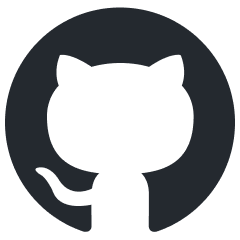
LLMInterviewQuestions
This repository contains LLM (Large language model) interview question asked in top companies like Google, Nvidia , Meta , Microsoft & fortune 500 companies.
Stars: 78
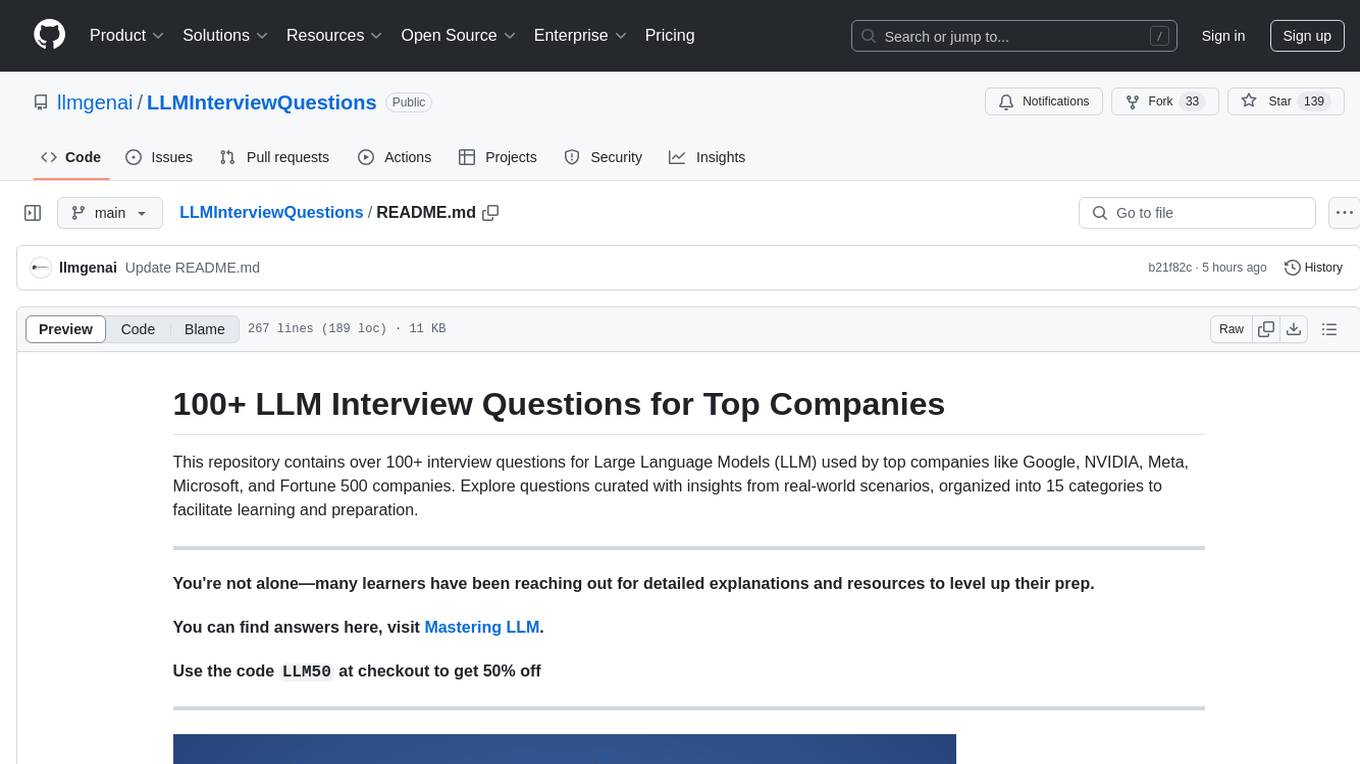
LLMInterviewQuestions is a repository containing over 100+ interview questions for Large Language Models (LLM) used by top companies like Google, NVIDIA, Meta, Microsoft, and Fortune 500 companies. The questions cover various topics related to LLMs, including prompt engineering, retrieval augmented generation, chunking, embedding models, internal working of vector databases, advanced search algorithms, language models internal working, supervised fine-tuning of LLM, preference alignment, evaluation of LLM system, hallucination control techniques, deployment of LLM, agent-based system, prompt hacking, and miscellaneous topics. The questions are organized into 15 categories to facilitate learning and preparation.
README:
This repository contains over 100+ interview questions for Large Language Models (LLM) used by top companies like Google, NVIDIA, Meta, Microsoft, and Fortune 500 companies. Explore questions curated with insights from real-world scenarios, organized into 15 categories to facilitate learning and preparation.
- Prompt Engineering & Basics of LLM
- Retrieval Augmented Generation (RAG)
- Chunking
- Embedding Models
- Internal Working of Vector Databases
- Advanced Search Algorithms
- Language Models Internal Working
- Supervised Fine-Tuning of LLM
- Preference Alignment (RLHF/DPO)
- Evaluation of LLM System
- Hallucination Control Techniques
- Deployment of LLM
- Agent-Based System
- Prompt Hacking
- Miscellaneous
- Case Studies
- What is the difference between Predictive/Discriminative AI and Generative AI?
- What is LLM, and how are LLMs trained?
- What is a token in the language model?
- How to estimate the cost of running SaaS-based and Open Source LLM models?
- Explain the Temperature parameter and how to set it.
- What are different decoding strategies for picking output tokens?
- What are different ways you can define stopping criteria in large language model?
- How to use stop sequences in LLMs?
- Explain the basic structure prompt engineering.
- Explain in-context learning
- Explain type of prompt engineering
- What are some of the aspect to keep in mind while using few-shots prompting?
- What are certain strategies to write good prompt?
- What is hallucination, and how can it be controlled using prompt engineering?
- How to improve the reasoning ability of LLM through prompt engineering?
- How to improve LLM reasoning if your COT prompt fails?
- how to increase accuracy, and reliability & make answers verifiable in LLM
- How does RAG work?
- What are some benefits of using the RAG system?
- When should I use Fine-tuning instead of RAG?
- What are the architecture patterns for customizing LLM with proprietary data?
- What is chunking, and why do we chunk our data?
- What factors influence chunk size?
- What are the different types of chunking methods?
- How to find the ideal chunk size?
- What are vector embeddings, and what is an embedding model?
- How is an embedding model used in the context of LLM applications?
- What is the difference between embedding short and long content?
- How to benchmark embedding models on your data?
- Suppose you are working with an open AI embedding model, after benchmarking accuracy is coming low, how would you further improve the accuracy of embedding the search model?
- Walk me through steps of improving sentence transformer model used for embedding?
- What is a vector database?
- How does a vector database differ from traditional databases?
- How does a vector database work?
- Explain difference between vector index, vector DB & vector plugins?
- You are working on a project that involves a small dataset of customer reviews. Your task is to find similar reviews in the dataset. The priority is to achieve perfect accuracy in finding the most similar reviews, and the speed of the search is not a primary concern. Which search strategy would you choose and why?
- Explain vector search strategies like clustering and Locality-Sensitive Hashing.
- How does clustering reduce search space? When does it fail and how can we mitigate these failures?
- Explain Random projection index?
- Explain Locality-sensitive hashing (LHS) indexing method?
- Explain product quantization (PQ) indexing method?
- Compare different Vector index and given a scenario, which vector index you would use for a project?
- How would you decide ideal search similarity metrics for the use case?
- Explain different types and challenges associated with filtering in vector DB?
- How to decide the best vector database for your needs?
- What are architecture patterns for information retrieval & semantic search?
- Why it’s important to have very good search
- How can you achieve efficient and accurate search results in large-scale datasets?
- Consider a scenario where a client has already built a RAG-based system that is not giving accurate results, upon investigation you find out that the retrieval system is not accurate, what steps you will take to improve it?
- Explain the keyword-based retrieval method
- How to fine-tune re-ranking models?
- Explain most common metric used in information retrieval and when it fails?
- If you were to create an algorithm for a Quora-like question-answering system, with the objective of ensuring users find the most pertinent answers as quickly as possible, which evaluation metric would you choose to assess the effectiveness of your system?
- I have a recommendation system, which metric should I use to evaluate the system?
- Compare different information retrieval metrics and which one to use when?
- How does hybrid search works?
- If you have search results from multiple methods, how would you merge and homogenize the rankings into a single result set?
- How to handle multi-hop/multifaceted queries?
- What are different techniques to be used to improved retrieval?
- Can you provide a detailed explanation of the concept of self-attention?
- Explain the disadvantages of the self-attention mechanism and how can you overcome it.
- What is positional encoding?
- Explain Transformer architecture in detail.
- What are some of the advantages of using a transformer instead of LSTM?
- What is the difference between local attention and global attention?
- What makes transformers heavy on computation and memory, and how can we address this?
- How can you increase the context length of an LLM?
- If I have a vocabulary of 100K words/tokens, how can I optimize transformer architecture?
- A large vocabulary can cause computation issues and a small vocabulary can cause OOV issues, what approach you would use to find the best balance of vocabulary?
- Explain different types of LLM architecture and which type of architecture is best for which task?
- What is fine-tuning, and why is it needed?
- Which scenario do we need to fine-tune LLM?
- How to make the decision of fine-tuning?
- How do you improve the model to answer only if there is sufficient context for doing so?
- How to create fine-tuning datasets for Q&A?
- How to set hyperparameters for fine-tuning?
- How to estimate infrastructure requirements for fine-tuning LLM?
- How do you fine-tune LLM on consumer hardware?
- What are the different categories of the PEFT method?
- What is catastrophic forgetting in LLMs?
- What are different re-parameterized methods for fine-tuning?
- At which stage you will decide to go for the Preference alignment type of method rather than SFT?
- What is RLHF, and how is it used?
- What is the reward hacking issue in RLHF?
- Explain different preference alignment methods.
- How do you evaluate the best LLM model for your use case?
- How to evaluate RAG-based systems?
- What are different metrics for evaluating LLMs?
- Explain the Chain of Verification.
- What are different forms of hallucinations?
- How to control hallucinations at various levels?
- Why does quantization not decrease the accuracy of LLM?
- What are the techniques by which you can optimize the inference of LLM for higher throughput?
- How to accelerate response time of model without attention approximation like group query attention?
- Explain the basic concepts of an agent and the types of strategies available to implement agents
- Why do we need agents and what are some common strategies to implement agents?
- Explain ReAct prompting with a code example and its advantages
- Explain Plan and Execute prompting strategy
- Explain OpenAI functions strategy with code examples
- Explain the difference between OpenAI functions vs LangChain Agents
- What is prompt hacking and why should we bother about it?
- What are the different types of prompt hacking?
- What are the different defense tactics from prompt hacking?
- How to optimize cost of overall LLM System?
- What are mixture of expert models (MoE)?
- How to build production grade RAG system, explain each component in detail ?
- What is FP8 variable and what are its advantages of it
- How to train LLM with low precision training without compromising on accuracy ?
- How to calculate size of KV cache
- Explain dimension of each layer in multi headed transformation attention block
- How do you make sure that attention layer focuses on the right part of the input?
- Case Study 1: LLM Chat Assistant with dynamic context based on query
- Case Study 2: Prompting Techniques
For answers for those questions please, visit Mastering LLM.
For Tasks:
Click tags to check more tools for each tasksFor Jobs:
Alternative AI tools for LLMInterviewQuestions
Similar Open Source Tools
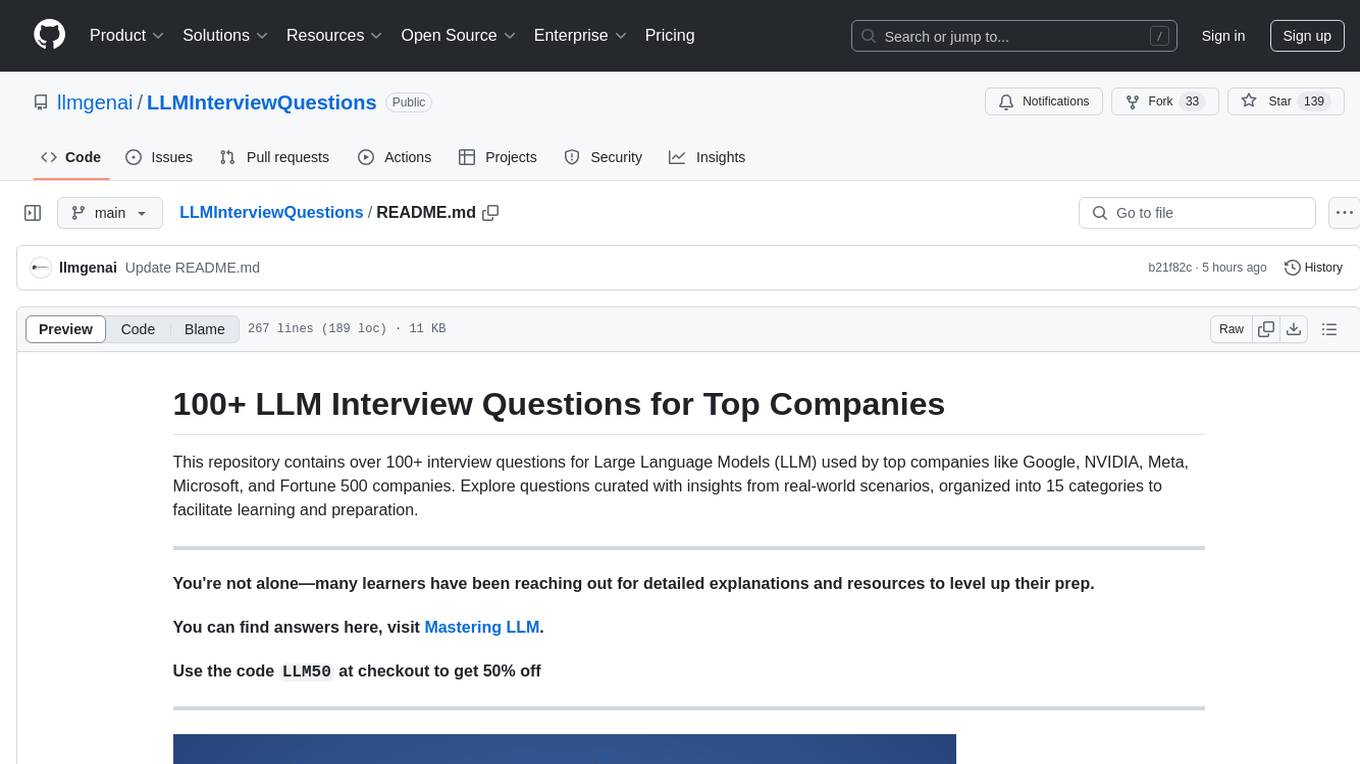
LLMInterviewQuestions
LLMInterviewQuestions is a repository containing over 100+ interview questions for Large Language Models (LLM) used by top companies like Google, NVIDIA, Meta, Microsoft, and Fortune 500 companies. The questions cover various topics related to LLMs, including prompt engineering, retrieval augmented generation, chunking, embedding models, internal working of vector databases, advanced search algorithms, language models internal working, supervised fine-tuning of LLM, preference alignment, evaluation of LLM system, hallucination control techniques, deployment of LLM, agent-based system, prompt hacking, and miscellaneous topics. The questions are organized into 15 categories to facilitate learning and preparation.
