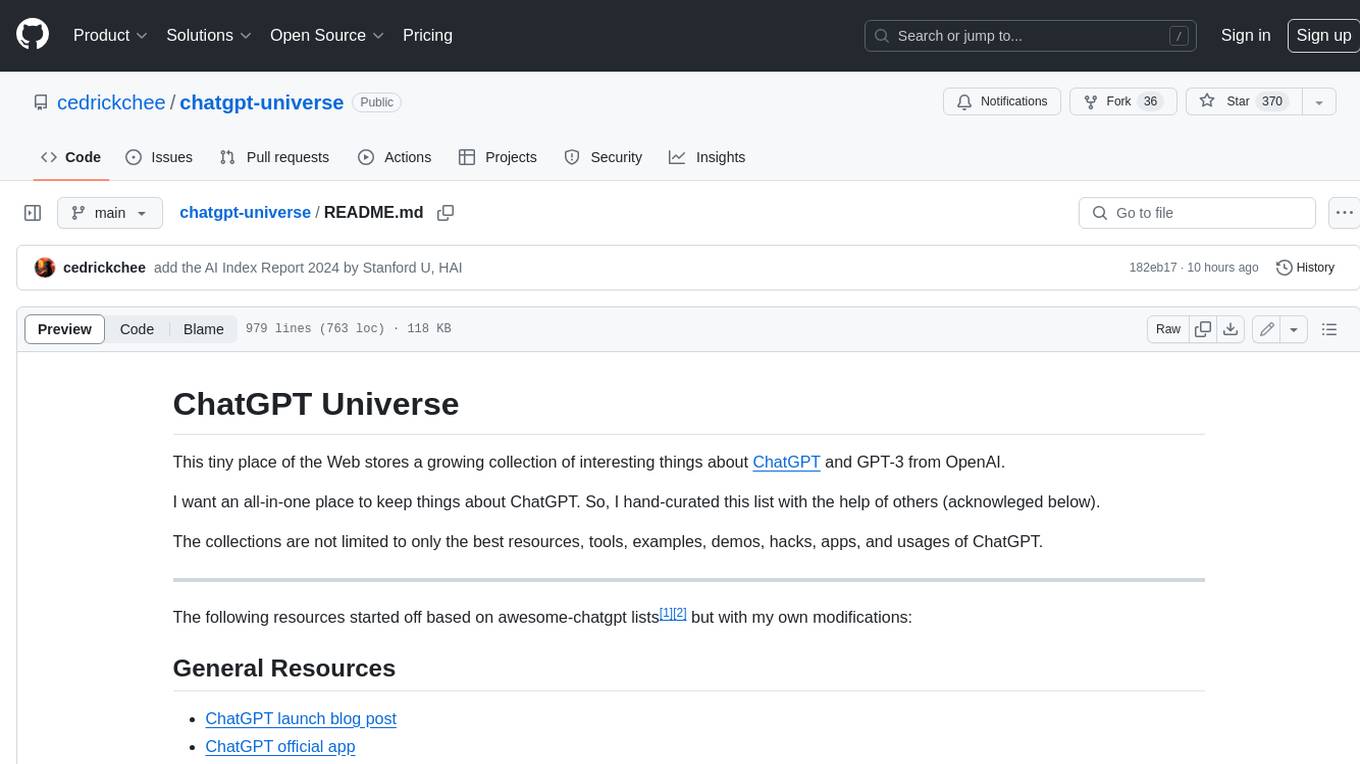
chatgpt-universe
ChatGPT is a large language model that can generate human-like text, translate languages, write different kinds of creative content, and answer your questions in a conversational way. It is trained on a massive amount of text data, and it is able to understand and respond to a wide range of natural language prompts. Here are 5 jobs suitable for this tool, in lowercase letters: 1. content writer 2. chatbot assistant 3. language translator 4. creative writer 5. researcher
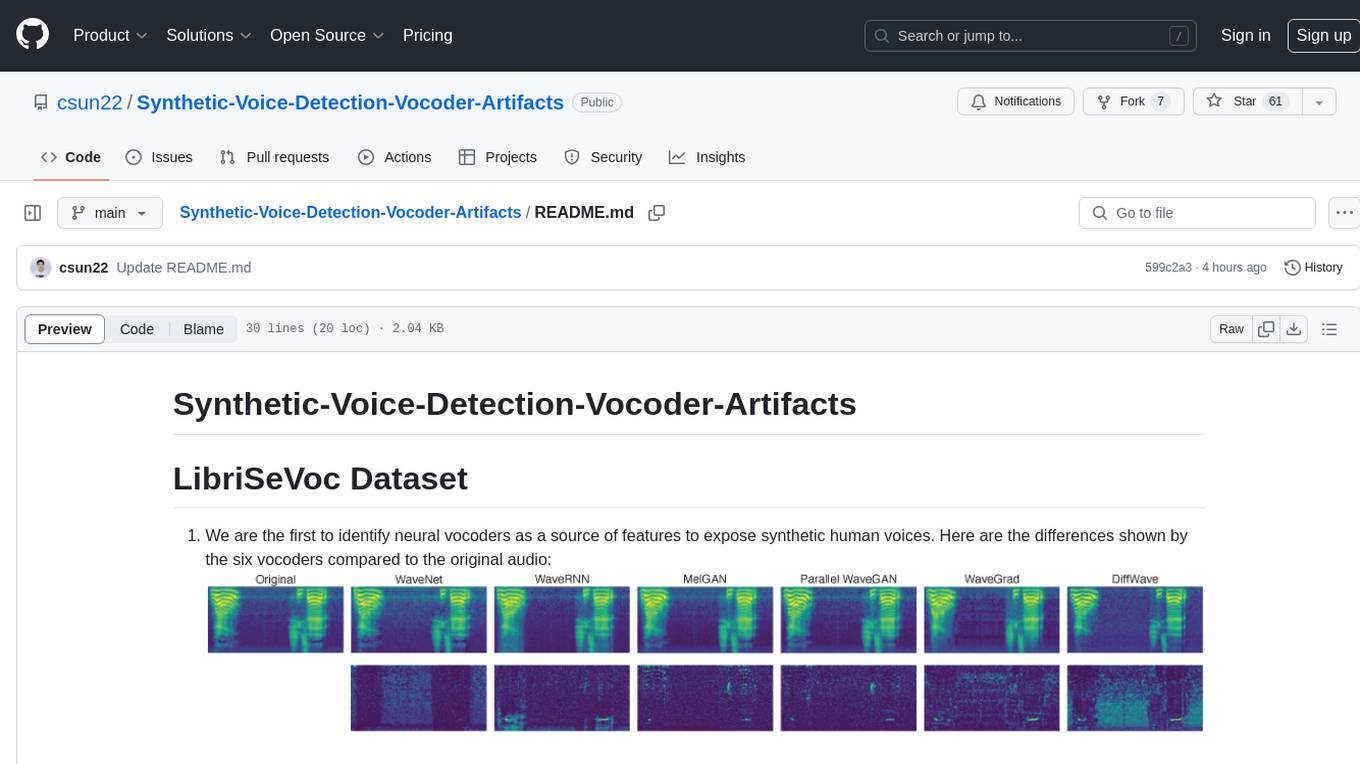
Synthetic-Voice-Detection-Vocoder-Artifacts
The Synthetic-Voice-Detection-Vocoder-Artifacts repository provides the LibriSeVoc dataset containing self-vocoding samples created with six state-of-the-art vocoders to expose and exploit vocoder artifacts. It also introduces a new approach for detecting synthetic human voices by identifying signal artifacts left by neural vocoders and enhancing the RawNet2 baseline. The repository includes a paper and dataset for further reference and offers instructions for training the model and testing it in the wild.
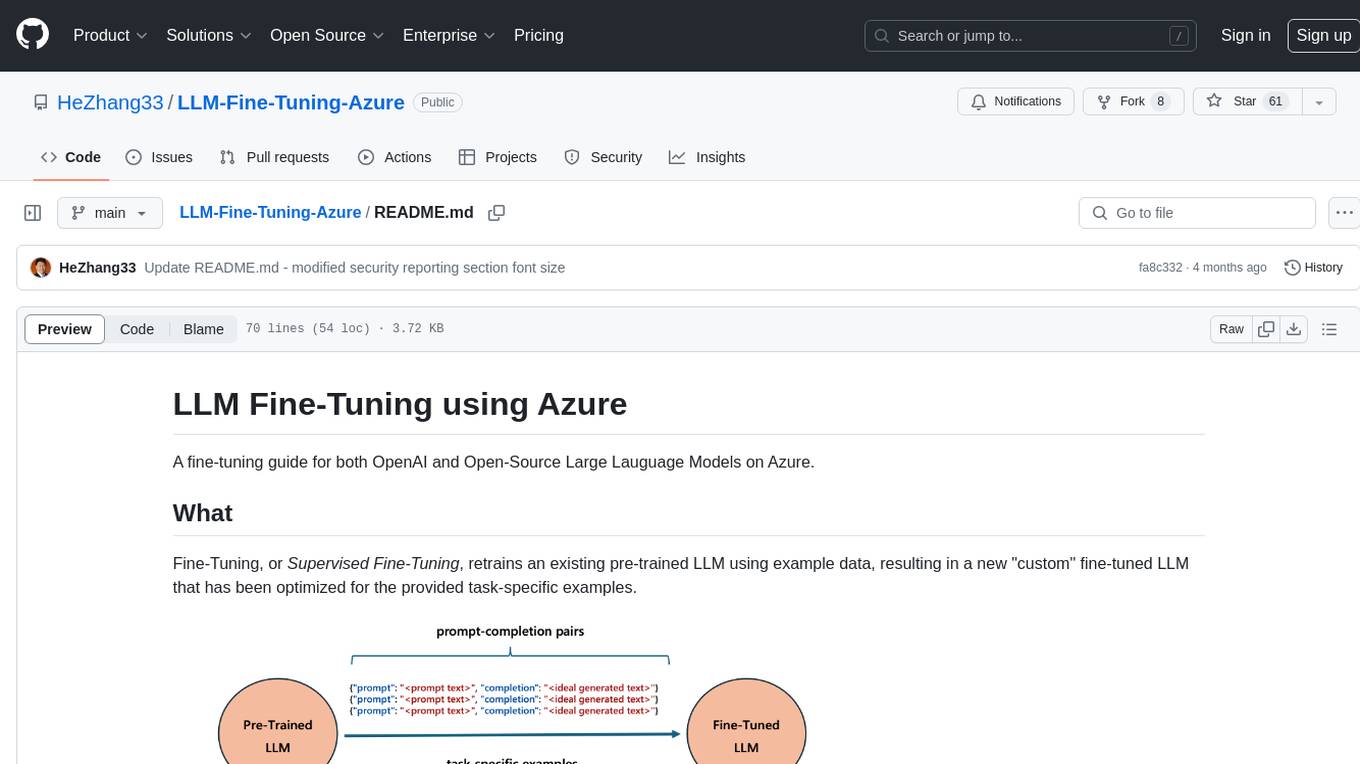
LLM-Fine-Tuning-Azure
A fine-tuning guide for both OpenAI and Open-Source Large Language Models on Azure. Fine-Tuning retrains an existing pre-trained LLM using example data, resulting in a new 'custom' fine-tuned LLM optimized for task-specific examples. Use cases include improving LLM performance on specific tasks and introducing information not well represented by the base LLM model. Suitable for cases where latency is critical, high accuracy is required, and clear evaluation metrics are available. Learning path includes labs for fine-tuning GPT and Llama2 models via Dashboards and Python SDK.
