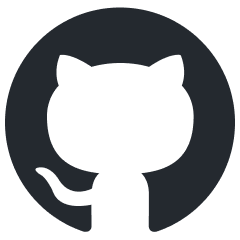
ai-toolkit
Various AI scripts. Mostly Stable Diffusion stuff.
Stars: 3861
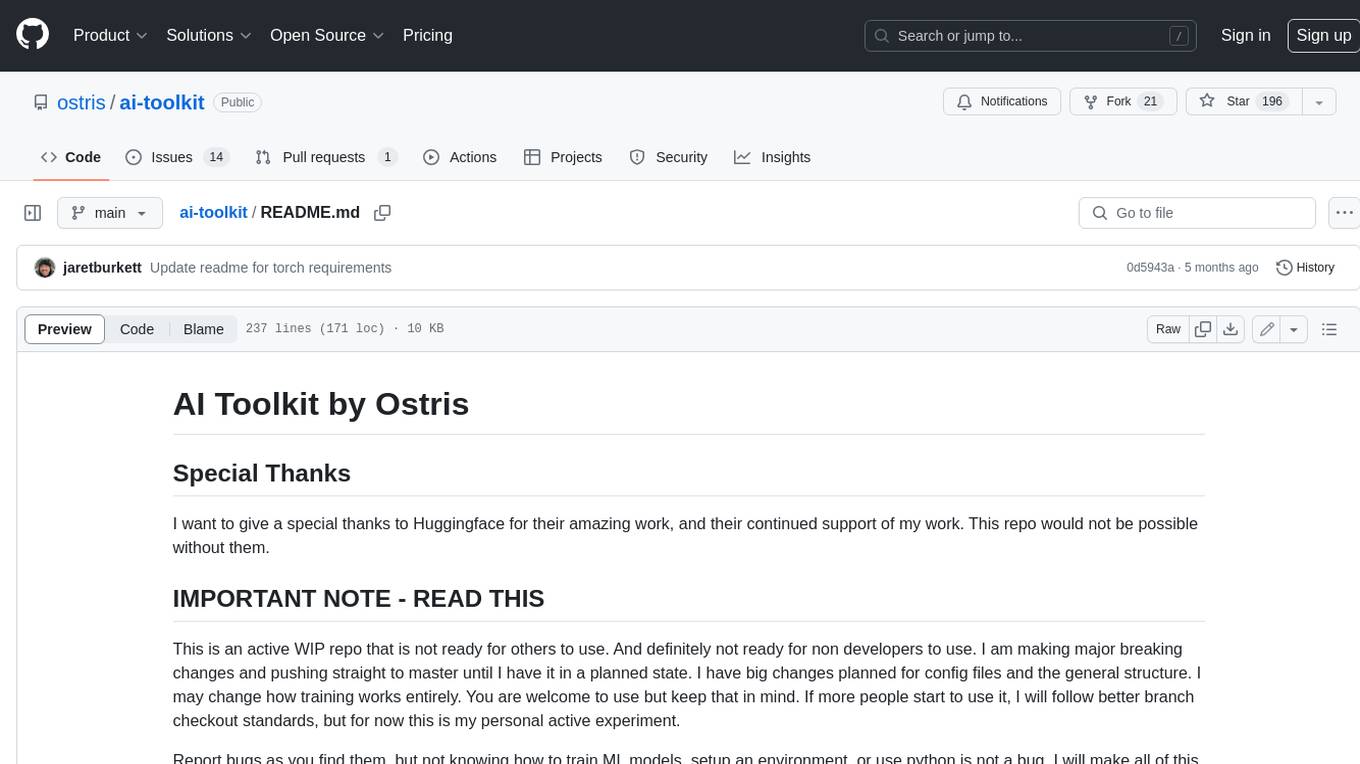
The AI Toolkit by Ostris is a collection of tools for machine learning, specifically designed for image generation, LoRA (latent representations of attributes) extraction and manipulation, and model training. It provides a user-friendly interface and extensive documentation to make it accessible to both developers and non-developers. The toolkit is actively under development, with new features and improvements being added regularly. Some of the key features of the AI Toolkit include: - Batch Image Generation: Allows users to generate a batch of images based on prompts or text files, using a configuration file to specify the desired settings. - LoRA (lierla), LoCON (LyCORIS) Extractor: Facilitates the extraction of LoRA and LoCON representations from pre-trained models, enabling users to modify and manipulate these representations for various purposes. - LoRA Rescale: Provides a tool to rescale LoRA weights, allowing users to adjust the influence of specific attributes in the generated images. - LoRA Slider Trainer: Enables the training of LoRA sliders, which can be used to control and adjust specific attributes in the generated images, offering a powerful tool for fine-tuning and customization. - Extensions: Supports the creation and sharing of custom extensions, allowing users to extend the functionality of the toolkit with their own tools and scripts. - VAE (Variational Auto Encoder) Trainer: Facilitates the training of VAEs for image generation, providing users with a tool to explore and improve the quality of generated images. The AI Toolkit is a valuable resource for anyone interested in exploring and utilizing machine learning for image generation and manipulation. Its user-friendly interface, extensive documentation, and active development make it an accessible and powerful tool for both beginners and experienced users.
README:
This is my research repo. I do a lot of experiments in it and it is possible that I will break things. If something breaks, checkout an earlier commit. This repo can train a lot of things, and it is hard to keep up with all of them.
My work on this project would not be possible without the amazing support of Glif and everyone on the team. If you want to support me, support Glif. Join the site, Join us on Discord, follow us on Twitter and come make some cool stuff with us
Requirements:
- python >3.10
- Nvidia GPU with enough ram to do what you need
- python venv
- git
Linux:
git clone https://github.com/ostris/ai-toolkit.git
cd ai-toolkit
git submodule update --init --recursive
python3 -m venv venv
source venv/bin/activate
# .\venv\Scripts\activate on windows
# install torch first
pip3 install torch
pip3 install -r requirements.txt
Windows:
git clone https://github.com/ostris/ai-toolkit.git
cd ai-toolkit
git submodule update --init --recursive
python -m venv venv
.\venv\Scripts\activate
pip install torch torchvision --index-url https://download.pytorch.org/whl/cu121
pip install -r requirements.txt
To get started quickly, check out @araminta_k tutorial on Finetuning Flux Dev on a 3090 with 24GB VRAM.
You currently need a GPU with at least 24GB of VRAM to train FLUX.1. If you are using it as your GPU to control
your monitors, you probably need to set the flag low_vram: true
in the config file under model:
. This will quantize
the model on CPU and should allow it to train with monitors attached. Users have gotten it to work on Windows with WSL,
but there are some reports of a bug when running on windows natively.
I have only tested on linux for now. This is still extremely experimental
and a lot of quantizing and tricks had to happen to get it to fit on 24GB at all.
FLUX.1-dev has a non-commercial license. Which means anything you train will inherit the non-commercial license. It is also a gated model, so you need to accept the license on HF before using it. Otherwise, this will fail. Here are the required steps to setup a license.
- Sign into HF and accept the model access here black-forest-labs/FLUX.1-dev
- Make a file named
.env
in the root on this folder -
Get a READ key from huggingface and add it to the
.env
file like soHF_TOKEN=your_key_here
FLUX.1-schnell is Apache 2.0. Anything trained on it can be licensed however you want and it does not require a HF_TOKEN to train. However, it does require a special adapter to train with it, ostris/FLUX.1-schnell-training-adapter. It is also highly experimental. For best overall quality, training on FLUX.1-dev is recommended.
To use it, You just need to add the assistant to the model
section of your config file like so:
model:
name_or_path: "black-forest-labs/FLUX.1-schnell"
assistant_lora_path: "ostris/FLUX.1-schnell-training-adapter"
is_flux: true
quantize: true
You also need to adjust your sample steps since schnell does not require as many
sample:
guidance_scale: 1 # schnell does not do guidance
sample_steps: 4 # 1 - 4 works well
- Copy the example config file located at
config/examples/train_lora_flux_24gb.yaml
(config/examples/train_lora_flux_schnell_24gb.yaml
for schnell) to theconfig
folder and rename it towhatever_you_want.yml
- Edit the file following the comments in the file
- Run the file like so
python run.py config/whatever_you_want.yml
A folder with the name and the training folder from the config file will be created when you start. It will have all checkpoints and images in it. You can stop the training at any time using ctrl+c and when you resume, it will pick back up from the last checkpoint.
IMPORTANT. If you press crtl+c while it is saving, it will likely corrupt that checkpoint. So wait until it is done saving
Please do not open a bug report unless it is a bug in the code. You are welcome to Join my Discord and ask for help there. However, please refrain from PMing me directly with general question or support. Ask in the discord and I will answer when I can.
To get started training locally with a with a custom UI, once you followed the steps above and ai-toolkit
is installed:
cd ai-toolkit #in case you are not yet in the ai-toolkit folder
huggingface-cli login #provide a `write` token to publish your LoRA at the end
python flux_train_ui.py
You will instantiate a UI that will let you upload your images, caption them, train and publish your LoRA
Example RunPod template: runpod/pytorch:2.2.0-py3.10-cuda12.1.1-devel-ubuntu22.04
You need a minimum of 24GB VRAM, pick a GPU by your preference.
- 1x A40 (48 GB VRAM)
- 19 vCPU 100 GB RAM
Custom overrides (you need some storage to clone FLUX.1, store datasets, store trained models and samples):
- ~120 GB Disk
- ~120 GB Pod Volume
- Start Jupyter Notebook
git clone https://github.com/ostris/ai-toolkit.git
cd ai-toolkit
git submodule update --init --recursive
python -m venv venv
source venv/bin/activate
pip install torch
pip install -r requirements.txt
pip install --upgrade accelerate transformers diffusers huggingface_hub #Optional, run it if you run into issues
- Create a new folder in the root, name it
dataset
or whatever you like. - Drag and drop your .jpg, .jpeg, or .png images and .txt files inside the newly created dataset folder.
- Get a READ token from here and request access to Flux.1-dev model from here.
- Run
huggingface-cli login
and paste your token.
- Copy an example config file located at
config/examples
to the config folder and rename it towhatever_you_want.yml
. - Edit the config following the comments in the file.
- Change
folder_path: "/path/to/images/folder"
to your dataset path likefolder_path: "/workspace/ai-toolkit/your-dataset"
. - Run the file:
python run.py config/whatever_you_want.yml
.
git clone https://github.com/ostris/ai-toolkit.git
cd ai-toolkit
git submodule update --init --recursive
python -m venv venv
source venv/bin/activate
pip install torch
pip install -r requirements.txt
pip install --upgrade accelerate transformers diffusers huggingface_hub #Optional, run it if you run into issues
- Run
pip install modal
to install the modal Python package. - Run
modal setup
to authenticate (if this doesn’t work, trypython -m modal setup
).
- Get a READ token from here and request access to Flux.1-dev model from here.
- Run
huggingface-cli login
and paste your token.
- Drag and drop your dataset folder containing the .jpg, .jpeg, or .png images and .txt files in
ai-toolkit
.
- Copy an example config file located at
config/examples/modal
to theconfig
folder and rename it towhatever_you_want.yml
. - Edit the config following the comments in the file, be careful and follow the example
/root/ai-toolkit
paths.
-
Set your entire local
ai-toolkit
path atcode_mount = modal.Mount.from_local_dir
like:code_mount = modal.Mount.from_local_dir("/Users/username/ai-toolkit", remote_path="/root/ai-toolkit")
-
Choose a
GPU
andTimeout
in@app.function
(default is A100 40GB and 2 hour timeout).
- Run the config file in your terminal:
modal run run_modal.py --config-file-list-str=/root/ai-toolkit/config/whatever_you_want.yml
. - You can monitor your training in your local terminal, or on modal.com.
- Models, samples and optimizer will be stored in
Storage > flux-lora-models
.
- Check contents of the volume by running
modal volume ls flux-lora-models
. - Download the content by running
modal volume get flux-lora-models your-model-name
. - Example:
modal volume get flux-lora-models my_first_flux_lora_v1
.
Datasets generally need to be a folder containing images and associated text files. Currently, the only supported
formats are jpg, jpeg, and png. Webp currently has issues. The text files should be named the same as the images
but with a .txt
extension. For example image2.jpg
and image2.txt
. The text file should contain only the caption.
You can add the word [trigger]
in the caption file and if you have trigger_word
in your config, it will be automatically
replaced.
Images are never upscaled but they are downscaled and placed in buckets for batching. You do not need to crop/resize your images. The loader will automatically resize them and can handle varying aspect ratios.
To train specific layers with LoRA, you can use the only_if_contains
network kwargs. For instance, if you want to train only the 2 layers
used by The Last Ben, mentioned in this post, you can adjust your
network kwargs like so:
network:
type: "lora"
linear: 128
linear_alpha: 128
network_kwargs:
only_if_contains:
- "transformer.single_transformer_blocks.7.proj_out"
- "transformer.single_transformer_blocks.20.proj_out"
The naming conventions of the layers are in diffusers format, so checking the state dict of a model will reveal
the suffix of the name of the layers you want to train. You can also use this method to only train specific groups of weights.
For instance to only train the single_transformer
for FLUX.1, you can use the following:
network:
type: "lora"
linear: 128
linear_alpha: 128
network_kwargs:
only_if_contains:
- "transformer.single_transformer_blocks."
You can also exclude layers by their names by using ignore_if_contains
network kwarg. So to exclude all the single transformer blocks,
network:
type: "lora"
linear: 128
linear_alpha: 128
network_kwargs:
ignore_if_contains:
- "transformer.single_transformer_blocks."
ignore_if_contains
takes priority over only_if_contains
. So if a weight is covered by both,
if will be ignored.
It may still work like that, but I have not tested it in a while.
A image generator that can take frompts from a config file or form a txt file and generate them to a
folder. I mainly needed this for an SDXL test I am doing but added some polish to it so it can be used
for generat batch image generation.
It all runs off a config file, which you can find an example of in config/examples/generate.example.yaml
.
Mere info is in the comments in the example
It is based on the extractor in the LyCORIS tool, but adding some QOL features
and LoRA (lierla) support. It can do multiple types of extractions in one run.
It all runs off a config file, which you can find an example of in config/examples/extract.example.yml
.
Just copy that file, into the config
folder, and rename it to whatever_you_want.yml
.
Then you can edit the file to your liking. and call it like so:
python3 run.py config/whatever_you_want.yml
You can also put a full path to a config file, if you want to keep it somewhere else.
python3 run.py "/home/user/whatever_you_want.yml"
More notes on how it works are available in the example config file itself. LoRA and LoCON both support extractions of 'fixed', 'threshold', 'ratio', 'quantile'. I'll update what these do and mean later. Most people used fixed, which is traditional fixed dimension extraction.
process
is an array of different processes to run. You can add a few and mix and match. One LoRA, one LyCON, etc.
Change <lora:my_lora:4.6>
to <lora:my_lora:1.0>
or whatever you want with the same effect.
A tool for rescaling a LoRA's weights. Should would with LoCON as well, but I have not tested it.
It all runs off a config file, which you can find an example of in config/examples/mod_lora_scale.yml
.
Just copy that file, into the config
folder, and rename it to whatever_you_want.yml
.
Then you can edit the file to your liking. and call it like so:
python3 run.py config/whatever_you_want.yml
You can also put a full path to a config file, if you want to keep it somewhere else.
python3 run.py "/home/user/whatever_you_want.yml"
More notes on how it works are available in the example config file itself. This is useful when making all LoRAs, as the ideal weight is rarely 1.0, but now you can fix that. For sliders, they can have weird scales form -2 to 2 or even -15 to 15. This will allow you to dile it in so they all have your desired scale
This is how I train most of the recent sliders I have on Civitai, you can check them out in my Civitai profile.
It is based off the work by p1atdev/LECO and rohitgandikota/erasing
But has been heavily modified to create sliders rather than erasing concepts. I have a lot more plans on this, but it is
very functional as is. It is also very easy to use. Just copy the example config file in config/examples/train_slider.example.yml
to the config
folder and rename it to whatever_you_want.yml
. Then you can edit the file to your liking. and call it like so:
python3 run.py config/whatever_you_want.yml
There is a lot more information in that example file. You can even run the example as is without any modifications to see how it works. It will create a slider that turns all animals into dogs(neg) or cats(pos). Just run it like so:
python3 run.py config/examples/train_slider.example.yml
And you will be able to see how it works without configuring anything. No datasets are required for this method. I will post an better tutorial soon.
You can now make and share custom extensions. That run within this framework and have all the inbuilt tools
available to them. I will probably use this as the primary development method going
forward so I dont keep adding and adding more and more features to this base repo. I will likely migrate a lot
of the existing functionality as well to make everything modular. There is an example extension in the extensions
folder that shows how to make a model merger extension. All of the code is heavily documented which is hopefully
enough to get you started. To make an extension, just copy that example and replace all the things you need to.
It is located in the extensions
folder. It is a fully finctional model merger that can merge as many models together
as you want. It is a good example of how to make an extension, but is also a pretty useful feature as well since most
mergers can only do one model at a time and this one will take as many as you want to feed it. There is an
example config file in there, just copy that to your config
folder and rename it to whatever_you_want.yml
.
and use it like any other config file.
This works, but is not ready for others to use and therefore does not have an example config. I am still working on it. I will update this when it is ready. I am adding a lot of features for criteria that I have used in my image enlargement work. A Critic (discriminator), content loss, style loss, and a few more. If you don't know, the VAE for stable diffusion (yes even the MSE one, and SDXL), are horrible at smaller faces and it holds SD back. I will fix this. I'll post more about this later with better examples later, but here is a quick test of a run through with various VAEs. Just went in and out. It is much worse on smaller faces than shown here.
- [X] Add proper regs on sliders
- [X] Add SDXL support (base model only for now)
- [ ] Add plain erasing
- [ ] Make Textual inversion network trainer (network that spits out TI embeddings)
- Huge memory rework and slider rework. Slider training is better thant ever with no more ram spikes. I also made it so all 4 parts of the slider algorythm run in one batch so they share gradient accumulation. This makes it much faster and more stable.
- Updated the example config to be something more practical and more updated to current methods. It is now a detail slide and shows how to train one without a subject. 512x512 slider training for 1.5 should work on 6GB gpu now. Will test soon to verify.