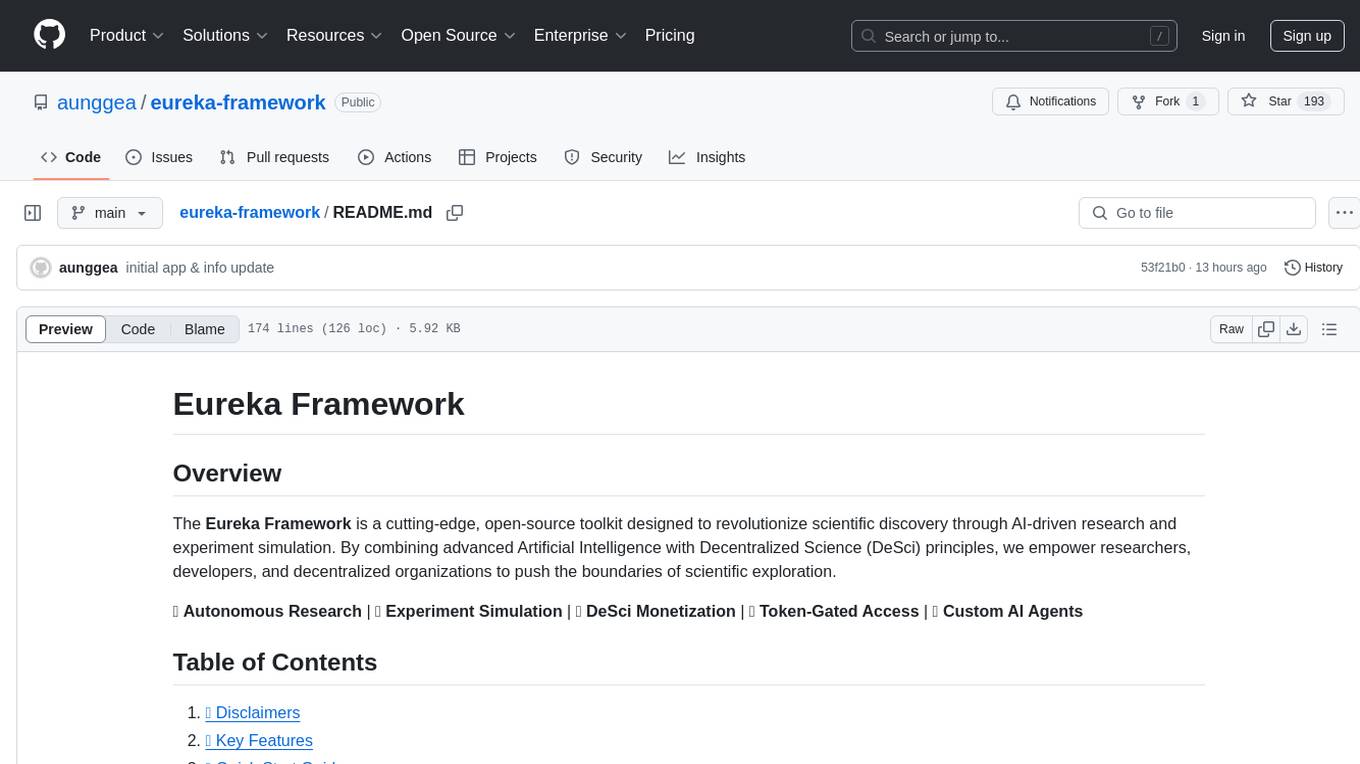
eureka-framework
The Eureka Framework is an open-source toolkit that leverages advanced Artificial Intelligence and Decentralized Science principles to revolutionize scientific discovery. It enables researchers, developers, and decentralized organizations to explore scientific papers, conduct AI-driven experiments, monetize research contributions, provide token-gated access to AI agents, and customize AI agents for specific research domains. The framework also offers features like a RESTful API, robust scheduler for task automation, and webhooks for real-time notifications, empowering users to automate research tasks, enhance productivity, and foster a committed research community.
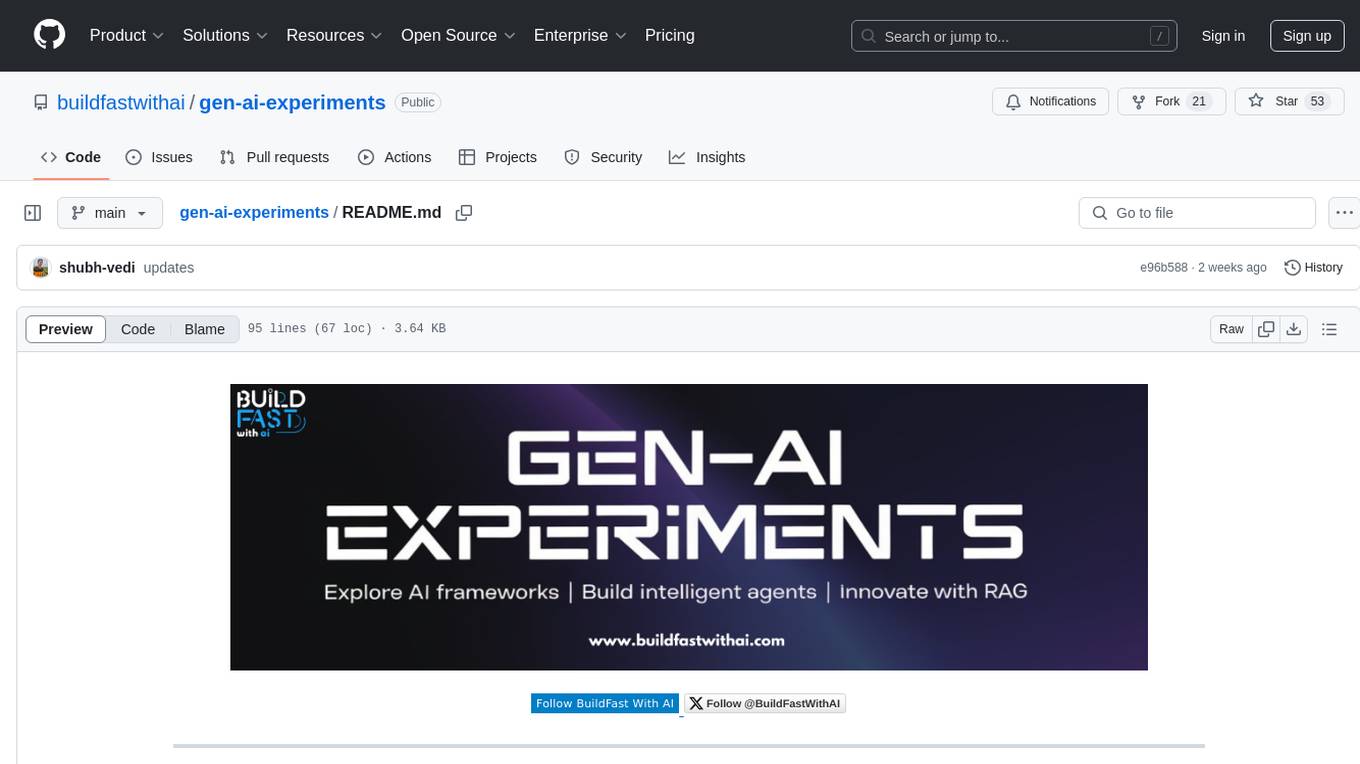
gen-ai-experiments
Gen-AI-Experiments is a structured collection of Jupyter notebooks and AI experiments designed to guide users through various AI tools, frameworks, and models. It offers valuable resources for both beginners and experienced practitioners, covering topics such as AI agents, model testing, RAG systems, real-world applications, and open-source tools. The repository includes folders with curated libraries, AI agents, experiments, LLM testing, open-source libraries, RAG experiments, and educhain experiments, each focusing on different aspects of AI development and application.
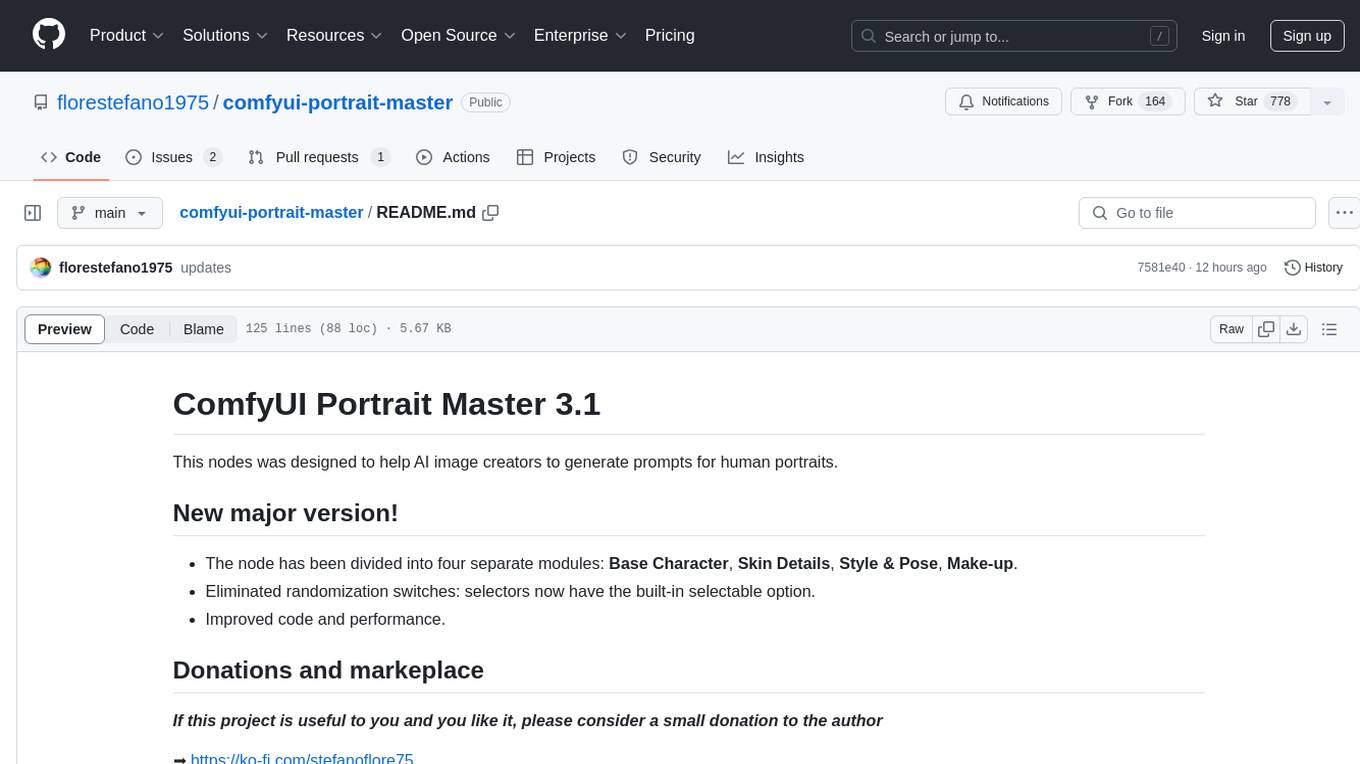
comfyui-portrait-master
ComfyUI Portrait Master 3.1 is a tool designed to assist AI image creators in generating prompts for human portraits. The tool offers various modules for customizing character details such as base character, skin details, style & pose, and makeup. Users can control parameters like shot type, gender, age, ethnicity mix, body type, facial features, hair details, skin imperfections, and more to create unique portrait prompts. The tool aims to enhance photorealism and provide a user-friendly interface for generating portrait prompts efficiently.
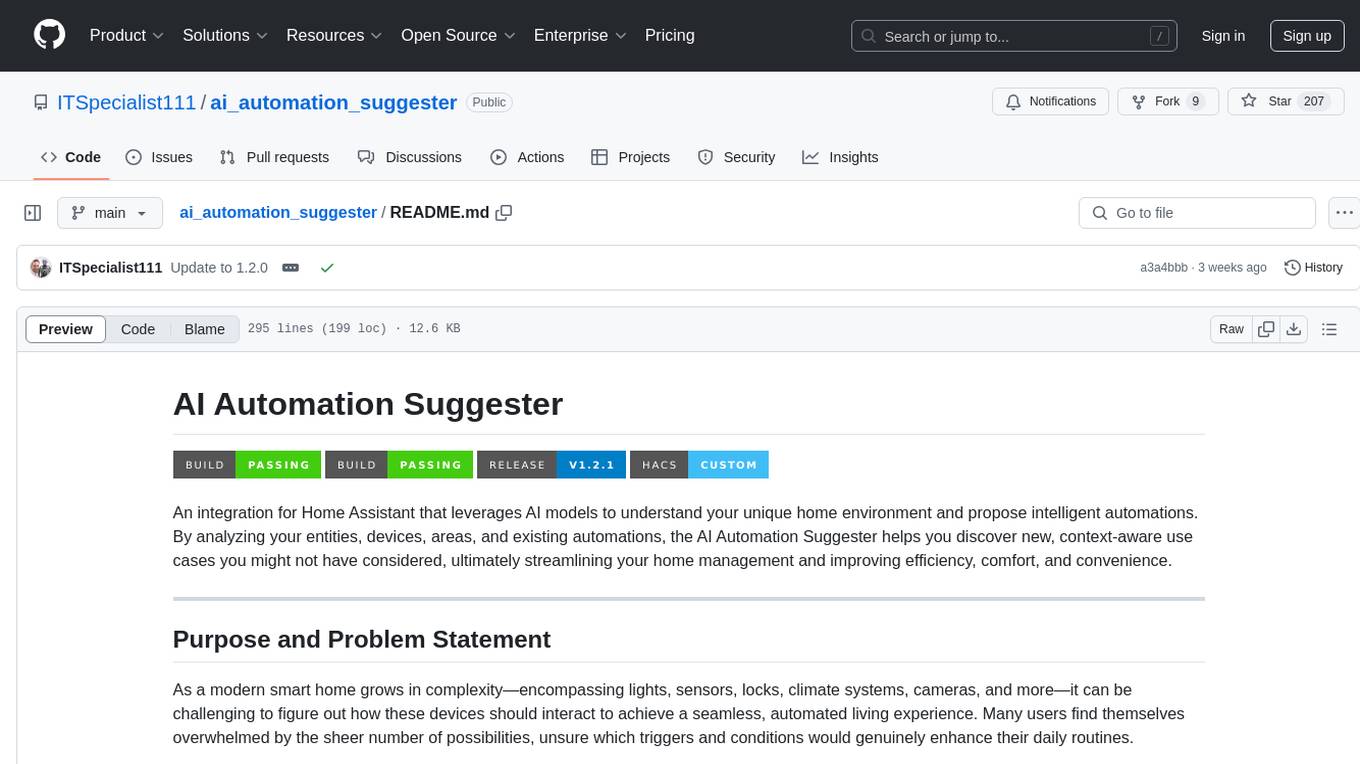
ai_automation_suggester
An integration for Home Assistant that leverages AI models to understand your unique home environment and propose intelligent automations. By analyzing your entities, devices, areas, and existing automations, the AI Automation Suggester helps you discover new, context-aware use cases you might not have considered, ultimately streamlining your home management and improving efficiency, comfort, and convenience. The tool acts as a personal automation consultant, providing actionable YAML-based automations that can save energy, improve security, enhance comfort, and reduce manual intervention. It turns the complexity of a large Home Assistant environment into actionable insights and tangible benefits.
