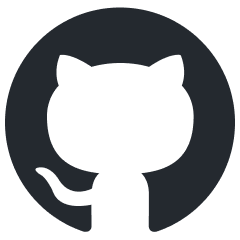
ail-framework
AIL framework - Analysis Information Leak framework
Stars: 696
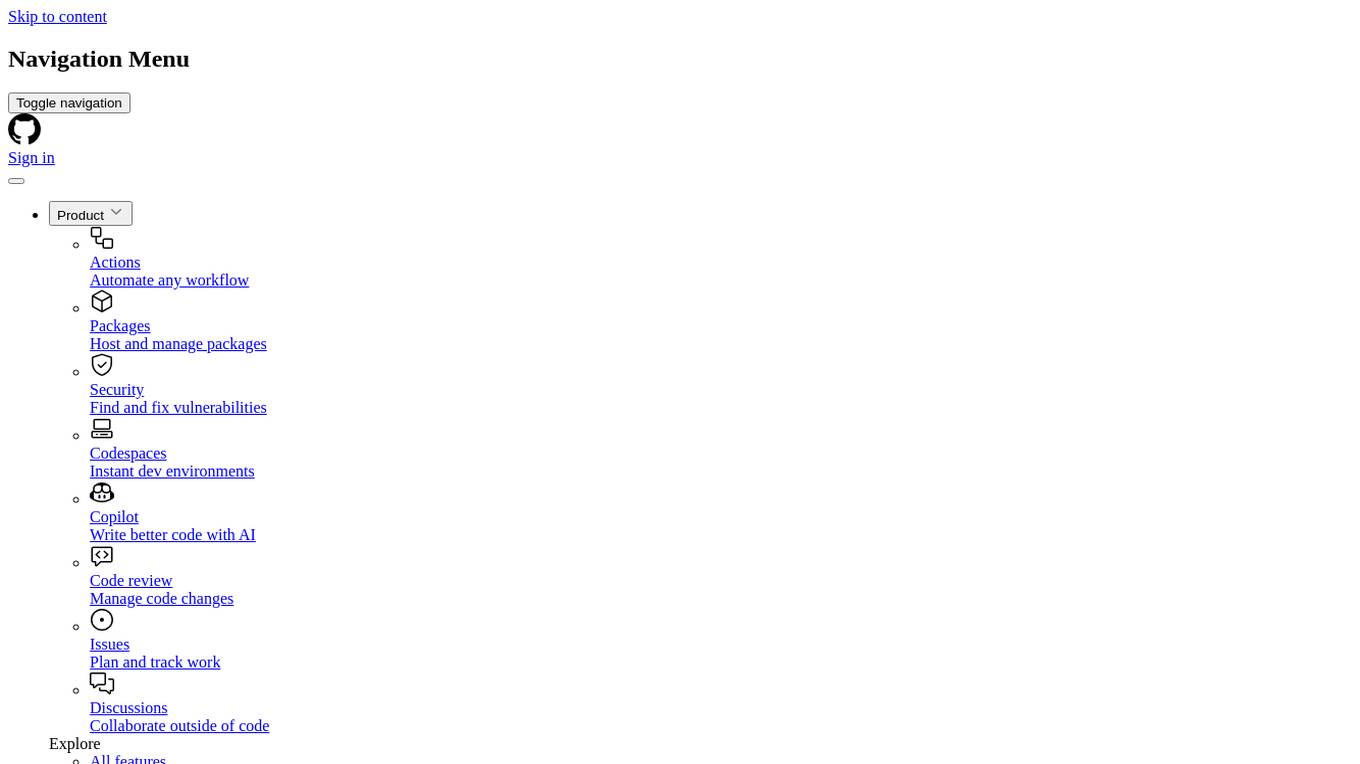
AIL framework is a modular framework to analyze potential information leaks from unstructured data sources like pastes from Pastebin or similar services or unstructured data streams. AIL framework is flexible and can be extended to support other functionalities to mine or process sensitive information (e.g. data leak prevention).
README:
Latest Release | |
CI | |
Gitter | |
Contributors | |
License |
AIL framework - Framework for Analysis of Information Leaks
AIL is a modular framework to analyse potential information leaks from unstructured data sources like pastes from Pastebin or similar services or unstructured data streams. AIL framework is flexible and can be extended to support other functionalities to mine or process sensitive information (e.g. data leak prevention).
AIL v5.0 introduces significant improvements and new features:
- Codebase Rewrite: The codebase has undergone a substantial rewrite, resulting in enhanced performance and speed improvements.
- Database Upgrade: The database has been migrated from ARDB to Kvrocks.
- New Correlation Engine: AIL v5.0 introduces a new powerful correlation engine with two new correlation types: CVE and Title.
- Enhanced Logging: The logging system has been improved to provide better troubleshooting capabilities.
- Tagging Support: AIL objects now support tagging, allowing users to categorize and label extracted information for easier analysis and organization.
- Trackers: Improved objects filtering, PGP and decoded tracking added.
- UI Content Visualization: The user interface has been upgraded to visualize extracted and tracked information.
- New Crawler Lacus: improve crawling capabilities.
- Modular Importers and Exporters: New importers (ZMQ, AIL Feeders) and exporters (MISP, Mail, TheHive) modular design. Allow easy creation and customization by extending an abstract class.
- Module Queues: improved the queuing mechanism between detection modules.
- New Object CVE and Title: Extract an correlate CVE IDs and web page titles.
- Modular architecture to handle streams of unstructured or structured information
- Default support for external ZMQ feeds, such as provided by CIRCL or other providers
- Multiple Importers and feeds support
- Each module can process and reprocess the information already analyzed by AIL
- Detecting and extracting URLs including their geographical location (e.g. IP address location)
- Extracting and validating potential leaks of credit card numbers, credentials, ...
- Extracting and validating leaked email addresses, including DNS MX validation
- Module for extracting Tor .onion addresses for further analysis
- Keep tracks of credentials duplicates (and diffing between each duplicate found)
- Extracting and validating potential hostnames (e.g. to feed Passive DNS systems)
- A full-text indexer module to index unstructured information
- Terms, Set of terms, Regex, typo squatting and YARA tracking and occurrence
- YARA Retro Hunt
- Many more modules for extracting phone numbers, credentials, and more
- Alerting to MISP to share found leaks within a threat intelligence platform using MISP standard
- Detecting and decoding encoded file (Base64, hex encoded or your own decoding scheme) and storing files
- Detecting Amazon AWS and Google API keys
- Detecting Bitcoin address and Bitcoin private keys
- Detecting private keys, certificate, keys (including SSH, OpenVPN)
- Detecting IBAN bank accounts
- Tagging system with MISP Galaxy and MISP Taxonomies tags
- UI submission
- Create events on MISP and cases on The Hive
- Automatic export on detection with MISP (events) and The Hive (alerts) on selected tags
- Extracted and decoded files can be searched by date range, type of file (mime-type) and encoding discovered
- Correlations engine and Graph to visualize relationships between decoded files (hashes), PGP UIDs, domains, username, and cryptocurrencies addresses
- Websites, Forums and Tor Hidden-Services hidden services crawler to crawl and parse output
- Domain availability monitoring to detect up and down of websites and hidden services
- Browsed hidden services are automatically captured and integrated into the analyzed output, including a blurring screenshot interface (to avoid "burning the eyes" of security analysts with sensitive content)
- Tor hidden services is part of the standard framework, all the AIL modules are available to the crawled hidden services
- Crawler scheduler to trigger crawling on demand or at regular intervals for URLs or Tor hidden services
To install the AIL framework, run the following commands:
# Clone the repo first
git clone https://github.com/ail-project/ail-framework.git
cd ail-framework
# For Debian and Ubuntu based distributions
./installing_deps.sh
# Launch ail
cd ~/ail-framework/
cd bin/
./LAUNCH.sh -l
The default installing_deps.sh is for Debian and Ubuntu based distributions.
Requirement:
- Python 3.8+
For Lacus Crawler and LibreTranslate installation instructions (if you want to use those features), refer to the HOWTO
To start AIL, use the following commands:
cd bin/
./LAUNCH.sh -l
You can access the AIL framework web interface at the following URL:
https://localhost:7000/
The default credentials for the web interface are located in the DEFAULT_PASSWORD
file, which is deleted when you change your password.
CIRCL organises training on how to use or extend the AIL framework. AIL training materials are available at https://github.com/ail-project/ail-training.
The API documentation is available in doc/api.md
HOWTO are available in HOWTO.md
For information on AIL's compliance with GDPR and privacy considerations, refer to the AIL information leaks analysis and the GDPR in the context of collection, analysis and sharing information leaks document.
this document provides an overview how to use AIL in a lawfulness context especially in the scope of General Data Protection Regulation.
If you use or reference AIL in an academic paper, you can cite it using the following BibTeX:
@inproceedings{mokaddem2018ail,
title={AIL-The design and implementation of an Analysis Information Leak framework},
author={Mokaddem, Sami and Wagener, G{\'e}rard and Dulaunoy, Alexandre},
booktitle={2018 IEEE International Conference on Big Data (Big Data)},
pages={5049--5057},
year={2018},
organization={IEEE}
}
Websites, Forums and Tor Hidden-Services
Copyright (C) 2014 Jules Debra
Copyright (c) 2021 Olivier Sagit
Copyright (C) 2014-2024 CIRCL - Computer Incident Response Center Luxembourg (c/o smile, security made in Lëtzebuerg, Groupement d'Intérêt Economique)
Copyright (c) 2014-2024 Raphaël Vinot
Copyright (c) 2014-2024 Alexandre Dulaunoy
Copyright (c) 2016-2024 Sami Mokaddem
Copyright (c) 2018-2024 Thirion Aurélien
This program is free software: you can redistribute it and/or modify
it under the terms of the GNU Affero General Public License as published by
the Free Software Foundation, either version 3 of the License, or
(at your option) any later version.
This program is distributed in the hope that it will be useful,
but WITHOUT ANY WARRANTY; without even the implied warranty of
MERCHANTABILITY or FITNESS FOR A PARTICULAR PURPOSE. See the
GNU Affero General Public License for more details.
You should have received a copy of the GNU Affero General Public License
along with this program. If not, see <http://www.gnu.org/licenses/>.
For Tasks:
Click tags to check more tools for each tasksFor Jobs:
Alternative AI tools for ail-framework
Similar Open Source Tools
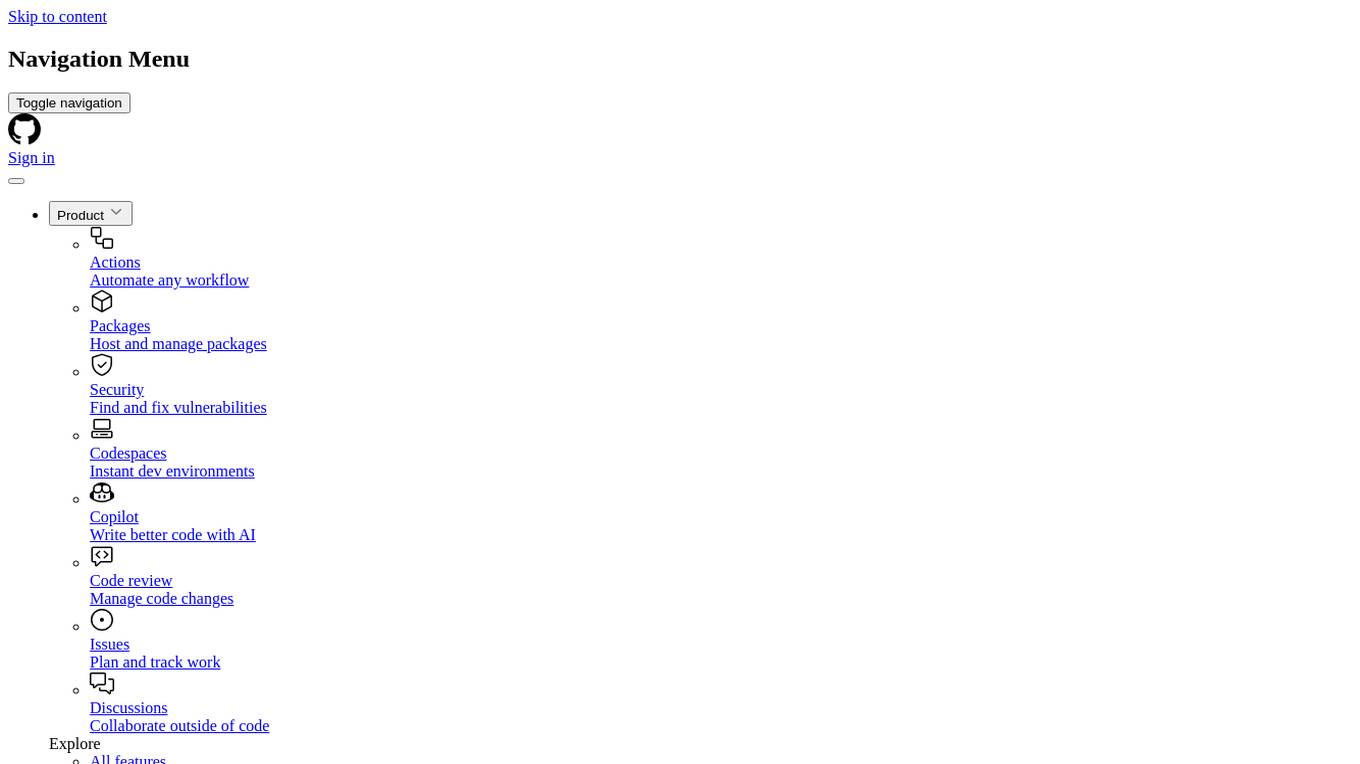
ail-framework
AIL framework is a modular framework to analyze potential information leaks from unstructured data sources like pastes from Pastebin or similar services or unstructured data streams. AIL framework is flexible and can be extended to support other functionalities to mine or process sensitive information (e.g. data leak prevention).