- Windows support bug fixes
- Extensions! Added functionality to make and share custom extensions for training, merging, whatever.
check out the example in the
extensions
folder. Read more about that above. - Model Merging, provided via the example extension.
Another big refactor to make SD more modular.
Made batch image generation script
Major changes and update. New LoRA rescale tool, look above for details. Added better metadata so Automatic1111 knows what the base model is. Added some experiments and a ton of updates. This thing is still unstable at the moment, so hopefully there are not breaking changes.
Unfortunately, I am too lazy to write a proper changelog with all the changes.
I added SDXL training to sliders... but.. it does not work properly. The slider training relies on a model's ability to understand that an unconditional (negative prompt) means you do not want that concept in the output. SDXL does not understand this for whatever reason, which makes separating out concepts within the model hard. I am sure the community will find a way to fix this over time, but for now, it is not going to work properly. And if any of you are thinking "Could we maybe fix it by adding 1 or 2 more text encoders to the model as well as a few more entirely separate diffusion networks?" No. God no. It just needs a little training without every experimental new paper added to it. The KISS principal.
Added "anchors" to the slider trainer. This allows you to set a prompt that will be used as a regularizer. You can set the network multiplier to force spread consistency at high weights
For Tasks:
Click tags to check more tools for each tasksFor Jobs:
Alternative AI tools for ai-toolkit
Similar Open Source Tools
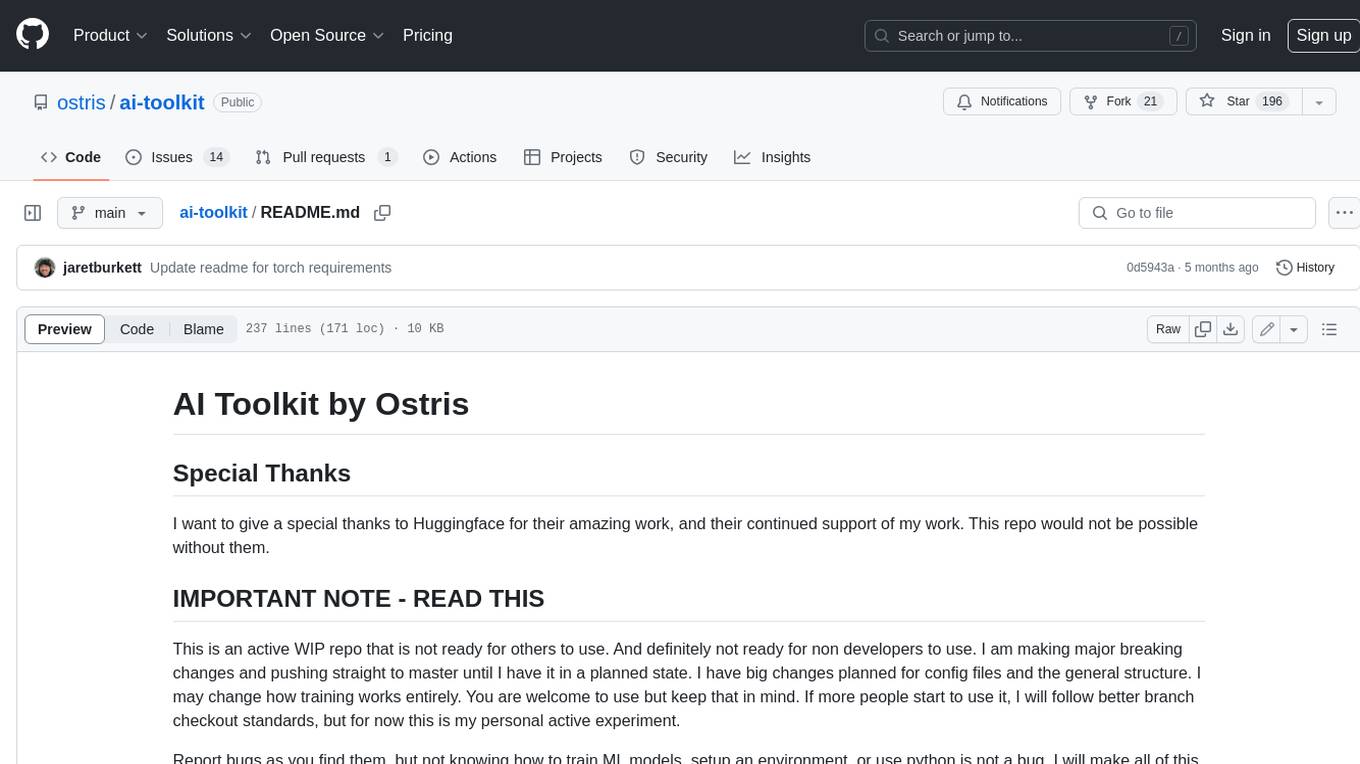
ai-toolkit
The AI Toolkit by Ostris is a collection of tools for machine learning, specifically designed for image generation, LoRA (latent representations of attributes) extraction and manipulation, and model training. It provides a user-friendly interface and extensive documentation to make it accessible to both developers and non-developers. The toolkit is actively under development, with new features and improvements being added regularly. Some of the key features of the AI Toolkit include: - Batch Image Generation: Allows users to generate a batch of images based on prompts or text files, using a configuration file to specify the desired settings. - LoRA (lierla), LoCON (LyCORIS) Extractor: Facilitates the extraction of LoRA and LoCON representations from pre-trained models, enabling users to modify and manipulate these representations for various purposes. - LoRA Rescale: Provides a tool to rescale LoRA weights, allowing users to adjust the influence of specific attributes in the generated images. - LoRA Slider Trainer: Enables the training of LoRA sliders, which can be used to control and adjust specific attributes in the generated images, offering a powerful tool for fine-tuning and customization. - Extensions: Supports the creation and sharing of custom extensions, allowing users to extend the functionality of the toolkit with their own tools and scripts. - VAE (Variational Auto Encoder) Trainer: Facilitates the training of VAEs for image generation, providing users with a tool to explore and improve the quality of generated images. The AI Toolkit is a valuable resource for anyone interested in exploring and utilizing machine learning for image generation and manipulation. Its user-friendly interface, extensive documentation, and active development make it an accessible and powerful tool for both beginners and experienced users.
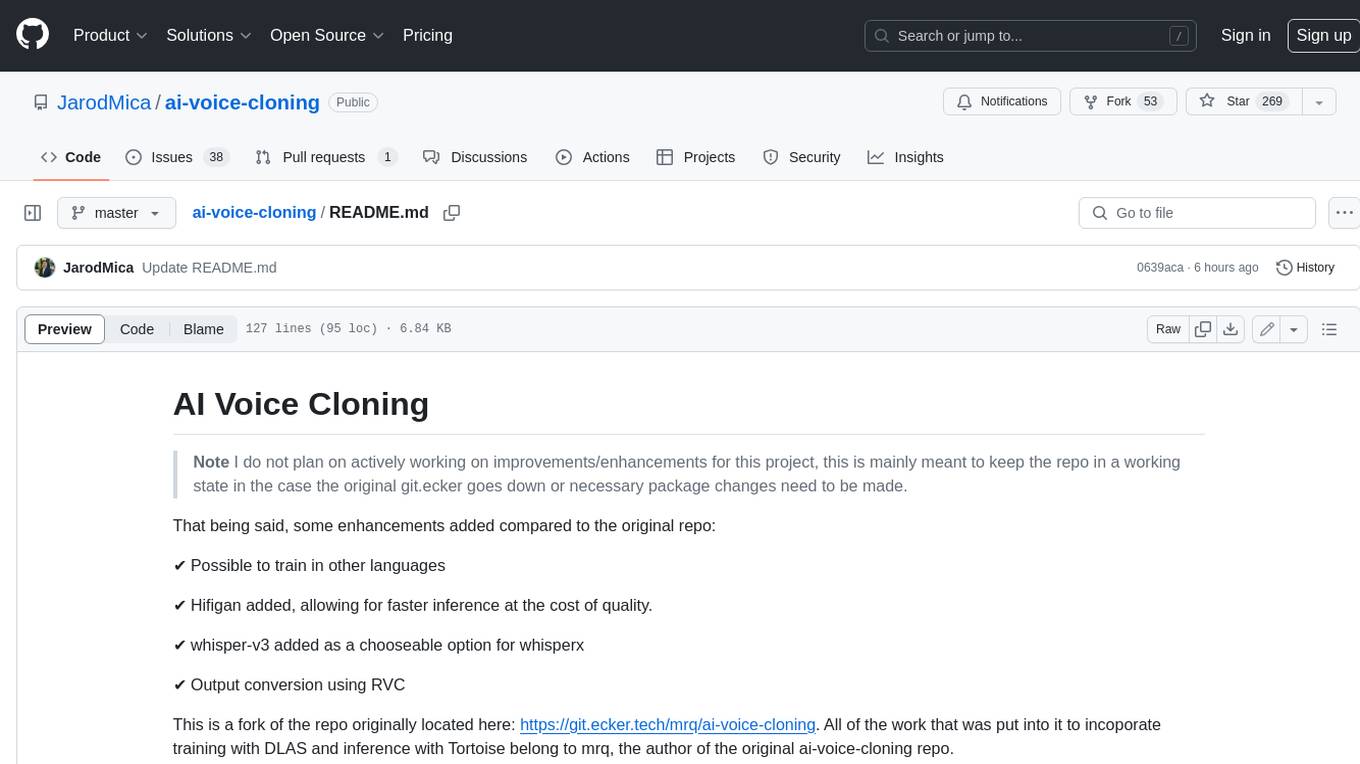
ai-voice-cloning
This repository provides a tool for AI voice cloning, allowing users to generate synthetic speech that closely resembles a target speaker's voice. The tool is designed to be user-friendly and accessible, with a graphical user interface that guides users through the process of training a voice model and generating synthetic speech. The tool also includes a variety of features that allow users to customize the generated speech, such as the pitch, volume, and speaking rate. Overall, this tool is a valuable resource for anyone interested in creating realistic and engaging synthetic speech.
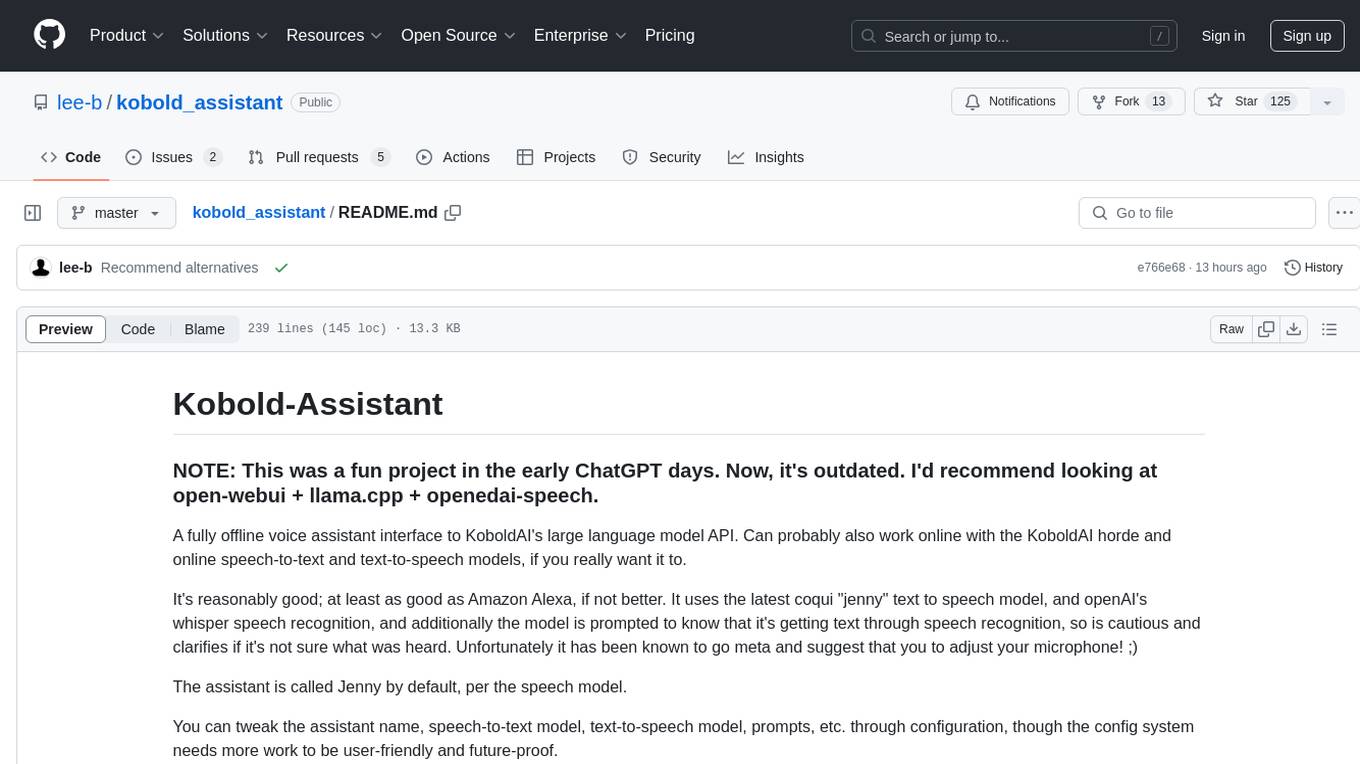
kobold_assistant
Kobold-Assistant is a fully offline voice assistant interface to KoboldAI's large language model API. It can work online with the KoboldAI horde and online speech-to-text and text-to-speech models. The assistant, called Jenny by default, uses the latest coqui 'jenny' text to speech model and openAI's whisper speech recognition. Users can customize the assistant name, speech-to-text model, text-to-speech model, and prompts through configuration. The tool requires system packages like GCC, portaudio development libraries, and ffmpeg, along with Python >=3.7, <3.11, and runs on Ubuntu/Debian systems. Users can interact with the assistant through commands like 'serve' and 'list-mics'.
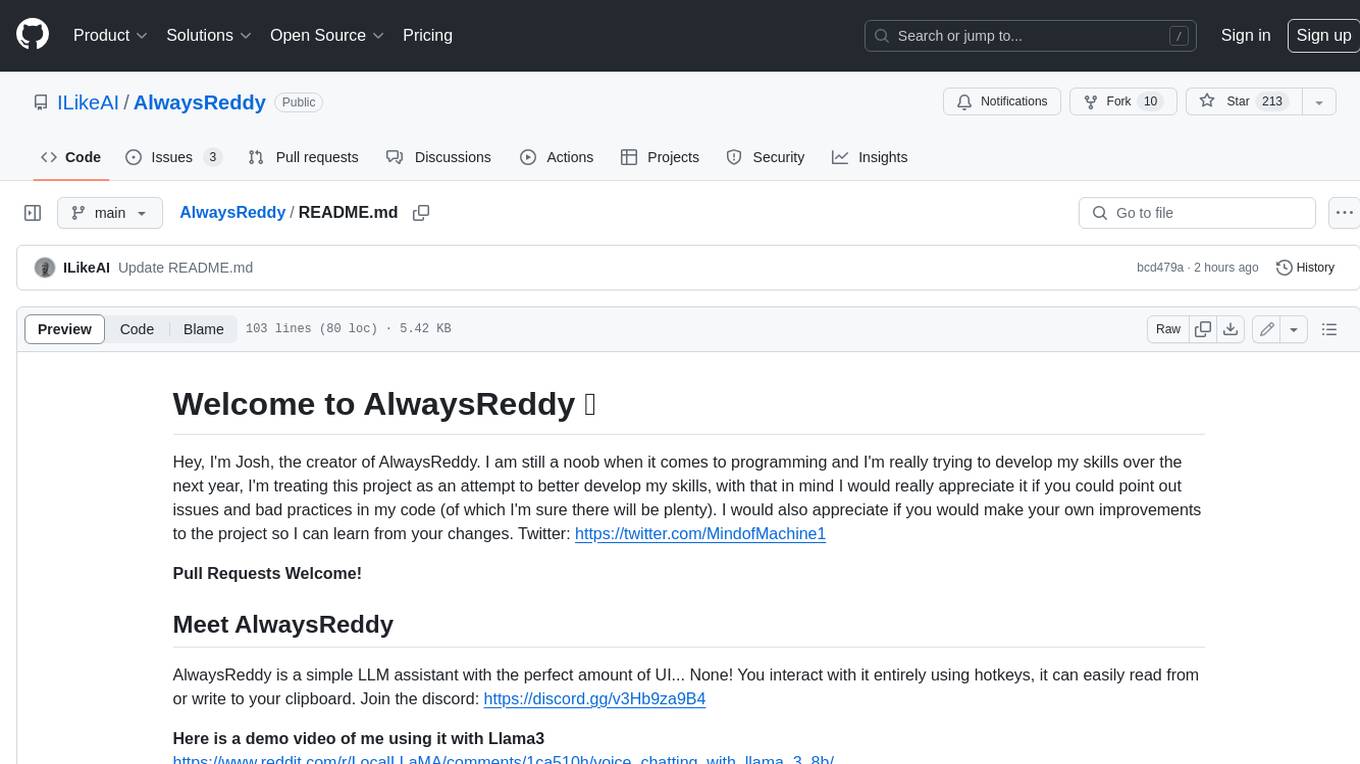
AlwaysReddy
AlwaysReddy is a simple LLM assistant with no UI that you interact with entirely using hotkeys. It can easily read from or write to your clipboard, and voice chat with you via TTS and STT. Here are some of the things you can use AlwaysReddy for: - Explain a new concept to AlwaysReddy and have it save the concept (in roughly your words) into a note. - Ask AlwaysReddy "What is X called?" when you know how to roughly describe something but can't remember what it is called. - Have AlwaysReddy proofread the text in your clipboard before you send it. - Ask AlwaysReddy "From the comments in my clipboard, what do the r/LocalLLaMA users think of X?" - Quickly list what you have done today and get AlwaysReddy to write a journal entry to your clipboard before you shutdown the computer for the day.