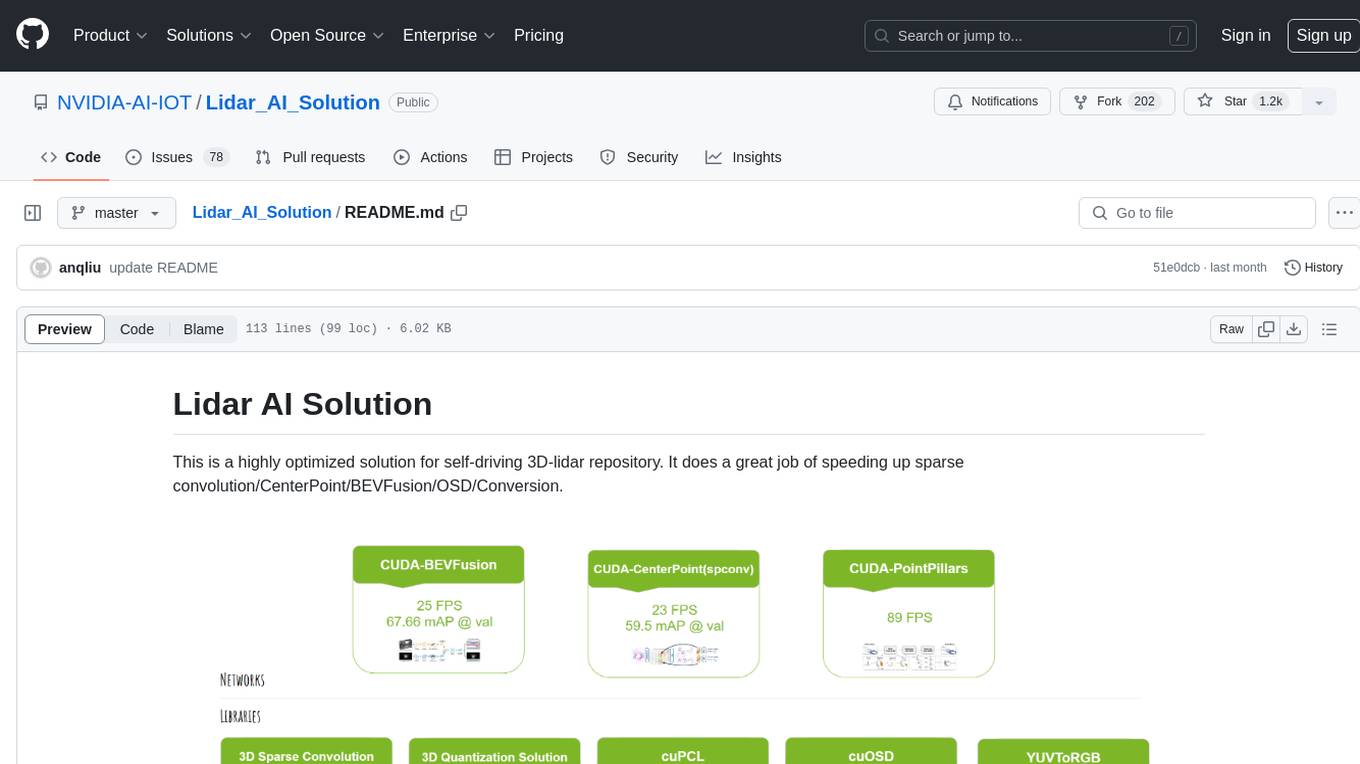
Lidar_AI_Solution
Lidar AI Solution is a highly optimized repository for self-driving 3D lidar, providing solutions for sparse convolution, BEVFusion, CenterPoint, OSD, and Conversion. It includes CUDA and TensorRT implementations for various tasks such as 3D sparse convolution, BEVFusion, CenterPoint, PointPillars, V2XFusion, cuOSD, cuPCL, and YUV to RGB conversion. The repository offers easy-to-use solutions, high accuracy, low memory usage, and quantization options for different tasks related to self-driving technology.
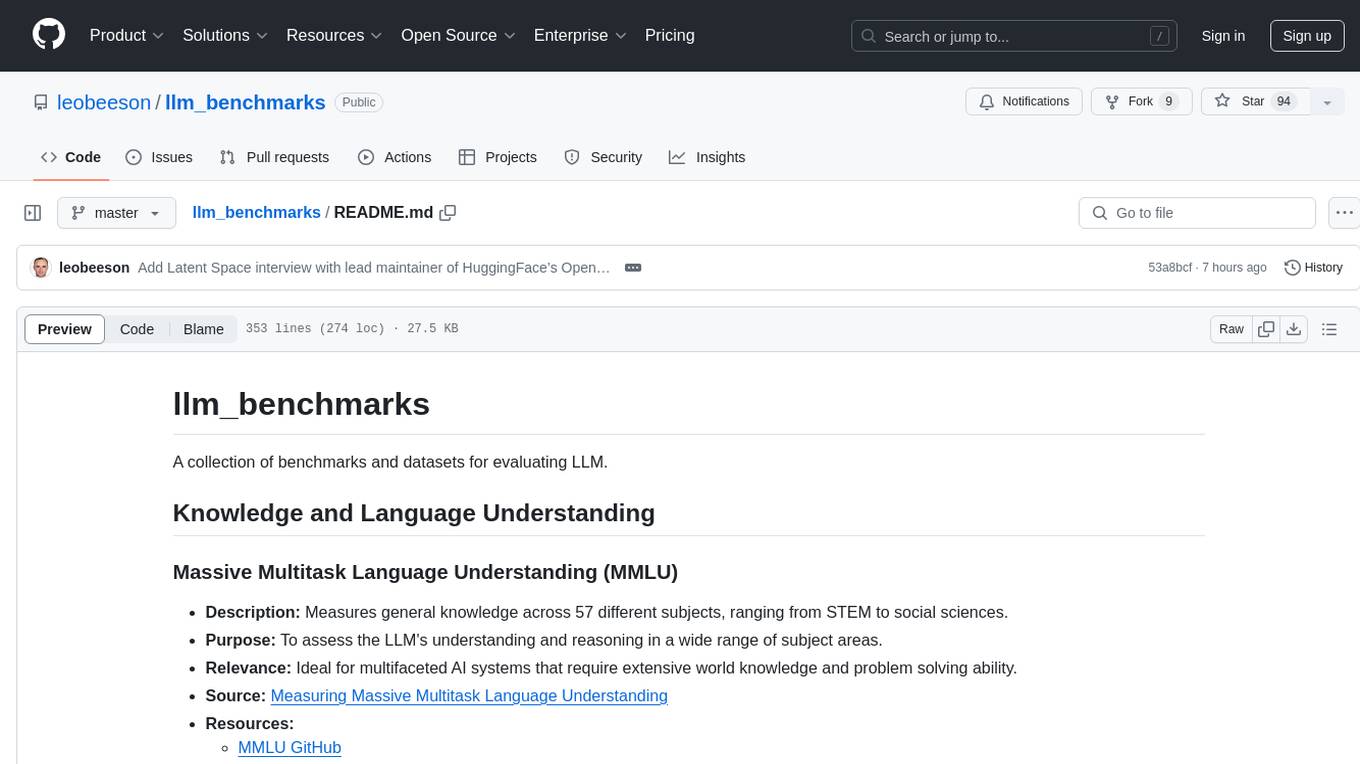
llm_benchmarks
llm_benchmarks is a collection of benchmarks and datasets for evaluating Large Language Models (LLMs). It includes various tasks and datasets to assess LLMs' knowledge, reasoning, language understanding, and conversational abilities. The repository aims to provide comprehensive evaluation resources for LLMs across different domains and applications, such as education, healthcare, content moderation, coding, and conversational AI. Researchers and developers can leverage these benchmarks to test and improve the performance of LLMs in various real-world scenarios.
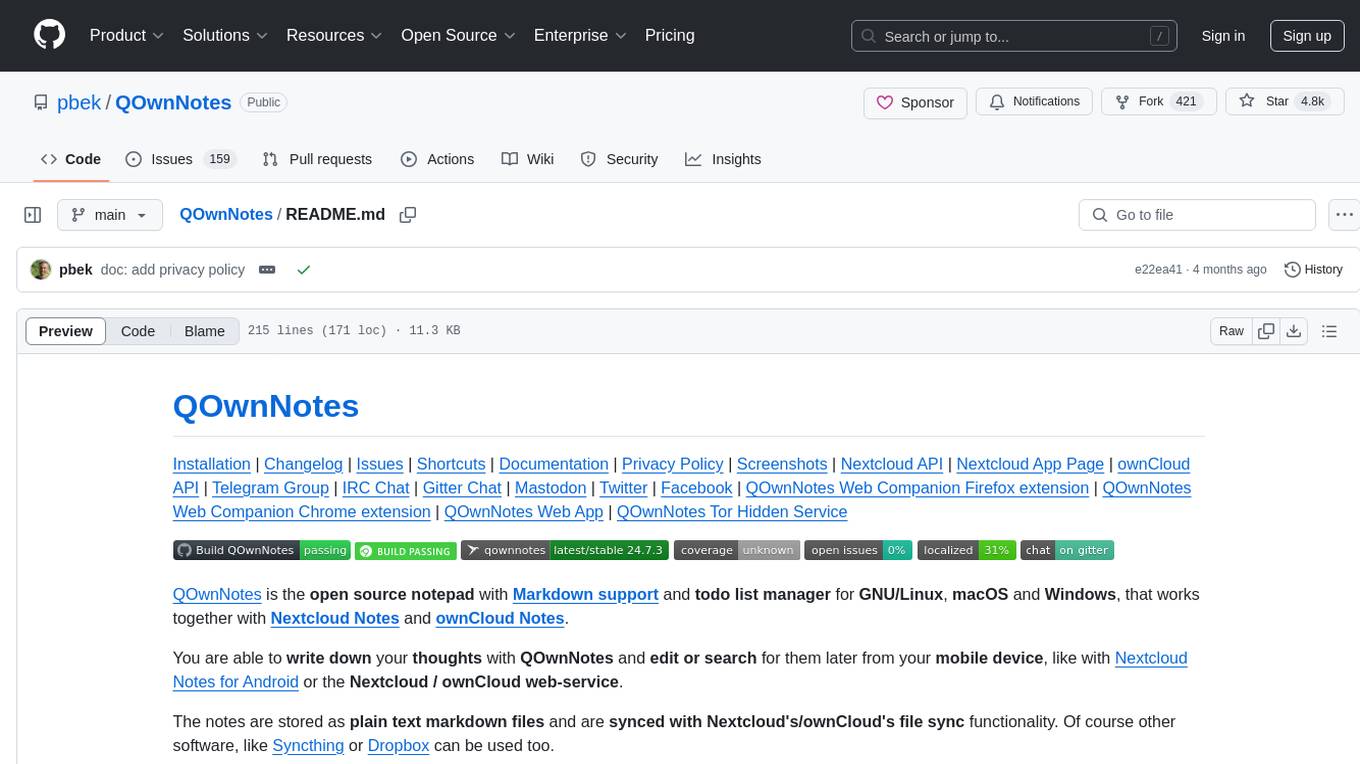
QOwnNotes
QOwnNotes is an open source notepad with Markdown support and todo list manager for GNU/Linux, macOS, and Windows. It allows you to write down thoughts, edit, and search for them later from mobile devices. Notes are stored as plain text markdown files and synced with Nextcloud's/ownCloud's file sync functionality. QOwnNotes offers features like multiple note folders, restoration of older versions and trashed notes, sub-string searching, customizable keyboard shortcuts, markdown highlighting, spellchecking, tabbing support, scripting support, encryption of notes, dark mode theme support, and more. It supports hierarchical note tagging, note subfolders, sharing notes on Nextcloud/ownCloud server, portable mode, Vim mode, distraction-free mode, full-screen mode, typewriter mode, Evernote and Joplin import, and is available in over 60 languages.
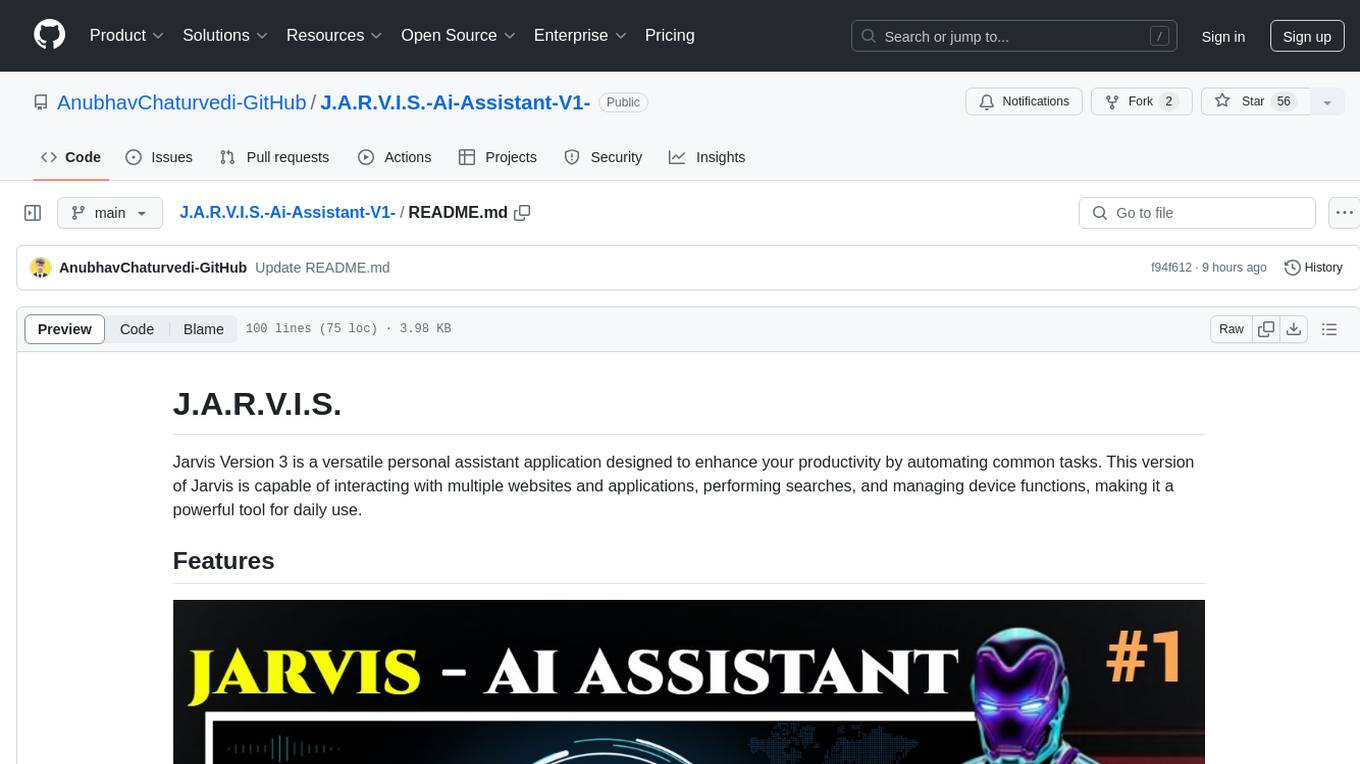
J.A.R.V.I.S.-Ai-Assistant-V1-
Jarvis Version 3 is a versatile personal assistant application designed to enhance productivity by automating common tasks. It can interact with websites and applications, perform searches, manage device functions, and control music. Users can give commands to open websites, search on Google or YouTube, scroll pages, manage applications, check time, internet speed, battery percentage, battery alerts, charging status, play music, and synchronize clapping with music. The tool offers features for web navigation, search functionality, scrolling, application management, device management, and music control.
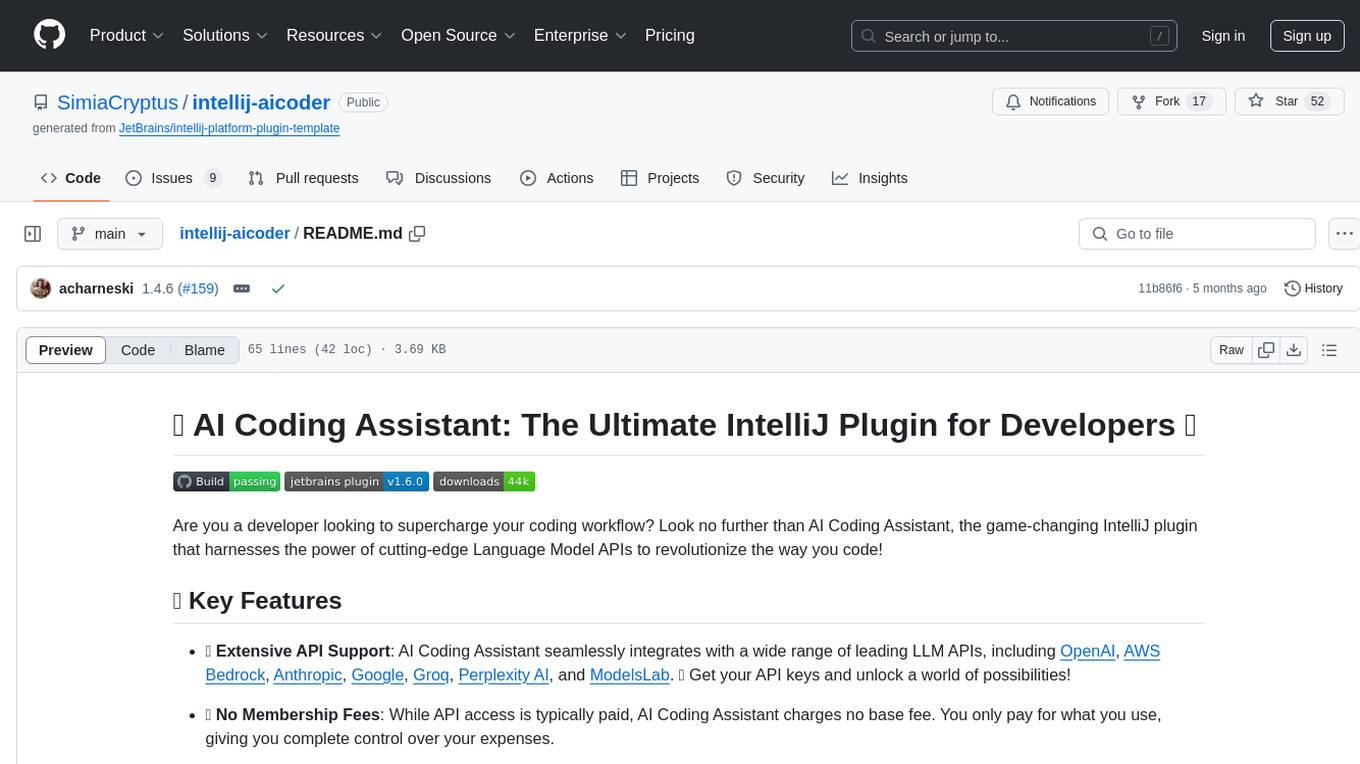
intellij-aicoder
AI Coding Assistant is a free and open-source IntelliJ plugin that leverages cutting-edge Language Model APIs to enhance developers' coding experience. It seamlessly integrates with various leading LLM APIs, offers an intuitive toolbar UI, and allows granular control over API requests. With features like Code & Patch Chat, Planning with AI Agents, Markdown visualization, and versatile text processing capabilities, this tool aims to streamline coding workflows and boost productivity.
