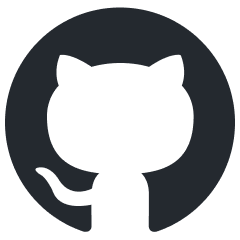
ciso-assistant-community
CISO Assistant is a one-stop-shop for GRC, covering Risk, AppSec and Audit Management and supporting +70 frameworks worldwide with auto-mapping: NIST CSF, ISO 27001, SOC2, CIS, PCI DSS, NIS2, CMMC, PSPF, GDPR, HIPAA, Essential Eight, NYDFS-500, DORA, NIST AI RMF, 800-53, 800-171, CyFun, CJIS, AirCyber, NCSC, ECC, SCF and so much more
Stars: 2563
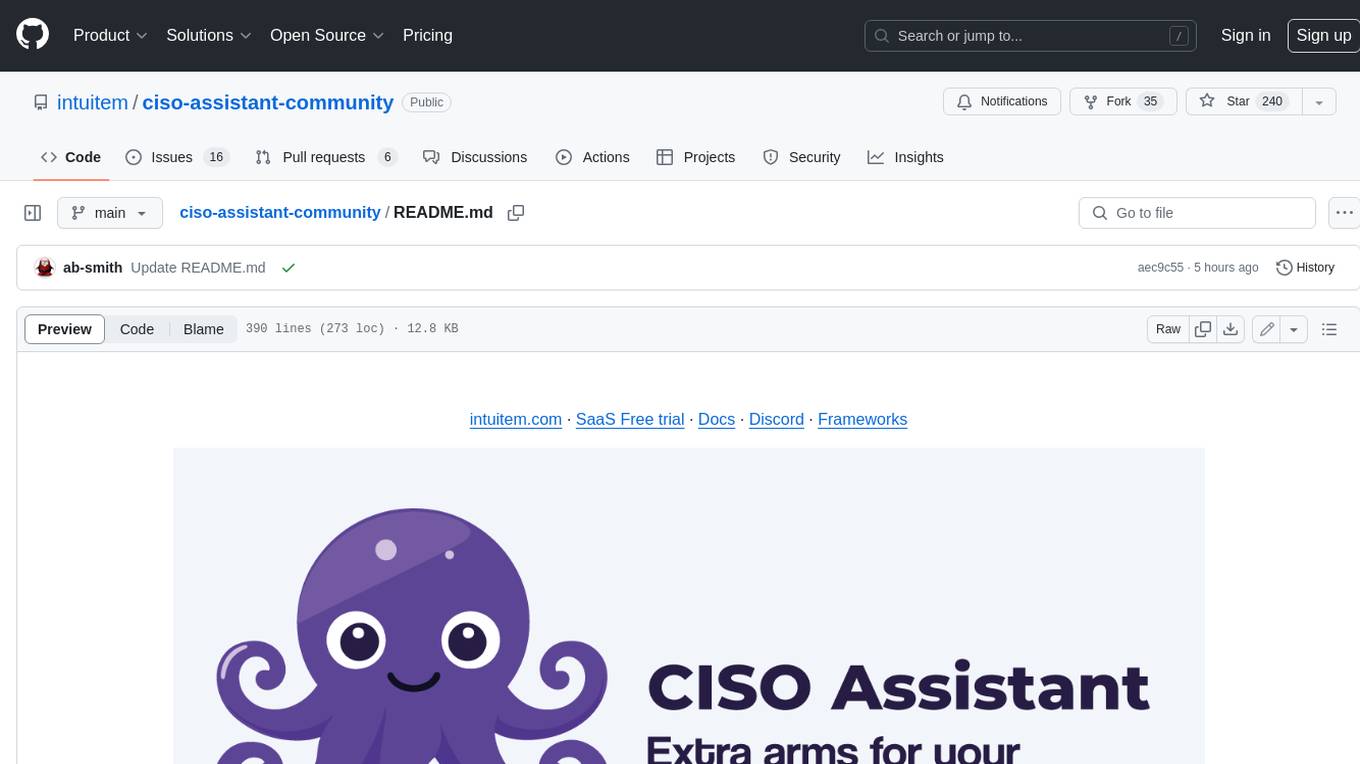
CISO Assistant is a tool that helps organizations manage their cybersecurity posture and compliance. It provides a centralized platform for managing security controls, threats, and risks. CISO Assistant also includes a library of pre-built frameworks and tools to help organizations quickly and easily implement best practices.
README:
Star the project 🌟 to get releases notification and help growing the community!
intuitem.com
·
SaaS Free trial
·
Roadmap
·
Docs
·
Languages
·
Discord
·
Frameworks
CISO Assistant brings a different take to GRC and Cyber Security Posture Management:
- by explicitly decoupling compliance from cybersecurity controls implementation
- has built-in standards, security controls and threats
- risk assessment and remediation plan follow-up
- allows to manage a catalog for security controls and threats
- you can bring your own framework as well using a simple syntax
- manage audit, evidences collection and report generation
Our vision is to provide a one stop shop for cyber security posture management and cover the layers of GRC (Governance, Risk and Compliance). As practitioners interacting with multiple cybersecurity and IT professionals, we have struggled with fragmentation and lack of efficient tooling. We keep improving CISO Assistant with anything that could bring clarity and productivity to cybersecurity teams and reduce the effort of research, audit management and paperwork.
CyberSecurity teams need to use GRC as a foundation to structure their program and implement the right tools and processes to mitigate the risks, and leave the rest to CISO Assistant 🐙
The vision of the tool is based on these fundamental objects:
There are other concepts and models to provide other features. The full details are available in the data model.
The decoupling concept is a pillar of the app and allows you to save a considerable amount of time:
- reuse previous assessments,
- assess a scope against multiple frameworks at the same time,
- leave the reporting formatting and sanity check to CISO assistant and focus on your fixes,
- balance controls implementation and compliance follow-up
Here is an illustration of the decoupling principle and its advantages:
https://github.com/user-attachments/assets/87bd4497-5cc2-4221-aeff-396f6b6ebe62
Here is an overview of CISO Assistant features and capabilities:
CISO Assistant is developed and maintained by intuitem, a French 🇫🇷 company specialized in Cyber Security, Cloud and Data/AI.
[!TIP] The easiest way to get started is through the free trial of cloud instance available here.
Alternatively, once you have Docker and Docker-compose installed, on your workstation or server:
clone the repo:
git clone https://github.com/intuitem/ciso-assistant-community.git
and run the starter script
./docker-compose.sh
If you are looking for other installation options, you might want to check the docs.
[!NOTE] The docker-compose script uses prebuilt Docker images supporting most of the standard hardware architecture. If you're using Windows, Make sure to have WSL installed and trigger the script within a WSL command line. It will feed Docker Desktop on your behalf.
The docker compose file can be adjusted to pass extra parameters to suit your setup (e.g. Mailer settings).
[!WARNING] If you're getting warnings or errors about image's platform not matching host platform, raise an issue with the details and we'll add it shortly after. You can also use
docker-compose-build.sh
instead (see below) to build for your specific architecture.
[!CAUTION] Don't use the
main
branch code directly for production as it's the merge upstream and can have breaking changes during our development. Either use thetags
for stable versions or prebuilt images.
Check out the online documentation on https://intuitem.gitbook.io/ciso-assistant.
- ISO 27001:2022 🌐
- NIST Cyber Security Framework (CSF) v1.1 🇺🇸
- NIST Cyber Security Framework (CSF) v2.0 🇺🇸
- NIS2 🇪🇺
- SOC2 🇺🇸
- PCI DSS 4.0 💳
- CMMC v2 🇺🇸
- PSPF 🇦🇺
- General Data Protection Regulation (GDPR): Full text and checklist from GDPR.EU 🇪🇺
- Essential Eight 🇦🇺
- NYDFS 500 with 2023-11 amendments 🇺🇸
- DORA (Act, RTS, ITS and GL) 🇪🇺
- NIST AI Risk Management Framework 🇺🇸🤖
- NIST SP 800-53 rev5 🇺🇸
- France LPM/OIV rules 🇫🇷
- CCB CyberFundamentals Framework 🇧🇪
- NIST SP-800-66 (HIPAA) 🏥
- HDS/HDH 🇫🇷
- OWASP Application Security Verification Standard (ASVS) 🐝🖥️
- RGS v2.0 🇫🇷
- AirCyber
✈️ 🌐 - Cyber Resilience Act (CRA) 🇪🇺
- TIBER-EU 🇪🇺
- NIST Privacy Framework 🇺🇸
- TISAX (VDA ISA) v5.1 and v6.0 🚘
- ANSSI hygiene guide 🇫🇷
- Essential Cybersecurity Controls (ECC) 🇸🇦
- CIS Controls v8* 🌐
- CSA CCM (Cloud Controls Matrix)* ☁️
- FADP (Federal Act on Data Protection) 🇨🇭
- NIST SP 800-171 rev2 (2021) 🇺🇸
- ANSSI : recommandations de sécurité pour un système d'IA générative 🇫🇷🤖
- NIST SP 800-218: Secure Software Development Framework (SSDF) 🖥️
- GSA FedRAMP rev5 ☁️🇺🇸
- Cadre Conformité Cyber France (3CF) v1 (2021)
✈️ 🇫🇷 - ANSSI : SecNumCloud ☁️🇫🇷
- Cadre Conformité Cyber France (3CF) v2 (2024)
✈️ 🇫🇷 - ANSSI : outil d’autoévaluation de gestion de crise cyber 💥🇫🇷
- BSI: IT-Grundschutz-Kompendium 🇩🇪
- NIST SP 800-171 rev3 (2024) 🇺🇸
- ENISA: 5G Security Controls Matrix 🇪🇺
- OWASP Mobile Application Security Verification Standard (MASVS) 🐝📱
- Agile Security Framework (ASF) - baseline - by intuitem 🤗
- ISO 27001:2013 🌐 (For legacy and migration)
- EU AI Act 🇪🇺🤖
- FBI CJIS 🇺🇸👮
- Operational Technology Cybersecurity Controls (OTCC) 🇸🇦
- Secure Controls Framework (SCF) 🇺🇸🌐
- NCSC Cyber Assessment Framework (CAF) 🇬🇧
- California Consumer Privacy Act (CCPA) 🇺🇸
- California Consumer Privacy Act Regulations 🇺🇸
- NCSC Cyber Essentials 🇬🇧
- Directive Nationale de la Sécurité des Systèmes d'Information (DNSSI) Maroc 🇲🇦
- Part-IS
✈️ 🇪🇺 - ENS Esquema Nacional de seguridad 🇪🇸
- Korea ISA ISMS-P 🇰🇷
- Swiss ICT minimum standard 🇨🇭
- Adobe Common Controls Framework (CCF) 🌐
- BSI Cloud Computing Compliance Criteria Catalogue (C5) 🇩🇪
- Référentiel d’Audit de la Sécurité des Systèmes d’Information, ANCS Tunisie 🇹🇳
- ECB Cyber resilience oversight expectations for financial market infrastructures 🇪🇺
- Mindeststandard-des-BSI-zur-Nutzung-externer-Cloud-Dienste (Version 2.1) 🇩🇪
- Formulaire d'évaluation de la maturité - niveau fondamental (DGA) 🇫🇷
- NIS2 technical and methodological requirements 2024/2690 🇪🇺
- Saudi Arabian Monetary Authority (SAMA) Cybersecurity Framework 🇸🇦
- Guide de sécurité des données (CNIL) 🇫🇷
- International Traffic in Arms Regulations (ITAR) 🇺🇸
- Federal Trade Commission (FTC) Standards for Safeguarding Customer Information 🇺🇸
- OWASP's checklist for LLM governance and security 🌐
- Recommandations pour les architectures des systèmes d’information sensibles ou à diffusion restreinte (ANSSI) 🇫🇷
- PGSSI-S (Politique Générale de Sécurité des Systèmes d'Information de Santé) 🇫🇷
- ANSSI : Recommandations de configuration d'un système GNU/Linux 🇫🇷
- PSSI-MCAS (Politique de sécurité des systèmes d’information pour les ministères chargés des affaires sociales) 🇫🇷
- ANSSI : Recommandations pour la protection des systèmes d'information essentiels 🇫🇷
- ANSSI : Recommandations de sécurité pour l'architecture d'un système de journalisation 🇫🇷
- ANSSI : Recommandations de sécurité relatives à TLS 🇫🇷
- New Zealand Information Security Manual (NZISM) 🇳🇿
- Clausier de sécurité numérique du Club RSSI Santé 🇫🇷
- Référentiel National de Sécurité de l’Information (RNSI), MPT Algérie 🇩🇿
- Misure minime di sicurezza ICT per le pubbliche amministrazioni, AGID Italia 🇮🇹
- Framework Nazionale CyberSecurity v2, FNCS Italia 🇮🇹
[!NOTE]
*
These frameworks require an extra manual step of getting the latest Excel sheet through their website as their license prevent direct usage.
Checkout the library and tools for the Domain Specific Language used and how you can define your own.
-
Indonesia PDP 🇮🇩
-
COBAC R-2024/01
-
ICO Data protection self-assessment 🇬🇧
-
NIST 800-82
-
ASD ISM 🇦🇺
-
Baseline informatiebeveiliging Overheid (BIO) 🇳🇱
-
and much more: just ask on Discord. If it's an open standard, we'll do it for you, free of charge 😉
A library can be a framework, a catalog of threats or reference controls, and even a custom risk matrix.
Take a look at the tools
directory and its dedicated README. The convert_library.py
script will help you create your library from a simple Excel file. Once you have structured your items in that format, just run the script and use the resulting yaml file.
You can also find some specific converters in the tools directory (e.g. for CIS or CCM Controls).
There is also a tool to facilitate the creation of mappings, called prepare_mapping.py
that will create an Excel file based on two framework libraries in yaml. Once properly filled, this Excel file can be processed by the convert_library.py
tool to get the resulting mapping library.
Join our open Discord community to interact with the team and other GRC experts.
The fastest and easiest way to get started is through the free trial of cloud instance available here.
To run CISO Assistant locally in a straightforward way, you can use Docker compose.
- Update docker
Make sure you have a recent version of docker (>= 25.0).
- Clone the repository
git clone https://github.com/intuitem/ciso-assistant-community.git
cd ciso-assistant-community
- Launch docker-compose script for prebuilt images:
./docker-compose.sh
Alternatively, you can use this variant to build the docker images for your specific architecture:
./docker-compose-build.sh
When asked for, enter your email and password for your superuser.
You can then reach CISO Assistant using your web browser at https://localhost:8443/
For the following executions, use "docker compose up" directly.
[!TIP] If you want a fresh install, simply delete the
db
directory, (default: backend/db) where the database is stored.
For docker setup on a remote server or hypervisor, checkout the specific instructions here
- Python 3.11+
- pip 20.3+
- poetry 1.8+
- node 18+
- npm 10.2+
- pnpm 9.0+
- yaml-cpp (brew install yaml-cpp libyaml or apt install libyaml-cpp-dev)
- Clone the repository.
git clone [email protected]:intuitem/ciso-assistant-community.git
cd ciso-assistant-community
- Create a file in the parent folder (e.g. ../myvars) and store your environment variables within it by copying and modifying the following code and replace
"<XXX>"
by your private values. Take care not to commit this file in your git repo.
Mandatory variables
All variables in the backend have handy default values.
Recommended variables
export DJANGO_DEBUG=True
# Default url is set to http://localhost:5173 but you can change it, e.g. to use https with a caddy proxy
export CISO_ASSISTANT_URL=https://localhost:8443
# Setup a development mailer with Mailhog for example
export EMAIL_HOST_USER=''
export EMAIL_HOST_PASSWORD=''
export [email protected]
export EMAIL_HOST=localhost
export EMAIL_PORT=1025
export EMAIL_USE_TLS=True
Other variables
# CISO Assistant will use SQLite by default, but you can setup PostgreSQL by declaring these variables
export POSTGRES_NAME=ciso-assistant
export POSTGRES_USER=ciso-assistantuser
export POSTGRES_PASSWORD=<XXX>
export POSTGRES_PASSWORD_FILE=<XXX> # alternative way to specify password
export DB_HOST=localhost
export DB_PORT=5432 # optional, default value is 5432
# Add a second backup mailer
export EMAIL_HOST_RESCUE=<XXX>
export EMAIL_PORT_RESCUE=587
export EMAIL_HOST_USER_RESCUE=<XXX>
export EMAIL_HOST_PASSWORD_RESCUE=<XXX>
export EMAIL_USE_TLS_RESCUE=True
# You can define the email of the first superuser, useful for automation. A mail is sent to the superuser for password initialization
export CISO_SUPERUSER_EMAIL=<XXX>
# By default, Django secret key is generated randomly at each start of CISO Assistant. This is convenient for quick test,
# but not recommended for production, as it can break the sessions (see
# this [topic](https://stackoverflow.com/questions/15170637/effects-of-changing-djangos-secret-key) for more information).
# To set a fixed secret key, use the environment variable DJANGO_SECRET_KEY.
export DJANGO_SECRET_KEY=...
# Logging configuration
export LOG_LEVEL=INFO # optional, default value is INFO. Available options: DEBUG, INFO, WARNING, ERROR, CRITICAL
export LOG_FORMAT=plain # optional, default value is plain. Available options: json, plain
# Authentication options
export AUTH_TOKEN_TTL=900 # optional, default value is 3600 seconds (60 minutes). It defines the time to live of the authentication token
export AUTH_TOKEN_AUTO_REFRESH=True # optional, default value is True. It defines if the token TTL should be refreshed automatically after each request authenticated with the token
- Install poetry
Visit the poetry website for instructions: https://python-poetry.org/docs/#installation
- Install required dependencies.
poetry install
- Recommended: Install the pre-commit hooks.
pre-commit install
- If you want to setup Postgres:
- Launch one of these commands to enter in Postgres:
psql as superadmin
sudo su postgres
psql
- Create the database "ciso-assistant"
create database ciso-assistant;
- Create user "ciso-assistantuser" and grant it access
create user ciso-assistantuser with password '<POSTGRES_PASSWORD>';
grant all privileges on database ciso-assistant to ciso-assistantuser;
- Apply migrations.
poetry run python manage.py migrate
- Create a Django superuser, that will be CISO Assistant administrator.