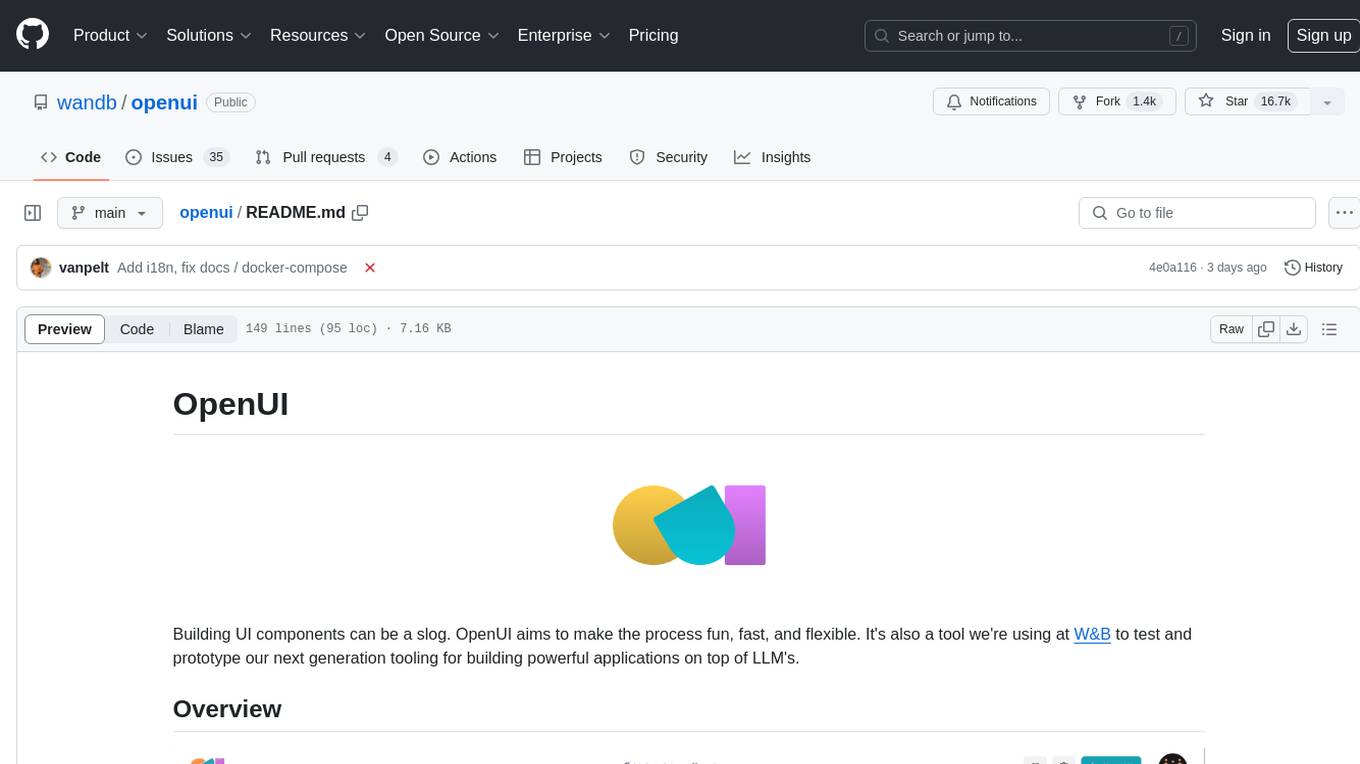
openui
OpenUI is a tool designed to simplify the process of building UI components by allowing users to describe UI using their imagination and see it rendered live. It supports converting HTML to React, Svelte, Web Components, etc. The tool is open source and aims to make UI development fun, fast, and flexible. It integrates with various AI services like OpenAI, Groq, Gemini, Anthropic, Cohere, and Mistral, providing users with the flexibility to use different models. OpenUI also supports LiteLLM for connecting to various LLM services and allows users to create custom proxy configs. The tool can be run locally using Docker or Python, and it offers a development environment for quick setup and testing.
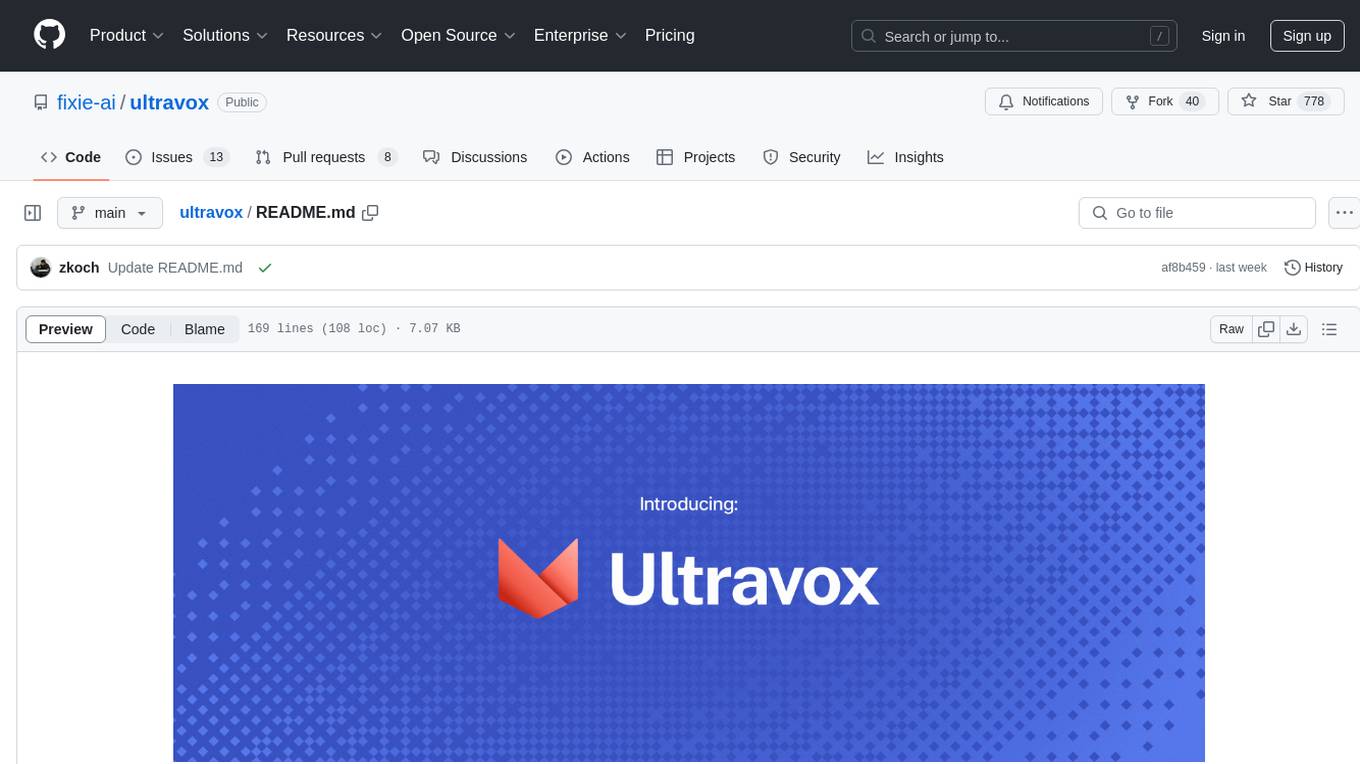
ultravox
Ultravox is a fast multimodal Language Model (LLM) that can understand both text and human speech in real-time without the need for a separate Audio Speech Recognition (ASR) stage. By extending Meta's Llama 3 model with a multimodal projector, Ultravox converts audio directly into a high-dimensional space used by Llama 3, enabling quick responses and potential understanding of paralinguistic cues like timing and emotion in human speech. The current version (v0.3) has impressive speed metrics and aims for further enhancements. Ultravox currently converts audio to streaming text and plans to emit speech tokens for direct audio conversion. The tool is open for collaboration to enhance this functionality.
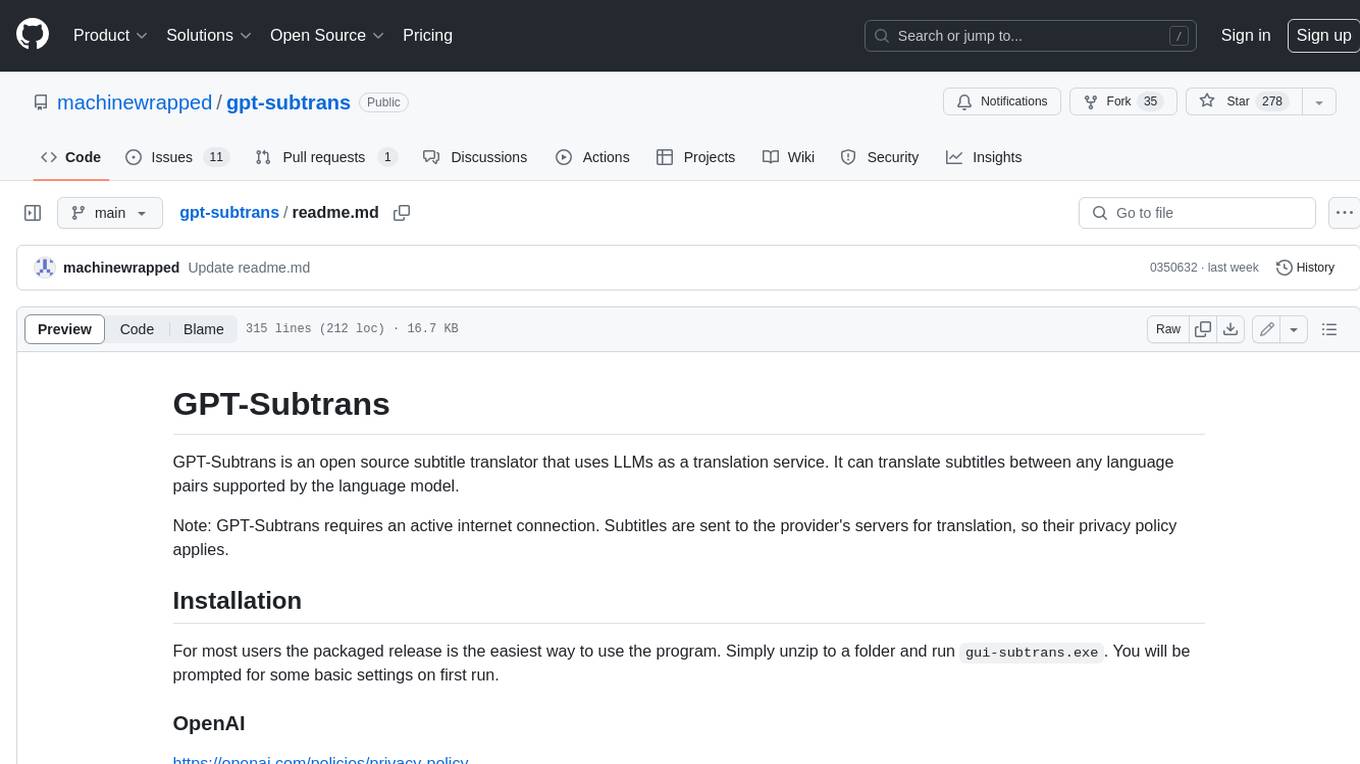
gpt-subtrans
GPT-Subtrans is an open-source subtitle translator that utilizes large language models (LLMs) as translation services. It supports translation between any language pairs that the language model supports. Note that GPT-Subtrans requires an active internet connection, as subtitles are sent to the provider's servers for translation, and their privacy policy applies.
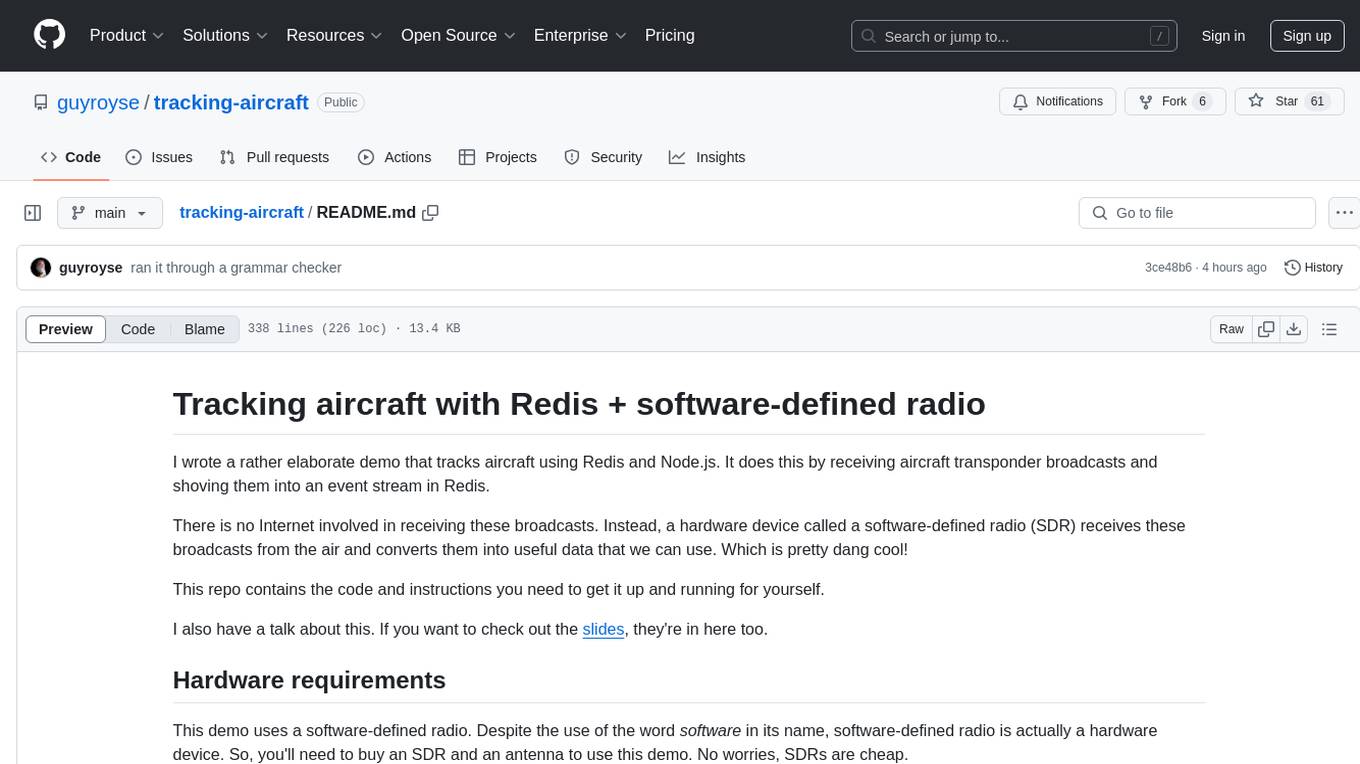
tracking-aircraft
This repository provides a demo that tracks aircraft using Redis and Node.js by receiving aircraft transponder broadcasts through a software-defined radio (SDR) and storing them in Redis. The demo includes instructions for setting up the hardware and software components required for tracking aircraft. It consists of four main components: Radio Ingestor, Flight Server, Flight UI, and Redis. The Radio Ingestor captures transponder broadcasts and writes them to a Redis event stream, while the Flight Server consumes the event stream, enriches the data, and provides APIs to query aircraft status. The Flight UI presents flight data to users in map and detail views. Users can run the demo by setting up the hardware, installing SDR software, and running the components using Docker or Node.js.
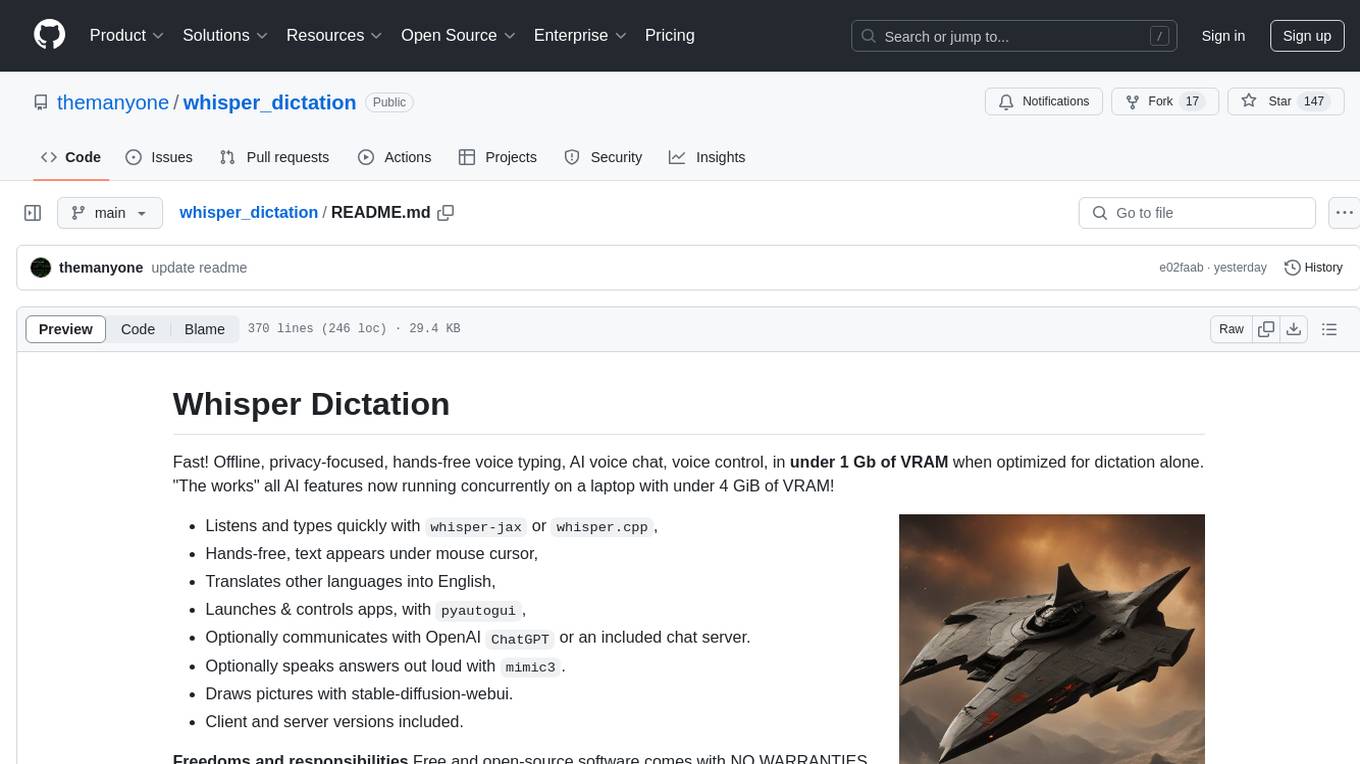
whisper_dictation
Whisper Dictation is a fast, offline, privacy-focused tool for voice typing, AI voice chat, voice control, and translation. It allows hands-free operation, launching and controlling apps, and communicating with OpenAI ChatGPT or a local chat server. The tool also offers the option to speak answers out loud and draw pictures. It includes client and server versions, inspired by the Star Trek series, and is designed to keep data off the internet and confidential. The project is optimized for dictation and translation tasks, with voice control capabilities and AI image generation using stable-diffusion API.