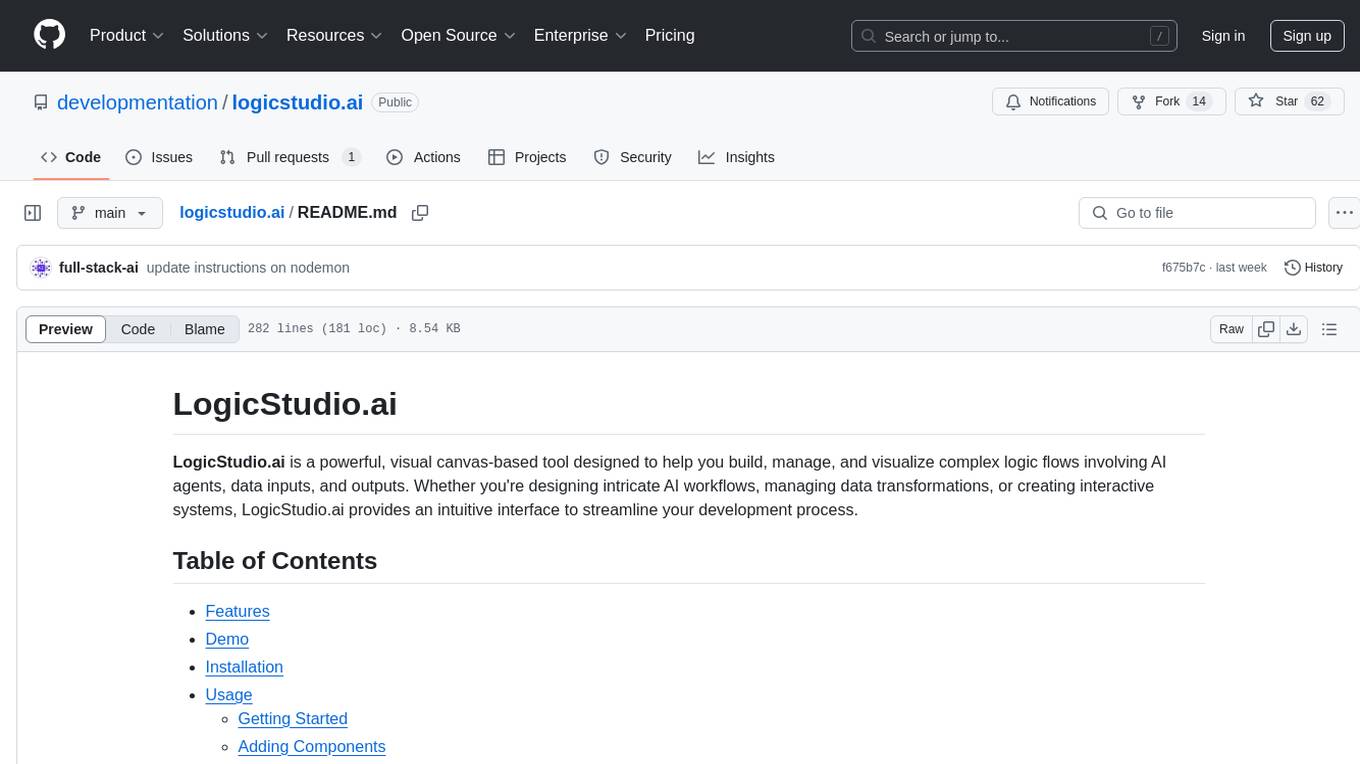
logicstudio.ai
LogicStudio.ai is a powerful visual canvas-based tool for building, managing, and visualizing complex logic flows involving AI agents, data inputs, and outputs. It provides an intuitive interface to streamline development processes by offering features like drag-and-drop canvas design, dynamic components, real-time connections, import/export capabilities, zoom & pan controls, file management, AI integration, editable views, and various output formats. Users can easily add, connect, configure, and manage components to create interactive systems and workflows.
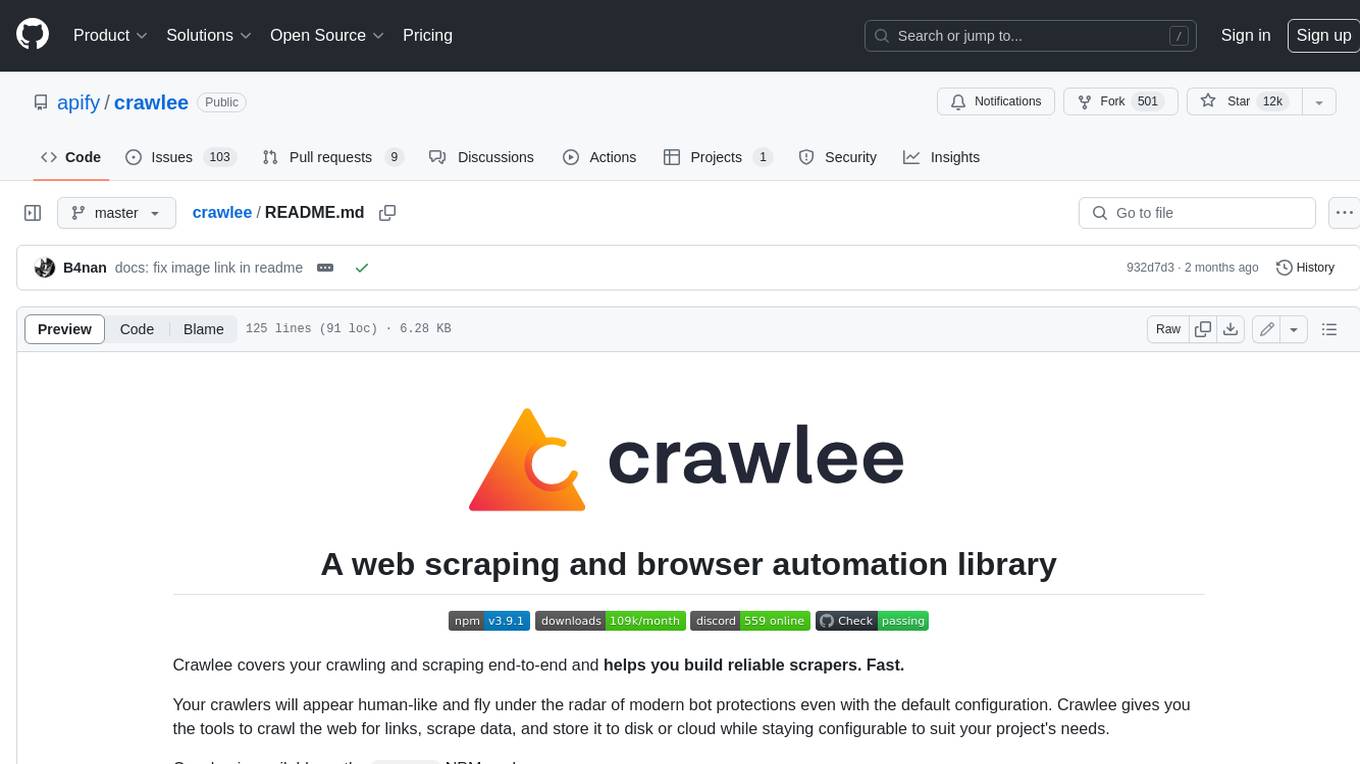
crawlee
Crawlee is a web scraping and browser automation library that helps you build reliable scrapers quickly. Your crawlers will appear human-like and fly under the radar of modern bot protections even with the default configuration. Crawlee gives you the tools to crawl the web for links, scrape data, and store it to disk or cloud while staying configurable to suit your project's needs.
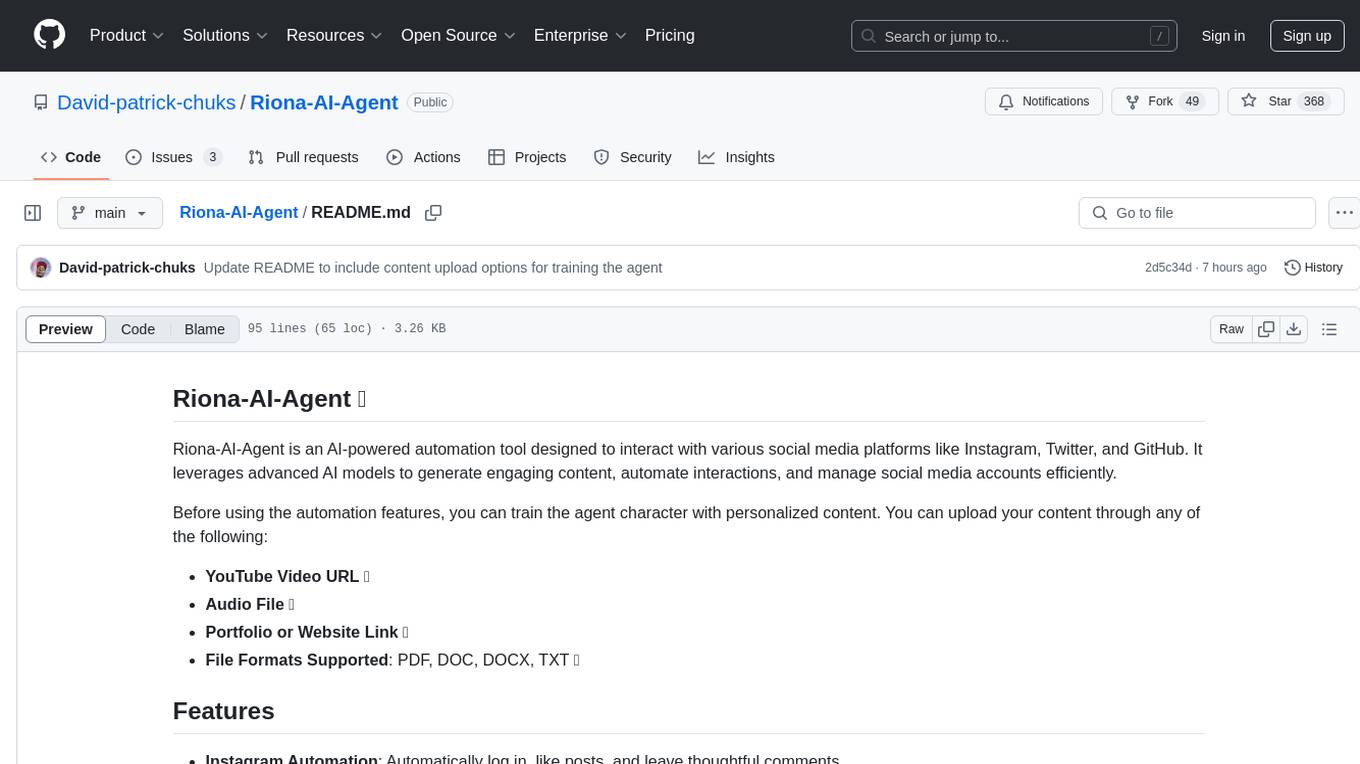
Riona-AI-Agent
Riona-AI-Agent is a versatile AI chatbot designed to assist users in various tasks. It utilizes natural language processing and machine learning algorithms to understand user queries and provide accurate responses. The chatbot can be integrated into websites, applications, and messaging platforms to enhance user experience and streamline communication. With its customizable features and easy deployment, Riona-AI-Agent is suitable for businesses, developers, and individuals looking to automate customer support, provide information, and engage with users in a conversational manner.
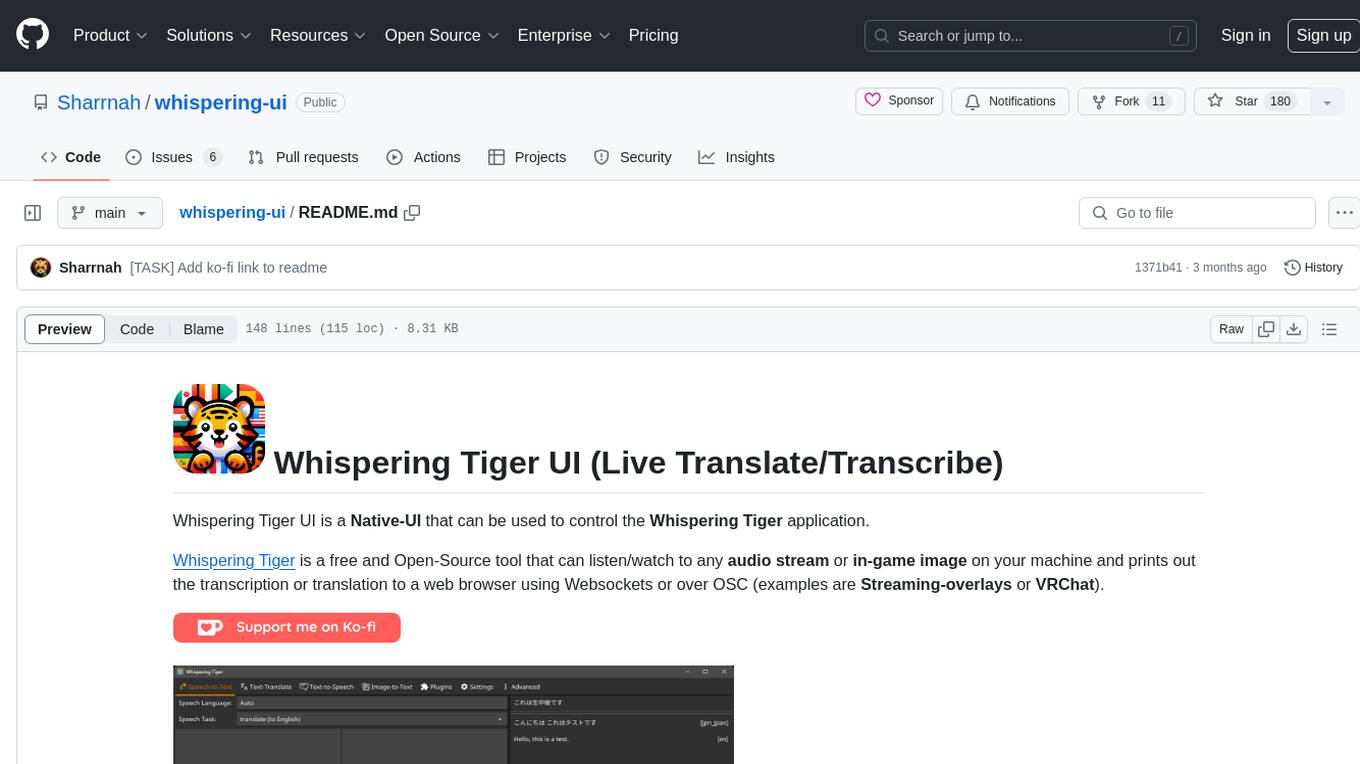
whispering-ui
Whispering Tiger UI is a Native-UI tool designed to control the Whispering Tiger application, a free and Open-Source tool that can listen/watch to audio streams or in-game images on your machine and provide transcription or translation to a web browser using Websockets or over OSC. It features a Native-UI for Windows, easy access to all Whispering Tiger features including transcription, translation, text-to-speech, and in-game image recognition. The tool supports loopback audio device, configuration saving/loading, plugin support for additional features, and auto-update functionality. Users can create profiles, configure audio devices, select A.I. devices for speech-to-text, and install/manage plugins for extended functionality.
