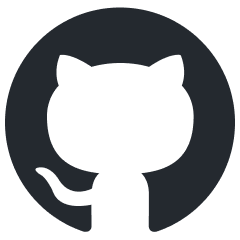
modelscope-agent
ModelScope-Agent: An agent framework connecting models in ModelScope with the world
Stars: 2657
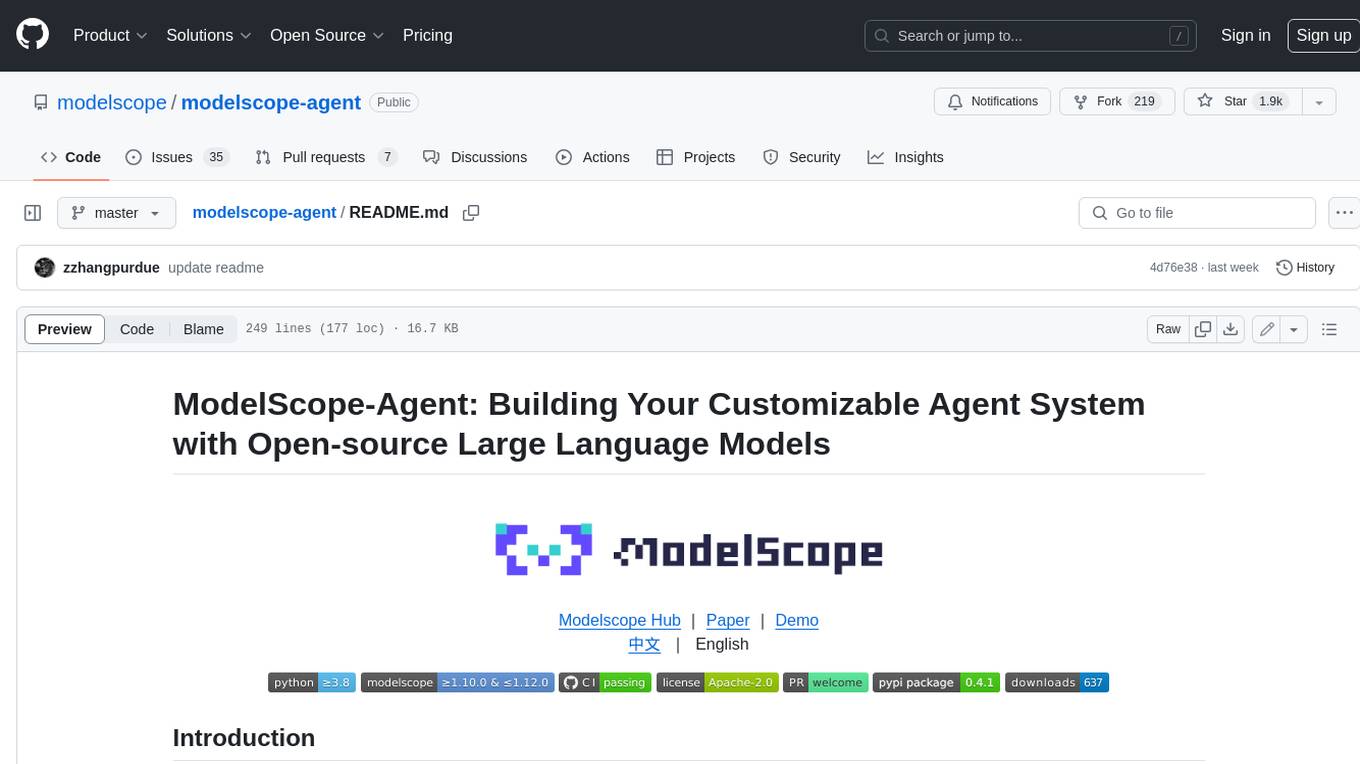
ModelScope-Agent is a customizable and scalable Agent framework. A single agent has abilities such as role-playing, LLM calling, tool usage, planning, and memory. It mainly has the following characteristics: - **Simple Agent Implementation Process**: Simply specify the role instruction, LLM name, and tool name list to implement an Agent application. The framework automatically arranges workflows for tool usage, planning, and memory. - **Rich models and tools**: The framework is equipped with rich LLM interfaces, such as Dashscope and Modelscope model interfaces, OpenAI model interfaces, etc. Built in rich tools, such as **code interpreter**, **weather query**, **text to image**, **web browsing**, etc., make it easy to customize exclusive agents. - **Unified interface and high scalability**: The framework has clear tools and LLM registration mechanism, making it convenient for users to expand more diverse Agent applications. - **Low coupling**: Developers can easily use built-in tools, LLM, memory, and other components without the need to bind higher-level agents.
README:
Modelscope Hub | Paper | Demo
中文  |  English
Modelscope-Agent is a customizable and scalable Agent framework. A single agent has abilities such as role-playing, LLM calling, tool usage, planning, and memory. It mainly has the following characteristics:
- Simple Agent Implementation Process: Simply specify the role instruction, LLM name, and tool name list to implement an Agent application. The framework automatically arranges workflows for tool usage, planning, and memory.
- Rich models and tools: The framework is equipped with rich LLM interfaces, such as Dashscope and Modelscope model interfaces, OpenAI model interfaces, etc. Built in rich tools, such as code interpreter, weather query, text to image, web browsing, etc., make it easy to customize exclusive agents.
- Unified interface and high scalability: The framework has clear tools and LLM registration mechanism, making it convenient for users to expand more diverse Agent applications.
- Low coupling: Developers can easily use built-in tools, LLM, memory, and other components without the need to bind higher-level agents.
- 🔥🔥🔥Aug 8, 2024: A new graph based code generation tool CodexGraph is released by Modelscope-Agent, it has been proved effective and versatile on various code related tasks, please check example.
- 🔥🔥Aug 1, 2024: A high efficient and reliable Data Science Assistant is running on Modelscope-Agent, please find detail in example.
- 🔥July 17, 2024: Parallel tool calling on Modelscope-Agent-Server, please find detail in doc.
- 🔥June 17, 2024: Upgrading RAG flow based on LLama-index, allow user to hybrid search knowledge by different strategies and modalities, please find detail in doc.
- 🔥June 6, 2024: With Modelscope-Agent-Server, Qwen2 could be used by OpenAI SDK with tool calling ability, please find detail in doc.
- 🔥June 4, 2024: Modelscope-Agent supported Mobile-Agent-V2arxiv,based on Android Adb Env, please check in the application.
- 🔥May 17, 2024: Modelscope-Agent supported multi-roles room chat in the gradio.
- May 14, 2024: Modelscope-Agent supported image input in
RolePlay
agents with latest OpenAI modelGPT-4o
. Developers can experience this feature by specifying theimage_url
parameter. - May 10, 2024: Modelscope-Agent launched a user-friendly
Assistant API
, and also provided aTools API
that executes utilities in isolated, secure containers, please find the document - Apr 12, 2024: The Ray version of multi-agent solution is on modelscope-agent, please find the document
- Mar 15, 2024: Modelscope-Agent and the AgentFabric (opensource version for GPTs) is running on the production environment of modelscope studio.
- Feb 10, 2024: In Chinese New year, we upgrade the modelscope agent to version v0.3 to facilitate developers to customize various types of agents more conveniently through coding and make it easier to make multi-agent demos. For more details, you can refer to #267 and #293 .
- Nov 26, 2023: AgentFabric now supports collaborative use in ModelScope's Creation Space, allowing for the sharing of custom applications in the Creation Space. The update also includes the latest GTE text embedding integration.
- Nov 17, 2023: AgentFabric released, which is an interactive framework to facilitate creation of agents tailored to various real-world applications.
- Oct 30, 2023: Facechain Agent released a local version of the Facechain Agent that can be run locally. For detailed usage instructions, please refer to Facechain Agent.
- Oct 25, 2023: Story Agent released a local version of the Story Agent for generating storybook illustrations. It can be run locally. For detailed usage instructions, please refer to Story Agent.
- Sep 20, 2023: ModelScope GPT offers a local version through gradio that can be run locally. You can navigate to the demo/msgpt/ directory and execute
bash run_msgpt.sh
. - Sep 4, 2023: Three demos, demo_qwen, demo_retrieval_agent and demo_register_tool, have been added, along with detailed tutorials provided.
- Sep 2, 2023: The preprint paper associated with this project was published.
- Aug 22, 2023: Support accessing various AI model APIs using ModelScope tokens.
- Aug 7, 2023: The initial version of the modelscope-agent repository was released.
clone repo and install dependency:
git clone https://github.com/modelscope/modelscope-agent.git
cd modelscope-agent && pip install -r requirements.txt
The ModelScope Notebook offers a free-tier that allows ModelScope user to run the FaceChain application with minimum setup, refer to ModelScope Notebook
# Step1: 我的notebook -> PAI-DSW -> GPU环境
# Step2: Download the [demo file](https://github.com/modelscope/modelscope-agent/blob/master/demo/demo_qwen_agent.ipynb) and upload it to the GPU.
# Step3: Execute the demo notebook in order.
The agent incorporates an LLM along with task-specific tools, and uses the LLM to determine which tool or tools to invoke in order to complete the user's tasks.
To start, all you need to do is initialize an RolePlay
object with corresponding tasks
- This sample code uses the qwen-max model, drawing tools and weather forecast tools.
- Using the qwen-max model requires replacing YOUR_DASHSCOPE_API_KEY in the example with your API-KEY for the code to run properly. YOUR_DASHSCOPE_API_KEY can be obtained here. The drawing tool also calls DASHSCOPE API (wanx), so no additional configuration is required.
- When using the weather forecast tool, you need to replace YOUR_AMAP_TOKEN in the example with your AMAP weather API-KEY so that the code can run normally. YOUR_AMAP_TOKEN is available here.
# 配置环境变量;如果您已经提前将api-key提前配置到您的运行环境中,可以省略这个步骤
import os
os.environ['DASHSCOPE_API_KEY']=YOUR_DASHSCOPE_API_KEY
os.environ['AMAP_TOKEN']=YOUR_AMAP_TOKEN
# 选用RolePlay 配置agent
from modelscope_agent.agents.role_play import RolePlay # NOQA
role_template = '你扮演一个天气预报助手,你需要查询相应地区的天气,并调用给你的画图工具绘制一张城市的图。'
llm_config = {'model': 'qwen-max', 'model_server': 'dashscope'}
# input tool name
function_list = ['amap_weather', 'image_gen']
bot = RolePlay(
function_list=function_list, llm=llm_config, instruction=role_template)
response = bot.run('朝阳区天气怎样?')
text = ''
for chunk in response:
text += chunk
Result
- Terminal runs
# 第一次调用llm的输出
Action: amap_weather
Action Input: {"location": "朝阳区"}
# 第二次调用llm的输出
目前,朝阳区的天气状况为阴天,气温为1度。
Action: image_gen
Action Input: {"text": "朝阳区城市风光", "resolution": "1024*1024"}
# 第三次调用llm的输出
目前,朝阳区的天气状况为阴天,气温为1度。同时,我已为你生成了一张朝阳区的城市风光图,如下所示:

An Agent
object consists of the following components:
-
LLM
: A large language model that is responsible to process your inputs and decide calling tools. -
function_list
: A list consists of available tools for agents.
Currently, configuration of Agent
may contain following arguments:
-
llm
: The llm config of this agent- When Dict: set the config of llm as {'model': '', 'api_key': '', 'model_server': ''}
- When BaseChatModel: llm is sent by another agent
-
function_list
: A list of tools- When str: tool names
- When Dict: tool cfg
-
storage_path
: If not specified otherwise, all data will be stored here in KV pairs by memory -
instruction
: the system instruction of this agent -
name
: the name of agent -
description
: the description of agent, which is used for multi_agent -
kwargs
: other potential parameters
Agent
, as a base class, cannot be directly initialized and called. Agent subclasses need to inherit it. They must implement function _run
, which mainly includes three parts: generation of messages/propmt, calling of llm(s), and tool calling based on the results of llm. We provide an implement of these components in RolePlay
for users, and you can also custom your components according to your requirement.
from modelscope_agent import Agent
class YourCustomAgent(Agent):
def _run(self, user_request, **kwargs):
# Custom your workflow
LLM is core module of agent, which ensures the quality of interaction results.
Currently, configuration of `` may contain following arguments:
-
model
: The specific model name will be passed directly to the model service provider. -
model_server
: provider of model services.
BaseChatModel
, as a base class of llm, cannot be directly initialized and called. The subclasses need to inherit it. They must implement function _chat_stream
and _chat_no_stream
, which correspond to streaming output and non-streaming output respectively.
Optionally implement chat_with_functions
and chat_with_raw_prompt
for function calling and text completion.
Currently we provide the implementation of three model service providers: dashscope (for qwen series models), zhipu (for glm series models) and openai (for all openai api format models). You can directly use the models supported by the above service providers, or you can customize your llm.
For more information please refer to docs/modules/llm.md
We provide several multi-domain tools that can be configured and used in the agent.
You can also customize your tools with set the tool's name, description, and parameters based on a predefined pattern by inheriting the base tool. Depending on your needs, call() can be implemented. An example of a custom tool is provided in demo_register_new_tool
You can pass the tool name or configuration you want to use to the agent.
# by tool name
function_list = ['amap_weather', 'image_gen']
bot = RolePlay(function_list=function_list, ...)
# by tool configuration
from langchain.tools import ShellTool
function_list = [{'terminal':ShellTool()}]
bot = RolePlay(function_list=function_list, ...)
# by mixture
function_list = ['amap_weather', {'terminal':ShellTool()}]
bot = RolePlay(function_list=function_list, ...)
-
image_gen
: Wanx Image Generation. DASHSCOPE_API_KEY needs to be configured in the environment variable. -
code_interpreter
: Code Interpreter -
web_browser
: Web Browsing -
amap_weather
: AMAP Weather. AMAP_TOKEN needs to be configured in the environment variable. -
wordart_texture_generation
: Word art texture generation. DASHSCOPE_API_KEY needs to be configured in the environment variable. -
web_search
: Web Searching. [] -
qwen_vl
: Qwen-VL image recognition. DASHSCOPE_API_KEY needs to be configured in the environment variable. -
style_repaint
: Character style redrawn. DASHSCOPE_API_KEY needs to be configured in the environment variable. -
image_enhancement
: Chasing shadow-magnifying glass. DASHSCOPE_API_KEY needs to be configured in the environment variable. -
text-address
: Geocoding. MODELSCOPE_API_TOKEN needs to be configured in the environment variable. -
speech-generation
: Speech generation. MODELSCOPE_API_TOKEN needs to be configured in the environment variable. -
video-generation
: Video generation. MODELSCOPE_API_TOKEN needs to be configured in the environment variable.
Please refer the multi-agent readme.
If you would like to learn more about the practical details of Agent, you can refer to our articles and video tutorials:
We appreciate your enthusiasm in participating in our open-source ModelScope-Agent project. If you encounter any issues, please feel free to report them to us. If you have built a new Agent demo and are ready to share your work with us, please create a pull request at any time! If you need any further assistance, please contact us via email at [email protected] or communication group!
Facechain is an open-source project for generating personalized portraits in various styles using facial images uploaded by users. By integrating the capabilities of Facechain into the modelscope-agent framework, we have greatly simplified the usage process. The generation of personalized portraits can now be done through dialogue with the Facechain Agent.
FaceChainAgent Studio Application Link: https://modelscope.cn/studios/CVstudio/facechain_agent_studio/summary
You can run it directly in a notebook/Colab/local environment: https://www.modelscope.cn/my/mynotebook
! git clone -b feat/facechain_agent https://github.com/modelscope/modelscope-agent.git
! cd modelscope-agent && ! pip install -r requirements.txt
! cd modelscope-agent/demo/facechain_agent/demo/facechain_agent && ! pip install -r requirements.txt
! pip install http://dashscope-cn-beijing.oss-cn-beijing.aliyuncs.com/zhicheng/modelscope_agent-0.1.0-py3-none-any.whl
! PYTHONPATH=/mnt/workspace/modelscope-agent/demo/facechain_agent && cd modelscope-agent/demo/facechain_agent/demo/facechain_agent && python app_v1.0.py
This project is licensed under the Apache License (Version 2.0).
