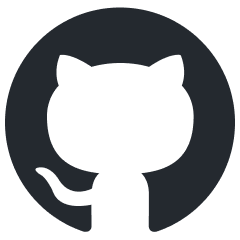
wikipedia-semantic-search
Semantic Search on Wikipedia with Upstash Vector
Stars: 371
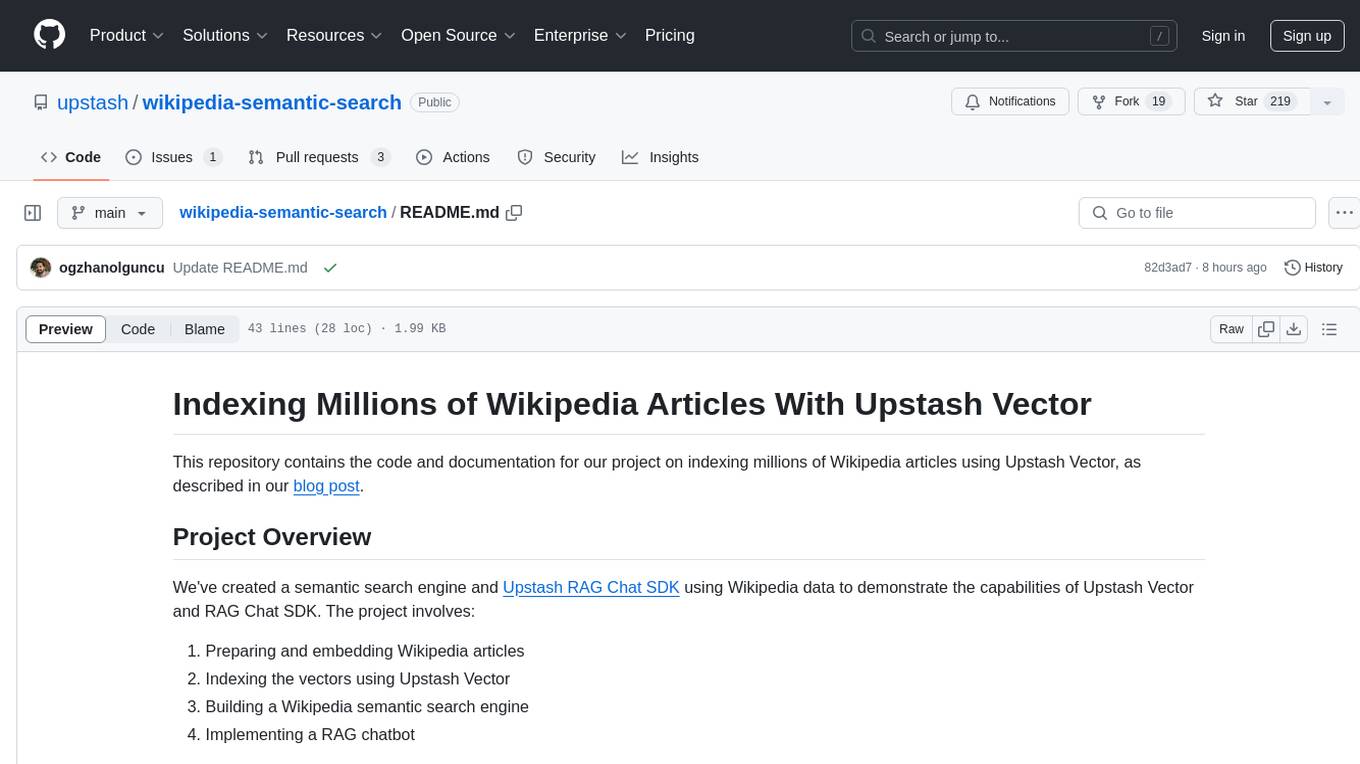
This repository showcases a project that indexes millions of Wikipedia articles using Upstash Vector. It includes a semantic search engine and a RAG chatbot SDK. The project involves preparing and embedding Wikipedia articles, indexing vectors, building a semantic search engine, and implementing a RAG chatbot. Key features include indexing over 144 million vectors, multilingual support, cross-lingual semantic search, and a RAG chatbot. Technologies used include Upstash Vector, Upstash Redis, Upstash RAG Chat SDK, SentenceTransformers, and Meta-Llama-3-8B-Instruct for LLM provider.
README:
This repository contains the code and documentation for our project on indexing millions of Wikipedia articles using Upstash Vector, as described in our blog post.
We've created a semantic search engine and Upstash RAG Chat SDK using Wikipedia data to demonstrate the capabilities of Upstash Vector and RAG Chat SDK. The project involves:
- Preparing and embedding Wikipedia articles
- Indexing the vectors using Upstash Vector
- Building a Wikipedia semantic search engine
- Implementing a RAG chatbot
- Indexed over 144 million vectors from Wikipedia articles in 11 languages
- Used BGE-M3 embedding model for multilingual support
- Implemented semantic search with cross-lingual capabilities
- Created a RAG chatbot using Upstash RAG Chat SDK
- Upstash Vector: For storing and querying vector embeddings
- Upstash Redis: For storing chat sessions
- Upstash RAG Chat SDK: For building the RAG Chat application
- SentenceTransformers: For generating embeddings
- Meta-Llama-3-8B-Instruct: As the LLM provider through QStash LLM APIs
To run the project locally, follow these steps:
- Go to Upstash Console to manage your databases:
- Create a new Vector database with embedding model support. You can choose the BGE-M3 model for multilingual support.
- Create a new Redis database for storing chat sessions.
- Copy the credentials for both Redis and Vector. Also copy the QStash credentials for using the upstash hosted LLM models.
Put the credentials in a .env
file in the root of the project. Your .env
file should look like this:
UPSTASH_VECTOR_REST_URL=
UPSTASH_VECTOR_REST_TOKEN=
UPSTASH_REDIS_REST_TOKEN=
UPSTASH_REDIS_REST_URL=
QSTASH_TOKEN=
- Populate your Vector index.
This project uses namespaces to store articles in different languages. So you have to upsert the vectors in the correct namespace. For english, upsert your vectors into the
en
namespace.
- Install the dependencies:
pnpm install
- Run the development server:
pnpm dev
We welcome contributions to improve this project. Please feel free to submit issues or pull requests.
- Wikipedia for providing the dataset
- Upstash for their vector database and RAG Chat SDK
- All contributors to the open-source libraries used in this project
For any questions or feedback about the project or Upstash Vector, please reach out to us at (add contact information).
Check out our live demo to see the project in action!
For Tasks:
Click tags to check more tools for each tasksFor Jobs:
Alternative AI tools for wikipedia-semantic-search
Similar Open Source Tools
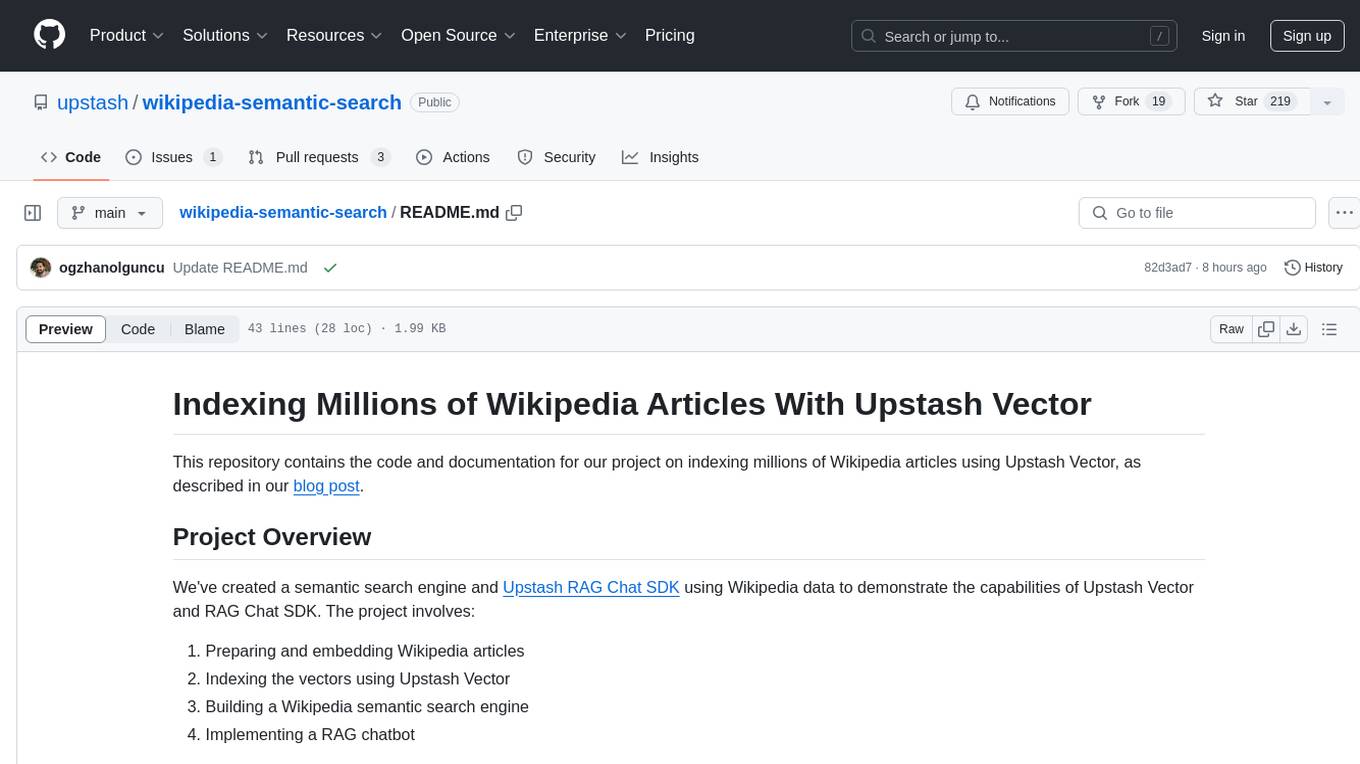
wikipedia-semantic-search
This repository showcases a project that indexes millions of Wikipedia articles using Upstash Vector. It includes a semantic search engine and a RAG chatbot SDK. The project involves preparing and embedding Wikipedia articles, indexing vectors, building a semantic search engine, and implementing a RAG chatbot. Key features include indexing over 144 million vectors, multilingual support, cross-lingual semantic search, and a RAG chatbot. Technologies used include Upstash Vector, Upstash Redis, Upstash RAG Chat SDK, SentenceTransformers, and Meta-Llama-3-8B-Instruct for LLM provider.
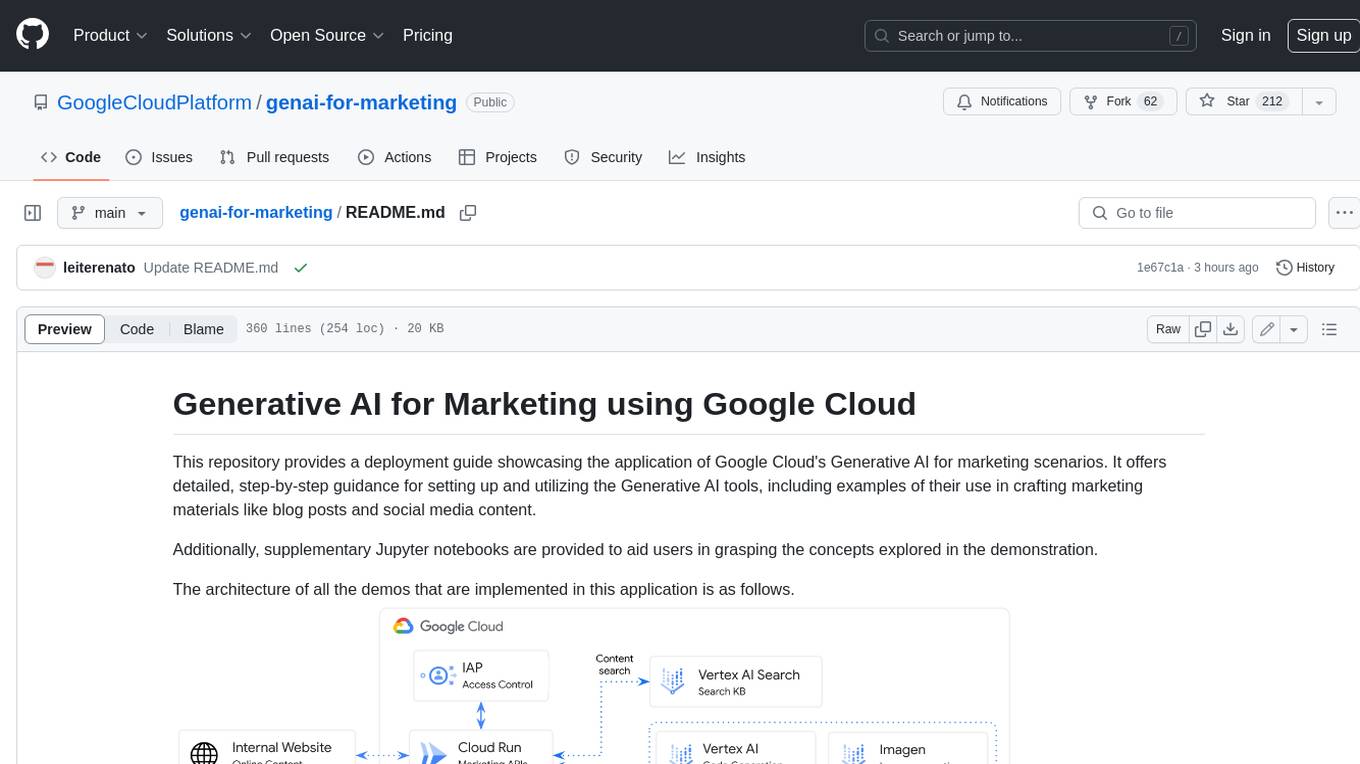
genai-for-marketing
This repository provides a deployment guide for utilizing Google Cloud's Generative AI tools in marketing scenarios. It includes step-by-step instructions, examples of crafting marketing materials, and supplementary Jupyter notebooks. The demos cover marketing insights, audience analysis, trendspotting, content search, content generation, and workspace integration. Users can access and visualize marketing data, analyze trends, improve search experience, and generate compelling content. The repository structure includes backend APIs, frontend code, sample notebooks, templates, and installation scripts.