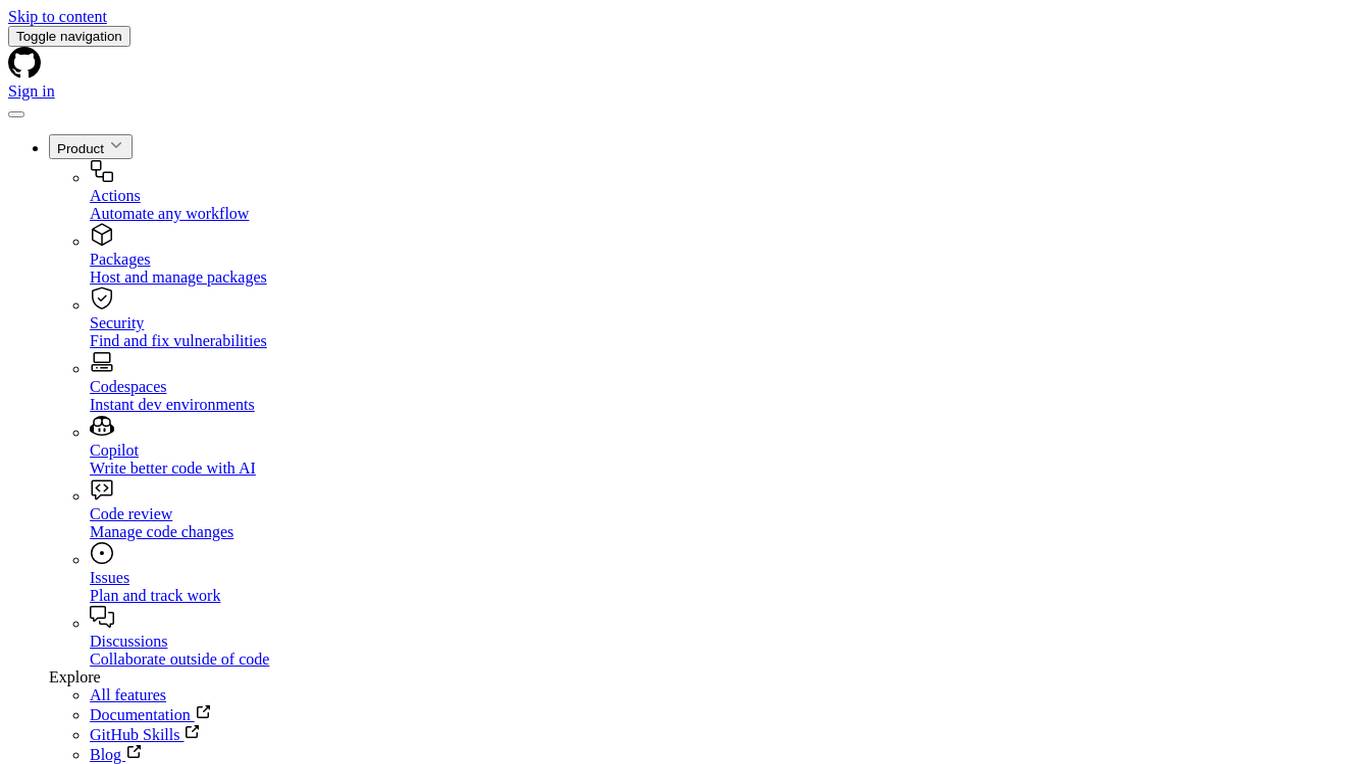
AIL-framework
AIL framework is a modular framework to analyze potential information leaks from unstructured data sources like pastes from Pastebin or similar services or unstructured data streams. AIL framework is flexible and can be extended to support other functionalities to mine or process sensitive information (e.g. data leak prevention).
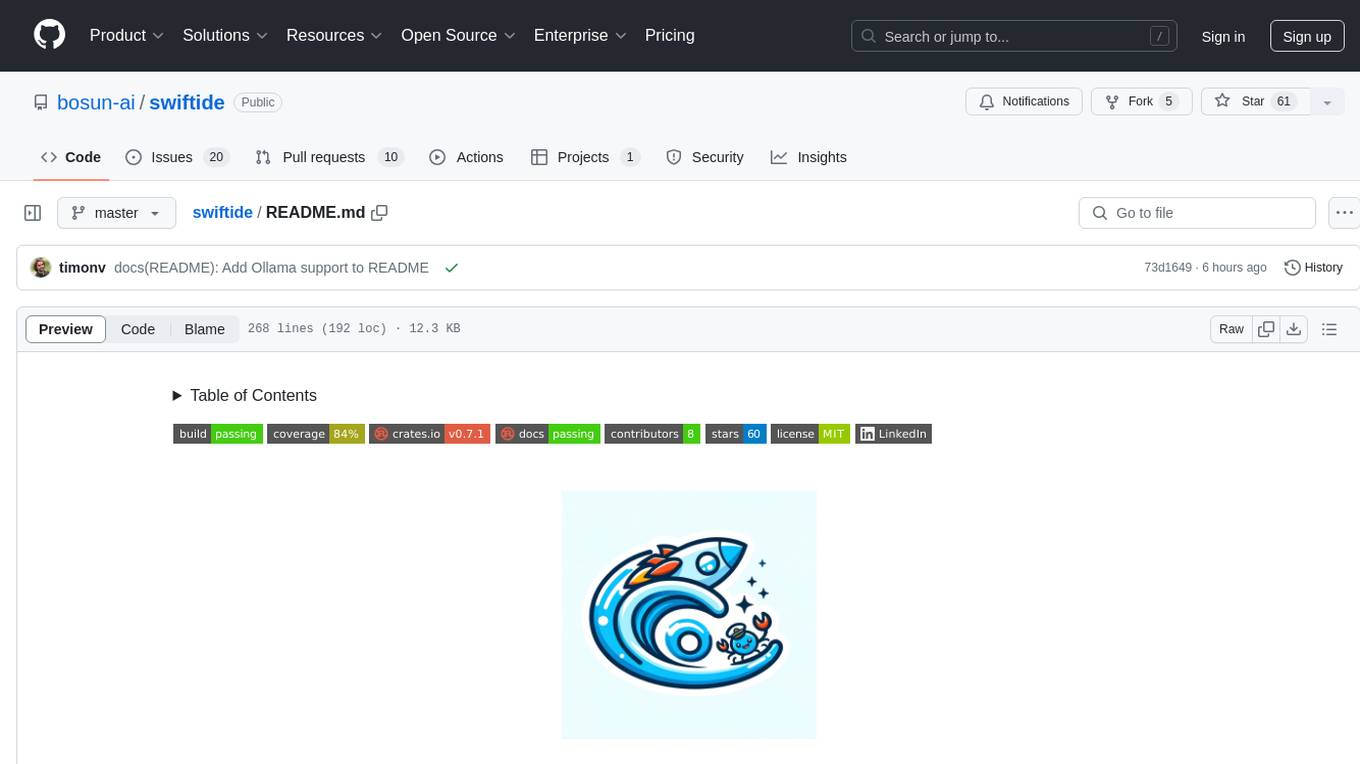
swiftide
Swiftide is a fast, streaming indexing and query library tailored for Retrieval Augmented Generation (RAG) in AI applications. It is built in Rust, utilizing parallel, asynchronous streams for blazingly fast performance. With Swiftide, users can easily build AI applications from idea to production in just a few lines of code. The tool addresses frustrations around performance, stability, and ease of use encountered while working with Python-based tooling. It offers features like fast streaming indexing pipeline, experimental query pipeline, integrations with various platforms, loaders, transformers, chunkers, embedders, and more. Swiftide aims to provide a platform for data indexing and querying to advance the development of automated Large Language Model (LLM) applications.
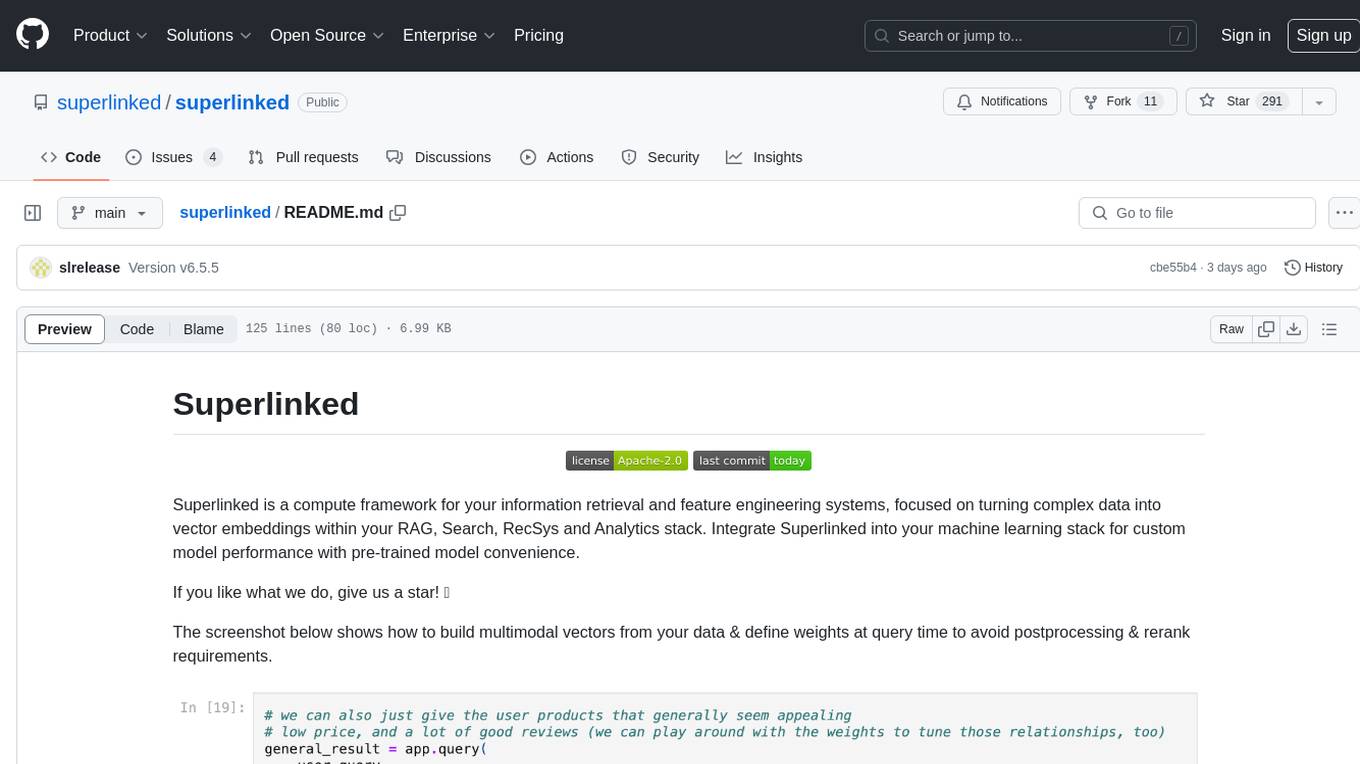
superlinked
Superlinked is a compute framework for information retrieval and feature engineering systems, focusing on converting complex data into vector embeddings for RAG, Search, RecSys, and Analytics stack integration. It enables custom model performance in machine learning with pre-trained model convenience. The tool allows users to build multimodal vectors, define weights at query time, and avoid postprocessing & rerank requirements. Users can explore the computational model through simple scripts and python notebooks, with a future release planned for production usage with built-in data infra and vector database integrations.
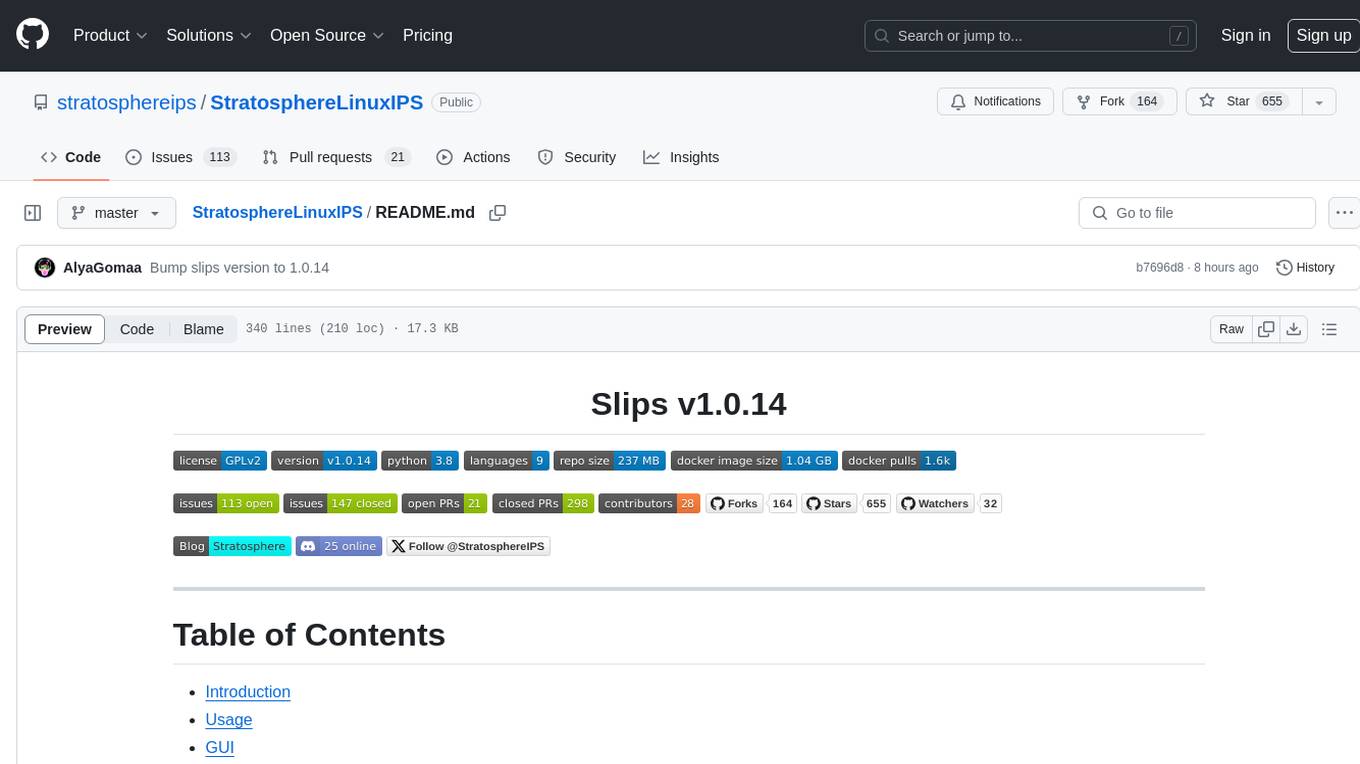
StratosphereLinuxIPS
Slips is a powerful endpoint behavioral intrusion prevention and detection system that uses machine learning to detect malicious behaviors in network traffic. It can work with network traffic in real-time, PCAP files, and network flows from tools like Suricata, Zeek/Bro, and Argus. Slips threat detection is based on machine learning models, threat intelligence feeds, and expert heuristics. It gathers evidence of malicious behavior and triggers alerts when enough evidence is accumulated. The tool is Python-based and supported on Linux and MacOS, with blocking features only on Linux. Slips relies on Zeek network analysis framework and Redis for interprocess communication. It offers a graphical user interface for easy monitoring and analysis.