For Tasks:
Click tags to check more tools for each tasksFor Jobs:
Alternative AI tools for modelscope-agent
Similar Open Source Tools
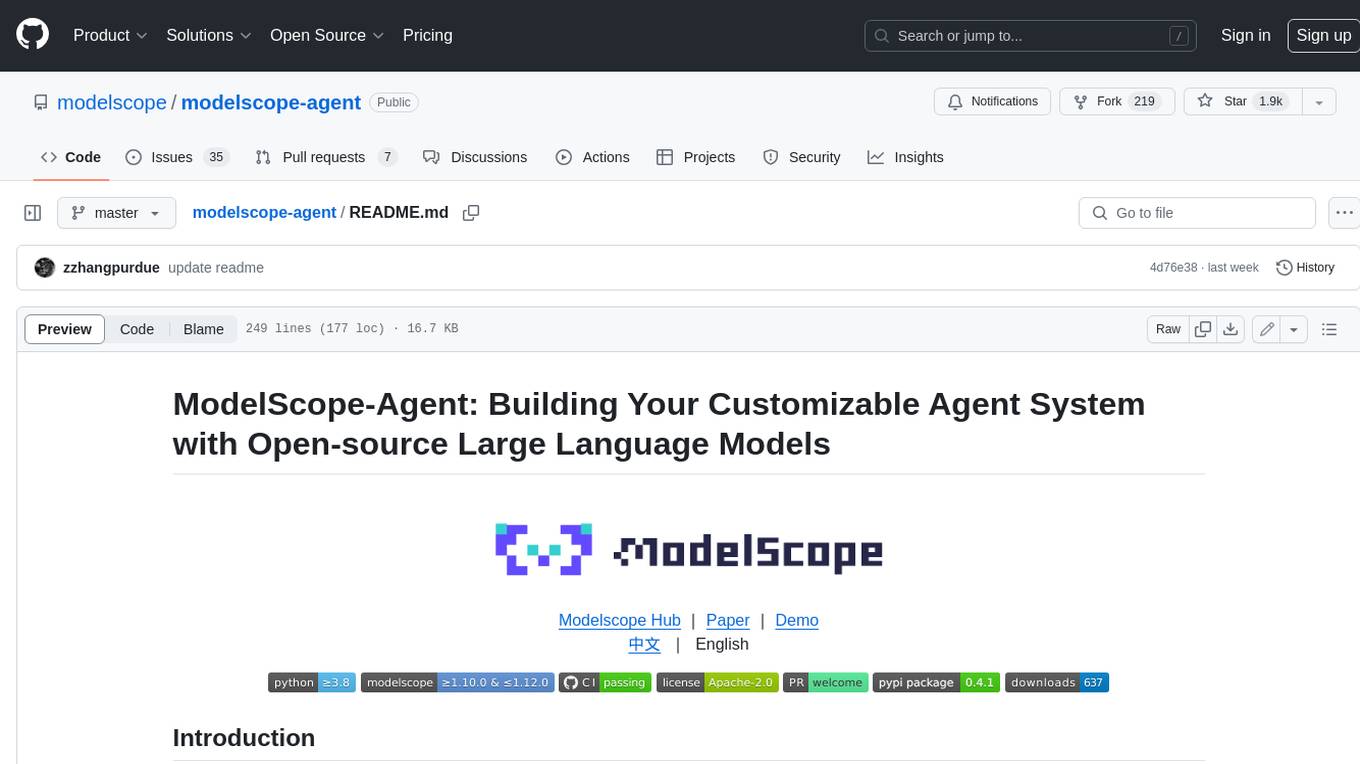
modelscope-agent
ModelScope-Agent is a customizable and scalable Agent framework. A single agent has abilities such as role-playing, LLM calling, tool usage, planning, and memory. It mainly has the following characteristics: - **Simple Agent Implementation Process**: Simply specify the role instruction, LLM name, and tool name list to implement an Agent application. The framework automatically arranges workflows for tool usage, planning, and memory. - **Rich models and tools**: The framework is equipped with rich LLM interfaces, such as Dashscope and Modelscope model interfaces, OpenAI model interfaces, etc. Built in rich tools, such as **code interpreter**, **weather query**, **text to image**, **web browsing**, etc., make it easy to customize exclusive agents. - **Unified interface and high scalability**: The framework has clear tools and LLM registration mechanism, making it convenient for users to expand more diverse Agent applications. - **Low coupling**: Developers can easily use built-in tools, LLM, memory, and other components without the need to bind higher-level agents.
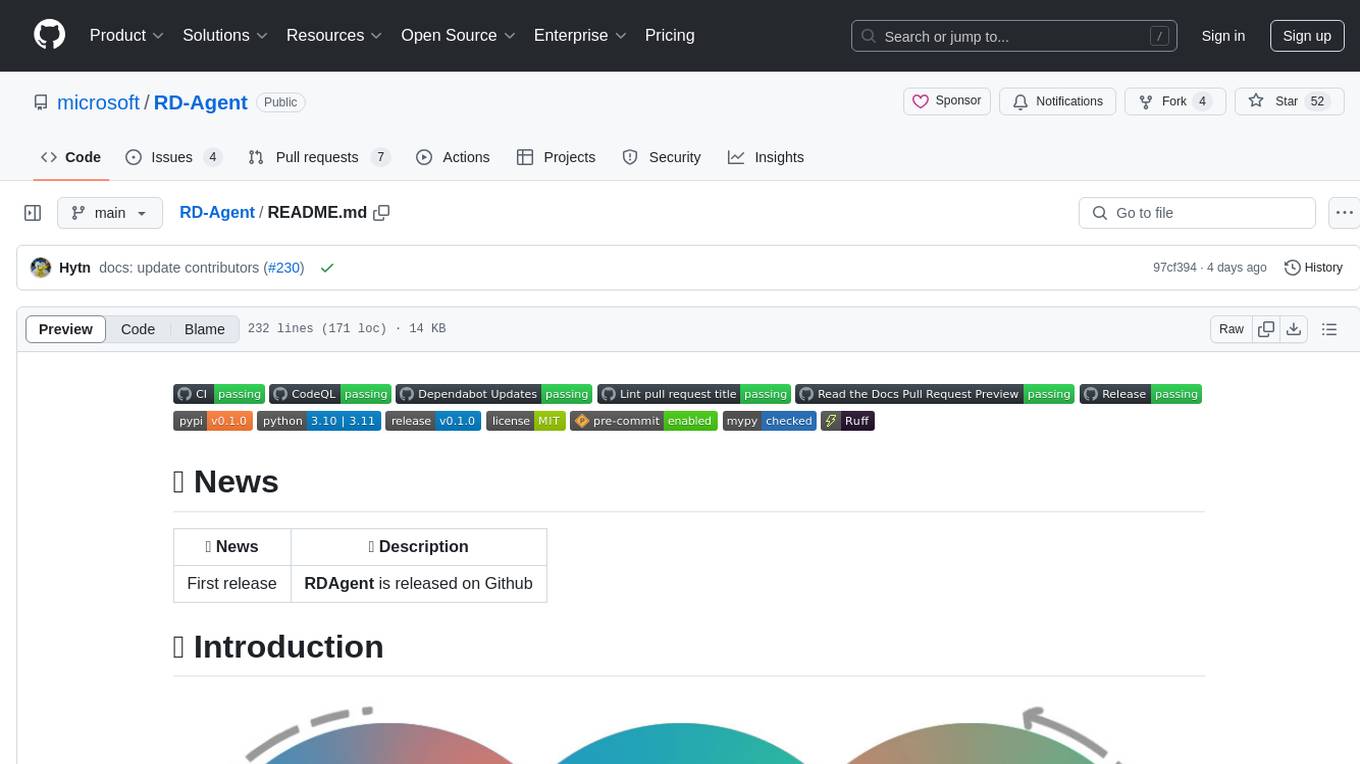
RD-Agent
RD-Agent is a tool designed to automate critical aspects of industrial R&D processes, focusing on data-driven scenarios to streamline model and data development. It aims to propose new ideas ('R') and implement them ('D') automatically, leading to solutions of significant industrial value. The tool supports scenarios like Automated Quantitative Trading, Data Mining Agent, Research Copilot, and more, with a framework to push the boundaries of research in data science. Users can create a Conda environment, install the RDAgent package from PyPI, configure GPT model, and run various applications for tasks like quantitative trading, model evolution, medical prediction, and more. The tool is intended to enhance R&D processes and boost productivity in industrial settings.
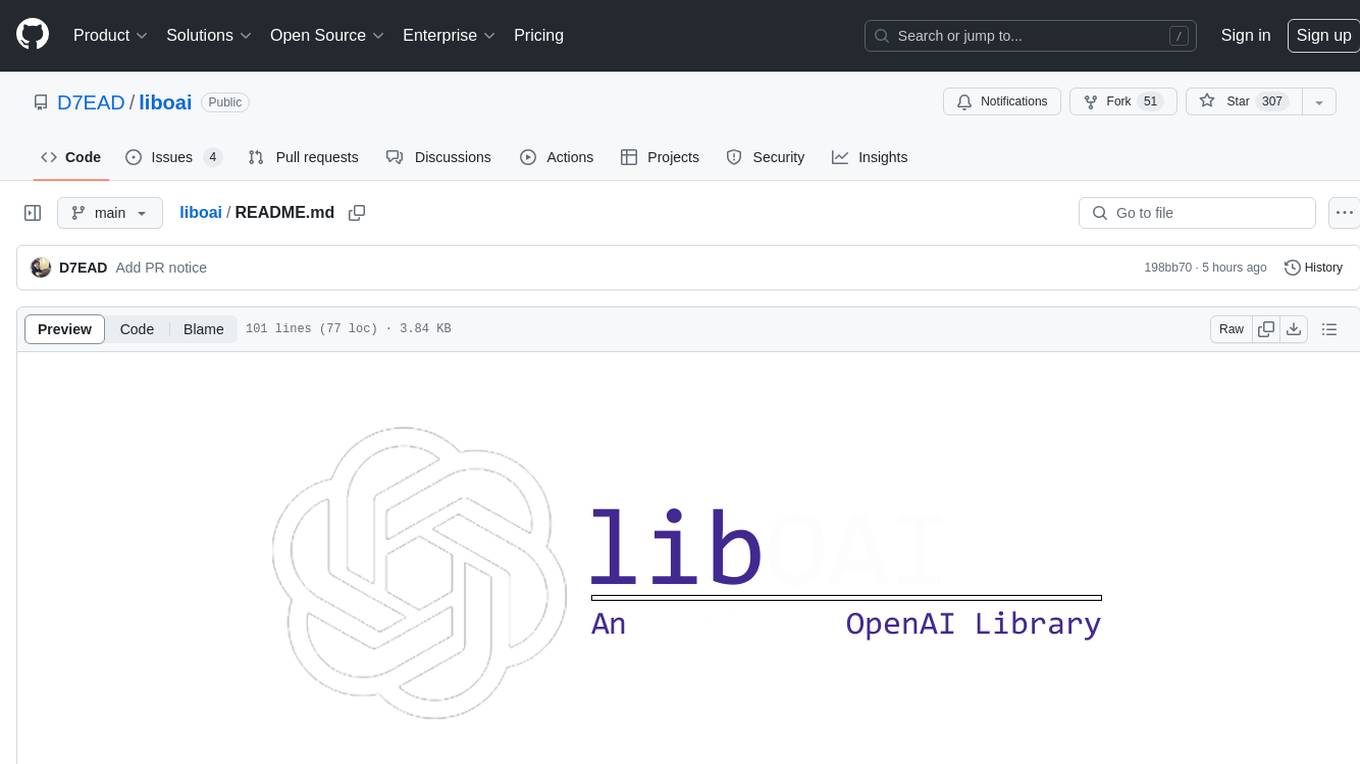
liboai
liboai is a simple C++17 library for the OpenAI API, providing developers with access to OpenAI endpoints through a collection of methods and classes. It serves as a spiritual port of OpenAI's Python library, 'openai', with similar structure and features. The library supports various functionalities such as ChatGPT, Audio, Azure, Functions, Image DALL·E, Models, Completions, Edit, Embeddings, Files, Fine-tunes, Moderation, and Asynchronous Support. Users can easily integrate the library into their C++ projects to interact with OpenAI services.
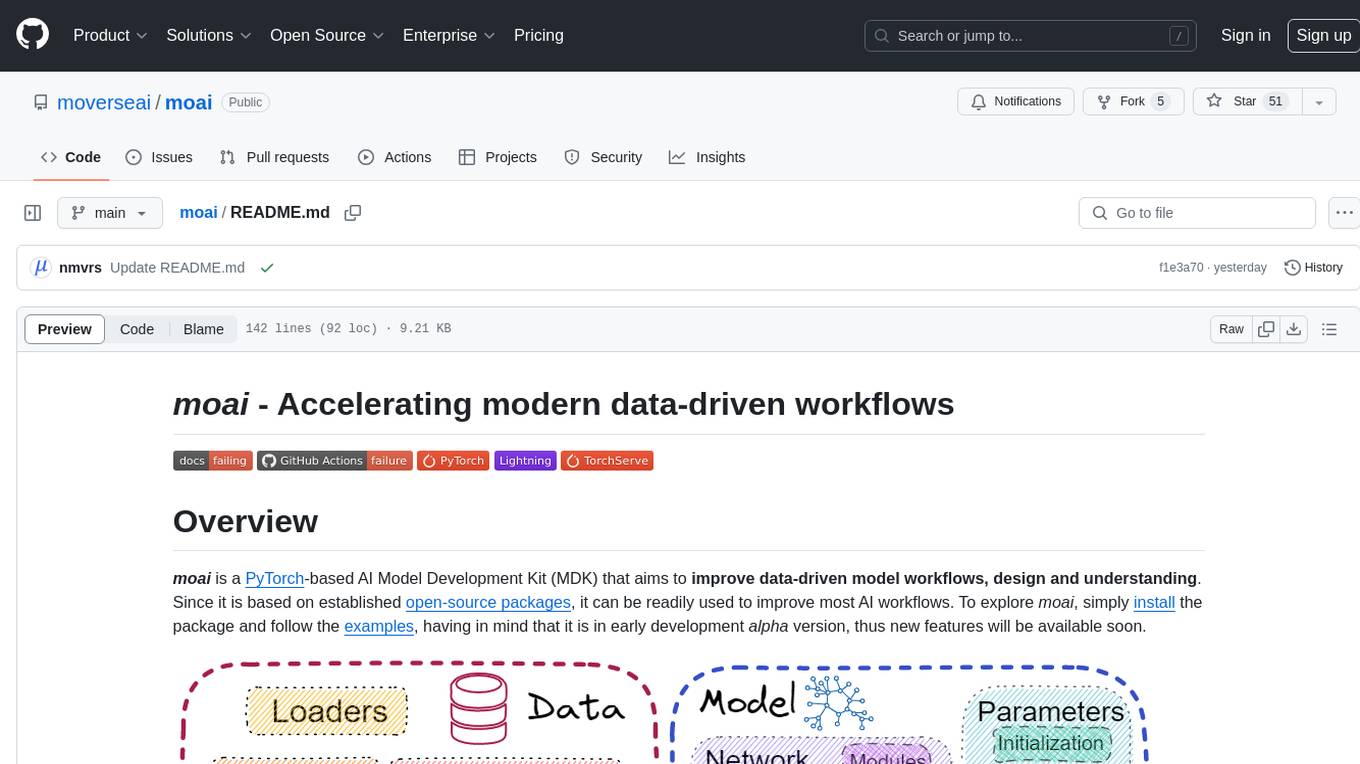
moai
moai is a PyTorch-based AI Model Development Kit (MDK) designed to improve data-driven model workflows, design, and understanding. It offers modularity via monads for model building blocks, reproducibility via configuration-based design, productivity via a data-driven domain modelling language (DML), extensibility via plugins, and understanding via inter-model performance and design aggregation. The tool provides specific integrated actions like play, train, evaluate, plot, diff, and reprod to support heavy data-driven workflows with analytics, knowledge extraction, and reproduction. moai relies on PyTorch, Lightning, Hydra, TorchServe, ONNX, Visdom, HiPlot, Kornia, Albumentations, and the wider open-source community for its functionalities.
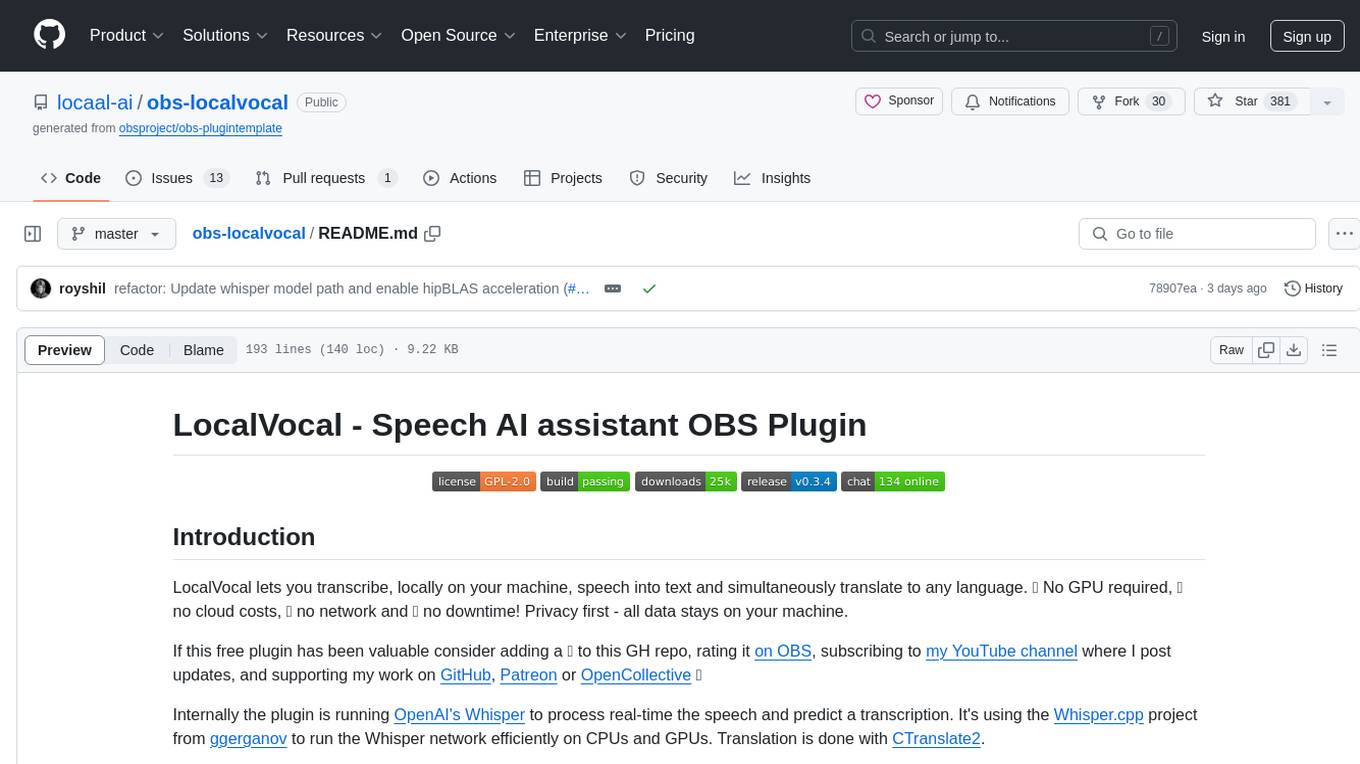
obs-localvocal
LocalVocal is a Speech AI assistant OBS Plugin that enables users to transcribe speech into text and translate it into any language locally on their machine. The plugin runs OpenAI's Whisper for real-time speech processing and prediction. It supports features like transcribing audio in real-time, displaying captions on screen, sending captions to files, syncing captions with recordings, and translating captions to major languages. Users can bring their own Whisper model, filter or replace captions, and experience partial transcriptions for streaming. The plugin is privacy-focused, requiring no GPU, cloud costs, network, or downtime.
