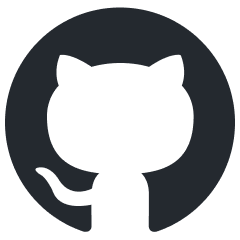
RD-Agent
Research and development (R&D) is crucial for the enhancement of industrial productivity, especially in the AI era, where the core aspects of R&D are mainly focused on data and models. We are committed to automating these high-value generic R&D processes through our open source R&D automation tool RD-Agent, which lets AI drive data-driven AI.
Stars: 4028
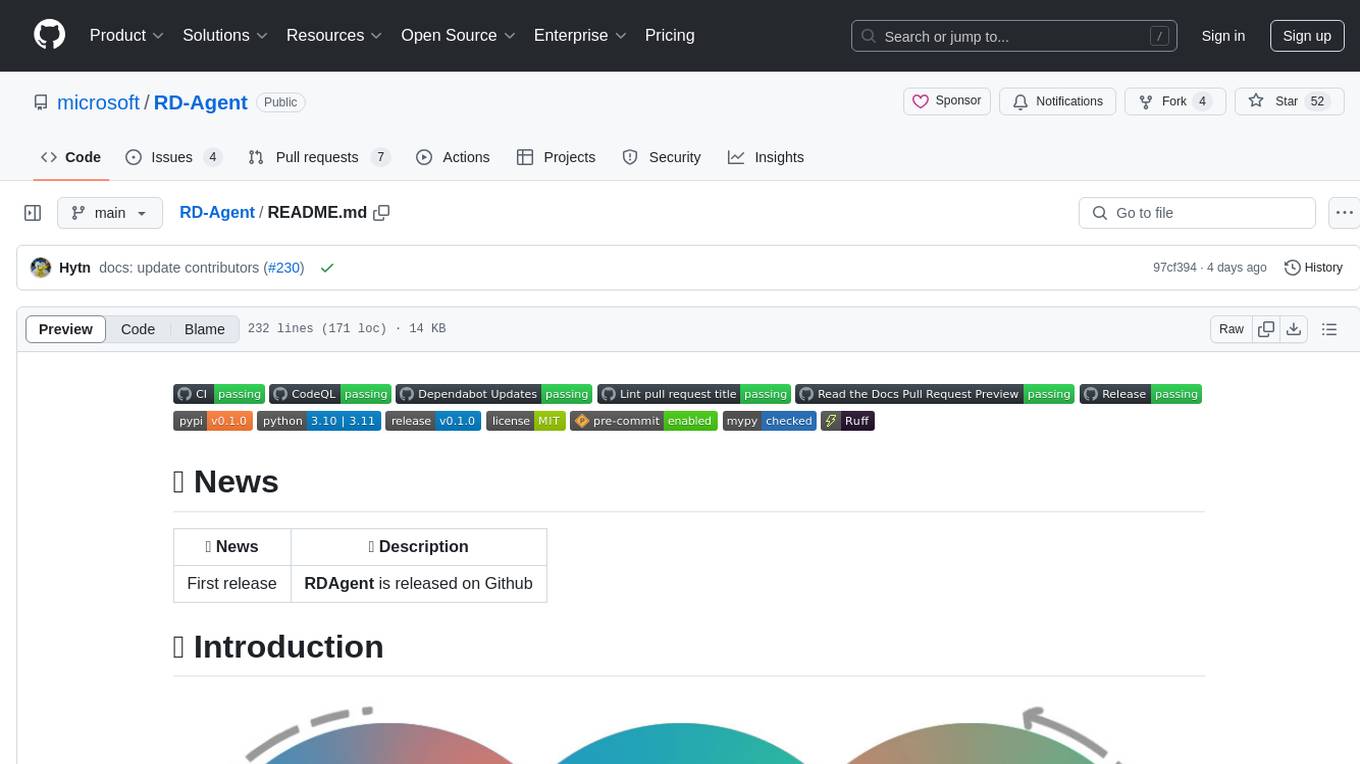
RD-Agent is a tool designed to automate critical aspects of industrial R&D processes, focusing on data-driven scenarios to streamline model and data development. It aims to propose new ideas ('R') and implement them ('D') automatically, leading to solutions of significant industrial value. The tool supports scenarios like Automated Quantitative Trading, Data Mining Agent, Research Copilot, and more, with a framework to push the boundaries of research in data science. Users can create a Conda environment, install the RDAgent package from PyPI, configure GPT model, and run various applications for tasks like quantitative trading, model evolution, medical prediction, and more. The tool is intended to enhance R&D processes and boost productivity in industrial settings.
README:
🖥️ Live Demo | 🎥 Demo Video
Check out our demo video showcasing the current progress of our Data Science Agent under development:
https://github.com/user-attachments/assets/3eccbecb-34a4-4c81-bce4-d3f8862f7305
🗞️ News | 📝 Description |
---|---|
Support LiteLLM Backend | We now fully support LiteLLM as a backend for integration with multiple LLM providers. |
More General Data Science Agent | 🚀Coming soon! |
Kaggle Scenario release | We release Kaggle Agent, try the new features! |
Official WeChat group release | We created a WeChat group, welcome to join! (🗪QR Code) |
Official Discord release | We launch our first chatting channel in Discord (🗪 |
First release | RDAgent is released on GitHub |
RDAgent aims to automate the most critical and valuable aspects of the industrial R&D process, and we begin with focusing on the data-driven scenarios to streamline the development of models and data. Methodologically, we have identified a framework with two key components: 'R' for proposing new ideas and 'D' for implementing them. We believe that the automatic evolution of R&D will lead to solutions of significant industrial value.
R&D is a very general scenario. The advent of RDAgent can be your
- 💰 Automatic Quant Factory (🎥Demo Video|
▶️ YouTube) - 🤖 Data Mining Agent: Iteratively proposing data & models (🎥Demo Video 1|
▶️ YouTube) (🎥Demo Video 2|▶️ YouTube) and implementing them by gaining knowledge from data. - 🦾 Research Copilot: Auto read research papers (🎥Demo Video|
▶️ YouTube) / financial reports (🎥Demo Video|▶️ YouTube) and implement model structures or building datasets. - 🤖 Kaggle Agent: Auto Model Tuning and Feature Engineering(🎥Demo Video Coming Soon...) and implementing them to achieve more in competitions.
- ...
You can click the links above to view the demo. We're continuously adding more methods and scenarios to the project to enhance your R&D processes and boost productivity.
Additionally, you can take a closer look at the examples in our 🖥️ Live Demo.
You can try above demos by running the following command:
Users must ensure Docker is installed before attempting most scenarios. Please refer to the official 🐳Docker page for installation instructions.
Ensure the current user can run Docker commands without using sudo. You can verify this by executing docker run hello-world
.
- Create a new conda environment with Python (3.10 and 3.11 are well-tested in our CI):
conda create -n rdagent python=3.10
- Activate the environment:
conda activate rdagent
- You can directly install the RDAgent package from PyPI:
pip install rdagent
- rdagent provides a health check that currently checks two things.
- whether the docker installation was successful.
- whether the default port used by the rdagent ui is occupied.
rdagent health_check
-
The demos requires following ability:
- ChatCompletion
- json_mode
- embedding query
-
For example: If you are using the
OpenAI API
, you have to configure your GPT model in the.env
file like this.cat << EOF > .env OPENAI_API_KEY=<replace_with_your_openai_api_key> # EMBEDDING_MODEL=text-embedding-3-small CHAT_MODEL=gpt-4-turbo EOF
-
However, not every API services support these features by default. For example:
AZURE OpenAI
, you have to configure your GPT model in the.env
file like this.cat << EOF > .env USE_AZURE=True EMBEDDING_OPENAI_API_KEY=<replace_with_your_azure_openai_api_key> EMBEDDING_AZURE_API_BASE=<replace_with_your_azure_endpoint> EMBEDDING_AZURE_API_VERSION=<replace_with_the_version_of_your_azure_openai_api> EMBEDDING_MODEL=text-embedding-3-small CHAT_OPENAI_API_KEY=<replace_with_your_azure_openai_api_key> CHAT_AZURE_API_BASE=<replace_with_your_azure_endpoint> CHAT_AZURE_API_VERSION=<replace_with_the_version_of_your_azure_openai_api> CHAT_MODEL=<replace_it_with_the_name_of_your_azure_chat_model> EOF
-
We now support LiteLLM as a backend for integration with multiple LLM providers. If you use LiteLLM Backend to use models, you can configure as follows:
cat << EOF > .env BACKEND=rdagent.oai.backend.LiteLLMAPIBackend # It can be modified to any model supported by LiteLLM. CHAT_MODEL=gpt-4o EMBEDDING_MODEL=text-embedding-3-small # The backend api_key fully follow the convention of litellm. OPENAI_API_KEY=<replace_with_your_openai_api_key>
-
For more configuration information, please refer to the documentation.
The 🖥️ Live Demo is implemented by the following commands(each item represents one demo, you can select the one you prefer):
-
Run the Automated Quantitative Trading & Iterative Factors Evolution: Qlib self-loop factor proposal and implementation application
rdagent fin_factor
-
Run the Automated Quantitative Trading & Iterative Model Evolution: Qlib self-loop model proposal and implementation application
rdagent fin_model
-
Run the Automated Medical Prediction Model Evolution: Medical self-loop model proposal and implementation application
(1) Apply for an account at PhysioNet.
(2) Request access to FIDDLE preprocessed data: FIDDLE Dataset.
(3) Place your username and password in.env
.cat << EOF >> .env DM_USERNAME=<your_username> DM_PASSWORD=<your_password> EOF
rdagent med_model
-
Run the Automated Quantitative Trading & Factors Extraction from Financial Reports: Run the Qlib factor extraction and implementation application based on financial reports
# 1. Generally, you can run this scenario using the following command: rdagent fin_factor_report --report_folder=<Your financial reports folder path> # 2. Specifically, you need to prepare some financial reports first. You can follow this concrete example: wget https://github.com/SunsetWolf/rdagent_resource/releases/download/reports/all_reports.zip unzip all_reports.zip -d git_ignore_folder/reports rdagent fin_factor_report --report_folder=git_ignore_folder/reports
-
Run the Automated Model Research & Development Copilot: model extraction and implementation application
# 1. Generally, you can run your own papers/reports with the following command: rdagent general_model <Your paper URL> # 2. Specifically, you can do it like this. For more details and additional paper examples, use `rdagent general_model -h`: rdagent general_model "https://arxiv.org/pdf/2210.09789"
-
Run the Automated Kaggle Model Tuning & Feature Engineering: self-loop model proposal and feature engineering implementation application
Using sf-crime (San Francisco Crime Classification) as an example.
- Register and login on the Kaggle website.
- Configuring the Kaggle API.
(1) Click on the avatar (usually in the top right corner of the page) ->Settings
->Create New Token
, A file calledkaggle.json
will be downloaded.
(2) Movekaggle.json
to~/.config/kaggle/
(3) Modify the permissions of the kaggle.json file. Reference command:chmod 600 ~/.config/kaggle/kaggle.json
- Join the competition: Click
Join the competition
->I Understand and Accept
at the bottom of the competition details page.
# Generally, you can run the Kaggle competition program with the following command: rdagent kaggle --competition <your competition name> # Specifically, you will need to first prepare some competition description files and configure the competition description file path, which you can follow for this specific example: # 1. Prepare the competition description files wget https://github.com/SunsetWolf/rdagent_resource/releases/download/kaggle_data/kaggle_data.zip unzip kaggle_data.zip -d git_ignore_folder/kaggle_data # 2. Add the competition description file path to the `.env` file. dotenv set KG_LOCAL_DATA_PATH "$(pwd)/git_ignore_folder/kaggle_data" # 3. run the application rdagent kaggle --competition sf-crime
Description of the above example:
- Kaggle competition data, contains two parts: competition description file (json file) and competition dataset (zip file). We prepare the competition description file for you, the competition dataset will be downloaded automatically when you run the program, as in the example.
- If you want to download the competition description file automatically, you need to install chromedriver, The instructions for installing chromedriver can be found in the documentation.
- The Competition List Available can be found here.
- Register and login on the Kaggle website.
-
You can run the following command for our demo program to see the run logs.
rdagent ui --port 19899 --log_dir <your log folder like "log/">
Note: Although port 19899 is not commonly used, but before you run this demo, you need to check if port 19899 is occupied. If it is, please change it to another port that is not occupied.
You can check if a port is occupied by running the following command.
rdagent health_check
We have applied RD-Agent to multiple valuable data-driven industrial scenarios.
In this project, we are aiming to build an Agent to automate Data-Driven R&D that can
- 📄 Read real-world material (reports, papers, etc.) and extract key formulas, descriptions of interested features and models, which are the key components of data-driven R&D .
- 🛠️ Implement the extracted formulas (e.g., features, factors, and models) in runnable codes.
- Due to the limited ability of LLM in implementing at once, build an evolving process for the agent to improve performance by learning from feedback and knowledge.
- 💡 Propose new ideas based on current knowledge and observations.
In the two key areas of data-driven scenarios, model implementation and data building, our system aims to serve two main roles: 🦾Copilot and 🤖Agent.
- The 🦾Copilot follows human instructions to automate repetitive tasks.
- The 🤖Agent, being more autonomous, actively proposes ideas for better results in the future.
The supported scenarios are listed below:
Scenario/Target | Model Implementation | Data Building |
---|---|---|
💹 Finance | 🤖 Iteratively Proposing Ideas & Evolving |
🤖 Iteratively Proposing Ideas & Evolving 🦾 Auto reports reading & implementation |
🩺 Medical | 🤖 Iteratively Proposing Ideas & Evolving |
- |
🏭 General | 🦾 Auto paper reading & implementation 🤖 Auto Kaggle Model Tuning |
🤖Auto Kaggle feature Engineering |
- RoadMap: Currently, we are working hard to add new features to the Kaggle scenario.
Different scenarios vary in entrance and configuration. Please check the detailed setup tutorial in the scenarios documents.
Here is a gallery of successful explorations (5 traces showed in 🖥️ Live Demo). You can download and view the execution trace using this command from the documentation.
Please refer to 📖readthedocs_scen for more details of the scenarios.
Automating the R&D process in data science is a highly valuable yet underexplored area in industry. We propose a framework to push the boundaries of this important research field.
The research questions within this framework can be divided into three main categories:
Research Area | Paper/Work List |
---|---|
Benchmark the R&D abilities | Benchmark |
Idea proposal: Explore new ideas or refine existing ones | Research |
Ability to realize ideas: Implement and execute ideas | Development |
We believe that the key to delivering high-quality solutions lies in the ability to evolve R&D capabilities. Agents should learn like human experts, continuously improving their R&D skills.
More documents can be found in the 📖 readthedocs.
@misc{chen2024datacentric,
title={Towards Data-Centric Automatic R&D},
author={Haotian Chen and Xinjie Shen and Zeqi Ye and Wenjun Feng and Haoxue Wang and Xiao Yang and Xu Yang and Weiqing Liu and Jiang Bian},
year={2024},
eprint={2404.11276},
archivePrefix={arXiv},
primaryClass={cs.AI}
}
In a data mining expert's daily research and development process, they propose a hypothesis (e.g., a model structure like RNN can capture patterns in time-series data), design experiments (e.g., finance data contains time-series and we can verify the hypothesis in this scenario), implement the experiment as code (e.g., Pytorch model structure), and then execute the code to get feedback (e.g., metrics, loss curve, etc.). The experts learn from the feedback and improve in the next iteration.