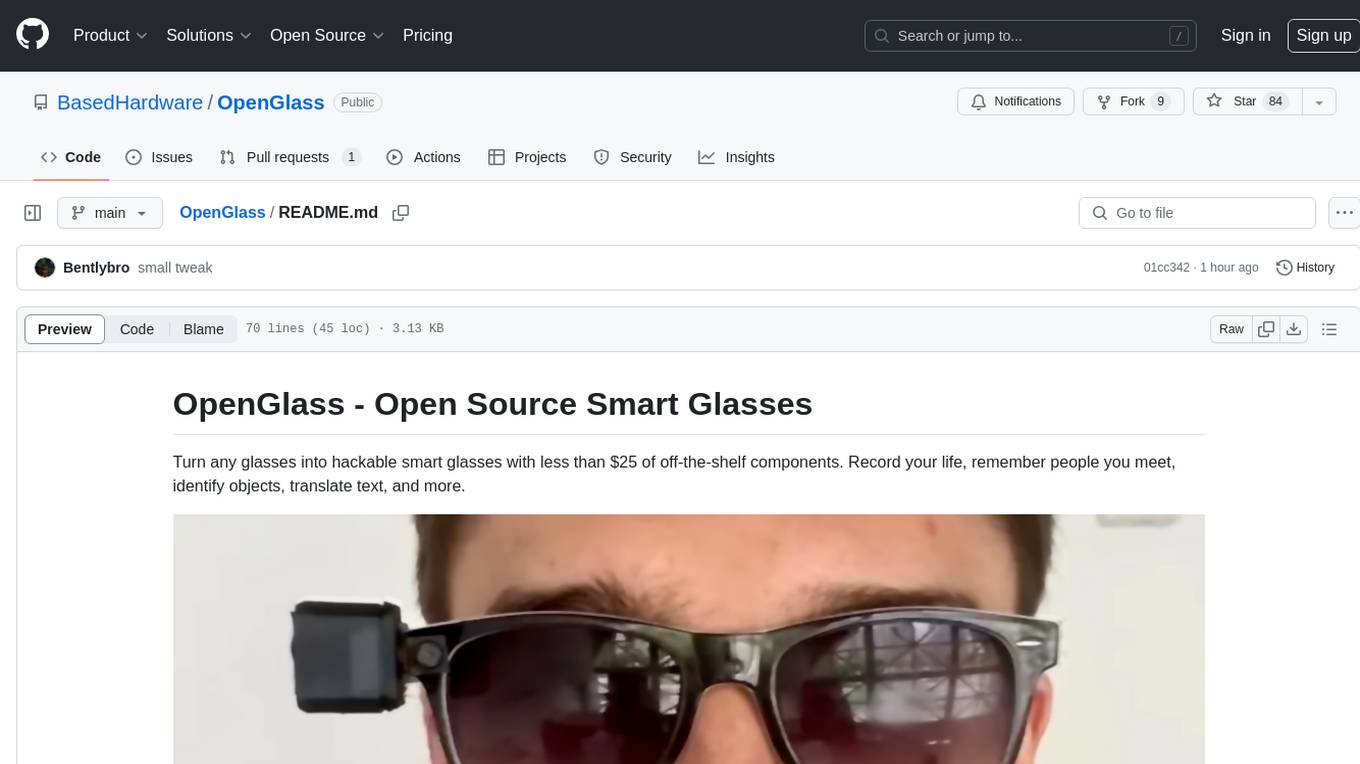
OpenGlass
OpenGlass is an open-source project that allows users to transform any regular glasses into smart glasses using affordable off-the-shelf components. With a cost of less than $25, users can enhance their glasses to record their daily activities, recognize people, identify objects, translate text, and more. The project provides detailed instructions on hardware setup and software installation, making it accessible for DIY enthusiasts and tech enthusiasts alike. By following the steps outlined in the repository, users can create their own smart glasses and explore various functionalities offered by the project.
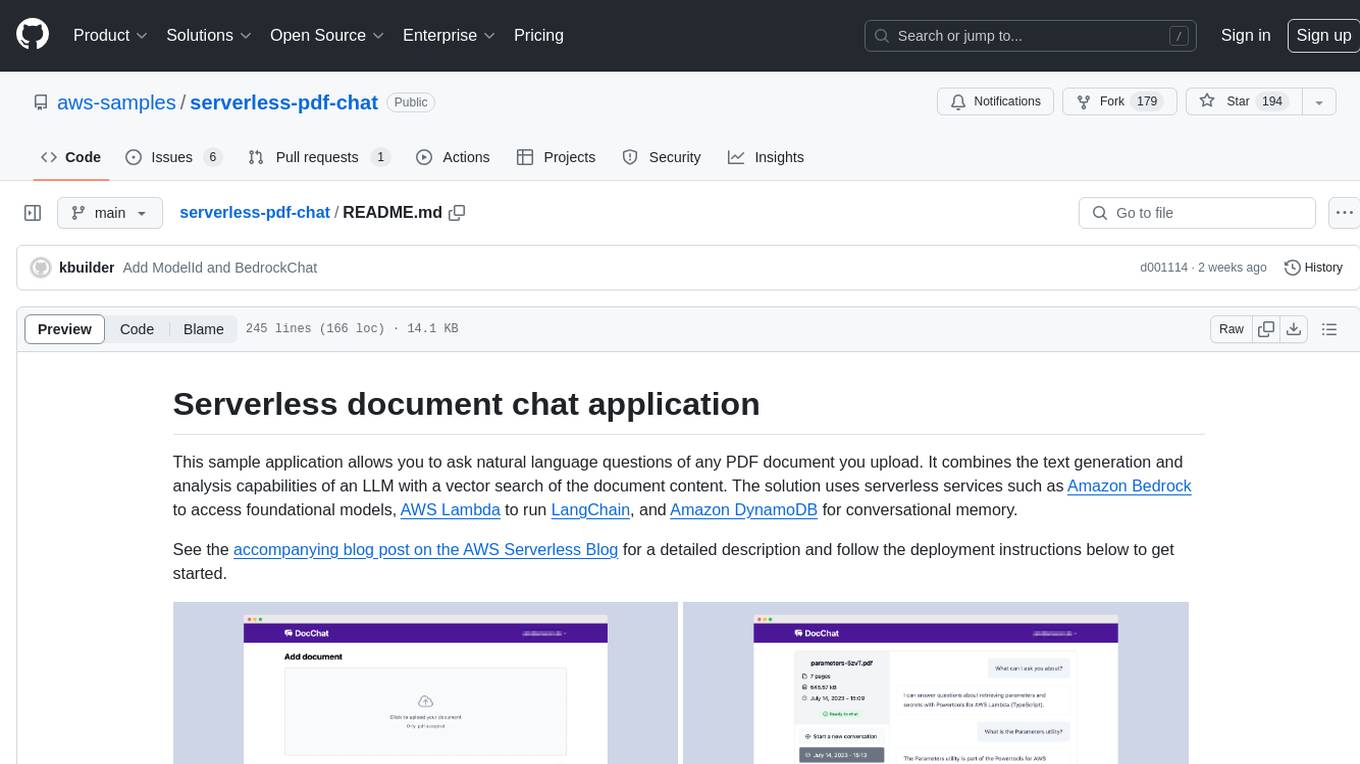
serverless-pdf-chat
The serverless-pdf-chat repository contains a sample application that allows users to ask natural language questions of any PDF document they upload. It leverages serverless services like Amazon Bedrock, AWS Lambda, and Amazon DynamoDB to provide text generation and analysis capabilities. The application architecture involves uploading a PDF document to an S3 bucket, extracting metadata, converting text to vectors, and using a LangChain to search for information related to user prompts. The application is not intended for production use and serves as a demonstration and educational tool.
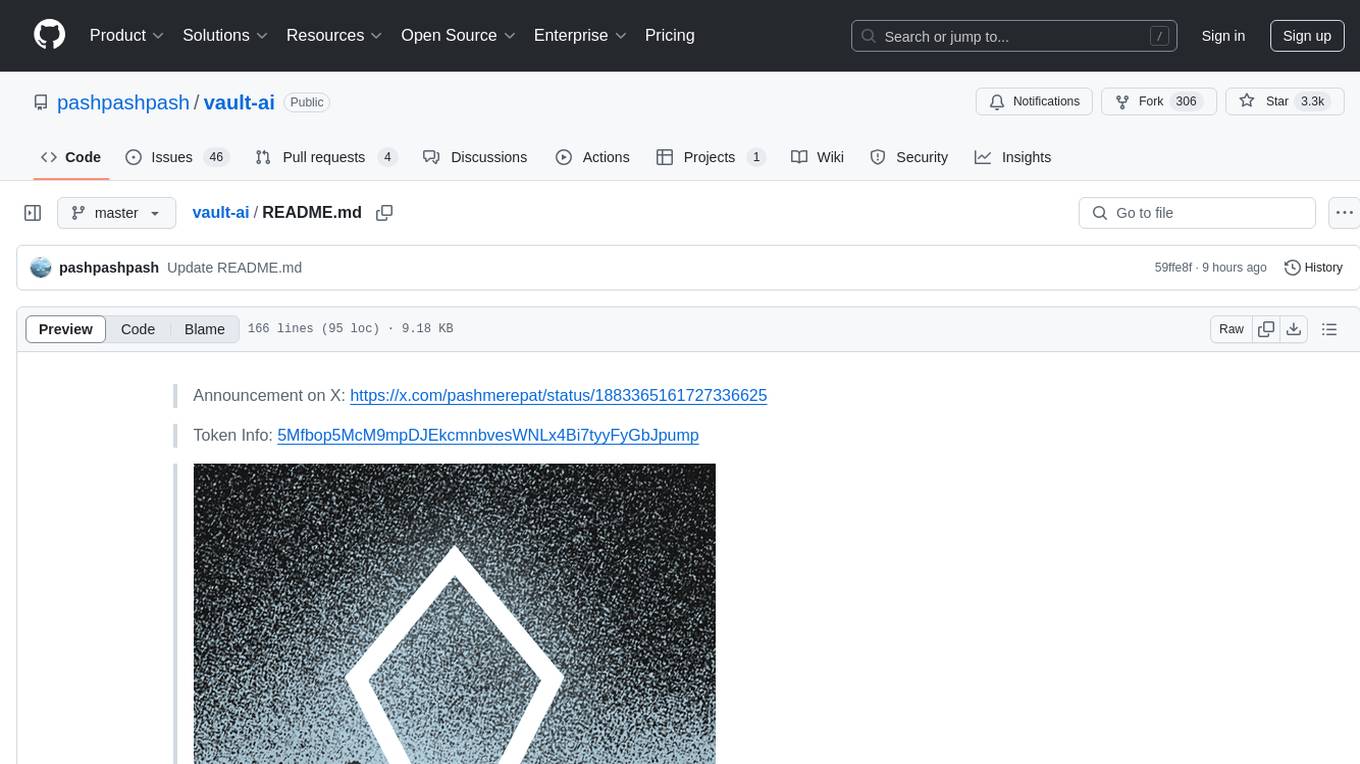
vault-ai
OP Vault is a tool that leverages the OP Stack (OpenAI + Pinecone Vector Database) to allow users to upload custom knowledgebase files and ask questions about their contents. It provides a user-friendly Golang server and React frontend for querying human-readable content like books and documents, making it valuable for knowledge extraction and question-answering. Users can upload entire libraries, receive specific answers with file and section references, and explore the power of the OP Stack in a practical interface.
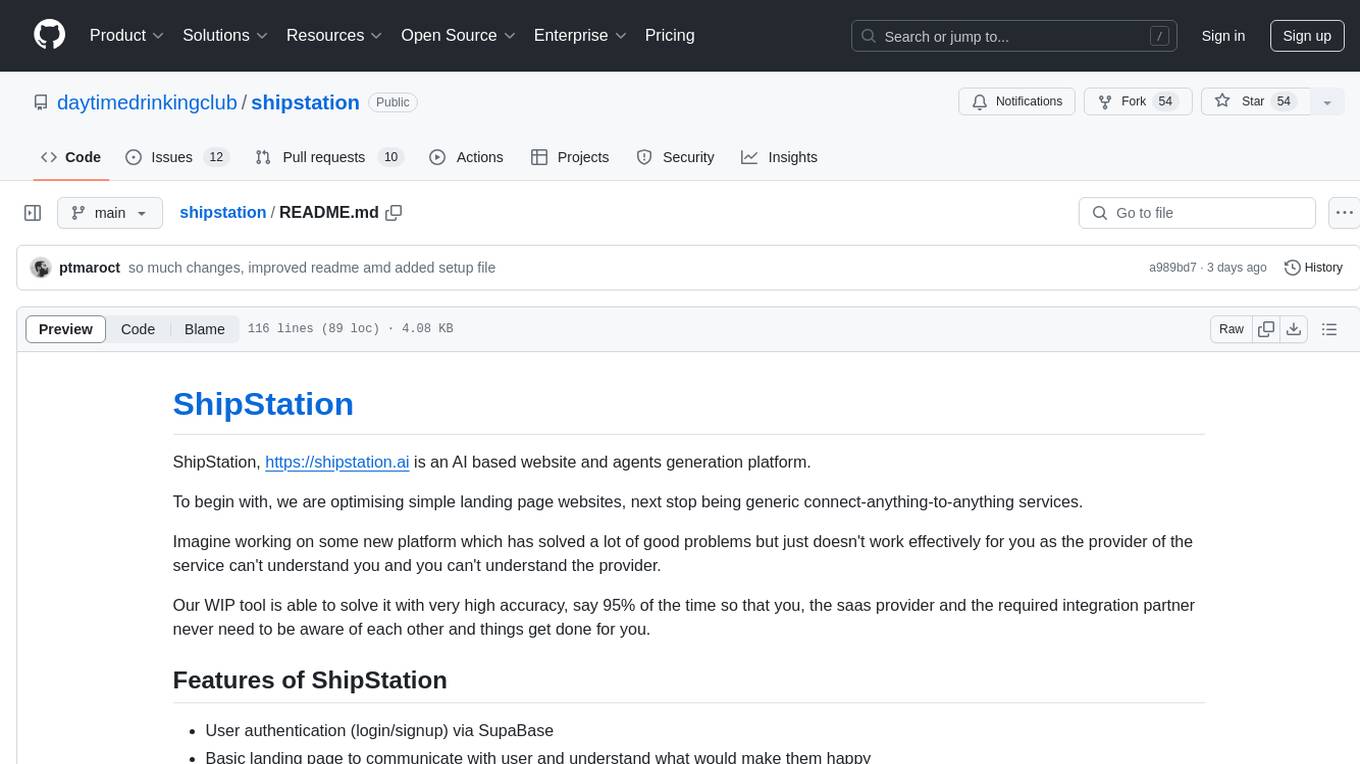
shipstation
ShipStation is an AI-based website and agents generation platform that optimizes landing page websites and generic connect-anything-to-anything services. It enables seamless communication between service providers and integration partners, offering features like user authentication, project management, code editing, payment integration, and real-time progress tracking. The project architecture includes server-side (Node.js) and client-side (React with Vite) components. Prerequisites include Node.js, npm or yarn, Anthropic API key, Supabase account, Tavily API key, and Razorpay account. Setup instructions involve cloning the repository, setting up Supabase, configuring environment variables, and starting the backend and frontend servers. Users can access the application through the browser, sign up or log in, create landing pages or portfolios, and get websites stored in an S3 bucket. Deployment to Heroku involves building the client project, committing changes, and pushing to the main branch. Contributions to the project are encouraged, and the license encourages doing good.
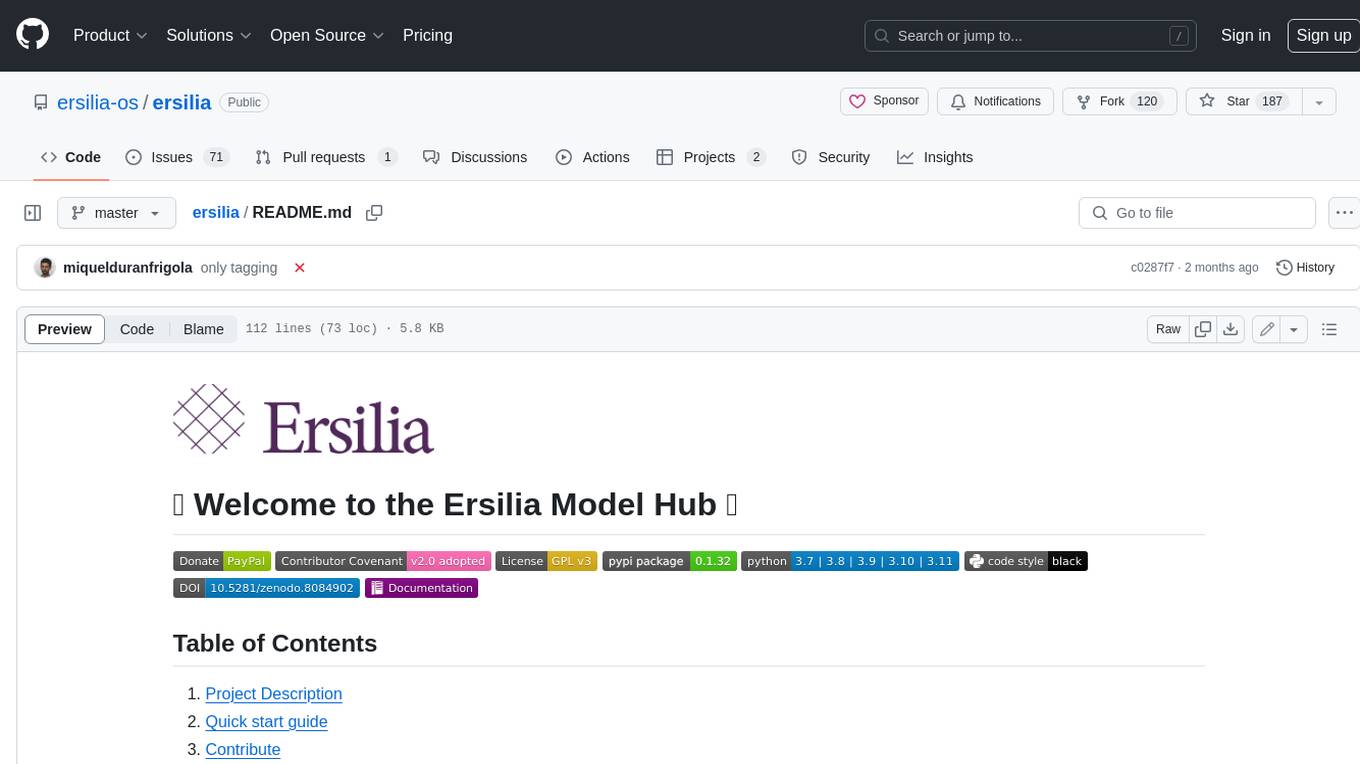
ersilia
The Ersilia Model Hub is a unified platform of pre-trained AI/ML models dedicated to infectious and neglected disease research. It offers an open-source, low-code solution that provides seamless access to AI/ML models for drug discovery. Models housed in the hub come from two sources: published models from literature (with due third-party acknowledgment) and custom models developed by the Ersilia team or contributors.