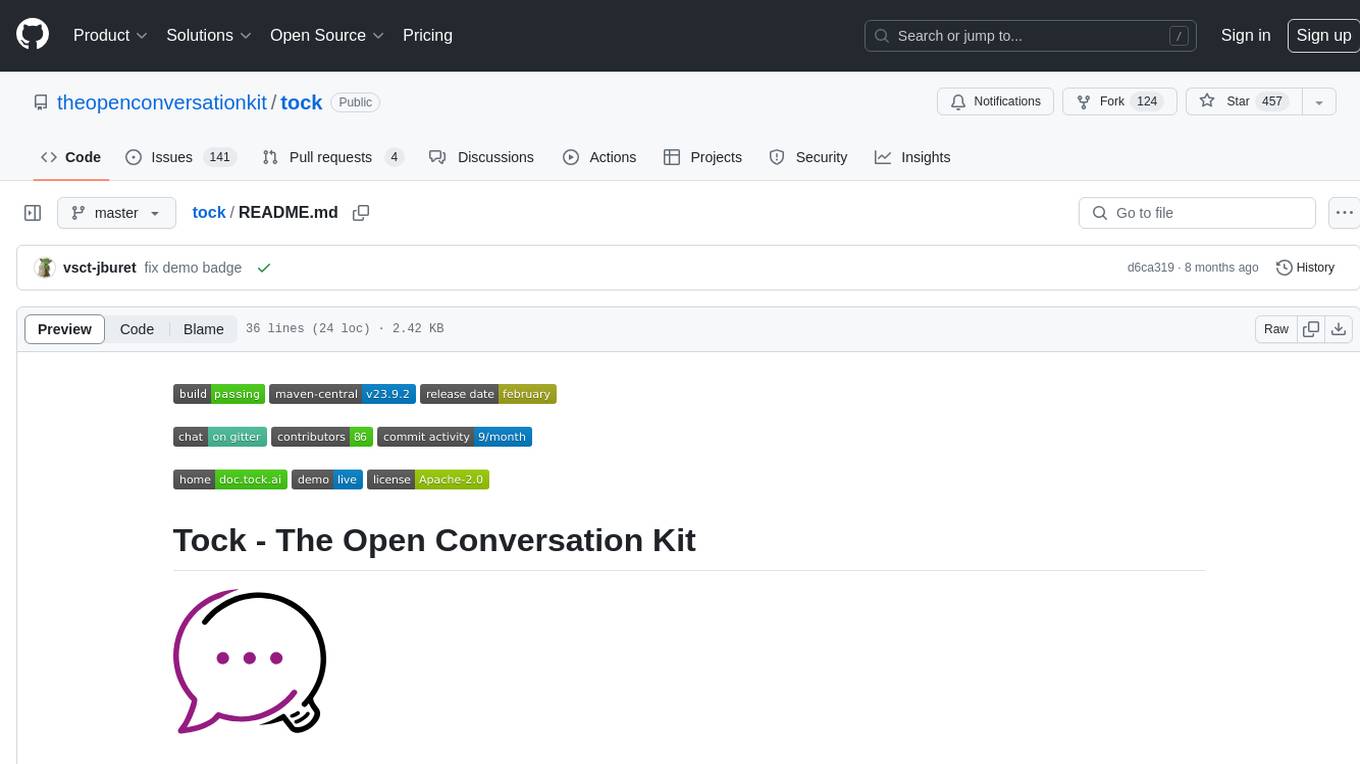
tock
Tock is an open conversational AI platform for building bots. It offers a natural language processing open source stack compatible with various tools, a user interface for building stories and analytics, a conversational DSL for different programming languages, built-in connectors for text/voice channels, toolkits for custom web/mobile integration, and the ability to deploy anywhere in the cloud or on-premise with Docker.
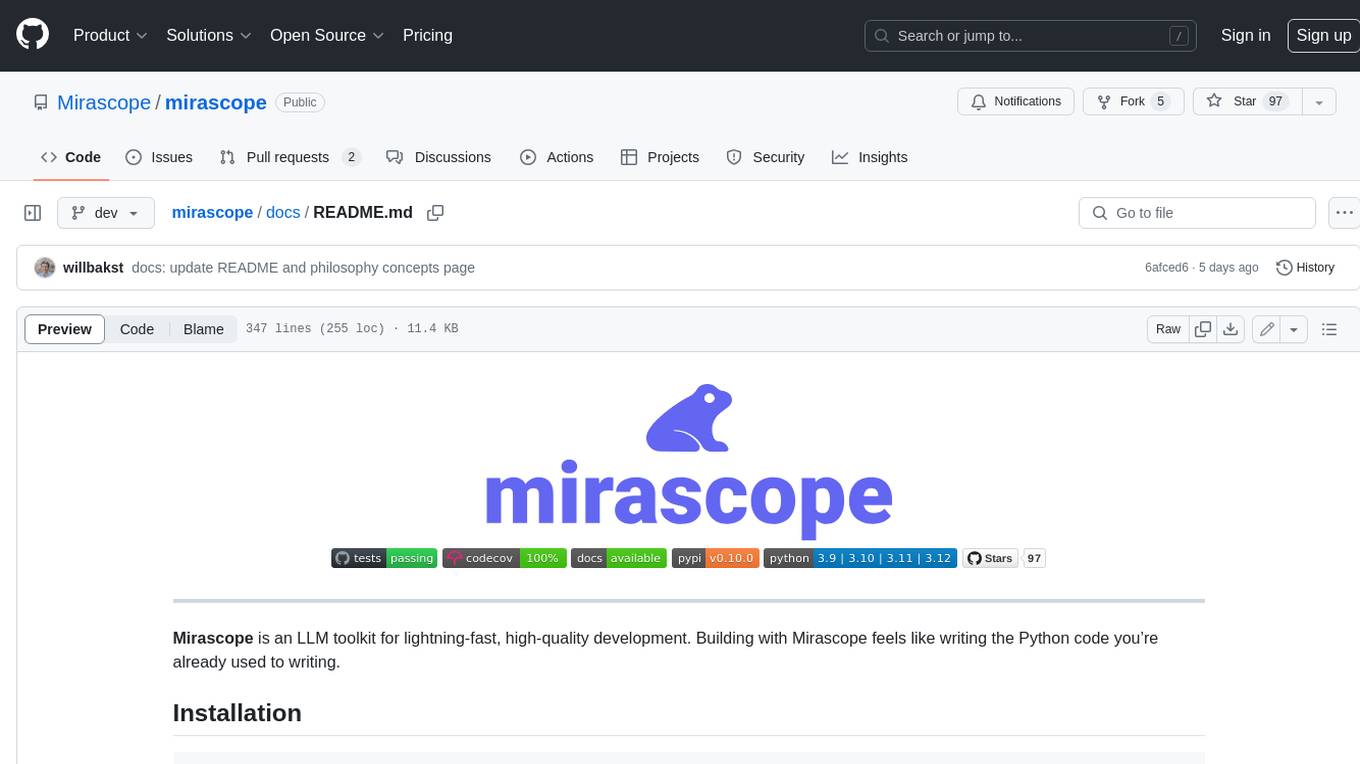
mirascope
Mirascope is an LLM toolkit for lightning-fast, high-quality development. Building with Mirascope feels like writing the Python code you’re already used to writing.
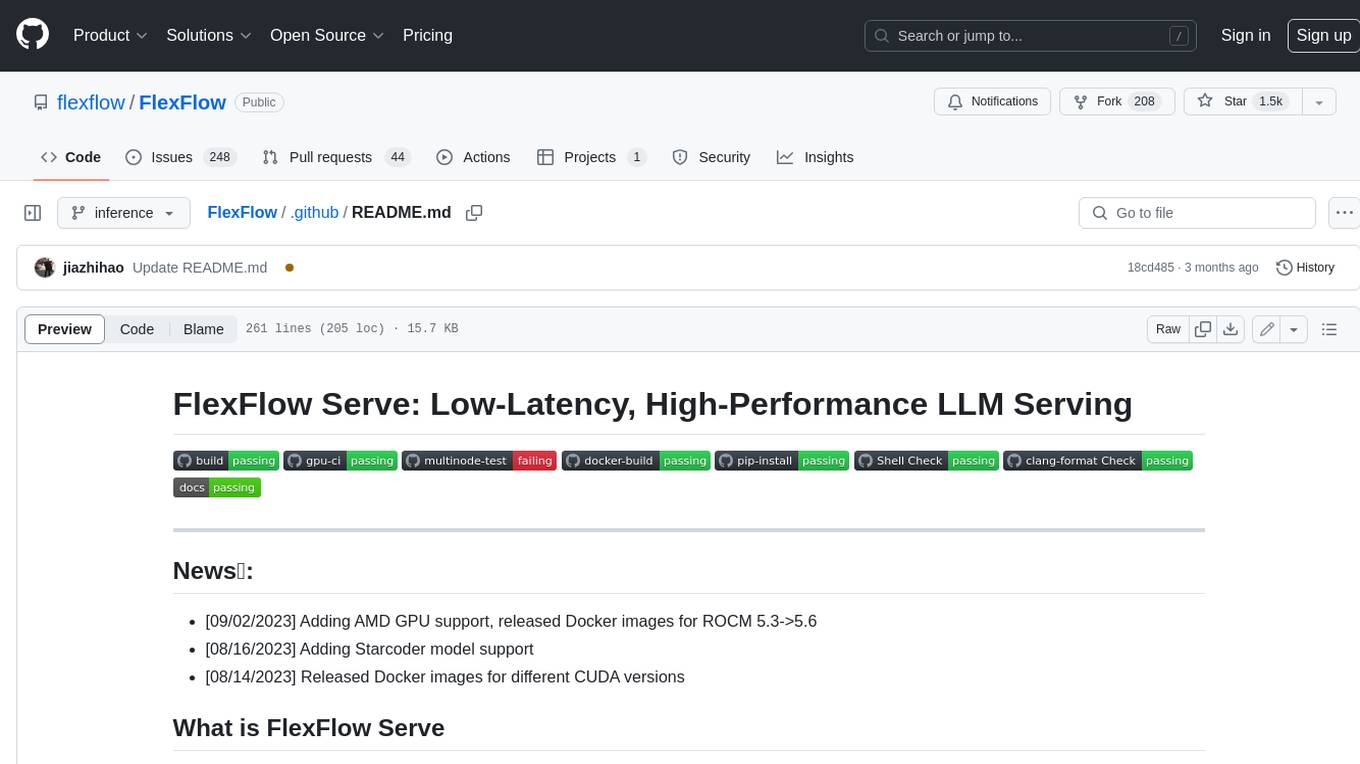
FlexFlow
FlexFlow Serve is an open-source compiler and distributed system for **low latency**, **high performance** LLM serving. FlexFlow Serve outperforms existing systems by 1.3-2.0x for single-node, multi-GPU inference and by 1.4-2.4x for multi-node, multi-GPU inference.
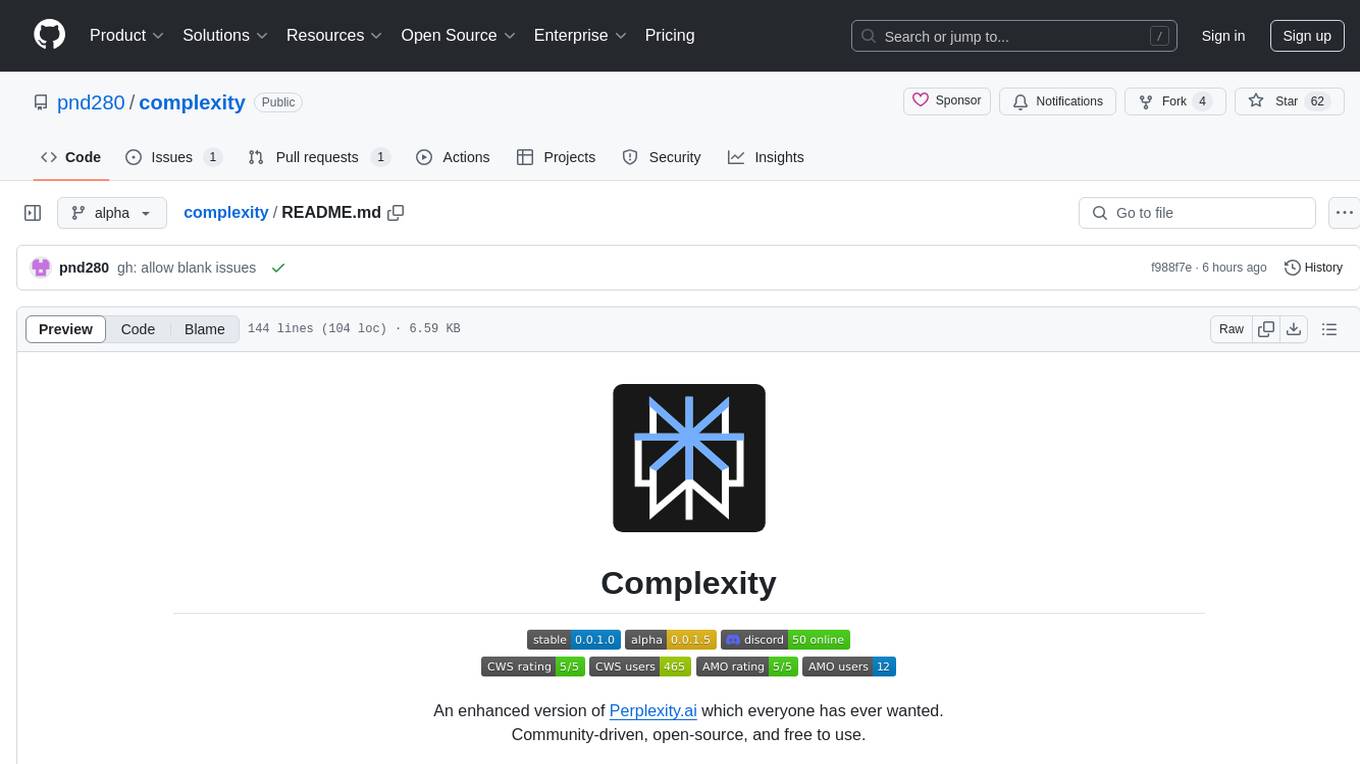
complexity
Complexity is a community-driven, open-source, and free third-party extension that enhances the features of Perplexity.ai. It provides various UI/UX/QoL tweaks, LLM/Image gen model selectors, a customizable theme, and a prompts library. The tool intercepts network traffic to alter the behavior of the host page, offering a solution to the limitations of Perplexity.ai. Users can install Complexity from Chrome Web Store, Mozilla Add-on, or build it from the source code.
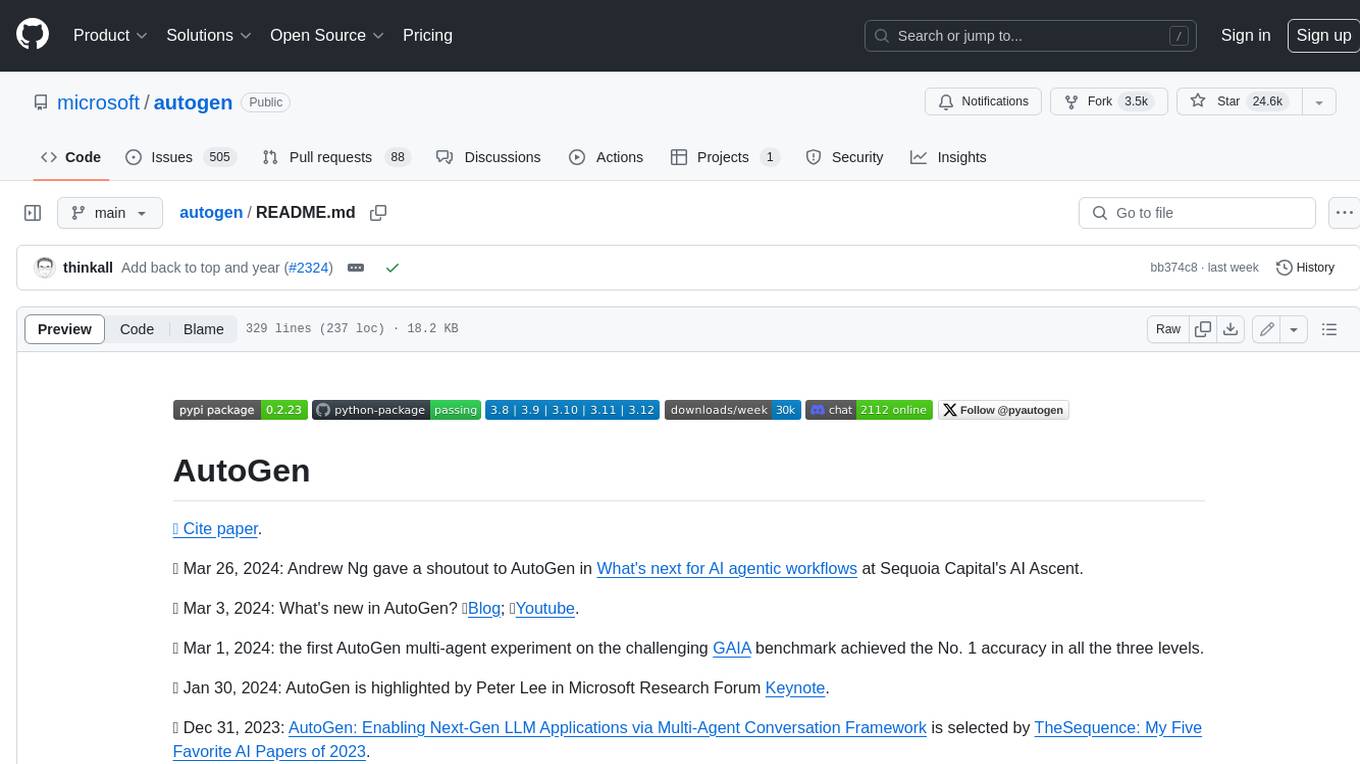
autogen
AutoGen is a framework that enables the development of LLM applications using multiple agents that can converse with each other to solve tasks. AutoGen agents are customizable, conversable, and seamlessly allow human participation. They can operate in various modes that employ combinations of LLMs, human inputs, and tools.