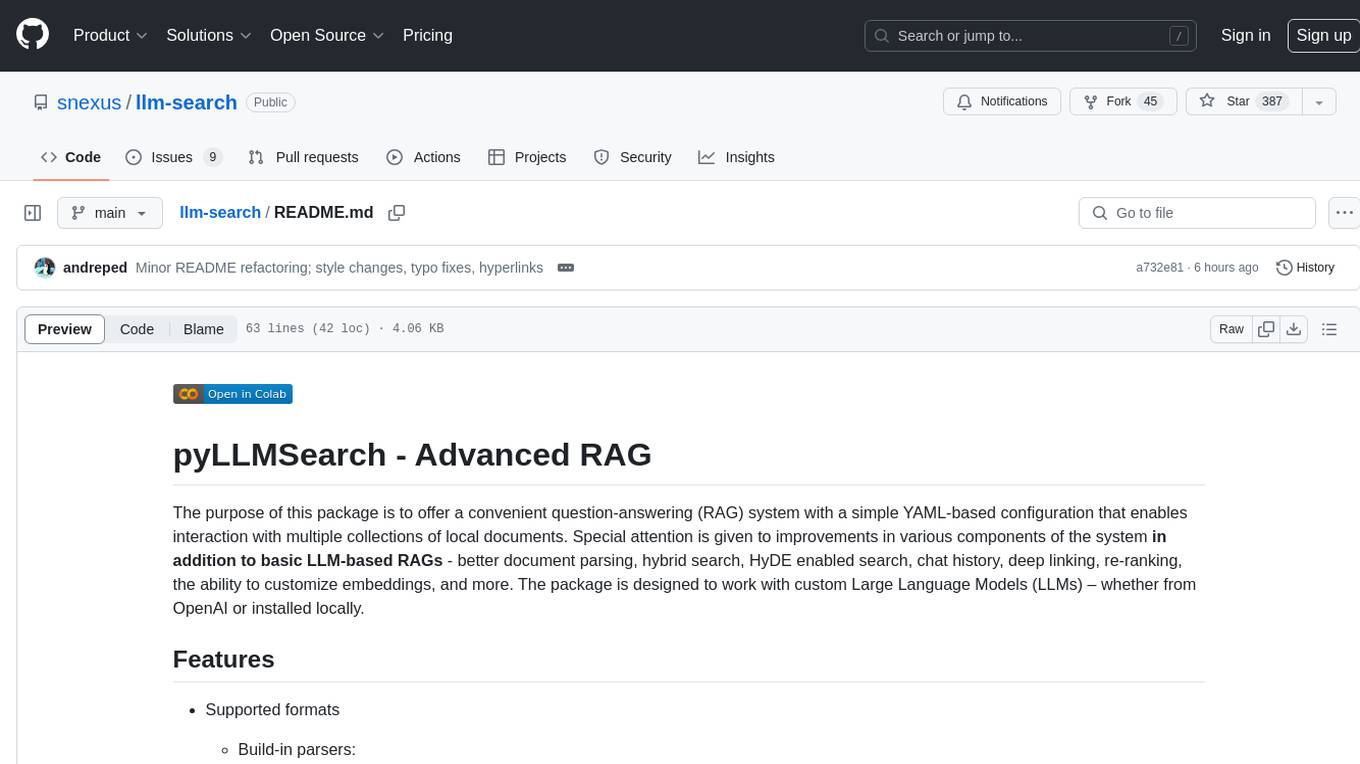
llm-search
pyLLMSearch is an advanced RAG system that offers a convenient question-answering system with a simple YAML-based configuration. It enables interaction with multiple collections of local documents, with improvements in document parsing, hybrid search, chat history, deep linking, re-ranking, customizable embeddings, and more. The package is designed to work with custom Large Language Models (LLMs) from OpenAI or installed locally. It supports various document formats, incremental embedding updates, dense and sparse embeddings, multiple embedding models, 'Retrieve and Re-rank' strategy, HyDE (Hypothetical Document Embeddings), multi-querying, chat history, and interaction with embedded documents using different models. It also offers simple CLI and web interfaces, deep linking, offline response saving, and an experimental API.
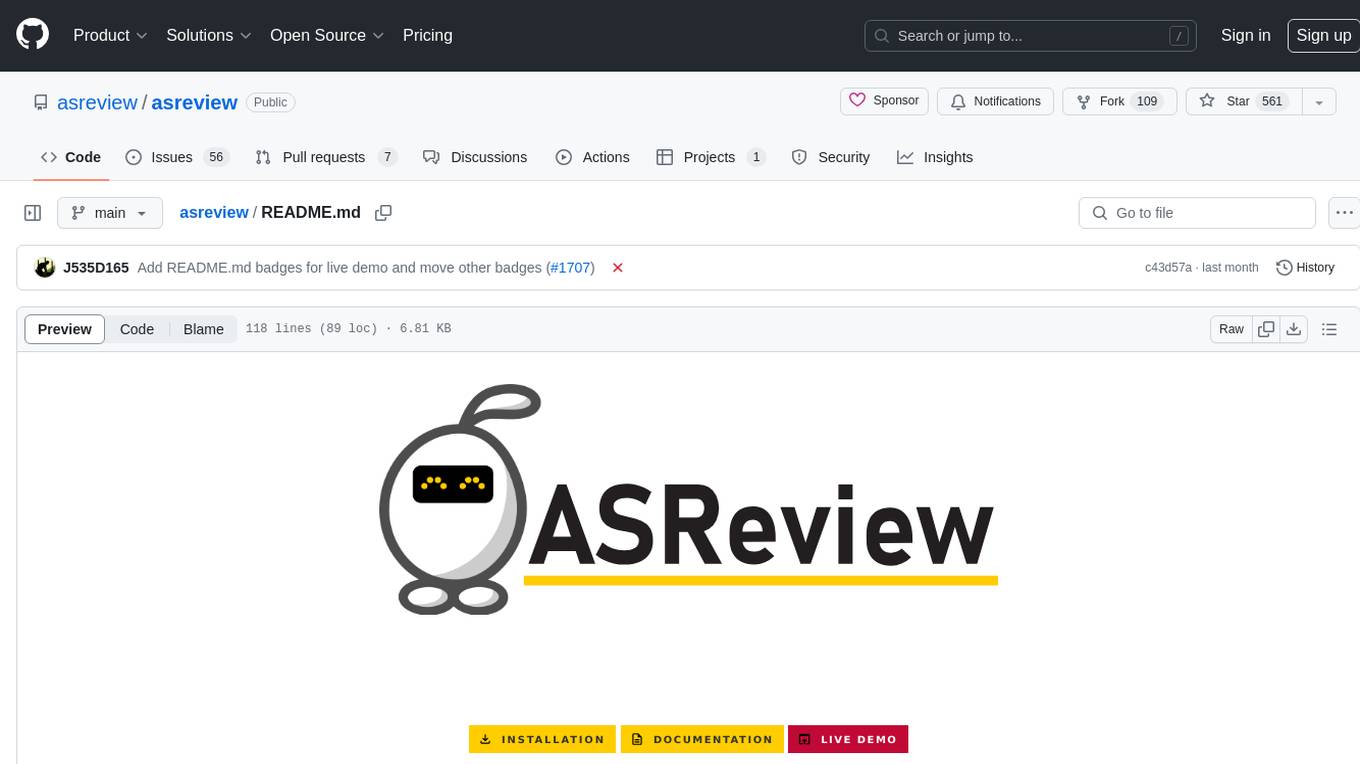
asreview
The ASReview project implements active learning for systematic reviews, utilizing AI-aided pipelines to assist in finding relevant texts for search tasks. It accelerates the screening of textual data with minimal human input, saving time and increasing output quality. The software offers three modes: Oracle for interactive screening, Exploration for teaching purposes, and Simulation for evaluating active learning models. ASReview LAB is designed to support decision-making in any discipline or industry by improving efficiency and transparency in screening large amounts of textual data.
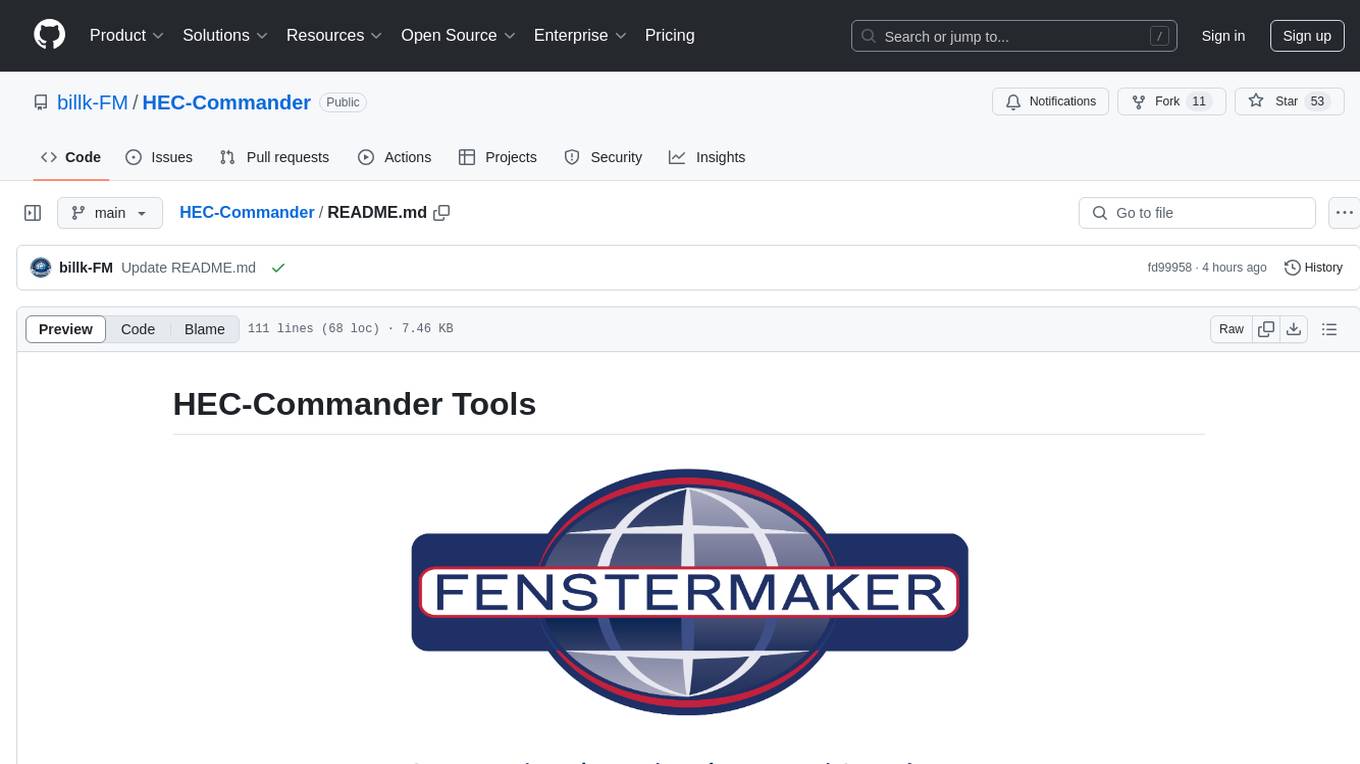
HEC-Commander
HEC-Commander Tools is a suite of python notebooks developed with AI assistance for water resource engineering workflows, providing automation for HEC-RAS and HEC-HMS through Jupyter Notebooks. It contains automation scripts for HEC-HMS, HEC-RAS, and DSS, along with miscellaneous tools. The repository also includes blog posts, ChatGPT assistants, and presentations related to H&H modeling and water resources workflows. Developed to support Region 4 of the Louisiana Watershed Initiative by Fenstermaker.