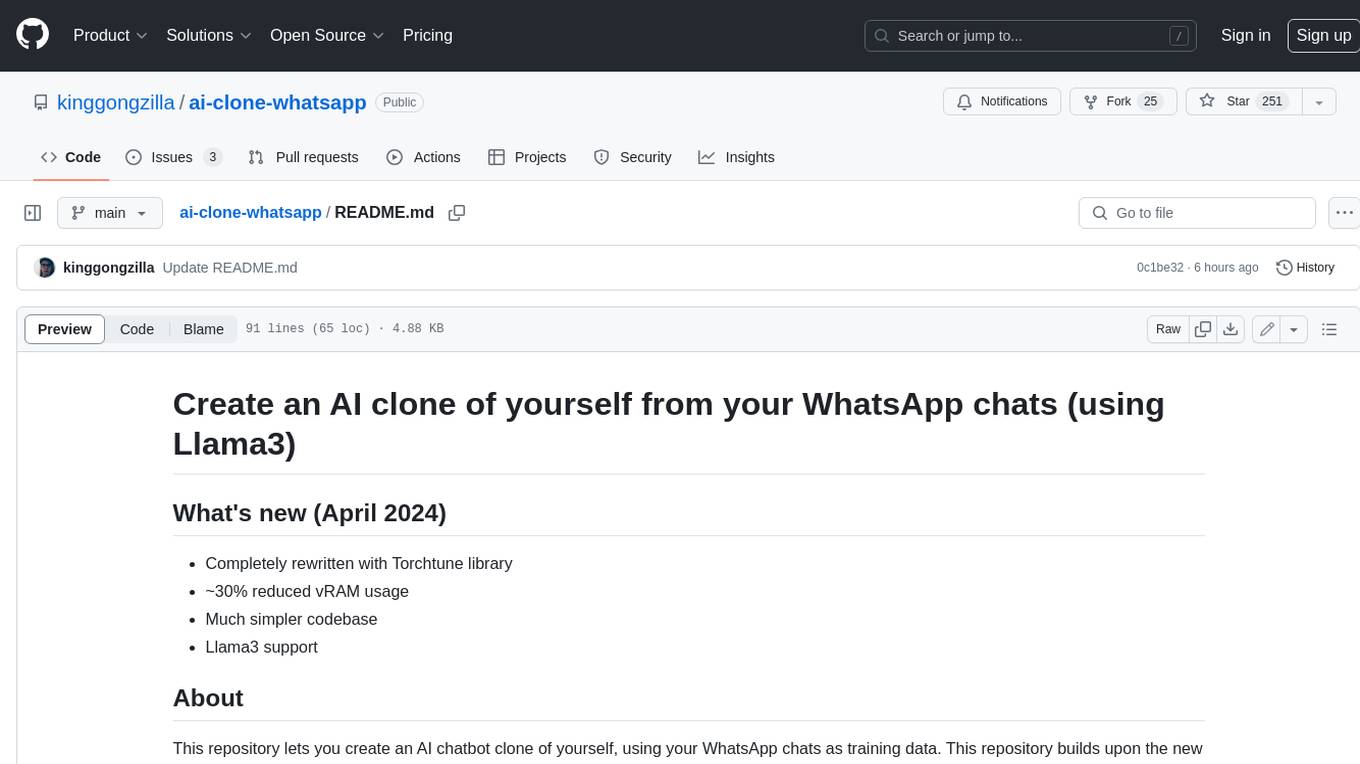
ai-clone-whatsapp
This repository provides a tool to create an AI chatbot clone of yourself using your WhatsApp chats as training data. It utilizes the Torchtune library for finetuning and inference. The code includes preprocessing of WhatsApp chats, finetuning models, and chatting with the AI clone via a command-line interface. Supported models are Llama3-8B-Instruct and Mistral-7B-Instruct-v0.2. Hardware requirements include approximately 16 GB vRAM for QLoRa Llama3 finetuning with a 4k context length. The repository addresses common issues like adjusting parameters for training and preprocessing non-English chats.
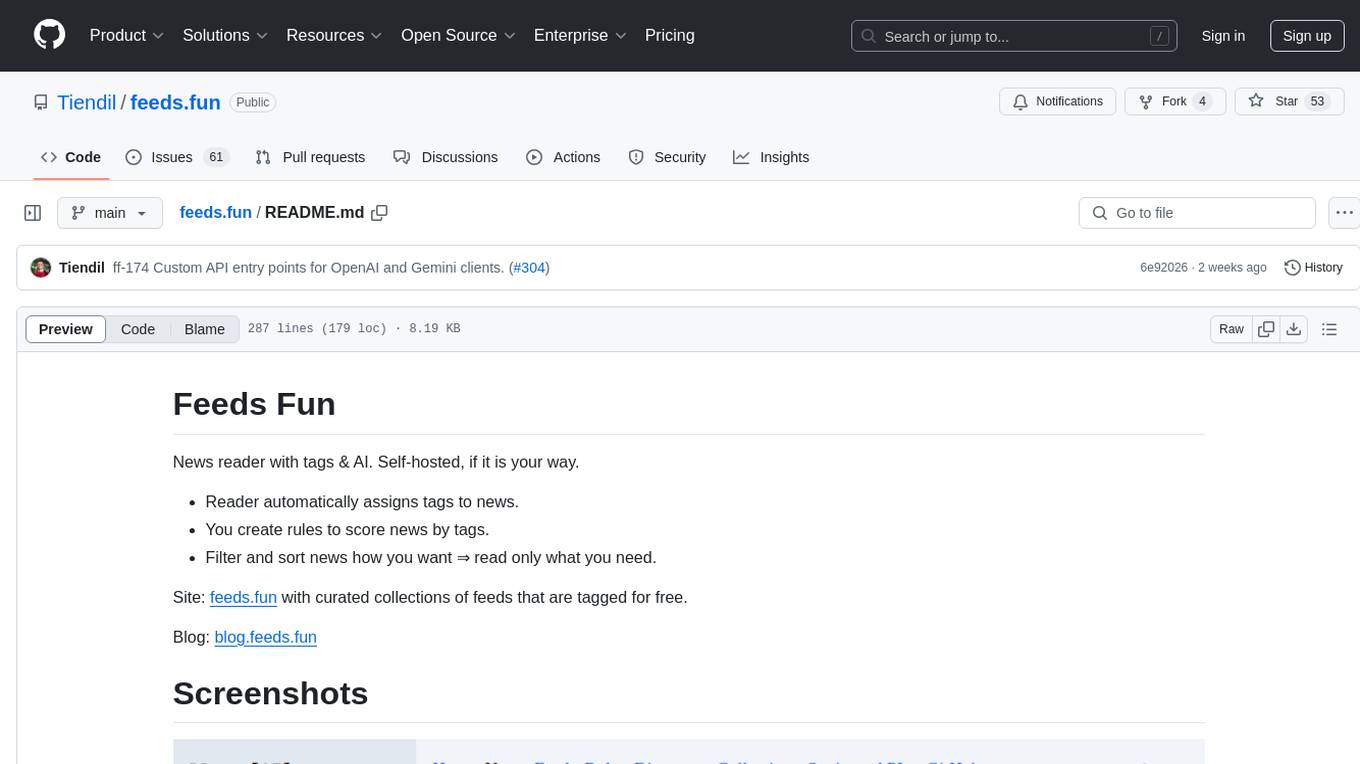
feeds.fun
Feeds Fun is a self-hosted news reader tool that automatically assigns tags to news entries. Users can create rules to score news based on tags, filter and sort news as needed, and track read news. The tool offers multi/single-user support, feeds management, and various features for personalized news consumption. Users can access the tool's backend as the ffun package on PyPI and the frontend as the feeds-fun package on NPM. Feeds Fun requires setting up OpenAI or Gemini API keys for full tag generation capabilities. The tool uses tag processors to detect tags for news entries, with options for simple and complex processors. Feeds Fun primarily relies on LLM tag processors from OpenAI and Google for tag generation.
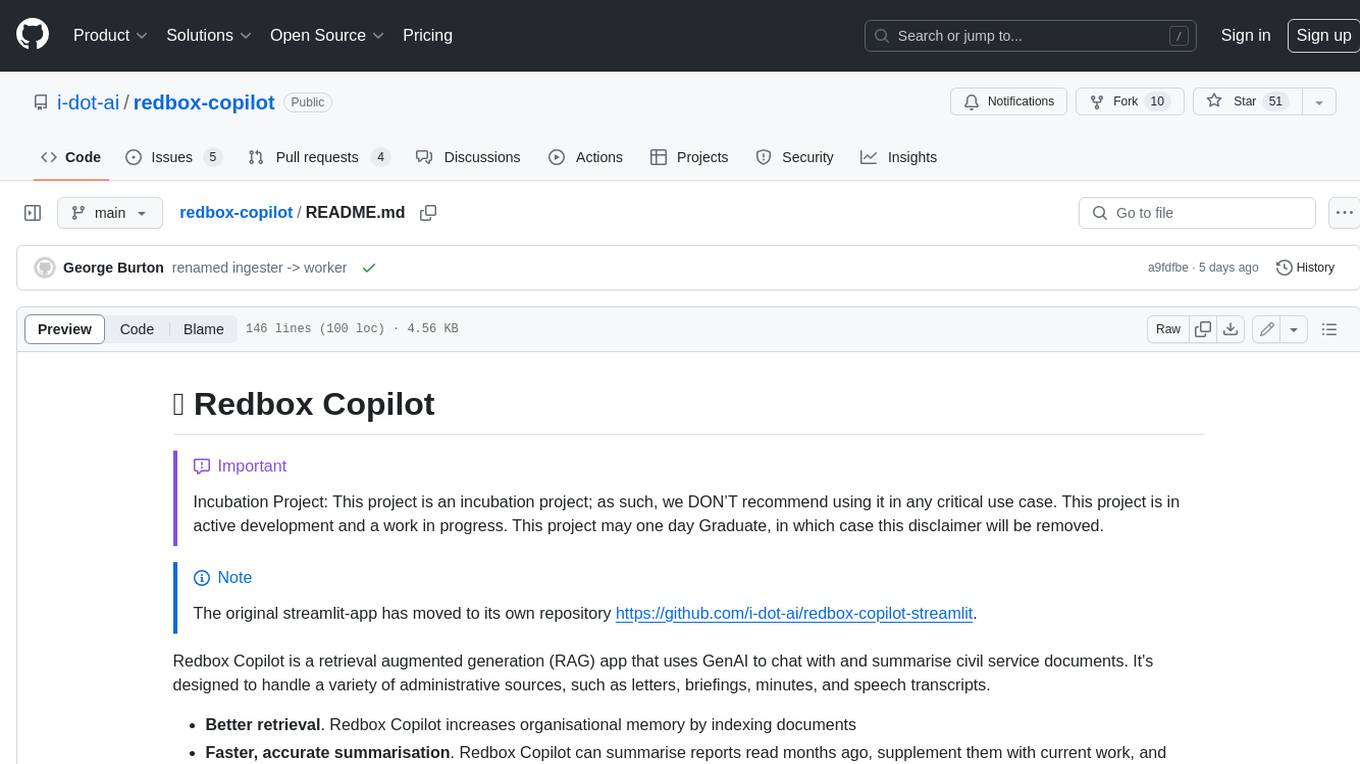
redbox-copilot
Redbox Copilot is a retrieval augmented generation (RAG) app that uses GenAI to chat with and summarise civil service documents. It increases organisational memory by indexing documents and can summarise reports read months ago, supplement them with current work, and produce a first draft that lets civil servants focus on what they do best. The project uses a microservice architecture with each microservice running in its own container defined by a Dockerfile. Dependencies are managed using Python Poetry. Contributions are welcome, and the project is licensed under the MIT License.
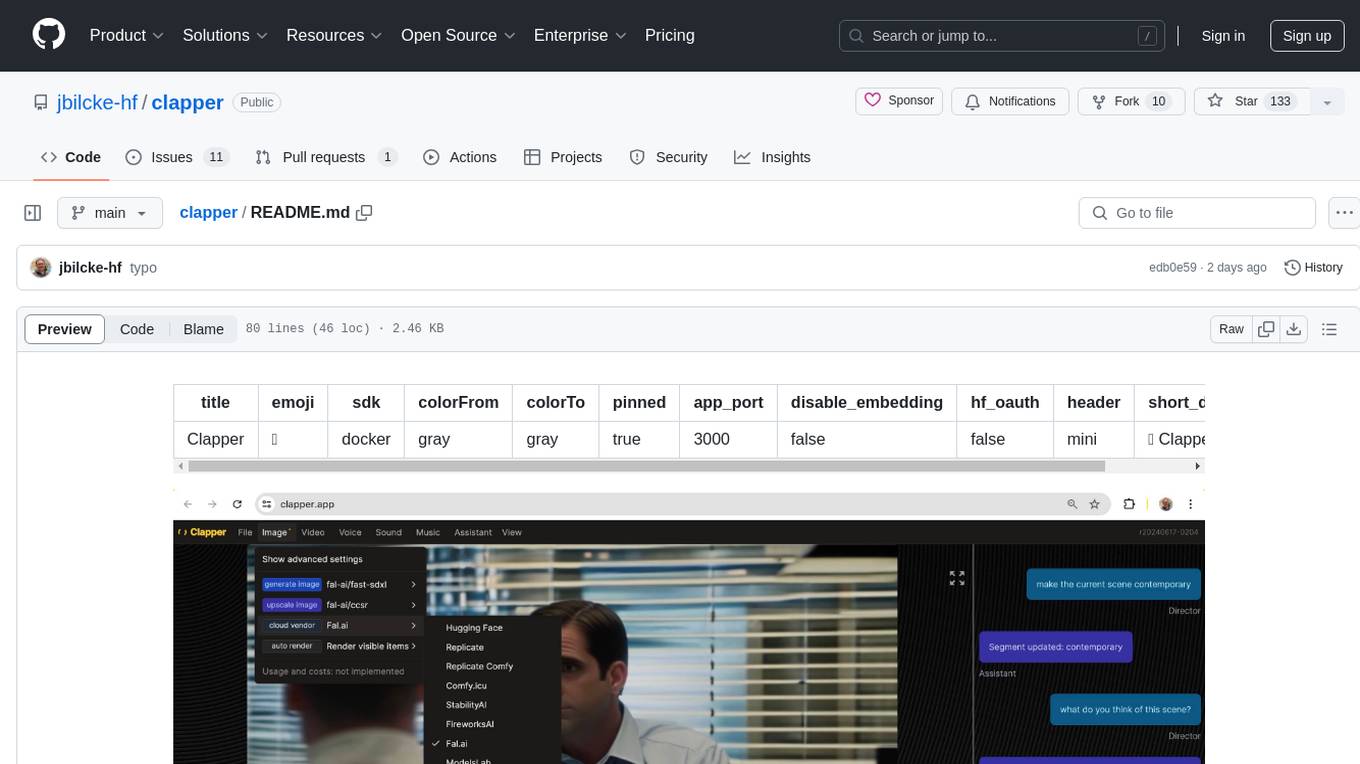
clapper
Clapper is an open-source AI story visualization tool that can interpret screenplays and render them into storyboards, videos, voice, sound, and music. It is currently in early development stages and not recommended for general use due to some non-functional features and lack of tutorials. A public alpha version is available on Hugging Face's platform. Users can sponsor specific features through bounties and developers can contribute to the project under the GPL v3 license. The tool lacks automated tests and code conventions like Prettier or a Linter.
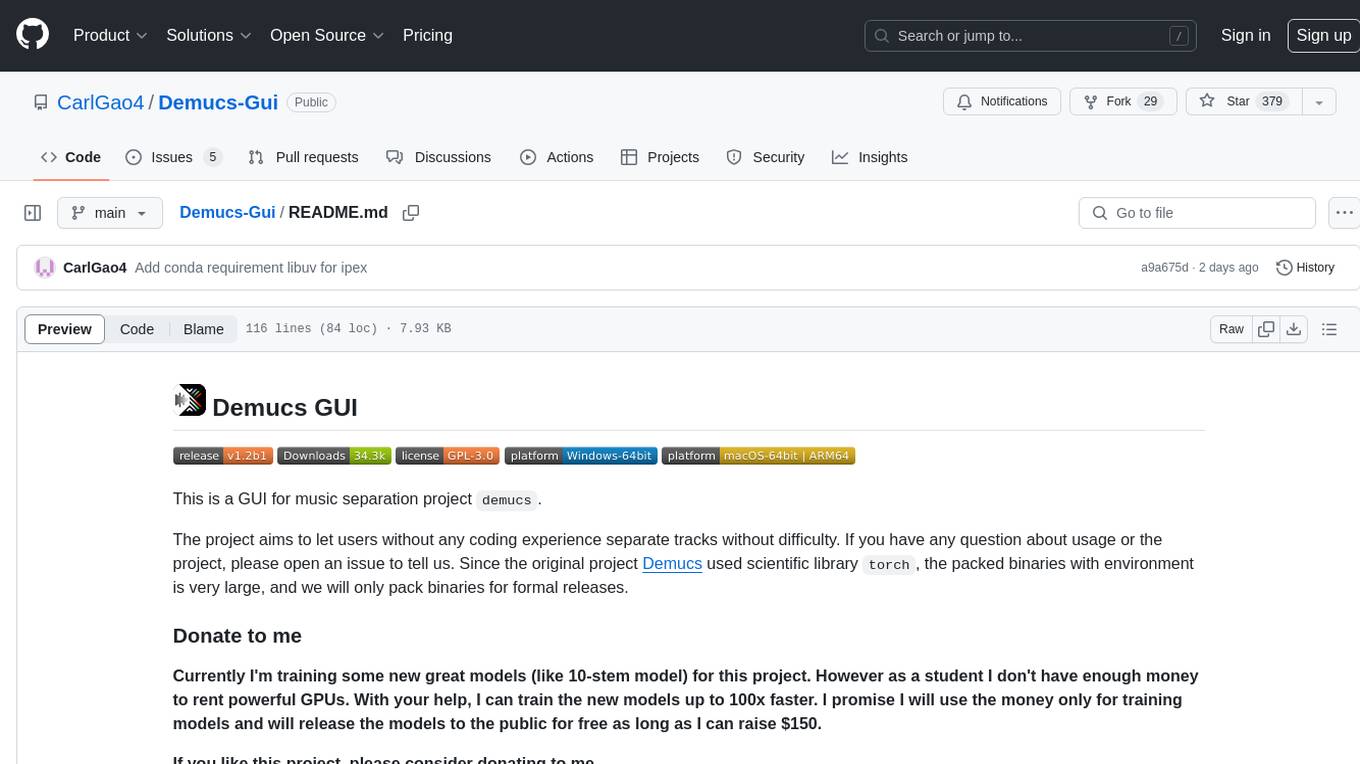
Demucs-Gui
Demucs GUI is a graphical user interface for the music separation project Demucs. It aims to allow users without coding experience to easily separate tracks. The tool provides a user-friendly interface for running the Demucs project, which originally used the scientific library torch. The GUI simplifies the process of separating tracks and provides support for different platforms such as Windows, macOS, and Linux. Users can donate to support the development of new models for the project, and the tool has specific system requirements including minimum system versions and hardware specifications.