Based on the principles above, we have established a basic method framework that continuously proposes hypotheses, verifies them, and gets feedback from the real-world practice. This is the first scientific research automation framework that supports linking with real-world verification.
For more detail, please refer to our 🖥️ Live Demo page.
@misc{yang2024collaborative,
title={Collaborative Evolving Strategy for Automatic Data-Centric Development},
author={Xu Yang and Haotian Chen and Wenjun Feng and Haoxue Wang and Zeqi Ye and Xinjie Shen and Xiao Yang and Shizhao Sun and Weiqing Liu and Jiang Bian},
year={2024},
eprint={2407.18690},
archivePrefix={arXiv},
primaryClass={cs.AI}
}
We welcome contributions and suggestions to improve RD-Agent. Please refer to the Contributing Guide for more details on how to contribute.
Before submitting a pull request, ensure that your code passes the automatic CI checks.
This project welcomes contributions and suggestions. Contributing to this project is straightforward and rewarding. Whether it's solving an issue, addressing a bug, enhancing documentation, or even correcting a typo, every contribution is valuable and helps improve RDAgent.
To get started, you can explore the issues list, or search for TODO:
comments in the codebase by running the command grep -r "TODO:"
.
Before we released RD-Agent as an open-source project on GitHub, it was an internal project within our group. Unfortunately, the internal commit history was not preserved when we removed some confidential code. As a result, some contributions from our group members, including Haotian Chen, Wenjun Feng, Haoxue Wang, Zeqi Ye, Xinjie Shen, and Jinhui Li, were not included in the public commits.
The RD-agent is provided “as is”, without warranty of any kind, express or implied, including but not limited to the warranties of merchantability, fitness for a particular purpose and noninfringement. The RD-agent is aimed to facilitate research and development process in the financial industry and not ready-to-use for any financial investment or advice. Users shall independently assess and test the risks of the RD-agent in a specific use scenario, ensure the responsible use of AI technology, including but not limited to developing and integrating risk mitigation measures, and comply with all applicable laws and regulations in all applicable jurisdictions. The RD-agent does not provide financial opinions or reflect the opinions of Microsoft, nor is it designed to replace the role of qualified financial professionals in formulating, assessing, and approving finance products. The inputs and outputs of the RD-agent belong to the users and users shall assume all liability under any theory of liability, whether in contract, torts, regulatory, negligence, products liability, or otherwise, associated with use of the RD-agent and any inputs and outputs thereof.
For Tasks:
Click tags to check more tools for each tasksFor Jobs:
Alternative AI tools for RD-Agent
Similar Open Source Tools
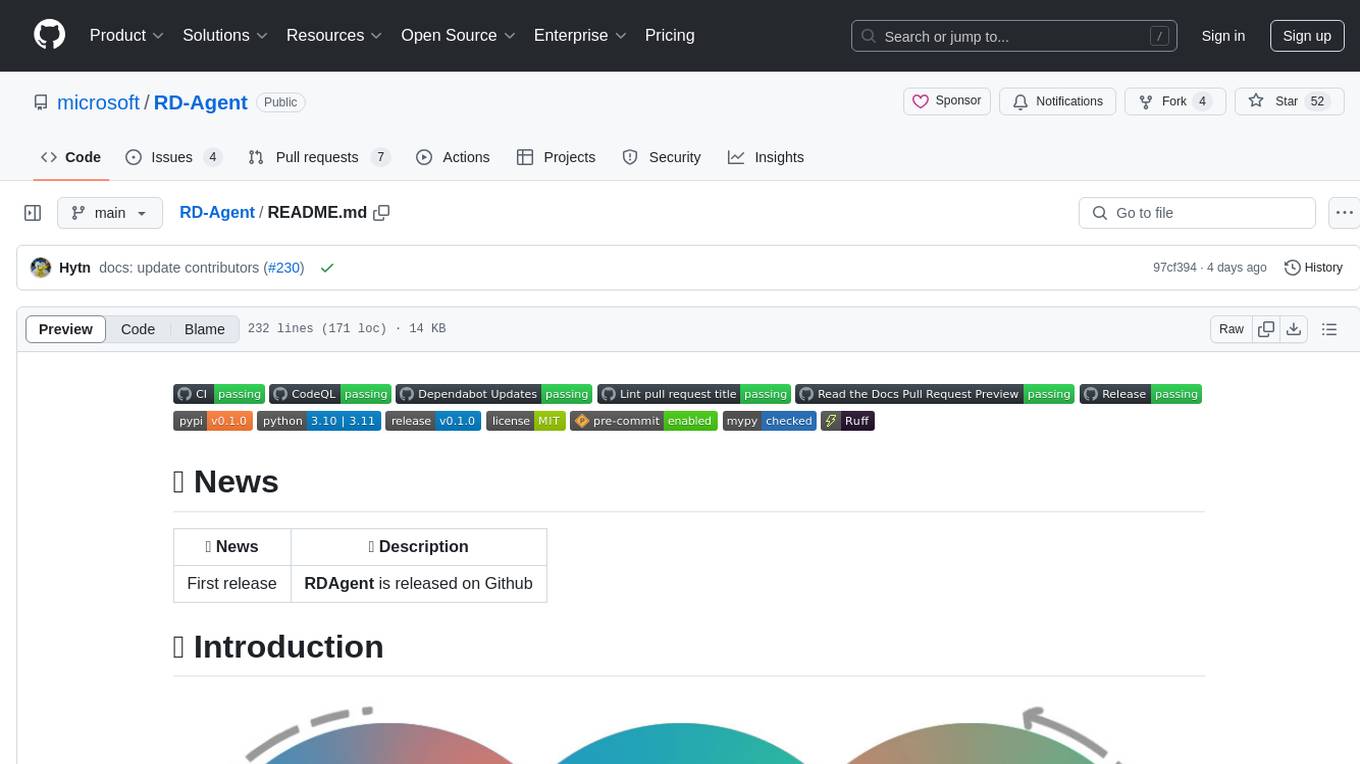
RD-Agent
RD-Agent is a tool designed to automate critical aspects of industrial R&D processes, focusing on data-driven scenarios to streamline model and data development. It aims to propose new ideas ('R') and implement them ('D') automatically, leading to solutions of significant industrial value. The tool supports scenarios like Automated Quantitative Trading, Data Mining Agent, Research Copilot, and more, with a framework to push the boundaries of research in data science. Users can create a Conda environment, install the RDAgent package from PyPI, configure GPT model, and run various applications for tasks like quantitative trading, model evolution, medical prediction, and more. The tool is intended to enhance R&D processes and boost productivity in industrial settings.
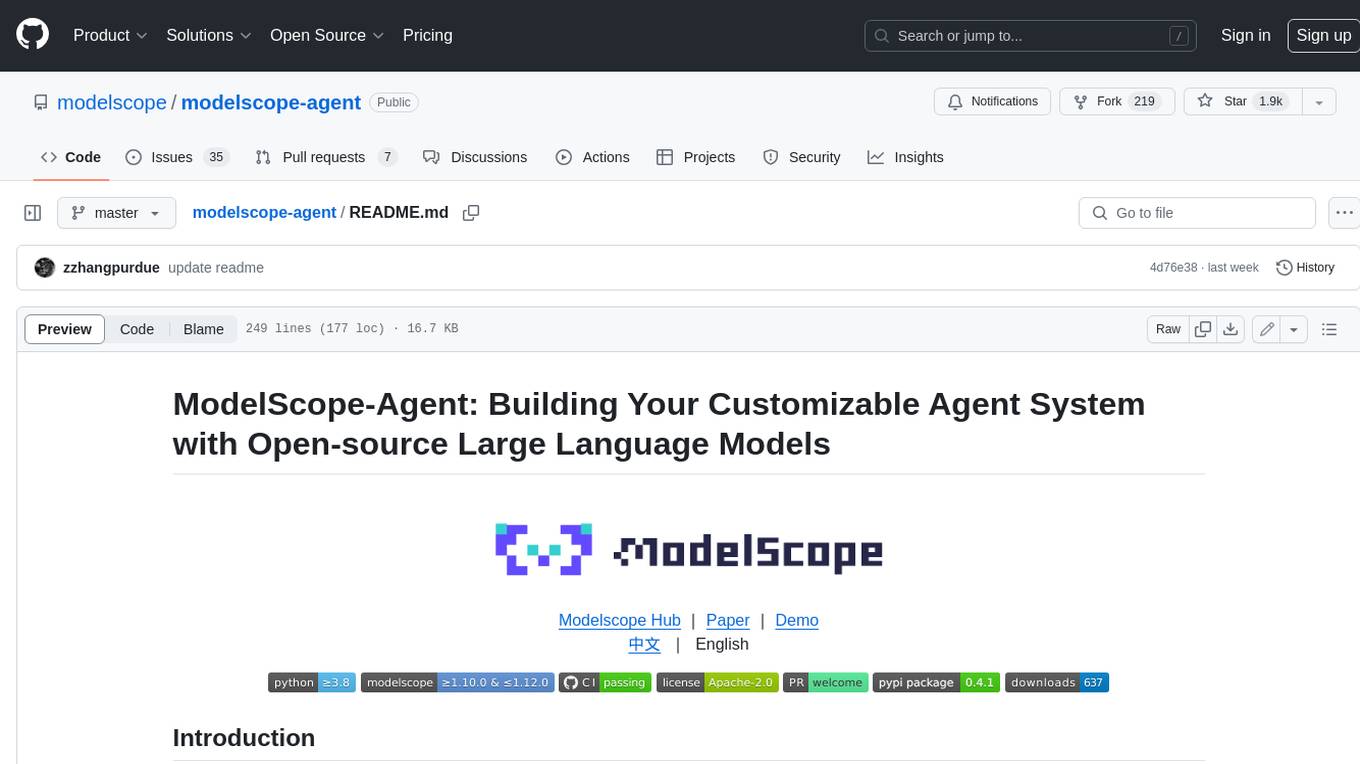
modelscope-agent
ModelScope-Agent is a customizable and scalable Agent framework. A single agent has abilities such as role-playing, LLM calling, tool usage, planning, and memory. It mainly has the following characteristics: - **Simple Agent Implementation Process**: Simply specify the role instruction, LLM name, and tool name list to implement an Agent application. The framework automatically arranges workflows for tool usage, planning, and memory. - **Rich models and tools**: The framework is equipped with rich LLM interfaces, such as Dashscope and Modelscope model interfaces, OpenAI model interfaces, etc. Built in rich tools, such as **code interpreter**, **weather query**, **text to image**, **web browsing**, etc., make it easy to customize exclusive agents. - **Unified interface and high scalability**: The framework has clear tools and LLM registration mechanism, making it convenient for users to expand more diverse Agent applications. - **Low coupling**: Developers can easily use built-in tools, LLM, memory, and other components without the need to bind higher-level agents.
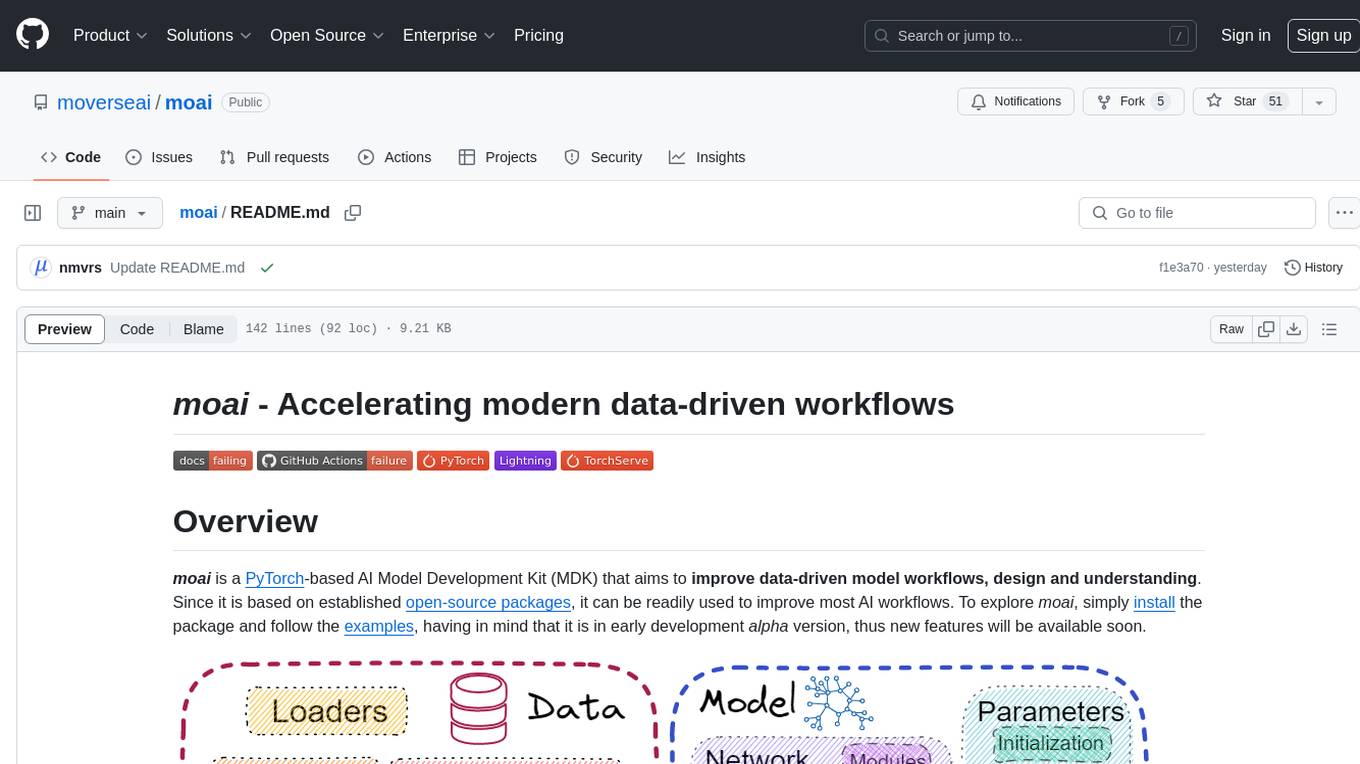
moai
moai is a PyTorch-based AI Model Development Kit (MDK) designed to improve data-driven model workflows, design, and understanding. It offers modularity via monads for model building blocks, reproducibility via configuration-based design, productivity via a data-driven domain modelling language (DML), extensibility via plugins, and understanding via inter-model performance and design aggregation. The tool provides specific integrated actions like play, train, evaluate, plot, diff, and reprod to support heavy data-driven workflows with analytics, knowledge extraction, and reproduction. moai relies on PyTorch, Lightning, Hydra, TorchServe, ONNX, Visdom, HiPlot, Kornia, Albumentations, and the wider open-source community for its functionalities.