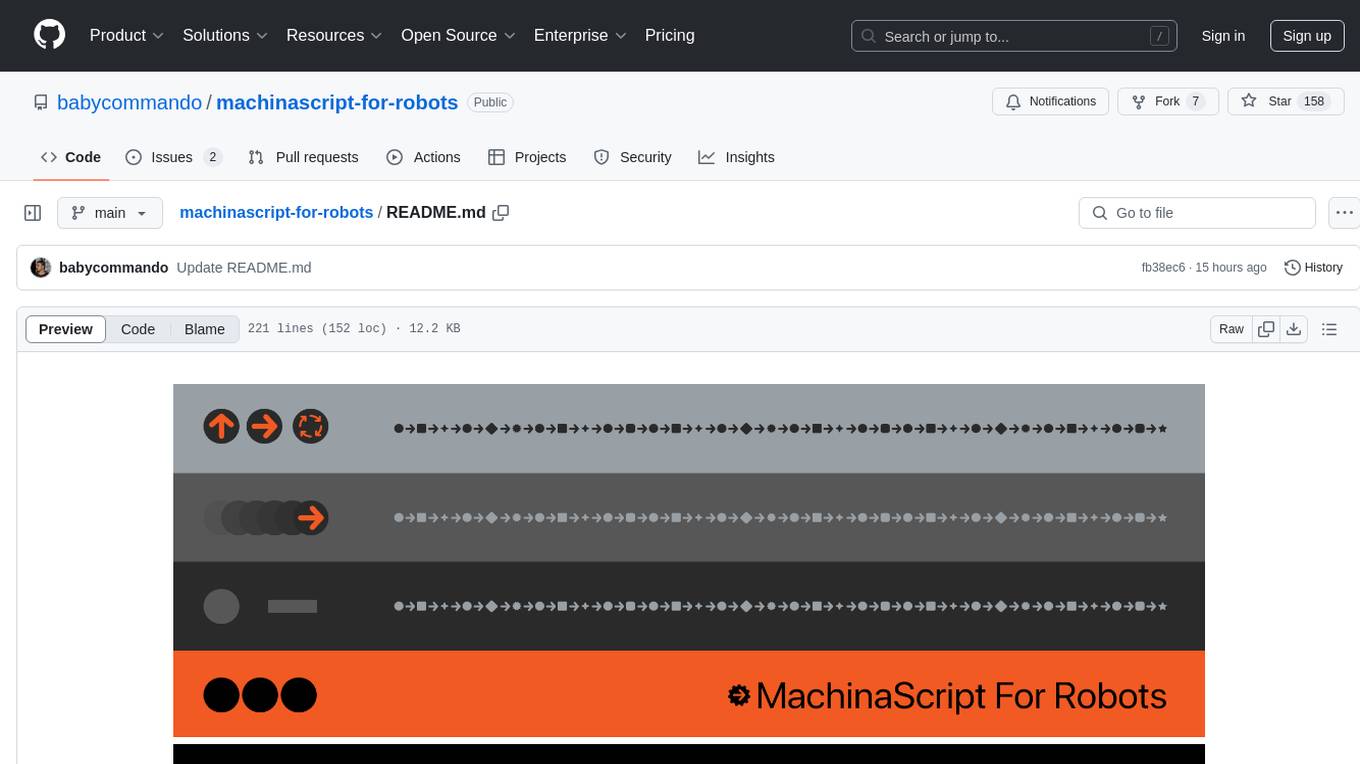
machinascript-for-robots
MachinaScript For Robots is a dynamic set of tools and a LLM-JSON-based language designed to empower humans in the creation of their own robots. It facilitates the animation of generative movements, the integration of personality, and the teaching of new skills with a high degree of autonomy. With MachinaScript, users can control a wide range of electronic components, including Arduinos, Raspberry Pis, servo motors, cameras, sensors, and more. The tool enables the creation of intelligent robots accessible to everyone, allowing for complex tasks to be performed with elegance and precision.
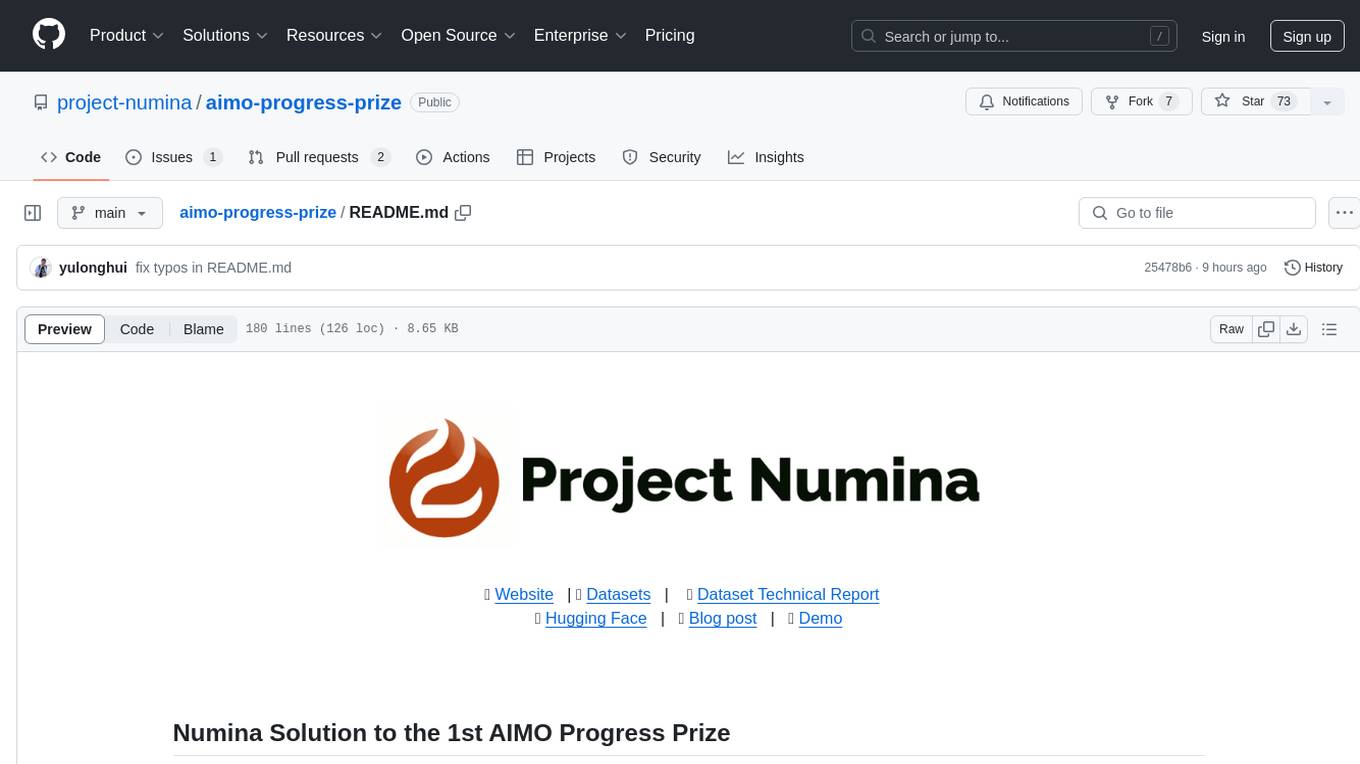
aimo-progress-prize
This repository contains the training and inference code needed to replicate the winning solution to the AI Mathematical Olympiad - Progress Prize 1. It consists of fine-tuning DeepSeekMath-Base 7B, high-quality training datasets, a self-consistency decoding algorithm, and carefully chosen validation sets. The training methodology involves Chain of Thought (CoT) and Tool Integrated Reasoning (TIR) training stages. Two datasets, NuminaMath-CoT and NuminaMath-TIR, were used to fine-tune the models. The models were trained using open-source libraries like TRL, PyTorch, vLLM, and DeepSpeed. Post-training quantization to 8-bit precision was done to improve performance on Kaggle's T4 GPUs. The project structure includes scripts for training, quantization, and inference, along with necessary installation instructions and hardware/software specifications.
For similar tasks
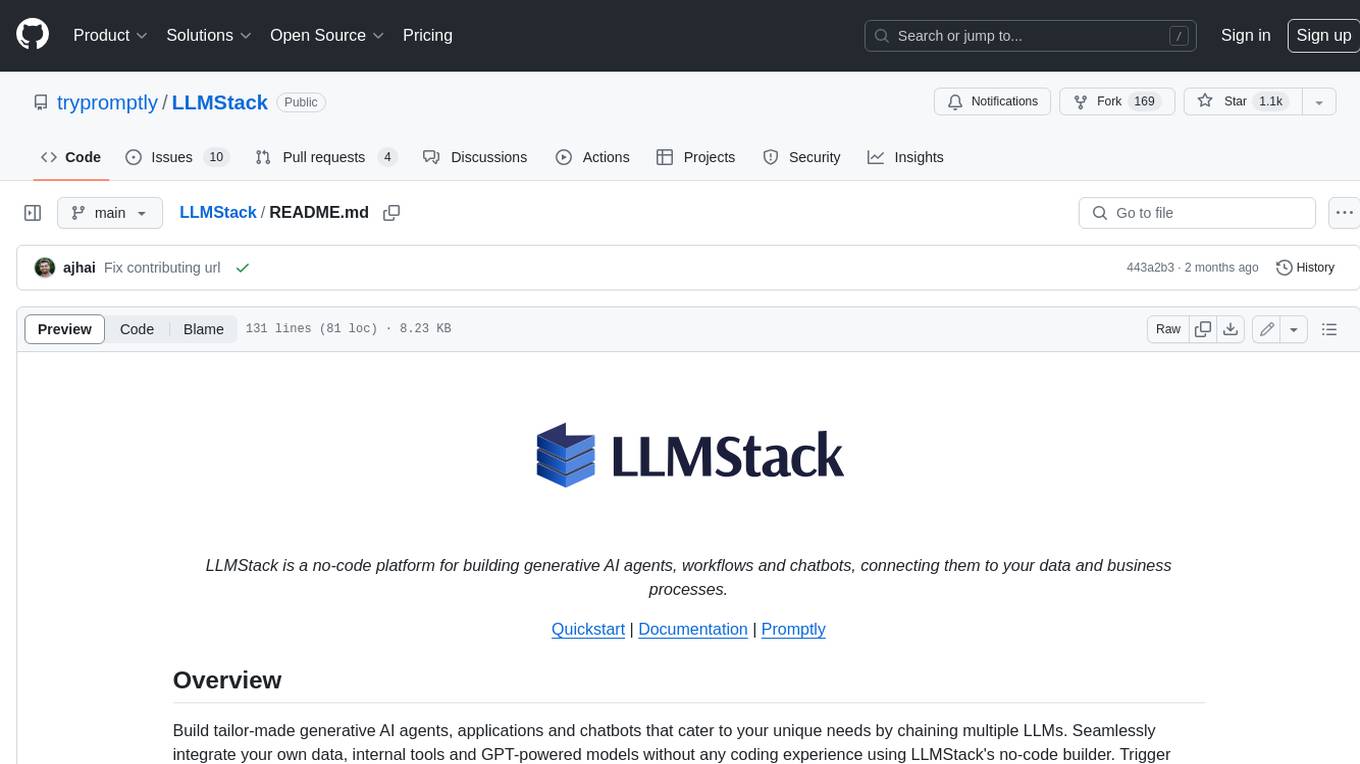
LLMStack
LLMStack is a no-code platform for building generative AI agents, workflows, and chatbots. It allows users to connect their own data, internal tools, and GPT-powered models without any coding experience. LLMStack can be deployed to the cloud or on-premise and can be accessed via HTTP API or triggered from Slack or Discord.