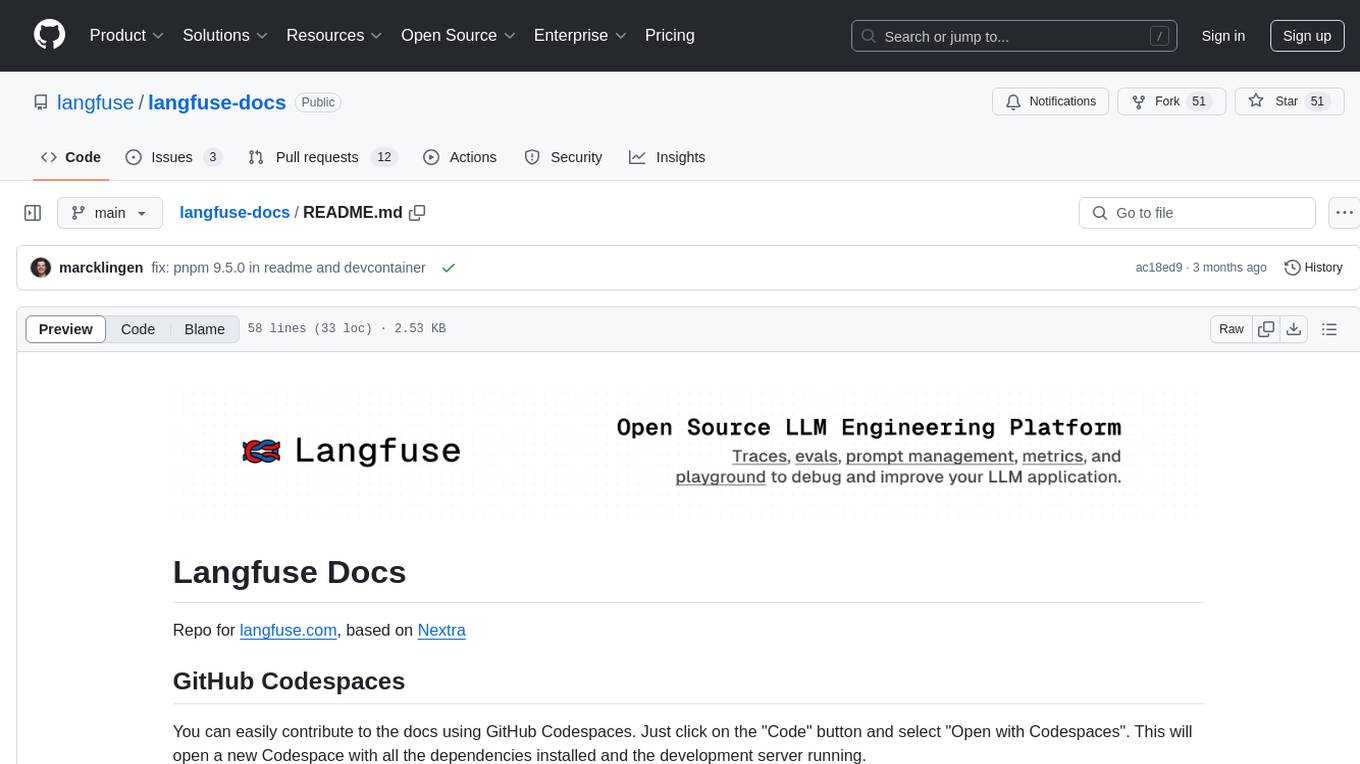
langfuse-docs
Langfuse Docs is a repository for langfuse.com, built on Nextra. It provides guidelines for contributing to the documentation using GitHub Codespaces and local development setup. The repository includes Python cookbooks in Jupyter notebooks format, which are converted to markdown for rendering on the site. It also covers media management for images, videos, and gifs. The stack includes Nextra, Next.js, shadcn/ui, and Tailwind CSS. Additionally, there is a bundle analysis feature to analyze the production build bundle size using @next/bundle-analyzer.
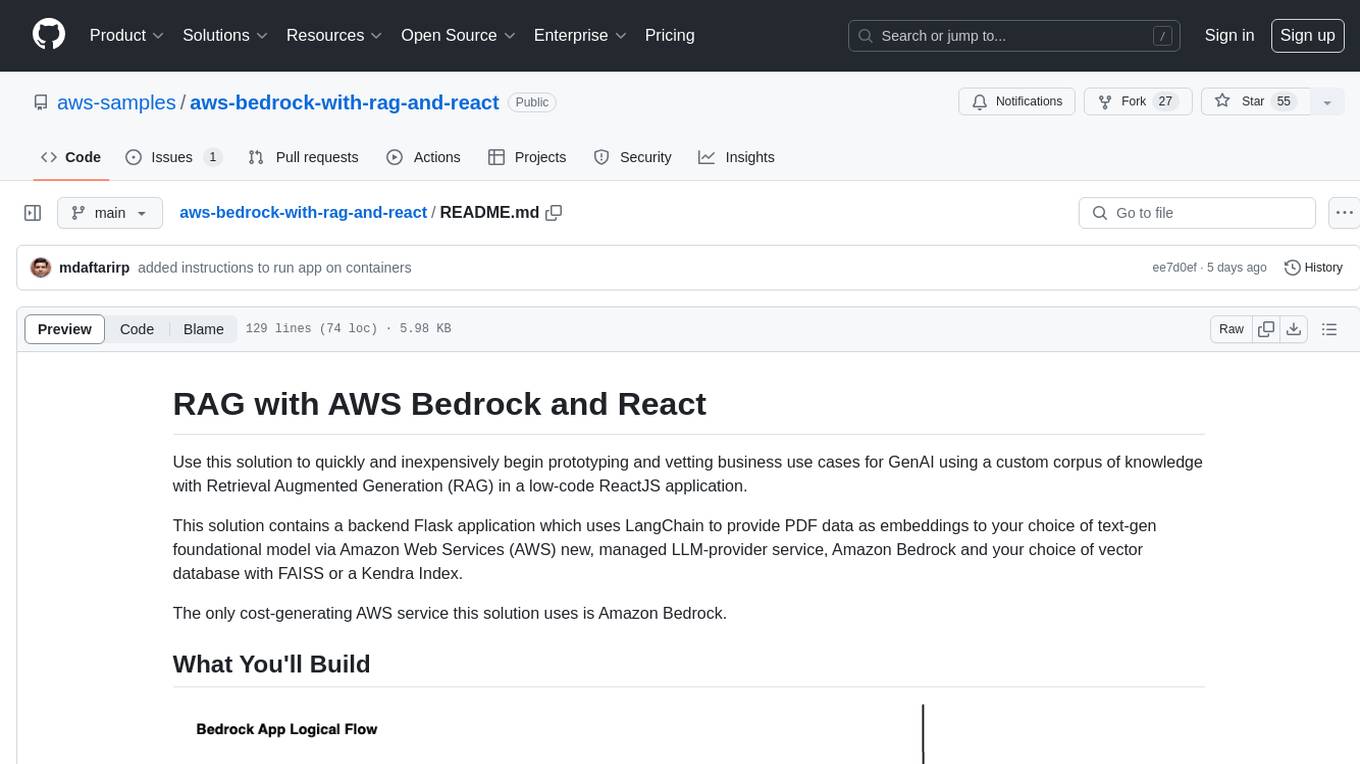
aws-bedrock-with-rag-and-react
This solution provides a low-code ReactJS application to prototype and vet business use cases for GenAI using Retrieval Augmented Generation (RAG). It includes a backend Flask application that uses LangChain to provide PDF data as embeddings to a text-gen model via Amazon Bedrock and a vector database with FAISS or Kendra Index. The solution utilizes Amazon Bedrock as the only cost-generating AWS service.
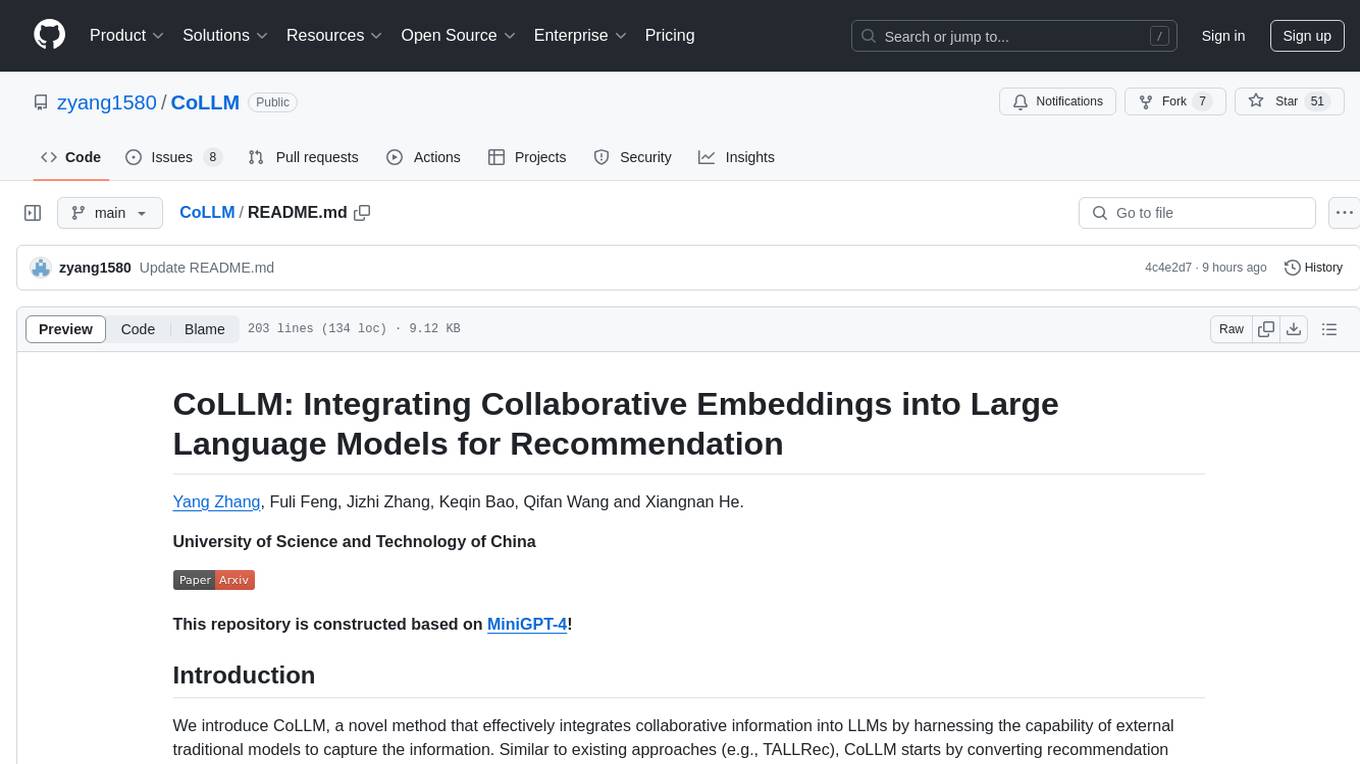
CoLLM
CoLLM is a novel method that integrates collaborative information into Large Language Models (LLMs) for recommendation. It converts recommendation data into language prompts, encodes them with both textual and collaborative information, and uses a two-step tuning method to train the model. The method incorporates user/item ID fields in prompts and employs a conventional collaborative model to generate user/item representations. CoLLM is built upon MiniGPT-4 and utilizes pretrained Vicuna weights for training.
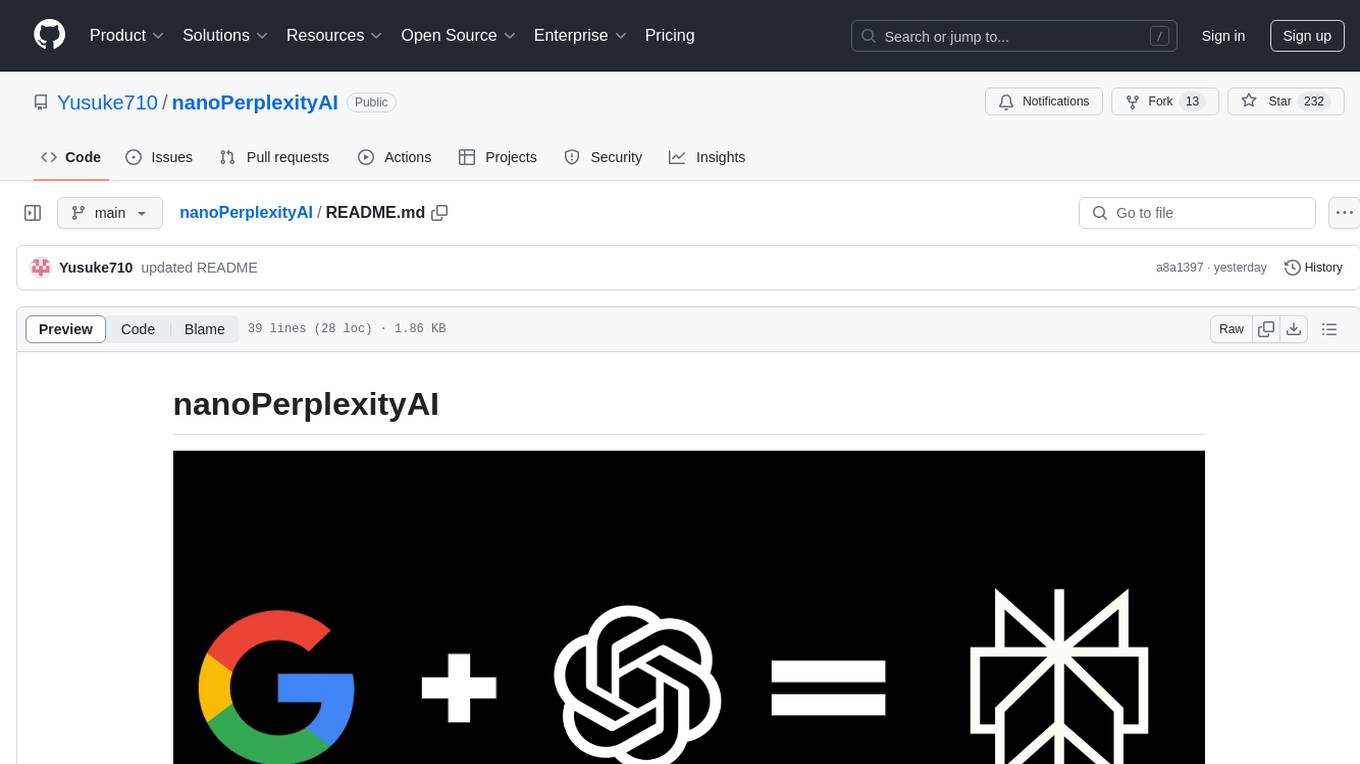
nanoPerplexityAI
nanoPerplexityAI is an open-source implementation of a large language model service that fetches information from Google. It involves a simple architecture where the user query is checked by the language model, reformulated for Google search, and an answer is generated and saved in a markdown file. The tool requires minimal setup and is designed for easy visualization of answers.