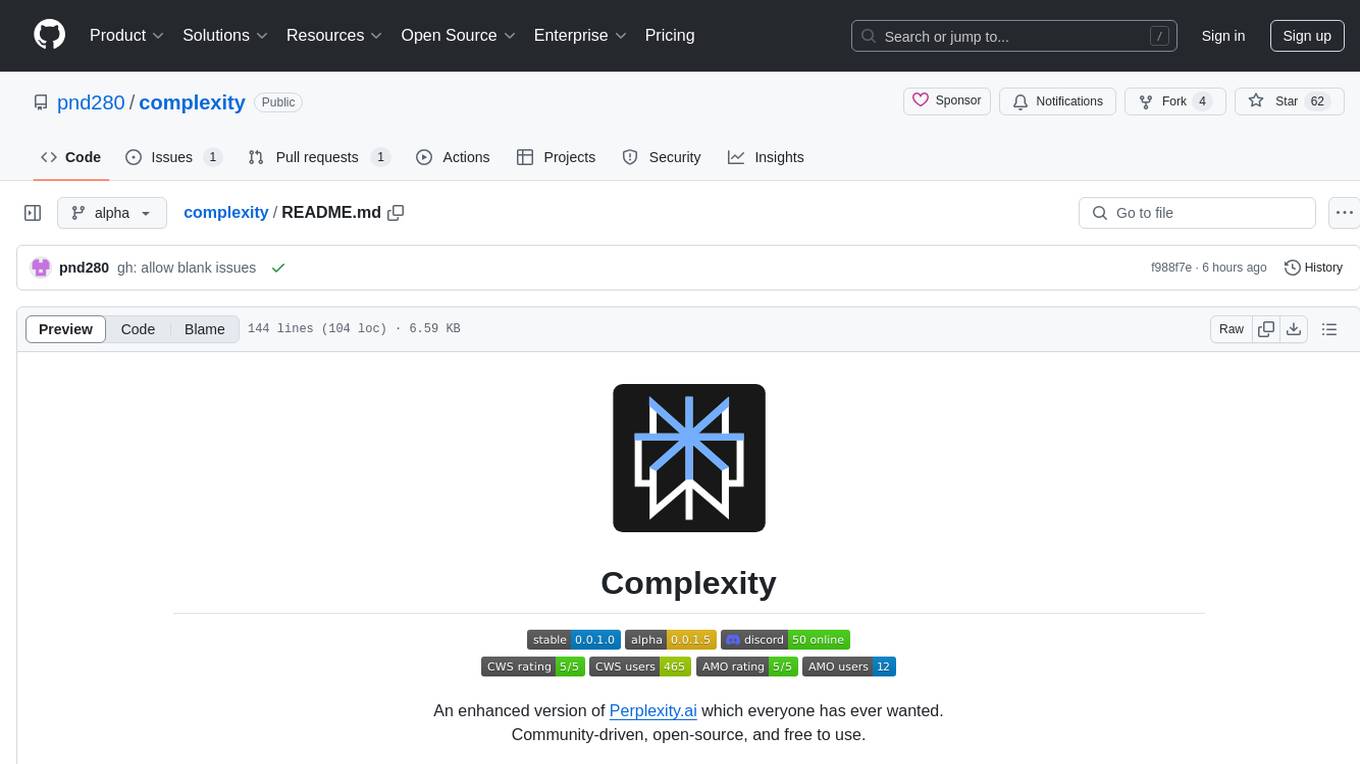
complexity
Complexity is a community-driven, open-source, and free third-party extension that enhances the features of Perplexity.ai. It provides various UI/UX/QoL tweaks, LLM/Image gen model selectors, a customizable theme, and a prompts library. The tool intercepts network traffic to alter the behavior of the host page, offering a solution to the limitations of Perplexity.ai. Users can install Complexity from Chrome Web Store, Mozilla Add-on, or build it from the source code.
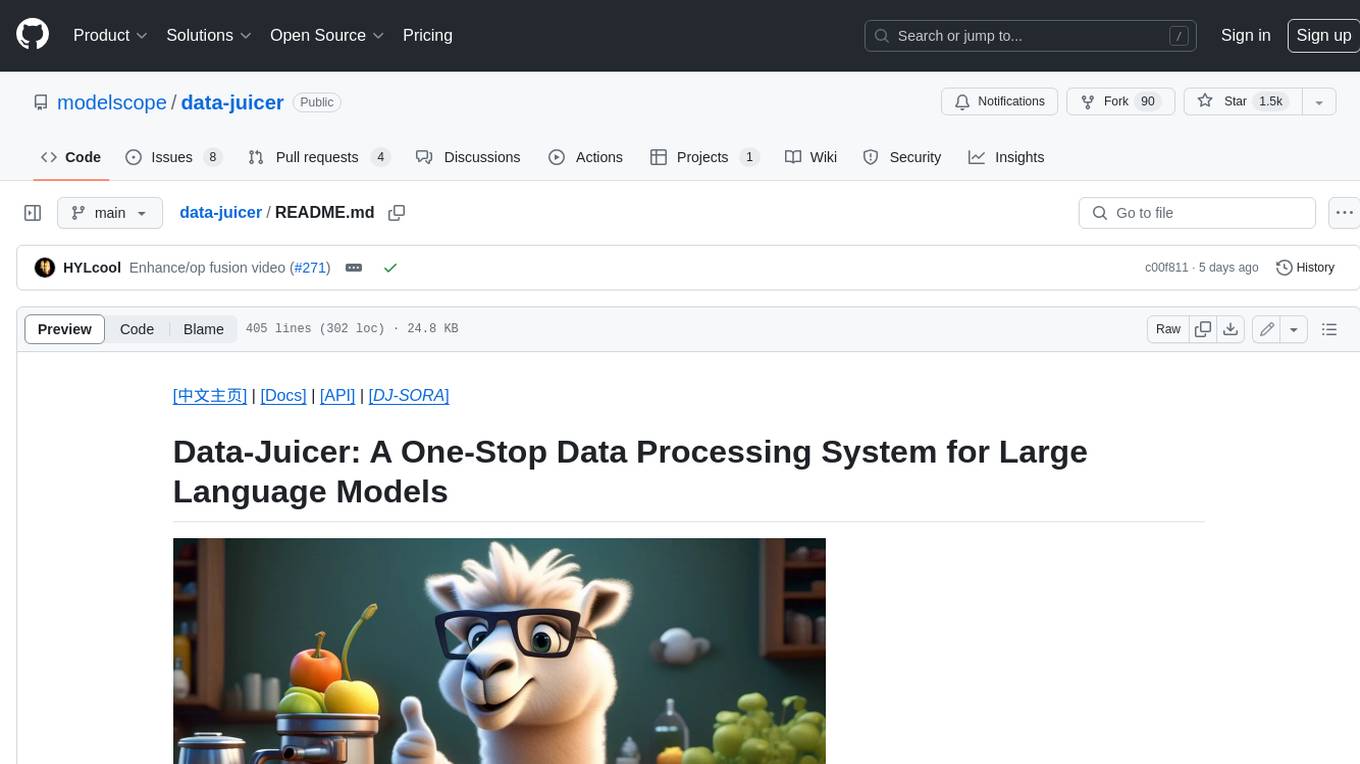
data-juicer
Data-Juicer is a one-stop data processing system to make data higher-quality, juicier, and more digestible for LLMs. It is a systematic & reusable library of 80+ core OPs, 20+ reusable config recipes, and 20+ feature-rich dedicated toolkits, designed to function independently of specific LLM datasets and processing pipelines. Data-Juicer allows detailed data analyses with an automated report generation feature for a deeper understanding of your dataset. Coupled with multi-dimension automatic evaluation capabilities, it supports a timely feedback loop at multiple stages in the LLM development process. Data-Juicer offers tens of pre-built data processing recipes for pre-training, fine-tuning, en, zh, and more scenarios. It provides a speedy data processing pipeline requiring less memory and CPU usage, optimized for maximum productivity. Data-Juicer is flexible & extensible, accommodating most types of data formats and allowing flexible combinations of OPs. It is designed for simplicity, with comprehensive documentation, easy start guides and demo configs, and intuitive configuration with simple adding/removing OPs from existing configs.
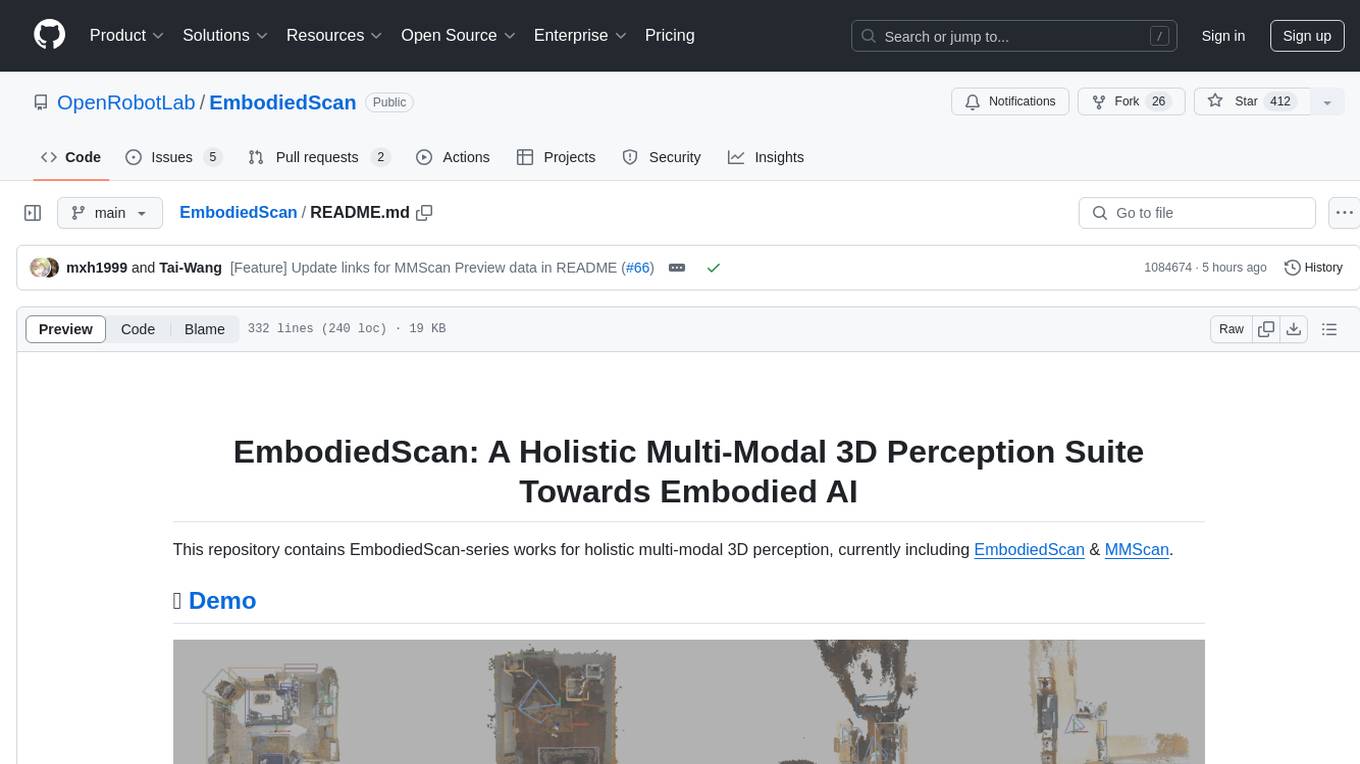
EmbodiedScan
EmbodiedScan is a holistic multi-modal 3D perception suite designed for embodied AI. It introduces a multi-modal, ego-centric 3D perception dataset and benchmark for holistic 3D scene understanding. The dataset includes over 5k scans with 1M ego-centric RGB-D views, 1M language prompts, 160k 3D-oriented boxes spanning 760 categories, and dense semantic occupancy with 80 common categories. The suite includes a baseline framework named Embodied Perceptron, capable of processing multi-modal inputs for 3D perception tasks and language-grounded tasks.
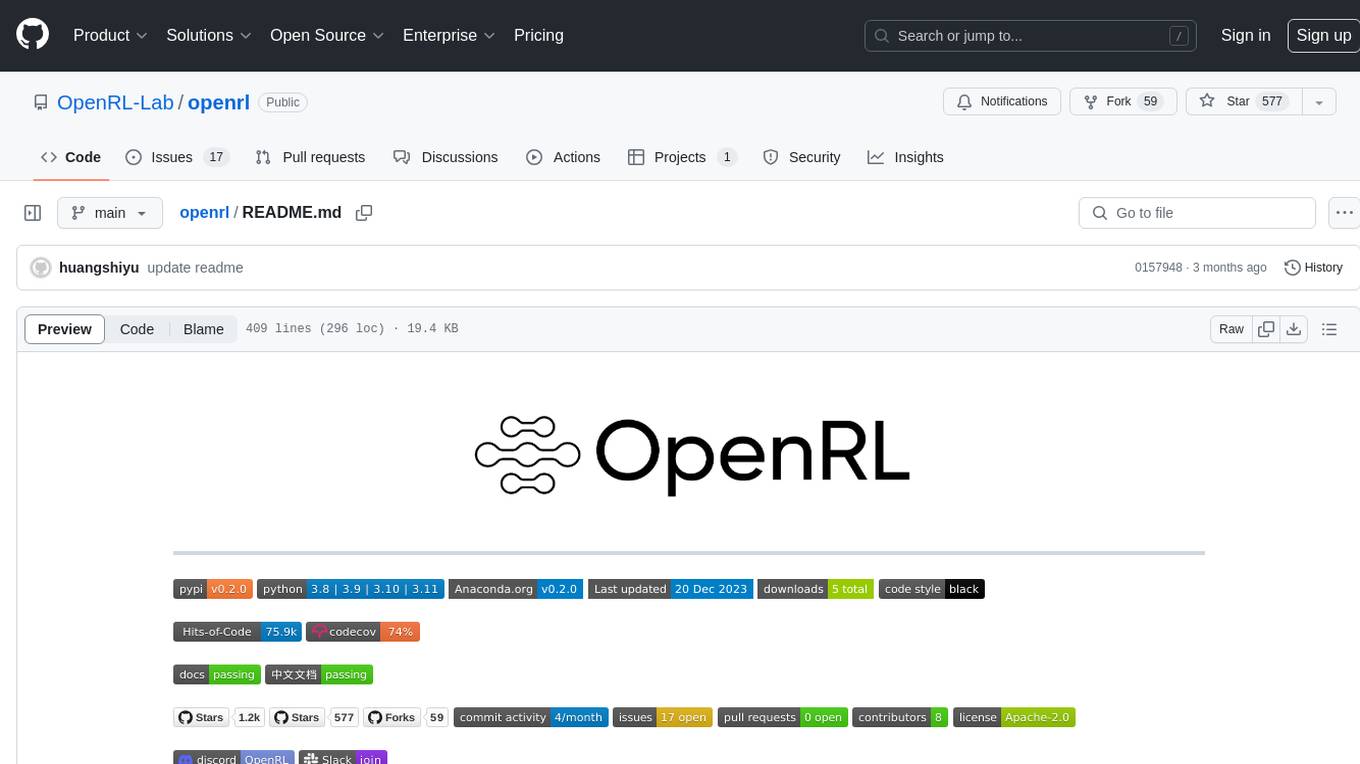
openrl
OpenRL is an open-source general reinforcement learning research framework that supports training for various tasks such as single-agent, multi-agent, offline RL, self-play, and natural language. Developed based on PyTorch, the goal of OpenRL is to provide a simple-to-use, flexible, efficient and sustainable platform for the reinforcement learning research community. It supports a universal interface for all tasks/environments, single-agent and multi-agent tasks, offline RL training with expert dataset, self-play training, reinforcement learning training for natural language tasks, DeepSpeed, Arena for evaluation, importing models and datasets from Hugging Face, user-defined environments, models, and datasets, gymnasium environments, callbacks, visualization tools, unit testing, and code coverage testing. It also supports various algorithms like PPO, DQN, SAC, and environments like Gymnasium, MuJoCo, Atari, and more.