If you have set a mailer and CISO_SUPERUSER_EMAIL variable, there's no need to create a Django superuser with
createsuperuser
, as it will be created automatically on first start. You should receive an email with a link to setup your password.
poetry run python manage.py createsuperuser
- Run development server.
poetry run python manage.py runserver
- Configure the git hooks for generating the build name.
cd .git/hooks
ln -fs ../../git_hooks/post-commit .
ln -fs ../../git_hooks/post-merge .
- for Huey (tasks runner)
- prepare a mailer for testing.
- run
python manage.py run_huey -w 2 -k process
or equivalent in a separate shell. - you can use
MAIL_DEBUG
to have mail on the console for easier debug
- cd into the frontend directory
cd frontend
- Install dependencies
npm install -g pnpm
pnpm install
- Start a development server (make sure that the django app is running)
pnpm run dev
- Reach the frontend on http://localhost:5173
[!NOTE] Safari will not properly work in this setup, as it requires https for secure cookies. The simplest solution is to use Chrome or Firefox. An alternative is to use a caddy proxy. This is the solution used in docker-compose, so you can use it as an example.
- Environment variables
All variables in the frontend have handy default values.
If you move the frontend on another host, you should set the following variable: PUBLIC_BACKEND_API_URL. Its default value is http://localhost:8000/api.
The PUBLIC_BACKEND_API_EXPOSED_URL is necessary for proper functioning of the SSO. It points to the URL of the API as seen from the browser. It should be equal to the concatenation of CISO_ASSISTANT_URL (in the backend) with "/api".
When you launch "node server" instead of "pnpm run dev", you need to set the ORIGIN variable to the same value as CISO_ASSISTANT_URL in the backend (e.g. http://localhost:3000).
The migrations are tracked by version control, https://docs.djangoproject.com/en/4.2/topics/migrations/#version-control
For the first version of the product, it is recommended to start from a clean migration.
Note: to clean existing migrations, type:
find . -path "*/migrations/*.py" -not -name "__init__.py" -delete
find . -path "*/migrations/*.pyc" -delete
After a change (or a clean), it is necessary to re-generate migration files:
poetry run python manage.py makemigrations
poetry run python manage.py migrate
These migration files should be tracked by version control.
To run API tests on the backend, simply type "pytest" in a shell in the backend folder.
To run functional tests on the frontend, do the following actions:
- in the frontend folder, launch the following command:
tests/e2e-tests.sh
The goal of the test harness is to prevent any regression, i.e. all the tests shall be successful, both for backend and frontend.
- The API documentation is available in dev mode on the
<backend_endpoint>/api/schema/swagger/
, for instance http://127.0.0.1:8000/api/schema/swagger/
To interact with it:
- call
/api/iam/login/
with your credentials in the body to get the token - pass it then as a header
Authorization: Token {token}
for your next calls. Notice it's Token not Bearer.
The docker-compose-prod.yml highlights a relevant configuration with a Caddy proxy in front of the frontend. It exposes API calls only for SSO. Note that docker-compose.yml exposes the full API, which is not yet recommended for production.
Set DJANGO_DEBUG=False for security reason.
[!NOTE] The frontend cannot infer the host automatically, so you need to either set the ORIGIN variable, or the HOST_HEADER and PROTOCOL_HEADER variables. Please see the sveltekit doc on this tricky issue. Beware that this approach does not work with "pnpm run dev", which should not be a worry for production.
[!NOTE] Caddy needs to receive a SNI header. Therefore, for your public URL (the one declared in CISO_ASSISTANT_URL), you need to use a FQDN, not an IP address, as the SNI is not transmitted by a browser if the host is an IP address. Another tricky issue!
- FR: French
- EN: English
- AR: Arabic
- PT: Portuguese
- ES: Spanish
- DE: German
- NL: Dutch
- IT: Italian
- PL: Polish
- RO: Romanian
- HI: Hindi
- UR: Urdu
- CS: Czech
- SV: Swedish
- ID: Indonesian
- Django - Python Web Development Framework
- SvelteKit - Frontend Framework
- eCharts - Charting library
- Gunicorn - Python WSGI HTTP Server for UNIX
- Caddy - The coolest reverse Proxy
- Gitbook - Documentation platform
- PostgreSQL - Open Source RDBMS
- SQLite - Open Source RDBMS
- Docker - Container Engine
- inlang - The ecosystem to globalize your software
Great care has been taken to follow security best practices. Please report any issue to [email protected].
This repository contains the source code for both the Open Source edition of CISO Assistant (Community Edition), released under the AGPL v3, as well as the commercial edition of CISO Assistant (Pro and Enterprise Editions), released under the intuitem Commercial Software License. This mono-repository approach is adopted for simplicity.
All the files within the top-level "enterprise" directory are released under the intuitem Commercial Software License.
All the files outside the top-level "enterprise" directory are released under the AGPLv3.
See LICENSE.md for details. For more details about the commercial editions, you can reach us on [email protected].
Unless otherwise noted, all files are © intuitem.
For Tasks:
Click tags to check more tools for each tasksFor Jobs:
Alternative AI tools for ciso-assistant-community
Similar Open Source Tools
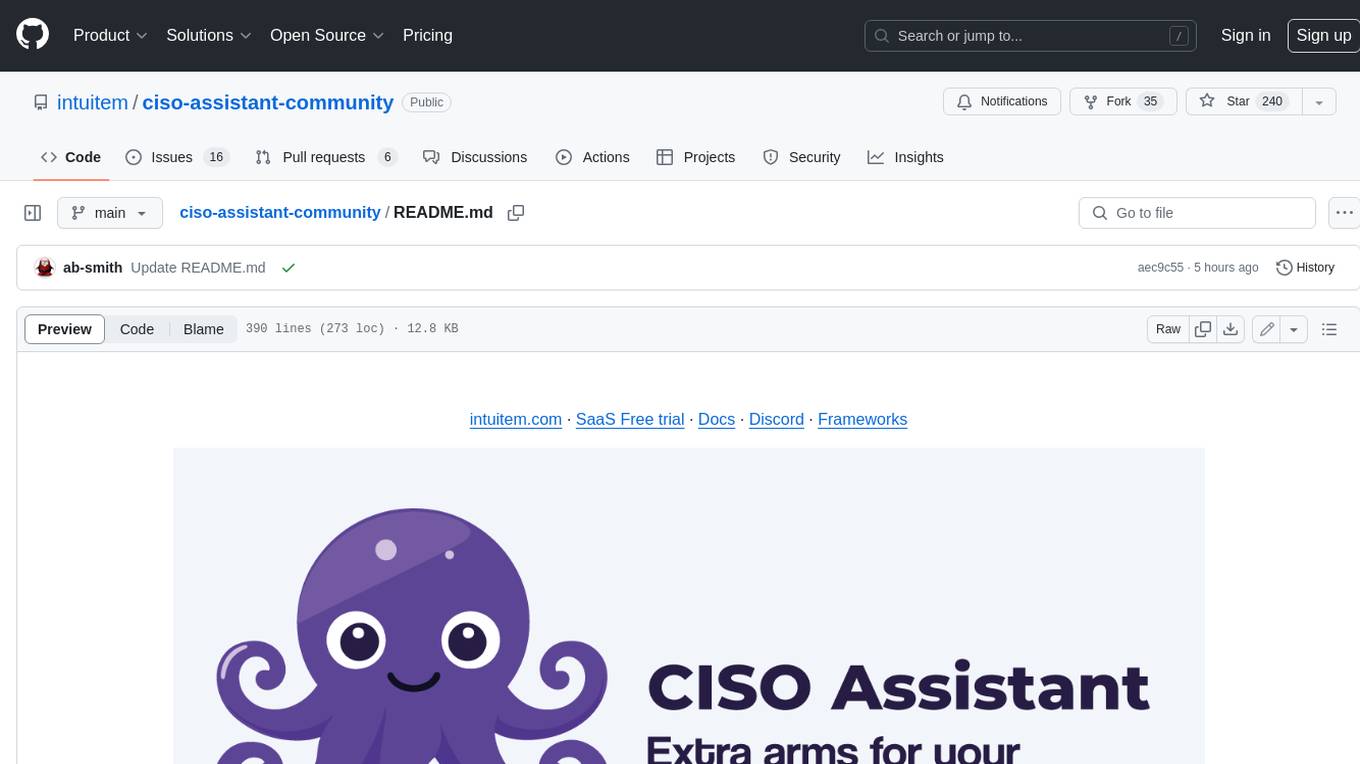
ciso-assistant-community
CISO Assistant is a tool that helps organizations manage their cybersecurity posture and compliance. It provides a centralized platform for managing security controls, threats, and risks. CISO Assistant also includes a library of pre-built frameworks and tools to help organizations quickly and easily implement best practices.
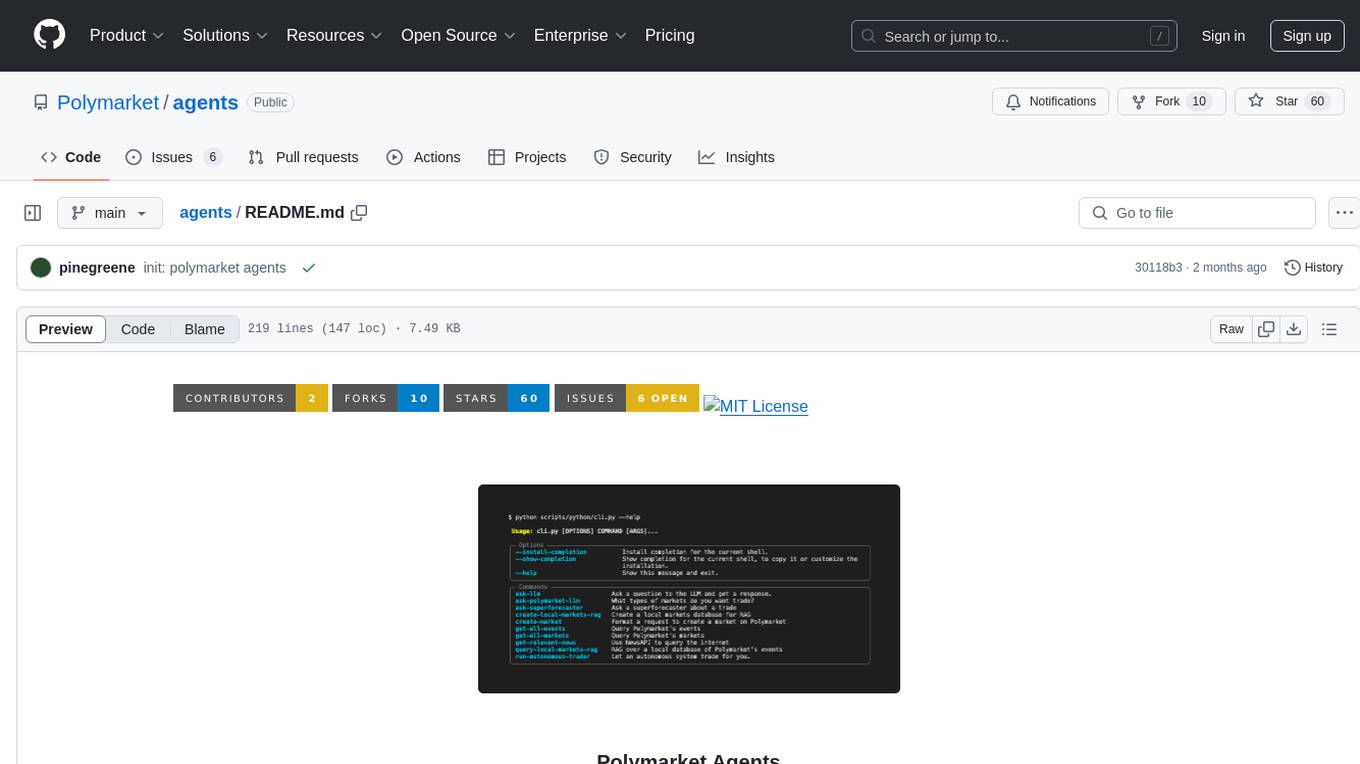
agents
Polymarket Agents is a developer framework and set of utilities for building AI agents to trade autonomously on Polymarket. It integrates with Polymarket API, provides AI agent utilities for prediction markets, supports local and remote RAG, sources data from various services, and offers comprehensive LLM tools for prompt engineering. The architecture features modular components like APIs and scripts for managing local environments, server set-up, and CLI for end-user commands.
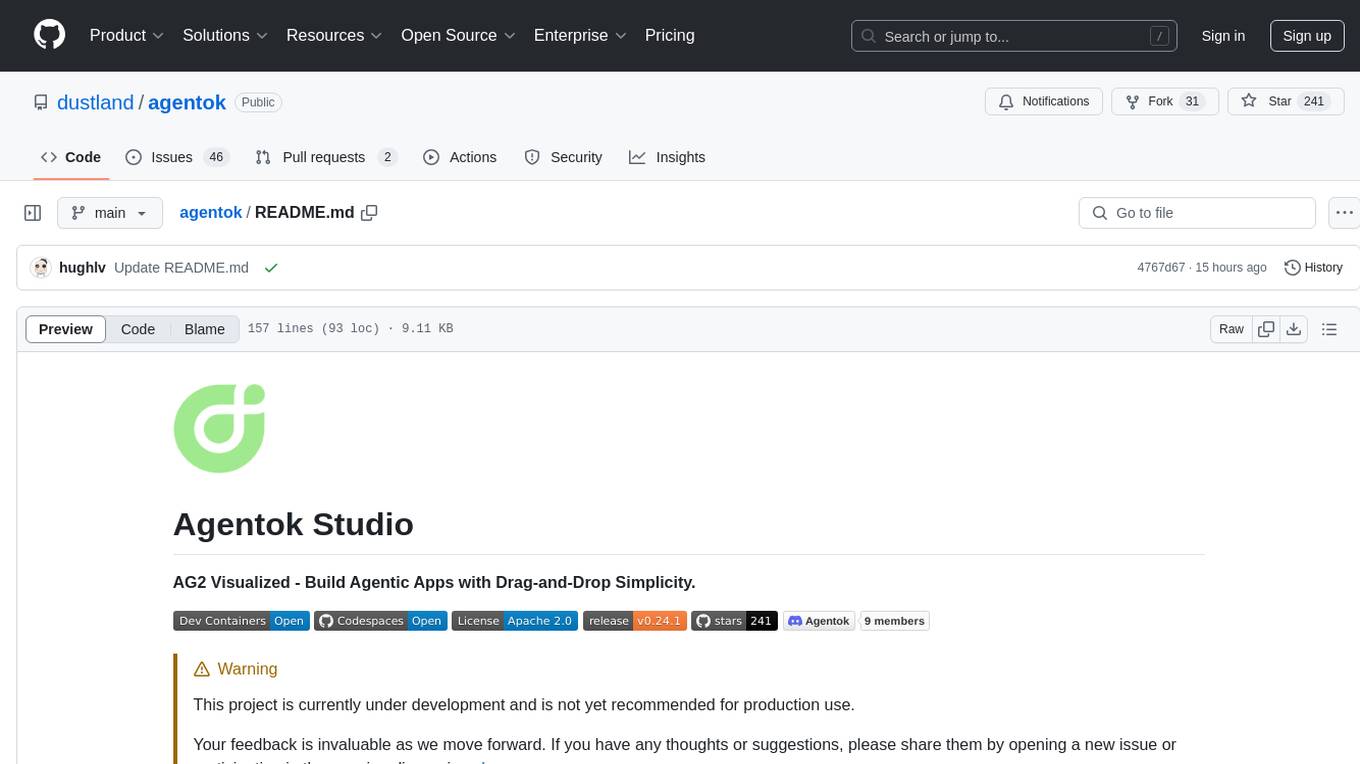
agentok
Agentok Studio is a tool built upon AG2, a powerful agent framework from Microsoft, offering intuitive visual tools to streamline the creation and management of complex agent-based workflows. It simplifies the process for creators and developers by generating native Python code with minimal dependencies, enabling users to create self-contained code that can be executed anywhere. The tool is currently under development and not recommended for production use, but contributions are welcome from the community to enhance its capabilities and functionalities.