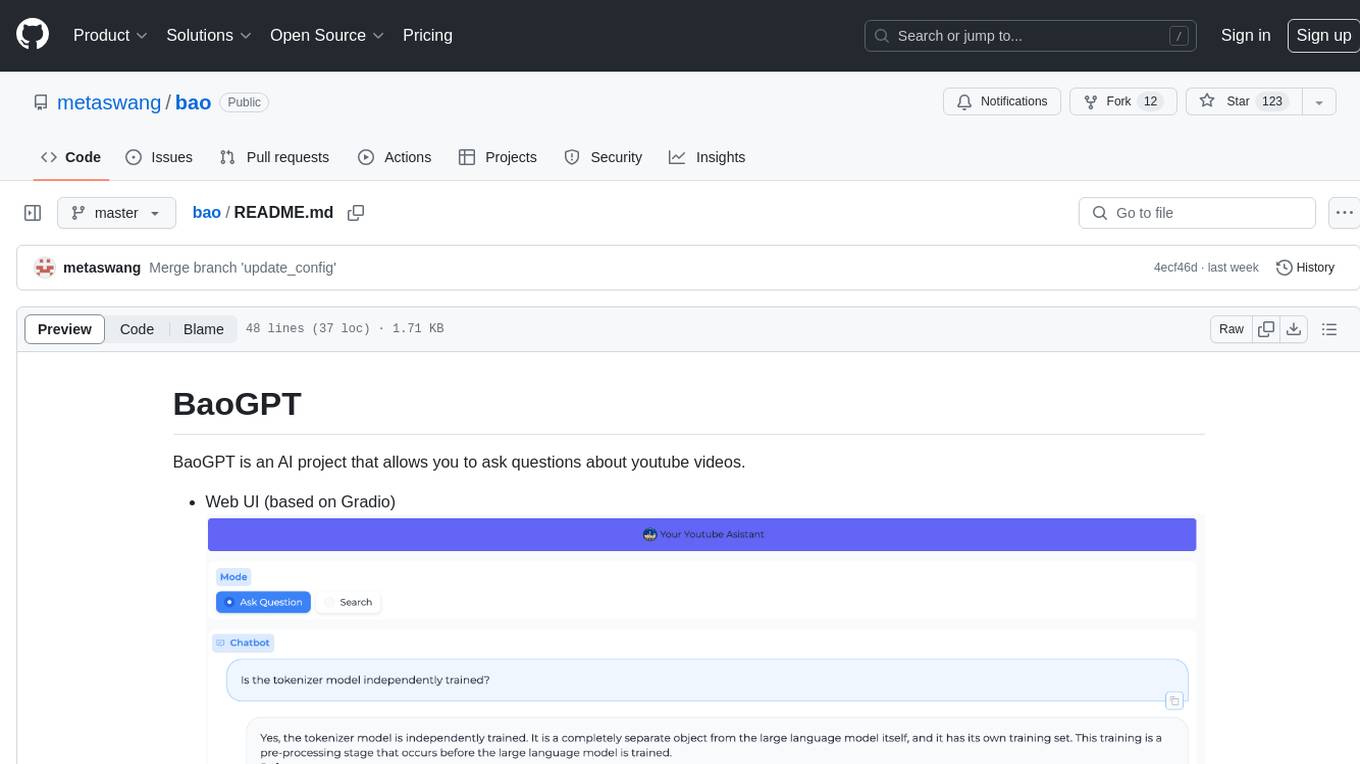
bao
BaoGPT is an AI project designed to facilitate asking questions about YouTube videos. It features a web UI based on Gradio and Discord integration. The tool utilizes a pipeline that routes input questions to either a greeting-like branch or a query & answer branch. The query analysis is performed by the LLM, which extracts attributes as filters and optimizes and rewrites questions for better vector retrieval in the vector DB. The tool then retrieves top-k candidates for grading and outputs final relative documents after grading. Lastly, the LLM performs summarization based on the reranking output, providing answers and attaching sources to the user.
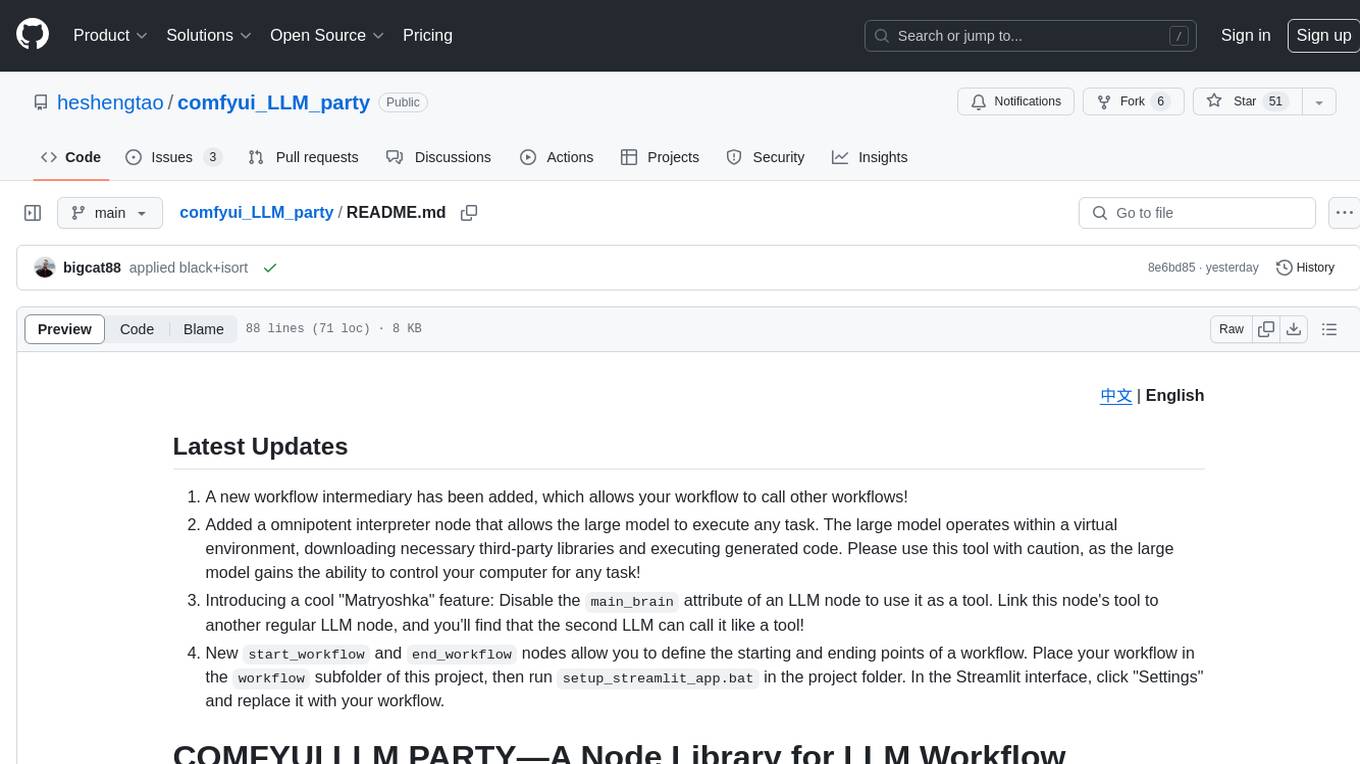
comfyui_LLM_party
COMFYUI LLM PARTY is a node library designed for LLM workflow development in ComfyUI, an extremely minimalist UI interface primarily used for AI drawing and SD model-based workflows. The project aims to provide a complete set of nodes for constructing LLM workflows, enabling users to easily integrate them into existing SD workflows. It features various functionalities such as API integration, local large model integration, RAG support, code interpreters, online queries, conditional statements, looping links for large models, persona mask attachment, and tool invocations for weather lookup, time lookup, knowledge base, code execution, web search, and single-page search. Users can rapidly develop web applications using API + Streamlit and utilize LLM as a tool node. Additionally, the project includes an omnipotent interpreter node that allows the large model to perform any task, with recommendations to use the 'show_text' node for display output.
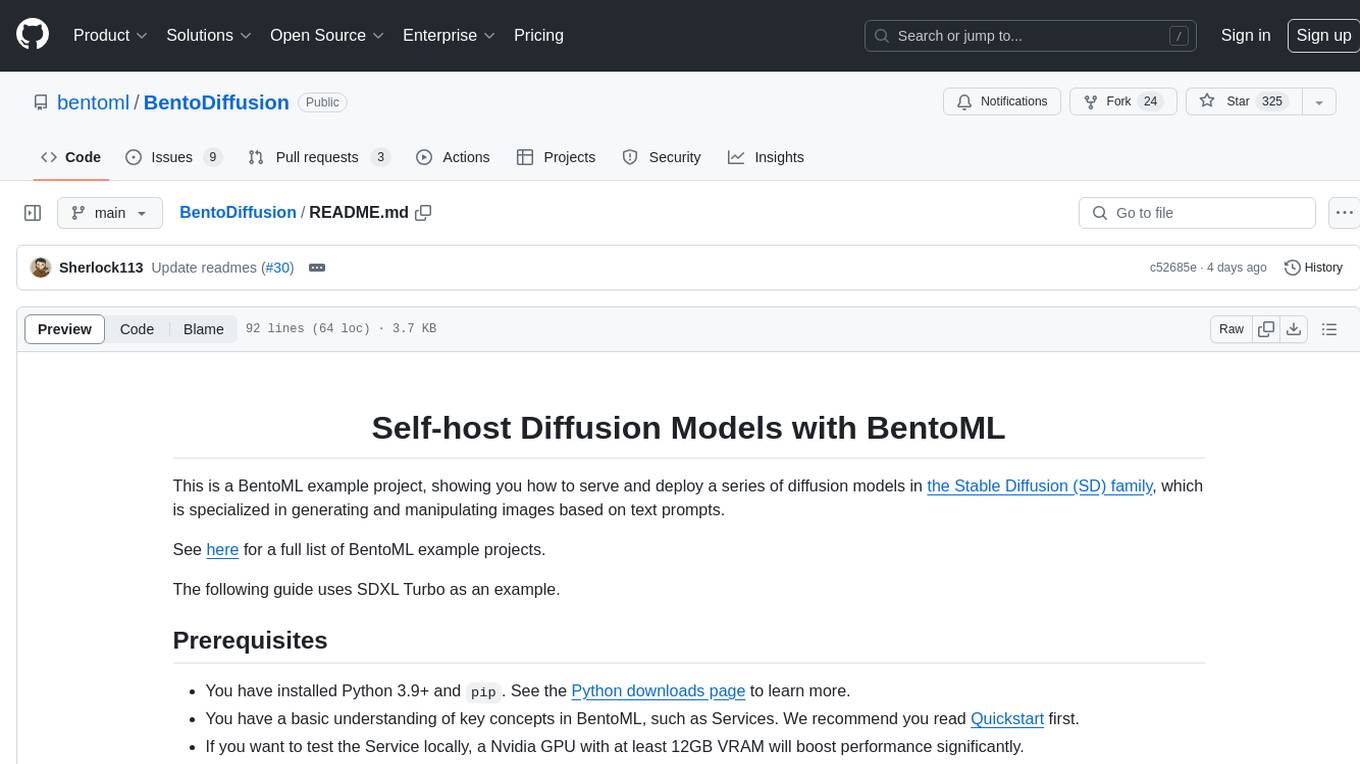
BentoDiffusion
BentoDiffusion is a BentoML example project that demonstrates how to serve and deploy diffusion models in the Stable Diffusion (SD) family. These models are specialized in generating and manipulating images based on text prompts. The project provides a guide on using SDXL Turbo as an example, along with instructions on prerequisites, installing dependencies, running the BentoML service, and deploying to BentoCloud. Users can interact with the deployed service using Swagger UI or other methods. Additionally, the project offers the option to choose from various diffusion models available in the repository for deployment.
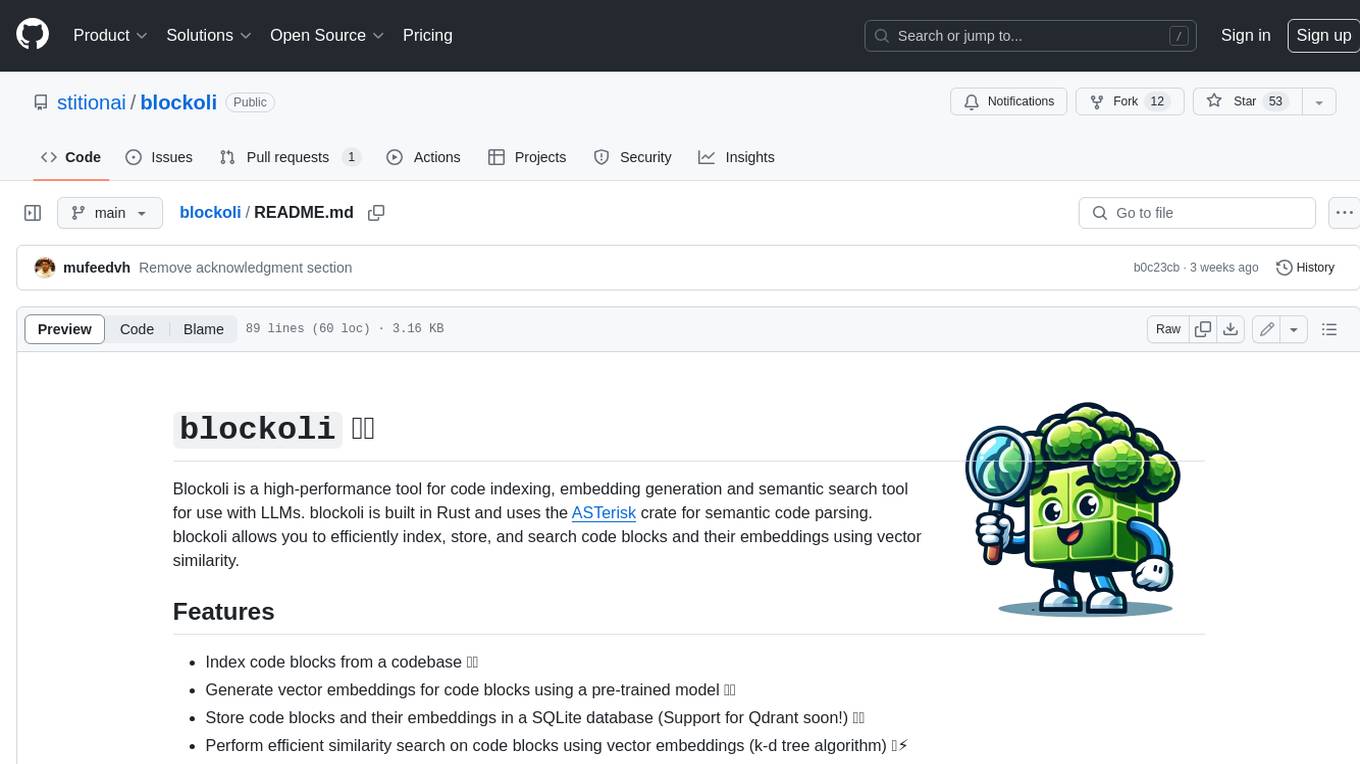
blockoli
Blockoli is a high-performance tool for code indexing, embedding generation, and semantic search tool for use with LLMs. It is built in Rust and uses the ASTerisk crate for semantic code parsing. Blockoli allows you to efficiently index, store, and search code blocks and their embeddings using vector similarity. Key features include indexing code blocks from a codebase, generating vector embeddings for code blocks using a pre-trained model, storing code blocks and their embeddings in a SQLite database, performing efficient similarity search on code blocks using vector embeddings, providing a REST API for easy integration with other tools and platforms, and being fast and memory-efficient due to its implementation in Rust.