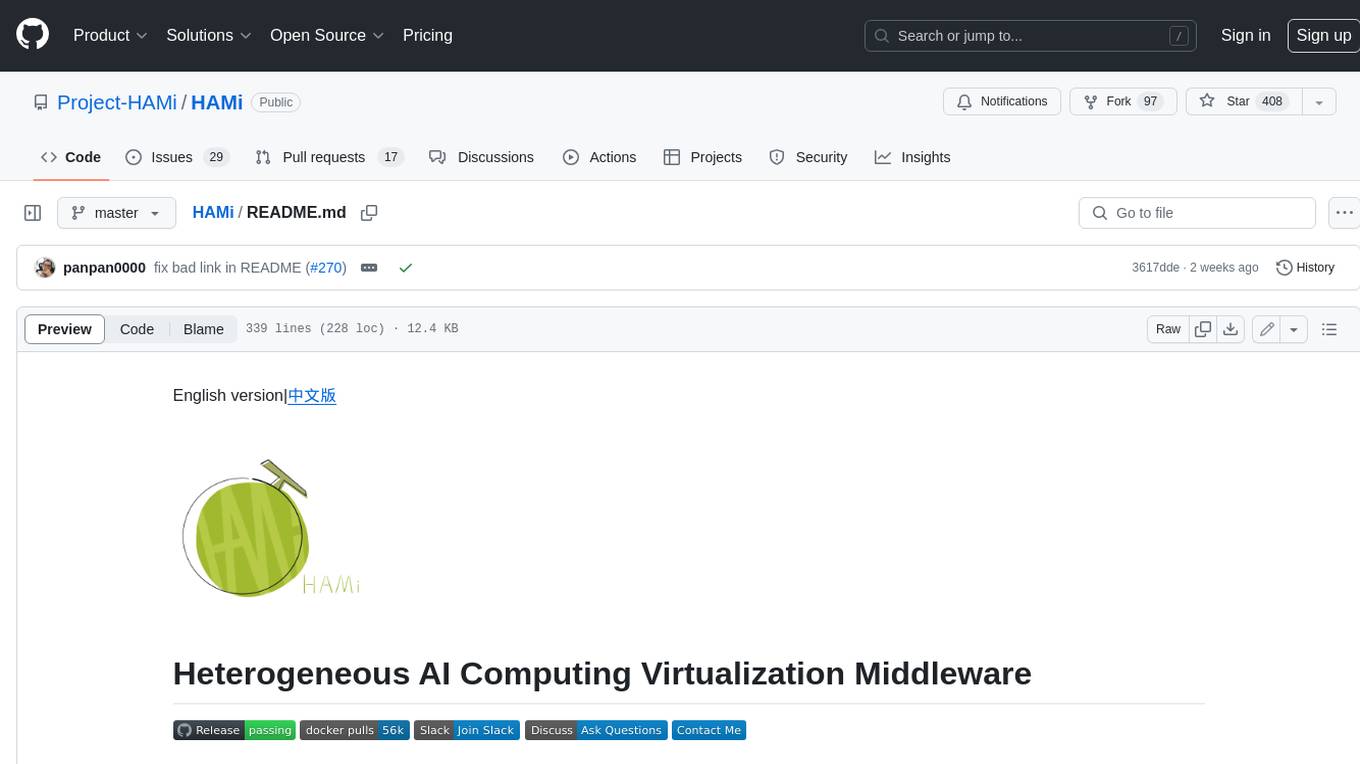
HAMi
HAMi is a Heterogeneous AI Computing Virtualization Middleware designed to manage Heterogeneous AI Computing Devices in a Kubernetes cluster. It allows for device sharing, device memory control, device type specification, and device UUID specification. The tool is easy to use and does not require modifying task YAML files. It includes features like hard limits on device memory, partial device allocation, streaming multiprocessor limits, and core usage specification. HAMi consists of components like a mutating webhook, scheduler extender, device plugins, and in-container virtualization techniques. It is suitable for scenarios requiring device sharing, specific device memory allocation, GPU balancing, low utilization optimization, and scenarios needing multiple small GPUs. The tool requires prerequisites like NVIDIA drivers, CUDA version, nvidia-docker, Kubernetes version, glibc version, and helm. Users can install, upgrade, and uninstall HAMi, submit tasks, and monitor cluster information. The tool's roadmap includes supporting additional AI computing devices, video codec processing, and Multi-Instance GPUs (MIG).
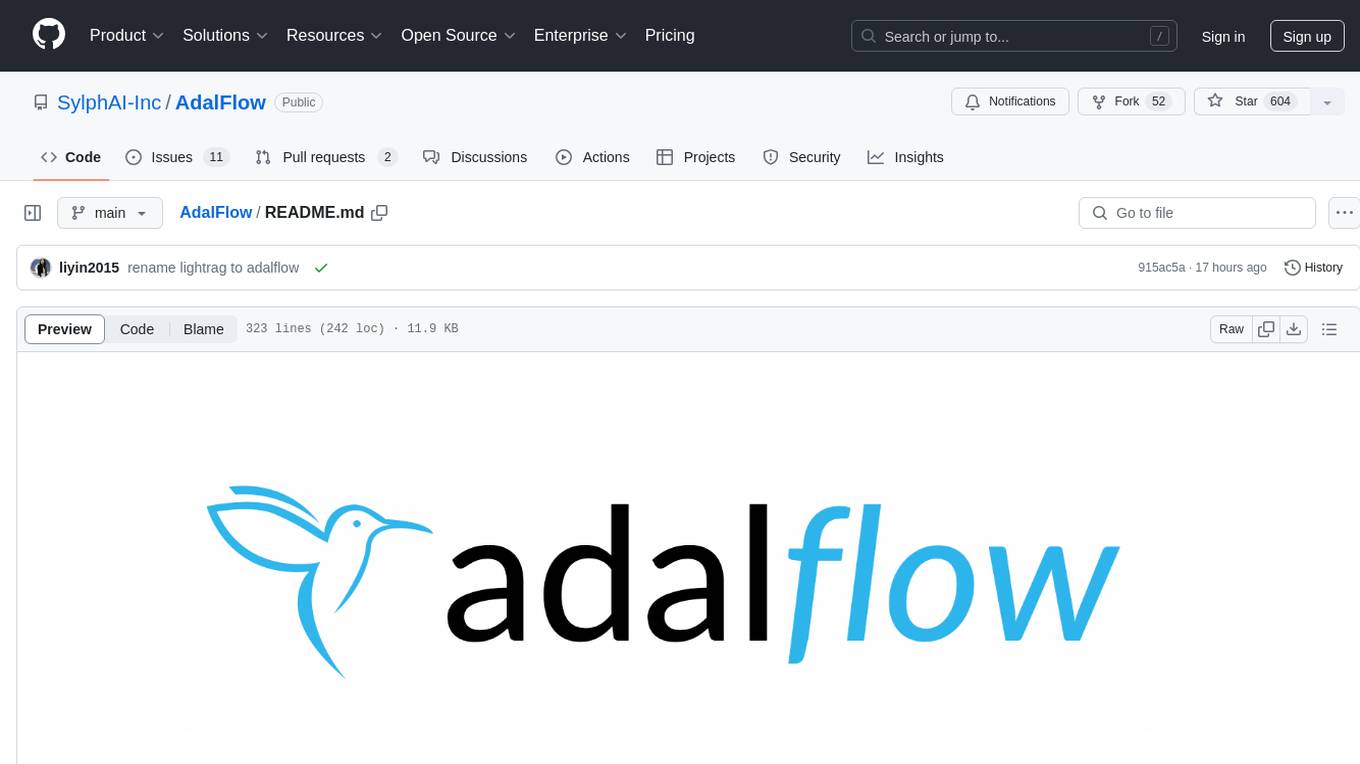
AdalFlow
AdalFlow is a library designed to help developers build and optimize Large Language Model (LLM) task pipelines. It follows a design pattern similar to PyTorch, offering a light, modular, and robust codebase. Named in honor of Ada Lovelace, AdalFlow aims to inspire more women to enter the AI field. The library is tailored for various GenAI applications like chatbots, translation, summarization, code generation, and autonomous agents, as well as classical NLP tasks such as text classification and named entity recognition. AdalFlow emphasizes modularity, robustness, and readability to support users in customizing and iterating code for their specific use cases.
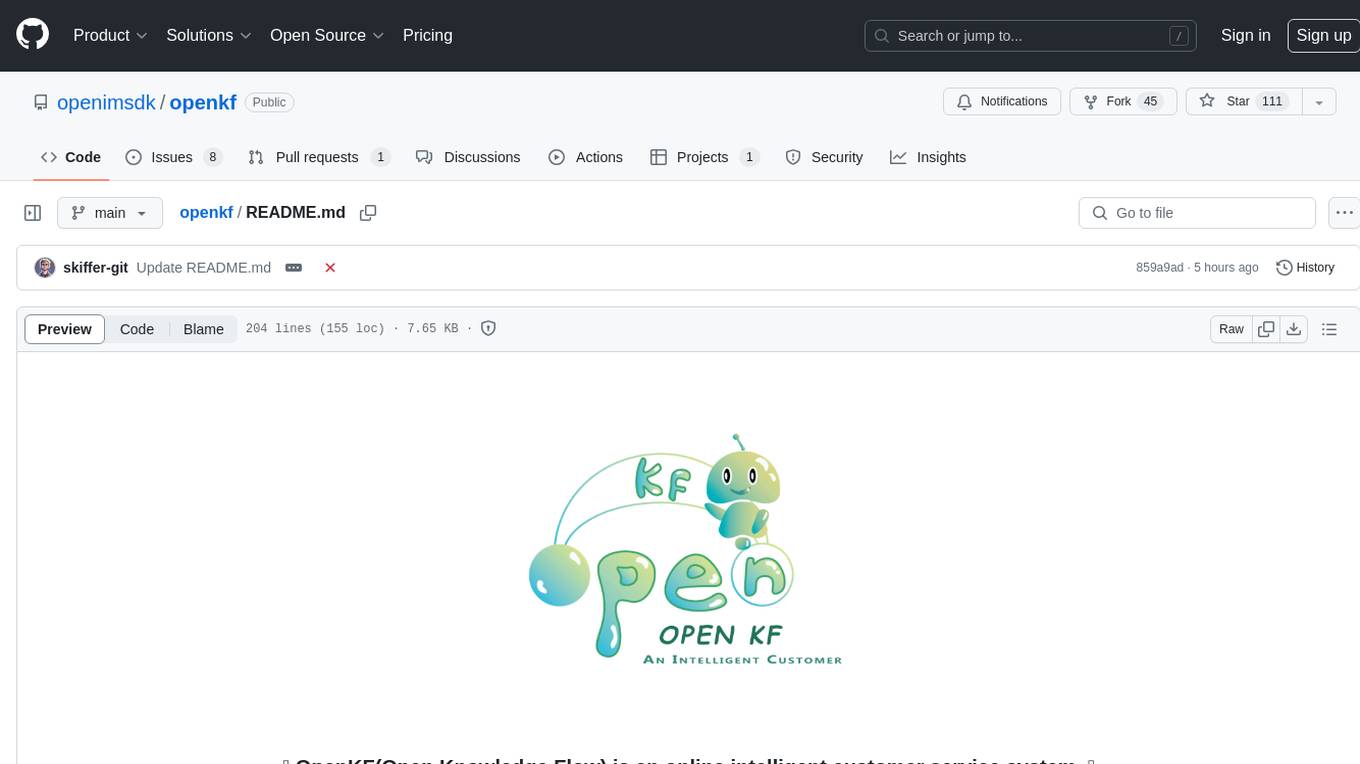
openkf
OpenKF (Open Knowledge Flow) is an online intelligent customer service system. It is an open-source customer service system based on OpenIM, supporting LLM (Local Knowledgebase) customer service and multi-channel customer service. It is easy to integrate with third-party systems, deploy, and perform secondary development. The system provides features like login page, config page, dashboard page, platform page, and session page. Users can quickly get started with OpenKF by following the installation and run instructions. The architecture follows MVC design with a standardized directory structure. The community encourages involvement through community meetings, contributions, and development. OpenKF is licensed under the Apache 2.0 license.
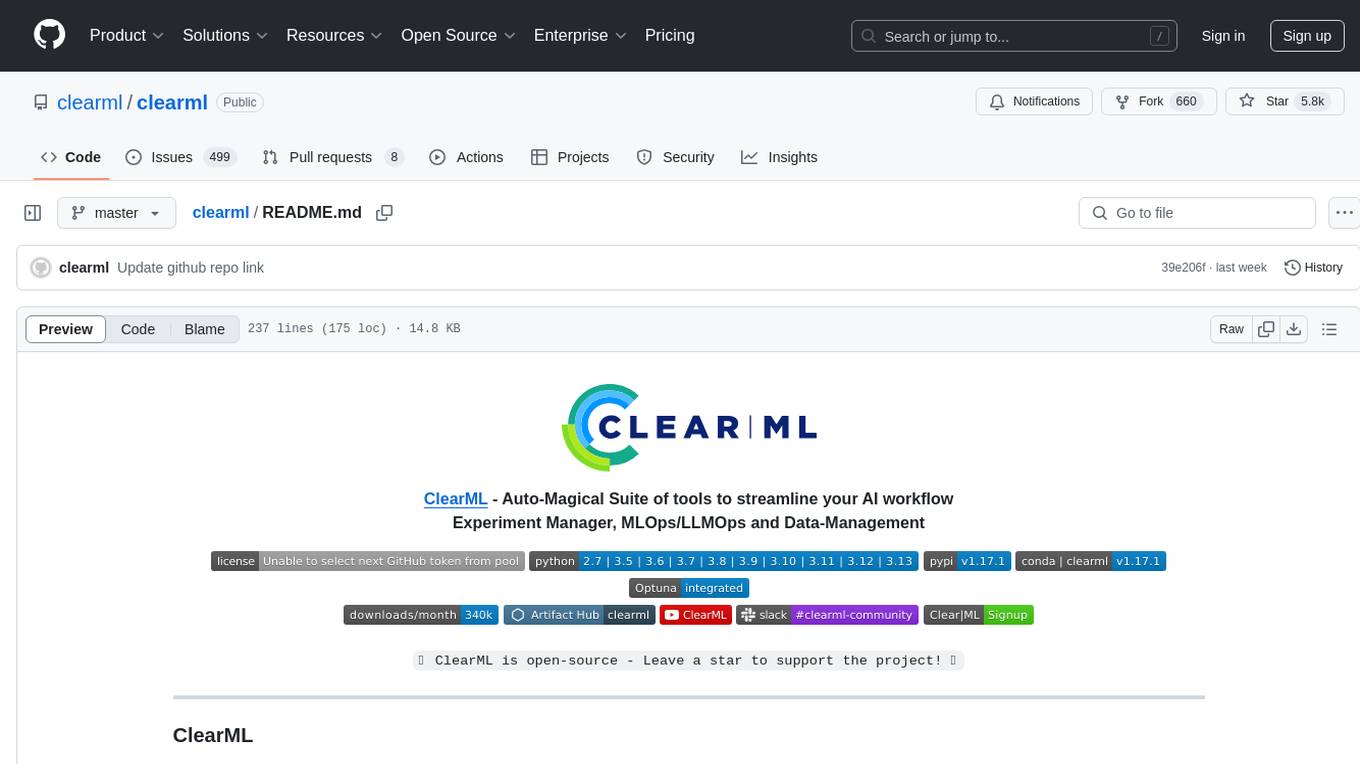
clearml
ClearML is an auto-magical suite of tools designed to streamline AI workflows. It includes modules for experiment management, MLOps/LLMOps, data management, model serving, and more. ClearML offers features like experiment tracking, model serving, orchestration, and automation. It supports various ML/DL frameworks and integrates with Jupyter Notebook and PyCharm for remote debugging. ClearML aims to simplify collaboration, automate processes, and enhance visibility in AI projects.