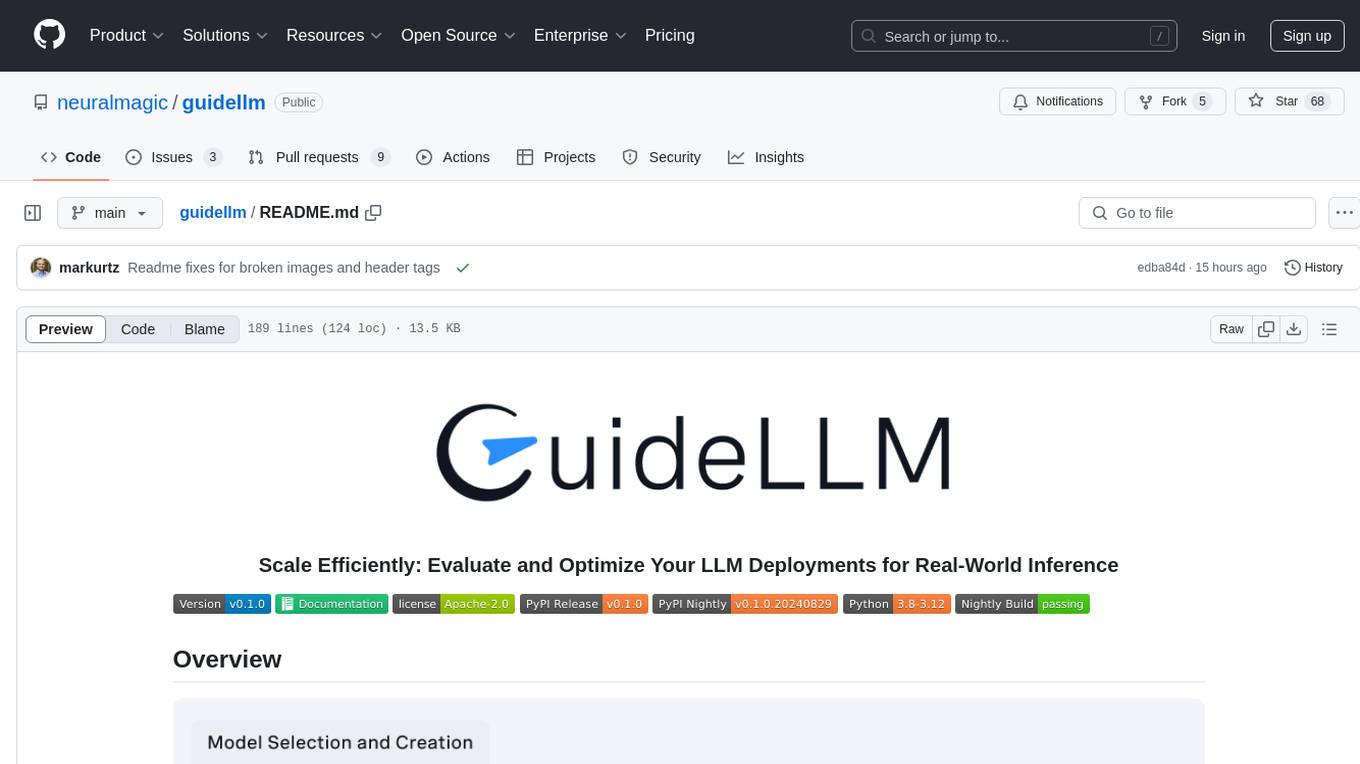
guidellm
GuideLLM is a powerful tool for evaluating and optimizing the deployment of large language models (LLMs). By simulating real-world inference workloads, GuideLLM helps users gauge the performance, resource needs, and cost implications of deploying LLMs on various hardware configurations. This approach ensures efficient, scalable, and cost-effective LLM inference serving while maintaining high service quality. Key features include performance evaluation, resource optimization, cost estimation, and scalability testing.
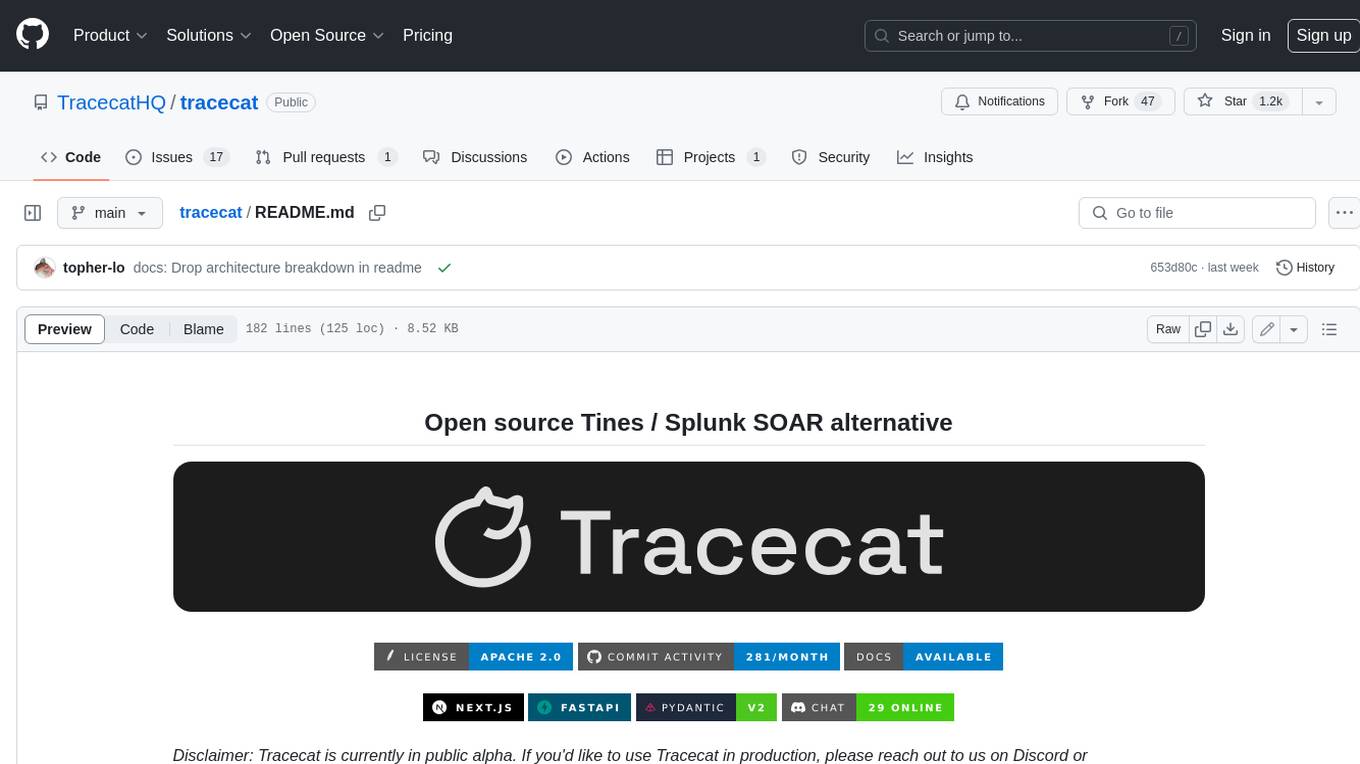
tracecat
Tracecat is an open-source automation platform for security teams. It's designed to be simple but powerful, with a focus on AI features and a practitioner-obsessed UI/UX. Tracecat can be used to automate a variety of tasks, including phishing email investigation, evidence collection, and remediation plan generation.
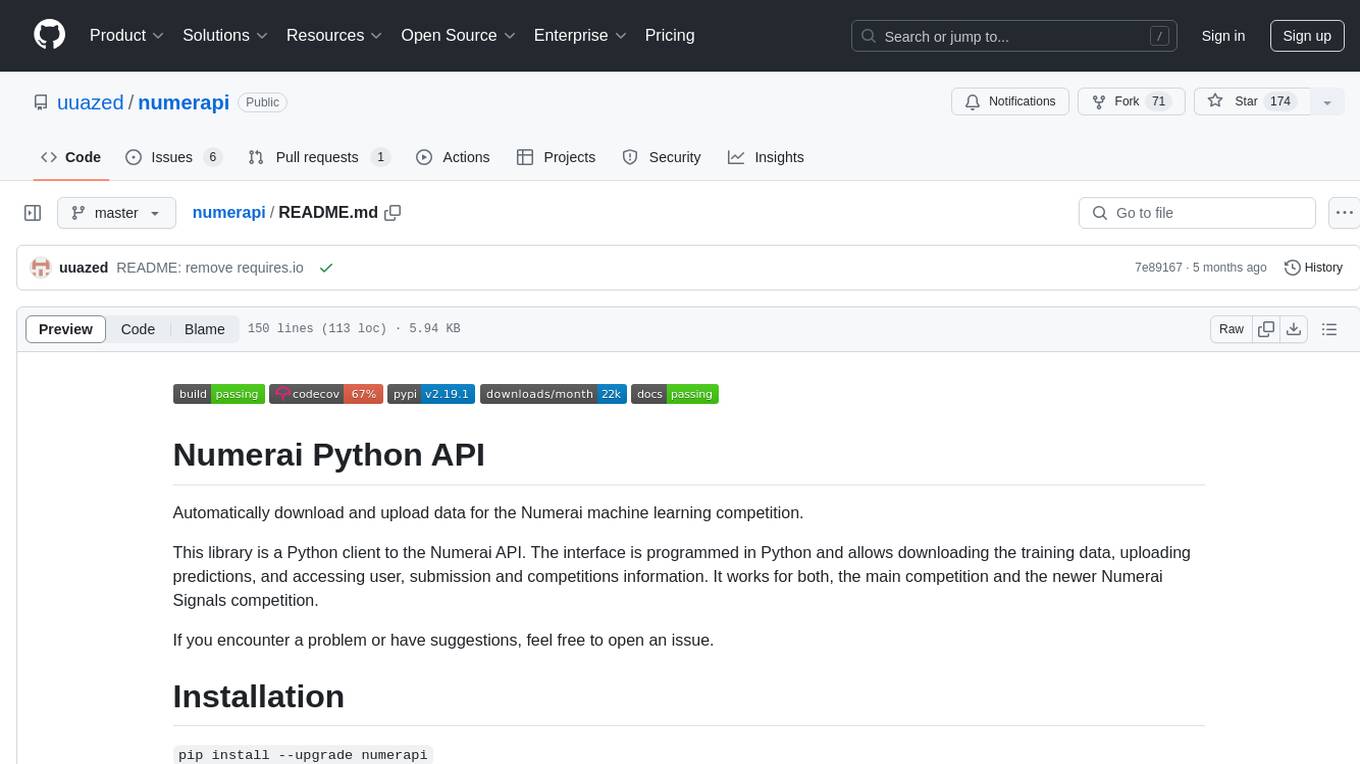
numerapi
Numerapi is a Python client to the Numerai API that allows users to automatically download and upload data for the Numerai machine learning competition. It provides functionalities for downloading training data, uploading predictions, and accessing user, submission, and competitions information for both the main competition and Numerai Signals competition. Users can interact with the API using Python modules or command line interface. Tokens are required for certain actions like uploading predictions or staking, which can be obtained from Numer.ai account settings. The tool also supports features like checking new rounds, getting leaderboards, and managing stakes.
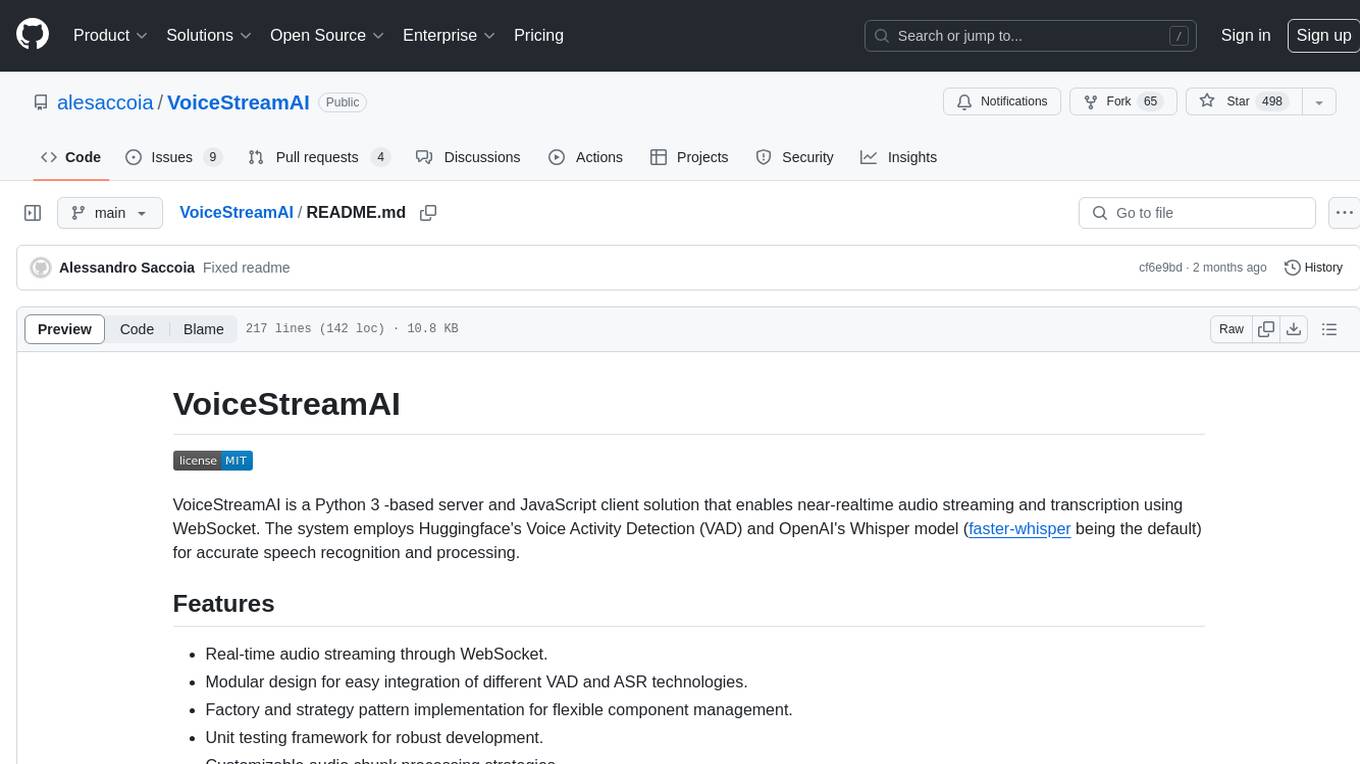
VoiceStreamAI
VoiceStreamAI is a Python 3-based server and JavaScript client solution for near-realtime audio streaming and transcription using WebSocket. It employs Huggingface's Voice Activity Detection (VAD) and OpenAI's Whisper model for accurate speech recognition. The system features real-time audio streaming, modular design for easy integration of VAD and ASR technologies, customizable audio chunk processing strategies, support for multilingual transcription, and secure sockets support. It uses a factory and strategy pattern implementation for flexible component management and provides a unit testing framework for robust development.
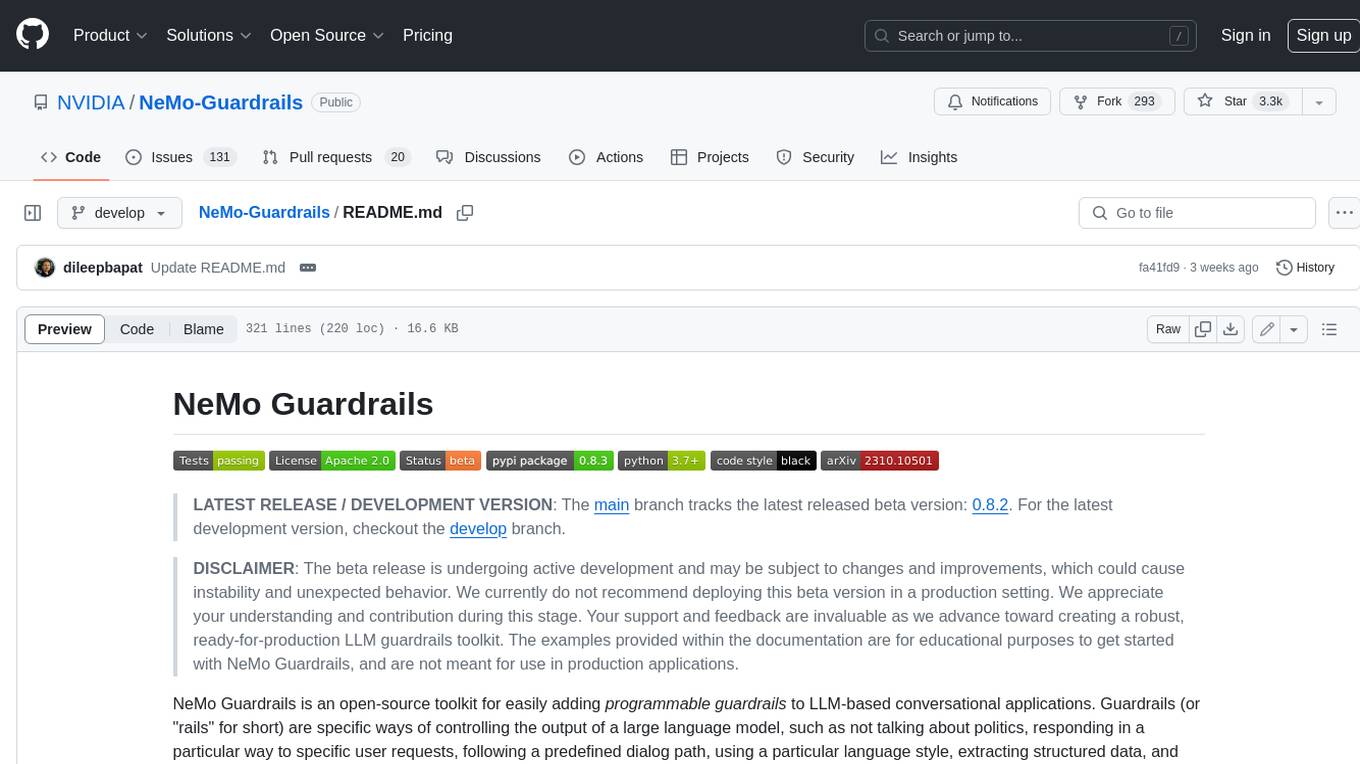
NeMo-Guardrails
NeMo Guardrails is an open-source toolkit for easily adding _programmable guardrails_ to LLM-based conversational applications. Guardrails (or "rails" for short) are specific ways of controlling the output of a large language model, such as not talking about politics, responding in a particular way to specific user requests, following a predefined dialog path, using a particular language style, extracting structured data, and more.