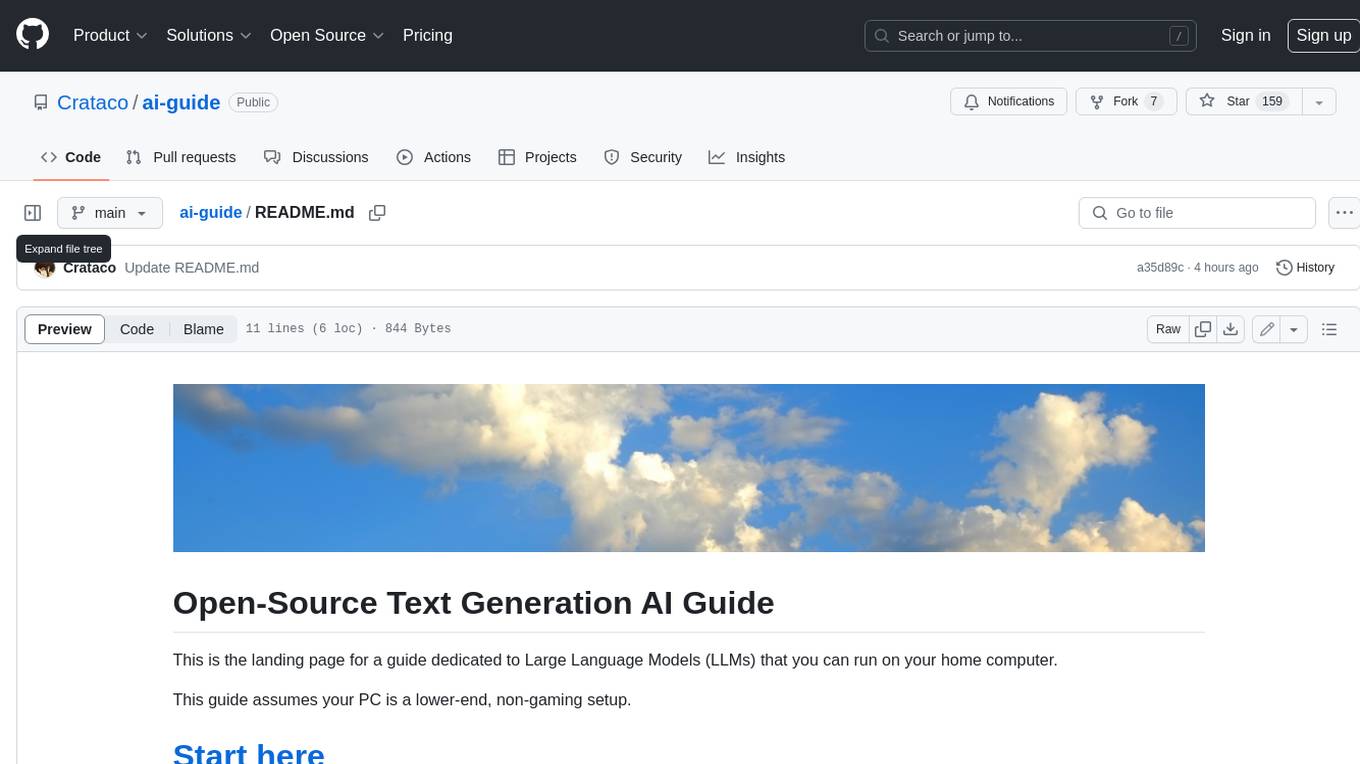
ai-guide
This guide is dedicated to Large Language Models (LLMs) that you can run on your home computer. It assumes your PC is a lower-end, non-gaming setup.
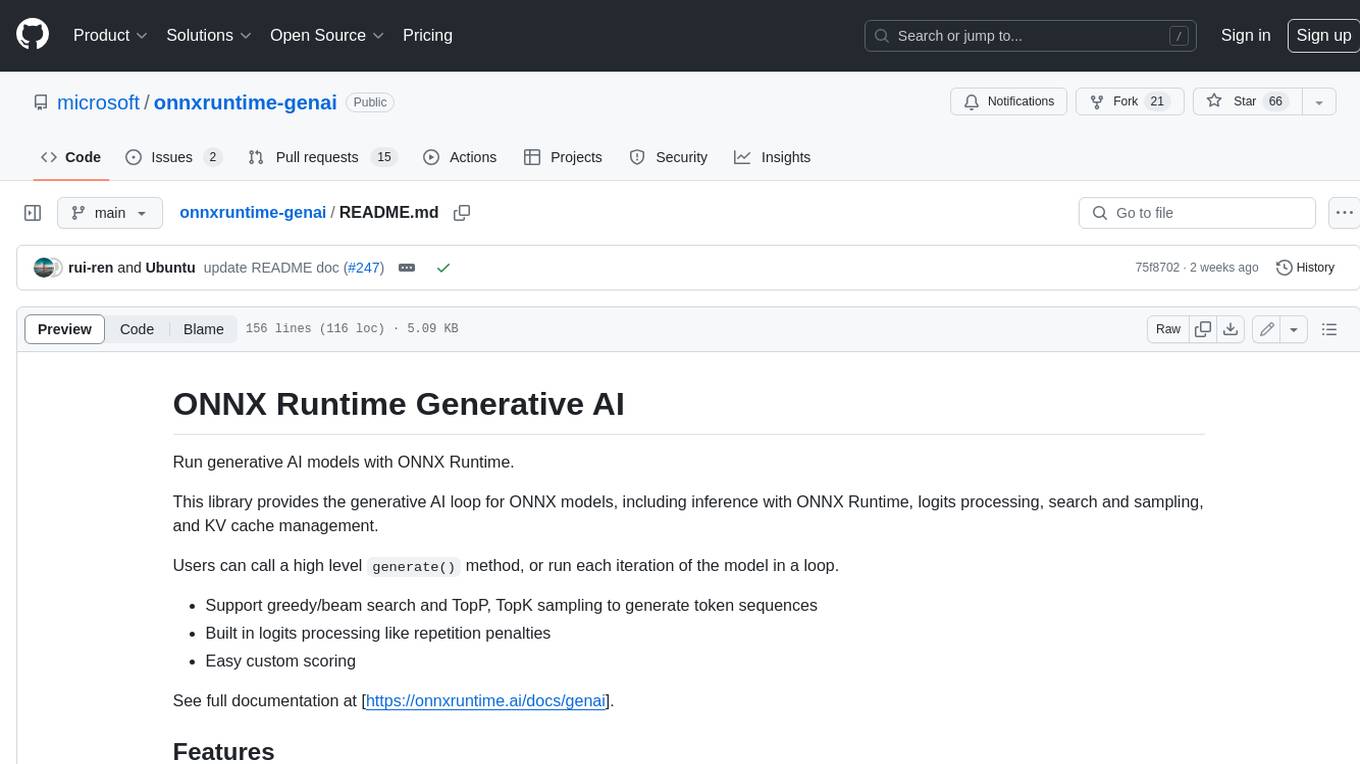
onnxruntime-genai
ONNX Runtime Generative AI is a library that provides the generative AI loop for ONNX models, including inference with ONNX Runtime, logits processing, search and sampling, and KV cache management. Users can call a high level `generate()` method, or run each iteration of the model in a loop. It supports greedy/beam search and TopP, TopK sampling to generate token sequences, has built in logits processing like repetition penalties, and allows for easy custom scoring.
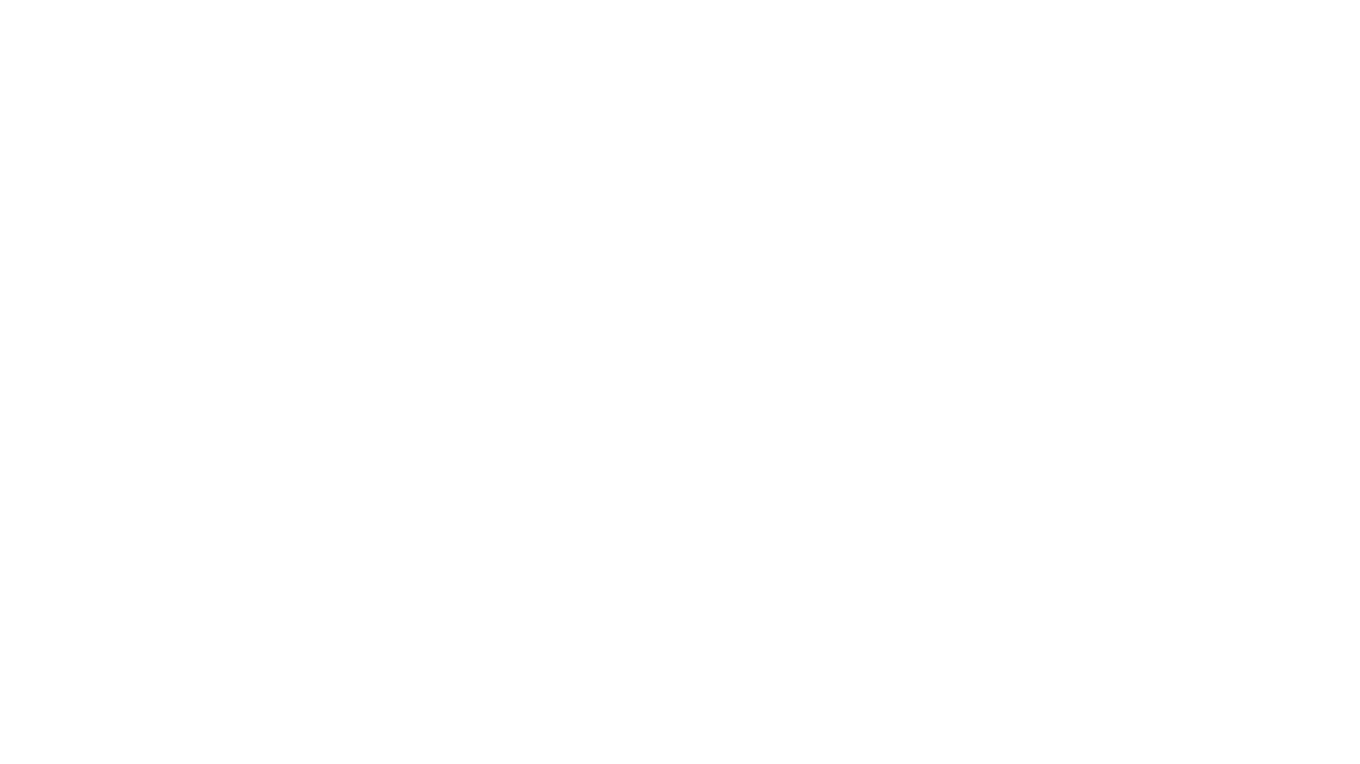
jupyter-ai
Jupyter AI connects generative AI with Jupyter notebooks. It provides a user-friendly and powerful way to explore generative AI models in notebooks and improve your productivity in JupyterLab and the Jupyter Notebook. Specifically, Jupyter AI offers: * An `%%ai` magic that turns the Jupyter notebook into a reproducible generative AI playground. This works anywhere the IPython kernel runs (JupyterLab, Jupyter Notebook, Google Colab, Kaggle, VSCode, etc.). * A native chat UI in JupyterLab that enables you to work with generative AI as a conversational assistant. * Support for a wide range of generative model providers, including AI21, Anthropic, AWS, Cohere, Gemini, Hugging Face, NVIDIA, and OpenAI. * Local model support through GPT4All, enabling use of generative AI models on consumer grade machines with ease and privacy.