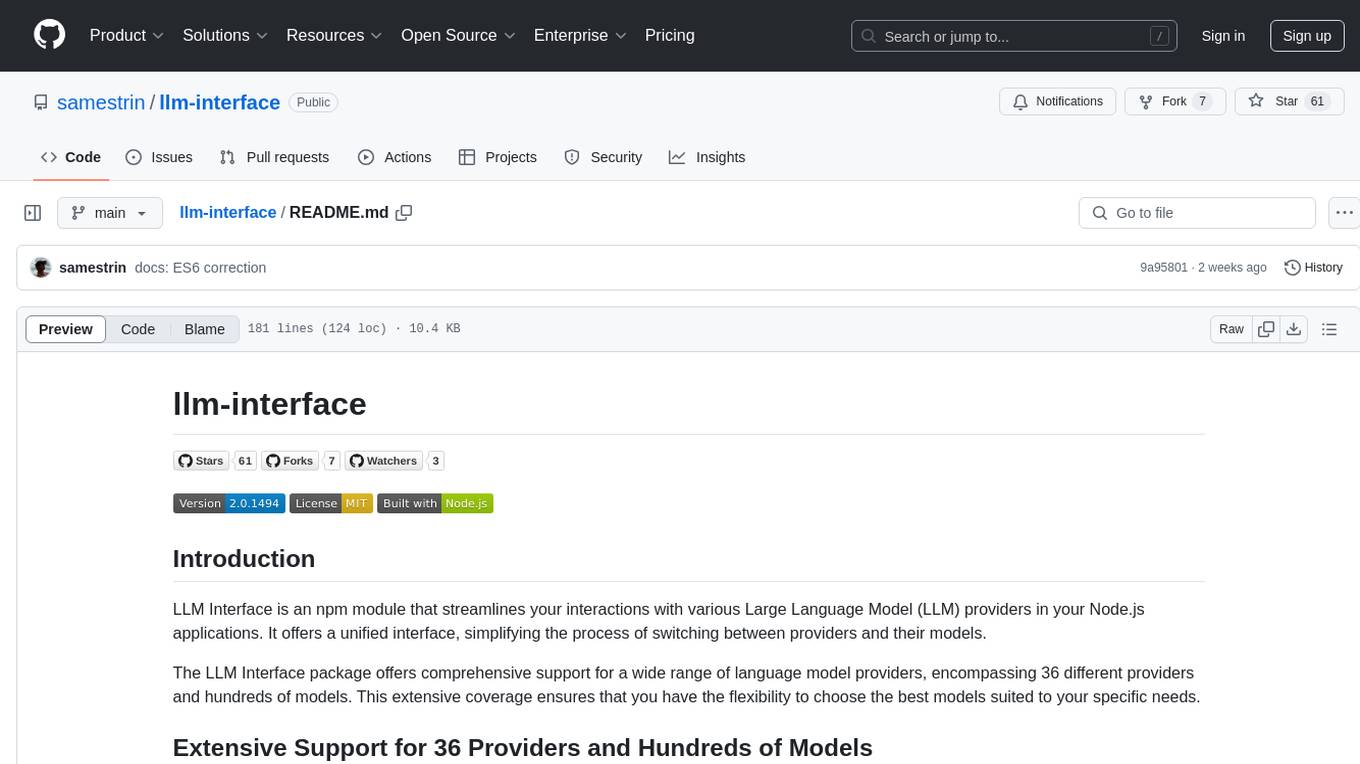
llm-interface
LLM Interface is an npm module that streamlines interactions with various Large Language Model (LLM) providers in Node.js applications. It offers a unified interface for switching between providers and models, supporting 36 providers and hundreds of models. Features include chat completion, streaming, error handling, extensibility, response caching, retries, JSON output, and repair. The package relies on npm packages like axios, @google/generative-ai, dotenv, jsonrepair, and loglevel. Installation is done via npm, and usage involves sending prompts to LLM providers. Tests can be run using npm test. Contributions are welcome under the MIT License.
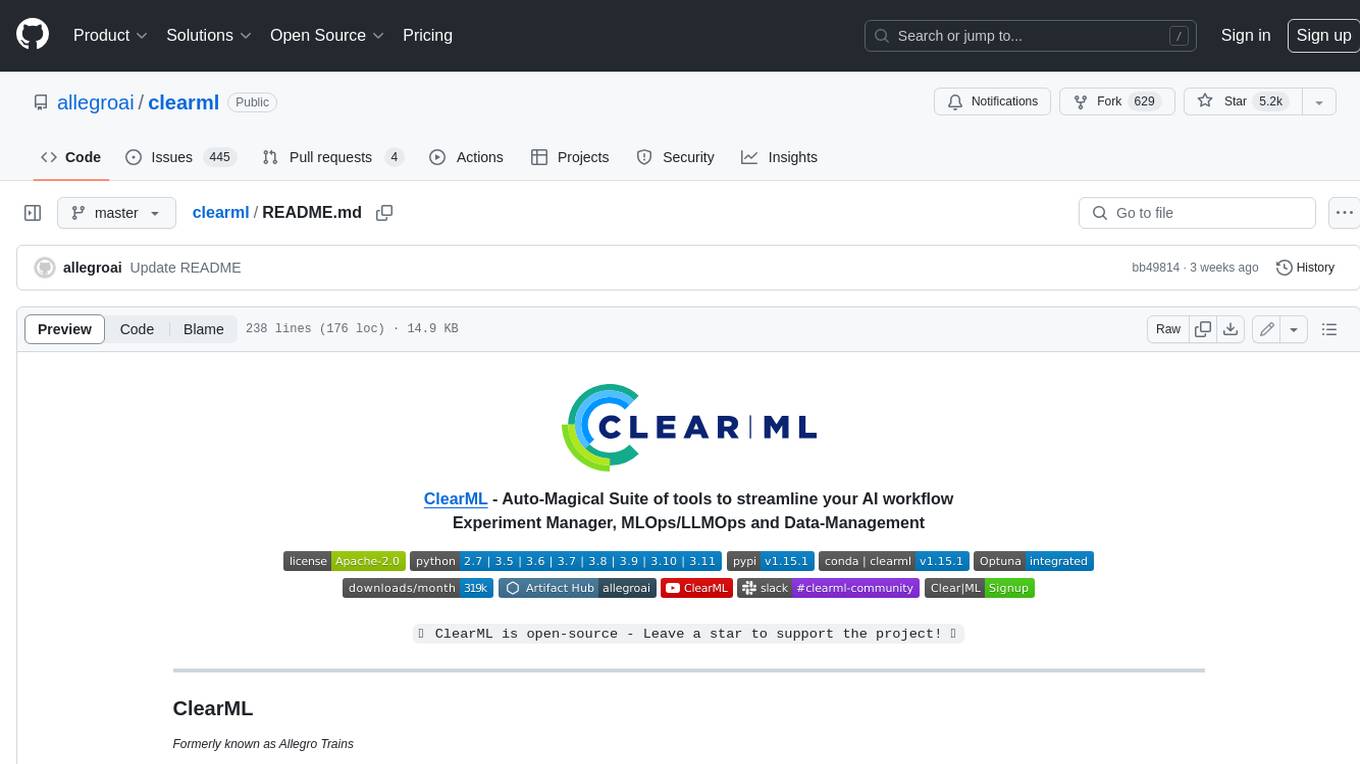
clearml
ClearML is a suite of tools designed to streamline the machine learning workflow. It includes an experiment manager, MLOps/LLMOps, data management, and model serving capabilities. ClearML is open-source and offers a free tier hosting option. It supports various ML/DL frameworks and integrates with Jupyter Notebook and PyCharm. ClearML provides extensive logging capabilities, including source control info, execution environment, hyper-parameters, and experiment outputs. It also offers automation features, such as remote job execution and pipeline creation. ClearML is designed to be easy to integrate, requiring only two lines of code to add to existing scripts. It aims to improve collaboration, visibility, and data transparency within ML teams.
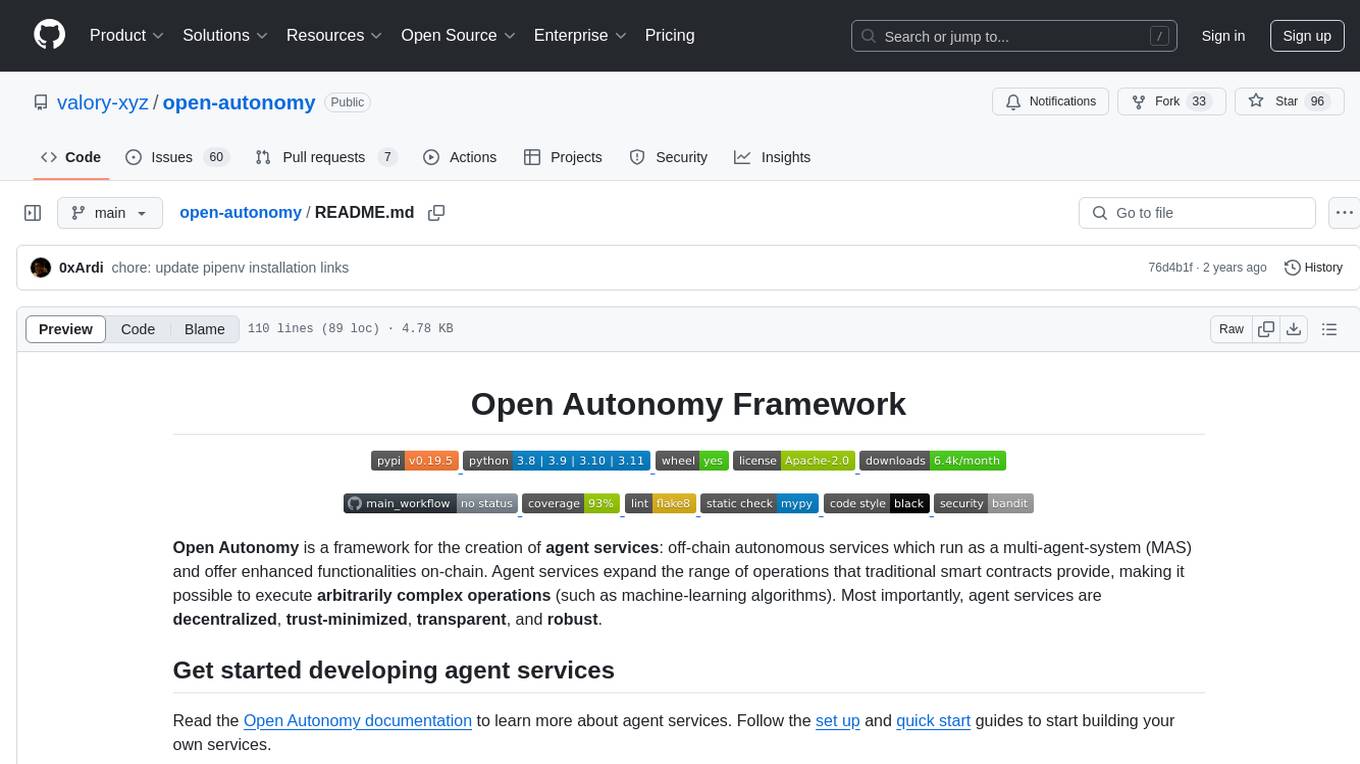
open-autonomy
Open Autonomy is a framework for creating agent services that run as a multi-agent-system and offer enhanced functionalities on-chain. It enables executing complex operations like machine-learning algorithms in a decentralized, trust-minimized, transparent, and robust manner.
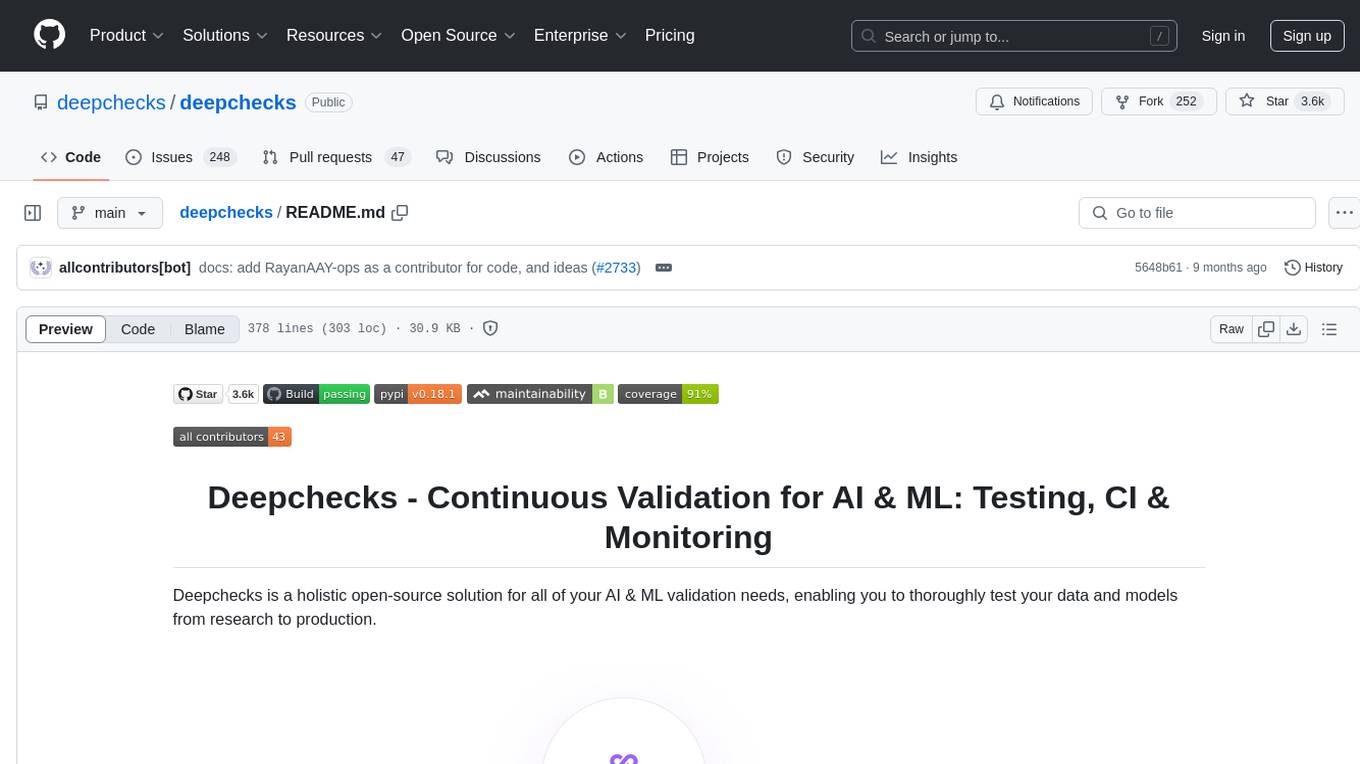
deepchecks
Deepchecks is a holistic open-source solution for AI & ML validation needs, enabling thorough testing of data and models from research to production. It includes components for testing, CI & testing management, and monitoring. Users can install and use Deepchecks for testing and monitoring their AI models, with customizable checks and suites for tabular, NLP, and computer vision data. The tool provides visual reports, pythonic/json output for processing, and a dynamic UI for collaboration and monitoring. Deepchecks is open source, with premium features available under a commercial license for monitoring components.