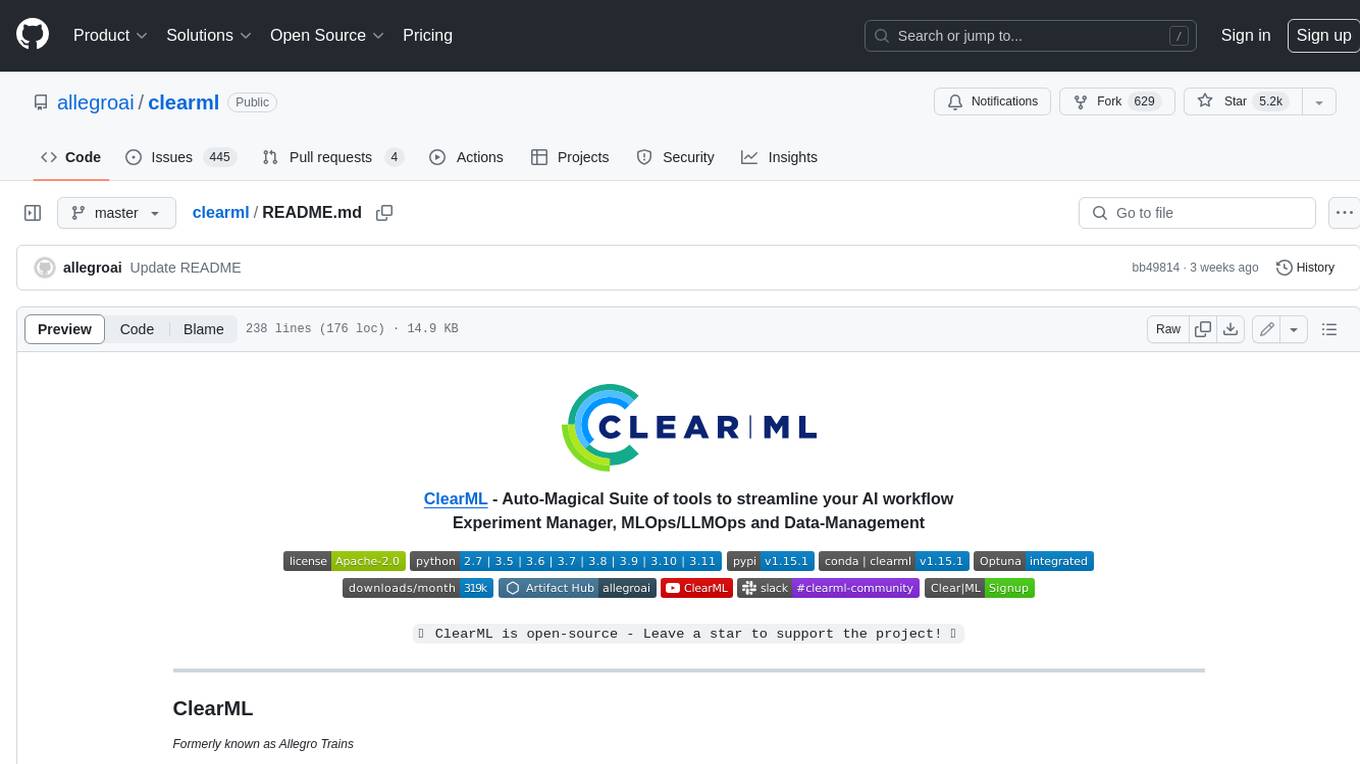
clearml
ClearML is a suite of tools designed to streamline the machine learning workflow. It includes an experiment manager, MLOps/LLMOps, data management, and model serving capabilities. ClearML is open-source and offers a free tier hosting option. It supports various ML/DL frameworks and integrates with Jupyter Notebook and PyCharm. ClearML provides extensive logging capabilities, including source control info, execution environment, hyper-parameters, and experiment outputs. It also offers automation features, such as remote job execution and pipeline creation. ClearML is designed to be easy to integrate, requiring only two lines of code to add to existing scripts. It aims to improve collaboration, visibility, and data transparency within ML teams.
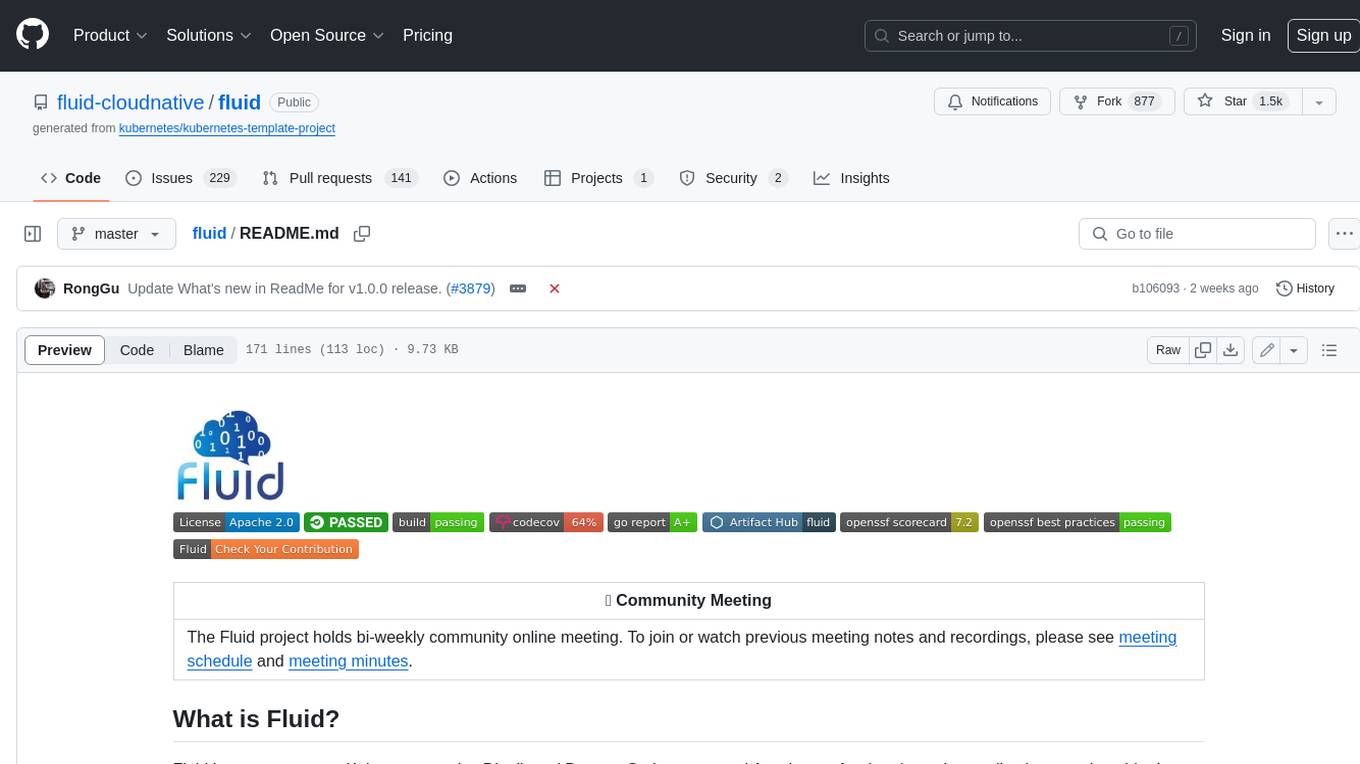
fluid
Fluid is an open source Kubernetes-native Distributed Dataset Orchestrator and Accelerator for data-intensive applications, such as big data and AI applications. It implements dataset abstraction, scalable cache runtime, automated data operations, elasticity and scheduling, and is runtime platform agnostic. Key concepts include Dataset and Runtime. Prerequisites include Kubernetes version > 1.16, Golang 1.18+, and Helm 3. The tool offers features like accelerating remote file accessing, machine learning, accelerating PVC, preloading dataset, and on-the-fly dataset cache scaling. Contributions are welcomed, and the project is under the Apache 2.0 license with a vendor-neutral approach.
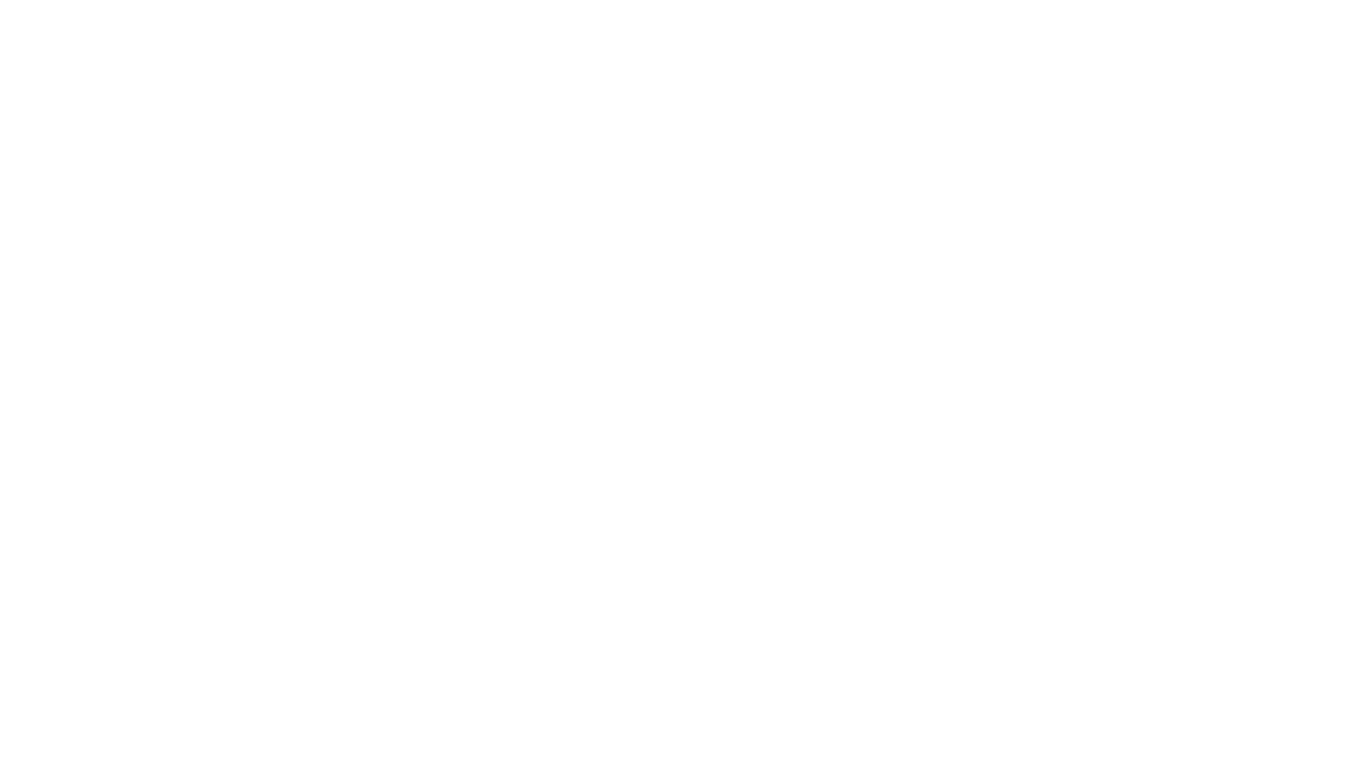
cleanlab
Cleanlab helps you **clean** data and **lab** els by automatically detecting issues in a ML dataset. To facilitate **machine learning with messy, real-world data** , this data-centric AI package uses your _existing_ models to estimate dataset problems that can be fixed to train even _better_ models.