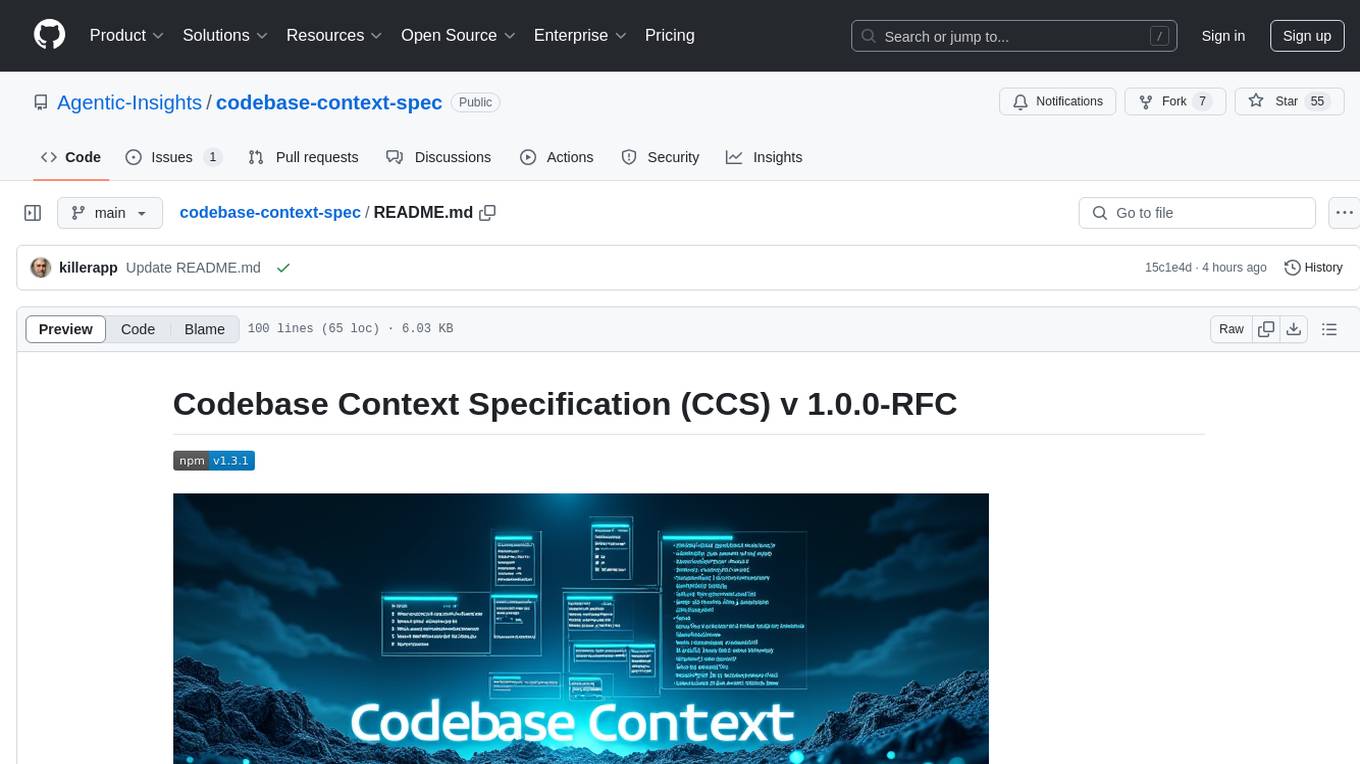
codebase-context-spec
The Codebase Context Specification (CCS) project aims to standardize embedding contextual information within codebases to enhance understanding for both AI and human developers. It introduces a convention similar to `.env` and `.editorconfig` files but focused on documenting code for both AI and humans. By providing structured contextual metadata, collaborative documentation guidelines, and standardized context files, developers can improve code comprehension, collaboration, and development efficiency. The project includes a linter for validating context files and provides guidelines for using the specification with AI assistants. Tooling recommendations suggest creating memory systems, IDE plugins, AI model integrations, and agents for context creation and utilization. Future directions include integration with existing documentation systems, dynamic context generation, and support for explicit context overriding.
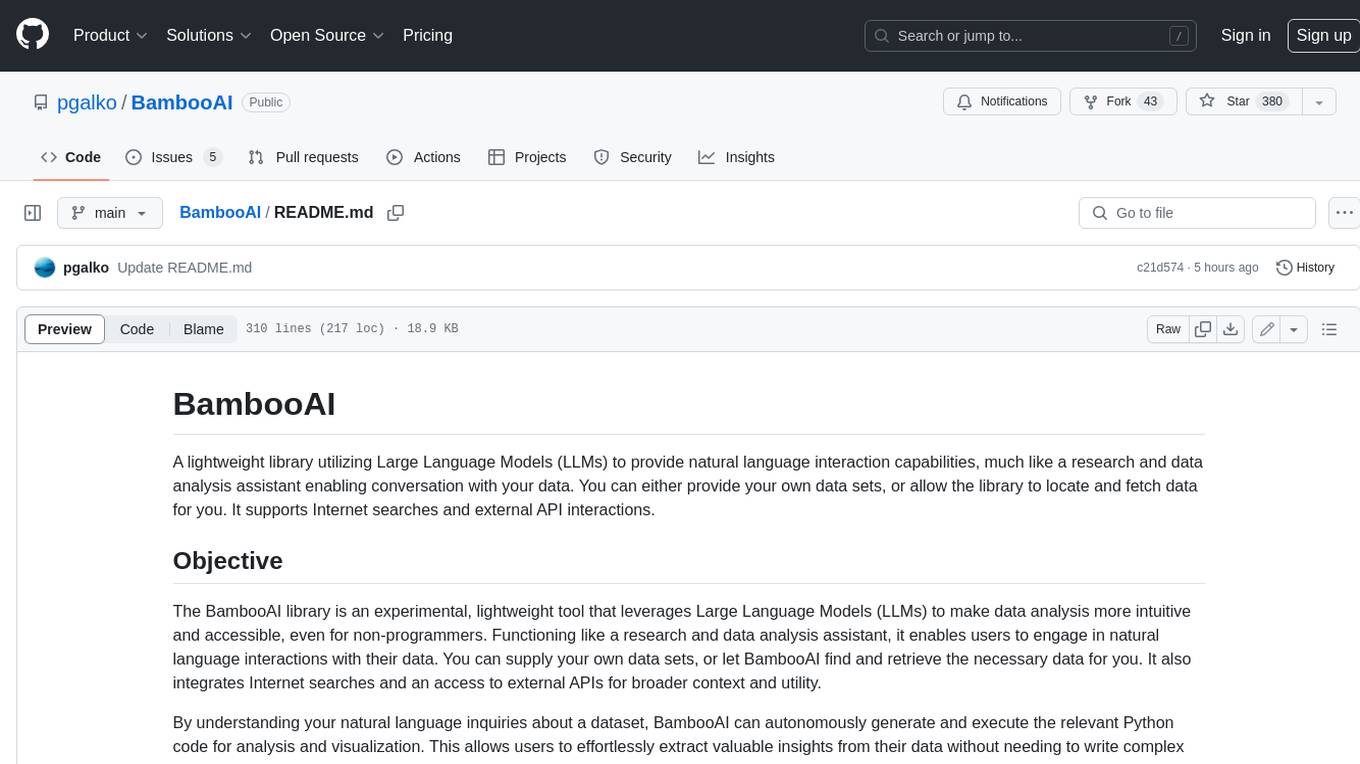
BambooAI
BambooAI is a lightweight library utilizing Large Language Models (LLMs) to provide natural language interaction capabilities, much like a research and data analysis assistant enabling conversation with your data. You can either provide your own data sets, or allow the library to locate and fetch data for you. It supports Internet searches and external API interactions.
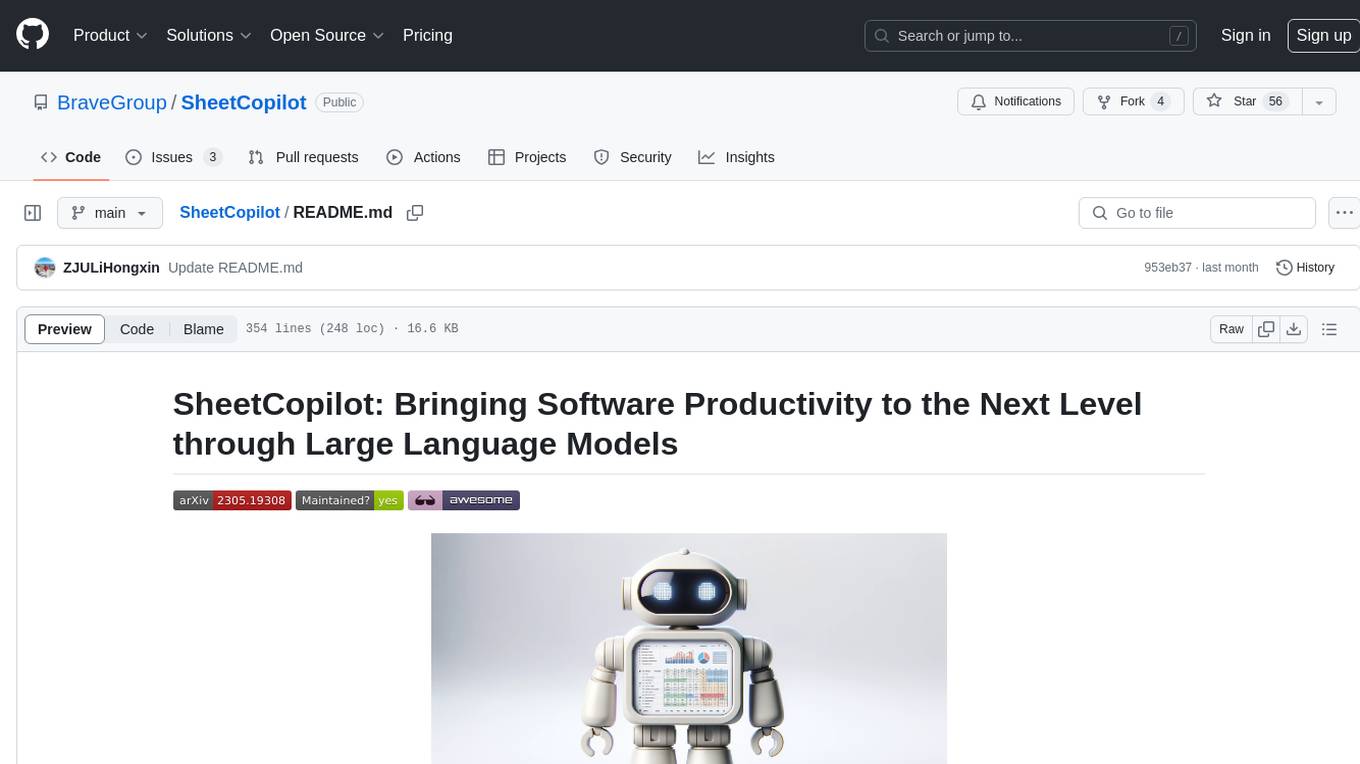
SheetCopilot
SheetCopilot is an assistant agent that manipulates spreadsheets by following user commands. It leverages Large Language Models (LLMs) to interact with spreadsheets like a human expert, enabling non-expert users to complete tasks on complex software such as Google Sheets and Excel via a language interface. The tool observes spreadsheet states, polishes generated solutions based on external action documents and error feedback, and aims to improve success rate and efficiency. SheetCopilot offers a dataset with diverse task categories and operations, supporting operations like entry & manipulation, management, formatting, charts, and pivot tables. Users can interact with SheetCopilot in Excel or Google Sheets, executing tasks like calculating revenue, creating pivot tables, and plotting charts. The tool's evaluation includes performance comparisons with leading LLMs and VBA-based methods on specific datasets, showcasing its capabilities in controlling various aspects of a spreadsheet.
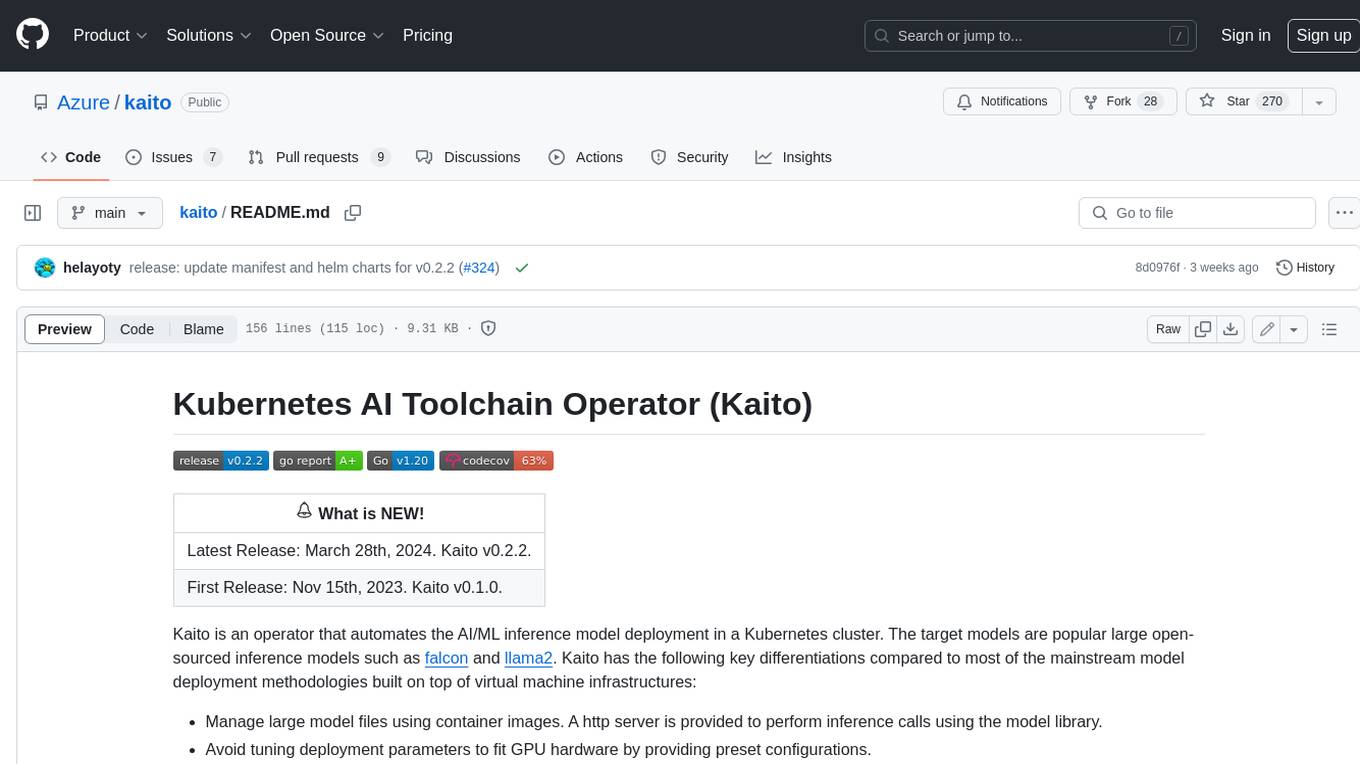
kaito
Kaito is an operator that automates the AI/ML inference model deployment in a Kubernetes cluster. It manages large model files using container images, avoids tuning deployment parameters to fit GPU hardware by providing preset configurations, auto-provisions GPU nodes based on model requirements, and hosts large model images in the public Microsoft Container Registry (MCR) if the license allows. Using Kaito, the workflow of onboarding large AI inference models in Kubernetes is largely simplified.
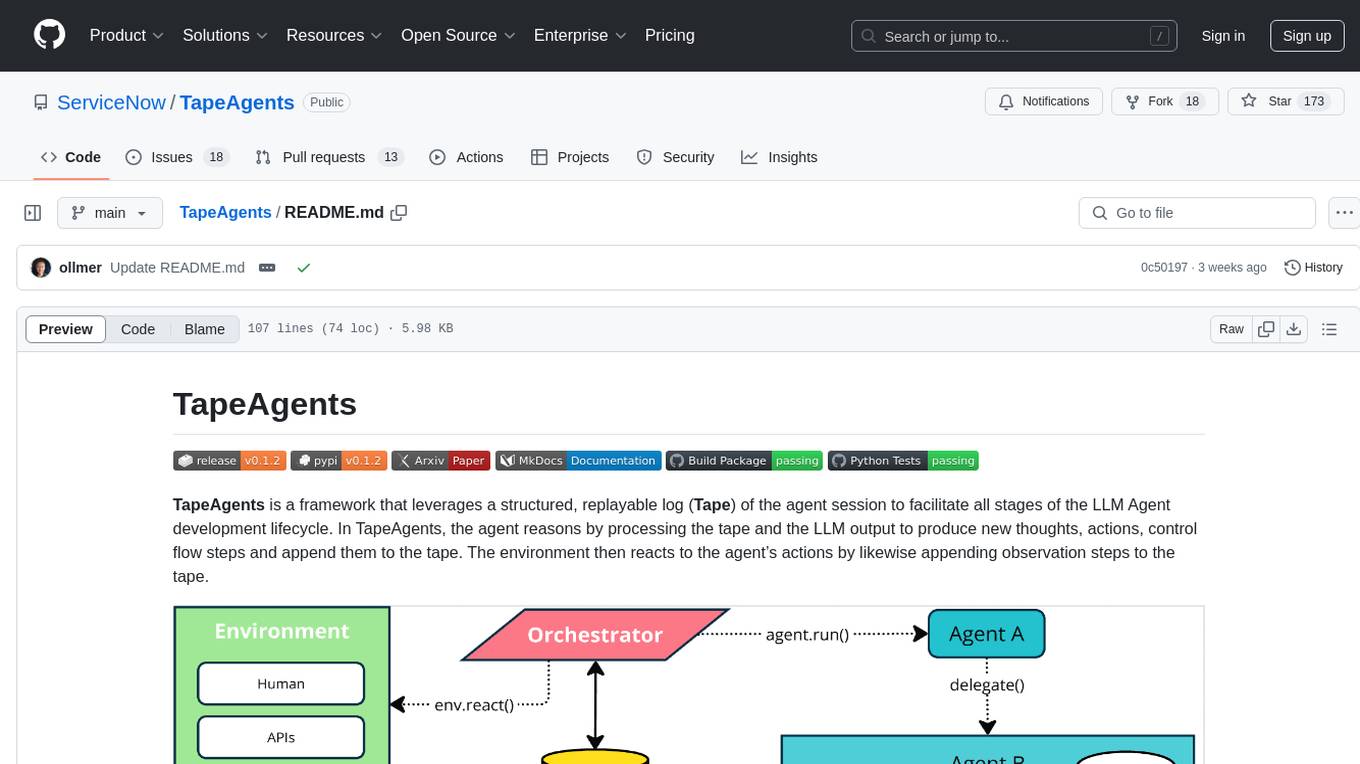
TapeAgents
TapeAgents is a framework that leverages a structured, replayable log of the agent session to facilitate all stages of the LLM Agent development lifecycle. The agent reasons by processing the tape and the LLM output to produce new thoughts, actions, control flow steps, and append them to the tape. Key features include building agents as low-level state machines or high-level multi-agent team configurations, debugging agents with TapeAgent studio or TapeBrowser apps, serving agents with response streaming, and optimizing agent configurations using successful tapes. The Tape-centric design of TapeAgents provides ultimate flexibility in project development, allowing access to tapes for making prompts, generating next steps, and controlling agent behavior.