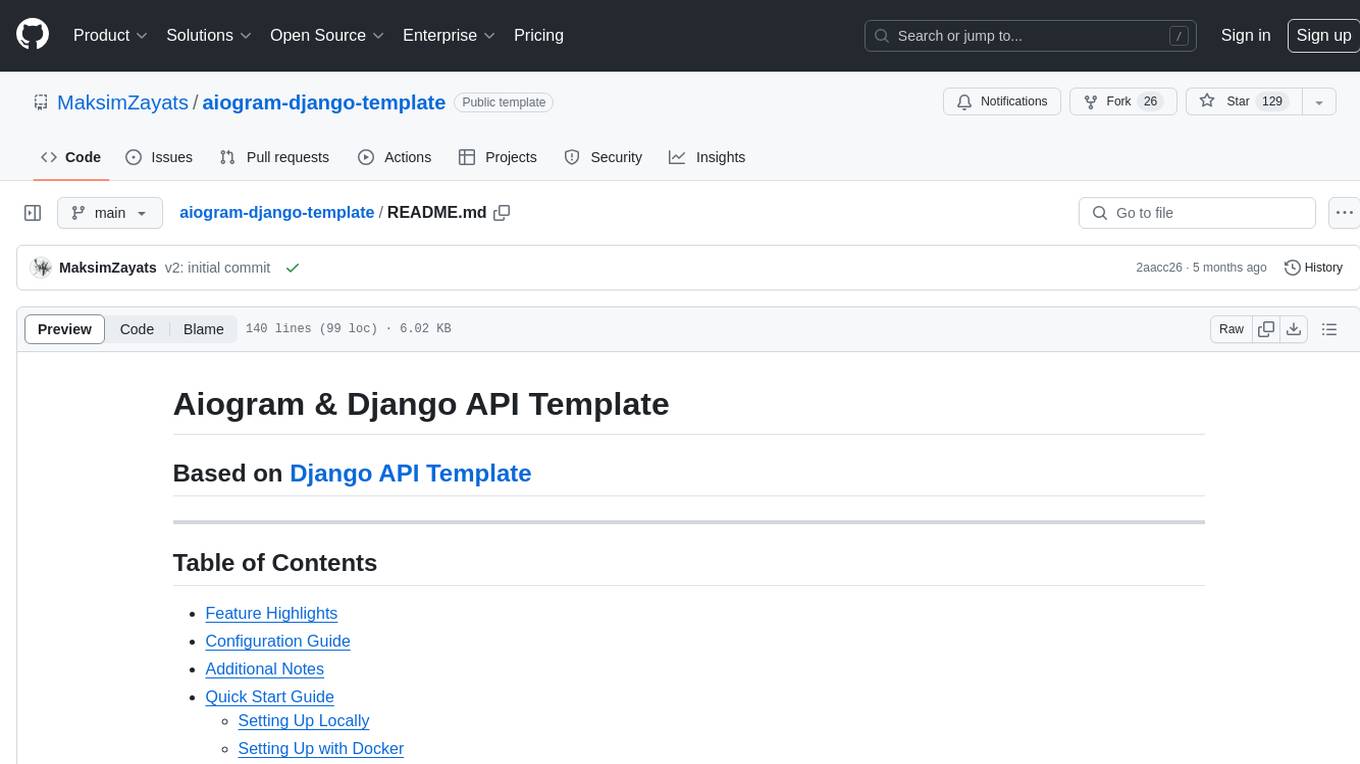
aiogram-django-template
Aiogram & Django API Template is a robust and secure Django template with advanced features like Docker integration, Celery for asynchronous tasks, Sentry for error tracking, Django Rest Framework for building APIs, and more. It provides scalability options, up-to-date dependencies, and integration with AWS S3 for storage. The template includes configuration guides for secrets, ports, performance tuning, application settings, CORS and CSRF settings, and database configuration. Security, scalability, and monitoring are emphasized for efficient Django API development.
For similar tasks
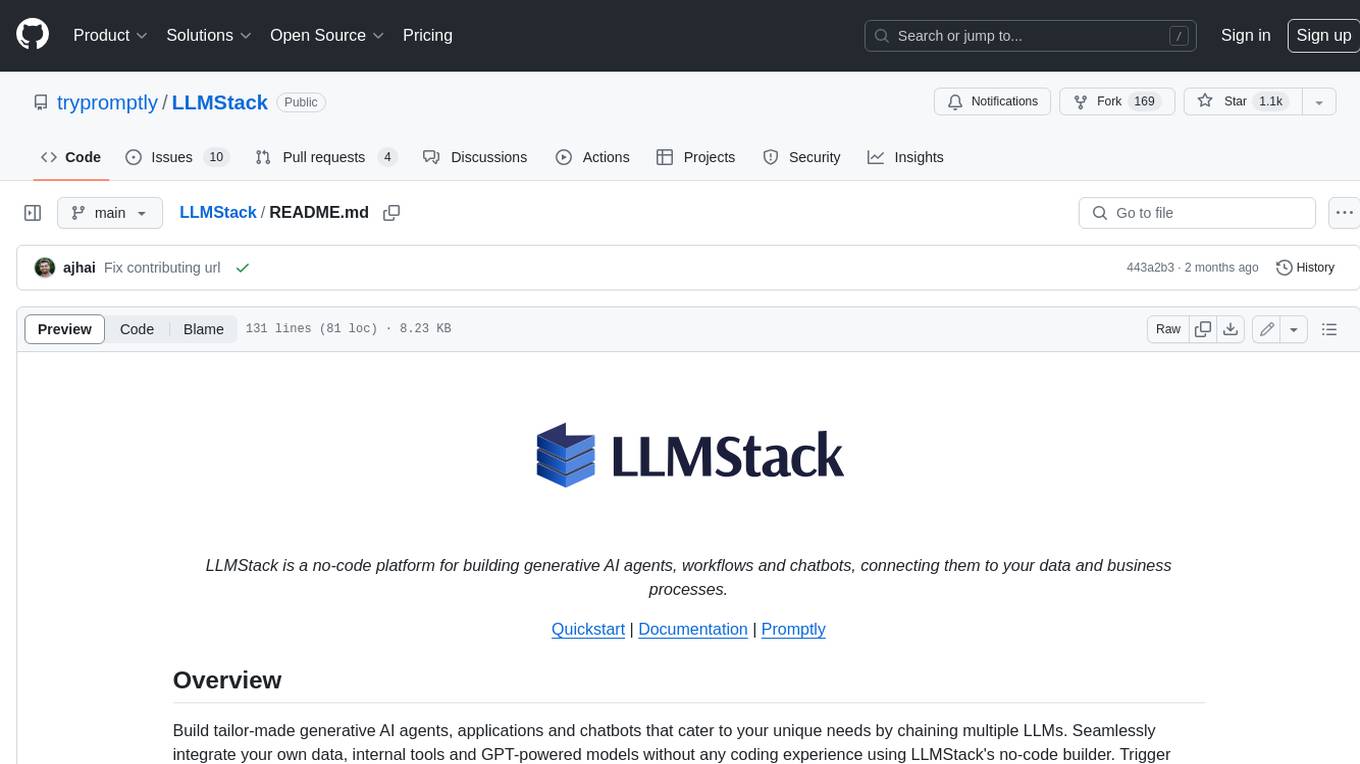
LLMStack
LLMStack is a no-code platform for building generative AI agents, workflows, and chatbots. It allows users to connect their own data, internal tools, and GPT-powered models without any coding experience. LLMStack can be deployed to the cloud or on-premise and can be accessed via HTTP API or triggered from Slack or Discord.
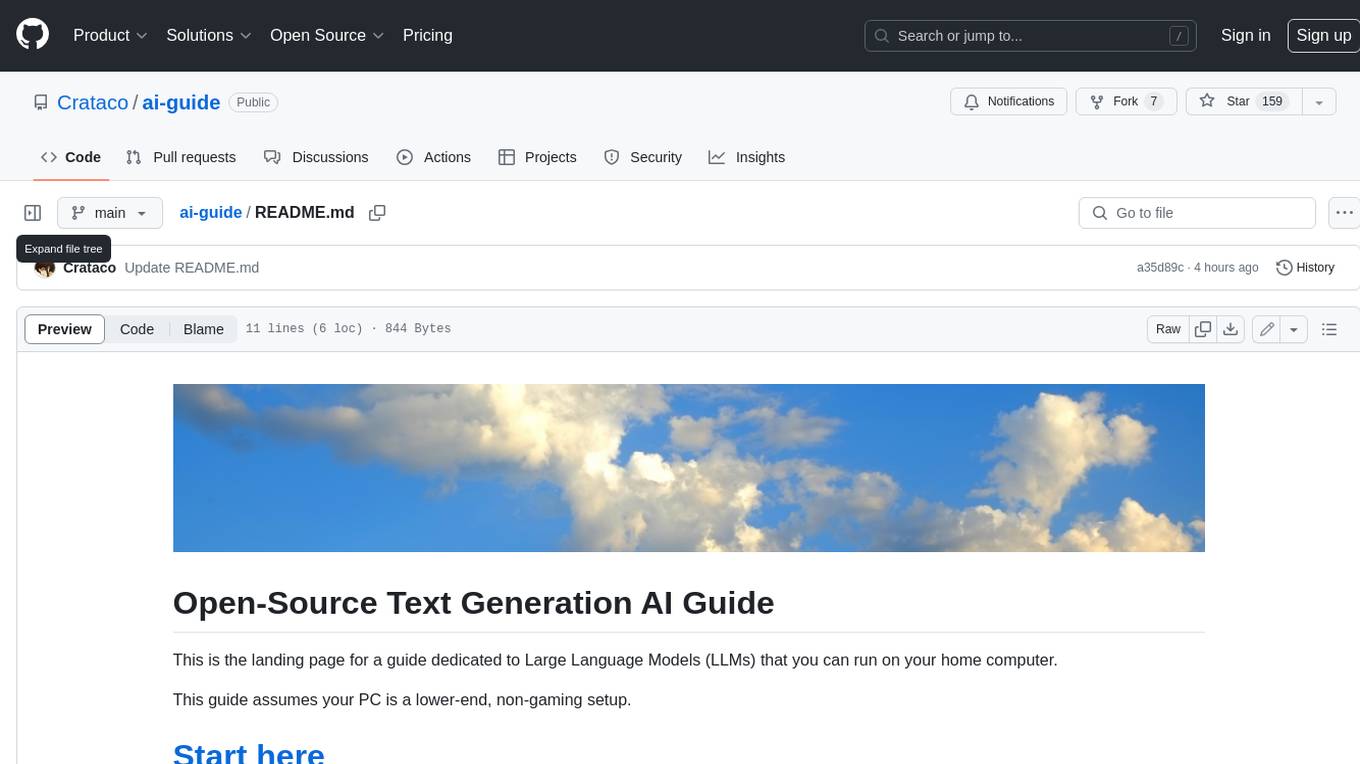
ai-guide
This guide is dedicated to Large Language Models (LLMs) that you can run on your home computer. It assumes your PC is a lower-end, non-gaming setup.
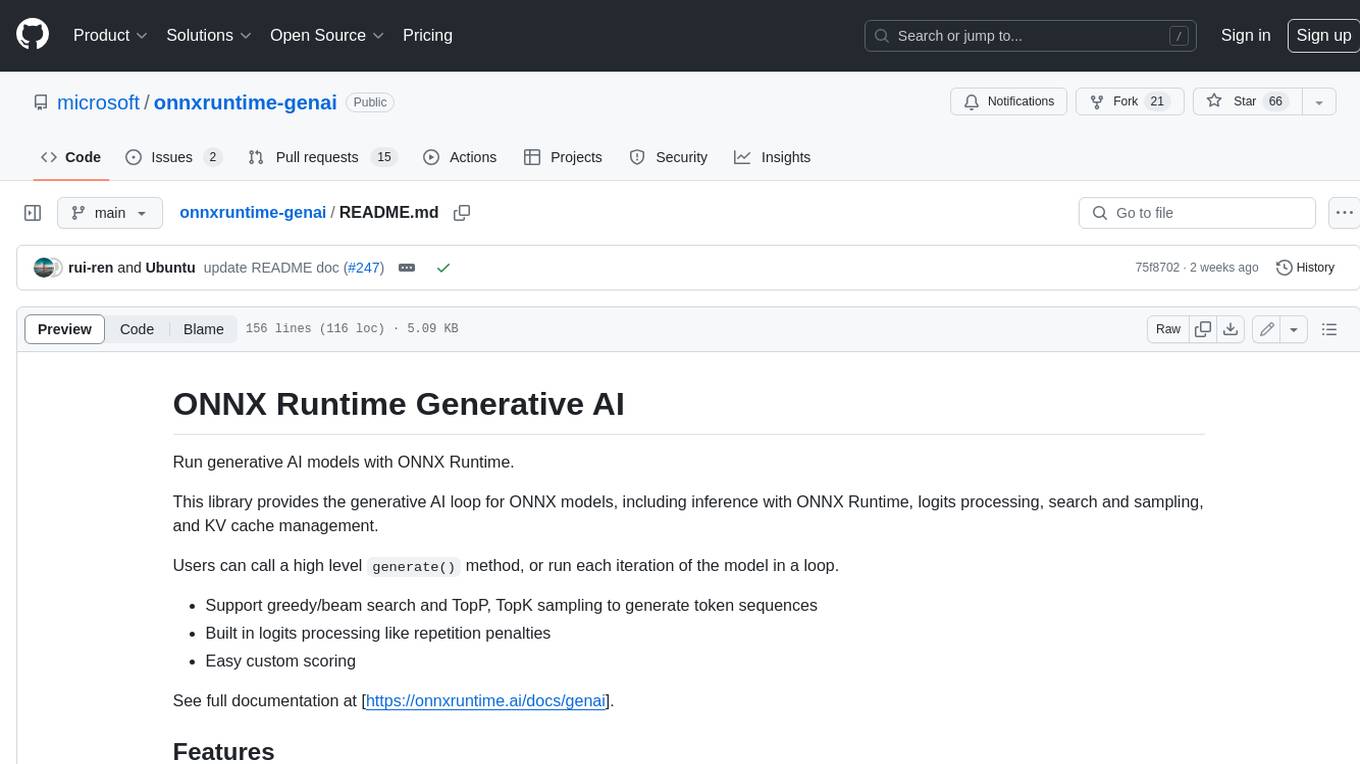
onnxruntime-genai
ONNX Runtime Generative AI is a library that provides the generative AI loop for ONNX models, including inference with ONNX Runtime, logits processing, search and sampling, and KV cache management. Users can call a high level `generate()` method, or run each iteration of the model in a loop. It supports greedy/beam search and TopP, TopK sampling to generate token sequences, has built in logits processing like repetition penalties, and allows for easy custom scoring.