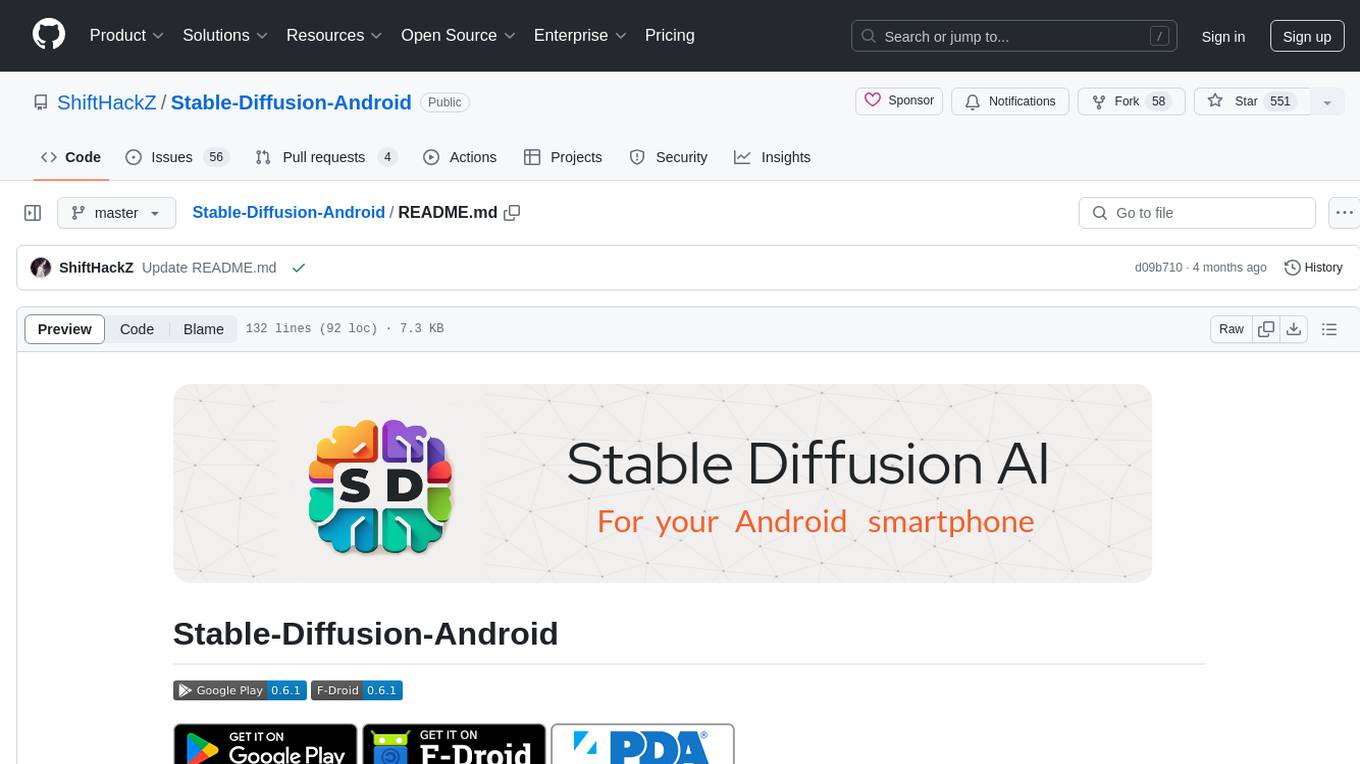
Stable-Diffusion-Android
Stable Diffusion AI is an easy-to-use app for generating images from text or other images. It allows communication with servers powered by various AI technologies like AI Horde, Hugging Face Inference API, OpenAI, StabilityAI, and LocalDiffusion. The app supports Txt2Img and Img2Img modes, positive and negative prompts, dynamic size and sampling methods, unique seed input, and batch image generation. Users can also inpaint images, select faces from gallery or camera, and export images. The app offers settings for server URL, SD Model selection, auto-saving images, and clearing cache.
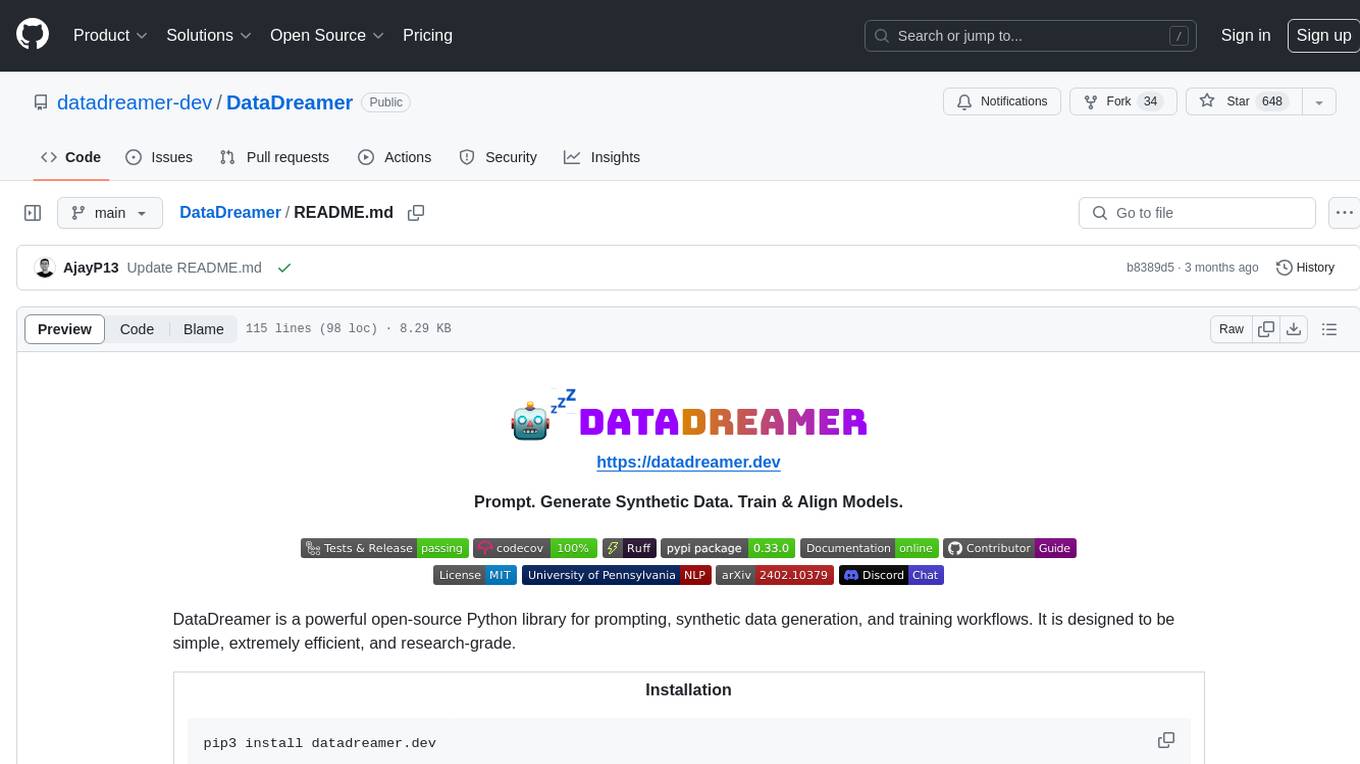
DataDreamer
DataDreamer is a powerful open-source Python library designed for prompting, synthetic data generation, and training workflows. It is simple, efficient, and research-grade, allowing users to create prompting workflows, generate synthetic datasets, and train models with ease. The library is built for researchers, by researchers, focusing on correctness, best practices, and reproducibility. It offers features like aggressive caching, resumability, support for bleeding-edge techniques, and easy sharing of datasets and models. DataDreamer enables users to run multi-step prompting workflows, generate synthetic datasets for various tasks, and train models by aligning, fine-tuning, instruction-tuning, and distilling them using existing or synthetic data.
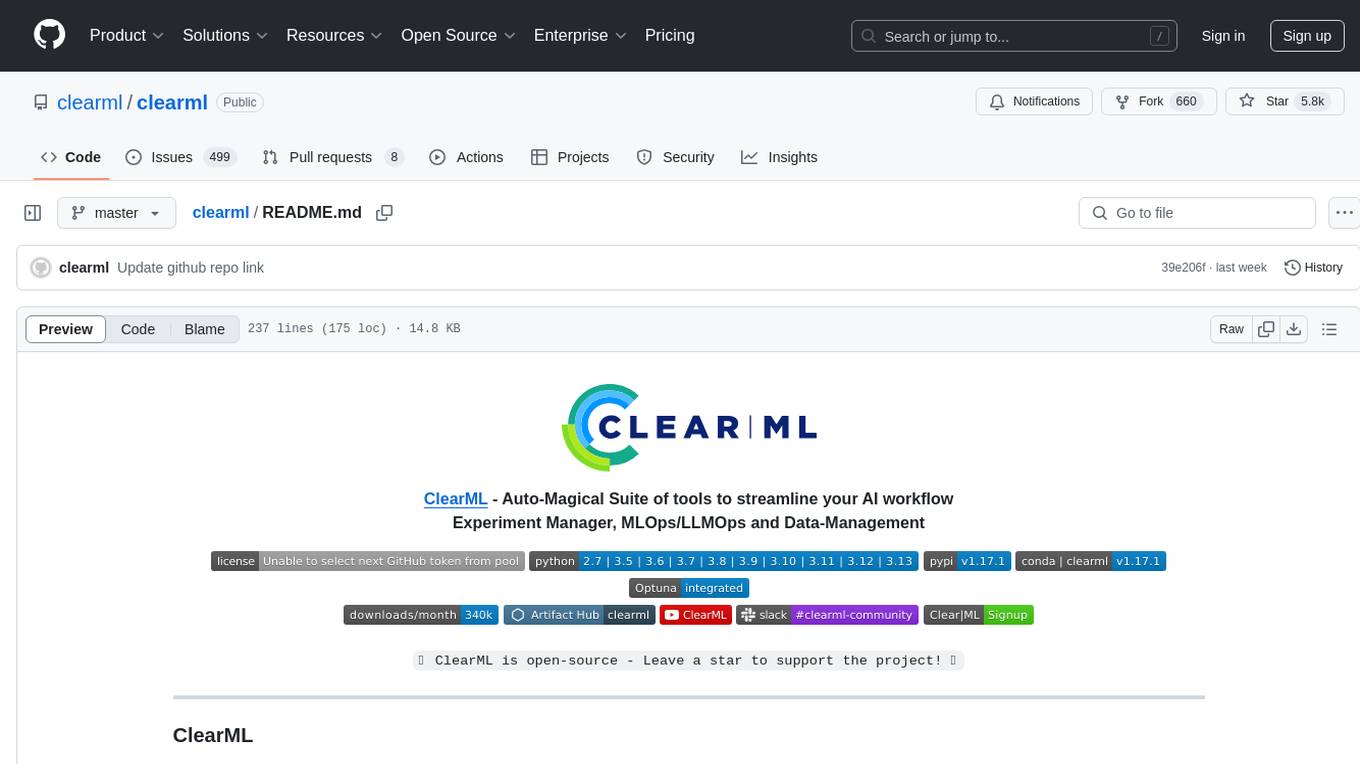
clearml
ClearML is an auto-magical suite of tools designed to streamline AI workflows. It includes modules for experiment management, MLOps/LLMOps, data management, model serving, and more. ClearML offers features like experiment tracking, model serving, orchestration, and automation. It supports various ML/DL frameworks and integrates with Jupyter Notebook and PyCharm for remote debugging. ClearML aims to simplify collaboration, automate processes, and enhance visibility in AI projects.
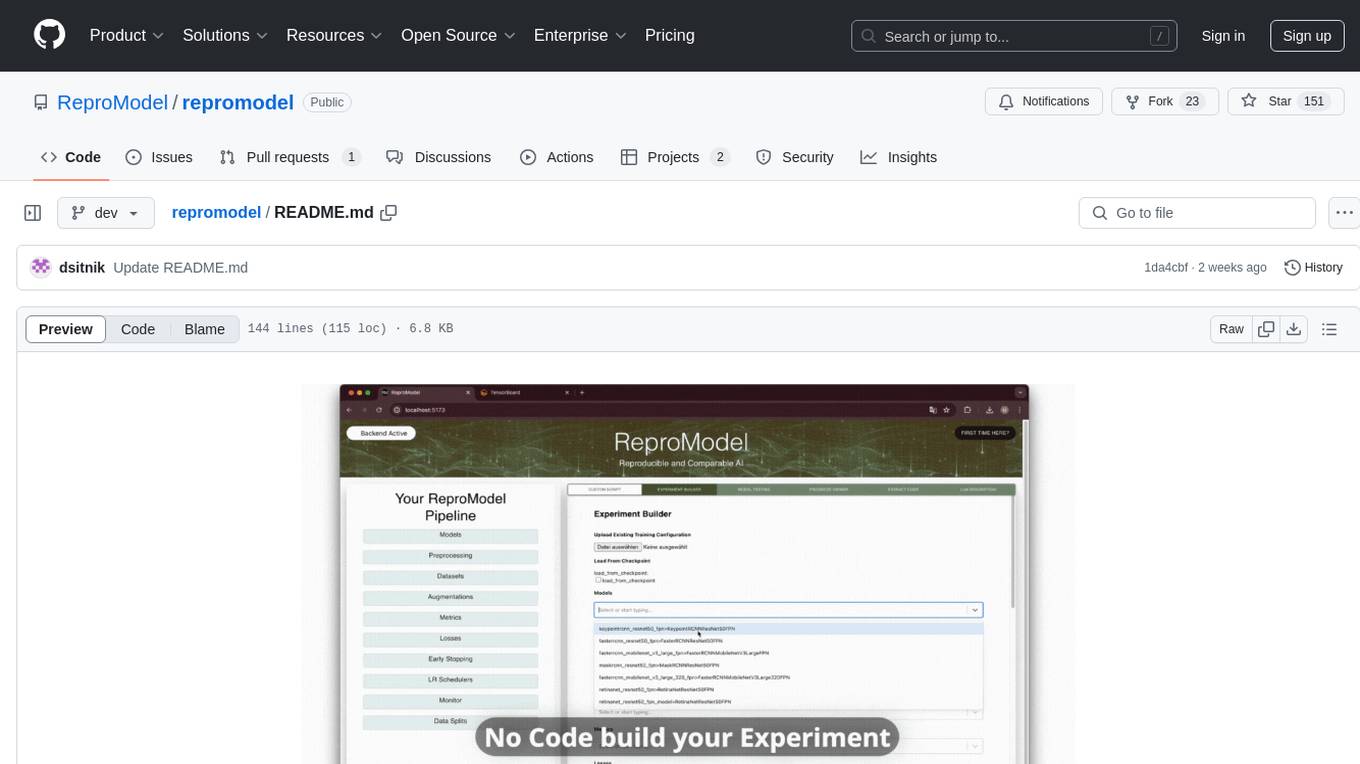
repromodel
ReproModel is an open-source toolbox designed to boost AI research efficiency by enabling researchers to reproduce, compare, train, and test AI models faster. It provides standardized models, dataloaders, and processing procedures, allowing researchers to focus on new datasets and model development. With a no-code solution, users can access benchmark and SOTA models and datasets, utilize training visualizations, extract code for publication, and leverage an LLM-powered automated methodology description writer. The toolbox helps researchers modularize development, compare pipeline performance reproducibly, and reduce time for model development, computation, and writing. Future versions aim to facilitate building upon state-of-the-art research by loading previously published study IDs with verified code, experiments, and results stored in the system.