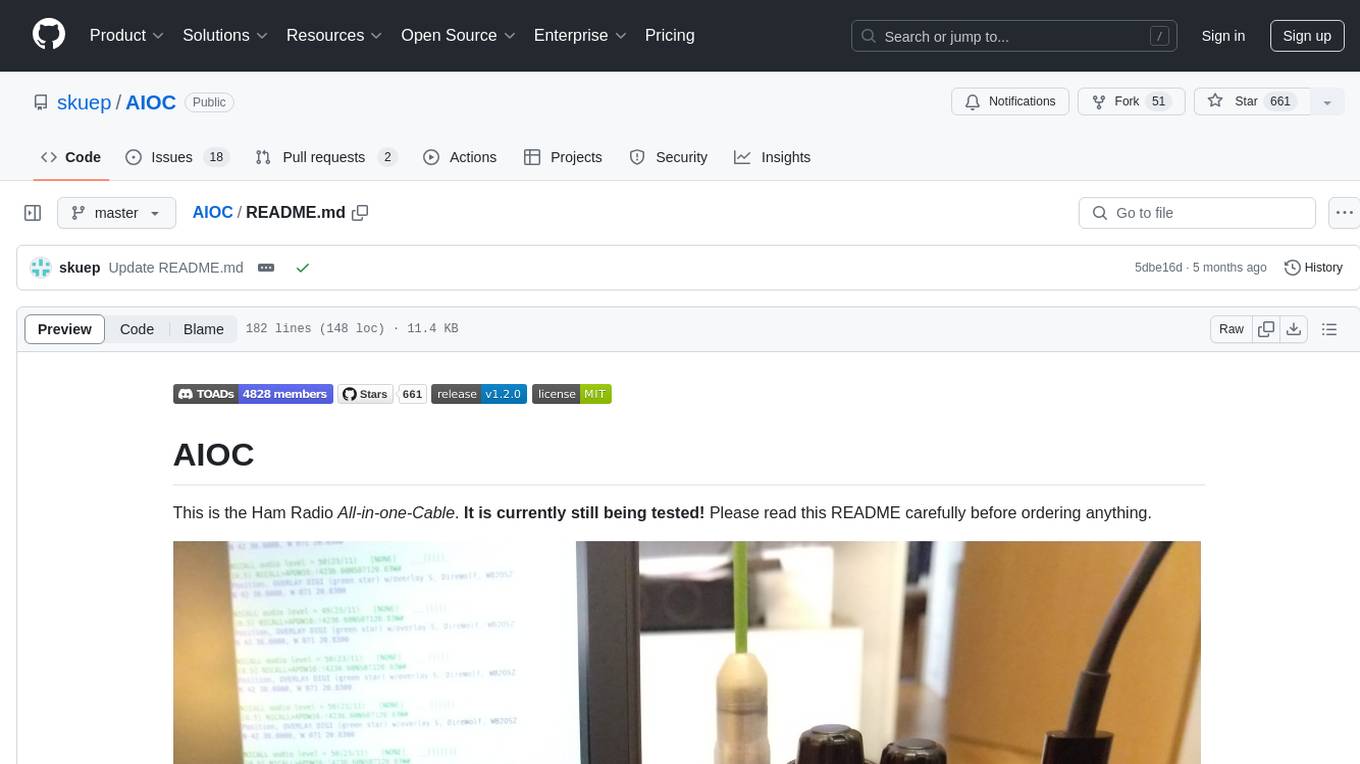
AIOC
AIOC is an All-in-one-Cable for Ham Radio enthusiasts, providing a cheap and hackable digital mode USB interface with features like sound-card, virtual tty, and CM108 compatible HID endpoint. It supports various software and tested radios for functions like programming, APRS, and Dual-PTT HTs. Users can fabricate and assemble the AIOC using specific instructions, and program it using STM32CubeIDE. The tool can be used for tasks like programming radios, asserting PTT, and accessing audio data channels. Future work includes configurable AIOC settings, virtual-PTT, and virtual-COS features.
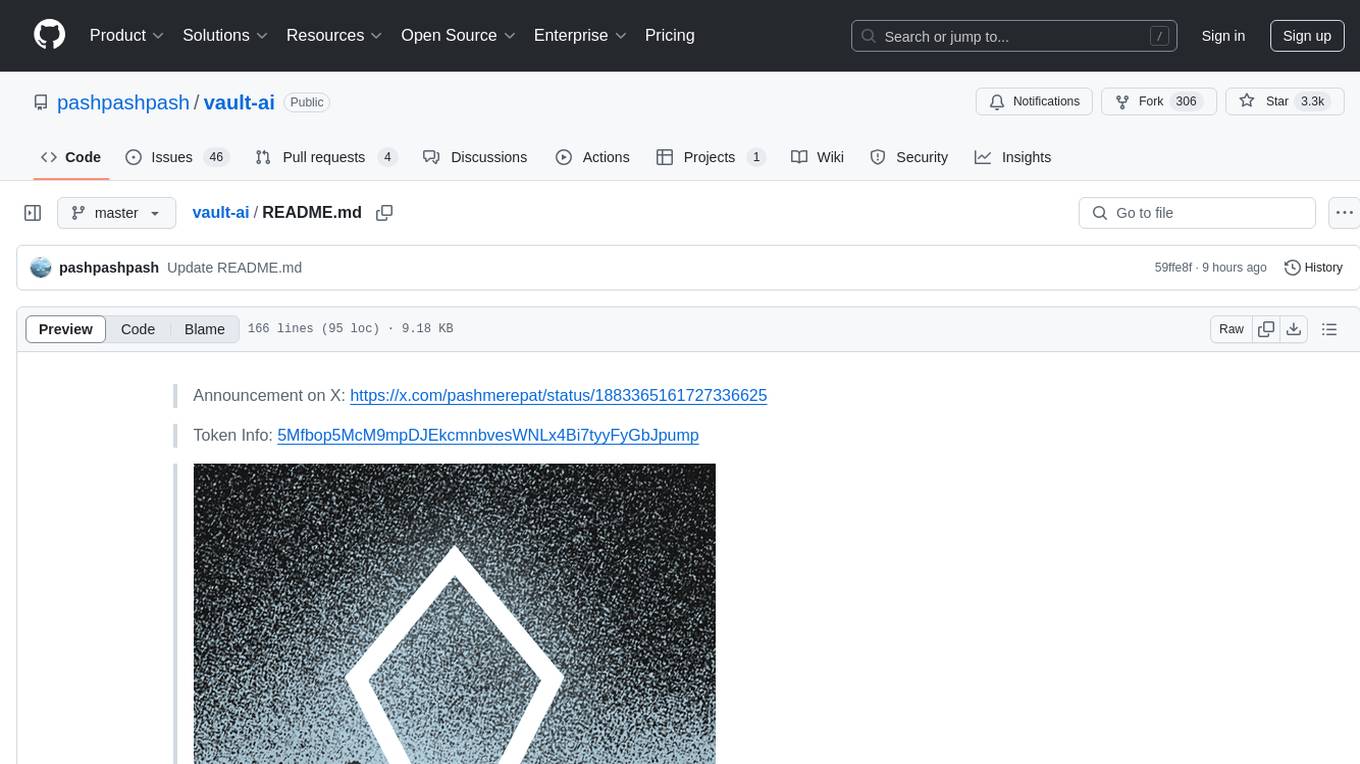
vault-ai
OP Vault is a tool that leverages the OP Stack (OpenAI + Pinecone Vector Database) to allow users to upload custom knowledgebase files and ask questions about their contents. It provides a user-friendly Golang server and React frontend for querying human-readable content like books and documents, making it valuable for knowledge extraction and question-answering. Users can upload entire libraries, receive specific answers with file and section references, and explore the power of the OP Stack in a practical interface.
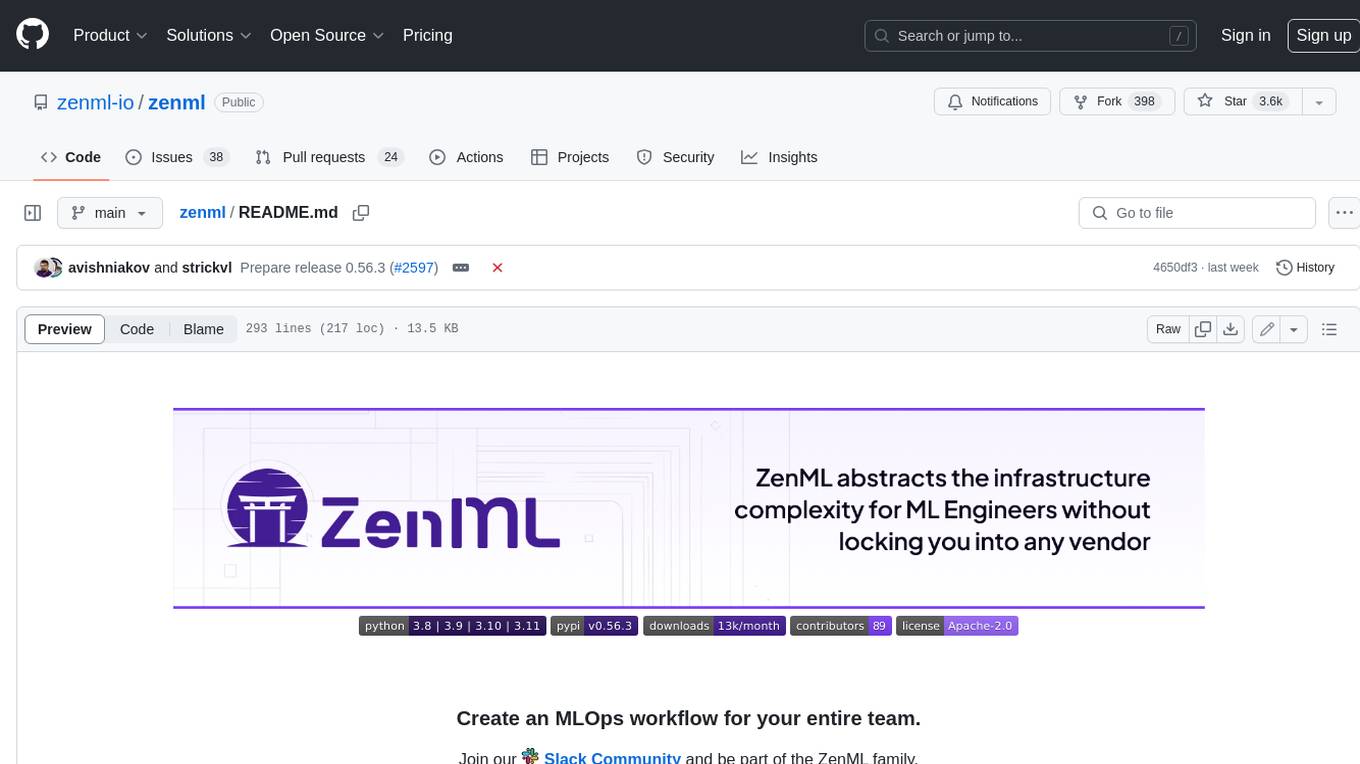
zenml
ZenML is an extensible, open-source MLOps framework for creating portable, production-ready machine learning pipelines. By decoupling infrastructure from code, ZenML enables developers across your organization to collaborate more effectively as they develop to production.
For similar tasks
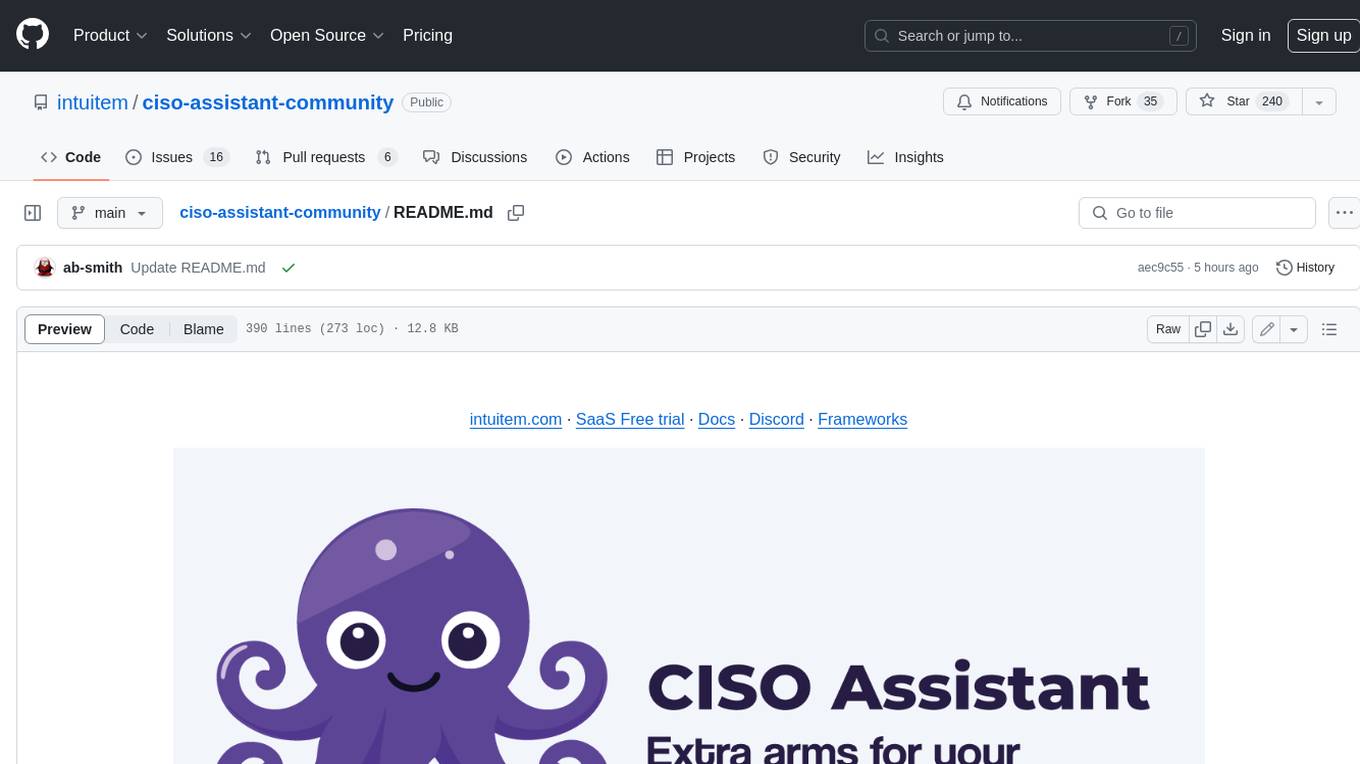
ciso-assistant-community
CISO Assistant is a tool that helps organizations manage their cybersecurity posture and compliance. It provides a centralized platform for managing security controls, threats, and risks. CISO Assistant also includes a library of pre-built frameworks and tools to help organizations quickly and easily implement best practices.
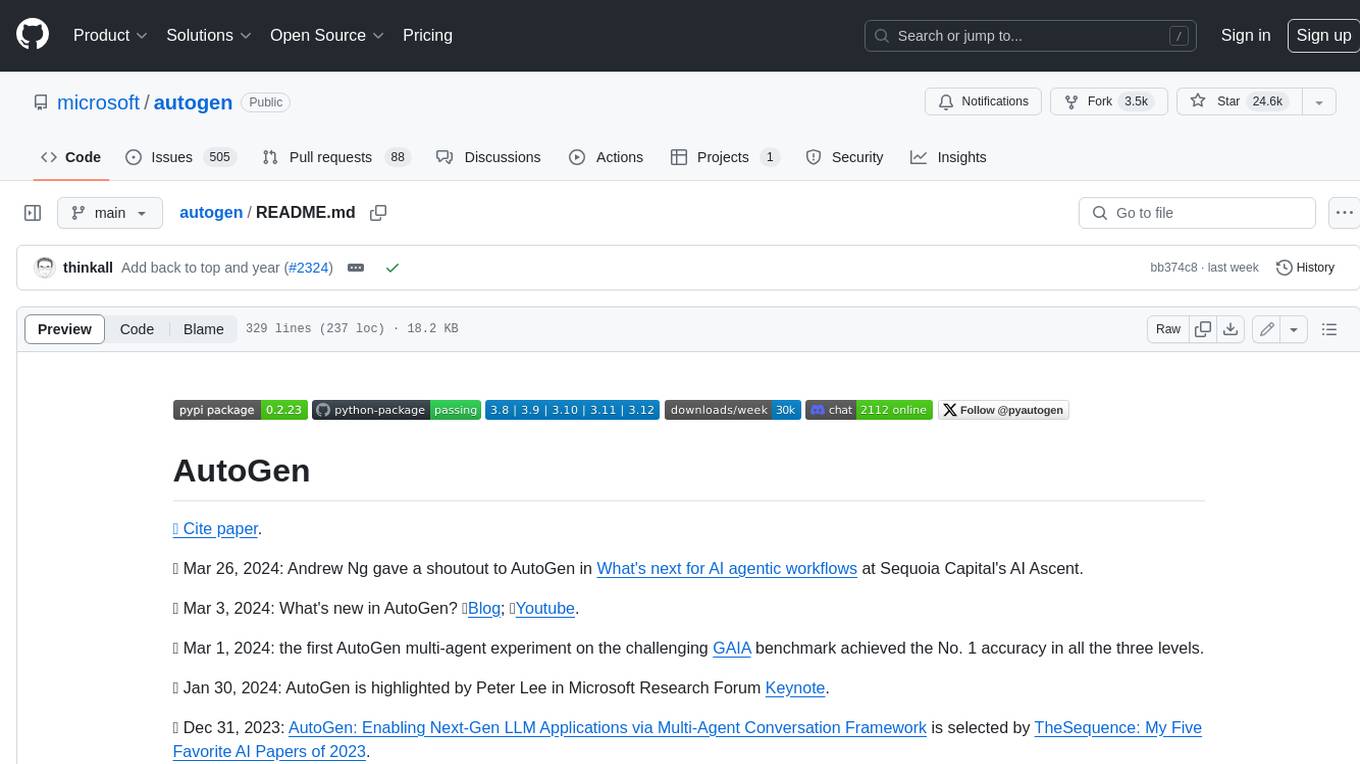
autogen
AutoGen is a framework that enables the development of LLM applications using multiple agents that can converse with each other to solve tasks. AutoGen agents are customizable, conversable, and seamlessly allow human participation. They can operate in various modes that employ combinations of LLMs, human inputs, and tools.