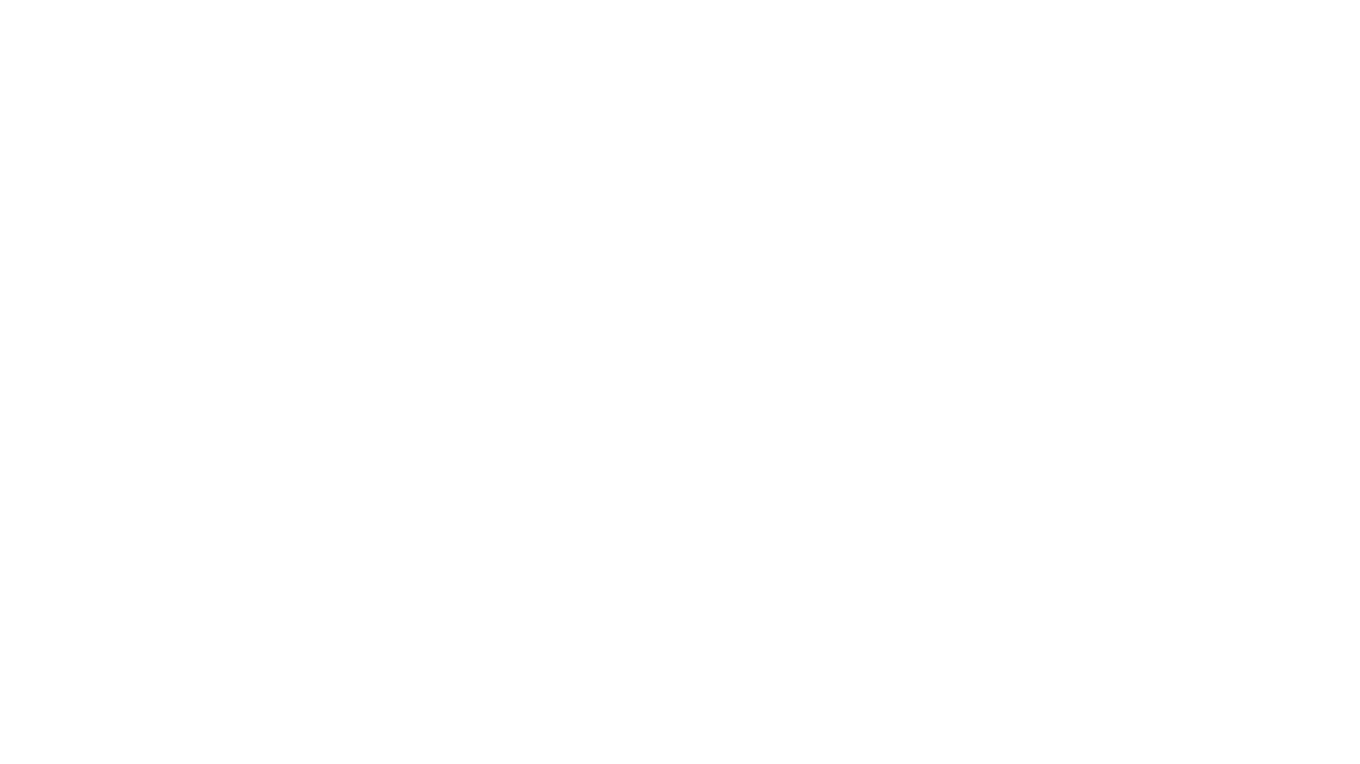
jupyter-ai
Jupyter AI connects generative AI with Jupyter notebooks. It provides a user-friendly and powerful way to explore generative AI models in notebooks and improve your productivity in JupyterLab and the Jupyter Notebook. Specifically, Jupyter AI offers: * An `%%ai` magic that turns the Jupyter notebook into a reproducible generative AI playground. This works anywhere the IPython kernel runs (JupyterLab, Jupyter Notebook, Google Colab, Kaggle, VSCode, etc.). * A native chat UI in JupyterLab that enables you to work with generative AI as a conversational assistant. * Support for a wide range of generative model providers, including AI21, Anthropic, AWS, Cohere, Gemini, Hugging Face, NVIDIA, and OpenAI. * Local model support through GPT4All, enabling use of generative AI models on consumer grade machines with ease and privacy.
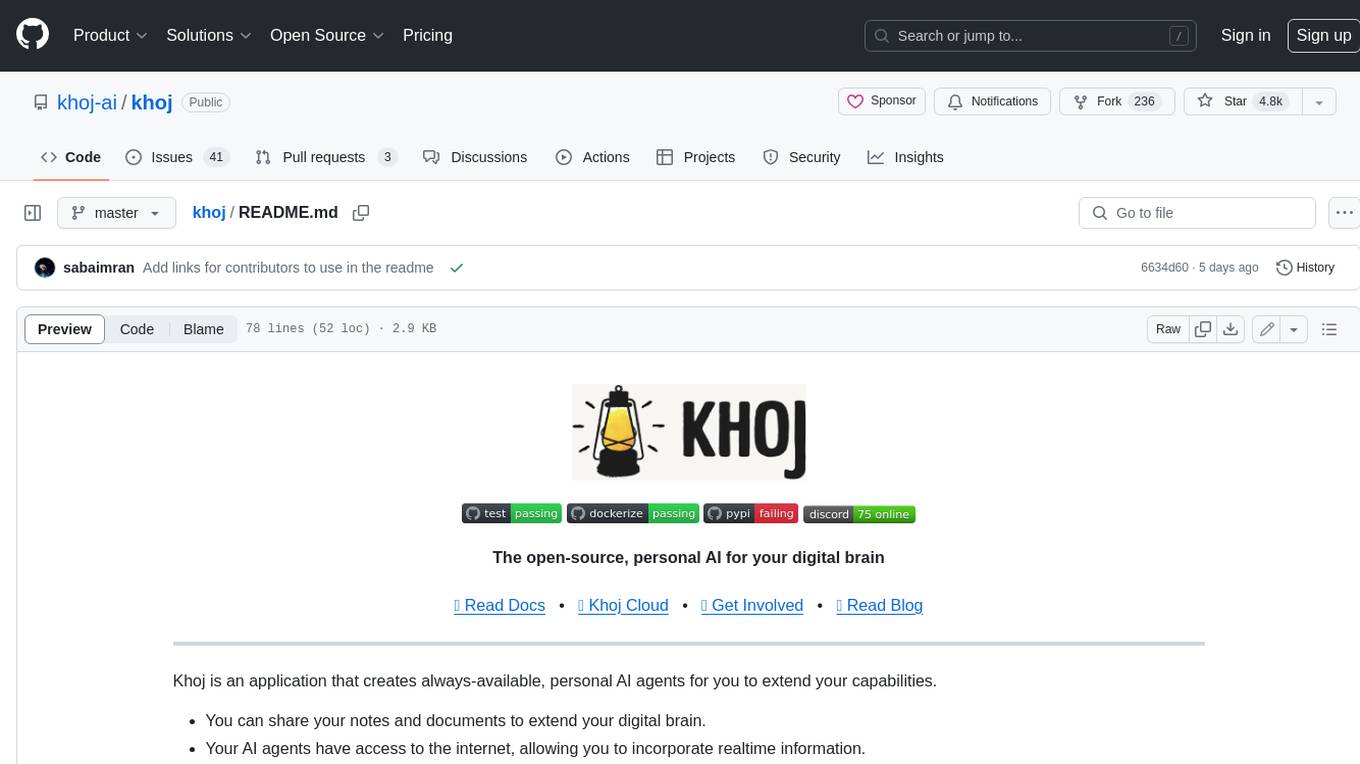
khoj
Khoj is an open-source, personal AI assistant that extends your capabilities by creating always-available AI agents. You can share your notes and documents to extend your digital brain, and your AI agents have access to the internet, allowing you to incorporate real-time information. Khoj is accessible on Desktop, Emacs, Obsidian, Web, and Whatsapp, and you can share PDF, markdown, org-mode, notion files, and GitHub repositories. You'll get fast, accurate semantic search on top of your docs, and your agents can create deeply personal images and understand your speech. Khoj is self-hostable and always will be.
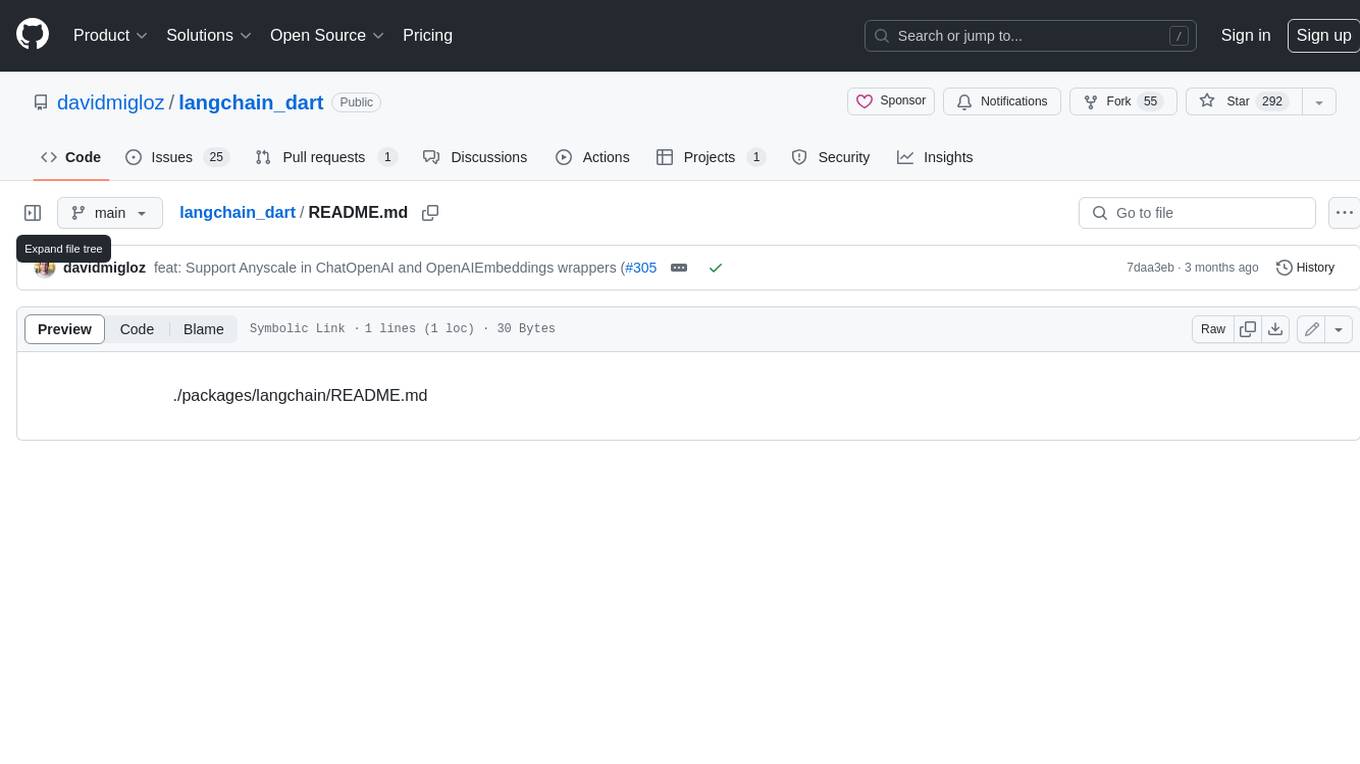
langchain_dart
LangChain.dart is a Dart port of the popular LangChain Python framework created by Harrison Chase. LangChain provides a set of ready-to-use components for working with language models and a standard interface for chaining them together to formulate more advanced use cases (e.g. chatbots, Q&A with RAG, agents, summarization, extraction, etc.). The components can be grouped into a few core modules: * **Model I/O:** LangChain offers a unified API for interacting with various LLM providers (e.g. OpenAI, Google, Mistral, Ollama, etc.), allowing developers to switch between them with ease. Additionally, it provides tools for managing model inputs (prompt templates and example selectors) and parsing the resulting model outputs (output parsers). * **Retrieval:** assists in loading user data (via document loaders), transforming it (with text splitters), extracting its meaning (using embedding models), storing (in vector stores) and retrieving it (through retrievers) so that it can be used to ground the model's responses (i.e. Retrieval-Augmented Generation or RAG). * **Agents:** "bots" that leverage LLMs to make informed decisions about which available tools (such as web search, calculators, database lookup, etc.) to use to accomplish the designated task. The different components can be composed together using the LangChain Expression Language (LCEL).
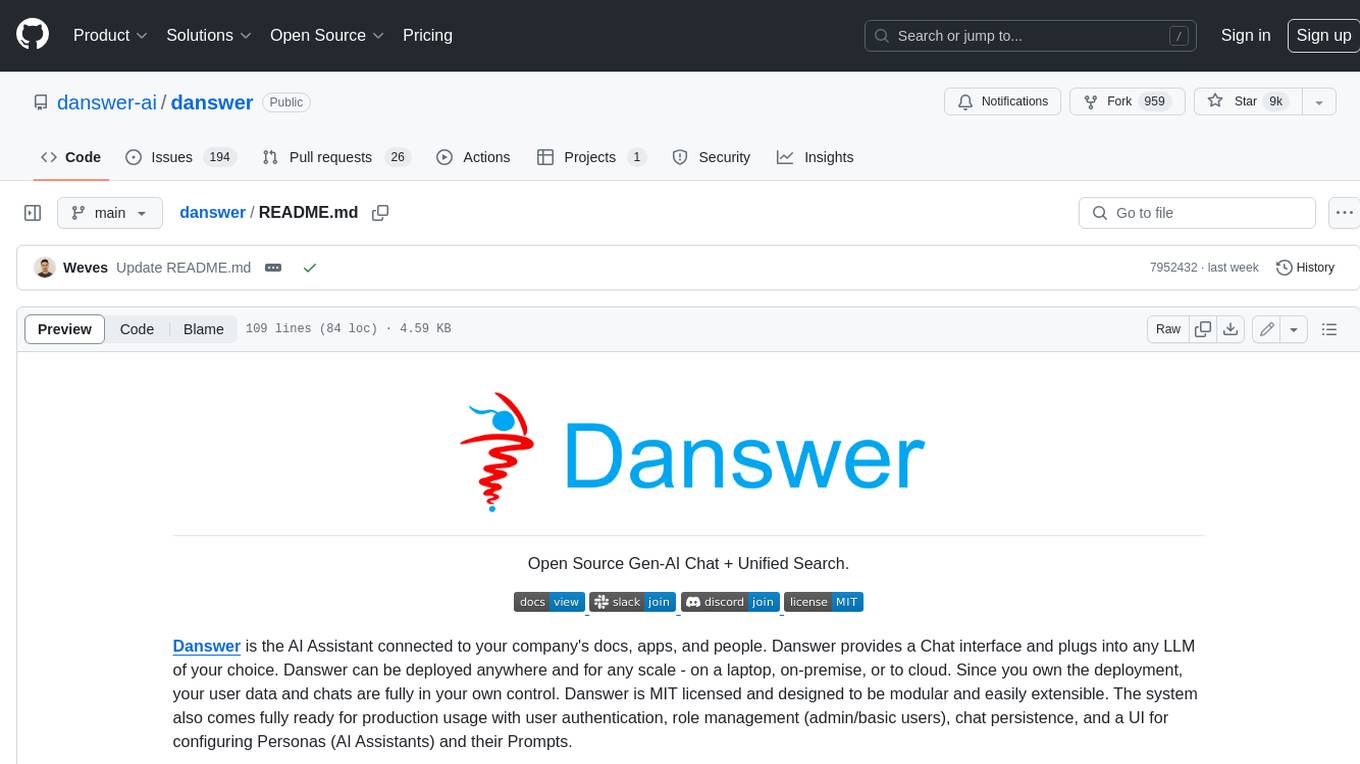
danswer
Danswer is an open-source Gen-AI Chat and Unified Search tool that connects to your company's docs, apps, and people. It provides a Chat interface and plugs into any LLM of your choice. Danswer can be deployed anywhere and for any scale - on a laptop, on-premise, or to cloud. Since you own the deployment, your user data and chats are fully in your own control. Danswer is MIT licensed and designed to be modular and easily extensible. The system also comes fully ready for production usage with user authentication, role management (admin/basic users), chat persistence, and a UI for configuring Personas (AI Assistants) and their Prompts. Danswer also serves as a Unified Search across all common workplace tools such as Slack, Google Drive, Confluence, etc. By combining LLMs and team specific knowledge, Danswer becomes a subject matter expert for the team. Imagine ChatGPT if it had access to your team's unique knowledge! It enables questions such as "A customer wants feature X, is this already supported?" or "Where's the pull request for feature Y?"