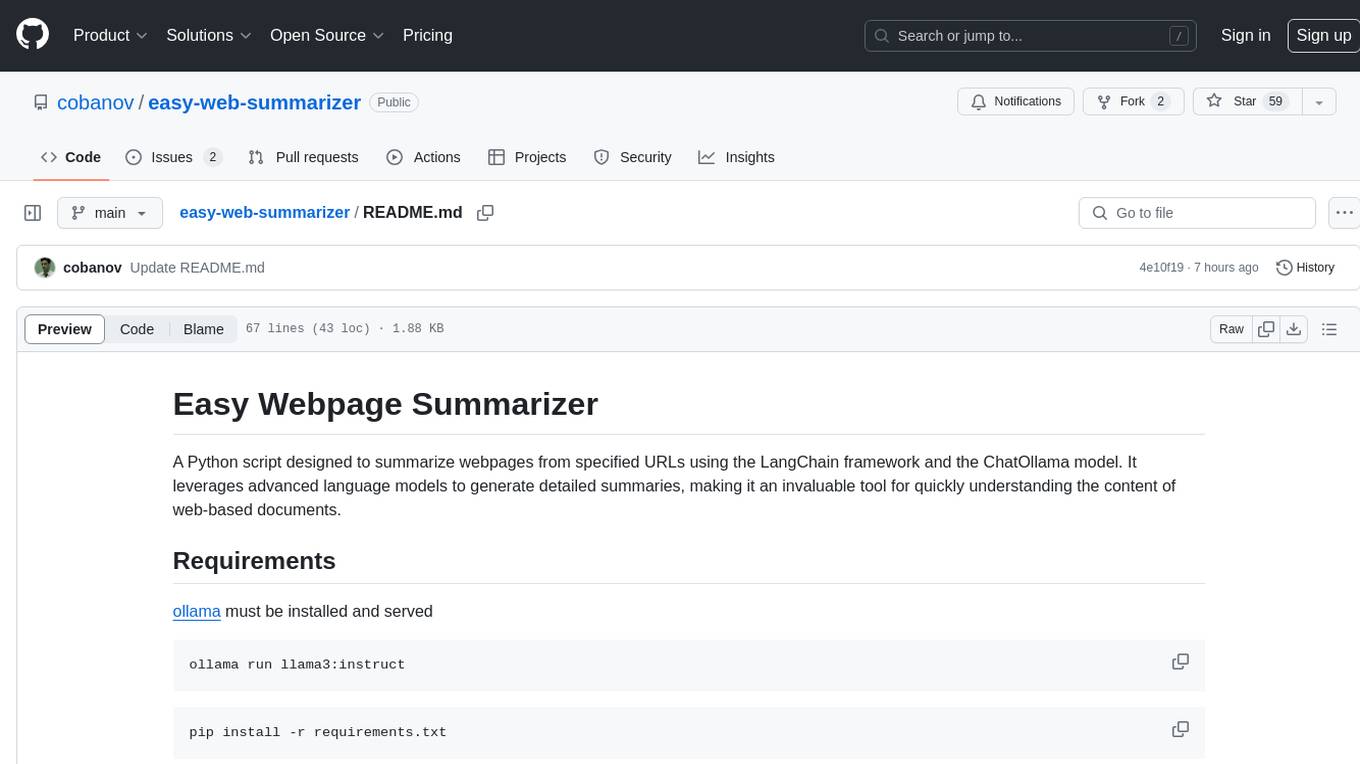
easy-web-summarizer
A Python script leveraging advanced language models to summarize webpages and youtube videos directly from URLs. It integrates with LangChain and ChatOllama for state-of-the-art summarization, providing detailed summaries for quick understanding of web-based documents. The tool offers a command-line interface for easy use and integration into workflows, with plans to add support for translating to different languages and streaming text output on gradio. It can also be used via a web UI using the gradio app. The script is dockerized for easy deployment and is open for contributions to enhance functionality and capabilities.
For similar tasks
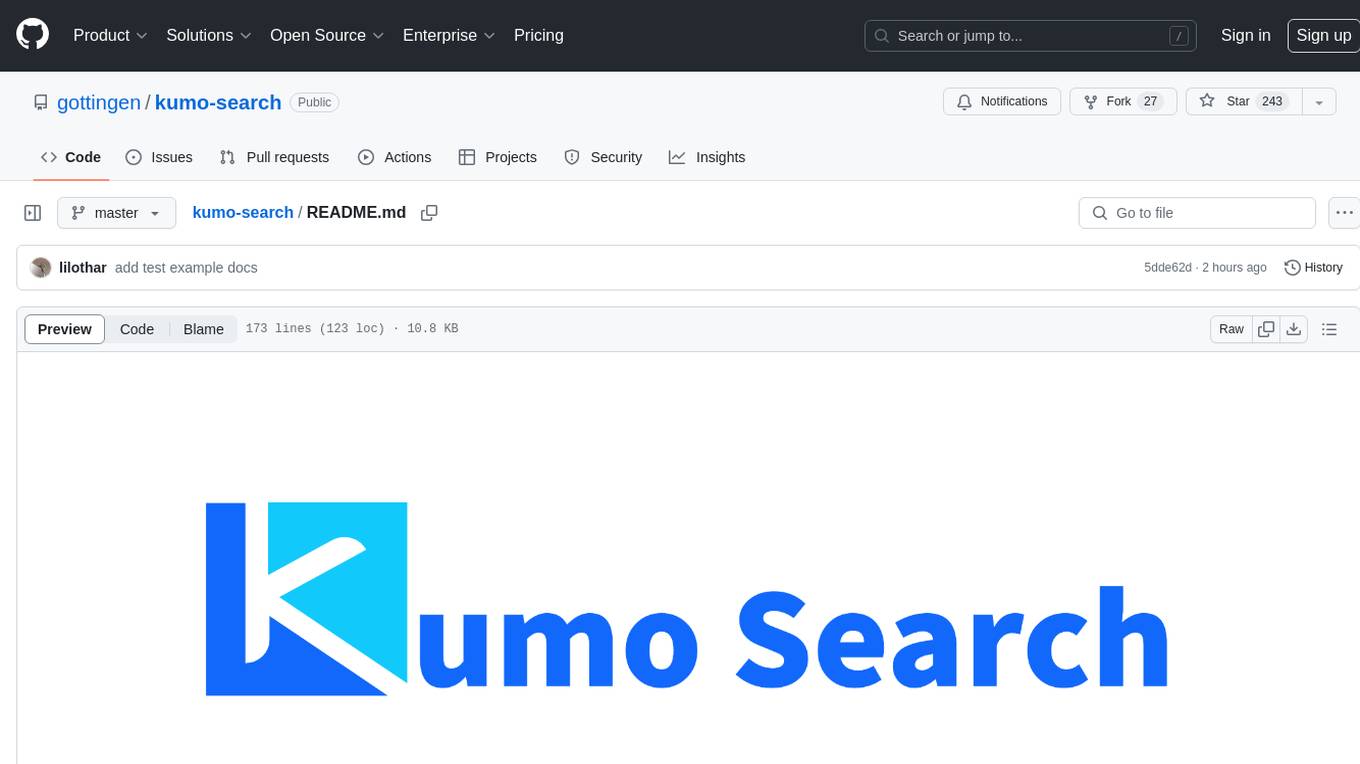
kumo-search
Kumo search is an end-to-end search engine framework that supports full-text search, inverted index, forward index, sorting, caching, hierarchical indexing, intervention system, feature collection, offline computation, storage system, and more. It runs on the EA (Elastic automic infrastructure architecture) platform, enabling engineering automation, service governance, real-time data, service degradation, and disaster recovery across multiple data centers and clusters. The framework aims to provide a ready-to-use search engine framework to help users quickly build their own search engines. Users can write business logic in Python using the AOT compiler in the project, which generates C++ code and binary dynamic libraries for rapid iteration of the search engine.
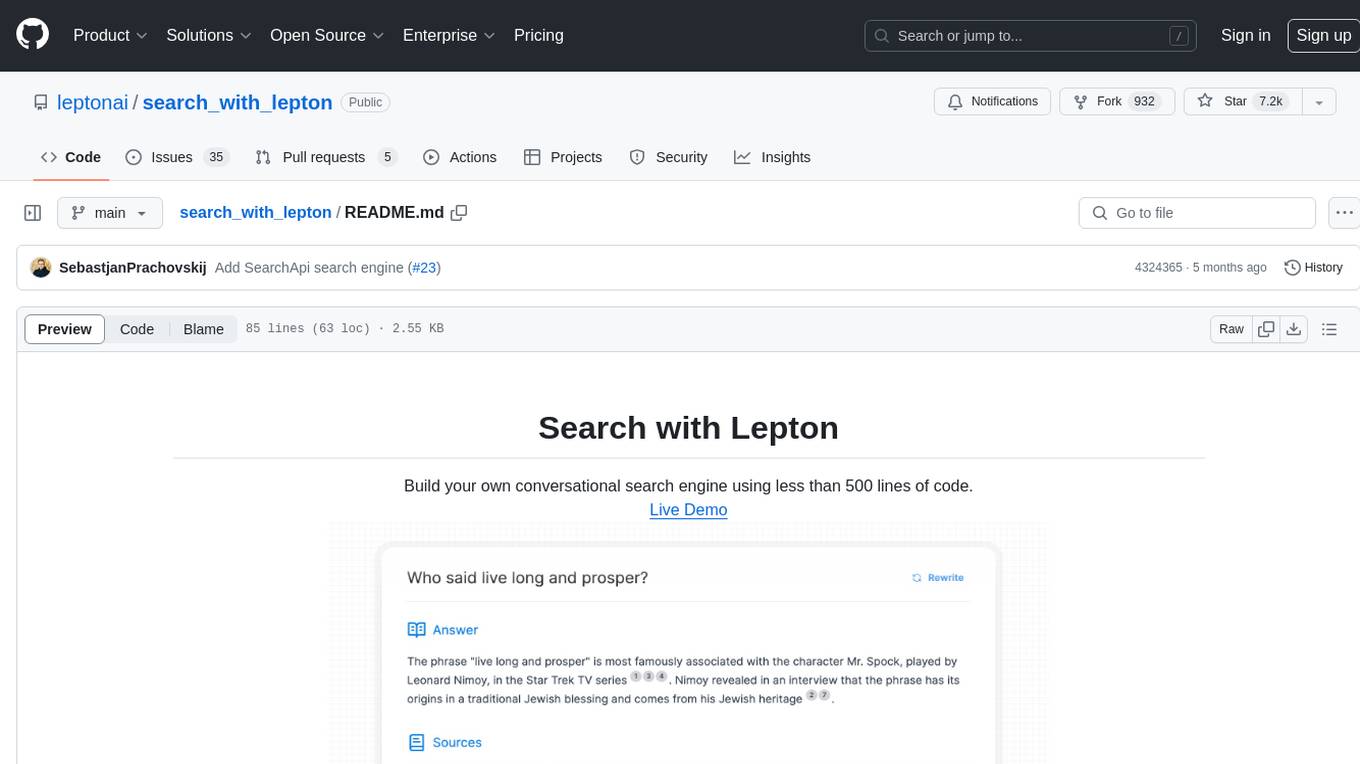
search_with_lepton
Build your own conversational search engine using less than 500 lines of code. Features built-in support for LLM, search engine, customizable UI interface, and shareable cached search results. Setup includes Bing and Google search engines. Utilize LLM and KV functions with Lepton for seamless integration. Easily deploy to Lepton AI or your own environment with one-click deployment options.
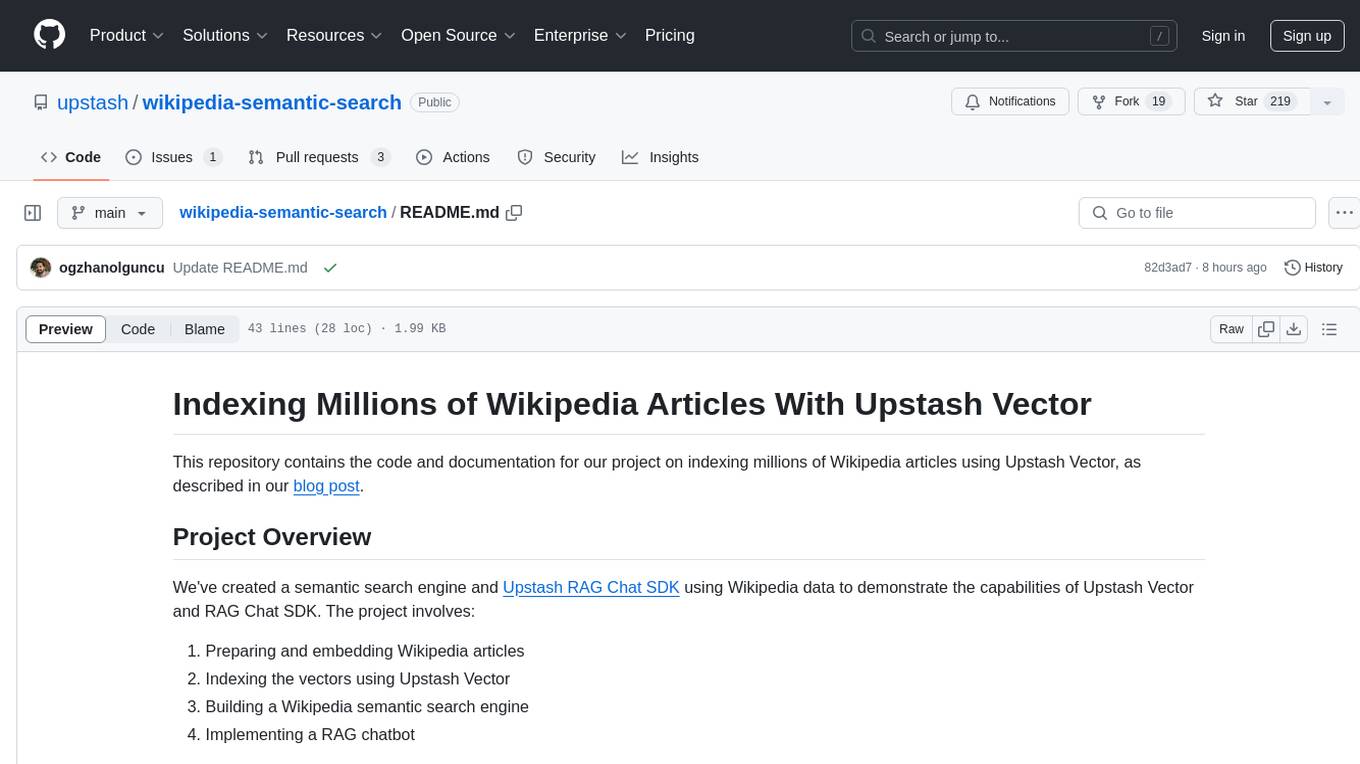
wikipedia-semantic-search
This repository showcases a project that indexes millions of Wikipedia articles using Upstash Vector. It includes a semantic search engine and a RAG chatbot SDK. The project involves preparing and embedding Wikipedia articles, indexing vectors, building a semantic search engine, and implementing a RAG chatbot. Key features include indexing over 144 million vectors, multilingual support, cross-lingual semantic search, and a RAG chatbot. Technologies used include Upstash Vector, Upstash Redis, Upstash RAG Chat SDK, SentenceTransformers, and Meta-Llama-3-8B-Instruct for LLM provider.