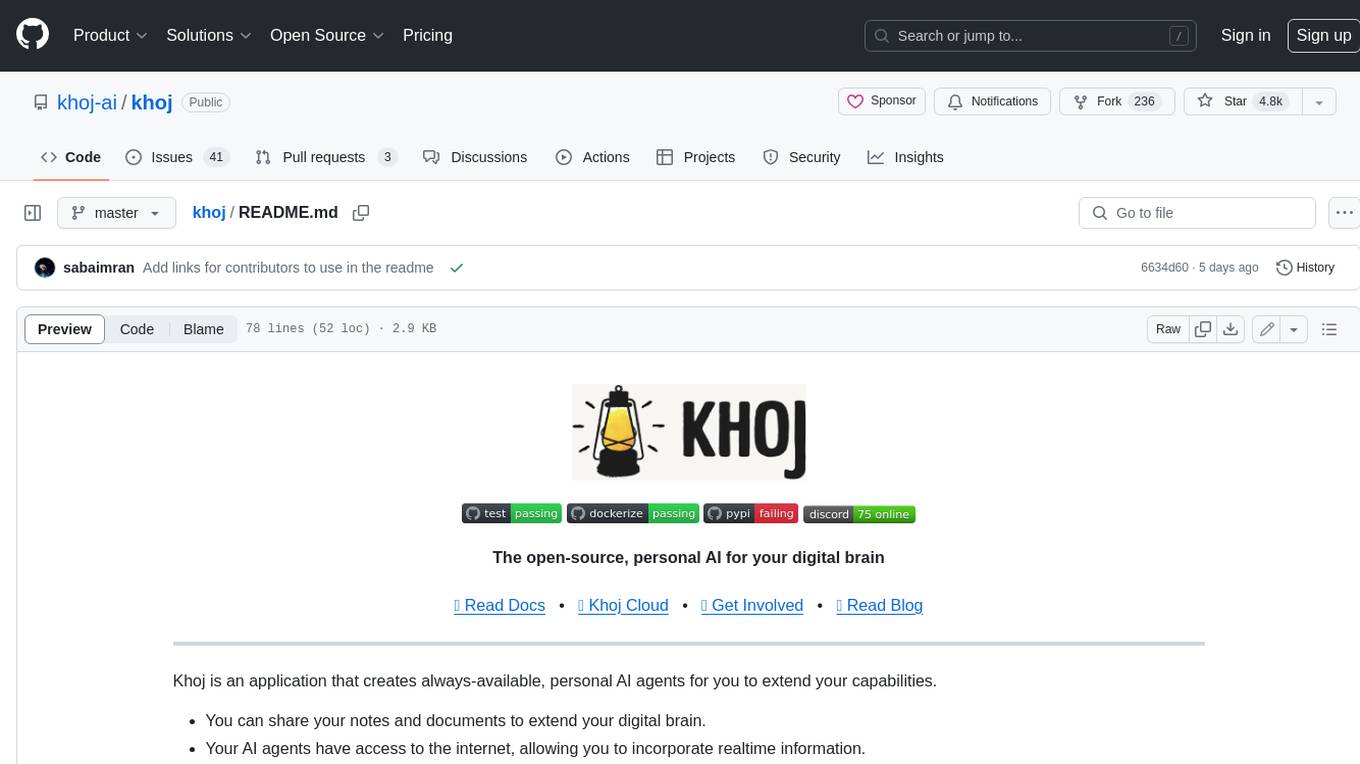
khoj
Khoj is an open-source, personal AI assistant that extends your capabilities by creating always-available AI agents. You can share your notes and documents to extend your digital brain, and your AI agents have access to the internet, allowing you to incorporate real-time information. Khoj is accessible on Desktop, Emacs, Obsidian, Web, and Whatsapp, and you can share PDF, markdown, org-mode, notion files, and GitHub repositories. You'll get fast, accurate semantic search on top of your docs, and your agents can create deeply personal images and understand your speech. Khoj is self-hostable and always will be.
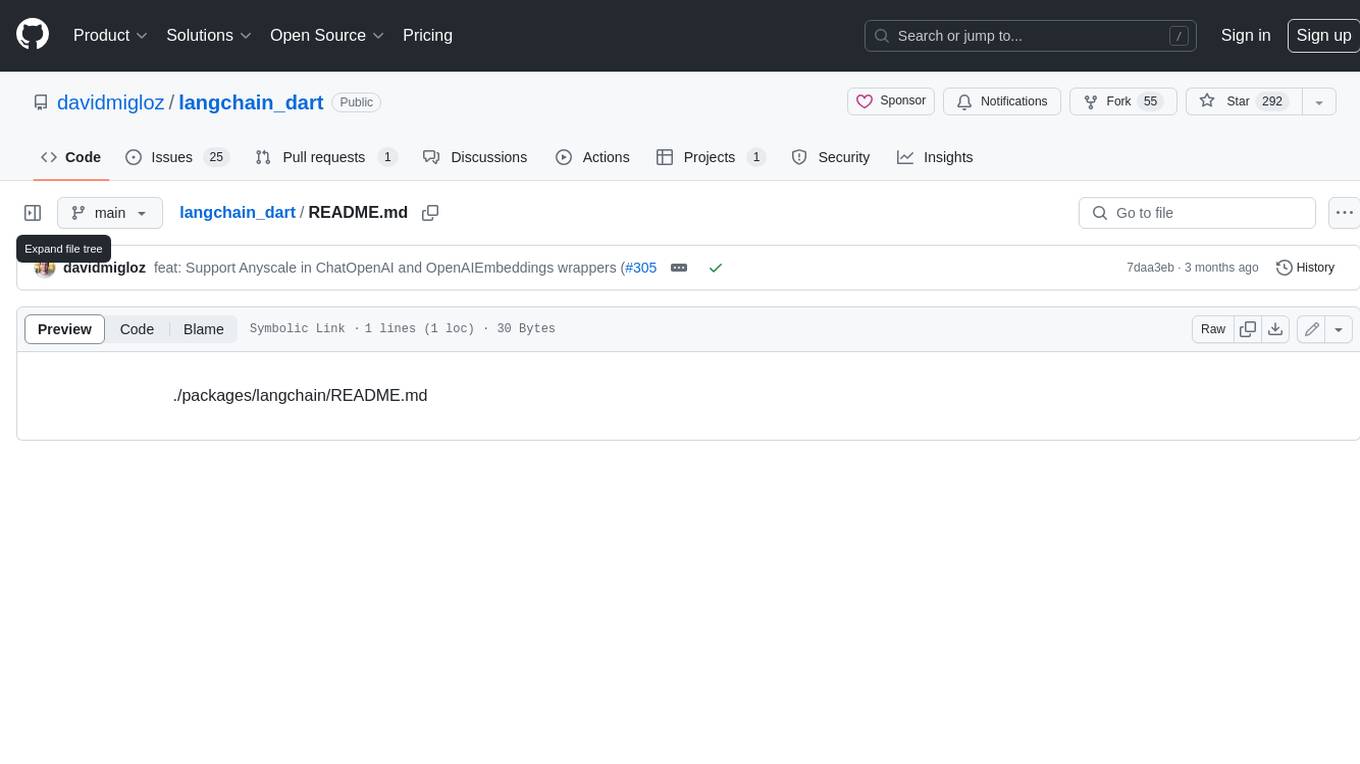
langchain_dart
LangChain.dart is a Dart port of the popular LangChain Python framework created by Harrison Chase. LangChain provides a set of ready-to-use components for working with language models and a standard interface for chaining them together to formulate more advanced use cases (e.g. chatbots, Q&A with RAG, agents, summarization, extraction, etc.). The components can be grouped into a few core modules: * **Model I/O:** LangChain offers a unified API for interacting with various LLM providers (e.g. OpenAI, Google, Mistral, Ollama, etc.), allowing developers to switch between them with ease. Additionally, it provides tools for managing model inputs (prompt templates and example selectors) and parsing the resulting model outputs (output parsers). * **Retrieval:** assists in loading user data (via document loaders), transforming it (with text splitters), extracting its meaning (using embedding models), storing (in vector stores) and retrieving it (through retrievers) so that it can be used to ground the model's responses (i.e. Retrieval-Augmented Generation or RAG). * **Agents:** "bots" that leverage LLMs to make informed decisions about which available tools (such as web search, calculators, database lookup, etc.) to use to accomplish the designated task. The different components can be composed together using the LangChain Expression Language (LCEL).
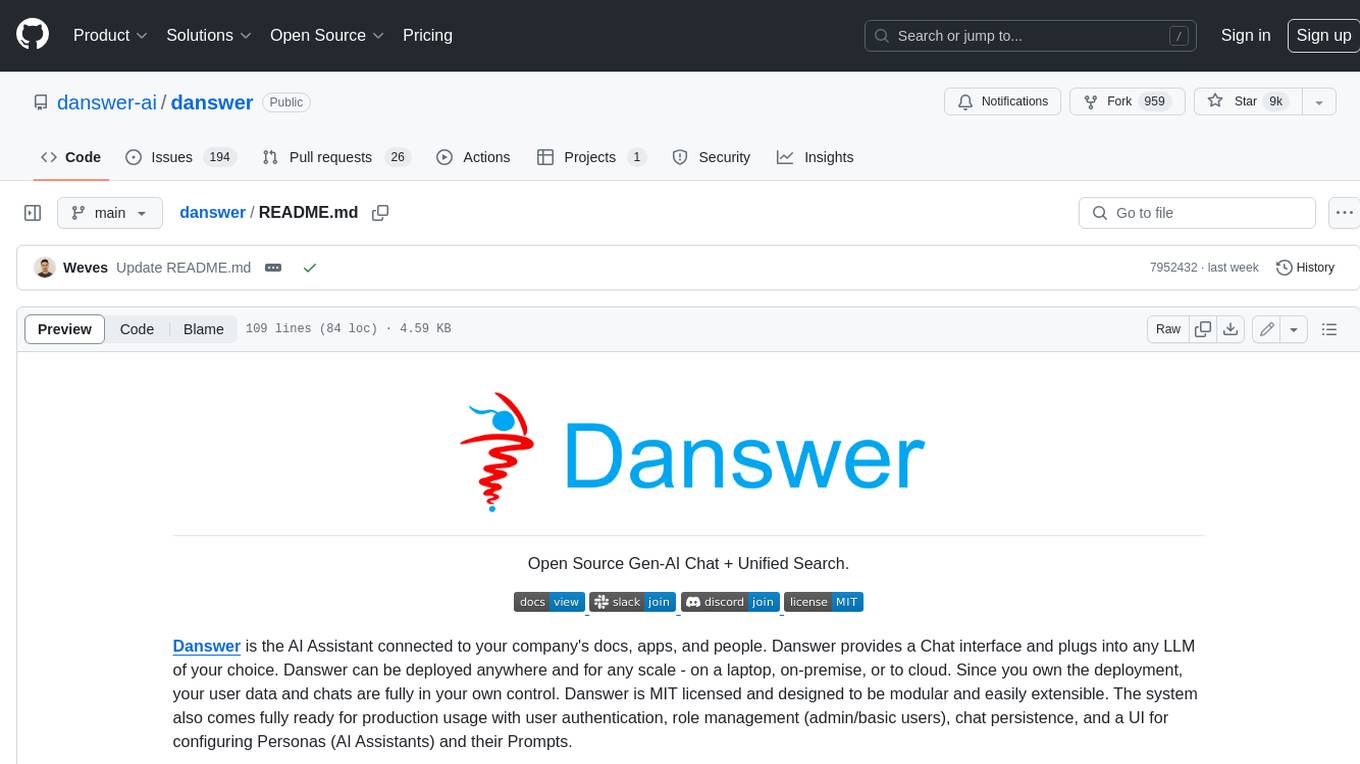
danswer
Danswer is an open-source Gen-AI Chat and Unified Search tool that connects to your company's docs, apps, and people. It provides a Chat interface and plugs into any LLM of your choice. Danswer can be deployed anywhere and for any scale - on a laptop, on-premise, or to cloud. Since you own the deployment, your user data and chats are fully in your own control. Danswer is MIT licensed and designed to be modular and easily extensible. The system also comes fully ready for production usage with user authentication, role management (admin/basic users), chat persistence, and a UI for configuring Personas (AI Assistants) and their Prompts. Danswer also serves as a Unified Search across all common workplace tools such as Slack, Google Drive, Confluence, etc. By combining LLMs and team specific knowledge, Danswer becomes a subject matter expert for the team. Imagine ChatGPT if it had access to your team's unique knowledge! It enables questions such as "A customer wants feature X, is this already supported?" or "Where's the pull request for feature Y?"