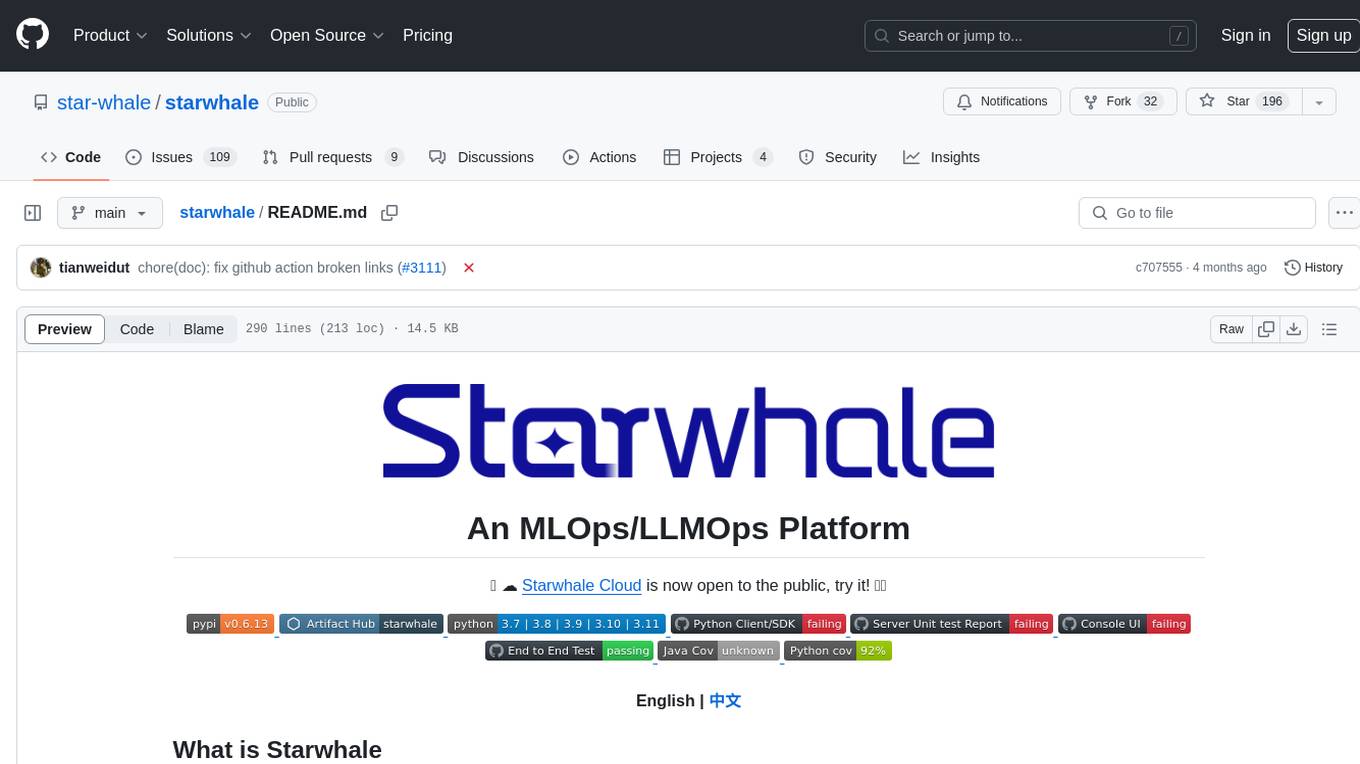
starwhale
Starwhale is an MLOps/LLMOps platform that brings efficiency and standardization to machine learning operations. It streamlines the model development lifecycle, enabling teams to optimize workflows around key areas like model building, evaluation, release, and fine-tuning. Starwhale abstracts Model, Runtime, and Dataset as first-class citizens, providing tailored capabilities for common workflow scenarios including Models Evaluation, Live Demo, and LLM Fine-tuning. It is an open-source platform designed for clarity and ease of use, empowering developers to build customized MLOps features tailored to their needs.
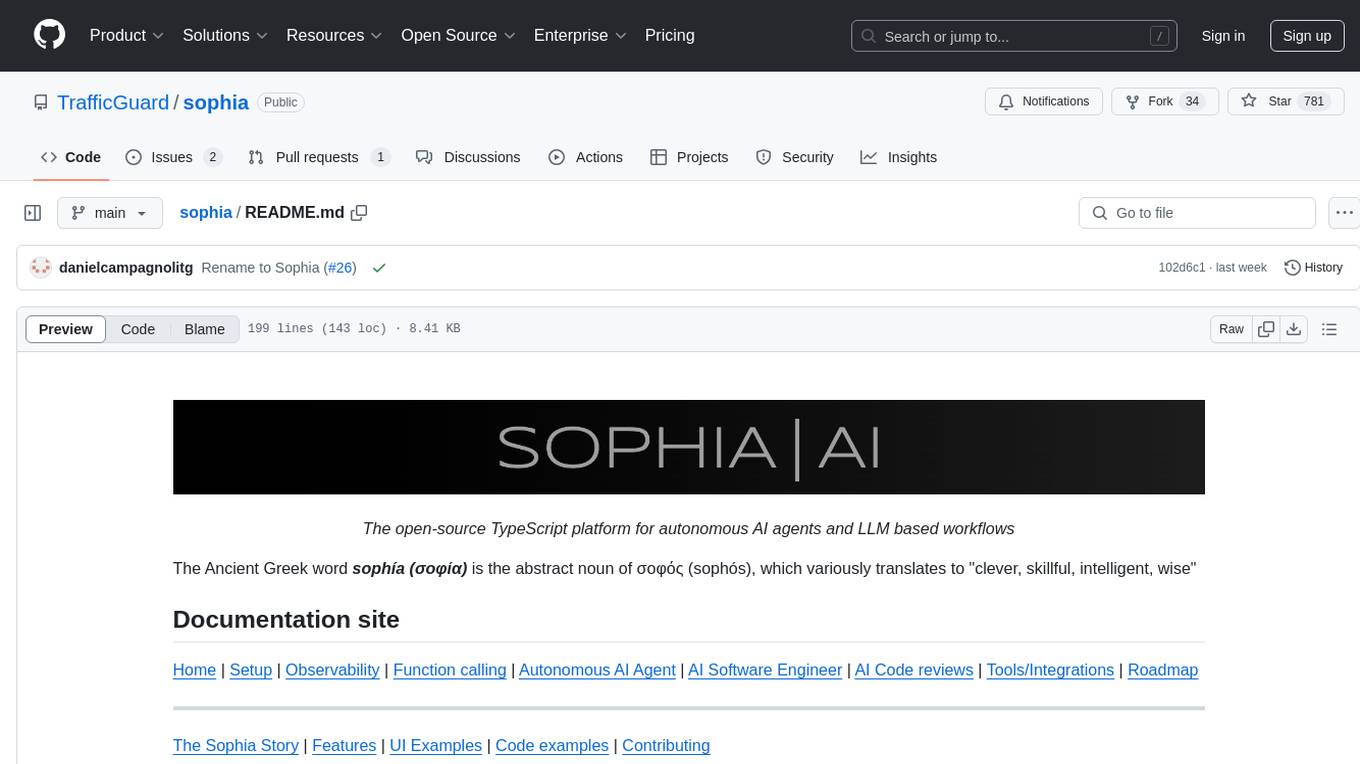
sophia
Sophia is an open-source TypeScript platform designed for autonomous AI agents and LLM based workflows. It aims to automate processes, review code, assist with refactorings, and support various integrations. The platform offers features like advanced autonomous agents, reasoning/planning inspired by Google's Self-Discover paper, memory and function call history, adaptive iterative planning, and more. Sophia supports multiple LLMs/services, CLI and web interface, human-in-the-loop interactions, flexible deployment options, observability with OpenTelemetry tracing, and specific agents for code editing, software engineering, and code review. It provides a flexible platform for the TypeScript community to expand and support various use cases and integrations.
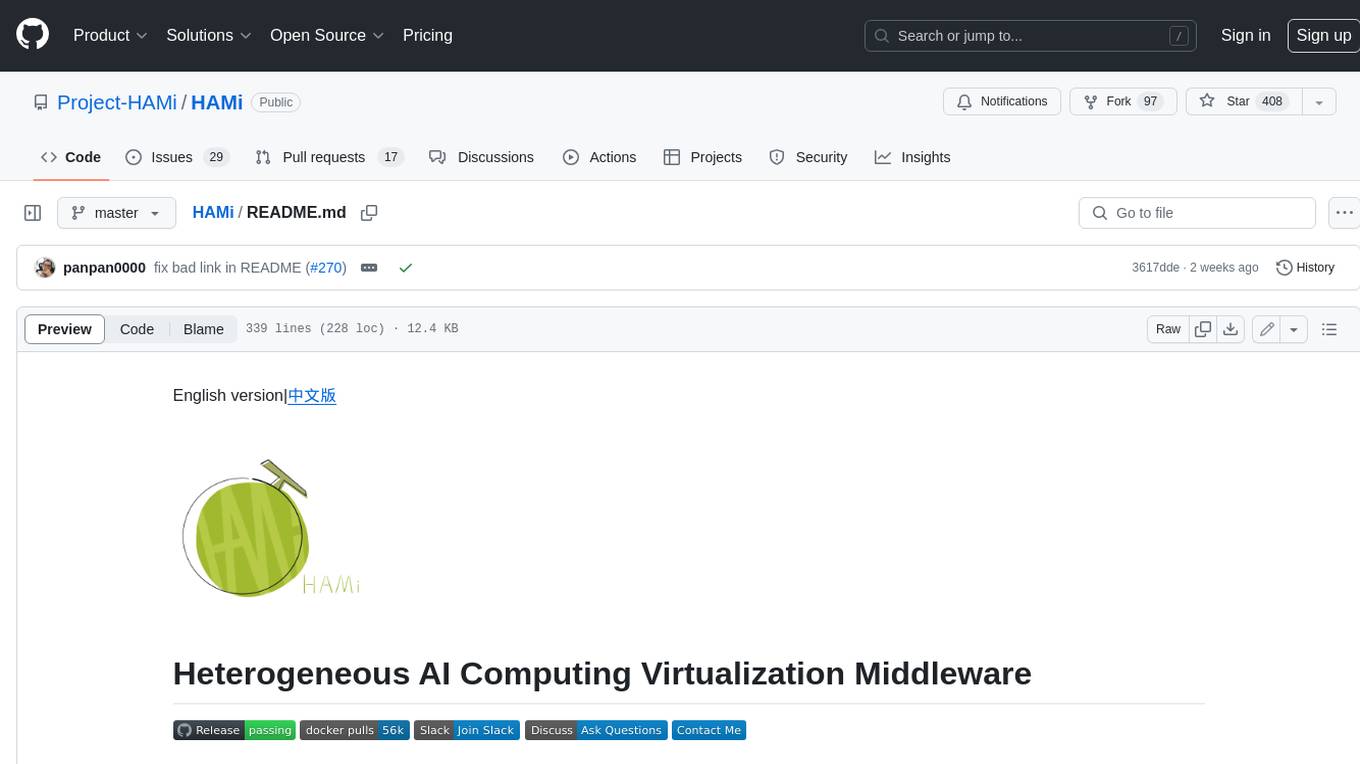
HAMi
HAMi is a Heterogeneous AI Computing Virtualization Middleware designed to manage Heterogeneous AI Computing Devices in a Kubernetes cluster. It allows for device sharing, device memory control, device type specification, and device UUID specification. The tool is easy to use and does not require modifying task YAML files. It includes features like hard limits on device memory, partial device allocation, streaming multiprocessor limits, and core usage specification. HAMi consists of components like a mutating webhook, scheduler extender, device plugins, and in-container virtualization techniques. It is suitable for scenarios requiring device sharing, specific device memory allocation, GPU balancing, low utilization optimization, and scenarios needing multiple small GPUs. The tool requires prerequisites like NVIDIA drivers, CUDA version, nvidia-docker, Kubernetes version, glibc version, and helm. Users can install, upgrade, and uninstall HAMi, submit tasks, and monitor cluster information. The tool's roadmap includes supporting additional AI computing devices, video codec processing, and Multi-Instance GPUs (MIG).