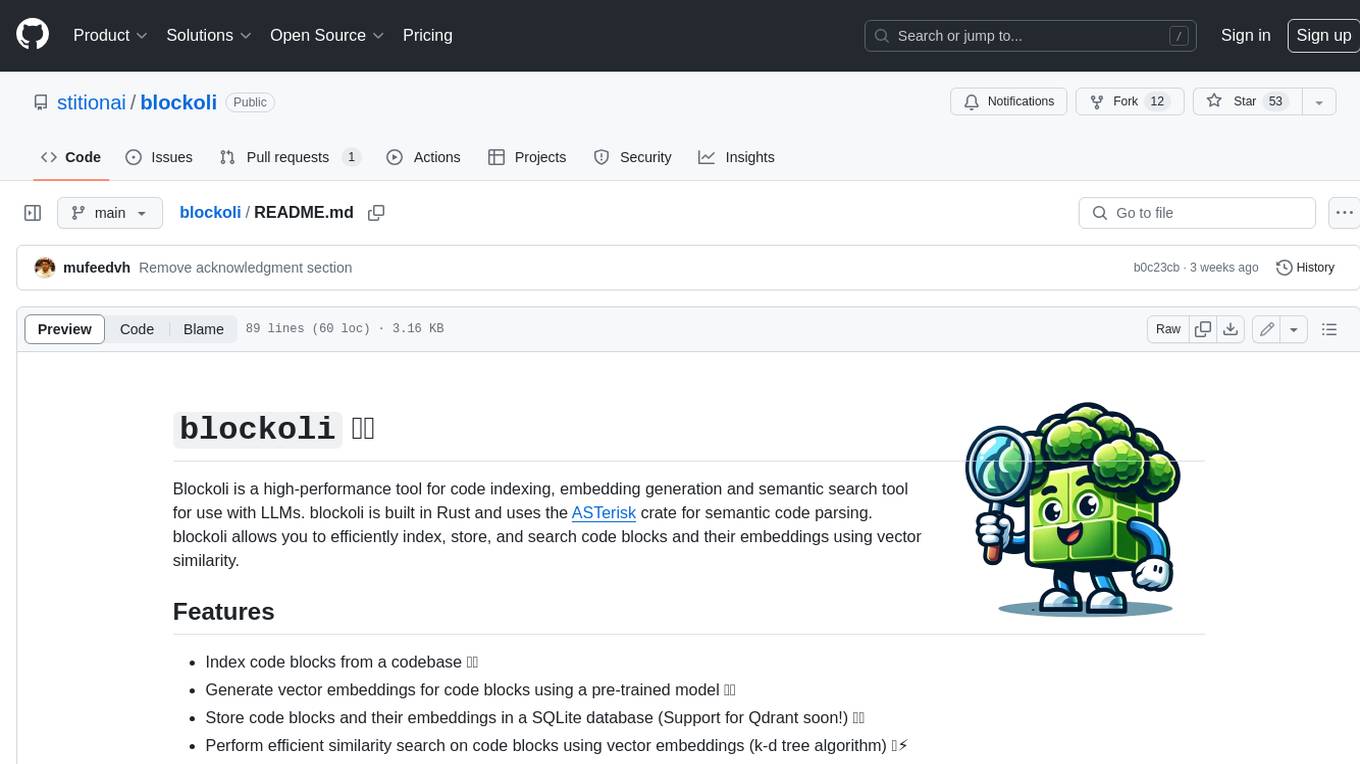
blockoli
Blockoli is a high-performance tool for code indexing, embedding generation, and semantic search tool for use with LLMs. It is built in Rust and uses the ASTerisk crate for semantic code parsing. Blockoli allows you to efficiently index, store, and search code blocks and their embeddings using vector similarity. Key features include indexing code blocks from a codebase, generating vector embeddings for code blocks using a pre-trained model, storing code blocks and their embeddings in a SQLite database, performing efficient similarity search on code blocks using vector embeddings, providing a REST API for easy integration with other tools and platforms, and being fast and memory-efficient due to its implementation in Rust.
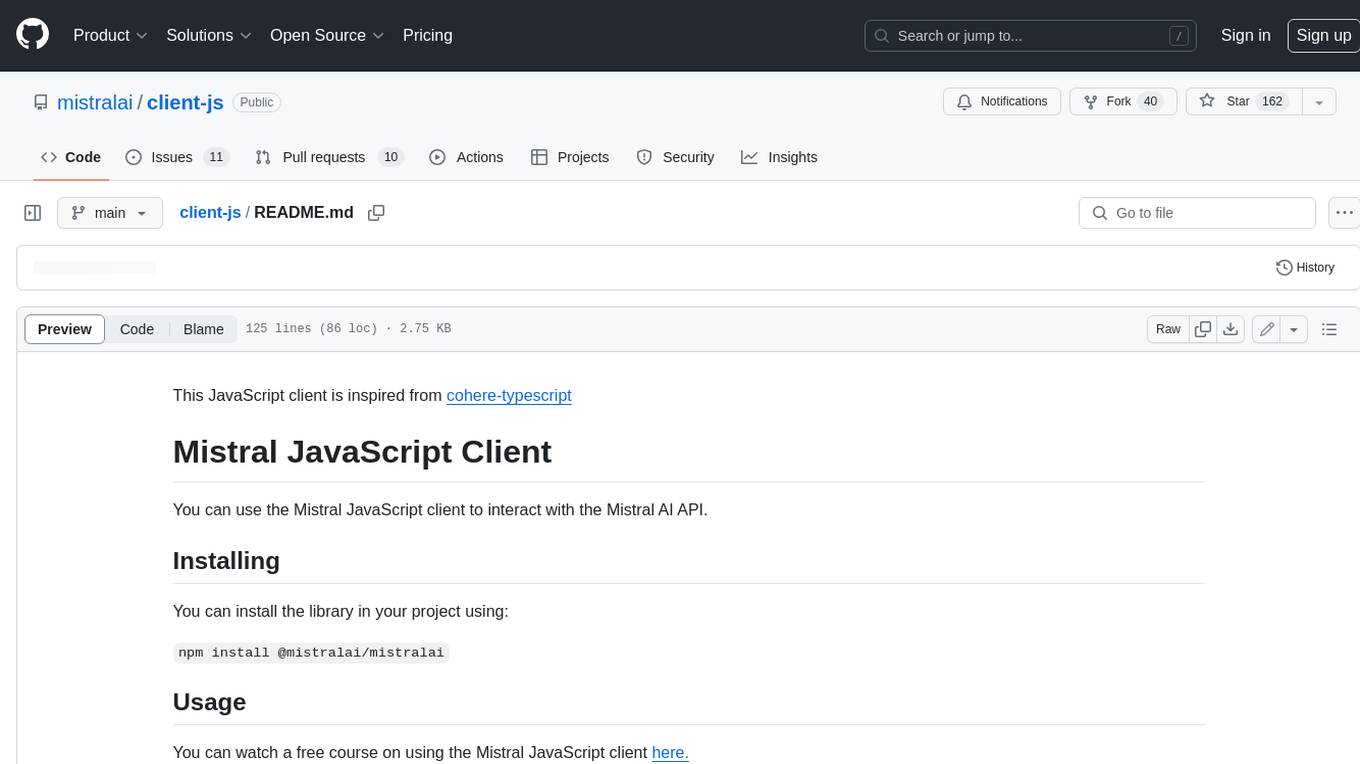
client-js
The Mistral JavaScript client is a library that allows you to interact with the Mistral AI API. With this client, you can perform various tasks such as listing models, chatting with streaming, chatting without streaming, and generating embeddings. To use the client, you can install it in your project using npm and then set up the client with your API key. Once the client is set up, you can use it to perform the desired tasks. For example, you can use the client to chat with a model by providing a list of messages. The client will then return the response from the model. You can also use the client to generate embeddings for a given input. The embeddings can then be used for various downstream tasks such as clustering or classification.
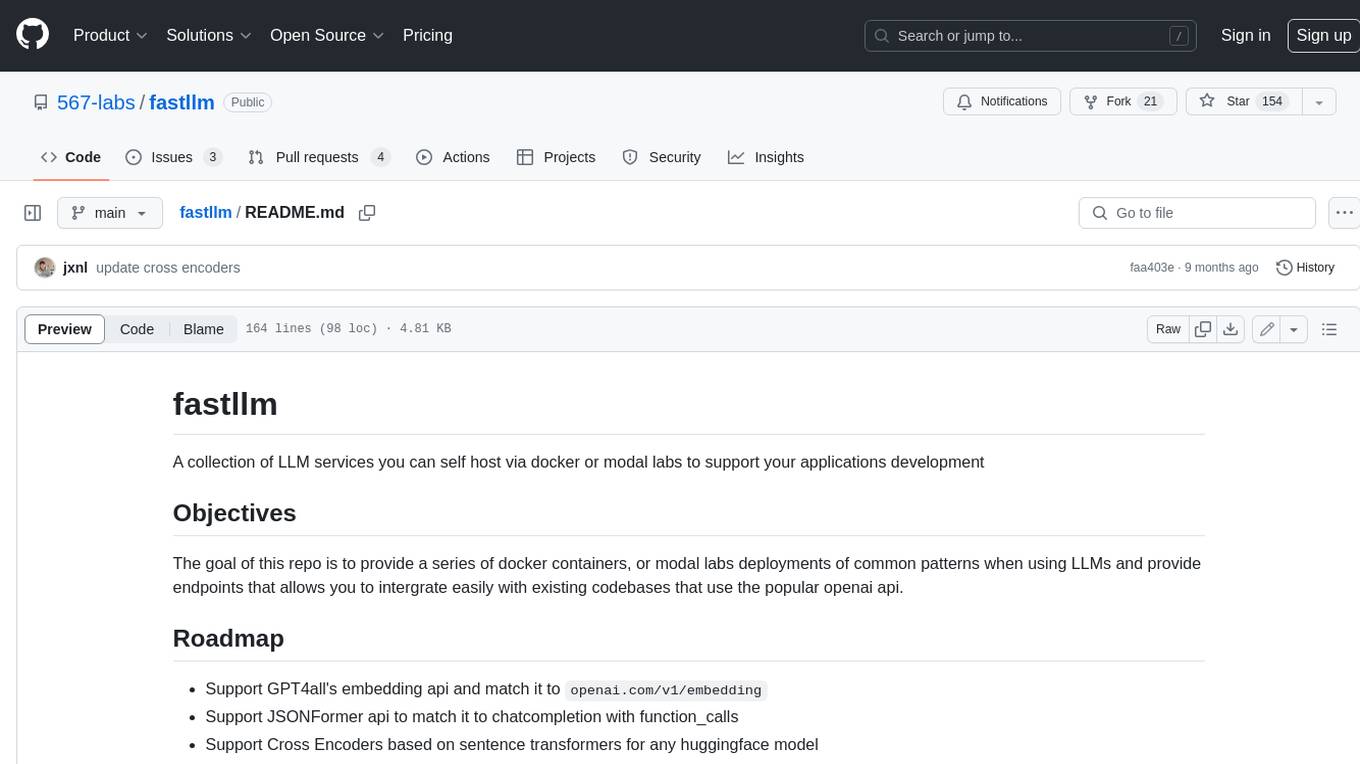
fastllm
A collection of LLM services you can self host via docker or modal labs to support your applications development. The goal is to provide docker containers or modal labs deployments of common patterns when using LLMs and endpoints to integrate easily with existing codebases using the openai api. It supports GPT4all's embedding api, JSONFormer api for chat completion, Cross Encoders based on sentence transformers, and provides documentation using MkDocs.
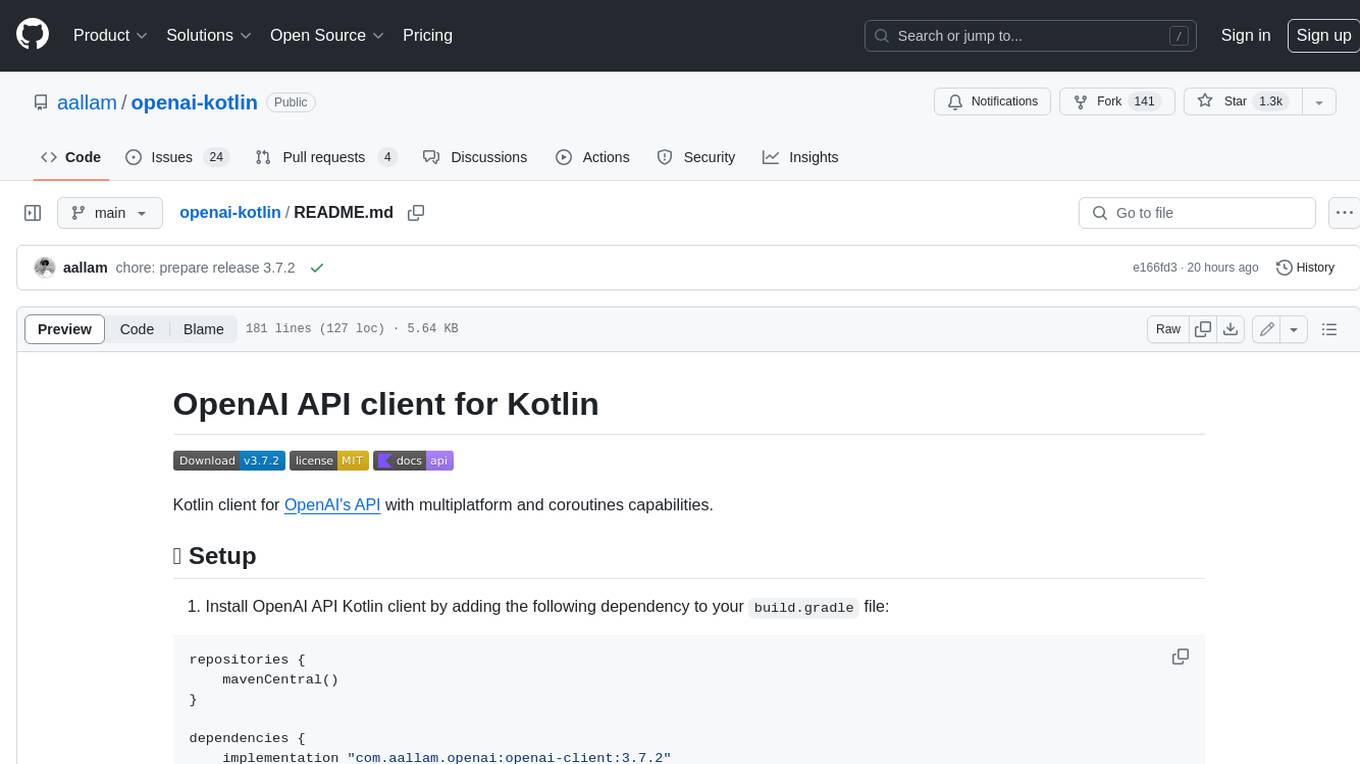
openai-kotlin
OpenAI Kotlin API client is a Kotlin client for OpenAI's API with multiplatform and coroutines capabilities. It allows users to interact with OpenAI's API using Kotlin programming language. The client supports various features such as models, chat, images, embeddings, files, fine-tuning, moderations, audio, assistants, threads, messages, and runs. It also provides guides on getting started, chat & function call, file source guide, and assistants. Sample apps are available for reference, and troubleshooting guides are provided for common issues. The project is open-source and licensed under the MIT license, allowing contributions from the community.