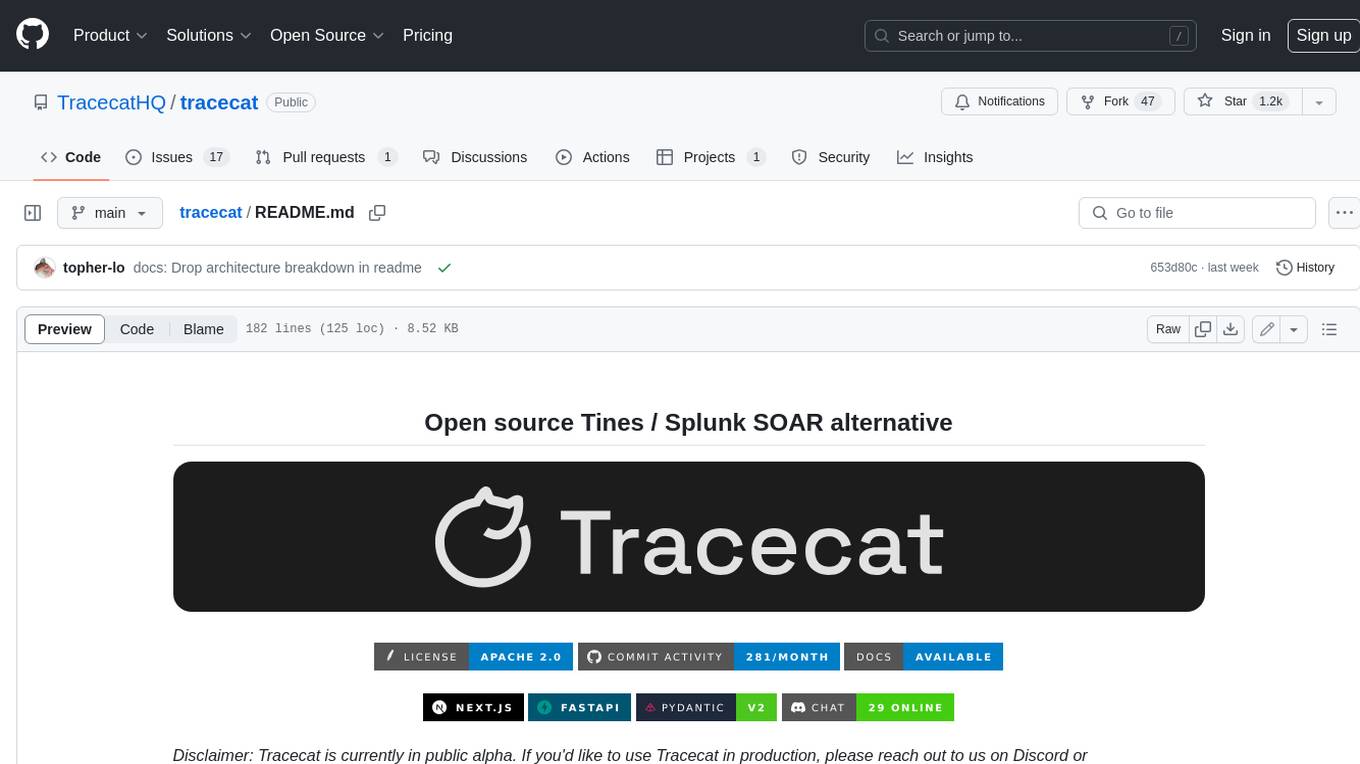
tracecat
Tracecat is an open-source automation platform for security teams. It's designed to be simple but powerful, with a focus on AI features and a practitioner-obsessed UI/UX. Tracecat can be used to automate a variety of tasks, including phishing email investigation, evidence collection, and remediation plan generation.
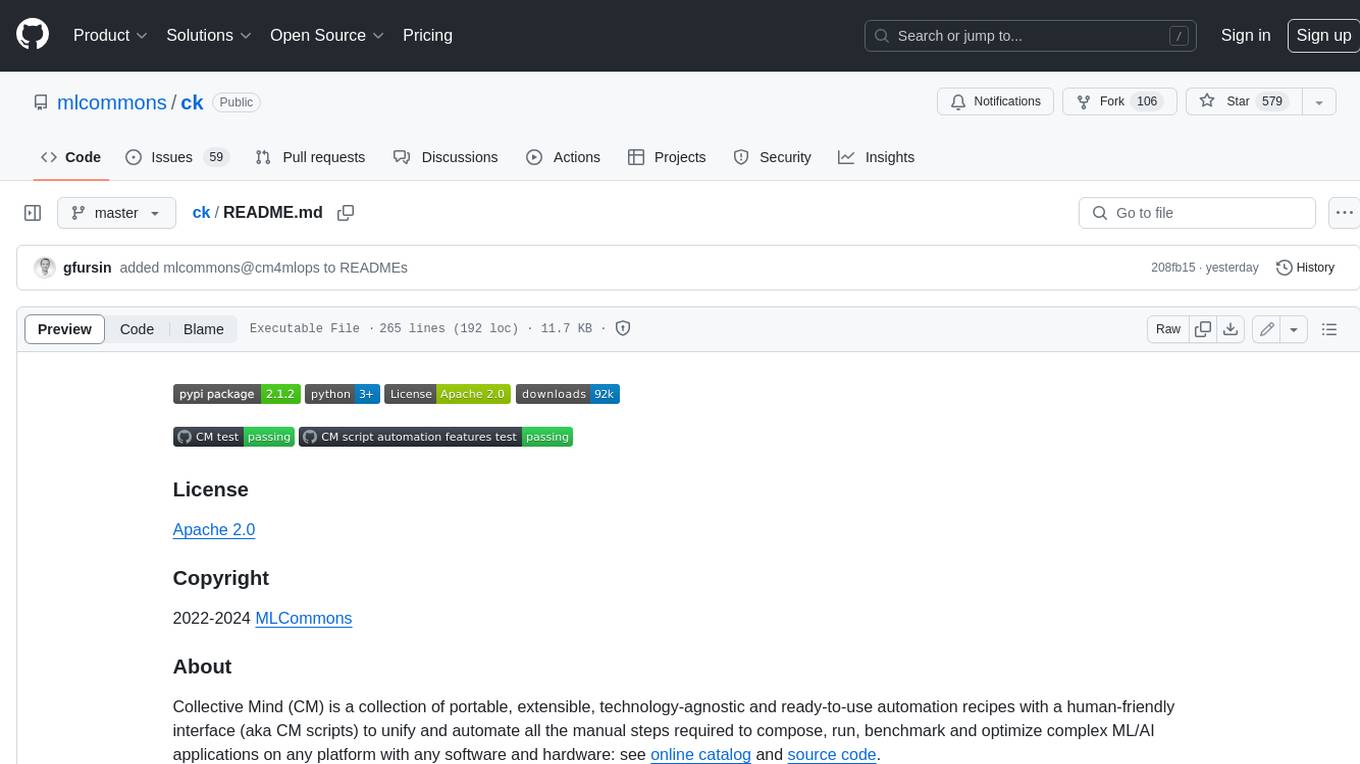
ck
Collective Mind (CM) is a collection of portable, extensible, technology-agnostic and ready-to-use automation recipes with a human-friendly interface (aka CM scripts) to unify and automate all the manual steps required to compose, run, benchmark and optimize complex ML/AI applications on any platform with any software and hardware: see online catalog and source code. CM scripts require Python 3.7+ with minimal dependencies and are continuously extended by the community and MLCommons members to run natively on Ubuntu, MacOS, Windows, RHEL, Debian, Amazon Linux and any other operating system, in a cloud or inside automatically generated containers while keeping backward compatibility - please don't hesitate to report encountered issues here and contact us via public Discord Server to help this collaborative engineering effort! CM scripts were originally developed based on the following requirements from the MLCommons members to help them automatically compose and optimize complex MLPerf benchmarks, applications and systems across diverse and continuously changing models, data sets, software and hardware from Nvidia, Intel, AMD, Google, Qualcomm, Amazon and other vendors: * must work out of the box with the default options and without the need to edit some paths, environment variables and configuration files; * must be non-intrusive, easy to debug and must reuse existing user scripts and automation tools (such as cmake, make, ML workflows, python poetry and containers) rather than substituting them; * must have a very simple and human-friendly command line with a Python API and minimal dependencies; * must require minimal or zero learning curve by using plain Python, native scripts, environment variables and simple JSON/YAML descriptions instead of inventing new workflow languages; * must have the same interface to run all automations natively, in a cloud or inside containers. CM scripts were successfully validated by MLCommons to modularize MLPerf inference benchmarks and help the community automate more than 95% of all performance and power submissions in the v3.1 round across more than 120 system configurations (models, frameworks, hardware) while reducing development and maintenance costs.
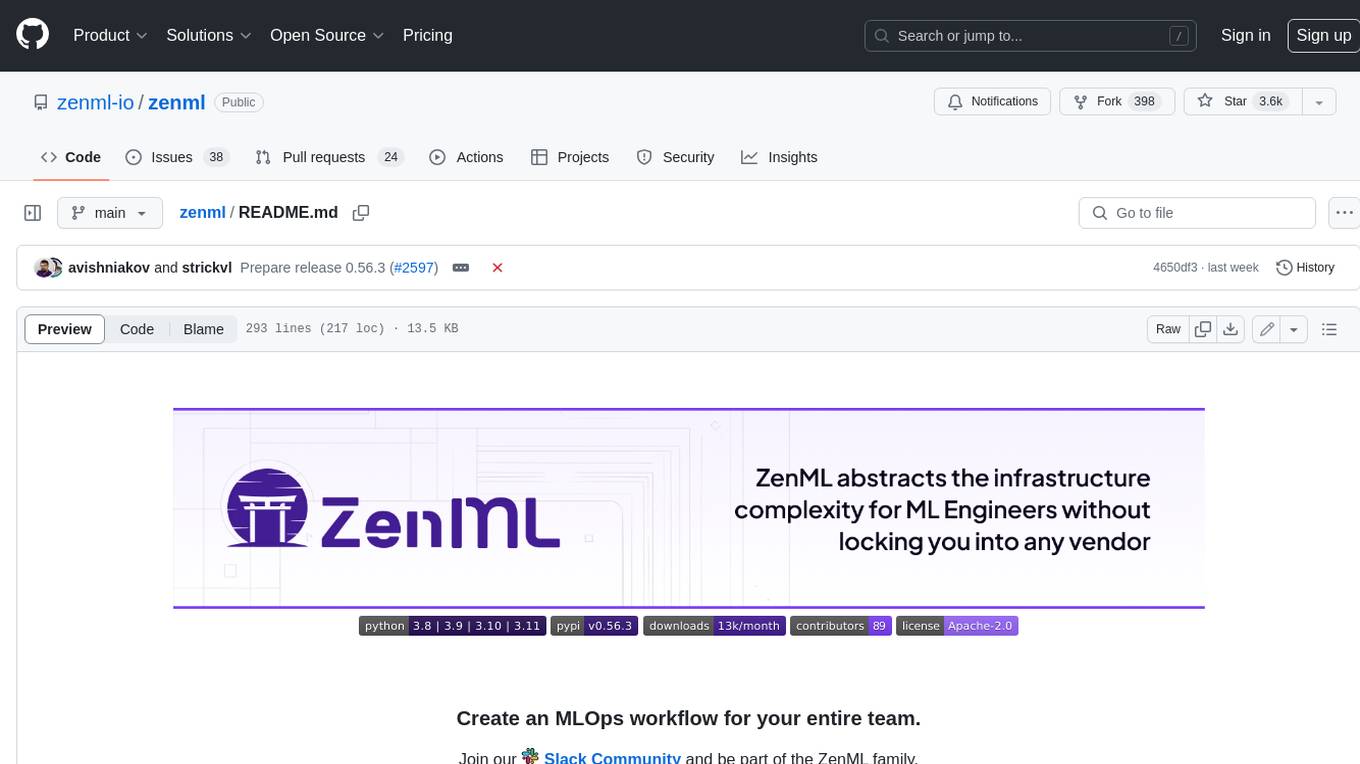
zenml
ZenML is an extensible, open-source MLOps framework for creating portable, production-ready machine learning pipelines. By decoupling infrastructure from code, ZenML enables developers across your organization to collaborate more effectively as they develop to production.
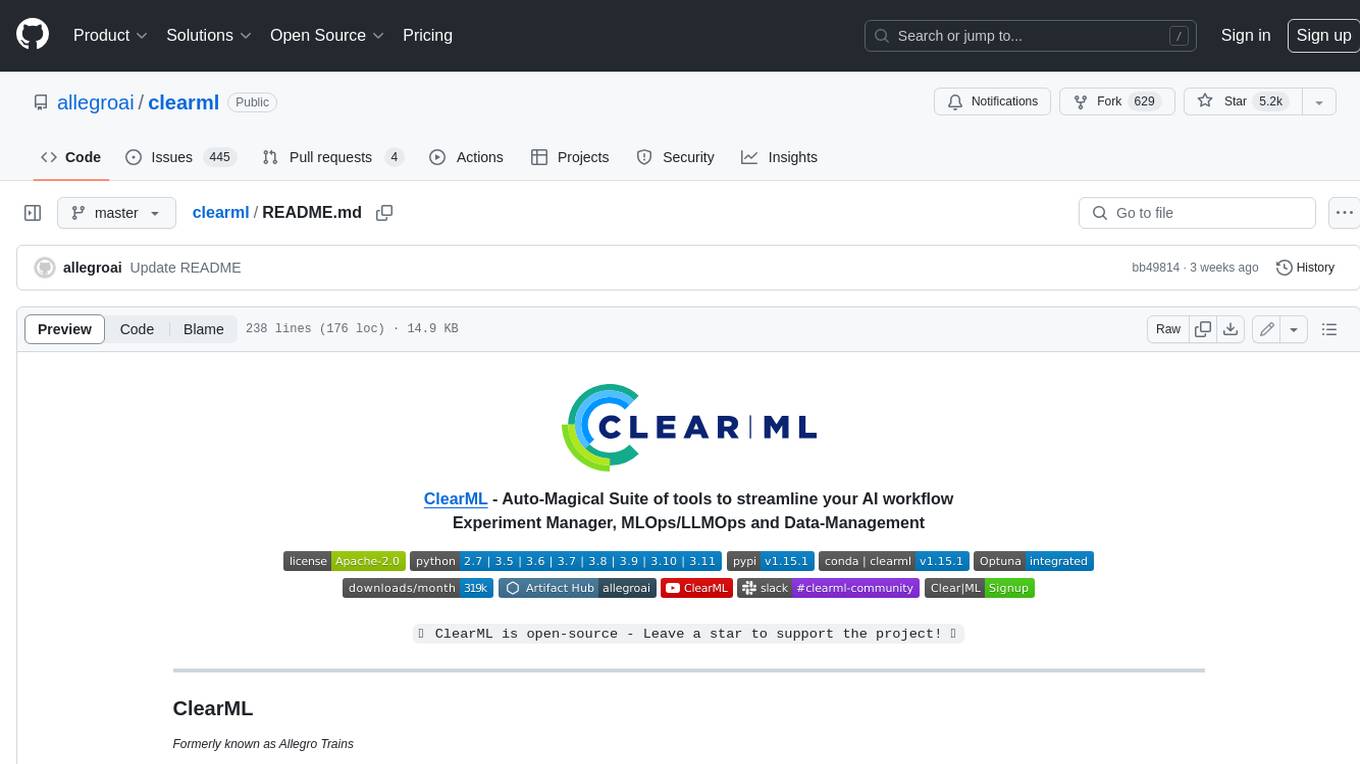
clearml
ClearML is a suite of tools designed to streamline the machine learning workflow. It includes an experiment manager, MLOps/LLMOps, data management, and model serving capabilities. ClearML is open-source and offers a free tier hosting option. It supports various ML/DL frameworks and integrates with Jupyter Notebook and PyCharm. ClearML provides extensive logging capabilities, including source control info, execution environment, hyper-parameters, and experiment outputs. It also offers automation features, such as remote job execution and pipeline creation. ClearML is designed to be easy to integrate, requiring only two lines of code to add to existing scripts. It aims to improve collaboration, visibility, and data transparency within ML teams.