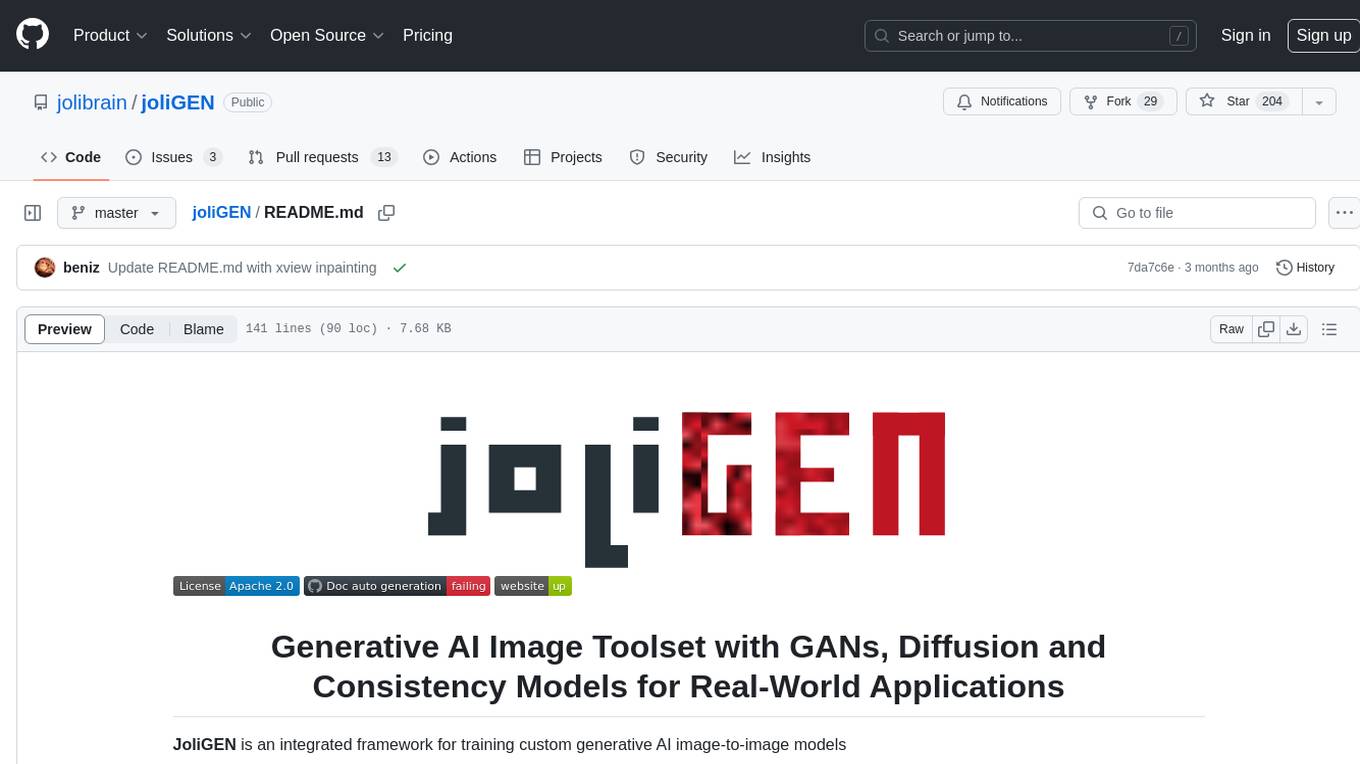
joliGEN
JoliGEN is an integrated framework for training custom generative AI image-to-image models. It implements GAN, Diffusion, and Consistency models for various image translation tasks, including domain and style adaptation with conservation of semantics. The tool is designed for real-world applications such as Controlled Image Generation, Augmented Reality, Dataset Smart Augmentation, and Synthetic to Real transforms. JoliGEN allows for fast and stable training with a REST API server for simplified deployment. It offers a wide range of options and parameters with detailed documentation available for models, dataset formats, and data augmentation.
For similar tasks
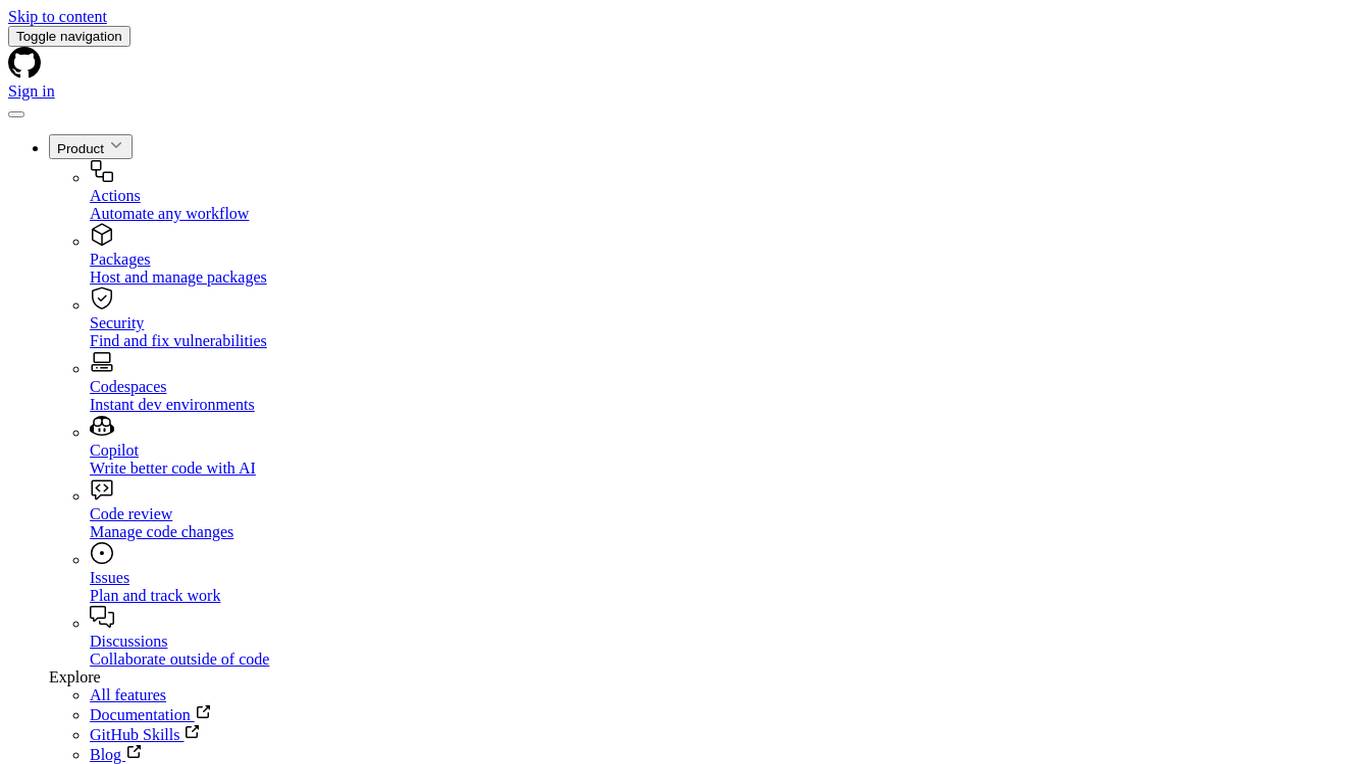
AIL-framework
AIL framework is a modular framework to analyze potential information leaks from unstructured data sources like pastes from Pastebin or similar services or unstructured data streams. AIL framework is flexible and can be extended to support other functionalities to mine or process sensitive information (e.g. data leak prevention).
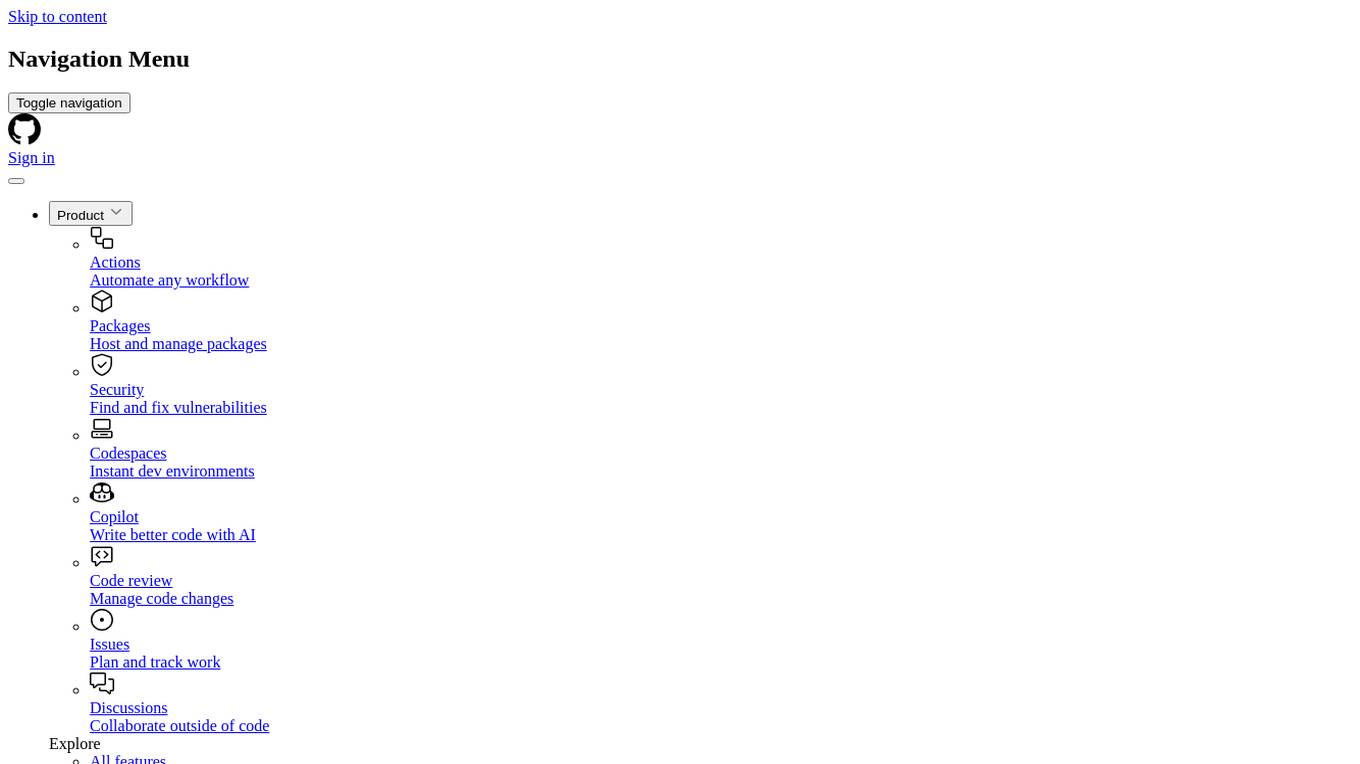
ail-framework
AIL framework is a modular framework to analyze potential information leaks from unstructured data sources like pastes from Pastebin or similar services or unstructured data streams. AIL framework is flexible and can be extended to support other functionalities to mine or process sensitive information (e.g. data leak prevention).
For similar jobs
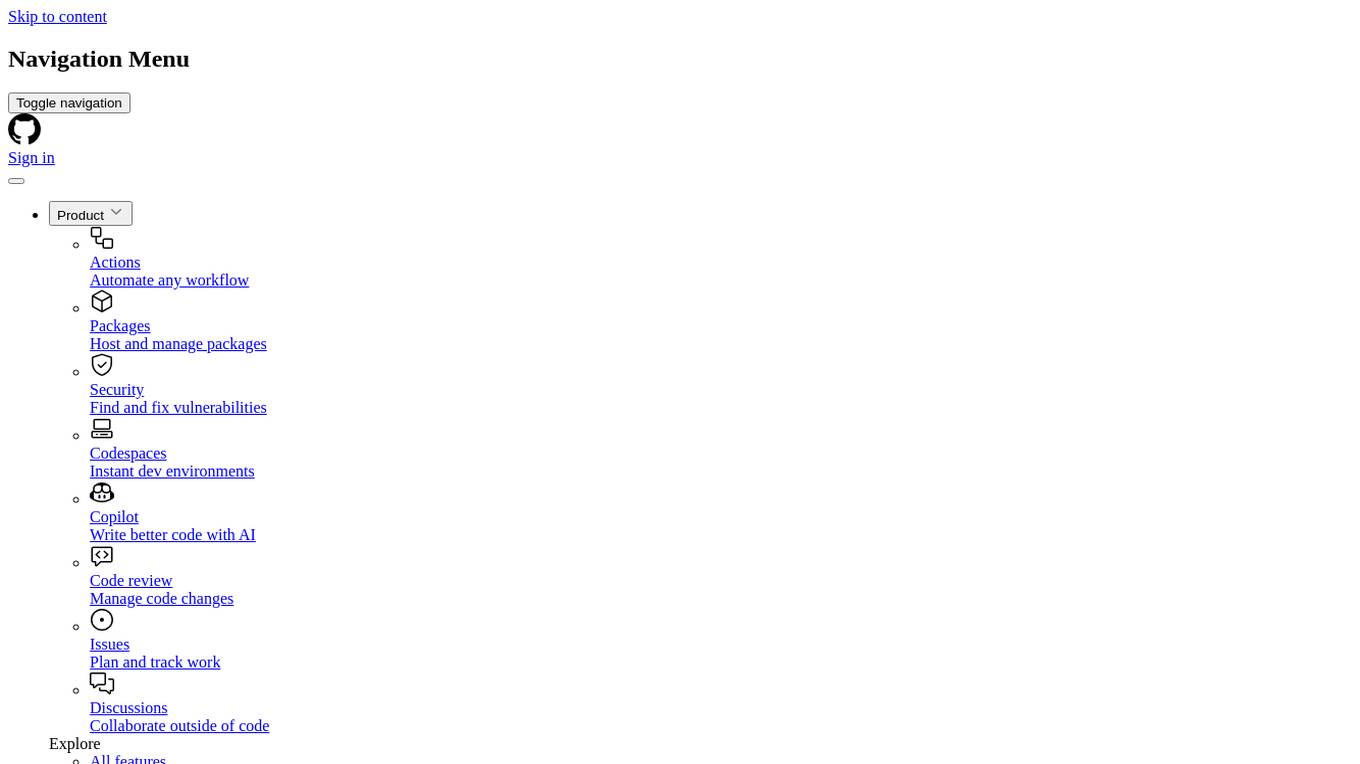
ail-framework
AIL framework is a modular framework to analyze potential information leaks from unstructured data sources like pastes from Pastebin or similar services or unstructured data streams. AIL framework is flexible and can be extended to support other functionalities to mine or process sensitive information (e.g. data leak prevention).