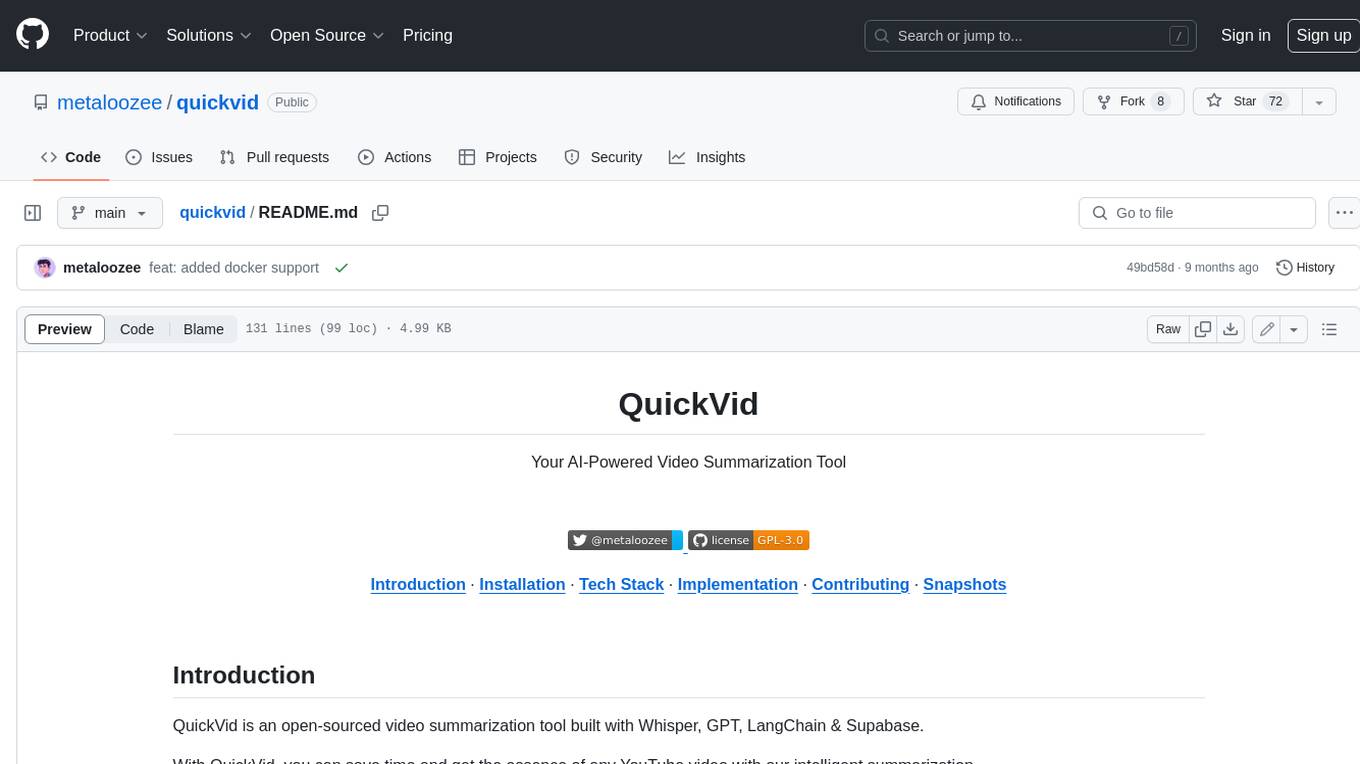
quickvid
QuickVid is an open-source video summarization tool that uses AI to generate summaries of YouTube videos. It is built with Whisper, GPT, LangChain, and Supabase. QuickVid can be used to save time and get the essence of any YouTube video with intelligent summarization.
For similar tasks
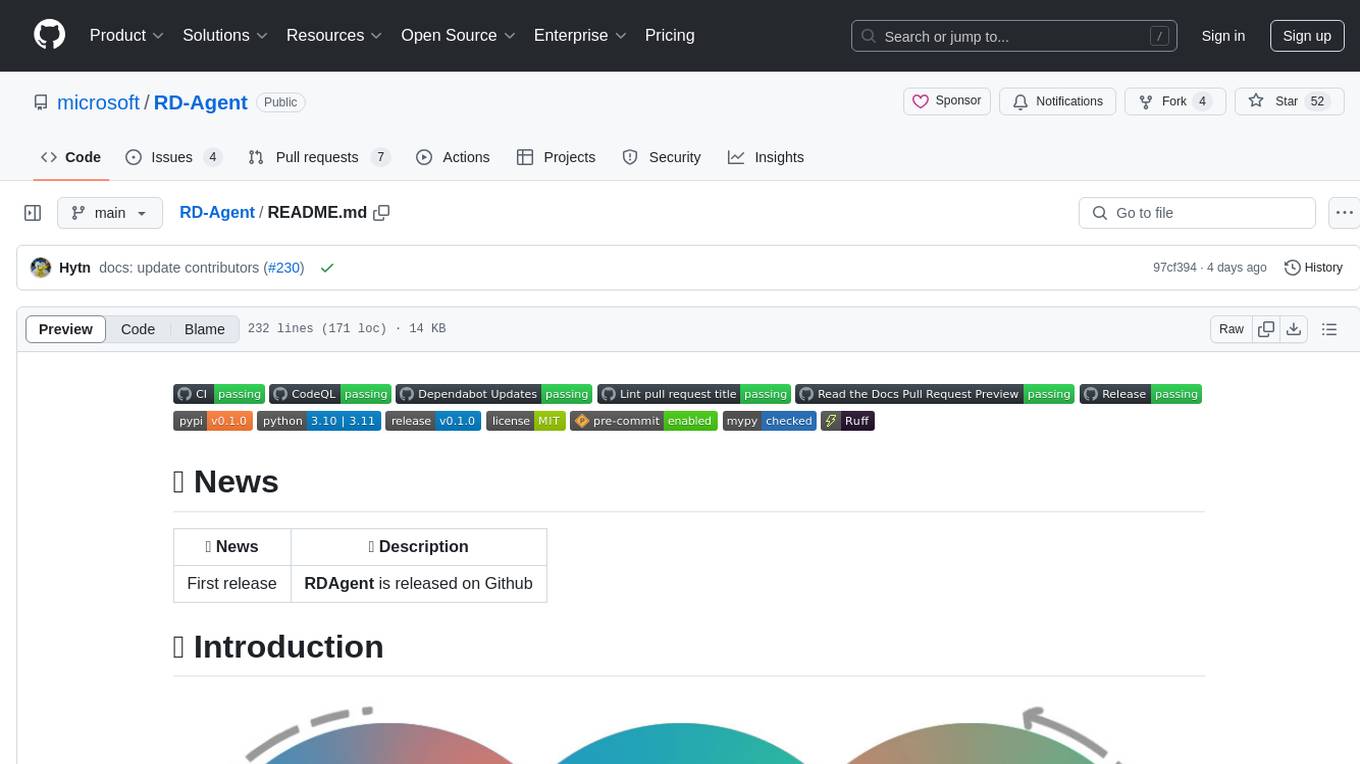
RD-Agent
RD-Agent is a tool designed to automate critical aspects of industrial R&D processes, focusing on data-driven scenarios to streamline model and data development. It aims to propose new ideas ('R') and implement them ('D') automatically, leading to solutions of significant industrial value. The tool supports scenarios like Automated Quantitative Trading, Data Mining Agent, Research Copilot, and more, with a framework to push the boundaries of research in data science. Users can create a Conda environment, install the RDAgent package from PyPI, configure GPT model, and run various applications for tasks like quantitative trading, model evolution, medical prediction, and more. The tool is intended to enhance R&D processes and boost productivity in industrial settings.
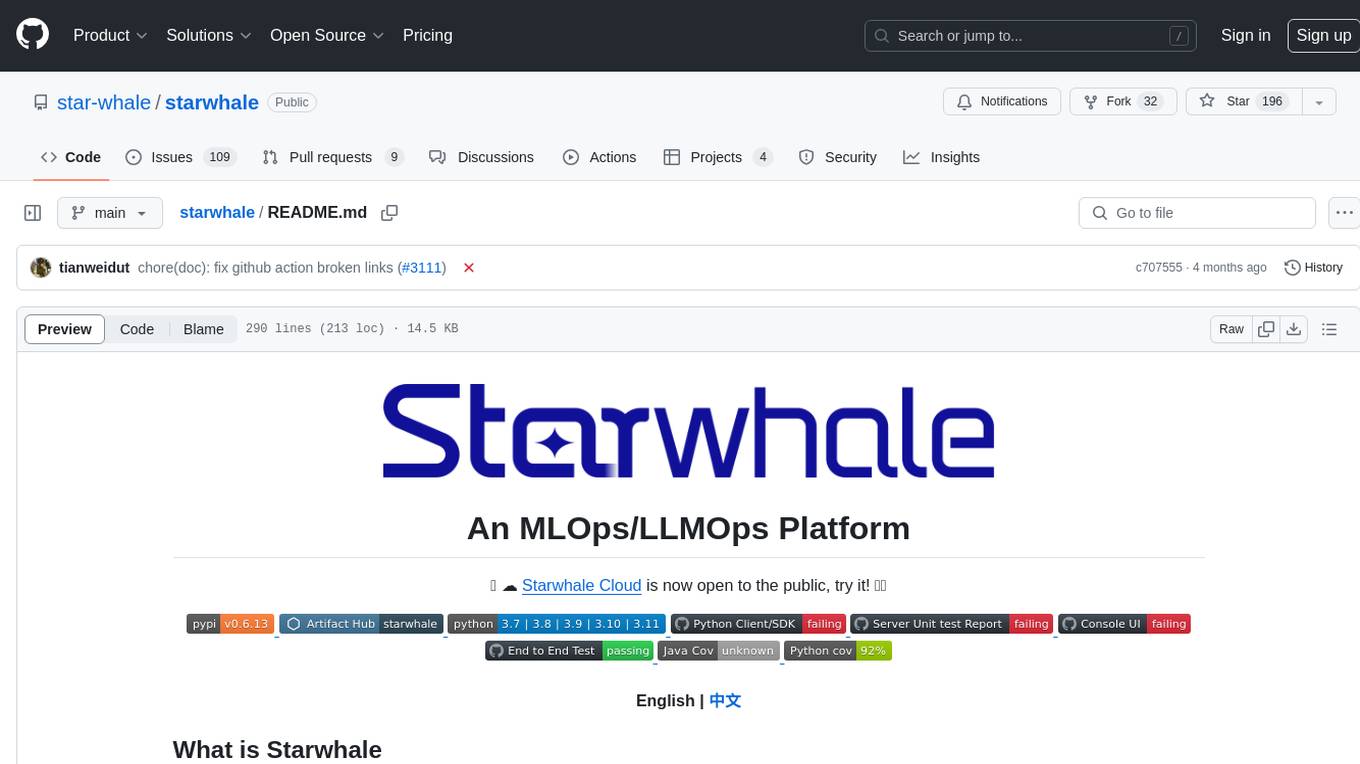
starwhale
Starwhale is an MLOps/LLMOps platform that brings efficiency and standardization to machine learning operations. It streamlines the model development lifecycle, enabling teams to optimize workflows around key areas like model building, evaluation, release, and fine-tuning. Starwhale abstracts Model, Runtime, and Dataset as first-class citizens, providing tailored capabilities for common workflow scenarios including Models Evaluation, Live Demo, and LLM Fine-tuning. It is an open-source platform designed for clarity and ease of use, empowering developers to build customized MLOps features tailored to their needs.
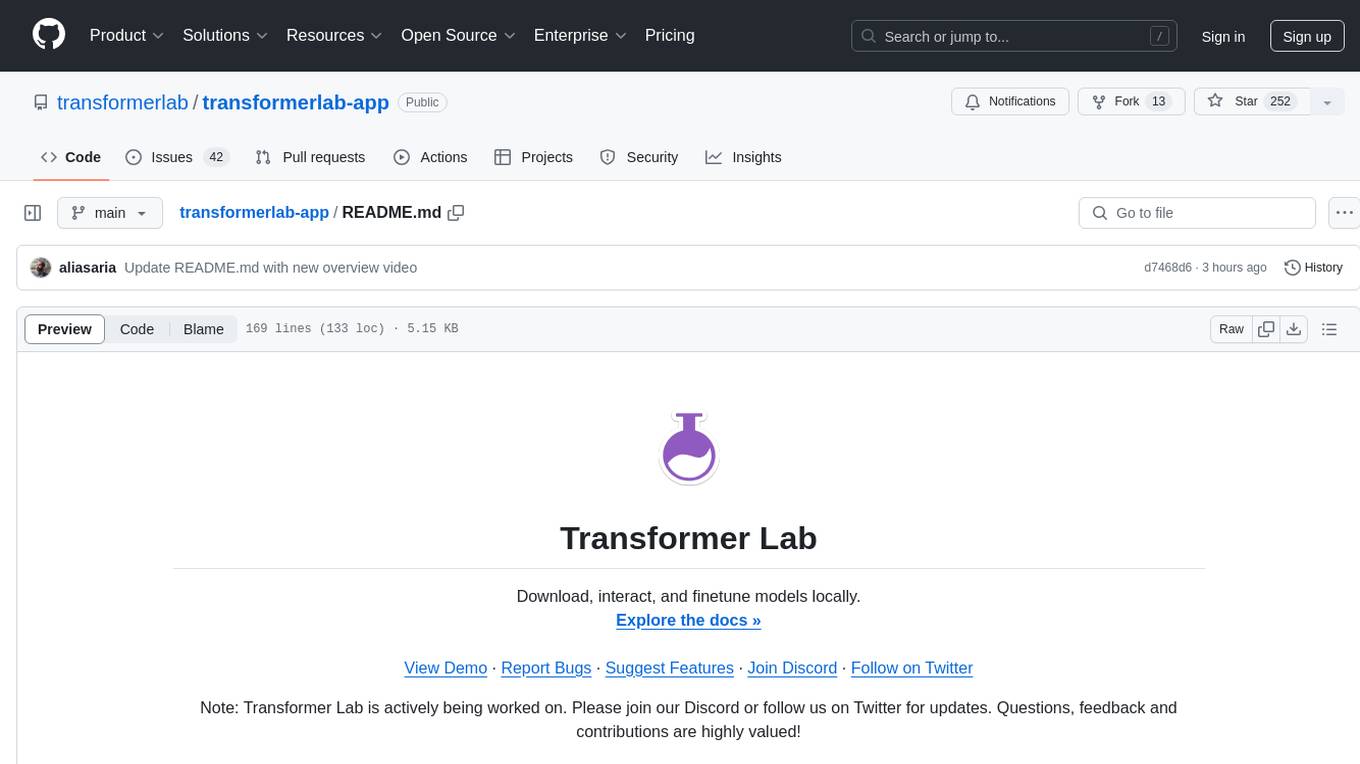
transformerlab-app
Transformer Lab is an app that allows users to experiment with Large Language Models by providing features such as one-click download of popular models, finetuning across different hardware, RLHF and Preference Optimization, working with LLMs across different operating systems, chatting with models, using different inference engines, evaluating models, building datasets for training, calculating embeddings, providing a full REST API, running in the cloud, converting models across platforms, supporting plugins, embedded Monaco code editor, prompt editing, inference logs, all through a simple cross-platform GUI.