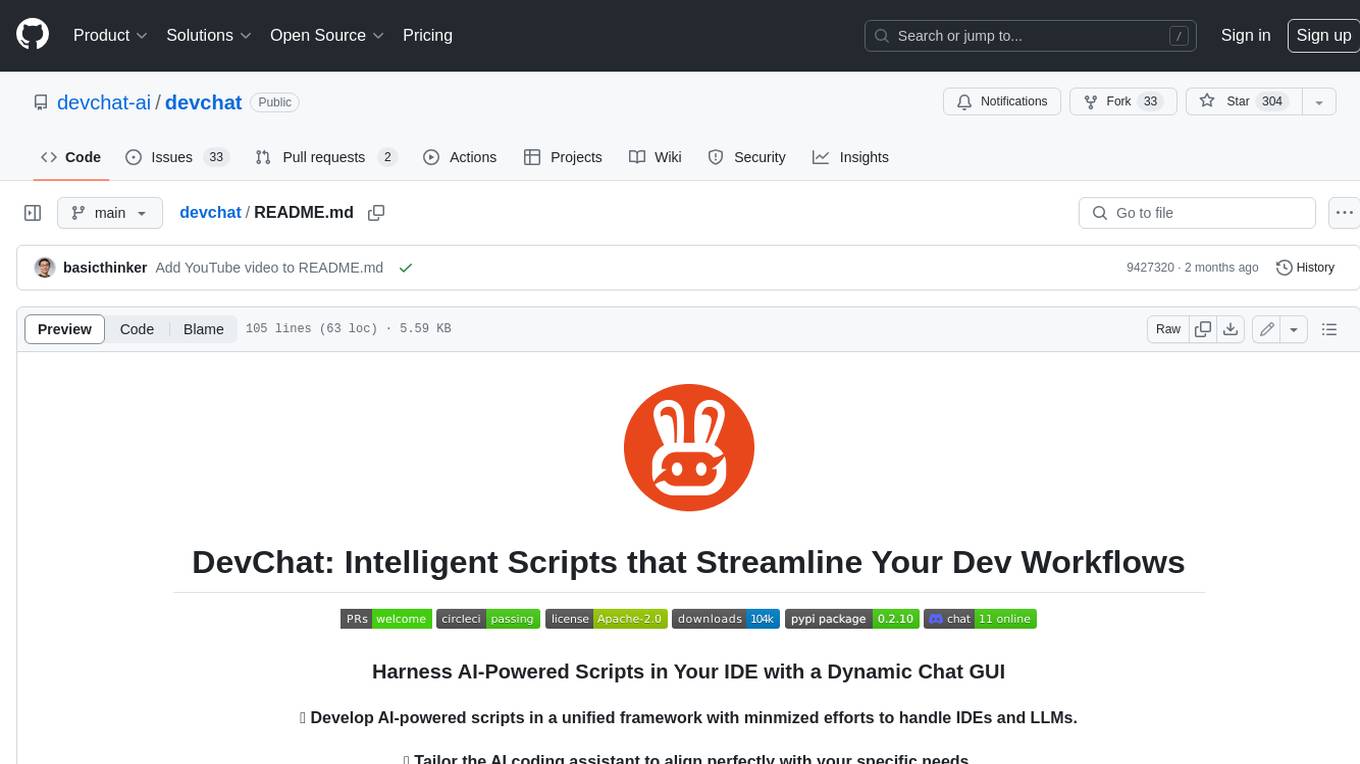
devchat
DevChat is an open-source workflow engine that enables developers to create intelligent, automated workflows for engaging with users through a chat panel within their IDEs. It combines script writing flexibility, latest AI models, and an intuitive chat GUI to enhance user experience and productivity. DevChat simplifies the integration of AI in software development, unlocking new possibilities for developers.
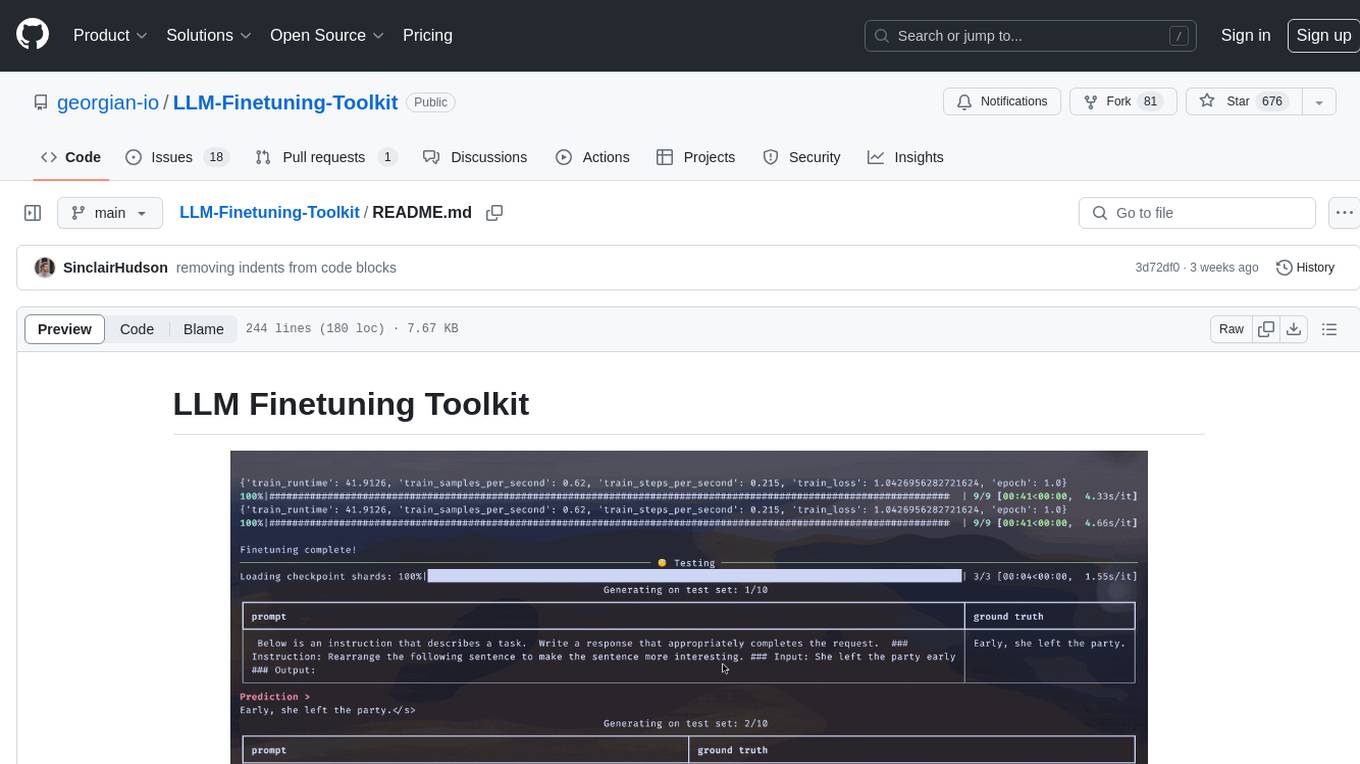
LLM-Finetuning-Toolkit
LLM Finetuning toolkit is a config-based CLI tool for launching a series of LLM fine-tuning experiments on your data and gathering their results. It allows users to control all elements of a typical experimentation pipeline - prompts, open-source LLMs, optimization strategy, and LLM testing - through a single YAML configuration file. The toolkit supports basic, intermediate, and advanced usage scenarios, enabling users to run custom experiments, conduct ablation studies, and automate fine-tuning workflows. It provides features for data ingestion, model definition, training, inference, quality assurance, and artifact outputs, making it a comprehensive tool for fine-tuning large language models.
For similar jobs
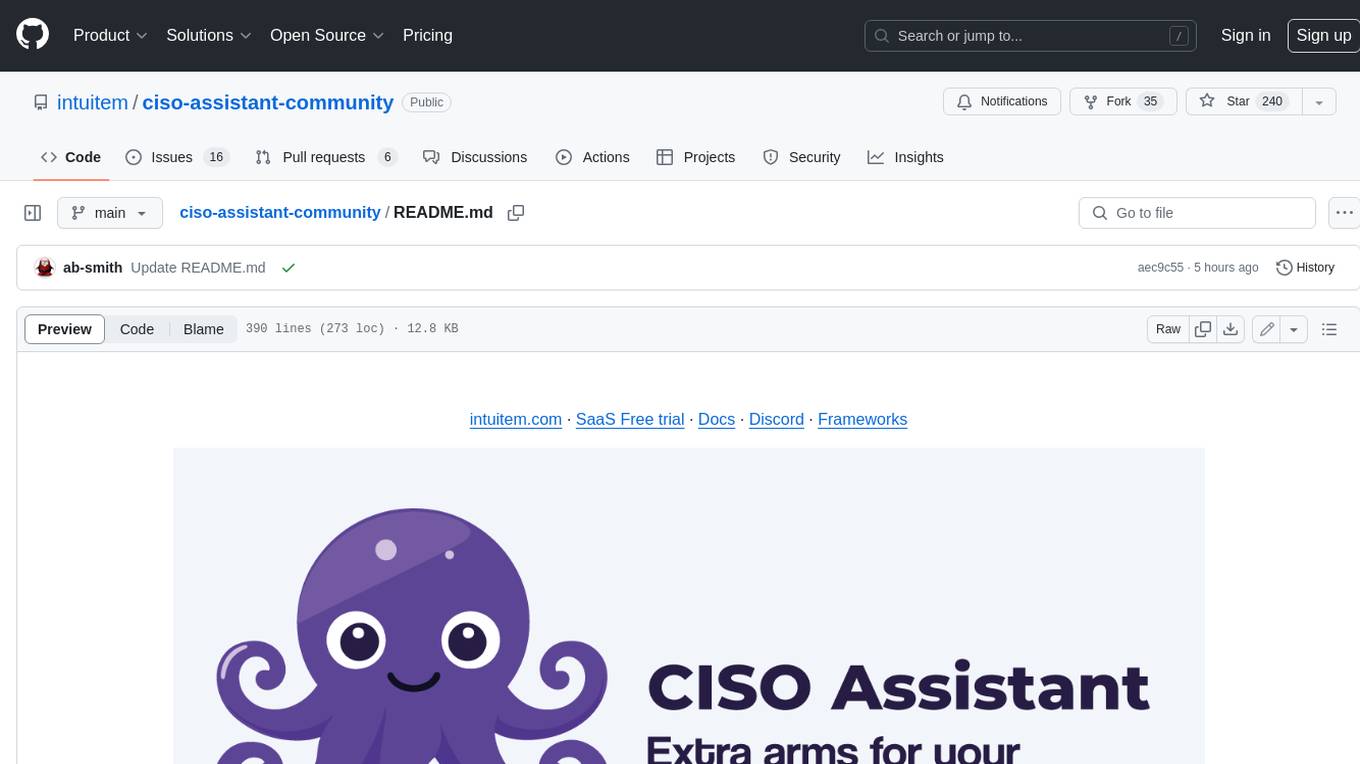
ciso-assistant-community
CISO Assistant is a tool that helps organizations manage their cybersecurity posture and compliance. It provides a centralized platform for managing security controls, threats, and risks. CISO Assistant also includes a library of pre-built frameworks and tools to help organizations quickly and easily implement best practices.
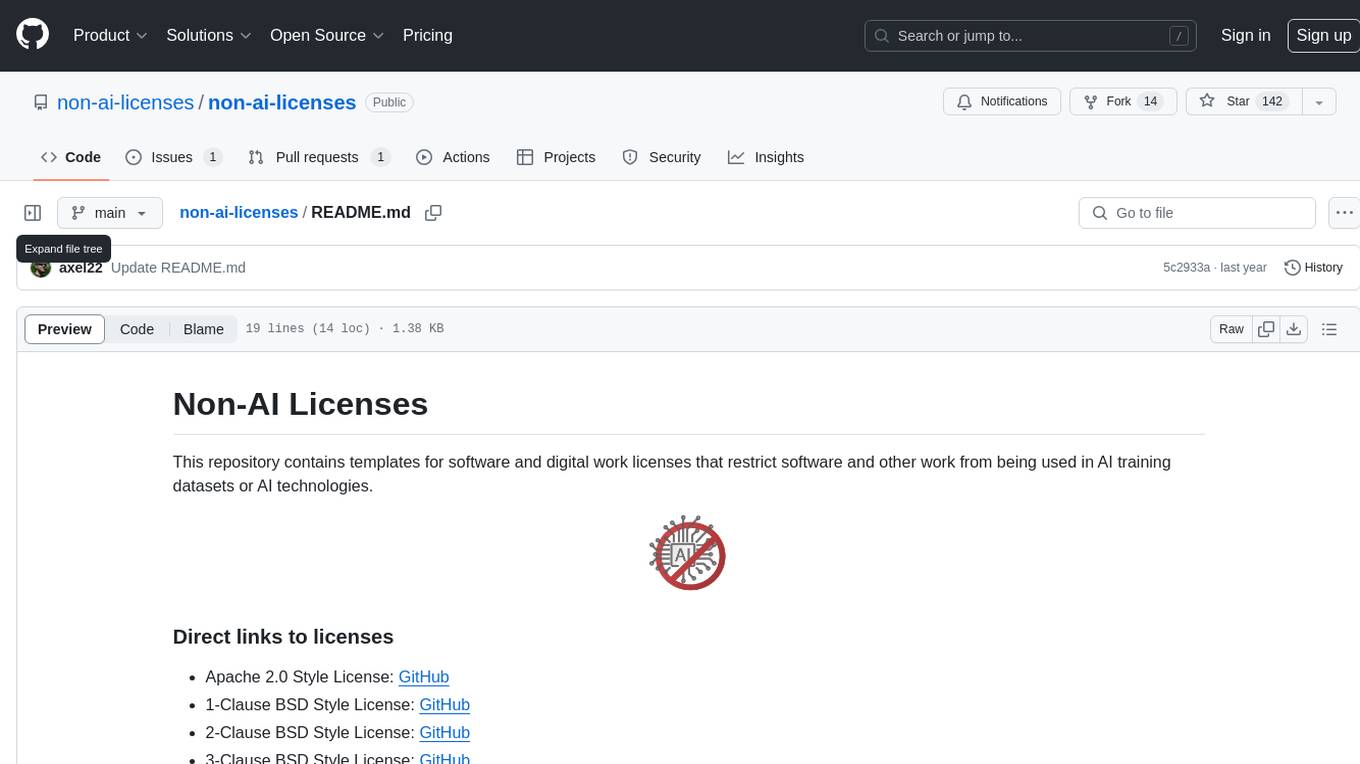
non-ai-licenses
This repository provides templates for software and digital work licenses that restrict usage in AI training datasets or AI technologies. It includes various license styles such as Apache, BSD, MIT, UPL, ISC, CC0, and MPL-2.0.
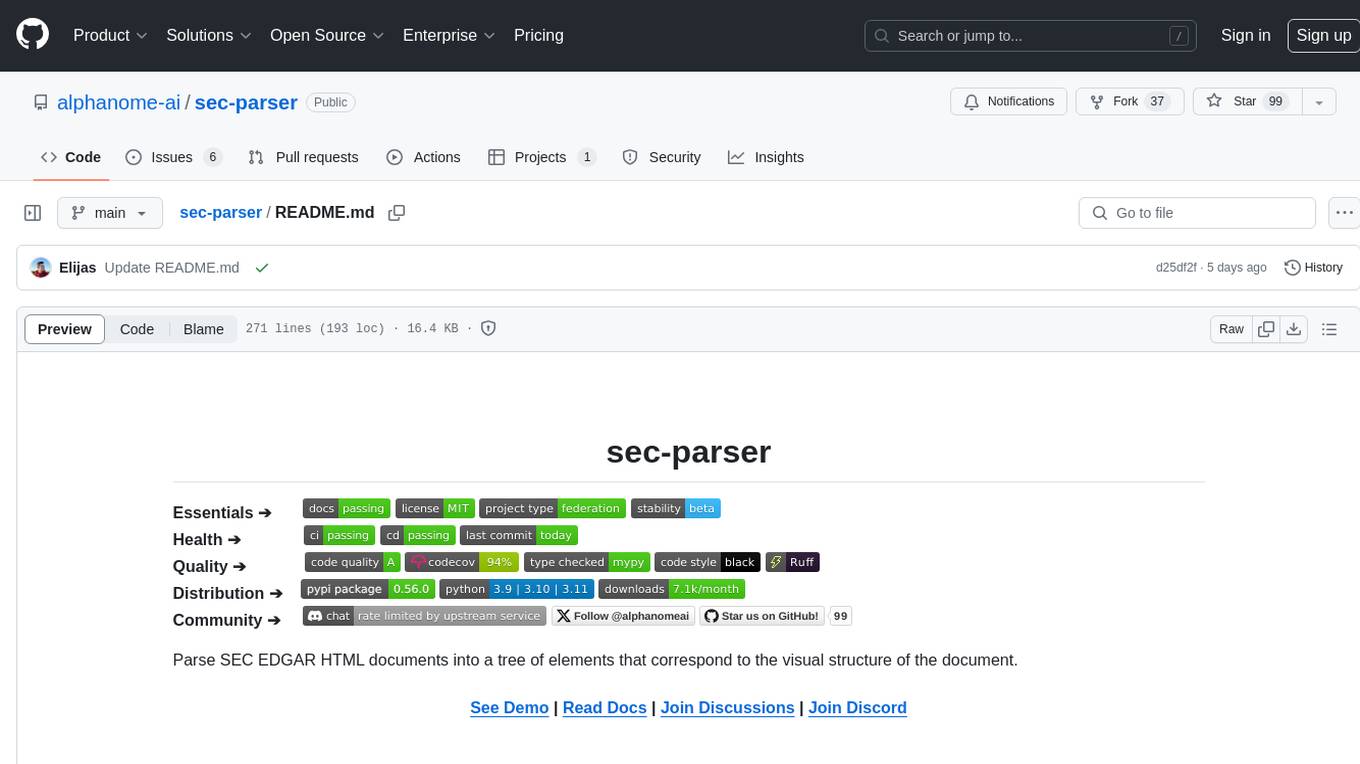
sec-parser
The `sec-parser` project simplifies extracting meaningful information from SEC EDGAR HTML documents by organizing them into semantic elements and a tree structure. It helps in parsing SEC filings for financial and regulatory analysis, analytics and data science, AI and machine learning, causal AI, and large language models. The tool is especially beneficial for AI, ML, and LLM applications by streamlining data pre-processing and feature extraction.