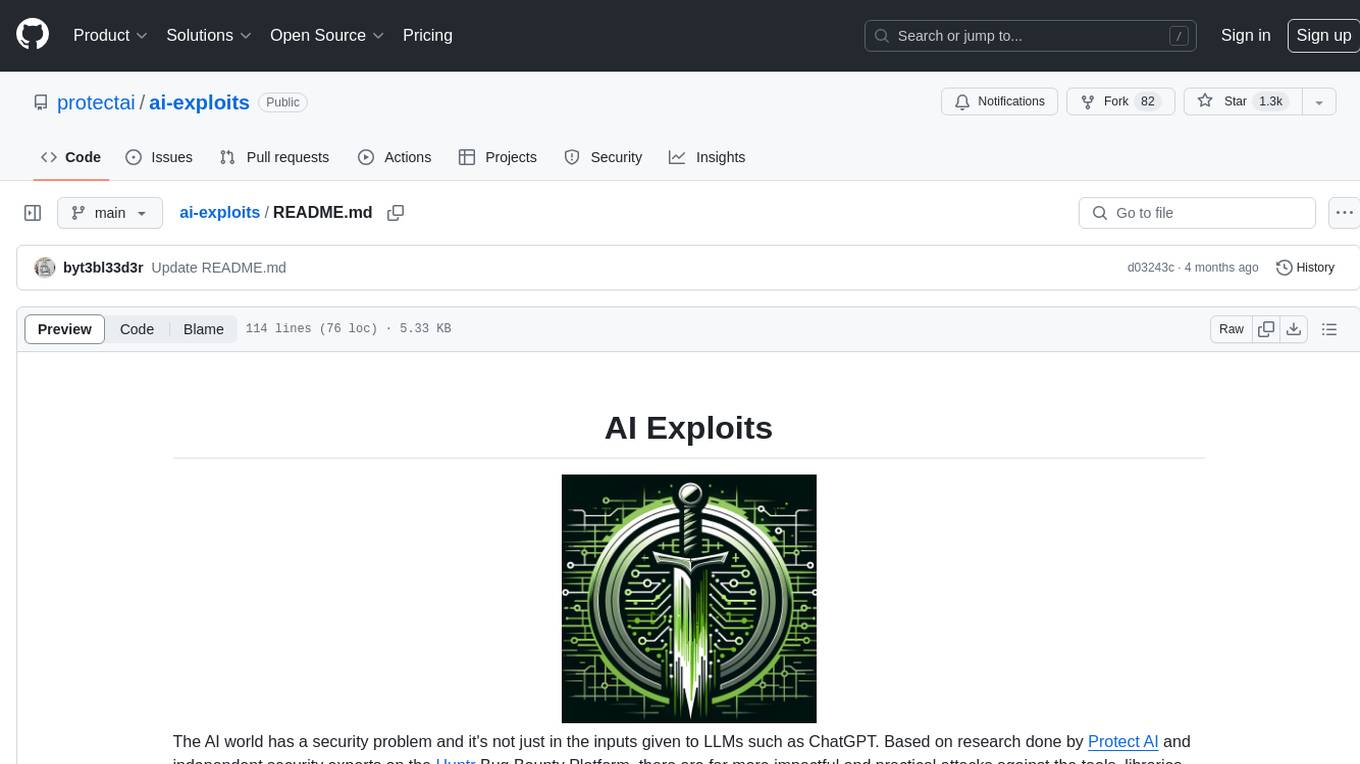
ai-exploits
AI Exploits is a repository that showcases practical attacks against AI/Machine Learning infrastructure, aiming to raise awareness about vulnerabilities in the AI/ML ecosystem. It contains exploits and scanning templates for responsibly disclosed vulnerabilities affecting machine learning tools, including Metasploit modules, Nuclei templates, and CSRF templates. Users can use the provided Docker image to easily run the modules and templates. The repository also provides guidelines for using Metasploit modules, Nuclei templates, and CSRF templates to exploit vulnerabilities in machine learning tools.
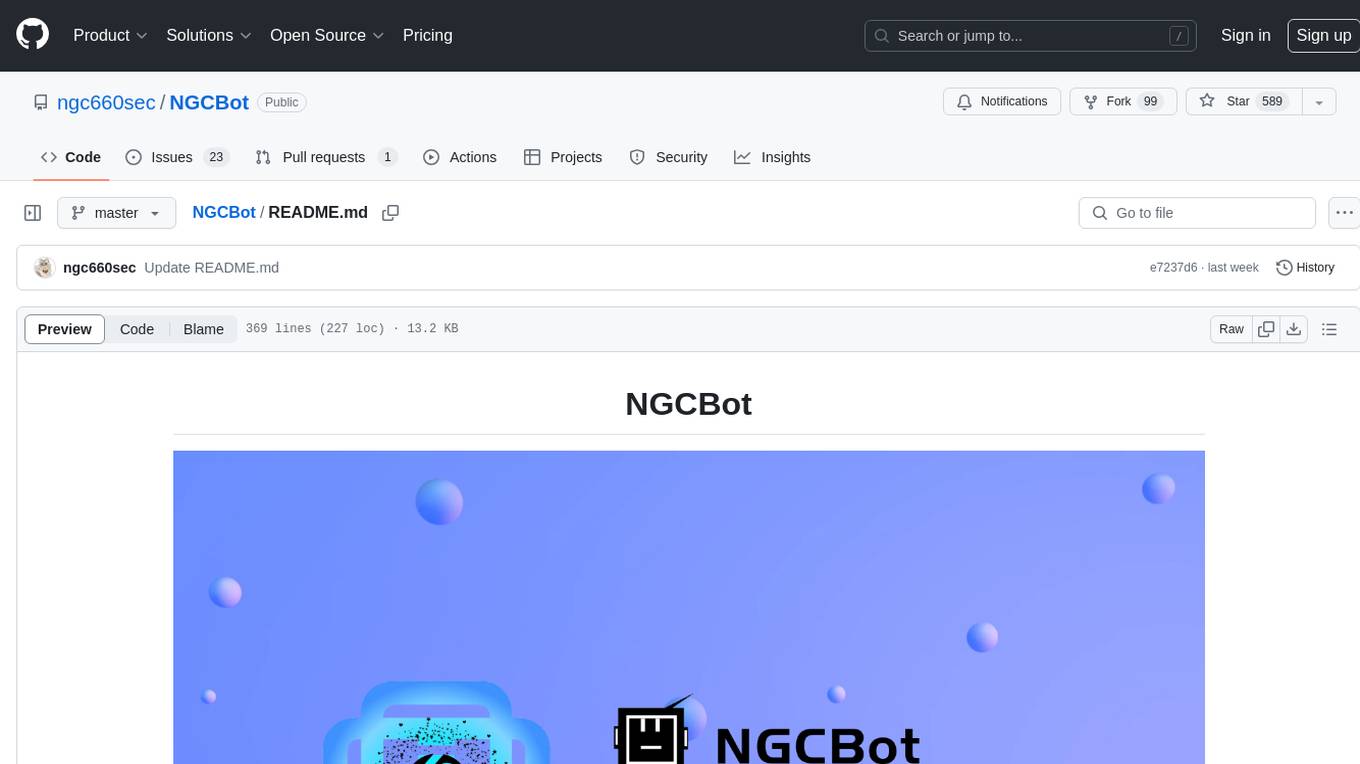
NGCBot
NGCBot is a WeChat bot based on the HOOK mechanism, supporting scheduled push of security news from FreeBuf, Xianzhi, Anquanke, and Qianxin Attack and Defense Community, KFC copywriting, filing query, phone number attribution query, WHOIS information query, constellation query, weather query, fishing calendar, Weibei threat intelligence query, beautiful videos, beautiful pictures, and help menu. It supports point functions, automatic pulling of people, ad detection, automatic mass sending, Ai replies, rich customization, and easy for beginners to use. The project is open-source and periodically maintained, with additional features such as Ai (Gpt, Xinghuo, Qianfan), keyword invitation to groups, automatic mass sending, and group welcome messages.
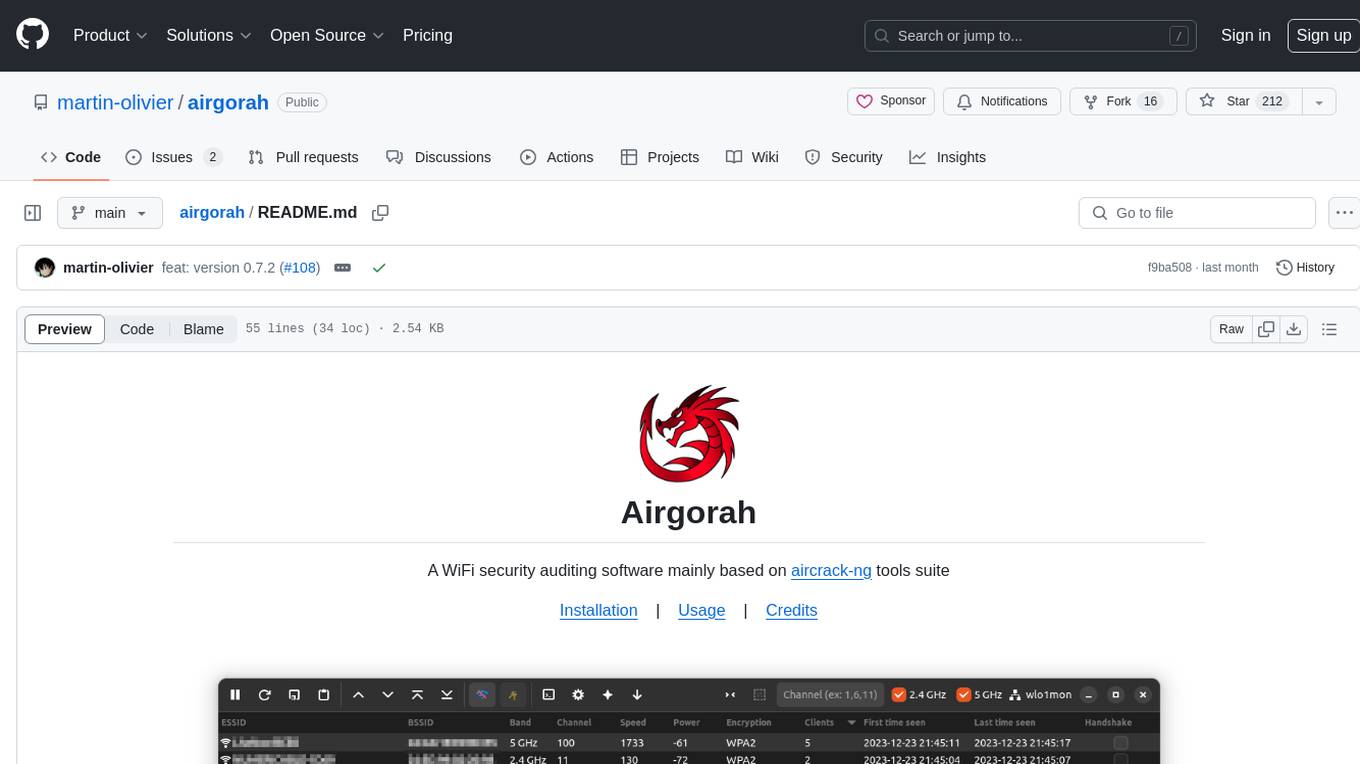
airgorah
Airgorah is a WiFi security auditing software written in Rust that utilizes the aircrack-ng tools suite. It allows users to capture WiFi traffic, discover connected clients, perform deauthentication attacks, capture handshakes, and crack access point passwords. The software is designed for testing and discovering flaws in networks owned by the user, and requires root privileges to run on Linux systems with a wireless network card supporting monitor mode and packet injection. Airgorah is not responsible for any illegal activities conducted with the software.