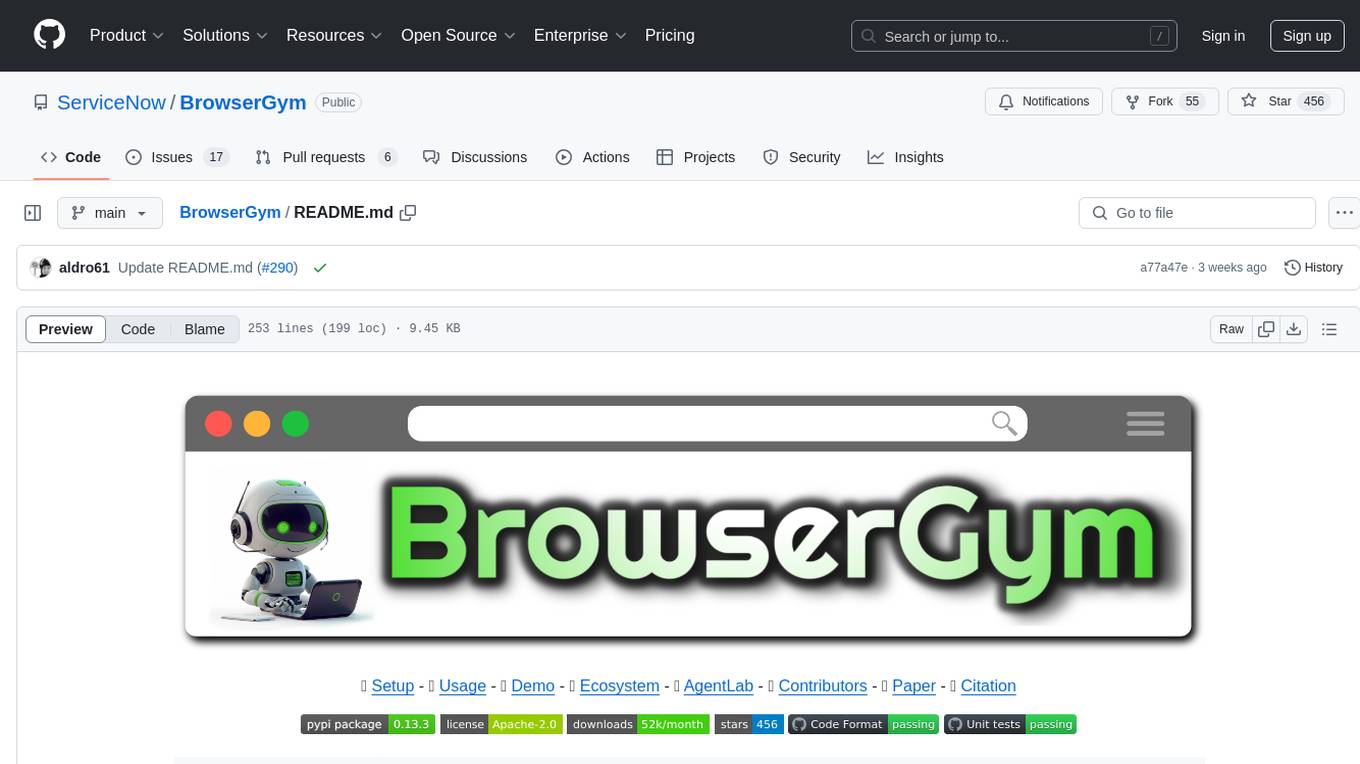
BrowserGym
BrowserGym is an open, easy-to-use, and extensible framework designed to accelerate web agent research. It provides benchmarks like MiniWoB, WebArena, VisualWebArena, WorkArena, AssistantBench, and WebLINX. Users can design new web benchmarks by inheriting the AbstractBrowserTask class. The tool allows users to install different packages for core functionalities, experiments, and specific benchmarks. It supports the development setup and offers boilerplate code for running agents on various tasks. BrowserGym is not a consumer product and should be used with caution.
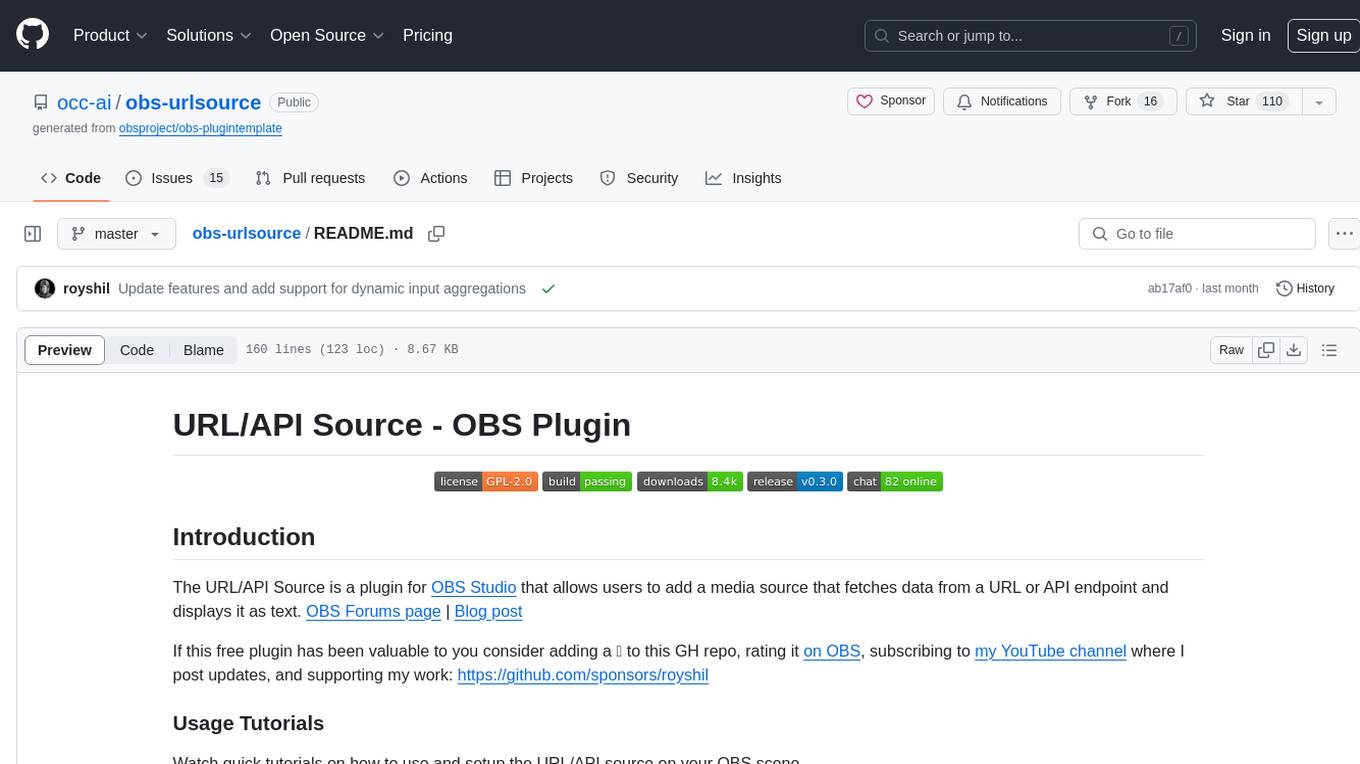
obs-urlsource
The URL/API Source is a plugin for OBS Studio that allows users to add a media source fetching data from a URL or API endpoint and displaying it as text. It supports input and output templating, various request types, output parsing (JSON, XML/HTML, Regex, CSS selectors), live data updating, output styling, and formatting. Future features include authentication, websocket support, more parsing options, request types, and output formats. The plugin is cross-platform compatible and actively maintained by the developer. Users can support the project on GitHub.
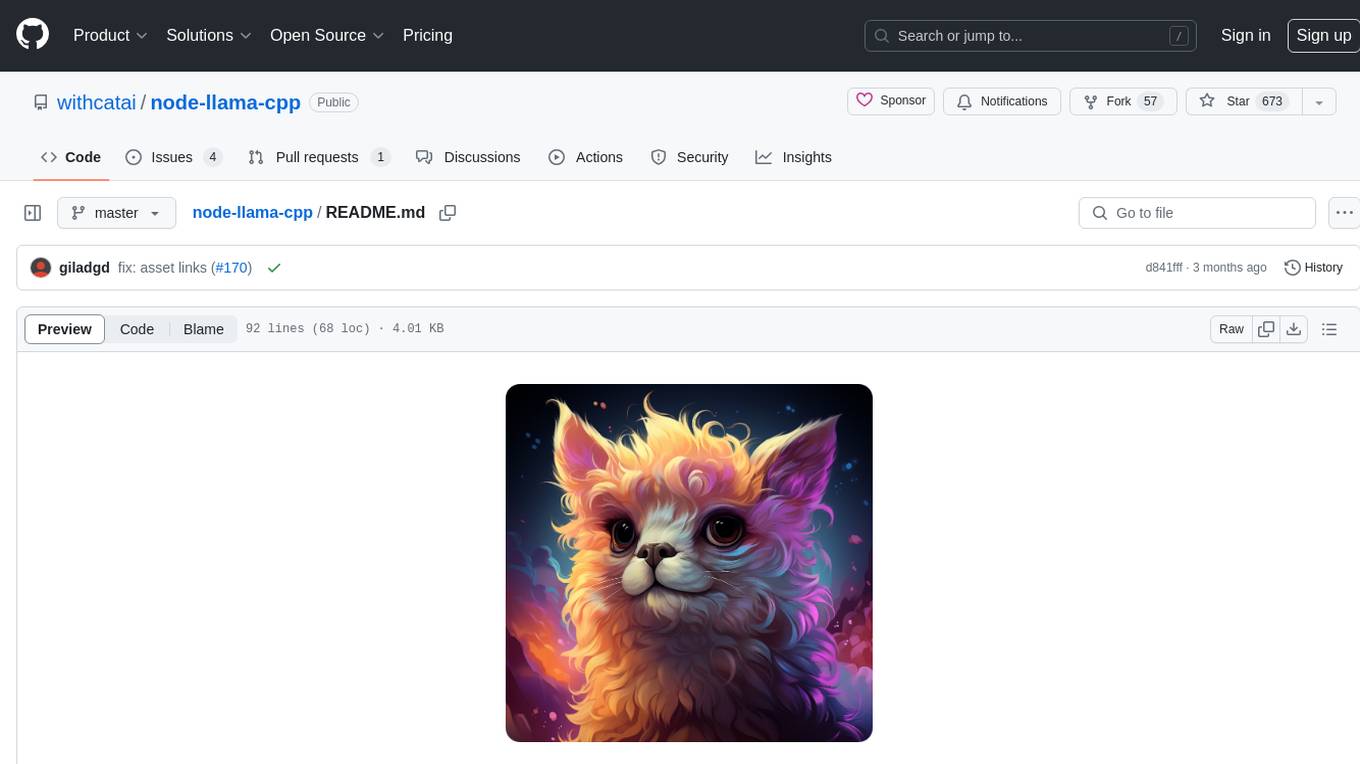
node-llama-cpp
node-llama-cpp is a tool that allows users to run AI models locally on their machines. It provides pre-built bindings with the option to build from source using cmake. Users can interact with text generation models, chat with models using a chat wrapper, and force models to generate output in a parseable format like JSON. The tool supports Metal and CUDA, offers CLI functionality for chatting with models without coding, and ensures up-to-date compatibility with the latest version of llama.cpp. Installation includes pre-built binaries for macOS, Linux, and Windows, with the option to build from source if binaries are not available for the platform.
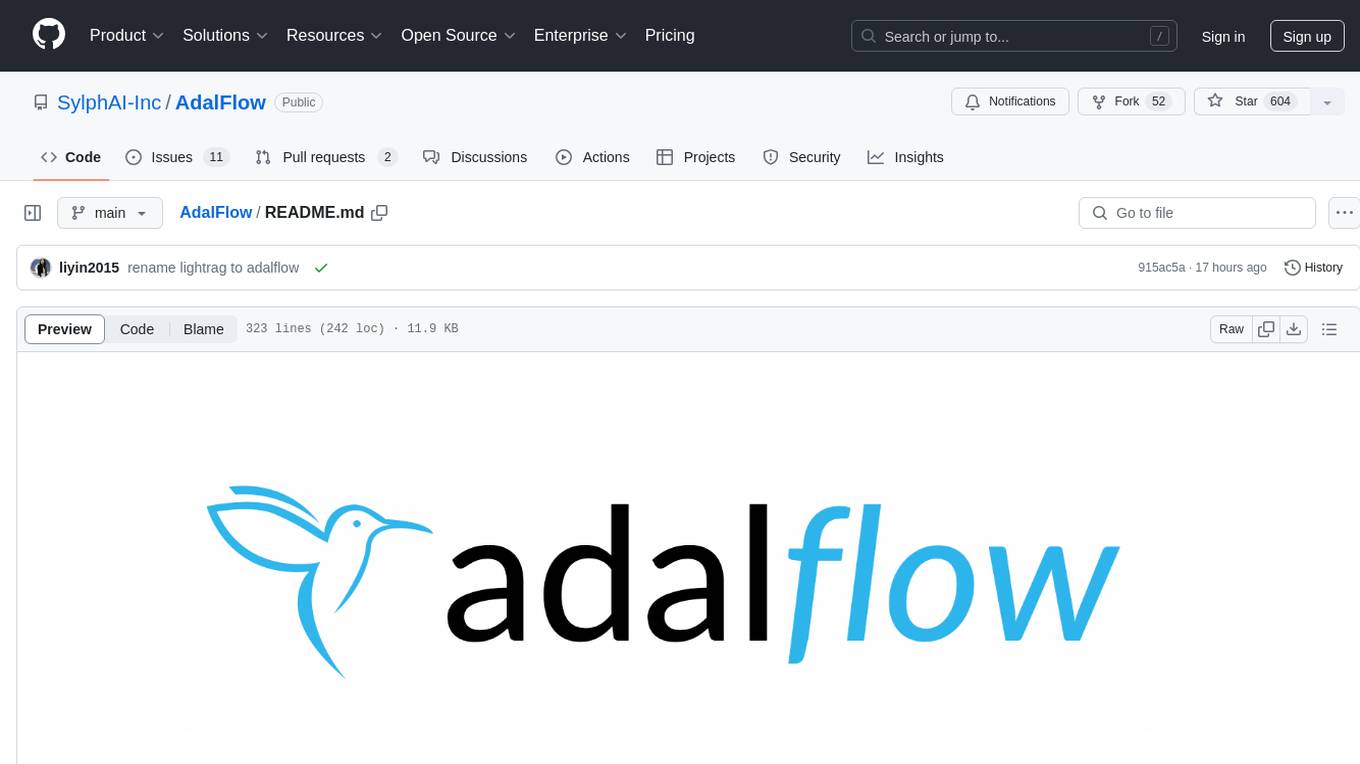
AdalFlow
AdalFlow is a library designed to help developers build and optimize Large Language Model (LLM) task pipelines. It follows a design pattern similar to PyTorch, offering a light, modular, and robust codebase. Named in honor of Ada Lovelace, AdalFlow aims to inspire more women to enter the AI field. The library is tailored for various GenAI applications like chatbots, translation, summarization, code generation, and autonomous agents, as well as classical NLP tasks such as text classification and named entity recognition. AdalFlow emphasizes modularity, robustness, and readability to support users in customizing and iterating code for their specific use cases.