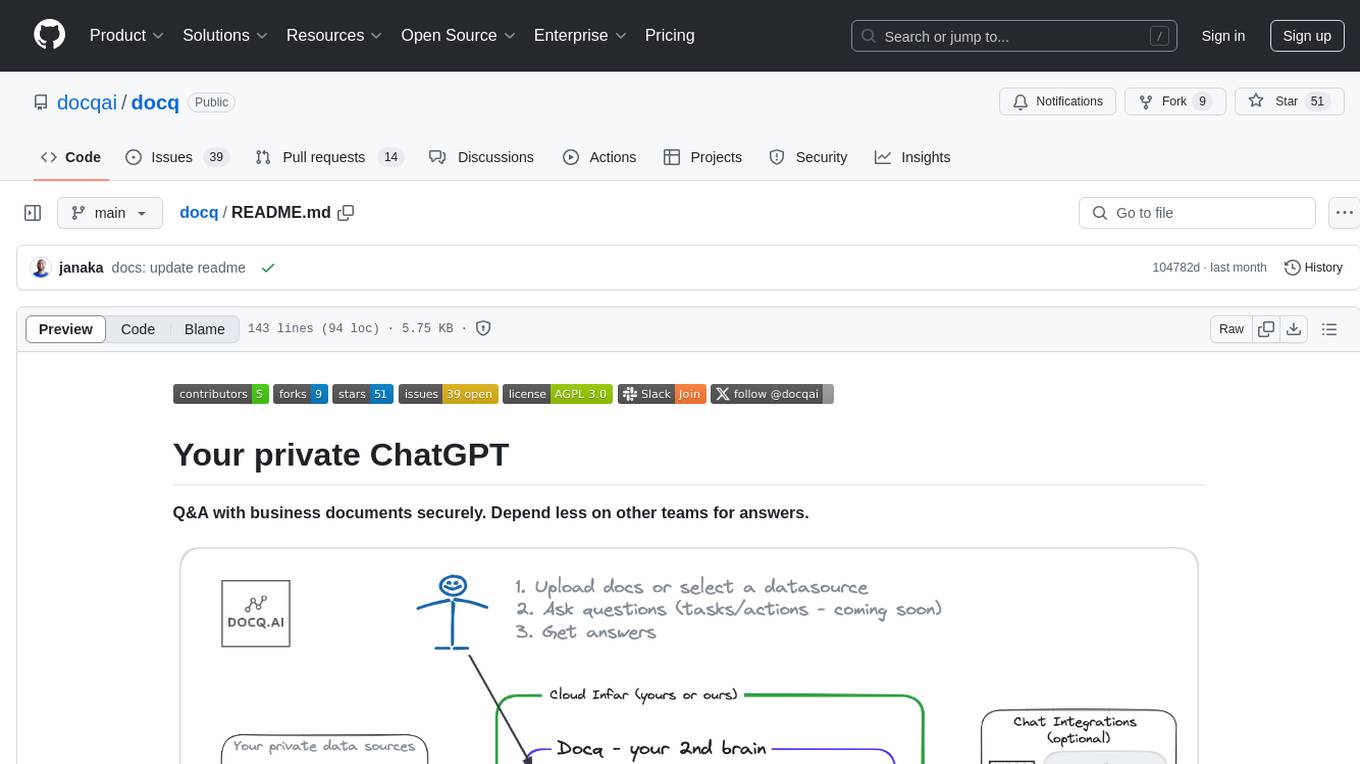
docq
Docq is a private and secure GenAI tool designed to extract knowledge from business documents, enabling users to find answers independently. It allows data to stay within organizational boundaries, supports self-hosting with various cloud vendors, and offers multi-model and multi-modal capabilities. Docq is extensible, open-source (AGPLv3), and provides commercial licensing options. The tool aims to be a turnkey solution for organizations to adopt AI innovation safely, with plans for future features like more data ingestion options and model fine-tuning.
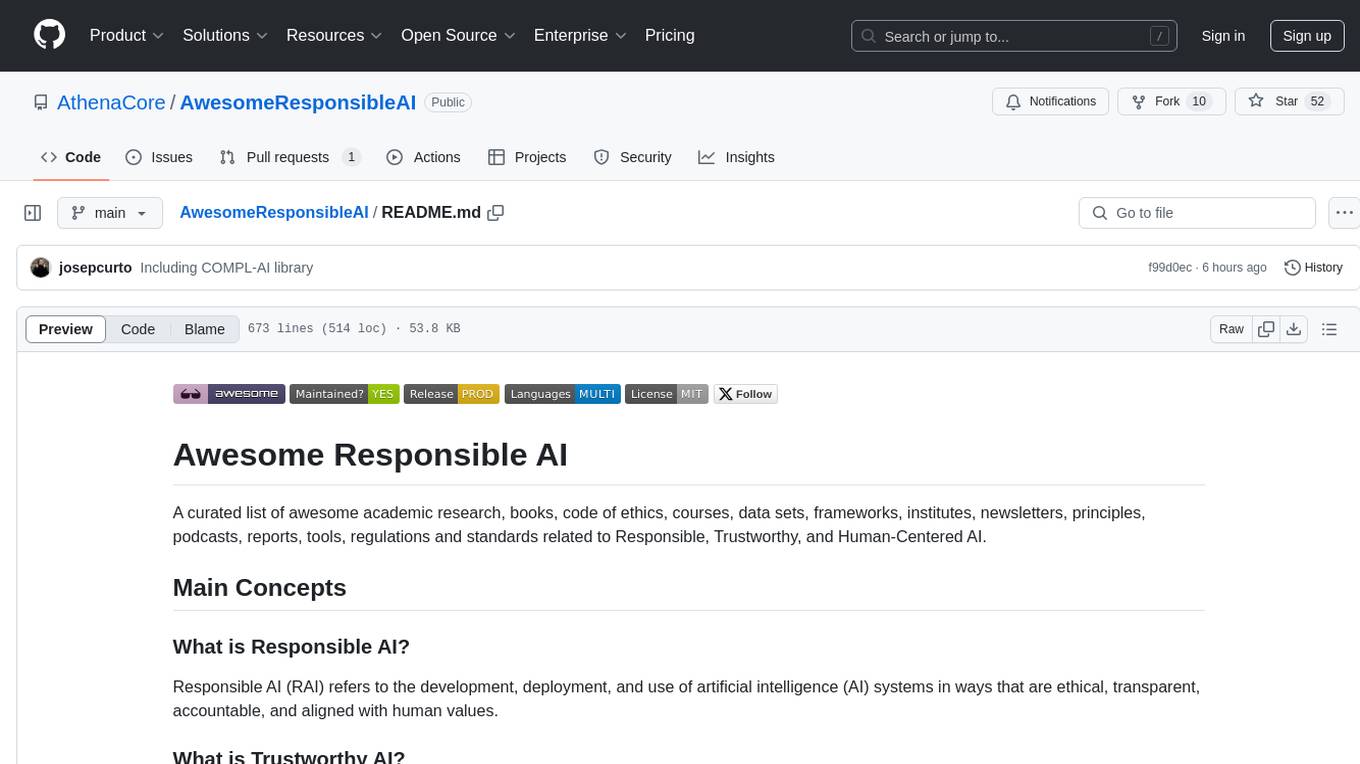
AwesomeResponsibleAI
Awesome Responsible AI is a curated list of academic research, books, code of ethics, courses, data sets, frameworks, institutes, newsletters, principles, podcasts, reports, tools, regulations, and standards related to Responsible, Trustworthy, and Human-Centered AI. It covers various concepts such as Responsible AI, Trustworthy AI, Human-Centered AI, Responsible AI frameworks, AI Governance, and more. The repository provides a comprehensive collection of resources for individuals interested in ethical, transparent, and accountable AI development and deployment.
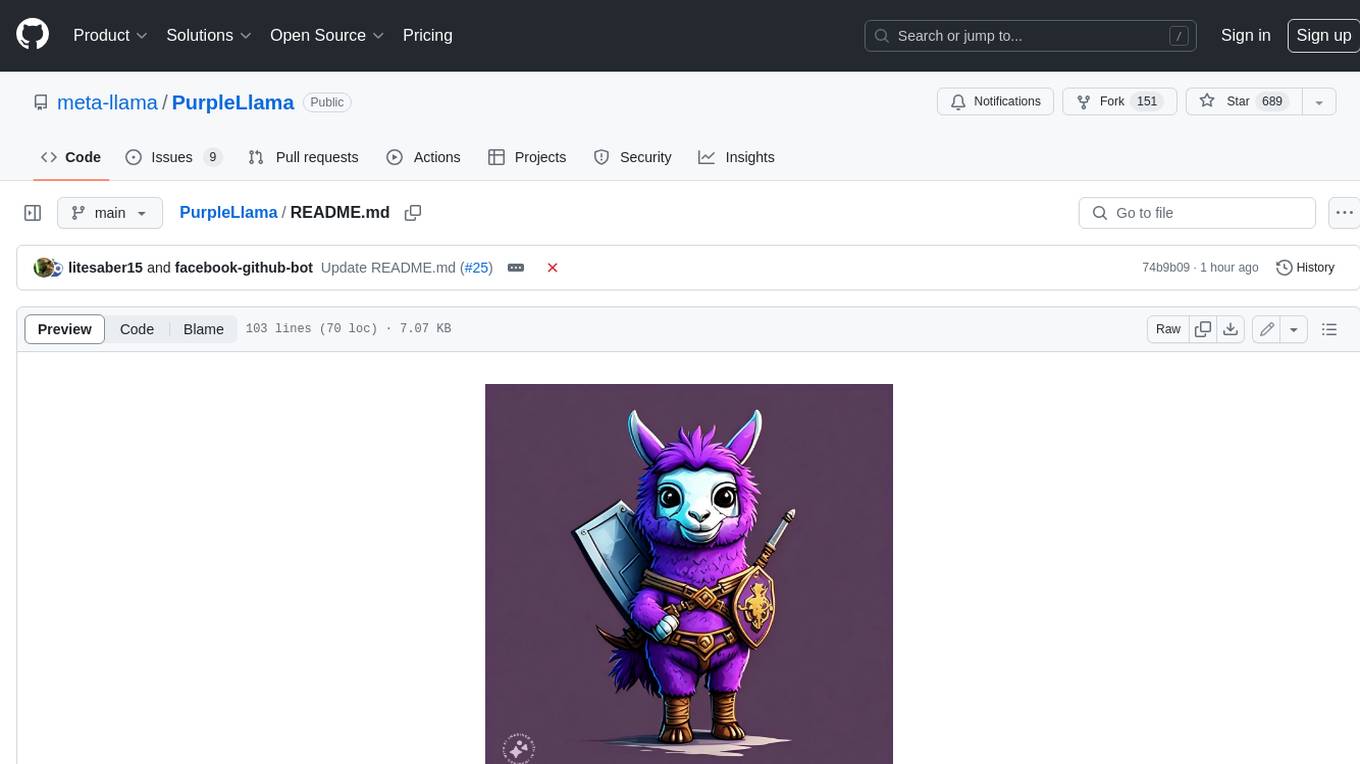
PurpleLlama
Purple Llama is an umbrella project that aims to provide tools and evaluations to support responsible development and usage of generative AI models. It encompasses components for cybersecurity and input/output safeguards, with plans to expand in the future. The project emphasizes a collaborative approach, borrowing the concept of purple teaming from cybersecurity, to address potential risks and challenges posed by generative AI. Components within Purple Llama are licensed permissively to foster community collaboration and standardize the development of trust and safety tools for generative AI.
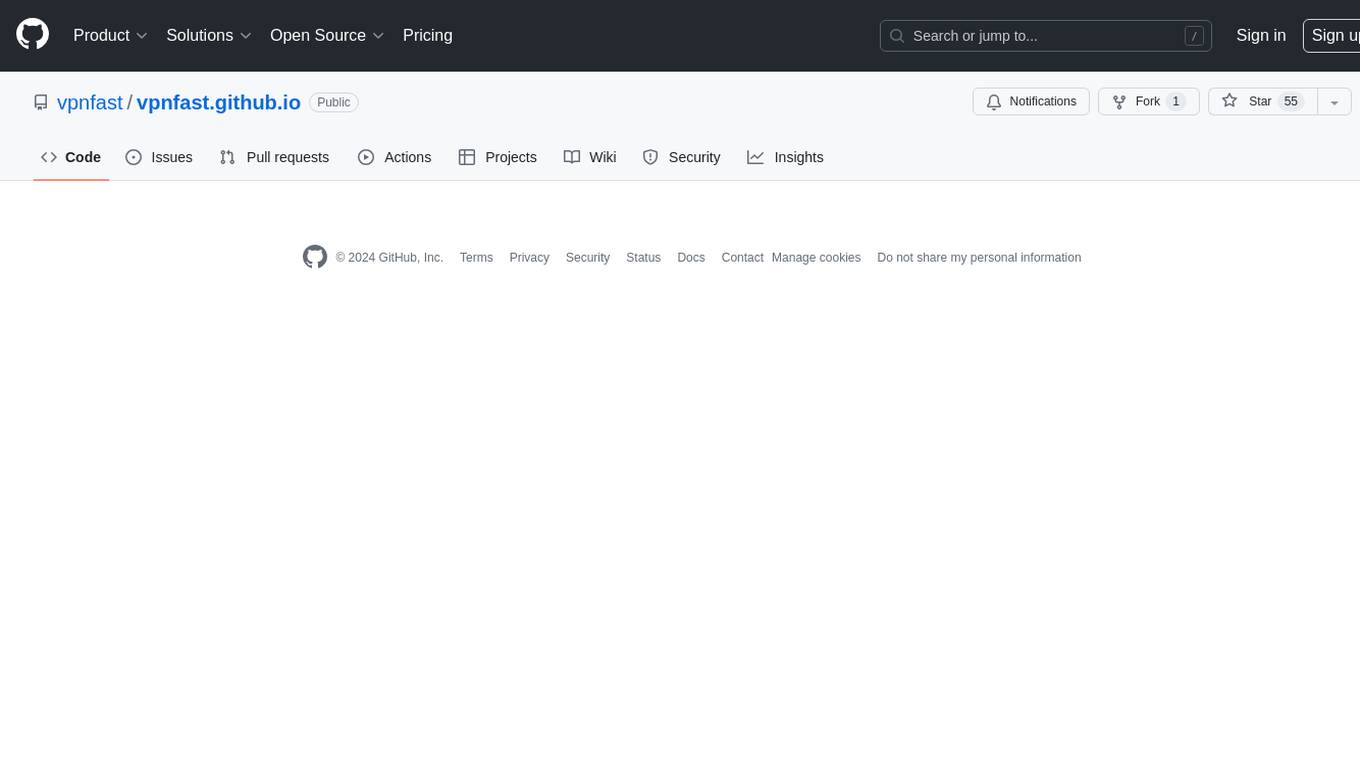
vpnfast.github.io
VPNFast is a lightweight and fast VPN service provider that offers secure and private internet access. With VPNFast, users can protect their online privacy, bypass geo-restrictions, and secure their internet connection from hackers and snoopers. The service provides high-speed servers in multiple locations worldwide, ensuring a reliable and seamless VPN experience for users. VPNFast is easy to use, with a user-friendly interface and simple setup process. Whether you're browsing the web, streaming content, or accessing sensitive information, VPNFast helps you stay safe and anonymous online.
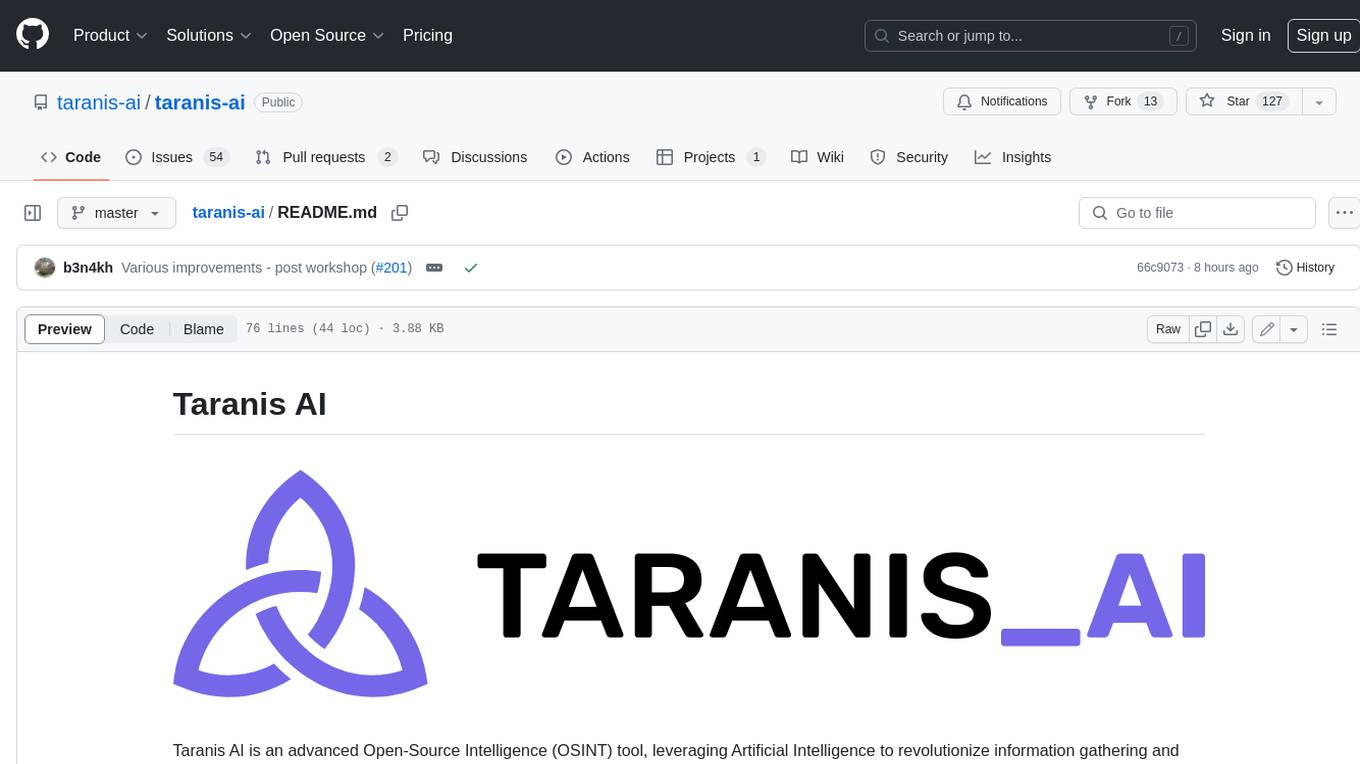
taranis-ai
Taranis AI is an advanced Open-Source Intelligence (OSINT) tool that leverages Artificial Intelligence to revolutionize information gathering and situational analysis. It navigates through diverse data sources like websites to collect unstructured news articles, utilizing Natural Language Processing and Artificial Intelligence to enhance content quality. Analysts then refine these AI-augmented articles into structured reports that serve as the foundation for deliverables such as PDF files, which are ultimately published.