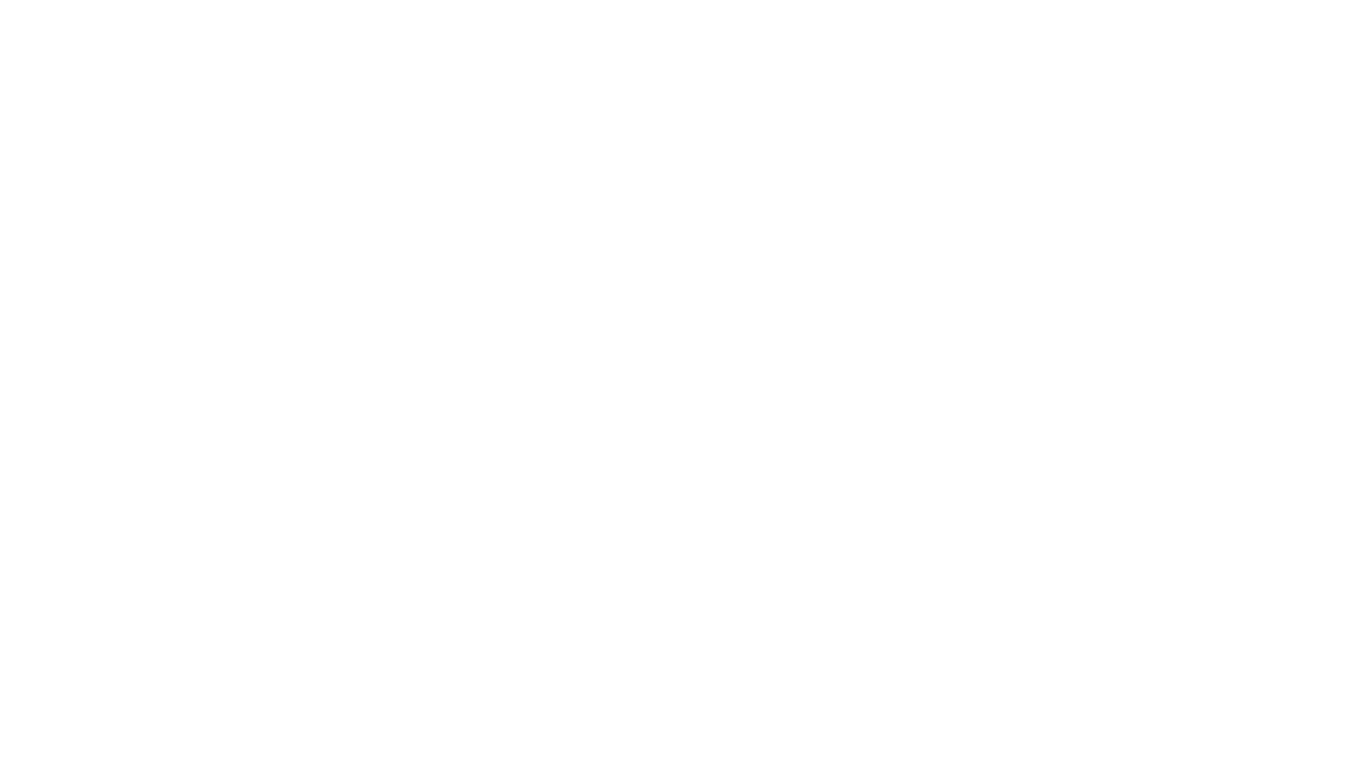
infinity
Infinity is an AI-native database designed for LLM applications, providing incredibly fast full-text and vector search capabilities. It supports a wide range of data types, including vectors, full-text, and structured data, and offers a fused search feature that combines multiple embeddings and full text. Infinity is easy to use, with an intuitive Python API and a single-binary architecture that simplifies deployment. It achieves high performance, with 0.1 milliseconds query latency on million-scale vector datasets and up to 15K QPS.
For similar jobs
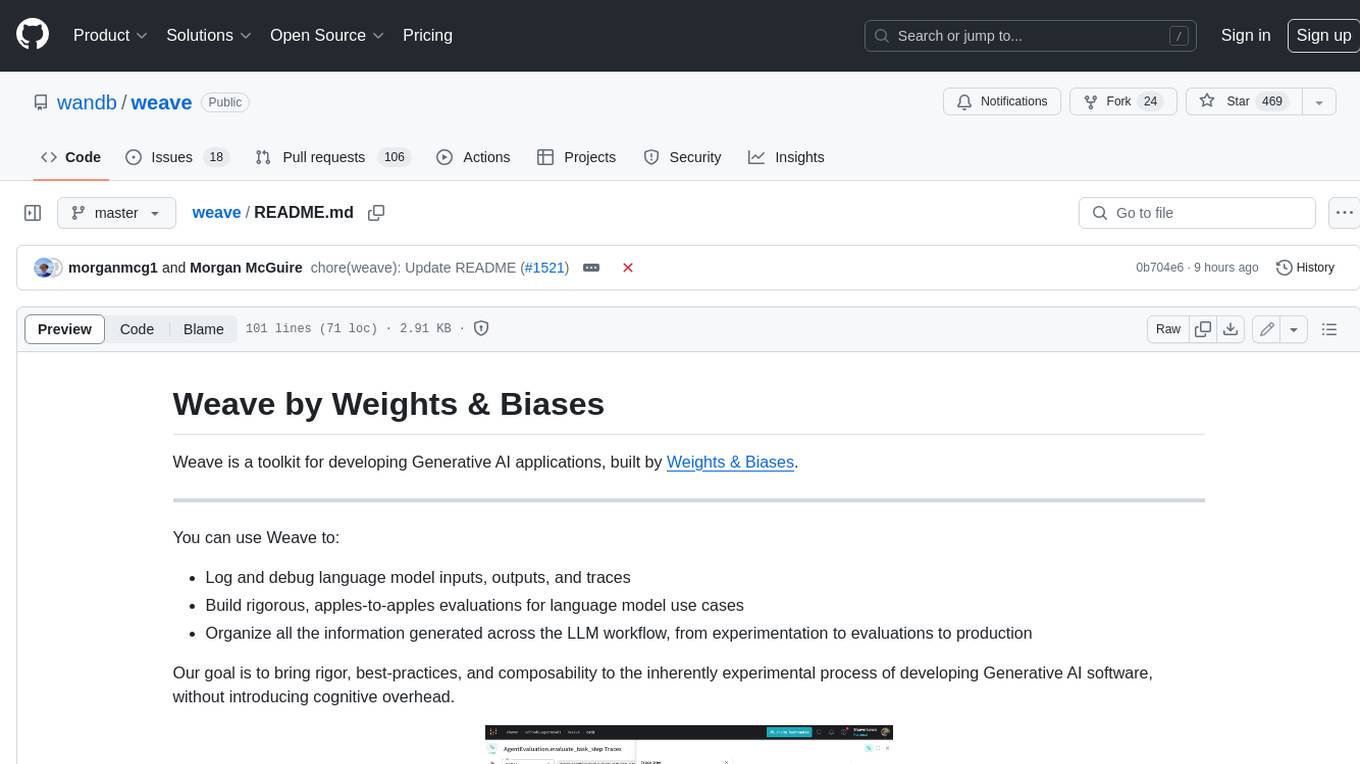
weave
Weave is a toolkit for developing Generative AI applications, built by Weights & Biases. With Weave, you can log and debug language model inputs, outputs, and traces; build rigorous, apples-to-apples evaluations for language model use cases; and organize all the information generated across the LLM workflow, from experimentation to evaluations to production. Weave aims to bring rigor, best-practices, and composability to the inherently experimental process of developing Generative AI software, without introducing cognitive overhead.
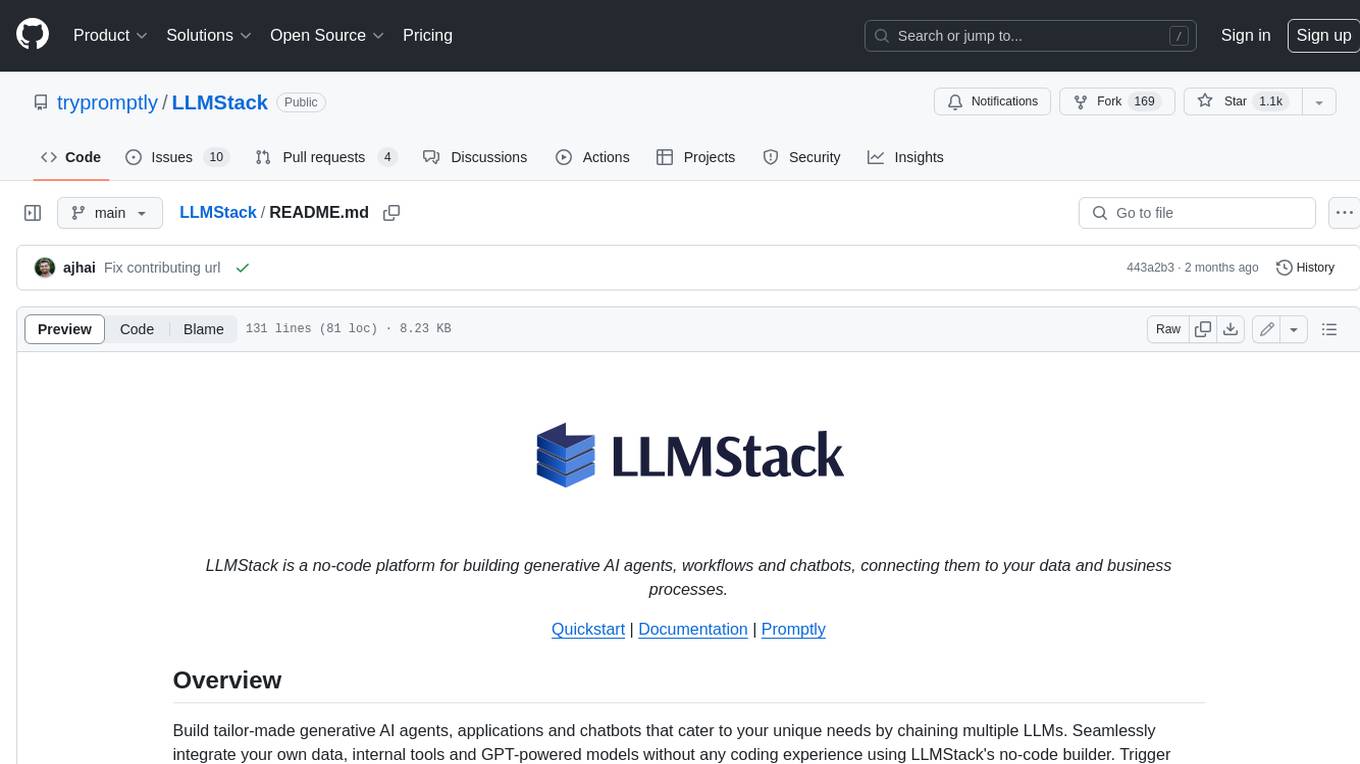
LLMStack
LLMStack is a no-code platform for building generative AI agents, workflows, and chatbots. It allows users to connect their own data, internal tools, and GPT-powered models without any coding experience. LLMStack can be deployed to the cloud or on-premise and can be accessed via HTTP API or triggered from Slack or Discord.
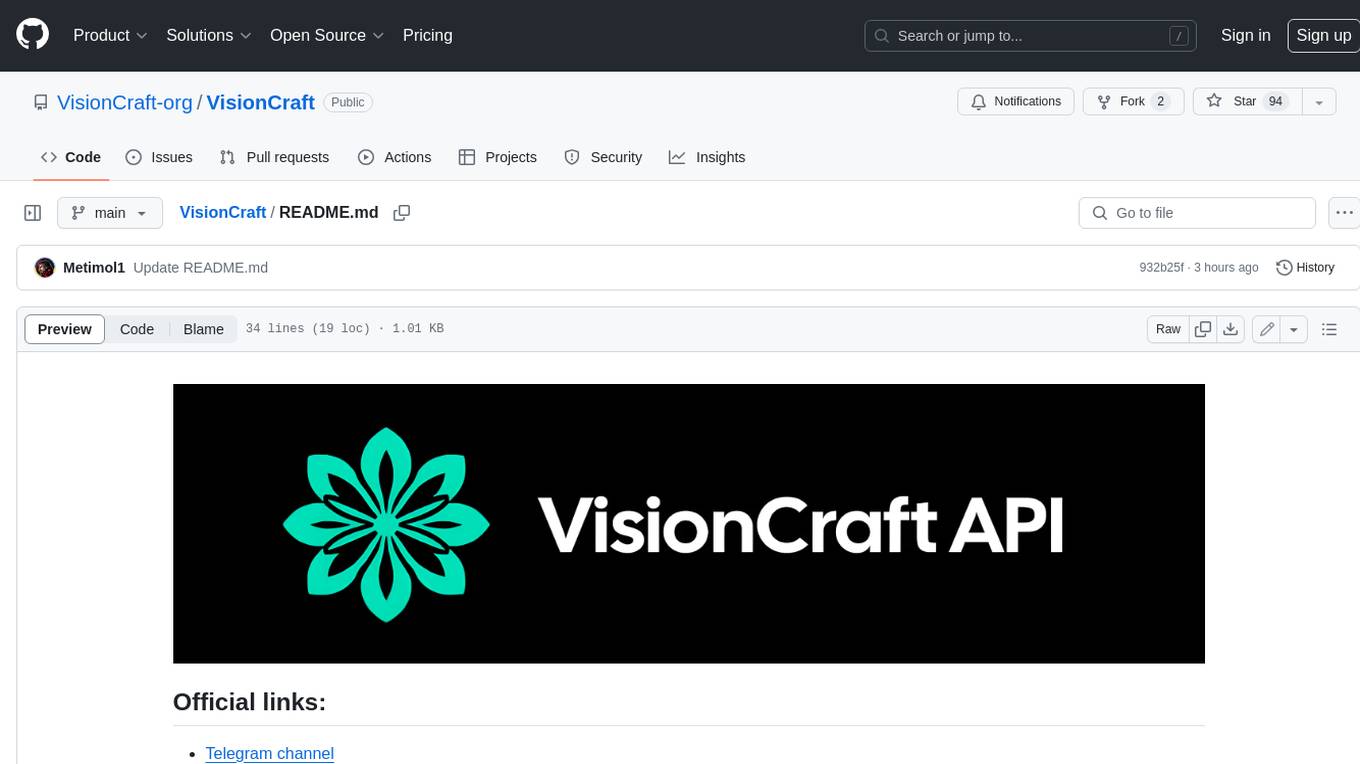
VisionCraft
The VisionCraft API is a free API for using over 100 different AI models. From images to sound.
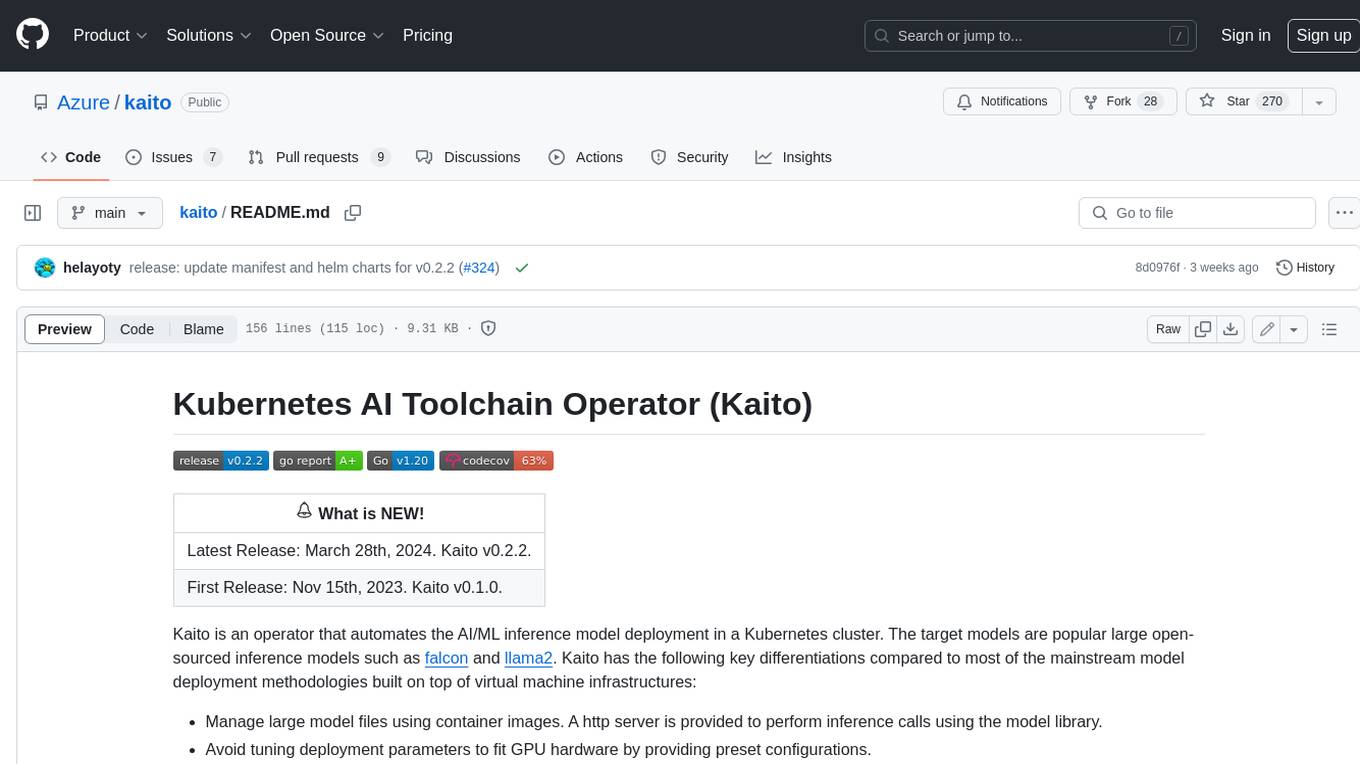
kaito
Kaito is an operator that automates the AI/ML inference model deployment in a Kubernetes cluster. It manages large model files using container images, avoids tuning deployment parameters to fit GPU hardware by providing preset configurations, auto-provisions GPU nodes based on model requirements, and hosts large model images in the public Microsoft Container Registry (MCR) if the license allows. Using Kaito, the workflow of onboarding large AI inference models in Kubernetes is largely simplified.