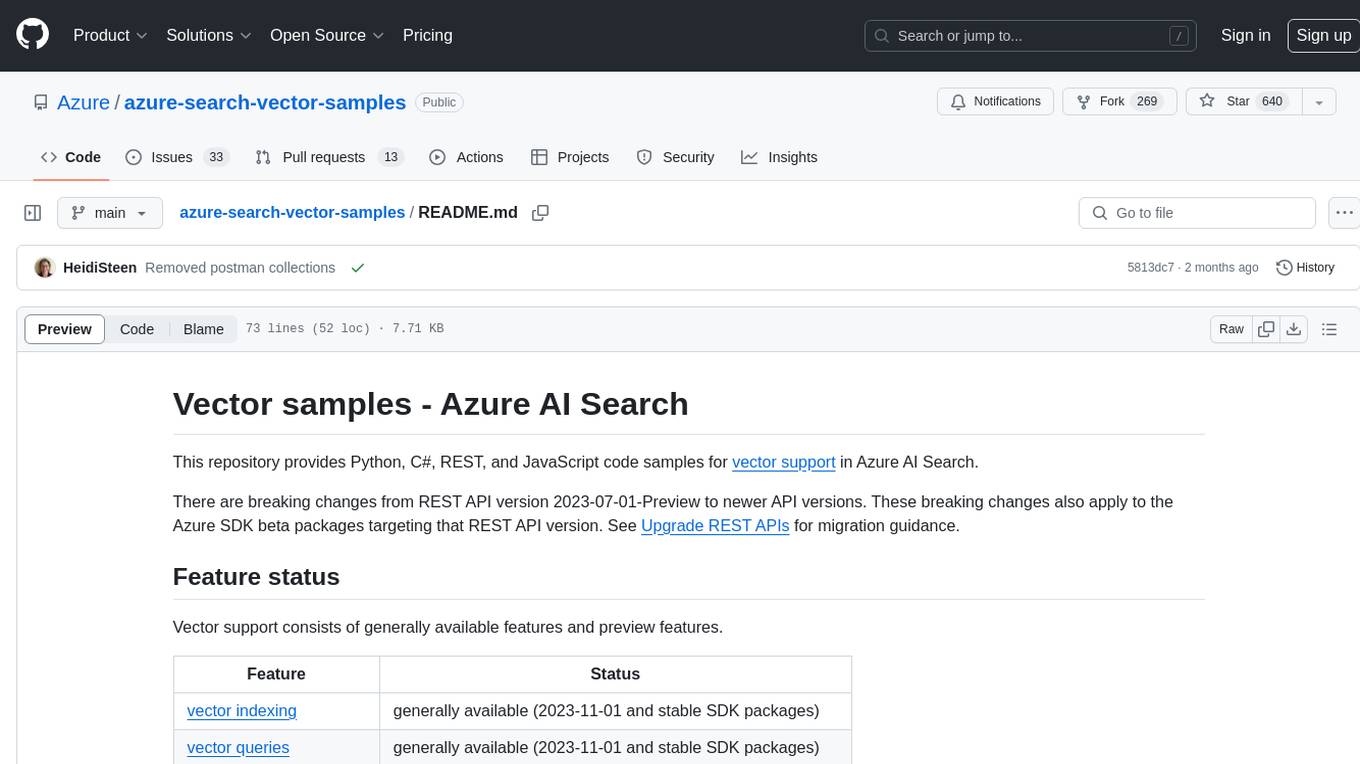
azure-search-vector-samples
This repository provides code samples in Python, C#, REST, and JavaScript for vector support in Azure AI Search. It includes demos for various languages showcasing vectorization of data, creating indexes, and querying vector data. Additionally, it offers tools like Azure AI Search Lab for experimenting with AI-enabled search scenarios in Azure and templates for deploying custom chat-with-your-data solutions. The repository also features documentation on vector search, hybrid search, creating and querying vector indexes, and REST API references for Azure AI Search and Azure OpenAI Service.
For similar jobs
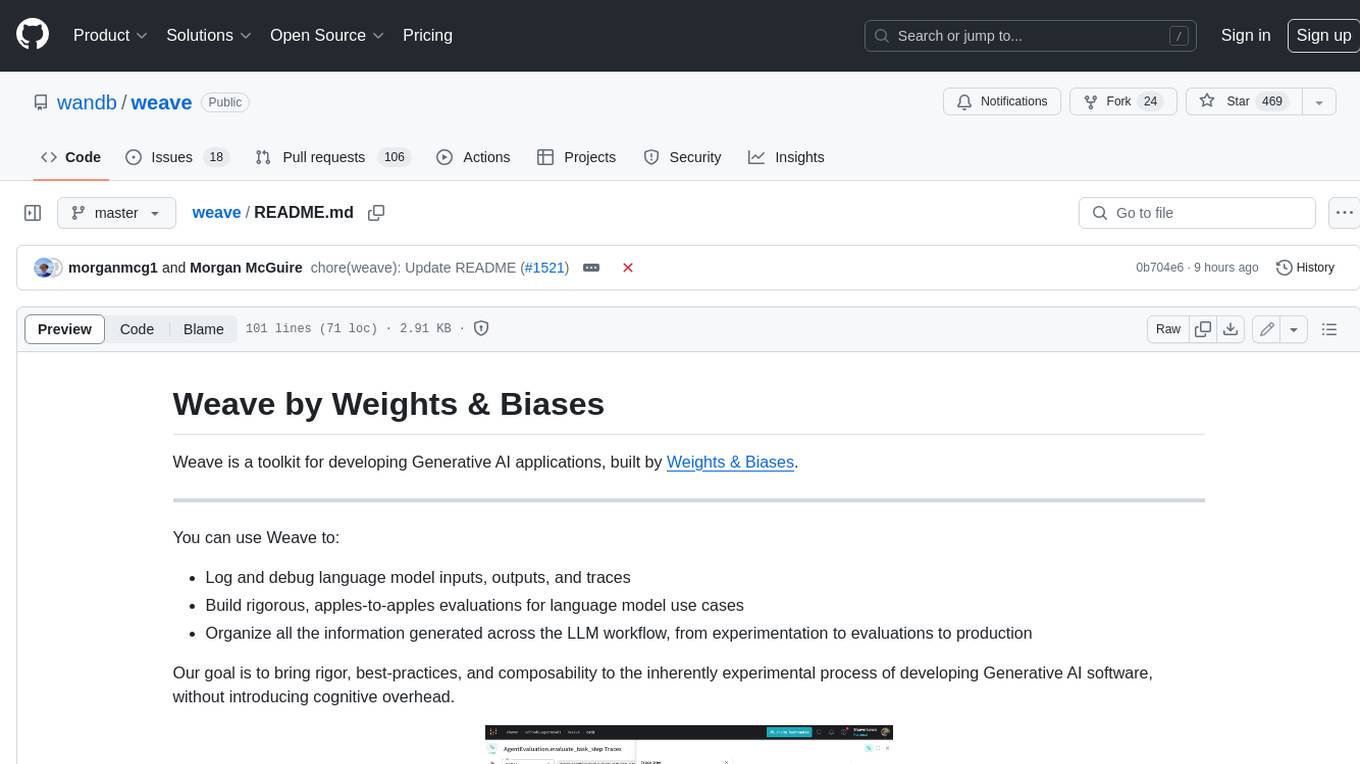
weave
Weave is a toolkit for developing Generative AI applications, built by Weights & Biases. With Weave, you can log and debug language model inputs, outputs, and traces; build rigorous, apples-to-apples evaluations for language model use cases; and organize all the information generated across the LLM workflow, from experimentation to evaluations to production. Weave aims to bring rigor, best-practices, and composability to the inherently experimental process of developing Generative AI software, without introducing cognitive overhead.
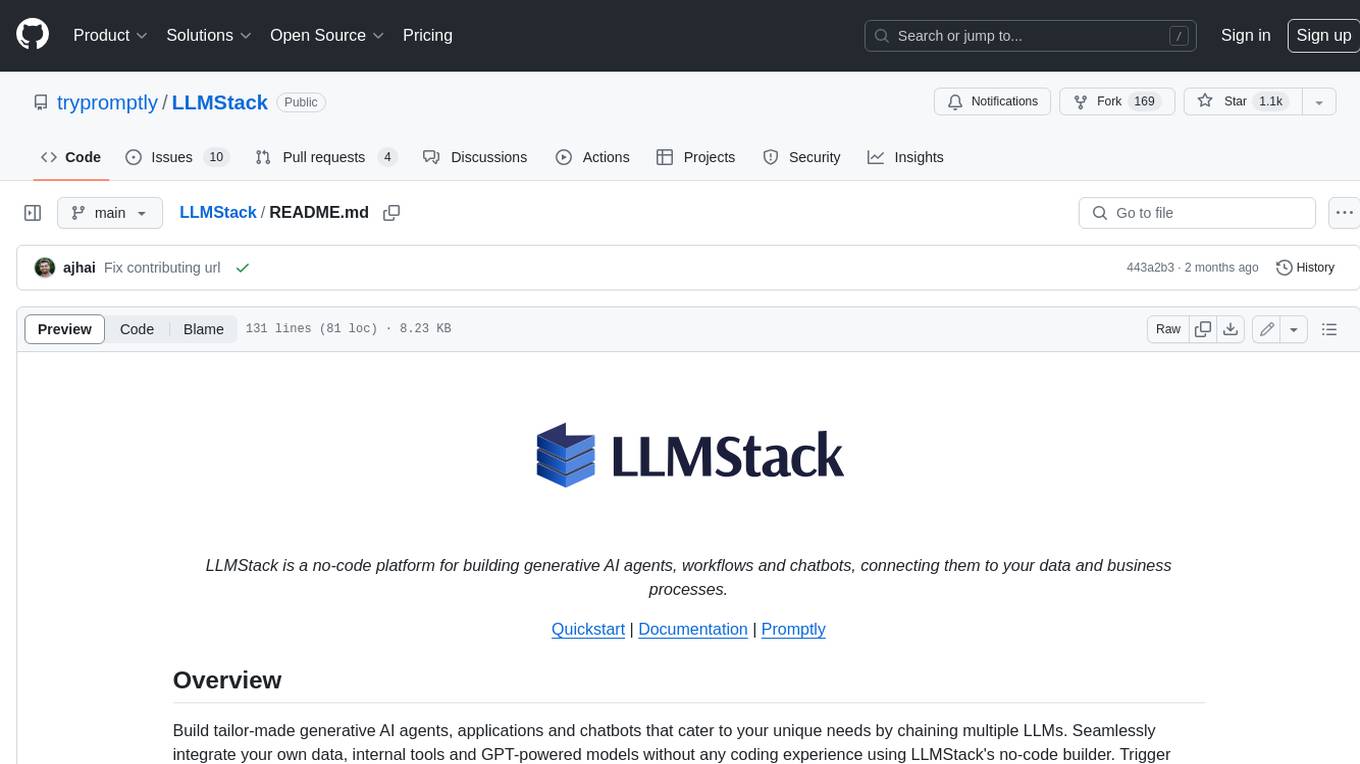
LLMStack
LLMStack is a no-code platform for building generative AI agents, workflows, and chatbots. It allows users to connect their own data, internal tools, and GPT-powered models without any coding experience. LLMStack can be deployed to the cloud or on-premise and can be accessed via HTTP API or triggered from Slack or Discord.
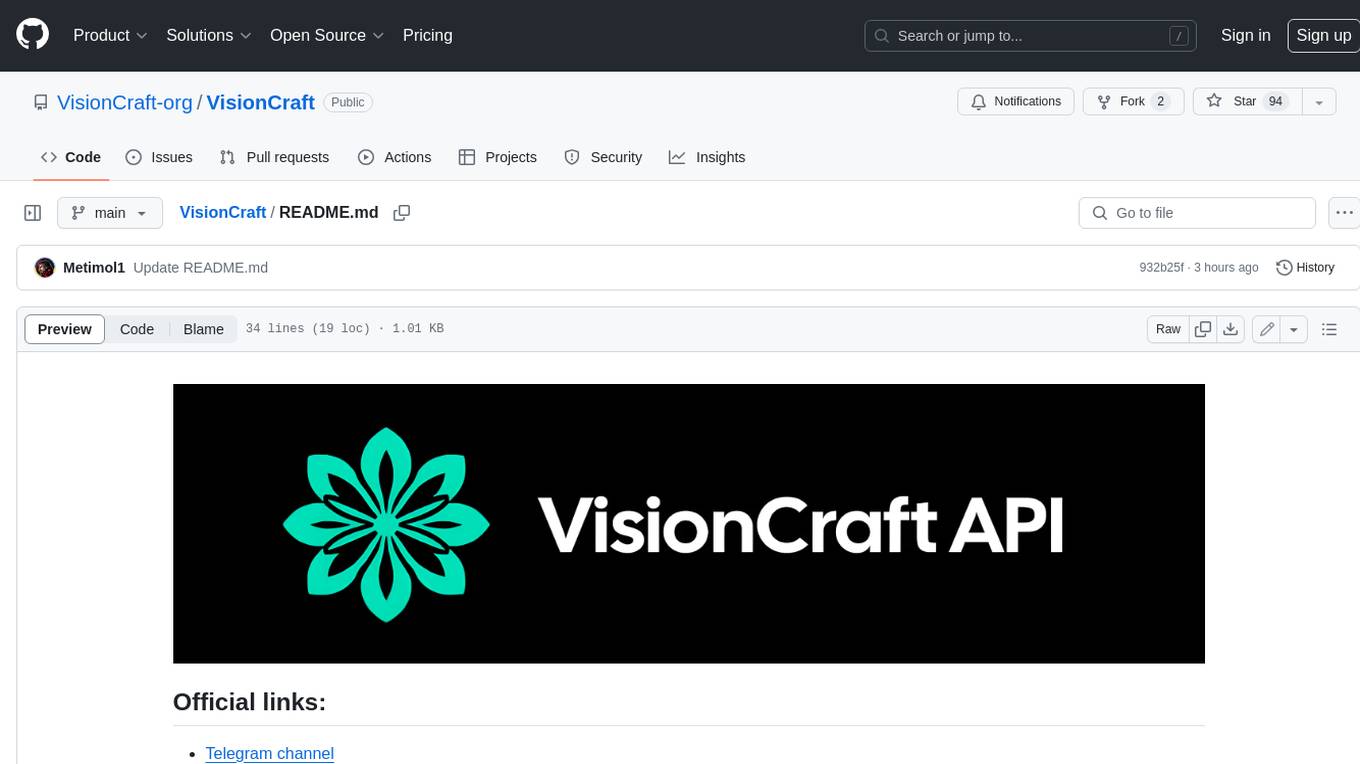
VisionCraft
The VisionCraft API is a free API for using over 100 different AI models. From images to sound.
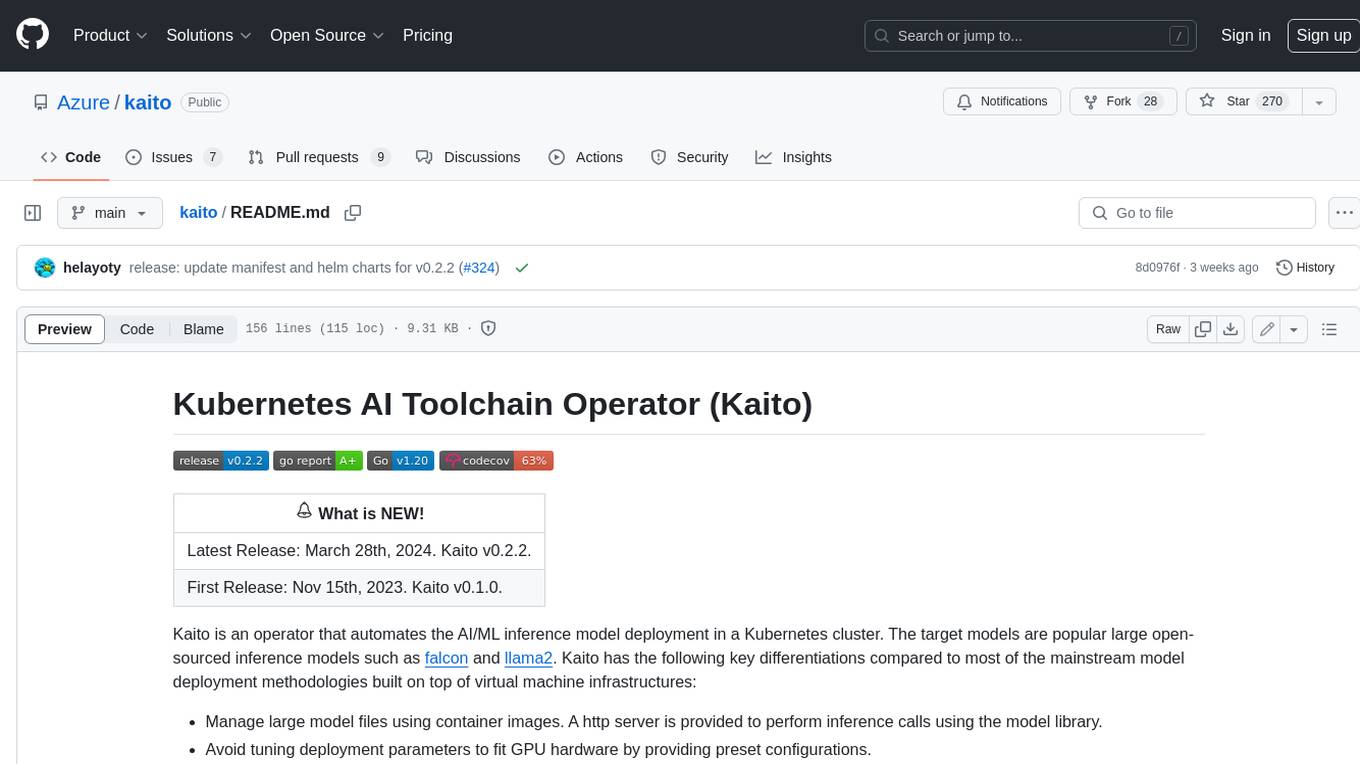
kaito
Kaito is an operator that automates the AI/ML inference model deployment in a Kubernetes cluster. It manages large model files using container images, avoids tuning deployment parameters to fit GPU hardware by providing preset configurations, auto-provisions GPU nodes based on model requirements, and hosts large model images in the public Microsoft Container Registry (MCR) if the license allows. Using Kaito, the workflow of onboarding large AI inference models in Kubernetes is largely simplified.