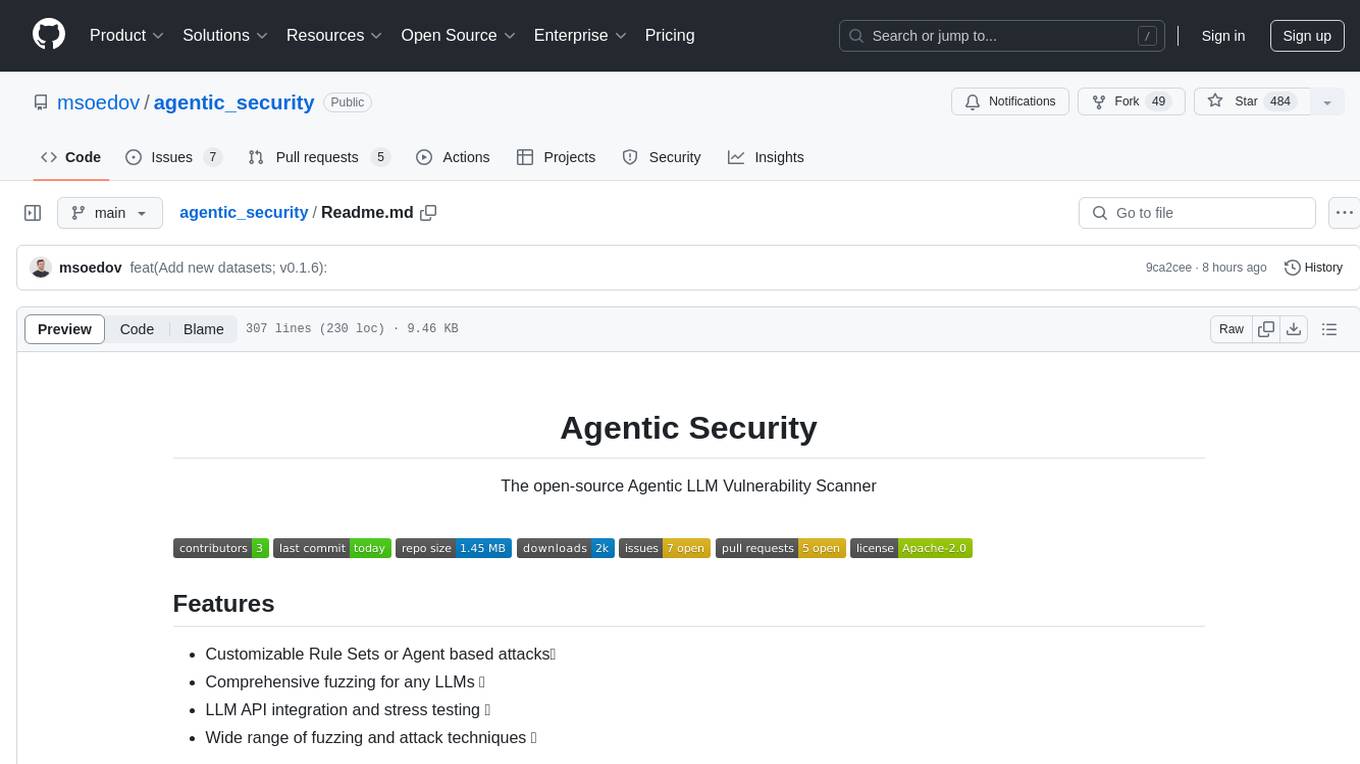
agentic_security
Agentic Security is an open-source vulnerability scanner designed for safety scanning, offering customizable rule sets and agent-based attacks. It provides comprehensive fuzzing for any LLMs, LLM API integration, and stress testing with a wide range of fuzzing and attack techniques. The tool is not a foolproof solution but aims to enhance security measures against potential threats. It offers installation via pip and supports quick start commands for easy setup. Users can utilize the tool for LLM integration, adding custom datasets, running CI checks, extending dataset collections, and dynamic datasets with mutations. The tool also includes a probe endpoint for integration testing. The roadmap includes expanding dataset variety, introducing new attack vectors, developing an attacker LLM, and integrating OWASP Top 10 classification.
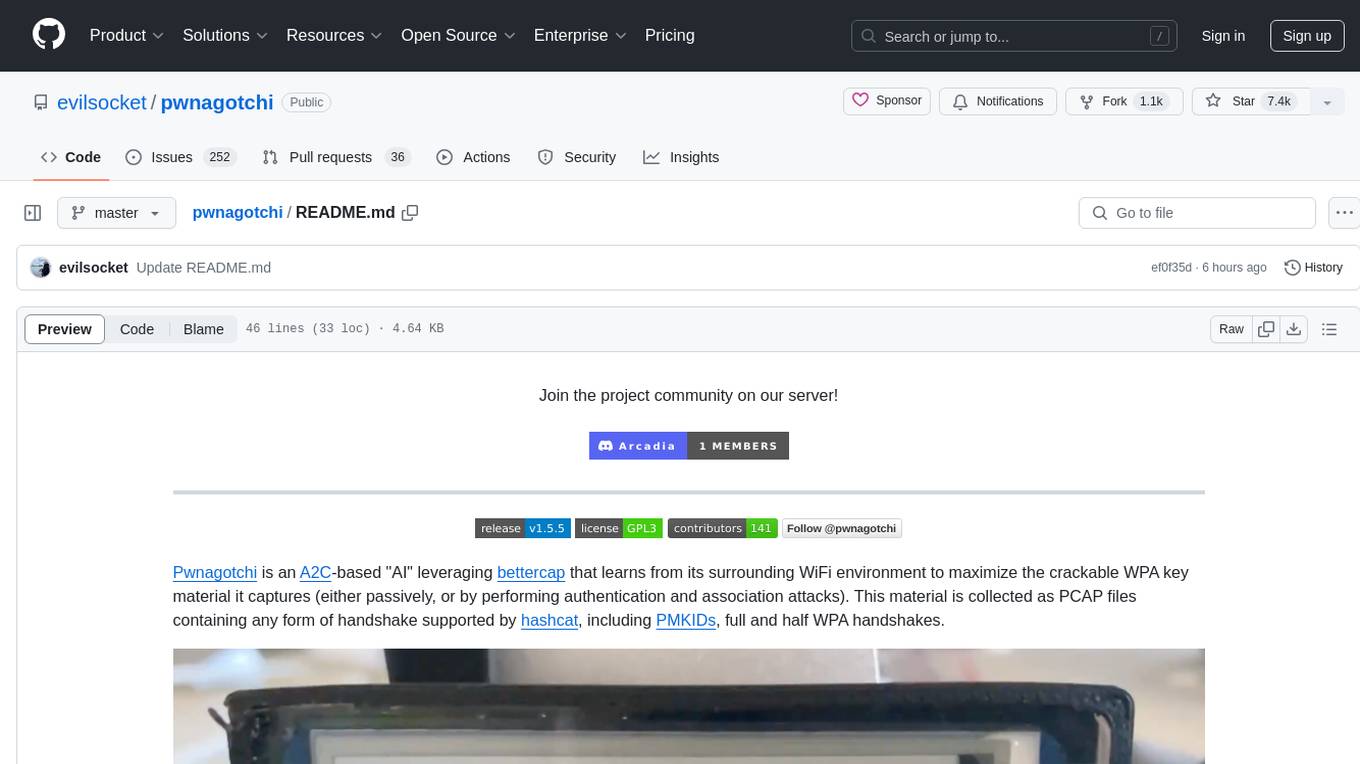
pwnagotchi
Pwnagotchi is an AI tool leveraging bettercap to learn from WiFi environments and maximize crackable WPA key material. It uses LSTM with MLP feature extractor for A2C agent, learning over epochs to improve performance in various WiFi environments. Units can cooperate using a custom parasite protocol. Visit https://www.pwnagotchi.ai for documentation and community links.
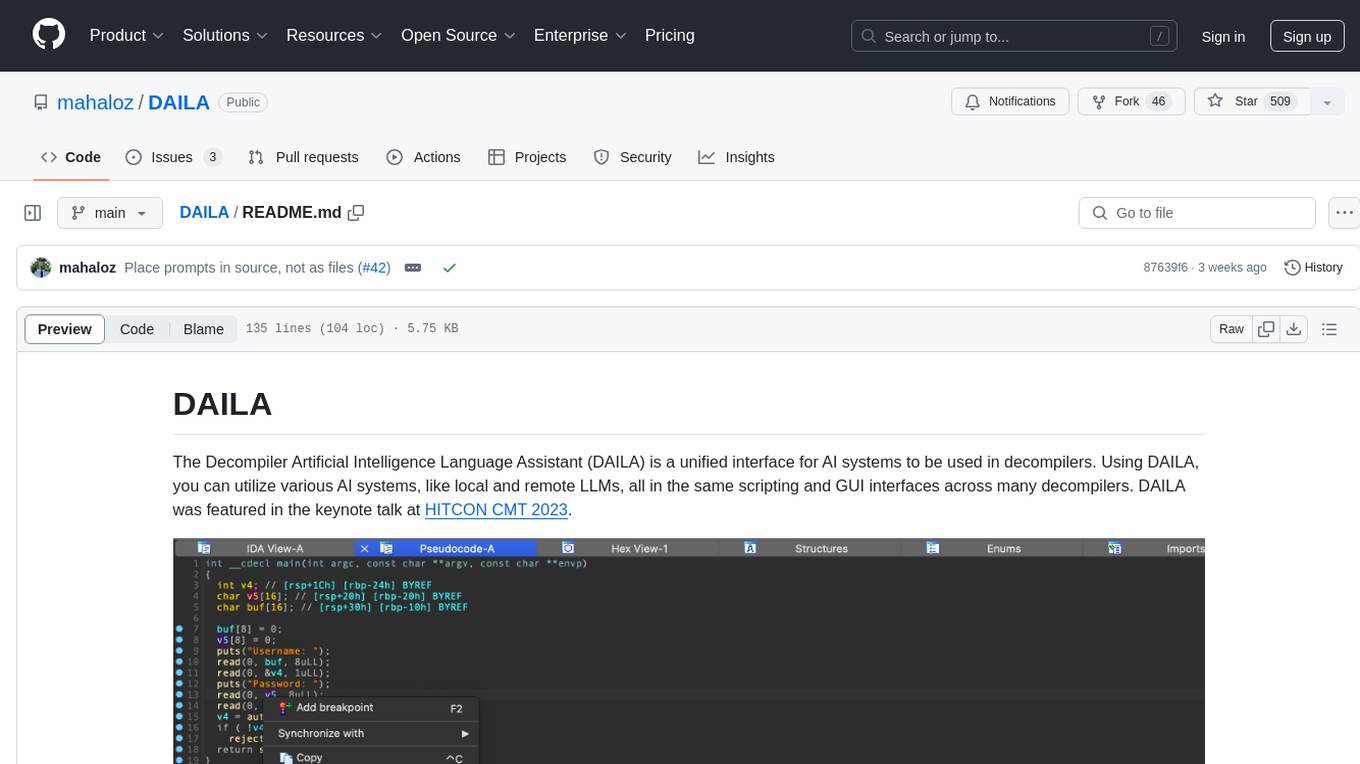
DAILA
DAILA is a unified interface for AI systems in decompilers, supporting various decompilers and AI systems. It allows users to utilize local and remote LLMs, like ChatGPT and Claude, and local models such as VarBERT. DAILA can be used as a decompiler plugin with GUI or as a scripting library. It also provides a Docker container for offline installations and supports tasks like summarizing functions and renaming variables in decompilation.
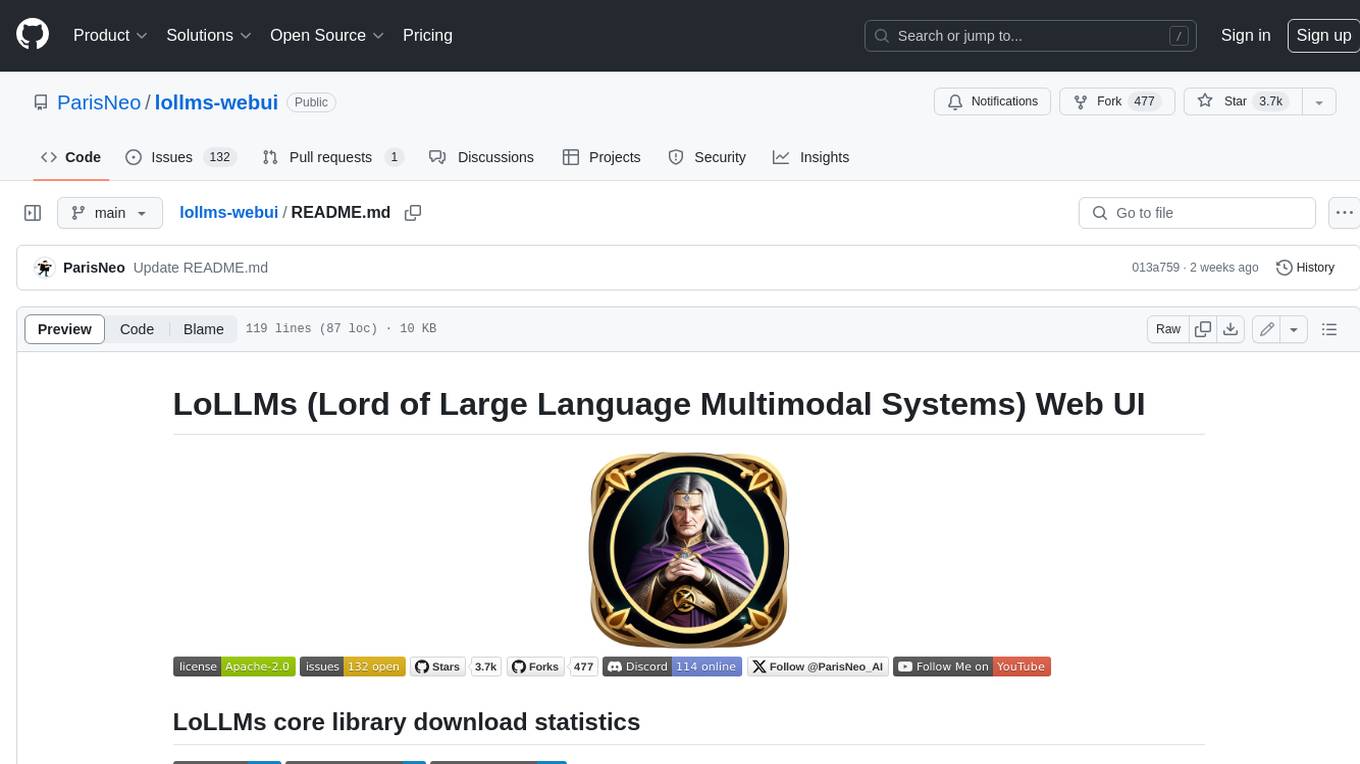
lollms-webui
LoLLMs WebUI (Lord of Large Language Multimodal Systems: One tool to rule them all) is a user-friendly interface to access and utilize various LLM (Large Language Models) and other AI models for a wide range of tasks. With over 500 AI expert conditionings across diverse domains and more than 2500 fine tuned models over multiple domains, LoLLMs WebUI provides an immediate resource for any problem, from car repair to coding assistance, legal matters, medical diagnosis, entertainment, and more. The easy-to-use UI with light and dark mode options, integration with GitHub repository, support for different personalities, and features like thumb up/down rating, copy, edit, and remove messages, local database storage, search, export, and delete multiple discussions, make LoLLMs WebUI a powerful and versatile tool.