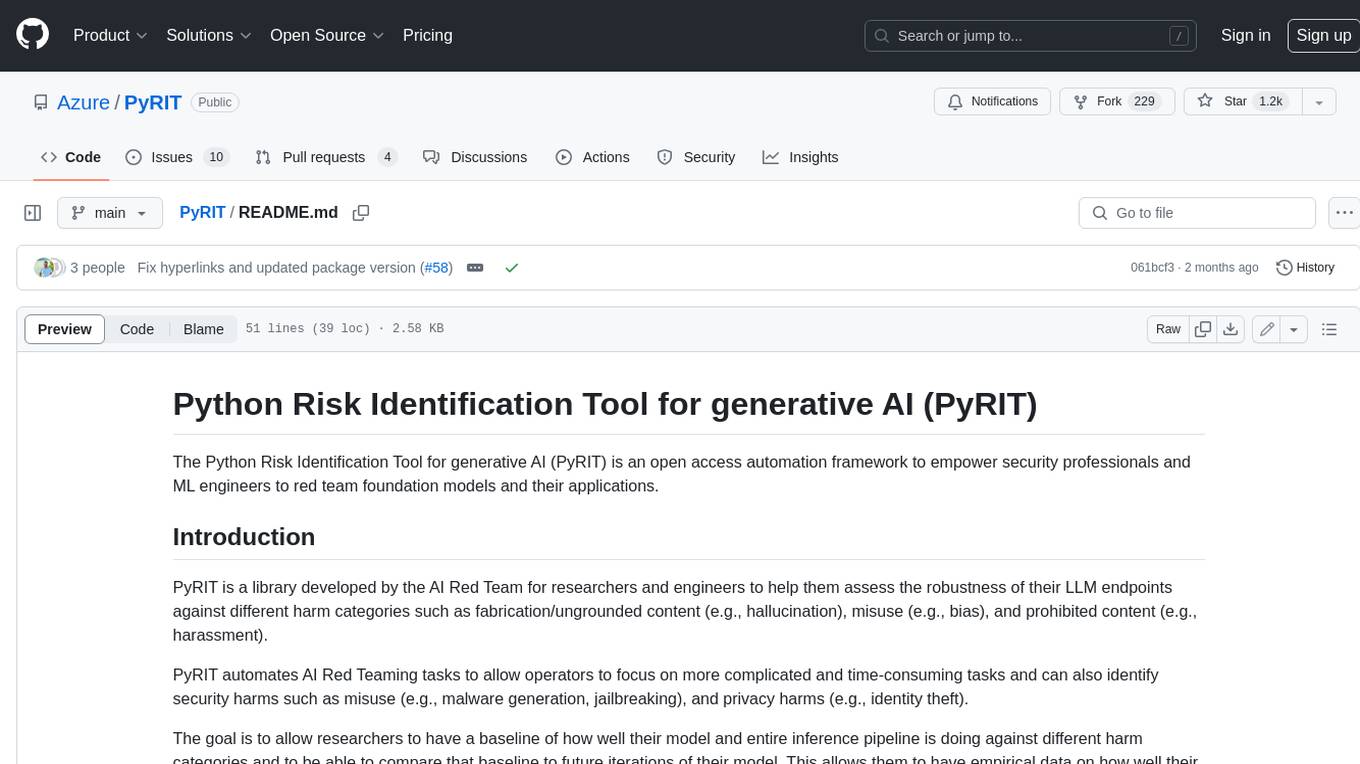
PyRIT
PyRIT is an open access automation framework designed to empower security professionals and ML engineers to red team foundation models and their applications. It automates AI Red Teaming tasks to allow operators to focus on more complicated and time-consuming tasks and can also identify security harms such as misuse (e.g., malware generation, jailbreaking), and privacy harms (e.g., identity theft). The goal is to allow researchers to have a baseline of how well their model and entire inference pipeline is doing against different harm categories and to be able to compare that baseline to future iterations of their model. This allows them to have empirical data on how well their model is doing today, and detect any degradation of performance based on future improvements.
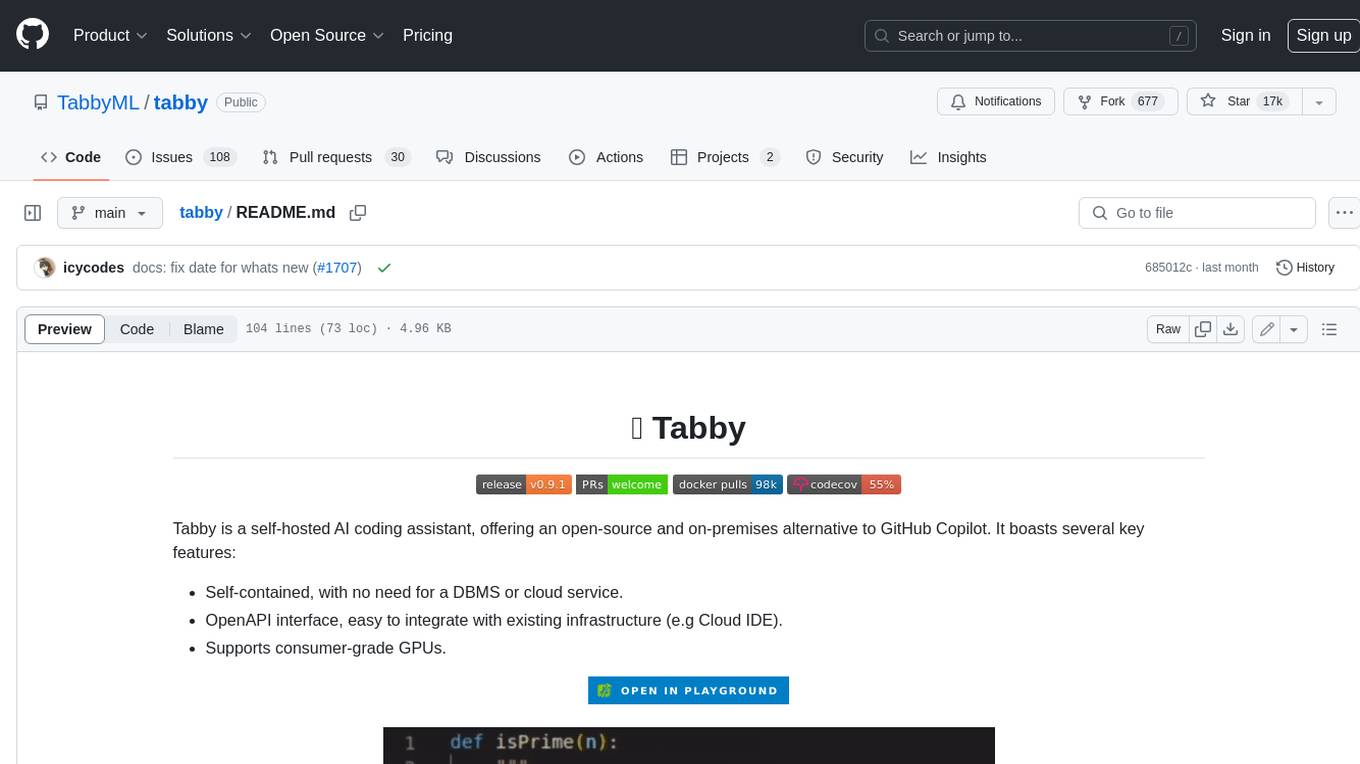
tabby
Tabby is a self-hosted AI coding assistant, offering an open-source and on-premises alternative to GitHub Copilot. It boasts several key features: * Self-contained, with no need for a DBMS or cloud service. * OpenAPI interface, easy to integrate with existing infrastructure (e.g Cloud IDE). * Supports consumer-grade GPUs.
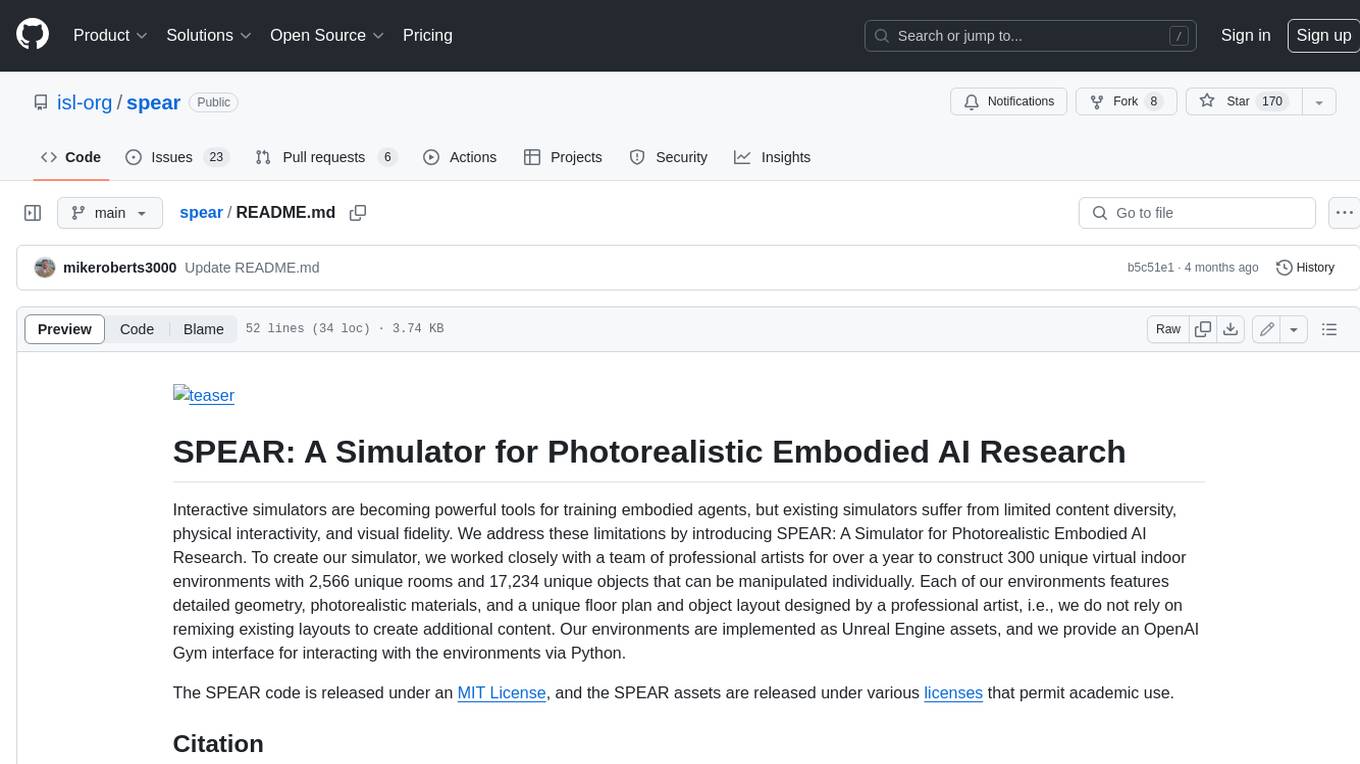
spear
SPEAR (Simulator for Photorealistic Embodied AI Research) is a powerful tool for training embodied agents. It features 300 unique virtual indoor environments with 2,566 unique rooms and 17,234 unique objects that can be manipulated individually. Each environment is designed by a professional artist and features detailed geometry, photorealistic materials, and a unique floor plan and object layout. SPEAR is implemented as Unreal Engine assets and provides an OpenAI Gym interface for interacting with the environments via Python.
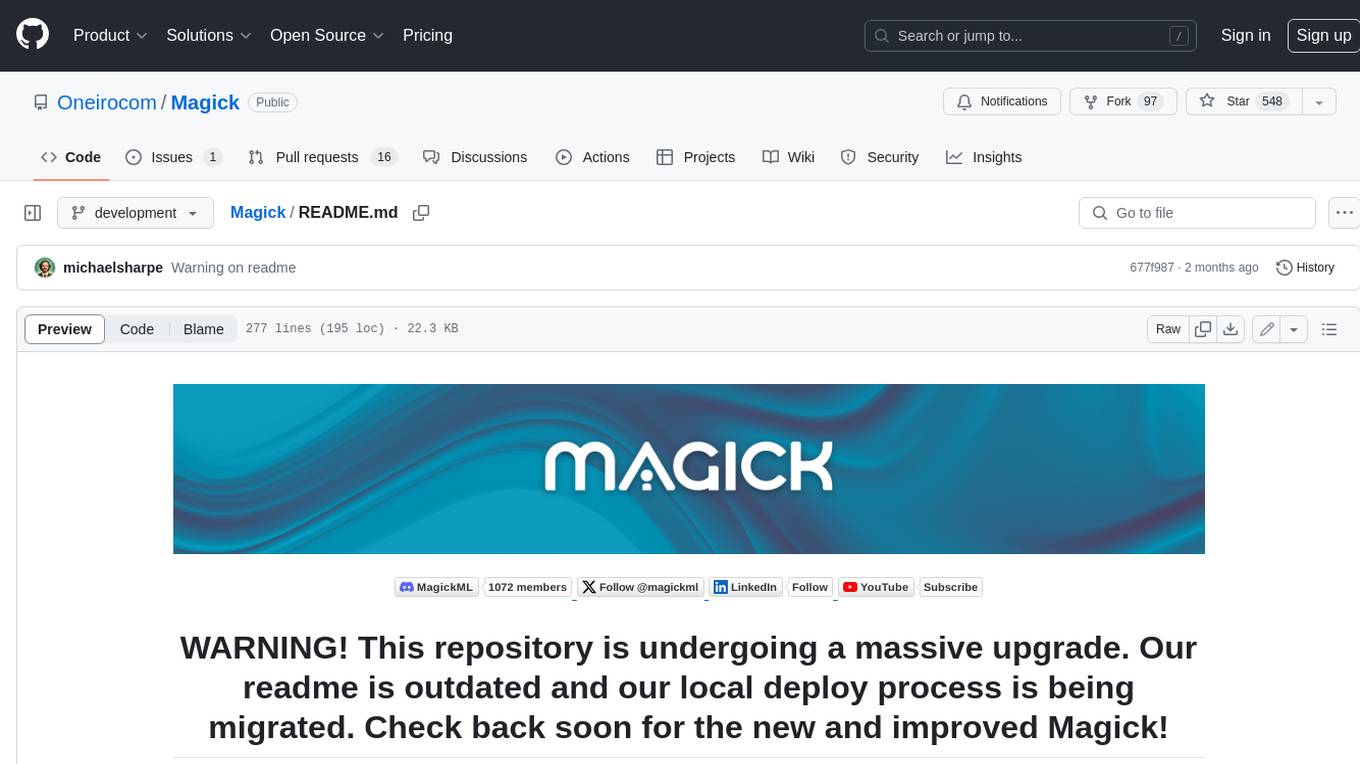
Magick
Magick is a groundbreaking visual AIDE (Artificial Intelligence Development Environment) for no-code data pipelines and multimodal agents. Magick can connect to other services and comes with nodes and templates well-suited for intelligent agents, chatbots, complex reasoning systems and realistic characters.