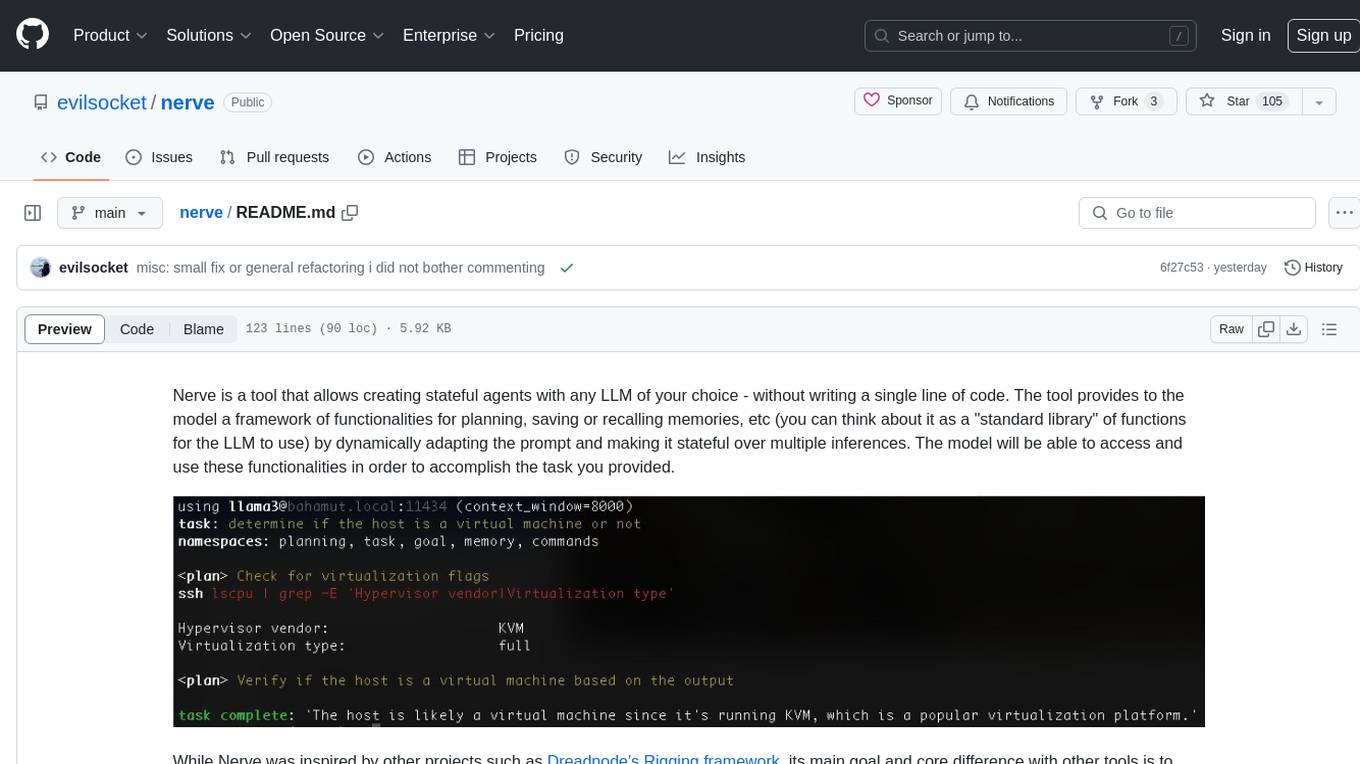
nerve
Nerve is a tool that allows creating stateful agents with any LLM of your choice without writing code. It provides a framework of functionalities for planning, saving, or recalling memories by dynamically adapting the prompt. Nerve is experimental and subject to changes. It is valuable for learning and experimenting but not recommended for production environments. The tool aims to instrument smart agents without code, inspired by projects like Dreadnode's Rigging framework.
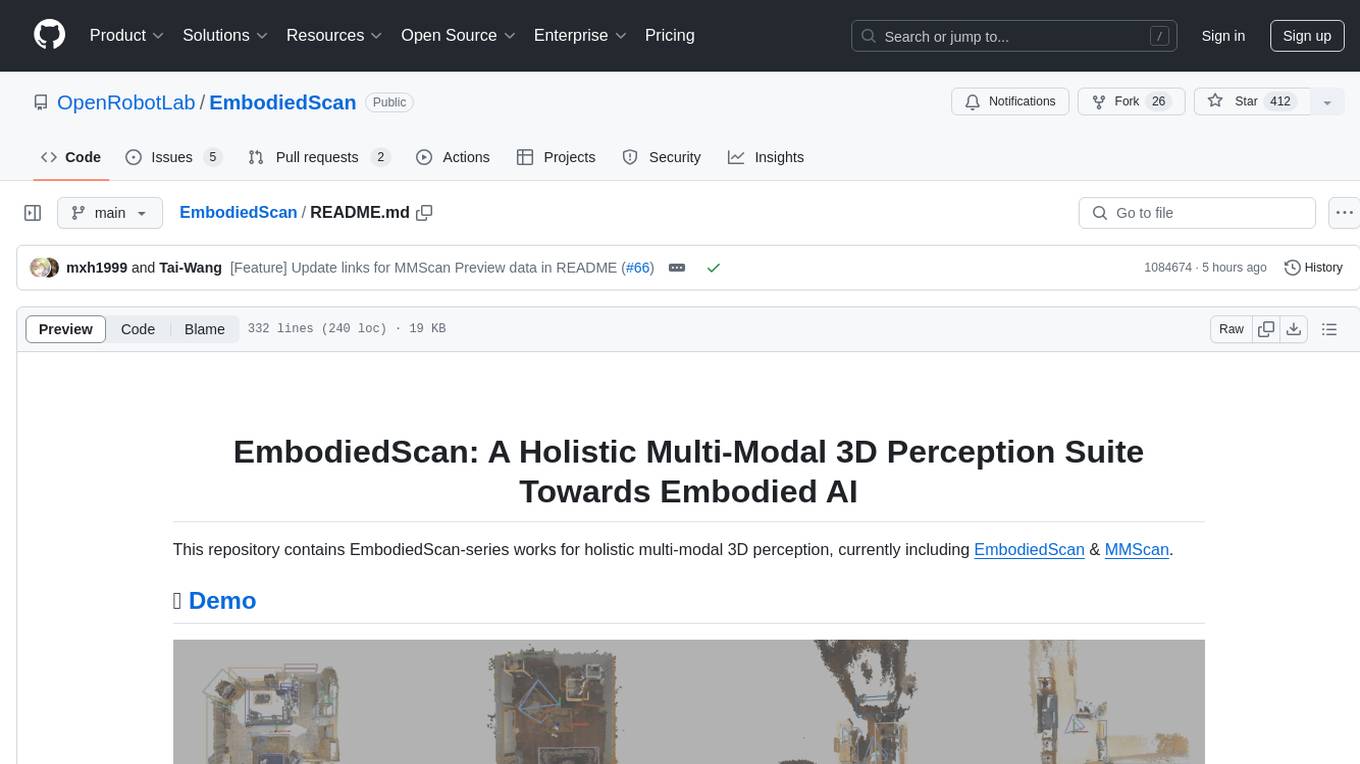
EmbodiedScan
EmbodiedScan is a holistic multi-modal 3D perception suite designed for embodied AI. It introduces a multi-modal, ego-centric 3D perception dataset and benchmark for holistic 3D scene understanding. The dataset includes over 5k scans with 1M ego-centric RGB-D views, 1M language prompts, 160k 3D-oriented boxes spanning 760 categories, and dense semantic occupancy with 80 common categories. The suite includes a baseline framework named Embodied Perceptron, capable of processing multi-modal inputs for 3D perception tasks and language-grounded tasks.
For similar tasks
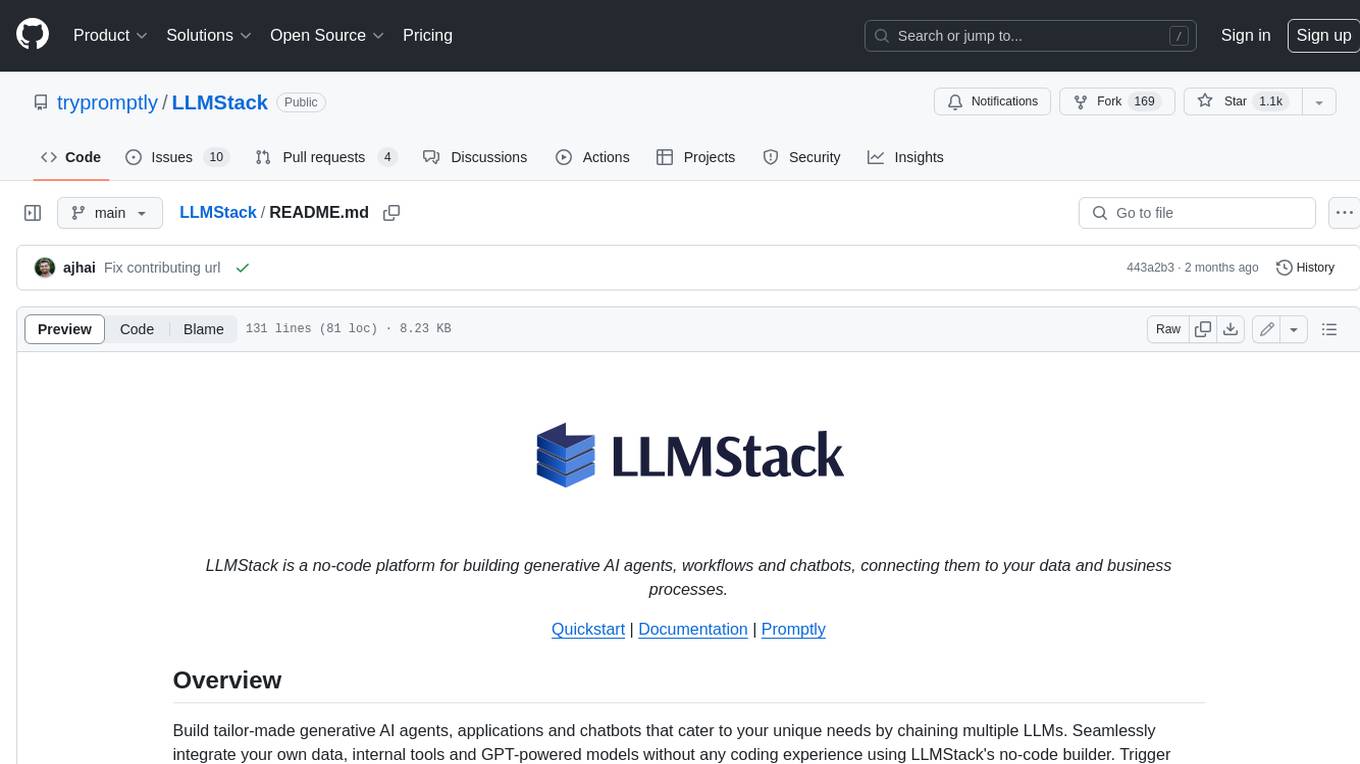
LLMStack
LLMStack is a no-code platform for building generative AI agents, workflows, and chatbots. It allows users to connect their own data, internal tools, and GPT-powered models without any coding experience. LLMStack can be deployed to the cloud or on-premise and can be accessed via HTTP API or triggered from Slack or Discord.
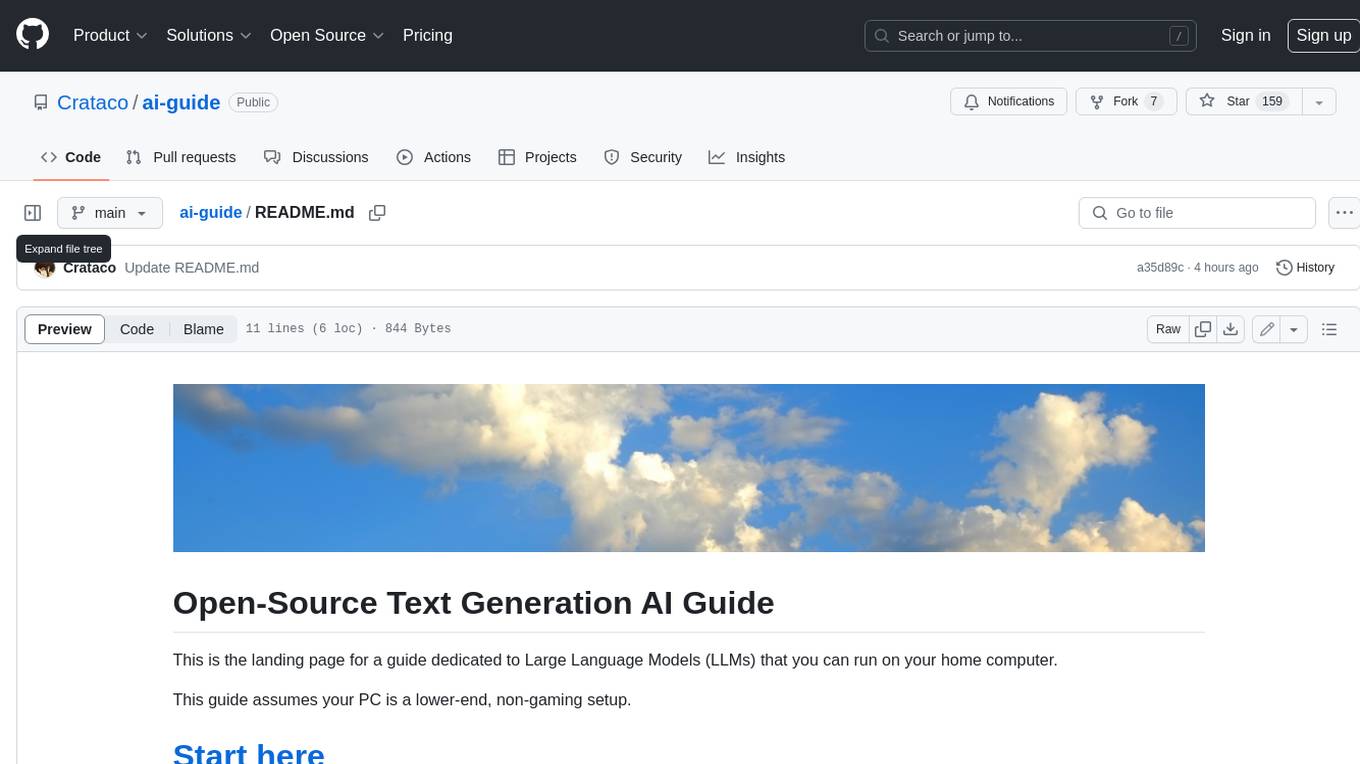
ai-guide
This guide is dedicated to Large Language Models (LLMs) that you can run on your home computer. It assumes your PC is a lower-end, non-gaming setup.
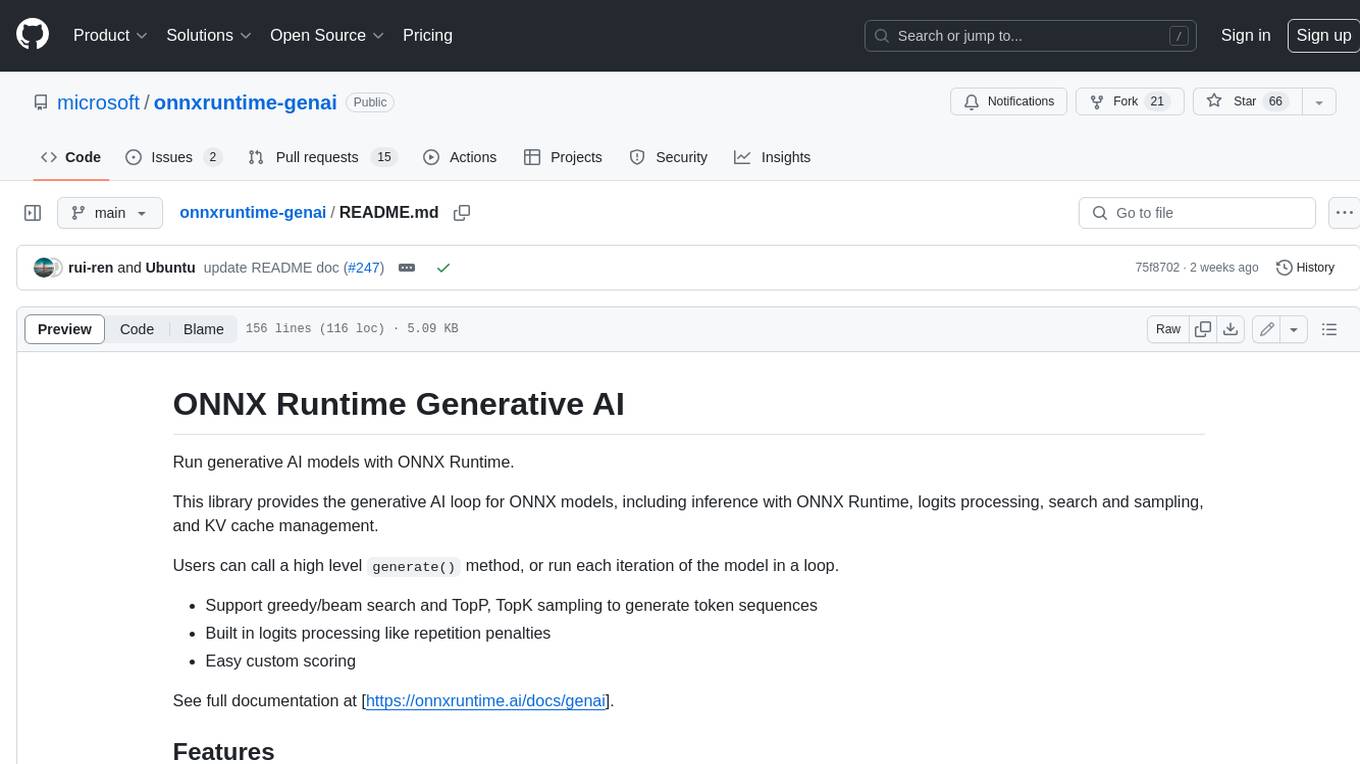
onnxruntime-genai
ONNX Runtime Generative AI is a library that provides the generative AI loop for ONNX models, including inference with ONNX Runtime, logits processing, search and sampling, and KV cache management. Users can call a high level `generate()` method, or run each iteration of the model in a loop. It supports greedy/beam search and TopP, TopK sampling to generate token sequences, has built in logits processing like repetition penalties, and allows for easy custom scoring.