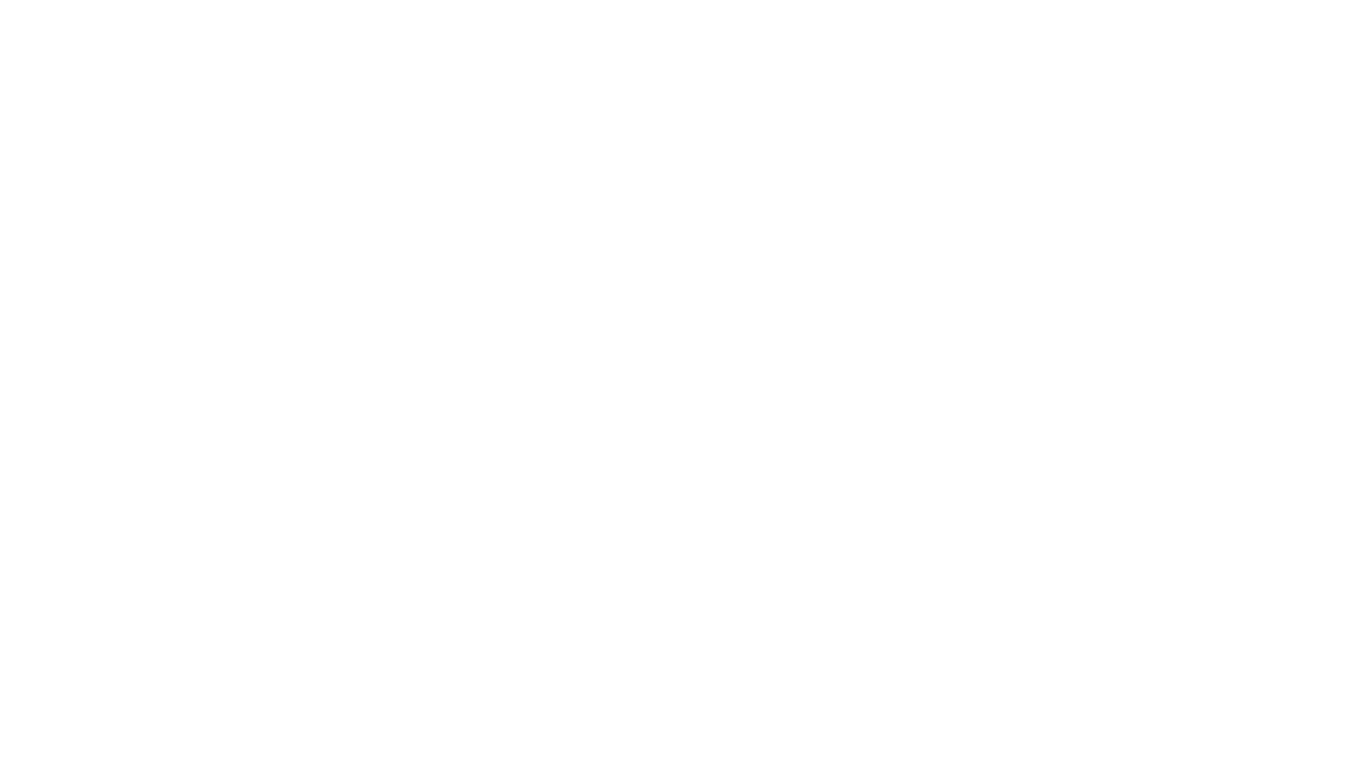
infinity
Infinity is an AI-native database designed for LLM applications, providing incredibly fast full-text and vector search capabilities. It supports a wide range of data types, including vectors, full-text, and structured data, and offers a fused search feature that combines multiple embeddings and full text. Infinity is easy to use, with an intuitive Python API and a single-binary architecture that simplifies deployment. It achieves high performance, with 0.1 milliseconds query latency on million-scale vector datasets and up to 15K QPS.
For similar jobs
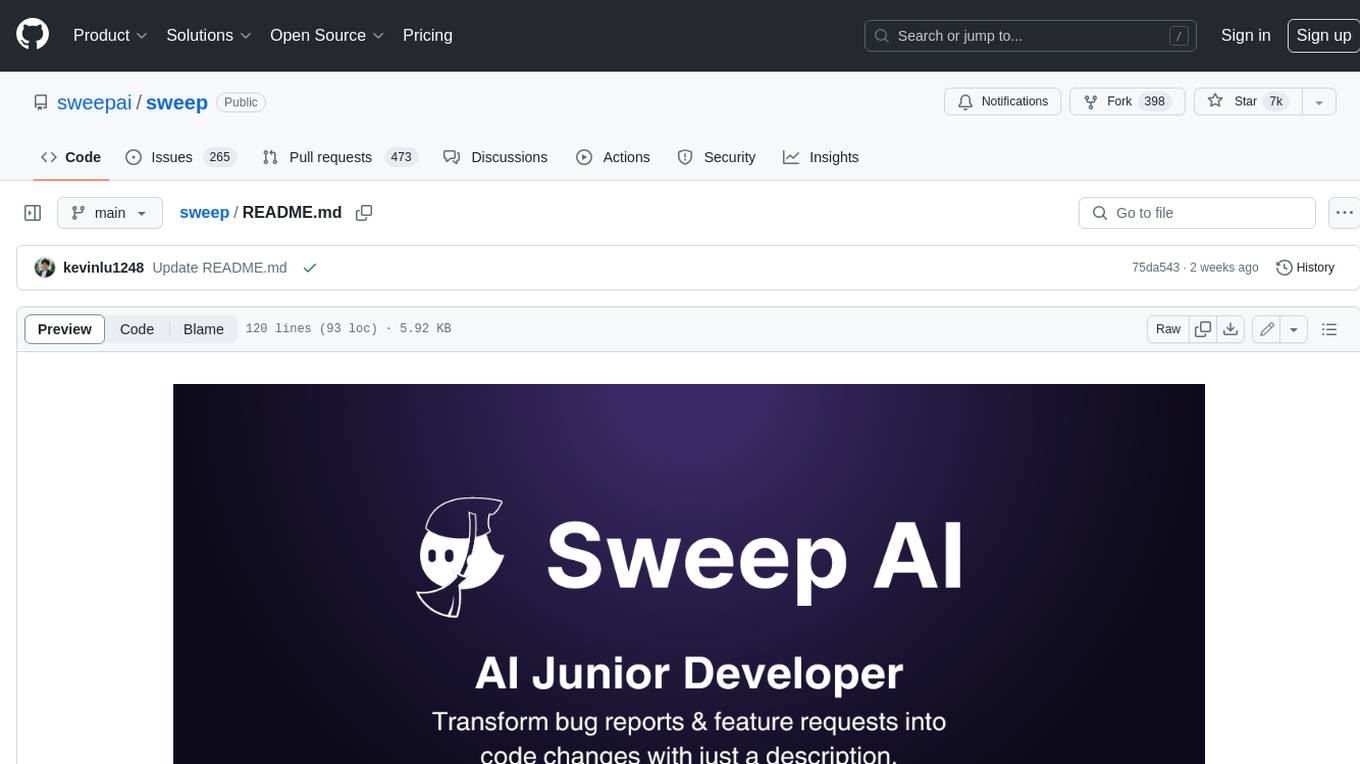
sweep
Sweep is an AI junior developer that turns bugs and feature requests into code changes. It automatically handles developer experience improvements like adding type hints and improving test coverage.
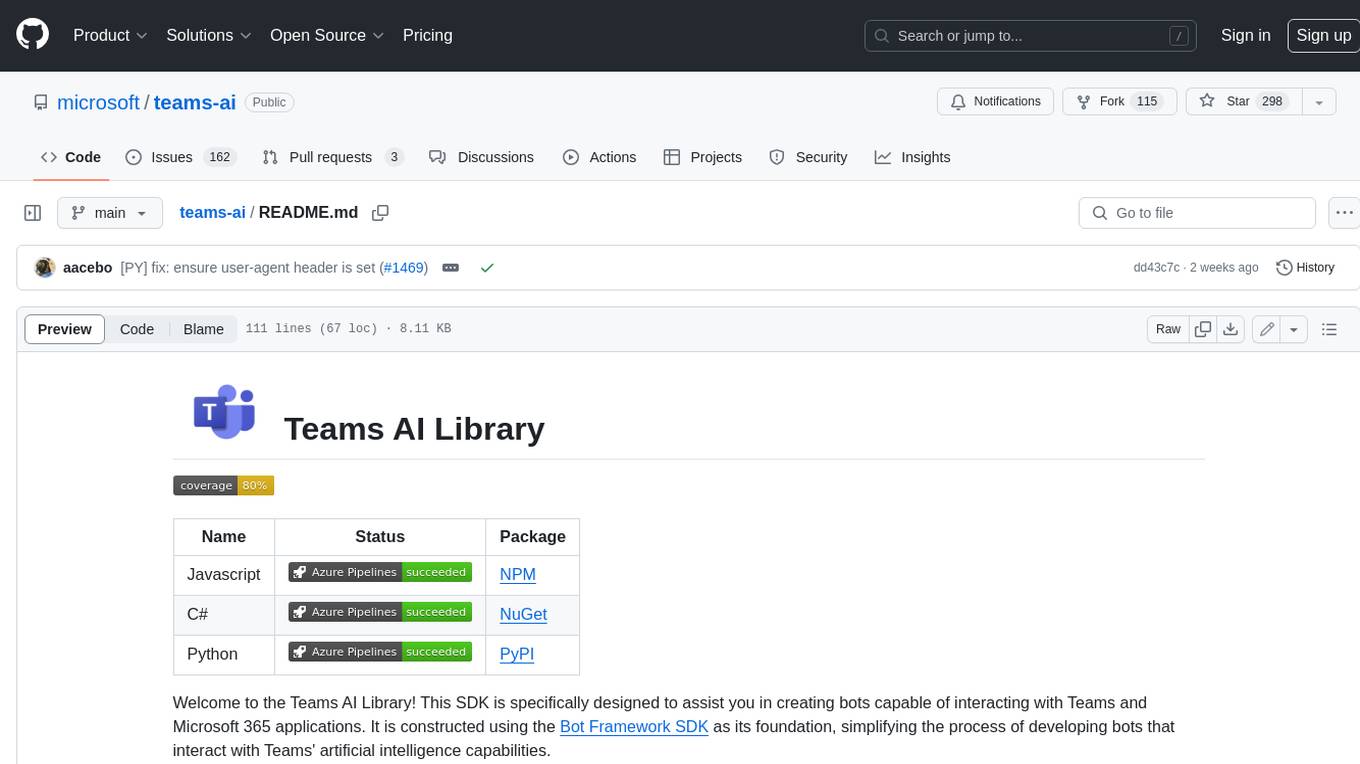
teams-ai
The Teams AI Library is a software development kit (SDK) that helps developers create bots that can interact with Teams and Microsoft 365 applications. It is built on top of the Bot Framework SDK and simplifies the process of developing bots that interact with Teams' artificial intelligence capabilities. The SDK is available for JavaScript/TypeScript, .NET, and Python.