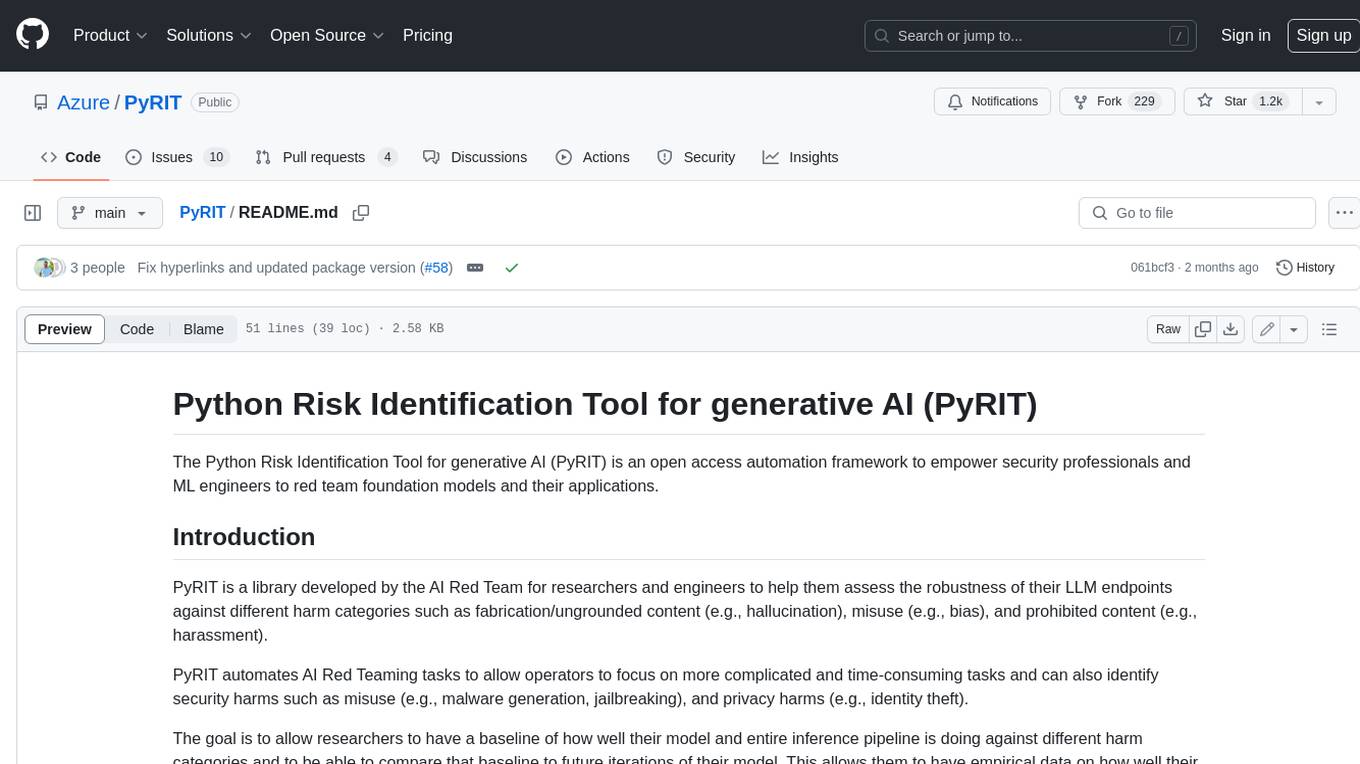
PyRIT
PyRIT is an open access automation framework designed to empower security professionals and ML engineers to red team foundation models and their applications. It automates AI Red Teaming tasks to allow operators to focus on more complicated and time-consuming tasks and can also identify security harms such as misuse (e.g., malware generation, jailbreaking), and privacy harms (e.g., identity theft). The goal is to allow researchers to have a baseline of how well their model and entire inference pipeline is doing against different harm categories and to be able to compare that baseline to future iterations of their model. This allows them to have empirical data on how well their model is doing today, and detect any degradation of performance based on future improvements.
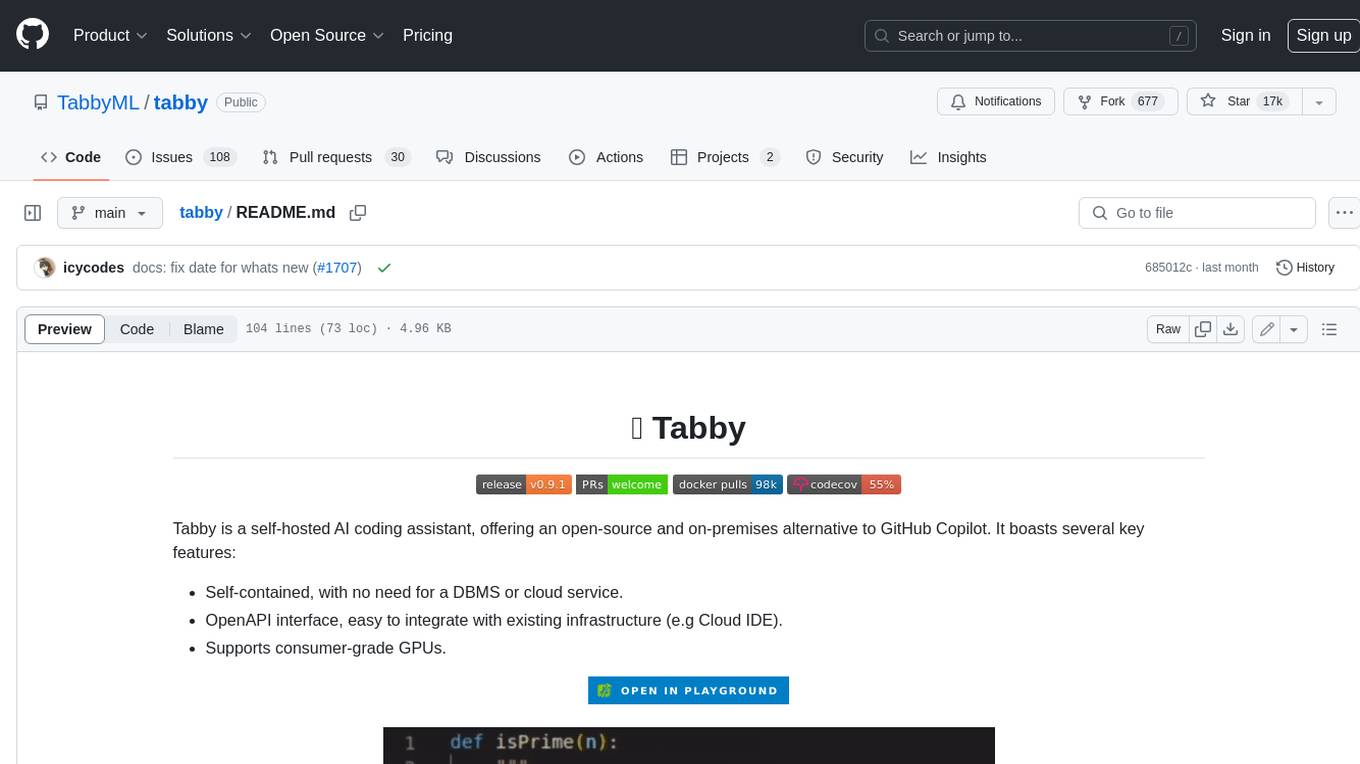
tabby
Tabby is a self-hosted AI coding assistant, offering an open-source and on-premises alternative to GitHub Copilot. It boasts several key features: * Self-contained, with no need for a DBMS or cloud service. * OpenAPI interface, easy to integrate with existing infrastructure (e.g Cloud IDE). * Supports consumer-grade GPUs.
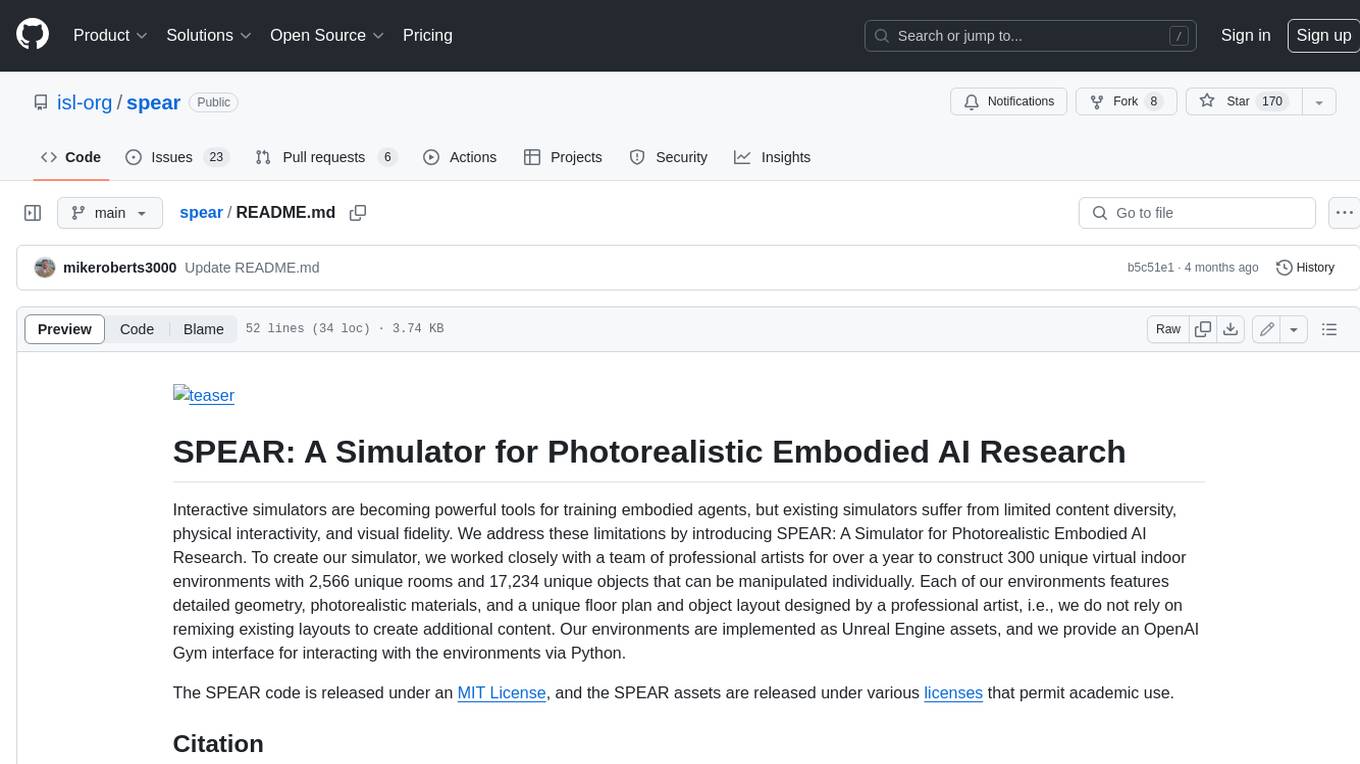
spear
SPEAR (Simulator for Photorealistic Embodied AI Research) is a powerful tool for training embodied agents. It features 300 unique virtual indoor environments with 2,566 unique rooms and 17,234 unique objects that can be manipulated individually. Each environment is designed by a professional artist and features detailed geometry, photorealistic materials, and a unique floor plan and object layout. SPEAR is implemented as Unreal Engine assets and provides an OpenAI Gym interface for interacting with the environments via Python.
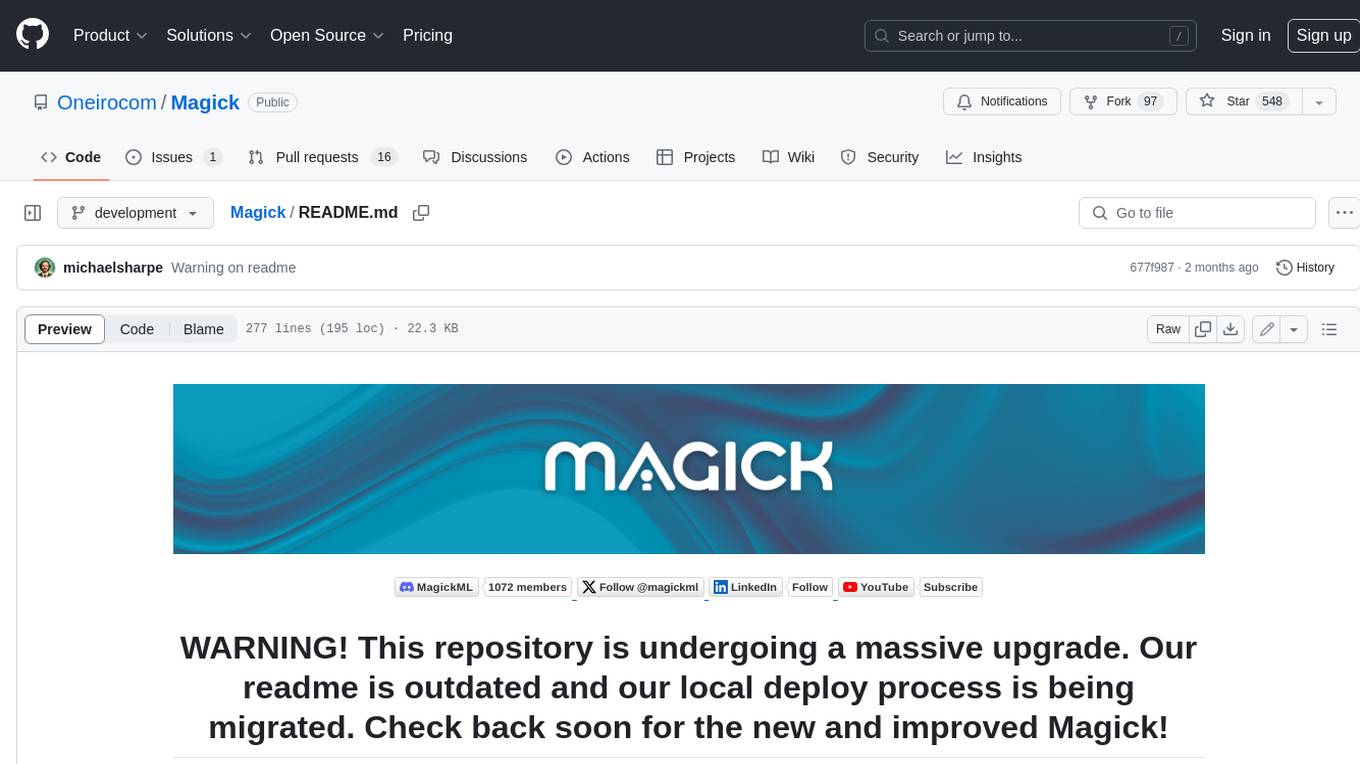
Magick
Magick is a groundbreaking visual AIDE (Artificial Intelligence Development Environment) for no-code data pipelines and multimodal agents. Magick can connect to other services and comes with nodes and templates well-suited for intelligent agents, chatbots, complex reasoning systems and realistic characters.