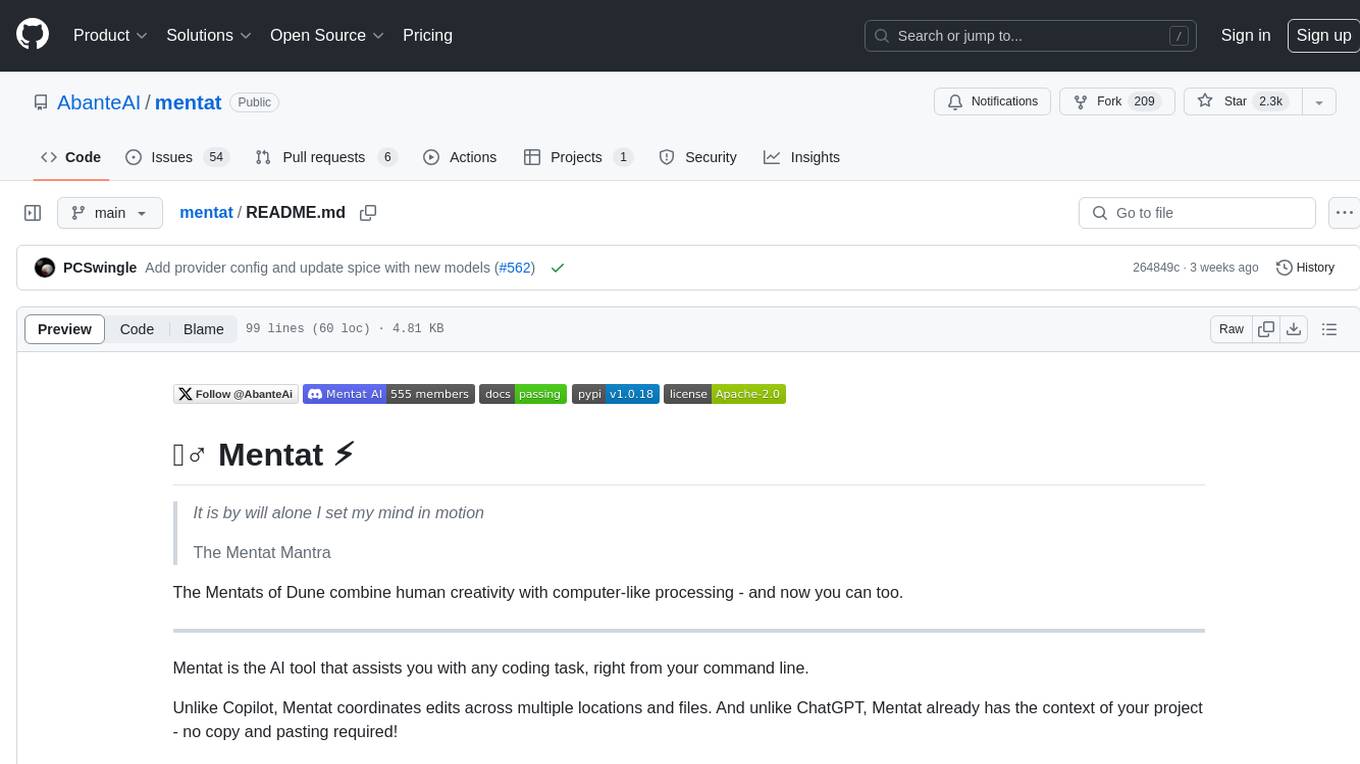
mentat
Mentat is an AI tool designed to assist with coding tasks directly from the command line. It combines human creativity with computer-like processing to help users understand new codebases, add new features, and refactor existing code. Unlike other tools, Mentat coordinates edits across multiple locations and files, with the context of the project already in mind. The tool aims to enhance the coding experience by providing seamless assistance and improving edit quality.
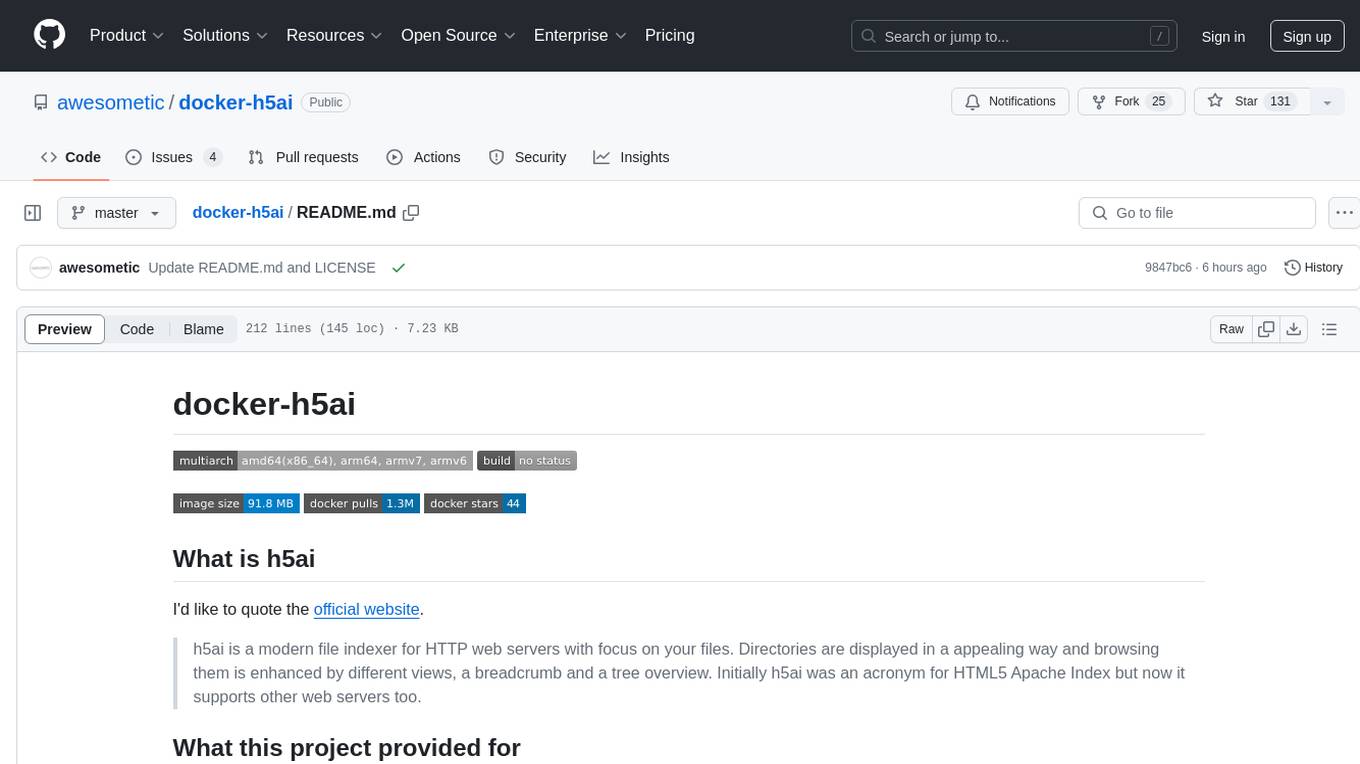
docker-h5ai
docker-h5ai is a Docker image that provides a modern file indexer for HTTP web servers, enhancing file browsing with different views, a breadcrumb, and a tree overview. It is built on Alpine Linux with Nginx and PHP, supporting h5ai 0.30.0 and enabling PHP 8 JIT compiler. The image supports multiple architectures and can be used to host shared files with customizable configurations. Users can set up authentication using htpasswd and run the image as a real-time service. It is recommended to use HTTPS for data encryption when deploying the service.
For similar tasks
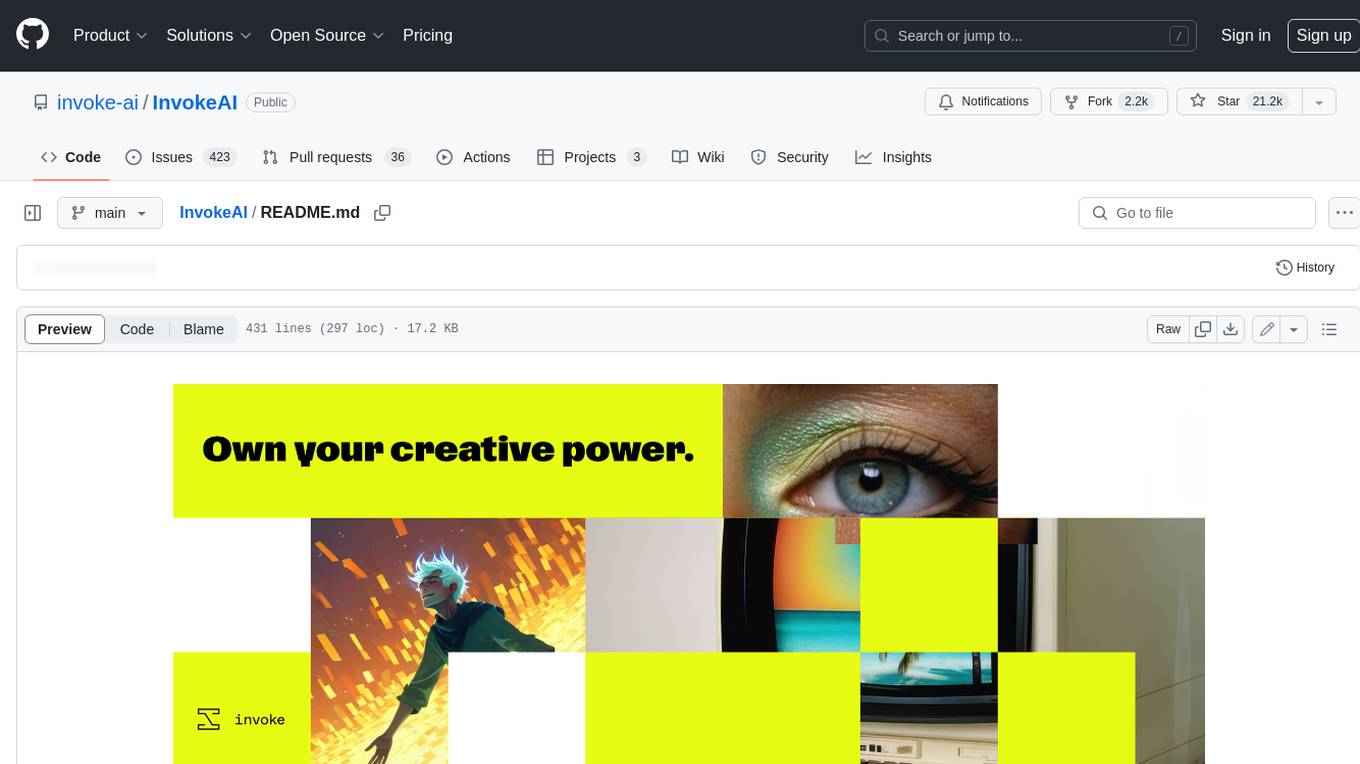
InvokeAI
InvokeAI is a leading creative engine built to empower professionals and enthusiasts alike. Generate and create stunning visual media using the latest AI-driven technologies. InvokeAI offers an industry leading Web Interface, interactive Command Line Interface, and also serves as the foundation for multiple commercial products.
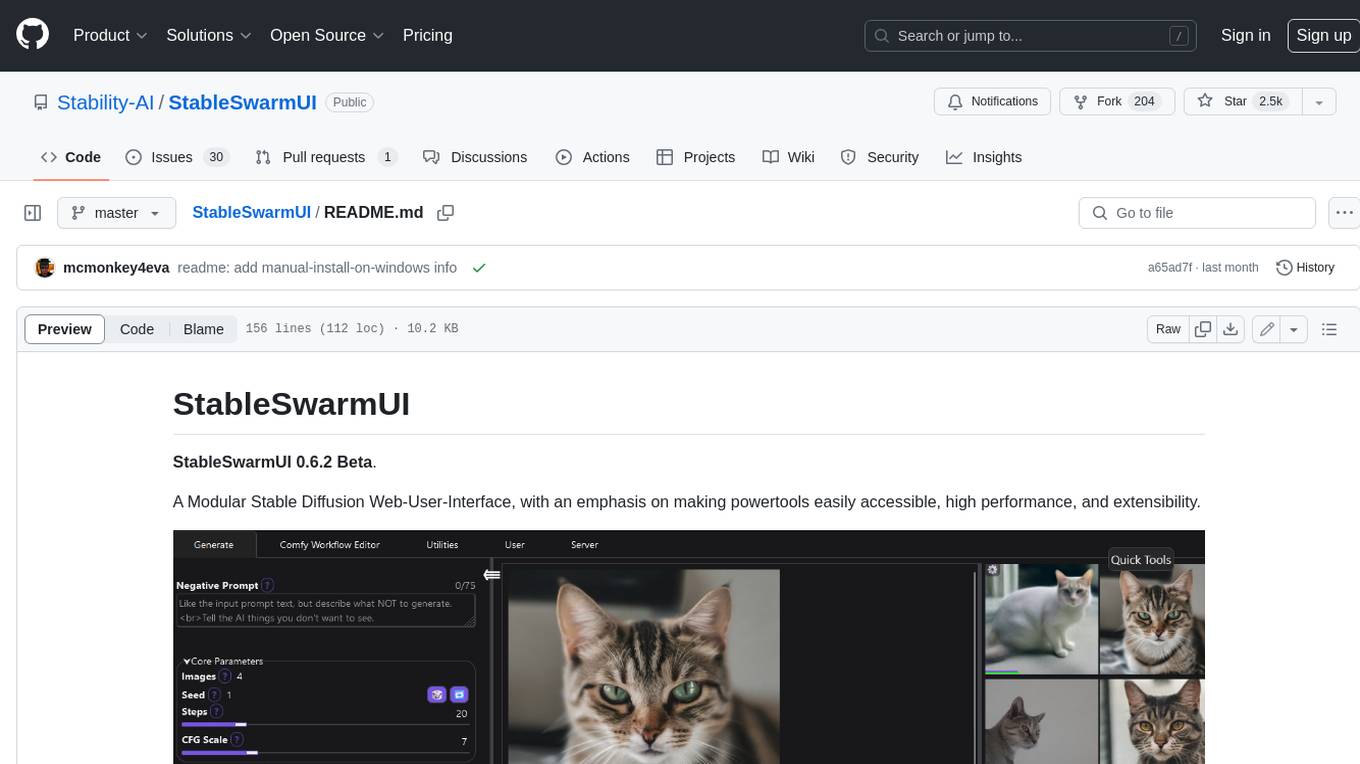
StableSwarmUI
StableSwarmUI is a modular Stable Diffusion web user interface that emphasizes making power tools easily accessible, high performance, and extensible. It is designed to be a one-stop-shop for all things Stable Diffusion, providing a wide range of features and capabilities to enhance the user experience.