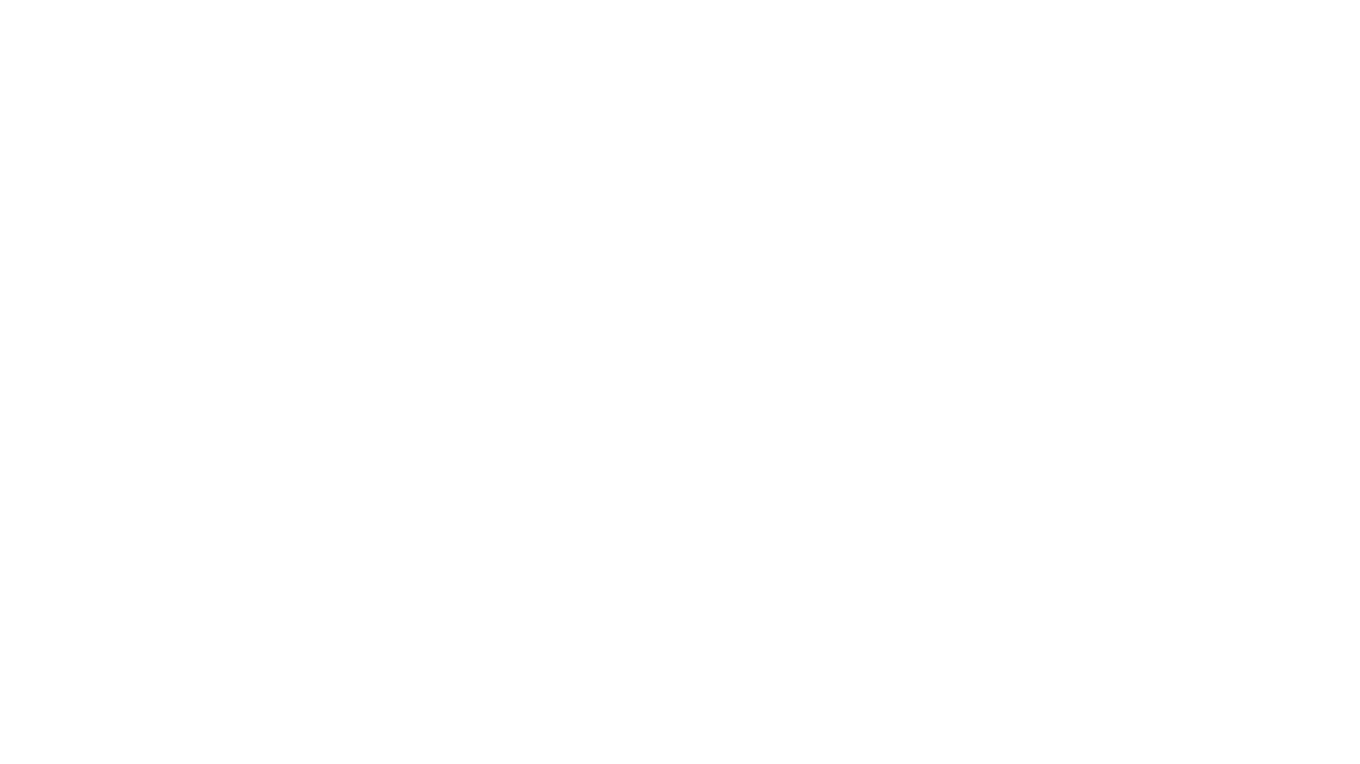
jupyter-ai
Jupyter AI connects generative AI with Jupyter notebooks. It provides a user-friendly and powerful way to explore generative AI models in notebooks and improve your productivity in JupyterLab and the Jupyter Notebook. Specifically, Jupyter AI offers: * An `%%ai` magic that turns the Jupyter notebook into a reproducible generative AI playground. This works anywhere the IPython kernel runs (JupyterLab, Jupyter Notebook, Google Colab, Kaggle, VSCode, etc.). * A native chat UI in JupyterLab that enables you to work with generative AI as a conversational assistant. * Support for a wide range of generative model providers, including AI21, Anthropic, AWS, Cohere, Gemini, Hugging Face, NVIDIA, and OpenAI. * Local model support through GPT4All, enabling use of generative AI models on consumer grade machines with ease and privacy.
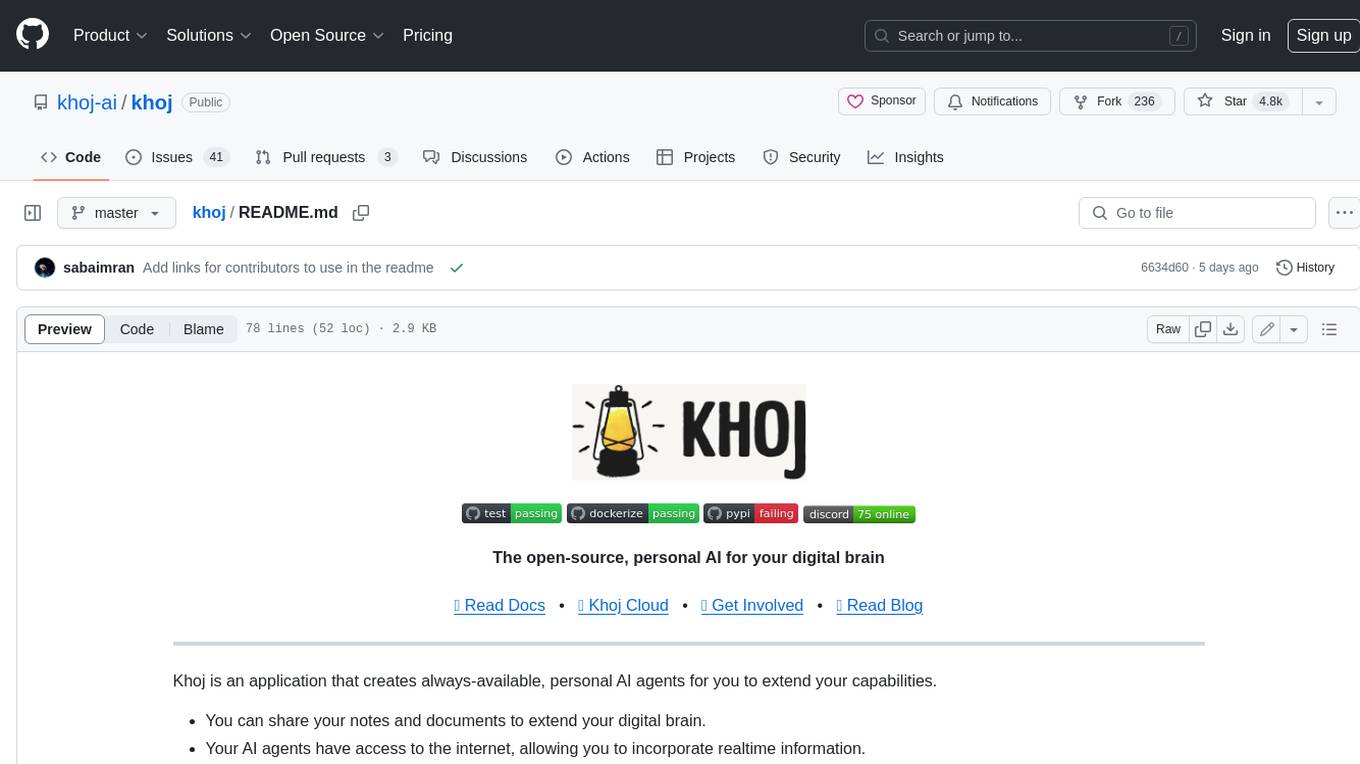
khoj
Khoj is an open-source, personal AI assistant that extends your capabilities by creating always-available AI agents. You can share your notes and documents to extend your digital brain, and your AI agents have access to the internet, allowing you to incorporate real-time information. Khoj is accessible on Desktop, Emacs, Obsidian, Web, and Whatsapp, and you can share PDF, markdown, org-mode, notion files, and GitHub repositories. You'll get fast, accurate semantic search on top of your docs, and your agents can create deeply personal images and understand your speech. Khoj is self-hostable and always will be.
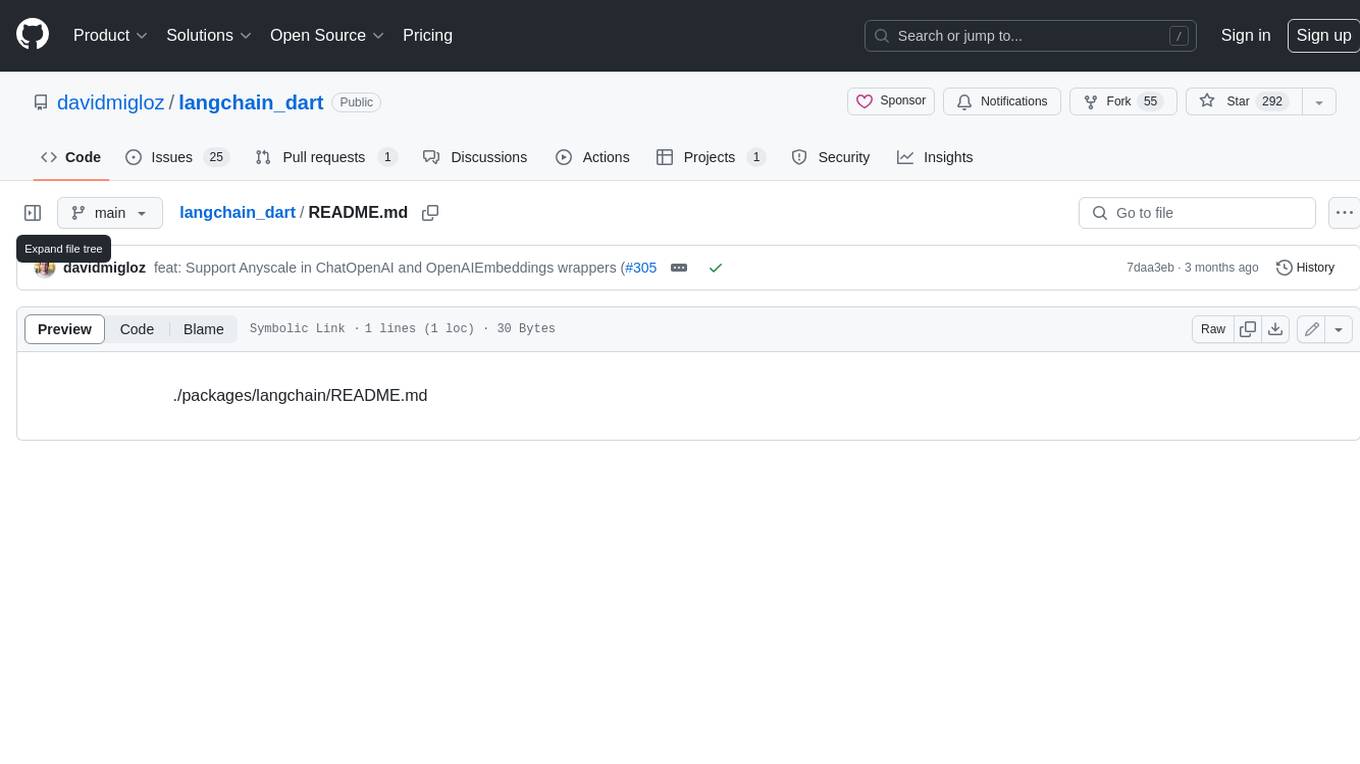
langchain_dart
LangChain.dart is a Dart port of the popular LangChain Python framework created by Harrison Chase. LangChain provides a set of ready-to-use components for working with language models and a standard interface for chaining them together to formulate more advanced use cases (e.g. chatbots, Q&A with RAG, agents, summarization, extraction, etc.). The components can be grouped into a few core modules: * **Model I/O:** LangChain offers a unified API for interacting with various LLM providers (e.g. OpenAI, Google, Mistral, Ollama, etc.), allowing developers to switch between them with ease. Additionally, it provides tools for managing model inputs (prompt templates and example selectors) and parsing the resulting model outputs (output parsers). * **Retrieval:** assists in loading user data (via document loaders), transforming it (with text splitters), extracting its meaning (using embedding models), storing (in vector stores) and retrieving it (through retrievers) so that it can be used to ground the model's responses (i.e. Retrieval-Augmented Generation or RAG). * **Agents:** "bots" that leverage LLMs to make informed decisions about which available tools (such as web search, calculators, database lookup, etc.) to use to accomplish the designated task. The different components can be composed together using the LangChain Expression Language (LCEL).
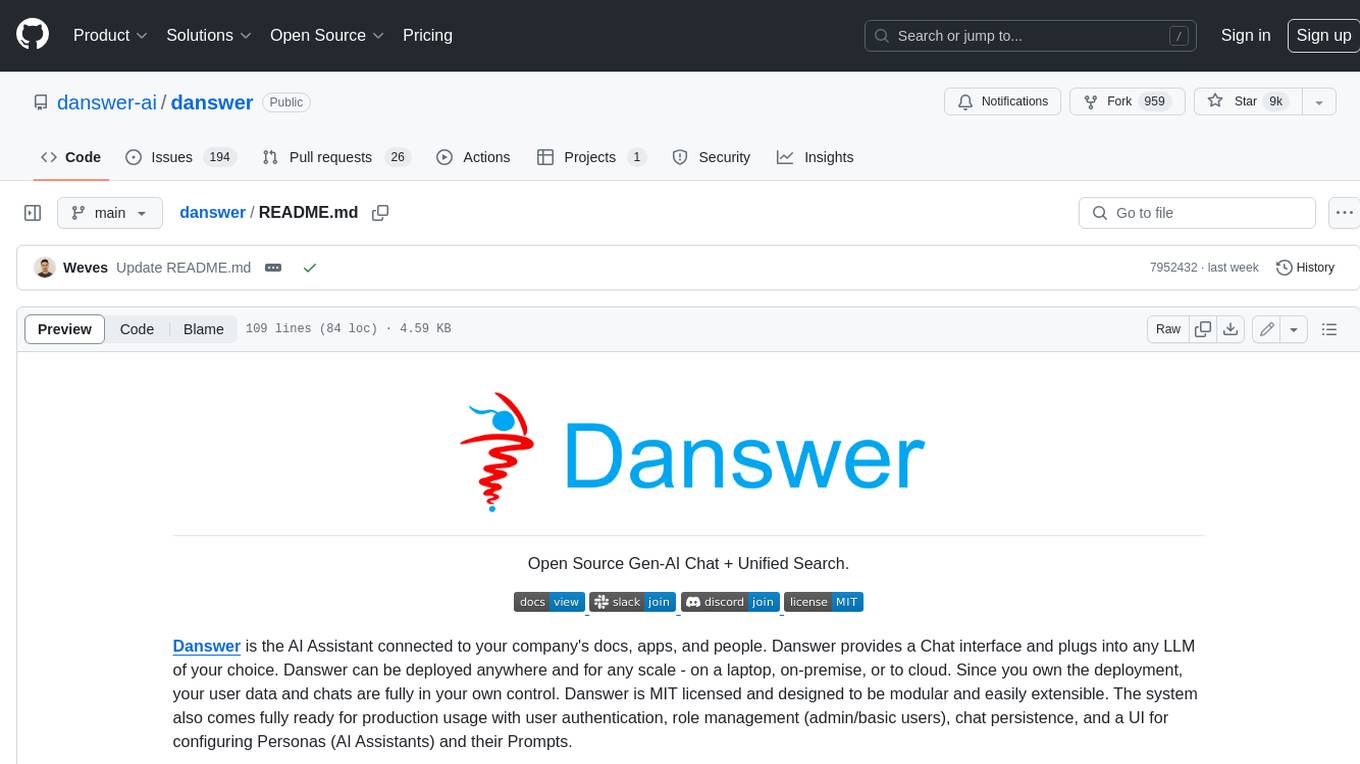
danswer
Danswer is an open-source Gen-AI Chat and Unified Search tool that connects to your company's docs, apps, and people. It provides a Chat interface and plugs into any LLM of your choice. Danswer can be deployed anywhere and for any scale - on a laptop, on-premise, or to cloud. Since you own the deployment, your user data and chats are fully in your own control. Danswer is MIT licensed and designed to be modular and easily extensible. The system also comes fully ready for production usage with user authentication, role management (admin/basic users), chat persistence, and a UI for configuring Personas (AI Assistants) and their Prompts. Danswer also serves as a Unified Search across all common workplace tools such as Slack, Google Drive, Confluence, etc. By combining LLMs and team specific knowledge, Danswer becomes a subject matter expert for the team. Imagine ChatGPT if it had access to your team's unique knowledge! It enables questions such as "A customer wants feature X, is this already supported?" or "Where's the pull request for feature Y?"