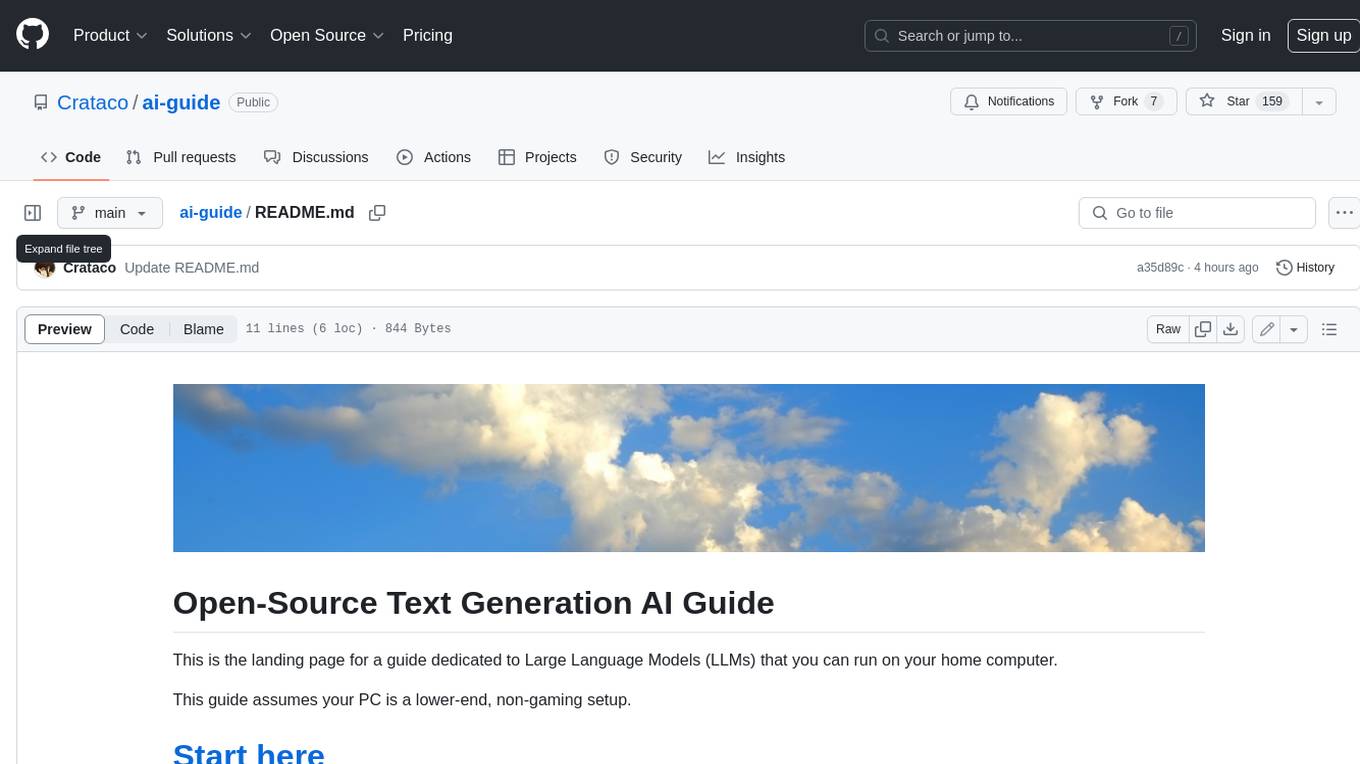
ai-guide
This guide is dedicated to Large Language Models (LLMs) that you can run on your home computer. It assumes your PC is a lower-end, non-gaming setup.
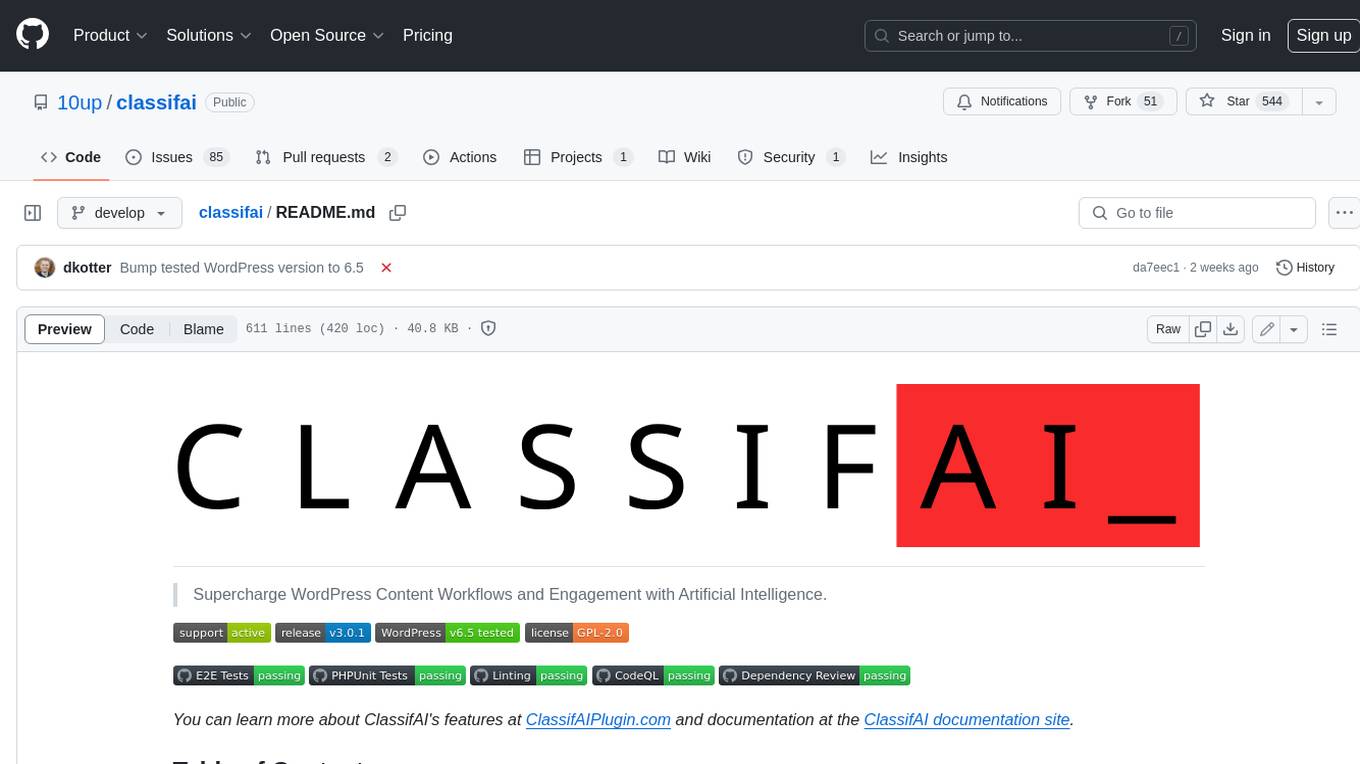
classifai
Supercharge WordPress Content Workflows and Engagement with Artificial Intelligence. Tap into leading cloud-based services like OpenAI, Microsoft Azure AI, Google Gemini and IBM Watson to augment your WordPress-powered websites. Publish content faster while improving SEO performance and increasing audience engagement. ClassifAI integrates Artificial Intelligence and Machine Learning technologies to lighten your workload and eliminate tedious tasks, giving you more time to create original content that matters.
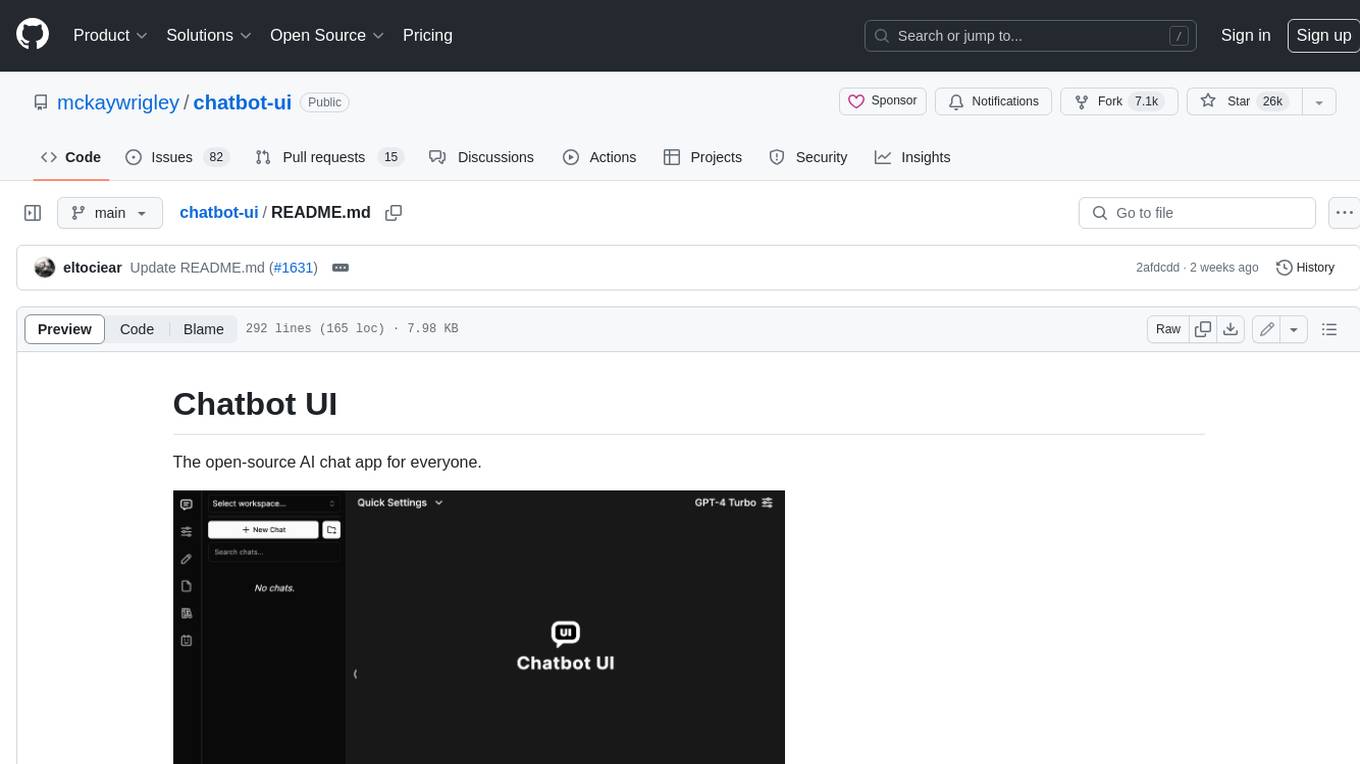
chatbot-ui
Chatbot UI is an open-source AI chat app that allows users to create and deploy their own AI chatbots. It is easy to use and can be customized to fit any need. Chatbot UI is perfect for businesses, developers, and anyone who wants to create a chatbot.