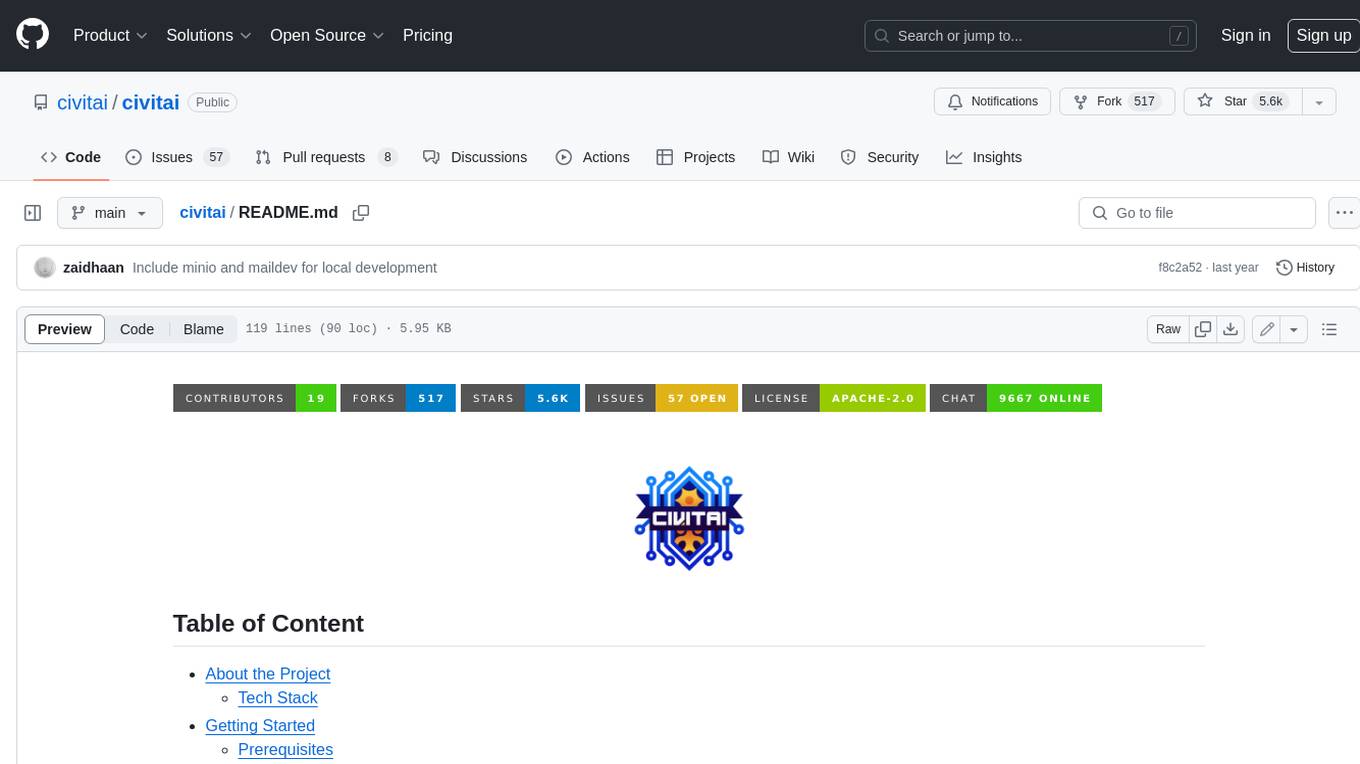
civitai
Civitai is a platform where people can share their stable diffusion models (textual inversions, hypernetworks, aesthetic gradients, VAEs, and any other crazy stuff people do to customize their AI generations), collaborate with others to improve them, and learn from each other's work. The platform allows users to create an account, upload their models, and browse models that have been shared by others. Users can also leave comments and feedback on each other's models to facilitate collaboration and knowledge sharing.
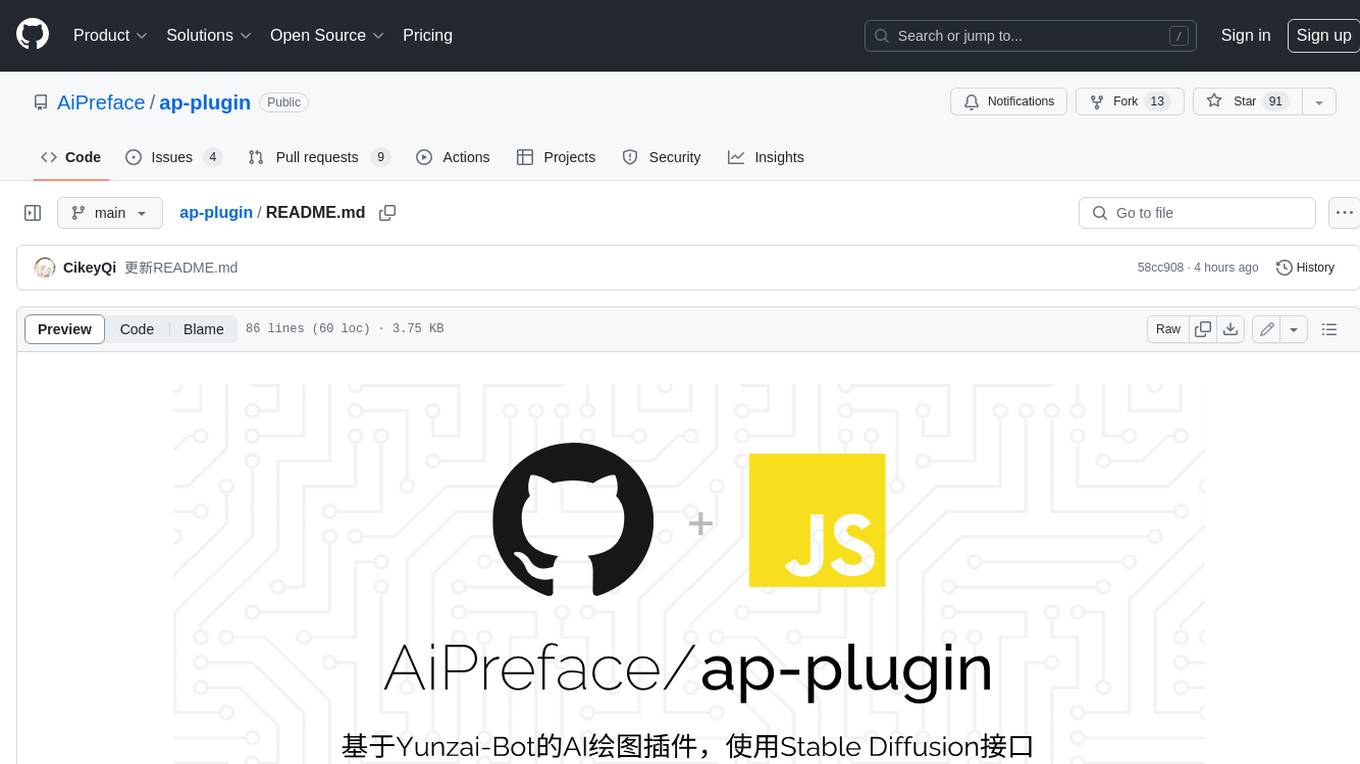
ap-plugin
AP-PLUGIN is an AI drawing plugin for the Yunzai series robot framework, allowing you to have a convenient AI drawing experience in the input box. It uses the open source Stable Diffusion web UI as the backend, deploys it for free, and generates a variety of images with richer functions.
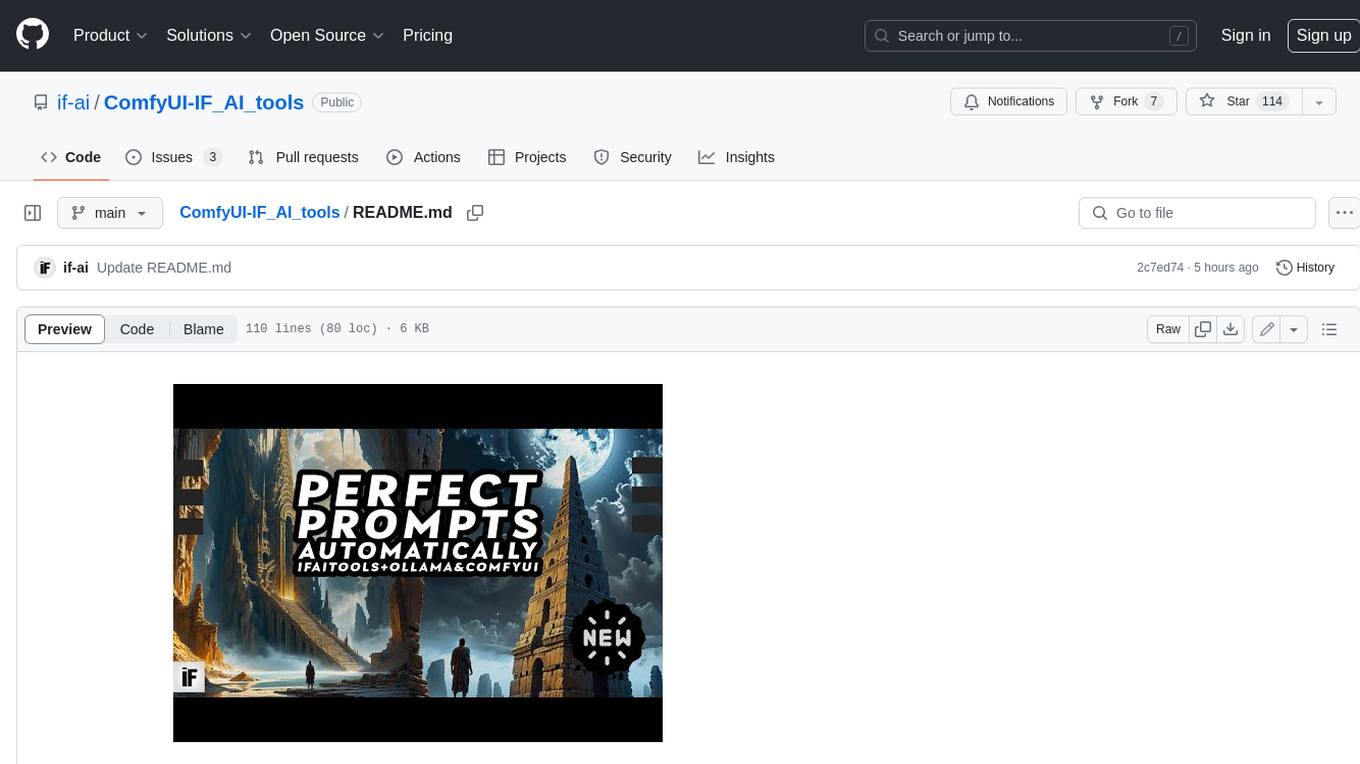
ComfyUI-IF_AI_tools
ComfyUI-IF_AI_tools is a set of custom nodes for ComfyUI that allows you to generate prompts using a local Large Language Model (LLM) via Ollama. This tool enables you to enhance your image generation workflow by leveraging the power of language models.
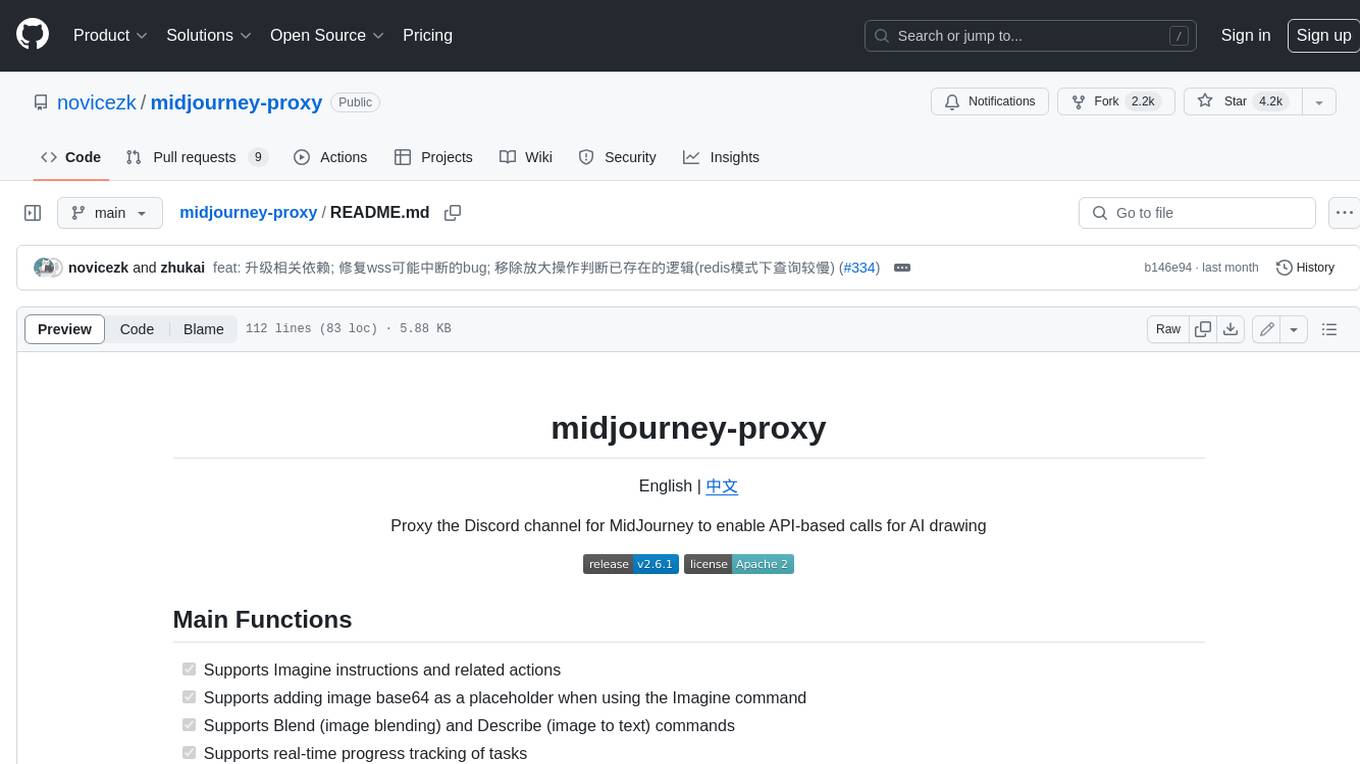
midjourney-proxy
Midjourney-proxy is a proxy for the Discord channel of MidJourney, enabling API-based calls for AI drawing. It supports Imagine instructions, adding image base64 as a placeholder, Blend and Describe commands, real-time progress tracking, Chinese prompt translation, prompt sensitive word pre-detection, user-token connection to WSS, multi-account configuration, and more. For more advanced features, consider using midjourney-proxy-plus, which includes Shorten, focus shifting, image zooming, local redrawing, nearly all associated button actions, Remix mode, seed value retrieval, account pool persistence, dynamic maintenance, /info and /settings retrieval, account settings configuration, Niji bot robot, InsightFace face replacement robot, and an embedded management dashboard.
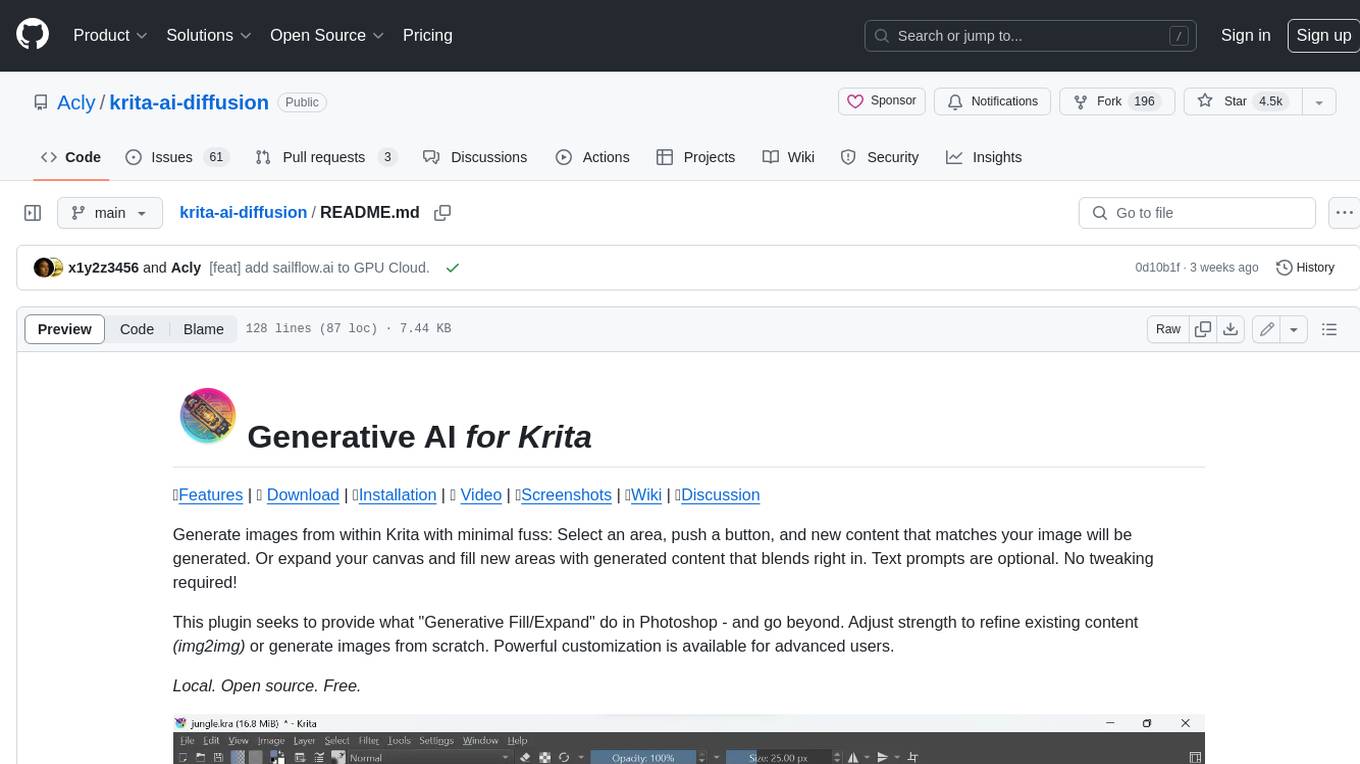
krita-ai-diffusion
Krita-AI-Diffusion is a plugin for Krita that allows users to generate images from within the program. It offers a variety of features, including inpainting, outpainting, generating images from scratch, refining existing content, live painting, and control over image creation. The plugin is designed to fit into an interactive workflow where AI generation is used as just another tool while painting. It is meant to synergize with traditional tools and the layer stack.