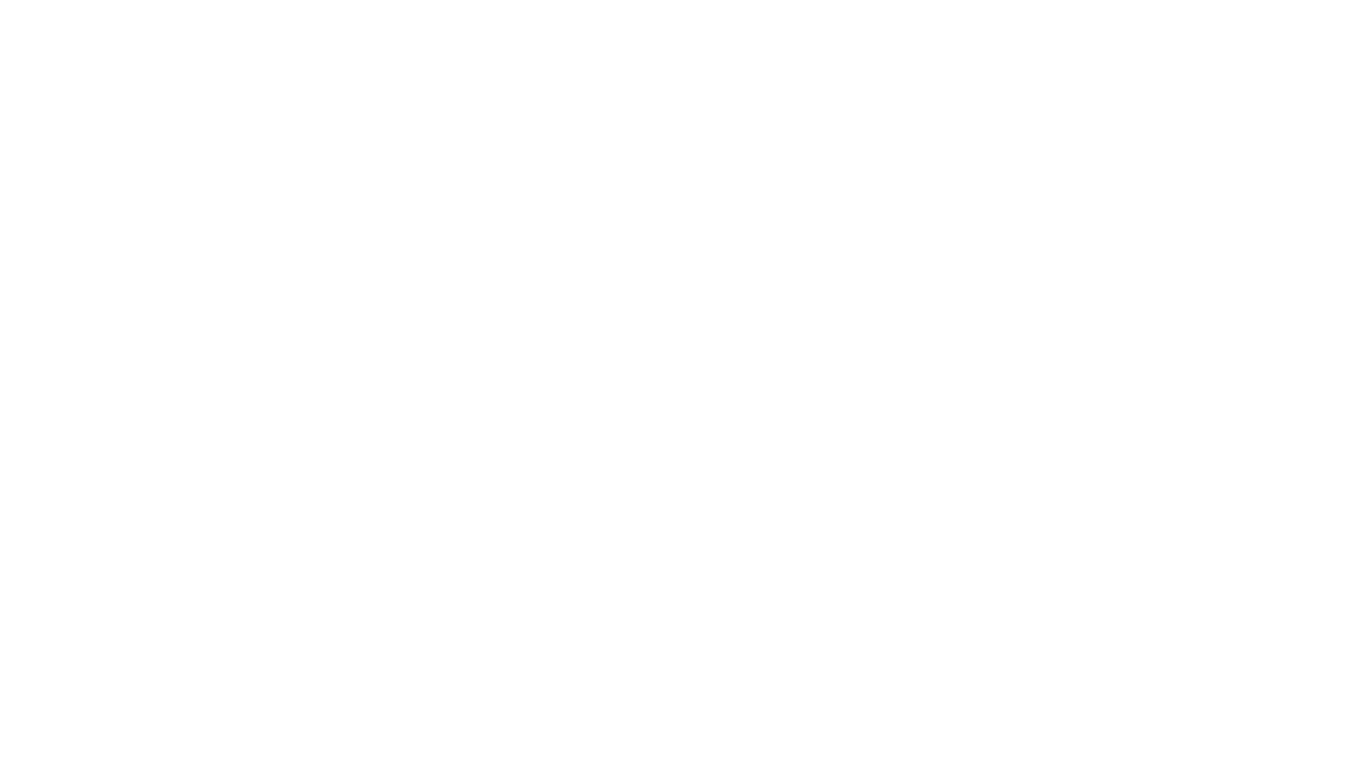
infinity
Infinity is an AI-native database designed for LLM applications, providing incredibly fast full-text and vector search capabilities. It supports a wide range of data types, including vectors, full-text, and structured data, and offers a fused search feature that combines multiple embeddings and full text. Infinity is easy to use, with an intuitive Python API and a single-binary architecture that simplifies deployment. It achieves high performance, with 0.1 milliseconds query latency on million-scale vector datasets and up to 15K QPS.
For similar jobs
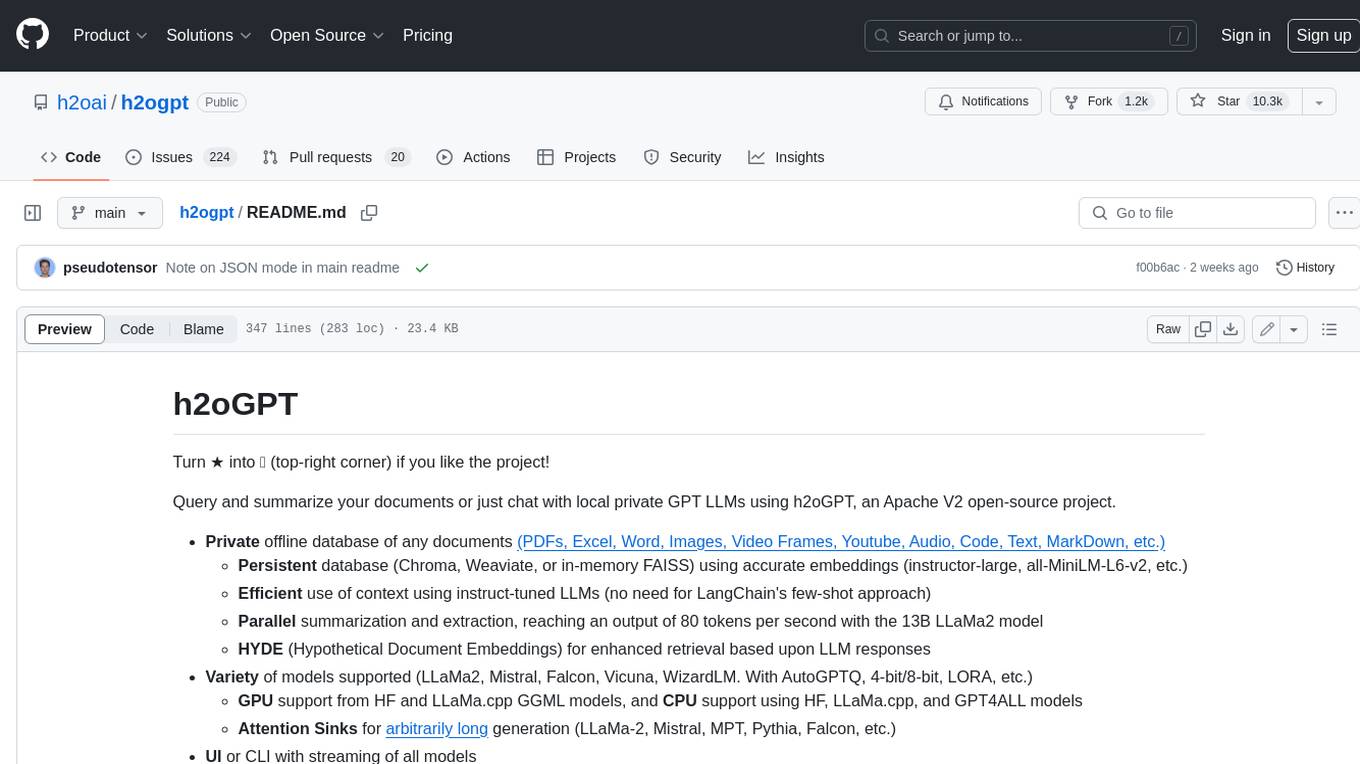
h2ogpt
h2oGPT is an Apache V2 open-source project that allows users to query and summarize documents or chat with local private GPT LLMs. It features a private offline database of any documents (PDFs, Excel, Word, Images, Video Frames, Youtube, Audio, Code, Text, MarkDown, etc.), a persistent database (Chroma, Weaviate, or in-memory FAISS) using accurate embeddings (instructor-large, all-MiniLM-L6-v2, etc.), and efficient use of context using instruct-tuned LLMs (no need for LangChain's few-shot approach). h2oGPT also offers parallel summarization and extraction, reaching an output of 80 tokens per second with the 13B LLaMa2 model, HYDE (Hypothetical Document Embeddings) for enhanced retrieval based upon LLM responses, a variety of models supported (LLaMa2, Mistral, Falcon, Vicuna, WizardLM. With AutoGPTQ, 4-bit/8-bit, LORA, etc.), GPU support from HF and LLaMa.cpp GGML models, and CPU support using HF, LLaMa.cpp, and GPT4ALL models. Additionally, h2oGPT provides Attention Sinks for arbitrarily long generation (LLaMa-2, Mistral, MPT, Pythia, Falcon, etc.), a UI or CLI with streaming of all models, the ability to upload and view documents through the UI (control multiple collaborative or personal collections), Vision Models LLaVa, Claude-3, Gemini-Pro-Vision, GPT-4-Vision, Image Generation Stable Diffusion (sdxl-turbo, sdxl) and PlaygroundAI (playv2), Voice STT using Whisper with streaming audio conversion, Voice TTS using MIT-Licensed Microsoft Speech T5 with multiple voices and Streaming audio conversion, Voice TTS using MPL2-Licensed TTS including Voice Cloning and Streaming audio conversion, AI Assistant Voice Control Mode for hands-free control of h2oGPT chat, Bake-off UI mode against many models at the same time, Easy Download of model artifacts and control over models like LLaMa.cpp through the UI, Authentication in the UI by user/password via Native or Google OAuth, State Preservation in the UI by user/password, Linux, Docker, macOS, and Windows support, Easy Windows Installer for Windows 10 64-bit (CPU/CUDA), Easy macOS Installer for macOS (CPU/M1/M2), Inference Servers support (oLLaMa, HF TGI server, vLLM, Gradio, ExLLaMa, Replicate, OpenAI, Azure OpenAI, Anthropic), OpenAI-compliant, Server Proxy API (h2oGPT acts as drop-in-replacement to OpenAI server), Python client API (to talk to Gradio server), JSON Mode with any model via code block extraction. Also supports MistralAI JSON mode, Claude-3 via function calling with strict Schema, OpenAI via JSON mode, and vLLM via guided_json with strict Schema, Web-Search integration with Chat and Document Q/A, Agents for Search, Document Q/A, Python Code, CSV frames (Experimental, best with OpenAI currently), Evaluate performance using reward models, and Quality maintained with over 1000 unit and integration tests taking over 4 GPU-hours.
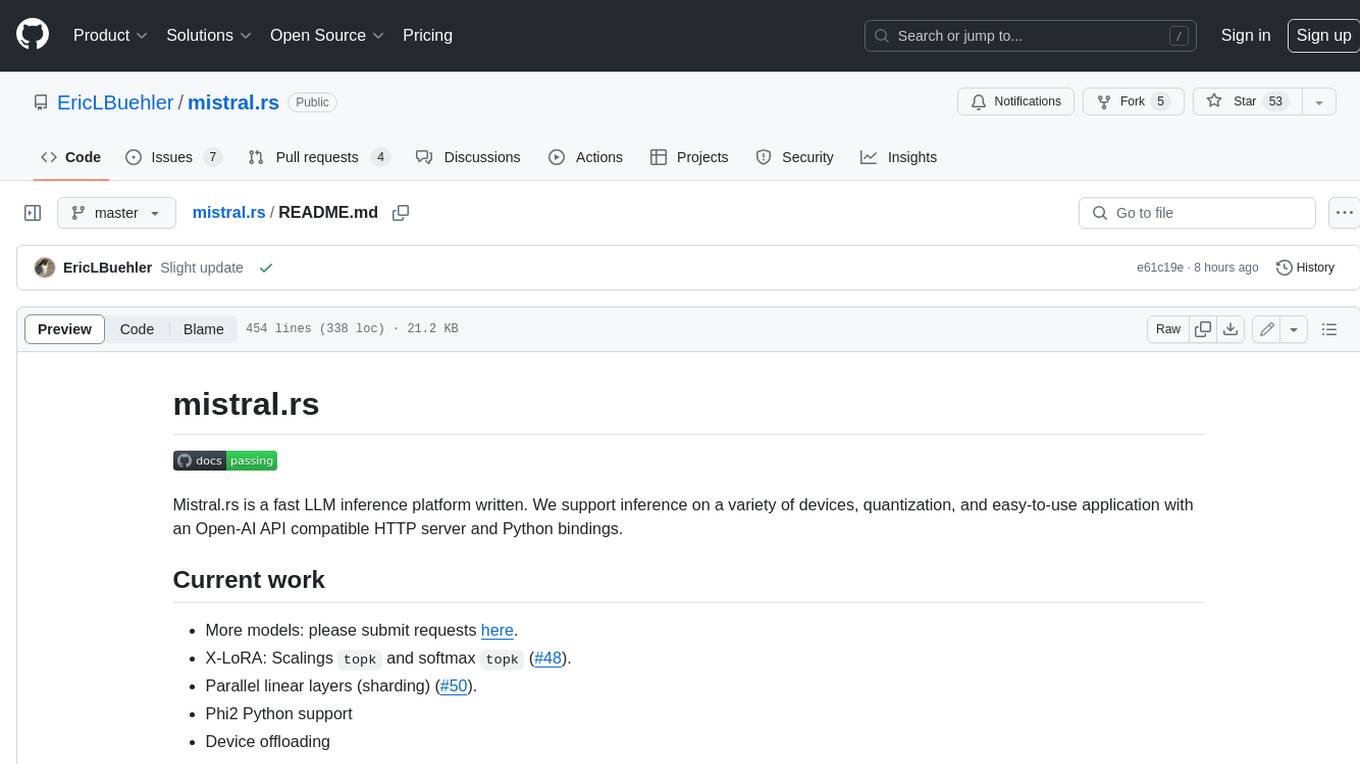
mistral.rs
Mistral.rs is a fast LLM inference platform written in Rust. We support inference on a variety of devices, quantization, and easy-to-use application with an Open-AI API compatible HTTP server and Python bindings.
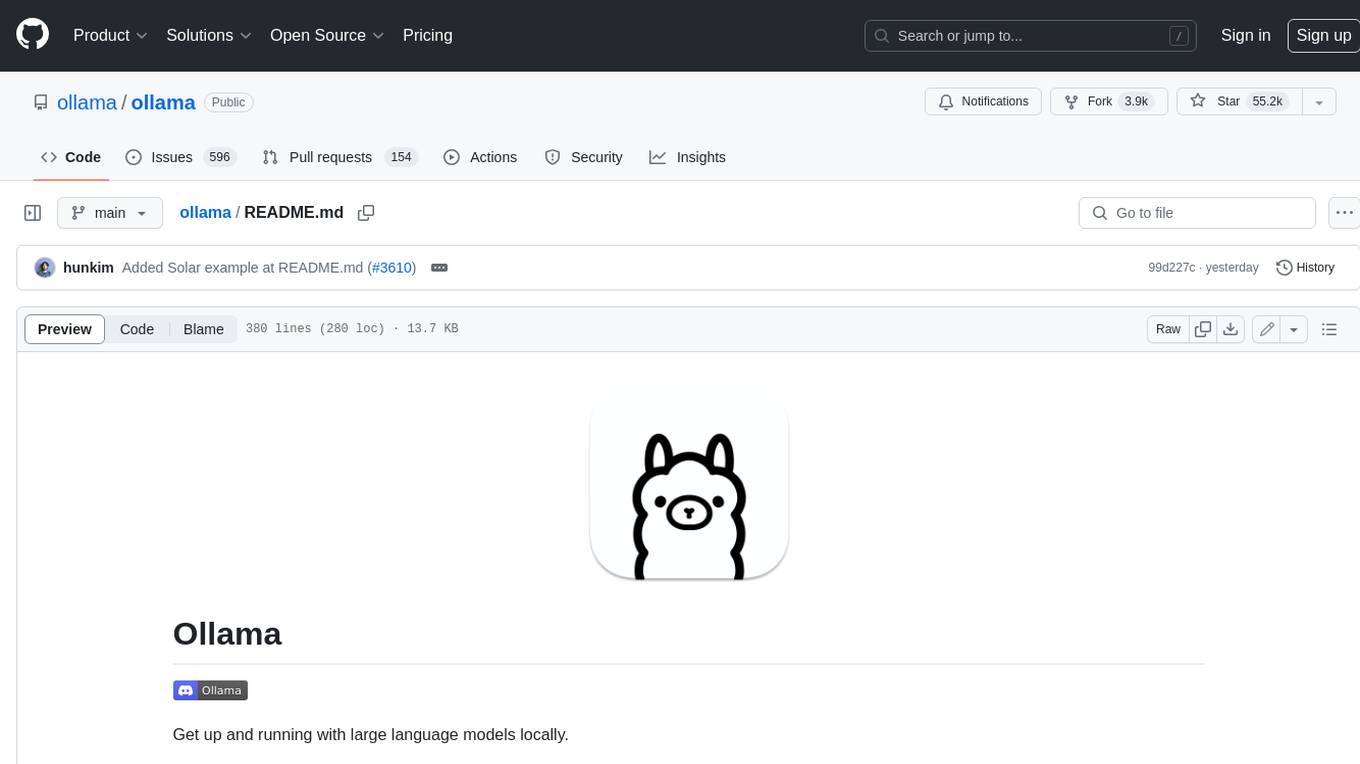
ollama
Ollama is a lightweight, extensible framework for building and running language models on the local machine. It provides a simple API for creating, running, and managing models, as well as a library of pre-built models that can be easily used in a variety of applications. Ollama is designed to be easy to use and accessible to developers of all levels. It is open source and available for free on GitHub.