For similar jobs
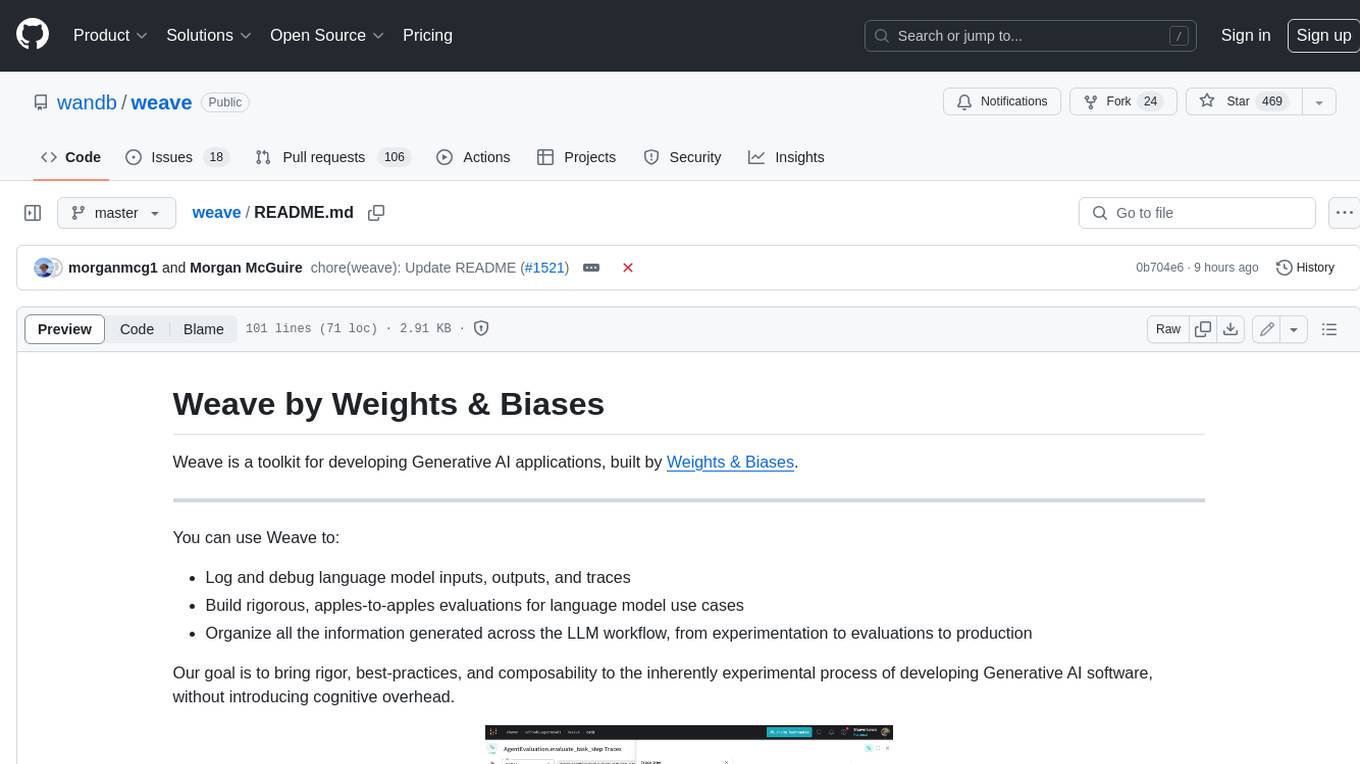
weave
Weave is a toolkit for developing Generative AI applications, built by Weights & Biases. With Weave, you can log and debug language model inputs, outputs, and traces; build rigorous, apples-to-apples evaluations for language model use cases; and organize all the information generated across the LLM workflow, from experimentation to evaluations to production. Weave aims to bring rigor, best-practices, and composability to the inherently experimental process of developing Generative AI software, without introducing cognitive overhead.
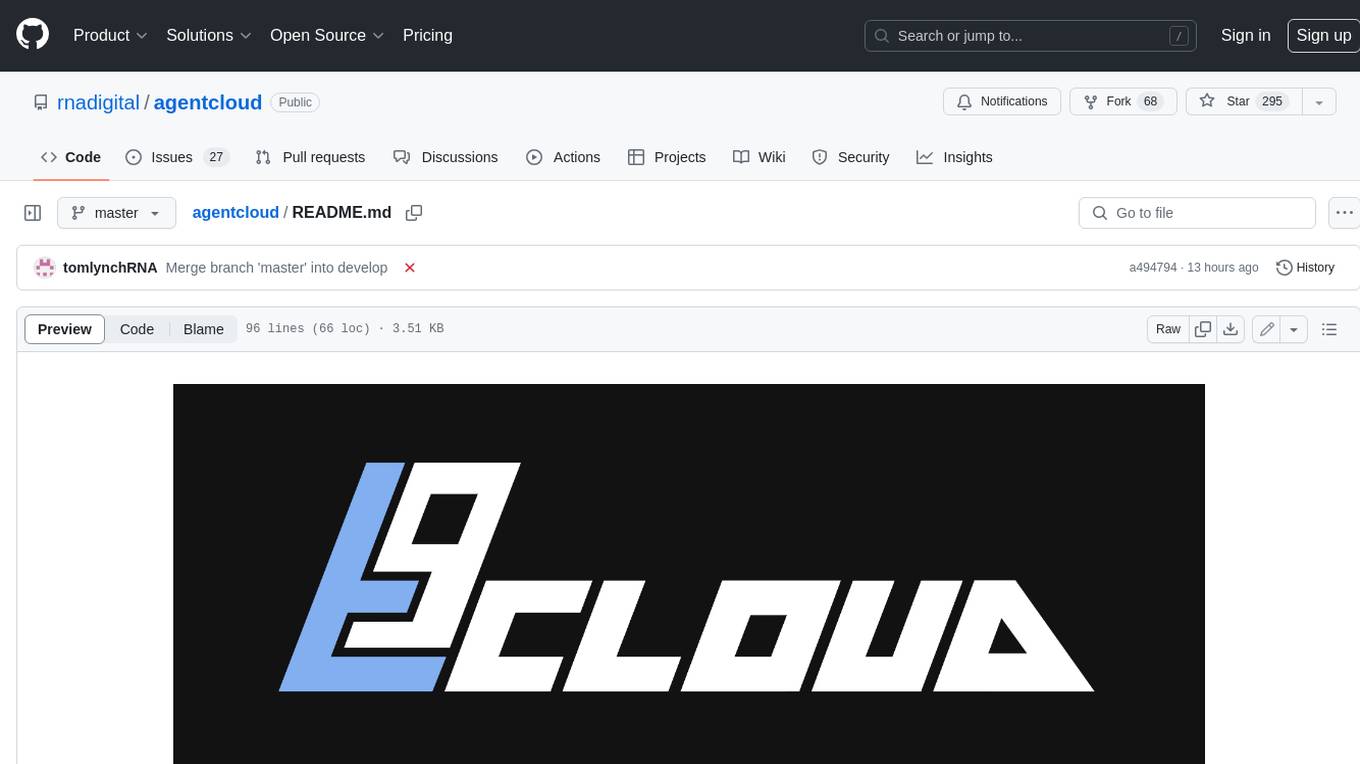
agentcloud
AgentCloud is an open-source platform that enables companies to build and deploy private LLM chat apps, empowering teams to securely interact with their data. It comprises three main components: Agent Backend, Webapp, and Vector Proxy. To run this project locally, clone the repository, install Docker, and start the services. The project is licensed under the GNU Affero General Public License, version 3 only. Contributions and feedback are welcome from the community.
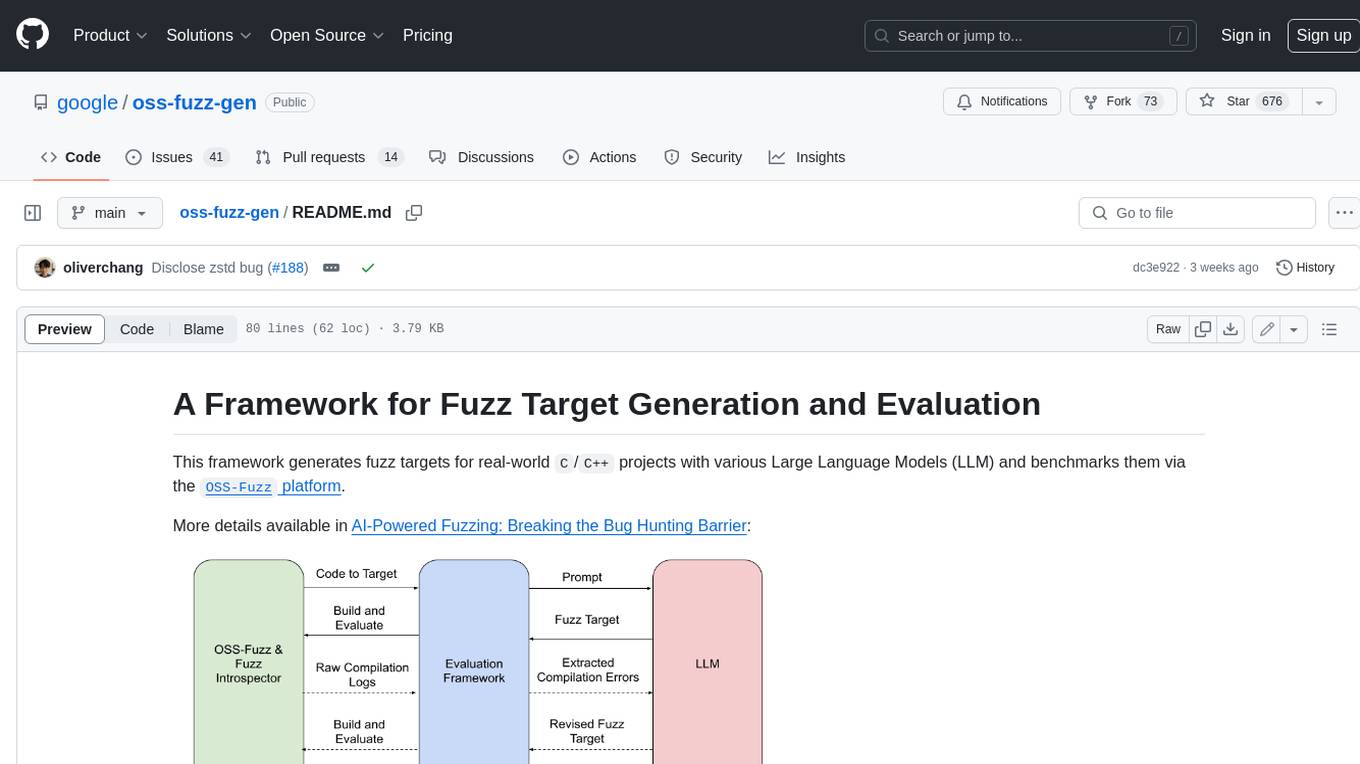
oss-fuzz-gen
This framework generates fuzz targets for real-world `C`/`C++` projects with various Large Language Models (LLM) and benchmarks them via the `OSS-Fuzz` platform. It manages to successfully leverage LLMs to generate valid fuzz targets (which generate non-zero coverage increase) for 160 C/C++ projects. The maximum line coverage increase is 29% from the existing human-written targets.
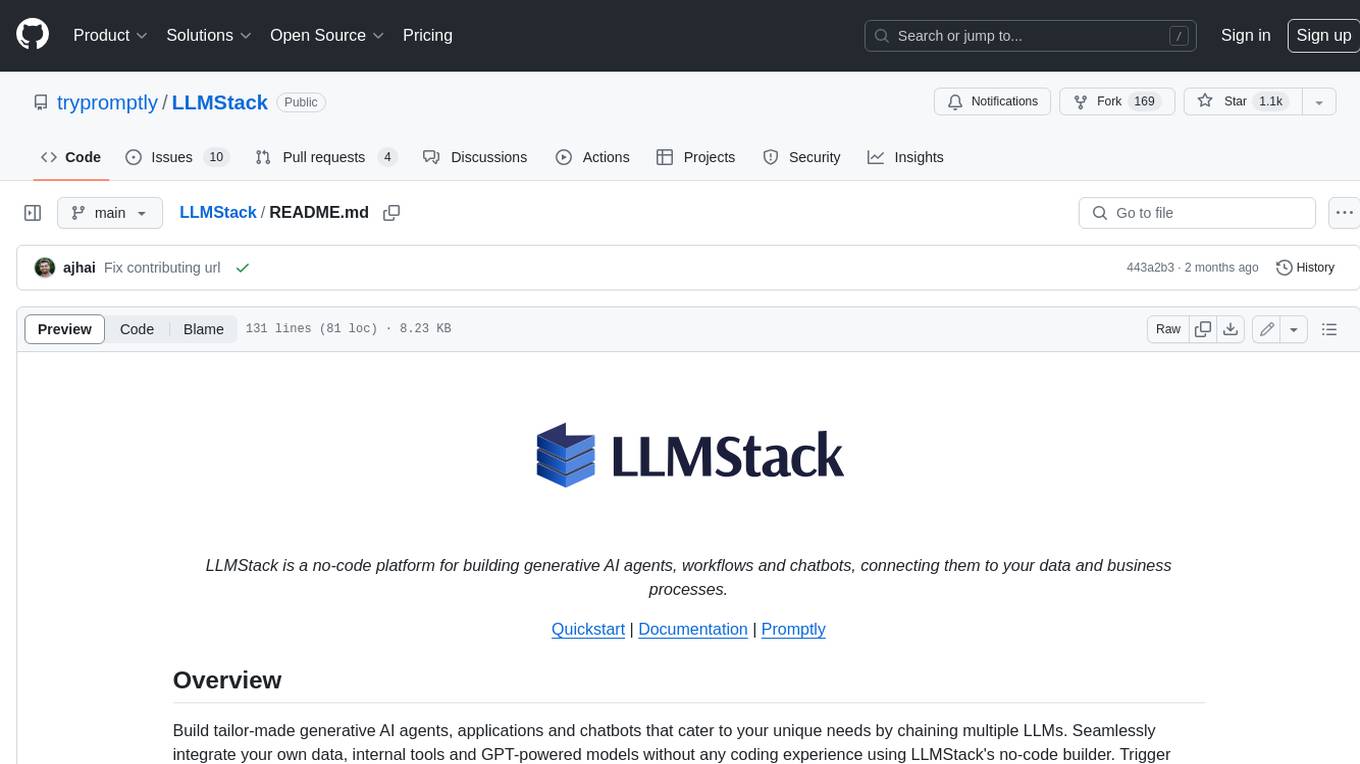
LLMStack
LLMStack is a no-code platform for building generative AI agents, workflows, and chatbots. It allows users to connect their own data, internal tools, and GPT-powered models without any coding experience. LLMStack can be deployed to the cloud or on-premise and can be accessed via HTTP API or triggered from Slack or Discord.