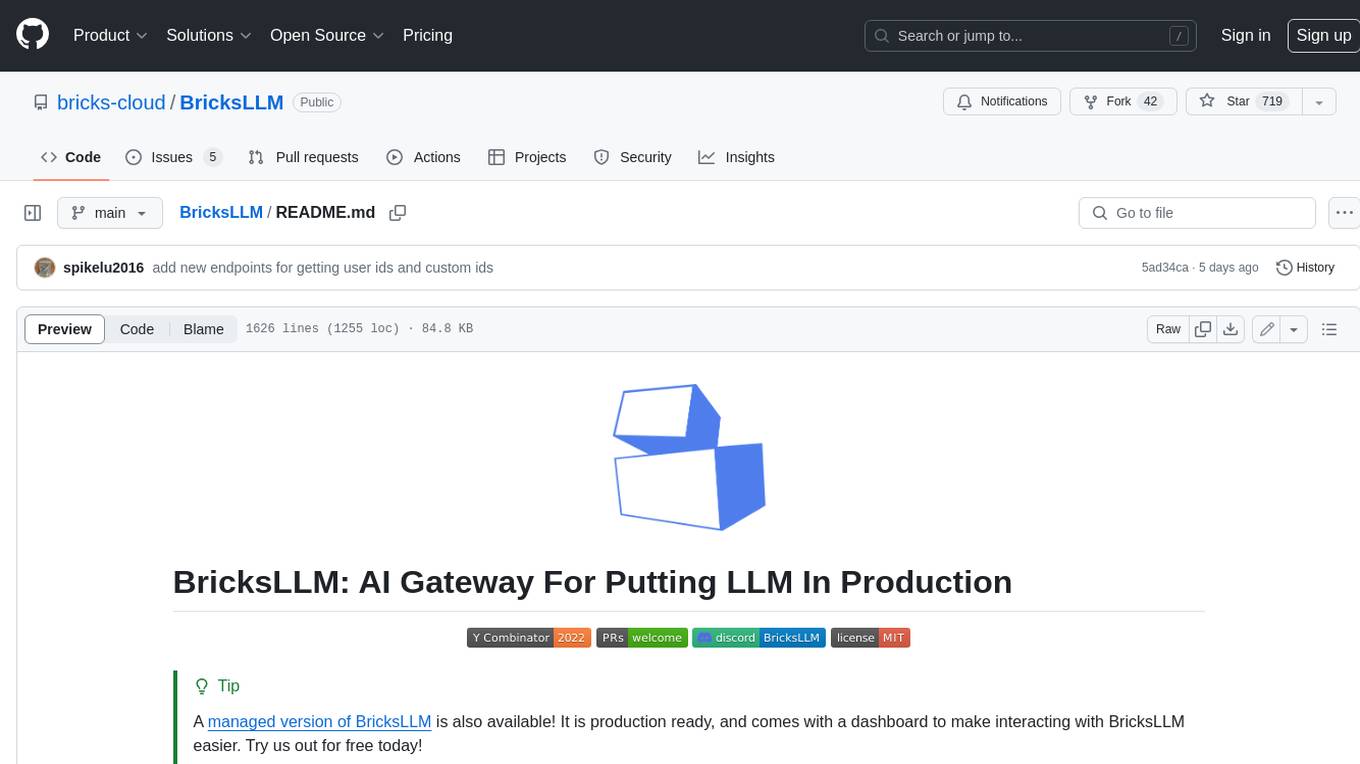
BricksLLM
BricksLLM is a cloud native AI gateway written in Go. Currently, it provides native support for OpenAI, Anthropic, Azure OpenAI and vLLM. BricksLLM aims to provide enterprise level infrastructure that can power any LLM production use cases. Here are some use cases for BricksLLM: * Set LLM usage limits for users on different pricing tiers * Track LLM usage on a per user and per organization basis * Block or redact requests containing PIIs * Improve LLM reliability with failovers, retries and caching * Distribute API keys with rate limits and cost limits for internal development/production use cases * Distribute API keys with rate limits and cost limits for students
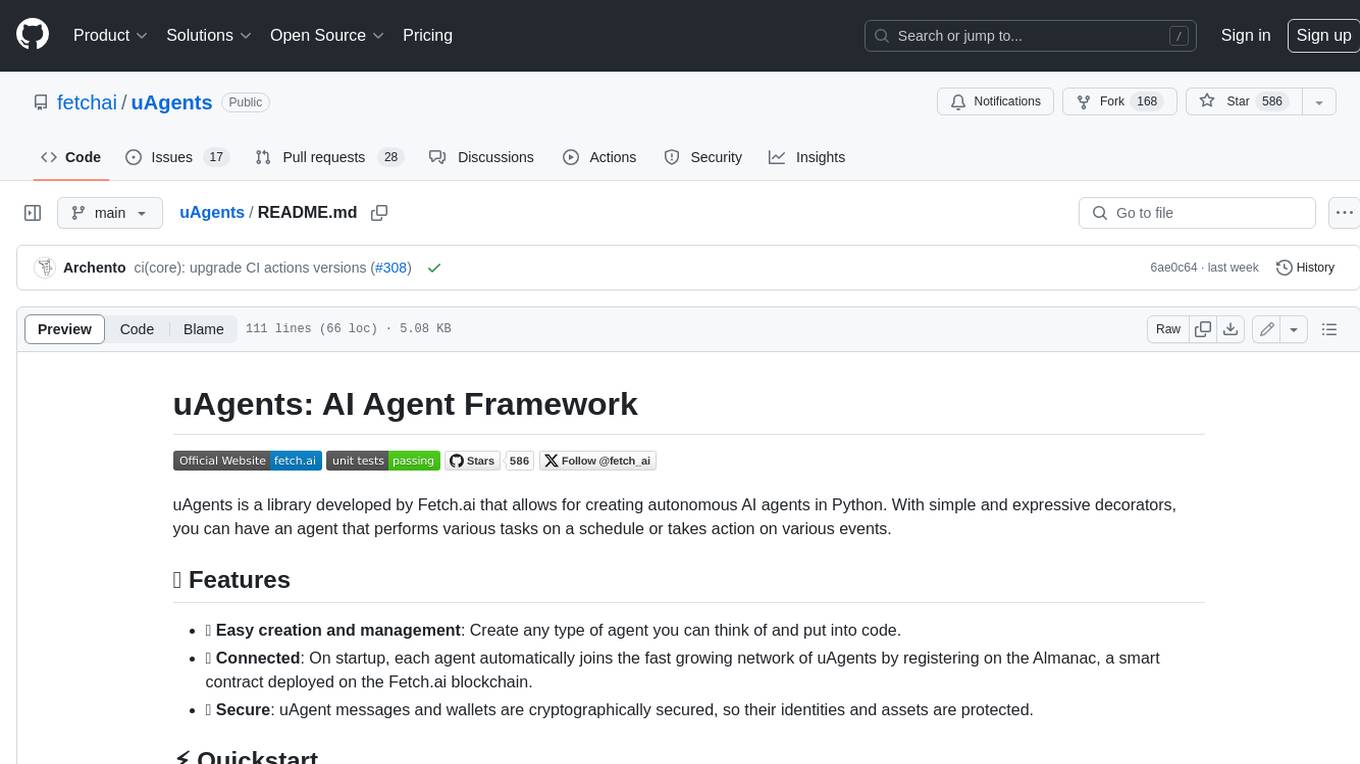
uAgents
uAgents is a Python library developed by Fetch.ai that allows for the creation of autonomous AI agents. These agents can perform various tasks on a schedule or take action on various events. uAgents are easy to create and manage, and they are connected to a fast-growing network of other uAgents. They are also secure, with cryptographically secured messages and wallets.
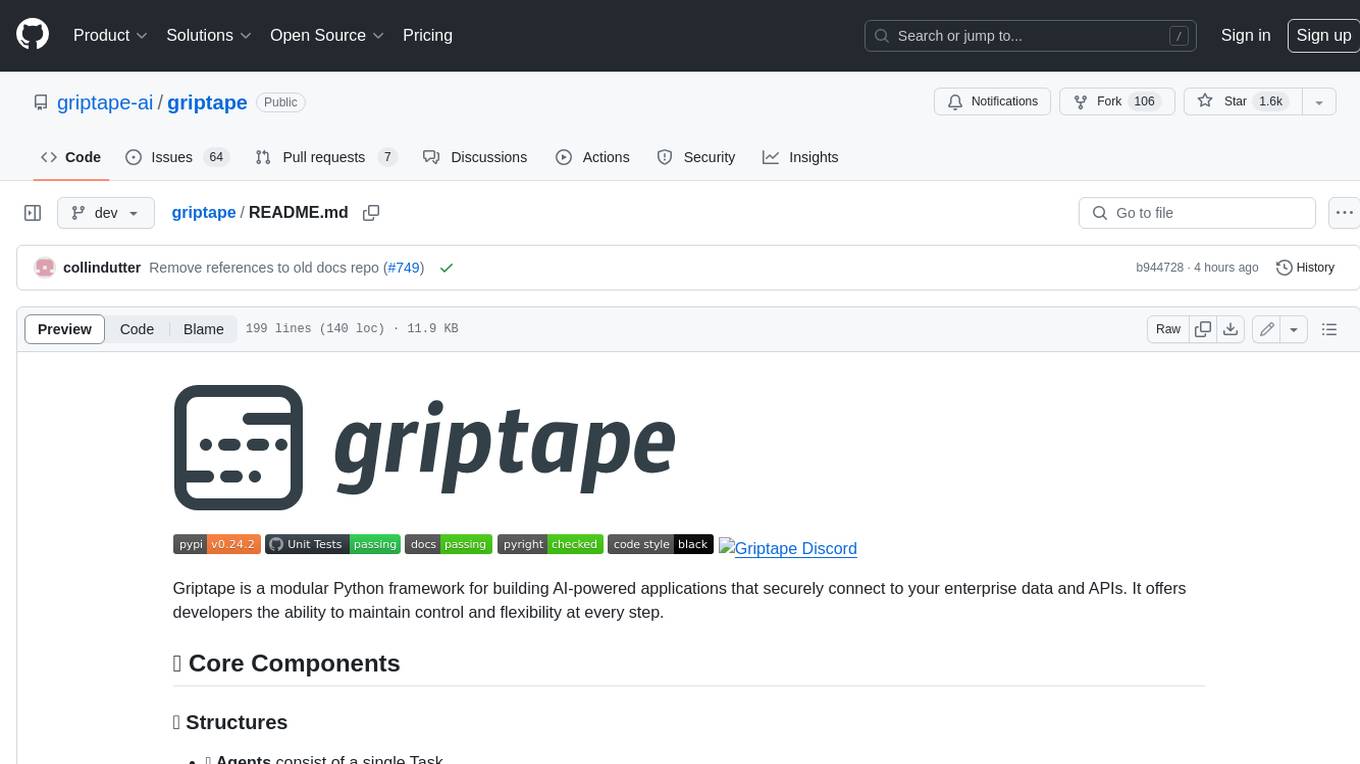
griptape
Griptape is a modular Python framework for building AI-powered applications that securely connect to your enterprise data and APIs. It offers developers the ability to maintain control and flexibility at every step. Griptape's core components include Structures (Agents, Pipelines, and Workflows), Tasks, Tools, Memory (Conversation Memory, Task Memory, and Meta Memory), Drivers (Prompt and Embedding Drivers, Vector Store Drivers, Image Generation Drivers, Image Query Drivers, SQL Drivers, Web Scraper Drivers, and Conversation Memory Drivers), Engines (Query Engines, Extraction Engines, Summary Engines, Image Generation Engines, and Image Query Engines), and additional components (Rulesets, Loaders, Artifacts, Chunkers, and Tokenizers). Griptape enables developers to create AI-powered applications with ease and efficiency.