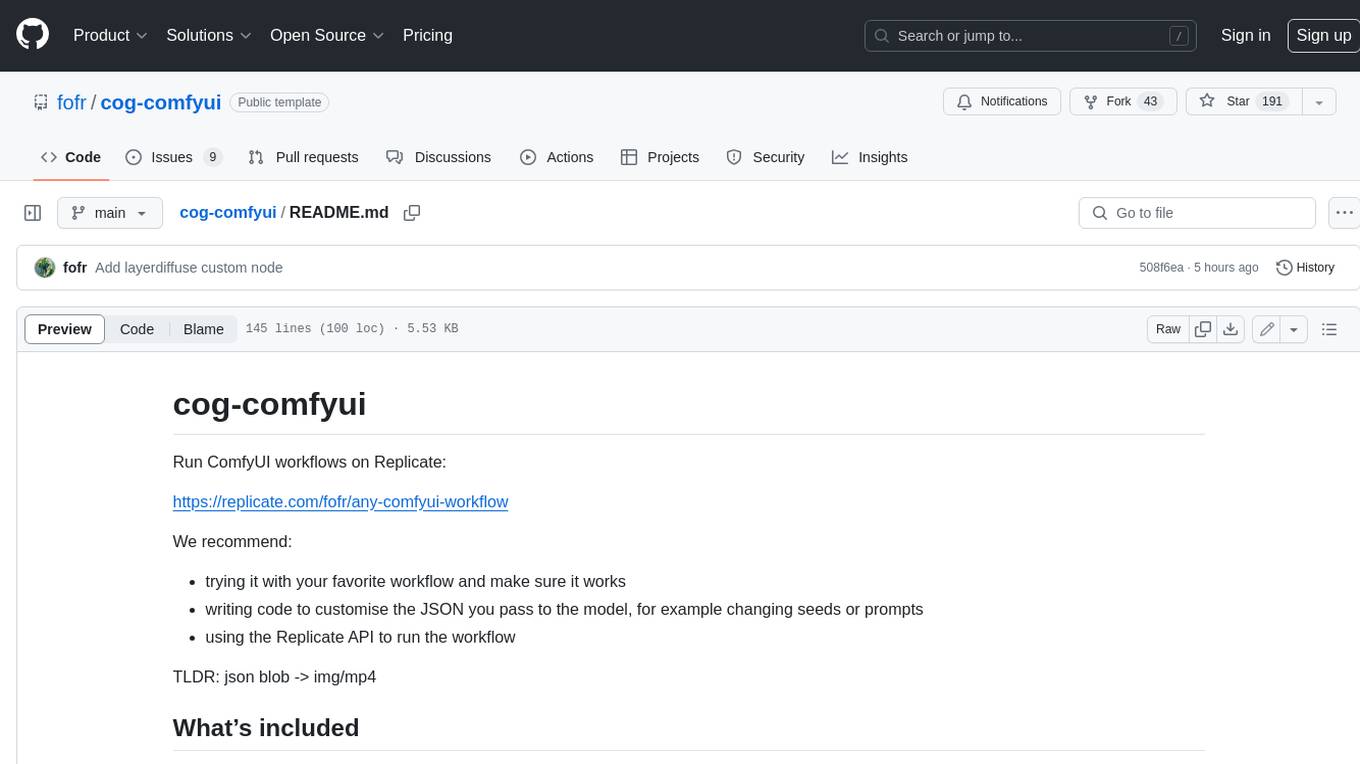
cog-comfyui
Cog-comfyui allows users to run ComfyUI workflows on Replicate. ComfyUI is a visual programming tool for creating and sharing generative art workflows. With cog-comfyui, users can access a variety of pre-trained models and custom nodes to create their own unique artworks. The tool is easy to use and does not require any coding experience. Users simply need to upload their API JSON file and any necessary input files, and then click the "Run" button. Cog-comfyui will then generate the output image or video file.
For similar jobs
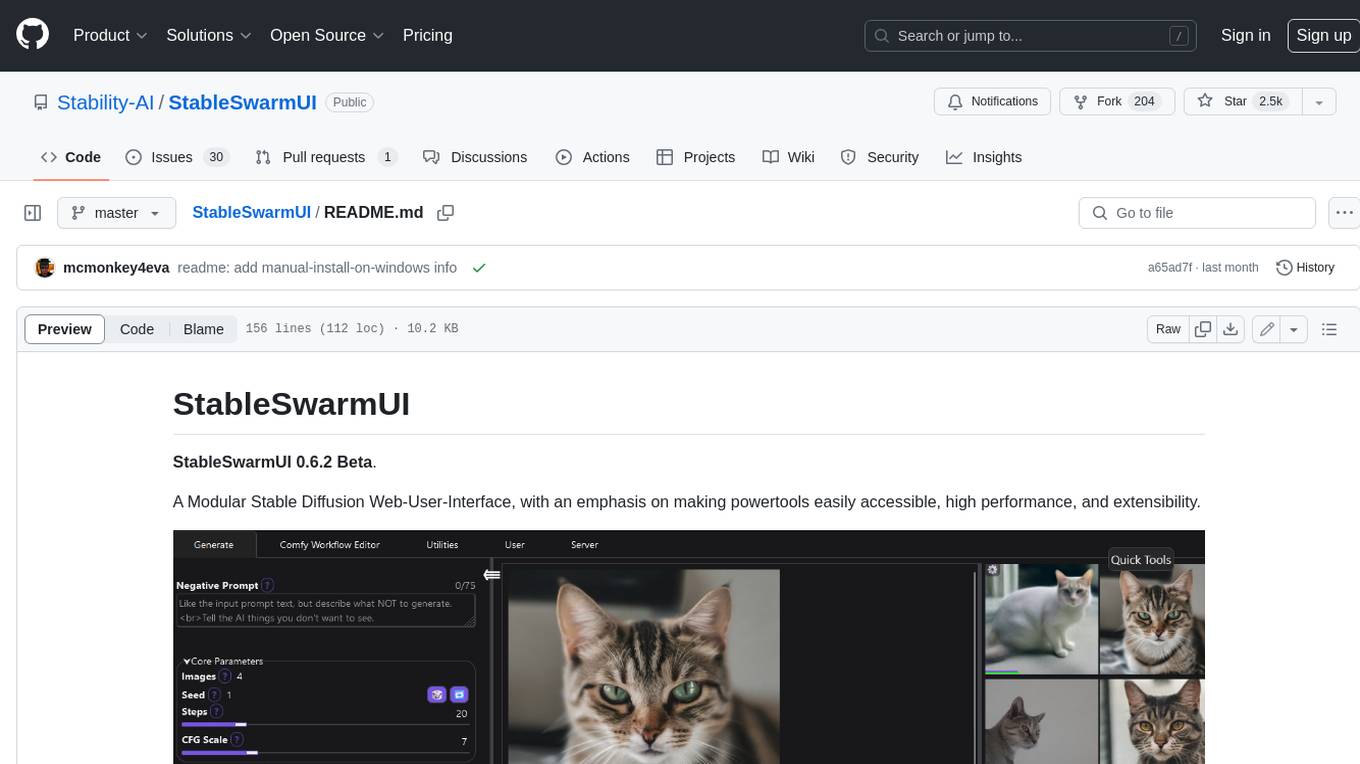
StableSwarmUI
StableSwarmUI is a modular Stable Diffusion web user interface that emphasizes making power tools easily accessible, high performance, and extensible. It is designed to be a one-stop-shop for all things Stable Diffusion, providing a wide range of features and capabilities to enhance the user experience.
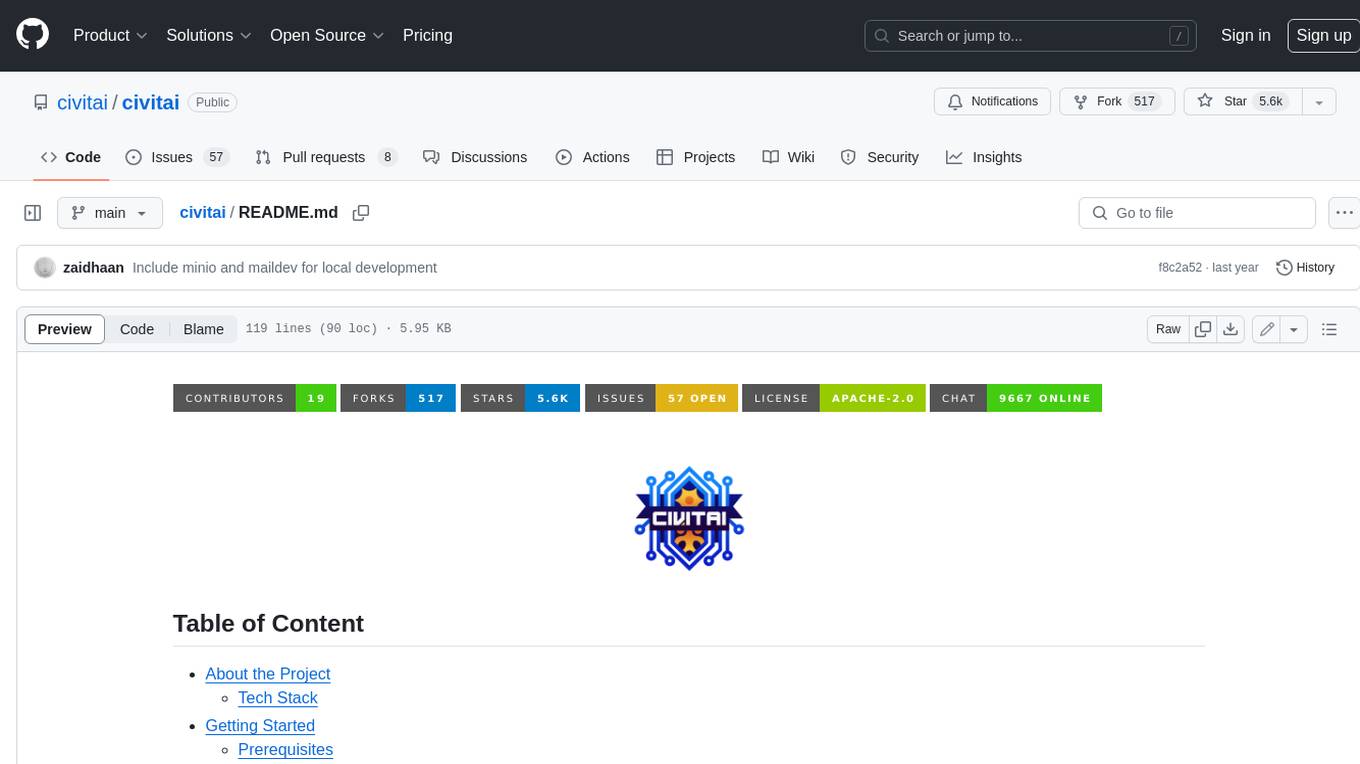
civitai
Civitai is a platform where people can share their stable diffusion models (textual inversions, hypernetworks, aesthetic gradients, VAEs, and any other crazy stuff people do to customize their AI generations), collaborate with others to improve them, and learn from each other's work. The platform allows users to create an account, upload their models, and browse models that have been shared by others. Users can also leave comments and feedback on each other's models to facilitate collaboration and knowledge sharing.
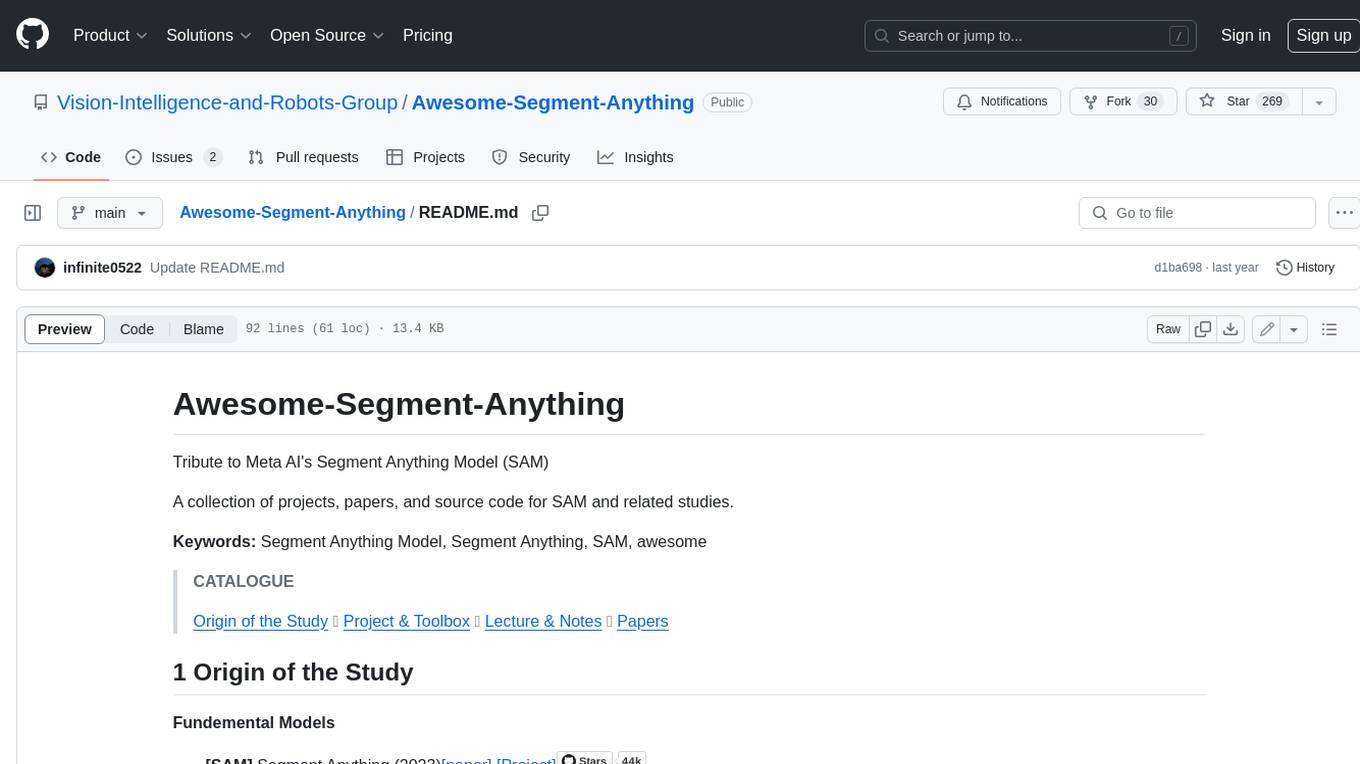
Awesome-Segment-Anything
The Segment Anything Model (SAM) is a powerful tool that allows users to segment any object in an image with just a few clicks. This makes it a great tool for a variety of tasks, such as object detection, tracking, and editing. SAM is also very easy to use, making it a great option for both beginners and experienced users.
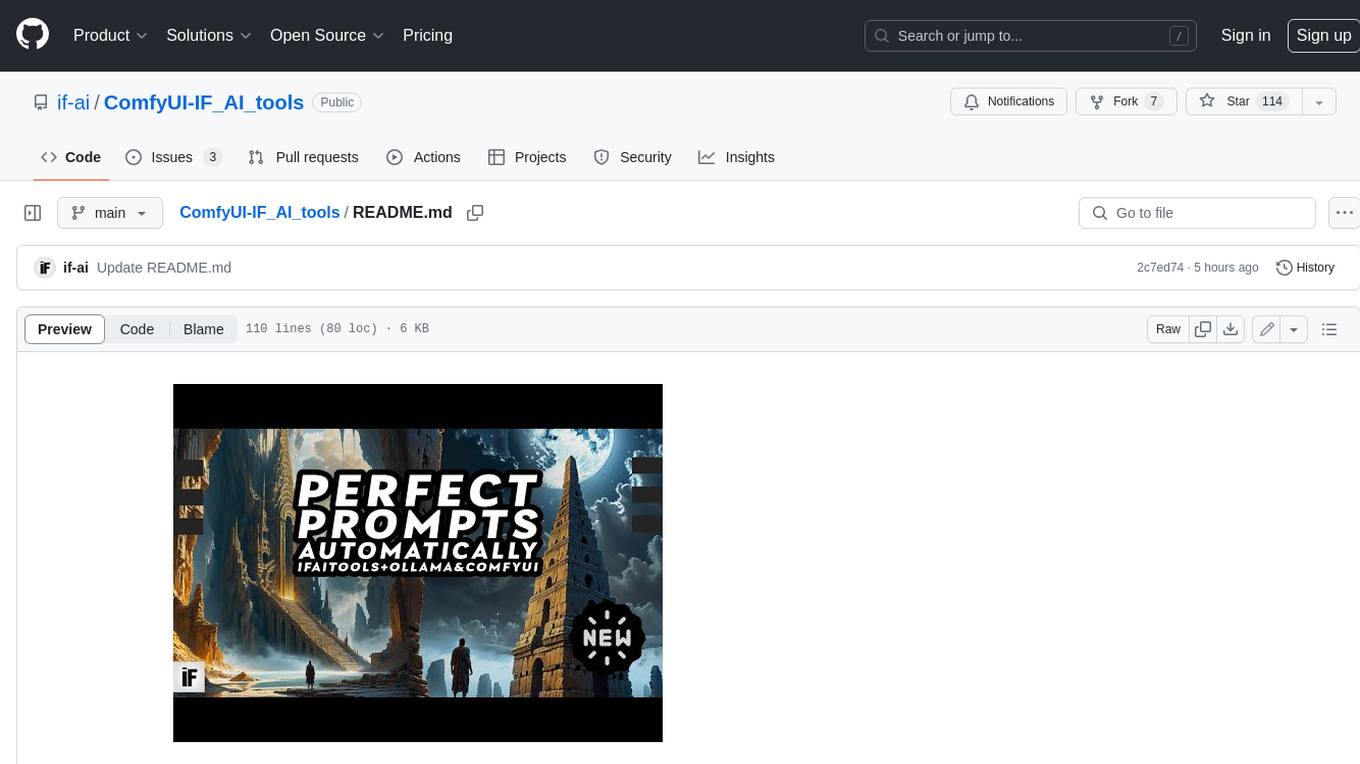
ComfyUI-IF_AI_tools
ComfyUI-IF_AI_tools is a set of custom nodes for ComfyUI that allows you to generate prompts using a local Large Language Model (LLM) via Ollama. This tool enables you to enhance your image generation workflow by leveraging the power of language models.