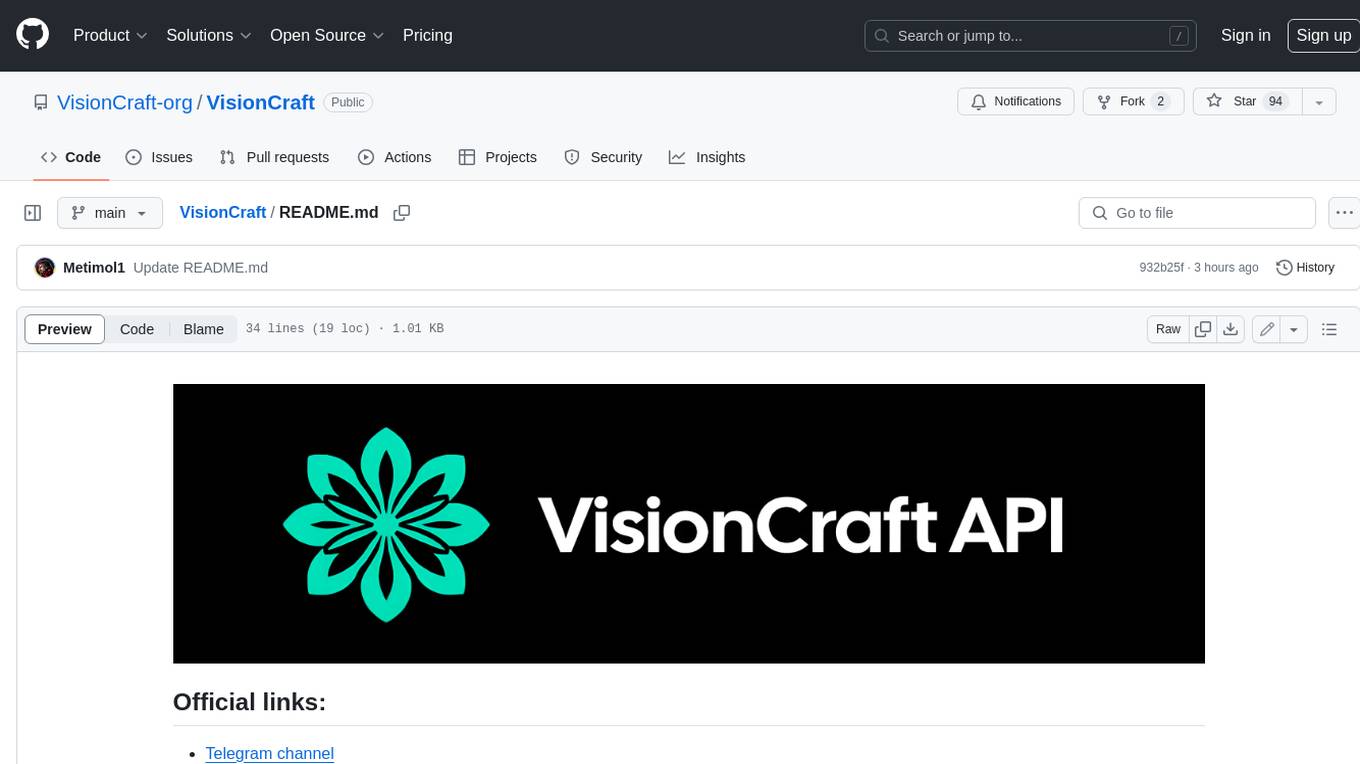
VisionCraft
The VisionCraft API is a free API for using over 100 different AI models. From images to sound.
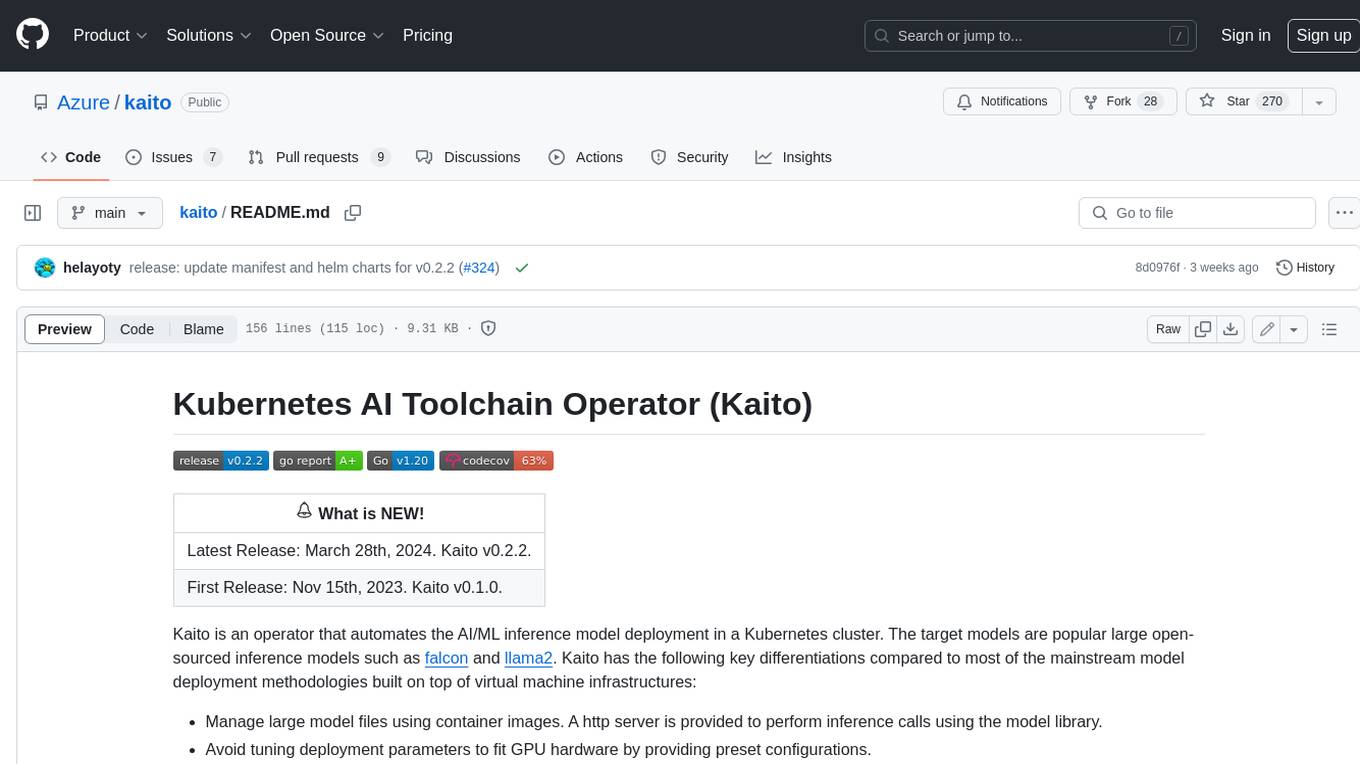
kaito
Kaito is an operator that automates the AI/ML inference model deployment in a Kubernetes cluster. It manages large model files using container images, avoids tuning deployment parameters to fit GPU hardware by providing preset configurations, auto-provisions GPU nodes based on model requirements, and hosts large model images in the public Microsoft Container Registry (MCR) if the license allows. Using Kaito, the workflow of onboarding large AI inference models in Kubernetes is largely simplified.
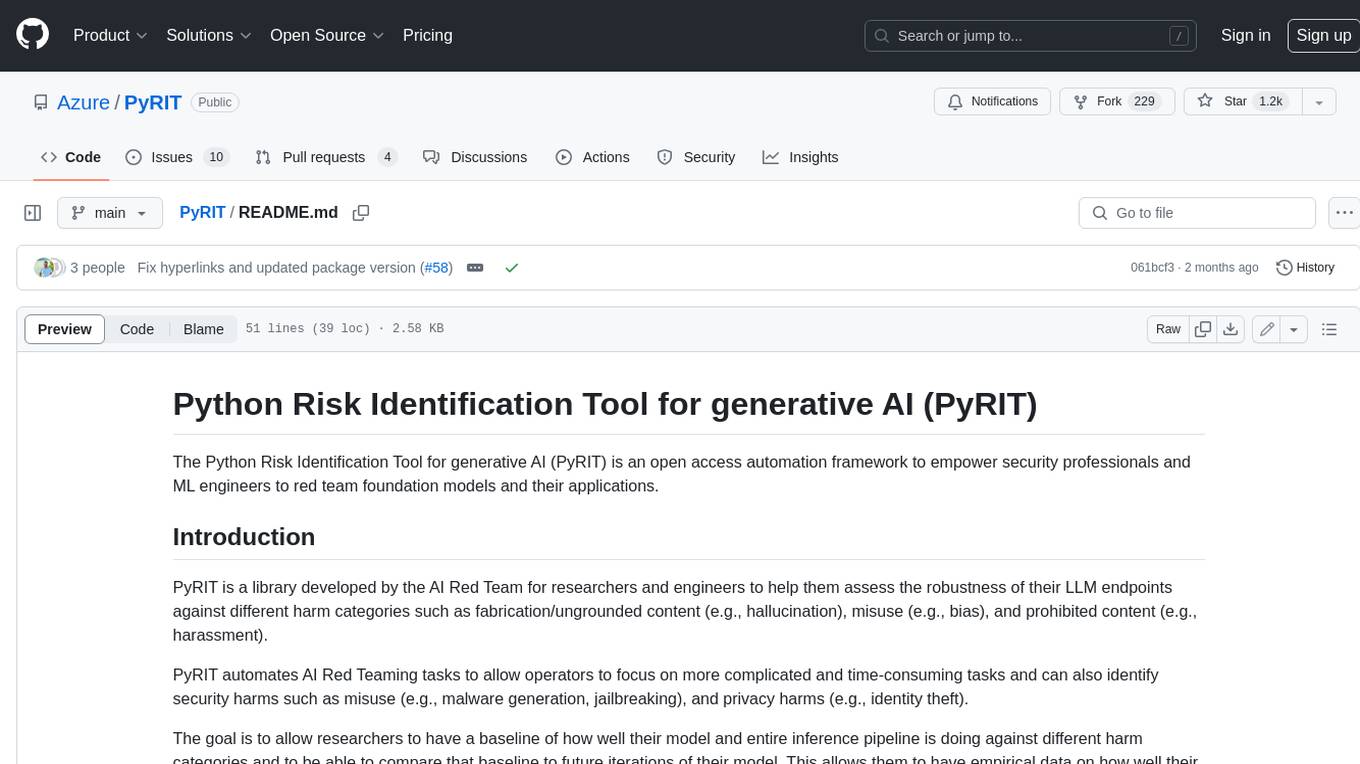
PyRIT
PyRIT is an open access automation framework designed to empower security professionals and ML engineers to red team foundation models and their applications. It automates AI Red Teaming tasks to allow operators to focus on more complicated and time-consuming tasks and can also identify security harms such as misuse (e.g., malware generation, jailbreaking), and privacy harms (e.g., identity theft). The goal is to allow researchers to have a baseline of how well their model and entire inference pipeline is doing against different harm categories and to be able to compare that baseline to future iterations of their model. This allows them to have empirical data on how well their model is doing today, and detect any degradation of performance based on future improvements.
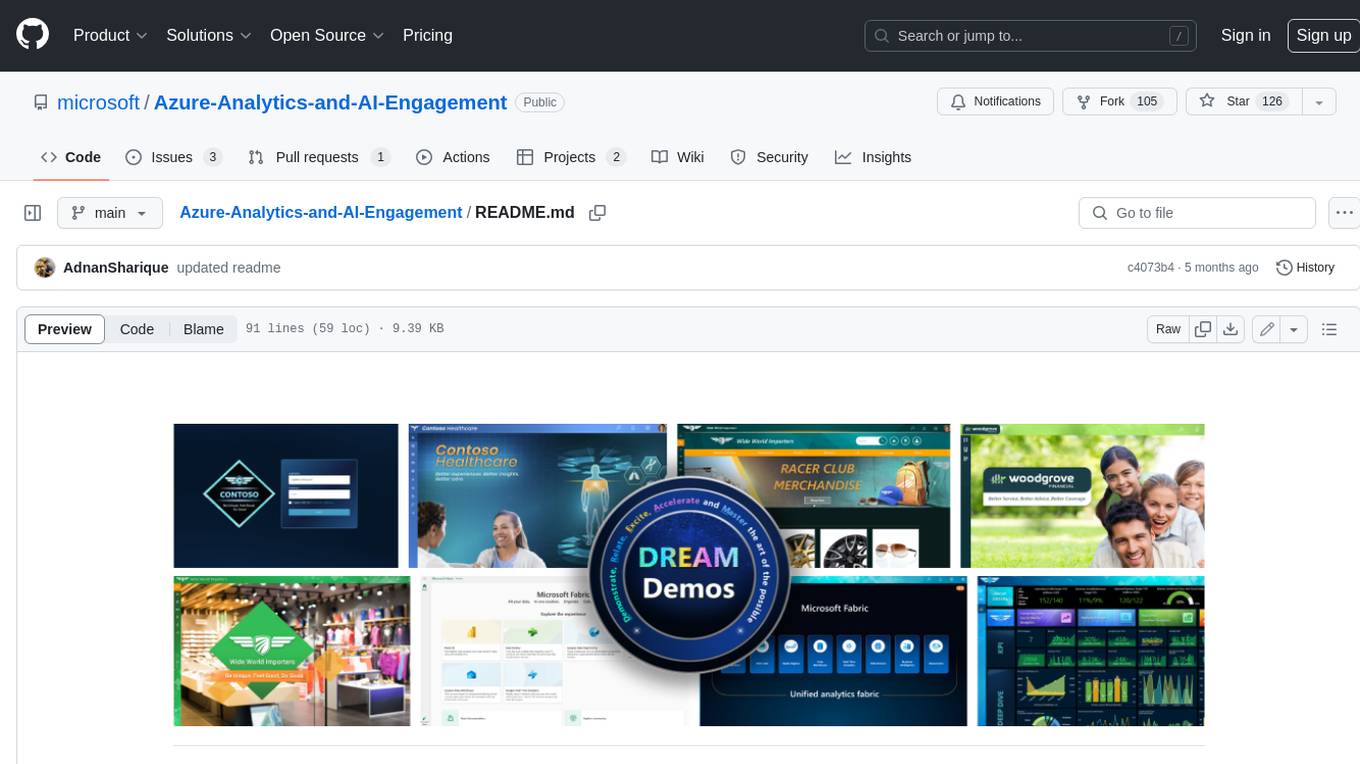
Azure-Analytics-and-AI-Engagement
The Azure-Analytics-and-AI-Engagement repository provides packaged Industry Scenario DREAM Demos with ARM templates (Containing a demo web application, Power BI reports, Synapse resources, AML Notebooks etc.) that can be deployed in a customer’s subscription using the CAPE tool within a matter of few hours. Partners can also deploy DREAM Demos in their own subscriptions using DPoC.