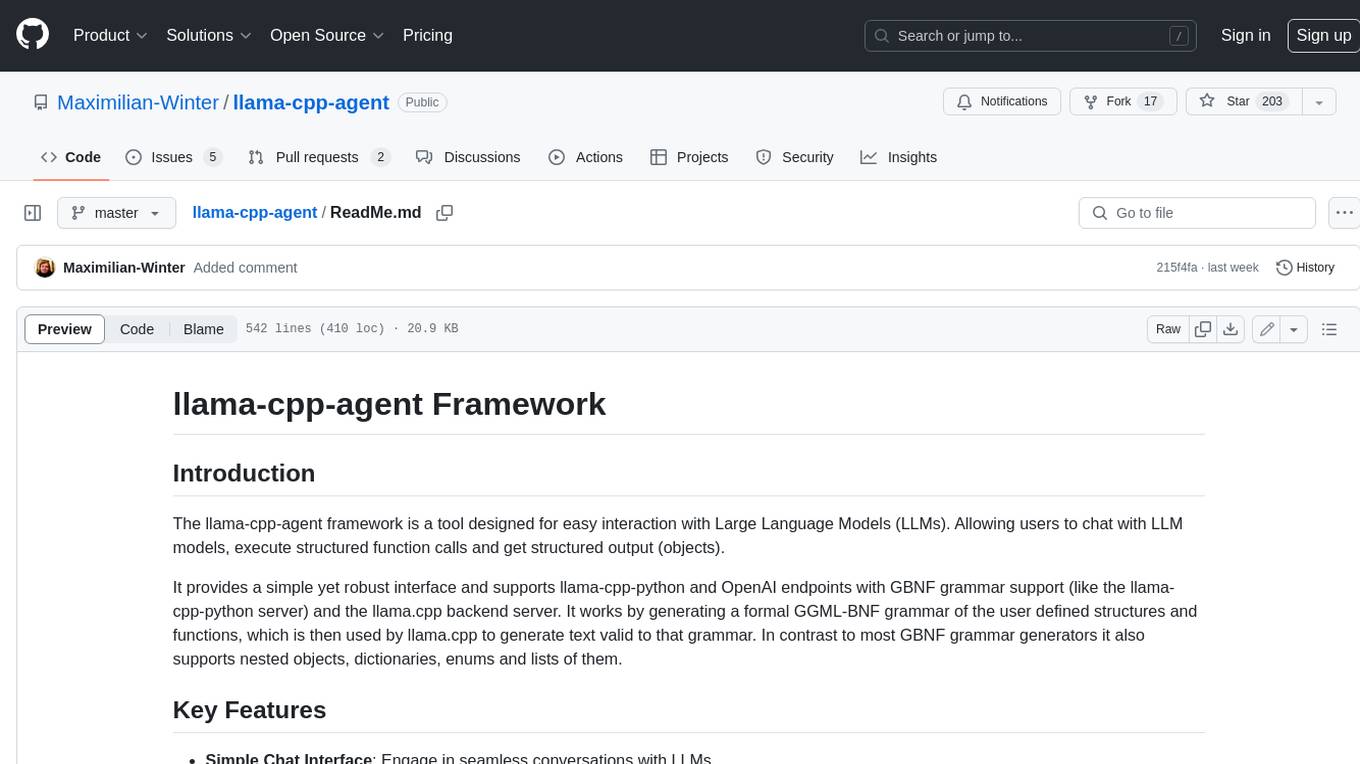
llama-cpp-agent
The llama-cpp-agent framework is a tool designed for easy interaction with Large Language Models (LLMs). Allowing users to chat with LLM models, execute structured function calls and get structured output (objects). It provides a simple yet robust interface and supports llama-cpp-python and OpenAI endpoints with GBNF grammar support (like the llama-cpp-python server) and the llama.cpp backend server. It works by generating a formal GGML-BNF grammar of the user defined structures and functions, which is then used by llama.cpp to generate text valid to that grammar. In contrast to most GBNF grammar generators it also supports nested objects, dictionaries, enums and lists of them.
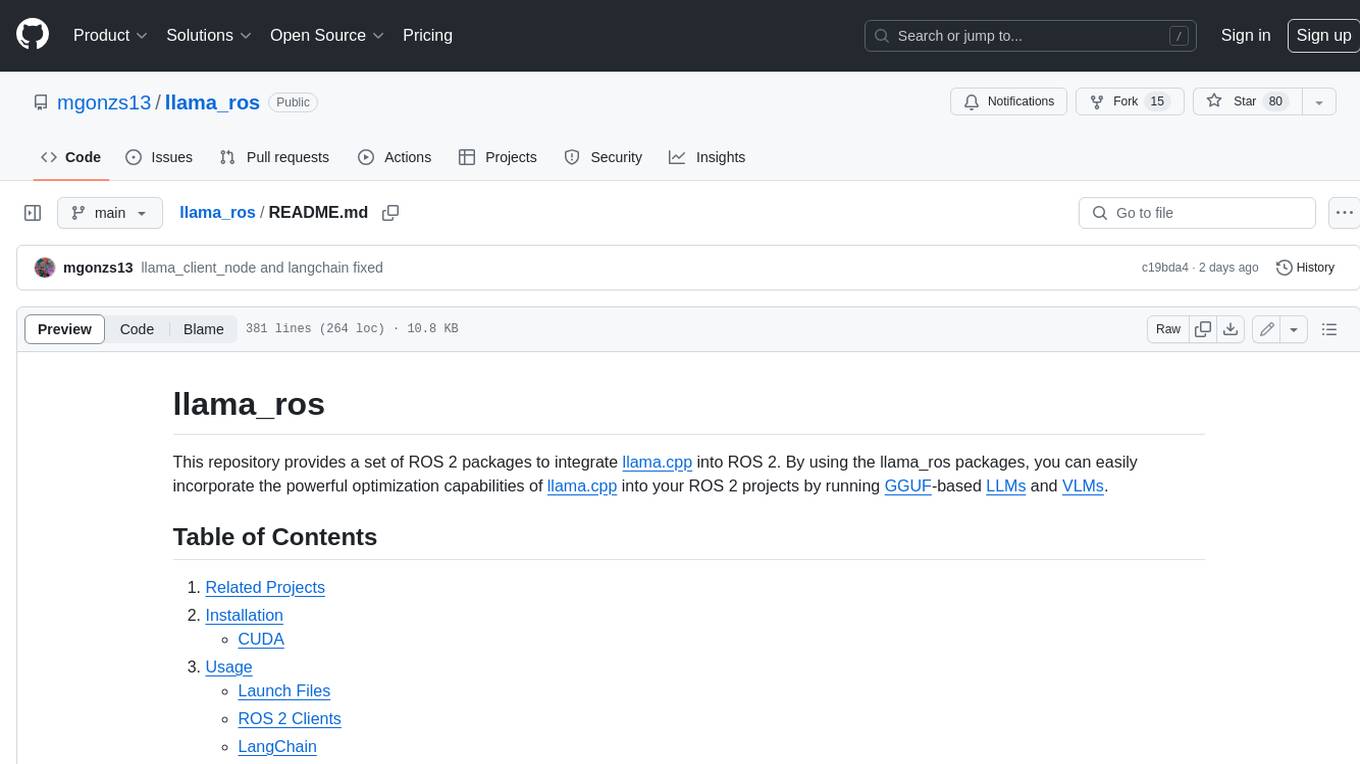
llama_ros
This repository provides a set of ROS 2 packages to integrate llama.cpp into ROS 2. By using the llama_ros packages, you can easily incorporate the powerful optimization capabilities of llama.cpp into your ROS 2 projects by running GGUF-based LLMs and VLMs.
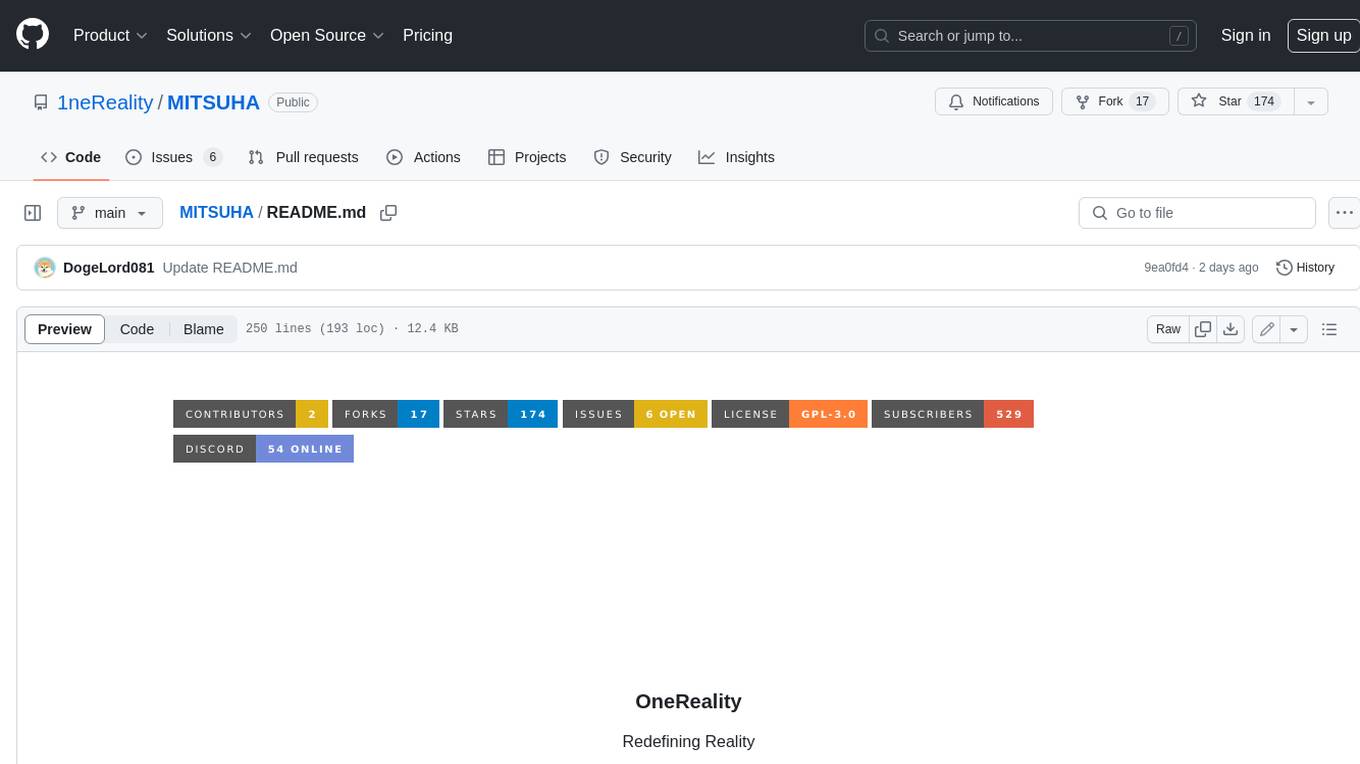
MITSUHA
OneReality is a virtual waifu/assistant that you can speak to through your mic and it'll speak back to you! It has many features such as: * You can speak to her with a mic * It can speak back to you * Has short-term memory and long-term memory * Can open apps * Smarter than you * Fluent in English, Japanese, Korean, and Chinese * Can control your smart home like Alexa if you set up Tuya (more info in Prerequisites) It is built with Python, Llama-cpp-python, Whisper, SpeechRecognition, PocketSphinx, VITS-fast-fine-tuning, VITS-simple-api, HyperDB, Sentence Transformers, and Tuya Cloud IoT.
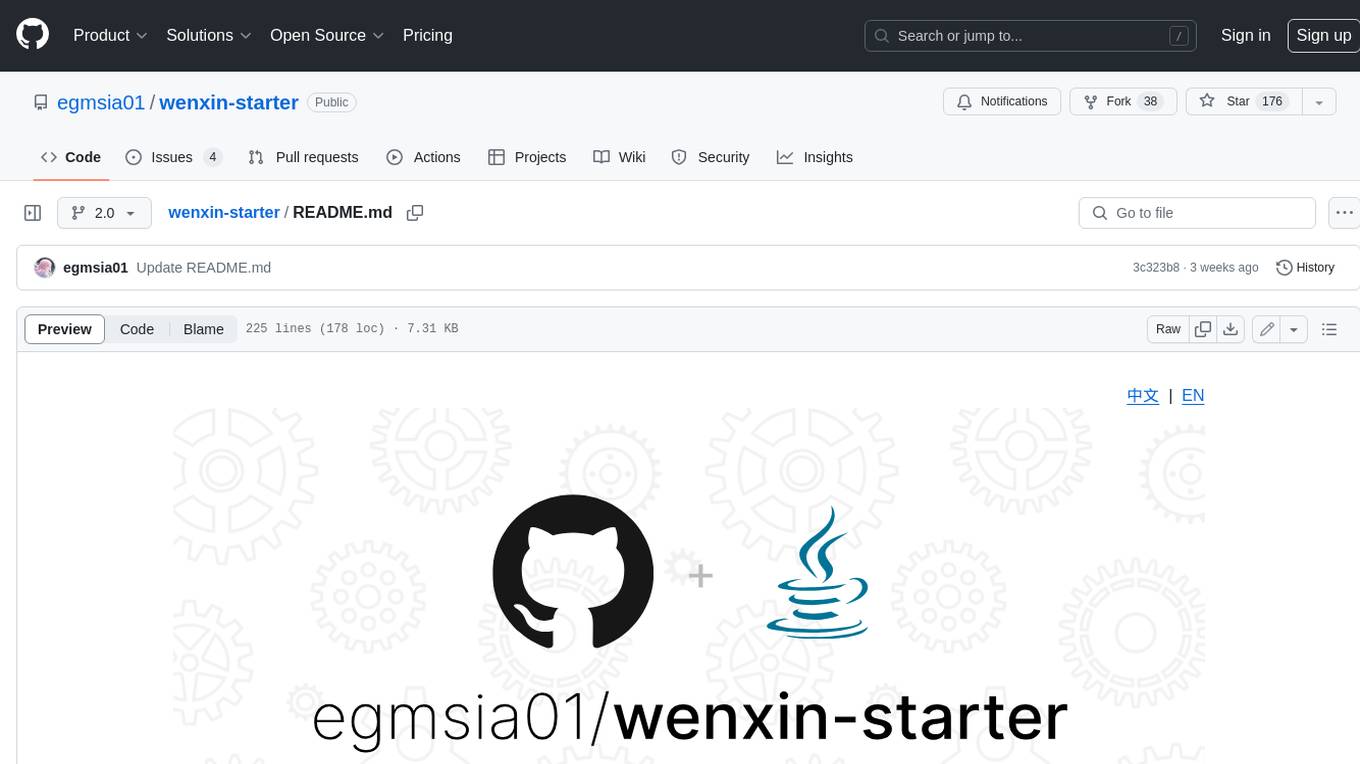
wenxin-starter
WenXin-Starter is a spring-boot-starter for Baidu's "Wenxin Qianfan WENXINWORKSHOP" large model, which can help you quickly access Baidu's AI capabilities. It fully integrates the official API documentation of Wenxin Qianfan. Supports text-to-image generation, built-in dialogue memory, and supports streaming return of dialogue. Supports QPS control of a single model and supports queuing mechanism. Plugins will be added soon.
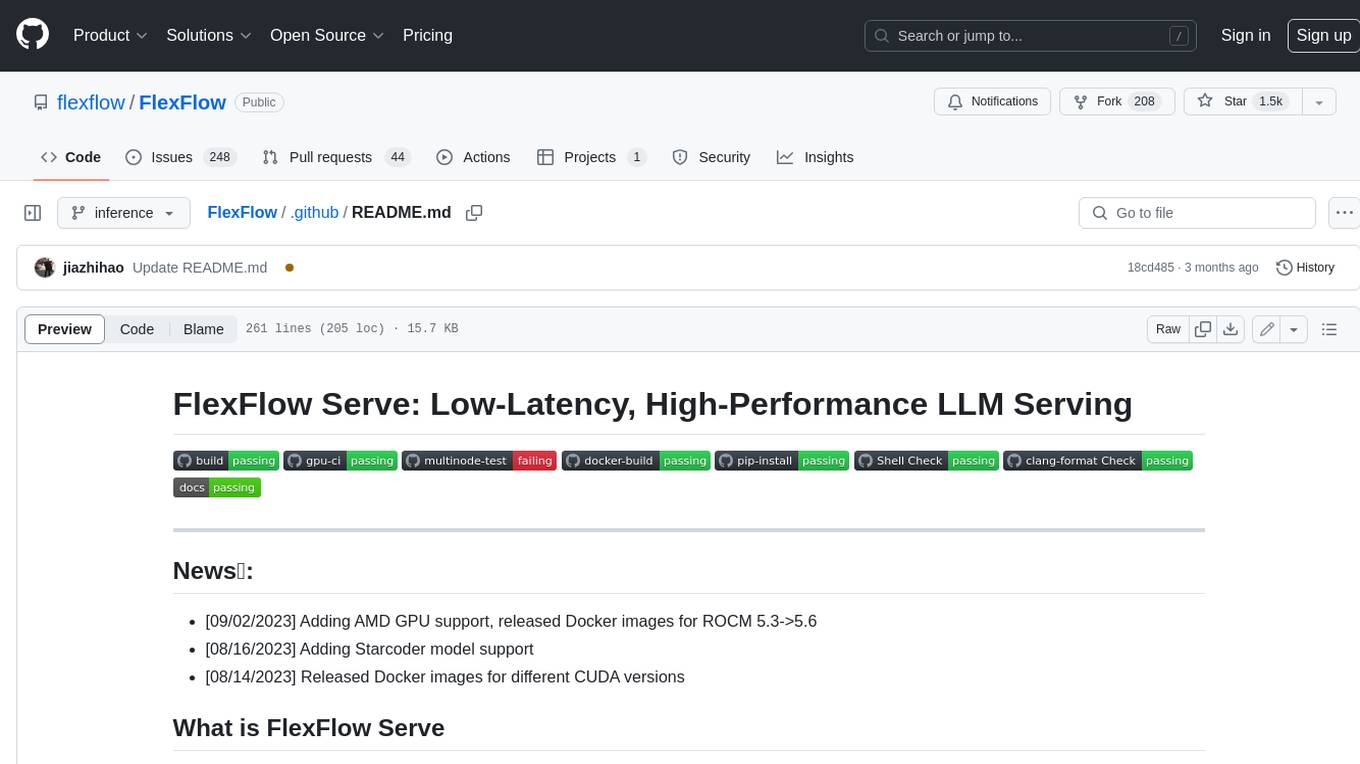
FlexFlow
FlexFlow Serve is an open-source compiler and distributed system for **low latency**, **high performance** LLM serving. FlexFlow Serve outperforms existing systems by 1.3-2.0x for single-node, multi-GPU inference and by 1.4-2.4x for multi-node, multi-GPU inference.