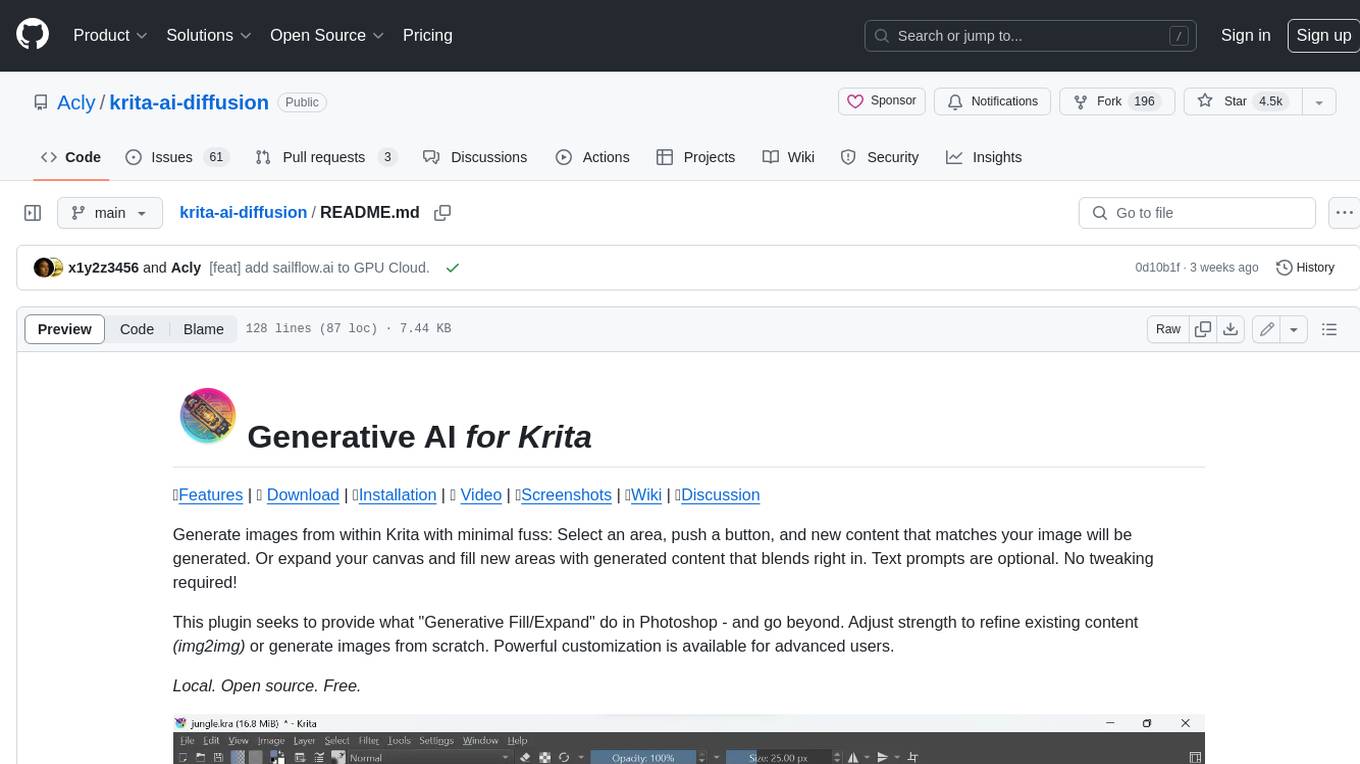
krita-ai-diffusion
Krita-AI-Diffusion is a plugin for Krita that allows users to generate images from within the program. It offers a variety of features, including inpainting, outpainting, generating images from scratch, refining existing content, live painting, and control over image creation. The plugin is designed to fit into an interactive workflow where AI generation is used as just another tool while painting. It is meant to synergize with traditional tools and the layer stack.
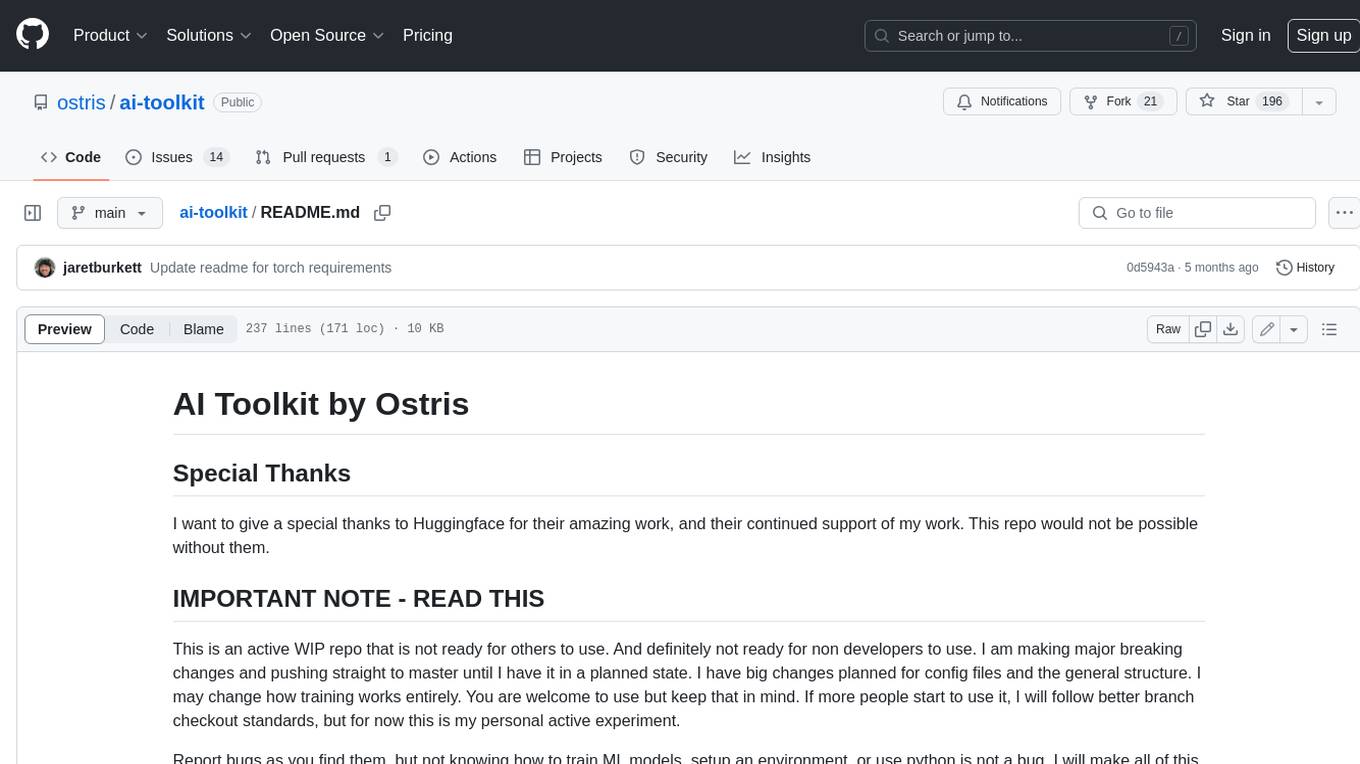
ai-toolkit
The AI Toolkit by Ostris is a collection of tools for machine learning, specifically designed for image generation, LoRA (latent representations of attributes) extraction and manipulation, and model training. It provides a user-friendly interface and extensive documentation to make it accessible to both developers and non-developers. The toolkit is actively under development, with new features and improvements being added regularly. Some of the key features of the AI Toolkit include: - Batch Image Generation: Allows users to generate a batch of images based on prompts or text files, using a configuration file to specify the desired settings. - LoRA (lierla), LoCON (LyCORIS) Extractor: Facilitates the extraction of LoRA and LoCON representations from pre-trained models, enabling users to modify and manipulate these representations for various purposes. - LoRA Rescale: Provides a tool to rescale LoRA weights, allowing users to adjust the influence of specific attributes in the generated images. - LoRA Slider Trainer: Enables the training of LoRA sliders, which can be used to control and adjust specific attributes in the generated images, offering a powerful tool for fine-tuning and customization. - Extensions: Supports the creation and sharing of custom extensions, allowing users to extend the functionality of the toolkit with their own tools and scripts. - VAE (Variational Auto Encoder) Trainer: Facilitates the training of VAEs for image generation, providing users with a tool to explore and improve the quality of generated images. The AI Toolkit is a valuable resource for anyone interested in exploring and utilizing machine learning for image generation and manipulation. Its user-friendly interface, extensive documentation, and active development make it an accessible and powerful tool for both beginners and experienced users.
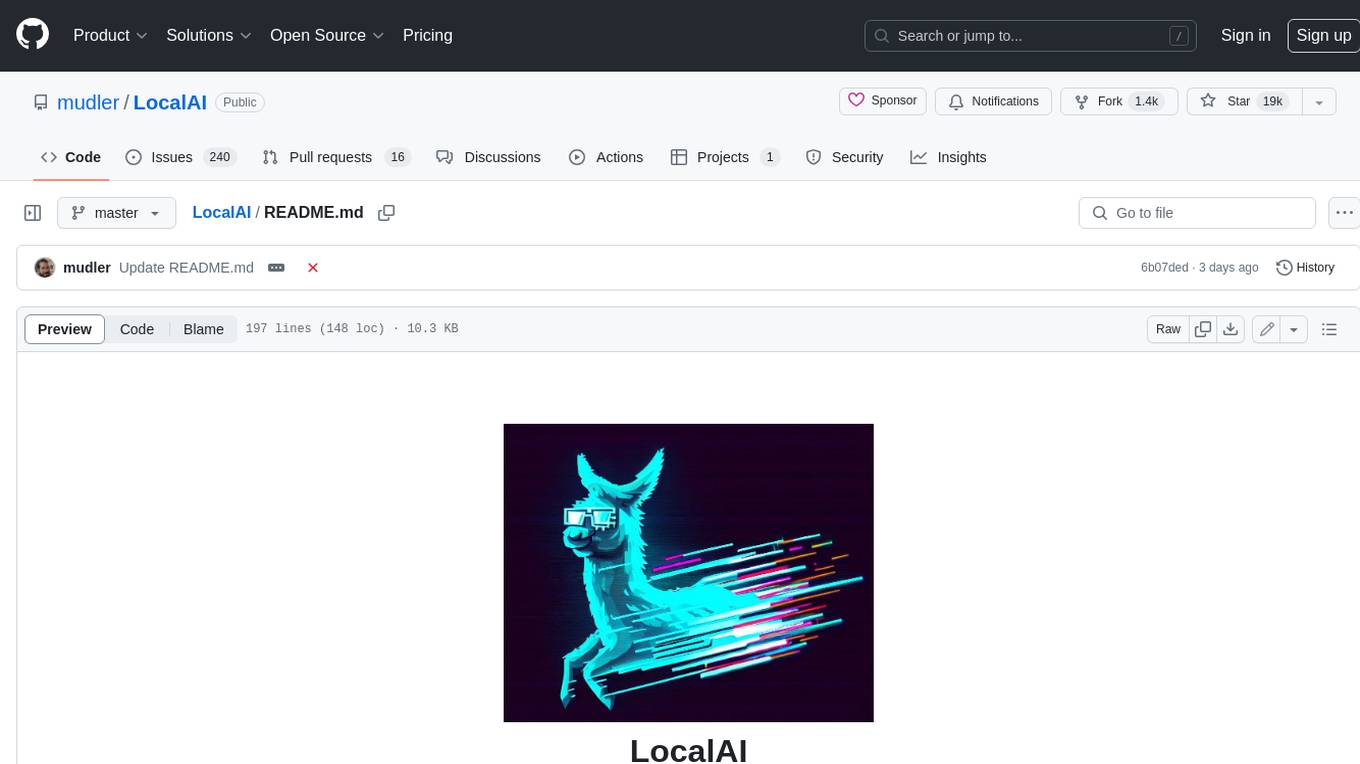
LocalAI
LocalAI is a free and open-source OpenAI alternative that acts as a drop-in replacement REST API compatible with OpenAI (Elevenlabs, Anthropic, etc.) API specifications for local AI inferencing. It allows users to run LLMs, generate images, audio, and more locally or on-premises with consumer-grade hardware, supporting multiple model families and not requiring a GPU. LocalAI offers features such as text generation with GPTs, text-to-audio, audio-to-text transcription, image generation with stable diffusion, OpenAI functions, embeddings generation for vector databases, constrained grammars, downloading models directly from Huggingface, and a Vision API. It provides a detailed step-by-step introduction in its Getting Started guide and supports community integrations such as custom containers, WebUIs, model galleries, and various bots for Discord, Slack, and Telegram. LocalAI also offers resources like an LLM fine-tuning guide, instructions for local building and Kubernetes installation, projects integrating LocalAI, and a how-tos section curated by the community. It encourages users to cite the repository when utilizing it in downstream projects and acknowledges the contributions of various software from the community.
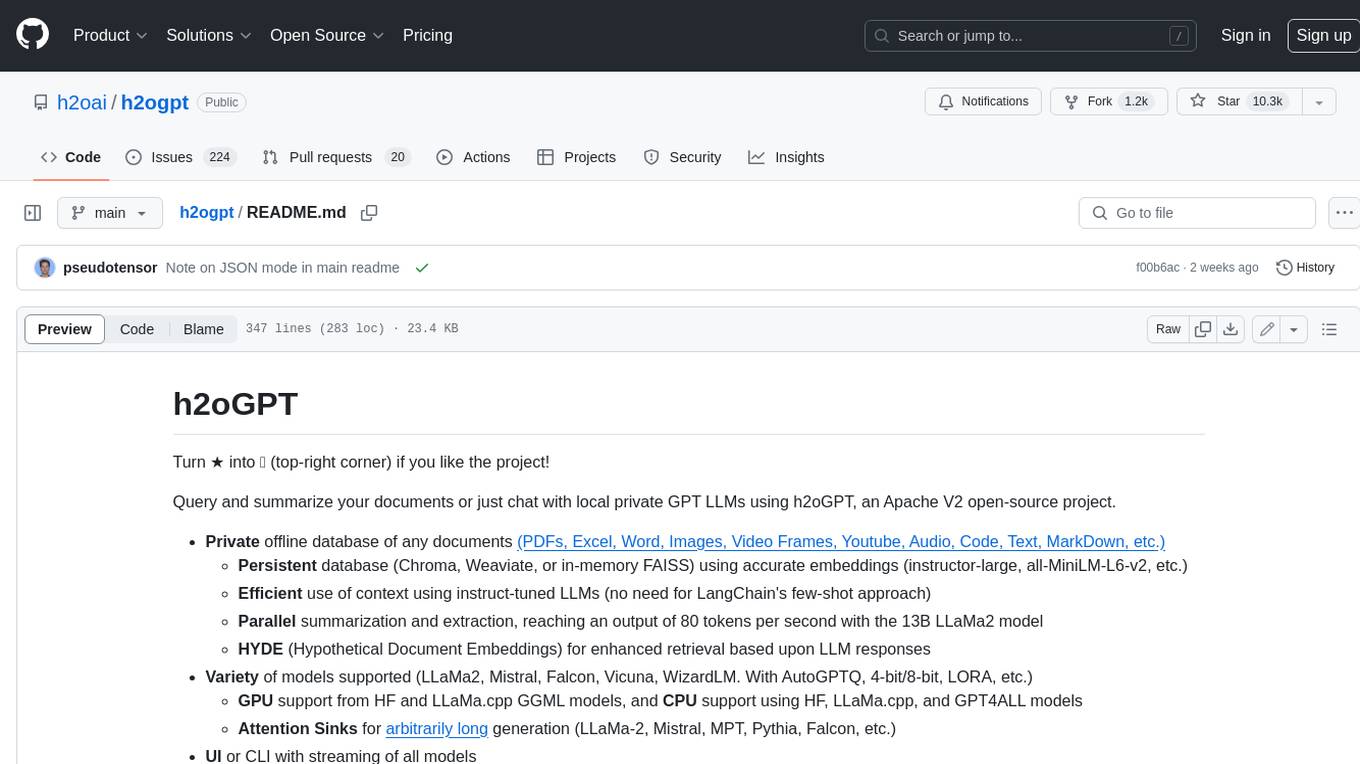
h2ogpt
h2oGPT is an Apache V2 open-source project that allows users to query and summarize documents or chat with local private GPT LLMs. It features a private offline database of any documents (PDFs, Excel, Word, Images, Video Frames, Youtube, Audio, Code, Text, MarkDown, etc.), a persistent database (Chroma, Weaviate, or in-memory FAISS) using accurate embeddings (instructor-large, all-MiniLM-L6-v2, etc.), and efficient use of context using instruct-tuned LLMs (no need for LangChain's few-shot approach). h2oGPT also offers parallel summarization and extraction, reaching an output of 80 tokens per second with the 13B LLaMa2 model, HYDE (Hypothetical Document Embeddings) for enhanced retrieval based upon LLM responses, a variety of models supported (LLaMa2, Mistral, Falcon, Vicuna, WizardLM. With AutoGPTQ, 4-bit/8-bit, LORA, etc.), GPU support from HF and LLaMa.cpp GGML models, and CPU support using HF, LLaMa.cpp, and GPT4ALL models. Additionally, h2oGPT provides Attention Sinks for arbitrarily long generation (LLaMa-2, Mistral, MPT, Pythia, Falcon, etc.), a UI or CLI with streaming of all models, the ability to upload and view documents through the UI (control multiple collaborative or personal collections), Vision Models LLaVa, Claude-3, Gemini-Pro-Vision, GPT-4-Vision, Image Generation Stable Diffusion (sdxl-turbo, sdxl) and PlaygroundAI (playv2), Voice STT using Whisper with streaming audio conversion, Voice TTS using MIT-Licensed Microsoft Speech T5 with multiple voices and Streaming audio conversion, Voice TTS using MPL2-Licensed TTS including Voice Cloning and Streaming audio conversion, AI Assistant Voice Control Mode for hands-free control of h2oGPT chat, Bake-off UI mode against many models at the same time, Easy Download of model artifacts and control over models like LLaMa.cpp through the UI, Authentication in the UI by user/password via Native or Google OAuth, State Preservation in the UI by user/password, Linux, Docker, macOS, and Windows support, Easy Windows Installer for Windows 10 64-bit (CPU/CUDA), Easy macOS Installer for macOS (CPU/M1/M2), Inference Servers support (oLLaMa, HF TGI server, vLLM, Gradio, ExLLaMa, Replicate, OpenAI, Azure OpenAI, Anthropic), OpenAI-compliant, Server Proxy API (h2oGPT acts as drop-in-replacement to OpenAI server), Python client API (to talk to Gradio server), JSON Mode with any model via code block extraction. Also supports MistralAI JSON mode, Claude-3 via function calling with strict Schema, OpenAI via JSON mode, and vLLM via guided_json with strict Schema, Web-Search integration with Chat and Document Q/A, Agents for Search, Document Q/A, Python Code, CSV frames (Experimental, best with OpenAI currently), Evaluate performance using reward models, and Quality maintained with over 1000 unit and integration tests taking over 4 GPU-hours.